the Creative Commons Attribution 4.0 License.
the Creative Commons Attribution 4.0 License.
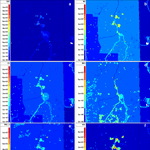
Emissions preparation and analysis for multiscale air quality modeling over the Athabasca Oil Sands Region of Alberta, Canada
Junhua Zhang
Michael D. Moran
Qiong Zheng
Paul A. Makar
Pegah Baratzadeh
George Marson
Peter Liu
Shao-Meng Li
The oil sands (OS) of Alberta, Canada, which are classified as unconventional oil, are the third-largest oil reserves in the world. We describe here a 6-year effort to improve the emissions data used for air quality (AQ) modeling of the roughly 100 km × 100 km oil extraction and processing industrial complex operating in the Athabasca Oil Sands Region (AOSR) of northeastern Alberta. This paper reviews the national, provincial, and sub-provincial emissions inventories that were available during the three phases of the study, supplemented by hourly SO2 and NOx emissions and stack characteristics for larger point sources measured by a continuous emission monitoring system (CEMS), as well as daily reports of SO2 from one AOSR facility for a 1-week period during a 2013 field campaign when the facility experienced upset conditions. Next it describes the creation of several detailed hybrid emissions inventories and the generation of model-ready emissions input files for the Global Environmental Multiscale–Modelling Air quality and CHemistry (GEM-MACH) AQ modeling system that were used during the 2013 field study and for various post-campaign GEM-MACH sensitivity studies, in particular for a high-resolution model domain with 2.5 km grid spacing covering much of western Canada and centered over the AOSR. Lastly, it compares inventory-based bottom-up emissions with aircraft-observation-based top-down emissions estimates. Results show that emissions values obtained from different data sources can differ significantly, such as a possible 10-fold difference in PM2.5 emissions and approximately 40 and 20 % differences for total VOC (volatile organic compound) and SO2 emissions. A novel emissions-processing approach was also employed to allocate emissions spatially within six large AOSR mining facilities in order to address the urban-scale spatial extent of the facilities and the high-resolution 2.5 km model grid. Gridded facility- and process-specific spatial surrogate fields that were generated using spatial information from GIS (geographic information system) shapefiles and satellite images were used to allocate non-smokestack emissions for each facility to multiple grid cells instead of treating these emissions as point sources and allocating them to a single grid cell as is normally done. Facility- and process-specific temporal profiles and VOC speciation profiles were also developed. The pre-2013 vegetation and land-use databases normally used to estimate biogenic emissions and meteorological surface properties were modified to account for the rapid change in land use in the study area due to marked, year-by-year changes in surface mining activities, including the 2013 opening of a new mine. Lastly, mercury emissions data were also processed in addition to the seven criteria-air-contaminant (CAC) species (NOx, VOC, SO2, NH3, CO, PM2.5, and PM10) to support AOSR mercury modeling activities. Six GEM-MACH modeling papers in this special issue used some of these new sets of emissions and land-use input files.
- Article
(4981 KB) -
Supplement
(1689 KB) - BibTeX
- EndNote
Alberta's oil sands (OS: see Appendix A for a list of acronyms), which consist of a mixture of bitumen, sand, clay, and water, are found in the Athabasca, Cold Lake, and Peace River areas of northern Alberta. Together these three areas cover 142 200 km2, about 21 % of the area of the province of Alberta (Alberta Energy, 2018) or about the same area as Greece. The Athabasca Oil Sands Region (AOSR) contributes the largest share of OS bitumen production: 82 % in 2015 (Alberta Energy Regulator, 2018a). There are two main methods used to produce oil from the bitumen, each of which has associated atmospheric emissions. For bitumen deposits buried less than 60 m or so below the surface, the oil sands are mined by open-pit mining methods, in which large excavators dig up oil sand ore and transfer it to heavy-hauler trucks for transport to crushers, where large ore lumps are broken up. The crushed ore is then mixed with hot water and transported to an extraction plant, where the bitumen is separated from the other components and then transferred to either an on-site or a remote upgrader to create synthetic crude oil. About 3 % of the OS area, mainly within the AOSR, can be surface mined but it accounts for about 20 % of the recoverable OS oil reserves. Oil sands in the remaining 97 % of the OS area are situated too deep for surface mining and can only be recovered by in situ extraction methods such as steam-assisted gravity drainage (Alberta Energy Regulator, 2018b). As of 2015, about 46 % of Alberta oil production from oil sands comes from surface mines in the AOSR (Alberta Energy Regulator, 2018a).
According to the 2013 National Pollutant Release Inventory (NPRI; Canada's legislated inventory of pollutant releases reported by industrial, commercial, and institutional facilities that meet certain reporting requirements), emissions from Alberta's OS sector account for 61, 34, and 14 % of the total reported VOC (volatile organic compound), SO2, and NOx emissions, respectively, for the province, whose NPRI total VOC, SO2, and NOx provincial emissions are the highest of the Canadian provinces (https://www.canada.ca/en/environment-climate-change/services/national-pollutant-release-inventory.html, last access: 15 July 2018). The OS industrial sector is also a significant source of PM (particulate matter) and CO emissions. Due to the complex nature of open-pit mining and the OS oil extraction processes, pollutants are mainly emitted from the following five processes: (1) exhaust emissions from off-road vehicles used for removal of the surface overburden and for excavation and transportation of the OS ore to an extraction plant; (2) pollutants emitted from processing taking place at the extraction and upgrading plants; (3) fugitive VOC emissions from mine faces, tailings ponds, and extraction plants; (4) fugitive dust emissions from surface disturbances such as the passage of the large vehicles belonging to the off-road mine fleets; and (5) wind-blown dust emissions from open surfaces such as mine faces and tailings-pond “beaches”. The emissions of criteria-air-contaminant (CAC) pollutants (NOx, VOC, SO2, NH3, CO, PM2.5, and PM10) from in situ OS extraction activities are believed to be lower currently than those of open-pit mining facilities based on the emissions reported to NPRI by facilities (https://www.canada.ca/en/environment-climate-change/services/national-pollutant-release-inventory.html, last access: 15 July 2018).
To support air quality (AQ) modeling activities that are part of the Governments of Canada and Alberta Joint Oil Sands Monitoring (JOSM) plan (see JOSM, 2011), emissions input files were created over the past 6 years in three successive phases for Environment and Climate Change Canada's (ECCC) Global Environmental Multiscale–Modelling Air quality and CHemistry (GEM-MACH) AQ modeling system, which was set up to conduct nested AQ forecasts at model horizontal grid spacings of 10 and 2.5 km (see Fig. S1 in the Supplement). The generation of emission input files was particularly challenging for the inner 2.5 km grid because the AOSR surface mining and processing facilities at the center of the grid are large, complex, and unconventional industrial facilities that cannot be well represented by standard emissions-processing approaches for point sources. At the beginning of emissions-related work for the JOSM plan in 2012 (referred to as phase 1, 2012–2013), considerable effort was invested in reviewing a number of available emissions inventories, compiling a hybrid emissions inventory, and preparing GEM-MACH emissions input files for multiple model grids to support AQ forecasting for an August–September 2013 AQ field campaign in the AOSR. Particular attention was paid to the emissions input files for the inner (2.5 km) model domain centered over the AOSR, since the model forecasts for this grid were the primary numerical guidance used during the field campaign period. Additional emissions input files were then developed for JOSM plan post-campaign AQ modeling activities in the second phase (2014–2015) based on new emissions-related information available after the field study and in the third phase (2016–2017) with updated emissions inventories, as well as new emissions estimates obtained from analysis of the 2013 field-study measurements.
GEM-MACH emissions input files developed during the first two phases using the SMOKE (Sparse Matrix Operator Kernel Emissions) emissions-processing system (https://www.cmascenter.org/smoke, last access: 15 July 2018) have been discussed in Zhang et al. (2015) and in a joint report by ECCC and AEP (Alberta Environment and Parks; formerly AESRD, Alberta Environment and Sustainable Resource Development) for the JOSM project (ECCC & AEP, 2016: hereinafter referred to as the JOSM report). This paper briefly summarizes the work of the first two phases but focuses on the development of new emissions input files during the third phase for the following GEM-MACH AQ modeling applications:
-
Base-case study for AQ forecasting and a long-term deposition study for the region (Makar et al., 2018) and for improvements for NH3 predictions (Whaley et al., 2018).
-
Model sensitivity study on the use of CEMS (continuous emission monitoring system) measurements of SO2, NOx, exit temperature, and flow rate (Akingunola et al., 2018; Gordon et al., 2017).
-
Model sensitivity study on the impact of updated VOC and PM2.5 emissions and speciation derived from surface measurements and from airborne measurements made during the 2013 field campaign (Stroud et al., 2018).
-
Mercury modeling over North America and the OS area using updated emissions (Fraser et al., 2018).
In the rest of this paper, Sect. 2 provides an overview of the various emissions inventories considered to build the base-case model emissions for all three phases. Challenges faced and approaches taken to compile a best-available hybrid emissions inventory for each of the three phases are discussed. Section 3 describes the emissions-processing methodology applied in phase 3 to generate base-case emissions. A land-cover database was also updated for biogenic emissions and for land-surface characterization to account for the rapid change in land use over this region. Next, Sect. 4 describes the emissions data and emissions processing used for several post-campaign emissions sensitivity studies. Lastly, Sect. 5 provides a summary of this work and gives recommendations for future updates and improvements of emissions for AOSR AQ modeling.
2.1 Review of emissions inventories used for JOSM phases 1 and 2 AQ modeling
In 2012, prior to the summer 2013 AOSR field study (Gordon et al., 2015; Liggio et al., 2016; Li et al., 2017), the national emissions inventories used to generate the emissions input files for ECCC's operational GEM-MACH AQ forecast model consisted of the AQ modeling version of the 2006 Canadian national Air Pollutant Emission Inventory (APEI) from ECCC, a projected 2012 United States National Emissions Inventory (NEI) from the U.S. Environmental Protection Agency (EPA) based on version 4 of the 2005 United States NEI, and the 1999 Mexican inventory (Moran et al., 2013a, 2014). The 2006 Canadian APEI represented a base year 7 years earlier than the field-study period, an important consideration for the AOSR due to its rapid development. For example, one of the five AOSR surface mining facilities in operation in 2012, the Canadian Natural Resources Limited (CNRL) Horizon mine (see Fig. 1), only began production in 2009. Hence, pollutant emissions from that mine were not available in the 2006 APEI. Thus, while the 2006 APEI was being used as the basis for national-scale operational AQ forecasting for Canada, it was not an ideal choice for high-resolution AQ modeling for the AOSR field study. A number of newer emissions inventories, however, had been developed for the AOSR area or for the province of Alberta, albeit not always for the purpose of supporting AQ modeling.
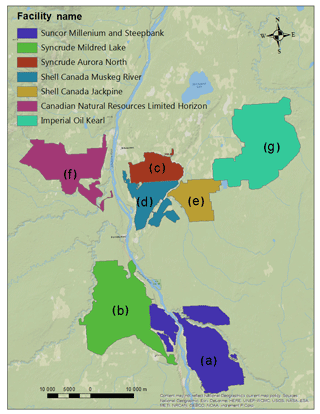
Figure 1Location of six AOSR surface mining and processing facilities: (a) Suncor Millenium and Steepbank, (b) Syncrude Mildred Lake, (c) Syncrude Aurora North, (d) Shell Canada Muskeg River and (e) Shell Canada Jackpine (reported to NPRI as one facility), (f) Canadian Natural Resources Limited Horizon, and (g) Imperial Oil Kearl (only started production in 2013, not considered in earlier inventories). The city of Fort McMurray is located about 10 km to the south.
After an intense review of 10 available national, provincial, and sub-provincial emissions inventories in 2012 (AESRD, 2013; Marson, 2013), a hybrid inventory was compiled for phase 1 and was used to prepare GEM-MACH-ready emissions input files for near-real-time GEM-MACH forecasts during the 2013 field study. Section S1 of the Supplement provides details about the creation of the phase 1 emissions files. After the field study, emissions were updated during the 2014–2015 period (phase 2) to incorporate newly available emissions information, including new versions of national inventories, measurements from CEMS attached to 17 smokestacks at four AOSR mining facilities for the field-study months of August and September 2013, and daily reports of SO2 emissions during a 1-week period in August 2013 when the CNRL Horizon facility experienced abnormal operating conditions. Details of the creation of the phase 2 emissions files are summarized in Sect. S2 of the Supplement.
2.2 Inventory updates for the phase 3 hybrid emissions inventory
After the generation of the phase 2 emissions input files for GEM-MACH, five important new sources of 2013-related emissions data became available:
-
The 2011 United States NEI version 1 from the U.S. EPA (Eyth et al., 2013).
-
The 2013 Canadian APEI.
- a.
2013 Canadian APEI version 1 from ECCC for all sectors, including the first version of reviewed, publicly available 2013 NPRI (released December 2014), except for on-road and off-road mobile source emissions (Sassi et al., 2016).
- b.
Second version of reviewed, publicly available 2013 NPRI (released December 2015).
- a.
-
The 2011 Canadian upstream oil and gas (UOG) point-source inventory for small and medium UOG facilities (Clearstone Engineering Ltd., 2014a, b, c) and a projected 2013 Canadian UOG inventory (created by ECCC as part of the 2013 APEI version 1).
-
CEMS measurements for all CEMS stacks with relatively large SO2 and/or NOx emissions in the province of Alberta for August and September 2013 (from AEP).
-
Top-down aircraft-measurement-based estimates of VOC emissions during the 2013 field-study period for four of the six AOSR mining facilities (Li et al., 2017) and aircraft-measurement-based size-resolved PM emissions for all six facilities.
There were large differences noted between the 2011 United States NEI and the older projected 2012 United States NEI (projected from the 2005 United States NEI) used in phases 1 and 2, despite the 1-year difference in base year. For example, the projected 2012 NEI SO2 emissions from all sectors were reduced by 48 % in the 2011 NEI, but NO2 emissions increased in the latter by 8 %, due mainly to a 40 % increase in on-road NOx emissions (Moran et al., 2015). Among the many reasons that may have contributed to these large differences between the two inventories, one is the change in the on-road emissions estimation tool used by the U.S. EPA from MOBILE6.2 and MOVES2010 (U.S. EPA, 2010) to SMOKE–MOVES2014 (U.S. EPA, 2015; Choi, 2016). Given that the 2011 United States NEI is a retrospective inventory based on actual activity data and CEMS data for base year 2011, it was chosen to replace the projected 2012 United States NEI used in phases 1 and 2 for the creation of the phase 3 emissions input files for base year 2013. Note, however, that the U.S. EPA's emissions trend data set suggests a reduction of NOx emissions by 8 % and SO2 emissions by 23 % between 2011 and 2013 (https://www.epa.gov/air-emissions-inventories/air-pollutant-emissions-trends-data, last access: 15 July 2018).
The first AQ modeling version (i.e., SMOKE-ready version) of the 2013 Canadian APEI (v1), which included point-source emissions from the first version (v1) of the reviewed, publicly available 2013 NPRI (released in late 2014), became available in early 2016 for most sectors, with the exception of the on-road and off-road mobile source sectors. There are significant differences for some sectors between the modified 2010 APEI used in phase 2 (Table S3 in the Supplement) and the 2013 APEI. Figure 2 shows a comparison of fugitive-dust PM2.5 emissions from four sectors for the province of Alberta. PM2.5 emissions from construction more than doubled from 2010 to 2013 due to a combination of increased construction activities and changes in the methodology used to estimate PM emissions for this sector (Environment Canada, 2014). Table 1 provides a comparison of facility-total VOC emissions for the six surface OS mining facilities used for phases 1 and 2 vs. phase 3. For phases 1 and 2 these emissions were 2010-NPRI-scaled CEMA VOC emissions (Tables S2 and S3), whereas for phase 3, version 2 (v2) of the 2013 NPRI, which became available in late 2015, was used (Table 2). VOC emissions from the Suncor Millenium and Steepbank facility were reduced from about 28 000 t yr−1 in phase 2 to 9500 t yr−1 in phase 3, a 64 % reduction; the Shell Canada Muskeg River and Jackpine mine had a similar percentage reduction. One additional complication is that facilities may submit modified reports to NPRI for past reporting years based on updated information, as can be seen by comparing the last two columns of Table 1, where reported total VOC emissions increased for Suncor Millenium and Steepbank, Syncrude Mildred Lake, and Syncrude Aurora North in the 2013 NPRI v2 (see also Li et al., 2017). One other important change evident in Table 1 is the inclusion of emissions from the Imperial Oil Kearl surface mine, which began production in 2013, in the two 2013 emission inventory versions.
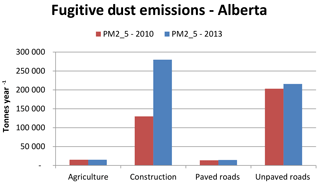
Figure 2Comparison of fugitive PM2.5 emissions for four sectors between 2010 APEI (used for phase 2) and 2013 APEI (used for phase 3) for the province of Alberta.
Emissions from smokestacks that are released at high-volume flow rates and high temperatures may rise much higher into the atmosphere than stack releases with lower volume flow rates and temperatures. As a consequence, AQ models such as GEM-MACH include specialized parameterizations to calculate this plume rise (see Akingunola et al., 2018; Gordon et al., 2017). However, the extent to which this information is reported depends on the regulatory environment. One limitation of the 2013 NPRI is that only emissions from stacks higher than 50 m must be reported separately. Emissions from all other shorter stacks are aggregated together with surface-level fugitive emissions and are treated as surface releases (ECCC, 2016). On the other hand, the 2009–2010 CEMA inventory has separate emissions information for all individual stacks. To allow plume rise to be calculated for stacks both above and below the NPRI reporting threshold, facility-total NPRI aggregate stack emissions were allocated proportionately to each stack in the CEMA inventory based on the 2009–2010 CEMA stack emissions.
There are a variety of activities with pollutant releases to air within any given facility's boundaries, and the type of activity may influence the type and amount of VOCs being emitted at the facility. The extent to which these activities can be identified to allow spatial allocation within a facility once again depends on the regulatory environment and the reporting requirements. Surface-level fugitive VOC emissions are reported to NPRI as facility-total emissions without differentiation between source type (e.g., mine faces, tailings ponds, and extraction and upgrading plants). To distribute 2013 NPRI fugitive VOC emissions spatially within an OS mining facility, process allocation factors calculated from the process-specific fugitive VOC emissions in the 2009–2010 CEMA inventory for each AOSR mining facility were used to allocate fugitive VOC emissions between mine faces, tailings ponds, and plants (similar to the procedure used in phase 2; see ECCC & AEP, 2016). For the Imperial Oil Kearl mine, which was not operating in 2010, 2013 fugitive VOC emissions were differentiated based on process allocation factors from the Shell Muskeg River and Jackpine facility given that both facilities use paraffinic froth treatment (PFT) technology to produce diluted bitumen, which is then transported through pipelines to off-site refineries for further processing (http://www.oilsandsmagazine.com/technical/mining/froth-treatment/paraffinic, last access: 15 July 2018; Li et al., 2017). However, due to the fact that the operation of a new mine during its first months may be quite different than a mine that has been operating for years, this was at best a necessary assumption with considerable uncertainty.
Table 2Summary of Canadian data sources used for generating JOSM phase 3 base-case emissions input files.
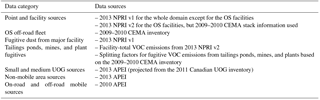
The UOG emissions input files generated for phase 2 were based in part on a year-2000 Canadian UOG inventory projected to 2010 (Table S3). After phase 2, a 2011 Canadian UOG inventory that was compiled for ECCC became available (Clearstone Engineering Ltd., 2014a, b, c). This new subinventory was then projected by ECCC to 2013 for inclusion in the 2013 APEI based on activity data and a methodology described in a letter report from Clearstone Engineering Ltd. (2014d). Figure 3 shows the national-level differences between the year-2000-based projected 2010 UOG inventory and the year-2011-based projected 2013 UOG inventory for the seven CAC pollutants, where about 95 % of the UOG facilities are located within the high-resolution OS modeling domain. VOC, CO, and NOx emissions are higher for the new subinventory by 27, 23, and 11 %, respectively, while SO2 emissions are 11 % lower. Thus, the projection of total UOG emissions from 2000 to 2010 that was used for phase 2 seems to have been reasonable in total. However, the number of UOG facilities with CAC emissions increased from about 207 000 in the 2000 UOG inventory to 334 000 in the 2011 UOG inventory, a 61 % increase. Figure S2 shows the locations of UOG facilities in the Ft. McMurray AOSR area for the 2000 and 2011 UOG inventories. We can see that some UOG facilities that existed in 2000 have been closed while many new facilities have opened since 2000. Updating the UOG inventory to the 2011-based 2013 projected inventory might thus be expected to have a significant impact on the spatial distribution of UOG emissions.
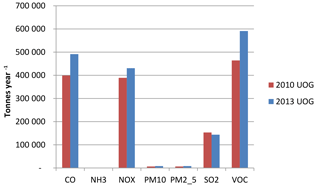
Figure 3Comparison of national CAC emissions between the year-2000-based projected 2010 UOG inventory and the year-2011-based projected 2013 UOG inventory.
Given the availability of these new emissions data sets, a synthesized phase 3 hybrid emissions inventory was created from the inventories listed in Table 2. As a complement to Table 1, which compared the VOC emissions from the AOSR mines used for the three phases, Tables S4 to S6 compare the facility-total emissions of other CAC species compiled for the three phases from three main source types: CEMA off-road mobile emissions, facility smokestack and area-source emissions, and road dust emissions. As described in the next section, further improvements were also made to the emissions-processing methodology before new phase 3 model-ready 2013 base-case emissions files were generated from the phase 3 hybrid inventory. Additional phase 3 emissions input files that were generated for GEM-MACH emissions sensitivity runs using an expanded set of CEMS measurements and aircraft-observation-based emissions estimates are then discussed in Sect. 4.
The same overall emissions-processing methodology described in Zhang et al. (2015) and the JOSM report (ECCC & AEP, 2016) was used in phase 3 to generate gridded, hourly, model-ready emissions fields for GEM-MACH using the SMOKE emissions-processing system. The three main steps required to process a typical emissions inventory that contains monthly or annual CAC emissions reported by jurisdiction for a small number of pollutants into gridded, hourly, model-ready emissions input files are (a) spatial disaggregation, (b) temporal disaggregation, and (c) chemical speciation (e.g., Dickson and Oliver, 1991; Houyoux et al., 2000; Moran et al., 2013b). Note that before spatial disaggregation (i.e., spatial allocation) can be performed, a set of spatial surrogate fields must first be generated on the model grid of interest for such proxy or surrogate fields as population, road density, and agricultural land use. Different inventories are then processed separately, often subinventory by subinventory (e.g., point sources, area sources, off-road sources, on-road sources), and as a last step some of the resulting gridded output fields may be merged.
Key aspects of the emissions-processing methodology for phase 3 that were specific to the AOSR emissions included the following:
-
Updated facility-specific and process-specific spatial surrogate fields were used (similar to phase 2) for the 10 km North American grid and 2.5 km western Canada grid based on GIS polygons of mine faces, tailings ponds, and plants for the six AOSR mining facilities (Fig. 1) in order to spatially allocate the surface area emissions from off-road fleet and fugitive sources between mine faces, tailings ponds, and plants. Emissions from individual smokestacks within these facilities, on the other hand, were treated as point-source emissions and assigned to the specific grid cells in which the stacks are located.
-
Facility-specific monthly temporal profiles for production-related emissions, such as emissions from off-road mine fleets and extraction plants, were generated based on facility-specific monthly production statistics for 2013 (Alberta Energy Regulator, 2014). Weekly and diurnal temporal profiles were treated as constant (i.e., flat) as a default because the AOSR mining facilities usually operate around the clock throughout the year (note, however, the discussion on CEMS emissions in Sect. 4.1). Temperature-based monthly temporal profiles were created for fugitive VOC emissions from mine faces and tailing ponds, similar to the methodology that has been used in past AOSR environmental impact assessment (EIA) submissions (e.g., Cenovus FCCL Ltd., 2010; Imperial Oil, 2005).
-
Facility-specific and process-specific VOC speciation profiles were created based on VOC speciation profiles compiled in the CEMA inventory (Davies et al., 2012; Zhang et al., 2015).
-
PM speciation profiles from version 4.3 of the U.S. EPA SPECIATE database (https://www.epa.gov/air-emissions-modeling/speciate-version-45-through-40, last access: 15 July 2018; Reff et al., 2009) were used to split PM emissions into six model chemical components: sulfate, nitrate, ammonium, elemental carbon, primary organic matter, and crustal material. Process-specific PM profiles were used for stack emissions based on the Source Classification Code (SCC) assigned to the stacks in the CEMA inventory (Davies et al., 2012). The “Unpaved Road Dust – Composite” PM speciation profile from SPECIATE v4.3 was used to speciate fugitive dust emissions from unpaved roads within each facility in the base-case emissions.
Another required emissions-processing step was to perform PM size disaggregation. As discussed in Makar et al. (2018) GEM-MACH may be configured to represent the PM size distribution with either 2 or 12 size bins. Accordingly, the PM emissions were processed twice, once for each representation of the PM size distribution. The two-bin version separates PM10 emissions into two size bins, PM2.5 (fine bin) and PM coarse bin (equal to PM10–PM2.5), whereas the 12-bin version separates PM10 emissions into the 10 size bins listed in Table 3, plus 2 larger size bins for diameters greater than 10 µm (note that the base-case emissions thus assumed no primary particulate emissions for sizes greater than 10 µm diameter). For the 12-bin PM emissions, generic PM size distribution profiles were applied for three broad source types (area, mobile, and point) based on 10 source-specific particle size distributions discussed in Eldering and Cass (1996). Figure 4 shows the distribution of the eight PM2.5 bins for these three source types. Mobile source PM2.5 emissions have a normal size distribution centered around 0.16 µm in diameter, but point-source and area-source PM2.5 emissions are skewed to the smaller and larger size bins, respectively.
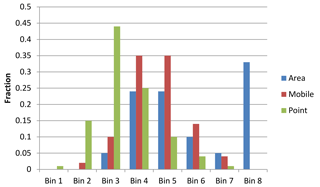
Figure 4Fractional distribution of the eight PM2.5 size bins for the 12-bin version of GEM-MACH modeling for three broad types of emissions sources.
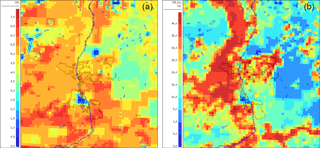
Figure 5(a) Leaf area index and (b) peak summer isoprene emissions computed on the 2.5 km for a portion of the 2.5 km OS grid centered on the AOSR study area from the original BELD3 database. The gray lines indicate the cleared areas within the boundaries of the six AOSR mining and processing facilities (see Fig. 1).
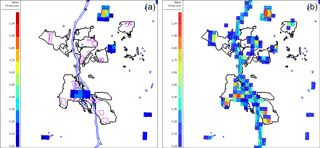
Figure 6(a) Inland water coverage for a portion of the 2.5 km OS grid centered on the six AOSR mining and processing facilities generated from the original land-cover database (only natural lakes); and (b) modified inland water coverage including tailings ponds and rivers. The black and pink lines in panel (a) indicate the cleared-land areas and the tailings ponds within the boundaries of the six AOSR mining and processing facilities, whereas the blues lines in panel (a) mark the boundaries of natural lakes and rivers.
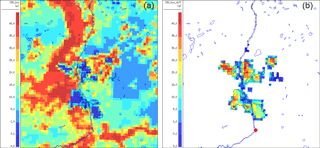
Figure 7(a) Modified biogenic isoprene emissions for a portion of the 2.5 km OS grid centered on the AOSR study area and (b) difference between the original and the modified isoprene emissions (original – modified). The gray lines indicate the cleared-land areas within the boundaries of the OS mining facilities. The location of Fort McMurray is indicated by the diamond symbol.
In addition to anthropogenic emissions, GEM-MACH must also consider natural emissions, including biogenic VOC emissions, which depend on local vegetation type and light and/or temperature conditions. GEM-MACH calculates biogenic emissions dynamically (that is, making use of the GEM meteorological model's predictions of temperatures and light levels during a simulation combined with vegetation-type-dependent biogenic emissions formulas from BEIS, Biogenic Emission Inventory System, v3.06). Vegetation type is described using the BELD3 (Biogenic Emissions Landuse Database, version 3) database, which contains 230 vegetation classes at 1 km resolution (Pierce et al., 2000). However, by 2013 the vegetation fields in the BELD3 database, which is based on early 1990s satellite imagery (Kinnee et al., 1997), were outdated over the AOSR mining area – much of the area that was forested in the 1990s was later cleared of forest cover during the construction of the AOSR mining facilities. This is illustrated in Fig. 5, which shows mean leaf area index (LAI) for the gridded vegetation and corresponding summer peak isoprene emissions computed from the original BELD3 database. Except for some areas within the two oldest AOSR mining facilities, Suncor Millenium–Steepbank and Syncrude Mildred Lake, LAI values and isoprene emissions over the other mining facilities as computed with the BELD3 database are erroneously high, due to the fact that these areas, which by 2013 had been cleared for surface mining, were still characterized in the database as forested. Furthermore, the only water bodies contained in the land-cover database over this area are natural lakes. The large artificial tailings ponds present in the mining facilities are not characterized as water covered in the database (Fig. 6a) even though in 2013 the tailings ponds in the AOSR covered an area of about 180 km2 (https://web.archive.org/web/20170727072144/http://www.energy.alberta.ca/OilSands/pdfs/FSTailings.pdf, last access: 15 July 2018), the equivalent of 29 grid cells on the OS 2.5 km grid. Tests of the GEM-MACH model's meteorology for plume-rise algorithm analysis have shown that these artificial water bodies can have a significant influence on local meteorology and atmospheric vertical stability. In addition, an examination of the default water-body field portion of the grid cells overlapping the Athabasca River (center of Fig. 6a, flowing from south to north) showed that the river was also not being treated as a body of water in the default meteorological model database. The accuracy of the land-use database thus influences both meteorological and biogenic emissions estimation accuracy.
The outdated land-cover characteristics over the AOSR area would thus have an impact on GEM-MACH predictions, particularly at high spatial resolutions. To improve the land-use and vegetation characterization of this area, masks for cleared land and artificial water bodies were generated as GIS polygons based on 2013 satellite images. Rivers were added using more detailed GIS water-body data. Figure 7a shows the biogenic isoprene emissions over the AOSR surface mining area after the modification (cf. Fig. 5b) and Fig. 7b shows the difference between the original and modified isoprene emissions. The modified inland water coverage is shown in Fig. 6b. By applying these masks to update vegetation and land-cover data, GEM-MACH-calculated biogenic emissions can be reduced by as much as 100 % for the cleared areas related to mining activities. Meteorological fields are also affected. For example, Fig. S3 shows that the predicted planetary boundary layer height over the OS facilities can be a few hundred meters lower than the surrounding areas, which is similar to the effect of natural lakes.
As an example of the emissions input files generated with the SMOKE emissions-processing system from the phase 3 inventory, Fig. S4 shows gridded August mean monthly emissions of six pollutant species for a portion of the 2.5 km OS grid centered on the AOSR study area. Similar to Fig. 7b, the locations of the six AOSR mining facilities can be seen clearly, but other emissions sources are also evident, such as on-road vehicle emissions and emissions from the city of Fort McMurray. GEM-MACH results from the use of the new phase 3 base-case emissions input files generated using these updated emissions inventories (Table 2), updated AOSR facility- and process-specific spatial surrogate fields, new AOSR facility-specific monthly temporal profiles and VOC speciation profiles, and updated BELD3 vegetation and land-use data sets are described in Makar et al. (2018).
In addition to the phase 3 base-case emissions input files described in Sect. 3, additional GEM-MACH emissions input files were generated using four special emissions data sets in order to examine the effects of specific changes to the emissions data on model predictions. These four data sets were (1) an expanded 2013 CEMS emissions data set, (2) 2013 OS field campaign aircraft-measurement-based top-down VOC emissions estimates, (3) 2013 OS field campaign aircraft-measurement-based top-down PM2.5 emissions estimates, and (4) updated mercury emissions. These additional GEM-MACH emissions input files were used for a number of phase 3 GEM-MACH sensitivity studies that are referenced in this section and described in detail elsewhere in this special issue.
Table 4Comparison of speciated annual ADOM-2 (Acid Deposition and Oxidant Model, version 2) model VOC species emissions (t yr−1) between base-case emissions from the 2013 NPRI version 2 (bottom-up) and the aircraft-observation-based estimates (top-down). Note that unknown or unreactive VOC species are not included. Suncor Millenium and Steepbank: Suncor – M/S; Syncrude Mildred Lake: Syncrude – ML; Shell Muskeg River and Jackpine: Shell – MR/J.
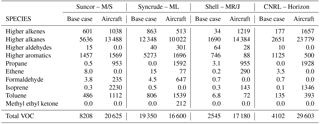
4.1 Expanded CEMS emissions data set
As noted in Sect. S2, CEMS-measured hourly SO2 and NOx emissions from 17 stacks within four AOSR mining facilities were used in phase 2 emissions processing for a GEM-MACH sensitivity test (ECCC & AEP, 2016; Makar et al., 2015; Zhang et al., 2015). This earlier work showed a relatively large impact of the better time-resolved CEMS data on model results. Recall that in Canada regulatory reporting at the national level requires only annual total emissions from large stacks; hence, details on specific time periods within the year are lost and calculations to reconstruct this time variation using each facility's operating schedule for the emitting activities can only be approximate at best. However, detailed CEMS records are reported to the Alberta provincial government. For phase 3, CEMS measurements from about 100 stacks at 33 facilities with relatively large SO2 or NOx emissions were obtained for the province of Alberta for August and September 2013. A sensitivity study was designed to investigate the impacts of both (i) CEMS-measured hourly SO2 and NOx emissions and (ii) CEMS-measured stack volume flow rates and exit temperatures on GEM-MACH predictions (Akingunola et al., 2018). For this study, the phase 3 base-case stack emissions (based on 2013 NPRI annual reporting of stack emissions) were replaced with the corresponding CEMS hourly measurements. For the phase 3 base-case emissions, the stack flow rate and exit temperature, which are used to calculate plume rise, were assumed to be static at the annual reported values. However, CEMS-measured stack exit temperature and flow rate often display significant temporal variation as shown in Fig. S5 for one example; hence, these measured values were saved in model-ready form for the 2-month period to evaluate their impact on model predictions.
Due to the NPRI reporting threshold that facility operators are not required to report stack-specific emission from smokestacks shorter than 50 m (Sect. 2.2), not all CEMS stacks could be matched to NPRI stacks. Overall, 38 of the 100 stacks in the expanded CEMS data set were matched with NPRI stacks at 20 facilities. However, since the 38 matched stacks were de facto all tall stacks with generally large emissions, emissions from the matched stacks account for 77 and 43 % of total SO2 and NOx emissions, respectively, from all NPRI point sources in Alberta. Figures S6 and S7 show comparisons by facility of SO2 and NOx emissions between the NPRI annual inventory and the 2-month CEMS measurements for SO2 and NOx, scaled up to annual values. Overall, these scaled CEMS-based estimates agree well with NPRI annual totals, in spite of the large short-term temporal variation shown in the CEMS measurements. This is reasonable since facilities are expected to base their reported annual stack emissions on CEMS measurements. Over shorter time intervals, however, the stack emissions levels may vary by up to several orders of magnitude, thus having a significant influence on model predictions. In addition, the differences between CEMS volume flow rates and exit temperatures and the annual reported values may also have a significant influence on plume dispersion and transformation of SO2 and NOx emitted from tall stacks. Akingunola et al. (2018) showed that model-predicted SO2 concentration could be changed by as much as 50 % and the NOx concentration by about 10 % using the CEMS-measured hourly stack flow rate and temperature. On the other hand, the use of the more realistic CEMS-measured volume flow rates and temperatures resulted in a slight degradation of model performance with a new, improved plume-rise algorithm.
4.2 Aircraft-measurement-based top-down VOC emissions estimates for AOSR mining facilities
Airborne measurements have recently been used to quantify emissions from various oil and gas fields. For example, Karion et al. (2013) estimated methane emissions over a western United States natural gas field, Peischl et al. (2015) quantified methane emissions as well from three United States shale production regions, and Li et al. (2017) estimated VOC emissions for four AOSR facilities during the 2013 OS field campaign. As described in Li et al. (2017), aircraft observations of VOC species concentrations were used to estimate facility-total emissions of individual VOC species using a mass-balance approach (Gordon et al., 2015) for the Suncor Millenium and Steepbank, Syncrude Mildred Lake, Shell Canada Muskeg River and Jackpine, and CNRL Horizon mining facilities (see Fig. 1). Comparisons between the aircraft-observation-based top-down estimates of individual VOC species emissions and the corresponding bottom-up emissions reported to NPRI by these four facilities showed differences in terms of the magnitude of both VOC species emissions and total VOC emissions (Li et al., 2017).
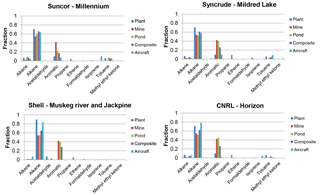
Figure 8Comparisons of facility-specific VOC speciation profiles for ADOM-2 mechanism for four AOSR mining facilities used for the base-case study with facility-specific profiles derived from aircraft observations. Different VOC speciation profiles for plants, mine faces, and tailings ponds were used for the base-case study. The “composite” VOC speciation profile for the base case is an emissions-weighted combination of the plant, mine-face, and tailings-pond profiles for each facility to allow comparison with the aircraft-based facility-specific VOC speciation profiles.
Some previous studies have shown that the use of aircraft-derived top-down emissions improved model performance. For example, in an attempt to understand high O3 events during winter time in a western United States oil and gas region, Ahmadov et al. (2015) compared AQ model performance using emissions from two different sources: (1) the U.S. EPA NEI (bottom-up) and (2) emissions derived from aircraft observations (top-down). They found that the top-down emissions improved model prediction of methane, other VOCs, and NOx. The use of these top-down emissions also captured the O3 episode better than using the bottom-up emissions. To assess the impact of the suggested uncertainty of VOC emissions for these four OS facilities on GEM-MACH predictions, emissions of the individual VOC species estimated from the aircraft observations (top-down) were mapped to the model VOC species used by GEM-MACH's ADOM-2 (Acid Deposition and Oxidant Model, version 2) gas-phase chemistry mechanism (Makar et al., 2003; Stroud et al., 2008) to replace the corresponding phase 3 base-case model VOC species emissions (bottom-up) for the four facilities.
Table 4 shows a comparison of facility-total emissions of ADOM-2 model VOC species between the phase 3 base-case emissions input files (bottom-up) and the aircraft-observation-based emissions input files (top-down). The aircraft-derived VOC emissions estimates shown in Table 4 were annualized by scaling daily values with seasonal variation factors as discussed in Li et al. (2017). Except for Syncrude Mildred Lake, the totals of the aircraft-observation-based top-down VOC emissions for these facilities are higher than the corresponding bottom-up base-case totals, ranging from a factor of 2.5 for Suncor Millenium and Steepbank to 6.7 for Shell Canada Muskeg River and Jackpine and 7.2 for CNRL Horizon. The relative rankings of the emissions by model VOC species also differ for the two data sources. Figure 8 compares the process-specific VOC speciation profiles for these four facilities that were used for the phase 3 base-case study based on the CEMA inventory (Davies et al., 2012; Zhang et al., 2015). Figure 8 also compares the inventory-based VOC speciation profiles (bottom-up) with the aircraft-observation-based VOC speciation profiles (top-down) by facility. As the emissions estimated from the aircraft observations corresponded to facility-total emissions, an emissions-weighted, base-case “composite” VOC speciation profile was created for each facility by combining the plant, mine-face, and tailings-pond VOC speciation profiles based on the emissions of each ADOM-2 model VOC species. Both the aircraft-observation-based VOC speciation profiles and the composite VOC profiles vary from facility to facility, but there are some differences between the two profile types. Consistent with Li et al. (2017), for example, the aircraft-observation-based VOC profiles have a higher propane emissions fraction and a much lower higher-aromatic emissions fraction than the composite profiles for all four facilities. The aircraft also measured significant amounts of isoprene emissions likely originated from bitumen vapor emissions from the Suncor Millenium and Steepbank and the CNRL Horizon facilities, which are not present in the corresponding bottom-up base-case profiles. Further studies are needed to confirm the source of non-biogenic isoprene emissions.
To generate model-ready emissions files, the aircraft-estimated top-down VOC emissions were first split by process based on the process-specific VOC emissions compiled for the base case and then spatially allocated within each facility based on the process-specific and facility-specific surrogates. Figure S8 shows spatial variations in the ratio of the gridded, model-ready, aircraft-observation-based higher-alkane emissions (top-down) to corresponding base-case emissions (bottom-up) for the GEM-MACH 2.5 km grid over the AOSR study area. Consistent with Table 4, the ADOM-2 higher-alkane emissions estimated from the top-down estimation are about 8 times higher for the Shell Canada Muskeg River and Jackpine and the CNRL Horizon facilities than corresponding bottom-up emissions from the 2013 NPRI but are closer for the Suncor Millenium and Steepbank and the Syncrude Mildred Lake facilities. The variations seen within individual facilities are due to different emission rates and different VOC speciation profiles for plants, mine faces, and tailings ponds. As expected there is no difference for areas outside of these four facilities. The new GEM-MACH emissions input files generated using the aircraft-observation-based VOC emissions have been used for a GEM-MACH sensitivity test (see Stroud et al., 2018).
4.3 Aircraft-measurement-based top-down PM emissions estimates for AOSR mining facilities
PM emissions from the AOSR mining facilities originate mainly from four major source categories: (1) emissions from plant stacks; (2) tailpipe emissions from the off-road mining fleet; (3) fugitive dust originating from various activities, such as excavation of oil sand ore, loading and unloading trucks, and wheel abrasion of surfaces by off-road vehicles; and (4) wind-blown dust. As summarized in Table 2, PM emissions from plant stacks and fugitive dust source categories were obtained from the 2013 NPRI, whereas emissions from tailpipe emissions were provided by the 2009–2010 CEMA inventory. However, none of the inventories included wind-blown dust emissions, and the estimates of anthropogenic fugitive dust emissions are highly uncertain. In addition, emissions of construction dust from one facility, the Imperial Oil Kearl mine, a portion of which was still under construction during the aircraft monitoring campaign, were expected to be large. In order to evaluate and potentially to improve these emissions estimates, top-down estimates of size-resolved PM emissions were also calculated based on aircraft measurements of size-resolved PM concentrations made during the 2013 AOSR field campaign for all six AOSR mining facilities.
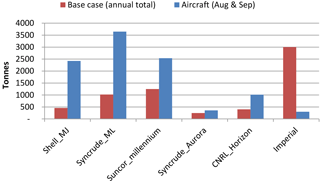
Figure 9Comparison of PM2.5 emissions between base-case annual emissions and aircraft-observation-based estimates for the 2 summer months (August and September) for the six AOSR mining facilities.
The 2013 aircraft campaign used a top-down mass-balance approach (Gordon et al., 2015) to determine PM emissions from all six AOSR surface mining facilities. For particles with a diameter in the range of 0.3 to 20 µm, a forward scattering spectrometer probe (FSSP) model 300 was deployed from a wing-mounted pod (Baumgardner et al., 1989) to measure the particle number-concentration size distribution in 30 size bins. An ultra-high sensitivity aerosol spectrometer (UHSAS) was used inboard to determine the number-concentration size distribution of particles with diameter from 0.06 to 1.00 µm in 99 size bins. Volume-concentration size distributions of particles were derived from these number-concentration size distributions from 0.06 to 20 µm by combining both sets of measurements from the two instruments. Size-dependent particle densities, varying from 1.5 to 2.5 g cm−3, were used to convert the volume-concentration size distributions to mass-concentration size distributions, based on the known mineralogy for the supermicron particles for the topsoil in the region and the known chemical composition for submicron particles from concurrent aerosol mass spectrometer measurements (Liggio et al., 2016). The resulting particle mass concentration size distributions were combined to match the 12-bin version of the GEM-MACH model particle size distribution. The mass-balance emission algorithm TERRA (Top-down Emission Rate Retrieval Algorithm) (Gordon et al., 2015) was then applied to these particle size bins to determine the particle mass emission rates for each bin. Uncertainties in the particle mass emission rate from each facility determined this way were estimated at approximately 36 % for supermicron particles and 32 % for submicron particles. Based on the aircraft observations, 68 % of the PM10 emissions are in the coarse mode (2.5 to 10 µm).
Figure 9 shows a comparison of facility-level PM2.5 emissions between the base-case inventory-based annual values (bottom-up) and the aircraft-observation-based estimates (top-down) for the 2 summer months (August and September) for the six AOSR facilities. Note that the latter were calculated for this comparison simply by assuming constant daily emissions throughout the 2 summer months. This avoided an annualization calculation, for which it is difficult to account for modulation by snow cover, frozen ground, or precipitation during wintertime. Moreover, the aircraft-observation-based top-down estimates were only used in GEM-MACH for summertime modeling. Except for the Imperial Oil Kearl facility, the PM2.5 emissions estimated from the top-down aircraft observations, even for just 2 summer months, were a factor of 1.5 to 5 larger than the bottom-up 2013 APEI PM2.5 annual emissions used for the phase 3 base-case emissions processing. One reason for the difference is that wind-blown dust is not included in the APEI inventory, which is compiled for anthropogenic emissions only. For the base-case bottom-up inventory, total PM2.5 emissions from off-road vehicle tailpipe emissions and stacks are 2272 t yr−1 (Tables S4 and S5) while road dust emissions are 4134 t yr−1 (Table S6). Thus, anthropogenic fugitive dust emissions account for 65 % of total PM2.5 emissions from the AOSR mines. Aircraft-observation-based estimated total PM2.5 emissions from all six facilities are about 10 300 t for the 2 summer months. If we assume that all of the unreported PM2.5 emissions come from natural wind-blown dust, then fugitive dust emissions will dominate total PM2.5 emissions from those facilities even more.
Figure 10 shows the observed size distribution of the first eight GEM-MACH size bins, which correspond to the PM2.5 size range (see Table 3). Although the size distribution of the PM2.5 emissions varies from facility to facility, 65–95 % of PM2.5 emissions are in bin 8 (diameter range from 1.28 to 2.56 µm), implying that the majority of the PM2.5 emissions are from fugitive-dust area sources (Eldering and Cass, 1996), either from dust kicked up by off-road mining vehicles or from wind-blown dust. Compared to the area-source PM size distribution profile used by SMOKE to process the bottom-up base-case emissions (Fig. 4), a much larger bin 8 mass fraction and smaller bin 1 to 7 (i.e., < 1.28 µm) mass fractions were observed by the aircraft for the AOSR mining facilities.
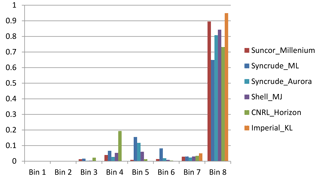
Figure 10PM2.5 size distribution derived from the aircraft observations for the six AOSR mining facilities.
An AOSR-specific PM chemical speciation profile consisting of six chemical components was also constructed for fugitive dust emissions from these facilities to replace the “Unpaved Road Dust – Composite” profile from the U.S. EPA SPECIATE v4.3 database (see Sect. 3). Wang et al. (2015) analyzed soil samples collected from 17 AOSR facility sites and 10 forest sites. The samples were further characterized as paved road dust, unpaved road dust, tailings sands, and overburden soil. Their analysis showed that PM speciation is clearly different between the dust collected from the facility sites and from the forest sites. For this study, the new AOSR-specific fugitive-dust PM speciation profile was compiled by averaging the site-specific profiles from all 17 facility sites from Wang et al. (2015) to represent surface PM speciation with the following three exceptions:
-
For the unpaved-road site S16, the elemental-carbon percentage seemed to be too large, which might be an artifact due to dry deposition from heavy-duty diesel exhaust (Wang et al., 2015). This site was excluded from the facility profile average in their study and was excluded in this study too.
-
The organic-carbon percentage for site S10 was much smaller and the elemental-carbon percentage was larger than those of other facility sites. That site was excluded from the organic-carbon range discussion in Wang et al. (2015) and was excluded here as well.
-
S17 is located on Highway 63, so it was also excluded from the facility average.
Figure 11 shows a comparison of the fugitive-dust PM speciation profile used for the phase 3 base-case emissions processing, which is the standard “Unpaved Road Dust – Composite” profile from the U.S. EPA SPECIATE v4.3 database, and the new profile described above. The organic-matter (OM = organic carbon + particulate non-carbon organic matter) percentage in the AOSR-specific PM speciation profile (21.8 %) is about 3 times larger than the fraction in the standard “Unpaved Road Dust – Composite” profile (7.6 %), suggesting that soils in the AOSR facilities contain more organic matter than soils in other areas. The crustal-material percentage decreases correspondingly, from over 91 to 76 %. The AOSR-specific PM speciation profile also has more sulfate and elemental carbon, but the fractions are relatively small.
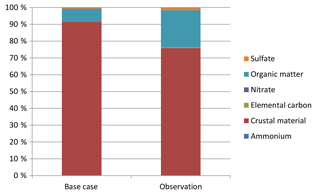
Figure 11Comparison of the fugitive-dust PM speciation profile used for the base-case study and the one compiled from soil analyses from Wang et al. (2015) for the AOSR mining facilities.
Figure S9 shows spatial variations in the ratio of the gridded aircraft-observation-based bin 8 OM emissions (top-down) to the corresponding base-case emissions (bottom-up) for the GEM-MACH 2.5 km grid over the AOSR study area. Except for the Imperial Oil Kearl facility, the top-down OM emissions are more than 2 orders of magnitude larger than those for the base-case study (bottom-up) due to the combination of higher PM emissions (Fig. 9), larger bin 8 mass fraction (Figs. 4 and 10), and the larger OM mass fraction (Fig. 11).
The new estimates of total fugitive dust emissions and the new PM size distribution and speciation profiles were used for two GEM-MACH sensitivity simulations. One of these simulations focussed on the impact of the increases of VOC and primary OM emissions on total organic aerosol and the formation of secondary organic aerosol (SOA; Stroud et al., 2018). The second examined the impact of the increased crustal-material emissions on regional acid deposition by making use of the Wang et al. (2015) PM speciation profile to further speciate the model's crustal material into a base-cation fraction (Makar et al., 2018). Similar to Ahmadov et al. (2015), Stroud et al. (2018) demonstrated that the measurement-derived top-down emissions improved the modeled VOC and organic aerosol (OA) concentration maxima in plumes. Bias was also improved for OA predictions. Their study suggested that intermediate volatile organic compound (IVOC) emissions need to be included as precursors to SOA for further improvement of SOA predictions. In their examination of acidifying deposition in the region, Makar et al. (2018) found that the new aircraft-based top-down emissions improved the model fit to observations, increasing correlation coefficients (R from 0.47 to 0.54) and improving slopes of the model-to-observation best-fit line (slope changed from 0.051 to 0.73, correcting most of the large underestimate in predicted base-cation deposition). The revised fugitive dust estimates from the aircraft study, while resulting in greatly improved model performance relative to the reported emissions, still resulted in an underestimate of base cations relative to observations, implying the need for further improvements to these emissions data.
4.4 Mercury emissions
Mercury emissions from the SMOKE-ready versions of the 2010 Canadian APEI and version 1 of the 2011 United States NEI (NEIv1) were used in phase 2 for creating gridded GEM-MACH-ready mercury emissions. In phase 3 these emissions input files were updated with two AOSR-specific adjustments. First, annual total mercury emissions to air from all NPRI facilities in the 2010 Canadian APEI, including the six AOSR mining facilities, were 3429 kg yr−1. In comparison, the annual total mercury emissions to air reported by all NPRI facilities for 2013 were 2529 kg yr−1, of which only 61 kg were emitted from the surface mining facilities. Thus, for the 2013 field study, the 2013 NPRI reported values were used for the model Hg emissions. Second, for the United States, mercury emissions from off-road vehicles were only available for the state of California in the SMOKE-ready version of the 2011 NEIv1 (https://www.epa.gov/air-emissions-modeling/2011-version-6-air-emissions-modeling-platforms, last access: 15 July 2018), whereas the original 2011 NEIv1 (https://www.epa.gov/air-emissions-inventories/2011-national-emissions-inventory-nei-data, last access: 15 July 2018) included off-road-mobile mercury emissions for other states as well. The amount of off-road-mobile mercury emissions for California was the same in the two inventory versions. Based on the original 2011 NEIv1 inventory, total annual off-road-mobile mercury emissions for the entire United States were 40.9 kg yr−1, of which 26.1 kg yr−1 was from California. Although these off-road-mobile mercury emissions were relatively small compared with other emissions sources (see Table 5) and more than 60 % of the off-road-mobile mercury emissions were from California, the second adjustment was to use off-road-mobile mercury emissions from the original 2011 NEIv1 to add in mercury emissions for the missing states in the off-road-mobile subinventory of the SMOKE-ready version of the 2011 United States NEIv1.
Table 5Sum of source-sector-specific mercury emissions (kg) for the 2011 United States inventory (version 1) and the 2010–2013 Canadian inventory.
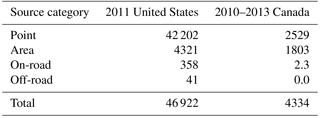
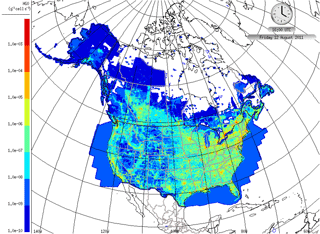
Figure 12Spatial distribution of phase 3 elemental mercury emissions for Canada and the United States for the 10 km continental model grid for 1 h in the afternoon in August. Note logarithmic spacing of the emissions contour intervals; white areas have emissions less than 10−10 g cell−1 s−1.
Table 5 presents a summary of source-specific anthropogenic mercury emissions used for phase 3 for both the United States and Canada. Total 2011 United States annual mercury emissions from all four broad categories were 46 992 kg, of which nearly 90 % was from point sources and the rest was mainly from area sources (9 %). Mercury emissions from on-road and off-road vehicles accounted for less than 1 % of total mercury emissions, and most of these vehicular emissions (90 %) came from on-road vehicles. The summary of 2010–2013 Canadian mercury emissions shows that point sources were the largest anthropogenic source of mercury emissions in Canada (58 %), followed by area sources (42 %), and on-road and off-road vehicle emissions contributed little. Total mercury emissions from Canada for 2010–2013 were about 9 % of those emitted in the United States for 2011. The two adjustments made for phase 3 reduced United States and Canadian anthropogenic mercury emissions by 885 kg yr−1 or less than 2 %. However, emissions of mercury from forest fires were also recognized as a major source (Fraser et al., 2018).
Three mercury species (elemental, divalent gas, and particulate) are considered in the mercury version of the GEM-MACH model (Fraser et al., 2018). Mercury emissions for the Canadian 2013 NPRI point-source emissions were pre-speciated based on the 2006 Canadian point-source emissions inventory used for the 2008 mercury assessment (UNEP, 2008). For other inventories, mercury emissions were reported as unspeciated totals in the 2010 Canadian APEI and the 2011 United States NEIv1. For these other inventories, mercury speciation was carried out using speciation profiles for nine broad source categories following the same methodology used in the U.S. EPA 2005 NEIv4.1 platform. The same profiles had also been used in the U.S. EPA 2002 v3 platform (see Tables 3–14 in U.S. EPA, 2011).
Figure 12 shows the spatial distribution of phase 3 elemental mercury emissions for both Canada and the United States on the 10 km GEM-MACH continental grid for 1 h in the afternoon in August. Most of the mercury emissions are from populated and industrial areas. Figure S10 shows the domain-average percentages of the three mercury species based on total emissions summed over the nine source categories. About 50, 30, and 20 % of the total mercury emissions are in the elemental, divalent gas, and particulate states, respectively. Fraser et al. (2018) present some results from the use of these phase 3 mercury emissions input files.
A number of sets of model-ready emissions input files have been prepared over the past 6 years in three successive phases for the GEM-MACH air quality modeling system in support of the Governments of Canada and Alberta Joint Oil Sands Monitoring (JOSM) plan. These emissions files were used by GEM-MACH to conduct nested AQ forecasts in support of an Oil Sands field campaign carried out in summer 2013 as well as ongoing experimental forecasts since then and retrospective model simulations and analyses for the field-study period. Two GEM-MACH grids were considered: a North American continental grid with 10 km grid spacing and a high-resolution western Canada grid with 2.5 km grid spacing centered over the Athabasca Oil Sands Region (AOSR) of northeastern Alberta, Canada.
Ten available emissions inventories covering the study area were reviewed in phase 1 (2012–2013) and a detailed synthesized or hybrid AQ modelers' emissions inventory was constructed. An important approach developed in phase 1 was to treat three types of major emissions sources within each AOSR mining facility – mine faces, tailings ponds, and extraction plants – as area sources rather than point sources due to their large spatial extent by developing and using three sets of facility-specific and process-specific spatial surrogate fields based on a 2010 GIS shapefile describing the AOSR mines. For phase 2 emissions processing from 2014 to 2015, more up-to-date emissions inventories and other relevant emissions information became available, including continuous emissions monitoring system (CEMS) data sets for 2013 for 17 smokestacks in four AOSR mining facilities and updated 2013-specific AOSR shapefiles.
This paper focused on the phase 3 emissions processing that was carried out from 2016 to 2017. Some of the gaps and recommendations raised in the JOSM report (ECCC & AEP, 2016) were addressed during this phase. Newer Canadian and US inventory compiled for, or close to, 2013 were used. An expanded CEMS data set of hourly SO2 and NOx emissions and smokestack operating characteristics for August–September 2013 was obtained for the entire province of Alberta, increasing the provincial total coverage of point-source SO2 and NOx emissions by CEMS measurements from 31 and 3 % to 77 and 43 %, respectively. New VOC and PM emissions estimates and chemical speciation profiles for the AOSR mining facilities that had been derived from on-site surface observations and aircraft observations made during the 2013 field campaign were processed for several GEM-MACH sensitivity studies. The aircraft-observation-based top-down VOC emissions were about 2 times larger than the bottom-up base-case emissions from the 2013 NPRI (Li et al., 2017). For PM emissions, 2-month PM emissions estimated from the top-down aircraft-observation-based emissions were even larger than the bottom-up NPRI annual emissions for five of the six facilities (Fig. 9). The VOC and PM chemical speciation profiles used to speciate emissions from the AOSR mines were also noticeably different than those used to process the phase 3 base-case emissions. A vegetation database used to estimate biogenic emissions and a land-cover database used in the parameterizations of land-surface processes and dry deposition were also modified to account for the rapid change in vegetation cover and land use in the AOSR region due to year-by-year changes in surface mining activities. In addition to CAC emissions, mercury emissions were also processed to support mercury modeling activities using newly available data sets.
This study also provides specific examples of some common issues related to the preparation of emissions input files for AQ models. First, there is always a time lag between a year of interest and the year in which an emissions inventory becomes available for that year of interest. Second, inventories are always subject to change due to reported corrections or to changes in estimation methodology. Third, if multiple inventories are available for the same region and the same base year, they are unlikely to be in perfect agreement. Fourth, a synthesized or hybrid inventory can provide a more accurate representation of emissions than any of its component inventories. Fifth, extra effort and investigation related to the specific year and region of interest can yield significant improvements over standard emissions-processing methodologies. And sixth, top-down emissions, such as those from aircraft observations, can be used to verify bottom-up emissions and to improve AQ modeling performance, as demonstrated by the companion AQ modeling papers in this special issue (Stroud et al., 2018; Makar et al., 2018).
Nevertheless, although improved sets of emissions input files were generated during phase 3 after a considerable effort to acquire and apply new sources of emissions data representative of the 2013 AOSR field-study period, there are still large uncertainties associated with these emissions. In the following, six areas that still need further improvement are described.
Top-down emissions estimates from aircraft measurements made in late summer 2013 during the AOSR field study show that VOC and PM emissions reported to the NPRI using currently accepted estimation methods might be underestimated for the AOSR facilities (Li et al., 2017). However, these measurements were made during a limited time period (4 weeks) and the mass-balance calculations used to estimate emissions were only applied to a relatively large area (Gordon et al., 2015; Li et al., 2017). Large variations in PM emissions results were also seen from flight to flight for the same facilities, probably related at least in part to the variation of mined volume of oil sands from day to day or recent precipitation. There are thus still issues with the spatial and temporal allocation of emissions to the right location at the right time.
The aircraft measurements also indicated that the VOC speciation reported to NPRI by individual AOSR mining facilities may need to be improved (Li et al., 2017), and additional VOC speciation data should be collected to improve speciation profiles. Moreover, these aircraft measurements were carried out at the facility level, but within these very large facilities the individual VOC species emitted from mine faces, tailings ponds, and plants can be very different. More aircraft measurements, especially at other times of year, and additional measurements of emissions at the sub-facility level, from mine faces, tailings ponds, and plants for multiple AOSR facilities, are needed to confirm and augment the findings of the 2013 field study and to further improve emissions factors, temporal profiles, and chemical speciation profiles used for OS emissions inventories and emissions processing (e.g., Small et al., 2015; Stantec Consulting Ltd. et al., 2016). Given the above differences between field-study measurements and reports, the AOSR mining facilities should also review the methodologies that they employ to estimate and report VOC emissions to NPRI.
The off-road mining fleets in the six AOSR mining facilities are a large source of NOx emissions, but large differences are seen in the emissions estimates for this source sector between different inventories. For example, the 2010 CEMA inventory lists 38 362 t of NOx emissions for this sector, but the 2010 APEI for the same year lists 27 786 t. The 2013 APEI then reduced NOx emissions from the OS off-road mining fleets to 12 370 t. Since mined oil sands increased by 17 % between 2010 and 2013, the significant drop of NOx emissions is probably due to different emissions factors being used for these two inventory years (possibly due in part to the introduction of cleaner heavy-hauler trucks: e.g., M.J. Bradley & Associates LLC, 2008).
Additional sources of information are needed to reconcile the differences amongst existing inventories. One possible data source is satellite remote sensing. For example, a methodology has been developed recently to use repeated satellite measurements of NO2 vertical column density over the AOSR to estimate NOx emissions (McLinden et al., 2014, 2016). Preliminary top-down results from satellite remote sensing show that area-source NOx emissions in the OS area, which are mainly from the off-road fleets, are about 38 kt yr−1 for 2013, comparable to the bottom-up 2010 CEMA inventory. The 2010 CEMA inventory was also deemed to have the best estimation of off-road emissions for the AOSR facilities (ECCC & AEP, 2016). Satellite remote sensing (e.g., McLinden et al., 2014; Shephard et al., 2015; Sioris et al., 2017) and ground-based remote sensing (e.g., Fioletov et al., 2016) should thus be considered in the future for emissions estimation and verification.
There have been ongoing efforts to improve the spatial allocation of emissions within the huge AOSR mining facilities using spatial surrogate fields generated from the locations of mine faces, tailings ponds, and extraction and upgrading plants. For example, the 2010 version of the shapefile used for generating these surrogates was updated in phase 2 based on 2013 satellite images (Zhang et al., 2015). Further improvements, however, are possible. As one example, the spatial surrogate used to allocate emissions from the off-road mining fleet currently allocates all of the emissions to the mine-face locations and does not account for the movement of the heavy-hauler trucks between the mine faces and the extraction plants. Year-specific shapefiles with locations of active mining areas and current boundaries of tailing ponds as well as activity data sets for the actual or average movement of mining vehicles and time spent at locations throughout the mine should be obtained to improve the spatial allocation of off-road emissions for the AOSR mining operations (ECCC & AEP, 2016)
Fugitive VOC emissions from tailing ponds and mine faces are currently provided as annual totals in the inventory. A temperature-based monthly temporal profile was used to allocate the annual emissions to each month while weekly and diurnal temporal profiles were assumed to be constant, which is likely not realistic. For example, nighttime emission rates over the mine faces are likely lower than daytime rates due to lower surface temperatures. In the future, model-predicted or locally measured hourly temperature and wind speed may be used to estimate hourly fugitive VOC emissions if the dependence of fugitive VOC emission rates on temperature and wind speed can be parameterized (Li et al., 2017). Snow cover over the mining areas and ice cover over the ponds during wintertime also affect fugitive VOC emissions and need to be considered. A related issue is that the tailings ponds are of different ages; some are receiving fresh tailings while others have been inactive for years, which may mean lower emission rates due to past off-gassing of more volatile components. Consideration should thus be given to tailings-pond age when allocating VOC emissions between different tailings ponds. A recently completed study (summer 2017) of tailings-pond emissions conducted by ECCC is expected to lead to improved estimates of emissions from these sources.
Top-down fugitive dust emissions estimates based on aircraft observations suggest large underestimates in the reported inventory totals, and GEM-MACH modeling suggests that even these revised estimates, or the fraction of their mass which is composed of base cations, might be underestimated (Makar et al., 2018). Further aircraft-based measurements of fugitive dust emissions and their speciation are needed to improve the emissions inventories used here. A parameterization of wind-blown dust emissions should also be added to GEM-MACH.
For mercury emissions, although unspeciated mercury emissions were obtained from inventories with base years close to 2013, chemical speciation was done crudely using speciation profiles for nine broad source categories. This methodology needs to be updated as more detailed speciation information becomes available in the future.
The pre-phase 3 Canadian CAC (criteria air contaminant) emissions inventory described in the JOSM report (ECCC & AEP, 2016) is available from the ECCC web page at http://donnees.ec.gc.ca/data/air/monitor/source-emissions-monitoring-oil-sands-region/source-emissions-oil-sands-region/?lang=en (ECCC, 2018). The Canadian SMOKE-ready CAC emissions inventories compiled for the phase 3 base case and sensitivity studies are available from the ECCC weblink http://collaboration.cmc.ec.gc.ca/cmc/arqi/ACP-2017-1215/CAC_inventory.tz (last access: 18 July 2018). The SMOKE-ready mercury emissions used for this study are available from the ECCC weblink http://collaboration.cmc.ec.gc.ca/cmc/arqi/ACP-2017-1215/Mercury_inventory.tz (last access: 18 July 2018). SMOKE-ready CAC emissions for the United States are available from the U.S. EPA's website for their emissions modeling platforms at https://www.epa.gov/air-emissions-modeling/emissions-modeling-platforms (EPA, 2018).
Acronym | Expansion |
AAEI | Alberta Air Emissions Inventory |
ADOM-2 | Acid Deposition and Oxidant Model, version 2 |
AEP | Alberta Environment and Parks (formerly AESRD) |
AER | Alberta Energy Regulator |
AESRD | Alberta Environment and Sustainable Resource Development (now AEP) |
AOSR | Athabasca Oil Sands Region |
APEI | Air Pollutant Emission Inventory |
AQ | air quality |
BEIS | Biogenic Emission Inventory System |
BELD | Biogenic Emissions Landuse Database |
CAC | criteria air contaminants |
CEMA | Cumulative Environmental Management Association |
CEMS | continuous emission monitoring system |
CNRL | Canadian Natural Resources Limited |
ECCC | Environment and Climate Change Canada |
EIA | environmental impact assessment |
EPA | Environmental Protection Agency (United States) |
EPEA | Environmental Protection and Enhancement Act (Alberta) |
FSSP | forward scattering spectrometer probe |
GEM-MACH | Global Environmental Multiscale–Modelling Air quality and CHemistry |
GIS | geographic information system |
JOSM | Joint Oil Sands Monitoring plan |
LAI | leaf area index |
LARP | Lower Athabasca Regional Plan |
NEI | National Emissions Inventory |
NPRI | National Pollutant Release Inventory |
OS | oil sands |
PFT | paraffinic froth treatment |
PM | particulate matter |
SCC | Source Classification Code |
SMOKE | Sparse Matrix Operator Kernel Emissions |
TERRA | Top-down Emission Rate Retrieval Algorithm |
UHSAS | ultra-high sensitivity aerosol spectrometer |
UOG | upstream oil and gas |
VOC | volatile organic compound |
WBEA | Wood Buffalo Environmental Association |
The supplement related to this article is available online at: https://doi.org/10.5194/acp-18-10459-2018-supplement.
JZ performed the analysis and compilation of the emissions inventory, generated the air quality model-ready emissions input files, and wrote and revised the manuscript. MDM oversaw the compilation of the emissions inventory and generation of emissions and wrote and revised the manuscript. QZ assisted with the analysis and compilation of inventory and with the generation of air quality model-ready emissions input files. PAM provided suggestions for improvements of emissions for air quality modeling. PB assisted with inventory analysis and preparation. GM performed the inventory analysis. PL estimated PM emissions based on aircraft observations. SML estimated VOC emissions based on aircraft observations.
The authors declare that they have no conflict of interest.
This article is part of the special issue “Atmospheric emissions from oil sands development and their transport, transformation and deposition (ACP/AMT inter-journal SI)”. It is not associated with a conference.
Emissions inventories used in this study were provided by the Pollutant
Inventory and Reporting Division of ECCC, the Cumulative Environmental
Management Association, and the U.S. Environmental Protection Agency. The
Alberta CEMS data were provided by Marilyn Albert, Ewa Przybylo-Komar,
Katelyn Mackay, and Tara-Lynn Carmody of Data Management and Stewardship,
Corporate Services Division, Alberta Environment and Parks. We also
appreciate the information provided by Sunny Cho and Richard Melick of Albert
Environment and Parks about emissions in the AOSR and the province of
Alberta. We thank our colleagues in the Program Integration Division of ECCC,
Alicia Berthiaume and Anne Monette, and colleagues in the Pollutant Inventory
and Reporting Division of ECCC for their careful internal review of the
manuscript. We also appreciate the external review comments provided by
Canada's Oil Sands Innovation Alliance (COSIA). Finally, we are very grateful
for the insightful comments on the manuscript from two anonymous reviewers.
The readability and quality of the paper was greatly improved by addressing
their comments. This project was supported by the Climate Change and Air Quality Program
of ECCC and the Joint Oil Sands Monitoring (JOSM) program of ECCC and AEP.
Edited by: Jan W.
Bottenheim
Reviewed by: two anonymous referees
AESRD (Alberta Environment & Sustainable Resource Development): Report on the Inventory of Oil Sands Inventories, internal report, April, 88 pp., 2013.
Ahmadov, R., McKeen, S., Trainer, M., Banta, R., Brewer, A., Brown, S., Edwards, P. M., de Gouw, J. A., Frost, G. J., Gilman, J., Helmig, D., Johnson, B., Karion, A., Koss, A., Langford, A., Lerner, B., Olson, J., Oltmans, S., Peischl, J., Pétron, G., Pichugina, Y., Roberts, J. M., Ryerson, T., Schnell, R., Senff, C., Sweeney, C., Thompson, C., Veres, P. R., Warneke, C., Wild, R., Williams, E. J., Yuan, B., and Zamora, R.: Understanding high wintertime ozone pollution events in an oil- and natural gas-producing region of the western US, Atmos. Chem. Phys., 15, 411–429, https://doi.org/10.5194/acp-15-411-2015, 2015.
Akingunola, A., Makar, P. A., Zhang, J., Darlington, A., Li, S.-M., Gordon, M., Moran, M. D., and Zheng, Q.: A chemical transport model study of plume-rise and particle size distribution for the Athabasca oil sands, Atmos. Chem. Phys., 18, 8667–8688, https://doi.org/10.5194/acp-18-8667-2018, 2018.
Alberta Energy: Facts and Statistics, Alberta Energy's website, available at: https://web.archive.org/web/20171223160423/http://www.energy.alberta.ca:80/OilSands/791.asp, last access: 15 July 2018.
Alberta Energy Regulator: ST39 Alberta Mineable Oil Sands Plant Statistics (Updated monthly, 3 months in arrears), Data in MS Excel, February, available at: https://www.aer.ca/documents/sts/ST39-2013.xls (last access: 18 May 2017), 2014.
Alberta Energy Regulator: ST98 Alberta's Energy Reserves and Supply/Demand Outlook, February, available at: http://www.aer.ca/providing-information/data-and-reports/statistical-reports/st98, last access: 15 July 2018a.
Alberta Energy Regulator: In situ Recovery, available at: http://www.aer.ca/providing-information/by-topic/oil-sands/in-situ-recovery, last access: 15 July 2018b.
Baumgardner, D., Dye, J. E., and Gandrud, B. W.: Calibration of the forward scattering spectrometer probe used on the ER-2 during the Airborne Antarctic Ozone Experiment, J. Geophys. Res., 94, 16475–16480, https://doi.org/10.1029/JD094iD14p16475, 1989.
Cenovus FCCL Ltd.: Environmental Impact Assessment – Cenovus FCCL Ltd. Narrows Lake Project, Volume 3 – Air quality, noise and health, 1607 pp., available at: https://open.alberta.ca/dataset/e41cfccf-1ab3-40ca-8efd-4079633216ac/resource/9f1ab94d-379c-4c7d-8544-ca709b3cacc4/download/Volume-3.pdf (last access: 19 May 2017), 2010.
Choi, D.: MOVES2014 Overview and Plans for the Future, report for FACA MOVES Review Work Group, available at: https://www.epa.gov/sites/production/files/2016-10/documents/moves2014-overview-and-plans-for-the-future.pdf (last access: 1 November 2017), 2016.
Clearstone Engineering Ltd.: Technical Report, Vol. 2, Overview of CAC and Other Priority Substance Emissions Inventory, prepared for Environment Canada, 354 pp., 2014a.
Clearstone Engineering Ltd.: Technical Report, Volume 3, UOG Emissions Inventory Methodology Manual, prepared for Environment Canada, 217 pp., 2014b.
Clearstone Engineering Ltd.: Technical Report, Volume 4, Compendium of Factors and Terminology, prepared for Environment Canada, 180 pp., 2014c.
Clearstone Engineering Ltd.: Letter Report, Documentation for UOG emission inventory extrapolation database (ExtrapolateR.accdb), prepared for Environment Canada, 17 pp., 2014d.
Davies, M., Person, R., Nopmongcol, U., Shah T., Vijayaraghavan, K., Morris, R., and Picard, D.: Lower Athabasca Region Source and Emission Inventory, report prepared by Stantec Consulting Ltd. and ENVIRON International Corporation for Cumulative Environmental Management Association – Air Working Group, 274 pp., available at: http://library.cemaonline.ca/ckan/dataset/0cfaa447-410a-4339-b51f-e64871390efe/resource/fba8a3b0-72df-45ed-bf12-8ca254fdd5b1/download/larsourceandemissionsinventory.pdf (last access: 24 October 2017), 2012.
Dickson, R. J. and Oliver, W. R.: Emissions models for regional air quality studies, Environ. Sci. Technol., 25, 1533–1535, 1991.
ECCC (Environment and Climate Change Canada): Guide for Reporting to the National Pollutant Release Inventory (NPRI), 2016 and 2017, available at: http://www.ec.gc.ca/inrp-npri/AFC98B81-A734-4E91-BD16-C5998F0DDE6B/2016-17 Guide for Reporting - EN.pdf (last access: 19 May 2017), 2016.
ECCC: Source Emissions, Oil Sands Region, available at: http://donnees.ec.gc.ca/data/air/monitor/source-emissions-monitoring-oil-sands-region/source-emissions-oil-sands-region/?lang=en, last access: 18 July 2018.
ECCC & AEP (Environment and Climate Change Canada & Alberta Environment and Parks): Joint Oil Sands Monitoring Program Emissions Inventory Compilation Report, 146 pp., available at: http://aep.alberta.ca/air/reports-data/documents/JOSM-EmissionsInventoryReport-Jun2016.pdf (last access: 15 July 2018), 2016.
Eldering, A. and Cass, G. R.: Source-oriented model for air pollutant effects on visibility, J. Geophys. Res., 101, 19343–19369, 1996.
Environment Canada: 2012 Air Pollutant Emissions Inventory & Trends: Methodology, Summary & Highlights, Internal report, 133 pp., 2014.
EPA: Emissions Modeling Platforms, available at: https://www.epa.gov/air-emissions-modeling/emissions-modeling-platforms, last access: 18 July 2018.
Eyth, A., Mason, R., and Zubrow, A.: Development and Status of EPA's 2011 Modeling Platform, 12th CMAS Conference, 28–30 October, Chapel Hill, North Carolina, available at: https://www.cmascenter.org/conference//2013/slides/eyth_development_status_2013.pptx (last access: 15 July 2018), 2013.
Fioletov, V. E., McLinden, C. A., Cede, A., Davies, J., Mihele, C., Netcheva, S., Li, S.-M., and O'Brien, J.: Sulfur dioxide (SO2) vertical column density measurements by Pandora spectrometer over the Canadian oil sands, Atmos. Meas. Tech., 9, 2961–2976, https://doi.org/10.5194/amt-9-2961-2016, 2016.
Fraser, A., Dastoor, A., and Ryjkov, A.: How important is biomass burning in Canada to mercury contamination?, Atmos. Chem. Phys., 18, 7263–7286, https://doi.org/10.5194/acp-18-7263-2018, 2018.
Gordon, M., Li, S.-M., Staebler, R., Darlington, A., Hayden, K., O'Brien, J., and Wolde, M.: Determining air pollutant emission rates based on mass balance using airborne measurement data over the Alberta oil sands operations, Atmos. Meas. Tech., 8, 3745–3765, https://doi.org/10.5194/amt-8-3745-2015, 2015.
Gordon, M., Makar, P. A., Staebler, R. M., Zhang, J., Akingunola, A., Gong, W., and Li, S.-M.: A Comparison of Plume Rise Algorithms to Stack Plume Measurements in the Athabasca Oil Sands, Atmos. Chem. Phys. Discuss., https://doi.org/10.5194/acp-2017-1093, in review, 2017.
Houyoux, M. R., Vukovich, J. M., Coats, Jr., C. J., Wheeler, N. J. M., and Kasibhatla, P. S.: Emission inventory development and processing for the Seasonal Model for Regional Air Quality (SMRAQ) project, J. Geophys. Res., 105, 9079–9090, 2000.
Imperial Oil Ltd.: Environmental Impact Assessment – Kearl Oil Sands Project, Volume 5 – Air quality & noise, 706 pp., available at: http://www.acee.gc.ca/050/documents_staticpost/cearref_16237/KR-0007-5.pdf (last access: 19 May 2017), 2005.
JOSM (Joint Oil Sands Monitoring Plan): Integrated Monitoring Plan for the Oil Sands: Air Quality Component, 72 pp., available at: http://publications.gc.ca/site/eng/394253/publication.html (last access: 6 November 2017), 2011.
Karion, A., Sweeney, C., Petron, G., Frost, G., Hardesty, R. M., Kofler, J., Miller, B. R., Newberger, T., Wolter, S., Banta, R., Brewer, A., Dlugokencky, E., Lang, P., Montzka, S. A., Schnell, R., Tans, P., Trainer, M., Zamora, R., and Conley, S.: Methane emissions estimate from airborne measurements over a western United States natural gas field, Geophys. Res. Lett., 40, 4393–4397, 2013.
Kinnee, E., Geron, C., and Pierce, T.: United States land use inventory for estimating biogenic ozone precursor emissions, Ecol. Appl., 7, 46–58, 1997.
Li, S.-M., Leithead, A., Moussa, S. G., Liggio, J., Moran, M. D., Wang, D., Hayden, K., Darlington, A., Gordon, M., Staebler, R., Makar, P. A., Stroud, C. A., McLaren, R., Liu, P. S. K., O'Brien, J., Mittermeier, R. L., Zhang, J., Marson, G., Cober, S. G., Wolde, M., and Wentzell, J. J. B.: Differences between measured and reported volatile organic compound emissions from oil sands facilities in Alberta, Canada, P. Natl. Acad. Sci. USA, 114, E3756–E3765, https://doi.org/10.1073/pnas.1617862114, 2017.
Liggio, J., Li, S.-M., Hayden, K., Taha, Y. M., Stroud., C., Darlington, A., Drollette, B. D., Gordon, M., Lee, P., Liu, P., Leithead, A., Moussa, S. G., Wang, D., O'Brien, J., Mittermeier, R. L., Brook, J. R., Lu, G., Staebler, R. M., Han, Y., Tokarek, T. W., Osthoff, H. D., Makar, P. A., Zhang, J., Plata, D. L., and Gentner, D. R.: Oil sands operations as a large source of secondary organic aerosols, Nature, 534, 91–94, https://doi.org/10.1038/nature17646, 2016.
Makar, P. A., Moran, M. D., Scholtz, M. T., and Taylor, A.: Speciation of volatile organic compound emissions for regional air quality modelling of particulate matter and ozone. J. Geophys. Res., 108, 4041, https://doi.org/10.1029/2001JD000797, 2003.
Makar, P. A., Zhang, J., Akingunola, A., Moran, M. D., Hayden, K., Li, S.-M., Staebler, R., and Aliabadi, A.: The Sensitivity of Model Plume Rise to Emissions Inputs, 7th International Workshop on Air Quality Forecasting Research (IWAQFR), 1–3 September 2015, College Park, MD, available at: http://www.arl.noaa.gov/documents/IWAQFR/Posters2015/S4_Makar_Poster_IWAQFR_2015.pdf (last access: 15 July 2018), 2015.
Makar, P. A., Akingunola, A., Aherne, J., Cole, A. S., Aklilu, Y.-A., Zhang, J., Wong, I., Hayden, K., Li, S.-M., Kirk, J., Scott, K., Moran, M. D., Robichaud, A., Cathcart, H., Baratzedah, P., Pabla, B., Cheung, P., Zheng, Q., and Jeffries, D. S.: Estimates of Exceedances of Critical Loads for Acidifying Deposition in Alberta and Saskatchewan, Atmos. Chem. Phys. Discuss., https://doi.org/10.5194/acp-2017-1094, in review, 2018.
Marson G.: Oil Sands Emission Inventories Review, draft internal report, Environment and Climate Change Canada, 56 pp., 2013.
McLinden, C. A., Fioletov, V., Boersma, K. F., Kharol, S. K., Krotkov, N., Lamsal, L., Makar, P. A., Martin, R. V., Veefkind, J. P., and Yang, K.: Improved satellite retrievals of NO2 and SO2 over the Canadian oil sands and comparisons with surface measurements, Atmos. Chem. Phys., 14, 3637–3656, https://doi.org/10.5194/acp-14-3637-2014, 2014.
McLinden, C. A., Fioletov, V., Krotkov, N. A., Li, C., Boersma, K. F., and Adams, C.: A decade of change in NO2 and SO2 over the Canadian oil sands as seen from space, Environ. Sci. Technol., 50, 331–337, 2016.
M.J. Bradley & Associates LLC: Evaluation of vehicle emissions reduction options for the oil sand mining fleet, final report prepared for Environment Canada, 40 pp., available at: http://www.mjbradley.com/sites/default/files/Oil Sands Retrofit Feasibility Study Mar08_0.pdf (last access: 8 September 2017), 2008.
Moran, M. D., Ménard, S., Gravel, S., Pavlovic, R., and Anselmo, D.: RAQDPS Versions 1.5.0 and 1.5.1: Upgrades to the CMC operational Regional Air Quality Deterministic Prediction System released in October 2012 and February 2013, Technical note, Canadian Meteorological Centre, Montreal, available at: http://collaboration.cmc.ec.gc.ca/cmc/cmoi/product_guide/docs/lib/op_systems/doc_opchanges/technote_raqdps_20130226_e.pdf (last access: 18 July 2018), 2013a.
Moran, M. D., Dastoor, A., and Morneau, G.: Long-range transport of air pollutants and regional and global AQ modelling, in: Air Quality Management – Canadian Perspectives on a Global Issue, edited by: Taylor, E. and McMillan, A., Springer, Dordrecht, 69–98, 2013b.
Moran, M. D., Ménard, S., Pavlovic, R., Anselmo, D., Antonopoulos, S., Makar, P. A., Gong, W., Stroud, C., Zhang, J., Zheng, Q., Robichaud, A., Landry, H., Beaulieu, P.-A., Gilbert, S., Chen, J., and Kallaur, A.: Recent advances in Canada's national operational AQ forecasting system. Air Pollution Modeling and its Application XXII, NATO Science for Peace and Security Series C: Environmental Security, edited by: Steyn, D. G., Builtjes, P. J. H., and Timmermans, R. M. A., Springer, Dordrecht, 215–220, https://doi.org/10.1007/978-94-007-5577-2_4, 2014.
Moran, M. D., Zheng, Q., Zhang, J., and Pavlovic, R.: RAQDPS Version 013: Upgrades to the CMC Operational Regional Air Quality Deterministic Prediction System Released in June 2015, Technical note, November 2015, Canadian Meteorological Centre, Montreal, 54 pp., available at: http://collaboration.cmc.ec.gc.ca/cmc/cmoi/product_guide/docs/lib/op_systems/doc_opchanges/Technical_Note_GEM-MACH10_v1.5.3+SET2.1.1_Emissions_9Nov2015.pdf (last access: 15 July 2018), 2015.
Peischl, J., Ryerson, T. B., Aikin, K. C., de Gouw, J. A., Gilman, J. B., Holloway, J. S., Lerner, B. M., Nadkarni, R., Neuman, J. A., Nowak, J. B., Trainer, M., Warneke, C., and Parrish, D. D.: Quantifying atmospheric methane emissions from the Haynesville, Fayetteville, and northeastern Marcellus shale gas production regions, J. Geophys. Res.-Atmos., 120, 2119–2139, 2015.
Pierce Jr., T. E., Kinnee, E. J., and Geron, C. D.: Development of a 1-km vegetation database for modeling biogenic fluxes of hydrocarbons and nitric oxide, Sixth International Conference on Air Surface Exchange of Gases and Particles, 3–7 July, Edinburgh, England, available at: https://www.epa.gov/sites/production/files/2015-08/beld3_web.ppsx (last access: 15 July 2018), 2000.
Reff, A., Bhave, P. V., Simon, H., Pace, T. G., Pouliot, G. A., Mobley, J. D., and Houyoux, M.: Emissions inventory of PM2.5 trace elements across the United States, Environ. Sci. Technol., 43, 5790–5796, 2009.
Sassi, M., Cousineau, S., Duhamel, A., Ménard, S., Racine, J., Zaganescu, C., and Mashayekhi, R.: The 2013 Canadian air quality modelling platform and the base and future cases used for policy regulations, 15th Annual CMAS Conference, 24–26 October 2016, Chapel Hill, NC, available at: https://www.cmascenter.org/conference//2016/abstracts/sassi_2013_canadian_2016.pdf (last access: 15 July 2018), 2016.
Shephard, M. W., McLinden, C. A., Cady-Pereira, K. E., Luo, M., Moussa, S. G., Leithead, A., Liggio, J., Staebler, R. M., Akingunola, A., Makar, P., Lehr, P., Zhang, J., Henze, D. K., Millet, D. B., Bash, J. O., Zhu, L., Wells, K. C., Capps, S. L., Chaliyakunnel, S., Gordon, M., Hayden, K., Brook, J. R., Wolde, M., and Li, S.-M.: Tropospheric Emission Spectrometer (TES) satellite observations of ammonia, methanol, formic acid, and carbon monoxide over the Canadian oil sands: validation and model evaluation, Atmos. Meas. Tech., 8, 5189–5211, https://doi.org/10.5194/amt-8-5189-2015, 2015.
Sioris, C. E., McLinden, C. A., Shephard, M. W., Fioletov, V. E., and Abboud, I.: Assessment of the aerosol optical depths measured by satellite-based passive remote sensors in the Alberta oil sands region, Atmos. Chem. Phys., 17, 1931–1943, https://doi.org/10.5194/acp-17-1931-2017, 2017.
Small, C. C., Cho, S., Hashishoa, Z., and Ulricha, A. C.: Emissions from oil sands tailings ponds: Review of tailings pond parameters and emission estimates, J. Petrol. Sci. Eng., 127, 490–501, 2015
Stantec Consulting Ltd., Clearstone Engineering Ltd., and Intrinsik Environmental Sciences Inc.: Air Pollutant and GHG Emissions from Mine Faces and Tailings Ponds, prepared for Alberta Environmental Monitoring, Evaluation and Reporting Agency, 413 pp., 2016.
Stroud, C. A., Morneau, G., Makar, P. A., Moran, M. D., Gong, W., Pabla, B., Zhang, J., Bouchet, V. S., Fox, D., Venkatesh, S., Wang, D., and Dann, T.: OH-reactivity of volatile organic compounds at urban and rural sites across Canada: Evaluation of air quality model predictions using speciated VOC measurements, Atmos. Environ., 42, 7746–7756, 2008.
Stroud, C. A., Makar, P. A., Zhang, J., Moran, M. D., Akingunola, A., Li, S.-M., Leithead, A., Hayden, K., and Siu, M.: Air Quality Predictions using Measurement-Derived Organic Gaseous and Particle Emissions in a Petrochemical-Dominated Region, Atmos. Chem. Phys. Discuss., https://doi.org/10.5194/acp-2018-93, in review, 2018.
UNEP Chemical Branch: The Global Atmospheric Mercury Assessment: Sources, Emissions and Transport, UNEP-Chemicals, Geneva, 42 pp., https://wedocs.unep.org/bitstream/handle/20.500.11822/11517/UNEP_GlobalAtmosphericMercuryAssessment_May2009.pdf?sequence=1&isAllowed=y (last access: 15 November 2017), 2008.
U.S. EPA (U.S. Environmental Protection Agency): Technical Support Document: Preparation of Emissions Inventories for the Version 4, 2005-based Platform, 73 pp., available at: ftp://ftp.epa.gov/EmisInventory/2005v4/2005_emissions_tsd_07jul2010.pdf (last access: 30 August 2017), 2010.
U.S. EPA (U.S. Environmental Protection Agency): Technical Support Document: Preparation of Emissions Inventories for the Version 4.1, 2005-based Platform, 76 pp., available at: https://www.epa.gov/sites/production/files/2015-11/documents/2005v4.1_main.pdf (last access: 15 November 2017), 2011.
U.S. EPA (U.S. Environmental Protection Agency): Technical Support Document: Preparation of Emissions Inventories for the Version 6.2, 2011 Emissions Modeling Platform, 204 pp., available at: https://www.epa.gov/sites/production/files/2015-10/documents/2011v6_2_2017_2025_emismod_tsd_aug2015.pdf (last access: 8 August 2017), 2015.
Wang, X., Chow, J. C., Kohl, S. D., Percy, K. E., Legge, A. H., and Watson, J. G.: Characterization of PM2.5 and PM10 fugitive dust source profiles in the Athabasca Oil Sands Region, J. Air Waste Manage., 65, 1421–1433, https://doi.org/10.1080/10962247.2015.1100693, 2015.
Whaley, C. H., Makar, P. A., Shephard, M. W., Zhang, L., Zhang, J., Zheng, Q., Akingunola, A., Wentworth, G. R., Murphy, J. G., Kharol, S. K., and Cady-Pereira, K. E.: Contributions of natural and anthropogenic sources to ambient ammonia in the Athabasca Oil Sands and north-western Canada, Atmos. Chem. Phys., 18, 2011–2034, https://doi.org/10.5194/acp-18-2011-2018, 2018.
Zhang, J., Zheng, Q., Moran, M. D., Makar, P. A., Akingunola, A., Li, S.-M., Marson, G., Gordon, M., Melick, R., and Cho, S.: Emissions preparation for high-resolution air quality modelling over the Athabasca oil sands region of Alberta, Canada, 21st Intern. Emissions Inventory Conference, 13–17 April, San Diego, 18 pp., available at: http://www.epa.gov/ttn/chief/conference/ei21/session1/zhang_emissions.pdf (last access: 15 July 2018), 2015.
- Abstract
- Introduction
- Emissions inventories used for the base-case emissions
- Phase 3 emissions processing for GEM-MACH 2013 base-case simulations
- Additional phase 3 emissions processing for GEM-MACH sensitivity and scenario studies
- Summary and future work
- Data availability
- Appendix A: List of acronyms used in the paper
- Author contributions
- Competing interests
- Special issue statement
- Acknowledgements
- References
- Supplement
- Abstract
- Introduction
- Emissions inventories used for the base-case emissions
- Phase 3 emissions processing for GEM-MACH 2013 base-case simulations
- Additional phase 3 emissions processing for GEM-MACH sensitivity and scenario studies
- Summary and future work
- Data availability
- Appendix A: List of acronyms used in the paper
- Author contributions
- Competing interests
- Special issue statement
- Acknowledgements
- References
- Supplement