the Creative Commons Attribution 4.0 License.
the Creative Commons Attribution 4.0 License.
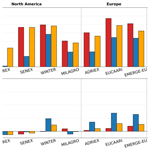
Exploring the processes controlling secondary inorganic aerosol: evaluating the global GEOS-Chem simulation using a suite of aircraft campaigns
Olivia G. Norman
Colette L. Heald
Solomon Bililign
Pedro Campuzano-Jost
Marc N. Fiddler
Jaime R. Green
Jose L. Jimenez
Katharina Kaiser
Jin Liao
Ann M. Middlebrook
Benjamin A. Nault
John B. Nowak
Johannes Schneider
André Welti
Secondary inorganic aerosols (sulfate, nitrate, and ammonium, SNA) are major contributors to fine particulate matter. Predicting concentrations of these species is complicated by the cascade of processes that control their abundance, including emissions, chemistry, thermodynamic partitioning, and removal. In this study, we use 11 flight campaigns to evaluate the GEOS-Chem model performance for SNA. Across all the campaigns, the model performance is best for sulfate (R2 = 0.51; normalized mean bias (NMB) = 0.11) and worst for nitrate (R2=0.22; NMB = 1.76), indicating substantive model deficiencies in the nitrate simulation. Thermodynamic partitioning reproduces the total particulate nitrate well (R2=0.79; NMB = 0.09), but actual partitioning (i.e., ε(NO NO TNO3) is challenging to assess given the limited sets of full gas- and particle-phase observations needed for ISORROPIA II. In particular, ammonia observations are not often included in aircraft campaigns, and more routine measurements would help constrain sources of SNA model bias. Model performance is sensitive to changes in emissions and dry and wet deposition, with modest improvements associated with the inclusion of different chemical loss and production pathways (i.e., acid uptake on dust, N2O5 uptake, and NO photolysis). However, these sensitivity tests show only modest reduction in the nitrate bias, with no improvement to the model skill (i.e., R2), implying that more work is needed to improve the description of loss and production of nitrate and SNA as a whole.
- Article
(7380 KB) - Full-text XML
-
Supplement
(8325 KB) - BibTeX
- EndNote
Aerosols (also known as particulate matter, PM) in our atmosphere are associated with poor air quality (Malm et al., 2000) and the attendant elevated risk of human premature mortality (Pope and Dockery, 2006; Huang et al., 2012), as well as changes in our climate (Lohmann and Feichter, 2005; Myhre et al., 2013). A major component of fine particulate matter (PM2.5) is secondary inorganic aerosols, which include sulfate (SO), nitrate (NO), and ammonium (NH). While other inorganic species, such as chloride (Cl−), can be locally important (Haskins et al., 2018; Gani et al., 2019), sulfate, nitrate, and ammonium (hereafter SNA) are the dominant contributors to secondary inorganic fine aerosol worldwide, contributing between one-third and three-quarters of measured fine non-refractory aerosols (Zhang et al., 2007). These inorganic aerosols are the major aerosol constituent responsible for the degradation of air quality associated with industrialization (e.g., in the United States and Europe in the 1970s and 1980s and China in the early 2000s) and subsequent improvements with the implementation of emissions control technology (Leibensperger et al., 2012; Geng et al., 2017). SNA are also the principal agents of historical aerosol climate forcing (IPCC, 2021). SNA themselves are not directly emitted but instead are formed in the atmosphere from precursor gases that have a range of natural and anthropogenic sources. However, connecting the response of SNA concentrations to changes in emissions can be challenging because many non-emission-related processes affect these aerosols (e.g., chemical oxidation, thermodynamic partitioning, wet and dry deposition; Pye et al., 2009; Paulot et al., 2017; Shah et al., 2018; Li et al., 2021; Zhai et al., 2021a). Understanding these formation and loss processes is key to characterizing aerosol trends and impacts on a global scale.
Emissions of sulfur dioxide (SO2), nitrogen oxides (NOx), and ammonia (NH3) provide the source for sulfate, nitrate, and ammonium aerosols. SO2 and NOx emissions are dominated by fossil fuel combustion. The major sources of NH3 are agricultural emissions, originating from livestock and fertilizer use, and from vehicular emissions in urban areas (e.g., Phan et al., 2013; Sun et al., 2017). Other important sources include volcanoes and the oxidation of oceanic dimethyl sulfide (for SO2), soils and biomass burning (for NH3 and NOx), and lightning (for NOx). Upon emission, SO2 is oxidized in both the gas and aqueous phase to form acidic sulfate aerosols. Similarly, the formation of inorganic nitrate is mainly through the oxidation of NOx into nitric acid (HNO3; Alexander et al., 2009). The very low-saturation vapor pressure of sulfuric acid implies that this species is primarily found in the particle phase (Seinfeld and Pandis, 2016). In contrast, thermodynamic partitioning controls the amount of nitrate and ammonium in the gas and particle phase (i.e., between HNO3 and NO for nitrate and NH3 and NH for ammonium). This partitioning is dependent on relative humidity, temperature, and pH, where higher relative humidity, lower temperature, and higher aerosol pH favors nitrate partitioning into the particle phase (Fountoukis and Nenes, 2007; Guo et al., 2016). Ammonia reacts with both acidic sulfate aerosols (to form different salts, e.g., ammonium bisulfate, ammonium sulfate) and nitrate (to form particulate ammonium nitrate; Seinfeld and Pandis, 2016). Volatile organic compounds (VOCs) can also act as a local control on SNA concentrations since they are directly connected to oxidation capacity and also are involved in alternative loss pathways for nitrate radicals (Aksoyoglu et al., 2017; Womack et al., 2019). Therefore, nitrate formation depends not only on the amount of NOx emitted but also on the amount of ammonia and sulfate, ambient conditions (relative humidity and temperature), and VOC and oxidant concentrations. Also relevant are the loss processes, which include dry and wet deposition (affecting both SNA and its precursors) and chemical losses (e.g., uptake by dust, nitrate photolysis). These formation and loss processes, and in turn SNA concentrations, are expected to respond to future changes in precursor emissions and climate (Dawson et al., 2007; Pye et al., 2009; Vasilakos et al., 2018; Aksoyoglu et al., 2020), but predicting the magnitude and direction of the response depends on how well models capture the complex, nonlinear system that describes the life cycle of atmospheric SNA.
Global atmospheric chemistry models incorporate these mechanisms of SNA production and loss. Past studies have evaluated the SNA simulation in a range of models using surface observations and aircraft campaigns; the results across models can vary substantially, particularly for nitrate (Mezuman et al., 2016; Bian et al., 2017; Chen et al., 2019; Nault et al., 2021; Reifenberg et al., 2022). Large variations in how nitrate production, partitioning, and loss is described drives differences in simulated nitrate, which can result in modeled total nitrate burden (fine plus coarse PM) varying by a factor of 13 (Bian et al., 2017), with some models underestimating nitrate and others overestimating nitrate. We also note that many global models neglect the formation of ammonium nitrate entirely (Gliß et al., 2021; Thornhill et al., 2021). Generally, the sulfate simulation is more consistent and reliable across the different models (Bian et al., 2017; Nault et al., 2021).
In this study, we use a single model (GEOS-Chem) to systemically evaluate SNA performance. Previous assessments of the global chemical transport model GEOS-Chem have focused on one region or used one specific field campaign. These model evaluation studies have found sulfate is well captured and that ammonium and nitrate are overestimated in Europe (Park et al., 2004), the US (Park et al., 2004; Heald et al., 2012; Zhang et al., 2012), and over South Korea (Travis et al., 2022; Zhai et al., 2023). More localized analyses in the US have shown exceptions to this trend, with underestimates in simulated nitrate in California (Heald et al., 2012; Schiferl et al., 2014) and an unbiased nitrate simulation in the northeastern US in wintertime (Shah et al., 2018). Various theories have been suggested to explain these model biases, including deficient emissions inventories (Park et al., 2004; Schiferl et al., 2014), underestimated deposition of HNO3 (Heald et al., 2012; Travis et al., 2022), overestimated N2O5 hydrolysis (Zhang et al., 2012; Heald et al., 2012), and uptake of acidic gases on coarse dust (Heald et al., 2012; Zhai et al., 2023). These studies provide insight into some of the key processes that may be misrepresented or missing from models such as GEOS-Chem that are adversely affecting simulated SNA concentrations. However, their local focus with various model versions (including changing descriptions of the chemistry and meteorology) make it challenging to generalize these results. Here, we use a suite of 11 aircraft campaigns spanning multiple regions of the world to provide a more comprehensive and consistent global evaluation of GEOS-Chem SNA performance. We also explore the key processes controlling SNA concentrations, identifying those that may contribute to model bias.
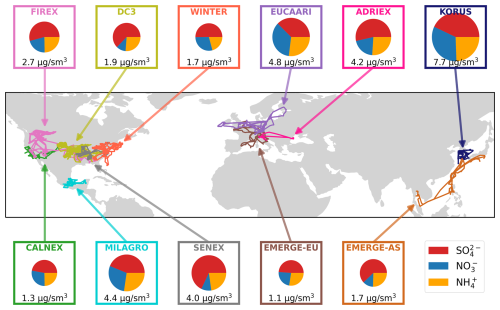
Figure 1Flight tracks for the airborne campaigns used in this analysis. Pie charts show mean relative contributions of sulfate (red), nitrate (blue), and ammonium (yellow) to total SNA for each individual campaign. The area of the pie charts are scaled based on the mean total SNA for each campaign, which is also reported below the pie chart. Only points below 5 km are included. Information about the year and season for each campaign is included in Table 1.
This study explores observations from 11 airborne campaigns that span different regions of the world and almost 2 decades (2004–2019). As a result, these campaigns represent a wide range of chemical regimes and emission scenarios. The campaigns are listed in Table 1, including the dates, locations, and primary references. These campaigns were selected because they all (1) share a common measurement technique for SNA concentrations and (2) are not representative of remote conditions, and thus they generally have higher concentrations of SNA that are well above detection limits. The campaigns all took place in the Northern Hemisphere in one of three general regions: North America (NA), Europe (EU), or Asia (AS). There are at least two campaigns in each area but with a large geographical sampling bias (> 50 % of the campaigns) towards campaigns in the NA region, particularly over the US. Figure 1 shows the campaign flight tracks. Figure 1 also shows panels for each campaign with a pie chart representing the fractional contribution of all three SNA species to the total measured SNA (measurements described below). Below each pie chart is the mean observed total SNA. Values are reported in units of µg sm−3, standardized at standard temperature and pressure (P=1013.25 hPa; T=273.15 K). To make a more direct comparison across campaigns with varying aircraft ceilings, only points below 5 km are included in Fig. 1. The total SNA concentrations are highest for KORUS-AQ, EUCAARI, MILAGRO, ADRIEX, and SENEX, indicative of the more significant influence of anthropogenic outflow during these campaigns. Generally, sulfate is the largest contributor to total SNA across all 11 campaigns. The nitrate fraction is higher for the three campaigns with the highest SNA concentrations (KORUS-AQ, EUCARRI, and MILAGRO) and for CalNex (associated with higher agricultural emissions) and WINTER (associated with colder temperature favoring particle-phase nitrate).
Table 1Details of all the campaigns used in this study, including the dates, regions, and primary references.
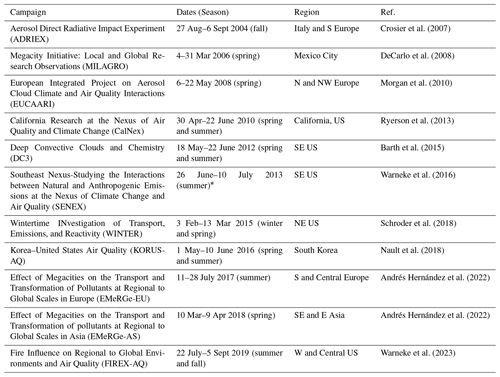
∗ The full campaign ran from 3 Jun–10 Jul 2014, but we remove points before 26 June because sensitivity issues with the AMS caused ammonium to be systematically higher than other species for earlier flights (Liao et al., 2017).
While we focus on campaigns influenced by anthropogenic sources, biomass burning also impacted some of the campaigns (i.e., FIREX-AQ, DC3, and MILAGRO). For FIREX-AQ, the main objective was to improve understanding of the impact of fires on air quality and climate, meaning both wildfires and prescribed agricultural burning in the US were intentionally sampled. The EMeRGe-EU and EMeRGe-AS campaigns were explicitly interested in air quality downwind of megacities in western Europe and Southeast Asia, respectively. We do not include the transit flights for the EMeRGe-AS campaign (corresponding to the flights on the first and last days between Germany and the United Arab Emirates). Other transit flights during EMeRGe-AS between megacity centers in Southeast Asia are included, which involved the sampling of cleaner, ocean air. Similarly, some flights for WINTER measured cleaner air over the Atlantic Ocean.
Across all the campaigns, Aerodyne aerosol mass spectrometers (AMSs; Canagaratna et al., 2007) measured sulfate, nitrate, and ammonium concentrations. An AMS measures sub-micrometer, non-refractory particles with approximately 30 % uncertainty for SNA species (Bahreini et al., 2009). Use of a single measurement technique is expected to reduce potential measurement bias between campaigns, though differences in instrument operation and models (Q-AMS, C-ToF-AMS, HR-ToF-AMS; see Supplementary Table 1 for AMS used in each campaign) may generate some variation.
The nitrate concentrations from the AMS include inorganic and organic nitrate. We use total nitrate in our analysis since the split between inorganic and organic nitrate is not available for all the campaigns. Previous work has shown that the percentage of total nitrate that is organic is highly dependent on total nitrate concentrations, ranging from 0 % at the highest amount of urban influence to 100 % at the cleanest conditions (Day et al., 2022). Given our selection of campaigns that are anthropogenically influenced, we expect inorganic nitrate to dominate total nitrate. We comment further on this in Sects. 3.1 and 4. Similarly, small fractions of the AMS sulfate may be due to organosulfates (Schueneman et al., 2021), and very small fractions of the AMS ammonium may be due to amines (Ge et al., 2024), but these apportionments are not typically reported, and possible contributions are neglected here.
We retain only the data points that have valid measurements for sulfate, nitrate, and ammonium. Observational data is filtered to remove plumes (sulfate, nitrate, ammonium concentrations that are greater than their respective 95th percentile) since the model is unable to capture these sub-grid processes successfully (Rastigejev et al., 2010). Observations are then averaged from their original resolution (using 1 min merge files when they are available) to the temporal and spatial resolution of the model. The majority of sampling occurred during the day, but some campaigns had more nighttime flights (e.g., 55 % of the valid points for WINTER are at nighttime). After filtering and averaging, there are 22 616 unique data points remaining that are used in our model–observation comparison.
3.1 General description
We use the GEOS-Chem chemical transport model version 13.3.4 (International GEOS-Chem User Community, 2021). Full-year simulations are performed at 2° × 2.5° horizontal resolution, while the campaign simulations make use of a finer resolution of 0.5° × 0.625° nested grid driven by boundary conditions from global 2° × 2.5° simulations. The model vertical domain is resolved into 47 hybrid-sigma layers extending from the surface to approximately 80 km altitude. All of the simulations are driven by the MERRA-2 assimilated meteorological data product from the NASA Goddard Global Modeling and Assimilation Office (GMAO). Boundary layer mixing is described using a non-local mixing scheme (Lin and McElroy, 2010). Following recommendations from Philip et al. (2016), time steps are 20 min for chemistry and 10 min for transport for the global simulations and 10 min for chemistry and 5 min for transport for the nested simulations.
GEOS-Chem includes detailed gas-phase chemistry coupled with the sulfate–nitrate–ammonium aerosol system (Park et al., 2004; Pye et al., 2009), with updates to HO2 uptake (Mao et al., 2013) and the reactive uptake of NO2, NO3, and N2O5 by aerosols and clouds (Holmes et al., 2019; McDuffie et al., 2018). Dust and sea salt aerosols are separated into different size bins (four bins for dust: 0.1–1.0 µm, 1.0–1.8 µm, 1.8–3.0 µm, 3.0–6.0 µm; two bins for sea salt: 0.01–0.5 µm, 0.5–8 µm). Sodium is calculated as a fraction of fine sea salt aerosol in GEOS-Chem (39.7 % by weight of sea salt). The model uses a bulk aerosol scheme with fixed log-normal modes to describe the size distribution of aerosols (Martin et al., 2003). A resistors-in-series scheme is used to describe gas dry deposition (Wesely, 1989; Wang et al., 1998) and size-dependent aerosol dry deposition (Zhang et al., 2001; Emerson et al., 2020). The wet-deposition scheme includes rainout, washout, and scavenging in moist convective updrafts for aerosols and gases (Amos et al., 2012; Liu et al., 2001; Wang et al., 2011, 2014). Thermodynamic partitioning between the gas and particle phase is described by the thermodynamic equilibrium aerosol model ISORROPIA II (Fountoukis and Nenes, 2007; Pye et al., 2009). ISORROPIA II is run using the default metastable mode, which assumes that all inorganic salts exist on the upper branch of the hygroscopic hysteresis curve. Acid uptake on dust (Fairlie et al., 2010) and nitrate photolysis (Shah et al., 2023) are optional processes in GEOS-Chem version 13.3.4 that we do not include in our model evaluation; however, we explore the effect of both of these processes on SNA in Sect. 5.5. When examining the impact of acid update on dust, we include nitrate and sulfate on dust in the smallest size bin (≤ 1 µm) in our model–observation comparisons.
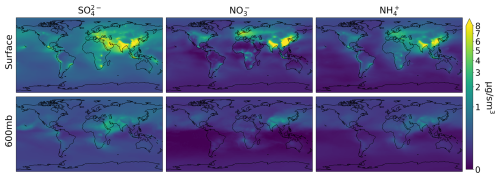
Figure 2Average annual concentrations of sulfate, nitrate, and ammonium at the surface and in the mid-troposphere (600 mb) for 2018.
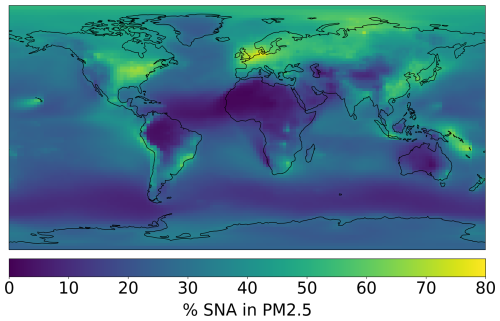
Figure 3Percent contribution of SNA to annual mean surface PM2.5 concentration based on a global simulation for 2018.
To match the observations, organic nitrate from the model (from isoprene and monoterpene precursors) is also included in nitrate. We use the complex scheme for organic aerosols described by Pai et al. (2020). However, we note that for the campaigns in this work, organic nitrate is a minor constituent of simulated total nitrate (median organic nitrate contribution is 0.1 % of total nitrate). The largest median organic nitrate fraction is simulated during SENEX (7.4 % of total nitrate), which was heavily influenced by biogenic sources in the southeastern US.
Each GEOS-Chem simulation is matched to the specific time and location of each airborne campaign. The majority of the emissions inventories used in this work are specific to a year. This includes the global anthropogenic emissions (comprising fossil fuel and agricultural sources) from the Community Emissions Database System (CEDS) v2 inventory, which also provides ship emissions (year-specific emissions up to 2017; Hoesly et al., 2018), biomass burning emissions from GFED4s (van der Werf et al., 2017), volcanic SO2 emissions (Carn et al., 2017), lightning emissions (Murray et al., 2012), sea salt emissions (Jaeglé et al., 2011), offline dust emissions (Meng et al., 2021), and offline soil NOx emissions (Hudman et al., 2012). Also included are dimethyl sulfide (DMS) emissions (Lana et al., 2011; Breider et al., 2017), aircraft emissions from AEIC 2009 (Stettler et al., 2011), and natural (soil, ocean, vegetation, wild animals) emissions of NH3 from GEIA Bouwman et al., 1997). Anthropogenic emissions for the United States are superseded by the EPA's National Emissions Inventory for 2016 (NEI 2016; Henderson and Freese, 2021). These emissions are also year-specific for all of our campaign runs, which are based on annual-scale factors derived from emissions trends from 2002–2020. By default, the NEI 2016 emissions inventory has weekday and weekend scaling factors applied to the NOx and SOx emissions. Time-of-day scaling factors are applied to all anthropogenic NOx and other fossil-burning emissions globally.
3.2 SNA budget in GEOS-Chem
Figure 2 shows the average global simulated distribution of sulfate, total (organic + inorganic) nitrate, and ammonium at the surface and in the mid-troposphere for the year 2018. Only fine sulfate and nitrate (not associated with sea salt or dust) are included to correspond to the fine-mode sampling by the AMS. Concentrations peak at the surface for all SNA species over India, East Asia, and Europe (annual mean concentrations > 8 µg sm−3), corresponding to regions with large anthropogenic precursor emissions. Smaller enhancements are visible over the US associated with lower emissions (e.g., stricter; Leibensperger et al., 2012). Other identifiable sources include biomass burning, volcanic emissions, and ocean sources (for sulfate). At the surface, SNA dominates (> 50 %) simulated PM2.5 concentrations across large swaths of the globe (Fig. 3), including near large population centers in the eastern US, Europe, and East Asia. Surface PM2.5 has been evaluated in GEOS-Chem previously and it is generally within 50% of the observations (Lee et al., 2017; Weagle et al., 2018; Zhai et al., 2021b). At the 600 mb level (Fig. 2), the same regions stand out as at the surface, but concentrations are generally low (∼ 1 µg sm−3). In the mid-troposphere, SO concentrations are higher and more uniform than NH and NO, reflecting the significant contributions of ocean sources to background SO and thermodynamics of ammonium and nitrate aerosols compared with sulfate aerosols.
Table 2 summarizes the budget for SNA and their precursors based on a 2018 simulation. All species have a similar lifetime of around 4–5 d. A significant amount of the emitted SOx (58 TgS yr−1) and DMS (19 TgS yr−1) is converted to sulfate and then lost to wet deposition (36 TgS yr−1). The precursor emissions for NO and NH are 50 TgN yr−1 for NOx and 68 TgN yr−1 for NH3. The budgets for sulfate, nitrate, and ammonium are generally within the range reported by Bian et al. (2017). The notable exceptions are that dry deposition of sulfate is lower in GEOS-Chem compared to all the other reported models (2.5–7.3 TgS yr−1) and that ammonia emissions exceed the range reported for the AeroCom III models in 2008 (47–58 TgN yr−1) (Bian et al., 2017). Dry deposition of ammonium (see Table 2) is also at the low end of the range reported in Bian et al. (2017) (1.3–16.3 TgN yr−1). However, across these models (and GEOS-Chem) dry-deposition loss generally makes up less than 20 % of the total loss due to deposition (Bian et al., 2017). In comparison, dry deposition of the precursor species (i.e., SO2, HNO3, NH3) is more important, contributing > 50 % of the total deposition loss of these precursors in GEOS-Chem. Other studies have shown that changes to the dry deposition of these precursors impacts SNA concentrations (Travis et al., 2022); this is discussed further in Sect. 5.3.
Table 2Summary of the 2018 global tropospheric budget in GEOS-Chem for SNA and their precursors. Note NO corresponds to fine, inorganic + organic nitrate. The lifetime is for dry and wet deposition only.
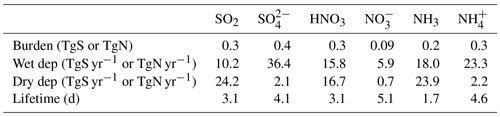
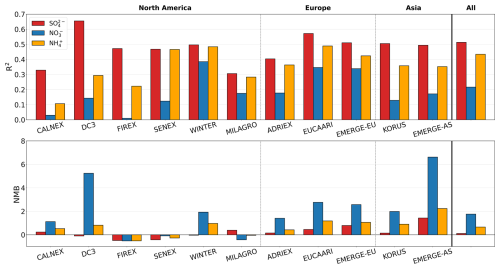
Figure 4GEOS-Chem model performance evaluated against each airborne campaign for sulfate (red), nitrate (blue), and ammonium (yellow) reported as R2 and NMB. Campaigns are grouped by the three general regions examined in this study. Model performance for all the campaigns merged into one dataset is shown under “All”.
We summarize the model evaluation of inorganic aerosol using two different statistical metrics: the coefficient of determination (R2) and the normalized mean bias (NMB). The ability of the model to capture variability is indicated by R2. NMB is the sum of the differences between each model and observation data point normalized by the sum of all the observations, where a positive (negative) NMB implies the model is overestimating (underestimating) the observations. It provides an idea of the relative bias irrespective of total concentration, which varies across these different campaigns. These statistics are calculated for the point-by-point comparison between the observations and model or, only where explicitly mentioned, using the vertical profiles. R values (not presented here) are all positive except for those corresponding to the NO vertical profiles (discussed in detail below) of two campaigns (CalNex and SENEX), where the model and observations show opposite trends with height. Figure 4 shows the R2 and NMB values for all the campaigns and the three SNA species. R2 values range from 0.01 (very poor) to 0.65 (variability in observations reasonably well captured). For all the campaigns, the model performance is best for sulfate (R2=0.51; NMB = 0.11) and notably worst for nitrate (R2=0.22; NMB = 1.76). Model performance for ammonium generally lies between that for nitrate and sulfate (R2 and NMB are 0.43 and 0.66 for all campaigns combined), reflecting the strong role that these acidic species play in the amount of ammonium formed. Better performance is expected for sulfate because the formation rates (under typical atmospheric conditions) are well understood and concentrations are not controlled by variable gas–particle partitioning. Figure 4 also demonstrates spatial variation in performance, with consistent high biases across all three species for the campaigns in Asia and Europe. In contrast, there is more variability by campaign and by species for the North American campaigns, with no apparent relationship in bias for these campaigns with year, season, or source influence. However, the high nitrate bias is more consistent with extreme overestimates (NMB > 2) seen across all three regions. When nitrate is scaled down based on the NMB across all the campaigns (NMB = 1.76), average surface PM2.5 concentrations across the Northern Hemisphere land decrease by 3.4 %, with maximum reductions of 25 % in the eastern US and East Asia and 33 % in Europe (Fig. S1 in the Supplement).
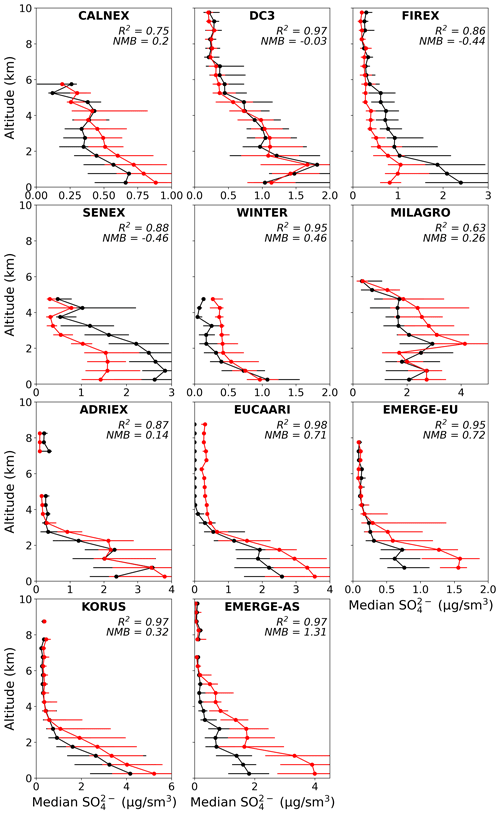
Figure 5Median vertical profile of observed (black) and simulated (red) sulfate concentrations. Points are binned to the nearest 0.5 km. Error bars represent the interquartile range. Altitude bins with less than 10 points per bin are not shown. R2 and NMB for the vertical variability are also reported for each campaign.
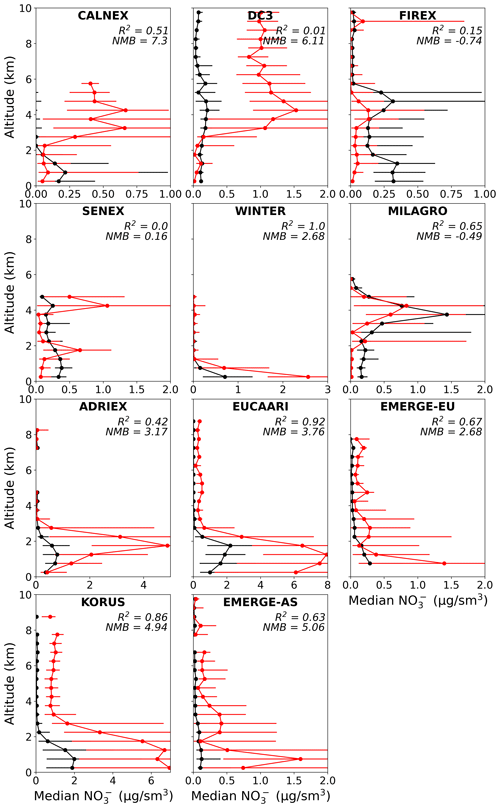
Figure 6Median vertical profile of observed (black) and simulated (red) nitrate concentrations. Points are binned to the nearest 0.5 km. Error bars represent the interquartile range. Altitude bins with less than 10 points per bin are not shown. R2 and NMB for the vertical variability is also reported for each campaign.
We examine if there is a connection between nitrate bias and the model gas ratio (GR, Fig. S2), which is the ratio of free ammonia ([NHx]–2[SO]) to total gas + particle nitrate (Ansari and Pandis, 1998). A GR > 1 indicates that the system is HNO3 limited, 0 < GR < 1 indicates that the system is NH3 limited, and GR < 0 indicates that the system is extremely NH3 limited and implies that sulfate is not fully neutralized. When NH3 is extremely limited, NO concentrations are lower and there is consistent negative bias in the simulated NO. This suggests that GEOS-Chem has an excessively strong NH3 limitation that is inhibiting some nitrate formation in these relatively clean (low-SNA-concentration) regions. However, these comparisons are also subject to measurement detection limits. The majority of the observations are characterized by GR > 0, which includes both ammonia limitation (0 < GR < 1) and HNO3 limitation (GR > 1); the simulated nitrate is positively biased in both cases, which indicates that the model bias is not the result of one specific precursor limitation.
Figures 5 and 6 show the vertical profile of median sulfate and nitrate, respectively, for each campaign. For sulfate, there are some modest underestimates and overestimates in magnitude across the campaigns. However, the model captures the generally consistent sulfate vertical profile shape, with most showing a peak at the surface and decreasing concentrations with altitude. The vertical profile of SO2 (Fig. S3) is also well captured by the model, but there is limited model skill for this species on a point-to-point basis (R2=0.31) which may degrade the sulfate simulation. The ratio SO SOx (for campaigns that have SO2 data) is well-captured for 4 of the 9 campaigns, but it is substantially overestimated for the remaining campaigns (CalNex, WINTER, MILAGRO, EMeRGe-EU, and EMeRGe-EU), particularly above the boundary layer (Fig. S4). For CalNex and MILAGRO, SO2 is underestimated and SO is overestimated (while total SOx is well captured), suggesting that oxidation may be overly rapid; for the other campaigns there is no evident relationship in the bias.
The shape of the observed vertical profile is less consistent for nitrate. For most campaigns, the model generally captures the vertical profile, albeit often with high biases both near the surface and aloft, especially for the European and Asian campaigns. However, in the case of the CalNex and DC3 campaigns, the model predicts peak nitrate concentrations aloft, which is not seen in the observations. The simulated nitrate also shows higher variability (i.e., larger interquartile range) compared to the observations and modeled sulfate. As indicated by the NO vertical profile for CalNex, this campaign measured many negative NO concentrations (25 % of all points), especially at higher altitudes (greater than 3 km all altitude bins have > 60 % negative points). While we do not remove these points for any of our model–observation comparisons, we note that the bias would remain but be modestly decreased if points below the detection limit were removed from our analysis. Observed and modeled ammonium profiles (Fig. S7) exhibit similar trends to nitrate, including the high-altitude peaks in simulated nitrate seen for CalNex and DC3, but generally exhibit less bias than nitrate.
The campaigns are influenced by a range of conditions that dictate the relative importance of particular processes. For example, some campaigns like EUCAARI and ADRIEX had strong inversions at the top of the boundary layer (BL) that led to increasing concentration of nitrate with height within the BL. Restricting the focus to points above the model-defined planetary boundary layer height (71 % of points) shows an improvement in R2 for NO across all campaigns (increases by < 0.01 to 0.13 relative to when all points are used), which implies that there is more model skill at capturing NO aloft. However, there is also an increase in the bias (NMB for NO increases to 2.91 for all the campaigns combined). Some campaigns (e.g., ADRIEX and EUCAARI) are less likely to be influenced by any deficiencies in the description of wet deposition in GEOS-Chem due to the lack of rainfall during the campaign (Crosier et al., 2007; Morgan et al., 2010). Others (e.g., DC3 and FIREX-AQ) may have biases associated with the challenges in capturing convective events. The exaggerated peak in simulated nitrate for DC3 could be associated with missing deposition because the storms are small compared to the spatial resolution of the model (Li et al., 2018). Consistent biases in vertical transport or precipitation are unlikely to explain the nitrate bias across these campaigns given that the model reproduces the expected vertical profiles for soluble species such as sulfate (Fig. 5) and for insoluble species such as CO (Fig. S8). In what follows, we use the merged dataset to focus on the universal response to processes; however, it is important to acknowledge that local biases in emissions and meteorology may degrade the model performance for individual campaigns, as explored in greater detail in campaign-specific studies.
As described in Sects. 2 and 3.1, the model and observed values for nitrate also include organic nitrate. Median observed nitrate concentrations are generally mid-range (0.05–0.7 µg sm−3) across most campaigns and at all altitudes, which implies these are, generally, environments where the relative contribution of organic nitrate could be significant (∼ 20 %–80 %) (Day et al., 2022). However, we find that the model organic nitrate contributes very little to total simulated nitrate concentrations across almost all the campaigns (Sect. 3.1). While this suggests that improvements to the organic nitrate description in GEOS-Chem are needed (Pai et al., 2020), it also indicates that the large positive bias in simulated nitrate is indicative of even greater deficiencies in the description of inorganic nitrate in GEOS-Chem. Furthermore, measurements of nitrate might be biased high for campaigns that used a C-ToF-AMS (CalNex, EMeRGe-AS, EMeRGe-EU, EUCAARI, SENEX), where the bias in observational nitrate is proportional to the organic mass concentrations (e.g., corrected nitrate measurements were 30 % lower than the measured values due to organics for one SENEX flight; Fry et al., 2018). Correcting for any overestimates in observed nitrate for these campaigns would worsen the model bias in nitrate.
In what follows, we examine potential causes of SNA model bias, with a focus on the nitrate bias, specifically the role that deposition, thermodynamic partitioning, chemistry, and/or emissions biases may play.
5.1 Evaluating thermodynamic partitioning
5.1.1 Evaluating thermodynamic partitioning in ISORROPIA II
First, we examine whether errors in the thermodynamic partitioning, represented via the ISORROPIA II scheme, could contribute to some of the model bias. Issues with partitioning, which can also act as a strong control on dry deposition and lifetime of total (gas- plus particle-phase) nitrate (TNO) and ammonium (NHx = NH3+ NH; Nenes et al., 2021), could contribute to the model SNA bias. ISORROPIA II, as implemented in GEOS-Chem and in forward mode, partitions TNO, NHx, and chloride (TCl HCl + Cl−) based on the total concentrations of these species, temperature (T), relative humidity (RH), and sodium and sulfate concentrations. It does not include cations associated with mineral dust (K+, Ca2+, and Mg2+), which are included in other implementations of ISORROPIA II.
The ability of ISORROPIA II to partition successfully can be evaluated by providing the observations as an input to a standalone version of ISORROPIA II (in forward mode) and comparing the predicted partitioning to the expected partitioning (i.e., the observations). However, none of the campaigns explored here included a complete set of measurements for the relevant species to fully evaluate partitioning. In particular, NH3, HCl, and Na+ were only measured for 2, 3, and 4 of the campaigns, respectively. We do not use the NH3 data collected for WINTER due to issues with the sample collection, as discussed in Guo et al. (2016), and we also do not use the NH3 data collected for FIREX-AQ because they only report enhancements in plumes that are not captured well by the model. Therefore, we undertake our evaluation of partitioning by substituting GEOS-Chem simulated values for these three species for all campaigns. In addition, we only consider the subset of campaigns where HNO3 and Cl− are measured, which leaves seven campaigns for our evaluation of ISORROPIA II. We filter the data as described in Sect. 2 and remove any points with missing or negative SNA, T, RH, HNO3, or Cl− to use as an input to ISORROPIA II. The resulting ISORROPIA II-predicted nitrate and ammonium concentrations do not agree perfectly with observations, although the overall NMB is small (Fig. 7). There are three input factors that may contribute to the imperfect performance in Fig. 7: the meteorology, the substituted model values, and measurement uncertainties.
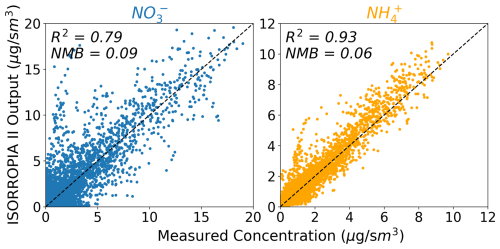
Figure 7Comparison of the expected (x axis) and ISORROPIA II-predicted (y axis) aerosol concentrations. Observations of T, RH, SO, NO, NH, HNO3, and Cl− are used as inputs into ISORROPIA II. Only the campaigns that include these measurements are represented.
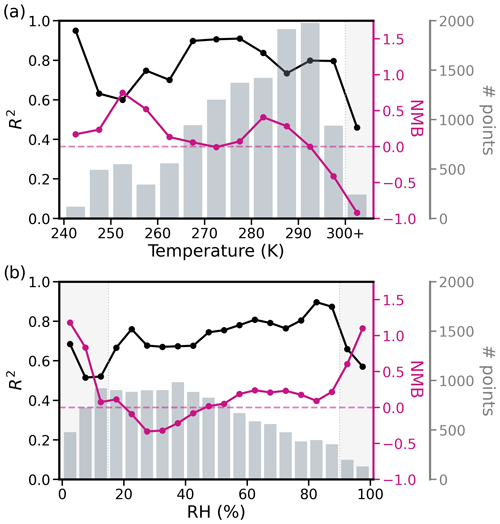
Figure 8R2 (black) and NMB (pink) for ISORROPIA II-predicted nitrate concentrations (with observations given as input) versus (a) temperature and (b) RH. Points are binned to the nearest 5 K and 5 % for the temperature and RH plots, respectively. Dark gray bars indicate the number of points in each bin. Light gray sections of the plot show which ranges of temperature and relative humidity result in worsened performance.
Figure 8 shows the relationship between ISORROPIA II performance (R2 and NMB) and temperature and RH specifically for NO. Performance degrades when RH < 15 % or RH > 90 % and T > 300 K. Previous work supports these observed limitations of ISORROPIA II's performance at very low humidity where under these conditions the aerosols are less likely to be in a completely liquid state (Ansari and Pandis, 2000; Malm and Day, 2001; Fountoukis and Nenes, 2007; Bertram et al., 2011). Also at very high humidity, there is exponential growth in the particle liquid water, which can lead to large uncertainties in the pH (Malm and Day, 2001; Guo et al., 2015). We therefore filter out these points (retaining only points where T<300 K and 15 ≤ RH < 90 %) in all subsequent analysis; however, we find that doing so only moderately improves the performance (R2 and NMB) of ISORROPIA II exhibited in Fig. 7 (impact on GEOS-Chem performance discussed in Sect. 5.1.2).
A more critical but difficult to assess factor is the use of model-substituted values for NH3, HCl, and Na+ concentrations. Figure 9 shows that for the limited campaigns where these species are measured, the model does not capture the observed variability (low R2), and in the case of sodium exhibits significant biases. Observations of sodium are limited, and the only available measurements are for bulk aerosol (< 4 µm), which does not align with the definition of sodium in GEOS-Chem (fraction of fine mode sea salt); these differences in size cut explain at least some of the discrepancy in Fig. 9. Observations for NH3 are only available for two of the campaigns (SENEX and CalNex). The near-zero NMB for NH3 in Fig. 9 is driven by large model overestimates for SENEX, with both overestimates and underestimates for CalNex. The variation in model performance could indicate that regional processes (e.g., emissions) dominate ammonia model bias.
For the two campaigns where NH3 measurements are available, we find that using these as inputs to ISORROPIA II, rather than model values, impacts the comparison between predicted and observed nitrate, with particularly large improvements in the R2 for CalNex (Figs. S9 and S10). Similar tests for Na+ and HCl had negligible impact on bias and R2, despite the clear inability of GEOS-Chem to capture the observed concentrations of these species (Fig. 9). We note that non-volatile cations, which other than Na+ are not accounted for in this implementation of ISORROPIA II, have been shown to shift partitioning, producing an average fine nitrate aerosol surface concentration that is 21 % higher than in a simulation with chemically inert dust (Karydis et al., 2016). This increase in nitrate is seen despite also introducing a loss pathway for HNO3 that reduces nitrate formation (discussed in more detail in Sect. 5.5). Figure 7 does not exhibit a systematic low bias in nitrate, suggesting that for the campaigns considered in this study, neglecting non-volatile cations does not produce noticeable partitioning bias.
Our evaluation of ISORROPIA II in Fig. 7 focuses on the aerosol nitrate and ammonium concentrations since these are the target species for our GEOS-Chem model simulation. A more explicit evaluation of the partitioning would explore the performance of ε(NO) (= NO TNO) and ε(NH) (= NH TNHx). However, the rarity of observed NH3 limits the dataset for which the observed partitioning can be fully assessed. For completeness we evaluate ε(NO) and ε(NH) using model-substituted ammonia concentrations as in Fig. 7. The resulting ISORROPIA II-predicted ε(NO) demonstrates little skill (R2=0.25), whereas ε(NH) is better captured (R2=0.78; Fig. S11 in Supplement). We identify no consistent relationship between the low R2 and other variables (e.g., other species, pH, concentrations) across the campaigns.
For the two campaigns with NH3 observations, replacing the GEOS-Chem-sourced NH3 values with the observed NH3 improves R2 for ε(NO) but at the cost of worsening R2 for ε(NH) (Figs. S9 and S10). We also explore the possibility of using estimated NH3 values for all campaigns. Following Guo et al. (2016), we iteratively solve for NH3 by cycling through different input TNHx values for ISORROPIA II until the expected concentration of NH is returned (or it fails to reach a solution). Using these new NH3 values improves agreement between observed and ISORROPIA II-predicted ε(NO) (R2=0.59). In particular, we note that we get a similar comparison between model and observed ε(NO) for WINTER as in Guo et al. (2016) (R2=0.61, NMB = −0.41, and performance is best when RH > 50 %). However, these estimated NH3 values greatly and unrealistically overestimate the observed NH3 from CalNex and SENEX (NMB = 0.48 and 11.39, respectively).
The limited evaluation of ε(NO) and ε(NH) possible here suggests that there may be some unresolved issues with partitioning as represented by ISORROPIA II. We note that our analysis assumes that the measurements are unbiased, there are no missing bases, and the system is in thermodynamic equilibrium. Representation of non-equilibrium thermodynamics can introduce some improvement in model bias for SNA but can also worsen model performance (Rosanka et al., 2024), suggesting that the missing non-equilibrium process in this work is unlikely a large contributor to the model bias shown here. More work is needed to fully evaluate ISORROPIA II performance for ammonium nitrate across a range of conditions, including using a full suite of gas- and aerosol-phase measurements. However, for the purposes of this broader investigation into ammonium nitrate performance within GEOS-Chem, we conclude that partitioning is not a dominant source of bias in the NO concentration comparisons (Fig. 7), and restricting the RH and T range can improve agreement between observations and model (Fig. 8).
5.1.2 Evaluating thermodynamic partitioning in GEOS-Chem
In addition to the comparisons shown in Fig. 9, here we explore whether there are biases in other model parameters that control thermodynamic partitioning and to what extent this may contribute to the GEOS-Chem biases in nitrate. Figure 10 shows the spread in these ISORROPIA II inputs for both the observations and the model. Where measured, HNO3 is generally overestimated by the model (NMB = 0.44). This could result from overestimated precursor emissions, excessive chemical production, or alternatively underestimated loss of HNO3 that could generate a high bias in HNO3 and in turn NO (discussed later). We also note that there is no systematic bias in the simulated NO TNO3 (Fig. S6). The overestimates and underestimates in this ratio are consistent with the NO bias seen in Fig. 6 and thus are not indicative of a partitioning bias, further supporting the analysis of the previous section. The model underestimates Cl− and does not capture the observed variability (low R2). Temperature is very well captured by the model (high R2, low NMB). The distribution of RH is similar between the model and observations in Fig. 10, but the lower R2 value indicates that there are differences in RH on a point-by-point basis. Some of the disagreement between observed and model RH can be explained by the observed RH being defined with respect to water, while the model RH is defined with respect to the relevant phase (ice, water, or a combination of the two) depending on temperature. This leads to greater discrepancies in RH aloft (Fig. S12). However, converting model RH to be with respect to water does not significantly alter ISORROPIA II-predicted partitioning and therefore does not contribute to the model bias.
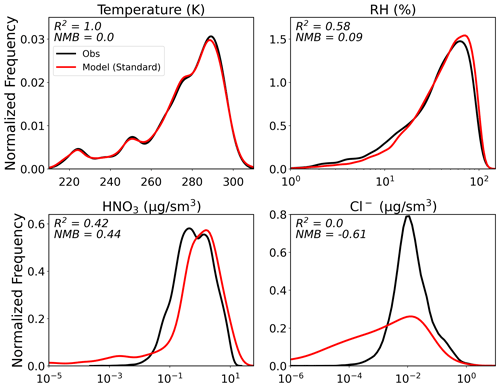
Figure 10Distribution of the observed and model values for the different variables needed as input to ISORROPIA II with reported R2 and NMB.
As in the previous section, we filter by RH and temperature (retaining points where model T<300 K and 15 ≤ model RH < 90 %) since Fig. 8 confirms that ISORROPIA II may not appropriately capture thermodynamic partitioning at the extremes of these T and RH values. A total of 20 % of the data points are eliminated by this filtering, with most of the points lost (72 %) from low altitudes (< 4 km). This filtering has a small effect on the model performance shown in Figs. 4–6. Sulfate performance is relatively unchanged (new R2 and NMB of 0.54 and 0.13 for all campaigns combined). R2 for all campaigns combined is decreased very minimally for NO (0.22 to 0.21) and NH (< 0.01 difference). The largest change after filtering is the reduction in NO NMB from 1.76 to 1.70. A small fraction of the model high-nitrate bias can therefore be explained by the temperature and RH range limitations, specifically for the partitioning by ISORROPIA II in GEOS-Chem. The comparison of ISORROPIA II-predicted pH using the observations and using the model values is also improved after filtering by T and RH (R2 goes from 0.28 to 0.32 and NMB from 0.32 to 0.19; see Fig. S13). For the remainder of this study, we remove points in these temperature and RH extremes and explore what processes might be responsible for the remaining nitrate bias.
We now test how the model values for T, RH, HNO3, Cl−, SO, NO, and NH impact the partitioning and contribute to the high NO bias in GEOS-Chem. Figure 11 shows a series of sensitivity tests where different combinations of modeled and observed values were given as an input to standalone ISORROPIA II. The bias of each sensitivity test relative to the “true” observed NO and NH is represented by the x and y axes, respectively.
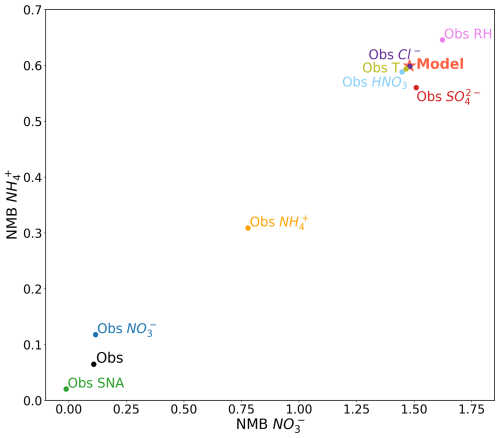
Figure 11Bias in NO and NH associated with different sensitivity tests with ISORROPIA II using all the available observed values (“Obs”), using all modeled values (“Model”), and when different observed values are substituted in for model values. Data are filtered to retain points where model T<300 K and 15 ≤ model RH < 90 %.
The “Obs” sensitivity case refers to when all the possible observations available for each campaign are used as input to ISORROPIA II. As in the previous section, we only use the campaigns that have HNO3 and Cl− measurements. We see that the ISORROPIA II-predicted nitrate and ammonium are only biased slightly high compared to observations when ISORROPIA II is driven by the entire (but incomplete) set of observed concentrations and meteorology (also seen in Fig. 7). We attribute this slight bias to the unmeasured species across the dataset in Sect. 5.1.1. The “Model” test case refers to using only the output from GEOS-Chem along the flight tracks as input to ISORROPIA II. The model is biased high compared to the observations, consistent with the results of the model evaluation in Sect. 4.
To identify whether any specific parameter drives the model bias, we substitute model values with observed values one at a time. When we replace the model temperature with the observed temperature, as in the “Obs T” run, we see a negligible impact on the partitioning, as expected given the match in observed and MERRA-2 temperature (Fig. 10). Similarly, substituting the observed HNO3, Cl−, and RH for model values (in three separate tests) produces little change in the thermodynamic partitioning, despite the biases seen between the model and observations in Fig. 10. As expected, the high bias in model HNO3 shifts the partitioning towards more particle phase. Despite an apparent high bias in model RH (NMB = 0.09; see Fig. 10), substituting observed RH for model RH results in less particle phase, which is associated with a low bias in model RH at higher NO and NH concentrations.
Using observed sulfate, which is generally lower than the model, as an input to ISORROPIA II produces less ammonium but more nitrate, which is as expected. However, the changes are relatively modest and do not suggest that sulfate model biases are responsible for the substantial biases in ammonium nitrate seen in GEOS-Chem. Greater improvements in predicted nitrate and ammonium concentrations result from using the observed ammonium or, more noticeably, the observed nitrate. The least biased ISORROPIA II prediction results from substituting in the observed sulfate, nitrate, and ammonium (“Obs SNA”), which nearly removes all bias for both nitrate and ammonium. This indicates that the GEOS-Chem model bias in nitrate and ammonium is largely a result of model SNA itself, rather than partitioning biases driven by meteorology, other aerosol constituents, or gas-phase precursors. However, we note that without a complete set of observed NH3 measurements, we cannot fully assess how biases in this species and the associated emissions may play a role in this model bias. We also note that while the magnitude of the NMB in NO and NH shown in Fig. 11 is sensitive to the subset of campaigns used, the general trends remain the same (i.e., changes in T, RH, Cl−, and HNO3 have low impact, while change in SNA has the largest).
The analyses above suggest that the GEOS-Chem model overestimate of nitrate (and ammonium) is likely the result of an excessive source or an underestimated or missing loss process for nitrate itself. We leverage the fast run time of standalone ISORROPIA II to run a multitude of sensitivity tests to explore how much TNO and NHx would need to change in GEOS-Chem to improve model performance. Figure 12 shows the model performance using NMB as the metric for NH and NO when the simulated values of TNO and NHx are scaled. All campaigns are included and are grouped by region to capture how changes on a regional scale could improve model performance. The NMB for the sum of ammonium and nitrate is also shown, where the swaths of gray (where NMB is near zero) indicate that there are different scalings of TNO and NHx that would all result in a similarly “most improved” simulation for both ammonium and nitrate. All three regions exhibit the same pattern, but the scaling factors are shifted up or down depending on regional model biases. For example, the North American campaigns, which are generally less biased (Fig. 4), require the least change (a 25 % reduction of TNO and/or NHx) to eliminate the bias. In contrast, the simulation would be most improved for the European and Asian campaigns with significant cuts (up to 50 %–75 %) to TNO, NHx, or both. In the coming sections, we explore how different production and loss processes in GEOS-Chem could reduce TNO and NHx in GEOS-Chem and in turn produce an improved simulation for ammonium nitrate.
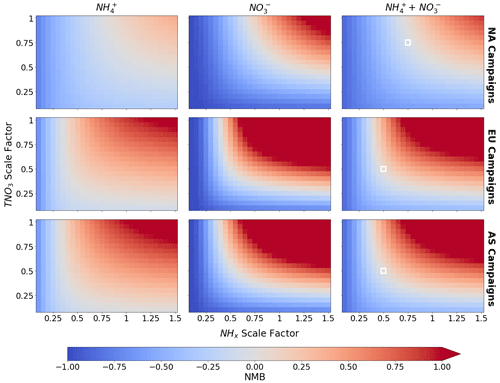
Figure 12ISORROPIA II performance across different sensitivity runs conducted by scaling NHx and TNO3 input from the baseline model values. Performance is reported as NMB for NH (first column), NO (middle column), and the sum of NH and NO (last column). Campaigns are grouped by region. Data are filtered to retain points where model T<300 K and 15 ≤ model RH < 90 %. White boxes in the last column indicate the scaling factors for NHx and TNO3 used in the full GEOS-Chem sensitivity test run (discussed in Sect. 5.2).
5.2 Response of SNA to changes in emissions
Overestimated precursor emissions in the model could drive the high bias in ammonium nitrate in GEOS-Chem. We conduct a sensitivity test where we assume that the entirety of the ammonium nitrate model bias is associated with emission uncertainties and use the concentration scalings for TNO and NHx from the previous section as a proxy for NOx and NH3 emissions in a GEOS-Chem sensitivity simulation. We cut both NOx and NH3 anthropogenic emissions by 50 % for the EU and AS regions and by 25 % for the NA region (scalings for each region are highlighted by the outlined white boxes in Fig. 12). Agricultural emissions, which are included in the anthropogenic emission inventories in GEOS-Chem, are also scaled down. The reduction in anthropogenic emissions is performed as a simple sensitivity to the dominant source value and does not imply that other smaller NOx sources (e.g., soil and lightning) are unbiased. The resulting GEOS-Chem model biases in nitrate and ammonium are both significantly reduced (Fig. 13). This confirms that our offline ISORROPIA II sensitivity tests shown in Fig. 12 are a reasonable proxy for precursor emission scaling. However, reductions in bias come without any significant improvement to the model's ability to capture the shape of the observed distribution or to model skill (see R2 values). In particular, despite the significant improvement at high NO concentrations, the lower NO concentrations (0.01–1 µg sm−3) are still significantly underestimated, suggesting that the biases at high and low concentrations may be driven by different factors. Furthermore, there is good agreement (within 10 %) between the current NH3 emissions from CEDS and top-down satellite-based emission estimates for North America, Europe, and eastern China (Luo et al., 2022). In addition, a regional emissions inventory for Asia is within ±25 % of NOx and NH3 emissions estimates from CEDS (Kurokawa and Ohara, 2020).
In addition, for those campaigns where NOx was measured, the model is almost consistently biased low in NOx (NMB = −0.29 across all campaigns) and overestimates the HNO3 : NOx concentration ratio (Fig. S14), which suggests that formation (and loss) of HNO3, rather than NOx emissions, may be overestimated (underestimated) in the model. Low NOx and high HNO3 biases could also indicate that oxidation is too fast in the model. Overly rapid oxidation could also contribute to the high SO SOx ratios seen across some campaigns (Fig. S4). While we do not explicitly investigate the potential role of oxidation on SNA model bias, we note that the mean tropospheric OH burden in GEOS-Chem is on the higher end of what is suggested by the literature (based on both observations and models; Bloss et al., 2005; Hu et al., 2018). Direct comparisons of GEOS-Chem to observations made at surface sites and during aircraft campaigns show that modeled OH (including its uncertainty) generally falls within the uncertainty range of measured OH but is generally higher in the model than the observations (Bloss et al., 2005; Christian et al., 2018; Kim et al., 2022). However, inconsistent biases in HNO3 across the campaigns suggest that model OH is not exclusively driving model bias. As mentioned above, changes to VOC emissions can also affect SNA concentrations, leading to possible reductions in concentration and the model bias presented here (e.g., Aksoyoglu et al., 2017); however, this effect is likely limited to near-surface regions with a higher potential for missing VOC reactivity and is unlikely to be an important driver of the high, consistent NO bias seen here in the free troposphere.
While reductions in the emissions in GEOS-Chem can eliminate the bias in the model simulation, the poor (and worsening) model skill is not ameliorated, suggesting that regional emissions biases alone are not responsible for the poor model performance for SNA.
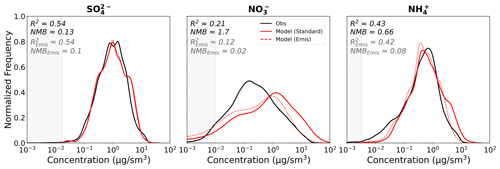
Figure 13Distribution plots of sulfate, nitrate, and ammonium mass concentrations across the all campaigns as in the observations (black), the standard GEOS-Chem model run (solid red), and the GEOS-Chem run with NOx and NH3 emissions reduced (“Emis”; dashed red). R2 and NMB are reported for both the standard (black text) and reduced emissions (grey text) simulations. Shaded regions indicate concentrations below the detection limit of the AMS (shown as the median DL across all campaigns). Extreme T and RH values have been filtered out as described in Sect. 5.1.2.
5.3 Sensitivity of SNA to dry-deposition changes
Dry deposition of SNA and its precursors is not well constrained. Evaluation of current model parameterizations for dry deposition are limited by a relatively small number of direct global measurements available for dry-deposition fluxes and large uncertainties in calculated deposition velocity (vd; Emerson et al., 2020). Travis et al. (2022) suggest some of the high bias in GEOS-Chem's nitrate and HNO3 during KORUS-AQ could be attributed to insufficient dry deposition on urban surfaces and see improvements in the model bias when vd for HNO3 is increased by a factor of 5. Heald et al. (2012) saw weak responses of global surface nitrate concentrations (decreased by < 10 %) when HNO3 dry deposition velocity was doubled.
Here we test how simulated global SNA responds to changes in vd using two sensitivity tests: one for changes in vd for all precursor gases (SO2, HNO3, and NH3; vd,prec) and the other for changes in vd for all the SNA species (vd,SNA). In both simulations, we increase vd by a factor of 2. We conduct these sensitivity tests for 1 year of simulation and not for all the campaigns (i.e., we do not provide comparisons of R2 and NMB). Figure 14 shows that relative changes in surface concentrations are minimal across all species and the two different sensitivity tests. Over land, surface NO is the most sensitive to the scaling of vd,prec and vd,SNA. Scaling vd,prec has a larger effect on SNA concentrations than scaling vd,SNA, demonstrating the more important role of dry deposition for the gas-phase precursors. However, while dry deposition of SNA in GEOS-Chem is on the lower end of other global models, dry deposition of precursors is on the higher end of these same models (Bian et al., 2017). Changing dry-deposition velocities has a lessened impact aloft, especially for the sensitivity test where vd,SNA was doubled (e.g., at the 800 mb level the maximum decrease for NO is 20 %), which confirms that the simulation of airborne measurements shown here is largely unaffected by uncertainties in dry deposition.
5.4 Sensitivity of SNA to wet-deposition changes
The wet-deposition scheme in GEOS-Chem accounts for rainout and washout in large-scale stratiform and convective precipitation and scavenging in convective updrafts (Jacob et al., 2000; Liu et al., 2001). These are highly parameterized processes that are empirically derived and remain uncertain. A recent update to the wet-deposition scheme in GEOS-Chem was developed by Luo et al. (2019, 2020), including changes that are relevant to SNA concentrations. The Luo et al. (2019, 2020) scheme updated the value for in-cloud condensed water (ICCW) to temporally and spatially vary based on MERRA-2 cloud and rainwater, as opposed to being a constant value. It also includes updated empirical washout coefficients for HNO3 and aerosols and rainout efficiencies for HNO3 and SO2 (Luo et al., 2019, 2020). Calculation of the effective Henry's law constant (H*) was updated to use a varying rainwater pH (for washout) and cloud water pH (for rainout and scavenging in convective updrafts), as opposed to a constant value of 4.5. Calculations of H* were also specifically updated for SO2 and NH3 with impacts on both wet and dry deposition (e.g., for the dry-deposition scheme, the average vd is 0.8–1 times the value from the standard simulation). The global annual mean burden for sulfate, nitrate, and ammonium are reduced by 32 %, 53 %, and 37 % under these changes in our 2018 simulation. SO2 and HNO3 global annual mean burdens decrease by 15 % and 56 %, respectively, in the simulation with the Luo et al. (2019, 2020) scheme. In contrast, the ammonia burden increases by 55 % as a result of partitioning favoring gas-phase TNHx when SO and TNO3 are reduced. We use the Luo et al. (2019, 2020) scheme to explore some of the sensitivity surrounding wet removal uncertainties through the lens of model performance for SNA.
Figure 15 shows the mass concentration distributions for all three SNA species across all campaigns for the observations and the two different wet-deposition schemes. Despite the addition of a geographically varying ICCW, which we might expect to better represent the regional variability in wet removal, there is no significant improvement in the R2. However, the new wet-deposition scheme substantially reduces the nitrate NMB from 1.70 to 1.02. The comparison suggests that the shifted nitrate distribution overestimates the lower concentrations; however, many of these concentrations may lie below the detection limit of the AMS and cannot be evaluated. The vertical profiles for nitrate show similar trends with shifts to lower concentrations at all altitudes, but no noticeable improvement in model performance compared to the profiles shown in Fig. 6 for the default model. The ammonium mass concentration distribution is also significantly shifted to lower concentrations, which improves the NMB. A similar reduction is seen for the sulfate mass concentration distribution, but the displacement to lower concentrations (not seen in the observations) slightly worsens the overall NMB (from 0.13 to −0.16). This suggests that the Luo et al. scheme may overestimate wet removal of SNA. Dutta and Heald (2023) also show that the Luo et al. deposition scheme results in a substantial overestimate of observed nitrate wet-deposition fluxes. This suggests that additional work is needed to optimize the removal efficiencies in GEOS-Chem considering the use of a physically varying ICCW. We note that smaller storms, which impacted some of the campaigns, may not be resolved at the resolution of the model, and therefore even with updates to the wet deposition scheme there is a limitation to how well the variability in wet removal can be captured. Finally, these comparisons emphasize that wet removal plays a major role in controlling the lifetime and abundance of SNA. Biases in the representation of these processes may explain some of the deficiencies in the simulation of model SNA concentrations.
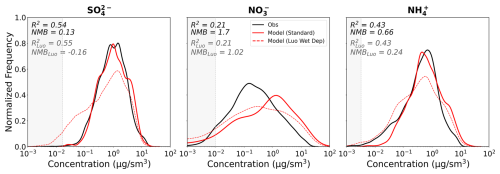
Figure 15Distribution plots of sulfate, nitrate, and ammonium mass concentrations across all the campaigns as observed (black), simulated in the standard GEOS-Chem model (solid red), and simulated in GEOS-Chem with the Luo et al. (2019, 2020) wet deposition scheme (dashed red). R2 and NMB are reported for both the standard (black text) and Luo et al. (grey text) simulations. Shaded regions indicate concentrations below the detection limit of the AMS (shown as the median DL across all campaigns). Extreme T and RH values have been filtered as described in Sect. 5.1.2.
5.5 The role of additional chemical sources and sinks in SNA bias
A missing chemical sink is another potential source of fine-mode SNA bias. Uptake of acidic gases (e.g., HNO3, SO2, H2SO4) by dust is one possible pathway. We find that for the two campaigns with the highest dust load (KORUS-AQ and EMeRGe-AS) acid uptake on dust, as implemented by Fairlie et al. (2010), improved the model's ability to capture SNA, but the impact was minimal. The largest impact was on nitrate where NMB was reduced by 0.04 and there was no change in model skill (R2), consistent with previous results (Fairlie et al., 2010). Zhai et al. (2023) show that including anthropogenic coarse dust in GEOS-Chem eliminated much of the nitrate overestimate for the KORUS-AQ campaign observations made in the Seoul Metropolitan Area (SMA). In the SMA, the average coarse-PM concentration at the surface was 23 µg m−3 for 2015 (Zhai et al., 2023), which is at the upper limit of what has been observed in Los Angeles and across European cities (range 5–23 µg m−3; Pakbin et al., 2010; Eeftens et al., 2012). Coarse anthropogenic PM is expected to be considerably less abundant outside of urban areas and aloft, and thus the campaigns explored here (including some individual flights during KORUS-AQ) would be relatively unaffected by this process, indicating that this is not a universal remedy for the GEOS-Chem nitrate simulation deficiencies.
Nitrate photolysis is another potential and uncertain pathway for nitrate loss. Studies generally relate the photolysis of nitrate to the photolysis of nitric acid by an enhancement factor (EF), with previous estimates for the EF ranging from 1–1000 (Romer et al., 2018; Shi et al., 2021; Ye et al., 2016). Shah et al. (2023) implemented a parameterization of NO photolysis in GEOS-Chem to address an observed underestimate in NO, where the EF scales from 10 to 100 depending on the concentration of sea salt aerosols relative to the concentration of NO. For two campaigns which are characterized by high calculated EFs and NO concentrations (MILAGRO and WINTER, with mean EFs of 0.47 and 0.29, respectively), adding the Shah et al. scheme leaves R2 unchanged and NMB negligibly altered (≤ 0.02) for all species. Therefore, nitrate photolysis, unless substantially more efficient than currently parameterized, cannot explain the large nitrate biases in the GEOS-Chem simulation.
We also consider the potential for an overestimated HNO3 source to explain the SNA bias, specifically N2O5 uptake by aerosols. N2O5 hydrolysis represents a significant pathway for inorganic nitrate formation, estimated to contribute 41 % of the inorganic nitrate source near the surface (Alexander et al., 2020) and 18 % of the tropospheric inorganic nitrate burden (Alexander et al., 2009). The N2O5 uptake coefficient () indicates the probability that N2O5 will be lost on an aerosol surface, leading to the formation of HNO3. The uptake parameter is dependent on numerous factors (e.g., aerosol composition, temperature, RH) and there remains uncertainty in the model parameterization of this process, with estimated values ranging over several orders of magnitude (Holmes et al., 2019; Macintyre and Evans, 2010; McDuffie et al., 2018). In a sensitivity test, we reduced the uptake coefficient of N2O5 in GEOS-Chem by 1 order of magnitude across all aerosol types for the WINTER and KORUS-AQ campaigns, which have the highest concentrations of N2O5. There was no significant impact on R2 (≤ 0.01), while the NMB for nitrate for these campaigns was reduced from 1.90 to 1.72; this suggests that the uncertainty in this pathway has a limited but non-negligible effect on the model's ability to capture SNA.
We explore the combined effect of all these updates to the chemical pathways (acid uptake by dust, reduced , and NO photolysis) on annual mean SNA. The global burden of both SO and NH are negligibly impacted (∼ 1 % decrease), but there is a 11 % reduction in the burden of NO. Figure 16 shows that the largest impact on SNA surface concentrations is for NO over the eastern US, Europe, India, and eastern China. Sulfate concentrations show modest increases downwind of regions where NO is decreased. A more damped effect on SNA concentrations is seen in the mid-troposphere. Collectively, known uncertainties in the chemical formation and loss processes (in the limits tested here) do not substantially perturb nitrate concentrations and cannot explain the model biases seen in our simulation.
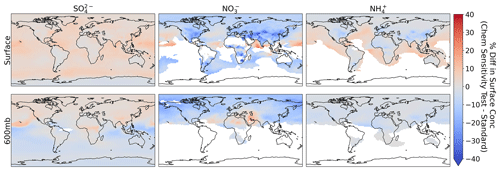
Figure 16Impact of updates to chemical pathways in GEOS-Chem (i.e., including acid uptake on dust, NO photolysis, and reducing ) on annual mean surface and mid-troposphere (600 mb) concentrations of sulfate, nitrate, and ammonium for 2018. Concentrations < 0.05 µg sm−3 are filtered out. Model nitrate and sulfate include nitrate and sulfate on dust in the smallest size bin.
Our evaluation of the global inorganic aerosol simulation in GEOS-Chem against observations from 11 airborne campaigns indicates that sulfate is generally well simulated in the model but that there is a systematic high bias in nitrate (and ammonium), with worse performance in Europe and Asia. We explore a range of factors that may contribute to the bias in nitrate.
We find that the ISORROPIA II model reproduces observed nitrate concentrations and conclude that thermodynamic partitioning is not responsible for the model nitrate bias. However, we identify that the variability in observed ε(NO) is not well captured with ISORROPIA II, but the evaluation of partitioning is incomplete given the limited set of ammonia observations. Extremely dry or saturated conditions, as well as the highest temperatures, are not well captured by ISORROPIA II, thereby degrading the GEOS-Chem model performance, particularly for nitrate. Removing these points modestly reduces the nitrate bias. Sensitivity tests using standalone ISORROPIA II suggest that the model bias in other species (HNO3, Cl−, Na+, HCl) are not responsible for the SNA bias. However, we find that partitioning is sensitive to NH3 concentrations and, for the two campaigns with ammonia measurements, the model evaluation demonstrates little skill and significant biases for this species. Ammonia is not routinely measured; our results indicate that additional measurements are sorely needed to further explore how ammonia biases may impact model simulations of nitrate. With the caveat that the impact of a potentially poor ammonia simulation on nitrate cannot be fully assessed, our analysis suggests that excessive sources or underestimated loss of nitrate in the model is the cause of the nitrate bias.
The model is sensitive to adjustments in emissions, deposition, and, very minimally, to different chemical loss and production updates (i.e., acid uptake on dust, N2O5 uptake, and NO photolysis), but none can explain the entirety of the high nitrate bias, or universally improve the model skill. Adjustments to the wet deposition scheme in GEOS-Chem show reductions in nitrate bias but worsen the model's ability to capture sulfate, suggesting that nitrate concentrations are very sensitive to wet removal processes, but these particular updates do not improve the model skill. A combination of changes to the emissions, deposition, and chemical production and loss may be able to close the high bias gap between model and observations, but more work is required to understand how to improve the model's ability to capture the variability in observed nitrate. We note that our comparisons assume that the fine-mode SNA is fully captured by the AMS observations. A high model bias in nitrate may result if a substantial fraction of fine aerosol nitrate extends beyond the 1 µm size (and is mis-characterized by the model as sub-micrometer as well). Measurements of the aerosol nitrate size distribution extending up to 2.5 µm are needed to explore this further. More routine geographically distributed measurements of wet deposition of TNO3 and dry deposition of HNO3 may also help constrain the nitrate life cycle. In addition, comprehensive measurements of NOy species (e.g., N2O5, PAN, HONO, organic nitrates) would help to evaluate NOy cycling in the model and in turn identify how biases in the chemical processes involving NOy impact inorganic particulate nitrate.
The model deficiencies in SNA highlighted in this paper have broader implications because of the role of SNA in climate and air quality. Despite numerous updates over the past decade to the description of chemical and physical processes that are relevant to nitrate formation in GEOS-Chem, model predictions of nitrate concentrations remain persistently biased high. The factor(s) contributing to the poor model skill and bias in SNA remain elusive. The grossly overestimated nitrate in GEOS-Chem implies that any policy-relevant studies for air quality and climate that employ this model will be similarly biased, including an over-emphasis on nitrogen-containing PM and a likely incorrect attribution of sectoral contributions to PM. Comprehensive measurements of particle- and gas-phase precursors in a range of environments would be invaluable to future efforts to identify the drivers of nitrate bias and to improve the fidelity of GEOS-Chem and possibly other models.
The data that support the findings of this study (https://doi.org/10.5281/zenodo.14029436, Norman and Heald, 2024) and the GEOS-Chem code for version 13.3.4 (https://doi.org/10.5281/zenodo.5764874, International GEOS-Chem User Community, 2021) are available. Observational data for MILAGRO (https://doi.org/10.5067/Aircraft/INTEXB/Aerosol-TraceGas, INTEX-B Science Team, 2013), DC3 (https://doi.org/10.5067/Aircraft/DC3/DC8/Aerosol-TraceGas, DC3 Science Team, 2018), KORUS-AQ (https://doi.org/10.5067/Suborbital/KORUSAQ/DATA01, KORUS-AQ Science Team, 2019), and FIREX-AQ (https://doi.org/10.5067/SUBORBITAL/FIREXAQ2019/DATA001, FIREX-AQ Science Team, 2023) are available through NASA LaRC Data Archive. Data for CalNex (https://csl.noaa.gov/groups/csl7/measurements/2010calnex/P3/DataDownload/DataDownloadMerged.html, CalNex Science Team, 2012) and SENEX (https://csl.noaa.gov/groups/csl7/measurements/2013senex/P3/DataDownload/mergeFiles.html, SENEX Science Team, 2014) are available via the NOAA ESRL data archive. Observational data for WINTER (https://data.eol.ucar.edu/master_lists/generated/winter, WINTER Science Team, 2016) are available through the NCAR EOL Archive. ADRIEX (https://data.ceda.ac.uk/badc/adriex/data/bae-146, ADRIEX Science Team, 2011) and EUCAARI (https://data.ceda.ac.uk/badc/faam/data/2008, EUCAARI Science Team, 2011) data are archived at the Centre for Environmental Data Analysis (CEDA). Observational data for EMeRGe-EU (https://halo-db.pa.op.dlr.de/mission/95, EMeRGe-EU Science Team, 2022) and EMeRGe-AS (https://halo-db.pa.op.dlr.de/mission/97, EMeRGe-AS Science Team, 2022) are publicly available via the HALO database.
The supplement related to this article is available online at: https://doi.org/10.5194/acp-25-771-2025-supplement.
CLH and OGN designed the study. OGN performed the simulations and led the analysis. PCJ, HC, JLJ, KK, JL, AMM, BAN, JS, and AW provided inorganic aerosol measurements used in the analysis. SB, MNF, JRG, and JBN provided gas-phase measurements used in the analysis. OGN and CLH wrote and edited the paper with input from the co-authors.
At least one of the (co-)authors is a member of the editorial board of Atmospheric Chemistry and Physics. The peer-review process was guided by an independent editor, and the authors also have no other competing interests to declare.
Publisher’s note: Copernicus Publications remains neutral with regard to jurisdictional claims made in the text, published maps, institutional affiliations, or any other geographical representation in this paper. While Copernicus Publications makes every effort to include appropriate place names, the final responsibility lies with the authors.
We also acknowledge the following investigators for providing measurements for SNA, HNO3, HCl, Na+, SO2, and CO: Andrew W. Rollins, J. Andy Neuman, Britton Stephens, Clifford Heizer, Felipe D. Lopez-Hilfiker, Glenn S. Diskin, L. Gregory Huey, Hans Schlager, Jack E. Dibb, Joel A. Thornton, John Crounse, John S. Holloway, Krystal T. Vasquez, Lisa Eirenschmalz, Lu Xu, Meghan Stell, Michael Lichtenstern, Michael Reeves, Michelle J. Kim, Paul O. Wennberg, Rodney J. Weber, Roya Bahreini, and Teresa Campos. The model simulations and analyses presented here were conducted using the “Svante” cluster, a facility located at MIT's Massachusetts Green High Performance Computing Center and jointly supported by the MIT Joint Program on the Science and Policy of Global Change; the Department of Earth, Atmospheric and Planetary Sciences; the Department of Civil and Environmental Engineering; the Institute for Data, Systems, and Society; and the Center for Global Change Science.
This research has been supported by the National Oceanic and Atmospheric Administration (grant no. NA19OAR4310180) and the NSF Division of Atmospheric and Geospace Sciences (grant no. AGS-2223070). Olivia G. Norman was partially supported by the Rasmussen Fellowship, a graduate fellowship for the department of Earth, Atmospheric, and Planetary Sciences at MIT. Pedro Campuzano-Jost, Benjamin A. Nault, and Jose L. Jimenez were supported by NASA (grant nos. 80NSSC21K1451 and 80NSSC23K0828). Benjamin A. Nault was also supported by NASA (grant no. 80NSSC22K0283). Joel Thornton also received an NSF grant that funded WINTER (grant no. AGS-1360745).
This paper was edited by Pedro Jimenez-Guerrero and reviewed by four anonymous referees.
ADRIEX Science Team: Aerosol Direct Radiative Impact Experiment (ADRIEX): In-situ airborne atmospheric measurements and atmospheric model data, Centre for Environmental Data Analysis [data set], https://data.ceda.ac.uk/badc/adriex/data/bae-146 (last access: 31 October 2024), 2011.
Aksoyoglu, S., Ciarelli, G., El-Haddad, I., Baltensperger, U., and Prévôt, A. S. H.: Secondary inorganic aerosols in Europe: sources and the significant influence of biogenic VOC emissions, especially on ammonium nitrate, Atmos. Chem. Phys., 17, 7757–7773, https://doi.org/10.5194/acp-17-7757-2017, 2017.
Aksoyoglu, S., Jiang, J., Ciarelli, G., Baltensperger, U., and Prévôt, A. S. H.: Role of ammonia in European air quality with changing land and ship emissions between 1990 and 2030, Atmos. Chem. Phys., 20, 15665–15680, https://doi.org/10.5194/acp-20-15665-2020, 2020.
Alexander, B., Hastings, M. G., Allman, D. J., Dachs, J., Thornton, J. A., and Kunasek, S. A.: Quantifying atmospheric nitrate formation pathways based on a global model of the oxygen isotopic composition (Δ17O) of atmospheric nitrate, Atmos. Chem. Phys., 9, 5043–5056, https://doi.org/10.5194/acp-9-5043-2009, 2009.
Alexander, B., Sherwen, T., Holmes, C. D., Fisher, J. A., Chen, Q., Evans, M. J., and Kasibhatla, P.: Global inorganic nitrate production mechanisms: comparison of a global model with nitrate isotope observations, Atmos. Chem. Phys., 20, 3859–3877, https://doi.org/10.5194/acp-20-3859-2020, 2020.
Amos, H. M., Jacob, D. J., Holmes, C. D., Fisher, J. A., Wang, Q., Yantosca, R. M., Corbitt, E. S., Galarneau, E., Rutter, A. P., Gustin, M. S., Steffen, A., Schauer, J. J., Graydon, J. A., Louis, V. L. St., Talbot, R. W., Edgerton, E. S., Zhang, Y., and Sunderland, E. M.: Gas-particle partitioning of atmospheric Hg(II) and its effect on global mercury deposition, Atmos. Chem. Phys., 12, 591–603, https://doi.org/10.5194/acp-12-591-2012, 2012.
Andrés Hernández, M. D., Hilboll, A., Ziereis, H., Förster, E., Krüger, O. O., Kaiser, K., Schneider, J., Barnaba, F., Vrekoussis, M., Schmidt, J., Huntrieser, H., Blechschmidt, A.-M., George, M., Nenakhov, V., Harlass, T., Holanda, B. A., Wolf, J., Eirenschmalz, L., Krebsbach, M., Pöhlker, M. L., Kalisz Hedegaard, A. B., Mei, L., Pfeilsticker, K., Liu, Y., Koppmann, R., Schlager, H., Bohn, B., Schumann, U., Richter, A., Schreiner, B., Sauer, D., Baumann, R., Mertens, M., Jöckel, P., Kilian, M., Stratmann, G., Pöhlker, C., Campanelli, M., Pandolfi, M., Sicard, M., Gómez-Amo, J. L., Pujadas, M., Bigge, K., Kluge, F., Schwarz, A., Daskalakis, N., Walter, D., Zahn, A., Pöschl, U., Bönisch, H., Borrmann, S., Platt, U., and Burrows, J. P.: Overview: On the transport and transformation of pollutants in the outflow of major population centres – observational data from the EMeRGe European intensive operational period in summer 2017, Atmos. Chem. Phys., 22, 5877–5924, https://doi.org/10.5194/acp-22-5877-2022, 2022.
Ansari, A. S. and Pandis, S. N.: Response of Inorganic PM to Precursor Concentrations, Environ. Sci. Technol., 32, 2706–2714, https://doi.org/10.1021/es971130j, 1998.
Ansari, A. S. and Pandis, S. N.: The effect of metastable equilibrium states on the partitioning of nitrate between the gas and aerosol phases, Atmos. Environ., 34, 157–168, https://doi.org/10.1016/S1352-2310(99)00242-3, 2000.
Bahreini, R., Ervens, B., Middlebrook, A. M., Warneke, C., de Gouw, J. A., DeCarlo, P. F., Jimenez, J. L., Brock, C. A., Neuman, J. A., Ryerson, T. B., Stark, H., Atlas, E., Brioude, J., Fried, A., Holloway, J. S., Peischl, J., Richter, D., Walega, J., Weibring, P., Wollny, A. G., and Fehsenfeld, F. C.: Organic aerosol formation in urban and industrial plumes near Houston and Dallas, Texas, J. Geophys. Res.-Atmos., 114, D00F16, https://doi.org/10.1029/2008JD011493, 2009.
Barth, M. C., Cantrell, C. A., Brune, W. H., Rutledge, S. A., Crawford, J. H., Huntrieser, H., Carey, L. D., MacGorman, D., Weisman, M., Pickering, K. E., Bruning, E., Anderson, B., Apel, E., Biggerstaff, M., Campos, T., Campuzano-Jost, P., Cohen, R., Crounse, J., Day, D. A., Diskin, G., Flocke, F., Fried, A., Garland, C., Heikes, B., Honomichl, S., Hornbrook, R., Huey, L. G., Jimenez, J. L., Lang, T., Lichtenstern, M., Mikoviny, T., Nault, B., O'Sullivan, D., Pan, L. L., Peischl, J., Pollack, I., Richter, D., Riemer, D., Ryerson, T., Schlager, H., Clair, J. S., Walega, J., Weibring, P., Weinheimer, A., Wennberg, P., Wisthaler, A., Wooldridge, P. J., and Ziegler, C.: The Deep Convective Clouds and Chemistry (DC3) Field Campaign, B. Am. Meteorol. Soc., 96, 1281–1309, https://doi.org/10.1175/BAMS-D-13-00290.1, 2015.
Bertram, A. K., Martin, S. T., Hanna, S. J., Smith, M. L., Bodsworth, A., Chen, Q., Kuwata, M., Liu, A., You, Y., and Zorn, S. R.: Predicting the relative humidities of liquid-liquid phase separation, efflorescence, and deliquescence of mixed particles of ammonium sulfate, organic material, and water using the organic-to-sulfate mass ratio of the particle and the oxygen-to-carbon elemental ratio of the organic component, Atmos. Chem. Phys., 11, 10995–11006, https://doi.org/10.5194/acp-11-10995-2011, 2011.
Bian, H., Chin, M., Hauglustaine, D. A., Schulz, M., Myhre, G., Bauer, S. E., Lund, M. T., Karydis, V. A., Kucsera, T. L., Pan, X., Pozzer, A., Skeie, R. B., Steenrod, S. D., Sudo, K., Tsigaridis, K., Tsimpidi, A. P., and Tsyro, S. G.: Investigation of global particulate nitrate from the AeroCom phase III experiment, Atmos. Chem. Phys., 17, 12911–12940, https://doi.org/10.5194/acp-17-12911-2017, 2017.
Bloss, W. J., Evans, M. J., Lee, J. D., Sommariva, R., Heard, D. E., and Pilling, M. J.: The oxidative capacity of the troposphere: Coupling of field measurements of OH and a global chemistry transport model, Faraday Discuss., 130, 425–436, https://doi.org/10.1039/B419090D, 2005.
Bouwman, A. F., Lee, D. S., Asman, W. A. H., Dentener, F. J., Van Der Hoek, K. W., and Olivier, J. G. J.: A global high-resolution emission inventory for ammonia, Global Biogeochem. Cy., 11, 561–587, https://doi.org/10.1029/97GB02266, 1997.
Breider, T. J., Mickley, L. J., Jacob, D. J., Ge, C., Wang, J., Payer Sulprizio, M., Croft, B., Ridley, D. A., McConnell, J. R., Sharma, S., Husain, L., Dutkiewicz, V. A., Eleftheriadis, K., Skov, H., and Hopke, P. K.: Multidecadal trends in aerosol radiative forcing over the Arctic: Contribution of changes in anthropogenic aerosol to Arctic warming since 1980, J. Geophys. Res.-Atmos., 122, 3573–3594, https://doi.org/10.1002/2016JD025321, 2017.
CalNex Science Team: CalNex, NOAA Chemical Sciences Laboratory [data set], https://csl.noaa.gov/groups/csl7/measurements/2010calnex/P3/DataDownload/DataDownloadMerged.html (last access: 31 October 2024), 2012.
Canagaratna, M. R., Jayne, J. T., Jimenez, J. L., Allan, J. D., Alfarra, M. R., Zhang, Q., Onasch, T. B., Drewnick, F., Coe, H., Middlebrook, A., Delia, A., Williams, L. R., Trimborn, A. M., Northway, M. J., DeCarlo, P. F., Kolb, C. E., Davidovits, P., and Worsnop, D. R.: Chemical and microphysical characterization of ambient aerosols with the aerodyne aerosol mass spectrometer, Mass Spectrom. Rev., 26, 185–222, https://doi.org/10.1002/mas.20115, 2007.
Carn, S. A., Fioletov, V. E., McLinden, C. A., Li, C., and Krotkov, N. A.: A decade of global volcanic SO2 emissions measured from space, Sci. Rep., 7, 44095, https://doi.org/10.1038/srep44095, 2017.
Chen, L., Gao, Y., Zhang, M., Fu, J. S., Zhu, J., Liao, H., Li, J., Huang, K., Ge, B., Wang, X., Lam, Y. F., Lin, C.-Y., Itahashi, S., Nagashima, T., Kajino, M., Yamaji, K., Wang, Z., and Kurokawa, J.: MICS-Asia III: multi-model comparison and evaluation of aerosol over East Asia, Atmos. Chem. Phys., 19, 11911–11937, https://doi.org/10.5194/acp-19-11911-2019, 2019.
Christian, K. E., Brune, W. H., Mao, J., and Ren, X.: Global sensitivity analysis of GEOS-Chem modeled ozone and hydrogen oxides during the INTEX campaigns, Atmos. Chem. Phys., 18, 2443–2460, https://doi.org/10.5194/acp-18-2443-2018, 2018.
Crosier, J., Allan, J. D., Coe, H., Bower, K. N., Formenti, P., and Williams, P. I.: Chemical composition of summertime aerosol in the Po Valley (Italy), northern Adriatic and Black Sea, Q. J. Roy. Meteorol. Soc., 133, 61–75, https://doi.org/10.1002/qj.88, 2007.
Dawson, J. P., Adams, P. J., and Pandis, S. N.: Sensitivity of PM2.5 to climate in the Eastern US: a modeling case study, Atmos. Chem. Phys., 7, 4295–4309, https://doi.org/10.5194/acp-7-4295-2007, 2007.
Day, D. A., Campuzano-Jost, P., Nault, B. A., Palm, B. B., Hu, W., Guo, H., Wooldridge, P. J., Cohen, R. C., Docherty, K. S., Huffman, J. A., de Sá, S. S., Martin, S. T., and Jimenez, J. L.: A systematic re-evaluation of methods for quantification of bulk particle-phase organic nitrates using real-time aerosol mass spectrometry, Atmos. Meas. Tech., 15, 459–483, https://doi.org/10.5194/amt-15-459-2022, 2022.
DC3 Science Team: DC3 Field Campaign Data from DC-8 aircraft, NASA Langley Research Center [data set], https://doi.org/10.5067/Aircraft/DC3/DC8/Aerosol-TraceGas, 2018.
DeCarlo, P. F., Dunlea, E. J., Kimmel, J. R., Aiken, A. C., Sueper, D., Crounse, J., Wennberg, P. O., Emmons, L., Shinozuka, Y., Clarke, A., Zhou, J., Tomlinson, J., Collins, D. R., Knapp, D., Weinheimer, A. J., Montzka, D. D., Campos, T., and Jimenez, J. L.: Fast airborne aerosol size and chemistry measurements above Mexico City and Central Mexico during the MILAGRO campaign, Atmos. Chem. Phys., 8, 4027–4048, https://doi.org/10.5194/acp-8-4027-2008, 2008.
Dutta, I. and Heald, C. L.: Exploring Deposition Observations of Oxidized Sulfur and Nitrogen as a Constraint on Emissions in the United States, J. Geophys. Res.-Atmos., 128, e2023JD039610, https://doi.org/10.1029/2023JD039610, 2023.
Eeftens, M., Tsai, M.-Y., Ampe, C., Anwander, B., Beelen, R., Bellander, T., Cesaroni, G., Cirach, M., Cyrys, J., de Hoogh, K., De Nazelle, A., de Vocht, F., Declercq, C., Dėdelė, A., Eriksen, K., Galassi, C., Gražulevičienė, R., Grivas, G., Heinrich, J., Hoffmann, B., Iakovides, M., Ineichen, A., Katsouyanni, K., Korek, M., Krämer, U., Kuhlbusch, T., Lanki, T., Madsen, C., Meliefste, K., Mölter, A., Mosler, G., Nieuwenhuijsen, M., Oldenwening, M., Pennanen, A., Probst-Hensch, N., Quass, U., Raaschou-Nielsen, O., Ranzi, A., Stephanou, E., Sugiri, D., Udvardy, O., Vaskövi, É., Weinmayr, G., Brunekreef, B., and Hoek, G.: Spatial variation of PM2.5, PM10, PM2.5 absorbance and PMcoarse concentrations between and within 20 European study areas and the relationship with NO2 – Results of the ESCAPE project, Atmos. Environ., 62, 303–317, https://doi.org/10.1016/j.atmosenv.2012.08.038, 2012.
EMeRGe-AS Science Team: Mission: EMeRGe-AS, HALO database [data set], https://halo-db.pa.op.dlr.de/mission/97 (last access: 31 October 2024), 2022.
EMeRGe-EU Science Team: Mission: EMeRGe-EU, HALO database [data set], https://halo-db.pa.op.dlr.de/mission/95 (last access: 31 October 2024), 2022.
Emerson, E. W., Hodshire, A. L., DeBolt, H. M., Bilsback, K. R., Pierce, J. R., McMeeking, G. R., and Farmer, D. K.: Revisiting particle dry deposition and its role in radiative effect estimates, P. Natl. Acad. Sci. USA, 117, 26076–26082, https://doi.org/10.1073/pnas.2014761117, 2020.
EUCAARI Science Team: Facility for Airborne Atmospheric Measurements (FAAM) flights, Centre for Environmental Data Analysis [data set], https://data.ceda.ac.uk/badc/faam/data/2008 (last access: 31 October 2024), 2011.
Fairlie, T. D., Jacob, D. J., Dibb, J. E., Alexander, B., Avery, M. A., van Donkelaar, A., and Zhang, L.: Impact of mineral dust on nitrate, sulfate, and ozone in transpacific Asian pollution plumes, Atmos. Chem. Phys., 10, 3999–4012, https://doi.org/10.5194/acp-10-3999-2010, 2010.
FIREX-AQ Science Team: FIREX-AQ Data, NASA Langley Research Center [data set], https://doi.org/10.5067/SUBORBITAL/FIREXAQ2019/DATA001, 2023.
Fountoukis, C. and Nenes, A.: ISORROPIA II: a computationally efficient thermodynamic equilibrium model for K+–Ca2+–Mg2+–NH–Na+–SO–NO–Cl−–H2O aerosols, Atmos. Chem. Phys., 7, 4639–4659, https://doi.org/10.5194/acp-7-4639-2007, 2007.
Fry, J. L., Brown, S. S., Middlebrook, A. M., Edwards, P. M., Campuzano-Jost, P., Day, D. A., Jimenez, J. L., Allen, H. M., Ryerson, T. B., Pollack, I., Graus, M., Warneke, C., de Gouw, J. A., Brock, C. A., Gilman, J., Lerner, B. M., Dubé, W. P., Liao, J., and Welti, A.: Secondary organic aerosol (SOA) yields from NO3 radical + isoprene based on nighttime aircraft power plant plume transects, Atmos. Chem. Phys., 18, 11663–11682, https://doi.org/10.5194/acp-18-11663-2018, 2018.
Gani, S., Bhandari, S., Seraj, S., Wang, D. S., Patel, K., Soni, P., Arub, Z., Habib, G., Hildebrandt Ruiz, L., and Apte, J. S.: Submicron aerosol composition in the world's most polluted megacity: the Delhi Aerosol Supersite study, Atmos. Chem. Phys., 19, 6843–6859, https://doi.org/10.5194/acp-19-6843-2019, 2019.
Ge, X., Sun, Y., Trousdell, J., Chen, M., and Zhang, Q.: Enhancing characterization of organic nitrogen components in aerosols and droplets using high-resolution aerosol mass spectrometry, Atmos. Meas. Tech., 17, 423–439, https://doi.org/10.5194/amt-17-423-2024, 2024.
Geng, G., Zhang, Q., Tong, D., Li, M., Zheng, Y., Wang, S., and He, K.: Chemical composition of ambient PM2.5 over China and relationship to precursor emissions during 2005–2012, Atmos. Chem. Phys., 17, 9187–9203, https://doi.org/10.5194/acp-17-9187-2017, 2017.
Gliß, J., Mortier, A., Schulz, M., Andrews, E., Balkanski, Y., Bauer, S. E., Benedictow, A. M. K., Bian, H., Checa-Garcia, R., Chin, M., Ginoux, P., Griesfeller, J. J., Heckel, A., Kipling, Z., Kirkevåg, A., Kokkola, H., Laj, P., Le Sager, P., Lund, M. T., Lund Myhre, C., Matsui, H., Myhre, G., Neubauer, D., van Noije, T., North, P., Olivié, D. J. L., Rémy, S., Sogacheva, L., Takemura, T., Tsigaridis, K., and Tsyro, S. G.: AeroCom phase III multi-model evaluation of the aerosol life cycle and optical properties using ground- and space-based remote sensing as well as surface in situ observations, Atmos. Chem. Phys., 21, 87–128, https://doi.org/10.5194/acp-21-87-2021, 2021.
Guo, H., Xu, L., Bougiatioti, A., Cerully, K. M., Capps, S. L., Hite Jr., J. R., Carlton, A. G., Lee, S.-H., Bergin, M. H., Ng, N. L., Nenes, A., and Weber, R. J.: Fine-particle water and pH in the southeastern United States, Atmos. Chem. Phys., 15, 5211–5228, https://doi.org/10.5194/acp-15-5211-2015, 2015.
Guo, H., Sullivan, A. P., Campuzano-Jost, P., Schroder, J. C., Lopez-Hilfiker, F. D., Dibb, J. E., Jimenez, J. L., Thornton, J. A., Brown, S. S., Nenes, A., and Weber, R. J.: Fine particle pH and the partitioning of nitric acid during winter in the northeastern United States, J. Geophys. Res.-Atmos., 121, 10355–10376, https://doi.org/10.1002/2016JD025311, 2016.
Haskins, J. D., Jaeglé, L., Shah, V., Lee, B. H., Lopez-Hilfiker, F. D., Campuzano-Jost, P., Schroder, J. C., Day, D. A., Guo, H., Sullivan, A. P., Weber, R., Dibb, J., Campos, T., Jimenez, J. L., Brown, S. S., and Thornton, J. A.: Wintertime Gas-Particle Partitioning and Speciation of Inorganic Chlorine in the Lower Troposphere Over the Northeast United States and Coastal Ocean, J. Geophys. Res.-Atmos., 123, 12897–12916, https://doi.org/10.1029/2018JD028786, 2018.
Heald, C. L., Collett Jr., J. L., Lee, T., Benedict, K. B., Schwandner, F. M., Li, Y., Clarisse, L., Hurtmans, D. R., Van Damme, M., Clerbaux, C., Coheur, P.-F., Philip, S., Martin, R. V., and Pye, H. O. T.: Atmospheric ammonia and particulate inorganic nitrogen over the United States, Atmos. Chem. Phys., 12, 10295–10312, https://doi.org/10.5194/acp-12-10295-2012, 2012.
Henderson, B. and Freese, L.: Preparation of GEOS-Chem Emissions from CMAQ, Zenodo, https://doi.org/10.5281/zenodo.5122827, 2021.
Hoesly, R. M., Smith, S. J., Feng, L., Klimont, Z., Janssens-Maenhout, G., Pitkanen, T., Seibert, J. J., Vu, L., Andres, R. J., Bolt, R. M., Bond, T. C., Dawidowski, L., Kholod, N., Kurokawa, J.-I., Li, M., Liu, L., Lu, Z., Moura, M. C. P., O'Rourke, P. R., and Zhang, Q.: Historical (1750–2014) anthropogenic emissions of reactive gases and aerosols from the Community Emissions Data System (CEDS), Geosci. Model Dev., 11, 369–408, https://doi.org/10.5194/gmd-11-369-2018, 2018.
Holmes, C. D., Bertram, T. H., Confer, K. L., Graham, K. A., Ronan, A. C., Wirks, C. K., and Shah, V.: The Role of Clouds in the Tropospheric NOx Cycle: A New Modeling Approach for Cloud Chemistry and Its Global Implications, Geophys. Res. Lett., 46, 4980–4990, https://doi.org/10.1029/2019GL081990, 2019.
Hu, L., Keller, C. A., Long, M. S., Sherwen, T., Auer, B., Da Silva, A., Nielsen, J. E., Pawson, S., Thompson, M. A., Trayanov, A. L., Travis, K. R., Grange, S. K., Evans, M. J., and Jacob, D. J.: Global simulation of tropospheric chemistry at 12.5 km resolution: performance and evaluation of the GEOS-Chem chemical module (v10-1) within the NASA GEOS Earth system model (GEOS-5 ESM), Geosci. Model Dev., 11, 4603–4620, https://doi.org/10.5194/gmd-11-4603-2018, 2018.
Huang, W., Cao, J., Tao, Y., Dai, L., Lu, S.-E., Hou, B., Wang, Z., and Zhu, T.: Seasonal Variation of Chemical Species Associated With Short-Term Mortality Effects of PM2.5 in Xi'an, a Central City in China, Am. J. Epidemiol., 175, 556–566, https://doi.org/10.1093/aje/kwr342, 2012.
Hudman, R. C., Moore, N. E., Mebust, A. K., Martin, R. V., Russell, A. R., Valin, L. C., and Cohen, R. C.: Steps towards a mechanistic model of global soil nitric oxide emissions: implementation and space based-constraints, Atmos. Chem. Phys., 12, 7779–7795, https://doi.org/10.5194/acp-12-7779-2012, 2012.
International GEOS-Chem User Community: geoschem/GCClassic: GEOS-Chem 13.3.4 (13.3.4), Zenodo [data set], https://doi.org/10.5281/zenodo.5764874, 2021.
INTEX-B Science Team: INTEX-B Data, NASA Langley Research Center [data set], https://doi.org/10.5067/Aircraft/INTEXB/Aerosol-TraceGas, 2013.
IPCC: Climate Change 2021: The Physical Science Basis. Contribution of Working Group I to the Sixth Assessment Report of the Intergovernmental Panel on Climate Change, edited by: Masson-Delmotte, V., Zhai, P., Pirani, A., Connors, S. L., Péan, C., Berger, S., Caud, N., Chen, Y., Goldfarb, L., Gomis, M. I., Huang, M., Leitzell, K., Lonnoy, E., Matthews, J. B. R., Maycock, T. K., Waterfield, T., Yelekçi, O., Yu, R., and Zhou, B., Cambridge University Press, Cambridge, United Kingdom, 2391 pp., https://doi.org/10.1017/9781009157896, 2023.
Jacob, D. J., Liu, H., Mari, C., and Yantosca, R. M.: Harvard wet deposition scheme for GMI, https://silo.tips/download/harvard-wet-deposition-scheme-for-gmi (last access: 31 October 2024), 2000.
Jaeglé, L., Quinn, P. K., Bates, T. S., Alexander, B., and Lin, J.-T.: Global distribution of sea salt aerosols: new constraints from in situ and remote sensing observations, Atmos. Chem. Phys., 11, 3137–3157, https://doi.org/10.5194/acp-11-3137-2011, 2011.
Karydis, V. A., Tsimpidi, A. P., Pozzer, A., Astitha, M., and Lelieveld, J.: Effects of mineral dust on global atmospheric nitrate concentrations, Atmos. Chem. Phys., 16, 1491–1509, https://doi.org/10.5194/acp-16-1491-2016, 2016.
Kim, H., Park, R. J., Kim, S., Brune, W. H., Diskin, G. S., Fried, A., Hall, S. R., Weinheimer, A. J., Wennberg, P., Wisthaler, A., Blake, D. R., and Ullmann, K.: Observed versus simulated OH reactivity during KORUS-AQ campaign: Implications for emission inventory and chemical environment in East Asia, Elementa: Sci. Anthrop., 10, 00030, https://doi.org/10.1525/elementa.2022.00030, 2022.
KORUS-AQ Science Team: KORUS-AQ Data, NASA Langley Research Center [data set], https://doi.org/10.5067/Suborbital/KORUSAQ/DATA01, 2019.
Kurokawa, J. and Ohara, T.: Long-term historical trends in air pollutant emissions in Asia: Regional Emission inventory in ASia (REAS) version 3, Atmos. Chem. Phys., 20, 12761–12793, https://doi.org/10.5194/acp-20-12761-2020, 2020.
Lana, A., Bell, T. G., Simó, R., Vallina, S. M., Ballabrera-Poy, J., Kettle, A. J., Dachs, J., Bopp, L., Saltzman, E. S., Stefels, J., Johnson, J. E., and Liss, P. S.: An updated climatology of surface dimethlysulfide concentrations and emission fluxes in the global ocean, Global Biogeochem. Cy., 25, GB1004, https://doi.org/10.1029/2010GB003850, 2011.
Lee, H.-M., Park, R. J., Henze, D. K., Lee, S., Shim, C., Shin, H.-J., Moon, K.-J., and Woo, J.-H.: PM2.5 source attribution for Seoul in May from 2009 to 2013 using GEOS-Chem and its adjoint model, Environ. Pollut., 221, 377–384, https://doi.org/10.1016/j.envpol.2016.11.088, 2017.
Leibensperger, E. M., Mickley, L. J., Jacob, D. J., Chen, W.-T., Seinfeld, J. H., Nenes, A., Adams, P. J., Streets, D. G., Kumar, N., and Rind, D.: Climatic effects of 1950–2050 changes in US anthropogenic aerosols – Part 1: Aerosol trends and radiative forcing, Atmos. Chem. Phys., 12, 3333–3348, https://doi.org/10.5194/acp-12-3333-2012, 2012.
Li, M., Zhang, Z., Yao, Q., Wang, T., Xie, M., Li, S., Zhuang, B., and Han, Y.: Nonlinear responses of particulate nitrate to NOx emission controls in the megalopolises of China, Atmos. Chem. Phys., 21, 15135–15152, https://doi.org/10.5194/acp-21-15135-2021, 2021.
Li, Y., Pickering, K. E., Barth, M. C., Bela, M. M., Cummings, K. A., and Allen, D. J.: Evaluation of Parameterized Convective Transport of Trace Gases in Simulation of Storms Observed During the DC3 Field Campaign, J. Geophys. Res.-Atmos., 123, 11238–11261, https://doi.org/10.1029/2018JD028779, 2018.
Liao, J., Brock, C. A., Murphy, D. M., Sueper, D. T., Welti, A., and Middlebrook, A. M.: Single-particle measurements of bouncing particles and in situ collection efficiency from an airborne aerosol mass spectrometer (AMS) with light-scattering detection, Atmos. Meas. Tech., 10, 3801–3820, https://doi.org/10.5194/amt-10-3801-2017, 2017.
Lin, J.-T. and McElroy, M. B.: Impacts of boundary layer mixing on pollutant vertical profiles in the lower troposphere: Implications to satellite remote sensing, Atmos. Environ., 44, 1726–1739, https://doi.org/10.1016/j.atmosenv.2010.02.009, 2010.
Liu, H., Jacob, D. J., Bey, I., and Yantosca, R. M.: Constraints from 210Pb and 7Be on wet deposition and transport in a global three-dimensional chemical tracer model driven by assimilated meteorological fields, J. Geophys. Res.-Atmos., 106, 12109–12128, https://doi.org/10.1029/2000JD900839, 2001.
Lohmann, U. and Feichter, J.: Global indirect aerosol effects: a review, Atmos. Chem. Phys., 5, 715–737, https://doi.org/10.5194/acp-5-715-2005, 2005.
Luo, G., Yu, F., and Schwab, J.: Revised treatment of wet scavenging processes dramatically improves GEOS-Chem 12.0.0 simulations of surface nitric acid, nitrate, and ammonium over the United States, Geosci. Model Dev., 12, 3439–3447, https://doi.org/10.5194/gmd-12-3439-2019, 2019.
Luo, G., Yu, F., and Moch, J. M.: Further improvement of wet process treatments in GEOS-Chem v12.6.0: impact on global distributions of aerosols and aerosol precursors, Geosci. Model Dev., 13, 2879–2903, https://doi.org/10.5194/gmd-13-2879-2020, 2020.
Luo, Z., Zhang, Y., Chen, W., Van Damme, M., Coheur, P.-F., and Clarisse, L.: Estimating global ammonia (NH3) emissions based on IASI observations from 2008 to 2018, Atmos. Chem. Phys., 22, 10375–10388, https://doi.org/10.5194/acp-22-10375-2022, 2022.
Macintyre, H. L. and Evans, M. J.: Sensitivity of a global model to the uptake of N2O5 by tropospheric aerosol, Atmos. Chem. Phys., 10, 7409–7414, https://doi.org/10.5194/acp-10-7409-2010, 2010.
Malm, W. C. and Day, D. E.: Estimates of aerosol species scattering characteristics as a function of relative humidity, Atmos. Environ., 35, 2845–2860, https://doi.org/10.1016/S1352-2310(01)00077-2, 2001.
Malm, W. C., Pitchford, M. L., Scruggs, M., Sisler, J. F., Ames, R., Copeland, S., Gebhart, K. A., and Day, D. E.: Spatial and Seasonal Patterns and Temporal Variability of Haze and Its Constituents in the United States: Report III, Coop. Inst. for Res. in the Atmos., Colo. State Univ., Fort Collins, Colorado, ISSN 0737-5352-47, 2000.
Mao, J., Fan, S., Jacob, D. J., and Travis, K. R.: Radical loss in the atmosphere from Cu-Fe redox coupling in aerosols, Atmos. Chem. Phys., 13, 509–519, https://doi.org/10.5194/acp-13-509-2013, 2013.
Martin, R. V., Jacob, D. J., Yantosca, R. M., Chin, M., and Ginoux, P.: Global and regional decreases in tropospheric oxidants from photochemical effects of aerosols, J. Geophys. Res.-Atmos., 108, 4097, https://doi.org/10.1029/2002JD002622, 2003.
McDuffie, E. E., Fibiger, D. L., Dubé, W. P., Lopez-Hilfiker, F., Lee, B. H., Thornton, J. A., Shah, V., Jaeglé, L., Guo, H., Weber, R. J., Michael Reeves, J., Weinheimer, A. J., Schroder, J. C., Campuzano-Jost, P., Jimenez, J. L., Dibb, J. E., Veres, P., Ebben, C., Sparks, T. L., Wooldridge, P. J., Cohen, R. C., Hornbrook, R. S., Apel, E. C., Campos, T., Hall, S. R., Ullmann, K., and Brown, S. S.: Heterogeneous N2O5 Uptake During Winter: Aircraft Measurements During the 2015 WINTER Campaign and Critical Evaluation of Current Parameterizations, J. Geophys. Res.-Atmos., 123, 4345–4372, https://doi.org/10.1002/2018JD028336, 2018.
Meng, J., Martin, R. V., Ginoux, P., Hammer, M., Sulprizio, M. P., Ridley, D. A., and van Donkelaar, A.: Grid-independent high-resolution dust emissions (v1.0) for chemical transport models: application to GEOS-Chem (12.5.0), Geosci. Model Dev., 14, 4249–4260, https://doi.org/10.5194/gmd-14-4249-2021, 2021.
Mezuman, K., Bauer, S. E., and Tsigaridis, K.: Evaluating secondary inorganic aerosols in three dimensions, Atmos. Chem. Phys., 16, 10651–10669, https://doi.org/10.5194/acp-16-10651-2016, 2016.
Morgan, W. T., Allan, J. D., Bower, K. N., Highwood, E. J., Liu, D., McMeeking, G. R., Northway, M. J., Williams, P. I., Krejci, R., and Coe, H.: Airborne measurements of the spatial distribution of aerosol chemical composition across Europe and evolution of the organic fraction, Atmos. Chem. Phys., 10, 4065–4083, https://doi.org/10.5194/acp-10-4065-2010, 2010.
Murray, L. T., Jacob, D. J., Logan, J. A., Hudman, R. C., and Koshak, W. J.: Optimized regional and interannual variability of lightning in a global chemical transport model constrained by LIS/OTD satellite data, J. Geophys. Res.-Atmos., 117, D20307, https://doi.org/10.1029/2012JD017934, 2012.
Myhre, G., Samset, B. H., Schulz, M., Balkanski, Y., Bauer, S., Berntsen, T. K., Bian, H., Bellouin, N., Chin, M., Diehl, T., Easter, R. C., Feichter, J., Ghan, S. J., Hauglustaine, D., Iversen, T., Kinne, S., Kirkevåg, A., Lamarque, J.-F., Lin, G., Liu, X., Lund, M. T., Luo, G., Ma, X., van Noije, T., Penner, J. E., Rasch, P. J., Ruiz, A., Seland, Ø., Skeie, R. B., Stier, P., Takemura, T., Tsigaridis, K., Wang, P., Wang, Z., Xu, L., Yu, H., Yu, F., Yoon, J.-H., Zhang, K., Zhang, H., and Zhou, C.: Radiative forcing of the direct aerosol effect from AeroCom Phase II simulations, Atmos. Chem. Phys., 13, 1853–1877, https://doi.org/10.5194/acp-13-1853-2013, 2013.
Nault, B. A., Campuzano-Jost, P., Day, D. A., Schroder, J. C., Anderson, B., Beyersdorf, A. J., Blake, D. R., Brune, W. H., Choi, Y., Corr, C. A., de Gouw, J. A., Dibb, J., DiGangi, J. P., Diskin, G. S., Fried, A., Huey, L. G., Kim, M. J., Knote, C. J., Lamb, K. D., Lee, T., Park, T., Pusede, S. E., Scheuer, E., Thornhill, K. L., Woo, J.-H., and Jimenez, J. L.: Secondary organic aerosol production from local emissions dominates the organic aerosol budget over Seoul, South Korea, during KORUS-AQ, Atmos. Chem. Phys., 18, 17769–17800, https://doi.org/10.5194/acp-18-17769-2018, 2018.
Nault, B. A., Campuzano-Jost, P., Day, D. A., Jo, D. S., Schroder, J. C., Allen, H. M., Bahreini, R., Bian, H., Blake, D. R., Chin, M., Clegg, S. L., Colarco, P. R., Crounse, J. D., Cubison, M. J., DeCarlo, P. F., Dibb, J. E., Diskin, G. S., Hodzic, A., Hu, W., Katich, J. M., Kim, M. J., Kodros, J. K., Kupc, A., Lopez-Hilfiker, F. D., Marais, E. A., Middlebrook, A. M., Andrew Neuman, J., Nowak, J. B., Palm, B. B., Paulot, F., Pierce, J. R., Schill, G. P., Scheuer, E., Thornton, J. A., Tsigaridis, K., Wennberg, P. O., Williamson, C. J., and Jimenez, J. L.: Chemical transport models often underestimate inorganic aerosol acidity in remote regions of the atmosphere, Commun. Earth Environ., 2, 1–13, https://doi.org/10.1038/s43247-021-00164-0, 2021.
Nenes, A., Pandis, S. N., Kanakidou, M., Russell, A. G., Song, S., Vasilakos, P., and Weber, R. J.: Aerosol acidity and liquid water content regulate the dry deposition of inorganic reactive nitrogen, Atmos. Chem. Phys., 21, 6023–6033, https://doi.org/10.5194/acp-21-6023-2021, 2021.
Norman, O. G. and Heald, C. L.: Exploring the processes controlling secondary inorganic aerosol: Evaluating the global GEOS-Chem simulation using a suite of aircraft campaigns, Zenodo [data set], https://doi.org/10.5281/zenodo.14029436, 2024.
Pai, S. J., Heald, C. L., Pierce, J. R., Farina, S. C., Marais, E. A., Jimenez, J. L., Campuzano-Jost, P., Nault, B. A., Middlebrook, A. M., Coe, H., Shilling, J. E., Bahreini, R., Dingle, J. H., and Vu, K.: An evaluation of global organic aerosol schemes using airborne observations, Atmos. Chem. Phys., 20, 2637–2665, https://doi.org/10.5194/acp-20-2637-2020, 2020.
Pakbin, P., Hudda, N., Cheung, K. L., Moore, K. F., and Sioutas, C.: Spatial and Temporal Variability of Coarse (PM10−2.5) Particulate Matter Concentrations in the Los Angeles Area, Aerosol Sci. Technol., 44, 514–525, https://doi.org/10.1080/02786821003749509, 2010.
Park, R. J., Jacob, D. J., Field, B. D., Yantosca, R. M., and Chin, M.: Natural and transboundary pollution influences on sulfate-nitrate-ammonium aerosols in the United States: Implications for policy, J. Geophys. Res.-Atmos., 109, D15204, https://doi.org/10.1029/2003JD004473, 2004.
Paulot, F., Fan, S., and Horowitz, L. W.: Contrasting seasonal responses of sulfate aerosols to declining SO2 emissions in the Eastern U.S.: Implications for the efficacy of SO2 emission controls, Geophys. Res. Lett., 44, 455–464, https://doi.org/10.1002/2016GL070695, 2017.
Phan, N.-T., Kim, K.-H., Shon, Z.-H., Jeon, E.-C., Jung, K., and Kim, N.-J.: Analysis of ammonia variation in the urban atmosphere, Atmos. Environ., 65, 177–185, https://doi.org/10.1016/j.atmosenv.2012.10.049, 2013.
Philip, S., Martin, R. V., and Keller, C. A.: Sensitivity of chemistry-transport model simulations to the duration of chemical and transport operators: a case study with GEOS-Chem v10-01, Geosci. Model Dev., 9, 1683–1695, https://doi.org/10.5194/gmd-9-1683-2016, 2016.
Pope, C. A. and Dockery, D. W.: Health Effects of Fine Particulate Air Pollution: Lines that Connect, J. Air Waste Manage. Assoc., 56, 709–742, https://doi.org/10.1080/10473289.2006.10464485, 2006.
Pye, H. O. T., Liao, H., Wu, S., Mickley, L. J., Jacob, D. J., Henze, D. K., and Seinfeld, J. H.: Effect of changes in climate and emissions on future sulfate-nitrate-ammonium aerosol levels in the United States, J. Geophys. Res.-Atmos., 114, D01205, https://doi.org/10.1029/2008JD010701, 2009.
Rastigejev, Y., Park, R., Brenner, M. P., and Jacob, D. J.: Resolving intercontinental pollution plumes in global models of atmospheric transport, J. Geophys. Res.-Atmos., 115, D02302, https://doi.org/10.1029/2009JD012568, 2010.
Reifenberg, S. F., Martin, A., Kohl, M., Bacer, S., Hamryszczak, Z., Tadic, I., Röder, L., Crowley, D. J., Fischer, H., Kaiser, K., Schneider, J., Dörich, R., Crowley, J. N., Tomsche, L., Marsing, A., Voigt, C., Zahn, A., Pöhlker, C., Holanda, B. A., Krüger, O., Pöschl, U., Pöhlker, M., Jöckel, P., Dorf, M., Schumann, U., Williams, J., Bohn, B., Curtius, J., Harder, H., Schlager, H., Lelieveld, J., and Pozzer, A.: Numerical simulation of the impact of COVID-19 lockdown on tropospheric composition and aerosol radiative forcing in Europe, Atmos. Chem. Phys., 22, 10901–10917, https://doi.org/10.5194/acp-22-10901-2022, 2022.
Romer, P. S., Wooldridge, P. J., Crounse, J. D., Kim, M. J., Wennberg, P. O., Dibb, J. E., Scheuer, E., Blake, D. R., Meinardi, S., Brosius, A. L., Thames, A. B., Miller, D. O., Brune, W. H., Hall, S. R., Ryerson, T. B., and Cohen, R. C.: Constraints on Aerosol Nitrate Photolysis as a Potential Source of HONO and NOx, Environ. Sci. Technol., 52, 13738–13746, https://doi.org/10.1021/acs.est.8b03861, 2018.
Rosanka, S., Tost, H., Sander, R., Jöckel, P., Kerkweg, A., and Taraborrelli, D.: How non-equilibrium aerosol chemistry impacts particle acidity: the GMXe AERosol CHEMistry (GMXe–AERCHEM, v1.0) sub-submodel of MESSy, Geosci. Model Dev., 17, 2597–2615, https://doi.org/10.5194/gmd-17-2597-2024, 2024.
Ryerson, T. B., Andrews, A. E., Angevine, W. M., Bates, T. S., Brock, C. A., Cairns, B., Cohen, R. C., Cooper, O. R., de Gouw, J. A., Fehsenfeld, F. C., Ferrare, R. A., Fischer, M. L., Flagan, R. C., Goldstein, A. H., Hair, J. W., Hardesty, R. M., Hostetler, C. A., Jimenez, J. L., Langford, A. O., McCauley, E., McKeen, S. A., Molina, L. T., Nenes, A., Oltmans, S. J., Parrish, D. D., Pederson, J. R., Pierce, R. B., Prather, K., Quinn, P. K., Seinfeld, J. H., Senff, C. J., Sorooshian, A., Stutz, J., Surratt, J. D., Trainer, M., Volkamer, R., Williams, E. J., and Wofsy, S. C.: The 2010 California Research at the Nexus of Air Quality and Climate Change (CalNex) field study: CalNex 2010 FIELD PROJECT OVERVIEW, J. Geophys. Res.-Atmos., 118, 5830–5866, https://doi.org/10.1002/jgrd.50331, 2013.
Schiferl, L. D., Heald, C. L., Nowak, J. B., Holloway, J. S., Neuman, J. A., Bahreini, R., Pollack, I. B., Ryerson, T. B., Wiedinmyer, C., and Murphy, J. G.: An investigation of ammonia and inorganic particulate matter in California during the CalNex campaign, J. Geophys. Res.-Atmos., 119, 1883–1902, https://doi.org/10.1002/2013JD020765, 2014.
Schroder, J. C., Campuzano-Jost, P., Day, D. A., Shah, V., Larson, K., Sommers, J. M., Sullivan, A. P., Campos, T., Reeves, J. M., Hills, A., Hornbrook, R. S., Blake, N. J., Scheuer, E., Guo, H., Fibiger, D. L., McDuffie, E. E., Hayes, P. L., Weber, R. J., Dibb, J. E., Apel, E. C., Jaeglé, L., Brown, S. S., Thornton, J. A., and Jimenez, J. L.: Sources and Secondary Production of Organic Aerosols in the Northeastern United States during WINTER, J. Geophys. Res.-Atmos., 123, 7771–7796, https://doi.org/10.1029/2018JD028475, 2018.
Schueneman, M. K., Nault, B. A., Campuzano-Jost, P., Jo, D. S., Day, D. A., Schroder, J. C., Palm, B. B., Hodzic, A., Dibb, J. E., and Jimenez, J. L.: Aerosol pH indicator and organosulfate detectability from aerosol mass spectrometry measurements, Atmos. Meas. Tech., 14, 2237–2260, https://doi.org/10.5194/amt-14-2237-2021, 2021.
Seinfeld, J. H. and Pandis, S. N.: Atmospheric Chemistry and Physics: From Air Pollution to Climate Change, John Wiley & Sons, 2197 pp., ISBN 9781119221166, 2016.
SENEX Science Team: SENEX, NOAA Chemical Sciences Laboratory [data set], https://csl.noaa.gov/groups/csl7/measurements/2013senex/P3/DataDownload/mergeFiles.html (last access: 31 October 2024), 2014.
Shah, V., Jaeglé, L., Thornton, J. A., Lopez-Hilfiker, F. D., Lee, B. H., Schroder, J. C., Campuzano-Jost, P., Jimenez, J. L., Guo, H., Sullivan, A. P., Weber, R. J., Green, J. R., Fiddler, M. N., Bililign, S., Campos, T. L., Stell, M., Weinheimer, A. J., Montzka, D. D., and Brown, S. S.: Chemical feedbacks weaken the wintertime response of particulate sulfate and nitrate to emissions reductions over the eastern United States, P. Natl. Acad. Sci. USA, 115, 8110–8115, https://doi.org/10.1073/pnas.1803295115, 2018.
Shah, V., Jacob, D. J., Dang, R., Lamsal, L. N., Strode, S. A., Steenrod, S. D., Boersma, K. F., Eastham, S. D., Fritz, T. M., Thompson, C., Peischl, J., Bourgeois, I., Pollack, I. B., Nault, B. A., Cohen, R. C., Campuzano-Jost, P., Jimenez, J. L., Andersen, S. T., Carpenter, L. J., Sherwen, T., and Evans, M. J.: Nitrogen oxides in the free troposphere: implications for tropospheric oxidants and the interpretation of satellite NO2 measurements, Atmos. Chem. Phys., 23, 1227–1257, https://doi.org/10.5194/acp-23-1227-2023, 2023.
Shi, Q., Tao, Y., Krechmer, J. E., Heald, C. L., Murphy, J. G., Kroll, J. H., and Ye, Q.: Laboratory Investigation of Renoxification from the Photolysis of Inorganic Particulate Nitrate, Environ. Sci. Technol., 55, 854–861, https://doi.org/10.1021/acs.est.0c06049, 2021.
Stettler, M. E. J., Eastham, S., and Barrett, S. R. H.: Air quality and public health impacts of UK airports. Part I: Emissions, Atmos. Environ., 45, 5415–5424, https://doi.org/10.1016/j.atmosenv.2011.07.012, 2011.
Sun, K., Tao, L., Miller, D. J., Pan, D., Golston, L. M., Zondlo, M. A., Griffin, R. J., Wallace, H. W., Leong, Y. J., Yang, M. M., Zhang, Y., Mauzerall, D. L., and Zhu, T.: Vehicle Emissions as an Important Urban Ammonia Source in the United States and China, Environ. Sci. Technol., 51, 2472–2481, https://doi.org/10.1021/acs.est.6b02805, 2017.
Thornhill, G. D., Collins, W. J., Kramer, R. J., Olivié, D., Skeie, R. B., O'Connor, F. M., Abraham, N. L., Checa-Garcia, R., Bauer, S. E., Deushi, M., Emmons, L. K., Forster, P. M., Horowitz, L. W., Johnson, B., Keeble, J., Lamarque, J.-F., Michou, M., Mills, M. J., Mulcahy, J. P., Myhre, G., Nabat, P., Naik, V., Oshima, N., Schulz, M., Smith, C. J., Takemura, T., Tilmes, S., Wu, T., Zeng, G., and Zhang, J.: Effective radiative forcing from emissions of reactive gases and aerosols – a multi-model comparison, Atmos. Chem. Phys., 21, 853–874, https://doi.org/10.5194/acp-21-853-2021, 2021.
Travis, K. R., Crawford, J. H., Chen, G., Jordan, C. E., Nault, B. A., Kim, H., Jimenez, J. L., Campuzano-Jost, P., Dibb, J. E., Woo, J.-H., Kim, Y., Zhai, S., Wang, X., McDuffie, E. E., Luo, G., Yu, F., Kim, S., Simpson, I. J., Blake, D. R., Chang, L., and Kim, M. J.: Limitations in representation of physical processes prevent successful simulation of PM2.5 during KORUS-AQ, Atmos. Chem. Phys., 22, 7933–7958, https://doi.org/10.5194/acp-22-7933-2022, 2022.
van der Werf, G. R., Randerson, J. T., Giglio, L., van Leeuwen, T. T., Chen, Y., Rogers, B. M., Mu, M., van Marle, M. J. E., Morton, D. C., Collatz, G. J., Yokelson, R. J., and Kasibhatla, P. S.: Global fire emissions estimates during 1997–2016, Earth Syst. Sci. Data, 9, 697–720, https://doi.org/10.5194/essd-9-697-2017, 2017.
Vasilakos, P., Russell, A., Weber, R., and Nenes, A.: Understanding nitrate formation in a world with less sulfate, Atmos. Chem. Phys., 18, 12765–12775, https://doi.org/10.5194/acp-18-12765-2018, 2018.
Wang, Q., Jacob, D. J., Fisher, J. A., Mao, J., Leibensperger, E. M., Carouge, C. C., Le Sager, P., Kondo, Y., Jimenez, J. L., Cubison, M. J., and Doherty, S. J.: Sources of carbonaceous aerosols and deposited black carbon in the Arctic in winter-spring: implications for radiative forcing, Atmos. Chem. Phys., 11, 12453–12473, https://doi.org/10.5194/acp-11-12453-2011, 2011.
Wang, Q., Jacob, D. J., Spackman, J. R., Perring, A. E., Schwarz, J. P., Moteki, N., Marais, E. A., Ge, C., Wang, J., and Barrett, S. R. H.: Global budget and radiative forcing of black carbon aerosol: Constraints from pole-to-pole (HIPPO) observations across the Pacific, J. Geophys. Res.-Atmos., 119, 195–206, https://doi.org/10.1002/2013JD020824, 2014.
Wang, Y., Jacob, D. J., and Logan, J. A.: Global simulation of tropospheric O3-NOx-hydrocarbon chemistry: 1. Model formulation, J. Geophys. Res.-Atmospheres, 103, 10713–10725, https://doi.org/10.1029/98JD00158, 1998.
Warneke, C., Trainer, M., de Gouw, J. A., Parrish, D. D., Fahey, D. W., Ravishankara, A. R., Middlebrook, A. M., Brock, C. A., Roberts, J. M., Brown, S. S., Neuman, J. A., Lerner, B. M., Lack, D., Law, D., Hübler, G., Pollack, I., Sjostedt, S., Ryerson, T. B., Gilman, J. B., Liao, J., Holloway, J., Peischl, J., Nowak, J. B., Aikin, K. C., Min, K.-E., Washenfelder, R. A., Graus, M. G., Richardson, M., Markovic, M. Z., Wagner, N. L., Welti, A., Veres, P. R., Edwards, P., Schwarz, J. P., Gordon, T., Dube, W. P., McKeen, S. A., Brioude, J., Ahmadov, R., Bougiatioti, A., Lin, J. J., Nenes, A., Wolfe, G. M., Hanisco, T. F., Lee, B. H., Lopez-Hilfiker, F. D., Thornton, J. A., Keutsch, F. N., Kaiser, J., Mao, J., and Hatch, C. D.: Instrumentation and measurement strategy for the NOAA SENEX aircraft campaign as part of the Southeast Atmosphere Study 2013, Atmos. Meas. Tech., 9, 3063–3093, https://doi.org/10.5194/amt-9-3063-2016, 2016.
Warneke, C., Schwarz, J. P., Dibb, J., Kalashnikova, O., Frost, G., Al-Saad, J., Brown, S. S., Brewer, Wm. A., Soja, A., Seidel, F. C., Washenfelder, R. A., Wiggins, E. B., Moore, R. H., Anderson, B. E., Jordan, C., Yacovitch, T. I., Herndon, S. C., Liu, S., Kuwayama, T., Jaffe, D., Johnston, N., Selimovic, V., Yokelson, R., Giles, D. M., Holben, B. N., Goloub, P., Popovici, I., Trainer, M., Kumar, A., Pierce, R. B., Fahey, D., Roberts, J., Gargulinski, E. M., Peterson, D. A., Ye, X., Thapa, L. H., Saide, P. E., Fite, C. H., Holmes, C. D., Wang, S., Coggon, M. M., Decker, Z. C. J., Stockwell, C. E., Xu, L., Gkatzelis, G., Aikin, K., Lefer, B., Kaspari, J., Griffin, D., Zeng, L., Weber, R., Hastings, M., Chai, J., Wolfe, G. M., Hanisco, T. F., Liao, J., Campuzano Jost, P., Guo, H., Jimenez, J. L., Crawford, J., and Team, T. F.-A. S.: Fire Influence on Regional to Global Environments and Air Quality (FIREX-AQ), J. Geophys. Res.-Atmos., 128, e2022JD037758, https://doi.org/10.1029/2022JD037758, 2023.
Weagle, C. L., Snider, G., Li, C., van Donkelaar, A., Philip, S., Bissonnette, P., Burke, J., Jackson, J., Latimer, R., Stone, E., Abboud, I., Akoshile, C., Anh, N. X., Brook, J. R., Cohen, A., Dong, J., Gibson, M. D., Griffith, D., He, K. B., Holben, B. N., Kahn, R., Keller, C. A., Kim, J. S., Lagrosas, N., Lestari, P., Khian, Y. L., Liu, Y., Marais, E. A., Martins, J. V., Misra, A., Muliane, U., Pratiwi, R., Quel, E. J., Salam, A., Segev, L., Tripathi, S. N., Wang, C., Zhang, Q., Brauer, M., Rudich, Y., and Martin, R. V.: Global Sources of Fine Particulate Matter: Interpretation of PM2.5 Chemical Composition Observed by SPARTAN using a Global Chemical Transport Model, Environ. Sci. Technol., 52, 11670–11681, https://doi.org/10.1021/acs.est.8b01658, 2018.
Wesely, M. L.: Parameterization of surface resistances to gaseous dry deposition in regional-scale numerical models, Atmos. Environ. (1967), 23, 1293–1304, https://doi.org/10.1016/0004-6981(89)90153-4, 1989.
WINTER Science Team: WINTER Data Sets, NCAR Earth Observing Laboratory [data set], https://data.eol.ucar.edu/master_lists/generated/winter (last access: 31 October 2024), 2016.
Womack, C. C., McDuffie, E. E., Edwards, P. M., Bares, R., de Gouw, J. A., Docherty, K. S., Dubé, W. P., Fibiger, D. L., Franchin, A., Gilman, J. B., Goldberger, L., Lee, B. H., Lin, J. C., Long, R., Middlebrook, A. M., Millet, D. B., Moravek, A., Murphy, J. G., Quinn, P. K., Riedel, T. P., Roberts, J. M., Thornton, J. A., Valin, L. C., Veres, P. R., Whitehill, A. R., Wild, R. J., Warneke, C., Yuan, B., Baasandorj, M., and Brown, S. S.: An Odd Oxygen Framework for Wintertime Ammonium Nitrate Aerosol Pollution in Urban Areas: NOx and VOC Control as Mitigation Strategies, Geophys. Res. Lett., 46, 4971–4979, https://doi.org/10.1029/2019GL082028, 2019.
Ye, C., Zhou, X., Pu, D., Stutz, J., Festa, J., Spolaor, M., Tsai, C., Cantrell, C., Mauldin, R. L., Campos, T., Weinheimer, A., Hornbrook, R. S., Apel, E. C., Guenther, A., Kaser, L., Yuan, B., Karl, T., Haggerty, J., Hall, S., Ullmann, K., Smith, J. N., Ortega, J., and Knote, C.: Rapid cycling of reactive nitrogen in the marine boundary layer, Nature, 532, 489–491, https://doi.org/10.1038/nature17195, 2016.
Zhai, S., Jacob, D. J., Wang, X., Liu, Z., Wen, T., Shah, V., Li, K., Moch, J. M., Bates, K. H., Song, S., Shen, L., Zhang, Y., Luo, G., Yu, F., Sun, Y., Wang, L., Qi, M., Tao, J., Gui, K., Xu, H., Zhang, Q., Zhao, T., Wang, Y., Lee, H. C., Choi, H., and Liao, H.: Control of particulate nitrate air pollution in China, Nat. Geosci., 14, 389–395, https://doi.org/10.1038/s41561-021-00726-z, 2021a.
Zhai, S., Jacob, D. J., Brewer, J. F., Li, K., Moch, J. M., Kim, J., Lee, S., Lim, H., Lee, H. C., Kuk, S. K., Park, R. J., Jeong, J. I., Wang, X., Liu, P., Luo, G., Yu, F., Meng, J., Martin, R. V., Travis, K. R., Hair, J. W., Anderson, B. E., Dibb, J. E., Jimenez, J. L., Campuzano-Jost, P., Nault, B. A., Woo, J.-H., Kim, Y., Zhang, Q., and Liao, H.: Relating geostationary satellite measurements of aerosol optical depth (AOD) over East Asia to fine particulate matter (PM2.5): insights from the KORUS-AQ aircraft campaign and GEOS-Chem model simulations, Atmos. Chem. Phys., 21, 16775–16791, https://doi.org/10.5194/acp-21-16775-2021, 2021b.
Zhai, S., Jacob, D. J., Pendergrass, D. C., Colombi, N. K., Shah, V., Yang, L. H., Zhang, Q., Wang, S., Kim, H., Sun, Y., Choi, J.-S., Park, J.-S., Luo, G., Yu, F., Woo, J.-H., Kim, Y., Dibb, J. E., Lee, T., Han, J.-S., Anderson, B. E., Li, K., and Liao, H.: Coarse particulate matter air quality in East Asia: implications for fine particulate nitrate, Atmos. Chem. Phys., 23, 4271–4281, https://doi.org/10.5194/acp-23-4271-2023, 2023.
Zhang, L., Gong, S., Padro, J., and Barrie, L.: A size-segregated particle dry deposition scheme for an atmospheric aerosol module, Atmos. Environ., 35, 549–560, https://doi.org/10.1016/S1352-2310(00)00326-5, 2001.
Zhang, L., Jacob, D. J., Knipping, E. M., Kumar, N., Munger, J. W., Carouge, C. C., van Donkelaar, A., Wang, Y. X., and Chen, D.: Nitrogen deposition to the United States: distribution, sources, and processes, Atmos. Chem. Phys., 12, 4539–4554, https://doi.org/10.5194/acp-12-4539-2012, 2012.
Zhang, Q., Jimenez, J. L., Canagaratna, M. R., Allan, J. D., Coe, H., Ulbrich, I., Alfarra, M. R., Takami, A., Middlebrook, A. M., Sun, Y. L., Dzepina, K., Dunlea, E., Docherty, K., DeCarlo, P. F., Salcedo, D., Onasch, T., Jayne, J. T., Miyoshi, T., Shimono, A., Hatakeyama, S., Takegawa, N., Kondo, Y., Schneider, J., Drewnick, F., Borrmann, S., Weimer, S., Demerjian, K., Williams, P., Bower, K., Bahreini, R., Cottrell, L., Griffin, R. J., Rautiainen, J., Sun, J. Y., Zhang, Y. M., and Worsnop, D. R.: Ubiquity and dominance of oxygenated species in organic aerosols in anthropogenically-influenced Northern Hemisphere midlatitudes, Geophys. Res. Lett., 34, L13801, https://doi.org/10.1029/2007GL029979, 2007.