the Creative Commons Attribution 4.0 License.
the Creative Commons Attribution 4.0 License.
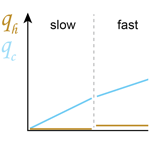
Microphysics regimes due to haze–cloud interactions: cloud oscillation and cloud collapse
Hamed Fahandezh Sadi
Raymond A. Shaw
Fabian Hoffmann
Aaron Wang
Mikhail Ovchinnikov
It is known that aqueous haze particles can be activated into cloud droplets in a supersaturated environment. However, haze–cloud interactions have not been fully explored, partly because haze particles are not represented in most cloud-resolving models. Here, we conduct a series of large-eddy simulations (LESs) of a cloud in a convection chamber using a haze-capable Eulerian-based bin microphysics scheme to explore haze–cloud interactions over a wide range of aerosol injection rates. Results show that the cloud is in a slow microphysics regime at low aerosol injection rates, where the cloud responds slowly to an environmental change and droplet deactivation is negligible. The cloud is in a fast microphysics regime at moderate aerosol injection rates, where the cloud responds quickly to an environmental change and haze–cloud interactions are important. More interestingly, two more microphysics regimes are observed at high aerosol injection rates due to haze–cloud interactions. Cloud oscillation is driven by the oscillation of the mean supersaturation around the critical supersaturation of aerosol due to haze–cloud interactions. Cloud collapse happens under weaker forcing of supersaturation where the chamber transfers cloud droplets to haze particles efficiently, leading to a significant decrease (collapse) in cloud droplet number concentration. One special case of cloud collapse is the haze-only regime. It occurs at extremely high aerosol injection rates, where droplet activation is inhibited, and the sedimentation of haze particles is balanced by the aerosol injection rate. Our results suggest that haze particles and their interactions with cloud droplets should be considered, especially in polluted conditions.
- Article
(6932 KB) - Full-text XML
- BibTeX
- EndNote
Atmospheric clouds play an important role in Earth's radiation balance and hydrological cycle. Their optical properties and precipitation efficiency are strongly influenced by cloud microphysical composition (e.g., droplet size and concentration) and processes (e.g., droplet formation and growth). It is known that cloud droplets in the atmosphere grow from aerosol particles, most of which contain water-soluble materials, such as sodium chloride or ammonium sulfate. These water-soluble aerosol particles first absorb water vapor in a subsaturated environment to become aqueous droplets (known as haze particles) through deliquescence. Haze particles can then be activated into cloud droplets in a sufficiently supersaturated environment (i.e., when relative humidity is higher than 100 %). The supersaturation needed to activate cloud droplets depends on aerosol properties as explained by Köhler theory (Twomey, 1959). Changes in aerosol properties from various anthropogenic and natural emissions can have a significant impact on clouds, thereby affecting the climate system substantially. So far, aerosol–cloud interaction remains one of the largest uncertainties in climate projection, partly because of the poor representation of cloud microphysical processes in models and incomplete understanding of those processes at the fundamental level (Morrison et al., 2020).
It is challenging to isolate the impact of aerosol on cloud properties and evolution in the real atmosphere because cloud microphysics, dynamics, and thermodynamics are coupled in a complex way. In addition, cloud properties fluctuate over time and space, making them difficult to thoroughly sample and interpret. In contrast, the Michigan Tech convection cloud chamber, also known as the Pi chamber, can maintain a steady-state cloud for several hours under well-controlled initial and boundary conditions (Chang et al., 2016). The Pi chamber produces a well-mixed supersaturated environment by maintaining a warm, humid bottom surface and a cool, humid top surface through Rayleigh–Bénard convection. The cloud is formed by continuously injecting aerosol particles into the supersaturated environment, and it can reach a steady state when the droplet activation rate is balanced by the droplet sedimentation rate. Cloud properties are controlled by aerosol properties (e.g., aerosol size, chemical composition, and injection rate) and boundary conditions (e.g., top and bottom temperatures – the driving factor to create a supersaturated environment). Steady-state cloud properties in the Pi chamber can be measured in great detail, which provides a unique opportunity to explore aerosol–cloud–turbulence interactions in well-controlled environments.
Previous Pi chamber experiments have shown that increasing aerosol injection rates result in higher cloud droplet number concentrations, smaller mean droplet radii, and narrower droplet size distributions (Chandrakar et al., 2016). These trends are consistent with results from cloud-resolving large-eddy simulations (LESs) of the Pi chamber (Thomas et al., 2019). Krueger (2020) derived an analytical expression for the equilibrium cloud droplet size distribution in a turbulent cloud chamber with the assumption of uniform supersaturation. This analytic droplet size distribution, along with three others that account for supersaturation fluctuations in different ways, has been compared with measured droplet size distributions in the Pi chamber (Chandrakar et al., 2020). Results show that all four analytical droplet size distributions match the observed distribution reasonably well for monodisperse aerosol injection. However, none of them matched well for polydisperse aerosol injections. Chandrakar et al. (2020) argued that it might be due to the Ostwald ripening effect (Korolev, 1995; Jensen and Nugent, 2017; Yang et al., 2018), which is not considered in those analytical models. Recently, Shaw et al. (2023) developed a theoretical model to describe the microphysical state of well-mixed monodisperse droplets in cloudy Rayleigh–Bénard convection. The model predicts that Nd∼nin and ql∼nin in the slow microphysics regime (i.e., at low aerosol injection rates), while and in the fast microphysics regime (i.e., at high aerosol injection rates), where Nd is the droplet number concentration, nin the aerosol injection rate, and ql the liquid water mixing ratio. The slow microphysics regime refers to a relatively clean condition where the cloud would respond slowly to an environmental change, while the fast microphysics regime refers to a relatively polluted condition where the cloud would respond quickly to an environmental change. Pi chamber observations confirm the nonlinear relationship between ql and nin in the fast microphysics regime (see Fig. 7 in Shaw et al., 2023), but more investigations are needed to evaluate the theory and its ability to represent microphysical properties in a convection cloud chamber.
Besides cloud droplets, observations using a digital optical particle counter show the existence of haze particles with diameters down to 0.6 µm (detection limit) in the Pi chamber (Prabhakaran et al., 2020). Results from direct numerical simulations with Lagrangian aerosol/droplet microphysics show that haze particles undergo multiple activation and deactivation cycles in a convection chamber (MacMillan et al., 2022). However, previous theoretical studies do not include the haze activation process for simplification (Krueger, 2020; Chandrakar et al., 2020; Shaw et al., 2023). In addition, most previous Pi chamber simulations do not fully resolve haze particles because in these simulations as well as in most atmospheric cloud simulations, droplets are formed directly from aerosol particles based on Twomey-type activation parameterizations (Twomey, 1959), in which aerosols are activated into cloud droplets if the environmental supersaturation is larger than the aerosol's critical supersaturation (Thomas et al., 2019; Grabowski, 2020). Recently, Yang et al. (2023) developed a haze-capable bin microphysics scheme to simulate the Pi chamber by directly calculating the condensational growth of haze and cloud droplets, which naturally resolves droplet activation process without further parameterization. Simulations using this haze-capable bin scheme can capture haze droplet size distributions, aligning well with simulations from a Lagrangian microphysics scheme, with the latter serving as the “truth” because it does not suffer numerical diffusion during droplet growth and advection (Morrison et al., 2018; Grabowski et al., 2019). Results also show that the simulated cloud properties using the haze-capable bin microphysics scheme agree reasonably well with those using Twomey-type activation. We refer to the Twomey-type activation scheme as the cloud condensation nuclei (CCN)-based bin microphysics scheme because it treats dry aerosols as CCN which behave like cloud droplets immediately after the environmental supersaturation is larger than a critical supersaturation (i.e., without resolving the growth of haze particles). Good agreement between the haze-capable and CCN-based bin microphysics schemes suggests that if we are only interested in the cloud microphysical properties, we could still use Twomey-type activation parameterizations. However, only two aerosol injection rates were used in Yang et al. (2023), and thus, it is not clear whether results from the CCN-based bin microphysics scheme will always be similar to those from the haze-capable bin microphysics scheme, especially in a low-supersaturation environment where haze–cloud interaction is important (e.g., Prabhakaran et al., 2020).
In this study, we conduct a series of large-eddy simulations of the Pi chamber using both CCN-based and haze-capable bin microphysics schemes over a wide range of aerosol injection rates. We aim to address the following questions:
- (a)
How do cloud microphysical properties change over a wide range of aerosol injection rates (for constant boundary conditions)?
- (b)
Do simulation results agree with previous theoretical studies?
- (c)
How important are haze–cloud interactions in the Pi chamber as well as in natural clouds?
Specifically, related to question (a), we aim to explore how the steady-state supersaturation, the mean droplet radius, Nd, and ql change with aerosol injection rate. For question (b), we aim to evaluate steady-state droplet size distribution predicted in Krueger (2020) and Chandrakar et al. (2020), as well as slow and fast microphysics regimes predicted in Shaw et al. (2023). Related to question (c), we aim to know whether cloud properties simulated by the CCN-based bin microphysics scheme are always consistent with those from the haze-capable bin microphysics scheme, as indicated by Yang et al. (2023), or if haze-capable microphysics must be used for certain atmospheric conditions. Note that the Pi chamber could be connected to some simple cloud systems like fog or non-drizzling shallow-layer clouds. Therefore, what we learn about haze–cloud interactions can be transferred. We want to understand the conditions under which haze–cloud interactions become important, connecting our work to a broader atmospheric science context.
We employ SAM-Chamber to conduct large-eddy simulations of the Pi chamber in this study. SAM-Chamber is an adapted and modified version of the System for Atmospheric Modeling (SAM; Khairoutdinov and Randall, 2003) with the major changes in the consideration of four side walls and the top surface to represent the chamber boundary condition (detailed in Thomas et al., 2019). SAM-Chamber has been used to simulate the Pi chamber to explore several topics, including the impact of various bin microphysics and advection schemes on Pi chamber simulations (Yang et al., 2022), impact of supersaturation fluctuations on droplet formation and growth (Prabhakaran et al., 2022; Anderson et al., 2023), development of a haze-capable microphysics scheme (Yang et al., 2023), investigation of drizzle initiation in larger convection chambers (Thomas et al., 2023; Wang et al., 2024c), glaciation of mixed-phase clouds (Wang et al., 2024a), and dual signatures of entrainment (Wang et al., 2024b). SAM-Chamber employed in this study is the one used in Wang et al. (2024c), where the wall fluxes of momentum, sensible heat, and moisture are modeled in accordance with Monin–Obukhov similarity theory (MOST; Monin and Obukhov, 1954) as before but with the following changes: (1) the roughness lengths for momentum (z0), sensible heat (zt), and moisture (zq) are tuned to match the mean fluxes obtained in the direct numerical simulations. (2) The hydrostatic stability on the side walls is assumed to be neutral, as the buoyancy is parallel rather than normal to the side walls. More details on the wall modeling are addressed in Wang et al. (2024c; see Sect. 2 and Appendix B therein).
(based on Wang et al., 2024c)(Yang et al., 2023)The model setup is summarized in Table 1. The temperature of the bottom surface is set to be 300 K, the top surface to be 280 K, and the side walls to be 290 K. In previous SAM-Chamber simulations (Thomas et al., 2019; Yang et al., 2022, 2023), the side walls were set to be subsaturated such that the domain-averaged supersaturation without cloud is about 2.5 % based on early chamber observations (Chandrakar et al., 2016). Subsaturated side walls serve as a sink for water vapor, tending to evaporate droplets nearby. Sidewalls have been improved (i.e., closer to be water saturated) recently in the real Pi chamber, such that clouds can form at much smaller top and bottom temperature differences (Prabhakaran et al., 2020). In this study, all surfaces are set to be saturated with respect to water. The impact of side wall conditions on cloud properties will be discussed later. The simulation domain is 2 m × 2 m × 1 m with 6.25 cm grid spacing in all three directions. This grid spacing falls in the inertial subrange, according to the direct numerical simulations with similar Reynolds number and Rayleigh number performed by Wang et al. (2024d).
To mimic continuous injection of salt particles, monodisperse sodium chloride aerosol particles with a dry radius of 62.5 nm are added in each grid box after each time step, as in previous studies (Yang et al., 2022, 2023). Cloud droplet formation and growth by condensation are simulated using either a CCN-based or haze-capable bin microphysics scheme. Both schemes are two-moment bin microphysics schemes based on Chen and Lamb (1994), with some differences detailed in Yang et al. (2023) and summarized below. For the CCN-based bin microphysics scheme (referred to as the CLCCN), droplet size distribution is represented by 33 mass-doubling bins starting from 1 µm radius. Dry aerosol particles stay in the aerosol category, and they will be moved to the first bin of the cloud category if the environmental supersaturation (in their grid box) is larger than the critical supersaturation of the aerosol (0.08 % for a salt particle of 62.5 nm in radius). Solute and curvature effects are not considered for droplet growth by condensation. Note that such treatment of cloud microphysical processes – Twomey-type parameterization of droplet formation and neglect of solute and curvature effects on droplet growth – is quite common in atmospheric cloud simulations. For the haze-capable bin microphysics scheme (referred to as the CLHaze), aqueous droplets (including haze and cloud) are represented by 40 mass-doubling bins starting from 0.1 µm radius. Dry aerosol particles initially become haze with the equilibrium size at a relative humidity of 90 % (same as in Yang et al., 2023). The growth of haze and cloud droplets via condensation is calculated explicitly with solute and curvature effects considered, and thus the activation process from haze particle to cloud droplet is naturally resolved. Following Yang et al. (2023), haze particles here refer to droplets with radii smaller than 1 µm, which is the bin edge closest to the critical radius of the aerosol (0.92 µm). In this study, we consider the solute and curvature effects for the growth of cloud droplets (radii larger than 1 µm) in both CLCCN and CLHaze schemes. The main difference between the CLCCN scheme and the CLHaze scheme is the way to handle droplet activation as detailed above. Although all chamber surfaces are saturated with respect to water, droplet deactivation by evaporation can still occur due to turbulent supersaturation fluctuations. For the CLCCN scheme, evaporated droplets will be moved to the aerosol category if their radii get smaller than 1 µm in radius (the deactivation process). For the CLHaze scheme, deactivated droplets remain as haze particles. Efflorescence is not considered, and if haze particles are less than 0.1 µm in radius, they stay in the smallest droplet bin. In both schemes, droplets can only be lost through the bottom surface due to sedimentation but not through the side walls.
Following the modeling studies by Yang et al. (2023) and Wang et al. (2024a, c, b), sodium chloride aerosol particles of a 62.5 nm radius are injected uniformly throughout the computational domain at a prescribed volumetric rate. A total of 25 aerosol injection rates (nin) are employed to explore their impact on cloud properties. nin ranges from 0.001 to 50 cm−3 s−1 in the following way: 0.001 to 0.005 cm−3 s−1 every 0.001 cm−3 s−1, 0.01 to 0.05 cm−3 s−1 every 0.01 cm−3 s−1, 0.1 to 0.5 cm−3 s−1 every 0.1 cm−3 s−1, 1.0 to 5.0 cm−3 s−1 every 1.0 cm−3 s−1, and 10.0 to 50.0 cm−3 s−1 every 10.0 cm−3 s−1. Note that 14 values of nin between 0.2 and 13 cm−3 s−1 were used in recent Pi chamber experiments (see Fig. 7 in Shaw et al., 2023), while only two values (0.25 and 2.5 cm−3 s−1) were used in the Pi chamber simulations by Yang et al. (2023). Here, we cover a range of nin that can be achieved in the Pi chamber, while extending nin to represent extremely clean and polluted conditions. Although these exceptionally small and large nin values might be difficult to achieve in the real chamber mainly due to the current limitations of aerosol injection, they are helpful to explore haze–cloud interactions in various microphysics regimes that will be discussed in the next section.
The time step is 0.02 s, and the total simulation is 1 h. The domain-averaged data are output every minute from the beginning of the simulation, while instantaneous 3-D data are output every 5 min in the second half of the simulation.
3.1 Impact of aerosol injection rate on bulk cloud properties
Figure 1 shows the impact of nin on droplet mean radius (rd), Nd, and ql. Here, ql is the liquid water mixing ratio. Specifically, rd and Nd are calculated only for cloud droplets whose radii are larger than 1 µm. for the CLHaze scheme, where qc is the cloud water mixing ratio (for droplets radii larger than 1 µm) and qh is the haze water mixing ratio (for droplets radii smaller than 1 µm), while ql=qc for the CLCCN scheme. Each dot in the figure represents a temporally averaged (over the second half an hour) and spatially averaged (over the whole domain) value for one aerosol injection rate when using either the CLCCN (black) or CLHaze (red) scheme. Results show that cloud microphysical properties based on these two schemes are similar, suggesting that using the Twomey-type activation parameterization is good enough to simulate bulk cloud properties, especially for Nd and ql.
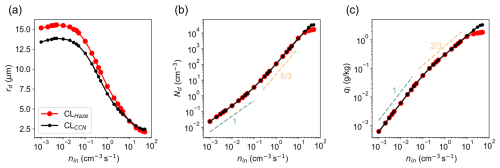
Figure 1Spatially averaged (over the whole domain) and temporally averaged (in the second half an hour) (a) mean droplet radius rd, (b) droplet number concentration Nd, and (c) liquid water mixing ratio ql at various aerosol injection rates. Black and red dots are results using CLCCN and CLHaze schemes, respectively. Each dot represents the average of the variable over the whole domain from the second half an hour. The light-green and yellow-colored dashed lines in (b) and (c) are scaling relationships based on Shaw et al. (2023) in slow and fast microphysics regimes, respectively. Note that we only consider cloud droplets whose radii are larger than 1 µm to calculate rd and Nd here.
The steady-state droplet size distributions based on the CLCCN and CLHaze schemes are shown in Fig. 2a–b. The distribution becomes narrower and shifts to smaller sizes with nin, consistent with previous Pi chamber observations (Chandrakar et al., 2016) and simulations (Thomas et al., 2019; Yang et al., 2023). The mode of small haze particles can only be captured by the CLHaze scheme and is enhanced as nin increases (Fig. 2b). We also compare the simulated size distributions with four analytical droplet size distributions: arexp (−br4) (Fig. 2c), arexp (−br2) (Fig. 2d), ar2exp (−br3) (Fig. 2e), and (Fig. 2f), where a and b represent the combinations of other variables and parameters except for r. All these analytical distributions use steady-state Nd and qc from the SAM-Chamber simulations as input to calculate the parameters a and b. The precise formulas are displayed in Fig. 2c–f. Chandrakar et al. (2020) detailed the assumptions regarding these analytical distributions and evaluated them with the Pi chamber observations. In short, arexp (−br4) is derived from the assumption of droplet growth in a constant supersaturation environment with size-dependent removal (Krueger, 2020), arexp (−br2) comes from droplet growth in a fluctuating supersaturation environment with size-independent removal (McGraw and Liu, 2006; Saito et al., 2019), ar2exp (−br3) results from the principle of maximum entropy assumption (Liu and Hallett, 1998), and comes from droplet growth in a fluctuating supersaturation environment with size-dependent removal (Chandrakar et al., 2020). Results show that the simulated cloud droplet size distributions are closer to arexp (−br4), ar2exp (−br3), and , compared to arexp (−br2), which produces significantly broader spectra (Fig. 2d). Furthermore, the haze mode is not captured by any analytical distribution, simply because none of those analytical models considers the full activation process – from haze particles to cloud droplets.
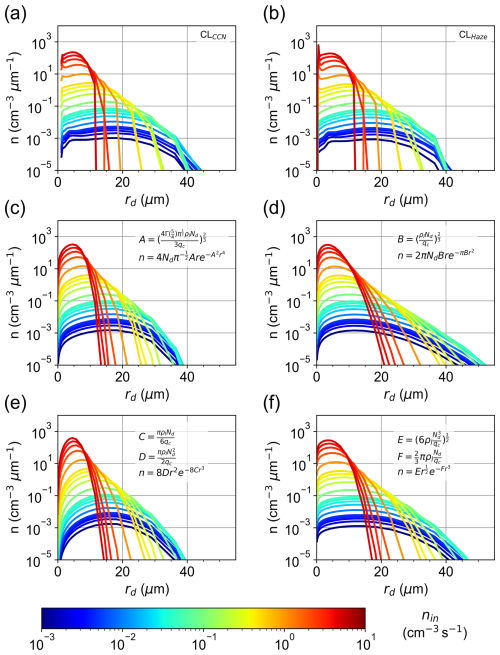
Figure 2Steady-state droplet size distributions for different aerosol injection rates when using (a) CLCCN and (b) CLHaze schemes. (c–f) Four analytical droplet size distributions using the domain-averaged Nd and qc as input, with the precise formulas displayed in the legend.
Slow and fast microphysics regimes are observed as shown in Fig. 1. The impact of nin on the mean supersaturation s and its standard deviation σs (see Fig. 3) indicates the physical origin of these two microphysics regimes and its connection to various activation regimes. The slow microphysics regime is observed when nin< 0.1 cm−3 s−1. In this regime, few droplets (i.e., very small Nd shown in Fig. 1b) grow in a high-supersaturation environment (Fig. 3a) before they fall out, leading to a roughly constant rd (Fig. 1a) and a linear relationship between nin and Nd (Fig. 1b) as well as ql (Fig. 1c) as predicted by Shaw et al. (2023). Based on the definition in Prabhakaran et al. (2020), the cloud is in the mean-supersaturation-dominated activation regime where s>>scrit.
When 0.1 cm−3 s−1 cm−3 s−1, the cloud is in the fast microphysics regime, in which more cloud droplets compete with each other for available water vapor needed for their condensational growth, leading to larger Nd and smaller rd. In this regime, rd, s, and σs decrease with nin, while and , consistent with theory. Based on the definition in Prabhakaran et al. (2020), the cloud is in the supersaturation-fluctuation-influenced activation regime (s>scrit and σs>scrit) or supersaturation-fluctuation-dominated activation regime (s<scrit and σs>scrit), but the latter is barely observed in our results.
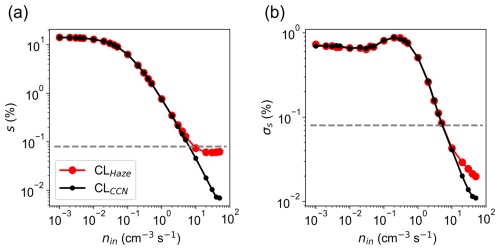
Figure 3Spatially averaged (over the whole domain) and temporally averaged (in the second half an hour) (a) mean supersaturation s and (b) standard deviation of supersaturation σs at various aerosol injection rates. Black and red dots are results using CLCCN and CLHaze schemes, respectively. Each dot represents the average of the variable over the whole domain from the second half an hour. The horizontal dashed line indicates the critical supersaturation of injected aerosols (0.08 %).
The scaling laws for Nd and ql do not work well for nin≥10.0 cm−3 s−1 when using the CLHaze scheme (Fig. 1b and c). Also note that both s and σs are smaller than scrit at these high aerosol injection rates, suggesting that droplet activation is strongly suppressed. It is interesting to see that s approaches a value that is slightly smaller than scrit when using the CLHaze scheme, while in contrast, s continuously decreases with nin and approaches 0 when using the CLCCN scheme. This is because the cloud system is buffered by a huge number of cloud droplets in the polluted condition and s should be close to the equilibrium supersaturation over droplets (which is scrit when using the CLHaze scheme where solute and curvature effects are considered or 0 when using the CLCCN scheme). This regime turns out to be very important for haze–cloud interactions, which will be explored in the following section.
Table 2Spatially and temporally averaged aerosol/haze number concentration (Na or Nh; cm−3), cloud droplet number concentration (Nd; cm−3), mean cloud droplet radius (rd; µm), droplet activation rate (Ract; cm−3 s−1), and droplet deactivation rate (Rdeact; cm−3 s−1) at different aerosol injection rates (nin; cm−3 s−1). Values before and after the slash are results when using the CLCCN and CLHaze schemes, respectively. Each value is averaged over the whole domain in the second half an hour at a given nin.
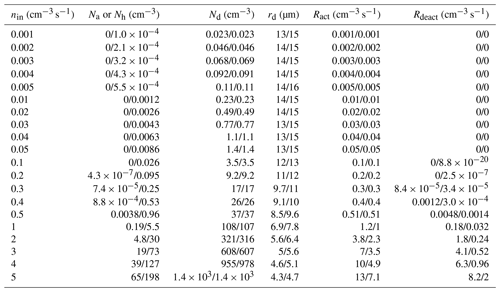
Table 2 summarizes the spatially and temporally averaged cloud microphysical properties for nin≤5.0 cm−3 s−1 when the scaling laws work reasonably well. Those variables include aerosol (when using the CLCCN scheme)/haze (when using the CLHaze scheme) number concentration (Na/Nh), cloud droplet number concentration (Nd), mean cloud droplet radius (rd), droplet activation rate (Ract), and deactivation rate (Rdeact). The droplet activation rate represents the number of newly formed cloud droplets per cubic centimeter per second, while the deactivation rate represents the reverse process. Note that the net activation rate (Ract−Rdeact, the last two columns in Table 2) is close to nin (the first column in Table 2) for each case suggesting that the cloud reaches a quasi-steady state. It is worth mentioning that although the simulated cloud properties using the two schemes are similar, unactivated particle concentration (Na or Nh), Ract, and Rdeact are quite different for nin≥1.0 cm−3 s−1. Our results suggest that haze–cloud interactions are important in the fast microphysics regime. The transition from the slow to the fast microphysics regime occurs when haze particles become important: % and % for nin≥1.0 cm−3 s−1 (Fig. 4).
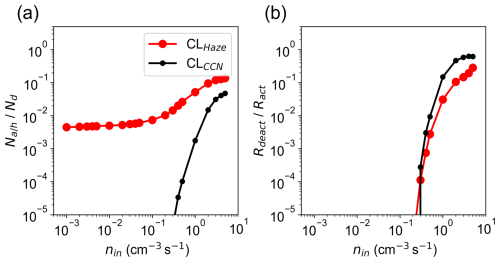
Figure 4(a) The ratio of the unactivated particle number concentration to the cloud droplet number concentration for different aerosol injection rates (nin). Unactivated particles are aerosol particles when using the CLCCN scheme or haze particles when using the CLHaze scheme. (b) The ratio of deactivation to activation rate for different nin.
Shaw et al. (2023) predicted that the transition from slow to fast microphysics regimes occurs at Da ≈1. Here Da is the Damköhler number, defined as the ratio of turbulent mixing time (τm) to phase relaxation time (τp) (see Eq. 1 in Lehmann et al., 2009). τp is inversely proportional to the product of Nd and rd, which can be determined from our simulation results. Take nin=0.1 cm−3 s−1 as an example, τp≈70 s, calculated from Nd=3.5 cm−3 and rd=12 µm based on Table 2 (using Eq. 18 in Korolev and Mazin, 2003). The apparent transition between slow and fast regimes as shown in Fig. 1 provides an opportunity to estimate τm, which is about 70 s for our boundary conditions (e.g., 20 K difference in top and bottom temperature), if we assume the transition occurs at Da ≈1. However, this value is larger than another estimate of τm via . Here, H=1 m is the chamber height, and vair≈0.1 m s−1 is the characteristic air speed in the chamber based on LESs, leading to τm on the order of 10 s. It is also larger than another estimate of s, where ϵ is the energy dissipation rate (about 0.005 m2 s−3 from the simulation).
3.2 Haze–cloud interactions in the polluted conditions
Figure 1c and d show that Nd and ql do not follow the aforementioned scaling laws for nin≥10 cm−3 s−1. In this section, we explore the reason for this departure and show that haze–cloud interaction in these extremely polluted conditions can lead to some new microphysics regimes, including cloud oscillation, cloud collapse, and haze only.
3.2.1 Cloud oscillation
One possible reason for the observed departure for Nd and ql in the polluted conditions (nin≥10 cm−3 s−1) is that the cloud does not reach a steady state after 1 h. To rule out this possibility, we extend the simulations of the largest five nin (10, 20, 30, 40, 50 cm−3 s−1) to a total simulation time of 10 h. Figure 5 shows the time series of domain-averaged ql, qc, Nd, Na (for the CLCCN scheme), Nh (for the CLHaze scheme), total particle concentration (NT), and rd. Note that ql≥qc and when using the CLHaze scheme, and the difference (ql−qc) is haze water mixing ratio (qh), while ql=qc and when using the CLCCN scheme. Results show that ql, Nd, and rd always reach a steady state when using the CLCCN scheme. Note that Na and NT increase with time for nin≥ 40 cm−3 s−1. This is because the sink of aerosol due to droplet activation is smaller than the source of aerosol due to aerosol injection, and thus aerosol particles accumulate. When using the CLHaze scheme, the cloud reaches a steady state for an aerosol injection rate of 10 cm−3 s−1, where ql is dominated by qc. In contrast, for nin≥20 cm−3 s−1, cloud microphysical properties (such as ql, qc, Nd, rd) oscillate. The oscillation period increases as nin increases, and the periods are 15, 20, 25, and 30 min for nin=20, 30, 40, and 50 cm−3 s−1. Meanwhile, the oscillation amplitude increases with nin. NT has a much smaller oscillation magnitude compared with Nd and Nh, suggesting that oscillations of Nh and Nc are out of phase. The local maximum of Nd corresponds to the local minimum of Nh, indicating the burst of droplet formation is due to the activation of a large number of haze particles. The ratio of qh (i.e., ql–qc) to ql increases with nin, and it can be up to 30 % for nin=50 cm−3 s−1. Note that the oscillation of the mean rd is mainly due to droplet activation/deactivation, not due to the physical growth/evaporation of cloud droplets. For example, the rapid formation of numerous small cloud droplets decreases the mean rd accordingly.
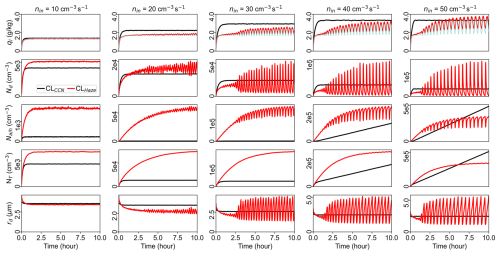
Figure 5Time series of domain-averaged ql (first row), Nd (second row), Na or Nh (third row), NT (fourth row), and rd (fifth row) for five different nin: 10, 20, 30, 40, and 50 cm−3 s−1. The light-blue line in the first row represents the cloud water mixing ratio (qc) when using the CLHaze scheme.
Figure 6 shows time series of domain-averaged activation rate (Ract), deactivation rate (Rdeact), supersaturation (s), standard deviation of supersaturation (σs), and surface precipitation rate (P). Here surface precipitation refers to the sedimentation of cloud droplets at the bottom surface. Results show that oscillations of bulk cloud properties when using the CLHaze scheme, as shown in Fig. 5, are associated with oscillations of process rates, like Ract, Rdeact, and P. It is interesting to see that s is close to scrit (about 0.08 %) when using the CLHaze scheme, while s decreases with nin and approaches 0 when using the CLCCN scheme. This is because the cloud system is buffered by a huge number of cloud droplets in the polluted condition and s should be close to the equilibrium supersaturation over droplets. This equilibrium supersaturation is scrit when using the CLHaze scheme where solute and curvature effects are considered, but it is 0 when using the CLCCN scheme. Because σs is much smaller than scrit at high injection rates, droplet activation is mainly controlled by the mean s. The oscillation of s around the scrit leads to the oscillation of droplet activation and further causes the oscillation of cloud properties.
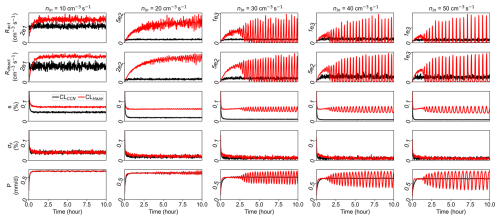
Figure 6Same cases in Fig. 5, time series of domain-averaged Ract (first row), Rdeact (second row), s (third row), σs (fourth row), and surface precipitation rate P (fifth row) for five different nin: 10, 20, 30, 40, and 50 cm−3 s−1.
Figure 7 shows the time evolution of mean profiles of cloud properties in the last hour of the simulation for nin=40 cm−3 s−1. We note that qc and qh oscillate out of phase (Fig. 7a vs. d), while ql is mainly influenced by qc (Fig. 7g). Larger qc (qh) corresponds to smaller Nd (Nh) and vice versa (Fig. 7a vs. b and d vs. e). The anti-correlation between qc and Nd is opposite to their scaling relationships in the slow and fast microphysics, which are qc∼Nd and , respectively (Shaw et al., 2023). The sharp increase in Nd (Fig. 7b) corresponds to a larger activation rate (Fig. 7c) due to the enhanced supersaturation (Fig. 7i), while the decrease in Nd corresponds to a larger deactivation rate and a smaller supersaturation. To better show the low value of Ract and Rdeact, we constrain the range of Ract and Rdeact to values below 240 cm−3 s−1 if their values are larger than it when plotting Fig. 7c and f. It can be seen that deactivation occurs in a much larger region (i.e., outside the top and bottom surfaces) and over a longer period within one cycle. However, the peak of Ract is larger than the peak of Rdeact (see Fig. 6 first and second rows). The net activation rate (Ract−Rdeact) averaged over one cycle should still be positive so that sedimentation is balanced by the net activation.
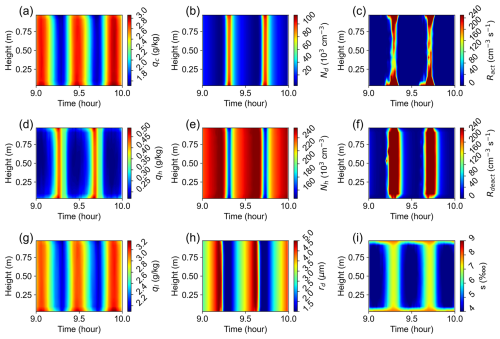
Figure 7Time evolution of mean profiles of (a) cloud water mixing ratio, qc; (b) cloud droplet number concentration, Nd; (c) activation rate, Ract; (d) haze water mixing ratio, qh; (e) haze number concentration, Nh; (f) deactivation rate, Rdeact; (g) total water mixing ratio, ql; (h) droplet radius, rd; and (i) supersaturation, s, for nin=40 cm−3 s−1 between 9 and 10 h when using the CLHaze scheme. It is the last simulation hour of Fig. 5, second column.
To further explore the mechanism of the oscillation, we pick one oscillation cycle for nin=40 cm−3 s−1. Figure 8 shows the phase diagram of four pairs of variables: Nh vs. Nd, qh vs. qc, qh vs. Nh, and qc vs. Nd. Each circle in the figure represents the domain-averaged value at one time and its color represents the domain-averaged supersaturation with the unit of , 1 per 10 000. The size of the circle represents the mean droplet radius in a relative way: a larger circle means a larger rd. The oscillation behavior can be explained by the circulation in the phase diagram. Taking Fig. 8d as an example: start from the lower left corner where qc and Nd are low, s is high, and rd is large. When s>scrit (scrit≈8
in this study), a huge number of droplets are activated leading to a sharp increase in Nd. Newly formed cloud droplets significantly decrease the mean rd, and they grow in slightly supersaturated conditions, leading to an increase in ql and a decrease in s. Shortly thereafter, Nd decreases because droplet activation is suppressed when s<scrit, and meanwhile, droplets are lost due to sedimentation and deactivation. Note that droplet loss is dominated by deactivation, and deactivation is driven by the mean supersaturation rather than supersaturation fluctuation because s oscillates around scrit while σs is much smaller than scrit as shown in Fig. 6. Droplet deactivation causes a recovery of Nh and an increase in qh (Fig. 8a, b). The decrease in Nd finally results in a decrease in ql and an increase in s. When s>scrit, another period starts. Note that droplet activation leads to an increase in Nd and a decrease in Nh simultaneously, thus causing the perfect anticorrelation between Nh and Nd (Fig. 8a). In contrast, mass and number concentrations (either qh vs. Nh or qc vs. Nd) peak at different times because it takes time for droplets/haze to grow. It is interesting to see that the oscillation evolves with time counterclockwise in the diagram (Fig. 8c) and the diagram (Fig. 8d), suggesting that the change in number concentration is ahead of the change in mass mixing ratio in their phases, analogous to a predator–prey dynamical system.
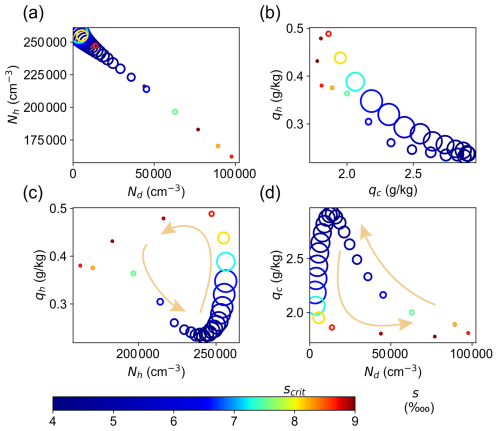
Figure 8The relationship between domain-averaged (a) Nh vs. Nd, (b) qh vs. qc, (c) qh vs. Nh, and (d) qc vs. Nd over one cycle of cloud oscillation at nin=40 cm−3 s−1. The size of the circle represents the mean droplet radius in a relative way; e.g., a larger circle means a larger rd. Its color stands for the domain-averaged supersaturation with a unit of , 1 per 10 000. The arrows in (c) and (d) represent its time evolution in one cycle.
3.2.2 Cloud oscillation in a box model
To make sure the oscillation is physical and not due to numerical artifacts from using an Eulerian-based bin microphysics scheme, we develop a box model using a particle-based microphysics approach to simulate a cloud in a convection chamber. The particle-based treatment, analogous to the Lagrangian droplet method, directly calculates and tracks the time evolution of droplet size. The well-mixed cloud system can be described by a set of differential equations detailed below.
Following Shaw et al. (2023), the time derivative of mean air temperature can be expressed as
where T0 is the reference temperature, which equals the mean temperature in the chamber without cloud droplets. L is the latent heat of vaporization of water, and cp is the specific heat of air. τm is the mixing timescale, which quantifies how efficient T can be restored to T0. Similarly, the time derivative of the water vapor mixing ratio is expressed as
where qv0 is the reference water vapor mixing ratio, which equals the mean water vapor mixing ratio in a cloud-free condition assuming both top and bottom surfaces are saturated with respect to water. The last terms in Eqs. (1) and (2) represent the impact of vapor diffusional growth () of droplets on T and qv, respectively.
To be consistent with the model setup of large-eddy simulations, monodisperse dry aerosol particles with radii of 62.5 nm are added at a constant rate using a particle-based super droplet method. Specifically, one new super particle (hereafter referred to as particle) is added at a constant rate: every second for nin≤5 cm−3 s−1 or every 20 s for the largest five nin to save computational time. Each particle represents numerous real particles per unit volume. We refer to this as multiplicity, denoted hereafter as ni, which represents the concentration of a particle with an index of i. Note that the multiplicity in this study is different from that in Lagrangian microphysics schemes (e.g., Shima et al., 2009; Hoffmann et al., 2015) in which it represents multiple number (instead of concentration) of identical droplets represented by the Lagrangian particle/superdroplet. The growth rate of droplet radius with an index of i is given by
where G is the growth factor, and s is the supersaturation depending on both T and qv. and are curvature and solute effects, respectively, in which A and B are constant for given thermodynamic and aerosol conditions (Eq. 6.6 in Rogers and Yau, 1996). The change in liquid water mixing ratio, which is linked to the last terms in Eqs. (1) and (2), can be calculated as the sum of mass change of all droplets,
Here ρa and ρl are air and liquid water densities, respectively.
Equations (1)–(4) are the governing equations to describe the bulk properties of a well-mixed cloud in a convection chamber. We use an ordinary differential equation solver to solve the above set of nonlinear and stiff equations (Brown et al., 1989). The total number of equations in the system depends on the number of particles. For example, if we have 100 particles at a given moment, the total number of equations to be solved is 102 (100 for ri, 1 for T, and 1 for qv). The same solver has been used in adiabatic cloud parcel models to properly calculate the growth of haze particles and the droplet activation process in the real atmosphere (Xue and Feingold, 2004; Chen et al., 2016; Yang et al., 2016).
Without sedimentation, the number of particles in the system would increase with time due to continuous injection, which eventually makes the system numerically unsolvable. In reality, the number of particles increases with time at the beginning, but it could reach a steady state if the rate of increase in particles due to injection is balanced by its loss rate due to sedimentation. To represent the impact of gravitational sedimentation, ni decreases with time as
where δt is set to be 1 s, and δni is the decreased amount of multiplicity of a particle with the index of i. τsed is the characteristic sedimentation time of a droplet with a radius of ri in a convection cloud chamber,
Here H is the chamber height of 1 m, and vt is the terminal velocity of a droplet with a radius of ri. If ni is smaller than a threshold of 10−10 cm−3, we remove that particle.
We conduct a total of 25 cases with the same forcing (i.e., T0, qv0, and τm) but different nin, which are the same as those used in previous large-eddy simulations. For a given nin, the multiplicity of a newly added particle (ni0) and the injection frequency are determined such that their product equals nin. For example, injection of a particle with ni0=0.5 cm−3 every second corresponds to nin=0.5 cm−3 s−1, while injection of a particle with ni0=200 cm−3 every 20 s corresponds to nin=10 cm−3 s−1. T0 and qv0 are set to be 290 K and 13.9 g kg−1, corresponding to a supersaturation of 15 % in the absence of all hydrometeors. This setup is consistent with the cloud-free humid condition in a convection chamber with a top temperature of 280 K and a bottom temperature of 300 K, with both surfaces saturated with respect to water. τm is set to be 165 s, such that the steady-state s from the box model (Fig. 9a) agrees with that from the LES (Fig. 1a). Note that the value of τm used here is not the same as the estimated τm (∼70 s) for Da=1 based on LES results above, but they are the same order of magnitude.
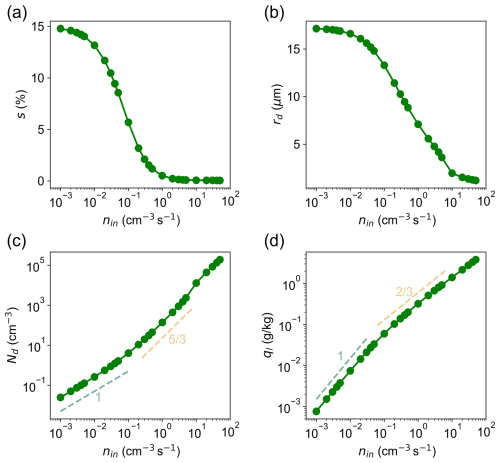
Figure 9Impact of nin on (a) supersaturation s, (b) mean droplet radius rd, (c) droplet number concentration Nd, and (d) liquid water mixing ratio ql based on a box model using a particle-based microphysics approach. Cloud oscillation occurs for the five largest nin values (10, 20, 30, 40, 50 cm−3 s−1), as shown in Fig. 10. For those cases, s, rd, Nd, and ql are averaged over one cycle. The light-green and yellow-colored dashed lines in (c) and (d) are scaling relationships based on Shaw et al. (2023) for slow and fast regimes, respectively.
Results show that the impact of nin on cloud properties based on the box model is consistent with those from LESs (compare Fig. 9 vs. Fig. 1). Slow and fast microphysics regimes are also captured by the box model (Fig. 9c, d). It is encouraging to see that the transition between slow and fast microphysics regimes occurs at around nin of 0.1 cm−3 s−1, which agrees well with LESs. The box model also captures cloud oscillation for the largest five nin (10, 20, 30, 40, 50 cm−3 s−1), as shown in Figs. 10 and 11. The oscillation frequency decreases with the increase in nin, which is consistent with LES results (Fig. 5). Note that, for cloud oscillation cases, s, rd, Nd, and ql in Fig. 9 are averaged over one cycle. It is interesting to see that Nd vs. nin and ql vs. nin agree better with the aforementioned scaling laws in the fast microphysics regime, compared with LESs (compare Fig. 9c, d vs. Fig. 1c, d). This might be due to the bias in representing droplet distribution when using a limited number of discretized bins in polluted conditions or the systematic difference between a 3-D LES and a box model.
3.2.3 Origin of cloud oscillation
Results from LES and box models show the existence of cloud oscillation at high nin, indicating that cloud oscillation is physically plausible, not due to numerical artifact. In this subsection, we discuss the physical origin of cloud oscillation and explain why the CLHaze scheme can simulate cloud oscillation, while the CLCCN scheme fails.
Time series of s shown in Figs. 6 and 11 provide more physical insights of cloud oscillation. The direct reason for cloud oscillation is that s oscillates around scrit when using the CLHaze scheme. To be clear, cloud oscillation mentioned in this study represents the oscillation of cloud bulk statistical properties. It is the oscillation of the whole well-mixed cloud system, not an individual droplet. The physical origin of cloud oscillation is due to the nonlinear interactions between haze and cloud droplets in a dynamic system:
-
First, the supersaturation s in the system is very close to scrit, and most of the time s<scrit. This can happen in a heavily polluted condition where there are many haze particles.
-
There is a forcing in the system to maintain the supersaturation. In the Pi chamber, the forcing is due to the temperature difference between the top and bottom surfaces. In the real atmosphere, the forcing can be due to adiabatic cooling (e.g., in a rising cloud parcel) or radiative cooling (e.g., radiation fog).
-
When s>scrit, a huge number of haze particles activate to cloud droplets and the consumption of water vapor due to droplet condensational growth leads to s<scrit.
-
Under s<scrit conditions, droplet activation is suppressed and droplet concentration decreases due to droplet deactivation and sedimentation.
-
Meanwhile, haze number concentration increases due to continuously aerosol injection and droplet deactivation.
-
s increases with the decrease in the sink of water vapor due to fewer cloud droplets and more haze particles, and when s>scrit, another cycle starts. In contrast, s approaches 0 when using the CLCCN scheme (black line in the third row of Fig. 6), suggesting that droplet activation is strongly suppressed in the bulk region.
Additionally, σs decreases with nin and approaches 0 due to the buffering effect of cloud droplets under polluted conditions (Fig. 6, fourth row). This suggests that droplet activation is controlled by the mean supersaturation instead of supersaturation fluctuation. This is why even though turbulence is not considered, the box model (Fig. 9) and the theoretical model (developed in Shaw et al., 2023) can still predict the scaling relationships in fast and slow microphysics regimes that are consistent with large-eddy simulations (Fig. 1) and Pi chamber experiments (Fig. 7 in Shaw et al., 2023). The nice performance of the theoretical model and the box model suggests that turbulence is not the direct factor in generating various microphysics regimes, including provoking cloud oscillation. As long as the cloud is well mixed (due to turbulence), various microphysics regimes (e.g., slow, fast, oscillation) can occur under different aerosol injection rates (for monodisperse aerosols like in this study).
It is interesting to see that droplet deactivation can still occur even though s is always positive in the box model (Fig. 11). This is also likely to be true in LESs when using the CLHaze scheme, in which s oscillates around scrit and σs≪scrit. One question is what drives droplet deactivation in a supersaturated environment. Here, droplet deactivation occurs due to the curvature effect: although haze particles can be activated to droplets when s>scrit, the subsequent decrease in s (like s oscillation in our case) can lead to droplet deactivation when s is smaller than the saturated saturation ratio over small cloud droplets (see green line in Fig. 1 of Nenes et al., 2001). Droplet deactivation in supersaturated conditions occurs when the phase relaxation time is much shorter than the droplet activation time. This phenomenon is closely relevant to the onset of the catastrophe discussed in Arabas and Shima (2017). In addition, there is one difference in handling droplet deactivation between the CLCCN and the CLHaze schemes. If droplets are deactivated, they go back to the dry aerosol category when using the CLCCN scheme. When using the CLHaze scheme, droplets stay as haze particles which can still consume water vapor and contribute to liquid water content. The latter has feedback in s which is critical to trigger cloud oscillation that we will discuss next.
When s<scrit, droplet activation is suppressed in the bulk region. This is true for both CLHaze and CLCCN schemes. However, when using the CLHaze scheme, the contribution of haze water content to the total liquid water content increases under this condition (s<scrit) due to continuous aerosol injection and droplet deactivation (as discussed above). The sink of water vapor via condensational growth decreases due to the decrease in cloud droplet concentration, which can lead to an increase in s, considering that the source of water vapor from chamber surfaces is constant. When s>scrit, droplet activation is active again. In contrast, when using the CLCCN scheme, haze water content is not considered, and cloud droplet content is equivalent to liquid water content. In addition, both s and σs are buffered to approach 0 under polluted conditions, and there is no restoring force to increase s.
3.2.4 Cloud collapse
For the simulations above, the side walls are set to be saturated with respect to water. In reality, the side walls in the Pi chamber could be subsaturated, which could enhance droplet deactivation. To investigate the impact of side wall humidity (RHwall) on cloud oscillation, we set RHwall to be 90 %, 70 %, 50 %, and 30 % for nin=20 cm−3 s−1. This is similar to the entrainment of subsaturated air into a natural cloud. Figure 12 shows the time series of domain-averaged ql, Nd, Na or Nh, NT, and rd, while Fig. 13 shows the corresponding Ract, Rdeact, s, σs, and P (as Figs. 5 and 6). Results indicate that ql decreases with RHwall (Fig. 12 first row). This is because subsaturated side walls serve as a water sink to evaporate droplets and thus enhance haze–cloud interactions. Note that qh can be as large as qc (e.g., for RHwall of 30 % at the end of the simulation), which cannot be captured when using the CLCCN scheme. The cloud always reaches a steady state when using the CLCCN scheme. In contrast, when using the CLHaze scheme, the cloud oscillates for RHwall of 90 and 70 %, but it can reach a steady state for RHwall of 50 %, and more interestingly, it tends to collapse for RHwall of 30 % (Fig. 12 second row). Here we define “cloud collapse” as the significant decrease in Nd at low RHwall conditions. It is also clear to see that the bulk s is negative in the cloud collapse regime (fourth row in Fig. 13). Note that the cloud does not dissipate completely because qc still reaches a steady state, probably due to droplet activation near the top and bottom surfaces where the local s can be still larger than scrit (similar to the high s observed near the surface in Wang et al. (2024a).
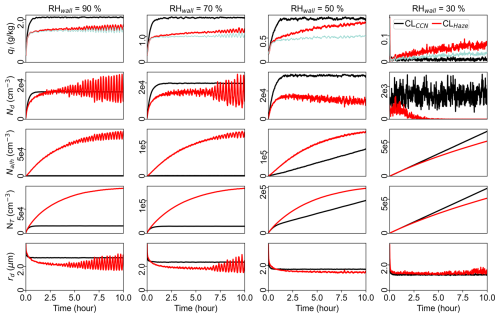
Figure 12Time series of domain-averaged ql (first row), Nd (second row), Na or Nh (third row), NT (fourth row), and rd (fifth row) at a nin of 20 cm−3 s−1 with four different size wall relative humidity levels, RHwall = 90 %, 70 %, 50 %, and 30 %. The light-blue line in the first row represents the cloud water mixing ratio (qc) when using the CLHaze scheme.
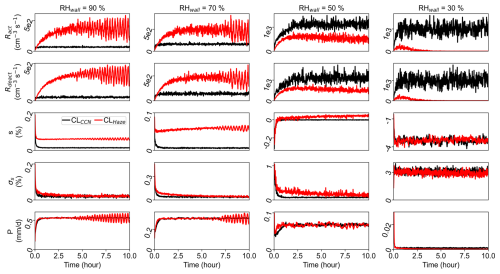
Figure 13Same cases in Fig. 12 but showing time series of domain-averaged Ract (first row), Rdeact (second row), s (third row), σs (fourth row), and P (fifth row) at a nin of 20 cm−3 s−1 with four different size wall relative humidity levels, RHwall = 90 %, 70 %, 50 %, and 30 %.
Our results suggest that cloud oscillation and cloud collapse result from haze–cloud interactions in a homogeneous and inhomogeneous supersaturation field, respectively. When the side walls are close to be saturated, the supersaturation field is almost homogeneous everywhere in the chamber except very close to the top and bottom surfaces. Such a homogeneous supersaturation field allows synchronized droplet activation or deactivation to occur throughout the entire chamber and thus leads to cloud oscillation as explained above and Fig. 8. However, when the side walls are considerably drier, the supersaturation field in the chamber is not homogeneous: air close to the side wall is subsaturated while air close to the center, top, and bottom surfaces is supersaturated. Such an inhomogeneous field causes droplet activation in one region and deactivation in another region. For a moderate dry side wall (i.e., RHwall of 50 %), a steady state might be reached if the net activation rate is balanced by the droplet sedimentation rate. For an extremely dry side wall (i.e., RHwall of 30 %), the chamber can be considered a machine to efficiently transfer cloud droplets to haze particles over time, leading to the cloud collapse.
3.2.5 Haze-only regime
So far, our results show that besides slow and fast microphysics regimes, there exists a cloud oscillation regime at a high aerosol injection rate due to haze–cloud interactions. In the oscillation regime, the oscillation frequency decreases and the haze number concentration increases as nin increases. It raises a question of what would happen if nin is extremely large. Would there be another regime in which there are only haze particles and no cloud droplets? Here, we develop a simple model to investigate the properties of a postulated haze-only regime.
Let us assume only haze particles exist in the chamber at an extremely high aerosol injection rate. Following the approach of Shaw et al. (2023) (Eqs. 56 and 57 therein), in the steady state, the mean air temperature would be higher than the reference temperature (i.e., T0, same as in our Eq. 1) due to latent heat release from the formation of haze particles,
Similarly, qv would be smaller than the reference water vapor mixing ratio (i.e., qv0, same as in our Eq. 2) due to water uptake by haze particles,
in Eqs. (7) and (8) indicates that only the contribution via diffusional growth is considered here. In the haze-only regime, condensation is dominated by the formation of haze particles,
Here req is the equilibrium haze particle radius at a given s<scrit, which depends on the environmental fractional relative humidity (RH ) and on properties of the substance. We assume that particles reach their equilibrium size within a very short time. req can be expressed as a function of RH for values near but smaller than unity based on Eq. (10) of Lewis (2019), where the constants are those for sodium chloride:
This expression is accurate to within 5 % for values of RH between 99 % and 100 % for dry aerosol radius (rdry) larger than 10 nm. A similar expression of req is also derived by Khvorostyanov and Curry (2007) (Eq. 16 therein).
Meanwhile, a steady-state haze-only system requires that the formation of haze particles through injection is balanced by their loss due to sedimentation,
Here Nh is the haze number concentration and τsed is the characteristic sedimentation time of haze particles with a radius of req (see Eq. 6).
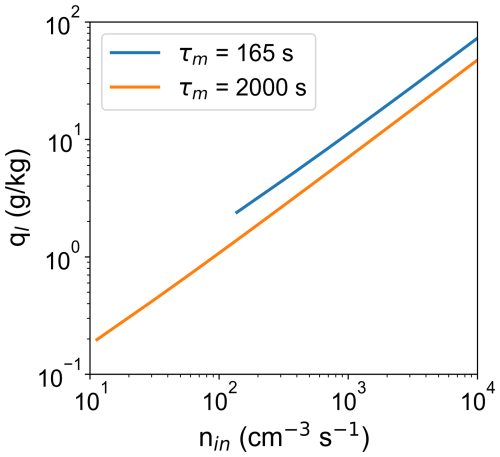
Figure 14Change of equilibrium liquid water mixing ratio with nin in the haze-only regime. Results are calculated numerically based on Eqs. (7) to (11), with T0=290 K and qv0=13.9 g kg−1. Blue and orange lines are for τm=165 and 2000 s, respectively. The left ends of the two lines are determined at RH =100 %.
For a given forcing (T0, qv0, and τm) and aerosol (rdry) condition, we can calculate the equilibrium liquid water mixing ratio at the haze-only steady state by solving Eqs. (7)–(11) numerically. For a direct comparison with the above results, we set T0=290 K, qv0=13.9 g kg−1, and τm=165 s, the same as those used in the box model. Figure 14 shows that ql increases with nin linearly in log–log space with a slope of about 0.83, which is steeper than that in the fast microphysics regime (0.67). Note that we only simulate the haze-only regime in the subsaturated environment here (i.e., RH <100 %; the left ends of the two lines in Fig. 14 are determined at RH =100 %), and the slope should be related to the RH dependence of req (Eq. 10). Results show that the required nin to reach this haze-only regime is extremely high, hundreds to thousands of cubic centimeters per second (cm−3 s−1), and ql is also exceptionally high, tens to hundreds of grams per kilogram (g kg−1). The main reason for the high nin and ql is that a huge number of slowly sedimenting haze particles are needed to balance the relatively strong forcing term to replenish water vapor so that s<0 all the time. Such high ql is likely unrealistic and unachievable in the real chamber due to factors not considered in the model (see the following section). However, if τm=2000 s, implying a much weaker forcing, ql in the haze-only regime can be less than 1 g kg−1 for a more realistic nin (Fig. 14).
So far, we have demonstrated the existence of the haze-only microphysics regime in an idealized scenario. One question is whether the haze-only regime is stable. We expect that the steady state in the haze-only regime is stable for a given nin. This is because the aerosol injection rate should be equal to the sedimentation rate of haze particles in the steady state (see Eq. 11). If there is a positive (or negative) perturbation of Nh, the sedimentation rate would increase (or decrease), leading to a net decreasing (or increasing) tendency in Nh for a given nin. This feedback is trying to bring Nh back to its steady-state value. Of course, this is only our conjecture, not a formal proof. Further efforts are needed to understand the onset of oscillation, the transition between the oscillation regime and haze-only regime, and the stability of the haze-only regime.
3.2.6 Impact of a haze sink
So far, the only sink for aerosol particles is activation. At high aerosol injection rates, activation is suppressed, and thus, they can accumulate when using the CLCCN scheme (see black lines in Figs. 5 and 12, third row). Similarly, the sink for haze particles is dominated by activation because their sedimentation speed is very small. We have shown that a chamber with subsaturated side walls can efficiently transfer cloud droplets to haze particles over time, leading to haze accumulation when using the CLHaze scheme (red line in Fig. 12, third row). In reality, these unactivated particles (aerosols or haze particles) can also be lost by side walls, coagulation, sedimentation, or droplet scavenging, preventing their concentration from approaching infinity.
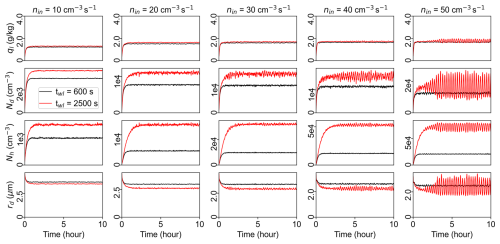
Figure 15Similar to Fig. 5, time series of domain-averaged ql (first row), Nd (second row), Nh (third row), and rd (fourth row) for five different nin, 10, 20, 30, 40, and 50 cm−3 s−1, but considering the loss of haze particles due to the side wall. Different line colors represent simulations using different wall-loss timescales (twl in Eq. 12): twl=600 s (black) and 2500 s (red).
To investigate the impact of the sink of haze particles on cloud properties, especially in the cloud oscillation regime, following Thomas et al. (2023) and Wang et al. (2024c) (Eq. 1 therein), a wall-loss timescale (twl) is applied to constrain Nh when using the CLHaze scheme as
Here Δt is the time step of the simulation, and δNh is the loss of haze particles due to walls after each time step. twl is 1 over the particle rate loss coefficient (β) due to the walls. β can be estimated from the deposition velocity (vdep) and the ratio of the wall area (A) to volume (V) (for the Pi chamber m−1): . For simplification (i.e., neglecting the impact of other factors, such as particle size and turbulence, on vdep), we set m s−1, a typical value for the deposition velocity for particles with a diameter of 2 µm (see Fig. 4 in Lai, 2002), which give us s−1 or twl=2500 s. Results show that oscillation still exists for nin≥ 20 cm−3 s−1 but with a smaller amplitude (red line in Fig. 15). The oscillation frequency is also higher than before (compare Figs. 5 and 15 for the same nin). Although we only consider the loss of haze particles due to walls here, there are some other types of haze sinks, such as Brownian coagulation (Baker and Charlson, 1990) and scavenging (Sellegri et al., 2003), which might lead to a smaller effective twl in the real chamber. For another sensitivity test, we set twl=600 s, the same value Thomas et al. (2019) used to constrain particle concentration for the Pi chamber simulation. Results show that the oscillation is barely seen (black line in Fig. 15). Also note that Nh increases with nin, but its value is 1 order of magnitude smaller than before (Fig. 15 vs. 5, third row). Combined with the “cloud collapse” findings, our results suggest that achieving a high concentration of haze particles and synchronized activation throughout the chamber are two key factors for the cloud to stay in the oscillation regime.
In this study, we conducted a series of large-eddy simulations of the Pi chamber using a haze-capable bin microphysics scheme (CLHaze) developed by Yang et al. (2023) to explore haze–cloud interactions over a wide range of aerosol injection rates (0.001 cm−3 s cm−3 s−1). Results are compared with simulations using a CCN-based bin microphysics scheme (CLCCN). The CLCCN scheme adopts a Twomey-type activation parameterization, which is widely used in atmospheric cloud simulations, while the CLHaze scheme can properly resolve the growth of haze particles and the activation process. Our objectives were to investigate (1) the influence of different aerosol injection rates on cloud properties and (2) the importance of haze–cloud interactions in a convection cloud chamber as well as in analogous natural cloud systems. For objective 1, we especially focused on the impact of nin on cloud droplet number concentration (Nd), liquid water mixing ratio (ql), and droplet size distribution and compared results with previous analytical studies (Krueger, 2020; Shaw et al., 2023). Objective 2 is motivated by Yang et al. (2023), showing that cloud microphysical properties gained with the CLCCN scheme are similar to those using the CLHaze scheme, raising the question of whether we need to consider haze–cloud interactions. However, only two aerosol injection rates were investigated in Yang et al. (2023). Here, we explored the consistency of the CLCCN scheme and the CLHaze scheme over a wider range of aerosol injection rates. Low-dimensional models are also employed to explore the impact of nin on cloud properties. In short, we confirm slow and fast microphysics regimes reported in previous studies (Shaw et al., 2023). We also find new microphysical regimes at high aerosol injection rates, cloud oscillation, and haze only, as illustrated in Fig. 16.
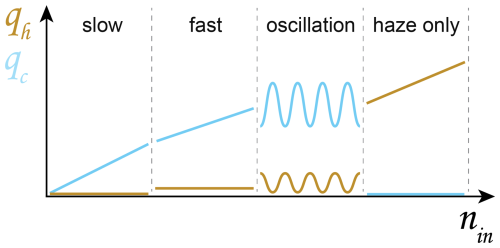
Figure 16A schematic illustration of qc or qh and nin relationships in different microphysics regimes: slow, fast, oscillation, and haze only.
Slow and fast microphysics regimes were observed at small and moderate aerosol injection rates, respectively. The change of cloud properties with aerosol injection rate in these two regimes agreed with previous analytical studies (Chandrakar et al., 2020; Shaw et al., 2023). Specifically, for small aerosol injection rates (nin<0.1 cm−3 s−1), the cloud was in the slow microphysics regime where droplets grow at a high supersaturation before they fall out, leading to a linear relationship between Nd and nin as well as ql and nin. For moderate aerosol injection rates (0.1 cm−3 s cm−3 s−1), the cloud was in the fast microphysics regime with and , consistent with the theoretical prediction in Shaw et al. (2023). In addition, droplet size distributions in the steady state became narrower and shifted to smaller sizes due to the increase in nin, and the shape of the distribution also agreed reasonably well with analytical estimates (Chandrakar et al., 2020; Liu and Hallett, 1998; Krueger, 2020). But those analytical estimates do not capture the distribution properties at large nin where haze mode is present.
The most striking phenomena are cloud oscillation, cloud collapse, and haze-only regimes that occur at high aerosol injection rates when using the CLHaze scheme. In contrast, cloud always reaches a steady state when using the CLCCN scheme. Haze–cloud interactions are responsible for the occurrence of these microphysics regimes. Specifically, in the cloud oscillation regime, s oscillates around scrit and σs≪scrit. Under this condition, the cloud system is buffered by a huge number of haze particles and cloud droplets. Droplet activation is controlled by the mean supersaturation rather than supersaturation fluctuation. Droplet deactivation can still occur in a supersaturated environment () due to the curvature effect. The oscillation of s around scrit leads to the oscillation of droplet activation and deactivation and further causes the oscillation of cloud properties. In a chamber with relatively humid side walls, the supersaturation is more homogeneous in the chamber, and droplets at different locations experience similar supersaturation, leading to synchronized activation (s>scrit) of a huge number of droplets across the whole chamber – the main reason for cloud oscillation. In contrast, cloud collapse occurs when the side walls are relatively dry. Under this condition, supersaturation in the chamber is more inhomogeneous: droplets close to the side walls tend to be deactivated to haze particles, while droplets away from the side walls tend to grow. The separation of droplet activation (in regions near the center, top, and bottom surfaces) and deactivation (in regions near the side walls) make the chamber an efficient machine to transfer cloud droplets to haze particles – the fundamental reason for cloud collapse. The haze-only regime occurred at extremely high aerosol injection rates. In this regime, s is much smaller than scrit, and it can be negative (corresponding to RH <100 %). Droplet activation is suppressed, and the formation of haze particles is balanced by their loss due to sedimentation.
In the real chamber, haze particles can also be removed through other mechanisms, such as wall loss and scavenging, which could constrain the haze number concentration. Therefore, clouds might struggle to achieve oscillation and haze-only regimes, especially when the source term to maintain high supersaturation is strong, e.g., a large temperature difference between top and bottom surfaces, like in this study. Haze–cloud oscillation is more likely to occur under conditions of weak supersaturation forcing, e.g., a small temperature difference between top and bottom surfaces in a convection chamber or small updraft velocity in the real atmosphere. Recently, Gutiérrez et al. (2024) solved coupled equations for droplet growth and supersaturation development in a rising cloud parcel. Their analysis also predicts the oscillation between haze and cloud droplets under certain conditions, e.g., low air vertical velocity and high aerosol number concentration. The fundamental reason for cloud oscillation stems from the nonlinear interactions in the coupled haze–cloud–supersaturation system (Arabas and Shima, 2017). Such a system is analogous to other predator–prey systems observed in nature, which causes similar oscillation behaviors, such as oscillation in open-cellular convection or in aerosol–cloud–precipitation system (Koren and Feingold, 2011). However, it should be mentioned that cloud oscillation reported in Gutiérrez et al. (2024) is not the same as oscillation reported in this study: they only have one size of droplet/haze that varies in time, while we have coexisting haze and cloud droplets.
Our results suggest that haze–cloud interactions are very important when air supersaturation is close to the critical supersaturation of aerosols. This condition happens in the Pi chamber at high aerosol injection rates, as shown in this study, and it can also occur in the atmosphere, for example, when cloud or fog is close to the source of intense natural and anthropogenic aerosol emissions. Studies have shown the possibility of fog consisting of just unactivated haze particles in a highly polluted environment (e.g., Klemm and Lin, 2016). The unactivated haze particles can significantly impact fog optical properties, such as visibility and radiation (Boutle et al., 2018), as well as cloud optical properties, i.e., cloud albedo (Hoffmann et al., 2022). Proper simulation of haze–cloud interactions requires resolving haze particles as well as the associated droplet activation and deactivation processes, rather than relying on Twomey-type activation parameterization. Also note that monodisperse aerosol with a dry radius of 62.5 nm is used in this study. We expect haze–cloud interaction might be more important for larger aerosol particles because their critical supersaturation gets smaller, their equilibrium wet radius gets larger, and the activation/deactivation timescale could get longer (Hoffmann, 2016). In addition, aerosol particles in nature vary in size and composition, and haze–cloud interactions might be more important for polydisperse aerosols (see Fig. 5 in Richter et al., 2021). Furthermore, unlike well-controlled environmental conditions in the cloud chamber, the boundary conditions of natural clouds or fogs change over time, which would also affect the microphysical regimes. The impact of haze–cloud interactions under real cloud conditions is worth exploring in the future.
The SAM model was kindly provided by Marat Khairoutdinov of Stony Brook University and is publicly available at http://rossby.msrc.sunysb.edu/SAM.html (Khairoutdinov, 2003). Data and Python code for figure generation are available at https://doi.org/10.5281/zenodo.14002522 (Yang and Hou, 2024).
FY, RAS, and FH: conceptualization. FY, HFS, and AW: code development and debug. FY and HFS: conducting simulations. FY and PH: data analysis and visualization. FY: original draft preparation. HFS, RAS, FH, PH, AW, and MO: writing (review and editing).
The contact author has declared that none of the authors has any competing interests.
Publisher's note: Copernicus Publications remains neutral with regard to jurisdictional claims made in the text, published maps, institutional affiliations, or any other geographical representation in this paper. While Copernicus Publications makes every effort to include appropriate place names, the final responsibility lies with the authors.
We thank Shin-ichiro Shima and the other reviewer for their valuable comments and suggestions. We also thank the editor Timothy Garrett for handling our paper and providing helpful comments. This work was supported by the Office of Science Biological and Environmental Research program as part of the Atmospheric Systems Research program. Brookhaven National Laboratory is operated by Battelle for the U.S. Department of Energy under contract DE-SC0012704. PNNL is operated for the Department of Energy by Battelle Memorial Institute under contract DE-AC05-76 RL01830. Hamed Fahandezh Sadi and Raymond A. Shaw were supported by NSF grant AGS-2133229. Fabian Hoffmann is supported by the German Research Foundation (DFG) under grant HO 6588/1-1. We thank Subin Thomas, who was involved with early large-eddy simulations that hinted at the possibility of cloud oscillations. We thank Ernie Lewis for helpful discussions that made us aware of Eq. (10). Fan Yang also thanks Kamal Kant Chandrakar and Silvio Schmalfuß for helpful discussions.
This work was supported by the Office of Science Biological and Environmental Research program as part of the Atmospheric Systems Research program. Brookhaven National Laboratory is operated by Battelle for the U.S. Department of Energy (contract no. DE-SC0012704). PNNL is operated for the Department of Energy by Battelle Memorial Institute (contract no. DE-AC05-76 RL01830). Hamed Fahandezh Sadi and Raymond A. Shaw were supported by NSF (grant no. AGS-2133229). Fabian Hoffmann is supported by the German Research Foundation (DFG) (grant no. HO 6588/1-1).
This paper was edited by Timothy Garrett and reviewed by Shin-ichiro Shima and one anonymous referee.
Anderson, J. C., Beeler, P., Ovchinnikov, M., Cantrell, W., Krueger, S., Shaw, R. A., Yang, F., and Fierce, L.: Enhancements in cloud condensation nuclei activity from turbulent fluctuations in supersaturation, Geophys. Res. Lett., 50, e2022GL102635, https://doi.org/10.1029/2022GL102635, 2023. a
Arabas, S. and Shima, S.: On the CCN (de)activation nonlinearities, Nonlin. Processes Geophys., 24, 535–542, https://doi.org/10.5194/npg-24-535-2017, 2017. a, b
Baker, M. B. and Charlson, R. J.: Bistability of CCN concentrations and thermodynamics in the cloud-topped boundary layer, Nature, 345, 142–145, https://doi.org/10.1038/345142a0, 1990. a
Boutle, I., Price, J., Kudzotsa, I., Kokkola, H., and Romakkaniemi, S.: Aerosol–fog interaction and the transition to well-mixed radiation fog, Atmos. Chem. Phys., 18, 7827–7840, https://doi.org/10.5194/acp-18-7827-2018, 2018. a
Brown, P. N., Byrne, G. D., and Hindmarsh, A. C.: VODE: A variable-coefficient ODE solver, SIAM journal on scientific and statistical computing, 10, 1038–1051, https://doi.org/10.1137/0910062, 1989. a
Chandrakar, K. K., Cantrell, W., Chang, K., Ciochetto, D., Niedermeier, D., Ovchinnikov, M., Shaw, R. A., and Yang, F.: Aerosol indirect effect from turbulence-induced broadening of cloud-droplet size distributions, P. Natl. Acad. Sci. USA, 113, 14243–14248, https://doi.org/10.1073/pnas.1612686113, 2016. a, b, c
Chandrakar, K. K., Saito, I., Yang, F., Cantrell, W., Gotoh, T., and Shaw, R. A.: Droplet size distributions in turbulent clouds: Experimental evaluation of theoretical distributions, Q. J. Roy. Meteor. Soc., 146, 483–504, https://doi.org/10.1002/qj.3692, 2020. a, b, c, d, e, f, g, h
Chang, K., Bench, J., Brege, M., Cantrell, W., Chandrakar, K., Ciochetto, D., Mazzoleni, C., Mazzoleni, L., Niedermeier, D., and Shaw, R.: A laboratory facility to study gas–aerosol–cloud interactions in a turbulent environment: The π chamber, B. Am. Meteorol. Soc., 97, 2343–2358, https://doi.org/10.1175/BAMS-D-15-00203.1, 2016. a
Chen, J., Liu, Y., Zhang, M., and Peng, Y.: New understanding and quantification of the regime dependence of aerosol-cloud interaction for studying aerosol indirect effects, Geophys. Res. Lett., 43, 1780–1787, https://doi.org/10.1002/2016GL067683, 2016. a
Chen, J.-P. and Lamb, D.: Simulation of cloud microphysical and chemical processes using a multicomponent framework. Part I: Description of the microphysical model, J. Atmos. Sci., 51, 2613–2630, https://doi.org/10.1175/1520-0469(1994)051<2613:SOCMAC>2.0.CO;2, 1994. a
Grabowski, W. W.: Comparison of Eulerian bin and Lagrangian particle-based schemes in simulations of Pi Chamber dynamics and microphysics, J. Atmos. Sci., 77, 1151–1165, https://doi.org/10.1175/JAS-D-19-0216.1, 2020. a
Grabowski, W. W., Morrison, H., Shima, S.-I., Abade, G. C., Dziekan, P., and Pawlowska, H.: Modeling of cloud microphysics: Can we do better?, B. Am. Meteorol. Soc., 100, 655–672, https://doi.org/10.1175/BAMS-D-18-0005.1, 2019. a
Gutiérrez, M. S., Chekroun, M. D., and Koren, I.: Dynamical regimes of CCN activation in adiabatic air parcels, arxiv [preprint], https://doi.org/10.48550/arXiv.2405.11545, 2024. a, b
Hoffmann, F.: The effect of spurious cloud edge supersaturations in Lagrangian cloud models: An analytical and numerical study, Mon. Weather Rev., 144, 107–118, https://doi.org/10.1175/MWR-D-15-0234.1, 2016. a
Hoffmann, F., Raasch, S., and Noh, Y.: Entrainment of aerosols and their activation in a shallow cumulus cloud studied with a coupled LCM–LES approach, Atmos. Res., 156, 43–57, https://doi.org/10.1016/j.atmosres.2014.12.008, 2015. a
Hoffmann, F., Mayer, B., and Feingold, G.: A parameterization of interstitial aerosol extinction and its application to marine cloud brightening, J. Atmos. Sci., 79, 2849–2862, https://doi.org/10.1175/JAS-D-22-0047.1, 2022. a
Jensen, J. B. and Nugent, A. D.: Condensational growth of drops formed on giant sea-salt aerosol particles, J. Atmos. Sci., 74, 679–697, https://doi.org/10.1175/JAS-D-15-0370.1, 2017. a
Khairoutdinov, M. F.: System for Atmospheric Modeling (SAM), Stony Brook University [code], http://rossby.msrc.sunysb.edu/SAM.html (last access: 19 March 2024), 2003. a
Khairoutdinov, M. F. and Randall, D. A.: Cloud resolving modeling of the ARM summer 1997 IOP: Model formulation, results, uncertainties, and sensitivities, J. Atmos. Sci., 60, 607–625, https://doi.org/10.1175/1520-0469(2003)060<0607:CRMOTA>2.0.CO;2, 2003. a
Khvorostyanov, V. I. and Curry, J. A.: Refinements to the Köhler's theory of aerosol equilibrium radii, size spectra, and droplet activation: Effects of humidity and insoluble fraction, J. Geophys. Res.-Atmos., 112, D5206, https://doi.org/10.1029/2006JD007672, 2007. a
Klemm, O. and Lin, N. H.: What causes observed fog trends: air quality or climate change?, Aerosol Air Qual. Res., 16, 1131–1142, https://doi.org/10.4209/aaqr.2015.05.0353, 2016. a
Koren, I. and Feingold, G.: Aerosol–cloud–precipitation system as a predator-prey problem, P. Natl. Acad. Sci. USA, 108, 12227–12232, https://doi.org/10.1073/pnas.1101777108, 2011. a
Korolev, A. V.: The influence of supersaturation fluctuations on droplet size spectra formation, J. Atmos. Sci., 52, 3620–3634, https://doi.org/10.1175/1520-0469(1995)052<3620:TIOSFO>2.0.CO;2, 1995. a
Korolev, A. V. and Mazin, I. P.: Supersaturation of water vapor in clouds, J. Atmos. Sci., 60, 2957–2974, https://doi.org/10.1175/1520-0469(2003)060<2957:SOWVIC>2.0.CO;2, 2003. a
Krueger, S. K.: Technical note: Equilibrium droplet size distributions in a turbulent cloud chamber with uniform supersaturation, Atmos. Chem. Phys., 20, 7895–7909, https://doi.org/10.5194/acp-20-7895-2020, 2020. a, b, c, d, e, f
Lai, A.: Particle deposition indoors: a review., Indoor air, 12, 211–214, https://doi.org/10.1034/j.1600-0668.2002.01159.x, 2002. a
Lehmann, K., Siebert, H., and Shaw, R. A.: Homogeneous and inhomogeneous mixing in cumulus clouds: Dependence on local turbulence structure, J. Atmos. Sci., 66, 3641–3659, https://doi.org/10.1175/2009JAS3012.1, 2009. a
Lewis, E. R.: The dependence of radius on relative humidity and solute mass at high relative humidities up to and including 100%, J. Geophys. Res.-Atmos., 124, 2105–2126, https://doi.org/10.1029/2018JD030008, 2019. a
Liu, Y. and Hallett, J.: On size distributions of cloud droplets growing by condensation: A new conceptual model, J. Atmos. Sci., 55, 527–536, https://doi.org/10.1175/1520-0469(1998)055<0527:OSDOCD>2.0.CO;2, 1998. a, b
MacMillan, T., Shaw, R. A., Cantrell, W. H., and Richter, D. H.: Direct numerical simulation of turbulence and microphysics in the Pi Chamber, Phys. Rev. Fluids, 7, 020501, https://doi.org/10.1103/PhysRevFluids.7.020501, 2022. a
McGraw, R. and Liu, Y.: Brownian drift-diffusion model for evolution of droplet size distributions in turbulent clouds, Geophys. Res. Lett., 33, L03802, https://doi.org/10.1029/2005GL023545, 2006. a
Monin, A. and Obukhov, A.: Basic laws of turbulent mixing in the atmosphere near the ground, Tr. Geofiz. Inst., Akad. Nauk SSSR, 24, 163–187, 1954. a
Morrison, H., Witte, M., Bryan, G. H., Harrington, J. Y., and Lebo, Z. J.: Broadening of modeled cloud droplet spectra using bin microphysics in an Eulerian spatial domain, J. Atmos. Sci., 75, 4005–4030, https://doi.org/10.1175/JAS-D-18-0055.1, 2018. a
Morrison, H., van Lier-Walqui, M., Fridlind, A. M., Grabowski, W. W., Harrington, J. Y., Hoose, C., Korolev, A., Kumjian, M. R., Milbrandt, J. A., Pawlowska, H., Posselt, D. J., Prat, O. P., Reimel, K. J., Shima, S. I., Diedenhoven, B., and Xue, L.: Confronting the challenge of modeling cloud and precipitation microphysics, J. Adv. Model. Earth Sy., 12, e2019MS001689, https://doi.org/10.1029/2019MS001689, 2020. a
Nenes, A., Ghan, S., Abdul-Razzak, H., Chuang, P. Y., and Seinfeld, J. H.: Kinetic limitations on cloud droplet formation and impact on cloud albedo, Tellus B, 53, 133–149, https://doi.org/10.3402/tellusb.v53i2.16569, 2001. a
Prabhakaran, P., Shawon, A. S. M., Kinney, G., Thomas, S., Cantrell, W., and Shaw, R. A.: The role of turbulent fluctuations in aerosol activation and cloud formation, P. Natl. Acad. Sci. USA, 117, 16831–16838, https://doi.org/10.1073/pnas.2006426117, 2020. a, b, c, d, e
Prabhakaran, P., Thomas, S., Cantrell, W., Shaw, R. A., and Yang, F.: Sources of stochasticity in the growth of cloud droplets: Supersaturation fluctuations versus turbulent transport, J. Atmos. Sci., 79, 3145–3162, https://doi.org/10.1175/JAS-D-22-0051.1, 2022. a
Richter, D. H., MacMillan, T., and Wainwright, C.: A Lagrangian cloud model for the study of marine fog, Bound.-Lay. Meteorol., 181, 523–542, https://doi.org/10.1007/s10546-020-00595-w, 2021. a
Rogers, R. R. and Yau, M. K.: A short course in cloud physics, 3rd edn., Burlington-Heinemann, MA, USA, ISBN 978-0750632157, 1989. a
Saito, I., Gotoh, T., and Watanabe, T.: Broadening of cloud droplet size distributions by condensation in turbulence, J. Meteorol. Soc. Jpn. Ser. II, 97, 867–891, https://doi.org/10.2151/jmsj.2019-049, 2019. a
Sellegri, K., Laj, P., Dupuy, R., Legrand, M., Preunkert, S., and Putaud, J.-P.: Size-dependent scavenging efficiencies of multicomponent atmospheric aerosols in clouds, J. Geophys. Res.-Atmos., 108, 4334, https://doi.org/10.1029/2002JD002749, 2003. a
Shaw, R. A., Thomas, S., Prabhakaran, P., Cantrell, W., Ovchinnikov, M., and Yang, F.: Fast and slow microphysics regimes in a minimalist model of cloudy Rayleigh-Bénard convection, Phys. Rev. Res., 5, 043018, https://doi.org/10.1103/PhysRevResearch.5.043018, 2023. a, b, c, d, e, f, g, h, i, j, k, l, m, n, o, p, q, r
Shima, S.., Kusano, K., Kawano, A., Sugiyama, T., and Kawahara, S.: The super-droplet method for the numerical simulation of clouds and precipitation: A particle-based and probabilistic microphysics model coupled with a non-hydrostatic model, Q. J. Roy. Meteor. Soc., 135, 1307–1320, https://doi.org/10.1002/qj.441, 2009. a
Thomas, S., Ovchinnikov, M., Yang, F., van der Voort, D., Cantrell, W., Krueger, S. K., and Shaw, R. A.: Scaling of an atmospheric model to simulate turbulence and cloud microphysics in the Pi Chamber, J. Adv. Model. Earth Sy., 11, 1981–1994, https://doi.org/10.1029/2019MS001670, 2019. a, b, c, d, e, f
Thomas, S., Yang, F., Ovchinnikov, M., Cantrell, W., and Shaw, R. A.: Scaling of turbulence and microphysics in a convection–cloud chamber of varying height, J. Adv. Model. Earth Sy., 15, e2022MS003304, https://doi.org/10.1029/2022MS003304, 2023. a, b
Twomey, S.: The nuclei of natural cloud formation part II: The supersaturation in natural clouds and the variation of cloud droplet concentration, Geofisica pura e applicata, 43, 243–249, https://doi.org/10.1007/BF01993560, 1959. a, b
Wang, A., Krueger, S., Chen, S., Ovchinnikov, M., Cantrell, W., and Shaw, R. A.: Glaciation of mixed-phase clouds: insights from bulk model and bin-microphysics large-eddy simulation informed by laboratory experiment, Atmos. Chem. Phys., 24, 10245–10260, https://doi.org/10.5194/acp-24-10245-2024, 2024a. a, b, c
Wang, A., Ovchinnikov, M., Yang, F., Cantrell, W., Yeom, J., and Shaw, R. A.: The Dual Nature of Entrainment-Mixing Signatures Revealed Through Large-Eddy Simulations of a Convection-Cloud Chamber, J. Atmos. Sci., https://doi.org/10.1175/JAS-D-24-0043.1, 2024b. a, b
Wang, A., Ovchinnikov, M., Yang, F., Shaw, R. A., and Schmalfuss, S.: Designing a convective cloud chamber for collision coalescence using atmospheric large-eddy simulation with bin microphysics scheme, J. Adv. Model. Earth Sy., 16, e2023MS003734, https://doi.org/10.1029/2023MS003734, 2024c. a, b, c, d, e, f, g
Wang, A., Yang, X. I. A., and Ovchinnikov, M.: An investigation of LES wall modeling for Rayleigh-Bénard convection via interpretable and physics-aware feedforward neural networks with DNS, J. Atmos. Sci., 81, 435–458, https://doi.org/10.1175/JAS-D-23-0094.1, 2024d. a
Xue, H. and Feingold, G.: A modeling study of the effect of nitric acid on cloud properties, J. Geophys. Res.-Atmos., 109, D18204, https://doi.org/10.1029/2004JD004750, 2004. a
Yang, F. and Hou, P.: Data and Code used in “Microphysics regimes due to haze-cloud interactions: cloud oscillation and cloud collapse”, Zenodo [code and data set], https://doi.org/10.5281/zenodo.14002523, 2024. a
Yang, F., Shaw, R., and Xue, H.: Conditions for super-adiabatic droplet growth after entrainment mixing, Atmos. Chem. Phys., 16, 9421–9433, https://doi.org/10.5194/acp-16-9421-2016, 2016. a
Yang, F., Kollias, P., Shaw, R. A., and Vogelmann, A. M.: Cloud droplet size distribution broadening during diffusional growth: ripening amplified by deactivation and reactivation, Atmos. Chem. Phys., 18, 7313–7328, https://doi.org/10.5194/acp-18-7313-2018, 2018. a
Yang, F., Ovchinnikov, M., Thomas, S., Khain, A., McGraw, R., Shaw, R. A., and Vogelmann, A. M.: Large-eddy simulations of a convection cloud chamber: Sensitivity to bin microphysics and advection, J. Adv. Model. Earth Sy., 14, e2021MS002895, https://doi.org/10.1029/2021MS002895, 2022. a, b, c
Yang, F., Hoffmann, F., Shaw, R. A., Ovchinnikov, M., and Vogelmann, A. M.: An intercomparison of large-eddy simulations of a convection cloud chamber using haze-capable bin and Lagrangian cloud microphysics schemes, J. Adv. Model. Earth Sy., 15, e2022MS003270, https://doi.org/10.1029/2022MS003270, 2023. a, b, c, d, e, f, g, h, i, j, k, l, m, n, o, p