the Creative Commons Attribution 4.0 License.
the Creative Commons Attribution 4.0 License.
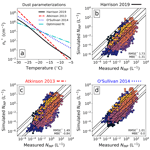
Gaps in our understanding of ice-nucleating particle sources exposed by global simulation of the UK Earth System Model
Alberto Sanchez-Marroquin
Daniel P. Grosvenor
Kirsty J. Pringle
Stephen R. Arnold
Benjamin J. Murray
Kenneth S. Carslaw
Changes in the availability of a subset of aerosol known as ice-nucleating particles (INPs) can substantially alter cloud microphysical and radiative properties. Despite very large spatial and temporal variability in INP properties, many climate models do not currently represent the link between (i) the global distribution of aerosols and INPs and (ii) primary ice production in clouds. Here we use the UK Earth System Model to simulate the global distribution of dust, marine-sourced, and black carbon INPs suitable for immersion-mode freezing of liquid cloud droplets over an annual cycle. The model captures the overall spatial and temporal distribution of measured INP concentrations, which is strongly influenced by the world's major mineral dust source regions. A negative bias in simulated versus measured INP concentrations at higher freezing temperatures points to incorrectly defined INP properties or a missing source of INPs. We find that the ability of the model to reproduce measured INP concentrations is greatly improved by representing dust as a mixture of mineralogical and organic ice-nucleating components, as present in many soils. To improve the agreement further, we define an optimized hypothetical parameterization of dust INP activity (ns(T)) as a function of temperature with a logarithmic slope of −0.175 K−1, which is much shallower than existing parameterizations (e.g. −0.35 K−1 for the K-feldspar data of Harrison et al., 2019). The results point to a globally important role for an organic component associated with mineral dust.
- Article
(13899 KB) - Full-text XML
-
Supplement
(1701 KB) - BibTeX
- EndNote
A subset of atmospheric aerosol particles known as ice-nucleating particles (INPs) have the potential to trigger ice formation when immersed in supercooled water droplets. This process is referred to as immersion-mode heterogeneous ice nucleation and is thought to be the dominant heterogeneous freezing mechanism in mixed-phase clouds (Murray et al., 2012; Burrows et al., 2022). Immersion-mode freezing generally operates between 0 and about −37 °C (Murray et al., 2012), below which homogeneous ice nucleation dominates (Koop and Murray, 2016; Herbert et al., 2015). Such primary ice formation in supercooled clouds is followed by several microphysical processes that can substantially alter the cloud radiative properties as well as the generation of precipitation on regional to global scales (Korolev et al., 2017; Vergara-Temprado et al., 2018b; Hawker et al., 2021; Liu et al., 2012; Vergara-Temprado et al., 2017; Shi and Liu, 2019). The importance of ice nucleation was demonstrated by Vergara-Temprado et al. (2018b), who found that defining a realistic INP population over the Southern Ocean leads to an improved simulation of the radiative properties of mixed-phase clouds. Estimates of equilibrium climate sensitivity (ECS) have been shown to be dependent on the supercooled liquid cloud fraction (Tan et al., 2016) and are sensitive to the magnitude of the cloud-phase feedback (Zelinka et al., 2020; Ceppi et al., 2017). Murray et al. (2021) hypothesize a link between the availability of INPs and the magnitude of the cloud-phase feedback, thereby linking the global distribution of INP concentrations to ECS estimates. The importance of ice formation for cloud radiative properties and feedbacks provides an incentive to include INPs as an active component of weather and climate models – i.e. treating ice formation as a process that depends on the ice-nucleating properties of transported aerosol particles.
Laboratory, field, and modelling studies have identified several components of aerosol particles that make them effective immersion-mode INPs (Murray and Liu, 2022; Burrows et al., 2022). Mineral dust is a globally important INP type due to its relatively high ice-nucleating ability and abundance (Augustin-Bauditz et al., 2014; Harrison et al., 2016; Ullrich et al., 2017; Kanji et al., 2017; Vergara-Temprado et al., 2017; Price et al., 2018; Harrison et al., 2019; Chatziparaschos et al., 2023). Most mineral dust is emitted from low-latitude arid source regions such as the Sahara and the Arabian Peninsula (Kok et al., 2021), although high-latitude cold environments are also potential sources of dust and INPs (Paramonov et al., 2018; Tobo et al., 2019; Sanchez-Marroquin et al., 2020; Meinander et al., 2022; Barr et al., 2023). Most of the mineral dust freezing behaviour below −15 °C is thought to be accounted for by the potassium-rich feldspar (K-feldspar) component of dust (Atkinson et al., 2013; Harrison et al., 2016; Vergara-Temprado et al., 2017; Harrison et al., 2019; Price et al., 2018; Harrison et al., 2022). Primary marine organic aerosol (PMOA) internally mixed with sea spray aerosol (SSA) is also a globally important type of INP (Schnell and Vali, 1976; Wilson et al., 2015; McCluskey et al., 2018a; Ickes et al., 2020; Irish et al., 2019; Zhao et al., 2021; Creamean et al., 2018). This marine-sourced INP is present in magnitudes that are comparable to dust INPs in regions far from major dust sources and is thought to be particularly relevant for the Southern Ocean (Vergara-Temprado et al., 2017, 2018b; McCluskey et al., 2018b, 2019, 2023; Zhao et al., 2021). Other particle types can contribute locally or regionally to the INP population, including primary biological aerosol particles such as fungal fragments, bacteria, or pollen (Pummer et al., 2012; Haga et al., 2013; Wex et al., 2015). Organic material associated with some mineral dusts, such as agricultural soil or alluvial material from river deltas, can also enhance the ice-nucleating ability of these dusts (Tobo et al., 2014; O'Sullivan et al., 2014; Pereira Freitas et al., 2023; Barr et al., 2023; Kawai et al., 2023; Sun et al., 2024), and volcanic ash can be transiently important (Steinke et al., 2011; Mangan et al., 2017; Maters et al., 2019, 2020). Carbonaceous material from biomass burning and anthropogenic activity has also been shown to act as an INP (Schill et al., 2016; Umo et al., 2015; Vergara-Temprado et al., 2018a). Modelling studies suggest it plays a minor role in the total INP population globally (Kanji et al., 2020; Schill et al., 2020; Vergara-Temprado et al., 2018a) but may influence INP concentrations in polluted regions alongside other anthropogenic components (Tian et al., 2022).
Global and regional models have been used to estimate the concentrations and seasonal cycles of INPs relevant for mixed-phase clouds from different sources (Lohmann and Diehl, 2006; Hoose et al., 2010; Burrows et al., 2013; Vergara-Temprado et al., 2017; Hummel et al., 2018; Huang et al., 2018; Vergara-Temprado et al., 2018a; McCluskey et al., 2019; Sanchez-Marroquin et al., 2020; Shi et al., 2022; Kawai et al., 2023; Chatziparaschos et al., 2023; Zhao et al., 2021; McCluskey et al., 2023; Schill et al., 2020). The simulated INP distributions have proven useful for understanding the relative importance of different INP types and in quantifying the impacts of INPs on clouds and climate (Vergara-Temprado et al., 2017; Shi and Liu, 2019; Shi et al., 2022; Chatziparaschos et al., 2023; DeMott et al., 2010; Hawker et al., 2021; Huang et al., 2018; Hummel et al., 2018; Kawai et al., 2023). Most models simulate dust INP concentrations based on simulated dust fields (Burrows et al., 2013; McCluskey et al., 2019; Shi et al., 2022) combined with laboratory measurements of INP activity of atmospheric dust (Niemand et al., 2012). Improved laboratory understanding of the specific minerals involved in ice nucleation, such as K-feldspar (Atkinson et al., 2013; Harrison et al., 2019) or quartz (Harrison et al., 2019), has enabled global models of INP concentrations based on these specific minerals (Vergara-Temprado et al., 2017; Chatziparaschos et al., 2023). The marine INP source has been represented by coupling either the simulated PMOA concentration (Vergara-Temprado et al., 2017; Burrows et al., 2013) or SSA surface area (McCluskey et al., 2019, 2018b) to laboratory-based parameterizations that describe their ice-nucleating ability (where environmental samples were analysed in a laboratory). Similarly, the role of carbonaceous INPs from biomass burning (Schill et al., 2020) and anthropogenic activity (Vergara-Temprado et al., 2018a) has been studied using simulated black carbon aerosol (BC) coupled to laboratory-based parameterizations (e.g. Schill et al., 2016, and Vergara-Temprado et al., 2018a)
The Met Office Unified Model (UM) has previously been used at the regional scale to study ice nucleation. Vergara-Temprado et al. (2018b) focused on cyclonic systems over the Southern Ocean and found that the cloud radiative properties are highly sensitive to the abundance of INPs. However, INPs were prescribed in the regional model based on separate global simulations in an offline aerosol model (Vergara-Temprado et al., 2017). Hawker et al. (2021) also used a regional configuration of the UM and focused on tropical deep convective clouds over the Atlantic Ocean, and they found that the definition of the immersion-mode freezing parameterization strongly affected the micro- and macroscale cloud properties. In this study we present a global INP model relevant for immersion-mode freezing of liquid water droplets. Our study builds on the work of Vergara-Temprado et al. (2017), who simulated INPs in the global chemical transport model GLOMAP (Global Model of Aerosol Processes, Mann et al., 2010). Here we use the Global Atmosphere configuration GA7.1 (Walters et al., 2019) of the Hadley Centre Global Environment Model (HadGEM). GA7.1 is the atmosphere-only setup of the HadGEM climate model, which includes a version of GLOMAP as part of the UK Chemistry and Aerosol (UKCA) sub-model. Although the aerosol model in this study (GLOMAP) is similar to that used in Vergara-Temprado et al. (2017), the aerosol emissions, transport, and removal processes are very different. An expanded global dataset of INP measurements is used to evaluate the model and identify missing INP sources. Our study constitutes a first step towards the full inclusion of the ice-nucleation process in the various Met Office modelling systems for weather and climate.
The paper is prepared as follows. Section 2 introduces the climate model and the methods by which we represent the three classes of INPs (dust, marine-sourced, and BC). In Sect. 3 we establish the efficacy of our model by evaluating the simulated seasonal cycles of dust, SSA, and PMOA concentrations against ambient aerosol measurements. Section 4 first introduces the global distribution of INPs simulated by our model and then evaluates the simulated INP concentrations against a dataset of INP measurements; the INP model evaluation includes global and regional evaluations (Sect. 4.2.1–4.2.2), as well as a focus on marine-sourced INPs in the Southern Ocean (Sect. 4.2.3). Following the outcomes of the evaluation we next focus on identifying missing INPs in our model; Sect. 5 introduces a number of possible sources and determines which are most likely to be contributing to our model bias. In Sect. 6 we summarize the main findings of our study and bring the results together to inform the direction of future studies.
The INP model in this study uses simulated aerosol concentrations generated by the UK Earth System Model version 1 (UKESM1), a climate configuration of the UM comprised of several coupled models detailed below. INP concentrations are calculated from the simulated aerosol fields using surface-area and mass-dependent parameterizations of immersion-mode freezing according to the singular description (Vali, 2014). This approach assumes that the time dependence of the ice-nucleation process is of second order compared with the temperature dependence and that ice nucleation occurs instantaneously at certain temperature-dependent active sites distributed on the surface of dust and BC particles or within the volume of SSA. The hypothesis of active sites is supported by experimental evidence (Holden et al., 2019, 2021) and can be used to explain in a reproducible way the freezing behaviour of water induced by most materials (Vali, 2014; DeMott et al., 2018). Therefore, although time dependence of nucleation is observed in laboratory measurements (Herbert et al., 2014; Knopf et al., 2020), for the vast majority of warm- and mixed-phase clouds the dominant driver for primary ice nucleation is via the cooling associated with vertical ascent.
2.1 The UK Earth System Model
UKESM1 is an Earth system model (Sellar et al., 2020) developed by the UK Met Office and the Natural Environment Research Council (NERC). The physical core of UKESM1 is the Global Coupled climate model 3.1 (GC3.1) comprised of the Global Atmosphere 7.1 (GA7.1) and Global Land 7.0 (GL7.0) configurations that represent the atmosphere–land–ocean–sea-ice interactions (Mulcahy et al., 2018; Walters et al., 2019). We use the atmosphere-only configuration of UKESM1. For these simulations we use a standard Atmospheric Model Intercomparison Project (AMIP) setup, whereby the sea-surface temperatures and sea ice fields are prescribed according to observed 2-D time series for the year 2010. Other relevant land-surface and ocean-surface variables are prescribed using climatologies for the year 2010, including ocean-surface chlorophyll concentrations which are used in our representation of marine-sourced INPs and discussed further in Sect. 2.3. The configuration has an atmospheric resolution of 1.25°×1.875° in latitude and longitude (about 135 km) and 85 vertical levels. We perform a free-running simulation (i.e. not nudged to reanalysis meteorology) for 20 years with prescribed fields (e.g. sea-surface temperature, surface properties, non-interactive aerosol emissions) taken from the year 2010 and calculate a mean annual cycle relevant for the present-day climate. The representation of aerosol emissions relevant for this study will be presented in the following sections. Although an evaluation of UKESM1 has been carried out by Mulcahy et al. (2020), given that we are employing the atmosphere-only configuration we present additional model evaluation of aerosol species most relevant for this study in Sect. 3.
2.2 Representation of dust INPs
We use dust concentrations simulated by the Coupled Large-scale Aerosol Simulator for Studies in Climate (CLASSIC) mineral dust scheme (Woodward, 2011) within the UM. CLASSIC prognostically represents dust in six size sections spanning diameters of 0.0632 to 63.2 µm, with interactive emissions calculated every 30 min. Dust emission occurs when the near-surface dust friction velocity exceeds a certain threshold value, which is dependent on the texture of the soil as well as the moisture content of the top layer. In the present study, dust is emitted only from model grid boxes that contain a non-zero fraction of bare soil. We assume that the ice-nucleation ability of the mineral component of soil dusts is determined by the K-feldspar content of the particles. This assumption is valid for the majority of desert dusts found worldwide (Atkinson et al., 2013; Harrison et al., 2022, 2019; Price et al., 2018; Chatziparaschos et al., 2023; Reicher et al., 2018), although it underestimates the ice-nucleation ability of soil dusts that contain organic ice-nucleating material, including agricultural soil dusts (O'Sullivan et al., 2014; Tobo et al., 2014) and high-latitude soil dusts (Tobo et al., 2019; Sanchez-Marroquin et al., 2020; Shi et al., 2022; Meinander et al., 2022; Barr et al., 2023). These organic-mineral-containing soil dusts are discussed in Sect. 5.2. Unlike Vergara-Temprado et al. (2017), we do not simulate the emission of K-feldspar as a distinct tracer. Instead, we simplify the model by assuming that 5 % of the dust mass and surface area is K-feldspar, consistent with mass fractions in atmospheric mineral dust particles (Atkinson et al., 2013; Harrison et al., 2019) and surface samples (Jeong, 2024). Although this mass fraction varies with location (about 1 to 20 %; Harrison et al., 2019; Nickovic et al., 2012; Harrison et al., 2022; Adebiyi et al., 2023) and particle size (Tanguy Claquin, 1999; Perlwitz et al., 2015), the error in INP abundance caused by using a fixed fraction is likely to be within the uncertainty of most INP measurement techniques. The assumed fraction of K-feldspar is discussed further in Sect. 5.1.
Using modelled dust concentrations, the dust INP number concentration in air (Ndust-INP) at a specific temperature T in each bin i is calculated following the singular description (DeMott, 1995; Murray et al., 2012; Vali, 2008) using Eq. 1.
In this equation, is the dust number concentration in each size bin (L−1 of air), is the fraction of the dust mass which corresponds to K-feldspar, ns(T) is the temperature-dependent density of ice-nucleating active sites of pure K-feldspar, and d is the mean diameter of the bin. We define ns(T) according to the parameterization of Harrison et al. (2019). The bins are summed to obtain the total Ndust-INP (L−1 of air).
2.3 Representation of marine-sourced INPs
For aerosol emitted from the ocean surface, two methods have previously been used to calculate INP activity. One is based on the mass concentration of atmospheric PMOA concentrations (Wilson et al., 2015; Zhao et al., 2021). This approach was used by Vergara-Temprado et al. (2017), obtaining good agreement with measurements of organic carbon. The reliability of the approach depends on the PMOA concentrations in the model, which are not well defined by measurements. McCluskey et al. (2019) used a simpler approach in which the ice-nucleating ability of PMOA is assumed to be proportional to the surface area of SSA, as defined by the measurements-based parameterization of McCluskey et al. (2018b). A model based on this assumption agreed well with INP measurements over the Southern Ocean and Mace Head (Ireland) (McCluskey et al., 2019). In our simulation we use the SSA-based approach to calculate marine-sourced INPs but test both approaches in Sect. 4.2.3.
For the SSA INPs we use SSA concentrations simulated by the modal version of the Global Model of Aerosol Processes (GLOMAP-mode). GLOMAP-mode, described in full by Mann et al. (2010) and Mulcahy et al. (2020), is a two-moment aerosol microphysics scheme that prognostically represents five log-normal aerosol modes ranging from particle diameters of 1 nm to 10 µm. SSA is present in two of the soluble modes (accumulation and coarse) and is internally mixed with sulfate, BC, and organic matter. The Nmarine-INP concentration is calculated following the singular description (DeMott, 1995; Murray et al., 2012; Vali, 2008) according to Eq. (2).
In order to perform these calculations, we extract the number, mass, standard deviation, and centre of the two aerosol modes that contain SSA. We then redistribute the SSA into six bins (denoted by i) from 0.1 to 10 µm. Using six bins reduces the precision in which the size distribution of the SSA can be described, but this only leads to minor effects on the marine-sourced INP concentration when compared to the uncertainties in the parameterization of the density of ice-nucleating active sites of the SSA. Using the number concentration of SSA in each bin, ni, we calculate the INP concentration in each bin using Eq. (2), where ns(T) is the density of ice-nucleating active sites given by McCluskey et al. (2018b). The concentration in all the bins is summed in order to calculate the total Nmarine-INP.
For the PMOA-based approach we use the model emissions of PMOA associated with SSA particles (Mulcahy et al., 2020). Briefly, the fraction of marine organic content in the SSA is correlated with the presence of chlorophyll in the sea surface and negatively correlated with the wind speed at 10 m. The sea–air flux of PMOA is calculated from the flux of SSA and the fraction of marine organic material in the SSA. Adding a source of PMOA in UKESM1 substantially improved the seasonal cycle of organic aerosol mass and cloud droplet number concentrations in the Southern Ocean (Mulcahy et al., 2020).
Although PMOA is added to the SSA particles, it is transported as aerosol organic matter along with other organic components from secondary organic aerosol and primary emissions. In order to separate the PMOA from the rest of the organic carbon, we therefore ran an additional simulation where the PMOA emissions were switched off. The difference in organic aerosol mass between the two simulations therefore represents the contribution from PMOA emissions. This method was successfully applied by Mulcahy et al. (2020) using UKESM1 to compare the modelled PMOA concentrations to measurements at Amsterdam Island. However, the organic aerosol components are not always linearly additive because of processes that influence other sources and sinks of organic aerosol. This results in errors in calculated PMOA primarily in regions where PMOA makes only a small contribution to total organic aerosol (mainly in the Northern Hemisphere). Because of these issues we restrict our analysis of PMOA INPs to the Southern Ocean boundary layer, where it is an important INP.
PMOA mass is converted into primary marine organic carbon assuming a mass ratio C PMOA (Wilson et al., 2015). The parameterization given by Wilson et al. (2015) is then used to convert the mass concentration of primary marine organic carbon into an INP concentration using Eq. (3), where [PMOC] is the mass concentration of primary marine organic carbon (in grams) and T is temperature (in kelvin).
Although the parameterization was developed for temperatures between −6 and −27 °C, we use it in the same range as the other parameterizations (−10 to −30 °C). This method relates the bulk mass of PMOA with a number of INPs. Given that PMOA is internally mixed with sea salt in SSA, for each aerosol mode we restrict Nmarine-INP,PMOA using the PMOA method to the number concentration of SSA.
2.4 Representation of black carbon INPs
In this model we represent INPs from biomass burning and anthropogenic activity using BC aerosol concentrations, which are simulated by GLOMAP-mode. Prescribed BC emissions in GLOMAP-mode include monthly-varying fluxes from biomass burning following van Marle et al. (2017) and annual mean fluxes from anthropogenic sources following Hoesly et al. (2018). BC is present in three soluble modes (Aitken, accumulation, and coarse) and one insoluble mode (Aitken). The NBC-INP concentration is calculated following the singular description according to Eq. (4).
Here is the BC number concentration in each size bin (ranging from 0.1 to 10 µm), with contributions from each aerosol size mode redistributed in the same manner as SSA in Eq. (2). We represent the ice-nucleating ability of BC aerosols using the ns(T) parameterization presented by Schill et al. (2020). This is based on INP measurements of wildfire emissions and is consistent with diesel exhaust (Schill et al., 2016) and soot (Vergara-Temprado et al., 2018a; Ullrich et al., 2017). The ns(T) values for wildfire emissions are slightly higher than diesel exhaust (Schill et al., 2020), so they can be seen as an upper estimate to the ice-nucleating ability of BC aerosol from all sources of simulated BC.
In this section we compare the simulated aerosol properties relevant for immersion-mode ice nucleation with in situ measurements from ground stations. We perform this analysis for dust, SSA, and PMOA. An in-depth evaluation of aerosol in UKESM1 was performed by Mulcahy et al. (2020). Using long-term ground-based observation networks (EMEP, IMPROVE, and EANET) Mulcahy et al. (2020) demonstrated that UKESM1 reproduces the global distribution and present-day trends of aerosol from both natural and anthropogenic sources with reasonable skill. A comparison with multiple satellite products shows good agreement with the spatial distribution and seasonal cycle of aerosol optical depth, although there is low bias close to dust source regions. Checa-Garcia et al. (2021) evaluated a number of Earth system models including UKESM1 with a specific focus on dust. The authors show that UKESM1 is able to generally capture the observed deposition flux, surface concentrations, and dust loading but under-represents the dust optical depth. For our INP model we are primarily interested in representing the dust concentrations. Therefore, the lack of skill in reproducing observed dust optical depths is of secondary importance to our analysis. BC mass concentrations simulated by UKESM1 have been compared to a network of ground-based observations by Mulcahy et al. (2020). The correlation coefficient between simulated BC and measured BC is 0.45 with a slightly negative bias. This model skill is consistent with other climate models (e.g. Tegen et al., 2019, and He et al., 2015). Therefore, we expect some associated uncertainty with our simulated BC INP concentrations, which we revisit later in the paper. We extend the UKESM1 evaluations performed by Mulcahy et al. (2020) and Checa-Garcia et al. (2021) with some additional focus on the three primary sources of INP types relevant for this study: dust (Fig. 1), SSA (Fig. 2), and PMOA (Fig. 3).
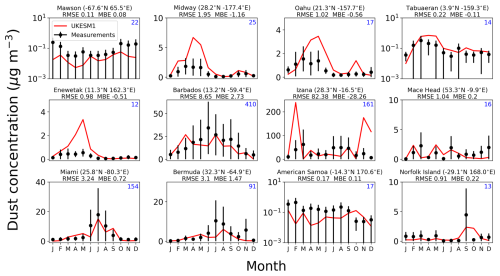
Figure 1Comparison of simulated dust concentrations with measurements. Lines correspond to the simulated monthly mean concentration at the location, and dots correspond to the monthly mean measurements from the Huneeus et al. (2011) database at 12 locations with standard deviations. The RMSE and MBE for each location are shown above each plot. The number shown in the top right of each plot is the mean number of measurements per month. Note that all stations have different y-axis scales, with Mawson, Tabuaeran, and American Samoa stations shown on a log scale.
The dataset of Huneeus et al. (2011) provides decadal-scale measurements of surface-level dust concentrations from several locations around the globe from the 1980s to the 1990s. Monthly mean measurements are shown alongside monthly mean simulated dust concentrations from the surface level of the model in Fig. 1. Overall, the model reproduces the relative magnitudes of dust concentrations and is able to capture seasonal variability. Model–measurement agreement is also good at remote locations far from dust sources, such as the Antarctic (Mawson) and the South Pacific Ocean (American Samoa and Norfolk Island). At Norfolk Island there is an inter-annually variable peak in September which is well reproduced, as are the low magnitudes (<10 µg m−3) at the other two stations. The greatest concentrations (20 to 100 µg m−3) are measured at Izana, Barbados, Bermuda, and Miami, which are all downwind from African dust source regions (Glaccum and Prospero, 1980; Prospero et al., 1981). Here, the model is able to capture the magnitudes and seasonal variability, though for winter months (November to February) the model over-predicts the dust concentrations in Izana by a factor of 4. This region, off the west coast of Africa, is subject to periodic plumes of dust that are strongly influenced by decadal-scale oscillations during the wintertime (Nakamae and Shiotani, 2013). Additionally our simulation uses some prescribed climatologies for the year 2010 so some discrepancy could be attributed to inter-annual or multi-decadal variability. A similar over-prediction (factor of 4) is observed in the tropical North Pacific island of Enewetak, influenced by the long-range transport of dust from Asia (Duce et al., 1980); similar factors could explain the discrepancies. Additional reasons for over-prediction are poorly represented dust removal processes and/or the insoluble nature of the simulated dust which would inhibit removal via cloud droplet activation. We revisit the representation and simulation of dust emissions in Sect. 5.
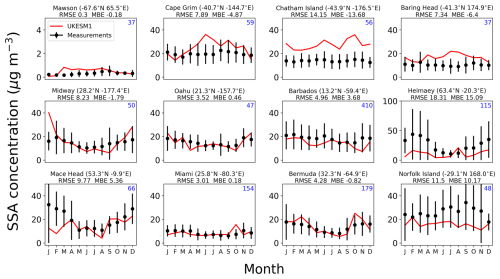
Figure 2Comparison of simulated sea spray aerosol (SSA) concentrations with measurements. Lines correspond to the simulated monthly mean concentration at the location (both accumulation and coarse modes), and dots correspond to monthly mean measurements. The RMSE and MBE for each location are shown above each plot. The number shown in the top right of each plot is the mean number of measurements per month. Note the different y-axis scales used for the Mawson and Heimaey stations.
The SSA evaluation is shown in Fig. 2. Monthly mean concentrations of accumulation and coarse modes are compared with historical measurements (1980s to 1990s) from the AEROCE (Atmosphere/Ocean Chemistry Experiment) and SEAREX (Sea/Air Exchange) programmes, available from the AeroCom benchmark data website (https://aerocom.met.no/data, last access: 26 January 2024). Compared to the dust, SSA measurements display considerably less variability amongst the 12 stations, with less pronounced seasonal variation in general. The model is within the range of measurement uncertainty in most stations, although it tends to over-predict at Chatham Island and Baring Head (both situated in the region of New Zealand). Although within measurement uncertainty, the model tends to consistently under-predict the magnitude at Heimaey (North Atlantic) throughout the annual cycle by up to a factor of 4. This is important as this station has the highest concentrations of all the stations, although it also displays the greatest inter-annual variability, which may account for the model discrepancy.
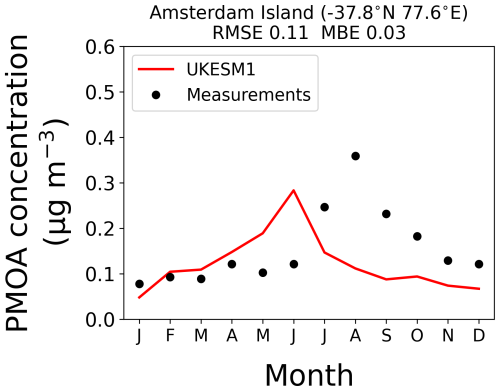
Figure 3Comparison of simulated primary marine organic aerosol (PMOA) concentrations in our simulation with measurements at Amsterdam Island. The line corresponds to the simulated monthly mean concentration at the location, and the dots correspond to monthly mean measurements. The RMSE and MBE are shown above the plot.
We compare simulated PMOA concentrations to measurements from Amsterdam Island (southern Indian Ocean) from Sciare et al. (2009) in Fig. 3. The discrepancies between the modelled and measured PMOA are consistent with Mulcahy et al. (2020), who also found that UKESM1 captures the magnitude and seasonality of the PMOA measurements in Amsterdam Island, but with the August peak concentration occurring about 2 months earlier in the model than in the measurements. This may be related to an early peak in simulated chlorophyll evident in Mulcahy et al. (2020), which is used to determine the PMOA emission flux. Nonetheless, the comparison suggests the model is able to capture the correct magnitude in PMOA, with the added caveat that there is a distinct lack of long-term PMOA measurements available to evaluate the model.
4.1 INP spatial distribution
The annual mean horizontal distribution of simulated INP number concentration from the sum of marine, dust, and BC sources () at an altitude of 500 m is shown in Fig. 4. A reference activation temperature of −20 °C is used to calculate NINP(T), which is the concentration that would be measured in a droplet freezing assay or an online INP counter at −20 °C, or if this air parcel were cooled to −20 °C. Dust INP concentrations (Fig. 4b) are greatest close to the major dust source regions of central and northern Africa, across much of Asia, and Australia. The outflow of these Northern Hemisphere (NH) dust sources results in elevated concentrations over the North Atlantic Ocean and North Pacific Ocean, whilst the South Pacific Ocean and Southern Ocean are influenced by outflow from Australia. There is a clear hemispheric gradient, with dust INP concentrations about 2 orders of magnitude higher in the northern high latitudes than in the south. Marine-sourced INP concentrations (Fig. 4d) are lower and less spatially variable than dust, with NINP(−20 °C) around 10−3 L−1 over much of the world's oceans. BC INP concentrations (Fig. 4f) are focused in regions of intense biomass burning (South America and central Africa) and regions influenced by anthropogenic pollution (Europe, North America, South Asia, and East Asia) with a maximum NBC-INP of ∼ 0.02 L−1 over East Asia. The total INP concentrations (Fig. 4a) are dominated by dust in most regions, with marine-sourced INPs making up a substantial fraction over the Southern Ocean (Fig. 4d). BC INPs contribute most strongly over the biomass burning regions, with less influence over the polluted continental regions (Fig. 4g). The presence of dust over many of the polluted regions, especially over Asia, suppresses the impact of BC INPs which reach a maximum contribution of ∼ 10 % over East Asia. This is consistent with previous global modelling studies that suggest a similarly weak role for BC INPs (Vergara-Temprado et al., 2018a; Schill et al., 2020; Kanji et al., 2020) and observational studies of anthropogenic-sourced INPs (Adams et al., 2020; Kanji et al., 2020; Zhang et al., 2022) in the immersion mode.
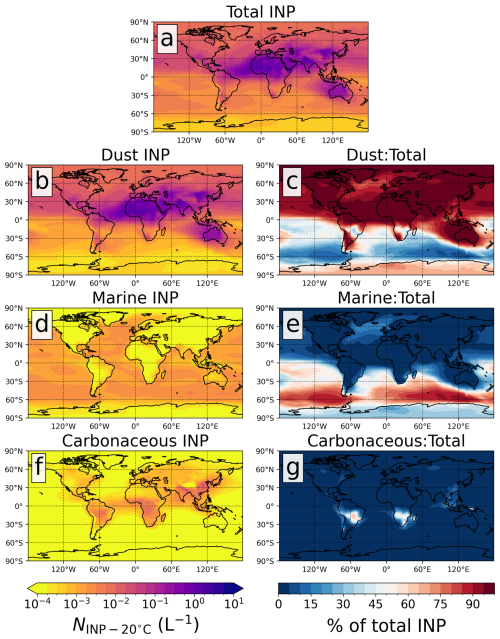
Figure 4Annual mean NINP(T) concentrations in the boundary layer at 500 m and a temperature of −20 °C for (a) total, (b) dust, (d) marine-sourced, and (f) BC NINP(−20 °C). The marine-sourced INPs are parameterized using SSA. Panels (c), (e), and (g) show the ratio (expressed as a percentage) of NINP(−20 °C) to total NINP(−20 °C) for dust, marine-sourced, and BC INPs, respectively, and it is used to highlight regions that are dominated by each source of INPs.
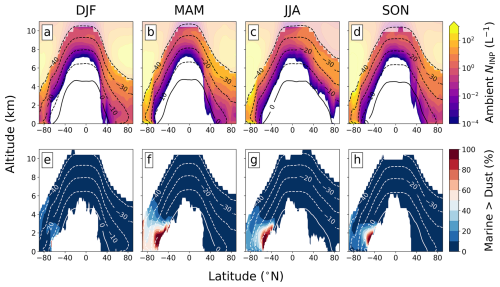
Figure 5Zonal mean profiles of NINP(Tamb) across the four seasons between the surface and an altitude of 11 km. Panels (a)–(d) show the total NINP(Tamb) with contour lines showing the seasonal mean ambient temperature at 10 °C intervals. Data for temperatures below −40 °C are muted as homogeneous freezing is likely to dominate over heterogeneous freezing. Filled contours in panels (e)–(h) show the percentage of grid boxes (over days and longitudes) where marine sources of NINP(Tamb) are larger than the contribution from the dust sources of NINP(Tamb).
Figure 5 shows the INP number concentration that is active at ambient temperatures, defined as NINP(Tamb). NINP(Tamb) is the INP concentration that we would expect to nucleate ice if activated to cloud droplets. The highest values of NINP(Tamb) occur at high latitudes or high altitudes where temperatures are the lowest. The NH high latitudes have relatively high concentrations throughout the lowest 4 km for most of the year, caused by the long-range transport of dust from the NH low-latitude regions. Figure 5a–d show that in the Southern Hemisphere non-negligible concentrations of NINP(Tamb) are only present within the lowest 2 km at latitudes south of 60° S, with concentrations greater than 102 L−1 for most of the year. The very cold lower-tropospheric temperatures present in this region enhance the activity of the very low concentrations of aerosols there that can act as INPs. In the tropics (30° S–30° N) INPs are active at mid-tropospheric altitudes (>6 km) where aerosol concentrations tend to be lower. Here INPs may play an important role in influencing radiative properties of deep convective clouds (Hawker et al., 2021). Figure 5e–h show that below 60° S both marine and dust sources contribute to NINP(Tamb), with marine sources more important over the Southern Ocean and dust more important over the Antarctic landmass. NINP(Tamb) values for BC INPs do not appreciably compete (<5 %) with either marine-sourced or dust INPs and have been left out of Fig. 5. The largest contribution of marine-sourced INPs occurs during March–May (MAM), while its minimum occurs during December–February (DJF). In contrast, the contribution of marine INP sources to NINP(Tamb) in the NH is negligible for most months of the year, only peaking at less than 5 % between October and March. Here in the NH, non-negligible NINP(Tamb) concentrations within the lower troposphere extend as far south as 40° N for all seasons except June–August (JJA). Although the temperatures in this region are not as cold as in the Southern Hemisphere polar region, there is a greater supply of dust from the tropical and subtropical dust sources present in the NH. The development of the Arctic polar dome, evident from SON through to MAM, results in very low temperatures and high INP concentrations close to the surface. These simulations are similar to Vergara-Temprado et al. (2017), but UKESM1 simulates a much smaller contribution of marine-sourced INPs to NINP(Tamb). This could be due to the different mechanisms used to represent marine INPs: in our study we use the SSA approach, whilst Vergara-Temprado et al. (2017) use the PMOA approach which predicts more INPs in the Southern Ocean. This is discussed further in Sect. 4.2.3.
4.2 Comparison with INP measurements
In this section we compare our INP model with a large set of ambient INP measurements made under immersion-mode conditions (at or above water saturation). We use the relevant measurements used in Vergara-Temprado et al. (2017), as well as a selection of recent measurements not included in that study (Ardon-Dryer and Levin, 2014; Price et al., 2018; McCluskey et al., 2018b; Si et al., 2018; Irish et al., 2019; Wex et al., 2019; Si et al., 2019; Jiang et al., 2020; Tobo et al., 2020; Sanchez-Marroquin et al., 2020, 2021; Tatzelt et al., 2021; Harrison et al., 2022) (see Table A1 for details; note we have not attempted to compile all available atmospheric INP data; rather we selected a subset of INP measurements with good global coverage). Figure 6 shows the location of all the measurements used in the comparison. For further analysis we split the measurements into zonal bands for the Arctic, NH mid-latitudes, NH subtropics, tropics, and the Southern Ocean. We additionally subset the Caribbean region to highlight the skill of the model in a region downwind of the Saharan dust source.
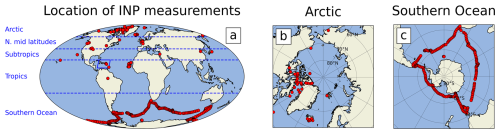
Figure 6Map indicating the location of the INP measurements used for the evaluation of our model across the globe (a), the Arctic (b), and the Southern Ocean (c). Some markers represent multiple measurements taken from fixed stations across the measurement time period. The measurements took place across several different time periods and not only during the year of our simulation (2010). Note that the number of markers in the map is not representative of the number of INP measurements. Several measurements carried out in a fixed ground-based station are represented using a single marker, given the fact that the location does not change across the measuring period, as opposed to ship- or aircraft-based measurements. Panel (a) shows the latitudinal divisions used in this analysis, which includes the Caribbean region marked by the square box.
4.2.1 Global evaluation
The simulated NINP and measured NINP are compared in Fig. 7. The INP model captures the overall spatial and temporal distribution of measured INP concentrations, which shows that the model is capable of representing the sources and transport of key INP types. The majority of the measurements are within 1.5 orders of magnitude of the simulated INP concentrations, which is comparable with the spread in INP concentrations typically observed at a given location over a month (e.g. Vogel et al., 2024). However, there is some discrepancy (root mean squared error (RMSE) of 1.73 and mean bias error (MBE) of −1.31), with the model under-predicting INP concentrations, especially at lower concentrations and higher temperatures.
Figure 7b shows the ratio between the simulated NINP and measured NINP as a function of temperature. In general, the underestimation of the INP concentrations is greater at higher temperatures, which explains why the bias is greatest for low INP concentrations in Fig. 7a. The higher bias at low concentrations was also reported by Vergara-Temprado et al. (2017), but here we show how the bias is related to the temperature rather than the concentration. A temperature-dependent bias shows that the cause is most likely related to the assumed INP properties of the particle (i.e. INP activity versus temperature) rather than the concentration of the aerosol particles themselves, so is not related to erroneous aerosol removal rates in the model unless such errors are also very strongly temperature dependent.
In Fig. 7c we link the INP concentration bias to the ns(T) parameterization. We express the bias as the temperature adjustment ns(Tmeasurement−Tbias) that would be required to obtain the measured INP concentration. A positive bias implies that the parameterization value of ns(T) is too low and would require a reduction in temperature of the same magnitude to higher values of ns(T). We define this as the temperature-dependent “parameterization bias” and use the metric ΔPbias to characterize the temperature dependence of the parameterization bias for the global (or regional) dataset. ΔPbias is calculated as the difference between the mean temperature bias at temperatures higher than −17 °C and lower than −22 °C. ΔPbias is −4.3 °C for the global dataset shown in Fig. 7c and can be attributed to the bias at the higher end of the temperature spectrum, which is consistent with a missing source of INP that is active at higher temperatures. One such candidate is a contribution of ice-active organic material that is known to be associated with many soils around the world (O'Sullivan et al., 2014; Tobo et al., 2014; Steinke et al., 2016, 2020; Chen et al., 2021). Additionally, or alternatively, bias may arise from an unrepresentative parameterization of mineral dust. We revisit these topics in Sect. 5 after evaluating INP properties in different regional environments.
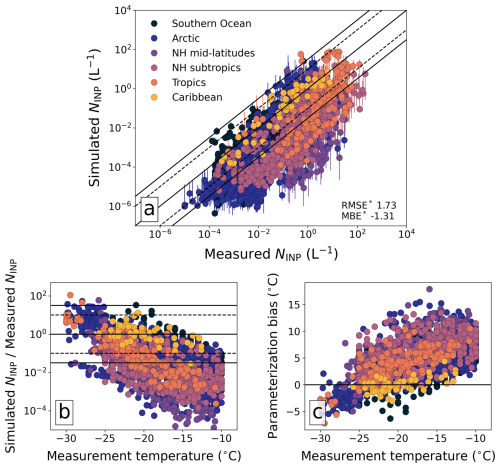
Figure 7Evaluation of the INP model. (a) Simulated NINP vs. measured NINP. The simulated concentration shown is the monthly median for the corresponding month of the measurement. Bars correspond to the variability in the monthly medians throughout the year in the model. Diagonal lines correspond to a bias of zero, 1 order of magnitude (dashed), and 1.5 orders of magnitude, respectively. Values of RMSE and MBE in log10 space are shown in the bottom-right corner. (b) Ratio between the simulated NINP and measured NINP versus measurement temperature. Horizontal lines correspond to a bias of zero, 1 order of magnitude, and 1.5 orders of magnitude, respectively. (c) Parameterization bias in the model for each measurement.
4.2.2 Regional evaluation
Figure 8a–f show model simulations versus measurements separated into the six regions. Here we see that the model performs better in some regions than others. Over the Southern Ocean simulated INP concentrations mostly lie within 1.5 orders of magnitude, with an overall negative bias at all concentrations. In the Arctic the model performs well at high INP concentrations but is increasingly biased low towards low concentrations. The biases are similar in the NH subtropics, which primarily includes measurements from Tokyo (Tobo et al., 2020) and Tel Aviv (Ardon-Dryer and Levin, 2014). The NH mid-latitude (western Europe and North America) measured concentrations show very little variation, with concentrations generally between 10−1 and 102 L−1. In contrast, the model predicts concentrations ranging from 10−6 to 101 L−1. This represents a considerable under-prediction in our INP model's ability to represent this region. The tropics include measurements made primarily in Cabo Verde and the Amazon. The Amazon measurements (mostly at higher INP concentrations) are well represented in the model, but Cabo Verde concentrations are consistently under-predicted. Measurements from the Caribbean region are well reproduced by the INP model and largely fall along the one-to-one line. This region is strongly influenced by trans-Atlantic dust from central Africa (Harrison et al., 2022), so it is surprising that we under-predict at Cabo Verde but not downwind in the Caribbean. A potential reason for this is an unrepresentative K-feldspar fraction applied to Cabo Verde dust, which has been reported to be much greater in Cabo Verde than in the Caribbean (Glaccum and Prospero, 1980; Prospero et al., 1981). It has been reported that the K-feldspar content in Cabo Verde is around 20 % (Kandler et al., 2011), whereas in Barbados values of around 1 % have been reported (Harrison et al., 2022; Kandler et al., 2018) In addition, mineralogical maps show spatial variability in feldspar content (Nickovic et al., 2012) and that some regions (e.g. the source of Caribbean dust; the Sahel) have a feldspar content close to our assumed 5 % whilst other regions (e.g. the Sahara) have higher contents. This spatial variability in feldspar content in both source regions and of transported dust in the atmosphere could perhaps explain why we under-predict the INP concentration in Cabo Verde. This is explored further in Sect. 5.1.
Figure 8g–l show the temperature-dependent parameterization bias in each region. The regional analysis is consistent with the global dataset (Fig. 7c). At temperatures higher than −15 °C all regions display a positive bias (temperature applied to parameterizations is too high), whereas at lower temperatures the parameterization bias tends towards negative values. Each region exhibits this behaviour, but this is more apparent in some than others. In the Caribbean there is a very weak temperature-dependent parameterization bias, whereas in the Arctic and NH mid-latitudes there is a strong temperature-dependent bias. This is consistent with the regional variations in Fig. 8a–f, suggesting that in most regions the model is missing a source of INPs active at higher temperatures (above about −15 °C). The analysis suggests relatively poorer model representation at latitudes north of 23.5° N, which coincides with NH sources of pollution, including North America, Europe, and parts of Asia. In Sect. 4.1 we showed that BC contributions to the total INP concentration were secondary when compared to mineral dust. Other components of anthropogenic pollution may contribute to the INP concentration; however, studies focusing on the impact of urban pollution report weak responses (Adams et al., 2020; Chen et al., 2018; Zhang et al., 2022; Yadav et al., 2019; Bi et al., 2019). Although it is difficult to rule out an additional contribution from anthropogenic aerosols, the temperature-dependent bias is apparent in all regions except for the Caribbean, suggesting this is unlikely. The obvious INP class that is thought to be common in the terrestrial mid-latitudes and is active above °C is that of biological/biogenic INPs (Kanji et al., 2017). We return to the topic of biogenic INPs in Sect. 5.2.
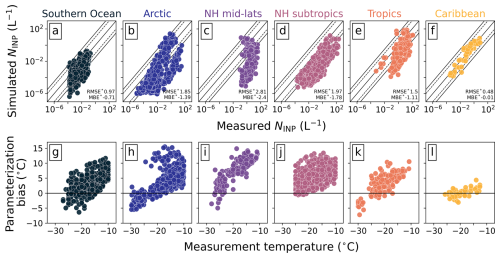
Figure 8NINP (a–f) and parameterization bias (g–l) as shown in Fig. 7a and c separated into the six regions of interest. The top row includes values of RMSE and MBE in log10 space. Diagonal lines in panels (g)–(l) correspond to a bias of zero, 1 order of magnitude (dashed), and 1.5 orders of magnitude.
4.2.3 PMOA versus SSA in the Southern Ocean
In this section we focus on the effect of assuming the marine-sourced INP concentrations are related to SSA or more specifically the PMOA component of the SSA particles. We restrict the comparison to the Southern Ocean as this is the only region for which we have measurements of both PMOA and SSA (Sect. 3), and it is where our simulated marine-sourced INPs are most important (Fig. 4).
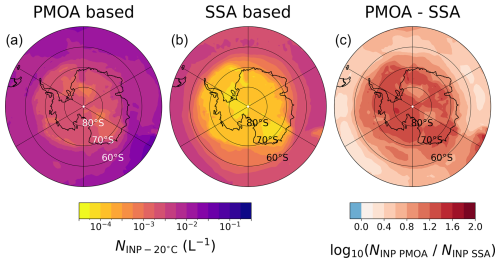
Figure 9Comparison of simulated annual mean marine-sourced INP concentrations using the two approaches (Sect. 2.3). (a) Marine NINP (−20 °C) concentration calculated using the PMOA approach at ground level. (b) Marine NINP (−20 °C) concentration calculated using the SSA approach at ground level. (c) Logarithmic difference between marine NINP (−20 °C) simulated using the PMOA and the SSA approaches (1 indicates a factor of 10 ratio). The area of the calculations has been restricted to the Southern Ocean for the reasons stated in Sect. 2.3.
Figure 9 shows the marine NINP at −20 °C calculated using the PMOA approach (Fig. 9a) and SSA approach (Fig. 9b). Figure 9c shows the difference in the order of magnitude between the two approaches. The PMOA approach produces NINP between 0.5 and 2 orders of magnitude higher than the SSA method. The difference between them is smaller in the northern regions of the Southern Ocean (50–60° S) as well as close to Antarctica. The spatial differences arise due to the underlying mechanisms of PMOA and SSA production, as well as removal processes in the aerosol microphysics scheme. PMOA emissions are dependent on surface concentrations of chlorophyll, whereas SSA emissions are wind speed dependent. SSA is removed from the atmosphere quicker than PMOA (Mann et al., 2010) as it is emitted into larger size modes. Differences in hygroscopicity between the two species also affect the removal rate through activation as cloud condensation nuclei. This acts to relatively enhance the transport of PMOA to the Antarctic landmass (Fig. 9c). The resulting latitudinal gradient (N–S) of the two aerosol types is more negative for SSA, driving an increasing difference in NINP between the two approaches.
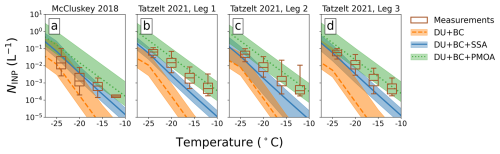
Figure 10Comparison between simulated NINP and measured NINP in the Southern Ocean. The panels include (a) the measurements of McCluskey et al. (2018a) and (b–d) the measurements reported for each of the legs of the circumnavigation by Tatzelt et al. (2021) (corresponding to the Indian Ocean, Pacific Ocean, and Atlantic Ocean; see Fig. S1). The simulated NINP, shown as lines and associated shading, is calculated for Ndust-INP+NBC-INP (DU+BC), (DU+BC+SSA), and (DU+BC+PMOA). Lines correspond to the median modelled concentration, while the shading corresponds to the 95 % interval over a time period and area comparable to the measurements. The measurements show the median and 25th and 75th percentiles of the data. The whiskers correspond to the 5th and 95th percentiles. Note that some data above −10 °C have been excluded from the analysis due to insufficient data at these higher temperatures.
To evaluate the two approaches we use measurements of NINP from McCluskey et al. (2018a) and Tatzelt et al. (2021). McCluskey et al. (2018a) made INP measurements during March 2016 as part of the Clouds, Aerosols, Precipitation, Radiation, and Atmospheric Composition Over the Southern Ocean (CAPRICORN) campaign, and Tatzelt et al. (2021) made INP measurements during the Antarctic Circumnavigation Expedition (ACE). ACE took place between December 2016 and March 2017 and was split into three legs (see Fig. S1 in the Supplement): Leg 1 took place from December to January south of the Indian Ocean, Leg 2 from January to February south of the Pacific Ocean, and Leg 3 from February to March south of the Atlantic Ocean. Though INP measurements from the Southern Ocean are scarce, the spatial coverage of the combined dataset provides valuable information from across the entire Southern Ocean, albeit for a single year.
Figure 10 shows that inclusion of marine-sourced INPs, from either approach, significantly enhances NINP when compared to dust-sourced INPs alone, especially at higher temperatures. In all cases, incorporating the marine source of INPs improves the agreement of the simulated NINP and measured NINP. For the McCluskey et al. (2018a) dataset (Fig. 10a), the SSA method produces a much better agreement to the measurements, whilst the PMOA-based method using the parameterization given by Wilson et al. (2015) tends to overestimate the measured concentrations. This is consistent with the results of McCluskey et al. (2019). However, for the three legs of the Tatzelt et al. (2021) dataset the approach that best matches the measurements is dependent on temperature. At lower temperatures (less than −20 °C) the measurements fall between the two approaches, whereas at higher temperatures, where most of the measurements are, the measurements are better reproduced by the PMOA-based approach. Incorrectly simulated aerosol concentrations (of SSA or PMOA) would result in a systematic bias in the simulated NINP; therefore, the temperature dependence of the bias suggests that the cause is related to the parameterizations of marine INP activity or that the model lacks an INP aerosol species that is active at higher temperatures. We will discuss this in the following section.
The PMOA-based approach produces higher concentrations than the SSA-based approach and better reproduces the measurement dataset. However, given the limited measurements in the region, it is difficult to state which approach is ultimately more appropriate. The PMOA parameterization is based on sea-surface microlayer samples from the Arctic and the North Atlantic (Wilson et al., 2015), and inspection of that data shows that the ice-nucleating activity (per mass of organic carbon) varies by up to a factor of 100 at any one temperature, with generally lower values found for samples in the North Atlantic compared to samples from the Arctic collected in the vicinity of sea ice. Comparable measurements in the Southern Ocean are not available. The SSA-based method used in this study underestimates some of the measurements given by Tatzelt et al. (2021) but produces an INP concentration within an order of magnitude of the measurements. We conclude that the SSA method produces a valid distribution of marine NINP over the Southern Ocean, and given it is far more simple to implement we recommend its use. It would be beneficial to repeat this analysis in other oceans and for other years, but this requires additional measurements that are currently lacking.
The analysis in Sect. 4 suggests our INP model is unable to reproduce the temperature dependence of NINP, with substantial bias at higher temperatures where INP concentrations are lowest. This suggests that INPs active at temperatures higher than −20 °C are either missing or currently incorrectly represented by our parameterizations. In this section we discuss potential causes of this discrepancy. Given that dust aerosol is the primary source of INPs in our model (Fig. 4), we focus on this aerosol species, though we also explore the potential for other species that are currently not simulated/represented by UKCA.
5.1 K-feldspar distribution and parameterizations
Several studies have shown that the ice-nucleating behaviour of mineral dust is primarily driven by the K-feldspar component (Atkinson et al., 2013; Harrison et al., 2016, 2019; Price et al., 2018; Harrison et al., 2022), which is the assumption we employ in this study. We have applied a constant value of 5 % K-feldspar content in our simulated dust, which is characteristic of transported dusts measured by Atkinson et al. (2013) and Harrison et al. (2019). However, we know that the K-feldspar content of soil and dust at the source is spatially variable (Nickovic et al., 2012), varying from ∼1 %–10 % (Jeong, 2024), and that larger dust particles will tend to contain relatively more K-feldspar than smaller particles (Nickovic et al., 2012). In Vergara-Temprado et al. (2017) the K-feldspar fraction of emitted dust in the model was driven by global maps of soil feldspar content and was enhanced in the larger aerosol size modes. Using this method results in a larger K-feldspar fraction close to dust source regions and a smaller fraction in remote regions. Despite the differences, the results and conclusions of that earlier study are very similar to ours, especially in the remote regions (e.g. Southern Ocean) where marine sources of INPs outcompete dust sources for much of the annual cycle. Therefore, it is reasonable to conclude that the missing high-temperature INPs in our model are not due to the spatial variability of K-feldspar that we neglect. However, the comparison between measurements in the Caribbean and the eastern tropical Atlantic indicate that there is some benefit in representing the variability in K-feldspar fraction in a similar manner to Vergara-Temprado et al. (2017).
A second source of potential model error arises from the temperature-dependent parameterization that links the dust K-feldspar content to its ice-nucleating ability. To explore this we have recalculated the global distributions of NINP using an alternative temperature dependence of INP activity given by Atkinson et al. (2013), shown in Fig. 11a alongside the default of Harrison et al. (2019) used in our INP model. We similarly assume a K-feldspar content of 5 %. This parameterization results in higher ns values compared to Harrison et al. (2019) across much of the temperature range but is consistent with the upper range of K-feldspar ice-nucleating activity from the compilation of literature data used by Harrison et al. (2019). The alternative K-feldspar parameterization leads to slightly better agreement between the model and the measurements (Fig. 11) in all regions except for the Caribbean, where the RMSE increases. Table 1 shows that globally the RMSE decreased from 1.73 to 1.49, ΔPbias has reduced from −4.3 to −3.0 °C, and the percentage of data with parameterization biases within a given threshold increased for all thresholds, except for the extreme cases where the parameterization bias exceeds 5 °C. This occurs because ns is higher than the original parameterization at temperatures below −12 °C (Fig. 11a). It is not possible to say whether the Atkinson et al. (2013) parameterization is more appropriate as some of the model–measurement uncertainty biases could be due to incorrectly simulated dust transport or size distributions, or K-feldspar variability as discussed previously. Future studies would benefit from INP, dust concentrations, and K-feldspar fraction measurements made over long time periods (months to years), which would provide a means to more robustly evaluate simulated INP concentrations.
Other neglected mineralogical components may also play a role. Chatziparaschos et al. (2023) recently presented a modelling study where atmospheric dust was partitioned into components of K-feldspar and quartz. Although quartz has a lower ice-nucleating ability than K-feldspar (Harrison et al., 2019), it is more abundant than K-feldspar (Atkinson et al., 2013). Chatziparaschos et al. (2023) found that although K-feldspar dominated the overall contribution to dust-sourced INP concentrations, there were some regions and some altitudes where the quartz was more important. The greatest impact was found at low temperatures around −30 °C; therefore, the addition of this component may improve our INP model at these temperatures but is unlikely to explain the missing INPs at higher temperatures. However, the active sites on quartz samples have been shown to be unstable, with some freshly milled quartz samples becoming less active with time when exposed to both liquid water and air (Harrison et al., 2019; Kumar et al., 2019). In contrast, the active sites on K-feldspars are stable, with activity being maintained after 10 d in liquid water and a shift of only ∼1 °C after 16 months (Harrison et al., 2016). The parameterization for quartz is for freshly ground material, which might be relevant for particles that have recently undergone the saltation process, but the ageing of quartz in the atmosphere may lead to an overestimate in the contribution of quartz to the INP population.
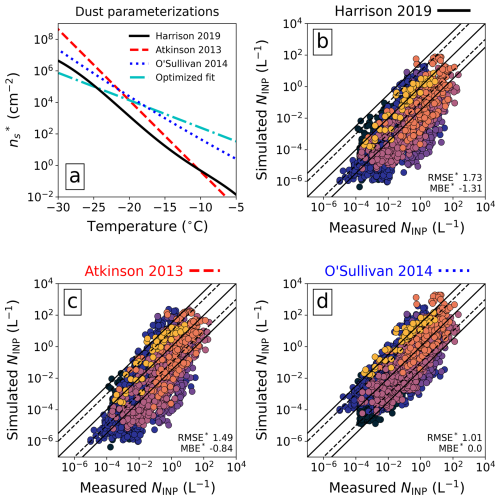
Figure 11Sensitivity of modelled dust INP concentrations to parameterization of ice-nucleating activity. (a) Temperature-dependent ns curves of the parameterizations for dust INPs, where ns for the Harrison et al. (2019) and Atkinson et al. (2013) curves is scaled by 5 % (accounting for dust K-feldspar content) to make all descriptions comparable. Panels (b), (c), and (d) show the simulated NINP vs. measured NINP in all regions for the Harrison et al. (2019), Atkinson et al. (2013), and O'Sullivan et al. (2014) parameterizations. Diagonal lines in panels (b)–(d) correspond to a bias of zero, 1 order of magnitude, and 1.5 orders of magnitude, respectively. The RMSE and MBE for each comparison are shown in the lower right of each panel, calculated using the log10 of each dataset.
5.2 Terrestrial biological material
Previous studies have shown that terrestrial biological material contributes to the INP population (Schnell and Vali, 1976; Conen et al., 2011; Morris et al., 2014; Tobo et al., 2014; Fröhlich-Nowoisky et al., 2015; Steinke et al., 2016; Augustin-Bauditz et al., 2016; O'Sullivan et al., 2018; Steinke et al., 2020; Cornwell et al., 2023; Gong et al., 2022; Sun et al., 2024). This can be in the form of primary biological aerosol particles such as fungal fragments, bacteria, and pollen (O'Sullivan et al., 2015; Fröhlich-Nowoisky et al., 2015; Palmero et al., 2011; Pouleur et al., 1992; Morris et al., 2014; Steinke et al., 2020) or in the form of organic material, which ultimately results from biological activity, such as macromolecules or other detritus attached to agricultural soil particles (O'Sullivan et al., 2015; Conen et al., 2011; O'Sullivan et al., 2016; Hill et al., 2016; Sun et al., 2024). Recent measurements in the Arctic suggest that dust emissions from high-latitude river sediment sources have similar activity to agricultural soils and also contain an important ice-active organic component (Barr et al., 2023; Meinander et al., 2022; Tobo et al., 2019).
Figure 12 shows that the total organic carbon (TOC) content of the topsoil layer can vary by around 1 order of magnitude across relatively small spatial scales. Some dust-producing regions such as the Sahara desert have typically low TOC contents (<1 kg C m−2), whereas other dust emission regions such as parts of North America and central Russia have relatively high TOC contents (>10 kg C m−2). We might expect the contribution of ice-active organic material to dust INPs to be larger close to regions where there are periodically conditions commensurate with biological activity (i.e. liquid water), such as agricultural or seasonally fertile areas as well as river flood plains. In contrast, dust from the most arid deserts, such as much of the Sahara, has a very low organic carbon content (Minasny et al., 2017, 2014; Price et al., 2012), and measurements indicate that dusts from these arid sources are controlled by their mineral content rather than any trace of organic material (Harrison et al., 2022) (see Fig. S3).
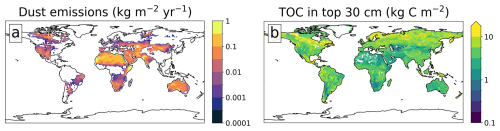
Figure 12Annual mean simulated dust emissions (a) and total organic carbon (TOC) content in the topsoil (0–30 cm) layer (b) taken from FAO et al. (2012).
Although some studies have attempted to include pollen grains, bacteria, and fungal spores in global models (Hoose et al., 2010; Spracklen and Heald, 2014; Cornwell et al., 2023), the lack of quantitative understanding of factors that determine fluxes of biological particles and of their ice-nucleating behaviour limits our capacity to represent all biological sources of INPs. Ice-nucleating proteins from soil fungus are known to bind to mineral dust particles and retain the ice-nucleating ability (O'Sullivan et al., 2016), whilst the membrane-bound proteins from bacteria can still nucleate ice after the cells are no longer viable and are fragmented (O'Sullivan et al., 2015; Murray et al., 2022; Bieber and Borduas-Dedekind, 2023). Additionally, pollen ice-nucleating macromolecules can be readily separated from the original pollen grains (Pummer et al., 2012; Murray et al., 2022). Hence, it has been suggested that these biological macromolecules or fragments of ice-nucleating biological entities might serve as a much more numerous group of INPs in the atmosphere than whole bacterial cells, pollen grains, or fungal spore (Rangel-Alvarado et al., 2015; O'Sullivan et al., 2015). Furthermore, the work that has been done on the global contribution of pollen, bacteria, and fungal spores to INP concentrations indicates that these species contribute very few INPs because particle concentrations in the atmosphere are much lower than concentrations of dust or SSA (Spracklen and Heald, 2014; Hoose et al., 2010).
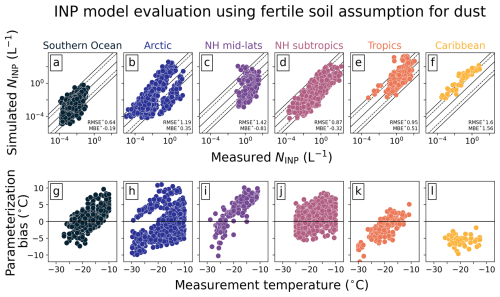
Figure 13Evaluation of the INP model using the fertile soil parameterization of O'Sullivan et al. (2014) to represent dust INPs. (a–f) Regional comparison between the simulated and measured concentrations and (g–l) parameterization bias as a function of measurement temperature in each region. Simulated INP concentrations shown in panels (a)–(f) are monthly medians for the corresponding month of the measurement. Diagonal lines in panels (a)–(f) correspond to a bias of zero, 1 order of magnitude (dashed), and 1.5 orders of magnitude.
Here we explore the potential effect of organic material attached to dust particles. We refer to soil dusts that contain organic material that results from biological activity as fertile soils, and we use desert soils to refer to the soils from arid regions containing very little organic matter. Fertile soils will likely produce mineral dust aerosol with more organic material associated with it than desert soils. In reality the biological content will vary between regions, e.g. Fig. 12b. Even soils in some semi-arid regions can contain organic matter and pose as a viable INP source (Schnell, 1974a, b), though airborne dust from northern Africa was found to contain no observable biological ice-nucleating components (Harrison et al., 2022). Although the global database of TOC in Fig. 12b may suggest a potential link between dust emissions and biological content, at present there are insufficient INP measurements to define the variability of activity we might expect due to the biological activity of fertile and desert soils. Therefore, as a simple first step and a reasonable approximation, we represent the ice-nucleating ability of all mineral dust as fertile soil according to the parameterization of O'Sullivan et al. (2014). The soils from the UK that O'Sullivan et al. (2014) analysed are consistent with the ice-nucleating activity of Argentinian soil dust (DeMott et al., 2018), Wyoming soil dust (Tobo et al., 2014), and high-latitude proglacial dust from the Copper River valley (Barr et al., 2023). A comparison of ns(T) (Fig. 11a) shows that the fertile soil parameterization is 2 orders of magnitude more active than that of K-feldspar (desert soils) at temperatures higher than −12 °C but is within a factor of 10 at temperatures below −20 °C. By representing the ice-nucleating ability of all mineral dust with the ice-nucleating ability of fertile soil we expect that we will over-estimate the INP concentration in some locations (such as the Caribbean, where dust was found to contain no appreciable organic ice-nucleating material), but this test will tell us if the organic material associated with fertile soils is a potential explanation for low-biased INP concentrations elsewhere in our model.
Table 1Table showing (top half) the RMSE and MBE between measurements and simulations using different dust INP parameterizations from the literature (Harrison et al., 2019; Atkinson et al., 2013; O'Sullivan et al., 2014) and an optimized parameterization; (middle) temperature dependence of the bias expressed using the metric ΔPbias; and (bottom half) percentage of data within parameterization bias thresholds.
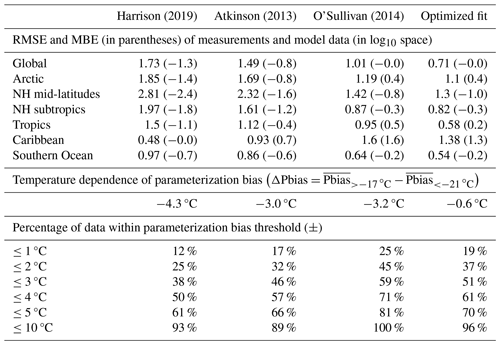
Figure 11d compares the model and measurements assuming a fertile soil parameterization for all dust. A regional comparison of NINP and parameterization bias is shown in Fig. 13. A fertile soil assumption considerably improves model–measurement agreement and removes a substantial fraction of the temperature-dependent bias in NINP. The ΔPbias has decreased from −4.3 to −3.2 °C, and global RMSE has reduced from 1.73 to 1.01 (MBE is now 0.0), contributed by improvements in all regions except for the Caribbean (Table 1). The greatest improvement occurs in the NH subtropics and mid-latitudes, where the RMSE falls from 2.0 and 2.8 to 0.9 and 1.4. In contrast, the RMSE in the Caribbean has increased from 0.5 to 1.6. We also see an improved representation in the Arctic, where RMSE has decreased from 1.8 to 1.2. This is consistent with the simulations of Kawai et al. (2023), who found that summertime (JJA) Arctic INP measurements were best reproduced using a parameterization (Tobo et al., 2019) that accounted for measured organic content associated with locally sourced surface dust.
These fertile soil simulations suggest that the organic component in fertile soil makes an important contribution to INP activity at temperatures higher than −20 °C where INP concentrations are usually low. The results also suggest that INPs around the world could be explained by a mixture of more- or less-fertile dust particles sourced from different regions. In the Caribbean, a fully fertile soil parameterization consistently overestimates measured INP concentrations (Fig. 13f), while the desert soil assumption (Table 1) produces realistic INP concentrations. This is a region heavily influenced by the major dust source regions of central and northern Africa where dust is mostly abiotic, as demonstrated by the heat tests performed on Caribbean samples (Harrison et al., 2022) and the low TOC values at the surface (<1 kg C m−2; Fig. 12b). Hence, the fact that INP concentrations in the Caribbean are better represented by the desert soil parameterization is consistent with the measurements and known sources of dust. In the Arctic, the fertile soil parameterization produces INP concentrations with mostly zero bias relative to the measurements (Fig. 13h) at temperatures higher than −15 °C, but the model is generally negatively biased below −20 °C. This suggests that the Arctic is influenced by dust sourced from both fertile and desert soils. There is good evidence that dust from the low latitudes (e.g. African continent) is transported to the high latitudes alongside dust from local sources (Shi et al., 2022), the latter of which is thought to contain a high fraction of biological material (Tobo et al., 2019; Kawai et al., 2023). Therefore, we hypothesize that representing the spatial variability of K-feldspar in desert soils and organic material in fertile soils will improve the ability of our model to simulate measured INP concentrations.
5.3 High-latitude dust
A third source of uncertainty we explore is the regional pattern of dust emissions. Much of the focus of global modelling centres is to better represent the size distributions and fluxes of dust from the major dust sources, such as those in Africa, the Middle East, and Asia. Historically, less attention is given to the weaker dust source regions, which include the high latitudes. However, these regions have been identified as substantial sources of local dust (Bullard et al., 2016; Arnalds et al., 2016; Dagsson-Waldhauserova et al., 2019; Shi et al., 2022; Meinander et al., 2022), and measurements of these dust particles show high INP activity (Sanchez-Marroquin et al., 2020; Barr et al., 2023; Tobo et al., 2019; Xi et al., 2022).
Simulating high-latitude dust (HLD) is challenging given that its emission mostly occurs in glaciofluvial valleys and is strongly affected by small-scale katabatic winds (Bullard et al., 2016; Prospero et al., 2012). The driving processes tend to occur at around 10 km scales, which are much smaller than the resolution of global models and thus rely on sub-grid-scale parameterizations. Despite the challenges, some studies have simulated HLD on global and regional scales (Leadbetter et al., 2012; Groot Zwaaftink et al., 2016, 2017; Tobo et al., 2019; Hamilton et al., 2019; Mingari et al., 2020; Sanchez-Marroquin et al., 2020; Shi et al., 2022). To explore the potential impact of HLD we use UM simulations that were performed by Walters et al. (2019) using the atmosphere model identical to ours, but with additional coupling of a land-surface model. In the model description paper the authors introduce the Met Office UM Global Atmosphere and Global Land configuration (GA7.1 and GL7.0). As part of this study the authors ran simulations of the atmosphere coupled to the Joint UK Land Environment Simulator (JULES) at different resolutions including N96 (135 km resolution; as used in our INP model), N216 (60 km resolution), and N512 (25 km resolution). Although these are all unable to explicitly resolve turbulent motion, we can use them to gain a perspective on the resolution dependence of dust emissions in our model. For the following analysis we will focus on the Arctic.
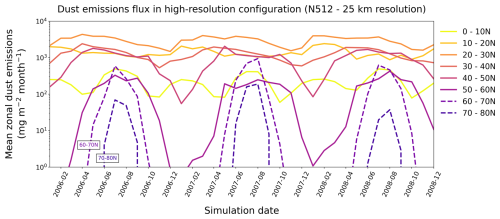
Figure 14Time series (January 2006–December 2008) of zonal mean dust emission fluxes in the NH from the high-resolution configuration (25 km) of UKESM simulations presented by Walters et al. (2019). Each coloured line shows the mean for a 10° latitude band from the Equator to 80° N. Latitudes south of 60° N are shown as solid lines, and latitudes north (the Arctic) are shown as dashed lines.
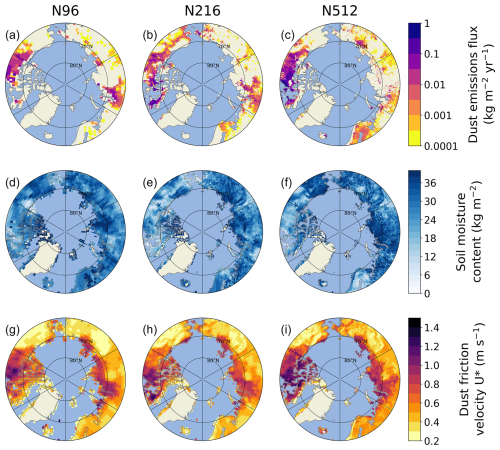
Figure 15Dust emissions and associated surface properties during July 2008 in the UM GA7.1 and GL7.0 simulations by Walters et al. (2019) at three resolutions over the Arctic region >60° N. Monthly mean values of (a–c) dust emission fluxes, (d–f) soil moisture content, and (g–i) dust friction velocity. The configurations (N96, N216, and N512) correspond to horizontal resolutions of approximately 135, 60, and 25 km.
Figure 14 shows NH zonal mean dust emissions fluxes in the N512 resolution simulations. Peak emissions for latitudes north of 30° N occur between April and October. In the Arctic region (north of 60° N) simulated dust emissions are negligible (below 1 mg m−2 per month) outside of these months and peak in July and August. Measurements from HLD sources (Bullard et al., 2016; Bullard and Mockford, 2018; Prospero et al., 2012) are consistent with the time period for simulated Arctic emissions (April–October) but suggest the month of peak emissions may occur earlier or later than July. This suggests even at the finest model resolution tested here UKESM may be unable to represent HLD emissions of dust from sub-grid-scale features such as valleys. Given that simulated emissions peak in July, we will focus on this month to investigate the role of model resolution dependence.
The July mean dust emission flux north of 60° N is shown for the three resolutions in Fig. 15a–c. We also show two of the primary drivers (see Sect. 4) of dust emissions in the model (Woodward et al., 2022): soil moisture content (Fig. 15d–f, shown for the top soil layer) and the dust friction velocity (Fig. 15g–i). At the coarsest resolution (135 km) dust emissions occur predominantly over North America and central Russia. As the resolution increases there are more numerous source regions, often with greater magnitudes. These new sources include coastal regions surrounding Greenland, Iceland, and the Arctic Archipelago, which likely correspond to small-scale glaciofluvial sites. Much of northern Scandinavia becomes a widespread source of dust in the finest resolution (25 km). Table 2 provides information on the July mean dust emissions and variability for the Arctic region (north of 60° N). As resolution increases, so does the annual mean flux and variability. Both the magnitude and variability (standard deviation) of the emissions increase 3-fold from the coarsest to finest resolution. This demonstrates that the overall emissions of Arctic HLD and the likelihood of extreme cases (in the form of relatively enhanced plumes of dust) are sensitive to the model resolution. This result may be specific to the model we use and its representation of dust emission processes. As an example, Shi et al. (2022) used a global model with 100 km horizontal resolution but were capable of simulating Arctic HLD emissions using a physically based dust emission model following Kok et al. (2014). Emissions in the Kok et al. (2014) dust model are dependent on wind friction velocity and the soil's threshold friction velocity, as in our model, but also include a term that accounts for increased emissions through saltation bombardment as the soil erodibility increases. Additionally, the model is primarily dependent on variables that are properties of the large-scale environment (soil and horizontal winds), which may help to suppress resolution dependence.
Table 2Table showing the annual mean dust emissions flux and standard deviation from inside the Arctic (north of 60° N) for three different resolution configurations of the UM–UKCA coupled to JULES. The figure in the parentheses is the typical horizontal grid scale.
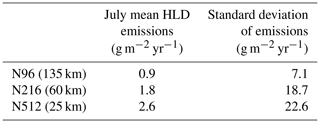
We explore the drivers of resolution dependence by focusing on the soil moisture content (Fig. 15d–f) and dust friction velocity (Fig. 15g–i). The soil moisture content is one of the key factors for dust uplift potential, with drier soil more readily emitted. Higher friction velocity also promotes more efficient dust uplift. The spatial distribution of soil moisture is generally similar across the scales, but the higher-resolution simulations are increasingly capable of resolving small-scale features. These include glacial valleys (visible throughout Russia and northern Asia) or sharp gradients that are smoothed out in the coarser scale (such as around the coastlines of Iceland and Greenland). Some regions have also become noticeably wetter or drier as resolution is increased, including the Alaskan North Slope and Scandinavia. The dust friction velocity displays similar sensitivity. The finer resolution permits sharper gradients and therefore more numerous extreme cases of high friction velocities. There is a particular sensitivity over the Arctic Archipelago, which drives some of the enhanced dust emissions. The reason behind these changes is beyond the scope of this paper but provides additional evidence that model resolution is important for representing Arctic HLD emissions. We hypothesize that an increase in model resolution beyond that investigated here would be required in order to reduce the discrepancy between the annual cycle of HLD emissions simulated by UKESM1 (Fig. 14) and as suggested by measurements (Prospero et al., 2012; Bullard et al., 2016; Bullard and Mockford, 2018). However, measurements of HLD are scarce, which makes evaluation difficult and provides a degree of uncertainty as to how much HLD our models should in fact simulate. This uncertainty can be observed in Shi et al. (2022), who find that although their model simulated a peak in summertime Arctic HLD at the Alert station (82.5° N, 20.3° W), this was not replicated in the measurements (Sirois and Barrie, 1999), which displayed either no seasonal cycle or a summertime minimum.
This section demonstrates that Arctic HLD emissions are sensitive to the model resolution. This may also hold true in the Southern Hemisphere, especially around the coastlines of Antarctica which are known to be potential sites for dust emissions (Asmi et al., 2018; Bory et al., 2010; Meinander et al., 2022; Kavan et al., 2017, 2018). Using a higher resolution in our simulations would likely increase the INP concentrations in our model, but this would occur primarily in the high latitudes and at all freezing temperatures. Therefore, weak HLD emissions do not explain the missing high-temperature INPs in our simulations. However, as the soil properties play a primary role in driving dust emissions, we recognize that using an interactive land-surface model would be a beneficial addition to our study and therefore recommend this for future studies focusing on HLD. Finally, there is a clear need for additional information on dust emissions from high-latitude environments, especially given that future trends suggest a shift towards more summertime melting and the appearance of either seasonal or permanent regions of bare soil that can produce dust (Meinander et al., 2022).
5.4 Optimized dust representation
Replacing the K-feldspar dust parameterization with a fertile soil assumption reduces the RMSE and some of the temperature-dependent ΔPbias (Table 1), but there remains a considerable parameterization bias towards the higher end of the temperature spectrum (Fig. 16). This suggests the INP spectrum has an even weaker temperature dependence than the fertile soils description from O'Sullivan et al. (2014). An alternative parameterization for dust INPs was produced to give the lowest combination of RMSE and ΔPbias when evaluated against the measurements. Figure 11a shows the optimized parameterization, which is described by , where T is in kelvin, and applicable for temperatures between −10 and −30 °C. Figure 16 shows that the ΔPbias has been substantially reduced from −3.2 °C (for the O'Sullivan et al., 2014, parameterization) to −0.6 °C, and the RMSE has reduced from 1.01 to 0.71. The optimized parameterization of ns(T) has a shallower temperature dependence than the other descriptions, with a value of −0.175 °C−1 compared to −0.35 and −0.28 °C−1 for the Harrison et al. (2019) and O'Sullivan et al. (2015) parameterizations. This temperature dependence is consistent with studies that have measured the ice-nucleating activity of soil and dust samples but shallower than most known INP species. Soil samples taken from four different regions of the world in Steinke et al. (2016) exhibit an ns(T) gradient of −0.15 °C−1, and dust samples from East Asian dust events in Chen et al. (2021) exhibit an ns(T) gradient around −0.19 °C−1. This suggests the material causing the shallow gradient is associated with the dust aerosol but requires further work to establish whether this is caused by organic material or another INP species. Figure 16 shows that the median parameterization bias is now close to 0 °C for measurement temperatures higher than −26 °C, but there remains a negative bias below this temperature. This supports our hypothesis that the mineral component, which exhibits a steeper temperature dependence (Fig. 11a), is important at lower temperatures, whereas the organic component (or material causing the shallow gradient) is important at higher temperatures. We note that the optimized fit is a simple exponential description that, if extrapolated to 0 °C, would be at odds with classical nucleation theory (Kashchiev, 2000). Measurements, such as those of O'Sullivan et al. (2014), demonstrate that the ice-nucleating activity of INP types often changes with temperature and rapidly decreases as the temperature approaches 0 °C. We therefore anticipate the gradient of the optimized representation, and associated ice-nucleating material, to not stay constant across the temperature spectrum.
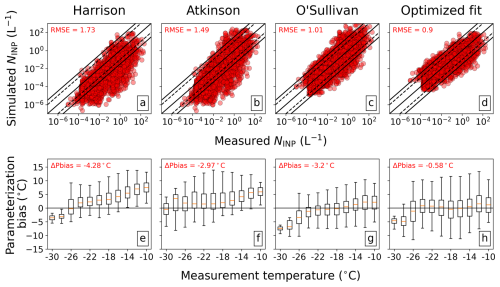
Figure 16Evaluation of the INP model using four representations of simulated dust INPs including three parameterizations of ns(T) from literature (Harrison et al., 2019; Atkinson et al., 2013; O'Sullivan et al., 2014) and the optimized parameterization. (a–d) Comparison between simulated and measured INP concentrations with the RMSE for each dataset shown in the upper-left corner. (e–h) Distribution of parameterization bias (in the form of boxplots) as a function of temperature for each representation. The ΔPbias value (measure of temperature dependence) for each representation is shown in the upper-left corner of panels (e)–(h).
In this study we present the first global simulations of ice-nucleating particles (INPs) relevant for immersion-mode freezing of cloud droplets using the atmosphere configuration of the UK Earth System Model (UKESM). The model is driven by three primary sources of INPs: mineral dust aerosol assuming that its ice-nucleating ability is determined by the K-feldspar content (Harrison et al., 2019), marine sources associated with sea spray aerosol (SSA) (McCluskey et al., 2018a) or the primary marine organic aerosol (PMOA) component of the SSA (Wilson et al., 2015), and black carbon (BC) sources from natural and anthropogenic processes. Multi-decadal simulations were run in order to quantify a mean annual cycle of INPs relevant for mixed-phase clouds.
The spatial distribution of INP concentrations in UKESM is dominated by the major dust source regions, whereas marine-sourced INPs are confined primarily to the oceanic regions and have less spatial variability. BC sources influence the INP concentration close to biomass burning regions (South America, central Africa, and Indonesia) and polluted regions (North America, Europe, and Asia), but concentrations tend to be lower than dust sources. In the Northern Hemisphere (NH), dust is the dominant INP in all regions, with marine-sourced INPs playing a negligible role even over the North Atlantic Ocean, North Pacific Ocean, and Arctic Ocean. The dominance of dust in the Arctic region, albeit with a local supply of marine-sourced INPs, highlights the important role of long-range dust transport from the low-latitude dust source regions to the NH high latitudes. Dust uplifted to high altitudes contributes to the availability of high-altitude INPs that may influence high-cloud radiative properties and humidification of the stratosphere (Hawker et al., 2021; Jensen et al., 2013). In contrast to the NH, marine-sourced INPs are the dominant source of INPs over large parts of the Southern Hemisphere (SH), particularly over the Southern Ocean, where only 10 %–20 % of annual mean INP concentrations are dust. Over the Antarctic landmass, dust supplies about 60 %–80 % of INPs, which is caused by transport of dust from non-local sources (given that we have no local sources simulated in the model). This is compatible with the trajectory analysis from Neff and Bertler (2015), who found that Patagonia and New Zealand were important suppliers of dust to Antarctica, and also with McConnell et al. (2007), who have associated the desertification of Patagonia with a doubling of dust deposition to Antarctica in the twentieth century. However, other studies have identified local dust sources (Asmi et al., 2018; Bory et al., 2010) that also may play a role, especially during Austral summer melt periods.
A comparison of simulated NINP against a large global dataset of measurements demonstrates that our INP model is able to represent the general spatial and temporal distribution of INP, which varies by roughly 4 orders of magnitude (calculated at an altitude of 500 m and −20 °C). However, the model tends to underestimate measured INP concentrations particularly when concentrations are low, which we show is caused by under-prediction at higher temperatures. As an average across all the measurements, the bias is equivalent to a 10 °C shift in the INP spectrum. Such a temperature-dependent bias occurs in all regions except for the Caribbean, which is downwind of the central Africa mineral dust sources. The tropics, NH subtropics, and NH mid-latitudes have a particularly strong temperature-dependent parameterization bias as well as an under-prediction of most measurements. The temperature dependence of the bias suggests that it cannot be due to incorrectly simulated dust transport or dust size distributions, which would cause a consistent bias across the whole temperature range.
Black carbon INPs are unlikely to be globally important but may influence regional concentrations. Over East Asia BC INPs from bio-fuel and fossil-fuel emissions represent a maximum of ∼10 % of total INPs measurable at the surface, whereas in South Asia, Europe, and North America the contribution is <5 %. Away from these regions the contribution is negligible. This is consistent with other global modelling studies (Vergara-Temprado et al., 2018a; Schill et al., 2020; Kanji et al., 2020) but suggests there may be a role for BC INPs on local scales and in synoptic conditions with low dust concentrations or in remote marine environments (Thomson et al., 2018). This is also consistent with some observational studies that suggest non-volatile organics contribute to the INP population (Tian et al., 2022; Zhao et al., 2019) but is inconsistent with others (Adams et al., 2020; Zhang et al., 2022; Yadav et al., 2019; Bi et al., 2019). The community would benefit from more compositional information on anthropogenic aerosols and the ice-nucleating activity of each component. In this study we only consider BC aerosols, yet other components, such as anthropogenic-sourced organic aerosol (Tian et al., 2022) or traffic-influenced dust emissions (Chen et al., 2024), may also act as locally important sources of INPs in polluted regions.
The INP representation and temperature-dependent model–measurement bias is improved when we assume that organic material is attached to the dust particles. The default ice-nucleation parameterization based on the K-feldspar content of dust (Harrison et al., 2019) was replaced with a parameterization based on agricultural soil samples (O'Sullivan et al., 2014) that accounts for an additional organic component. We refer to this class as fertile soil, and we use desert soil to refer to soils containing very little organic matter whose ice-nucleating ability is dependent on the mineralogical component. The fertile soil parameterization enhanced the ice-nucleating activity of dust particularly at high temperatures (greater than −15 °C), reducing some of the temperature-dependent bias (ΔPbias) from −4.3 to −3.2 °C and decreasing the RMSE of the global dataset from 1.73 to 1.01. Most improvement was found in the NH mid-latitudes and NH subtropics where the model was originally poorest. However, agreement with the Caribbean measurements is worsened and is instead best represented assuming no organic component. This demonstrates that not all dust sources can be assumed to be represented as fertile soils. We hypothesize that there is likely spatial variability in the ice-active organic component of fertile soil, which may be related to factors such as rainfall, soil organic carbon, or soil biodiversity. We aim to explore this in future studies.
An optimized parameterization of ns(T) for dust INPs suggests the best representation exhibits a shallower temperature-dependent parameterization than current descriptions. The optimized parameterization substantially reduced ΔPbias from −3.2 °C for the O'Sullivan et al. (2014) fertile soils parameterization to −0.6 °C whilst reducing the RMSE for INP concentrations from 1.01 to 0.71. This was achieved primarily due to the weaker temperature dependence of ns(T), which has a logarithmic slope of −0.175 °C, compared to −0.35 and −0.28 °C for the Harrison et al. (2019) and O'Sullivan et al. (2014) parameterizations. Though such gradients have been observed in laboratory studies (Steinke et al., 2016; Chen et al., 2021), further work is required to identify the aerosol material causing this and whether it is associated with the dust or is a distinct INP species that needs to be represented.
Arctic INPs are particularly important for mixed-phase clouds in cold air outbreaks, and it has been hypothesized that local sources may be important (Murray et al., 2021). We therefore investigated the model's ability to represent such sources. A comparison of simulated Arctic dust emissions using three model configurations with resolutions of 135 (this study), 60, and 25 km demonstrated that summertime high-latitude dust (HLD) emissions and their variability are considerably dependent on model resolution. Mean emissions and their variability increase 3-fold from the coarsest to the finest resolution. This suggests our INP model is missing some sources of HLD and may contribute to the misrepresentation of Arctic INP measurements. We recommend an exploration of alternative methods to reproduce HLD emissions in UKESM alongside much-needed measurements from around the Arctic in order to robustly evaluate and ground the models.
Over the Southern Ocean, we found that the simulated INP concentration is sensitive to the method used to represent the ice-nucleating ability of organics-enriched SSA. A parameterization using the PMOA content of the SSA (Vergara-Temprado et al., 2017; Burrows et al., 2013) produces more INPs than a simpler parameterization based on the SSA surface area (McCluskey et al., 2018b). The difference between the two approaches increases with proximity to the Antarctic landmass, which can be linked to differences in the emission processes of PMOA and SSA. A comparison of the two methods was made with INP measurements from the Southern Ocean. At the lowest temperatures ( °C) both methods produced values of NINP consistent with the measurements, whereas at higher temperatures ( °C) the PMOA method is more representative. The SSA method is technically easier to represent in the model as PMOA is not regularly included as a prognostic aerosol species in aerosol microphysics models (including UKCA). Therefore, although the PMOA method is likely the more appropriate representation, we conclude that the SSA method is a reasonable pragmatic approximation for marine-sourced INPs. We note that PMOA emissions are likely more sensitive to future climate and/or inter-annual variability in oceanic productivity than the SSA.
Our results suggest several directions for the improvement of global INP models. There is a clear need for evaluations to be made on longer timescales that can capture diurnal cycles, synoptic-scale events, seasonal cycles, and inter-annual variability. This will provide a robust test of whether models can capture the key transport pathways between source and receptor regions. The evaluations will additionally enable better separation of INPs into particular particle compositions emitted from particular locations, which is needed to build a model that represents sources, transport, and removal of INPs. For this to be possible we require long-term INP measurements that span these timescales. Remote-sensing platforms provide an excellent opportunity to make such measurements, yet complex cloud dynamical and microphysical processes may obscure any link between INP availability and the resulting bulk-scale cloud properties (Korolev et al., 2017; Morrison et al., 2020). This uncertainty associated with cloud microphysical processes restricts the use of remote-sensing observations for quantification of INP concentrations (e.g. Carlsen and David, 2022, and Zhang et al., 2012). An alternative direction is extending current INP measurements to provide greater spatial coverage and longer sampling periods. Several recent campaigns in the Arctic (Sze et al., 2023; Creamean et al., 2022) and those using the Portable Ice Nucleation Experiment (PINE) cloud chamber instruments (Möhler et al., 2021; Brasseur et al., 2022; Adams et al., 2021; Wilbourn et al., 2024; Vogel et al., 2024) are capable of making these measurements. In future work we aim to repeat this evaluation and focus on these longer records, and we encourage continued effort in extending these types of measurements. The analysis of simulated INP concentrations at ambient conditions in Fig. 5 highlights that INPs are active within the lowest 5 km of the atmosphere north of 40° N and south of 40° S. Therefore, we recommend in situ INP measurements from within the lower atmosphere are targeted within these latitudes to gain a representative dataset for evaluation. We also simulate INPs in the tropical mid-troposphere (∼10 km) that may influence tropical high-cloud radiative properties, yet there is a distinct lack of INP measurements at these altitudes. We recommend targeting these altitudes for sampling of air and INP analysis. The in situ sampling should be complemented by building on our existing knowledge of INP activity from surface samples at important source regions, such as Africa, the Middle East, and Australia, and HLD sources that may play a disproportionately important role. Finally, if dust emissions are associated with fertile soils it is likely that marine-sourced INP concentrations in the Southern Hemisphere will become less important, to an extent that it may be reasonable to assume global INP activity can be represented by dust alone. This would simplify the requirements for adding interactive INPs into climate models. This hypothesis would require additional measurements in the SH, especially on longer timescales that can capture the seasonal cycles of dust, SSA, and PMOA and help constrain the primary drivers of INP activity in the Southern Ocean.
The INP distribution we present here provides a valuable tool for the community. Running a fully coupled aerosol microphysics scheme adds considerable computational expense to simulations; hence, cloud-ice microphysics schemes are not commonly coupled to aerosol fields. For simplicity, temperature-dependent parameterizations are often used to calculate INP concentrations (e.g. Meyers et al., 1992, and Cooper, 1986); however, they cannot represent spatial or temporal variability. Others are more complex and are dependent on concentrations of large (over 0.5 µm diameter) non-SSA particles (DeMott et al., 2010) or mineral dust particles (DeMott et al., 2015). Our model builds on these complex parameterizations and provides a 4D climatology of INP concentrations for use in global and regional modelling studies, as well as other relevant applications. Though we do not fully understand the mechanisms involved, the INP distribution using the fertile soil parameterization given by O'Sullivan et al. (2014) is able to best represent the INP measurements. This informs us on the direction of future model developments, with a need to represent dust aerosol emissions from both desert and fertile soils, rather than just a single class.
Table A1Details of the INP measurements used for evaluation. The campaign name is included alongside the reference where available. The spatial distribution of measurements can be found in Fig. 6 and individual datasets compared against the INP model in Fig. A1.
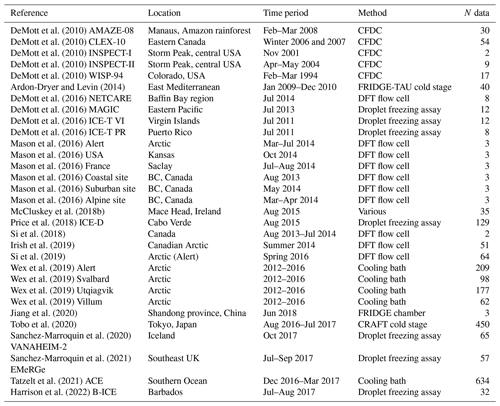
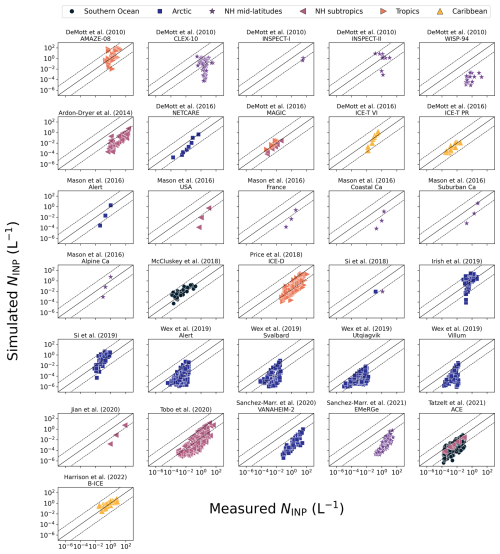
Figure A1Evaluation of the INP model against individual measurement datasets, detailed in Table A1. Dust INPs are represented using the K-feldspar-based parameterization of Harrison et al. (2019). Marine-sourced INPs are represented with the parameterization of McCluskey et al. (2018a) applied to the surface area of SSA. The simulated concentration shown is the monthly median for the corresponding month of the measurement. The solid line corresponds to a bias of zero and the dashed lines to a bias of 1.5 orders of magnitude. Geographical locations, shown in Fig. 6, are depicted by different marker shape/colour combinations.
All data relevant for producing the figures and values and the necessary Python scripts are available on Zenodo at https://doi.org/10.5281/zenodo.11186250 (Herbert, 2024).
The supplement related to this article is available online at: https://doi.org/10.5194/acp-25-291-2025-supplement.
BJM and KSC designed the study. ASM and KJP configured the model and ASM ran the simulations. RJH and ASM developed the analysis codes and performed the analysis, with assistance from KJP and DPG. BJM, KSC, and SRA contributed to discussions and the direction of analysis. RJH and ASM led the manuscript preparation with contributions from all co-authors.
At least one of the (co-)authors is a member of the editorial board of Atmospheric Chemistry and Physics. The peer-review process was guided by an independent editor, and the authors also have no other competing interests to declare.
Publisher’s note: Copernicus Publications remains neutral with regard to jurisdictional claims made in the text, published maps, institutional affiliations, or any other geographical representation in this paper. While Copernicus Publications makes every effort to include appropriate place names, the final responsibility lies with the authors.
This work used Monsoon2, a collaborative high-performance computing facility funded by the Met Office and the Natural Environment Research Council, and JASMIN (http://jasmin.ac.uk/, last access: 7 October 2024), the UK collaborative data analysis facility.
This research has been supported by the Natural Environment Research Council (grant no. NE/T00648X/1) and the Centre for Environmental Modelling And Computation (CEMAC).
This paper was edited by Dantong Liu and reviewed by two anonymous referees.
Adams, M. P., Tarn, M. D., Sanchez-Marroquin, A., Porter, G. C. E., O'Sullivan, D., Harrison, A. D., Cui, Z., Vergara-Temprado, J., Carotenuto, F., Holden, M. A., Daily, M. I., Whale, T. F., Sikora, S. N. F., Burke, I. T., Shim, J.-U., McQuaid, J. B., and Murray, B. J.: A Major Combustion Aerosol Event Had a Negligible Impact on the Atmospheric Ice-Nucleating Particle Population, J. Geophys. Res.-Atmos., 125, https://doi.org/10.1029/2020JD032938, 2020. a, b, c
Adams, M. P., Atanasova, N. S., Sofieva, S., Ravantti, J., Heikkinen, A., Brasseur, Z., Duplissy, J., Bamford, D. H., and Murray, B. J.: Ice nucleation by viruses and their potential for cloud glaciation, Biogeosciences, 18, 4431–4444, https://doi.org/10.5194/bg-18-4431-2021, 2021. a
Adebiyi, A., Kok, J. F., Murray, B. J., Ryder, C. L., Stuut, J.-B. W., Kahn, R. A., Knippertz, P., Formenti, P., Mahowald, N. M., Pérez García-Pando, C., Klose, M., Ansmann, A., Samset, B. H., Ito, A., Balkanski, Y., Di Biagio, C., Romanias, M. N., Huang, Y., and Meng, J.: A review of coarse mineral dust in the Earth system, Aeolian Res., 60, 100849, https://doi.org/10.1016/j.aeolia.2022.100849, 2023. a
Ardon-Dryer, K. and Levin, Z.: Ground-based measurements of immersion freezing in the eastern Mediterranean, Atmos. Chem. Phys., 14, 5217–5231, https://doi.org/10.5194/acp-14-5217-2014, 2014. a, b, c
Arnalds, O., Dagsson-Waldhauserova, P., and Olafsson, H.: The Icelandic volcanic aeolian environment: Processes and impacts – A review, Aeolian Res., 20, 176–195, https://doi.org/10.1016/j.aeolia.2016.01.004, 2016. a
Asmi, E., Neitola, K., Teinilä, K., Rodriguez, E., Virkkula, A., Backman, J., Bloss, M., Jokela, J., Lihavainen, H., Leeuw, G. D., Paatero, J., Aaltonen, V., Mei, M., Gambarte, G., Copes, G., Albertini, M., Fogwill, G. P., Ferrara, J., Barlasina, M. E., and Sánchez, R.: Primary sources control the variability of aerosol optical properties in the Antarctic Peninsula, Tellus B, 70, 1414571, https://doi.org/10.1080/16000889.2017.1414571, 2018. a, b
Atkinson, J., Murray, B., Woodhouse, M., Whale, T., Baustian, K., Carslaw, K., Dobbie, S., O'Sullivan, D., and Malkin, T.: The importance of feldspar for ice nucleation by mineral dust in mixed-phase clouds, Nature, 498, 355–358, https://doi.org/10.1038/nature12278, 2013. a, b, c, d, e, f, g, h, i, j, k, l, m
Augustin-Bauditz, S., Wex, H., Kanter, S., Ebert, M., Niedermeier, D., Stolz, F., Prager, A., and Stratmann, F.: The immersion mode ice nucleation behavior of mineral dusts: A comparison of different pure and surface modified dusts, Geophys. Res. Lett., 41, 7375–7382, 2014. a
Augustin-Bauditz, S., Wex, H., Denjean, C., Hartmann, S., Schneider, J., Schmidt, S., Ebert, M., and Stratmann, F.: Laboratory-generated mixtures of mineral dust particles with biological substances: characterization of the particle mixing state and immersion freezing behavior, Atmos. Chem. Phys., 16, 5531–5543, https://doi.org/10.5194/acp-16-5531-2016, 2016. a
Barr, S. L., Wyld, B., McQuaid, J. B., III, R. R. N., and Murray, B. J.: Southern Alaska as a source of atmospheric mineral dust and ice-nucleating particles, Sci. Adv., 9, eadg3708, https://doi.org/10.1126/sciadv.adg3708, 2023. a, b, c, d, e, f
Bi, K., McMeeking, G. R., Ding, D. P., Levin, E. J. T., DeMott, P. J., Zhao, D. L., Wang, F., Liu, Q., Tian, P., Ma, X. C., Chen, Y. B., Huang, M. Y., Zhang, H. L., Gordon, T. D., and Chen, P.: Measurements of Ice Nucleating Particles in Beijing, China, J. Geophys. Res.-Atmos., 124, 8065–8075, https://doi.org/10.1029/2019JD030609, 2019. a, b
Bieber, P. and Borduas-Dedekind, N.: High-speed cryo-microscopy proves that ice-nucleating proteins of Pseudomonas syringae trigger freezing at hydrophobic interfaces, ChemRxiv, https://doi.org/10.26434/chemrxiv-2023-m016b, 2023. a
Bory, A., Wolff, E., Mulvaney, R., Jagoutz, E., Wegner, A., Ruth, U., and Elderfield, H.: Multiple sources supply eolian mineral dust to the Atlantic sector of coastal Antarctica: Evidence from recent snow layers at the top of Berkner Island ice sheet, Earth Planet. Sc. Lett., 291, 138–148, https://doi.org/10.1016/j.epsl.2010.01.006, 2010. a, b
Brasseur, Z., Castarède, D., Thomson, E. S., Adams, M. P., Drossaart van Dusseldorp, S., Heikkilä, P., Korhonen, K., Lampilahti, J., Paramonov, M., Schneider, J., Vogel, F., Wu, Y., Abbatt, J. P. D., Atanasova, N. S., Bamford, D. H., Bertozzi, B., Boyer, M., Brus, D., Daily, M. I., Fösig, R., Gute, E., Harrison, A. D., Hietala, P., Höhler, K., Kanji, Z. A., Keskinen, J., Lacher, L., Lampimäki, M., Levula, J., Manninen, A., Nadolny, J., Peltola, M., Porter, G. C. E., Poutanen, P., Proske, U., Schorr, T., Silas Umo, N., Stenszky, J., Virtanen, A., Moisseev, D., Kulmala, M., Murray, B. J., Petäjä, T., Möhler, O., and Duplissy, J.: Measurement report: Introduction to the HyICE-2018 campaign for measurements of ice-nucleating particles and instrument inter-comparison in the Hyytiälä boreal forest, Atmos. Chem. Phys., 22, 5117–5145, https://doi.org/10.5194/acp-22-5117-2022, 2022. a
Bullard, J. E. and Mockford, T.: Seasonal and decadal variability of dust observations in the Kangerlussuaq area, west Greenland, Arct. Antarct. Alp. Res., 50, e1415854, https://doi.org/10.1080/15230430.2017.1415854, 2018. a, b
Bullard, J. E., Baddock, M., Bradwell, T., Crusius, J., Darlington, E., Gaiero, D., Gassó, S., Gisladottir, G., Hodgkins, R., McCulloch, R., McKenna-Neuman, C., Mockford, T., Stewart, H., and Thorsteinsson, T.: High-latitude dust in the Earth system, Rev. Geophys., 54, 447–485, https://doi.org/10.1002/2016rg000518, 2016. a, b, c, d
Burrows, S. M., Hoose, C., Pöschl, U., and Lawrence, M. G.: Ice nuclei in marine air: biogenic particles or dust?, Atmos. Chem. Phys., 13, 245–267, https://doi.org/10.5194/acp-13-245-2013, 2013. a, b, c, d
Burrows, S. M., McCluskey, C. S., Cornwell, G., Steinke, I., Zhang, K., Zhao, B., Zawadowicz, M., Raman, A., Kulkarni, G., China, S., Zelenyuk, A., and DeMott, P. J.: Ice-Nucleating Particles That Impact Clouds and Climate: Observational and Modeling Research Needs, Rev. Geophys., 60, e2021RG000745, https://doi.org/10.1029/2021RG000745, 2022. a, b
Carlsen, T. and David, R. O.: Spaceborne Evidence That Ice-Nucleating Particles Influence High-Latitude Cloud Phase, Geophys. Res. Lett., 49, e2022GL098041, https://doi.org/10.1029/2022GL098041, 2022. a
Ceppi, P., Brient, F., Zelinka, M. D., and Hartmann, D. L.: Cloud feedback mechanisms and their representation in global climate models, WIREs Climate Change, 8, e465, https://doi.org/10.1002/wcc.465, 2017. a
Chatziparaschos, M., Daskalakis, N., Myriokefalitakis, S., Kalivitis, N., Nenes, A., Gonçalves Ageitos, M., Costa-Surós, M., Pérez García-Pando, C., Zanoli, M., Vrekoussis, M., and Kanakidou, M.: Role of K-feldspar and quartz in global ice nucleation by mineral dust in mixed-phase clouds, Atmos. Chem. Phys., 23, 1785–1801, https://doi.org/10.5194/acp-23-1785-2023, 2023. a, b, c, d, e, f, g
Checa-Garcia, R., Balkanski, Y., Albani, S., Bergman, T., Carslaw, K., Cozic, A., Dearden, C., Marticorena, B., Michou, M., van Noije, T., Nabat, P., O'Connor, F. M., Olivié, D., Prospero, J. M., Le Sager, P., Schulz, M., and Scott, C.: Evaluation of natural aerosols in CRESCENDO Earth system models (ESMs): mineral dust, Atmos. Chem. Phys., 21, 10295–10335, https://doi.org/10.5194/acp-21-10295-2021, 2021. a, b
Chen, J., Wu, Z., Augustin-Bauditz, S., Grawe, S., Hartmann, M., Pei, X., Liu, Z., Ji, D., and Wex, H.: Ice-nucleating particle concentrations unaffected by urban air pollution in Beijing, China, Atmos. Chem. Phys., 18, 3523–3539, https://doi.org/10.5194/acp-18-3523-2018, 2018. a
Chen, J., Wu, Z., Chen, J., Reicher, N., Fang, X., Rudich, Y., and Hu, M.: Size-resolved atmospheric ice-nucleating particles during East Asian dust events, Atmos. Chem. Phys., 21, 3491–3506, https://doi.org/10.5194/acp-21-3491-2021, 2021. a, b, c
Chen, J., Wu, Z., Gong, X., Qiu, Y., Chen, S., Zeng, L., and Hu, M.: Anthropogenic Dust as a Significant Source of Ice-Nucleating Particles in the Urban Environment, Earth's Future, 12, e2023EF003738, https://doi.org/10.1029/2023EF003738, 2024. a
Conen, F., Morris, C. E., Leifeld, J., Yakutin, M. V., and Alewell, C.: Biological residues define the ice nucleation properties of soil dust, Atmos. Chem. Phys., 11, 9643–9648, https://doi.org/10.5194/acp-11-9643-2011, 2011. a, b
Cooper, W. A.: Ice Initiation in Natural Clouds, Meteor. Mon., 21, 29–32, https://doi.org/10.1175/0065-9401-21.43.29, 1986. a
Cornwell, G. C., McCluskey, C. S., Hill, T. C., Levin, E. T., Rothfuss, N. E., Tai, S.-L., Petters, M. D., DeMott, P. J., Kreidenweis, S., Prather, K. A., and Burrows, S. M.: Bioaerosols are the dominant source of warm-temperature immersion-mode INPs and drive uncertainties in INP predictability, Sci. Adv., 9, eadg3715, https://doi.org/10.1126/sciadv.adg3715, 2023. a, b
Creamean, J. M., Kirpes, R. M., Pratt, K. A., Spada, N. J., Maahn, M., de Boer, G., Schnell, R. C., and China, S.: Marine and terrestrial influences on ice nucleating particles during continuous springtime measurements in an Arctic oilfield location, Atmos. Chem. Phys., 18, 18023–18042, https://doi.org/10.5194/acp-18-18023-2018, 2018. a
Creamean, J. M., Barry, K., Hill, T. C. J., Hume, C., DeMott, P. J., Shupe, M. D., Dahlke, S., Willmes, S., Schmale, J., Beck, I., Hoppe, C. J. M., Fong, A., Chamberlain, E., Bowman, J., Scharien, R., and Persson, O.: Annual cycle observations of aerosols capable of ice formation in central Arctic clouds, Nat. Commun., 13, 3537, https://doi.org/10.1038/s41467-022-31182-x, 2022. a
Dagsson-Waldhauserova, P., Renard, J. B., Olafsson, H., Vignelles, D., Berthet, G., Verdier, N., and Duverger, V.: Vertical distribution of aerosols in dust storms during the Arctic winter, Sci. Rep., 9, 16122, https://doi.org/10.1038/s41598-019-51764-y, 2019. a
DeMott, P.: Quantitative descriptions of ice formation mechanisms of silver iodide-type aerosols, Atmos. Res., 38, 63–99, https://doi.org/10.1016/0169-8095(94)00088-U, 1995. a, b
DeMott, P. J., Prenni, A. J., Liu, X., Kreidenweis, S. M., Petters, M. D., Twohy, C. H., Richardson, M. S., Eidhammer, T., and Rogers, D. C.: Predicting global atmospheric ice nuclei distributions and their impacts on climate, P. Natl. Acad. Sci. USA, 107, 11217–11222, https://doi.org/10.1073/pnas.0910818107, 2010. a, b, c, d, e, f, g
DeMott, P. J., Prenni, A. J., McMeeking, G. R., Sullivan, R. C., Petters, M. D., Tobo, Y., Niemand, M., Möhler, O., Snider, J. R., Wang, Z., and Kreidenweis, S. M.: Integrating laboratory and field data to quantify the immersion freezing ice nucleation activity of mineral dust particles, Atmos. Chem. Phys., 15, 393–409, https://doi.org/10.5194/acp-15-393-2015, 2015. a
DeMott, P. J., Hill, T. C. J., McCluskey, C. S., Prather, K. A., Collins, D. B., Sullivan, R. C., Ruppel, M. J., Mason, R. H., Irish, V. E., Lee, T., Hwang, C. Y., Rhee, T. S., Snider, J. R., McMeeking, G. R., Dhaniyala, S., Lewis, E. R., Wentzell, J. J. B., Abbatt, J., Lee, C., Sultana, C. M., Ault, A. P., Axson, J. L., Martinez, M. D., Venero, I., Santos-Figueroa, G., Stokes, M. D., Deane, G. B., Mayol-Bracero, O. L., Grassian, V. H., Bertram, T. H., Bertram, A. K., Moffett, B. F., and Franc, G. D.: Sea spray aerosol as a unique source of ice nucleating particles, P. Natl. Acad. Sci. USA, 113, 5797–5803, https://doi.org/10.1073/pnas.1514034112, 2016. a, b, c, d
DeMott, P. J., Möhler, O., Cziczo, D. J., Hiranuma, N., Petters, M. D., Petters, S. S., Belosi, F., Bingemer, H. G., Brooks, S. D., Budke, C., Burkert-Kohn, M., Collier, K. N., Danielczok, A., Eppers, O., Felgitsch, L., Garimella, S., Grothe, H., Herenz, P., Hill, T. C. J., Höhler, K., Kanji, Z. A., Kiselev, A., Koop, T., Kristensen, T. B., Krüger, K., Kulkarni, G., Levin, E. J. T., Murray, B. J., Nicosia, A., O'Sullivan, D., Peckhaus, A., Polen, M. J., Price, H. C., Reicher, N., Rothenberg, D. A., Rudich, Y., Santachiara, G., Schiebel, T., Schrod, J., Seifried, T. M., Stratmann, F., Sullivan, R. C., Suski, K. J., Szakáll, M., Taylor, H. P., Ullrich, R., Vergara-Temprado, J., Wagner, R., Whale, T. F., Weber, D., Welti, A., Wilson, T. W., Wolf, M. J., and Zenker, J.: The Fifth International Workshop on Ice Nucleation phase 2 (FIN-02): laboratory intercomparison of ice nucleation measurements, Atmos. Meas. Tech., 11, 6231–6257, https://doi.org/10.5194/amt-11-6231-2018, 2018. a, b
Duce, R. A., Unni, C. K., Ray, B. J., Prospero, J. M., and Merrill, J. T.: Long-Range Atmospheric Transport of Soil Dust from Asia to the Tropical North Pacific: Temporal Variability, Science, 209, 1522–1524, http://www.jstor.org/stable/1684974, 1980. a
FAO, IIASA, ISRIC, ISSCAS, and JRC: Harmonized World Soil Database (version 1.2), Food and Agriculture Organization of the United Nations (FAO), International Institute for Applied Systems Analysis (IIASA), ISRIC – World Soil Information, Institute of Soil Science – Chinese Academy of Sciences (ISSCAS), Joint Research Centre of the European Commission (JRC), Laxenburg, Austria, https://doi.org/10.4060/cc3823en, 2012. a
Fröhlich-Nowoisky, J., Hill, T. C. J., Pummer, B. G., Yordanova, P., Franc, G. D., and Pöschl, U.: Ice nucleation activity in the widespread soil fungus Mortierella alpina, Biogeosciences, 12, 1057–1071, https://doi.org/10.5194/bg-12-1057-2015, 2015. a, b
Glaccum, R. A. and Prospero, J. M.: Saharan aerosols over the tropical North Atlantic – Mineralogy, Mar. Geol., 37, 295–321, https://doi.org/10.1016/0025-3227(80)90107-3, 1980. a, b
Gong, X., Radenz, M., Wex, H., Seifert, P., Ataei, F., Henning, S., Baars, H., Barja, B., Ansmann, A., and Stratmann, F.: Significant continental source of ice-nucleating particles at the tip of Chile's southernmost Patagonia region, Atmos. Chem. Phys., 22, 10505–10525, https://doi.org/10.5194/acp-22-10505-2022, 2022. a
Groot Zwaaftink, C. D., Grythe, H., Skov, H., and Stohl, A.: Substantial contribution of northern high-latitude sources to mineral dust in the Arctic, J. Geophys. Res.-Atmos., 121, 13678–13697, https://doi.org/10.1002/2016jd025482, 2016. a
Groot Zwaaftink, C. D., Arnalds, Ó., Dagsson-Waldhauserova, P., Eckhardt, S., Prospero, J. M., and Stohl, A.: Temporal and spatial variability of Icelandic dust emissions and atmospheric transport, Atmos. Chem. Phys., 17, 10865–10878, https://doi.org/10.5194/acp-17-10865-2017, 2017. a
Haga, D. I., Iannone, R., Wheeler, M. J., Mason, R., Polishchuk, E. A., Fetch Jr., T., van der Kamp, B. J., McKendry, I. G., and Bertram, A. K.: Ice nucleation properties of rust and bunt fungal spores and their transport to high altitudes, where they can cause heterogeneous freezing, J. Geophys. Res.-Atmos., 118, 7260–7272, https://doi.org/10.1002/jgrd.50556, 2013. a
Hamilton, D. S., Scanza, R. A., Feng, Y., Guinness, J., Kok, J. F., Li, L., Liu, X., Rathod, S. D., Wan, J. S., Wu, M., and Mahowald, N. M.: Improved methodologies for Earth system modelling of atmospheric soluble iron and observation comparisons using the Mechanism of Intermediate complexity for Modelling Iron (MIMI v1.0), Geosci. Model Dev., 12, 3835–3862, https://doi.org/10.5194/gmd-12-3835-2019, 2019. a
Harrison, A. D., Whale, T. F., Carpenter, M. A., Holden, M. A., Neve, L., O'Sullivan, D., Vergara Temprado, J., and Murray, B. J.: Not all feldspars are equal: a survey of ice nucleating properties across the feldspar group of minerals, Atmos. Chem. Phys., 16, 10927–10940, https://doi.org/10.5194/acp-16-10927-2016, 2016. a, b, c, d
Harrison, A. D., Lever, K., Sanchez-Marroquin, A., Holden, M. A., Whale, T. F., Tarn, M. D., McQuaid, J. B., and Murray, B. J.: The ice-nucleating ability of quartz immersed in water and its atmospheric importance compared to K-feldspar, Atmos. Chem. Phys., 19, 11343–11361, https://doi.org/10.5194/acp-19-11343-2019, 2019. a, b, c, d, e, f, g, h, i, j, k, l, m, n, o, p, q, r, s, t, u, v, w, x, y
Harrison, A. D., O'Sullivan, D., Adams, M. P., Porter, G. C. E., Blades, E., Brathwaite, C., Chewitt-Lucas, R., Gaston, C., Hawker, R., Krüger, O. O., Neve, L., Pöhlker, M. L., Pöhlker, C., Pöschl, U., Sanchez-Marroquin, A., Sealy, A., Sealy, P., Tarn, M. D., Whitehall, S., McQuaid, J. B., Carslaw, K. S., Prospero, J. M., and Murray, B. J.: The ice-nucleating activity of African mineral dust in the Caribbean boundary layer, Atmos. Chem. Phys., 22, 9663–9680, https://doi.org/10.5194/acp-22-9663-2022, 2022. a, b, c, d, e, f, g, h, i, j, k
Hawker, R. E., Miltenberger, A. K., Wilkinson, J. M., Hill, A. A., Shipway, B. J., Cui, Z., Cotton, R. J., Carslaw, K. S., Field, P. R., and Murray, B. J.: The temperature dependence of ice-nucleating particle concentrations affects the radiative properties of tropical convective cloud systems, Atmos. Chem. Phys., 21, 5439–5461, https://doi.org/10.5194/acp-21-5439-2021, 2021. a, b, c, d, e
He, J., Zhang, Y., Glotfelty, T., He, R., Bennartz, R., Rausch, J., and Sartelet, K.: Decadal simulation and comprehensive evaluation of CESM/CAM5.1 with advanced chemistry, aerosol microphysics, and aerosol-cloud interactions, J. Adv. Model. Earth Sy., 7, 110–141, https://doi.org/10.1002/2014MS000360, 2015. a
Herbert, R. J.: Dataset for manuscript “Gaps in our understanding of ice-nucleating particle sources exposed by global simulation of the UK climate model”, Zenodo [data set], https://doi.org/10.5281/zenodo.11186250, 2024. a
Herbert, R. J., Murray, B. J., Whale, T. F., Dobbie, S. J., and Atkinson, J. D.: Representing time-dependent freezing behaviour in immersion mode ice nucleation, Atmos. Chem. Phys., 14, 8501–8520, https://doi.org/10.5194/acp-14-8501-2014, 2014. a
Herbert, R. J., Murray, B. J., Dobbie, S. J., and Koop, T.: Sensitivity of liquid clouds to homogenous freezing parameterizations, Geophys. Res. Lett., 42, 1599–1605, https://doi.org/10.1002/2014GL062729, 2015. a
Hill, T. C. J., DeMott, P. J., Tobo, Y., Fröhlich-Nowoisky, J., Moffett, B. F., Franc, G. D., and Kreidenweis, S. M.: Sources of organic ice nucleating particles in soils, Atmos. Chem. Phys., 16, 7195–7211, https://doi.org/10.5194/acp-16-7195-2016, 2016. a
Hoesly, R. M., Smith, S. J., Feng, L., Klimont, Z., Janssens-Maenhout, G., Pitkanen, T., Seibert, J. J., Vu, L., Andres, R. J., Bolt, R. M., Bond, T. C., Dawidowski, L., Kholod, N., Kurokawa, J.-I., Li, M., Liu, L., Lu, Z., Moura, M. C. P., O'Rourke, P. R., and Zhang, Q.: Historical (1750–2014) anthropogenic emissions of reactive gases and aerosols from the Community Emissions Data System (CEDS), Geosci. Model Dev., 11, 369–408, https://doi.org/10.5194/gmd-11-369-2018, 2018. a
Holden, M. A., Whale, T. F., Tarn, M. D., O'Sullivan, D., Walshaw, R. D., Murray, B. J., Meldrum, F. C., and Christenson, H. K.: High-speed imaging of ice nucleation in water proves the existence of active sites, Sci. Adv., 5, eaav4316, https://doi.org/10.1126/sciadv.aav4316, 2019. a
Holden, M. A., Campbell, J. M., Meldrum, F. C., Murray, B. J., and Christenson, H. K.: Active sites for ice nucleation differ depending on nucleation mode, P. Natl. Acad. Sci. USA, 118, https://doi.org/10.1073/pnas.2022859118, 2021. a
Hoose, C., Kristjánsson, J. E., Chen, J.-P., and Hazra, A.: A Classical-Theory-Based Parameterization of Heterogeneous Ice Nucleation by Mineral Dust, Soot, and Biological Particles in a Global Climate Model, J. Atmos. Sci., 67, 2483–2503, https://doi.org/10.1175/2010JAS3425.1, 2010. a, b, c
Huang, W. T. K., Ickes, L., Tegen, I., Rinaldi, M., Ceburnis, D., and Lohmann, U.: Global relevance of marine organic aerosol as ice nucleating particles, Atmos. Chem. Phys., 18, 11423–11445, https://doi.org/10.5194/acp-18-11423-2018, 2018. a, b
Hummel, M., Hoose, C., Pummer, B., Schaupp, C., Fröhlich-Nowoisky, J., and Möhler, O.: Simulating the influence of primary biological aerosol particles on clouds by heterogeneous ice nucleation, Atmos. Chem. Phys., 18, 15437–15450, https://doi.org/10.5194/acp-18-15437-2018, 2018. a, b
Huneeus, N., Schulz, M., Balkanski, Y., Griesfeller, J., Prospero, J., Kinne, S., Bauer, S., Boucher, O., Chin, M., Dentener, F., Diehl, T., Easter, R., Fillmore, D., Ghan, S., Ginoux, P., Grini, A., Horowitz, L., Koch, D., Krol, M. C., Landing, W., Liu, X., Mahowald, N., Miller, R., Morcrette, J.-J., Myhre, G., Penner, J., Perlwitz, J., Stier, P., Takemura, T., and Zender, C. S.: Global dust model intercomparison in AeroCom phase I, Atmos. Chem. Phys., 11, 7781–7816, https://doi.org/10.5194/acp-11-7781-2011, 2011. a, b
Ickes, L., Porter, G. C. E., Wagner, R., Adams, M. P., Bierbauer, S., Bertram, A. K., Bilde, M., Christiansen, S., Ekman, A. M. L., Gorokhova, E., Höhler, K., Kiselev, A. A., Leck, C., Möhler, O., Murray, B. J., Schiebel, T., Ullrich, R., and Salter, M. E.: The ice-nucleating activity of Arctic sea surface microlayer samples and marine algal cultures, Atmos. Chem. Phys., 20, 11089–11117, https://doi.org/10.5194/acp-20-11089-2020, 2020. a
Irish, V. E., Hanna, S. J., Willis, M. D., China, S., Thomas, J. L., Wentzell, J. J. B., Cirisan, A., Si, M., Leaitch, W. R., Murphy, J. G., Abbatt, J. P. D., Laskin, A., Girard, E., and Bertram, A. K.: Ice nucleating particles in the marine boundary layer in the Canadian Arctic during summer 2014, Atmos. Chem. Phys., 19, 1027–1039, https://doi.org/10.5194/acp-19-1027-2019, 2019. a, b, c
Jensen, E. J., Diskin, G., Lawson, R. P., Lance, S., Bui, T. P., Hlavka, D., McGill, M., Pfister, L., Toon, O. B., and Gao, R.: Ice nucleation and dehydration in the Tropical Tropopause Layer, P. Natl. Acad. Sci. USA, 110, 2041–2046, https://doi.org/10.1073/pnas.1217104110, 2013. a
Jeong, G. Y.: Microanalysis and mineralogy of Asian and Saharan dust, Journal of Analytical Science and Technology, 15, 10, https://doi.org/10.1186/s40543-024-00425-5, 2024. a, b
Jiang, H., Yin, Y., Chen, K., Chen, Q., He, C., and Sun, L.: The measurement of ice nucleating particles at Tai'an city in East China, Atmos. Res., 232, 104684, https://doi.org/10.1016/j.atmosres.2019.104684, 2020. a, b
Kandler, K., Schütz, L., Jäckel, S., Lieke, K., Emmel, C., Müller-Ebert, D., Ebert, M., Scheuvens, D., Schladitz, A., Šegvić, B., Wiedensohler, A., and Weinbruch, S.: Ground-based off-line aerosol measurements at Praia, Cape Verde, during the Saharan Mineral Dust Experiment: microphysical properties and mineralogy, Tellus B, 63, 459–474, https://doi.org/10.1111/j.1600-0889.2011.00546.x, 2011. a
Kandler, K., Schneiders, K., Ebert, M., Hartmann, M., Weinbruch, S., Prass, M., and Pöhlker, C.: Composition and mixing state of atmospheric aerosols determined by electron microscopy: method development and application to aged Saharan dust deposition in the Caribbean boundary layer, Atmos. Chem. Phys., 18, 13429–13455, https://doi.org/10.5194/acp-18-13429-2018, 2018. a
Kanji, Z. A., Ladino, L. A., Wex, H., Boose, Y., Burkert-Kohn, M., Cziczo, D. J., and Krämer, M.: Overview of Ice Nucleating Particles, Meteor. Mon., 58, 1.1–1.33, https://doi.org/10.1175/AMSMONOGRAPHS-D-16-0006.1, 2017. a, b
Kanji, Z. A., Welti, A., Corbin, J. C., and Mensah, A. A.: Black Carbon Particles Do Not Matter for Immersion Mode Ice Nucleation, Geophys. Res. Lett., 47, e2019GL086764, https://doi.org/10.1029/2019GL086764, 2020. a, b, c, d
Kashchiev, D.: Nucleation, Elsevier, ISBN 978-0-08-053783-2, 2000. a
Kavan, J., Ondruch, J., Nývlt, D., Hrbáček, F., Carrivick, J. L., and Láska, K.: Seasonal hydrological and suspended sediment transport dynamics in proglacial streams, James Ross Island, Antarctica, Geogr. Ann. A, 99, 38–55, https://doi.org/10.1080/04353676.2016.1257914, 2017. a
Kavan, J., Dagsson-Waldhauserova, P., Renard, J. B., Láska, K., and Ambrožová, K.: Aerosol Concentrations in Relationship to Local Atmospheric Conditions on James Ross Island, Antarctica, Front. Earth Sci., 6, 207, https://doi.org/10.3389/feart.2018.00207, 2018. a
Kawai, K., Matsui, H., and Tobo, Y.: Dominant Role of Arctic Dust With High Ice Nucleating Ability in the Arctic Lower Troposphere, Geophys. Res. Lett., 50, e2022GL102470, https://doi.org/10.1029/2022GL102470, 2023. a, b, c, d, e
Knopf, D. A., Alpert, P. A., Zipori, A., Reicher, N., and Rudich, Y.: Stochastic nucleation processes and substrate abundance explain time-dependent freezing in supercooled droplets, npj Climate and Atmospheric Science, 3, 1–9, https://doi.org/10.1038/s41612-020-0106-4, 2020. a
Kok, J. F., Mahowald, N. M., Fratini, G., Gillies, J. A., Ishizuka, M., Leys, J. F., Mikami, M., Park, M.-S., Park, S.-U., Van Pelt, R. S., and Zobeck, T. M.: An improved dust emission model – Part 1: Model description and comparison against measurements, Atmos. Chem. Phys., 14, 13023–13041, https://doi.org/10.5194/acp-14-13023-2014, 2014. a, b
Kok, J. F., Adebiyi, A. A., Albani, S., Balkanski, Y., Checa-Garcia, R., Chin, M., Colarco, P. R., Hamilton, D. S., Huang, Y., Ito, A., Klose, M., Li, L., Mahowald, N. M., Miller, R. L., Obiso, V., Pérez García-Pando, C., Rocha-Lima, A., and Wan, J. S.: Contribution of the world's main dust source regions to the global cycle of desert dust, Atmos. Chem. Phys., 21, 8169–8193, https://doi.org/10.5194/acp-21-8169-2021, 2021. a
Koop, T. and Murray, B. J.: A physically constrained classical description of the homogeneous nucleation of ice in water, J. Chem. Phys., 145, 211915, https://doi.org/10.1063/1.4962355, 2016. a
Korolev, A., McFarquhar, G., Field, P. R., Franklin, C., Lawson, P., Wang, Z., Williams, E., Abel, S. J., Axisa, D., Borrmann, S., Crosier, J., Fugal, J., Krämer, M., Lohmann, U., Schlenczek, O., Schnaiter, M., and Wendisch, M.: Mixed-Phase Clouds: Progress and Challenges, Meteor. Mon., 58, 5.1–5.50, https://doi.org/10.1175/AMSMONOGRAPHS-D-17-0001.1, 2017. a, b
Kumar, A., Marcolli, C., and Peter, T.: Ice nucleation activity of silicates and aluminosilicates in pure water and aqueous solutions – Part 2: Quartz and amorphous silica, Atmos. Chem. Phys., 19, 6035–6058, https://doi.org/10.5194/acp-19-6035-2019, 2019. a
Leadbetter, S. J., Hort, M. C., von Löwis, S., Weber, K., and Witham, C. S.: Modeling the resuspension of ash deposited during the eruption of Eyjafjallajökull in spring 2010, J. Geophys. Res.-Atmos., 117, D00U10, https://doi.org/10.1029/2011jd016802, 2012. a
Liu, X., Shi, X., Zhang, K., Jensen, E. J., Gettelman, A., Barahona, D., Nenes, A., and Lawson, P.: Sensitivity studies of dust ice nuclei effect on cirrus clouds with the Community Atmosphere Model CAM5, Atmos. Chem. Phys., 12, 12061–12079, https://doi.org/10.5194/acp-12-12061-2012, 2012. a
Lohmann, U. and Diehl, K.: Sensitivity Studies of the Importance of Dust Ice Nuclei for the Indirect Aerosol Effect on Stratiform Mixed-Phase Clouds, J. Atmos. Sci., 63, 968–982, https://doi.org/10.1175/JAS3662.1, 2006. a
Mangan, T. P., Atkinson, J. D., Neuberg, J. W., O’Sullivan, D., Wilson, T. W., Whale, T. F., Neve, L., Umo, N. S., Malkin, T. L., and Murray, B. J.: Heterogeneous Ice Nucleation by Soufriere Hills Volcanic Ash Immersed in Water Droplets, Plos One, 12, 1–11, https://doi.org/10.1371/journal.pone.0169720, 2017. a
Mann, G. W., Carslaw, K. S., Spracklen, D. V., Ridley, D. A., Manktelow, P. T., Chipperfield, M. P., Pickering, S. J., and Johnson, C. E.: Description and evaluation of GLOMAP-mode: a modal global aerosol microphysics model for the UKCA composition-climate model, Geosci. Model Dev., 3, 519–551, https://doi.org/10.5194/gmd-3-519-2010, 2010. a, b, c
Mason, R. H., Si, M., Chou, C., Irish, V. E., Dickie, R., Elizondo, P., Wong, R., Brintnell, M., Elsasser, M., Lassar, W. M., Pierce, K. M., Leaitch, W. R., MacDonald, A. M., Platt, A., Toom-Sauntry, D., Sarda-Estève, R., Schiller, C. L., Suski, K. J., Hill, T. C. J., Abbatt, J. P. D., Huffman, J. A., DeMott, P. J., and Bertram, A. K.: Size-resolved measurements of ice-nucleating particles at six locations in North America and one in Europe, Atmos. Chem. Phys., 16, 1637–1651, https://doi.org/10.5194/acp-16-1637-2016, 2016. a, b, c, d, e, f
Maters, E. C., Dingwell, D. B., Cimarelli, C., Müller, D., Whale, T. F., and Murray, B. J.: The importance of crystalline phases in ice nucleation by volcanic ash, Atmos. Chem. Phys., 19, 5451–5465, https://doi.org/10.5194/acp-19-5451-2019, 2019. a
Maters, E. C., Cimarelli, C., Casas, A. S., Dingwell, D. B., and Murray, B. J.: Volcanic ash ice-nucleating activity can be enhanced or depressed by ash-gas interaction in the eruption plume, Earth Planet. Sc. Lett., 551, 116587, https://doi.org/10.1016/j.epsl.2020.116587, 2020. a
McCluskey, C. S., Hill, T. C. J., Humphries, R. S., Rauker, A. M., Moreau, S., Strutton, P. G., Chambers, S. D., Williams, A. G., McRobert, I., Ward, J., Keywood, M. D., Harnwell, J., Ponsonby, W., Loh, Z. M., Krummel, P. B., Protat, A., Kreidenweis, S. M., and DeMott, P. J.: Observations of Ice Nucleating Particles Over Southern Ocean Waters, Geophys. Res. Lett., 45, 11989–11997, https://doi.org/10.1029/2018GL079981, 2018a. a, b, c, d, e, f, g
McCluskey, C. S., Ovadnevaite, J., Rinaldi, M., Atkinson, J., Belosi, F., Ceburnis, D., Marullo, S., Hill, T. C. J., Lohmann, U., Kanji, Z. A., O'Dowd, C., Kreidenweis, S. M., and DeMott, P. J.: Marine and Terrestrial Organic Ice-Nucleating Particles in Pristine Marine to Continentally Influenced Northeast Atlantic Air Masses, J. Geophys. Res.-Atmos., 123, 6196–6212, https://doi.org/10.1029/2017JD028033, 2018b. a, b, c, d, e, f, g
McCluskey, C. S., DeMott, P. J., Ma, P.-L., and Burrows, S. M.: Numerical Representations of Marine Ice-Nucleating Particles in Remote Marine Environments Evaluated Against Observations, Geophys. Res. Lett., 46, 7838–7847, https://doi.org/10.1029/2018GL081861, 2019. a, b, c, d, e, f, g
McCluskey, C. S., Gettelman, A., Bardeen, C. G., DeMott, P. J., Moore, K. A., Kreidenweis, S. M., Hill, T. C. J., Barry, K. R., Twohy, C. H., Toohey, D. W., Rainwater, B., Jensen, J. B., Reeves, J. M., Alexander, S. P., and McFarquhar, G. M.: Simulating Southern Ocean Aerosol and Ice Nucleating Particles in the Community Earth System Model Version 2, J. Geophys. Res.-Atmos., 128, e2022JD036955, https://doi.org/10.1029/2022JD036955, 2023. a, b
McConnell, J. R., Aristarain, A. J., Banta, J. R., Edwards, P. R., and Simões, J. C.: 20th-Century doubling in dust archived in an Antarctic Peninsula ice core parallels climate change and desertification in South America, P. Natl. Acad. Sci. USA, 104, 5743–5748, https://doi.org/10.1073/pnas.0607657104, 2007. a
Meinander, O., Dagsson-Waldhauserova, P., Amosov, P., Aseyeva, E., Atkins, C., Baklanov, A., Baldo, C., Barr, S. L., Barzycka, B., Benning, L. G., Cvetkovic, B., Enchilik, P., Frolov, D., Gassó, S., Kandler, K., Kasimov, N., Kavan, J., King, J., Koroleva, T., Krupskaya, V., Kulmala, M., Kusiak, M., Lappalainen, H. K., Laska, M., Lasne, J., Lewandowski, M., Luks, B., McQuaid, J. B., Moroni, B., Murray, B., Möhler, O., Nawrot, A., Nickovic, S., O’Neill, N. T., Pejanovic, G., Popovicheva, O., Ranjbar, K., Romanias, M., Samonova, O., Sanchez-Marroquin, A., Schepanski, K., Semenkov, I., Sharapova, A., Shevnina, E., Shi, Z., Sofiev, M., Thevenet, F., Thorsteinsson, T., Timofeev, M., Umo, N. S., Uppstu, A., Urupina, D., Varga, G., Werner, T., Arnalds, O., and Vukovic Vimic, A.: Newly identified climatically and environmentally significant high-latitude dust sources, Atmos. Chem. Phys., 22, 11889–11930, https://doi.org/10.5194/acp-22-11889-2022, 2022. a, b, c, d, e, f
Meyers, M. P., DeMott, P. J., and Cotton, W. R.: New Primary Ice-Nucleation Parameterizations in an Explicit Cloud Model, J. Appl. Meteorol. Clim., 31, 708–721, https://doi.org/10.1175/1520-0450(1992)031<0708:NPINPI>2.0.CO;2, 1992. a
Minasny, B., McBratney, A. B., Malone, B. P., Lacoste, M., and Walter, C.: Quantitatively Predicting Soil Carbon Across Landscapes, Springer International Publishing, Cham, 45–57, ISBN 978-3-319-04084-4, https://doi.org/10.1007/978-3-319-04084-4_5, 2014. a
Minasny, B., Malone, B. P., McBratney, A. B., Angers, D. A., Arrouays, D., Chambers, A., Chaplot, V., Chen, Z.-S., Cheng, K., Das, B. S., Field, D. J., Gimona, A., Hedley, C. B., Hong, S. Y., Mandal, B., Marchant, B. P., Martin, M., McConkey, B. G., Mulder, V. L., O'Rourke, S., de Forges, A. C. R., Odeh, I., Padarian, J., Paustian, K., Pan, G., Poggio, L., Savin, I., Stolbovoy, V., Stockmann, U., Sulaeman, Y., Tsui, C.-C., Vågen, T.-G., van Wesemael, B., and Winowiecki, L.: Soil carbon 4 per mille, Geoderma, 292, 59–86, https://doi.org/10.1016/j.geoderma.2017.01.002, 2017. a
Mingari, L., Folch, A., Dominguez, L., and Bonadonna, C.: Volcanic Ash Resuspension in Patagonia: Numerical Simulations and Observations, Atmosphere, 11, 977, https://doi.org/10.3390/atmos11090977, 2020. a
Möhler, O., Adams, M., Lacher, L., Vogel, F., Nadolny, J., Ullrich, R., Boffo, C., Pfeuffer, T., Hobl, A., Weiß, M., Vepuri, H. S. K., Hiranuma, N., and Murray, B. J.: The Portable Ice Nucleation Experiment (PINE): a new online instrument for laboratory studies and automated long-term field observations of ice-nucleating particles, Atmos. Meas. Tech., 14, 1143–1166, https://doi.org/10.5194/amt-14-1143-2021, 2021. a
Morris, C. E., Conen, F., Alex Huffman, J., Phillips, V., Pöschl, U., and Sands, D. C.: Bioprecipitation: a feedback cycle linking Earth history, ecosystem dynamics and land use through biological ice nucleators in the atmosphere, Glob. Change Biol., 20, 341–351, https://doi.org/10.1111/gcb.12447, 2014. a, b
Morrison, H., van Lier-Walqui, M., Fridlind, A. M., Grabowski, W. W., Harrington, J. Y., Hoose, C., Korolev, A., Kumjian, M. R., Milbrandt, J. A., Pawlowska, H., Posselt, D. J., Prat, O. P., Reimel, K. J., Shima, S.-I., van Diedenhoven, B., and Xue, L.: Confronting the Challenge of Modeling Cloud and Precipitation Microphysics, J. Adv. Model. Earth Sy., 12, e2019MS001689, https://doi.org/10.1029/2019MS001689, 2020. a
Mulcahy, J. P., Jones, C., Sellar, A., Johnson, B., Boutle, I. A., Jones, A., Andrews, T., Rumbold, S. T., Mollard, J., Bellouin, N., Johnson, C. E., Williams, K. D., Grosvenor, D. P., and McCoy, D. T.: Improved Aerosol Processes and Effective Radiative Forcing in HadGEM3 and UKESM1, J. Adv. Model. Earth Sy., 10, 2786–2805, https://doi.org/10.1029/2018MS001464, 2018. a
Mulcahy, J. P., Johnson, C., Jones, C. G., Povey, A. C., Scott, C. E., Sellar, A., Turnock, S. T., Woodhouse, M. T., Abraham, N. L., Andrews, M. B., Bellouin, N., Browse, J., Carslaw, K. S., Dalvi, M., Folberth, G. A., Glover, M., Grosvenor, D. P., Hardacre, C., Hill, R., Johnson, B., Jones, A., Kipling, Z., Mann, G., Mollard, J., O'Connor, F. M., Palmiéri, J., Reddington, C., Rumbold, S. T., Richardson, M., Schutgens, N. A. J., Stier, P., Stringer, M., Tang, Y., Walton, J., Woodward, S., and Yool, A.: Description and evaluation of aerosol in UKESM1 and HadGEM3-GC3.1 CMIP6 historical simulations, Geosci. Model Dev., 13, 6383–6423, https://doi.org/10.5194/gmd-13-6383-2020, 2020. a, b, c, d, e, f, g, h, i, j, k
Murray, B. J. and Liu, X.: Chapter 15 - Ice-nucleating particles and their effects on clouds and radiation, in: Aerosols and Climate, edited by: Carslaw, K. S., Elsevier, 619–649, ISBN 978-0-12-819766-0, https://doi.org/10.1016/B978-0-12-819766-0.00014-6, 2022. a
Murray, B. J., O'Sullivan, D., Atkinson, J. D., and Webb, M. E.: Ice nucleation by particles immersed in supercooled cloud droplets, Chem. Soc. Rev., 41, 6519–6554, https://doi.org/10.1039/C2CS35200A, 2012. a, b, c, d
Murray, B. J., Carslaw, K. S., and Field, P. R.: Opinion: Cloud-phase climate feedback and the importance of ice-nucleating particles, Atmos. Chem. Phys., 21, 665–679, https://doi.org/10.5194/acp-21-665-2021, 2021. a, b
Murray, K. A., Kinney, N. L. H., Griffiths, C. A., Hasan, M., Gibson, M. I., and Whale, T. F.: Pollen derived macromolecules serve as a new class of ice-nucleating cryoprotectants, Sci. Rep., 12, 12295, https://doi.org/10.1038/s41598-022-15545-4, 2022. a, b
Nakamae, K. and Shiotani, M.: Interannual variability in Saharan dust over the North Atlantic Ocean and its relation to meteorological fields during northern winter, Atmos. Res., 122, 336–346, https://doi.org/10.1016/j.atmosres.2012.09.012, 2013. a
Neff, P. D. and Bertler, N. A. N.: Trajectory modeling of modern dust transport to the Southern Ocean and Antarctica, J. Geophys. Res.-Atmos., 120, 9303–9322, https://doi.org/10.1002/2015JD023304, 2015. a
Nickovic, S., Vukovic, A., Vujadinovic, M., Djurdjevic, V., and Pejanovic, G.: Technical Note: High-resolution mineralogical database of dust-productive soils for atmospheric dust modeling, Atmos. Chem. Phys., 12, 845–855, https://doi.org/10.5194/acp-12-845-2012, 2012. a, b, c, d
Niemand, M., Möhler, O., Vogel, B., Vogel, H., Hoose, C., Connolly, P., Klein, H., Bingemer, H., DeMott, P., Skrotzki, J., and Leisner, T.: A Particle-Surface-Area-Based Parameterization of Immersion Freezing on Desert Dust Particles, J. Atmos. Sci., 69, 3077–3092, https://doi.org/10.1175/JAS-D-11-0249.1, 2012. a
O'Sullivan, D., Murray, B. J., Malkin, T. L., Whale, T. F., Umo, N. S., Atkinson, J. D., Price, H. C., Baustian, K. J., Browse, J., and Webb, M. E.: Ice nucleation by fertile soil dusts: relative importance of mineral and biogenic components, Atmos. Chem. Phys., 14, 1853–1867, https://doi.org/10.5194/acp-14-1853-2014, 2014. a, b, c, d, e, f, g, h, i, j, k, l, m, n, o, p
O'Sullivan, D., Murray, B. J., Ross, J. F., and Webb, M. E.: The adsorption of fungal ice-nucleating proteins on mineral dusts: a terrestrial reservoir of atmospheric ice-nucleating particles, Atmos. Chem. Phys., 16, 7879–7887, https://doi.org/10.5194/acp-16-7879-2016, 2016. a, b
O'Sullivan, D., Adams, M. P., Tarn, M. D., Harrison, A. D., Vergara-Temprado, J., Porter, G. C. E., Holden, M. A., Sanchez-Marroquin, A., Carotenuto, F., Whale, T. F., McQuaid, J. B., Walshaw, R., Hedges, D. H. P., Burke, I. T., Cui, Z., and Murray, B. J.: Contributions of biogenic material to the atmospheric ice-nucleating particle population in North Western Europe, Sci. Re., 8, 13821, https://doi.org/10.1038/s41598-018-31981-7, 2018. a
O'Sullivan, D., Murray, B. J., Ross, J. F., Whale, T. F., Price, H. C., Atkinson, J. D., Umo, N. S., and Webb, M. E.: The relevance of nanoscale biological fragments for ice nucleation in clouds, Sci. Rep., 5, 8082, https://doi.org/10.1038/srep08082, 2015. a, b, c, d, e
Palmero, D., Rodríguez, J. M., de Cara, M., Camacho, F., Iglesias, C., and Tello, J. C.: Fungal microbiota from rain water and pathogenicity of Fusarium species isolated from atmospheric dust and rainfall dust, J. Ind. Microbiol. Biot., 38, 13–20, https://doi.org/10.1007/s10295-010-0831-5, 2011. a
Paramonov, M., David, R. O., Kretzschmar, R., and Kanji, Z. A.: A laboratory investigation of the ice nucleation efficiency of three types of mineral and soil dust, Atmos. Chem. Phys., 18, 16515–16536, https://doi.org/10.5194/acp-18-16515-2018, 2018. a
Pereira Freitas, G., Adachi, K., Conen, F., Heslin-Rees, D., Krejci, R., Tobo, Y., Yttri, K. E., and Zieger, P.: Regionally sourced bioaerosols drive high-temperature ice nucleating particles in the Arctic, Nat. Commun., 14, 5997, https://doi.org/10.1038/s41467-023-41696-7, 2023. a
Perlwitz, J. P., Pérez García-Pando, C., and Miller, R. L.: Predicting the mineral composition of dust aerosols – Part 1: Representing key processes, Atmos. Chem. Phys., 15, 11593–11627, https://doi.org/10.5194/acp-15-11593-2015, 2015. a
Pouleur, S., Richard, C., Martin, J.-G., and Antoun, H.: Ice Nucleation Activity in Fusarium acuminatum and Fusarium avenaceum, Appl. Environ. Microb., 58, 2960–2964, https://doi.org/10.1128/aem.58.9.2960-2964.1992, 1992. a
Price, H. C., Baustian, K. J., McQuaid, J. B., Blyth, A., Bower, K. N., Choularton, T., Cotton, R. J., Cui, Z., Field, P. R., Gallagher, M., Hawker, R., Merrington, A., Miltenberger, A., Neely III, R. R., Parker, S. T., Rosenberg, P. D., Taylor, J. W., Trembath, J., Vergara-Temprado, J., Whale, T. F., Wilson, T. W., Young, G., and Murray, B. J.: Atmospheric Ice-Nucleating Particles in the Dusty Tropical Atlantic, J. Geophys. Res.-Atmos., 123, 2175–2193, https://doi.org/10.1002/2017JD027560, 2018. a, b, c, d, e, f
Price, S. P., Bradford, M. A., and Ashton, M. S.: Characterizing Organic Carbon Stocks and Flows in Forest Soils, Springer Netherlands, Dordrecht, 7–30, ISBN 978-94-007-2232-3, https://doi.org/10.1007/978-94-007-2232-3_2, 2012. a
Prospero, J. M., Glaccum, R. A., and Nees, R. T.: Atmospheric transport of soil dust from Africa to South America, Nature, 289, 570–572, https://doi.org/10.1038/289570a0, 1981. a, b
Prospero, J. M., Bullard, J. E., and Hodgkins, R.: High-latitude dust over the North Atlantic: inputs from Icelandic proglacial dust storms, Science, 335, 1078–82, https://doi.org/10.1126/science.1217447, 2012. a, b, c
Pummer, B. G., Bauer, H., Bernardi, J., Bleicher, S., and Grothe, H.: Suspendable macromolecules are responsible for ice nucleation activity of birch and conifer pollen, Atmos. Chem. Phys., 12, 2541–2550, https://doi.org/10.5194/acp-12-2541-2012, 2012. a, b
Rangel-Alvarado, R. B., Nazarenko, Y., and Ariya, P. A.: Snow-borne nanosized particles: Abundance, distribution, composition, and significance in ice nucleation processes, J. Geophys. Res.-Atmos., 120, 11760–11774, https://doi.org/10.1002/2015JD023773, 2015. a
Reicher, N., Segev, L., and Rudich, Y.: The WeIzmann Supercooled Droplets Observation on a Microarray (WISDOM) and application for ambient dust, Atmos. Meas. Tech., 11, 233–248, https://doi.org/10.5194/amt-11-233-2018, 2018. a
Sanchez-Marroquin, A., Arnalds, O., Baustian-Dorsi, K. J., Browse, J., Dagsson-Waldhauserova, P., Harrison, A. D., Maters, E. C., Pringle, K. J., Vergara-Temprado, J., Burke, I. T., McQuaid, J. B., Carslaw, K. S., and Murray, B. J.: Iceland is an episodic source of atmospheric ice-nucleating particles relevant for mixed-phase clouds, Sci. Adv., 6, eaba8137, https://doi.org/10.1126/sciadv.aba8137, 2020. a, b, c, d, e, f, g
Sanchez-Marroquin, A., West, J. S., Burke, I. T., McQuaid, J. B., and Murray, B. J.: Mineral and biological ice-nucleating particles above the South East of the British Isles, Environ. Sci. Atmos., 1, 176–191, https://doi.org/10.1039/D1EA00003A, 2021. a, b
Schill, G. P., Jathar, S. H., Kodros, J. K., Levin, E. J. T., Galang, A. M., Friedman, B., Link, M. F., Farmer, D. K., Pierce, J. R., Kreidenweis, S. M., and DeMott, P. J.: Ice-nucleating particle emissions from photochemically aged diesel and biodiesel exhaust, Geophys. Res. Lett., 43, 5524–5531, https://doi.org/10.1002/2016GL069529, 2016. a, b, c
Schill, G. P., DeMott, P. J., Emerson, E. W., Rauker, A. M. C., Kodros, J. K., Suski, K. J., Hill, T. C. J., Levin, E. J. T., Pierce, J. R., Farmer, D. K., and Kreidenweis, S. M.: The contribution of black carbon to global ice nucleating particle concentrations relevant to mixed-phase clouds, P/ Natl. Acad. Sci. USA, 117, 22705–22711, https://doi.org/10.1073/pnas.2001674117, 2020. a, b, c, d, e, f, g
Schnell, R. and Vali, G.: Biogenic ice nuclei: Part I. Terrestrial and marine sources, J. Atmos. Sci., 33, 1554–1564, 1976. a, b
Schnell, R. C.: Biogenic Sources of Atmospheric Ice Nuclei, University of Wyoming, 1974a. a
Schnell, R. C.: Biogenic and inorganic ice nuclei sources in the drought-stricken areas of the Sahel, Tech. rep., Rockefeller Foundation, New York City, New York, 1974b. a
Sciare, J., Favez, O., Sarda-Estève, R., Oikonomou, K., Cachier, H., and Kazan, V.: Long-term observations of carbonaceous aerosols in the Austral Ocean atmosphere: Evidence of a biogenic marine organic source, J. Geophys. Res.-Atmos., 114, D15302, https://doi.org/10.1029/2009JD011998, 2009. a
Sellar, A. A., Walton, J., Jones, C. G., Wood, R., Abraham, N. L., Andrejczuk, M., Andrews, M. B., Andrews, T., Archibald, A. T., de Mora, L., Dyson, H., Elkington, M., Ellis, R., Florek, P., Good, P., Gohar, L., Haddad, S., Hardiman, S. C., Hogan, E., Iwi, A., Jones, C. D., Johnson, B., Kelley, D. I., Kettleborough, J., Knight, J. R., Köhler, M. O., Kuhlbrodt, T., Liddicoat, S., Linova-Pavlova, I., Mizielinski, M. S., Morgenstern, O., Mulcahy, J., Neininger, E., O'Connor, F. M., Petrie, R., Ridley, J., Rioual, J.-C., Roberts, M., Robertson, E., Rumbold, S., Seddon, J., Shepherd, H., Shim, S., Stephens, A., Teixiera, J. C., Tang, Y., Williams, J., Wiltshire, A., and Griffiths, P. T.: Implementation of U.K. Earth System Models for CMIP6, J. Adv. Model. Earth Sy., 12, e2019MS001946, https://doi.org/10.1029/2019MS001946, 2020. a
Shi, Y. and Liu, X.: Dust Radiative Effects on Climate by Glaciating Mixed-Phase Clouds, Geophys. Res. Lett., 46, 6128–6137, https://doi.org/10.1029/2019GL082504, 2019. a, b
Shi, Y., Liu, X., Wu, M., Zhao, X., Ke, Z., and Brown, H.: Relative importance of high-latitude local and long-range-transported dust for Arctic ice-nucleating particles and impacts on Arctic mixed-phase clouds, Atmos. Chem. Phys., 22, 2909–2935, https://doi.org/10.5194/acp-22-2909-2022, 2022. a, b, c, d, e, f, g, h, i
Si, M., Irish, V. E., Mason, R. H., Vergara-Temprado, J., Hanna, S. J., Ladino, L. A., Yakobi-Hancock, J. D., Schiller, C. L., Wentzell, J. J. B., Abbatt, J. P. D., Carslaw, K. S., Murray, B. J., and Bertram, A. K.: Ice-nucleating ability of aerosol particles and possible sources at three coastal marine sites, Atmos. Chem. Phys., 18, 15669–15685, https://doi.org/10.5194/acp-18-15669-2018, 2018. a, b
Si, M., Evoy, E., Yun, J., Xi, Y., Hanna, S. J., Chivulescu, A., Rawlings, K., Veber, D., Platt, A., Kunkel, D., Hoor, P., Sharma, S., Leaitch, W. R., and Bertram, A. K.: Concentrations, composition, and sources of ice-nucleating particles in the Canadian High Arctic during spring 2016, Atmos. Chem. Phys., 19, 3007–3024, https://doi.org/10.5194/acp-19-3007-2019, 2019. a, b
Sirois, A. and Barrie, L. A.: Arctic lower tropospheric aerosol trends and composition at Alert, Canada: 1980–1995, J. Geophys. Res.-Atmos., 104, 11599–11618, https://doi.org/10.1029/1999JD900077, 1999. a
Spracklen, D. V. and Heald, C. L.: The contribution of fungal spores and bacteria to regional and global aerosol number and ice nucleation immersion freezing rates, Atmos. Chem. Phys., 14, 9051–9059, https://doi.org/10.5194/acp-14-9051-2014, 2014. a, b
Steinke, I., Möhler, O., Kiselev, A., Niemand, M., Saathoff, H., Schnaiter, M., Skrotzki, J., Hoose, C., and Leisner, T.: Ice nucleation properties of fine ash particles from the Eyjafjallajökull eruption in April 2010, Atmos. Chem. Phys., 11, 12945–12958, https://doi.org/10.5194/acp-11-12945-2011, 2011. a
Steinke, I., Funk, R., Busse, J., Iturri, A., Kirchen, S., Leue, M., Möhler, O., Schwartz, T., Schnaiter, M., Sierau, B., Toprak, E., Ullrich, R., Ulrich, A., Hoose, C., and Leisner, T.: Ice nucleation activity of agricultural soil dust aerosols from Mongolia, Argentina, and Germany, J. Geophys. Res.-Atmos., 121, 13559–13576, https://doi.org/10.1002/2016JD025160, 2016. a, b, c, d
Steinke, I., Hiranuma, N., Funk, R., Höhler, K., Tüllmann, N., Umo, N. S., Weidler, P. G., Möhler, O., and Leisner, T.: Complex plant-derived organic aerosol as ice-nucleating particles – more than the sums of their parts?, Atmos. Chem. Phys., 20, 11387–11397, https://doi.org/10.5194/acp-20-11387-2020, 2020. a, b, c
Sun, Y., Zhu, Y., Qi, Y., Chen, L., Mu, J., Shan, Y., Yang, Y., Nie, Y., Liu, P., Cui, C., Zhang, J., Liu, M., Zhang, L., Wang, Y., Wang, X., Tang, M., Wang, W., and Xue, L.: Measurement report: Atmospheric ice nuclei in the Changbai Mountains (2623 m a.s.l.) in northeastern Asia, Atmos. Chem. Phys., 24, 3241–3256, https://doi.org/10.5194/acp-24-3241-2024, 2024. a, b, c
Sze, K. C. H., Wex, H., Hartmann, M., Skov, H., Massling, A., Villanueva, D., and Stratmann, F.: Ice-nucleating particles in northern Greenland: annual cycles, biological contribution and parameterizations, Atmos. Chem. Phys., 23, 4741–4761, https://doi.org/10.5194/acp-23-4741-2023, 2023. a
Tan, I., Storelvmo, T., and Zelinka, M. D.: Observational constraints on mixed-phase clouds imply higher climate sensitivity, Science, 352, 224–227, 2016. a
Tanguy Claquin, Michael Schulz, Y. B.: Modeling the mineralogy of atmospheric dust sources, J. Geophys. Res.-Atmos., 104, 22243–22256, https://doi.org/10.1029/1999JD900416, 1999. a
Tatzelt, C., Henning, S., Welti, A., Baccarini, A., Hartmann, M., Gysel-Beer, M., van Pinxteren, M., Modini, R. L., Schmale, J., and Stratmann, F.: Circum-Antarctic abundance and properties of CCN and INPs, Atmos. Chem. Phys., 22, 9721–9745, https://doi.org/10.5194/acp-22-9721-2022, 2022. a, b, c, d, e, f, g
Tegen, I., Neubauer, D., Ferrachat, S., Siegenthaler-Le Drian, C., Bey, I., Schutgens, N., Stier, P., Watson-Parris, D., Stanelle, T., Schmidt, H., Rast, S., Kokkola, H., Schultz, M., Schroeder, S., Daskalakis, N., Barthel, S., Heinold, B., and Lohmann, U.: The global aerosol–climate model ECHAM6.3–HAM2.3 – Part 1: Aerosol evaluation, Geosci. Model Dev., 12, 1643–1677, https://doi.org/10.5194/gmd-12-1643-2019, 2019. a
Thomson, E. S., Weber, D., Bingemer, H. G., Tuomi, J., Ebert, M., and Pettersson, J. B. C.: Intensification of ice nucleation observed in ocean ship emissions, Sci. Rep., 8, 1111, https://doi.org/10.1038/s41598-018-19297-y, 2018. a
Tian, P., Liu, D., Bi, K., Huang, M., Wu, Y., Hu, K., Li, R., He, H., Ding, D., Hu, Y., Liu, Q., Zhao, D., Qiu, Y., Kong, S., and Xue, H.: Evidence for Anthropogenic Organic Aerosols Contributing to Ice Nucleation, Geophys. Res. Lett., 49, e2022GL099990, https://doi.org/10.1029/2022GL099990, 2022. a, b, c
Tobo, Y., DeMott, P. J., Hill, T. C. J., Prenni, A. J., Swoboda-Colberg, N. G., Franc, G. D., and Kreidenweis, S. M.: Organic matter matters for ice nuclei of agricultural soil origin, Atmos. Chem. Phys., 14, 8521–8531, https://doi.org/10.5194/acp-14-8521-2014, 2014. a, b, c, d, e
Tobo, Y., Adachi, K., DeMott, P. J., Hill, T. C. J., Hamilton, D. S., Mahowald, N. M., Nagatsuka, N., Ohata, S., Uetake, J., Kondo, Y., and Koike, M.: Glacially sourced dust as a potentially significant source of ice nucleating particles, Nat. Geosci., 12, 253–258, https://doi.org/10.1038/s41561-019-0314-x, 2019. a, b, c, d, e, f, g
Tobo, Y., Uetake, J., Matsui, H., Moteki, N., Uji, Y., Iwamoto, Y., Miura, K., and Misumi, R.: Seasonal Trends of Atmospheric Ice Nucleating Particles Over Tokyo, J. Geophys. Res.-Atmos., 125, e2020JD033658, https://doi.org/10.1029/2020JD033658, 2020. a, b, c
Ullrich, R., Hoose, C., Möhler, O., Niemand, M., Wagner, R., Höhler, K., Hiranuma, N., Saathoff, H., and Leisner, T.: A New Ice Nucleation Active Site Parameterization for Desert Dust and Soot, J. Atmos. Sci., 74, 699–717, https://doi.org/10.1175/JAS-D-16-0074.1, 2017. a, b
Umo, N. S., Murray, B. J., Baeza-Romero, M. T., Jones, J. M., Lea-Langton, A. R., Malkin, T. L., O'Sullivan, D., Neve, L., Plane, J. M. C., and Williams, A.: Ice nucleation by combustion ash particles at conditions relevant to mixed-phase clouds, Atmos. Chem. Phys., 15, 5195–5210, https://doi.org/10.5194/acp-15-5195-2015, 2015. a
Vali, G.: Repeatability and randomness in heterogeneous freezing nucleation, Atmos. Chem. Phys., 8, 5017–5031, https://doi.org/10.5194/acp-8-5017-2008, 2008. a, b
Vali, G.: Interpretation of freezing nucleation experiments: singular and stochastic; sites and surfaces, Atmos. Chem. Phys., 14, 5271–5294, https://doi.org/10.5194/acp-14-5271-2014, 2014. a, b
van Marle, M. J. E., Kloster, S., Magi, B. I., Marlon, J. R., Daniau, A.-L., Field, R. D., Arneth, A., Forrest, M., Hantson, S., Kehrwald, N. M., Knorr, W., Lasslop, G., Li, F., Mangeon, S., Yue, C., Kaiser, J. W., and van der Werf, G. R.: Historic global biomass burning emissions for CMIP6 (BB4CMIP) based on merging satellite observations with proxies and fire models (1750–2015), Geosci. Model Dev., 10, 3329–3357, https://doi.org/10.5194/gmd-10-3329-2017, 2017. a
Vergara-Temprado, J., Murray, B. J., Wilson, T. W., O'Sullivan, D., Browse, J., Pringle, K. J., Ardon-Dryer, K., Bertram, A. K., Burrows, S. M., Ceburnis, D., DeMott, P. J., Mason, R. H., O'Dowd, C. D., Rinaldi, M., and Carslaw, K. S.: Contribution of feldspar and marine organic aerosols to global ice nucleating particle concentrations, Atmos. Chem. Phys., 17, 3637–3658, https://doi.org/10.5194/acp-17-3637-2017, 2017. a, b, c, d, e, f, g, h, i, j, k, l, m, n, o, p, q, r, s, t
Vergara-Temprado, J., Holden, M. A., Orton, T. R., O'Sullivan, D., Umo, N. S., Browse, J., Reddington, C., Baeza-Romero, M. T., Jones, J. M., Lea-Langton, A., Williams, A., Carslaw, K. S., and Murray, B. J.: Is Black Carbon an Unimportant Ice-Nucleating Particle in Mixed-Phase Clouds?, J. Geophys. Res.-Atmos., 123, 4273–4283, https://doi.org/10.1002/2017JD027831, 2018a. a, b, c, d, e, f, g, h
Vergara-Temprado, J., Miltenberger, A. K., Furtado, K., Grosvenor, D. P., Shipway, B. J., Hill, A. A., Wilkinson, J. M., Field, P. R., Murray, B. J., and Carslaw, K. S.: Strong control of Southern Ocean cloud reflectivity by ice-nucleating particles, P. Natl. Acad. Sci. USA, 115, 2687–2692, https://doi.org/10.1073/pnas.1721627115, 2018b. a, b, c, d
Vogel, F., Adams, M. P., Lacher, L., Foster, P. B., Porter, G. C. E., Bertozzi, B., Höhler, K., Schneider, J., Schorr, T., Umo, N. S., Nadolny, J., Brasseur, Z., Heikkilä, P., Thomson, E. S., Büttner, N., Daily, M. I., Fösig, R., Harrison, A. D., Keskinen, J., Proske, U., Duplissy, J., Kulmala, M., Petäjä, T., Möhler, O., and Murray, B. J.: Ice-nucleating particles active below −24 °C in a Finnish boreal forest and their relationship to bioaerosols, Atmos. Chem. Phys., 24, 11737–11757, https://doi.org/10.5194/acp-24-11737-2024, 2024. a, b
Walters, D., Baran, A. J., Boutle, I., Brooks, M., Earnshaw, P., Edwards, J., Furtado, K., Hill, P., Lock, A., Manners, J., Morcrette, C., Mulcahy, J., Sanchez, C., Smith, C., Stratton, R., Tennant, W., Tomassini, L., Van Weverberg, K., Vosper, S., Willett, M., Browse, J., Bushell, A., Carslaw, K., Dalvi, M., Essery, R., Gedney, N., Hardiman, S., Johnson, B., Johnson, C., Jones, A., Jones, C., Mann, G., Milton, S., Rumbold, H., Sellar, A., Ujiie, M., Whitall, M., Williams, K., and Zerroukat, M.: The Met Office Unified Model Global Atmosphere 7.0/7.1 and JULES Global Land 7.0 configurations, Geosci. Model Dev., 12, 1909–1963, https://doi.org/10.5194/gmd-12-1909-2019, 2019. a, b, c, d, e
Wex, H., Augustin-Bauditz, S., Boose, Y., Budke, C., Curtius, J., Diehl, K., Dreyer, A., Frank, F., Hartmann, S., Hiranuma, N., Jantsch, E., Kanji, Z. A., Kiselev, A., Koop, T., Möhler, O., Niedermeier, D., Nillius, B., Rösch, M., Rose, D., Schmidt, C., Steinke, I., and Stratmann, F.: Intercomparing different devices for the investigation of ice nucleating particles using Snomax® as test substance, Atmos. Chem. Phys., 15, 1463–1485, https://doi.org/10.5194/acp-15-1463-2015, 2015. a
Wex, H., Huang, L., Zhang, W., Hung, H., Traversi, R., Becagli, S., Sheesley, R. J., Moffett, C. E., Barrett, T. E., Bossi, R., Skov, H., Hünerbein, A., Lubitz, J., Löffler, M., Linke, O., Hartmann, M., Herenz, P., and Stratmann, F.: Annual variability of ice-nucleating particle concentrations at different Arctic locations, Atmos. Chem. Phys., 19, 5293–5311, https://doi.org/10.5194/acp-19-5293-2019, 2019. a, b, c, d, e
Wilbourn, E. K., Lacher, L., Guerrero, C., Vepuri, H. S. K., Höhler, K., Nadolny, J., Pantoya, A. D., Möhler, O., and Hiranuma, N.: Measurement report: A comparison of ground-level ice-nucleating-particle abundance and aerosol properties during autumn at contrasting marine and terrestrial locations, Atmos. Chem. Phys., 24, 5433–5456, https://doi.org/10.5194/acp-24-5433-2024, 2024. a
Wilson, T., Ladino, L., Alpert, P., Breckels, M., Brooks, I., Browse, J., Burrows, S., Carslaw, K., Huffman, J., Judd, C., Kilthau, W., Mason, R., Mcfiggans, G., Miller, L., Nájera, J., Polishchuk, E., Rae, S., Schiller, C., Si, M., and Murray, B.: A marine biogenic source of atmospheric ice-nucleating particles, Nature, 525, 234–238, https://doi.org/10.1038/nature14986, 2015. a, b, c, d, e, f, g
Woodward, S.: Technical Note 87 Mineral Dust in HadGEM 2 March 2011, Tech. rep., Met Office Hadley Centre, 2011. a
Woodward, S., Sellar, A. A., Tang, Y., Stringer, M., Yool, A., Robertson, E., and Wiltshire, A.: The simulation of mineral dust in the United Kingdom Earth System Model UKESM1, Atmos. Chem. Phys., 22, 14503–14528, https://doi.org/10.5194/acp-22-14503-2022, 2022. a
Xi, Y., Xu, C., Downey, A., Stevens, R., Bachelder, J. O., King, J., Hayes, P. L., and Bertram, A. K.: Ice nucleating properties of airborne dust from an actively retreating glacier in Yukon, Canada, Environmental Science: Atmospheres, 2, 714–726, https://doi.org/10.1039/D1EA00101A, 2022. a
Yadav, S., Venezia, R. E., Paerl, R. W., and Petters, M. D.: Characterization of Ice-Nucleating Particles Over Northern India, J. Geophys. Res.-Atmos., 124, 10467–10482, https://doi.org/10.1029/2019JD030702, 2019. a, b
Zelinka, M. D., Myers, T. A., McCoy, D. T., Po-Chedley, S., Caldwell, P. M., Ceppi, P., Klein, S. A., and Taylor, K. E.: Causes of Higher Climate Sensitivity in CMIP6 Models, Geophys. Res. Lett., 47, e2019GL085782, https://doi.org/10.1029/2019GL085782, 2020. a
Zhang, C., Wu, Z., Chen, J., Chen, J., Tang, L., Zhu, W., Pei, X., Chen, S., Tian, P., Guo, S., Zeng, L., Hu, M., and Kanji, Z. A.: Ice-nucleating particles from multiple aerosol sources in the urban environment of Beijing under mixed-phase cloud conditions, Atmos. Chem. Phys., 22, 7539–7556, https://doi.org/10.5194/acp-22-7539-2022, 2022. a, b, c
Zhang, D., Wang, Z., Heymsfield, A., Fan, J., Liu, D., and Zhao, M.: Quantifying the impact of dust on heterogeneous ice generation in midlevel supercooled stratiform clouds, Geophys. Res. Lett., 39, L18805, https://doi.org/10.1029/2012GL052831, 2012. a
Zhao, B., Wang, Y., Gu, Y., Liou, K.-N., Jiang, J. H., Fan, J., Liu, X., Huang, L., and Yung, Y. L.: Ice nucleation by aerosols from anthropogenic pollution, Nat. Geosci., 12, 602–607, https://doi.org/10.1038/s41561-019-0389-4, 2019. a
Zhao, X., Liu, X., Burrows, S. M., and Shi, Y.: Effects of marine organic aerosols as sources of immersion-mode ice-nucleating particles on high-latitude mixed-phase clouds, Atmos. Chem. Phys., 21, 2305–2327, https://doi.org/10.5194/acp-21-2305-2021, 2021. a, b, c, d
- Abstract
- Introduction
- Methods
- Aerosol model evaluation
- The INP model
- Evidence for additional INP types
- Conclusions
- Appendix A: Details of INP measurements
- Code and data availability
- Author contributions
- Competing interests
- Disclaimer
- Acknowledgements
- Financial support
- Review statement
- References
- Supplement
- Abstract
- Introduction
- Methods
- Aerosol model evaluation
- The INP model
- Evidence for additional INP types
- Conclusions
- Appendix A: Details of INP measurements
- Code and data availability
- Author contributions
- Competing interests
- Disclaimer
- Acknowledgements
- Financial support
- Review statement
- References
- Supplement