the Creative Commons Attribution 4.0 License.
the Creative Commons Attribution 4.0 License.
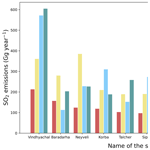
SO2 emissions derived from TROPOMI observations over India using a flux-divergence method with variable lifetimes
Ronald J. van der A
Henk Eskes
Jason E. Williams
Nicolas Theys
Athanasios Tsikerdekis
Pieternel F. Levelt
The rapid development of the economy and the implementation of environmental policies adapted in India have led to fast changes of regional SO2 emissions. We present a monthly SO2 emission inventory for India covering December 2018 to November 2023 based on the Tropospheric Monitoring Instrument (TROPOMI) Level-2 COBRA SO2 dataset, using an improved flux-divergence method and estimated local SO2 lifetime, which includes both its chemical loss and dry deposition. We update the methodology to use the daily CAMS model output estimates of the hydroxyl-radical distribution as well as the measured dry deposition velocity to account for the variability in the tropospheric SO2 lifetime. It is the first effort to derive the local SO2 lifetime for application in the divergence method. The results show the application of the local SO2 lifetime improves the accuracy of SO2 emissions estimation when compared to calculations using a constant lifetime. Our improved flux-divergence method reduced the spreading of the point-source emissions compared to the standard flux-divergence method. Our derived averaged SO2 emissions covering the recent 5 years are about 5.2 Tg yr−1 with a monthly mean uncertainty of 40 %, which is lower than the bottom-up emissions of 11.0 Tg yr−1 from CAMS-GLOB-ANT v5.3. The total emissions from the 92 largest point-source emissions are estimated to be 2.9 Tg yr−1, lower than the estimation of 5.2 Tg yr−1 from the global SO2 catalog MSAQSO2LV4. We claim that the variability in the SO2 lifetime is important to account for in estimating top-down SO2 emissions.
- Article
(10384 KB) - Full-text XML
-
Supplement
(33227 KB) - BibTeX
- EndNote
Sulfur dioxide (SO2) is a reactive gas-phase air pollutant released through natural processes, such as volcanic eruptions and passive degassing (Oppenheimer et al., 2011; Carn et al., 2017), as well as anthropogenic activities, primarily from thermal power plants, fossil fuel combustion, and metal smelting and refining (Smith et al., 2011; Klimont et al., 2013; Serbula et al., 2014). After being released into the atmosphere, SO2 is primarily oxidized in the gas phase by the hydroxyl radical (OH) to form sulfuric acid (H2SO4(g)) or scavenged into cloud droplets and subsequential oxidized to form sulfate (SO) via the reaction of ozone and hydrogen peroxide (Steinfeld, 1998). Gaseous SO2 and particulate SO have detrimental effects on human health via increasing the particulate matter concentrations (PM1.0, PM2.5). Exposure to SO2 pollution, whether long or short term, is associated with increased respiratory morbidity (Chen et al., 2007; Clark Nina et al., 2010; Chen et al., 2012; Rodriguez-Villamizar et al., 2015). Sulfuric acid rain induces acidification in both aquatic and terrestrial ecosystems, causing harm to animals and plants (Larssen et al., 2006; Shukla et al., 2013). Additionally, SO contributes to reduced visibility (Leaderer et al., 1979) and acts as a precursor of cloud formation via increasing the cloud condensation nuclei (CCN), subsequently impacting regional and global climate (Lelieveld and Heintzenberg, 1992; Arnold, 2006).
There have been profound changes regarding global anthropogenic SO2 emissions in the past decades. Specifically, global SO2 emissions decreased by 31 % between 1990–2015 due to the mitigation efforts in Europe and the USA which have reduced regional SO2 emissions, while East Asia witnessed a 70 % increase in 1990–2005, followed by a decreasing trend thereafter (Kuttippurath et al., 2022). Contrary to the declining trend in China (Klimont et al., 2013; Li et al., 2017b; Zheng et al., 2018; van der A et al., 2017; Qu et al., 2019), Indian emissions surged from 4.5 to 15.0 Tg S yr−1 between 1990 and 2015 (Crippa et al., 2018; Aas et al., 2019), after which India became the world's largest emitter of anthropogenic SO2 (Li et al., 2017b, a). Given India's substantial dependence on coal-based thermal power plants to fulfill its growing energy demand, it is anticipated that the emissions will continue to rise driven by population growth and economic development (Venkataraman et al., 2018).
With the development of satellite-based measuring instruments, not only the large SO2 sources but also the weaker ones can be monitored from space. These satellite measurements provide effective near real-time information, including SO2 vertical column densities (VCDs) and data quality (QA value), to locate potential SO2 hot spots and estimate point-source emission terms. During the 1980s, only SO2 emitted from large volcano eruptions could be monitored from space by the Total Ozone Mapping Spectrometer (TOMS) and the Solar Backscattered Ultraviolet (SBUV) instruments (Krueger, 1983; McPeters et al., 1984; Krueger et al., 2000). After that, the Global Ozone Monitoring Experiment (GOME), launched in 1995, enabled the detection of large industrial SO2 sources for the first time (Eisinger and Burrows, 1998; Khokhar et al., 2008). Subsequently, the SCanning Imaging Absorption spectroMeter for Atmospheric CHartographY (SCIAMACHY) instrument launched in 2002 (Bovensmann et al., 1999), the Global Ozone Monitoring Experiment-2 (GOME 2) instrument launched in 2006 (Callies et al., 2000), and the Dutch–Finnish Ozone Monitoring Instrument (OMI) instrument launched in 2004 (Levelt et al., 2006) were used to detect sources and monitor emissions from human activities with greater detail (Carn et al., 2007; Lee et al., 2011; McLinden et al., 2016). Half of the reported anthropogenic sources can be detected and quantified with OMI SO2 measurements (Fioletov et al., 2015, 2016). Nowadays, the Tropospheric Monitoring Instrument (TROPOMI) on the ESA Copernicus Sentinel-5P satellite has become one of the most widely used satellite-based monitoring instruments (Veefkind et al., 2012; Theys et al., 2017). TROPOMI supplies daily global coverage for SO2 tropospheric vertical column densities (TVCDs) from 2018 to the present. The measurements have a horizontal resolution of approximately 5.5 km × 3.5 km (7 km × 3.5 km before 6 August 2019) at nadir viewing geometry. The TROPOMI SO2 product reprocessed by the Covariance-Based Retrieval Algorithm (COBRA) has largely reduced the SO2 noise level and uncertainties as compared to earlier SO2 datasets derived from TROPOMI or other satellite instruments (Theys et al., 2021). It makes the SO2 measurements more sensitive to minor SO2 sources down to 8.0 Gg yr−1(Theys et al., 2021), which indicates that more SO2 sources can be detected and quantified with the COBRA datasets (Fioletov et al., 2023).
With the significant advancement of satellite-based monitoring instruments over the past decades, a variety of inversion methods have been developed to constrain emissions more efficiently. Data assimilation has been used by combining satellite observations and a chemical transport model (CTM) to derive emissions of trace gases, such as NOx (Miyazaki et al., 2017; Mijling and van der A, 2012), volatile organic compounds (VOCs; Koohkan et al., 2013), CH4 (Meirink et al., 2008), and SO2 (Tsikerdekis et al., 2023). The mass balance method is a less expensive approach for deriving emissions directly from satellite observations without involving a CTM. For example, Leue et al. (2001) and Martin et al. (2003) started to calculate the NOx emissions based solely on sink terms, ignoring the effect of atmospheric transport. Recently, Fioletov et al. (2011, 2015, 2016, 2023) identified SO2 point sources using a plume fitting method and quantified emissions based on the mass balance principle with a fixed 6 h effective time. Beirle et al. (2011) used the plume fitting method to derive the NOx emissions from the large megacity sources and a mean lifetime of NO2 of 4 h. Later, Beirle et al. (2019) determined the total NOx emissions using the divergence method, while also calculating point-source emissions using a 2D-Gaussian peak fitting method with a fixed 4 h lifetime. It is noteworthy that the sink term, controlled by the tropospheric lifetime, plays a crucial role in determining the final emission terms according to the mass balance principle. However, previous studies have assumed a constant lifetime for the sink term estimation, which can lead to the spreading of emissions (Beirle et al., 2019). Consequently, deriving realistic local SO2 lifetimes, which vary from several hours to several days (Chin et al., 2000; Hains et al., 2008; Lee et al., 2011; Green et al., 2019), is crucial to calculate quantitatively accurate SO2 emissions.
In this study, we will constrain Indian SO2 emissions for the period December 2018 to November 2023 based on daily TROPOMI SO2 observations. The flux-divergence method, i.e., combining the independently derived SO2 sink and divergence, is used to obtain local emissions. Since the sink term is determined by the lifetime, we will initially derive the SO2 local effective lifetime by incorporating the SO2 chemical loss and dry deposition. Subsequently, we will improve the divergence method to generate a high-resolution 0.1° × 0.1° emission map, mitigating the smoothing of the emission map. We will estimate the SO2 emissions using the derived SO2 local lifetimes and the enhanced divergence method. We then will conduct a comparative analysis with existing bottom-up and top-down emission data. The article is organized as follows: the datasets for the divergence and sink term calculation and SO2 emissions datasets against which our results are compared are introduced in Sect. 2. Section 3 discusses the basic principles of emission calculation and the method to derive the SO2 lifetimes. Section 4 illustrates the magnitude of the spreading in the original divergence method and how we reduce this smoothing of the emission map on various spatial resolutions. The uncertainties associated with the resulting SO2 emission estimates are discussed in Sect. 5. The regional Indian emission estimations, comparisons with respect to existing estimates, and emission changes during the study period are given in Sect. 6. Section 7 discusses the uncertainties which are difficult to quantify. Finally, in Sect. 8 we present our conclusions.
2.1 Satellite observations and wind field datasets
TROPOMI on the ESA Copernicus Sentinel-5P satellite was launched on 13 October 2017 (Veefkind et al., 2012). TROPOMI is a hyperspectral nadir sensor consisting of UV–Vis–NIR spectrometers, monitoring key atmospheric species with high accuracy, including NO2 O3, SO2, CH4, CO, and HCHO as well as aerosol and cloud information. The Sentinel-5P satellite overpass time is about 13:30 local time (LT). The spatial resolution for the center of the swath is approximately 5.5 km × 3.5 km (7 km × 3.5 km before 6 August 2019) in nadir and 5.5 km × 6 km on average over the swath. In this study, the SO2 emissions are based on the TROPOMI SO2 product reprocessed by the Covariance-Based Retrieval Algorithm (COBRA) (Theys et al., 2021). The TROPOMI Level-2 COBRA SO2 data v01.00.01 are extracted from 1 December 2018 to 30 November 2023 for the SO2 divergence calculation. To ensure the high quality of the measurements, only data with a QA value larger than 0.5 and surface height lower than 3 km are used (https://data-portal.s5p-pal.com/product-docs/so2cbr/S5P-BIRA-PRF-SO2CBR_1.0.pdf, last access: 29 July 2024). Wind field information is needed for the divergence calculation. We used the wind field from the daily operational 12 h forecasts of European Centre for Medium-Range Weather Forecasts (ECMWF) with a resolution of 0.25° × 0.25° (https://www.ecmwf.int/en/forecasts, last access: 29 July 2024). The wind fields are interpolated at the mid-point of the planetary boundary layer (PBL).
2.2 Copernicus Atmospheric Monitoring Service (CAMS) datasets
CAMS has been regularly publishing global forecasts for atmospheric composition from 2015 to present on the ECMWF website (https://ads.atmosphere.copernicus.eu, last access: 29 July 2024) (referred to as the CAMS forecast datasets hereafter). The forecast itself uses ECMWF's Integrated Forecast System (IFS) for the data assimilation and modeling of the concentration of over 50 chemical species (including SO2 and OH), 7 different types of aerosols, and several meteorological factors provided with a resolution of 0.4° × 0.4°. The CAMS forecast datasets are available for 137 vertical layers with a temporal resolution of 3 h.
Calculating the chemical lifetimes of SO2 involves deriving a monthly mean OH climatology (derived from 5-year OH concentration as detailed in Sect. 3.2). This climatology is based on the monthly mean OH concentrations accessible within the CAMS forecast datasets. Specifically, the OH concentrations averaged within the PBL at 06:00 UTC (11:30 LT) are used. To ensure a stable OH climatology less influenced by extreme weather events, such as large-scale precipitation occurring on individual days, the monthly mean OH concentrations are averaged over the years from 2018 to 2023. Since cloud cover has minimal influence on the OH concentration over India (Duncan et al., 2024), we did not apply any filtering on OH data based on cloud fraction when computing the OH climatology.
2.3 SO2 emission and source datasets
Indian SO2 emissions taken from the bottom-up inventories (i.e., the Emissions Database for Global Atmospheric Research version 8 (EDGARv8) between 2018 and 2022 (Crippa et al., 2024); CAMS global anthropogenic monthly emissions version 5.3 (CAMS-GLOB-ANTv5.3) (Soulie et al., 2024) from 2018 to 2023; and the top-down SO2 global catalog, the Multi-Satellite Air Quality Sulfur Dioxide (SO2) database Long-Term L4 Global V2 (referred to as MSAQSO2L4 hereafter) (Fioletov et al., 2023) from 2019 to 2022) are used for the comparison of the final emission fluxes. The total Indian emissions from CAMS-GLOB-ANT v5.3 and EDGAR v8 show little variation in recent years, about 11.0 Tg yr−1 in each year. The total emissions of India's 92 large point sources from MSAQSO2LV4 are 5.3, 4.9, 5.2, 5.6 Tg yr−1 during 2019 to 2022, respectively. The locations of Indian thermal power plants we use in this study originate from the Open Infrastructure Map (https://openinframap.org/stats/area/India, last access: 29 July 2024).
This flux-divergence method is initially proposed by Beirle et al. (2019) and has been refined and applied in estimating emissions of trace gases like NOx (Beirle et al., 2021) and CH4 (Liu et al., 2021). Here we apply it for the derivation of SO2 emissions. The steady-state equation governing the flux-divergence method is described as follows:
with D, E. and S being the terms of divergence, emission. and sink of SO2, respectively. This equation shows that the SO2 emissions are obtained by adding estimates of SO2 divergence and sink terms. The two main steps, the divergence calculation and the sink calculation, are discussed below.
3.1 Calculation of the divergence
Equation (2) defines divergence (D) as the continuity equation of the flux (F), incorporating SO2 VCDs (V) and wind field data (w):
Note that because both VCDs and wind information are available on a grid scale rather than a continuous state, the second-order central finite difference method (SOCFDM) is used to approximate the divergence. The daily divergence of a grid cell needs to be derived for both x and y directions (see the one-dimensional example in the Supplement).
3.2 Calculation of the sink term
The relation between sink term, atmospheric density, and lifetime can be expressed as
with S the SO2 sink term, the SO2 VCD, and τ the SO2 effective lifetime. The SO2 VCDs are taken from the satellite measurements. The SO2 lifetime is determined by various processes in the atmosphere responsible for removing SO2, including deposition and chemical loss. As the deposition and chemical loss occur simultaneously, the SO2 effective lifetime τ is defined as follows:
where τc is the chemical lifetime, and τd is the lifetime related to SO2 dry deposition.
3.2.1 Calculation of the chemical lifetime
Notably, TROPOMI can “see” SO2 only in the cloud-free part of the pixel, leaving SO2 concentrations within or beneath clouds being unmeasurable. We assume that the resulting SO2 has had no interaction with clouds; thus the resulting lifetime derived for SO2 pertains to cloud-free conditions in a constrained region. Oxidization by OH(g) determines the SO2 chemical lifetime in the atmosphere under cloud-free conditions (Blitz et al., 2003; Long et al., 2017; Green et al., 2019). This reaction occurs primarily during daytime hours with maximum sunlight under humid conditions. Considering the TROPOMI overpass time is 13:00 LT, coinciding with peak OH concentrations and favorable conditions for the SO2 + OH reaction, we assume SO2 lifetime dominance via OH oxidation. Therefore, we use the model-simulated OH concentration at 11:30 LT, which is closest to the TROPOMI overpass time from CAMS forecast datasets, to calculate the chemical lifetime τc (s−1) as follows:
with k being the chemical rate coefficient (molec.−1 cm3 s−1) and [OH] denoting the OH concentration (molec. cm−3), i.e., OH column density, within the PBL divided by the PBL height. The rate coefficient k depends on the atmospheric temperature and is calculated following Table 2-1 in Vladimir et al. (2015). Due to the OH concentrations exhibiting a clear seasonal cycle (Lelieveld et al., 2016), we derive a monthly OH climatology (December 2018 to November 2023) and calculate k to estimate the SO2 chemical lifetime per month per grid cell as shown in Fig. S1. The estimated SO2 monthly mean chemical lifetime varies from 16 to 34 h. While the distribution of the SO2 chemical lifetime does not show big differences within the same season, it has a clear seasonality, with the lowest chemical lifetime occurring in summer and the highest in winter. The chemical lifetimes averaged for the whole of India in winter, spring, summer, and autumn are 31, 18, 16, and 22 h, respectively. The variation in SO2 chemical lifetime is also notable across various regions. The SO2 chemical lifetime in northern regions is larger than that in the south, with an exception occurring in summer when there is less spatial variation in lifetime. This is because more OH can be generated at low latitudes in the lower to middle troposphere due to the small solar zenith angle and high concentration of water vapor (Crutzen and Zimmermann, 1991; Spivakovsky et al., 2000). As these papers show, the OH concentration near the Equator remains consistently high throughout all seasons, leading to less variable chemical lifetimes in southern India compared to the north (Fig. S1).
3.2.2 Deposition lifetime
Wet and dry deposition influences the SO2 lifetime in the atmosphere. However, given that all SO2 is measured in cloud-free areas, our analysis only considers the impact of dry deposition, i.e., direct loss to the surface. Previous studies have indicated that SO2 dry deposition lifetimes span several days (Matsuda et al., 2006; Faloona et al., 2009; Hayden et al., 2021). Here we use 0.4 cm s−1 as a general dry deposition velocity, which is based on measurements from Hicks (2006), Myles et al. (2007), and Faloona et al. (2009). The SO2 monthly dry deposition lifetime within the PBL height is calculated by dividing the PBL height (from ECMWF data) by 0.4 cm s−1 (Slinn et al., 1978). As shown in Fig. S2, the Indian SO2 monthly mean dry deposition lifetime varies from 55 to 135 h, with the longest lifetime occurring in spring. The dry deposition lifetimes averaged over the whole of India in winter, spring, summer, and autumn are 62, 120, 75, and 70 h, respectively. The lifetime is longer in spring due to the higher PBL in this season.
3.2.3 The SO2 effective lifetime
Following Eq. (4) we combine the SO2 chemical lifetime and dry deposition terms to calculate the SO2 monthly effective lifetime for each grid cell to derive the local sink term. The SO2 monthly mean effective lifetime in India varies from 12 to 19 h (Fig. S3). Figure 1 displays SO2 effective lifetimes averaged for each season. The SO2 seasonal mean lifetimes averaged for India in winter, spring, summer, and autumn are 19, 15, 12, and 16 h, respectively. After considering the SO2 dry deposition, the annual mean SO2 effective lifetime decreases by 27 % compared to only considering the chemical loss, reducing the fraction transported away from strong point sources. Comparing the SO2 lifetime derived here with the lifetimes proposed in the literature shows that our estimates are similar to other independent model-based lifetime estimates (Lee et al., 2011) and ground-measurement-based lifetime estimates (Hains et al., 2008). We therefore argue that on average our calculated SO2 lifetime is reasonable. Furthermore, it has a latitude and seasonal dependency that is often lacking in other inversion methods.
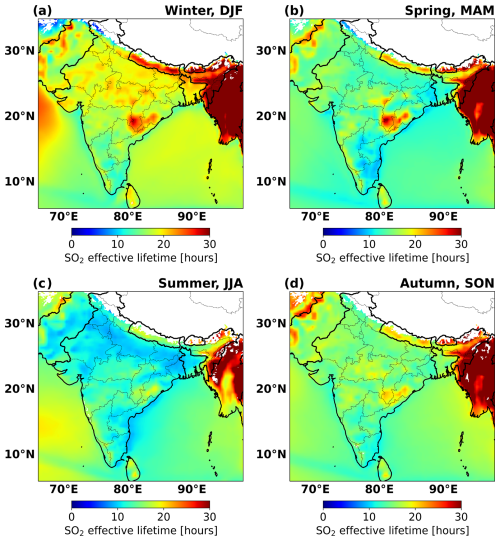
Figure 1SO2 seasonal mean effective lifetime in India. Lifetime in each season is averaged for the period from December 2018 to November 2023. (a) Winter DJF: December–January–February. (b) Spring MAM: March–April–May. (c) Summer JJA: June–July–August. (d) Autumn SON: September–October–November. The white region represents the areas with surface heights larger than 3 km or the areas without high-quality SO2 measurements. These regions are not discussed in this study.
3.2.4 The SO2 effective lifetime validation
The monthly mean SO2 effective lifetime is calculated based on OH oxidation and the SO2 dry deposition. We assume negligible influence on lifetime from SO2 wet deposition and other chemical reactions occurring in the cloud's droplets in terms of monthly mean lifetime, especially since we use only cloud-free observations. To show this, we derive a monthly mean SO2 lifetime () from the CAMS model by considering all SO2 producing processes and all kinds of sink according to Eq. (6),
with C being the total SO2 concentration and E the total SO2 emissions. We sum both the concentrations and the emissions of the model for each month covering the whole of India to derive a monthly mean averaged C and E for the whole of India. Figure 2 shows the monthly in 2019–2020 and 2022–2023 based on the CAMS model. This model-intrinsic SO2 lifetime of each month consistently exceeds 7 h. The lowest lifetime is in summer, around 9.5 h on average, while the longest lifetime is in winter, around 25.5 h on average. The lifetime in spring and autumn is comparable, around 19 h on average. Note that the CAMS model includes both dry and wet deposition of SO2. The noticeable monthly/seasonal variation of lifetime aligns well with our calculations based on the OH oxidation and SO2 dry deposition, indicating our calculated SO2 lifetime will not change significantly, even if wet deposition and other chemical reactions are considered now. At the same time, we see a large variation both spatially and in the average from month to month. Therefore, we will use the monthly averaged local lifetime from here on.
3.3 Emission calculation
The final emission term is the sum of the flux-divergence term and the sink term and can be expressed as
where is the flux divergence of the SO2 concentrations, is the wind divergence, and the last term describes the sink. The wind divergence term considers the vertical transport, which contributes to the divergence of the wind and can affect the calculated emissions. To calculate this wind divergence term, we follow the method described in Bryan (2022) to remove the wind divergence from the equation. To minimize the impact of noise on the SO2 measurements, we average the divergence over each season. Emissions for each month are then calculated by summing the monthly sink term and the divergence term of the corresponding season. The divergence calculation can be conducted on different spatial scales. Given the target resolution for the emissions is 0.1° × 0.1°, the divergence calculation can be conducted on a 0.1° × 0.1° regular grid cell (which corresponds to an approximate surface area of 10 km × 10 km) after integrating the measured SO2 VCDs to the regular grid cells. Since the emission map resolution is limited by the pixel scale of TROPOMI, we also calculate the divergence based on the original TROPOMI-measured pixels (on an along- × cross-track grid, about 5.5 km × 3.5 km at nadir varying with the viewing angle) (de Foy and Schauer, 2022) and later integrate the divergence to the regular grid cells of 0.1° × 0.1°. The integration from the TROPOMI pixels to regular grid cells is based on the weight of the overlap areas. The divergence calculations on different spatial resolutions mentioned above are conducted in this study. The final emissions are calculated from the divergence map with the best performance.
3.4 Closed-loop validation approach
To verify both the flux-divergence method and the derived OH climatology, we have tested our method using the simulated data from CAMS forecast datasets with the known input emissions CAMS-GLOB-ANT v4.2 (Fig. 3). We use the simulated SO2 VCDs within the PBL and the wind field at the mid-point of the PBL from the CAMS forecast datasets (0.4° × 0.4°) from December 2019 to November 2020 to calculate the CAMS top-down SO2 emissions with the flux-divergence method, in which the sink term is calculated following Sect. 3.2. The CAMS-GLOB-ANT v4.2 data (Soulie et al., 2024), which are applied in the CAMS forecast datasets across 2019/2020, are used for comparison with the CAMS top-down SO2 emissions. If they align closely, it indicates that the lifetime and flux-divergence method work well in this process.
To verify the performance of the flux-divergence method, it is initially tested in a closed-loop validation to calculate the CAMS top-down SO2 emissions with a resolution of 0.4° × 0.4°. Figure 4a shows the model input emissions (CAMS-GLOB-ANT v4.2), and Fig. 4b shows the CAMS top-down SO2 emissions derived with the original flux-divergence method (hereafter referred to as the classic divergence method (CDM)). The total CAMS top-down SO2 emissions for the Indian domain are 15.0 Tg yr−1, close to 13.6 Tg yr−1 calculated in the CAMS-GLOB-ANT v4.2. However, the distribution differences between the two maps are significant. The map of Fig. 4a shows a more distinct emission signal at precise locations representing point sources, whereas the emission map from Fig. 4b shows a noticeable spreading effect of point sources. This effect leads to a large difference in the emissions at the source locations. The spreading effect in the emissions derived with the CDM is a result of using the SOCFDM to approximate the continuity equation of the divergence calculation (Eq. 1), since it effectively involves a linear interpolation. To show this, Eq. (1) used to calculate the divergence in grid cell i along x direction can be rewritten as
Here, Fx(i) denotes the flux of SO2 in grid cell i along the x direction, and Δx is the resolution of the grid-scale data. Then, the divergence in grid cell i along x direction can be expressed as
with DRE(i) and DLE(i) representing the divergence at the right edge and the left edge of grid cell i. Thus, the divergence of each grid cell is essentially a linear interpolation of the divergence at the grid cell edges. If we perceive the divergence interpolation as a divergence allocation, the linear interpolation of the divergence essentially means that half of the divergence is allocated to the source location grid cell, while the remaining half is allocated to the grid cell adjacent to the source location grid cell, resulting in the spreading effect (Fig. S4c).
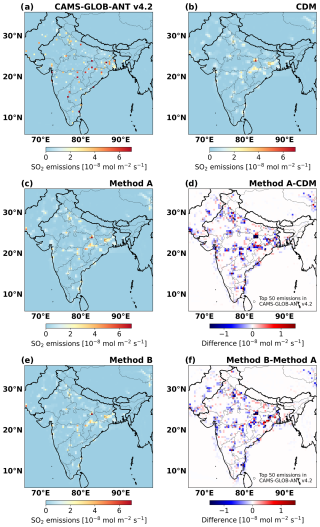
Figure 4The CAMS model input and CAMS top-down SO2 emission distribution in the winter season (December–January–February) of 2019/2020. The emissions from (a) the CAMS-GLOB-ANT v4.2 inventory and emissions derived with (b) the CDM, (c) method A, and (e) method B are shown. Panel (d) shows the difference in emissions between the CDM and method A. Panel (f) shows the difference in emissions between the CDM and method B. The black circles represent the locations of the top 50 emissions in the CAMS-GLOB-ANT v4.2 inventory.
As the spreading is a result of the discrete steps in SOCFDM, the improvements mainly focus on using different divergence interpolation/allocation methods to reduce the spreading and make the emission signals “sharper” in the source locations. In the one-dimensional situation along the wind, the highest SO2 concentration occurs downwind of the source (Fig. S4b). The largest SO2 VCD gradient is displayed around the source, especially upwind (Fig. S4a). Considering this distribution, we conduct method A, assigning all of the edge divergence to the grid cell, whose opposite edge has the larger SO2 VCD gradient (see formula in Sect. S5 in the Supplement). Figure 4c using method A shows that the spreading effect is reduced efficiently compared to Fig. 5b using the CDM. The most notable improvements are observed in the source locations, suggesting that method A can yield a higher-quality emission inventory. However, compared to the input emissions used in CAMS and shown in Fig. 5a, method A still shows a clear spreading effect. Although method A is very effective in a theoretical one-dimensional example, it is much less efficient in two dimensions, where the grid cells and wind direction (i.e., the plume) are usually not aligned. The highest SO2 concentration downwind of the source can be dispersed across multiple grid cells in the two-dimensional situation. Therefore, the peak concentration usually occurs at the source location (See Fig. S5). Based on this, we have developed a more advanced methodology (hereafter referred to as method B), which allocates all of the edge divergence to the grid cell with the larger SO2 VCD (See formula in Sect. S6 in the Supplement). The emission map derived with method B provides better results when compared to the CDM as shown in Fig. 5f and method A as shown in Fig. S6b. It is noteworthy that only the distribution is different between emissions derived with the CDM, method A, and method B. The total amount of SO2 emissions derived with the different methods remains the same. This is because the total divergence over the domain equals zero, and the total emission amount is solely determined by the SO2 sink term. We subsequently adopt method B to calculate divergence at the resolution of 0.1° × 0.1° (about 10 km × 10 km) and at the original TROPOMI-measured pixels (along × cross, about 5.5 km × 3.5 km at nadir), respectively. The divergence of the TROPOMI-measured pixels are also gridded to 0.1° × 0.1° afterwards. From Fig. 5 we see that emissions from point sources derived from the TROPOMI-measured pixels are more convergent to the point-source location (less smoothing), although the background noise also seems enhanced. For each test, method B shows emission maps with a higher spatial resolution than the other methods. Considering the outcome of these tests, our calculated emissions will be based on the divergence on TROPOMI-measured pixels derived with method B. For emissions of an individual point source (e.g., a power plant), we will sum all emissions in the 5 × 5 grid cells around the point source because part of the spreading effect still remains in the results.
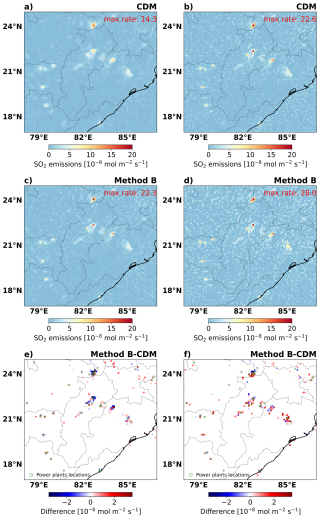
Figure 5The SO2 emission distribution in the winter season (DJF) of 2019/2020 in a selected domain (17–25° N, 78–87° E) with large thermal power plants (a–d). The emissions (a, c) are derived from the divergence calculated directly on a 0.1° resolution using the CDM (a) and method B (c). (b, d) Emissions are derived based on the divergence calculated on the TROPOMI-measured pixels using the CDM (b) and method B (d). (e, f) The difference in emissions between method B and the CDM (method B − CDM) and for the divergence calculated directly on 0.1° resolution (e) and derived on the TROPOMI-measured pixels (f). The green circles represent the locations of thermal power plants with annual power generation larger than 1000 MW (from Open Infrastructure Map, https://openinframap.org/stats/area/India).
As the uncertainty is mainly determined by the sink term, the SO2 emissions uncertainty involves the uncertainties from the measured SO2 VCDs and those associated with the SO2 effective lifetime, of which the latter are primarily related to the OH concentrations and dry deposition velocity. The SO2 VCD uncertainty is mainly from the calculation of air mass factors (AMFs). Here we apply an averaged AMF uncertainty of about 30 % for the individual measurement column, which is estimated from the S5P/TROPOMI SO2 ATBD file (https://sentinel.esa.int/documents/247904/2476257/Sentinel-5P-ATBD-SO2-TROPOMI, last access: 29 July 2024). Considering there are 17 effective measurements on average for each month across India, the uncertainty from AMFs for monthly mean SO2 VCDs is calculated to be about 7 % . The uncertainty associated with the dry deposition velocity only has a second-order effect on the SO2 effective lifetime, with the uncertainty in the OH term dominating. If the dry deposition velocity increases by 100 %, the effective lifetime for SO2 is only reduced by 20 %. Since there is a lack of validation of OH concentration due to a scarcity of measurements, we assume the differences of the simulated OH by the IFS model with various chemistry schemes (IFS(CB05BASCOE), IFS(MOZART), IFS(MOCAGE)) as an estimate of the OH uncertainty, which can reach up to 50 % (Huijnen et al., 2019). Changes in the OH density by ±50 % generally translate to a maximum uncertainty of 60 % increase or a 20 % decrease in SO2 effective lifetime. Consequently, the uncertainties of Indian emissions mainly involve the uncertainties from SO2 VCDs and from the CAMS OH concentrations. Combining the uncertainties leads to an emission uncertainty ranging from a maximum of −42 % to +33 %. Although the measured SO2 plume has no interaction with the clouds during the TROPOMI overpass, the SO2 may interact with clouds before and after this time to influence the effective SO2 lifetime. Therefore, we take an uncertainty of 40 %, which is larger than the averaged uncertainty (35 %), for SO2 monthly emissions.
6.1 Calculation of the SO2 emissions and the emission detection threshold
We calculate annual SO2 emissions over India for the period December 2018 to November 2023 (5 years). The 5-year-averaged annual SO2 emission map in Fig. 6a effectively captures large emission hot spots. But the noise on the data hampers precise differentiation of the weakest SO2 point sources. To address this, we assess the noise level on the measurement or the emission detection threshold from a selected ocean region (within 5–18° N and 85–90° E), which typically contains no strong ship or other emissions. The frequency distribution of annual SO2 emissions (or background noise) within the selected region approximates a normal distribution with σ = 0.52 Gg yr−1 as depicted by the blue bars in Fig. S7. We define the detection threshold as 4 times σ (about 2.0 Gg yr−1 per grid cell). The emissions sources above the detection threshold are shown in Fig. 6b–d. It displays a good location alignment with the source locations detected in MSAQSO2LV4 and the known thermal power plants.
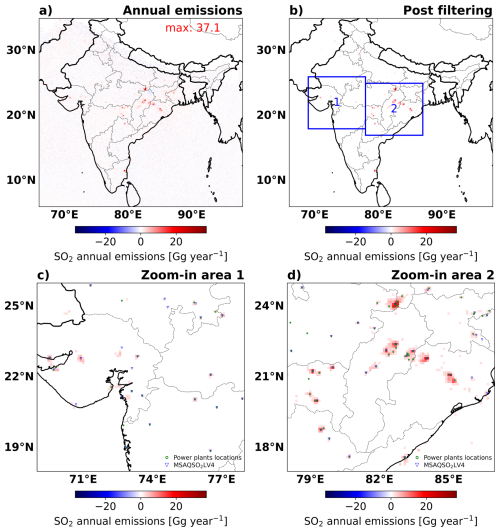
Figure 6(a) The SO2 annual mean emissions averaged between December 2018 to November 2023. Panel (b) shows the emissions above the detection threshold of 2.0 Gg yr−1. Panels (c) and (d) show the emissions of the zoomed-in views of area 1 and 2, respectively. The blue triangles represent the source locations identified by MSAQSO2L4. The green circles represent the locations of thermal power plants with annual power generation larger than 500 MW from the Open Infrastructure map (https://openinframap.org/stats/area/India). The range of the color bar is scaled with the maximum value.
The annual mean emissions for the whole of India from December 2018 to November 2023 are approximately 5.7, 4.2, 5.1, 5.1, and 5.7 Tg yr−1, with the 5-year-averaged SO2 emissions being 5.2 Tg yr−1, with an uncertainty of ±12 % . The sudden reduction in SO2 emissions in 2020 corresponds to the declining trend of coal consumption in the same year (IEA, 2023) likely due to the effects of the COVID-19 pandemic on energy consumption (Levelt et al., 2022). The Indian SO2 emissions show a seasonality: the emissions in winter (DJF) are on average 0.50 Tg per month, in spring (MAM) 0.57 Tg per month, in summer (JJA) 0.25 Tg per month, and in autumn (SON) 0.41 Tg per month. During the summer season more additional power capacity from hydropower and wind power is available (related to the monsoon), and less energy from coal powerplants is needed (IEA, 2023).
6.2 Comparison against other Indian SO2 emissions datasets
We compare our SO2 emission fluxes against those taken from the global catalog MSAQSO2L4 for 92 strong SO2 point sources. The total SO2 emissions of 92 point sources averaged over 5 years are 2.9 Tg yr−1, notably lower than 5.2 Tg yr−1 in MSAQSO2L4. The scatter plot in Fig. 7 shows the annual emissions averaged over the 5-year study period. The strong and significant correlation (P < 0.05) between the two emission datasets results in a Pearson R value of 0.87, confirming the efficiency and accuracy of the divergence method for detection of strong point sources. To further explore the differences in these emissions terms depicted in Fig. 7a, we also calculate the emissions assuming a constant SO2 lifetime of 6 h assumed in MSAQSO2L4 by Fioletov et al. (2023). This adjustment increases our SO2 emissions to 4.0 Tg yr−1, which is closer to the total emissions of the MSAQSO2L4 (Fig. 7b). But we see a noticeable smoothing effect and an overall positive bias on emissions estimated with a fixed 6 h lifetime compared to the emissions estimated with a local, variable lifetime, especially around the source location (Figs. 7c, d and S8). This indicates that the lifetime of 6 h is too short, and the application of a non-constant SO2 lifetime to constrain SO2 emissions is more realistic. Consequently, we suggest that the real SO2 emissions in India are lower than emissions estimated with a fixed 6 h lifetime.
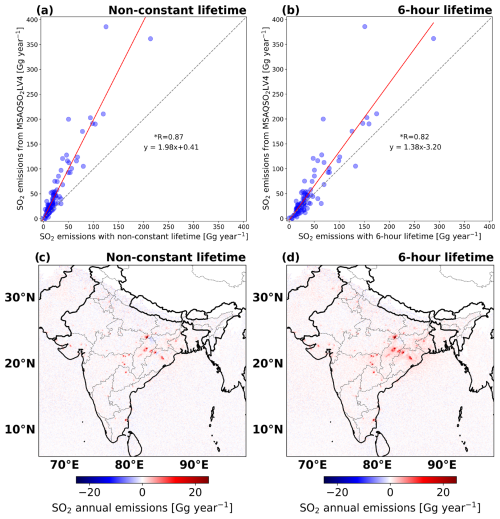
Figure 7(a) Comparison between SO2 emissions in this study derived using a variable lifetime (x axis) and the corresponding SO2 emissions from the MSAQSO2LV4 catalog (y axis). (b) Same as panel (a) but for emissions derived with a 6 h lifetime on the x axis. (c) SO2 emissions derived with the non-constant lifetime. (d) Same as panel (c) but for emissions derived with a 6 h lifetime. The point-source emissions from MSAQSO2LV4 are averaged from 2019 to 2022. The emissions from this study are averaged from December 2018 to November 2023.
To further compare the emissions to other inventories, we select our top 10 highest emission sources (see locations in Fig. S9). Our top 10 sources are associated with thermal power stations, emitting in total 1.1 Tg yr−1, which accounts for 21 % of all SO2 emissions in India. The comparison with the global catalog MSAQSO2LV4 and the bottom-up emission inventories, EDGAR v8 and CAMS-GLOB-ANT v5.3, is shown in Fig. 8. Generally, the emissions from our top 10 sources are lower than those reported by the other inventories. Except for Chandrapur (20.01° N, 79.29° E) and Durgaphur (23.55° N, 87.21° E), our top 10 sources are also listed in the Indian top 10 sources from MSAQSO2LV4. The largest emitter, Vindhyachal, representing 5 × 5 grid cells around the Vindhyachal Superpower Station (24.9° N, 82.68° E), is also the largest SO2 emission source in CAMS-GLOB-ANT v5.3 and EDGAR v8. Neyveli (11.55° N, 79.44° E) is the largest SO2 emitter in the MSAQSO2L4 and is the third largest in our inventory. Within the 5 × 5 grid cells of Neyveli, several coal power plants are situated near a lignite mine. Our comparison of the highest emitter (Neyveli) in Fig. 7a and b indicates that the emission disparities between our inventory and MSAQSO2L4 cannot be solely attributed to different lifetimes, suggesting that the choice of inversion method can also play a key role in constraining emissions.
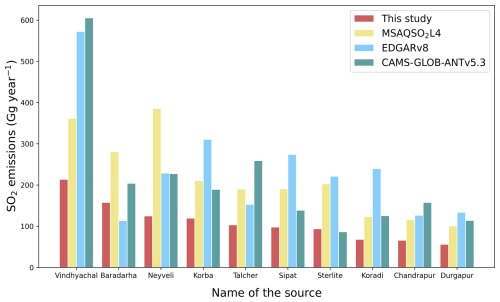
Figure 8A comparison of SO2 emission estimates from our 10 largest point sources in India using the global catalog MSAQSO2LV4, EDGAR v8, and CAMS-GLOB-ANT v5.3 datasets. The sources are sorted by descending order of our emissions. The x label lists the name of each source (i.e., power plant). For the inventories, the total emissions within 5 × 5 grid cells centered by the source location are used for comparison. Emissions from MSAQSO2LV4 are averaged from 2019 to 2022. Emissions from EDGAR v8 are averaged from December 2018 to November 2022. Emissions from other inventories are averaged from December 2018 to November 2023.
We calculate Indian SO2 emissions to be 5.2 Tg yr−1 using the SO2 local lifetime and 12.0 Tg yr−1 using a fixed 6 h lifetime. The country-total emissions obtained with a local lifetime are about 50 % lower than the reported emissions in the most used bottom-up inventories, i.e., CAMS-GLOB-ANT and EDGAR. The CAMS-GLOB-ANT v5.3 inventory estimates that India emitted 11 Tg yr−1 SO2 in 2023. However, the CAMS-model-simulated SO2 densities, driven by CAMS-GLOB-ANT v5.3, are much higher (by a factor of 2) than the TROPOMI measurements (see the comparison for 2023 in Fig. 9). The emissions at the large source locations show big differences between the two maps. Many strong source signals in the west of India are shown on the CAMS map, while they are not visible on the TROPOMI map. Considering the good data quality of the TROPOMI observations (Theys et al., 2021; De Smedt et al., 2021), we suggest that the difference in Fig. 9 is primarily due to a positive bias in model input emissions. It is noted that the SO2 lifetime in CAMS model may be overestimated and contribute to the higher simulated SO2 concentration, even though the OH level, which mainly determines the SO2 lifetime under cloud-free conditions, is similar between CAMS results (Fig. S10) and the previous studies (Hewitt and Harrison, 1985; Lelieveld et al., 2004; Souri et al., 2024).
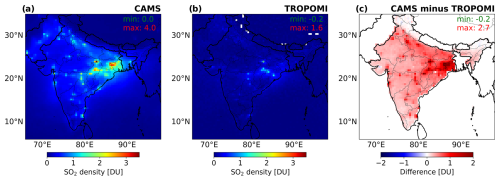
Figure 9Indian SO2 vertical column densities (VCDs) averaged in 2023 from (a) the CAMS global composition forecast dataset and (b) the TROPOMI Level-2 COBRA dataset (at about the overpass time of 06:00 UTC). We integrate the TROPOMI observations to a resolution of 0.4° × 0.4°, the same as the CAMS datasets. Panel (c) is the difference obtained by subtracting (b) from (a). The data of the same days are used for comparison. The CAMS SO2 density with total cloud coverage larger than 30 % is excluded from the averaging.
The total SO2 emissions in India were similar in 2019 and 2023, with lower emissions in the years in between. To explore the changes in detail, the difference in emissions between 2019 and 2023 of each point source is shown in Fig. 10. Overall, the total point-source emissions are estimated to be 2.8 Tg yr−1 in 2019 and 3.0 Tg yr−1 in 2023. The point sources exhibiting the largest changes belong to our top 10 sources. The emissions of Vindhyachal, the point source showing the largest decrease, were reduced by 17 %, which is about 43 Gg yr−1. This reduction may be partially attributed to the initiation of a carbon capture project at the Vindhyachal plant started in August 2022 (PTI, 2022), which likely mitigates some of the SO2 emissions (Wang et al., 2011; Corvisier et al., 2013; Gimeno et al., 2017). The largest increasing emitter, Baradarha, increased over 75 %, which is in total 107 Gg yr−1 of SO2 emissions.
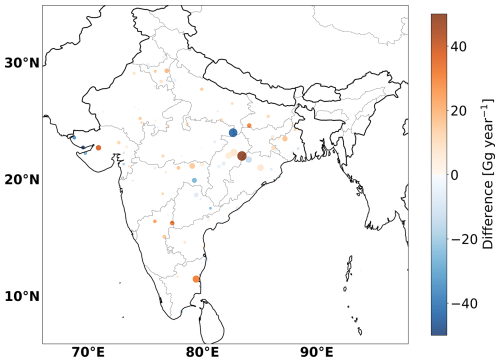
Figure 10Absolute changes in the derived SO2 emissions for the most important point sources between December 2018 and November 2023. The circle size denotes the size of the emissions in the last year (December 2022 to November 2023). The circle color means changes in the last year compared to the emissions in the first year (December 2018 to November 2019).
The hard-to-quantify factors influencing the lifetime and emissions are discussed here. First, our grid-averaged (about 40 km × 40 km) OH climatology may not resolve detailed chemical variation within the pollutant plumes, particularly those involving the interaction between SO2 and OH. Krol et al. (2024) studied the chemistry within the NOx plumes and observed low OH concentrations near the strong NOx sources (within an average of 10 km) and enhanced OH away from the sources. This suggests that our 40 km averaged OH climatology cannot capture this OH decline and may underestimate the SO2 lifetime near the large NOx sources. Furthermore, the variation of OH concentration between 10 km to 40 km is roughly limited to 10 %; see Fig. 7b from the study of Krol et al. (2024). We have considered these effects in our error estimate of the lifetime. Second, we did not consider the heterogenous SO2 reactions on wet aerosols. We suppose this impact on SO2 lifetime can be neglected in our study. Analysis of the dominant term for conversion in the CAMS system shows aqueous-phase chemistry is the dominant term related to sulfate production rather than heterogeneous reactions. We therefore assume wet aerosols are a negligible source term (which typically have low pH and slow sulfate production; Gillani et al., 1981). Even though the atmospheric SO2 in the gas phase can convert to the aqueous phase and be oxidized to form sulfate on the wet surface of aerosols or within clouds, these reactions typically occur on hazy days with high relative humidity and PM2.5 level (Ge et al., 2021). These meteorological conditions are generally not favored on days with minimal cloud coverage, as achieving the necessary high relative humidity is difficult with ample sunlight at noon. Finally, we only calculate the lifetime for SO2 in cloud-free regions, excluding the SO2 wet deposition and the reactions within the clouds. This is actually the lifetime we need in our inversion since the TROPOMI observations of SO2 plumes are limited to cloud-free scenes.
In this study, we derived Indian SO2 emissions using an improved flux-divergence method including a non-constant SO2 effective lifetime for cloud-free conditions. The improved divergence method largely removes the spreading effect on emissions that is typically introduced by the discretization in calculating the divergence. The non-constant lifetime approach is more representative with respect to season and latitude as compared to adopting a fixed lifetime for the derivation of emission fluxes, especially for short-living species like SO2. Based on the non-constant lifetime, the improved divergence method further constrains the SO2 emissions more closely to its source. The SO2 effective lifetime in India for cloud-free conditions, derived from the SO2 chemical lifetime and dry deposition lifetime, is calculated for each grid cell. The SO2 chemical lifetime is primarily derived using an OH monthly climatology (December 2018 to November 2023) from CAMS simulations. The variability in the monthly mean SO2 effective lifetime varies from 16 to 34 h, with the longer chemical lifetime occurring in the winter season. The seasonality of the SO2 chemical lifetime is driven by the OH concentration, which is largely influenced by sunlight. Significantly different chemical lifetimes were also noted across various regions within the same season. The chemical lifetime in northern India is generally larger than in the south in spring, winter, and autumn. The SO2 monthly dry deposition lifetime varies from 55 to 135 h. After accounting for the SO2 dry deposition, the seasonality and regional variation of lifetime are reduced. The SO2 effective lifetime is 27 % lower on average compared to the chemical lifetime. The SO2 monthly mean effective lifetime varies from 12 to 19 h with uncertainties of −20 % to +60 %. Our local effective lifetime calculations align with a recent study, demonstrating that the species lifetime varies spatially due to the spatial variation of the influencing factor (Krol et al., 2024).
Since the data are available on a grid scale instead of a continuous state, the divergence calculation will introduce a spreading effect to the calculated SO2 divergence and emissions. To reduce the spreading effect, we have tested two divergence allocation methods on the resolution of 0.4° × 0.4°, 0.1° × 0.1°, and TROPOMI-measured pixels and concluded that assigning all flux divergence to the grid cell with the larger SO2 VCD improved the results. After the implementation of the improved flux-divergence method, the smoothing of the emission map is mitigated efficiently. An emission map with more distinct emission signals has been obtained.
Implementing the improved method with a non-constant SO2 lifetime, we calculated the SO2 emissions for India from December 2018 to November 2023. The total annual SO2 emissions we found for this period is about 5.2 Tg yr−1 with a monthly mean uncertainty of 40 %. The total annual SO2 emissions decreased from 2019 to 2020 due to the COVID-19 quarantine measures and then gradually increased to the same level as before COVID-19 in 2023. In contrast to the trend from MSAQSO2LV4 showing that the SO2 emissions reaching its highest point in 2022, our emissions in 2022 are the same as those in 2021 and lower than the emissions in 2019 and 2023. Even though the total power generation in 2022 is higher than the previous years (https://powermin.gov.in/en/content/power-sector-glance-all-India, last access: 29 July 2024), the comparable emissions between 2021 and 2022 might be a result of the growth of renewable and non-fossil fuel power generation in 2022 (https://powermin.gov.in/en/content/overview, last access: 29 July 2024).
The 92 SO2 large point sources are compared with the global catalog MSAQSO2LV4. Our total emissions of 2.9 Tg yr−1 are lower than the total emissions from MSAQSO2LV4 of 5.2 Tg yr−1. The difference is mainly because Fioletov et al. (2023) used a fixed 6 h lifetime for calculating emissions, while our derived monthly effective lifetimes varied from 12 to 19 h. Using the fixed 6 h lifetime can result in a smoothing of emission map with the divergence method and may lead to overestimation of the emissions. Our results show the SO2 emissions of the 92 point sources in India are similar between 2019 and 2023. The SO2 emissions at the largest point source, Vindhyachal, show a reduction in recent years. This might be due to the initiation of a carbon capture project at Vindhyachal.
With the improvement in the divergence method and locally derived variability in the lifetime, gridded SO2 emissions over a large area can be estimated efficiently. This method can be applied to any region in the world to derive SO2 emissions with a 0.1° × 0.1° resolution based on TROPOMI observations. For those regions with more northerly latitudes than 40° N (e.g., northern China, eastern Europe), the latitude- and season-dependent SO2 lifetime with the improved divergence calculation approach has the potential to significantly improve the top-down derivation of SO2 emission estimates. This paper is considered a first step towards addressing the lifetime variability in the inversion methodology.
The TROPOMI Level-2 COBRA SO2 data created by the Royal Belgian Institute for Space Aeronomy (BIRA-IASB) are publicly available on the PAL website (https://data-portal.s5p-pal.com/products/so2cbr.html, Theys, 2024a) and the BIRA website (https://distributions.aeronomie.be, Theys, 2024b; Theys et al., 2021). The daily operational 12 h forecast wind field data created by the European Centre for Medium-Range Weather Forecasts (ECMWF) are available at https://www.ecmwf.int/en/forecasts/datasets/open-data (ECMWF, 2024). CAMS global atmospheric composition forecast data created by the Copernicus Atmospheric Monitoring Service (CAMS) are available on the Copernicus website with a login account (https://ads.atmosphere.copernicus.eu/datasets/cams-global-atmospheric-composition-forecasts?tab=overview, CAMS, 2024). Indian SO2 emissions data created by the Emissions Database for Global Atmospheric Research version 8 (EDGARv8) are available at https://edgar.jrc.ec.europa.eu/dataset_ap81 (Crippa, 2024). CAMS Global anthropogenic emissions data are publicly available on the ECCAD website (https://permalink.aeris-data.fr/CAMS-GLOB-ANT, Soulie, 2024; Soulie et al., 2024). SO2 global catalog MSAQSO2L4 data created by Fioletov et al. (2023) are available on the NASA Goddard Earth Sciences (GES) Data and Information Services Center (DISC) website (https://doi.org/10.5067/MEASURES/SO2/DATA406, Fioletov et al., 2022). Indian power plant locations from the OpenStreetMap database are publicly available at https://openinframap.org/stats/area/India/plants (OpenStreetMap contributors, 2024; © OpenStreetMap contributors, ODbL).
The supplement related to this article is available online at https://doi.org/10.5194/acp-25-1851-2025-supplement.
YC: formal analysis, writing. RJvdA: physical and technical support of the divergence method, conceptualization, paper review, and editing. JD: physical and technical support of the divergence method, conceptualization, paper review, and editing. HE: technical support of the divergence method, wind field reprocessing, paper review, and editing. JEW: support of the related chemistry, paper review, and editing. NT: support of SO2 COBRA datasets, paper review, and editing. AT: support of CAMS datasets, paper review, and editing. PFL: paper review and editing.
The contact author has declared that none of the authors has any competing interests.
Publisher's note: Copernicus Publications remains neutral with regard to jurisdictional claims made in the text, published maps, institutional affiliations, or any other geographical representation in this paper. While Copernicus Publications makes every effort to include appropriate place names, the final responsibility lies with the authors.
We acknowledge the team of ECCAD, EDGAR, ECMWF, Copernicus Project, and all the other investigators who have obtained the data used in this study and made them available online.
We acknowledge Felipe Cifuentes for inspiring the closed-loop validation approach and Lotte Bryan for developing the wind divergence removal method.
Nicolas Theys acknowledges support from ESA S5P ATM-MPC, ESA S5P-PAL, and Belgium Prodex TRACE-S5P projects.
This research has been supported by the China Scholarship Council (grant no. 202206330028).
This paper was edited by Jianzhong Ma and reviewed by Chris McLinden and two anonymous referees.
Aas, W., Mortier, A., Bowersox, V., Cherian, R., Faluvegi, G., Fagerli, H., Hand, J., Klimont, Z., Galy-Lacaux, C., Lehmann, C. M. B., Myhre, C. L., Myhre, G., Olivié, D., Sato, K., Quaas, J., Rao, P. S. P., Schulz, M., Shindell, D., Skeie, R. B., Stein, A., Takemura, T., Tsyro, S., Vet, R., and Xu, X.: Global and regional trends of atmospheric sulfur, Sci. Rep., 9, 953, https://doi.org/10.1038/s41598-018-37304-0, 2019.
Arnold, F.: Atmospheric Aerosol and Cloud Condensation Nuclei Formation: A Possible Influence of Cosmic Rays?, Space Sci. Rev., 125, 169–186, https://doi.org/10.1007/s11214-006-9055-4, 2006.
Beirle, S., Boersma, K. F., Platt, U., Lawrence, M. G., and Wagner, T.: Megacity emissions and lifetimes of nitrogen oxides probed from space, Science, 333, 1737–1739, https://doi.org/10.1126/science.1207824, 2011.
Beirle, S., Borger, C., Dörner, S., Li, A., Hu, Z., Liu, F., Wang, Y., and Wagner, T.: Pinpointing nitrogen oxide emissions from space, Sci. Adv., 5, eaax9800, https://doi.org/10.1126/sciadv.aax9800, 2019.
Beirle, S., Borger, C., Dörner, S., Eskes, H., Kumar, V., de Laat, A., and Wagner, T.: Catalog of NOx emissions from point sources as derived from the divergence of the NO2 flux for TROPOMI, Earth Syst. Sci. Data, 13, 2995–3012, https://doi.org/10.5194/essd-13-2995-2021, 2021.
Blitz, M. A., Hughes, K. J., and Pilling, M. J.: Determination of the High-Pressure Limiting Rate Coefficient and the Enthalpy of Reaction for OH + SO2, J. Phys. Chem. A, 107, 1971–1978, https://doi.org/10.1021/jp026524y, 2003.
Bovensmann, H., Burrows, J. P., Buchwitz, M., Frerick, J., Noël, S., Rozanov, V. V., Chance, K. V., and Goede, A. P. H.: SCIAMACHY: Mission Objectives and Measurement Modes, J. Atmos. Sci., 56, 127–150, https://doi.org/10.1175/1520-0469(1999)056<0127:SMOAMM>2.0.CO;2, 1999.
Bryan, L.: The Flux Divergence Method Applied to Nitrogen Emissions in The Netherlands, Master thesis, TU Delft Electrical Engineering, Mathematics and Computer Science, Delft University of Technology, https://resolver.tudelft.nl/uuid:6e7e611b-9a7a-4886-8eb8-f1a172516d99 (last access: 11 February 2025), 2022.
Callies, J., Corpaccioli, E., Eisinger, M., Hahne, A., and Lefebvre, A.: GOME-2-Metop's second-generation sensor for operational ozone monitoring, ESA Bull., 102, 28–36, 2000.
Carn, S. A., Krueger, A. J., Krotkov, N. A., Yang, K., and Levelt, P. F.: Sulfur dioxide emissions from Peruvian copper smelters detected by the Ozone Monitoring Instrument, Geophys. Res. Lett., 34, L09801, https://doi.org/10.1029/2006GL029020, 2007.
Carn, S. A., Fioletov, V. E., McLinden, C. A., Li, C., and Krotkov, N. A.: A decade of global volcanic SO2 emissions measured from space, Sci. Rep., 7, 44095, https://doi.org/10.1038/srep44095, 2017.
Chen, R., Huang, W., Wong, C.-M., Wang, Z., Quoc Thach, T., Chen, B., and Kan, H.: Short-term exposure to sulfur dioxide and daily mortality in 17 Chinese cities: The China air pollution and health effects study (CAPES), Environ. Res., 118, 101–106, https://doi.org/10.1016/j.envres.2012.07.003, 2012.
Chen, T. M., Gokhale, J., Shofer, S., and Kuschner, W. G.: Outdoor air pollution: nitrogen dioxide, sulfur dioxide, and carbon monoxide health effects, Am. J. Med. Sci., 333, 249–256, https://doi.org/10.1097/MAJ.0b013e31803b900f, 2007.
Chin, M., Savoie, D. L., Huebert, B. J., Bandy, A. R., Thornton, D. C., Bates, T. S., Quinn, P. K., Saltzman, E. S., and De Bruyn, W. J.: Atmospheric sulfur cycle simulated in the global model GOCART: Comparison with field observations and regional budgets, J. Geophys. Res.-Atmos., 105, 24689–24712, https://doi.org/10.1029/2000JD900385, 2000.
Clark Nina, A., Demers Paul, A., Karr Catherine, J., Koehoorn, M., Lencar, C., Tamburic, L., and Brauer, M.: Effect of Early Life Exposure to Air Pollution on Development of Childhood Asthma, Environ. Health Perspect., 118, 284–290, https://doi.org/10.1289/ehp.0900916, 2010.
Copernicus Atmospheric Monitoring Service (CAMS): CAMS global atmospheric composition forecast data, Copernicus Atmospheric Monitoring Service [data set], https://ads.atmosphere.copernicus.eu/datasets/cams-global-atmospheric-composition-forecasts?tab=overview (last access: 29 July 2024), 2024.
Corvisier, J., Bonvalot, A.-F., Lagneau, V., Chiquet, P., Renard, S., Sterpenich, J., and Pironon, J.: Impact of Co-injected Gases on CO2 Storage Sites: Geochemical Modeling of Experimental Results, Enrgy. Proced., 37, 3699–3710, https://doi.org/10.1016/j.egypro.2013.06.264, 2013.
Crippa, M.: Indian SO2 emissions (EDGARv8), Emissions Database for Global Atmospheric Research (EDGAR), European Commission, Joint Research Centre (JRC) [data set], https://edgar.jrc.ec.europa.eu/dataset_ap81 (last access: 29 July 2024), 2024.
Crippa, M., Guizzardi, D., Muntean, M., Schaaf, E., Dentener, F., van Aardenne, J. A., Monni, S., Doering, U., Olivier, J. G. J., Pagliari, V., and Janssens-Maenhout, G.: Gridded emissions of air pollutants for the period 1970–2012 within EDGAR v4.3.2, Earth Syst. Sci. Data, 10, 1987–2013, https://doi.org/10.5194/essd-10-1987-2018, 2018.
Crippa, M., Guizzardi, D., Pagani, F., Schiavina, M., Melchiorri, M., Pisoni, E., Graziosi, F., Muntean, M., Maes, J., Dijkstra, L., Van Damme, M., Clarisse, L., and Coheur, P.: Insights into the spatial distribution of global, national, and subnational greenhouse gas emissions in the Emissions Database for Global Atmospheric Research (EDGAR v8.0), Earth Syst. Sci. Data, 16, 2811–2830, https://doi.org/10.5194/essd-16-2811-2024, 2024.
Crutzen, P. J. and Zimmermann, P. H.: The changing photochemistry of the troposphere, Tellus B, 43, 136–151, https://doi.org/10.1034/j.1600-0889.1991.t01-1-00012.x, 1991.
de Foy, B. and Schauer, J. J.: An improved understanding of NOx emissions in South Asian megacities using TROPOMI NO2 retrievals, Environ. Res. Lett., 17, 024006, https://doi.org/10.1088/1748-9326/ac48b4, 2022.
De Smedt, I., Pinardi, G., Vigouroux, C., Compernolle, S., Bais, A., Benavent, N., Boersma, F., Chan, K.-L., Donner, S., Eichmann, K.-U., Hedelt, P., Hendrick, F., Irie, H., Kumar, V., Lambert, J.-C., Langerock, B., Lerot, C., Liu, C., Loyola, D., Piters, A., Richter, A., Rivera Cárdenas, C., Romahn, F., Ryan, R. G., Sinha, V., Theys, N., Vlietinck, J., Wagner, T., Wang, T., Yu, H., and Van Roozendael, M.: Comparative assessment of TROPOMI and OMI formaldehyde observations and validation against MAX-DOAS network column measurements, Atmos. Chem. Phys., 21, 12561–12593, https://doi.org/10.5194/acp-21-12561-2021, 2021.
Duncan, B. N., Anderson, D. C., Fiore, A. M., Joiner, J., Krotkov, N. A., Li, C., Millet, D. B., Nicely, J. M., Oman, L. D., St. Clair, J. M., Shutter, J. D., Souri, A. H., Strode, S. A., Weir, B., Wolfe, G. M., Worden, H. M., and Zhu, Q.: Opinion: Beyond global means – novel space-based approaches to indirectly constrain the concentrations of and trends and variations in the tropospheric hydroxyl radical (OH), Atmos. Chem. Phys., 24, 13001–13023, https://doi.org/10.5194/acp-24-13001-2024, 2024.
Eisinger, M. and Burrows, J. P.: Tropospheric sulfur dioxide observed by the ERS-2 GOME instrument, Geophys. Res. Lett., 25, 4177–4180, https://doi.org/10.1029/1998GL900128, 1998.
European Centre for Medium-Range Weather Forecasts (ECMWF): Daily operational 12 h forecast wind field data, ECMWF [data set], https://www.ecmwf.int/en/forecasts/datasets/open-data (last access: 29 July 2024), 2024.
Faloona, I., Conley, S. A., Blomquist, B., Clarke, A. D., Kapustin, V., Howell, S., Lenschow, D. H., and Bandy, A. R.: Sulfur dioxide in the tropical marine boundary layer: dry deposition and heterogeneous oxidation observed during the Pacific Atmospheric Sulfur Experiment, J. Atmos. Chem., 63, 13–32, https://doi.org/10.1007/s10874-010-9155-0, 2009.
Fioletov, V., McLinden, C. A., Griffin, D., Abboud, I., Krotkov, N., Leonard, P. J. T., Li, C., Joiner, J., Theys, N., and Carn, S.: Multi-Satellite Air Quality Sulfur Dioxide (SO2) Database Long-Term L4 Global V2, edited by: Leonard, P., Greenbelt, MD, USA, Goddard Earth Science Data and Information Services Center (GES DISC) [data set], https://doi.org/10.5067/MEASURES/SO2/DATA406, 2022.
Fioletov, V. E., McLinden, C. A., Krotkov, N., Moran, M. D., and Yang, K.: Estimation of SO2 emissions using OMI retrievals, Geophys. Res. Lett., 38, L21811, https://doi.org/10.1029/2011GL049402, 2011.
Fioletov, V. E., McLinden, C. A., Krotkov, N., and Li, C.: Lifetimes and emissions of SO2 from point sources estimated from OMI, Geophys. Res. Lett., 42, 1969–1976, https://doi.org/10.1002/2015gl063148, 2015.
Fioletov, V. E., McLinden, C. A., Krotkov, N., Li, C., Joiner, J., Theys, N., Carn, S., and Moran, M. D.: A global catalogue of large SO2 sources and emissions derived from the Ozone Monitoring Instrument, Atmos. Chem. Phys., 16, 11497–11519, https://doi.org/10.5194/acp-16-11497-2016, 2016.
Fioletov, V. E., McLinden, C. A., Griffin, D., Abboud, I., Krotkov, N., Leonard, P. J. T., Li, C., Joiner, J., Theys, N., and Carn, S.: Version 2 of the global catalogue of large anthropogenic and volcanic SO2 sources and emissions derived from satellite measurements, Earth Syst. Sci. Data, 15, 75–93, https://doi.org/10.5194/essd-15-75-2023, 2023.
Ge, W., Liu, J., Yi, K., Xu, J., Zhang, Y., Hu, X., Ma, J., Wang, X., Wan, Y., Hu, J., Zhang, Z., Wang, X., and Tao, S.: Influence of atmospheric in-cloud aqueous-phase chemistry on the global simulation of SO2 in CESM2, Atmos. Chem. Phys., 21, 16093–16120, https://doi.org/10.5194/acp-21-16093-2021, 2021.
Gillani, N. V., Kohli, S., and Wilson, W. E.: Gas-to-particle conversion of sulfur in power plant plumes – I. Parametrization of the conversion rate for dry, moderately polluted ambient conditions, Atmos. Environ., 15, 2293–2313, https://doi.org/10.1016/0004-6981(81)90261-4, 1981.
Gimeno, B., Artal, M., Velasco, I., Blanco, S. T., and Fernández, J.: Influence of SO2 on CO2 storage for CCS technology: Evaluation of CO2 SO2 co-capture, Appl. Energ., 206, 172–180, https://doi.org/10.1016/j.apenergy.2017.08.048, 2017.
Green, J. R., Fiddler, M. N., Holloway, J. S., Fibiger, D. L., McDuffie, E. E., Campuzano-Jost, P., Schroder, J. C., Jimenez, J. L., Weinheimer, A. J., Aquino, J., Montzka, D. D., Hall, S. R., Ullmann, K., Shah, V., Jaeglé, L., Thornton, J. A., Bililign, S., and Brown, S. S.: Rates of Wintertime Atmospheric SO2 Oxidation based on Aircraft Observations during Clear-Sky Conditions over the Eastern United States, J. Geophys. Res.-Atmos., 124, 6630–6649, https://doi.org/10.1029/2018JD030086, 2019.
Hains, J. C., Taubman, B. F., Thompson, A. M., Stehr, J. W., Marufu, L. T., Doddridge, B. G., and Dickerson, R. R.: Origins of chemical pollution derived from Mid-Atlantic aircraft profiles using a clustering technique, Atmos. Environ., 42, 1727–1741, https://doi.org/10.1016/j.atmosenv.2007.11.052, 2008.
Hayden, K., Li, S.-M., Makar, P., Liggio, J., Moussa, S. G., Akingunola, A., McLaren, R., Staebler, R. M., Darlington, A., O'Brien, J., Zhang, J., Wolde, M., and Zhang, L.: New methodology shows short atmospheric lifetimes of oxidized sulfur and nitrogen due to dry deposition, Atmos. Chem. Phys., 21, 8377–8392, https://doi.org/10.5194/acp-21-8377-2021, 2021.
Hewitt, C. N. and Harrison, R. M.: Tropospheric concentrations of the hydroxyl radical – a review, Atmos. Environ., 19, 545–554, https://doi.org/10.1016/0004-6981(85)90033-2, 1985.
Hicks, B. B.: Dry deposition to forests – On the use of data from clearings, Agr. Forest Meteorol., 136, 214–221, https://doi.org/10.1016/j.agrformet.2004.06.013, 2006.
Huijnen, V., Pozzer, A., Arteta, J., Brasseur, G., Bouarar, I., Chabrillat, S., Christophe, Y., Doumbia, T., Flemming, J., Guth, J., Josse, B., Karydis, V. A., Marécal, V., and Pelletier, S.: Quantifying uncertainties due to chemistry modelling – evaluation of tropospheric composition simulations in the CAMS model (cycle 43R1), Geosci. Model Dev., 12, 1725–1752, https://doi.org/10.5194/gmd-12-1725-2019, 2019.
IEA: India Energy Outlook 2023, International Energy Agency, IEA, Paris, https://www.iea.org/reports/india-energy-outlook-2021 (last access: 29 July 2024), 2023.
Khokhar, M. F., Platt, U., and Wagner, T.: Temporal trends of anthropogenic SO2 emitted by non-ferrous metal smelters in Peru and Russia estimated from Satellite observations, Atmos. Chem. Phys. Discuss., 8, 17393–17422, https://doi.org/10.5194/acpd-8-17393-2008, 2008.
Klimont, Z., Smith, S. J., and Cofala, J.: The last decade of global anthropogenic sulfur dioxide: 2000–2011 emissions, Environ. Res. Lett., 8, 014003, https://doi.org/10.1088/1748-9326/8/1/014003, 2013.
Koohkan, M. R., Bocquet, M., Roustan, Y., Kim, Y., and Seigneur, C.: Estimation of volatile organic compound emissions for Europe using data assimilation, Atmos. Chem. Phys., 13, 5887–5905, https://doi.org/10.5194/acp-13-5887-2013, 2013.
Krol, M., van Stratum, B., Anglou, I., and Boersma, K. F.: Evaluating NOx stack plume emissions using a high-resolution atmospheric chemistry model and satellite-derived NO2 columns, Atmos. Chem. Phys., 24, 8243–8262, https://doi.org/10.5194/acp-24-8243-2024, 2024.
Krueger, A., Schaefer, S., Krotkov, N., Bluth, G., and Barker, S.: Ultraviolet Remote Sensing of Volcanic Emissions, Geoph. Monog. Series, 116, 25–43, https://doi.org/10.1029/GM116p0025, 2000.
Krueger, A. J.: Sighting of El Chichón Sulfur Dioxide Clouds with the Nimbus 7 Total Ozone Mapping Spectrometer, Science, 220, 1377–1379, https://doi.org/10.1126/science.220.4604.1377, 1983.
Kuttippurath, J., Patel, V. K., Pathak, M., and Singh, A.: Improvements in SO2 pollution in India: role of technology and environmental regulations, Environ. Sci. Pollut. Res., 29, 78637–78649, https://doi.org/10.1007/s11356-022-21319-2, 2022.
Larssen, T., Lydersen, E., Tang, D., He, Y., Gao, J., Liu, H., Duan, L., Seip, H. M., Vogt, R. D., and Mulder, J.: Acid rain in China, Environ. Sci. Technol., 40, 418–425, https://doi.org/10.1016/s0269-7491(99)00279-1, 2006.
Leaderer, B. P., Holford, T. R., and Stolwijk, J. A. J.: Relationship between Sulfate Aerosol and Visibility, J. Air Pollut. Control Assoc., 29, 154–157, 1979.
Lee, C., Martin, R. V., van Donkelaar, A., Lee, H., Dickerson, R. R., Hains, J. C., Krotkov, N., Richter, A., Vinnikov, K., and Schwab, J. J.: SO2 emissions and lifetimes: Estimates from inverse modeling using in situ and global, space-based (SCIAMACHY and OMI) observations, J. Geophys. Res.-Atmos., 116, D06304, https://doi.org/10.1029/2010JD014758, 2011.
Lelieveld, J. and Heintzenberg, J.: Sulfate Cooling Effect on Climate Through In-Cloud Oxidation of Anthropogenic SO2, Science, 258, 117–120, https://doi.org/10.1126/science.258.5079.117, 1992.
Lelieveld, J., Dentener, F. J., Peters, W., and Krol, M. C.: On the role of hydroxyl radicals in the self-cleansing capacity of the troposphere, Atmos. Chem. Phys., 4, 2337–2344, https://doi.org/10.5194/acp-4-2337-2004, 2004.
Lelieveld, J., Gromov, S., Pozzer, A., and Taraborrelli, D.: Global tropospheric hydroxyl distribution, budget and reactivity, Atmos. Chem. Phys., 16, 12477–12493, https://doi.org/10.5194/acp-16-12477-2016, 2016.
Leue, C., Wenig, M., Wagner, T., Klimm, O., Platt, U., and Jähne, B.: Quantitative analysis of NO x emissions from Global Ozone Monitoring Experiment satellite image sequences, J. Geophys. Res.-Atmos., 106, 5493–5505, https://doi.org/10.1029/2000JD900572, 2001.
Levelt, P. F., Oord, G. H. J. v. d., Dobber, M. R., Malkki, A., Huib, V., Johan de, V., Stammes, P., Lundell, J. O. V., and Saari, H.: The ozone monitoring instrument, IEEE T. Geosci. Remote, 44, 1093–1101, https://doi.org/10.1109/TGRS.2006.872333, 2006.
Levelt, P. F., Stein Zweers, D. C., Aben, I., Bauwens, M., Borsdorff, T., De Smedt, I., Eskes, H. J., Lerot, C., Loyola, D. G., Romahn, F., Stavrakou, T., Theys, N., Van Roozendael, M., Veefkind, J. P., and Verhoelst, T.: Air quality impacts of COVID-19 lockdown measures detected from space using high spatial resolution observations of multiple trace gases from Sentinel-5P/TROPOMI, Atmos. Chem. Phys., 22, 10319–10351, https://doi.org/10.5194/acp-22-10319-2022, 2022.
Li, C., McLinden, C., Fioletov, V., Krotkov, N., Carn, S., Joiner, J., Streets, D., He, H., Ren, X., Li, Z., and Dickerson, R. R.: India Is Overtaking China as the World's Largest Emitter of Anthropogenic Sulfur Dioxide, Sci. Rep., 7, 14304, https://doi.org/10.1038/s41598-017-14639-8, 2017a.
Li, M., Liu, H., Geng, G. N., Hong, C. P., Liu, F., Song, Y., Tong, D., Zheng, B., Cui, H. Y., Man, H. Y., Zhang, Q., and He, K. B.: Anthropogenic emission inventories in China: a review, Natl. Sci. Rev., 4, 834–866, https://doi.org/10.1093/nsr/nwx150, 2017b.
Liu, M., van der A, R., van Weele, M., Eskes, H., Lu, X., Veefkind, P., de Laat, J., Kong, H., Wang, J., Sun, J., Ding, J., Zhao, Y., and Weng, H.: A New Divergence Method to Quantify Methane Emissions Using Observations of Sentinel-5P TROPOMI, Geophys. Res. Lett., 48, e2021GL094151, https://doi.org/10.1029/2021GL094151, 2021.
Long, B., Bao, J. L., and Truhlar, D. G.: Reaction of SO(2) with OH in the atmosphere, Phys. Chem. Chem. Phys., 19, 8091–8100, https://doi.org/10.1039/c7cp00497d, 2017.
Martin, R. V., Jacob, D. J., Chance, K., Kurosu, T. P., Palmer, P. I., and Evans, M. J.: Global inventory of nitrogen oxide emissions constrained by space-based observations of NO2 columns, J. Geophys. Res.-Atmos., 108, 4537, https://doi.org/10.1029/2003JD003453, 2003.
Matsuda, K., Watanabe, I., Wingpud, V., Theramongkol, P., and Ohizumi, T.: Deposition velocity of O3 and SO2 in the dry and wet season above a tropical forest in northern Thailand, Atmos. Environ., 40, 7557–7564, https://doi.org/10.1016/j.atmosenv.2006.07.003, 2006.
McLinden, C. A., Fioletov, V., Shephard, M. W., Krotkov, N., Li, C., Martin, R. V., Moran, M. D., and Joiner, J.: Space-based detection of missing sulfur dioxide sources of global air pollution, Nat. Geosci., 9, 496–500, https://doi.org/10.1038/ngeo2724, 2016.
McPeters, R. D., Heath, D. F., and Schlesinger, B. M.: Satellite observation of SO2 from El Chichon: Identification and measurement, Geophys. Res. Lett., 11, 1203–1206, https://doi.org/10.1029/GL011i012p01203, 1984.
Meirink, J. F., Bergamaschi, P., and Krol, M. C.: Four-dimensional variational data assimilation for inverse modelling of atmospheric methane emissions: method and comparison with synthesis inversion, Atmos. Chem. Phys., 8, 6341–6353, https://doi.org/10.5194/acp-8-6341-2008, 2008.
Mijling, B. and van der A, R. J.: Using daily satellite observations to estimate emissions of short-lived air pollutants on a mesoscopic scale, J. Geophys. Res.-Atmos., 117, D17302, https://doi.org/10.1029/2012JD017817, 2012.
Miyazaki, K., Eskes, H., Sudo, K., Boersma, K. F., Bowman, K., and Kanaya, Y.: Decadal changes in global surface NOx emissions from multi-constituent satellite data assimilation, Atmos. Chem. Phys., 17, 807–837, https://doi.org/10.5194/acp-17-807-2017, 2017.
Myles, L., Meyers, T. P., and Robinson, L.: Relaxed eddy accumulation measurements of ammonia, nitric acid, sulfur dioxide and particulate sulfate dry deposition near Tampa, FL, USA, Environ. Res. Lett., 2, 034004, https://doi.org/10.1088/1748-9326/2/3/034004, 2007.
OpenStreetMap contributors: Indian power plant location, OpenStreetMap [data set], https://openinframap.org/stats/area/India/plants (last access: 29 July 2024), 2024.
Oppenheimer, C., Scaillet, B., and Martin, R. S.: Sulfur Degassing From Volcanoes: Source Conditions, Surveillance, Plume Chemistry and Earth System Impacts, Rev. Mineral. Geochem., 73, 363–421, https://doi.org/10.2138/rmg.2011.73.13, 2011.
Press Trust of India (PTI): NTPC starts capturing CO2 from flue gas stream at Vindhyachal plant, Press Trust of India (PTI), https://energy.economictimes.indiatimes.com/news/oil-and-gas/ntpc-starts-capturing-co2-from-flue-gas-stream-at-vindhyachal-plant/93661446 (last access: 5 February 2025), 2022.
Qu, Z., Henze, D. K., Li, C., Theys, N., Wang, Y., Wang, J., Wang, W., Han, J., Shim, C., Dickerson, R. R., and Ren, X.: SO2 Emission Estimates Using OMI SO2 Retrievals for 2005–2017, J. Geophys. Res.-Atmos., 124, 8336–8359, https://doi.org/10.1029/2019JD030243, 2019.
Rodriguez-Villamizar, L. A., Magico, A., Osornio-Vargas, A., and Rowe, B. H.: The Effects of Outdoor Air Pollution on the Respiratory Health of Canadian Children: A Systematic Review of Epidemiological Studies, Can. Respir. J., 22, 263427, https://doi.org/10.1155/2015/263427, 2015.
Serbula, S., Ţivkovic, D., Radojevic, A., Kalinovic, T., and Kalinovic, J.: Emission of SO2 and SO from copper smelter and its influence on the level of total s in soil and moss in Bor and the surroundings, Hem. Ind., 69, 18–18, https://doi.org/10.2298/HEMIND131003018S, 2014.
Shukla, J., Sundar, S., S., and Naresh, R.: Modeling and analysis of the acid rain formation due to precipitation and its effect on plant species, Nat. Resour. Model., 26, 53–65, 2013.
Slinn, W. G. N., Hasse, L., Hicks, B. B., Hogan, A. W., Lal, D., Liss, P. S., Munnich, K. O., Sehmel, G. A., and Vittori, O.: Some aspects of the transfer of atmospheric trace constituents past the air-sea interface, Atmos. Environ., 12, 2055–2087, https://doi.org/10.1016/0004-6981(78)90163-4, 1978.
Smith, S. J., van Aardenne, J., Klimont, Z., Andres, R. J., Volke, A., and Delgado Arias, S.: Anthropogenic sulfur dioxide emissions: 1850–2005, Atmos. Chem. Phys., 11, 1101–1116, https://doi.org/10.5194/acp-11-1101-2011, 2011.
Soulie, A.: CAMS Global anthropogenic emissions, ECCAD [data set], https://permalink.aeris-data.fr/CAMS-GLOB-ANT (last access: 29 July 2024), 2024.
Soulie, A., Granier, C., Darras, S., Zilbermann, N., Doumbia, T., Guevara, M., Jalkanen, J.-P., Keita, S., Liousse, C., Crippa, M., Guizzardi, D., Hoesly, R., and Smith, S. J.: Global anthropogenic emissions (CAMS-GLOB-ANT) for the Copernicus Atmosphere Monitoring Service simulations of air quality forecasts and reanalyses, Earth Syst. Sci. Data, 16, 2261–2279, https://doi.org/10.5194/essd-16-2261-2024, 2024.
Souri, A. H., Duncan, B. N., Strode, S. A., Anderson, D. C., Manyin, M. E., Liu, J., Oman, L. D., Zhang, Z., and Weir, B.: Enhancing long-term trend simulation of the global tropospheric hydroxyl (TOH) and its drivers from 2005 to 2019: a synergistic integration of model simulations and satellite observations, Atmos. Chem. Phys., 24, 8677–8701, https://doi.org/10.5194/acp-24-8677-2024, 2024.
Spivakovsky, C. M., Logan, J. A., Montzka, S. A., Balkanski, Y. J., Foreman-Fowler, M., Jones, D. B. A., Horowitz, L. W., Fusco, A. C., Brenninkmeijer, C. A. M., Prather, M. J., Wofsy, S. C., and McElroy, M. B.: Three-dimensional climatological distribution of tropospheric OH: Update and evaluation, J. Geophys. Res.-Atmos., 105, 8931–8980, https://doi.org/10.1029/1999JD901006, 2000.
Steinfeld, J. I.: Atmospheric Chemistry and Physics: From Air Pollution to Climate Change, Environment: Science and Policy for Sustainable Development, 40, 26–26, https://doi.org/10.1080/00139157.1999.10544295, 1998.
Theys, N.: TROPOMI Level-2 COBRA SO2 data, Royal Belgian Institute for Space Aeronomy (BIRA-IASB) [data set], https://data-portal.s5p-pal.com/products/so2cbr.html (last access: 29 July 2024), 2024a.
Theys, N.: TROPOMI Level-2 COBRA SO2 data, Royal Belgian Institute for Space Aeronomy (BIRA-IASB) [data set], https://distributions.aeronomie.be (last access: 29 July 2024), 2024b.
Theys, N., De Smedt, I., Yu, H., Danckaert, T., van Gent, J., Hörmann, C., Wagner, T., Hedelt, P., Bauer, H., Romahn, F., Pedergnana, M., Loyola, D., and Van Roozendael, M.: Sulfur dioxide retrievals from TROPOMI onboard Sentinel-5 Precursor: algorithm theoretical basis, Atmos. Meas. Tech., 10, 119–153, https://doi.org/10.5194/amt-10-119-2017, 2017.
Theys, N., Fioletov, V., Li, C., De Smedt, I., Lerot, C., McLinden, C., Krotkov, N., Griffin, D., Clarisse, L., Hedelt, P., Loyola, D., Wagner, T., Kumar, V., Innes, A., Ribas, R., Hendrick, F., Vlietinck, J., Brenot, H., and Van Roozendael, M.: A sulfur dioxide Covariance-Based Retrieval Algorithm (COBRA): application to TROPOMI reveals new emission sources, Atmos. Chem. Phys., 21, 16727–16744, https://doi.org/10.5194/acp-21-16727-2021, 2021.
Tsikerdekis, A., Hasekamp, O. P., Schutgens, N. A. J., and Zhong, Q.: Assimilation of POLDER observations to estimate aerosol emissions, Atmos. Chem. Phys., 23, 9495–9524, https://doi.org/10.5194/acp-23-9495-2023, 2023.
van der A, R. J., Mijling, B., Ding, J., Koukouli, M. E., Liu, F., Li, Q., Mao, H., and Theys, N.: Cleaning up the air: effectiveness of air quality policy for SO2 and NOx emissions in China, Atmos. Chem. Phys., 17, 1775–1789, https://doi.org/10.5194/acp-17-1775-2017, 2017.
Veefkind, J. P., Aben, I., McMullan, K., Förster, H., de Vries, J., Otter, G., Claas, J., Eskes, H. J., de Haan, J. F., Kleipool, Q., van Weele, M., Hasekamp, O., Hoogeveen, R., Landgraf, J., Snel, R., Tol, P., Ingmann, P., Voors, R., Kruizinga, B., Vink, R., Visser, H., and Levelt, P. F.: TROPOMI on the ESA Sentinel-5 Precursor: A GMES mission for global observations of the atmospheric composition for climate, air quality and ozone layer applications, Remote Sens. Environ., 120, 70–83, https://doi.org/10.1016/j.rse.2011.09.027, 2012.
Venkataraman, C., Brauer, M., Tibrewal, K., Sadavarte, P., Ma, Q., Cohen, A., Chaliyakunnel, S., Frostad, J., Klimont, Z., Martin, R. V., Millet, D. B., Philip, S., Walker, K., and Wang, S.: Source influence on emission pathways and ambient PM2.5 pollution over India (2015–2050), Atmos. Chem. Phys., 18, 8017–8039, https://doi.org/10.5194/acp-18-8017-2018, 2018.
Vladimir, O., James, B., Sander, S., J, D., Barker, J., Robert, H., Kolb, C., Michael, K., David, W., and Wine, P.: Chemical Kinetics and Photochemical Data for Use in Atmospheric Studies, Evaluation No. 18, JPL Publication 15-10, Jet Propulsion Laboratory, Pasadena, http://jpldataeval.jpl.nasa.gov (last access: 11 February 2025), 2015.
Wang, J., Ryan, D., Anthony, E., Wildgust, N., and Aiken, T.: Effects of Impurities on CO2 Transport, Injection and Storage, Enrgy. Proced., 4, 3071–3078, https://doi.org/10.1016/j.egypro.2011.02.219, 2011.
Zheng, B., Tong, D., Li, M., Liu, F., Hong, C., Geng, G., Li, H., Li, X., Peng, L., Qi, J., Yan, L., Zhang, Y., Zhao, H., Zheng, Y., He, K., and Zhang, Q.: Trends in China's anthropogenic emissions since 2010 as the consequence of clean air actions, Atmos. Chem. Phys., 18, 14095–14111, https://doi.org/10.5194/acp-18-14095-2018, 2018.