the Creative Commons Attribution 4.0 License.
the Creative Commons Attribution 4.0 License.
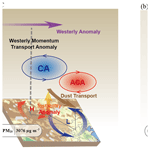
Distinctive dust weather intensities in North China resulted from two types of atmospheric circulation anomalies
Qianyi Huo
Zhicong Yin
Xiaoqing Ma
Huijun Wang
Dust weather in North China (NC; 34–42° N, 105–120° E) has worsened in recent years, posing adverse impacts on the environment, human health, and the economy. A super dust storm that occurred on 15 March 2021 raised Beijing's PM10 (particulate matter with a diameter less than 10 µm) concentrations above 7000 µg m−3, while 2023 witnessed the highest spring dust weather frequency in nearly a decade. Previous research has primarily focused on the role of the Mongolian cyclone in influencing dust weather in NC, with less attention given to other synoptic systems. Additionally, the differences in PM10 concentrations in NC caused by different synoptic systems have not yet been quantified. This study demonstrates that the Mongolian cyclone was responsible for 61.7 % of the dust weather in NC, while the remaining 38.3 % was primarily caused by the cold high. The dust intensity induced by the Mongolian cyclone was stronger than that of the cold high, with average maximum PM10 concentrations of 3076 and 2391 µg m−3, respectively. The three-dimensional structure of atmospheric circulation anomalies and related dynamic mechanisms of the two types were concluded. To comprehensively forecast the two types of dust weather, a common predictor was constructed based on the 500 hPa cyclonic anomaly and anticyclonic anomaly circulation systems. These findings contribute to enhancing the comprehension of dust weather in NC and offer insights for both dust weather forecasting and climate prediction.
- Article
(8824 KB) - Full-text XML
-
Supplement
(3427 KB) - BibTeX
- EndNote
Dust weather frequently occurs in spring over the northern region of China and has a number of negative effects on the environment, human health, and economy. The strong wind, which induces the dust weather, has the potential to inflict severe damage on infrastructure and results in soil erosion, thereby exerting detrimental effects on agricultural productivity (Ahmadzai et al., 2023). Simultaneously, the entrainment of dust particles by the strong wind leads to an increase in PM10 (particulate matter with a diameter less than 10 µm) concentration (Krasnov et al., 2016), which reduces air quality and poses a threat to human health, increasing the risk of respiratory and cardiovascular diseases (Lwin et al., 2023). In addition, dust aerosols reduce visibility (Gui et al., 2022), impeding people's travel and adversely affecting traffic safety.
North China (NC; 34–42° N, 105–120° E) is the region, apart from Northwest China, where spring dust weather is most frequent and intense and which has the highest average PM10 concentration (Li et al., 2022; Zhang et al., 2023). In contrast to previous studies that examined dust weather across the entire northern region of China, this study specifically focuses on NC. With a dense population and developed economy, and playing a vital role in China's economy, politics, culture, and agriculture, studying dust weather in NC is of great importance. In response to global warming, extreme weather has been intensifying and occurring more frequently in China (Yin et al., 2023b). A severe dust storm reoccurred in the spring of 2021 after an absence for more than 10 years in NC (Zhang et al., 2022). During 14–16 March 2021, the hourly observed PM10 concentration exceeded the monitoring threshold in Ulanqab (> 9985 µg m−3) and reached an extraordinarily high value in Beijing (> 7400 µg m−3; Yin et al., 2022). In the spring of 2023, there was a notable increase in the frequency of dust weather, reaching the highest level observed in recent decades (Yin et al., 2023a).
The generation of dust weather requires both dust source conditions and dynamic conditions. The primary dust sources of dust weather include deserts and sandy areas in arid and semi-arid regions (Huang et al., 2014). In East Asia, the Gobi Desert located in the Mongolian Plateau and northern China is a major source of sandstorms (Zhang et al., 2023). In recent years, China has made significant progress in combating land desertification (Zhang and Huisingh, 2018). However, during the dust weather events in March and April 2023, over 42 % of the dust concentration in NC originated from cross-border transport from Mongolia (Chen et al., 2023a). Strong winds and thermally unstable atmospheric stratification were the primary dynamic conditions for the formation of dust weather (Wu et al., 2023), which facilitated the entrainment and uplift of dust particles (Zhao et al., 2022). The intensity of turbulence and the structure of the boundary layer affect the lifting and diffusion capabilities of sand and dust (Shao, 2008). The combined effect of thermal (i.e., unstable stratification) and dynamic (i.e., near-surface wind shear) factors enhances turbulent motion, leading to increased wind erosion, which favors the lifting of sand and dust (Wiggs, 2011).
The long-distance transport of dust aerosols in the lower atmosphere is regulated by regional synoptic systems (Huang et al., 2014). Meanwhile, the frequent dust weather activities in East Asia in spring are closely related to mid-latitude synoptic-scale cyclone activities (Qian et al., 2002). The Mongolian cyclone is the primary synoptic system causing dust weather in NC (Li et al., 2022). Both the severe dust storm events on 15 March 2021 and on 22 March 2023 were attributed to the Mongolian cyclone (Mu et al., 2023; Yin et al., 2023a). The appearance of the Mongolian cyclone triggered strong gusts and unstable thermal conditions, disturbed loose surfaces, and lifted surface dust into the air through upward motion (Tian et al., 2023). As the Mongolian cyclone moved eastward, the northerly winds behind it transported dust from the source area southward, thereby affecting NC (Takemi and Seino, 2005).
Recent weather and climate studies on the atmospheric circulation systems related to dust weather in NC have primarily focused on the frequency, intensity, and physical processes of the Mongolian cyclone (Wu et al., 2016; Bueh et al., 2022; Chen et al., 2023b; Gao et al., 2024). However, the Mongolian cyclone alone cannot explain all instances of dust weather. According to the daily maximum observed PM10 concentration in NC, a dust event caused by a cold high resulted in a relatively low PM10 concentration of 1247 µg m−3 on 14 March 2023 (Fig. S1a in the Supplement). This event went largely unnoticed. However, on 22 March 2023, a severe dust storm, brought by a Mongolian cyclone (Fig. S1b), led to higher PM10 concentrations of 9993 µg m−3, garnering more attention (Yin et al., 2023a). Research on subjective and objective classifications of dust weather has been conducted (Liu et al., 2004; Yun et al., 2013; Yi et al., 2021). These studies indicate that besides the Mongolian cyclone, other synoptic systems such as the cold high also played significant roles in causing dust weather in North China.
This study used PM10 concentrations as indicators to investigate the differences in the intensity of dust weather caused by the Mongolian cyclone and other synoptic systems. The differences and similarities in atmospheric circulation anomalies between the two types were compared. In order to comprehensively predict both types of dust weather, common anomalous circulation systems for the two types have been identified, and a common predictor has been established. This study helps enhance the understanding of dust weather in NC and provides references for dust weather forecasting and climate prediction.
2.1 Data
Hourly observed station PM10 and PM2.5 concentrations in March, April, and May from 2015 to 2023 are derived from the China National Environmental Monitoring Centre and publicly accessible at https://quotsoft.net/air/ (last access: 6 April 2024). The study area is located in NC, specifically within the range of 34–42° N, 105–120° E. PM10 and PM2.5 concentration data from 556 stations in NC have been utilized for selecting dust weather days, with negative and missing values excluded. The fifth-generation European Centre for Medium-Range Weather Forecasts (ERA5) provided hourly reanalysis meteorology data with the horizontal resolution of 0.25° × 0.25° on pressure and surface levels in spring (March, April, and May) from 2015 to 2023 (Hersbach et al., 2023a, b). The variables include geopotential height at 500 hPa (Z500), zonal and meridional winds at 10 m (UV10), zonal and meridional winds at 850 hPa (UV850), zonal winds at 200 hPa (U200), sea level pressure (SLP), temperature at 1000 hPa (T1000), temperature at 850 hPa (T850), vertical velocity at 500 hPa (ω500), 10 m wind gust (Gust10), surface air temperature (SAT), planetary boundary layer height (PBLH), vertical velocity (ω), zonal and meridional winds, divergence (div), and specific humidity (q) from 1000 to 200 hPa on 23 pressure levels.
The Normalized Difference Vegetation Index (NDVI) quantifies the vegetation by measuring the difference between near-infrared and red light. Gridded NDVI data, with a horizontal resolution of 1° × 1° in March 2023, were obtained from the National Oceanic and Atmospheric Administration's (NOAA) National Centers for Environmental Information (Vermote, 2019). The Copernicus Climate Change Service (C3S) provided seasonal forecast products from the European Centre for Medium-Range Weather Forecasts (ECMWF) SEAS5.1; Deutscher Wetterdienst (DWD) GCFS2.0 and GCFS2.1; and Météo-France (MF) System 6, System 7, and System 8 (Copernicus Climate Change Service, Climate Data Store, 2018a, b). In this study, the Z500 and SLP data for spring were initialized annually on 1 February (1-month lead) with a temporal resolution of 12 h and a spatial resolution of 1° × 1°. The daily ensemble mean data for each variable are used in this study.
2.2 Method
During dust events, PM10 concentrations significantly increase, at least doubling or even increasing by tens of times (Dulam et al., 2014). In this study, the maximum PM10 concentration was utilized to confirm the occurrence of dust weather in NC. In order to better identify dust days, the daily maximum PM10 concentrations were selected from the actual hourly observed values for each station in NC. The station with the highest PM10 concentration in NC was chosen, and the maximum value at that station was used as a reference for selecting dust day. The changes in daily maximum PM10 concentrations observed in NC during spring 2015–2023 are illustrated in Fig. S2. The first and third quartiles of the PM10 concentration series in spring 2015–2023 were 426.5 and 1019 µg m−3, respectively. Referring to selected percentile values, two PM10 concentration thresholds were chosen at 500 and 1000 µg m−3. Periods when PM10 concentrations exceeded 1000 µg m−3 were defined as high concentration periods, while periods below 500 µg m−3 were categorized as low concentration periods. In this study, the dates corresponding to the maximum PM10 concentrations during high PM10 concentration periods were designated as dust days (Fig. S2), representing days most significantly impacted by dust in NC during dust events. Conversely, the dates corresponding to the minimum PM10 concentrations during low PM10 concentration periods were designated as non-dust days (Fig. S2).
Considering potential anthropogenic influences, the PM2.5 concentrations were obtained at the same site and time as the maximum PM10 concentrations on the dust days, and the PM2.5 PM10 ratios were calculated. A lower PM2.5 PM10 ratio indicates the presence of a significant amounts of coarse particles in the air, associated with natural sources such as dust storms, while a higher PM2.5 PM10 ratio suggests pollution from anthropogenic sources (Sugimoto et al., 2016). In NC, the average PM2.5 PM10 ratio typically ranges from 0.5 to 0.7 and exhibits distinct seasonal variations, being lower in spring (Wang et al., 2015). To eliminate the influence of anthropogenic aerosols, 2 d were excluded from the selected dust days as the PM2.5 PM10 ratio exceeded 0.5 on these days.
According to the synoptic definition of the extratropical cyclone (Shou, 2006), the Mongolian cyclone was identified based on the following criteria: (1) the lowest SLP within the range of 40–55° N, 100–130° E should not exceed 1010 hPa. (2) The average pressure gradient within a ±2.5° latitude and longitude range around the lowest SLP must be equal to or greater than 0.55 hPa per 100 km. The vertical air temperature difference (VATD) was defined as T1000–T850, which indicates the thermal atmospheric instability. The vertical transport of westerly momentum was defined as , where < 0 represents downward fluxes (Zhong et al., 2019). The composites of the variables were computed based on the daily mean values. All anomalies were calculated relative to the daily mean values in spring from 2015 to 2023. The correlation coefficients in this study were calculated using Pearson correlation.
The primary surface synoptic system leading to dust weather in NC was the Mongolian cyclone. Additionally, other synoptic systems could also contribute to dust weather affecting NC (Liu et al., 2004). By objectively identifying the presence of the Mongolian cyclone, dust days were further classified into two categories. As depicted in the original SLP fields for the two types, the main surface synoptic systems for the two types of dust days were the Mongolian cyclone and cold high, respectively (Fig. S1c, d). Therefore, the two types of dust days were, respectively, named Mongolian cyclone (MC) type and cold high (CH) types. Dust days caused by Mongolian cyclones (MC type) accounted for a significant portion during the spring seasons from 2015 to 2023, at 61.7 %. Other synoptic systems, mainly cold high systems (CH type), accounted for 38.3 % of the total dust days. Figure 1c illustrates the temporal distribution of MC days, CH days, and non-dust days in spring from 2015 to 2023.
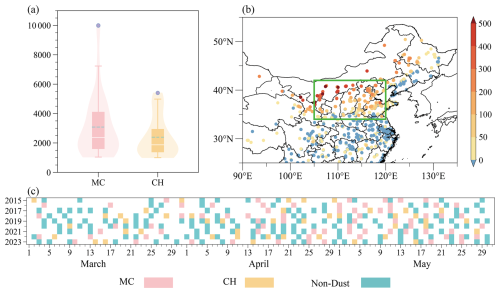
Figure 1(a) Box plots of daily maximum PM10 concentrations (units: µg m−3) in NC during MC days (pink) and CH days (orange). The dashed cyan lines and blue dots in the box plot represent average PM10 concentrations and outlier values. Density distributions of PM10 concentrations are shown by pink and orange shading for MC days and CH days, respectively. (b) The composite differences of observed daily maximum PM10 concentrations (scatter, units: µg m−3) during MC days relative to CH days. The green box indicates NC. (c) The temporal distribution of MC days, CH days, and non-dust days in spring from 2015 to 2023.
There are distinct differences in PM10 concentrations between the MC and CH types. Both of the two types exhibited high PM10 concentrations in NC (Fig. S3). Compared to the CH type, the MC type resulted in higher PM10 concentrations and showed more pronounced extremes (Fig. 1a). The outliers in the PM10 concentration box plot for the MC type in Fig. 1a included the severe dust storms on 15 March 2021 and 22 March 2023. During dust days, the dust particles that caused the increase in PM10 concentrations originated from the dust source area. From the NDVI, it can be observed that there was a significant lack of vegetation cover in the northwest direction outside NC (Fig. S3a). This area (NDVI < 0.1), serving as an external dust source region, could provide favorable conditions for dust emissions (Wang et al., 2021). From the spatial distribution differences in PM10 concentrations, it can be observed that the MC type resulted in relatively higher PM10 concentrations in NC, especially in its northern region (Fig. 1b).
During dust days of MC and CH types, there were strong anomalous northerly winds to the north of NC at the surface (Fig. 2d, e). During MC days, due to the presence of the Mongolian cyclone (Fig. S1c), there was a significant negative SLP anomaly in the eastern part of East Asia, with a positive anomaly in the west (Fig. 2a). The rear part of the low-pressure anomaly exhibited strong anomalous northwest winds (Fig. 2d). During CH days, the northern part of East Asia exhibited a significant cold high-pressure anomaly, with a low-pressure anomaly in the south (Fig. 2b). Between the two anomalous circulations, there were northeasterly anomalous winds to the north of NC (Fig. 2e). The easterly wind anomaly components weakened the westerly wind components of the surface winds in the CH type. For CH days, the actual wind direction was more northerly compared to MC days (Fig. S1c, d).
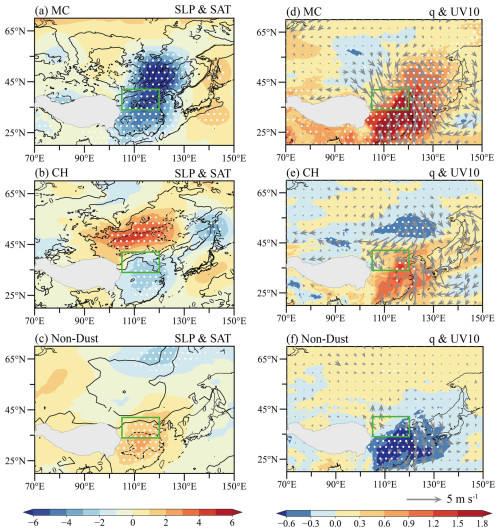
Figure 2(a) Composite anomalies of SLP (shading, units: hPa) and SAT (contour, units: K) during MC days. White dots indicate that SLP anomalies exceed the 95 % confidence level. Panels (b) and (c) are the same as panel (a) but for CH days and non-dust days. (d) Composite anomalies of q (shading, units: 10−3 kg kg−1) and UV10 (vectors, units: m s−1) during MC days. White dots indicate that q anomalies exceed the 95 % confidence level. Panels (e) and (f) are the same as panel (d) but for CH days and non-dust days. The green boxes in panels (a)–(f) represent NC.
Compared to the CH type, the MC type exhibited stronger dynamic instability, manifested by higher anomalies in Gust10 (Fig. S4a, b). Additionally, the increase in VATD favored atmospheric thermal instability in NC and the surrounding external dust source areas (Fig. S4a, b). The combination of dynamic and thermal instability enhanced turbulence in the atmospheric boundary layer (Garratt, 1992). The anomalously intensified turbulence aided in lifting surface dust particles into the atmosphere (Wiggs, 2011), while also elevating the PBLH in NC and the surrounding external dust source areas (Fig. S4c, d). The higher PBLH provided favorable conditions for the dispersion of dust particles from external dust source areas towards NC (Shao, 2008). It is observed that there were anomalous divergent winds over the external dust source areas on MC and CH days (Fig. 2d, e). The anomalous northerly wind facilitated the transport of dust particles from external dust source areas to NC (Fig. 2d, e), leading to the increase in PM10 concentrations. The anomalous divergent winds not only aided in outward transport of dust particles but also suppressed the transport of moisture towards the dust source areas. This resulted in anomalously dry conditions near the surface of the external dust source areas (Fig. 2d, e), promoting dust emissions.
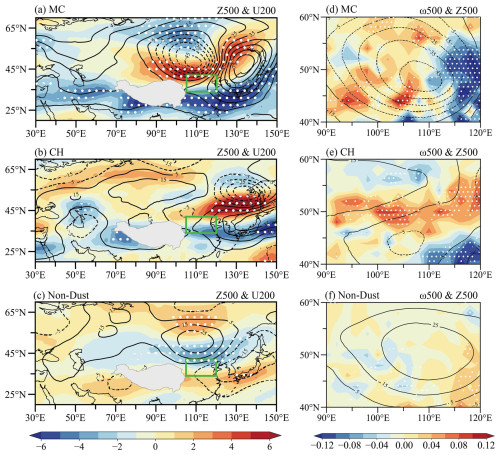
Figure 3(a) Composite anomalies of Z500 (contour, units: gpm) and U200 (shading, units: m s−1) during MC days. White dots indicate that U200 anomalies exceed the 95 % confidence level. Panels (b) and (c) are the same as panel (a) but for CH days and non-dust days. The green boxes in panels (a)–(c) represent NC. (d) Composite anomalies of ω500 (shading, units: Pa s−1) and Z500 (contour, units: gpm) during MC days. White dots indicate that ω500 anomalies exceed the 95 % confidence level. Panels (e) and (f) are the same as panel (d) but for CH days and non-dust days.
Although there were significant differences in the surface circulation anomaly patterns between the MC and CH types, some similar features could be observed in the middle to upper troposphere. The MC type and CH type displayed intensified westerly winds over the mid-latitude East Asia region at 200 hPa (Fig. 3a, b). At 500 hPa, both the MC and CH types exhibited cyclonic anomalies to the north of NC and anticyclonic anomalies to the east of NC (Fig. 3a, b). Compared to the CH type, the MC type showed stronger negative geopotential height anomalies at 500 hPa, with the center located in the northern part outside of NC (Fig. 3a). In contrast, the geopotential height anomalies to the north of NC at 500 hPa were weaker for the CH type (Fig. 3b). Since this 500 hPa cyclonic anomaly is located adjacent to the northern part of NC and over the sparsely vegetated external dust source area to the northwest of NC (Fig. S3a), we hypothesize that this 500 hPa cyclonic anomaly (CA) is a key anomalous circulation system influencing dust activities in NC. The vertical circulation structure of CA was further analyzed. During dust days, CA exhibited an asymmetric vertical structure (Fig. 3d, e). For the MC type, the western part of the CA showed positive ω anomalies, while the eastern part showed negative ω anomalies (Fig. 3d). For the CH type, the positive ω anomalies were more biased towards the northwest of CA, with negative ω anomalies in the southeastern part of CA (Fig. 3e).
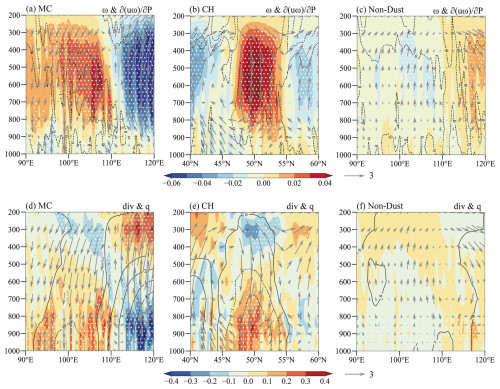
Figure 4Composite anomalies of zonal component of the vertical circulation average over 40–60° N, 90–120° E during MC days: (a) the variables include ω (shading, units: Pa s−1) and downward transport of westerly momentum (< 0, dashed contour, units: 10−3 m s−2). White dots indicate that ω anomalies exceed the 95 % confidence level. The vectors represent ω (magnified 100 times) and zonal wind. (d) The variables include divergence (shading, units: 10−5 s−1) and q (contour, units: 10−4 kg kg−1). White dots indicate that divergence anomalies exceed the 95 % confidence level. The vectors represent ω (magnified 100 times) and zonal wind. Panels (c) and (f) are the same as panels (a) and (d), respectively, but for non-dust days. Composite anomalies of meridional component of the vertical circulation average over 40–60° N, 90–120° E during CH days: (b) the variables include ω (shading, units: Pa s−1) and downward transport of westerly momentum (< 0, dashed contour, units: 10−3 m s−2). White dots indicate that ω anomalies exceed the 95 % confidence level. The vectors represent ω (magnified 100 times) and meridional wind. (e) The variables include divergence (shading, units: 10−5 s−1) and q (contour, units: 10−4 kg kg−1). White dots indicate that divergence anomalies exceed the 95 % confidence level. The vectors represent ω (magnified 100 times) and meridional wind.
The vertical circulation anomalies associated with CA were primarily related to the emission and transport of dust from the external dust source areas outside of NC. The anomalous subsidence facilitated the downward transport of westerly momentum from the upper troposphere to near the surface (Fig. 4a, b), favoring the enhancement of anomalies in V850 and Gust10 (Fig. S4). With the strengthening of westerly momentum exchange, the conversion of kinetic energy toward turbulent flow was enhanced (Liu and Liu, 2011). Furthermore, the anomalous subsidence also helped to transport dry and cold air from the mid-troposphere to layers closer to the surface (Fig. 4d, e). The intrusion of cold air helped to disrupt the stable boundary layer, resulting in increased atmospheric thermal instability and turbulent kinetic energy (Liu and Liu, 2011). The stronger turbulence not only lifted surface dust particles but also led to higher PBLH (Fig. S4c, d). The anomalous divergence in the lower troposphere provided favorable conditions for the outward spread of dust particles (Fig. 4d, e). Anomalous convergence zones promoted the aggregation of dust particles and transported them to higher altitudes through anomalous upward motion (Fig. 4d, e). This prolonged the settling time of dust particles and facilitated their long-distance transport.
The east–west contrast in the vertical structure of the CA was weaker for the CH type compared to the MC type (Fig. S5a), whereas the north–south contrast for the CH type was more pronounced (Fig. 4b, e). For the CH type, the enhanced anomalous meridional vertical circulation increased the importance of the dust source areas to the north of NC (Fig. S3a). The vegetation cover outside of NC along the northern direction was relatively better than that along the northwest direction outside of NC, indicated by higher NDVI (Fig. S3a). This might lead to lower PM10 concentrations in NC during CH days compared to MC days. Furthermore, in NC and external dust source areas, meteorological variable anomalies of the CH type exhibited spatial distribution characteristics similar to the MC type but with weaker intensities (Figs. 2d and e and S4). Spatial correlation coefficient results indicated a significant linear correlation between these meteorological variables and the maximum PM10 concentrations in NC (Fig. S6). These results partially explained why the PM10 concentrations on CH days are lower relative to MC days.
For comparison, the atmospheric circulation anomalies during non-dust days were also analyzed. The atmospheric circulation anomalies during non-dust days exhibited distribution characteristics opposite to those on dust days. The southeastern part of East Asia showed positive SLP anomalies, while the northwestern part exhibited negative SLP anomalies and warm anomalies (Fig. 2c). The wind speed anomalies in NC and its surrounding areas were relatively low, with anomalous southerly winds being conducive to the transport of moisture from the south, leading to higher q (Fig. 2f). At 500 hPa, an anticyclonic anomaly was observed to the north of NC, while to the east of NC a cyclonic anomaly occurred (Fig. 3c). In the upper troposphere, the westerly winds in the mid-latitudes of East Asia were significantly weakened (Fig. 3c), and the subsidence and downward momentum transport weakened (Figs. 3f and 4c). These circulation anomalies were unfavorable for the occurrence of dust weather.
Table 1The definition of meteorological indices related to dust weather in NC and correlation coefficients (R) of observed daily maximum PM10 concentrations over NC with each index in spring from 2015 to 2023. All indices were the normalized area-averaged corresponding variables for their corresponding areas. All the correlation coefficients exceed the 99 % confidence level.
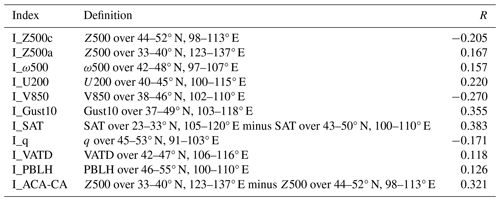
Table 2The correlation coefficients of I_Z500c, I_Z500a and I_ACA-CA with other meteorological indices (Table 1) in spring from 2015 to 2023. All the correlation coefficients exceed the 99 % confidence level. The symbol “–” denotes that the correlation coefficient is not statistically significant and therefore has been excluded from the analysis.

The analysis results in Sect. 4 indicate that the anomalous circulation patterns and meteorological conditions during MC days, CH days, and non-dust days exhibited a certain linear correlation with the maximum PM10 concentration in NC. It is also found that the 500 hPa cyclonic anomaly (CA) may be a key anomalous circulation system influencing MC and CH types. In order to comprehensively predict dust weather of the MC and CH types, we defined a series of meteorological indices to explore the common anomalous circulation systems influencing these two dust weather types. We calculated indices by selecting regions with the most significant spatial correlation coefficients between meteorological variables and PM10 concentrations. These indices exhibited significant correlations with the maximum PM10 concentration in NC with confidence levels exceeding 99 % (Table 1). The composite values of the meteorological indices before normalization also showed significant differences during MC days, CH days, and non-dust days (Fig. 5b–i), correlating with the composite PM10 concentrations of the three categories (Fig. 1a). The 500 hPa cyclonic anomaly (CA) and anticyclonic anomaly (ACA) circulation systems were represented by the 500 hPa geopotential height indices I_Z500c and I_Z500a (Table 1). While I_Z500c showed significant correlations with other related meteorological indices, it could not explain the anomalies in VATD and PBLH well on dust days (Table 2). If only CA is considered, it may not be sufficient to provide the thermodynamic instability conditions for dust weather. When considering the role of ACA, it can be observed that I_Z500a exhibited a stronger correlation with VATD and PBLH, with correlation coefficients reaching 0.639 and 0.534, respectively (Table 2). However, ACA alone may not explain the anomalies in specific humidity (q) on dust days effectively.
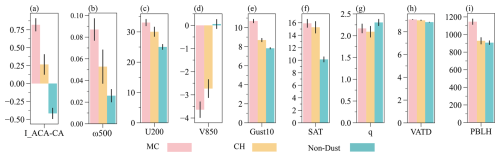
Figure 5Composite meteorological index (a) I_ACA-CA and composite meteorological variables (composite values of corresponding meteorological indices before normalization in Table 1): (b) ω500 (unit: Pa s−1), (c) U200 (unit: m s−1), (d) V850 (unit: m s−1), (e) Gust10 (unit: m s−1), (f) SAT (unit: K), (g) q (unit: 10−3 kg kg−1), (h) VATD (unit: K), and (i) PBLH (unit: m) during MC days, CH days, and non-dust days. The black error bars indicate the standard error.
By considering CA and ACA together, calculating the difference in Z500 between them and normalizing it, the index I_ACA-CA was defined. I_ACA-CA is significantly correlated with the maximum PM10 concentration in NC (R = 0.321, exceeding the 99 % confidence level), with an absolute correlation coefficient higher than I_Z500c ( = 0.205) and I_Z500a ( = 0.167). During MC days, CH days, and non-dust days, the composite values of I_ACA-CA also showed significant differences, corresponding to the composite PM10 concentration values during these days (Fig. 5a). Furthermore, I_ACA-CA exhibited significant correlations with meteorological conditions and horizontal circulation influencing dust weather in NC (Table 2), consistent with the physical mechanisms described in Sect. 4. Therefore, CA and ACA are closely related to dust weather in NC and are key anomalous circulation systems. I_ACA-CA is designated as a common predictor for the two types of dust days in NC. Figure 6 displays the daily I_ACA-CA values in spring from 2015 to 2023, where positive I_ACA-CA captured 84.5 % of MC days, 63.6 % of CH days, and 76.5 % of dust days overall. All the dust days in 2016 and 2023 were captured by I_ACA-CA (Fig. 6). In 2021, only one instance of lower PM10 concentration was not captured, with the rest being captured (Fig. 6). In correspondence with the positive I_ACA-CA observed 2 d, 1 d (I_ACA-CA > its 1 standard deviation), and 0 d (I_ACA-CA > its 1 standard deviation) in advance, successful capture rates of 56.5 %, 69.6 %, and 76.5 % for dust days were achieved. These high percentages suggest that the reinforced positive I_ACA-CA significantly contributed to the high PM10 concentrations in NC.
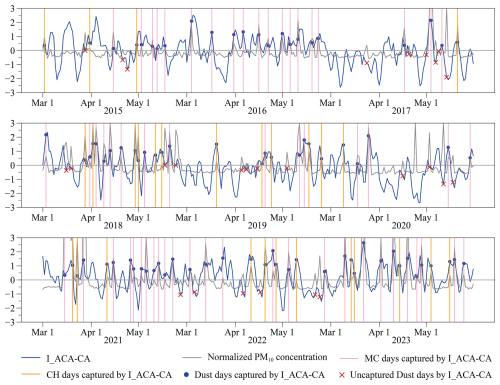
Figure 6Daily I_ACA-CA (blue line) and normalized daily maximum PM10 concentrations observed in NC (grey line) in March (Mar), April (Apr), and May from 2015 to 2023. The blue dots indicate the dust days captured when I_ACA-CA > 0 and the corresponding I_ACA-CA value. The pink and yellow vertical lines indicate the MC days and CH days captured when I_ACA-CA > 0, respectively. The red crosses represent the dust days that I_ACA-CA failed to capture.
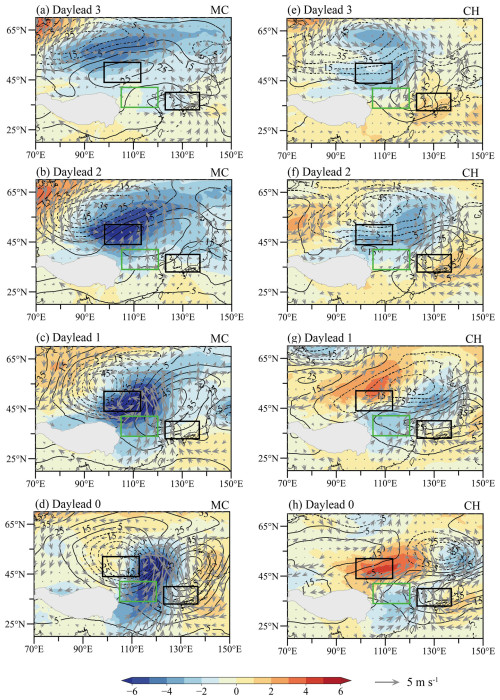
Figure 7(a–d) Lead composite evolution of Z500 (contour, unit: gpm) anomalies, SLP (shading, unit: hPa) anomalies, and UV850 (vectors, units: m s−1) anomalies during MC days. Panels (e)–(h) are the same as panels (a)–(d) but for CH days. The green boxes in panels (a)–(h) represent NC, while the black boxes represent the region for calculating I_ACA-CA.
The evolution of CA, ACA, and related atmospheric circulation anomalies before MC days and CH days was further investigated (Fig. 7). Prior to both types of dust days, CA and ACA moved eastward towards NC (Fig. 7). For the MC type, CA and ACA from western Siberia and Lake Baikal gradually strengthened as they moved eastward (Fig. 7a–d). The development of the Mongolian cyclone intensified (Fig. S7a–d), accompanied by an eastward strengthening of the associated surface low-pressure anomaly and cyclonic winds to the northwest of NC (Fig. 7a–c). As the surface low pressure anomaly moved eastward, the anomalous southerly winds north of NC shifted to anomalous northerly winds (Fig. 7c, d). The actual wind directions changed from westerly to northwesterly (Fig. S7c, d). For the CH type, CA and ACA were relatively positioned more to the east (Fig. 7e), with CA moving eastward from central Siberia and gradually weakening, while ACA moving eastward from Northeast Asia gradually strengthened (Fig. 7e–h). The cold high intensified as it moved eastward (Fig. S7e–g), accompanied by an eastward strengthening of the surface high-pressure anomaly (Fig. 7e–g). One day before the CH-type dust day, the surface high-pressure anomaly replaced the low-pressure anomaly to the north of NC (Fig. 7g, h), and the wind anomalies north of NC shifted from southwest to northeast (Fig. 7f–h). The actual wind directions changed from westerly to northerly directions (Fig. S7f–h). In summary, the development and movement of CA and ACA aligned with the occurrence and development of MC days and CH days in NC. The common predictor I_ACA-CA served as a meaningful indicator for predicting dust weather in NC.
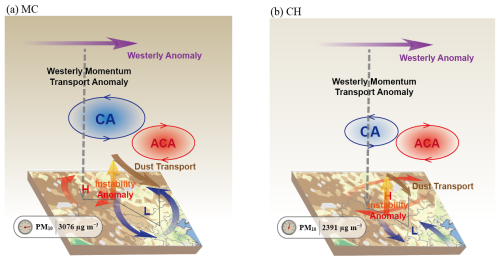
Figure 8Schematic diagram for the three-dimensional atmospheric circulation anomalies and related dynamic processes of (a) MC-type and (b) CH-type dust weather with distinct PM10 concentrations in NC. The 500 hPa cyclonic anomaly (CA) and anticyclonic anomaly (ACA) are the key anomalous circulation systems for the two types. “L” and “H”, respectively, represent surface low-pressure anomalies and high-pressure anomalies. The anomalous gust winds and thermal instability near the dust source area favored dust lifting. Enhanced 200 hPa westerly winds, with momentum transport downward, favored further increases in surface wind speeds. Anomalous northerly winds facilitated the emission and transport of dust particles. The shading on the surface represents NDVI in March 2023. The directions of the arrows indicate anomalous airflow directions. The average maximum PM10 concentrations of MC and CH days are demonstrated in the bottom left of each panel.
In recent years, the frequency and intensity of dust weather in NC have been increasing, with many adverse effects on human health, national economy, and ecological environment. This study selected dust days based on PM10 concentrations in NC in spring from 2015 to 2023. By objectively identifying the presence of the Mongolian cyclone and based on the main surface synoptic systems, dust days were classified into MC type (61.7 %) and CH type (38.3 %). The PM10 concentrations on MC days were higher and more extreme compared to those on CH days, with average maximum PM10 concentrations at 3076 and 2391 µg m−3. The three-dimensional atmospheric circulation anomaly structures and relevant dynamic processes of the two types of dust weather with distinct PM10 concentrations in NC are illustrated (Fig. 8). The 500 hPa cyclonic and anticyclonic circulation anomalies have been investigated to be correlated with both types of dust days. In order to comprehensively forecast the two types of dust weather, a common predictor has been constructed based on the anomalous circulation systems at 500 hPa, providing insights for dust weather forecasting and climate prediction in NC.
This study differs from traditional weather classification studies by not further categorizing weather types beyond the Mongolian cyclone. The circulation anomalies related to the two types of dust weather were explored to identify the common predictor. The study designates the peak PM10 concentration during periods when the daily maximum PM10 concentration exceeds 1000 µg m−3 as dust days, representing a typical dust event. This aims to capture the most significant circulation anomalies and extreme conditions during dust events. The transitional phase during the development and cessation of dust weather in NC has not been included in this study. Previous studies have investigated the relationship between PM10 concentration thresholds and traditional dust weather levels, but a unified standard has not yet been established. According to the standards used in previous studies (Wan et al., 2004; Wang et al., 2008), dust days identified by the 1000 µg m−3 PM10 concentration threshold primarily correspond to the traditional meteorological classifications of blowing dust, sand and dust storm, and severe sand and dust storm. Furthermore, dust weather identified by PM10 concentrations may differ from traditional dust weather defined based on visibility and other meteorological phenomena. For example, in the years 2015–2023, dust days defined by PM10 concentrations were most frequent in May, rather than in April as seen in previous studies. However, the increase in the number of dust days in May may be a recent trend that requires further study.
The common predictor (I_ACA-CA) of the two dust weather types in NC was identified. The research findings demonstrated that the I_ACA-CA had good indicative significance for dust weather in NC. Previous studies have primarily focused on the role of Mongolian cyclones in North China's dust weather. However, in addition to Mongolian cyclones, systems like the cold high can also lead to dust storms in North China. The common predictor offers a more comprehensive prediction for both types of dust weather compared to solely considering the Mongolian cyclone, capturing more dust days. The ability of the C3S seasonal forecast model to reproduce I_ACA-CA was further assessed. The I_ACA-CA calculated by ECMWF, DWD, and MF seasonal forecast models with a 1-month lead captured around 50 % of spring dust days when positive. These ratios are all lower than the capture rate of I_ACA-CA for dust weather (76.5 %) calculated from ERA5 data, indicating the need for further improvement. It is worth noting that due to the lower spatial resolution (1° × 1°) of the C3S model forecast data relative to the ERA5 data (0.25° × 0.25°), the SLP produced by the C3S model failed to effectively identify the presence of the Mongolian cyclone. Therefore, the introduction of the common predictor (I_ACA-CA) is of great significance for dust weather prediction in NC. However, due to constraints imposed by PM10 concentration observation data, the study period only covered the years 2015 to 2023. Further research is needed to test the effectiveness of the I_ACA-CA indicator over longer time series and to utilize it for predicting future dust weather.
All data used in this article can be publicly downloaded. Hourly PM10 and PM2.5 concentration data are available at https://quotsoft.net/air/ (China National Environmental Monitoring Centre, 2024). The hourly ERA5 reanalysis dataset is available at https://doi.org/10.24381/cds.bd0915c6 (Hersbach et al., 2023a) and https://doi.org/10.24381/cds.adbb2d47 (Hersbach et al., 2023b). The NDVI data can be acquired from https://doi.org/10.7289/V5ZG6QH9 (Vermote, 2019). The C3S seasonal forecast data are available at https://doi.org/10.24381/cds.50ed0a73 (Copernicus Climate Change Service, Climate Data Store, 2018a) and https://doi.org/10.24381/cds.181d637e (Copernicus Climate Change Service, Climate Data Store, 2018b).
The supplement related to this article is available online at: https://doi.org/10.5194/acp-25-1711-2025-supplement.
ZY and HW designed the research. ZY, QH, and XM performed the research. QH prepared the manuscript with contributions from all co-authors.
The contact author has declared that none of the authors has any competing interests.
Publisher's note: Copernicus Publications remains neutral with regard to jurisdictional claims made in the text, published maps, institutional affiliations, or any other geographical representation in this paper. While Copernicus Publications makes every effort to include appropriate place names, the final responsibility lies with the authors.
We acknowledge the financial support from the National Natural Science Foundation of China for this work.
This research has been supported by the National Natural Science Foundation of China (grant no. 42088101).
This paper was edited by Stephanie Fiedler and reviewed by three anonymous referees.
Ahmadzai, H., Malhotra, A., and Tutundjian, S.: Assessing the impact of sand and dust storm on agriculture: Empirical evidence from Mongolia, PLoS One, 18, e0269271, https://doi.org/10.1371/journal.pone.0269271, 2023.
Bueh, C., Zhuge, A., Xie, Z., Yong, M., and Purevjav, G.: The development of a powerful Mongolian cyclone on 14–15 March 2021: Eddy energy analysis, Atmos. Ocean. Sci. Lett., 15, 100259, https://doi.org/10.1016/j.aosl.2022.100259, 2022.
Chen, S. Y., Zhao, D., Huang, J. P., He, J. Q., Chen, Y., Chen, J. Y., Bi, H. R., Lou, G. T., Du, S. K., Zhang, Y., and Yang, F.: Mongolia Contributed More than 42 % of the Dust Concentrations in Northern China in March and April 2023, Adv. Atmos. Sci., 40, 1549–1557, https://doi.org/10.1007/s00376-023-3062-1, 2023a.
Chen, Y., Chen, S. Y., Zhou, J., Zhao, D., Bi, H. R., Zhang, Y., Alam, K., Yu, H. P., Yang, Y. X., and Chen, J. Y.: A super dust storm enhanced by radiative feedback, NPJ Clim. Atmos. Sci., 6, 90, https://doi.org/10.1038/s41612-023-00418-y, 2023b.
China National Environmental Monitoring Centre: Hourly PM10 concentration data, China National Environmental Monitoring Centre [data set], https://quotsoft.net/air/ (last access: 6 April 2024), 2024.
Copernicus Climate Change Service, Climate Data Store: Seasonal forecast subdaily data on pressure levels. Copernicus Climate Change Service (C3S) Climate Data Store (CDS) [data set], https://doi.org/10.24381/cds.50ed0a73, 2018a.
Copernicus Climate Change Service, Climate Data Store: Seasonal forecast daily and subdaily data on single levels. Copernicus Climate Change Service (C3S) Climate Data Store (CDS) [data set], https://doi.org/10.24381/cds.181d637e, 2018b.
Dulam, J., Shinoda, M., Kimura, R., Batbold, A., and Amarjargal, D.: Quantitative Analysis on Windblown Dust Concentrations of PM10 (PM2.5) during Dust Events in Mongolia, Aeolian Res., 14, 3–13, https://doi.org/10.1016/j.aeolia.2014.04.005, 2014.
Gao, J., Ding, T., and Gao, H.: Dominant circulation pattern and moving path of the Mongolian Cyclone for the severe sand and dust storm in China, Atmos. Res., 301, 107272, https://doi.org/10.1016/j.atmosres.2024.107272, 2024.
Garratt J. R.: The Atmospheric Boundary Layer, Cambridge University Press, Cambridge, ISBN 0521467454, 1992.
Gui, K., Yao, W., Che, H., An, L., Zheng, Y., Li, L., Zhao, H., Zhang, L., Zhong, J., Wang, Y., and Zhang, X.: Record-breaking dust loading during two mega dust storm events over northern China in March 2021: aerosol optical and radiative properties and meteorological drivers, Atmos. Chem. Phys., 22, 7905–7932, https://doi.org/10.5194/acp-22-7905-2022, 2022.
Hersbach, H., Bell, B., Berrisford, P., Biavati, G., Horányi, A., Muñoz Sabater, J., Nicolas, J., Peubey, C., Radu, R., Rozum, I., Schepers, D., Simmons, A., Soci, C., Dee, D., and Thépaut, J.-N.: ERA5 hourly data on pressure levels from 1940 to present, Copernicus Climate Change Service (C3S) Climate Data Store (CDS) [data set], https://doi.org/10.24381/cds.bd0915c6, 2023a.
Hersbach, H., Bell, B., Berrisford, P., Biavati, G., Horányi, A., Muñoz Sabater, J., Nicolas, J., Peubey, C., Radu, R., Rozum, I., Schepers, D., Simmons, A., Soci, C., Dee, D., and Thépaut, J.-N.: ERA5 hourly data on single levels from 1940 to present, Copernicus Climate Change Service (C3S) Climate Data Store (CDS) [data set], https://doi.org/10.24381/cds.adbb2d47, 2023b.
Huang, J. P., Wang, T. H., Wang, W. C., Li, Z. Q., and Yan, H. R.: Climate effects of dust aerosols over East Asian arid and semiarid regions, J. Geophys. Res.-Atmos., 119, 11398–11416, https://doi.org/10.1002/2014JD021796, 2014.
Krasnov, H., Katra, I., and Friger, M.: Increase in dust storm related PM10 concentrations: A time series analysis of 2001–2015, Environ. Pollut., 213, 36–42, https://doi.org/10.1016/j.envpol.2015.10.021, 2016.
Li, J. D., Hao, X., Liao, H., Yue, X., Li, H., Long, X., and Li, N: Predominant type of dust storms that influences air quality over northern China and future projections, Earths Future, 10, e2022EF002649, https://doi.org/10.1029/2022EF002649, 2022.
Liu, J., Qian, Z., Jiang, X., and Zheng M.: A Study on Weather Types of Super Severe Dust Storms in North China, Plateau Meteor., 23, 540–547, 2004 (in Chinese).
Liu, S. K. and Liu, S. D.: Atmospheric dynamics, 2nd edn., Peking University Press, Beijing, China, 143–147, ISBN 9787301161586, 2011.
Lwin, K. S., Tobias, A., Chua, P. L., Yuan, L., Thawonmas, R., Ith, S., Htay, Z. W., Yu, L. S., Yamasaki, L., Roqué, M., Querol, X., Fussell, J. C., Nadeau, K. C., Stafoggia, M., Saliba, N. A., Sheng Ng, C. F., and Hashizume, M.: Effects of Desert Dust and Sandstorms on Human Health: A Scoping Review, GeoHealth, 7, e2022GH000728, https://doi.org/10.1029/2022GH000728, 2023.
Mu, F., Luiz, E. W., and Fiedler, S.: On the dynamics and air-quality impact of the exceptional East Asian dust outbreak in mid-March 2021, Atmos. Res., 292, 106846, https://doi.org/10.1016/j.atmosres.2023.106846, 2023.
Qian, W. H., Quan, L. S., and Shi, S. Y.: Variations of the Dust Storm in China and its Climatic Control, J. Climate, 15, 1216–1229, https://doi.org/10.1175/1520-0442(2002)015<1216:VOTDSI>2.0.CO;2, 2002.
Shao, Y. P.: Physics and Modelling of Wind Erosion, 2nd edn., Springer Dordrecht, 456 pp., https://doi.org/10.1007/978-1-4020-8895-7, 2008.
Shou, S. W.: Synoptic Analysis, China Meteorological Press, Beijing, 361 pp., ISBN 9787502934576, 2006 (in Chinese).
Sugimoto, N., Shimizu, A., Matsui, I., and Nishikawa, M.: A method for estimating the fraction of mineral dust in particulate matter using PM2.5-to-PM10 ratios, Particuology, 28, 114–120, https://doi.org/10.1016/j.partic.2015.09.005, 2016.
Takemi, T. and Seino, N.: Dust storms and cyclone tracks over the arid regions in east Asia in spring, J. Geophys. Res., 110, D18S11, https://doi.org/10.1029/2004JD004698, 2005.
Tian, Y., Pan, X. L., Jing, Y. J., Zhang, Y. T., Yao, W. J., Liu, H., Lei, S. D., and Wang, Z. F.: East Asia dust storms in spring 2021: Transport mechanisms and impacts on China, Atmos. Res., 290, 106773, https://doi.org/10.1016/j.atmosres.2023.106773, 2023.
Vermote, E.: NOAA Climate Data Record (CDR) of AVHRR Normalized Difference Vegetation Index (NDVI), Version 5, NOAA National Centers for Environmental Information [data set], https://doi.org/10.7289/V5ZG6QH9, 2019.
Wan, B., Kang, X., Zhang, J., Tong, Y., Tang, G., and Li, X.: Research on classification of dust and sand storm basic on particular concentration, Environ. Monit. China, 20, 8–11, https://doi.org/10.3969/j.issn.1002-6002.2004.03.003, 2004 (in Chinese).
Wang, S., Yu, Y., Zhang, X. X., Lu, H., Zhang, X. Y., and Xu, Z.: Weakened dust activity over China and Mongolia from 2001 to 2020 associated with climate change and land-use management, Environ. Res. Lett., 16, 124056, https://doi.org/10.1088/1748-9326/ac3b79, 2021.
Wang, Y. Q., Zhang, X. Y., Gong, S. L., Zhou, C. H., Hu, X. Q., Liu, H. L., Niu, T., and Yang, Y. Q.: Surface observation of sand and dust storm in East Asia and its application in CUACE/Dust, Atmos. Chem. Phys., 8, 545–553, https://doi.org/10.5194/acp-8-545-2008, 2008.
Wang, Y. Q., Zhang, X. Y., Sun, J. Y., Zhang, X. C., Che, H. Z., and Li, Y.: Spatial and temporal variations of the concentrations of PM10, PM2.5 and PM1 in China, Atmos. Chem. Phys., 15, 13585–13598, https://doi.org/10.5194/acp-15-13585-2015, 2015.
Wiggs, G. F. S.: Sediment Mobilisation by the Wind, In Arid Zone Geomorphology: Process, Form and Change in Drylands, 3rd edn., edited by: Thomas, D. S. G., Wiley, 455–486, https://doi.org/10.1002/9780470710777, 2011.
Wu, C. L., Lin, Z. H., He, J. X., Zhang, M. H., Liu, X. H., Zhang, R. J., and Brown, H.: A process-oriented evaluation of dust emission parameterizations in CESM: Simulation of a typical severe dust storm in East Asia, J. Adv. Model. Earth Syst., 8, 1432–1452, https://doi.org/10.1002/2016MS000723, 2016.
Wu, J., Li, C., Zhu, X. W., Qiu, Y. L., Tang, Y. X., and Ma, X. H.: Comparative analysis of meteorological factors and sand source conditions in sand and dust weather events in northern China during the spring of 2021 and 2022, Trans. Atmos. Sci., 46, 950–960, https://doi.org/10.13878/j.cnki.dqkxxb.20230313001, 2023 (in Chinese).
Yi, Z., Wang, Y., Chen, W., Guo, B., Zhang, B., Che, H., and Zhang, X.: Classification of the Circulation Patterns Related to Strong Dust Weather in China Using a Combination of the Lamb–Jenkinson and k-Means Clustering Methods, Atmosphere, 12, 1545, https://doi.org/10.3390/atmos12121545, 2021.
Yin, Z. C., Wan, Y., Zhang, Y. J., and Wang, H. J.: Why super sandstorm 2021 in North China?, Natl. Sci. Rev., 9, nwab165, https://doi.org/10.1093/nsr/nwab165, 2022.
Yin, Z. C., Huo, Q. Y., Ma, X. Q., Zhang, Y. J., Ma, X. H., and Wang., H. J.: Mechanisms of dust source accumulation and synoptic disturbance triggering the 2023 spring sandstorm in northern China, Trans. Atmos. Sci., 46, 321–331, https://doi.org/10.13878/j.cnki.dqkxxb.20230501007, 2023a (in Chinese).
Yin, Z. C., Zhou, B. T., Duan, M. K., Chen, H. S., and Wang, H. J.: Climate extremes become increasingly fierce in China, Innovation, 4, 100406, https://doi.org/10.1016/j.xinn.2023.100406, 2023b.
Yun, J., Jiang, X., Meng, X., Wu, X., and Ying, C.: Comparative Analyses on Some Statistic Characteristics between Cold Front and Mongolian Cyclone Duststorm Processes, Plateau Meteor., 32, 423–434, http://www.gyqx.ac.cn/CN/10.7522/j.issn.1000-0534.2012.00041 (last access: 6 February 2025), 2013 (in Chinese).
Zhang, L., Fan, F., Wu, H., Zou, Y., Zhou, Z., Zhang, X., and Gao, S.: Diagnosis of sandstorm weather process and analysis of sand pollution transportation in northern China from 14th to 16th, March 2021, Acta Scientiae Circumstantiae, 42, 1–13, https://doi.org/10.13671/j.hjkxxb.2021.0452, 2022 (in Chinese).
Zhang, X. X., Lei, J. Q., Wu, S. X., Li, S. Y., Liu, L. Y., Wang, Z. F., Huang, S. Y., Guo, Y. H., Wang, Y. D., Tang, X., and Zhou, J.: Spatiotemporal evolution of aeolian dust in China: An insight into the synoptic records of 1984–2020 and nationwide practices to combat desertification, Land. Degrad. Dev., 34, 2005–2023, https://doi.org/10.1002/ldr.4585, 2023.
Zhang, Z. H. and Huisingh, D.: Combating desertification in China: Monitoring, control, management and revegetation, J. Clean. Prod., 182, 765–775, https://doi.org/10.1016/j.jclepro.2018.01.233, 2018.
Zhao, D., Chen, S. Y., and Chen, Y.: Comparative analysis of two typical dust storm processes over East Asia, J. Lanzhou Univ. Nat. Sci., 58, 313–322, https://doi.org/10.13885/j.issn.0455-2059.2022.03.005, 2022 (in Chinese).
Zhong, W., Yin, Z., and Wang, H.: The relationship between anticyclonic anomalies in northeastern Asia and severe haze in the Beijing–Tianjin–Hebei region, Atmos. Chem. Phys., 19, 5941–5957, https://doi.org/10.5194/acp-19-5941-2019, 2019.
- Abstract
- Introduction
- Data and method
- PM10 concentration differences between regional synoptic systems
- Large-scale atmospheric circulation anomalies
- A common predictor for the two dust weather types
- Conclusion and discussion
- Data availability
- Author contributions
- Competing interests
- Disclaimer
- Acknowledgements
- Financial support
- Review statement
- References
- Supplement
- Abstract
- Introduction
- Data and method
- PM10 concentration differences between regional synoptic systems
- Large-scale atmospheric circulation anomalies
- A common predictor for the two dust weather types
- Conclusion and discussion
- Data availability
- Author contributions
- Competing interests
- Disclaimer
- Acknowledgements
- Financial support
- Review statement
- References
- Supplement