the Creative Commons Attribution 4.0 License.
the Creative Commons Attribution 4.0 License.
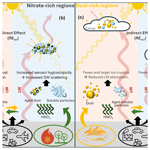
Impact of mineral dust on the global nitrate aerosol direct and indirect radiative effect
Alexandros Milousis
Klaus Klingmüller
Alexandra P. Tsimpidi
Jasper F. Kok
Maria Kanakidou
Athanasios Nenes
Vlassis A. Karydis
Nitrate (NO) aerosol is projected to increase dramatically in the coming decades and may become the dominant inorganic particle species. This is due to the continued strong decrease in SO2 emissions, which is not accompanied by a corresponding decrease in NOx and especially NH3 emissions. Thus, the radiative effect (RE) of NO aerosol may become more important than that of SO aerosol in the future. The physicochemical interactions of mineral dust particles with gas and aerosol tracers play an important role in influencing the overall RE of dust and non-dust aerosols but can be a major source of uncertainty due to their lack of representation in many global climate models. Therefore, this study investigates how and to what extent dust affects the current global NO aerosol radiative effect through both radiation (REari) and cloud interactions (REaci) at the top of the atmosphere (TOA). For this purpose, multiyear simulations nudged towards the observed atmospheric circulation were performed with the global atmospheric chemistry and climate model EMAC, while the thermodynamics of the interactions between inorganic aerosols and mineral dust were simulated with the thermodynamic equilibrium model ISORROPIA-lite. The emission flux of the mineral cations Na+, Ca2+, K+, and Mg2+ is calculated as a fraction of the total aeolian dust emission based on the unique chemical composition of the major deserts worldwide. Our results reveal positive and negative shortwave and longwave radiative effects in different regions of the world via aerosol–radiation interactions and cloud adjustments. Overall, the NO aerosol direct effect contributes a global cooling of −0.11 W m−2, driven by fine-mode particle cooling at short wavelengths. Regarding the indirect effect, it is noteworthy that NO aerosol exerts a global mean warming of +0.17 W m−2. While the presence of NO aerosol enhances the ability of mineral dust particles to act as cloud condensation nuclei (CCN), it simultaneously inhibits the formation of cloud droplets from the smaller anthropogenic particles. This is due to the coagulation of fine anthropogenic CCN particles with the larger nitrate-coated mineral dust particles, which leads to a reduction in total aerosol number concentration. This mechanism results in an overall reduced cloud albedo effect and is thus attributed as warming.
- Article
(6928 KB) - Full-text XML
-
Supplement
(2913 KB) - BibTeX
- EndNote
Atmospheric aerosols are among the most complex components of the Earth's climate system. This is due not only to the diversity of their origins, with many natural and anthropogenic emission sources, but also to their extremely varied chemical composition and properties. The many mechanisms by which they interact with each other and with physical entities such as radiation, clouds, land, and oceans add to their complexity and play a critical role in the energy balance of the planet (Arias et al., 2021). The most direct way in which aerosols affect the Earth's energy balance is through their interactions with solar shortwave (SW) and terrestrial longwave (LW) radiation (IPCC, 2013). Overall, the radiative effect due to aerosol–radiation interactions (REari) is mainly dominated by the scattering of SW radiation back to space (negative radiative effect, generating a cooling of the climate system) and the absorption of LW radiation (positive radiative effect, generating a warming of the climate system) (Gao et al., 2018; Tsigaridis and Kanakidou, 2018). Aerosols belonging to the black and/or brown carbon family, together with mineral dust particles, contribute to absorption (Kanakidou et al., 2005; Zhang et al., 2017; Wong et al., 2019), while the main inorganic aerosol components, such as sulfate and nitrate, as well as a significant amount of organic carbon, contribute mainly to scattering (Kirchstetter et al., 2004; (Bond and Bergstrom, 2006; Klingmüller et al., 2019; Zhang, 2020). However, mineral dust can also influence the behavior of the REari of anthropogenic pollution. Dust particles alter the anthropogenic radiative effect of aerosol–radiation interactions by reducing the loading of anthropogenic aerosols (either by coagulating with them or by adsorption of their precursor inorganic trace gases), leading to less scattering of solar radiation and thus a warming effect (Kok et al., 2023).
Atmospheric aerosols can also indirectly affect the Earth's energy balance by forming clouds, controlling cloud optical thickness and scattering properties, and altering their precipitation and lifetime (IPCC, 2013). Atmospheric aerosols act as cloud condensation nuclei (CCN), providing a suitable surface for water vapor to condense, leading to the formation of liquid droplets that develop into a corresponding liquid cloud (Lance et al., 2004). Such clouds are referred to as warm clouds and are typically found in the lower troposphere (Khain and Pinsky, 2018). However, there is constant competition between small and large particles for the available amount of water vapor (Barahona et al., 2010; Morales Betancourt and Nenes, 2014). Under the same humidity conditions, the presence of small particles will lead to the formation of small droplets with high number concentrations, while the presence of larger particles will lead to the formation of large droplets but with lower number concentrations. Depending on the size characteristics of its particle population, a warm cloud will exhibit different optical properties, with a population dominated by smaller particles generally being more reactive in the SW spectrum. The change in cloud reflectivity due to the presence of aerosols is referred to as the first radiative effect due to aerosol–cloud interactions (REaci) and was first described by Twomey (1977). The small size of anthropogenic aerosols results in an overall smaller cloud droplet size, which reduces precipitation efficiency and thus increases cloud lifetime. This contributes to cloud reflectivity and is referred to as the second radiative effect of aerosol–cloud interactions, first described by Albrecht (1989). These two indirect effects are considered equally important for the total indirect radiative effect of aerosols (Lohmann and Feichter, 2005). Atmospheric aerosols exert a net cooling effect that can partially mask the warming effect of greenhouse gases; therefore, the recent decline in anthropogenic aerosol concentrations may accelerate global warming (Urdiales-Flores et al., 2023). Overall, the radiative effect due to aerosol–cloud interactions is considered the main source of existing uncertainty in the effective (total) radiative effect of aerosols in the atmosphere (Myhre et al., 2014; Seinfeld et al., 2016).
Mineral dust influences the anthropogenic radiative effect through aerosol–cloud interactions in several ways that can result in either a net warming or net cooling effect. Dust particles can increase the cloud droplet number concentration (CDNC) in remote areas since through chemical aging by pollutants (Nenes et al., 2014; Karydis et al., 2017), dust particles become more hygroscopic and require lower supersaturation thresholds for activation (Karydis et al., 2011). This is caused by the transfer of anthropogenic pollutants towards remote desert regions, which enhances the solubility of dust particles. In such regions, this mostly results in increased cloud albedo and a net cooling effect. However, dust particles also tend to reduce the availability of smaller anthropogenic CCN. This is due to intrusions of aged dust particles into polluted environments, which reduce the numbers of smaller aerosols through increased coagulation with them. This results in lower cloud reflectivity (albedo) and thus a net warming effect (Klingmüller et al., 2020). Furthermore, when dust is above or below low-level clouds, the resulting effect of local heating is an increase in total cloud cover due to enhanced temperature inversion or enhanced upward vertical motion, respectively (Kok et al., 2023). On the other hand, when dust is present inside low-level clouds, local heating enhances in-cloud evaporation, resulting in an overall decrease in cloud cover. Kok et al. (2023) showed that the amount of desert dust in the atmosphere has increased since the mid-19th century, causing an overall cooling effect on the Earth that masks up to 8 % of the warming caused by greenhouse gases. If the increase in dust were halted, the previously hidden additional warming potential of greenhouse gases could lead to slightly faster climate warming.
NO is expected to dominate the global aerosol composition in the coming decades due to the predicted limited availability of SO following the abrupt decline in SO2 emissions, which will not necessarily be accompanied by proportional reductions in NOx and NH3 emissions (Bellouin et al., 2011; Hauglustaine et al., 2014). Excess NO is expected to exert a cooling REari by scattering SW radiation (Bauer et al., 2007a; Xu and Penner, 2012; Myhre et al., 2013; IPCC, 2013; Li et al., 2015), but the REaci is much more complex and complicated and can lead to both cooling and warming. Mineral dust thus becomes a key factor, as it is one of the main promoters of NO aerosol formation, providing a very suitable surface for gaseous HNO3 condensation to the aerosol phase (Karydis et al., 2011; Trump et al., 2015). In addition to HNO3 adsorption, heterogeneous reactions on the surface of dust particles are known to promote nitrate formation (Krueger et al., 2004; Hodzic et al., 2006). The most important pathway through which this occurs is N2O5 hydrolysis, with a yield for aerosol nitrate of ∼ 2 (Seisel et al., 2005; Tang et al., 2012). At the same time, other reactions, such as NO2 oxidation, contribute to much slower nitrate production and are of major importance mainly during short periods of dust pollution events (Li et al., 2024). These processes affect not only the optical properties of dust aerosols, which will influence their overall REari, but also how they can alter cloud formation and microphysics. NO aerosols increase the hygroscopicity of mineral dust (Kelly et al., 2007) by providing layers of soluble material on their surface, thus increasing their ability to act as CCN (Karydis et al., 2017). In doing so, they also increase the size of dust particles through hygroscopic growth and therefore their coagulation efficiency. Thus, nitrate–dust interactions are a complex mechanism that ultimately affects climatology in a variety of ways. The role of mineral dust in modifying the influence of NO aerosols in the global REaci is not yet well understood. This study aims to focus on the extent of the REari and REaci of NO aerosols and on how interactions with mineral dust regulate both on a global scale.
This study is organized as follows: in Sect. 2, details of the modeling setup for conducting the global simulations as well as the treatment of dust–nitrate interactions in the model are discussed and the methodology for calculating the global REari and REaci of NO aerosols is explained. Section 3 presents the main results for the global REari for coarse and fine NO aerosols for the base case simulation and the sensitivity cases listed in Table 1. Section 4 presents the results for the global REaci of total NO aerosols, while Sect. 5 includes the feedback mechanism of dust–nitrate interactions with cloud microphysics. Finally, the main conclusions and a general discussion on the scope of the study are presented in Sect. 6.
Table 1Differences between the base case and all sensitivity simulations performed.
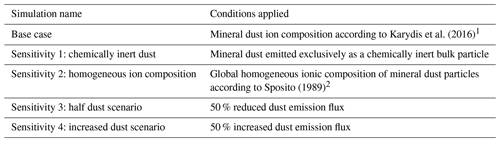
1 The ionic composition of the dust particles with respect to the mineral ions Ca2+, Mg2+, K+, and Na+ depends on the chemical composition of the soil in each grid cell, which is estimated from the desert soil composition maps of Klingmüller et al. (2018) based on the fraction of mineral ions found in Karydis et al. (2016). 2 The ionic composition of the dust particles is homogeneous and held constant in all grid cells where dust is present. The dust particles are a mixture of bulk species and the mineral ions Na+, K+, Ca2+, and Mg2+ with mass fractions of 94 %, 1.2 %, 1.5 %, 2.4 %, and 0.9 %, respectively.
2.1 Model setup
The simulations were performed with the global atmospheric chemistry and climate model EMAC (ECHAM/MESSy) (Jöckel et al., 2006), which includes several submodels describing atmospheric processes and their interactions with oceans, land, and human influences. These submodels are linked through the Modular Earth Submodel System (MESSy) (Jöckel et al., 2005) to a base model, the 5th Generation European Center Hamburg General Circulation Model (ECHAM) (Roeckner et al., 2006). The submodel system used in this work includes the MECCA submodel, which performs the gas-phase chemistry calculations (Sander et al., 2019). The SCAV submodel is responsible for the in-cloud liquid-phase chemistry and wet deposition processes (Tost et al., 2006, 2007b), while DRYDEP and SEDI are used to compute the dry deposition of gases and aerosols and gravitational settling, respectively (Kerkweg et al., 2006). All aerosol microphysical processes are calculated by the GMXe submodel (Pringle et al., 2010a, b), where aerosols are divided into four lognormal size modes (nucleation, Aitken, accumulation, and coarse). Each mode is defined in terms of aerosol number concentration, mean dry radius, and geometric standard deviation (sigma). The mean dry radius for each mode is allowed to vary within fixed bounds (0.5–6 nm for nucleation, 6–60 nm for Aitken, 60–700 nm for accumulation, and above 700 nm for coarse) and the sigma is fixed and equal to 1.59 for the first three size modes and 2 for the coarse mode. The coagulation of aerosols is also handled by GMXe, following Vignati et al. (2004), and the coagulation coefficients for Brownian motion are calculated according to Fuchs and Davies (1964). The partitioning between the gas and aerosol phases is calculated using the ISORROPIA-lite thermodynamic module (Kakavas et al., 2022) as implemented in EMAC by Milousis et al. (2024). The optical properties of the aerosols and the radiative transfer calculations are simulated by the submodels AEROPT (Dietmüller et al., 2016) and RAD (Dietmüller et al., 2016), respectively. AEROPT can be called several times within a model time step with different settings for the aerosol properties. More details are given in Sect. 2.3.1. All cloud properties and microphysical processes are simulated by the CLOUD submodel (Roeckner et al., 2006) using the two-moment microphysical scheme of Lohmann and Ferrachat (2010) for liquid and ice clouds. The activation processes of liquid cloud droplets and ice crystals follow the physical treatment of Morales Betancourt and Nenes (2014) and Barahona and Nenes (2009), respectively, as described by Karydis et al. (2017) and Bacer et al. (2018). More details are given in Sect. 2.3.2.
The meteorology for each of the simulations was nudged by ERA5 reanalysis data (C3S, 2017); thus, this study estimates the radiative effect of nitrate aerosols with respect to REari and REaci separately, rather than the effective (total) radiative effect, as this would require multiple free-run simulations with prescribed sea surface temperatures for each case separately. The spectral resolution used for each simulation was T63L31, which corresponds to a grid resolution of 1.875° × 1.875 and 31 vertical layers up to 25 km in height. The period covered by the simulations is from 2007 to 2018, with the first year representing the model spin-up period.
Anthropogenic aerosol and trace gas emissions were taken from the CMIP6 database (O'Neill et al., 2016) according to the SSP370 scenario. Natural NH3 emissions (from land and ocean) were based on the GEIA database (Bouwman et al., 1997), and natural volcanic SO2 emissions were taken from the AEROCOM database (Dentener et al., 2006). Biogenic NO emissions from soils were calculated online according to the algorithm of Yienger and Levy (1995), while lightning-produced NOx was also calculated online by the LNOx submodel (Tost et al., 2007a) using the parameterization of Grewe et al. (2001). Dimethyl sulfide (DMS) emissions from the oceans are calculated online by the AIRSEA submodel (Pozzer et al., 2006). Sea salt emissions are based on the AEROCOM database (Dentener et al., 2006) following the chemical composition reported by Seinfeld and Pandis (2016), i.e., 30.6 % Na+, 3.7 % Mg2+, 1.2 % Ca2+, 1.1 % K+, and 55 % Cl−. Dust emissions are calculated online using the parameterization of Astitha et al. (2012). In this scheme, while the surface friction velocity is the most important parameter for the amount of the emitted dust flux, the meteorological information for each grid cell is also taken into account. Dust particles are emitted in the accumulation and coarse size modes of the insoluble fraction but can be transferred to the soluble fraction after coagulation with other soluble species and/or by condensation of soluble material on their surface. Both processes are treated and calculated by GMXe and ISORROPIA-lite. The emissions of mineral ions (Ca2+, Mg2+, K+, and Na+) are estimated as a fraction of the total dust emission flux based on the soil chemical composition of each grid cell. This is done using desert soil composition maps from Klingmüller et al. (2018), which are based on the mineral ion fractions from Karydis et al. (2016). These mineral ions are treated as individual species that are part of the aerosol in each size mode and are assumed to be well mixed with the rest of the aerosol species considered (i.e., dust, black carbon, organics, inorganic ions). The aerosol composition within each of the seven modes considered is uniform in size (internally mixed) but may vary between modes (externally mixed).
To assess the impact of changes in mineral dust chemistry and emissions on the global NO aerosol REari and REaci, four additional sensitivity simulations were performed (Table 1). In the first sensitivity simulation, mineral dust is described only by a bulk, chemically inert species. In this case, there is no uptake of HNO3 by the dust particles due to acid–base interactions with the non-volatile cations (NVCs), so it remains in the gas phase. In the second sensitivity case, the chemical composition of the mineral dust was assumed to be spatially uniform, with a percentage distribution for bulk dust, Na+, K+, Ca2+, and Mg2+ particles assumed to be 94 %, 1.2 %, 1.5 %, 2.4 %, and 0.9 %, respectively, according to Sposito (1989). Finally, two additional simulations were performed to assess the impact of the global mineral dust budget on the results, where the dust emission fluxes were first halved and then increased by 50 % to account for the historical increase in global dust mass load since pre-industrial times, as reconstructed by Kok et al. (2023). The particle size distribution of the emitted dust mass remained unchanged in all sensitivity simulations.
Overall, the EMAC model is well established in the literature for its ability to accurately predict organic and inorganic aerosol concentrations and compositions, aerosol optical depth, acid deposition, gas-phase mixing ratios, cloud properties, and meteorological parameters (de Meij et al., 2012; Pozzer et al., 2012, 2022; Tsimpidi et al., 2016, 2017; Karydis et al., 2016, 2017; Bacer et al., 2018; Milousis et al., 2024); factually replicate dust emissions (Astitha et al., 2012; Abdelkader et al., 2015; Klingmüller et al., 2018); and provide realistic estimates for CCN and CDNC (Chang et al., 2017; Karydis et al., 2017; Fanourgakis et al., 2019). Here, a comparison of the performance of the model in estimating the surface mass concentrations of PM2.5 NO and total PM10 aerosols is provided in the Supplement (Figs. S2, S3 and Tables S1, S2). In addition, the ability of the model to estimate CDNCs is evaluated (Fig. S4 and Table S3). The comparison is made with observations of PM2.5 nitrate aerosols from regional networks in the polluted Northern Hemisphere covering the regions of East Asia (EANET, the Acid Deposition Monitoring Network in East Asia, 2024), Europe (EMEP, European Monitoring and Evaluation Programme, 2024), and the USA for urban (EPA-CASTNET, U.S. Environmental Protection Agency Clean Air Status and Trends Network, 2024) and rural (IMPROVE, Interagency Monitoring of Protected Visual Environments, 2024) locations. The comparison with observations of surface mass PM10 aerosols also covers the aforementioned monitoring networks, with the exception of the EPA. Finally, the CDNCs estimated by the base case simulation are compared with the CDNCs observed in different regions of the planet (continental, polluted, and clean marine) over different time periods, but also altitudes, as found in Karydis et al. (2017) and all relevant references therein.
2.2 Treatment of dust–nitrate interactions
The interactions between mineral dust and nitrate aerosols play a crucial role in altering the size distribution and optical properties of both species and can also strongly influence cloud microphysical processes (Fig. 1). Therefore, these interactions affect both the REari and the REaci of both nitrate and dust aerosols. First, the adsorption of HNO3 onto the surface of dust particles is a process that strongly promotes the formation of nitrate aerosols on dust (Karydis et al., 2016). We treat this condensation process using the GMXe submodel. Specifically, the quantity of gas-phase species that are able to kinetically condense within a model time step (equal to 10 min in this study) is calculated according to the diffusion-limited condensation theory of Vignati et al. (2004). The diffusive flux of gas on a single particle surface for each size mode i is described by the condensation coefficient Ci according to Fuchs and Davies (1964) and is estimated from the following function as found in Vignati et al. (2004).
where rgi is the geometric mean radius of the size mode i, D is the diffusion coefficient, and s is an accommodation coefficient for each gas species treated that has the assigned values of 1 for H2SO4 (Vignati et al. 2004), 0.1 for HNO3, 0.064 for HCl, and 0.09 for NH3 (Pringle et al., 2010a, b). v is the mean thermal velocity of the molecule and Δ is the mean free path length of the gas molecule (the distance from which the kinetic regime applies with respect to the particle).This information is then passed to the ISORROPIA-lite thermodynamic module to calculate the gas–aerosol partitioning. Specifically, the module receives as input the ambient temperature and humidity along with the diffusion-limited concentrations of H2SO4, NH3, HNO3, and HCl; the concentrations of the non-volatile cations (NVCs) Na+, K+, Ca2+, and Mg2+; and the concentrations of the ions SO, NO, NH, and Cl− present in the aerosol phase from the previous time step. The module then calculates the equilibrium reactions of the NO anion with the NVCs, depending on their abundance with respect to the SO anion, taking into account mass conservation, electroneutrality, water activity equations, and precalculated activity coefficients for specific ionic pairs (Fountoukis and Nenes, 2007; Kakavas et al., 2022). Therefore, in all cases where mineral dust is considered chemically active, all reactions of nitrate aerosols with NVCs are treated. The salts that may be formed are assumed to be completely deliquesced as follows.
Salt deliquescence over a range of relative humidities is treated by the mutual deliquescence relative humidity (MDRH) approach of Wexler and Seinfeld (1991). In a multicomponent salt mixture, the MDRH determines the humidity value above which all salts are considered to be saturated. In this study, if the wet aerosol is below the MDRH, it does not crystalize and remains in a supersaturated aqueous solution (Kakavas et al., 2022), with all salts completely deliquesced. More information on equilibrium reactions and equilibrium constants as well as the corresponding thermodynamic equilibrium calculations can be found in Fountoukis and Nenes (2007). It should be noted that in this study nitrate production on dust particles occurs not only via the thermodynamic equilibrium between gas-phase HNO3 and particulate nitrate, but also via heterogeneous chemistry by hydrolysis of N2O5 on the dust surface. This chemical formation pathway is the most dominant for heterogeneous nitrate production (Seisel et al., 2005; Tang et al., 2012), while others, such as NO2 oxidation during dust pollution events over polluted regions (Li et al., 2024), do not show such high yields under normal conditions. On the other hand, consideration of sulfate production by heterogeneous chemistry on dust would theoretically result in slightly reduced amounts of particulate nitrate in some cases due to acidification of dust particles inhibiting partitioning of HNO3 to the aerosol phase (Nenes et al., 2020). Overall, full consideration of heterogeneous chemistry on dust could change simulated nitrate aerosol concentrations only slightly and episodically, and therefore changes to radiative effect estimates are not expected to be critical.
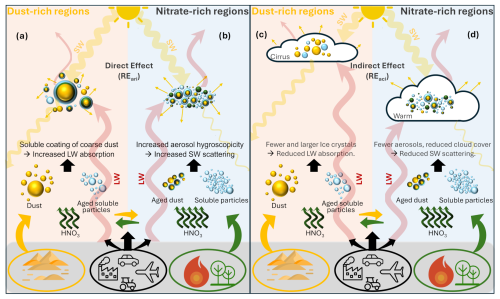
Figure 1Conceptual illustration of how dust–nitrate interactions affect the total NO (left) REari and (right) REaci. (a) In dust-rich environments, nitric acid transported from anthropogenic pollution and biomass burning regions interacts with mineral cations to form a soluble coating on the surface of dust particles. The dominant effect of these interactions is an enhanced LW absorption (warming REari) by the coarse dust particles. (b) In nitrate-rich environments, the intrusion of dust particles and their subsequent interaction with freshly formed nitric acid lead to an overall increase in aerosol hygroscopicity and thus a stronger SW reflection (cooling REari). (c) In dust-rich environments, the number of ice crystals in cirrus clouds is reduced while their size is increased due to the interaction of dust particles with the transported HNO3. This results in an optical thinning of the ice clouds, which leads to less trapping of outgoing LW radiation (cooling REaci). (d) In nitrate-rich environments, the increased wet radius of aged dust particles leads to enhanced coagulation with smaller particles, resulting in a decrease in the number of smaller aerosols and, in turn, a decrease in the number of activated particles in cloud droplets by smaller aerosols, which ultimately leads to a reduction in the backscattering of SW radiation by warm clouds (warming REari).
The coating of dust particles by nitrate aerosols during gas–aerosol partitioning calculations is an important process that leads to an increase in dust solubility and hygroscopicity (Laskin et al., 2005). Therefore, after these processes have taken place, a large fraction of the originally insoluble dust particles has become soluble (Fig. 1a), which leads to changes in their optical properties, as their increased ability to absorb water makes them more efficient in extinguishing SW radiation and absorbing and emitting LW radiation (Fig. 1a, b) (Kok et al., 2023). The transfer to the soluble fraction after coating with soluble material is handled by the GMXe submodel, which also provides key aerosol attributes necessary for the calculation of the dust optical properties (see Sect. 2.3).
In general, the changes in the properties of dust particles through their interactions with nitrate aerosols will result in more efficient removal rates, mainly through wet deposition, due to their higher hygroscopicity and increased size (Fan et al., 2004). The reduced number of dust particles that can act as ice nuclei (IN) and their increased size can lead to an optical thinning of cirrus clouds (Fig. 1c) (Kok et al., 2023). Furthermore, the changes induced by dust–nitrate interactions reduce the activation of smaller aerosols in warm clouds (Fig. 1d). In particular, the enhanced hygroscopicity of dust particles will lead to a faster depletion of the available supersaturation, as they act as giant CCN that absorb large amounts of water vapor to activate into cloud droplets (Karydis et al., 2017). In addition, the population of smaller aerosols will also be depleted by increased coagulation with the large dust particles. As a consequence of the different degrees of complexity of the dust–nitrate interactions, it is very important to note that they do not always result in a linear response in terms of how they affect climate through their subsequent interactions with radiation, clouds, or both.
2.3 Radiative effect calculation
To calculate the global REari and REaci of NO aerosols, the optical properties from the AEROPT submodel and the radiative transfer calculations from the RAD submodel were used. First, AEROPT provides the aerosol extinction (absorption and scattering) coefficients, the single-scattering albedo, and the aerosol asymmetry factor for each grid cell with a vertical distribution analogous to the vertical resolution used. The GMXe submodel is used to provide input of aerosol attributes for the calculation of aerosol optical properties, which is done online using 3D look-up tables. The tables provide information on the real and imaginary parts of the refractive index and the Mie size parameter per size mode (Dietmüller et al., 2016). Then, the radiative scheme of RAD uses the particle number weighted average of the extinction cross section, the single-scattering albedo, and the asymmetry factor as input for the radiative transfer calculations. In addition to AEROPT, RAD takes input from the submodels ORBIT (Earth orbital parameters), CLOUDOPT (cloud optical properties) (Dietmüller et al., 2016), and IMPORT (import of external datasets) to calculate the radiative transfer properties for longwave and shortwave radiation fluxes separately. Both the AEROPT and RAD submodels can be invoked multiple times within a model time step, each time with different settings for the aerosol optical properties, allowing radiative transfer estimates for identical climatological conditions. This is of paramount importance for the calculation of the REari of aerosols since any effects due to possibly different climatological conditions must be eliminated. Henceforth, all references to RE estimates, as well as net, longwave, and shortwave flux quantities, will refer to the top of the atmosphere (TOA) only.
2.3.1 Radiative effect from aerosol–radiation interactions (REari)
To estimate the global REari of all aerosols as well as that of total, coarse, and fine NO aerosols, three simulations were performed for each sensitivity case in Table 1. In the first simulation all aerosol species are present. In the second simulation NO aerosols are completely removed by turning off their formation by removing the pathway of HNO3 formation through both NO2 oxidation and N2O5 hydrolysis, leaving no available HNO3 to condense on the aerosol via equilibrium partitioning and form nitrate. In the third simulation, coarse-mode NO aerosols are removed by allowing HNO3 to condense only on the fine mode (i.e., the sum of the three smaller lognormal size modes: nucleation, Aitken, and accumulation). For each of these three simulations, the radiative transfer routines are called twice for each time step. One call uses the normal aerosol optical properties of the existing population, and the other call uses an aerosol optical depth equal to 0 to emulate an atmosphere without aerosols. Essentially, the global REari of each simulation can be calculated by taking the difference between the net fluxes between the two calls. More specifically, the first simulation will yield the REari of the total aerosol load (F1,ari hereafter), the second simulation will yield the REari of all aerosols except NO (F2,ari below), and the third simulation will yield the REari of all aerosols except the coarse-mode NO (F3,ari below). Since the above estimates of the radiative effect were computed using the exact same climatology, its effect was effectively eliminated. However, in order to isolate the NO aerosol radiative effect, it is also essential to disable any aerosol–cloud interactions; otherwise the cooling effect would be severely underestimated because cloud scattering would make aerosol scattering less relevant (Ghan et al., 2012). For this purpose, the simplest cloud scheme available in the EMAC model is used, which calculates the cloud microphysics according to Lohmann and Roeckner (1996), who empirically relate the cloud droplet number concentration to the sulfate aerosol mass (Boucher and Lohmann, 1995) and specifically to its monthly mean values as derived from the sulfur cycle of the ECHAM5 circulation model (Feichter et al., 1996). The cloud coverage is estimated according to Tompkins (2002) with the use of prognostic equations for the water phases and the distribution moments. To disable aerosol–cloud interactions, no aerosol activation routines are used to avoid coupling with the activation schemes. Overall, the global REari values of total, coarse, and fine NO aerosols are obtained as follows:
-
(FN,ari) = F1,ari-F2,ari,
-
(FcN,ari) = F1,ari-F3,ari,
-
(FfN,ari) = F3,ari-F2,ari.
2.3.2 Radiative effect from aerosol–cloud interactions (REaci)
In this work we estimate the effect of total NO aerosols on the calculated global REaci. Climatology plays a crucial role in aerosol–cloud interactions and simulating a “fine-only NO atmosphere”, as done for the REari calculations, would produce an unrealistic climatological scenario, since coarse-mode NO is strongly associated with cations in mineral dust particles (Karydis et al., 2016), making them quite effective as CCN (Karydis et al., 2017). Therefore, the REaci calculations require two additional simulations for each sensitivity case separately: one with all aerosols present and one with the entire NO aerosol load removed by turning off their formation as described in the previous section. The global REaci is then given by
-
(FN,aci) = FFN - FN,ari,
where FFN is the total NO aerosol feedback radiative effect. Since FN,ari is calculated using the methodology described in Sect. 2.3.1, it is only necessary to estimate FFN. This is equal to the difference in net fluxes between the two additional simulations. There is no need to emulate an aerosol-free atmosphere here since any differences induced by different climatologies must be included. The two simulations performed for the calculation of FFN use the cloud formation scheme as described in Lohmann and Ferrachat (2010), which uses prognostic equations for the water phases and the bulk cloud microphysics. In addition, the empirical cloud cover scheme of Sundqvist et al. (1989) is used. For the cloud droplet formation, the CDNC activation scheme of Morales Betancourt and Nenes (2014) is used, which includes the adsorption activation of mineral dust as described in Karydis et al. (2017). The effect of dust–nitrate interactions on clouds presented here refers to the lowest level of cloud formation at 940 hPa. For the ice crystal number concentration (ICNC), the activation scheme of Barahona and Nenes (2009) is used, which calculates the ice crystal size distribution through heterogeneous and homogeneous freezing as well as ice crystal growth.
3.1 Base case
The global average REari of total NO aerosols at the top of the atmosphere was found to be −0.11 W m−2, which is within the reported range of the estimated present-day all-sky direct radiative effect of total NO aerosols by other studies (Liao et al., 2004; Bauer et al., 2007a, b; Bellouin et al., 2011; Xu and Penner, 2012; Heald et al., 2014) (Table S4). The NO cooling of the REari calculated by EMAC is driven by the scattering of SW radiation (equal to −0.34 W m−2), which outweighs the warming due to absorption of LW radiation (equal to +0.23 W m−2) (Table 2). The REari of the total NO aerosol shows a clearly contrasting behavior with respect to the size mode considered (Table 2; Fig. 2).
Table 2Net, longwave, and shortwave global mean TOA REari of total, coarse, and fine NO aerosols for the base case and each sensitivity case simulation.
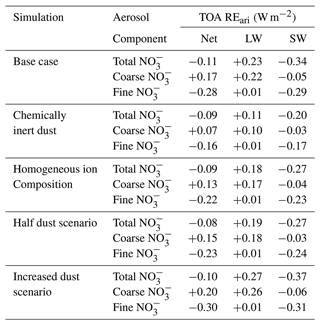
In particular, the coarse particles show a net warming effect of +0.17 W m−2 (Fig. 2i) and contribute to 96 % of the LW warming of the total nitrate, while only contributing 15 % of the radiative cooling in the SW spectrum (−0.05 W m−2). The LW warming is strongest over the dust belt zone and especially over the Sahara, the Middle East, and the northern face of the Himalayan plateau, while the contribution over other arid regions such as the Atacama, Gobi, Taklimakan, and Mojave deserts is significant. These regions are characterized by moderate to high concentrations of coarse NO aerosols due to the adsorption of HNO3 on desert soil particles (Karydis et al., 2016; Milousis et al., 2024). Therefore, the warming due to absorption of terrestrial LW radiation by coarse-mode nitrates interacting with mineral dust is the strongest over these areas (see Fig. 1a), ranging from +1.5 to +5 W m−2 (Fig. 2iii). On the other hand, the cooling exerted by coarse nitrate aerosol through the SW REari is more pronounced over areas where it interacts strongly with high concentrations of mineral dust particles (see Fig. 1b). Such areas include the Congo Basin, where HNO3 from tropical forest biomass burning interacts with Saharan mineral dust particles; the Middle East and northern Indian regions, where anthropogenic HNO3 emissions interact with mineral dust particles from the Sahara and Taklimakan deserts, respectively; and the East Asian region, where HNO3 emissions from Chinese megacities interact with mineral dust particles from the Gobi Desert. These regions can lead to an average cooling of up to −3.5 W m−2 (Fig. 2v).
Interestingly, there is no significant cooling from SW interactions over the Sahara for the coarse mode. This phenomenon can be attributed to two factors, the first related to nitrate–dust interactions and the second related to the characteristics of the region. Specifically, because the underlying desert surface is very bright, its absorption in this part of the spectrum is less than that of the particles above it, which means that the desert surface can scatter radiation more effectively than the particles above it. This is further enhanced by the growth of coarse-mode particles there (see Fig. 4x and Sect. 5.1), which increases the absorption cross section of the particles. All this leads to an overall attenuation of the cooling effect over this region and sometimes even to local warming (Fig. 2v).

Figure 2Global mean TOA net REari for (i) coarse and (ii) fine NO aerosols, longwave REari for (iii) coarse and (iv) fine NO aerosols, and shortwave REari for (v) coarse and (vi) fine NO aerosols as calculated by EMAC from the base case simulation.
In contrast to the radiative effect of coarse NO particles, the REari of fine NO particles is an overall cooling of −0.28 W m−2 (Fig. 2ii). Fine nitrates have a negligible 4 % contribution to the warming in the LW spectrum (Fig. 2iv) but account for 85 % of the net cooling of the total nitrate aerosols (Fig. 2vi). The cooling induced by fine NO aerosols from scattering of SW radiation is stronger (up to −5 W m−2) over regions of high anthropogenic activity, particularly the East Asian and Indian regions, where fine nitrates dominate the total nitrate aerosol load. The regions of West Africa and the Amazon Basin are characterized by moderate fine nitrate concentrations, and the cooling observed there is enhanced by HNO3 associated with biomass burning interacting with fresh and aged Saharan dust particles, respectively, which are dominated by accumulation-mode sizes in the absence of coarse-mode nitrates. Finally, other polluted regions such as North America and Europe also show SW cooling up to −2 W m−2.
3.2 Sensitivity of REari estimates
The comparison of the calculated total NO radiative effect due to interactions with net, LW, and SW radiation for the sensitivity cases listed in Table 1 can be found in Table 2, which shows each of the estimates. Consideration of nitrate interactions with mineral dust cations can greatly affect the NO REari estimates. Assuming that mineral dust particles are inert, the estimated warming due to LW radiation interactions for total nitrate aerosols is 52 % weaker than in the base case where dust reactivity is considered. Similarly, the cooling effect exerted by all nitrate aerosols through interactions with SW radiation is estimated to be 41 % weaker under the assumption that mineral dust is non-reactive. Both estimates are lower when mineral dust is assumed to be chemically inert, since HNO3 is no longer effectively adsorbed on dust particles. However, since both the estimated warming and cooling are weaker, the effects partially cancel each other out, resulting in a net cooling effect (−0.09 W m−2) that is 18 % weaker compared to the base case calculations. Assuming a homogeneous ionic composition for the dust results in SW cooling and LW warming for total nitrate aerosols being 21 % and 22 % lower, respectively, weakening the estimate for the net cooling REari by 18 % (−0.09 W m−2). The net direct radiative effect of total NO is the same for the cases where dust is assumed to have a homogeneous chemical composition and where it has no chemical identity, indicating the importance of both aspects for the impact of dust–nitrate interactions on the direct radiative effect.
In the half dust scenario, the total nitrate aerosol LW warming estimate is 17 % weaker than in the base case, while the total nitrate aerosol SW estimate is even more so (21 %), resulting in a lower net cooling estimate of −0.08 W m−2. Finally, the increased dust scenario shows the strongest total nitrate aerosol LW warming effect (17 % increase over the base case) due to an increase in coarse-mode nitrate. At the same time, the cooling effect of total nitrate aerosols due to interactions with SW radiation shows a smaller increase of 9 %. Thus, accounting for the historical increase in mineral dust emissions results in a net cooling estimate of −0.10 W m−2, which is smaller than the base case. Interestingly, the behavior of the global total NO REari does not exhibit linearity with respect to the global dust load. This is not surprising since the nitrate–dust interactions themselves are not linearly correlated, and a given increase or decrease in dust emissions does not lead to an analogous change in nitrate aerosol levels. For example, Karydis et al. (2016) have shown that moving from a scenario in which nitrate–dust chemistry is not considered to one in which it is, but with half dust emissions, resulted in a 39 % increase in the tropospheric burden of nitrate aerosols. However, moving from a scenario with half to full dust emissions, the corresponding increase was only 9 %. In our case, moving from the chemically inert dust scenario to the half dust scenario led to an 18 % increase in atmospheric nitrate aerosol burden, while moving from the half dust scenario to the base case led to an additional 8 % increase, and finally moving from the base case to the increased dust scenario led to an even smaller increase of 5 %.
There are several reasons for this nonlinearity between changes in dust load and nitrate production. Firstly, since the adsorption of HNO3 onto dust particles is the main driver of nitrate production on dust, over desert areas (where the change in dust load takes place) the amount of nitric acid present is the limiting factor for such production, rather than the amount of dust itself. Secondly, when more dust is present in the atmosphere, the combination of its increased coating with the higher aerosol numbers tends to result in its more efficient removal by wet deposition as well as coagulation. This inherently affects nitrate production, which does not increase in proportion to the increase in dust.
4.1 Base case
The global average REaci of total NO aerosols at the top of the atmosphere was found to be +0.17 W m−2. In contrast, an estimate of the REaci of nitrate aerosols by Xu and Penner (2012) showed only a trivial cooling effect for particulate NO (−0.01 W m−2). Similar to the REari, the net REaci estimated by EMAC is driven by the effect on the SW part of the spectrum, which causes a warming effect of +0.27 W m−2, while the effect on the LW radiation causes an average cooling of −0.10 W m−2 (Table 3). Overall, the net REaci of total NO aerosols is reversed compared to the net REari; i.e., REaci exerts a strong cooling effect over regions where REari exerts a warming effect and vice versa (Fig. 3i). The reason for this is that the regions contributing to a cooling REari are dominated by smaller-sized nitrate aerosols and vice versa. Therefore, the size characteristics of the dominant nitrate aerosol population lead to different effects on the cloud optical properties as discussed in Sect. 1. For example, as the dominance of smaller nitrate aerosols decreases over a particular region, the optical thinning of low-level clouds will have an opposite effect on the REaci (Fig. 1d). Details of the mechanism by which nitrate–dust interactions affect cloud microphysical processes are discussed in Sect. 5. Over North America and Europe, REaci causes a warming effect of up to +3 W m−2, driven solely by the effect on SW radiation (Fig. 3iii). Over the regions of East Asia and the Amazon and Congo basins, REaci reaches a maximum of +5 W m−2, driven by the effect on both the SW (up to +4 W m−2) and LW (up to +1.5 W m−2) parts of the radiation spectrum. The cooling effect of REaci (up to −2 W m−2) extends mainly between the equatorial line and the Tropic of Cancer, mainly due to the interaction of nitrate aerosols with desert dust particles (e.g., from the Sahara) and their effect on the terrestrial spectrum (LW) (Figs. 1c, 3ii). The cooling effect of dust interactions with anthropogenic particles in the LW spectrum corroborates the findings of Klingmüller et al. (2020) and is attributed to the reduced ice-water path due to the depletion of small aerosols, which in turn leads to less trapped outgoing terrestrial radiation. In addition, Kok et al. (2023) note how the presence of dust particles leads to an optical thinning of cirrus clouds by reducing the number of ice crystals while increasing their size, which also leads to less trapping of outgoing LW radiation and thus a cooling effect (Fig. 1c). On the other hand, the warming effect of dust interactions with anthropogenic particles in the SW spectrum requires further investigation and is therefore discussed in more detail in Sect. 5.
4.2 Sensitivity of REaci estimates
Table 3 shows the comparison of the net, LW, and SW contributions of total NO to the REaci at the top of the atmosphere as calculated by the base case simulation and all sensitivity cases considered. By assuming chemically inert dust, the calculated net REaci of nitrate decreases by 35 %, resulting in a net warming of +0.11 W m−2. As with the REari estimate, this sensitivity case produces the largest deviation from the base case among all sensitivity simulations for both the SW (37 % less warming) and LW (40 % less cooling) estimates. This is due to the fact that the absence of dust–nitrate interactions does not have such a large impact on the population of aerosols and activated particles (see also Sect. 5). The assumption of a homogeneous ionic composition of the mineral dust leads to a weakened LW cooling estimate of 10 % and a weakened SW warming estimate of 19 %, resulting in a net NO REaci of +0.13 W m−2 (24 % lower than in the base case).
The reduced dust emissions result in a 15 % weaker warming in the SW spectrum and a 20 % weaker cooling in the LW spectrum, leading to an overall NO REaci of +0.15 W m−2 (12 % weaker than the base case scenario). This is because the reduced loading of nitrate aerosols, especially in the coarse mode, in the half dust scenario results in less absorption of LW radiation (Fig. 1c) (hence less cooling). Similarly, the effect of dust–nitrate interactions on the activation of smaller particles (Fig. 1d) is less drastic and results in a weaker inhibition of SW radiation scattering (hence less warming; see also Sect. 5). Finally, increased dust emissions in the increased dust scenario show a 10 % increase in the LW cooling and an 8 % decrease in the SW warming effect, surprisingly resulting in a net warming (+0.14 W m−2) that is lower than in the half dust scenario. The reason that this scenario results in more LW cooling than the base case is that the increased quantity of dust particles leads to even more optical thinning of the ice clouds and therefore even less trapping of LW radiation (more cooling). However, the reason why the SW warming estimate is lower than the base case is more complicated. First, the transition from the half dust scenario to the base case and then to the increased dust scenario does not lead to an analogous increase in the nitrate aerosol burden (see Sect. 3.2). Moreover, since the number of aerosols has increased from the increased dust scenario to the base case, but the relative humidity has remained largely the same, there is more competition for water vapor because it is now distributed over a larger population. As a result, the wet radius increase in the presence of nitrates is not as strong in the increased dust scenario compared to the base case, and the depletion of smaller-sized particles is also not as strong (not shown). The implications of the depletion of the aerosol population in the presence of nitrate aerosols for the microphysical processes of warm clouds, and consequently for SW warming, are discussed in the next section.
5.1 Maximum supersaturation, hygroscopicity, and wet radius
To further investigate the cause of the positive REaci induced by the NO aerosols, their effect on the aerosol population characteristics as well as on the cloud microphysics is investigated with respect to the lowest-forming cloud level of 940 hPa. For this purpose, a sensitivity simulation is performed assuming a “nitrate-aerosol-free” (NAF) atmosphere, in which the formation of NO aerosols has been switched off, but an advanced cloud scheme is considered which is the same as the one described in Sect. 2.3.2. This is essentially the same setup that was used for the estimation of the total nitrate aerosol feedback radiative effect. This simulation is used to determine whether the presence of NO aerosols has a significant effect on the hygroscopicity and size of atmospheric aerosols and ultimately on the maximum supersaturation developed during cloud formation. Over polluted areas affected by transported dust air masses from surrounding arid areas, the presence of NO aerosols can increase the CCN activity of the large mineral dust particles, resulting in a reduction of the maximum supersaturation and inhibiting the activation of the small anthropogenic particles into cloud droplets (Klingmüller et al., 2020). Results from the NAF sensitivity simulation support this hypothesis over parts of eastern and central Asia, where the maximum supersaturation decreases by up to 0.05 %. In contrast, the presence of NO aerosols increases maximum supersaturation by up to 0.2 % over North America, Europe, the Middle East, and parts of southern Asia (Fig. 4ii). Therefore, changes in maximum supersaturation caused by the presence of NO aerosols cannot explain their warming effect through the REaci.
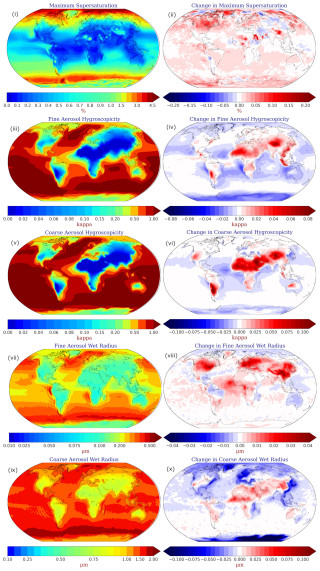
Figure 4(i) Global mean maximum supersaturation, fine-aerosol (iii) hygroscopicity, and (v) wet radius, as well as coarse-aerosol (vii) hygroscopicity and (ix) wet radius, as calculated by EMAC from the base case simulation at the altitude of 940 hPa. Absolute difference between the base case and nitrate-aerosol-free (NAF) sensitivity simulation in (ii) maximum supersaturation, fine-aerosol (iv) hygroscopicity, and (vi) wet radius, as well as coarse-aerosol (viii) hygroscopicity and (x) wet radius at the altitude of 940 hPa. Red indicates higher values calculated by the base case simulation in the presence of NO aerosols.
The presence of NO has a significant effect on the hygroscopicity of both fine and coarse aerosols and consequently on their wet radius, as shown in Fig. 1a and b and Fig. 4. This is most evident for coarse desert dust particles, which mix with NO aerosols from urban and forest regions, increasing their hygroscopicity by an order of magnitude (up to 0.1), especially over the African–Asian dust belt and the Atacama Desert in South America (Fig. 4vi). Aerosol hygroscopicity is similarly increased for the fine-mode particles both near arid regions and over the highly industrialized region of Southeast Asia (Fig. 4iv). The low values of the hygroscopic parameter of the fine-aerosol population, especially over the dust belt zone, are largely due to the higher proportion of insoluble fine particles present over these regions (Fig. S5). This is also observed over other regions with similarly low fine-aerosol hygroscopicity (southern Africa, South America, and the western US). Nevertheless, the estimates of aerosol kappa values at 940 hPa are broadly consistent with the results of Pringle et al. (2010c). On the other hand, the aerosol hygroscopicity for the two size modes is only slightly reduced, by up to 0.06 (or < 10 %) over the oceans and coasts of Europe and East Asia, due to interactions of NO with sea salt particles, reducing their hygroscopicity. The increased ability of both coarse dust aerosols and smaller aerosols to absorb water leads to an increase in their wet radius, but in different parts of the world. For example, fine particle sizes increase by up to 0.04 µm (up to 40 %), mostly over regions of high anthropogenic activity (North America, Europe, and East Asia) (Fig. 4viii). On the other hand, coarse-mode particle sizes are increased by up to 0.1 µm (up to 10 %) over the forests of central Africa and the African–Asian dust belt zone (Fig. 4x), while showing a similar decrease near the coasts of the polluted Northern Hemisphere due to the effect of NO on the hygroscopicity of sea salt.
5.2 Number concentrations of aerosol and activated particles
Figure 5 shows the effect of NO on the number concentration of fine and coarse aerosols between the base case and the NAF sensitivity simulation, as well as the total aerosol population. The presence of NO aerosols decreases the total aerosol number concentration over forests and polluted regions (see also Fig. 1d). This behavior is driven solely by the decrease in smaller particle sizes, as the effect is minimal for the coarser particles (Figs. 5ii, iv). The largest decrease is calculated over East and South Asia (up to 1000 cm−3 or 10 %), while decreases of up to 200 cm−3 on average (∼ 10 %) are found over Europe, the USA, and central Africa. This effect is directly related to the increased wet radius of the aerosol population (Fig. 4viii) over these regions and thus to its depositional efficiency. In addition, coarse dust particles become more hygroscopic due to interactions with NO aerosols that increase in size, resulting in increased coagulation with the smaller anthropogenic particles, which reduces their abundance.
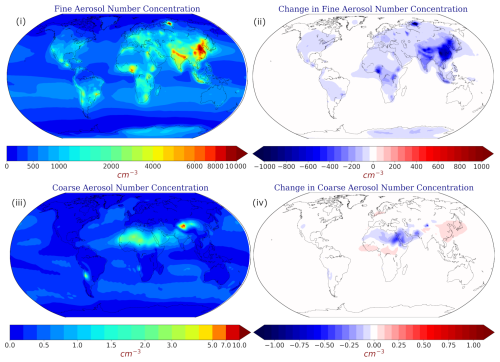
Figure 5Global mean number concentration of (i) fine and (iii) coarse aerosols as calculated by EMAC from the base case simulation at the altitude of 940 hPa. Absolute difference between the base case and the nitrate-aerosol-free (NAF) sensitivity simulation in the number concentration of (ii) fine and (iv) coarse aerosols at the altitude of 940 hPa. Blue indicates that number concentrations are lower in the presence of NO aerosols.
The reduced aerosol number concentration in the presence of NO can lead to a reduction of particles that are also activated into cloud droplets. Such behavior can be seen in Fig. 6, which shows the effect of NO on the number concentration of activated fine and coarse particles in cloud droplets between the base case and the NAF sensitivity simulation. The reduction in the total number of activated cloud droplets is almost entirely due to the reduction in smaller-sized particles (Figs. 6ii, iv). A reduction in the total number of activated droplets of up to 30 cm−3 or 10 % is observed over the USA, Amazon, Europe, central Africa, and parts of the Middle East, while this reduction reaches up to 100 cm−3 (10 %) over Southeast Asia, where the largest reductions in aerosol numbers are also calculated (Fig. 4ii). In turn, these are the regions where the warming effect of NO aerosols on the calculated mean REaci is strongest (Fig. 3i). The small increase in activated droplets (∼ 10 cm−3 or 1 %) over Beijing, which concerns the fine-mode particles, is most likely because their number concentration decreases with increasing size. The high aerosol number concentration there, which is the global maximum (Fig. 5i), results in a hotspot of more readily activated particles in the presence of NO. On the other hand, the CDNC decreases slightly over the Sahara due to the more efficient deposition capacity of coarse dust particles due to their interactions with nitrate aerosols, which is also reflected in the decrease in aerosol number (Fig. 6iv). Overall, the lower particle number in the presence of NO aerosols hinders the ability of the smaller anthropogenic particles to activate into cloud droplets, leading to a reduced cloud cover and thus a reduced cloud albedo effect. Therefore, not only is less LW radiation absorbed, but also, more importantly, less SW radiation is scattered back to space, resulting in an overall warming of the net average REaci for total NO aerosols.
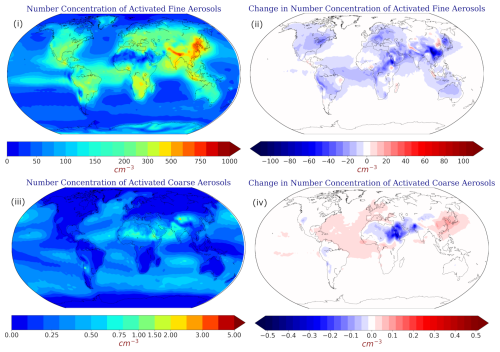
Figure 6Global mean number concentration of activated (i) fine and (iii) coarse aerosols as calculated by EMAC from the base case simulation at the altitude of 940 hPa. Absolute difference between the base case and the nitrate-aerosol-free (NAF) sensitivity simulation in the number concentration of activated (ii) fine and (iv) coarse aerosols at the altitude of 940 hPa. Blue indicates that number concentrations are lower in the presence of NO aerosols.
This study presents the effects of interactions between mineral dust and NO aerosols on the present-day global TOA radiative effect of the latter. We investigate how the presence of dust affects the radiative effect of NO aerosols through aerosol interactions with both radiation and separately with clouds (REari and REaci, respectively). Sensitivity simulations are also performed, varying both the mineral dust composition and its emissions, to assess the effect on the calculated NO aerosol radiative effect.
It was found that the global average net REari of total NO aerosols is −0.11 W m−2, which is mainly due to the cooling from the shortwave part of the radiation spectrum due to scattering, equal to −0.34 W m−2. A warming from the longwave part of the spectrum due to absorption was found to be +0.23 W m−2 on global average and was mainly located over regions with high concentrations of coarse NO aerosols. SW cooling was also observed in these regions, but also over regions of high anthropogenic activity, mainly over the polluted Northern Hemisphere. The behavior of the REari was opposite when considering different sizes of NO aerosols. Specifically, the coarse mode was responsible for 96 % of the estimated warming in the LW part of the spectrum but 15 % of the estimated cooling in the SW part of the spectrum. On the other hand, the contribution of the fine mode to the LW warming was negligible, but it was the main contributor to the SW cooling, accounting for 85 % of the net estimate. The sensitivity experiments revealed that the chemistry of the mineral dust is the most important factor in changing the estimated REari of the total NO aerosols. In particular, LW warming is most affected by this assumption, being 52 % weaker after assuming chemically inert dust emissions, while the SW cooling is reduced by 41 % compared to the base case simulation, amounting to a net cooling of −0.09 W m−2. A globally homogeneous ionic composition for mineral dust had a smaller effect in the LW (22 % decrease) and SW (21 % decrease) but resulted in the same net estimate of −0.09 W m−2. Halving the dust emissions resulted in weaker estimates for LW and SW by 17 % and 21 %, respectively, and the lowest overall net REari of −0.08 W m−2. On the other hand, a 50 % increase in dust emissions increased both LW warming and SW cooling by 17 % and 9 %, respectively, resulting in a net cooling REari of −0.10 W m−2, indicating the strong nonlinear relationship of nitrate–dust interactions and how they affect the radiative effect estimates.
The global average net REaci of total NO aerosols was +0.17 W m−2 due to the effect on the shortwave portion of the spectrum. This was found to be +0.27 W m−2, while the cooling from the longwave part was −0.10 W m−2. Spatially, the net REaci is reversed compared to the net REari for total NO aerosols, where regions responsible for a strong SW cooling of the REari contribute to a strong SW warming of the REaci and vice versa. This is due to the fact that nitrate–dust interactions challenge the dominance of smaller particles over heavily polluted regions, reducing the reflectivity of warm clouds and thus having an opposite effect on the REaci. The sensitivity experiments again showed that the consideration of the mineral dust chemistry is the most important aspect for the calculation of the REaci of the total NO aerosols. When the dust was assumed to be chemically inert, the LW and SW estimates were up to 40 % weaker, resulting in a net warming of +0.11 W m−2. Assuming a homogeneous ion composition resulted in a smaller weakening of the estimates (up to 18 %) and a net warming of +0.13 W m−2. When dust emissions were halved, the LW cooling was reduced slightly more than in the base case, resulting in a net warming of +0.15 W m−2. The 50 % increase in dust emissions had the largest effect on LW behavior (10 % increase), but surprisingly the net estimate (+0.14 W m−2) was smaller than in the half dust scenario. The reason for this is that the SW estimate did not increase but decreased by 8 % due to the fact that in this scenario the increased nitrate burden causes increased competition for the available supersaturation and the effect of dust–nitrate interactions on the smaller aerosol populations is not as emphasized as in the base case.
The total NO aerosol REaci shows a positive sign, which is attributed to a reduced cloud albedo effect. More specifically, although the presence or absence of NO aerosols in the atmosphere did not significantly affect the total available maximum supersaturation, it did alter both the hygroscopicity and wet radii of the aerosols. In the presence of NO, the hygroscopicity of aerosols over deserts was increased by up to an order of magnitude, leading to an increase in their wet radius of up to 10 %, with an even larger increase of up to 40 % for smaller particles over urban regions. Therefore, in the presence of NO aerosols, the depletion of fine particles by coagulation with coarser particles (i.e., mineral dust) is enhanced and further increases the size of the coarse particles. The reduction in the number of aerosols is up to 10 % in some regions, with maximum reductions calculated over Southeast Asia. This reduction in the number of fine aerosols leads to a reduction in the number of cloud droplets activated by fine aerosols (also up to 10 %), which would otherwise have absorbed more outgoing longwave radiation and, more importantly, scattered more incoming shortwave radiation. Thus, the reduced cloud albedo effect leads to a cooling in the longwave part of the spectrum, which is offset by a strong warming in the shortwave part, overall resulting in a net warming of the atmosphere.
The chemistry–climate model simulations presented here suggest that NO aerosol–radiation interactions lead to a net effect of −0.11 W m−2 (cooling) driven by fine NO aerosol, while NO aerosol–cloud interactions lead to a net effect of +0.17 W m−2 (warming) driven mainly by coarse-mode NO aerosol.
The usage of MESSy (Modular Earth Submodel System) and access to the source code are licensed to all affiliates of institutions which are members of the MESSy Consortium. Institutions can become a member of the MESSy Consortium by signing the MESSy Memorandum of Understanding. More information can be found on the MESSy Consortium website: http://www.messy-interface.org (last access: 22 May 2024). The code used in this study is based on MESSy version 2.55 and is archived with a restricted-access DOI (https://doi.org/10.5281/zenodo.8379120, The MESSy Consortium, 2023). The data produced in the study are available from the authors upon request.
The supplement related to this article is available online at: https://doi.org/10.5194/acp-25-1333-2025-supplement.
AM and VAK wrote the paper with contributions from KK, APT, JFK, MK, and AN. VAK planned the research with contributions from APT, MK, and AN. AM, KK, and VAK designed the methodology for the radiative effect calculations. AM performed the simulations and analyzed the results, assisted by VAK and APT. All the authors discussed the results and contributed to the paper.
At least one of the (co-)authors is a member of the editorial board of Atmospheric Chemistry and Physics. The peer-review process was guided by an independent editor, and the authors also have no other competing interests to declare.
Publisher’s note: Copernicus Publications remains neutral with regard to jurisdictional claims made in the text, published maps, institutional affiliations, or any other geographical representation in this paper. While Copernicus Publications makes every effort to include appropriate place names, the final responsibility lies with the authors.
This article is part of the special issue “The Modular Earth Submodel System (MESSy) (ACP/GMD inter-journal SI)”. It is not associated with a conference.
This work was supported by the project FORCeS funded from the European Union's Horizon 2020 research and innovation program under grant agreement no. 821205. JFK was funded by the National Science Foundation (NSF) Directorate for Geosciences under grants 1856389 and 2151093. The work described in this paper has received funding from the Initiative and Networking Fund of the Helmholtz Association through the project “Advanced Earth System Modelling Capacity (ESM)”. The authors gratefully acknowledge the Earth System Modelling Project (ESM) for funding this work by providing computing time on the ESM partition of the supercomputer JUWELS (Alvarez, 2021) at the Jülich Supercomputing Centre (JSC).
This research has been supported by Horizon 2020 (grant no. 821205) and the Directorate for Geosciences (grant nos. 1856389 and 2151093).
The article processing charges for this open-access publication were covered by the Forschungszentrum Jülich.
This paper was edited by Joshua Fu and reviewed by Kan Huang and one anonymous referee.
Abdelkader, M., Metzger, S., Mamouri, R. E., Astitha, M., Barrie, L., Levin, Z., and Lelieveld, J.: Dust–air pollution dynamics over the eastern Mediterranean, Atmos. Chem. Phys., 15, 9173–9189, https://doi.org/10.5194/acp-15-9173-2015, 2015.
Albrecht, B. A.: Aerosols, Cloud Microphysics, and Fractional Cloudiness, Science, 245, 1227–1230, https://doi.org/10.1126/science.245.4923.1227 , 1989.
Alvarez, D.: JUWELS cluster and booster: Exascale pathfinder with modular supercomputing architecture at juelich supercomputing Centre, J. Large-Scale Res. Fac., 7, A183–A183, https://doi.org/10.17815/jlsrf-7-183, 2021.
Arias, P. A., Bellouin, N., Coppola, E., Jones, R. G., Krinner, G., Marotzke, J., Naik, V., Palmer, M. D., Plattner, G.-K., Rogelj, J., Rojas, M., Sillmann, J., Storelvmo, T., Thorne, P. W., Trewin, B., Achuta Rao, K., Adhikary, B., Allan, R. P., Armour, K., Bala, G., Barimalala, R., Berger, S., Canadell, J. G., Cassou, C., Cherchi, A., Collins, W., Collins, W. D., Connors, S. L., Corti, S., Cruz, F., Dentener, F. J., Dereczynski, C., Di Luca, A., Diongue Niang, A., Doblas-Reyes, F. J., Dosio, A., Douville, H., Engelbrecht, F., Eyring, V., Fischer, E., Forster, P., Fox-Kemper, B., Fuglestvedt, J. S., Fyfe, J. C., Gillett, N. P., Goldfarb, L., Gorodetskaya, I., Gutierrez, J. M., Hamdi, R., Hawkins, E., Hewitt, H. T., Hope, P., Islam, A. S., Jones, C., Kaufman, D. S., Kopp, R. E., Kosaka, Y., Kossin, J., Krakovska, S., Lee, J.-Y., Li, J., Mauritsen, T., Maycock, T. K., Meinshausen, M., Min, S.-K., Monteiro, P. M. S., Ngo-Duc, T., Otto, F., Pinto, I., Pirani, A., Raghavan, K., Ranasinghe, R., Ruane, A. C., Ruiz, L., Sallée, J.-B., Samset, B. H., Sathyendranath, S., Seneviratne, S. I., Sörensson, A. A., Szopa, S., Takayabu, I., Tréguier, A.-M., van den Hurk, B., Vautard, R., von Schuckmann, K., Zaehle, S., Zhang, X., and Zickfeld, K.: Technical Summary, in: Climate Change 2021: The Physical Science Basis. Contribution of Working Group I to the Sixth Assessment Report of the Intergovernmental Panel on Climate Change, edited by: Masson-Delmotte, V., Zhai, P., Pirani, A., Connors, S. L., Péan, C., Berger, S., Caud, N., Chen, Y., Goldfarb, L., Gomis, M. I., Huang, M., Leitzell, K., Lonnoy, E., Matthews, J. B. R., Maycock, T. K., Waterfield, T., Yelekçi, O., Yu, R., and Zhou, B., Cambridge University Press, Cambridge, United Kingdom and New York, NY, USA, https://doi.org/10.1017/9781009157896.002, 2021.
Astitha, M., Lelieveld, J., Abdel Kader, M., Pozzer, A., and de Meij, A.: Parameterization of dust emissions in the global atmospheric chemistry-climate model EMAC: impact of nudging and soil properties, Atmos. Chem. Phys., 12, 11057–11083, https://doi.org/10.5194/acp-12-11057-2012, 2012.
Bacer, S., Sullivan, S. C., Karydis, V. A., Barahona, D., Krämer, M., Nenes, A., Tost, H., Tsimpidi, A. P., Lelieveld, J., and Pozzer, A.: Implementation of a comprehensive ice crystal formation parameterization for cirrus and mixed-phase clouds in the EMAC model (based on MESSy 2.53), Geosci. Model Dev., 11, 4021–4041, https://doi.org/10.5194/gmd-11-4021-2018, 2018.
Barahona, D. and Nenes, A.: Parameterizing the competition between homogeneous and heterogeneous freezing in cirrus cloud formation – monodisperse ice nuclei, Atmos. Chem. Phys., 9, 369–381, https://doi.org/10.5194/acp-9-369-2009, 2009.
Barahona, D., West, R. E. L., Stier, P., Romakkaniemi, S., Kokkola, H., and Nenes, A.: Comprehensively accounting for the effect of giant CCN in cloud activation parameterizations, Atmos. Chem. Phys., 10, 2467–2473, https://doi.org/10.5194/acp-10-2467-2010, 2010.
Bauer, S. E., Koch, D., Unger, N., Metzger, S. M., Shindell, D. T., and Streets, D. G.: Nitrate aerosols today and in 2030: a global simulation including aerosols and tropospheric ozone, Atmos. Chem. Phys., 7, 5043–5059, https://doi.org/10.5194/acp-7-5043-2007, 2007a.
Bauer, S. E., Mishchenko, M. I., Lacis, A. A., Zhang, S., Perlwitz, J., and Metzger, S. M.: Do sulfate and nitrate coatings on mineral dust have important effects on radiative properties and climate modeling?, J. Geophys. Res.-Atmos., 112, D6, https://doi.org/10.1029/2005JD006977, 2007b.
Bellouin, N., Rae, J., Jones, A., Johnson, C., Haywood, J., and Boucher, O.: Aerosol forcing in the Climate Model Intercomparison Project (CMIP5) simulations by HadGEM2-ES and the role of ammonium nitrate, J. Geophys. Res.-Atmos., 116, D20, https://doi.org/10.1029/2011JD016074, 2011.
Bond, T. C. and Bergstrom, R. W.: Light absorption by carbonaceous particles: An investigative review, Aerosol Sci. Technol., 40, 27–67, https://doi.org/10.1080/02786820500421521 , 2006.
Boucher, O. and Lohmann, U.: The sulfate-CCN-cloud albedo effect, Tellus B, 47, 281–300, https://doi.org/10.3402/tellusb.v47i3.16048, 1995.
Bouwman, A. F., Lee, D. S., Asman, W. A. H., Dentener, F. J., VanderHoek, K. W., and Olivier, J. G. J.: A global high-resolution emission inventory for ammonia, Global Biogeochem. Cy., 11, 561–587, https://doi.org/10.1029/97GB02266, 1997.
Chang, D. Y., Lelieveld, J., Tost, H., Steil, B., Pozzer, A., and Yoon, J.: Aerosol physicochemical effects on CCN activation simulated with the chemistry-climate model EMAC, Atmos. Environ., 162, 127–140, https://doi.org/10.1016/j.atmosenv.2017.03.036, 2017.
de Meij, A., Pozzer, A., Pringle, K. J., Tost, H., and Lelieveld, J.: EMAC model evaluation and analysis of atmospheric aerosol properties and distribution with a focus on the Mediterranean region, Atmos. Res., 114, 38–69, https://doi.org/10.1016/j.atmosres.2012.05.014, 2012.
Dentener, F., Kinne, S., Bond, T., Boucher, O., Cofala, J., Generoso, S., Ginoux, P., Gong, S., Hoelzemann, J. J., Ito, A., Marelli, L., Penner, J. E., Putaud, J.-P., Textor, C., Schulz, M., van der Werf, G. R., and Wilson, J.: Emissions of primary aerosol and precursor gases in the years 2000 and 1750 prescribed data-sets for AeroCom, Atmos. Chem. Phys., 6, 4321–4344, https://doi.org/10.5194/acp-6-4321-2006, 2006.
Dietmüller, S., Jöckel, P., Tost, H., Kunze, M., Gellhorn, C., Brinkop, S., Frömming, C., Ponater, M., Steil, B., Lauer, A., and Hendricks, J.: A new radiation infrastructure for the Modular Earth Submodel System (MESSy, based on version 2.51), Geosci. Model Dev., 9, 2209–2222, https://doi.org/10.5194/gmd-9-2209-2016, 2016.
European Monitoring and Evaluation Programme (EMEP): EBAS database online, https://projects.nilu.no/ccc/index.html (last access: 3 September 2024), 2024.
Fan, S.-M., Horowitz, L. W., Levy Ii, H., and Moxim, W. J.: Impact of air pollution on wet deposition of mineral dust aerosols, Geophys. Res. Lett., 31, 2, https://doi.org/10.1029/2003GL018501, 2004.
Fanourgakis, G. S., Kanakidou, M., Nenes, A., Bauer, S. E., Bergman, T., Carslaw, K. S., Grini, A., Hamilton, D. S., Johnson, J. S., Karydis, V. A., Kirkevåg, A., Kodros, J. K., Lohmann, U., Luo, G., Makkonen, R., Matsui, H., Neubauer, D., Pierce, J. R., Schmale, J., Stier, P., Tsigaridis, K., van Noije, T., Wang, H., Watson-Parris, D., Westervelt, D. M., Yang, Y., Yoshioka, M., Daskalakis, N., Decesari, S., Gysel-Beer, M., Kalivitis, N., Liu, X., Mahowald, N. M., Myriokefalitakis, S., Schrödner, R., Sfakianaki, M., Tsimpidi, A. P., Wu, M., and Yu, F.: Evaluation of global simulations of aerosol particle and cloud condensation nuclei number, with implications for cloud droplet formation, Atmos. Chem. Phys., 19, 8591–8617, https://doi.org/10.5194/acp-19-8591-2019, 2019.
Feichter, J., Kjellström, E., Rodhe, H., Dentener, F., Lelieveldi, J., and Roelofs, G. J.: Simulation of the tropospheric sulfur cycle in a global climate model, Atmos. Environ., 30, 1693–1707, https://doi.org/10.1016/1352-2310(95)00394-0, 1996.
Fountoukis, C. and Nenes, A.: ISORROPIA II: a computationally efficient thermodynamic equilibrium model for K+–Ca2+–Mg2+–NH–Na+–SO–NO–Cl−–H2O aerosols, Atmos. Chem. Phys., 7, 4639–4659, https://doi.org/10.5194/acp-7-4639-2007, 2007.
Fuchs, N. A. and Davies, C. N.: The mechanics of aerosols, Pergamon Press, Oxford, ISBN 9780486660554, 1964.
Gao, M., Ji, D., Liang, F., and Liu, Y.: Attribution of aerosol direct radiative forcing in China and India to emitting sectors, Atmos. Environ., 190, 35–42, https://doi.org/10.1016/j.atmosenv.2018.07.011 , 2018.
Ghan, S. J., Liu, X., Easter, R. C., Zaveri, R., Rasch, P. J., Yoon, J. H., and Eaton, B.: Toward a Minimal Representation of Aerosols in Climate Models: Comparative Decomposition of Aerosol Direct, Semidirect, and Indirect Radiative Forcing, J. Climate, 25, 6461–6476, https://doi.org/10.1175/JCLI-D-11-00650.1, 2012.
Grewe, V., Brunner, D., Dameris, M., Grenfell, J. L., Hein, R., Shindell, D., and Staehelin, J.: Origin and variability of upper tropospheric nitrogen oxides and ozone at northern mid-latitudes, Atmos. Environ., 35, 3421–3433, https://doi.org/10.1016/S1352-2310(01)00134-0 , 2001.
Hauglustaine, D. A., Balkanski, Y., and Schulz, M.: A global model simulation of present and future nitrate aerosols and their direct radiative forcing of climate, Atmos. Chem. Phys., 14, 11031–11063, https://doi.org/10.5194/acp-14-11031-2014, 2014.
Heald, C. L., Ridley, D. A., Kroll, J. H., Barrett, S. R. H., Cady-Pereira, K. E., Alvarado, M. J., and Holmes, C. D.: Contrasting the direct radiative effect and direct radiative forcing of aerosols, Atmos. Chem. Phys., 14, 5513–5527, https://doi.org/10.5194/acp-14-5513-2014, 2014.
Hodzic, A., Bessagnet, B., and Vautard, R.: A model evaluation of coarse-mode nitrate heterogeneous formation on dust particles, Atmos. Environ., 40, 4158–4171, https://doi.org/10.1016/j.atmosenv.2006.02.015, 2006.
Interagency Monitoring of Protected Visual Environment (IM-PROVE): Federal Land Manager Environmental Database, https://vista.cira.colostate.edu/Improve/improve-data/ (last access: 3 September 2024), 2024.
IPCC: Climate Change 2013: The Physical Science Basis. Contribution of Working Group I to the Fifth Assessment Report of the Intergovernmental Panel on Climate Change, edited by: Stocker, T. F., Qin, D., Plattner, G.-K., Tignor, M., Allen, S. K., Boschung, J., Nauels, A., Xia, Y., Bex, V., and Midgley, P. M., Cambridge University Press, Cambridge, United Kingdom and New York, NY, https://doi.org/10.1017/CBO9781107415324, USA, 1535 pp., 2013.
Jöckel, P., Sander, R., Kerkweg, A., Tost, H., and Lelieveld, J.: Technical Note: The Modular Earth Submodel System (MESSy) – a new approach towards Earth System Modeling, Atmos. Chem. Phys., 5, 433–444, https://doi.org/10.5194/acp-5-433-2005, 2005.
Jöckel, P., Tost, H., Pozzer, A., Brühl, C., Buchholz, J., Ganzeveld, L., Hoor, P., Kerkweg, A., Lawrence, M. G., Sander, R., Steil, B., Stiller, G., Tanarhte, M., Taraborrelli, D., van Aardenne, J., and Lelieveld, J.: The atmospheric chemistry general circulation model ECHAM5/MESSy1: consistent simulation of ozone from the surface to the mesosphere, Atmos. Chem. Phys., 6, 5067–5104, https://doi.org/10.5194/acp-6-5067-2006, 2006.
Kakavas, S., Pandis, S. N., and Nenes, A.: ISORROPIA-Lite: A Comprehensive Atmospheric Aerosol Thermodynamics Module for Earth System Models, Tellus B, 74, 1–23, https://doi.org/10.16993/tellusb.33, 2022.
Kanakidou, M., Seinfeld, J. H., Pandis, S. N., Barnes, I., Dentener, F. J., Facchini, M. C., Van Dingenen, R., Ervens, B., Nenes, A., Nielsen, C. J., Swietlicki, E., Putaud, J. P., Balkanski, Y., Fuzzi, S., Horth, J., Moortgat, G. K., Winterhalter, R., Myhre, C. E. L., Tsigaridis, K., Vignati, E., Stephanou, E. G., and Wilson, J.: Organic aerosol and global climate modelling: a review, Atmos. Chem. Phys., 5, 1053–1123, https://doi.org/10.5194/acp-5-1053-2005, 2005.
Karydis, V. A., Kumar, P., Barahona, D., Sokolik, I. N., and Nenes, A.: On the effect of dust particles on global cloud condensation nuclei and cloud droplet number, J. Geophys. Res.-Atmos., 116, D23204, https://doi.org/10.1029/2011JD016283, 2011.
Karydis, V. A., Tsimpidi, A. P., Pozzer, A., Astitha, M., and Lelieveld, J.: Effects of mineral dust on global atmospheric nitrate concentrations, Atmos. Chem. Phys., 16, 1491–1509, https://doi.org/10.5194/acp-16-1491-2016, 2016.
Karydis, V. A., Tsimpidi, A. P., Bacer, S., Pozzer, A., Nenes, A., and Lelieveld, J.: Global impact of mineral dust on cloud droplet number concentration, Atmos. Chem. Phys., 17, 5601–5621, https://doi.org/10.5194/acp-17-5601-2017, 2017.
Kelly, J. T., Chuang, C. C., and Wexler, A. S.: Influence of dust composition on cloud droplet formation, Atmos. Environ., 41, 2904–2916, https://doi.org/10.1016/j.atmosenv.2006.12.008, 2007.
Kerkweg, A., Buchholz, J., Ganzeveld, L., Pozzer, A., Tost, H., and Jöckel, P.: Technical Note: An implementation of the dry removal processes DRY DEPosition and SEDImentation in the Modular Earth Submodel System (MESSy), Atmos. Chem. Phys., 6, 4617–4632, https://doi.org/10.5194/acp-6-4617-2006, 2006.
Khain, A. P. and Pinsky, M.: Physical Processes in Clouds and Cloud Modeling, Cambridge University Press, 4–14 pp., ISBN 9781139049481, 2018.
Kirchstetter, T. W., Novakov, T., and Hobbs, P. V.: Evidence that the spectral dependence of light absorption by aerosols is affected by organic carbon, J. Geophys. Res.-Atmos., 109, D21, https://doi.org/10.1029/2004JD004999 , 2004.
Klingmüller, K., Metzger, S., Abdelkader, M., Karydis, V. A., Stenchikov, G. L., Pozzer, A., and Lelieveld, J.: Revised mineral dust emissions in the atmospheric chemistry–climate model EMAC (MESSy 2.52 DU_Astitha1 KKDU2017 patch), Geosci. Model Dev., 11, 989–1008, https://doi.org/10.5194/gmd-11-989-2018, 2018.
Klingmüller, K., Lelieveld, J., Karydis, V. A., and Stenchikov, G. L.: Direct radiative effect of dust–pollution interactions, Atmos. Chem. Phys., 19, 7397–7408, https://doi.org/10.5194/acp-19-7397-2019, 2019.
Klingmüller, K., Karydis, V. A., Bacer, S., Stenchikov, G. L., and Lelieveld, J.: Weaker cooling by aerosols due to dust–pollution interactions, Atmos. Chem. Phys., 20, 15285–15295, https://doi.org/10.5194/acp-20-15285-2020, 2020.
Kok, J. F., Storelvmo, T., Karydis, V. A., Adebiyi, A. A., Mahowald, N. M., Evan, A. T., He, C., and Leung, D. M.: Mineral dust aerosol impacts on global climate and climate change, Nat. Rev. Earth Environ., 4, 71–86, https://doi.org/10.1038/s43017-022-00379-5 , 2023.
Krueger, B. J., Grassian, V. H., Cowin, J. P., and Laskin, A.: Heterogeneous chemistry of individual mineral dust particles from different dust source regions: the importance of particle mineralogy, Atmos. Environ., 38, 6253–6261, https://doi.org/10.1016/j.atmosenv.2004.07.010, 2004.
Lance, S., Nenes, A., and Rissman, T. A.: Chemical and dynamical effects on cloud droplet number: Implications for estimates of the aerosol indirect effect, J. Geophys. Res.-Atmospheres, 109, D22, https://doi.org/10.1029/2004JD004596, 2004.
Laskin, A., Wietsma, T. W., Krueger, B. J., and Grassian, V. H.: Heterogeneous chemistry of individual mineral dust particles with nitric acid: A combined CCSEM/EDX, ESEM, and ICP-MS study, J. Geophys. Res.-Atmos., 110, D10, https://doi.org/10.1029/2004JD005206, 2005.
Li, J., Wang, W.-C., Liao, H., and Chang, W.: Past and future direct radiative forcing of nitrate aerosol in East Asia, Theor. Appl. Climatol., 121, 445–458, https://doi.org/10.1007/s00704-014-1249-1, 2015.
Li, X., Yu, Z., Yue, M., Liu, Y., Huang, K., Chi, X., Nie, W., Ding, A., Dong, X., and Wang, M.: Impact of mineral dust photocatalytic heterogeneous chemistry on the formation of the sulfate and nitrate: A modelling study over East Asia, Atmos. Environ., 316, 120166, https://doi.org/10.1016/j.atmosenv.2023.120166, 2024.
Liao, H., Seinfeld, J. H., Adams, P. J., and Mickley, L. J.: Global radiative forcing of coupled tropospheric ozone and aerosols in a unified general circulation model, J. Geophys. Res.-Atmos., 109, D16, https://doi.org/10.1029/2003JD004456 , 2004.
Lohmann, U. and Feichter, J.: Global indirect aerosol effects: a review, Atmos. Chem. Phys., 5, 715–737, https://doi.org/10.5194/acp-5-715-2005, 2005.
Lohmann, U. and Roeckner, E.: Design and performance of a new cloud microphysics scheme developed for the ECHAM general circulation model, Clim. Dynam., 12, 557–572, https://doi.org/10.1007/BF00207939 , 1996.
Lohmann, U. and Ferrachat, S.: Impact of parametric uncertainties on the present-day climate and on the anthropogenic aerosol effect, Atmos. Chem. Phys., 10, 11373–11383, https://doi.org/10.5194/acp-10-11373-2010, 2010.
Milousis, A., Tsimpidi, A. P., Tost, H., Pandis, S. N., Nenes, A., Kiendler-Scharr, A., and Karydis, V. A.: Implementation of the ISORROPIA-lite aerosol thermodynamics model into the EMAC chemistry climate model (based on MESSy v2.55): implications for aerosol composition and acidity, Geosci. Model Dev., 17, 1111–1131, https://doi.org/10.5194/gmd-17-1111-2024, 2024.
Morales Betancourt, R. and Nenes, A.: Understanding the contributions of aerosol properties and parameterization discrepancies to droplet number variability in a global climate model, Atmos. Chem. Phys., 14, 4809–4826, https://doi.org/10.5194/acp-14-4809-2014, 2014.
Myhre, G., Samset, B. H., Schulz, M., Balkanski, Y., Bauer, S., Berntsen, T. K., Bian, H., Bellouin, N., Chin, M., Diehl, T., Easter, R. C., Feichter, J., Ghan, S. J., Hauglustaine, D., Iversen, T., Kinne, S., Kirkevåg, A., Lamarque, J.-F., Lin, G., Liu, X., Lund, M. T., Luo, G., Ma, X., van Noije, T., Penner, J. E., Rasch, P. J., Ruiz, A., Seland, Ø., Skeie, R. B., Stier, P., Takemura, T., Tsigaridis, K., Wang, P., Wang, Z., Xu, L., Yu, H., Yu, F., Yoon, J.-H., Zhang, K., Zhang, H., and Zhou, C.: Radiative forcing of the direct aerosol effect from AeroCom Phase II simulations, Atmos. Chem. Phys., 13, 1853–1877, https://doi.org/10.5194/acp-13-1853-2013, 2013.
Myhre, G., Shindell, D., Bréon, F.-M., Collins, W., Fuglestvedt, J., Huang, J., Koch, D., Lamarque, J.-F., Lee, D., Mendoza, B., Nakajima, T., Robock, A., Stephens, G., Takemura, T., and Zhang, H., Anthropogenic and Natural Radiative Forcing. Climate Change 2013 – The Physical Science Basis, Cambridge University Press, 659–740 pp., https://doi.org/10.1017/CBO9781107415324.018, 2014.
Nenes, A., Murray, B., and Bougiatioti, A.: Mineral Dust and its Microphysical Interactions with Clouds, in: Mineral Dust, edited by: Knippertz, P. and Stuut, J. B., Springer, Dordrecht, https://doi.org/10.1007/978-94-017-8978-3_12, 2014.
Nenes, A., Pandis, S. N., Weber, R. J., and Russell, A.: Aerosol pH and liquid water content determine when particulate matter is sensitive to ammonia and nitrate availability, Atmos. Chem. Phys., 20, 3249–3258, https://doi.org/10.5194/acp-20-3249-2020, 2020.
O'Neill, B. C., Tebaldi, C., van Vuuren, D. P., Eyring, V., Friedlingstein, P., Hurtt, G., Knutti, R., Kriegler, E., Lamarque, J.-F., Lowe, J., Meehl, G. A., Moss, R., Riahi, K., and Sanderson, B. M.: The Scenario Model Intercomparison Project (ScenarioMIP) for CMIP6, Geosci. Model Dev., 9, 3461–3482, https://doi.org/10.5194/gmd-9-3461-2016, 2016.
Pozzer, A., Jöckel, P., Sander, R., Williams, J., Ganzeveld, L., and Lelieveld, J.: Technical Note: The MESSy-submodel AIRSEA calculating the air-sea exchange of chemical species, Atmos. Chem. Phys., 6, 5435–5444, https://doi.org/10.5194/acp-6-5435-2006, 2006.
Pozzer, A., de Meij, A., Pringle, K. J., Tost, H., Doering, U. M., van Aardenne, J., and Lelieveld, J.: Distributions and regional budgets of aerosols and their precursors simulated with the EMAC chemistry-climate model, Atmos. Chem. Phys., 12, 961–987, https://doi.org/10.5194/acp-12-961-2012, 2012.
Pozzer, A., Reifenberg, S. F., Kumar, V., Franco, B., Kohl, M., Taraborrelli, D., Gromov, S., Ehrhart, S., Jöckel, P., Sander, R., Fall, V., Rosanka, S., Karydis, V., Akritidis, D., Emmerichs, T., Crippa, M., Guizzardi, D., Kaiser, J. W., Clarisse, L., Kiendler-Scharr, A., Tost, H., and Tsimpidi, A.: Simulation of organics in the atmosphere: evaluation of EMACv2.54 with the Mainz Organic Mechanism (MOM) coupled to the ORACLE (v1.0) submodel, Geosci. Model Dev., 15, 2673–2710, https://doi.org/10.5194/gmd-15-2673-2022, 2022.
Pringle, K. J., Tost, H., Message, S., Steil, B., Giannadaki, D., Nenes, A., Fountoukis, C., Stier, P., Vignati, E., and Lelieveld, J.: Description and evaluation of GMXe: a new aerosol submodel for global simulations (v1), Geosci. Model Dev., 3, 391–412, https://doi.org/10.5194/gmd-3-391-2010, 2010a.
Pringle, K. J., Tost, H., Metzger, S., Steil, B., Giannadaki, D., Nenes, A., Fountoukis, C., Stier, P., Vignati, E., and Lelieveld, J.: Corrigendum to ”Description and evaluation of GMXe: a new aerosol submodel for global simulations (v1)” published in Geosci. Model Dev., 3, 391–412, 2010, Geosci. Model Dev., 3, 413–413, https://doi.org/10.5194/gmd-3-413-2010, 2010b.
Pringle, K. J., Tost, H., Pozzer, A., Pöschl, U., and Lelieveld, J.: Global distribution of the effective aerosol hygroscopicity parameter for CCN activation, Atmos. Chem. Phys., 10, 5241–5255, https://doi.org/10.5194/acp-10-5241-2010, 2010c.
Roeckner, E., Brokopf, R., Esch, M., Giorgetta, M., Hagemann, S., Kornblueh, L., Manzini, E., Schlese, U., and Schulzweida, U.: Sensitivity of simulated climate to horizontal and vertical resolution in the ECHAM5 atmosphere model, J. Climate, 19, 3771–3791, https://doi.org/10.1175/JCLI3824.1, 2006.
Sander, R., Baumgaertner, A., Cabrera-Perez, D., Frank, F., Gromov, S., Grooß, J.-U., Harder, H., Huijnen, V., Jöckel, P., Karydis, V. A., Niemeyer, K. E., Pozzer, A., Riede, H., Schultz, M. G., Taraborrelli, D., and Tauer, S.: The community atmospheric chemistry box model CAABA/MECCA-4.0, Geosci. Model Dev., 12, 1365–1385, https://doi.org/10.5194/gmd-12-1365-2019, 2019.
Seinfeld, J. H. and Pandis, S. N.: Atmospheric chemistry and physics from air pollution to climate change, John Wiley & Sons, ISBN 1118947401, 2016.
Seinfeld, J. H., Bretherton, C., Carslaw, K. S., Coe, H., DeMott, P. J., Dunlea, E. J., Feingold, G., Ghan, S., Guenther, A. B., Kahn, R., Kraucunas, I., Kreidenweis, S. M., Molina, M. J., Nenes, A., Penner, J. E., Prather, K. A., Ramanathan, V., Ramaswamy, V., Rasch, P. J., Ravishankara, A. R., Rosenfeld, D., Stephens, G., and Wood, R.: Improving our fundamental understanding of the role of aerosol-cloud interactions in the climate system, P. Natl. Acad. Sci. USA, 113, 5781–5790, https://doi.org/10.1073/pnas.1514043113, 2016.
Seisel, S., Börensen, C., Vogt, R., and Zellner, R.: Kinetics and mechanism of the uptake of N2O5 on mineral dust at 298 K, Atmos. Chem. Phys., 5, 3423–3432, https://doi.org/10.5194/acp-5-3423-2005, 2005.
Sposito, G.: The Chemistry of Soils, Oxford University Press, New York, ISBN 9780190630881, 1989.
Sundqvist, H., Berge, E., and Kristjánsson, J. E.: Condensation and Cloud Parameterization Studies with a Mesoscale Numerical Weather Prediction Model, Mon. Weather Rev., 117, 1641–1657, https://doi.org/10.1175/1520-0493(1989)117<1641:CACPSW>2.0.CO;2, 1989.
Tang, M. J., Thieser, J., Schuster, G., and Crowley, J. N.: Kinetics and mechanism of the heterogeneous reaction of N2O5 with mineral dust particles, Phys. Chem. Chem. Phys., 14, 8551–8561, https://doi.org/10.1039/C2CP40805H, 2012.
The Acid Deposition Monitoring Network in East Asia: EANET Data on the Acid Deposition in the East Asian Region, https://monitoring.eanet.asia/document/public/index (last access: 3 September 2024), 2024.
The MESSy Consortium: The Modular Earth Submodel System (2.55.2_842-isorropia-light), Zenodo, https://doi.org/10.5281/zenodo.8379120, 2023.
Tompkins, A. M.: A Prognostic Parameterization for the Subgrid-Scale Variability of Water Vapor and Clouds in Large-Scale Models and Its Use to Diagnose Cloud Cover, J. Atmos. Sci., 59, 1917–1942, https://doi.org/10.1175/1520-0469(2002)059<1917:APPFTS>2.0.CO;2, 2002.
Tost, H., Jöckel, P., Kerkweg, A., Sander, R., and Lelieveld, J.: Technical note: A new comprehensive SCAVenging submodel for global atmospheric chemistry modelling, Atmos. Chem. Phys., 6, 565–574, https://doi.org/10.5194/acp-6-565-2006, 2006.
Tost, H., Jöckel, P., and Lelieveld, J.: Lightning and convection parameterisations – uncertainties in global modelling, Atmos. Chem. Phys., 7, 4553–4568, https://doi.org/10.5194/acp-7-4553-2007, 2007a.
Tost, H., Jöckel, P., Kerkweg, A., Pozzer, A., Sander, R., and Lelieveld, J.: Global cloud and precipitation chemistry and wet deposition: tropospheric model simulations with ECHAM5/MESSy1, Atmos. Chem. Phys., 7, 2733–2757, https://doi.org/10.5194/acp-7-2733-2007, 2007b.
Trump, E. R., Fountoukis, C., Donahue, N. M., and Pandis, S. N.: Improvement of simulation of fine inorganic PM levels through better descriptions of coarse particle chemistry, Atmos. Environ., 102, 274–281, https://doi.org/10.1016/j.atmosenv.2014.11.059, 2015.
Tsigaridis, K. and Kanakidou, M.: The present and future of secondary organic aerosol direct forcing on climate, Curr. Clim. Change Rep., 4, 84–98, https://doi.org/10.1007/s40641-018-0092-3 , 2018.
Tsimpidi, A. P., Karydis, V. A., Pandis, S. N., and Lelieveld, J.: Global combustion sources of organic aerosols: model comparison with 84 AMS factor-analysis data sets, Atmos. Chem. Phys., 16, 8939–8962, https://doi.org/10.5194/acp-16-8939-2016, 2016.
Tsimpidi, A. P., Karydis, V. A., Pandis, S. N., and Lelieveld, J.: Global-scale combustion sources of organic aerosols: sensitivity to formation and removal mechanisms, Atmos. Chem. Phys., 17, 7345–7364, https://doi.org/10.5194/acp-17-7345-2017, 2017.
Twomey, S.: The Influence of Pollution on the Shortwave Albedo of Clouds, J. Atmos. Sci., 34, 1149–1152, https://doi.org/10.1175/1520-0469(1977)034<1149:TIOPOT>2.0.CO;2 , 1977.
Urdiales-Flores, D., Zittis, G., Hadjinicolaou, P., Osipov, S., Klingmüller, K., Mihalopoulos, N., Kanakidou, M., Economou, T., and Lelieveld, J.: Drivers of accelerated warming in Mediterranean climate-type regions, npj Clim. Atmos. Sci., 6, 97, https://doi.org/10.1038/s41612-023-00423-1, 2023.
U.S. Environmental Protection Agency Clean Air Markets Division Clean Air Status and Trends Network (CASTNET): CASTNET Data, https://www.epa.gov/castnet (last access: 3 September 2024), 2024.
Vignati, E., Wilson, J., and Stier, P.: M7: An efficient size-resolved aerosol microphysics module for large-scale aerosol transport models, J. Geophys. Res.-Atmos., 109, D22, https://doi.org/10.1029/2003JD004485, 2004.
Wexler, A. S. and Seinfeld, J. H.: Second-generation inorganic aerosol model, Atmos. Environ. A, 25, 2731–2748, https://doi.org/10.1016/0960-1686(91)90203-J, 1991.
Wong, J. P. S., Tsagkaraki, M., Tsiodra, I., Mihalopoulos, N., Violaki, K., Kanakidou, M., Sciare, J., Nenes, A., and Weber, R. J.: Atmospheric evolution of molecular-weight-separated brown carbon from biomass burning, Atmos. Chem. Phys., 19, 7319–7334, https://doi.org/10.5194/acp-19-7319-2019, 2019.
Xu, L. and Penner, J. E.: Global simulations of nitrate and ammonium aerosols and their radiative effects, Atmos. Chem. Phys., 12, 9479–9504, https://doi.org/10.5194/acp-12-9479-2012, 2012.
Yienger, J. J. and Levy, H.: EMPIRICAL-MODEL OF GLOBAL SOIL-BIOGENIC NOX EMISSIONS, J. Geophys. Res.-Atmos., 100, D6, https://doi.org/10.1029/95JD00370, 1995.
Zhang, Y., Forrister, H., Liu, J., Dibb, J., Anderson, B., Schwarz, J. P., Perring, A. E., Jimenez, J. L., Campuzano-Jost, P., Wang, Y., Nenes, A., and Weber, R. J.: Top-of-atmosphere radiative forcing affected by brown carbon in the upper troposphere, Nat. Geosci., 10, 486–489, https://doi.org/10.1038/ngeo2960, 2017.
Zhang, B.: The effect of aerosols to climate change and society, J. Geosci. Environ. Protect., 8, 55, https://doi.org/10.4236/gep.2020.88006 , 2020.
- Abstract
- Introduction
- Methodology
- Radiative effect from aerosol–radiation interactions (REari)
- Radiative effect from aerosol–cloud interactions (REaci)
- Effect of NO aerosols on cloud microphysics
- Conclusions and discussion
- Code and data availability
- Author contributions
- Competing interests
- Disclaimer
- Special issue statement
- Acknowledgements
- Financial support
- Review statement
- References
- Supplement
- Abstract
- Introduction
- Methodology
- Radiative effect from aerosol–radiation interactions (REari)
- Radiative effect from aerosol–cloud interactions (REaci)
- Effect of NO aerosols on cloud microphysics
- Conclusions and discussion
- Code and data availability
- Author contributions
- Competing interests
- Disclaimer
- Special issue statement
- Acknowledgements
- Financial support
- Review statement
- References
- Supplement