the Creative Commons Attribution 4.0 License.
the Creative Commons Attribution 4.0 License.
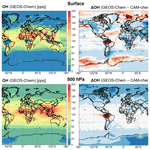
Intercomparison of GEOS-Chem and CAM-chem tropospheric oxidant chemistry within the Community Earth System Model version 2 (CESM2)
Louisa K. Emmons
Elizabeth W. Lundgren
Laura Hyesung Yang
Ruijun Dang
Shixian Zhai
Yunxiao Tang
Makoto M. Kelp
Nadia K. Colombi
Sebastian D. Eastham
Thibaud M. Fritz
Daniel J. Jacob
Tropospheric ozone is a major air pollutant and greenhouse gas. It is also the primary precursor of OH, the main tropospheric oxidant. Global atmospheric chemistry models show large differences in their simulations of tropospheric ozone budgets. Here we implement the widely used GEOS-Chem atmospheric chemistry module as an alternative to CAM-chem within the Community Earth System Model version 2 (CESM2). We compare the resulting GEOS-Chem and CAM-chem simulations of tropospheric ozone and related species within CESM2 to observations from ozonesondes, surface sites, the ATom-1 aircraft campaign over the Pacific and Atlantic, and the KORUS-AQ aircraft campaign over the Seoul Metropolitan Area. We find that GEOS-Chem and CAM-chem within CESM2 have similar tropospheric ozone budgets and concentrations usually within 5 ppb but important differences in the underlying processes including (1) photolysis scheme (no aerosol effects in CAM-chem), (2) aerosol nitrate photolysis, (3) N2O5 cloud uptake, (4) tropospheric halogen chemistry, and (5) ozone deposition to the oceans. Global tropospheric OH concentrations are the same in both models, but there are large regional differences reflecting the above processes. Carbon monoxide is lower in CAM-chem (and lower than observations), at least in part because of higher OH concentrations in the Northern Hemisphere and insufficient production from isoprene oxidation in the Southern Hemisphere. CESM2 does not scavenge water-soluble gases in convective updrafts, leading to some upper-tropospheric biases. Comparison to KORUS-AQ observations shows an overestimate of ozone above 4 km altitude in both models, which at least in GEOS-Chem is due to inadequate scavenging of particulate nitrate in convective updrafts in CESM2, leading to excessive NO production from nitrate photolysis. The KORUS-AQ comparison also suggests insufficient boundary layer mixing in CESM2. This implementation and evaluation of GEOS-Chem in CESM2 contribute to the MUSICA vision of modularizing tropospheric chemistry in Earth system models.
- Article
(7881 KB) - Full-text XML
- BibTeX
- EndNote
Ozone is a central species in atmospheric chemistry. It is a major air pollutant and greenhouse gas and the primary source of the hydroxyl radical (OH), which is the main tropospheric oxidant (Monks et al., 2015). It is produced within the troposphere by complicated chemical mechanisms involving hydrogen oxide radicals (), nitrogen oxide radicals (), volatile organic compounds (VOCs), and ozone itself. It is extensively observed from surface sites, aircraft, sondes, and satellites and is thus an important indicator of skill for chemical transport models (Hu et al., 2017). At the same time, comparisons with observations can be successful for the wrong reasons. Extensive intercomparisons of global models often show similar tropospheric ozone burdens but large differences in chemical source and sink magnitudes (Wu et al., 2007; Young et al., 2018), implying large differences in sensitivity to perturbations. This is a particular problem for chemistry–climate models that aim to quantify chemical feedbacks on climate change.
Here we compare two state-of-the-science atmospheric chemistry modules, GEOS-Chem and CAM-chem, within the Community Earth System Model (CESM2) (Danabasoglu et al., 2020). CAM-chem is the resident atmospheric chemistry module in CESM2 and as such has a large user base (Lamarque et al., 2012; Tilmes et al., 2015, 2016; Emmons et al., 2020). GEOS-Chem is used by hundreds of research groups worldwide as an offline chemical transport model (CTM) driven by the GEOS archive of external meteorological data (Bey et al., 2001). Offline here is defined by contrast to online models that perform their own simulations of atmospheric dynamics (Brasseur and Jacob, 2017). GEOS-Chem is grid-independent and modularized so that the chemical module describing local operations in 1-D model columns (including emissions, chemistry, and deposition) is separated from the transport module (Long et al., 2015). This allows independent implementation of the GEOS-Chem chemical module in online models, where chemical transport is done as part of the simulation of atmospheric dynamics (Hu et al., 2018; Lin et al., 2020; Lu et al., 2020; Keller et al., 2021). The GEOS-Chem chemical module has been previously coupled to the WRF and GEOS meteorological models to investigate aerosol–chemistry–climate feedbacks (Feng et al., 2021; Moch et al., 2022) and powers the GEOS global chemical forecasts (GEOS-CF) (Keller et al., 2021). The same GEOS-Chem scientific code base is used as in the offline CTM such that version updates developed for the CTM can be seamlessly passed on to the online applications.
Fritz et al. (2022) implemented the GEOS-Chem chemical module in CESM2 as the first application of that module to an open-source Earth system model (ESM) for community use. GEOS-Chem offers an alternative representation of atmospheric chemistry to CAM-chem within CESM2, contributing to the MUSICA (MUlti-Scale Infrastructure for Chemistry and Aerosols; Pfister et al., 2020) vision for CESM of allowing users to choose among a range of options for atmospheric chemistry. The GEOS-Chem emission component (HEMCO; Keller et al., 2014) has been previously implemented in MUSICA (Lin et al., 2021). Fritz et al. (2022) presented general comparisons between GEOS-Chem and CAM-chem in the CESM2 environment. They found good agreement between the two modules for stratospheric ozone but lower tropospheric ozone in GEOS-Chem due to tropospheric halogen chemistry not considered in CAM-chem. They found several challenges in the implementation of the GEOS-Chem chemical module within CESM2. For example, CESM2 uses the MAM4 (Modal Aerosol Model version 4; Liu et al., 2016) modal aerosol microphysics to simulate aerosol–cloud interactions and aerosol–radiation interactions, while GEOS-Chem uses either bulk or sectional representations of aerosol microphysics. CESM2 does not couple convective transport with scavenging of water-soluble species, but this is a major process in the GEOS-Chem CTM to prevent unphysical buildup of water-soluble species in the upper troposphere (Balkanski et al., 1993; Liu et al., 2001). If convective transport and scavenging are applied sequentially, instead of being coupled, then water-soluble species can reach the upper troposphere in deep convective updrafts and disperse on the model grid scale to avoid scavenging. Indeed, Fritz et al. (2022) found large overestimates of upper-tropospheric aerosol in GEOS-Chem within CESM2 as compared to the offline GEOS-Chem.
Our work builds on the Fritz et al. (2022) initial implementation of GEOS-Chem in CESM2 to address the previous challenges and to give a more thorough evaluation with observations and intercomparison with CAM-chem. We focus on tropospheric ozone and related oxidant chemistry from both a global perspective (ozonesonde and ATom-1 aircraft observations) and polluted conditions over East Asia (KORUS-AQ aircraft observations). KORUS-AQ, conducted in May–June 2016, is of particular interest because of the previously identified large differences between offline GEOS-Chem and CAM-chem in simulating the aircraft observations including 20–30 ppb differences in ozone (Park et al., 2021). We analyze the individual processes driving differences between GEOS-Chem and CAM-chem and use observations to arbitrate when possible. This process-based intercomparison of GEOS-Chem and CAM-chem leverages the unique capability of comparing these two major representations side by side in a common ESM environment where specific causes of model differences can be attributed to different representations of chemistry. As part of resolving differences in photolysis rates, we implement into CAM-chem the Fast-JX photolysis scheme used in GEOS-Chem (Bian and Prather, 2002), further contributing to the MUSICA vision of process-level modularization of atmospheric chemistry models.
2.1 CESM2, CAM-chem, and HEMCO
We use a beta version of CESM 2.3 including the CAM6 Community Atmosphere Model (CAM tag version cam6_3_095), which has provided the basis for the integration of the GEOS-Chem module into the mainline CESM code. All simulations are for the year 2016 with an 18-month initialization period. The year was chosen for evaluation with the ATom (Wofsy et al., 2018) and KORUS-AQ (Crawford et al., 2021) aircraft campaigns. We use a global 0.9°×1.25° grid with 32 vertical layers up to 2 hPa. We use the “F” compsets in CESM, which use active atmosphere and land models with prescribed sea surface temperatures, sea ice, and greenhouse gases for current climate (CMIP6 SSP2-4.5 scenario). The model reproduces a given meteorological year by nudging winds and temperature (using the FCnudged
configuration in CAM6) to a 3-hourly MERRA2 meteorological reanalysis produced by the NASA Global Modeling and Assimilation Office. This nudging is done with a 50 h relaxation time that allows CAM to generate its own physics, including the hydrological cycle and the effects of aerosols on clouds.
CAM-chem is the standard representation of tropospheric–stratospheric chemistry in CESM2, currently using the MOZART-TS1 (Model for OZone And Related chemical Tracers; Emmons et al., 2020) mechanism and the Modal Aerosol Model with four modes (MAM4; Liu et al., 2016) as the default. MOZART-TS1 includes 229 chemical species and 541 reactions. Photolysis is calculated using a lookup table based on the Tropospheric Ultraviolet and Visible (TUV) radiation model, which takes into account the impact of clouds but not aerosols (Kinnison et al., 2007). A sensitivity simulation developed for this project uses Fast-JX instead of the TUV lookup table for photolysis.
The CAM-chem version in our work uses HEMCO for emissions but is otherwise unmodified. HEMCO is the standard emission component of GEOS-Chem (Keller et al., 2014), now implemented in CESM as part of MUSICA (Lin et al., 2021). It allows the use of any emission inventories on any grid to be supplied to the model in netCDF format at runtime with options to add, supersede, and scale emissions. Here we use the same emissions in GEOS-Chem and CAM-chem processed through HEMCO. This includes global anthropogenic emissions from the CEDSv2 inventory (Community Emissions Data System; McDuffie et al., 2021) superseded by the KORUSv5 inventory (Woo et al., 2020) over East Asia. Fire emissions are from the GFED4.1s inventory (van der Werf et al., 2017; Randerson et al., 2018). HEMCO has extensions to use emission modules dependent on environmental variables, and this is applied to soil NOx emissions from Hudman et al. (2012) and ocean iodine emissions from Sherwen et al. (2016a, b). We otherwise use emissions computed from other modules in CESM to enforce consistency of the atmospheric chemistry simulation with other CESM components. This includes biogenic VOC emissions from MEGANv2.1 (Guenther et al., 2012) computed with the Community Land Model (CLM) and lightning NOx, dust, and sea salt emissions from CAM (Price et al., 1997; Mahowald et al., 2006a, b; Lamarque et al., 2012).
2.2 GEOS-Chem within CESM2
Unless explicitly written otherwise, GEOS-Chem in this work refers to the online implementation of the GEOS-Chem chemical module within the CESM2 model and not the offline CTM. We use GEOS-Chem version 14.1.1 (https://doi.org/10.5281/zenodo.7696632, The International GEOS-Chem User Community, 2023) with the addition of particulate nitrate (p) photolysis following Shah et al. (2023), which was subsequently implemented in version 14.2.0 (https://doi.org/10.5281/zenodo.8411433). The same GEOS-Chem chemical module and MERRA-2 meteorological fields are used in CESM2 and in the offline CTM simulations presented here. The GEOS-Chem chemical mechanism has 286 species and 914 reactions with a development history independent of MOZART-TS1. It features recent major updates to NOx heterogeneous and cloud chemistry (Holmes et al., 2019), isoprene chemistry (Bates and Jacob, 2019), aromatic chemistry (Bates et al., 2021), and Cl–Br–I tropospheric halogen chemistry (Wang et al., 2021). Photolysis is calculated using the Fast-JX model (Bian and Prather, 2002) with consistent aerosol and overhead column ozone information from the GEOS-Chem simulation (Eastham et al., 2014). No aerosol microphysics is included here so that aerosol concentrations are represented by the bulk masses of their chemical components (Park et al., 2004; Pai et al., 2020) but with four size bins for dust and two for sea salt aerosol (Alexander et al., 2005; Fairlie et al., 2010).
Fritz et al. (2022) describe the original implementation of GEOS-Chem within CESM2. They developed an interface to pass input data to GEOS-Chem, run the GEOS-Chem chemical module, and export the updated chemical species concentrations. The interface converts between the bulk aerosols in GEOS-Chem and the modal aerosols in MAM4 for aerosol–radiation and aerosol–cloud interactions. Coupling of the GEOS-Chem chemical module to CESM2 required the adaptation of several components for compatibility with CESM2 or consistency with CAM-chem. We summarize in Table 1 the important differences between the atmospheric chemistry representations in CAM-chem, GEOS-Chem within CESM2, and the offline GEOS-Chem CTM.
Table 1Major differences between CAM-chem and GEOS-Chem simulations.
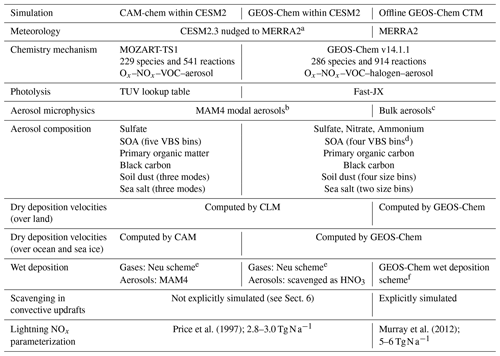
a With 50 h relaxation time, nudging U, V, and T. b GEOS-Chem bulk aerosols masses are mapped to MAM4 modes for aerosol–radiation and aerosol–cloud interaction effects within CESM2. See Fritz et al. (2022) for the species mapping between GEOS-Chem species to MAM4 aerosols. c Sectional aerosol microphysics are available in GEOS-Chem (Yu and Luo, 2009; Kodros and Pierce, 2017) but are not used here. d SOA denotes secondary organic aerosol; VBS denotes volatility basis set. GEOS-Chem here uses the complex SOA option from Pye et al. (2010). e Neu and Prather (2012). f Liu et al. (2001) for water-soluble aerosols and Amos et al. (2012) for gases.
Here we make several improvements and corrections to the original implementation of GEOS-Chem within CESM2 by Fritz et al. (2022). We simulate nucleation in MAM4 by passing the gas-phase H2SO4 production rate computed in GEOS-Chem from the SO2 + OH reaction. We add an aerosol sink in the upper troposphere and lower stratosphere following Hodzic et al. (2015, 2016) to compensate for CESM2's omission of coupling convective transport and scavenging. We correct the sea surface temperatures passed to HEMCO, which results in inorganic iodine emissions being 1 % of the previously incorrectly calculated value. We also add numerous GEOS-Chem diagnostics for analyzing model output, including individual reaction rates and total production and loss rates for individual species.
Figure 1 shows the mean photolysis frequencies (J values) for NO2 () and O3 to O(1D) (JO1D) simulated by the GEOS-Chem model (with Fast-JX) and the difference with CAM-chem (with TUV lookup table) in surface air in July. Photolysis rates in GEOS-Chem with Fast-JX are generally lower than in CAM-chem with TUV. Differences for are typically 0 %–10 % over oceans and 10 %–20 % over land, while differences for JO1D are typically 10 %–20 % over oceans and 20 %–40 % over land. There are some larger differences in polluted and open-fire regions, such as in East Asia and Siberia, and at high latitudes.
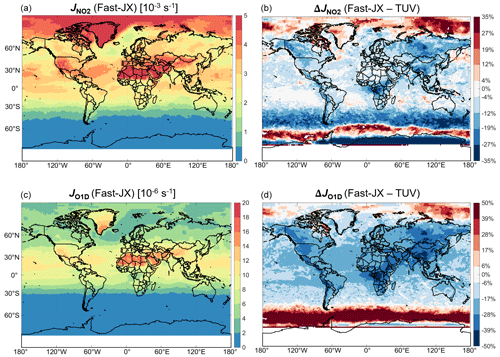
Figure 1Mean photolysis frequencies for NO2 () and O3 to O(1D) (JO1D) in surface air in July 2016. The left panels (a, c) show the values computed by Fast-JX within GEOS-Chem. The right panels (b, d) show the differences (Δ) with the values computed by TUV within CAM-chem.
Fast-JX and TUV use the same spectroscopic data from the NASA JPL recommendations (Burkholder et al., 2020). Fast-JX includes aerosol extinction, but TUV does not, which explains the larger differences over polluted and open-fire regions. Differences over the oceans are mainly due to clouds. While Fast-JX and TUV both represent effects of cloud extinction, treatment of cloud scattering between the two schemes is different. The effects of aerosol–cloud interactions on cloud properties through MAM4 cause GEOS-Chem and CAM-chem to have different cloud optical depths that can lead to further differences. Cloud effects are particularly large at high latitudes because of extensive cloud cover and low sun angles. Sensitivity simulations for clear sky (no cloud or aerosol extinction input to the photolysis schemes) show smaller differences between Fast-JX and TUV, generally less than 10 % for and less than 20 % for JO1D, while sensitivity simulations with Fast-JX implemented in CAM-chem show less than 5 % differences for and JO1D everywhere compared to Fast-JX implemented in GEOS-Chem.
Figure 2 shows photolysis frequencies from the KORUS-AQ and ATom-1 campaigns derived from actinic flux measurements (Hall et al., 2018; Crawford et al., 2021), compared to the photolysis frequencies computed by Fast-JX and TUV sampled along the aircraft flight tracks. values agree within 10 %, and there is no systematic bias relative to observations. Fast-JX values tend to be higher than TUV at high altitudes, and this can be attributed to cloud effects as discussed above. JO1D values also show good agreement for ATom-1, but observed values for KORUS-AQ are much lower than for ATom-1 in the same season, which is captured by Fast-JX but not by TUV (which is 30 % too high). We find that the overestimate of JO1D by TUV during KORUS-AQ is due in part to not accounting for aerosol extinction. Comparison of clear-sky J values shows that there is some additional unidentified factor causing TUV to be too high during KORUS-AQ, and this disappears when Fast-JX is implemented in CAM-chem. In what follows the CAM-chem simulation uses the TUV lookup table, but we will comment as appropriate on the effect of switching to Fast-JX.
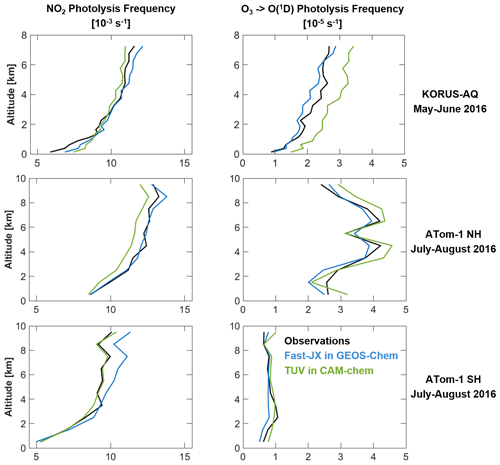
Figure 2Median vertical profiles of and JO1D from the KORUS-AQ aircraft campaign over the Seoul Metropolitan Area (SMA; 37–37.6° N, 126.6–127.7° E) in May–June 2016 and the ATom-1 aircraft campaign over the Pacific and Atlantic oceans in July–August 2016. Observations from in situ measurements of actinic fluxes (Hall et al., 2018) are compared to CESM2 values using the Fast-JX scheme within GEOS-Chem and the TUV scheme within CAM-chem. The model values are sampled along the aircraft flight tracks.
Table 2 shows global tropospheric ozone and OH budgets from GEOS-Chem and CAM-chem compared to the literature. Ozone budgets from the two models and the multi-model mean in Young et al. (2018) are within 10 % of each other. The larger chemical production and shorter chemical lifetime in GEOS-Chem are mainly due to photolysis of particulate nitrate (Shah et al., 2023), without which chemical production in GEOS-Chem decreases by 10 % to 4902 Tg a−1, and the tropospheric ozone burden decreases by 5 % to 332 Tg. The lower dry deposition in GEOS-Chem reflects lower ozone deposition to the ocean (Pound et al., 2020). GEOS-Chem and CAM-chem have the same global OH concentrations, on the high end of the range of values from the ACCMIP and CCMI model ensembles (Naik et al., 2013; Zhao et al., 2019). The lifetime of methylchloroform against loss to tropospheric OH is 5.4 and 5.3 years, respectively, in GEOS-Chem and CAM-chem, 15 % lower than 6.3 ± 0.4 years inferred from observations (Prather et al., 2012).
Table 2Global budgets of tropospheric ozone and OH.a
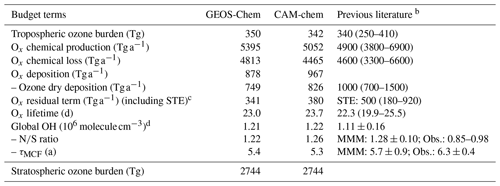
a Annual mean values for 2016 from GEOS-Chem and CAM-chem in CESM2. The troposphere is defined by [O3]<150 ppb (Young et al., 2013). The budget is for the odd oxygen (Ox) family to account for rapid cycling between Ox species: , where X is Cl, Br, or I. CAM-chem does not include particulate nitrate or tropospheric halogen species.
b Means and ranges from Young et al. (2018) (33 models) for ozone and Naik et al. (2013) (16 ACCMIP models) for OH, for the year 2000. MMM: multi-model mean. Obs.: observation-derived estimates.
c Residual of mass balance between tropospheric chemical production and loss, Ox deposition, and accumulation. This term represents an estimate of stratosphere–troposphere exchange (STE) in the absence of advective flux diagnostics in CESM2. The accumulation term in the GEOS-Chem and CAM-chem models over 2016 is 0.4 and −4.9 Tg a−1, respectively.
d Global annual mean air-mass-weighted OH concentration in the troposphere. NS ratio denotes the ratio between the two hemispheres. τMCF denotes the lifetime of atmospheric methylchloroform against oxidation by tropospheric OH.
Figure 3 shows the spatial distribution of annual mean OH concentrations simulated by GEOS-Chem and the difference with CAM-chem. Despite having the same global mean OH concentrations, the two models have large regional differences. GEOS-Chem is up to 30 % lower than CAM-chem over the continents, particularly over polluted regions, due to lower J(O1D) and possibly higher OH reactivity. Over the Amazon and Congo basins where NOx is low, isoprene does not titrate OH in GEOS-Chem due to recent updates in isoprene oxidation chemistry incorporating H-shift isomerization of isoprene–hydroxy–peroxy radicals to recycle OH, which sustains OH under low-NO conditions (Bates and Jacob, 2019).
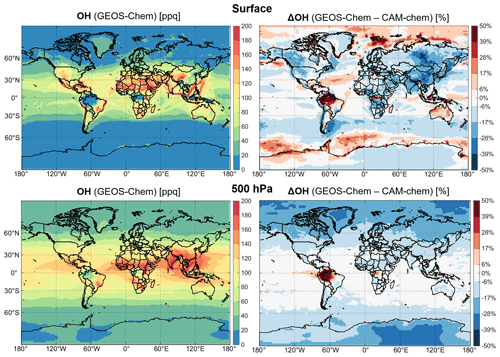
Figure 3Annual mean OH concentrations in surface air and at 500 hPa in GEOS-Chem within CESM2 and differences with CAM-chem. Percentage differences are relative to CAM-chem. Values are for 2016.
Figure 4 shows annual mean surface and 500 hPa ozone and NOx concentrations simulated by GEOS-Chem and differences with CAM-chem. Ozone differences are generally smaller than 5 ppb, indicating a remarkable degree of agreement. The largest surface differences are at southern midlatitudes due to slower ozone deposition to the ocean in GEOS-Chem. At 500 hPa, GEOS-Chem has lower ozone at high latitudes due to tropospheric halogen chemistry. This chemistry increases ozone destruction through catalytic ozone loss cycles driven by iodine and bromine and decreases ozone production by conversion of NOx to halogen nitrates. Tropospheric halogen chemistry is not represented in the default configuration of CAM-chem. N2O5 uptake in clouds, included in GEOS-Chem (Holmes et al., 2019) but not in CAM-chem, also contributes to the lower GEOS-Chem ozone at high northern latitudes. Particulate nitrate photolysis in GEOS-Chem corrects for a missing NOx source in the remote troposphere (Shah et al., 2023) and accounts for the higher NOx and ozone than CAM-chem over the oceans.
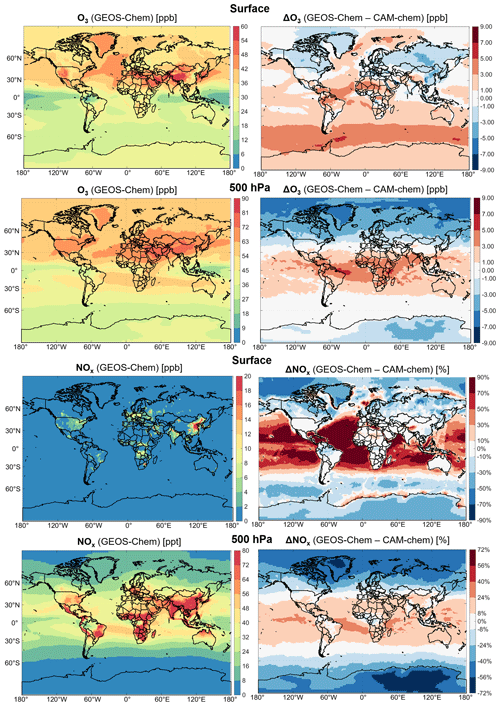
Figure 4Annual mean ozone and NOx concentrations in surface air and at 500 hPa in GEOS-Chem within CESM2 and differences with CAM-chem. Percentage differences are relative to CAM-chem. Values are for 2016.
Table 3 shows global budget terms in the troposphere from sensitivity simulations varying the most important differences between GEOS-Chem and CAM-chem. The largest controlling factors for tropospheric ozone differences between GEOS-Chem and CAM-chem are nitrate photolysis and tropospheric halogen chemistry, which increase and decrease the tropospheric ozone burden by 5 % and 4 %, respectively. The global tropospheric NOx burden is 4 % lower in GEOS-Chem than CAM-chem because of conversion to halogen nitrates and use of Fast-JX offsetting the effect of nitrate photolysis. Using Fast-JX for photolysis in CAM-chem results in a 7 % decrease in tropospheric NOx, which we attribute to lower in surface air over continents (Fig. 1).
Table 3Global tropospheric budget terms from different configurations of GEOS-Chem and CAM-chem in CESM2.a
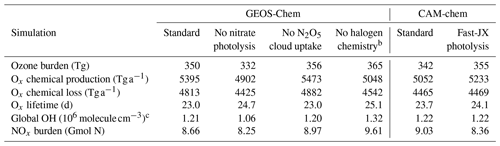
a Refer to footnotes in Table 2. The standard entries replicate those of Table 2.
b Zeroing out reaction rates for halogen reactions in the troposphere.
c Global annual mean air-mass-weighted OH concentration in the troposphere.
Table 2 includes a residual term in the tropospheric ozone budget as a balance between the chemical production, chemical loss, deposition, and (negligible) accumulation terms. This residual term of 341–380 Tg a−1 is expected to represent stratosphere–troposphere exchange (STE), which is not explicitly diagnosed in CESM2, and falls within the range of literature values listed in Table 2. The residual changes slightly in the sensitivity simulations of Table 3 in a way that is consistent with the tropospheric ozone burden, as increasing tropospheric ozone decreases STE while increasing deposition.
Fritz et al. (2022) previously found tropospheric ozone in GEOS-Chem to be 30 % lower than CAM-chem in the extratropics because of halogen chemistry, but iodine emissions in that simulation were 100-fold too high because the interface to HEMCO erroneously passed 2 m temperature instead of sea surface temperature to the iodine emissions module (Sect. 2.2). With corrected iodine emissions, we find only a 4 % decrease of tropospheric ozone in GEOS-Chem due to tropospheric halogen chemistry. The magnitude of the effect of halogen chemistry on ozone is uncertain, ranging from 10 % to 19 % in previous implementations in offline GEOS-Chem (Sherwen et al., 2016b; Wang et al., 2021) and CAM-chem (Saiz-Lopez et al., 2012), but all models report lower-tropospheric ozone as a result. We find that halogen chemistry has a smaller effect on tropospheric ozone in GEOS-Chem within CESM2 than offline due to weaker wind speeds and lower sea surface temperatures in CESM2, resulting in weaker sea salt and gaseous iodine emissions.
Figure 5 compares annual mean ozone vertical profiles simulated by GEOS-Chem and CAM-chem to ozonesonde observations for 2016 from the World Ozone and Ultraviolet Radiation Data Centre (WOUDC), averaged across nine regions following Tilmes et al. (2012). Figure 6 compares April 2016 monthly mean surface ozone simulated by GEOS-Chem and CAM-chem to background surface ozone observations from 10 sites of the NOAA ESRL Global Monitoring Division (McClure-Begley et al., 2013) and 5 remote sites in China under the World Meteorological Organization Global Atmosphere Watch Programme. Both models match the observations well and are within 5–10 ppb of each other. GEOS-Chem has lower ozone at high northern latitudes (up to 10 ppb at the surface) because of halogen chemistry.
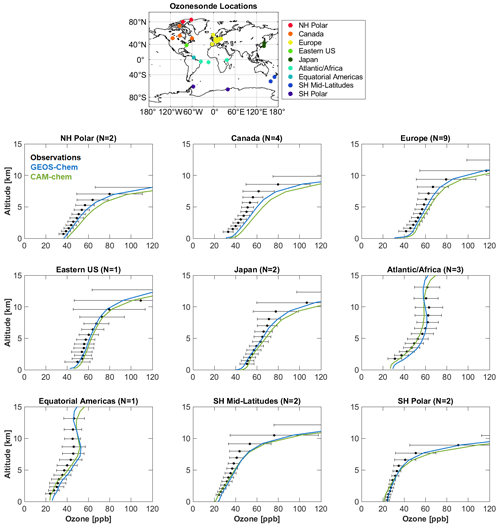
Figure 5Comparison of annual mean ozone vertical profiles to 2016 ozonesonde observations simulated by GEOS-Chem and CAM-chem. The regions average a number N of observing sites as given by Tilmes et al. (2012). Horizontal bars are standard deviations of the means across the N sites.
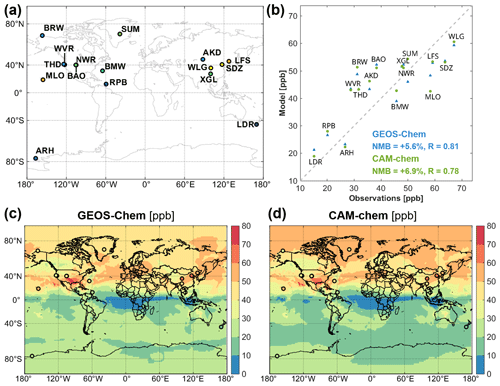
Figure 6Monthly mean surface ozone concentrations in GEOS-Chem and CAM-chem within CESM2 for April 2016 and comparison to surface observations. References for the observations are given in the text.
Figure 7 shows tropospheric profiles of OH, NO, and CO simulated by GEOS-Chem and CAM-chem over the oceans in comparison to observations from the ATom-1 campaign. Model profiles compared to aircraft observations are computed at model runtime by sampling the two closest time steps and four closest grid boxes to the time-varying flight track data and then interpolated to the aircraft time and location. The 0.9°×1.25° resolution of the simulation is well adapted to the scales sampled by ATom. Both models generally agree with OH observations within uncertainty. Both models fit NO observations within a factor of 2 in the Northern Hemisphere but have large underestimates in the Southern Hemisphere. This underestimate is a known model issue in previous offline GEOS-Chem simulations (Travis et al., 2020) and is not correctable by nitrate photolysis because particulate nitrate concentrations in the Southern Hemisphere are low (Shah et al., 2023). Observations show a NO increase in the upper troposphere of the Southern Hemisphere that is captured by CAM-chem but not GEOS-Chem. Previous work has shown that offline GEOS-Chem simulations capture this increase of NO in the upper troposphere (Shah et al., 2023). A sensitivity GEOS-Chem simulation without tropospheric halogen chemistry, as shown in Fig. 6, also captures this increase. The difference is due to CESM2 not accounting for the scavenging in convective updrafts of soluble halogen gases such as HBr and HOBr. This increases the formation of stable halogen nitrates in the upper troposphere where thermolysis and hydrolysis are slow (Wang et al., 2021).
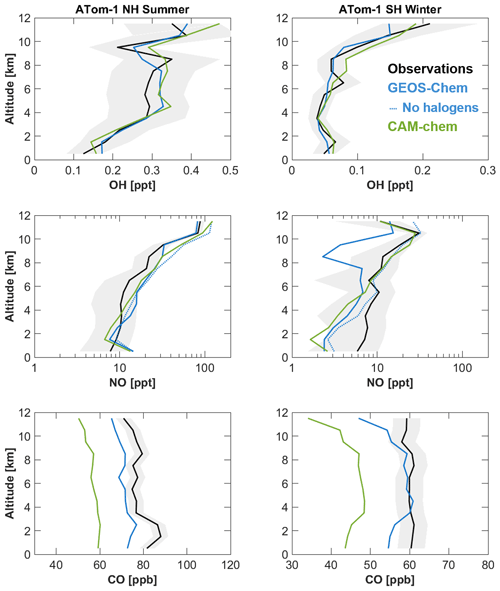
Figure 7Median vertical profiles of OH, NO, and CO concentrations from the ATom-1 field campaign (July–August 2016) and from the GEOS-Chem and CAM-chem models within CESM2. Observations are separated between Northern Hemisphere and Southern Hemisphere (NH and SH), filtered to remove influences from biomass burning (CH3CN>200 ppt; Travis et al., 2020) and binned in 1 km intervals. Shaded areas correspond to the measurement accuracy.
Both models underestimate CO in the Northern Hemisphere, which is a known issue attributed to excessive OH (Gaubert et al., 2020) or missing emissions of CO and its precursors (Park et al., 2021; Tang et al., 2023). CAM-chem has 10–20 ppb lower CO globally compared to GEOS-Chem that is likely driven by differences in OH. In the Southern Hemisphere the difference is driven by improvements in isoprene oxidation in GEOS-Chem by Bates and Jacob (2019), which recycles OH through H-shift isomerization of isoprene–hydroxy–peroxy radicals under low-NO conditions, seen in observations by Wells et al. (2020). This leads to faster in situ isoprene oxidation and a higher CO yield. This is not included in CAM-chem's default MOZART-TS1 mechanism used in this work but is included in the updated MOZART-TS2 mechanism (Schwantes et al., 2020, 2022).
We use comparison to observations from the KORUS-AQ campaign (1 May to 10 June 2016) over the Seoul Metropolitan Area (SMA; 37–37.6° N, 126.6–127.7° E) as illustrative of a polluted atmosphere. Figure 8 shows median concentration profiles of oxidants and related species. Observations are compared to GEOS-Chem and CAM-chem sampled along the flight tracks and to GEOS-Chem sensitivity simulations without particulate nitrate photolysis and without the nitrate correction applied in CESM2 for lack of scavenging in convective updrafts. Also shown in the figure are vertical profiles from an offline nested GEOS-Chem simulation at 0.25°×0.3125° resolution reported by Yang et al. (2023). We see that GEOS-Chem without particulate nitrate photolysis, as reported in the model intercomparison of Park et al. (2021), is much lower than observed and 20 ppb lower than the standard model. CAM-chem was the only model to successfully reproduce observed ozone in the Park et al. (2021) intercomparison, and this was attributed to its stratospheric ozone influx, but here GEOS-Chem uses the same dynamics and hence the same stratospheric influx. The success of CAM-chem in KORUS-AQ reflects instead its non-accounting of tropospheric halogen chemistry as a sink of ozone, which in GEOS-Chem needs to be compensated by particulate nitrate photolysis. Both models are too high compared to observations above 4 km altitude, which is due at least in GEOS-Chem to excessive particulate nitrate resulting from inadequate convective scavenging.
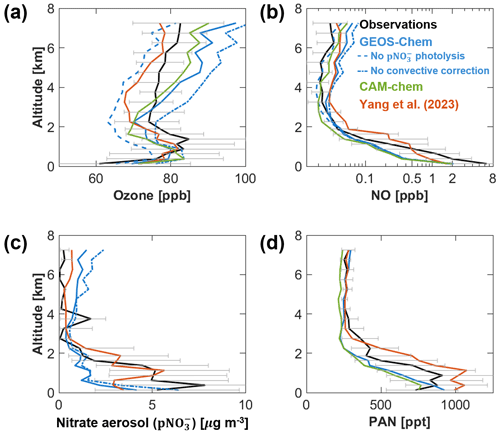
Figure 8Median tropospheric vertical profiles of species concentrations over the Seoul Metropolitan Area (SMA; 37–37.6° N, 126.6–127.7° E) during the KORUS-AQ aircraft campaign in May–June 2016. Observations are compared to GEOS-Chem and CAM-chem simulations within CESM2 and to the offline GEOS-Chem simulation reported by Yang et al. (2023). Results from GEOS-Chem sensitivity simulations with no particulate nitrate photolysis and no correction for scavenging of nitrate in wet convective updrafts are also shown. The vertical profiles are constructed by binning data into 0.25 km intervals below 2 km altitude and 0.5 km intervals above 2 km altitude. Horizontal bars represent the interquartile range of the observations in the given vertical bin.
Particulate nitrate photolysis increases free-tropospheric NOx and ozone production, but this depends on the nitrate concentration. CAM-chem does not simulate nitrate. Because GEOS-Chem nitrate is not removed in convective updrafts in the CESM2 environment, our standard implementation within CESM2 corrects nitrate using the same photolytic sink that CAM-chem applies for SOA with a rate of and no products (Hodzic et al., 2015, 2016) to avoid buildup in the upper troposphere. But that correction is apparently insufficient because particulate nitrate is overestimated relative to observations above 4 km altitude, an overestimate not seen in the offline GEOS-Chem simulation of Yang et al. (2023) and which would be worse if we did not apply the correction (Fig. 8b and c). A solution would be to replace CESM convective transport with the GEOS-Chem offline convective transport and scavenging module using archived CESM convective mass fluxes, and this has been done before when coupling GEOS-Chem to the GEOS and Beijing Climate Center (BCC) ESMs, which had the same problem of not scavenging water-soluble species in convective updrafts (Yu et al., 2018; Lu et al., 2020). A more comprehensive solution would be to include scavenging of water-soluble species in the CESM2 convection scheme. This is implemented for MAM aerosols (Wang et al., 2013) but not for gas-phase species or aerosols only represented in GEOS-Chem, including nitrate.
The simulations of particulate nitrate and peroxyacetyl nitrate (PAN) within CESM show a sharp drop of concentrations with altitude above the surface, whereas the observations and the offline GEOS-Chem simulation of Yang et al. (2023) show a mixed-layer structure extending to 1–2 km altitude. This likely reflects a bias in the CESM2 boundary layer mixing scheme that would need to be investigated further. Boundary layer mixing in the offline GEOS-Chem model is a standard non-local scheme from Lin and McElroy (2010). The PAN simulations in GEOS-Chem and CAM-chem otherwise agree closely, indicating similar production from VOC chemistry, and are lower than the offline GEOS-Chem simulation, which includes additional emissions of volatile chemical products (VCPs) as a source of acetaldehyde leading to PAN production (Yang et al., 2023).
GEOS-Chem has been implemented as an atmospheric chemistry module in the NCAR Community Earth System Model (CESM2) to serve as alternative to CAM-chem and contribute to the MUSICA vision of plug-and-play modularization of atmospheric chemistry within CESM (Pfister et al., 2020). Here we presented an intercomparison and evaluation with observations of tropospheric oxidant simulations with these two modules. The intercomparison covered the full year of 2016, allowing evaluation with the ATom-1 aircraft campaign over the remote Pacific and Atlantic and the KORUS-AQ aircraft campaign over the Seoul Metropolitan Area (SMA). Both GEOS-Chem and CAM-chem used the same emissions processed through HEMCO (Lin et al., 2021) and the same coupling to other CESM2 modules.
GEOS-Chem uses the Fast-JX scheme for photolysis, while CAM-chem uses a lookup table based on TUV. Both schemes agree to within 10 % when compared to and JO1D photolysis frequencies observations in ATom-1, but observations in KORUS-AQ show that CAM-chem overestimates JO1D, while GEOS-Chem does not. One major difference is that TUV does not account for extinction by aerosols, while Fast-JX does. We implemented Fast-JX in CAM-chem and find that it resolves most of the photolysis differences with GEOS-Chem.
Global tropospheric ozone budget terms in GEOS-Chem and CAM-chem agree within 10 %, compared to a much wider spread in the literature and due in part to canceling effects. Differences between the two models are mostly driven by aerosol nitrate photolysis, N2O5 uptake in clouds, and tropospheric halogen chemistry, all of which are included in GEOS-Chem but not in CAM-chem. Aerosol nitrate photolysis in GEOS-Chem produces NOx and enhances ozone production, compensating for losses from N2O5 uptake in clouds and tropospheric halogen chemistry. Annual mean ozone concentrations agree within 5 ppb between GEOS-Chem and CAM-chem almost everywhere. Lower ozone deposition to the oceans in GEOS-Chem results in higher surface ozone at southern midlatitudes. Tropospheric halogen chemistry results in lower ozone at high northern latitudes. Tropospheric NOx in GEOS-Chem is higher than CAM-chem in the tropics due to nitrate photolysis and lower at high latitudes due to N2O5 uptake by cloud and formation of halogen nitrates. The global mean tropospheric OH concentration is identical between the two models, but there are large differences over the continents driven by photolysis and by isoprene chemistry.
Both GEOS-Chem and CAM-chem show good agreement with annual mean ozonesonde observations and background surface ozone observations over the range of latitudes. Comparison to ATom-1 observations in July–August 2016 shows good agreement for OH concentrations in both the Northern Hemisphere and Southern Hemisphere (NH and SH) within the measurement accuracy and for NOx in the NH, but NOx in the SH is underestimated. GEOS-Chem shows a depletion of NOx in the SH upper troposphere that is due to formation of halogen nitrates and is not seen in the observations. However, the offline GEOS-Chem simulation does not show this problem. One issue in CESM2 is the lack of scavenging of water-soluble species, including halogen radical reservoirs in convective updrafts. Both GEOS-Chem and CAM-chem underestimate CO in the NH, but CAM-chem is consistently lower than GEOS-Chem due to higher OH in the NH and suppression of CO production from isoprene oxidation in the SH.
Comparison with KORUS-AQ aircraft observations allowed model evaluation for polluted conditions. Ozone concentrations in GEOS-Chem and CAM-chem are higher than observed above 4 km altitude, which in GEOS-Chem is due to excessive particulate nitrate photolyzing to produce excessive NOx. Lack of scavenging of water-soluble species in convective updrafts is a major shortcoming in CESM2 that hinders proper representation of nitrate in the upper troposphere. Simulation of peroxyacetyl nitrate (PAN) in KORUS-AQ shows good agreement between GEOS-Chem and CAM-chem and with observations, indicating consistency in the VOC chemistry producing PAN. However, the decreases of PAN and particulate nitrate mixing ratios with altitude in the lower 2 km are much sharper than observed or simulated by the offline GEOS-Chem model, implying insufficient boundary layer mixing in CESM2.
Overall, we have shown that GEOS-Chem provides a high-quality simulation of tropospheric oxidant chemistry in CESM2 and can contribute modules for alternative representations of atmospheric chemistry to serve the MUSICA vision.
A fork of an alpha version (cam6_3_095) of the Community Atmosphere Model (CAM) including GEOS-Chem is available at https://github.com/geoschem/CAM (Fritz et al., 2023) and is used in this work. CAM-chem using HEMCO for emissions is implemented in the mainline CAM code as of cam6_3_118 (https://github.com/ESCOMP/CAM/tree/cam6_3_118, The Community Atmosphere Model Developers, 2023a). GEOS-Chem within CESM2 is implemented in the mainline CAM code as of cam6_3_147 (https://github.com/escomp/cam/tree/cam6_3_147, The Community Atmosphere Model Developers, 2023b).
LKE, SDE, and DJJ conceived of the project, acquired funding, and supervised the work. HL, EWL, XF, SDE, and TMF performed software development. HL, LKE, LHY, XF, RD, SZ, YT, MMK, NKC, and DJJ analyzed the model results. SZ prepared the KORUSv5 emission inventory for input into HEMCO and CESM2. HL performed the visualization and preparation of the original draft. All authors contributed to review and editing of the manuscript.
The contact author has declared that none of the authors has any competing interests.
Publisher's note: Copernicus Publications remains neutral with regard to jurisdictional claims made in the text, published maps, institutional affiliations, or any other geographical representation in this paper. While Copernicus Publications makes every effort to include appropriate place names, the final responsibility lies with the authors.
This work was supported by the Atmospheric Chemistry Program of the US National Science Foundation. This material is based upon work supported by the National Center for Atmospheric Research, which is a major facility sponsored by the National Science Foundation under cooperative agreement no. 1852977. We would like to acknowledge high-performance computing support from Cheyenne: HPE/SGI ICE XA System (https://doi.org/10.5065/D6RX99HX; Computational and Information Systems Laboratory, 2019) and Derecho: HPE Cray EX System (https://doi.org/10.5065/qx9a-pg09, Computational and Information Systems Laboratory, 2024), provided by NCAR's Computational and Information Systems Laboratory (CISL) and sponsored by the National Science Foundation.
This research has been supported by the National Science Foundation (grant no. AGS-2228359).
This paper was edited by Suvarna Fadnavis and reviewed by three anonymous referees.
Alexander, B., Park, R. J., Jacob, D. J., Li, Q. B., Yantosca, R. M., Savarino, J., Lee, C. C. W., and Thiemens, M. H.: Sulfate formation in sea-salt aerosols: Constraints from oxygen isotopes, J. Geophys. Res.-Atmos., 110, D10307, https://doi.org/10.1029/2004JD005659, 2005.
Amos, H. M., Jacob, D. J., Holmes, C. D., Fisher, J. A., Wang, Q., Yantosca, R. M., Corbitt, E. S., Galarneau, E., Rutter, A. P., Gustin, M. S., Steffen, A., Schauer, J. J., Graydon, J. A., Louis, V. L. St., Talbot, R. W., Edgerton, E. S., Zhang, Y., and Sunderland, E. M.: Gas-particle partitioning of atmospheric Hg(II) and its effect on global mercury deposition, Atmos. Chem. Phys., 12, 591–603, https://doi.org/10.5194/acp-12-591-2012, 2012.
Balkanski, Y. J., Jacob, D. J., Gardner, G. M., Graustein, W. C., and Turekian, K. K.: Transport and residence times of tropospheric aerosols inferred from a global three-dimensional simulation of Pb-210, J. Geophys. Res., 98, 20573–20586, https://doi.org/10.1029/93JD02456, 1993.
Bates, K. H. and Jacob, D. J.: A new model mechanism for atmospheric oxidation of isoprene: global effects on oxidants, nitrogen oxides, organic products, and secondary organic aerosol, Atmos. Chem. Phys., 19, 9613–9640, https://doi.org/10.5194/acp-19-9613-2019, 2019.
Bates, K. H., Jacob, D. J., Li, K., Ivatt, P. D., Evans, M. J., Yan, Y., and Lin, J.: Development and evaluation of a new compact mechanism for aromatic oxidation in atmospheric models, Atmos. Chem. Phys., 21, 18351–18374, https://doi.org/10.5194/acp-21-18351-2021, 2021.
Bey, I., Jacob, D. J., Yantosca, R. M., Logan, J. A., Field, B. D., Fiore, A. M., Li, Q., Liu, H. Y., Mickley, L. J., and Schultz, M. G.: Global modeling of tropospheric chemistry with assimilated meteorology: Model description and evaluation, J. Geophys. Res., 106, 23073–23095, https://doi.org/10.1029/2001JD000807, 2001.
Bian, H. and Prather, M. J.: Fast-J2: Accurate Simulation of Stratospheric Photolysis in Global Chemical Models, J. Atmos. Chem., 41, 281–296, https://doi.org/10.1023/A:1014980619462, 2002.
Brasseur, G. P. and Jacob, D. J.: Modeling of Atmospheric Chemistry, Cambridge University Press, Cambridge, UK, https://doi.org/10.1017/9781316544754, 2017.
Burkholder, J. B., Sander, S. P., Abbatt, J., Barker, J. R., Cappa, C., Crounse, J. D., Dibble, T. S., Huie, R. E., Kolb, C. E., Kurylo, M. J., Orkin, V. L., Percival, C. J., Wilmouth, D. M., and Wine, P. H.: Chemical kinetics and photochemical data for use in atmospheric studies, Evaluation no. 19, Tech. Rep. JPL Publication 19-5, Jet Propulsion Laboratory, Pasadena, CA, https://jpldataeval.jpl.nasa.gov/pdf/NASA-JPL Evaluation 19-5.pdf (last access: 31 July 2024), 2020.
Computational and Information Systems Laboratory: Cheyenne: HPE/SGI ICE XA System (University Community Computing), National Center for Atmospheric Research, Boulder, CO, https://doi.org/10.5065/D6RX99HX, 2019.
Computational and Information Systems Laboratory: Derecho: HPE Cray EX System (University Community Computing), National Center for Atmospheric Research, Boulder, CO, https://doi.org/10.5065/qx9a-pg09, 2024.
Crawford, J. H., Ahn, J.-Y., Al-Saadi, J., Chang, L., Emmons, L. K., Kim, J., Lee, G., Park, J.-H., Park, R. J., Woo, J. H., Song, C.-K., Hong, J.-H., Hong, Y.-D., Lefer, B. L., Lee, M., Lee, T., Kim, S., Min, K.-E., Yum, S. S., Shin, H. J., Kim, Y.-W., Choi, J.-S., Park, J.-S., Szykman, J. J., Long, R. W., Jordan, C. E., Simpson, I. J., Fried, A., Dibb, J. E., Cho, S., and Kim, Y. P.: The Korea–United States Air Quality (KORUS-AQ) field study, Elem. Sci. Anth., 9, 00163, https://doi.org/10.1525/elementa.2020.00163, 2021.
Danabasoglu, G., Lamarque, J.-F., Bacmeister, J., Bailey, D. A., DuVivier, A. K., Edwards, J., Emmons, L. K., Fasullo, J., Garcia, R., Gettelman, A., Hannay, C., Holland, M. M., Large, W. G., Lauritzen, P. H., Lawrence, D. M., Lenaerts, J. T. M., Lindsay, K., Lipscomb, W. H., Mills, M. J., Neale, R., Oleson, K. W., Otto-Bliesner, B., Philips, A. S., Sacks, W., Tilmes, S., van Kampenhout, L., Vertenstein, M., Bertini, A., Dennis, J., Deser, C., Fischer, C., Fox-Kemper, B., Kay, J. E., Kinnison, D., Kushner, P. J., Larson, V. E., Long, M. C., Mickelson, S., Moore, J. K., Nienhouse, E., Polvani, L., Rasch, P. J., and Strand, W. G.: The Community Earth System Model Version 2 (CESM2), J. Adv. Model. Earth Sy., 12, e2019MS001916, https://doi.org/10.1029/2019MS001916, 2020.
Eastham, S. D., Weisenstein, D. K., and Barrett, S. R. H.: Development and evaluation of the unified tropospheric–stratospheric chemistry extension (UCX) for the global chemistry-transport model GEOS-Chem, Atmos. Environ., 89, 52–63, https://doi.org/10.1016/j.atmosenv.2014.02.001, 2014.
Emmons, L. K., Schwantes, R. H., Orlando, J. J., Tyndall, G., Kinnison, D., Lamarque, J.-F., Marsh, D., Mills, M. J., Tilmes, S., Bardeen, C., Buchholz, R. R., Conley, A., Gettelman, A., Garcia, R., Simpson, I., Blake, D. R., Meinardi, S., and Pétron, G.: The chemistry mechanism in the community earth system model version 2 (CESM2), J. Adv. Model. Earth Syst., 12, e2019MS001882, https://doi.org/10.1029/2019MS001882, 2020.
Fairlie, T. D., Jacob, D. J., Dibb, J. E., Alexander, B., Avery, M. A., van Donkelaar, A., and Zhang, L.: Impact of mineral dust on nitrate, sulfate, and ozone in transpacific Asian pollution plumes, Atmos. Chem. Phys., 10, 3999–4012, https://doi.org/10.5194/acp-10-3999-2010, 2010.
Feng, X., Lin, H., Fu, T.-M., Sulprizio, M. P., Zhuang, J., Jacob, D. J., Tian, H., Ma, Y., Zhang, L., Wang, X., Chen, Q., and Han, Z.: WRF-GC (v2.0): online two-way coupling of WRF (v3.9.1.1) and GEOS-Chem (v12.7.2) for modeling regional atmospheric chemistry–meteorology interactions, Geosci. Model Dev., 14, 3741–3768, https://doi.org/10.5194/gmd-14-3741-2021, 2021.
Fritz, T. M., Eastham, S. D., Emmons, L. K., Lin, H., Lundgren, E. W., Goldhaber, S., Barrett, S. R. H., and Jacob, D. J.: Implementation and evaluation of the GEOS-Chem chemistry module version 13.1.2 within the Community Earth System Model v2.1, Geosci. Model Dev., 15, 8669–8704, https://doi.org/10.5194/gmd-15-8669-2022, 2022.
Gaubert, B., Emmons, L. K., Raeder, K., Tilmes, S., Miyazaki, K., Arellano Jr., A. F., Elguindi, N., Granier, C., Tang, W., Barré, J., Worden, H. M., Buchholz, R. R., Edwards, D. P., Franke, P., Anderson, J. L., Saunois, M., Schroeder, J., Woo, J.-H., Simpson, I. J., Blake, D. R., Meinardi, S., Wennberg, P. O., Crounse, J., Teng, A., Kim, M., Dickerson, R. R., He, H., Ren, X., Pusede, S. E., and Diskin, G. S.: Correcting model biases of CO in East Asia: impact on oxidant distributions during KORUS-AQ, Atmos. Chem. Phys., 20, 14617–14647, https://doi.org/10.5194/acp-20-14617-2020, 2020.
Fritz, T. M., Lin, H. P., Lundgren, E. W., Eastham, S. D., Emmons, L. K., Goldhaber, S., Feng, X., Barrett, S. R. H., and Jacob, D. J.: GEOS-Chem chemistry module version 14.1.2 within the Community Earth System Model version 2 [code], GitHub [code], https://github.com/geoschem/CAM (last access: 31 July 2024), 2023.
Guenther, A. B., Jiang, X., Heald, C. L., Sakulyanontvittaya, T., Duhl, T., Emmons, L. K., and Wang, X.: The Model of Emissions of Gases and Aerosols from Nature version 2.1 (MEGAN2.1): an extended and updated framework for modeling biogenic emissions, Geosci. Model Dev., 5, 1471–1492, https://doi.org/10.5194/gmd-5-1471-2012, 2012.
Hall, S. R., Ullmann, K., Prather, M. J., Flynn, C. M., Murray, L. T., Fiore, A. M., Correa, G., Strode, S. A., Steenrod, S. D., Lamarque, J.-F., Guth, J., Josse, B., Flemming, J., Huijnen, V., Abraham, N. L., and Archibald, A. T.: Cloud impacts on photochemistry: building a climatology of photolysis rates from the Atmospheric Tomography mission, Atmos. Chem. Phys., 18, 16809–16828, https://doi.org/10.5194/acp-18-16809-2018, 2018.
Hodzic, A., Madronich, S., Kasibhatla, P. S., Tyndall, G., Aumont, B., Jimenez, J. L., Lee-Taylor, J., and Orlando, J.: Organic photolysis reactions in tropospheric aerosols: effect on secondary organic aerosol formation and lifetime, Atmos. Chem. Phys., 15, 9253–9269, https://doi.org/10.5194/acp-15-9253-2015, 2015.
Hodzic, A., Kasibhatla, P. S., Jo, D. S., Cappa, C. D., Jimenez, J. L., Madronich, S., and Park, R. J.: Rethinking the global secondary organic aerosol (SOA) budget: stronger production, faster removal, shorter lifetime, Atmos. Chem. Phys., 16, 7917–7941, https://doi.org/10.5194/acp-16-7917-2016, 2016.
Holmes, C. D., Bertram, T. H., Confer, K. L., Graham, K. A., Ronan, A. C., Wirks, C. K., and Shah, V.: The Role of Clouds in the Tropospheric NOx Cycle: A New Modeling Approach for Cloud Chemistry and Its Global Implications, Geophys. Res. Lett., 46, 4980–4990, https://doi.org/10.1029/2019GL081990, 2019.
Hu, L., Jacob, D. J., Liu, X., Zhang, Y., Kim, P. S., Sulprizio, M. P., and Yantosca, R. M.: Global budget of tropospheric ozone: Evaluating recent model advances with satellite (OMI), aircraft (IAGOS), and ozonesonde observations, Atmos. Environ., 167, 323–334, https://doi.org/10.1016/j.atmosenv.2017.08.036, 2017.
Hu, L., Keller, C. A., Long, M. S., Sherwen, T., Auer, B., Da Silva, A., Nielsen, J. E., Pawson, S., Thompson, M. A., Trayanov, A. L., Travis, K. R., Grange, S. K., Evans, M. J., and Jacob, D. J.: Global simulation of tropospheric chemistry at 12.5 km resolution: performance and evaluation of the GEOS-Chem chemical module (v10-1) within the NASA GEOS Earth system model (GEOS-5 ESM), Geosci. Model Dev., 11, 4603–4620, https://doi.org/10.5194/gmd-11-4603-2018, 2018.
Hudman, R. C., Moore, N. E., Mebust, A. K., Martin, R. V., Russell, A. R., Valin, L. C., and Cohen, R. C.: Steps towards a mechanistic model of global soil nitric oxide emissions: implementation and space based-constraints, Atmos. Chem. Phys., 12, 7779–7795, https://doi.org/10.5194/acp-12-7779-2012, 2012.
Keller, C. A., Long, M. S., Yantosca, R. M., Da Silva, A. M., Pawson, S., and Jacob, D. J.: HEMCO v1.0: a versatile, ESMF-compliant component for calculating emissions in atmospheric models, Geosci. Model Dev., 7, 1409–1417, https://doi.org/10.5194/gmd-7-1409-2014, 2014.
Keller, C. A., Knowland, K. E., Duncan, B. N., Liu, J., Anderson, D. C., Das, S., Lucchesi, R. A., Lundgren, E. W., Nicely, J. M., Nielsen, E., Ott, L. E., Saunders, E., Strode, S. A., Wales, P. A., Jacob, D. J., and Pawson, S.: Description of the NASA GEOS Composition Forecast Modeling System GEOS-CF v1.0, J. Adv. Model. Earth Sy., 13, e2020MS002413, https://doi.org/10.1029/2020MS002413, 2021.
Kodros, J. K. and Pierce, J. R.: Important global and regional differences in aerosol cloud-albedo effect estimates between simulations with and without prognostic aerosol microphysics, J. Geophys. Res.-Atmos., 122, 4003–4018, https://doi.org/10.1002/2016JD025886, 2017.
Kinnison, D. E., Brasseur, G. P., and Walters, S.: Sensitivity of chemical tracers to meteorological parameters in the MOZART-3 chemical transport model, J. Geophys. Res.-Atmos., 112, D15, https://doi.org/10.1029/2003JD004473, 2007.
Lamarque, J.-F., Emmons, L. K., Hess, P. G., Kinnison, D. E., Tilmes, S., Vitt, F., Heald, C. L., Holland, E. A., Lauritzen, P. H., Neu, J., Orlando, J. J., Rasch, P. J., and Tyndall, G. K.: CAM-chem: description and evaluation of interactive atmospheric chemistry in the Community Earth System Model, Geosci. Model Dev., 5, 369–411, https://doi.org/10.5194/gmd-5-369-2012, 2012.
Lin, H., Feng, X., Fu, T.-M., Tian, H., Ma, Y., Zhang, L., Jacob, D. J., Yantosca, R. M., Sulprizio, M. P., Lundgren, E. W., Zhuang, J., Zhang, Q., Lu, X., Zhang, L., Shen, L., Guo, J., Eastham, S. D., and Keller, C. A.: WRF-GC (v1.0): online coupling of WRF (v3.9.1.1) and GEOS-Chem (v12.2.1) for regional atmospheric chemistry modeling – Part 1: Description of the one-way model, Geosci. Model Dev., 13, 3241–3265, https://doi.org/10.5194/gmd-13-3241-2020, 2020.
Lin, H., Jacob, D. J., Lundgren, E. W., Sulprizio, M. P., Keller, C. A., Fritz, T. M., Eastham, S. D., Emmons, L. K., Campbell, P. C., Baker, B., Saylor, R. D., and Montuoro, R.: Harmonized Emissions Component (HEMCO) 3.0 as a versatile emissions component for atmospheric models: application in the GEOS-Chem, NASA GEOS, WRF-GC, CESM2, NOAA GEFS-Aerosol, and NOAA UFS models, Geosci. Model Dev., 14, 5487–5506, https://doi.org/10.5194/gmd-14-5487-2021, 2021.
Lin, J.-T. and McElroy, M. B.: Impacts of boundary layer mixing on pollutant vertical profiles in the lower troposphere: Implications to satellite remote sensing, Atmos. Environ., 44, 1726–1739, 2010.
Liu, H., Jacob, D. J., Bey, I., and Yantosca, R. M.: Constraints from 210Pb and 7Be on wet deposition and transport in a global three-dimensional chemical tracer model driven by assimilated meteorological fields, J. Geophys. Res., 106, 12109–12128, https://doi.org/10.1029/2000JD900839, 2001.
Liu, X., Ma, P.-L., Wang, H., Tilmes, S., Singh, B., Easter, R. C., Ghan, S. J., and Rasch, P. J.: Description and evaluation of a new four-mode version of the Modal Aerosol Module (MAM4) within version 5.3 of the Community Atmosphere Model, Geosci. Model Dev., 9, 505–522, https://doi.org/10.5194/gmd-9-505-2016, 2016.
Long, M. S., Yantosca, R., Nielsen, J. E., Keller, C. A., da Silva, A., Sulprizio, M. P., Pawson, S., and Jacob, D. J.: Development of a grid-independent GEOS-Chem chemical transport model (v9-02) as an atmospheric chemistry module for Earth system models, Geosci. Model Dev., 8, 595–602, https://doi.org/10.5194/gmd-8-595-2015, 2015.
Lu, X., Zhang, L., Wu, T., Long, M. S., Wang, J., Jacob, D. J., Zhang, F., Zhang, J., Eastham, S. D., Hu, L., Zhu, L., Liu, X., and Wei, M.: Development of the global atmospheric chemistry general circulation model BCC-GEOS-Chem v1.0: model description and evaluation, Geosci. Model Dev., 13, 3817–3838, https://doi.org/10.5194/gmd-13-3817-2020, 2020.
Mahowald, N. M., Lamarque, J.-F., Tie, X. X., and Wolff, E.: Sea-salt aerosol response to climate change: Last Glacial Maximum, preindustrial, and doubled carbon dioxide climates, J. Geophys. Res.-Atmos., 111, D05303, https://doi.org/10.1029/2005JD006459, 2006a.
Mahowald, N. M., Muhs, D. R., Levis, S., Rasch, P. J., Yoshioka, M., Zender, C. S., and Luo, C.: Change in atmospheric mineral aerosols in response to climate: Last glacial period, preindustrial, modern, and doubled carbon dioxide climates, J. Geophys. Res.-Atmos., 111, D10202, https://doi.org/10.1029/2005JD006653, 2006b.
McClure-Begley, A., Petropavlovskikh, I., Oltmans, S., and NOAA ESRL: Earth System Research Laboratory Ozone Water Vapor Group Surface Ozone Measurements, Version 1, NOAA National Centers for Environmental Information [data set], https://doi.org/10.7289/V57P8WBF, 2023.
McDuffie, E. E., Martin, R. V., Spadaro, J. V., Burnett, R., Smith, S. J., O'Rourke, P., Hammer, M. S., van Donkelaar, A., Bindle, L., Shah, V., Jaeglé, L., Luo, G., Yu, F., Adeniran, J. A., Lin, J., and Brauer, M.: Source sector and fuel contributions to ambient PM2.5 and attributable mortality across multiple spatial scales, Nat. Commun., 12, 3594, https://doi.org/10.1038/s41467-021-23853-y, 2021.
Moch, J. M., Mickley, L. J., Keller, C. A., Bian, H., Lundgren, E. W., Zhai, S., and Jacob, D. J.: Aerosol-Radiation Interactions in China in Winter: Competing Effects of Reduced Shortwave Radiation and Cloud-Snowfall-Albedo Feedbacks Under Rapidly Changing Emissions, J. Geophys. Res.-Atmos., 127, e2021JD035442, https://doi.org/10.1029/2021JD035442, 2022.
Monks, P. S., Archibald, A. T., Colette, A., Cooper, O., Coyle, M., Derwent, R., Fowler, D., Granier, C., Law, K. S., Mills, G. E., Stevenson, D. S., Tarasova, O., Thouret, V., von Schneidemesser, E., Sommariva, R., Wild, O., and Williams, M. L.: Tropospheric ozone and its precursors from the urban to the global scale from air quality to short-lived climate forcer, Atmos. Chem. Phys., 15, 8889–8973, https://doi.org/10.5194/acp-15-8889-2015, 2015.
Murray, L. T., Jacob, D. J., Logan, J. A., Hudman, R. C., and Koshak, W. J.: Optimized regional and interannual variability of lightning in a global chemical transport model constrained by LIS/OTD satellite data, J. Geophys. Res.-Atmos., 117, 2012JD017934, https://doi.org/10.1029/2012JD017934, 2012.
Naik, V., Voulgarakis, A., Fiore, A. M., Horowitz, L. W., Lamarque, J.-F., Lin, M., Prather, M. J., Young, P. J., Bergmann, D., Cameron-Smith, P. J., Cionni, I., Collins, W. J., Dalsøren, S. B., Doherty, R., Eyring, V., Faluvegi, G., Folberth, G. A., Josse, B., Lee, Y. H., MacKenzie, I. A., Nagashima, T., van Noije, T. P. C., Plummer, D. A., Righi, M., Rumbold, S. T., Skeie, R., Shindell, D. T., Stevenson, D. S., Strode, S., Sudo, K., Szopa, S., and Zeng, G.: Preindustrial to present-day changes in tropospheric hydroxyl radical and methane lifetime from the Atmospheric Chemistry and Climate Model Intercomparison Project (ACCMIP), Atmos. Chem. Phys., 13, 5277–5298, https://doi.org/10.5194/acp-13-5277-2013, 2013.
Neu, J. L. and Prather, M. J.: Toward a more physical representation of precipitation scavenging in global chemistry models: cloud overlap and ice physics and their impact on tropospheric ozone, Atmos. Chem. Phys., 12, 3289–3310, https://doi.org/10.5194/acp-12-3289-2012, 2012.
Pai, S. J., Heald, C. L., Pierce, J. R., Farina, S. C., Marais, E. A., Jimenez, J. L., Campuzano-Jost, P., Nault, B. A., Middlebrook, A. M., Coe, H., Shilling, J. E., Bahreini, R., Dingle, J. H., and Vu, K.: An evaluation of global organic aerosol schemes using airborne observations, Atmos. Chem. Phys., 20, 2637–2665, https://doi.org/10.5194/acp-20-2637-2020, 2020.
Park, R., Jacob, D. J., Field, B. D., Yantosca, R. M., and Chin, M.: Natural and transboundary pollution influences on sulfate-nitrate-ammonium aerosols in the United States: Implications for policy, J. Geophys. Res.-Atmos., 109, 2003JD004474, https://doi.org/10.1029/2003JD004473, 2004.
Park, R. J., Oak, Y. J., Emmons, L. K., Kim, C.-H., Pfister, G. G., Carmichael, G. R., Saide, P. E., Cho, S.-Y., Kim, S., Woo, J.-H., Crawford, J. H., Gaubert, B., Lee, H.-J., Park, S.-Y., Jo, Y.-J., Gao, M., Tang, B., Stanier, C. O., Shin, S. S., Park, H. Y., Bae, C., and Kim, E.: Multi-model intercomparisons of air quality simulations for the KORUS-AQ campaign, Elem. Sci. Anth., 9, 00139, https://doi.org/10.1525/elementa.2021.00139, 2021.
Pfister, G. G., Eastham, S. D., Arellano, A. F., Aumont, B., Barsanti, K. C., Barth, M. C., Conley, A., Davis, N. A., Emmons, L. K., Fast, J. D., Arlene, M. F., Gaubert, B., Goldhaber, S., Granier, C., Grell, G. A., Guevara, M., Henze, D. K., Hodzic, A., Liu, X., Marsh, D. R., Orlando, J. J., Plane, J. M. C., Polvani, L. M., Rosenlof, K. H., Steiner, A. L., Jacob, D. J., and Brasseur, G. P.: A MUlti-Scale Infrastructure for Chemistry and Aerosols-MUSICA, B. Am. Meteorol. Soc., 101, E1743–E1760, https://doi.org/10.1175/BAMS-D-19-0331.1, 2020.
Pound, R. J., Sherwen, T., Helmig, D., Carpenter, L. J., and Evans, M. J.: Influences of oceanic ozone deposition on tropospheric photochemistry, Atmos. Chem. Phys., 20, 4227–4239, https://doi.org/10.5194/acp-20-4227-2020, 2020.
Prather, M. J., Holmes, C. D., and Hsu, J.: Reactive greenhouse gas scenarios: Systematic exploration of uncertainties and the role of atmospheric chemistry, Geophys. Res. Lett., 39, L09803, https://doi.org/10.1029/2012GL051440, 2012.
Price, C., Penner, J., and Prather, M.: NOx from lightning: 1. Global distribution based on lightning physics, J. Geophys. Res.-Atmos., 102, 5929–5941, https://doi.org/10.1029/96JD03504, 1997.
Pye, H. O. T., Chan, A. W. H., Barkley, M. P., and Seinfeld, J. H.: Global modeling of organic aerosol: the importance of reactive nitrogen (NOx and NO3), Atmos. Chem. Phys., 10, 11261–11276, https://doi.org/10.5194/acp-10-11261-2010, 2010.
Randerson, J. T., van der Werf, G. R., Giglio, L., Collatz, G. J., and Kasibhatla, P. S.: Global Fire Emissions Database, Version 4, (GFEDv4), ORNL DAAC, Oak Ridge, Tennessee, USA, https://doi.org/10.3334/ORNLDAAC/1293, 2018.
Saiz-Lopez, A., Lamarque, J.-F., Kinnison, D. E., Tilmes, S., Ordóñez, C., Orlando, J. J., Conley, A. J., Plane, J. M. C., Mahajan, A. S., Sousa Santos, G., Atlas, E. L., Blake, D. R., Sander, S. P., Schauffler, S., Thompson, A. M., and Brasseur, G.: Estimating the climate significance of halogen-driven ozone loss in the tropical marine troposphere, Atmos. Chem. Phys., 12, 3939–3949, https://doi.org/10.5194/acp-12-3939-2012, 2012.
Schwantes, R. H., Emmons, L. K., Orlando, J. J., Barth, M. C., Tyndall, G. S., Hall, S. R., Ullmann, K., St. Clair, J. M., Blake, D. R., Wisthaler, A., and Bui, T. P. V.: Comprehensive isoprene and terpene gas-phase chemistry improves simulated surface ozone in the southeastern US, Atmos. Chem. Phys., 20, 3739–3776, https://doi.org/10.5194/acp-20-3739-2020, 2020.
Schwantes, R. H., Lacey, F. G., Tilmes, S., Emmons, L. K., Lauritzen, P. H., Walters, S., Callaghan, P., Zarzycki, C. M., Barth, M. C., Jo, D. S., Bacmeister, J. T., Neale, R. B., Vitt, F., Kluzek, E., Roozitalab, B., Hall, S. R., Ullmann, K., Warneke, C., Peischl, J., Pollack, I. B., Flocke, F., Wolfe, G. M., Hanisco, T. F., Keutsch, F. N., Kaiser, J., Bui, T. P. V., Jimenez, J. L., Campuzano-Jost, P., Apel, E. C., Hornbrook, R. S., Hills, A. J., Yuan, B., and Wisthaler, A.: Evaluating the Impact of Chemical Complexity and Horizontal Resolution on Tropospheric Ozone Over the Conterminous US With a Global Variable Resolution Chemistry Model, J. Adv. Model. Earth Sy., 14, e2021MS002889, https://doi.org/10.1029/2021MS002889, 2022.
Shah, V., Jacob, D. J., Dang, R., Lamsal, L. N., Strode, S. A., Steenrod, S. D., Boersma, K. F., Eastham, S. D., Fritz, T. M., Thompson, C., Peischl, J., Bourgeois, I., Pollack, I. B., Nault, B. A., Cohen, R. C., Campuzano-Jost, P., Jimenez, J. L., Andersen, S. T., Carpenter, L. J., Sherwen, T., and Evans, M. J.: Nitrogen oxides in the free troposphere: implications for tropospheric oxidants and the interpretation of satellite NO2 measurements, Atmos. Chem. Phys., 23, 1227–1257, https://doi.org/10.5194/acp-23-1227-2023, 2023.
Sherwen, T., Evans, M. J., Carpenter, L. J., Andrews, S. J., Lidster, R. T., Dix, B., Koenig, T. K., Sinreich, R., Ortega, I., Volkamer, R., Saiz-Lopez, A., Prados-Roman, C., Mahajan, A. S., and Ordóñez, C.: Iodine's impact on tropospheric oxidants: a global model study in GEOS-Chem, Atmos. Chem. Phys., 16, 1161–1186, https://doi.org/10.5194/acp-16-1161-2016, 2016a.
Sherwen, T., Schmidt, J. A., Evans, M. J., Carpenter, L. J., Großmann, K., Eastham, S. D., Jacob, D. J., Dix, B., Koenig, T. K., Sinreich, R., Ortega, I., Volkamer, R., Saiz-Lopez, A., Prados-Roman, C., Mahajan, A. S., and Ordóñez, C.: Global impacts of tropospheric halogens (Cl, Br, I) on oxidants and composition in GEOS-Chem, Atmos. Chem. Phys., 16, 12239–12271, https://doi.org/10.5194/acp-16-12239-2016, 2016b.
Tang, Z., Jiang, Z., Chen, J., Yang, P., and Shen, Y.: The capabilities of the adjoint of GEOS-Chem model to support HEMCO emission inventories and MERRA-2 meteorological data, Geosci. Model Dev., 16, 6377–6392, https://doi.org/10.5194/gmd-16-6377-2023, 2023.
The Community Atmosphere Model Developers: The Community Atmosphere Model version 6.3.118 [code], GitHub [code], https://github.com/ESCOMP/CAM/tree/cam6_3_118 (last access: 31 July 2024), 2023a.
The Community Atmosphere Model Developers: The Community Atmosphere Model version 6.3.147 [code], GitHub [code], https://github.com/ESCOMP/CAM/tree/cam6_3_147 (last access: 31 July 2024), 2023b.
The International GEOS-Chem User Community: geoschem/geos-chem: GEOS-Chem 14.1.1 (14.1.1) Zenodo [code], https://doi.org/10.5281/zenodo.7696632, 2023.
Tilmes, S., Lamarque, J.-F., Emmons, L. K., Conley, A., Schultz, M. G., Saunois, M., Thouret, V., Thompson, A. M., Oltmans, S. J., Johnson, B., and Tarasick, D.: Technical Note: Ozonesonde climatology between 1995 and 2011: description, evaluation and applications, Atmos. Chem. Phys., 12, 7475–7497, https://doi.org/10.5194/acp-12-7475-2012, 2012.
Tilmes, S., Lamarque, J.-F., Emmons, L. K., Kinnison, D. E., Ma, P.-L., Liu, X., Ghan, S., Bardeen, C., Arnold, S., Deeter, M., Vitt, F., Ryerson, T., Elkins, J. W., Moore, F., Spackman, J. R., and Val Martin, M.: Description and evaluation of tropospheric chemistry and aerosols in the Community Earth System Model (CESM1.2), Geosci. Model Dev., 8, 1395–1426, https://doi.org/10.5194/gmd-8-1395-2015, 2015.
Tilmes, S., Lamarque, J.-F., Emmons, L. K., Kinnison, D. E., Marsh, D., Garcia, R. R., Smith, A. K., Neely, R. R., Conley, A., Vitt, F., Val Martin, M., Tanimoto, H., Simpson, I., Blake, D. R., and Blake, N.: Representation of the Community Earth System Model (CESM1) CAM4-chem within the Chemistry-Climate Model Initiative (CCMI), Geosci. Model Dev., 9, 1853–1890, https://doi.org/10.5194/gmd-9-1853-2016, 2016.
Travis, K. R., Heald, C. L., Allen, H. M., Apel, E. C., Arnold, S. R., Blake, D. R., Brune, W. H., Chen, X., Commane, R., Crounse, J. D., Daube, B. C., Diskin, G. S., Elkins, J. W., Evans, M. J., Hall, S. R., Hintsa, E. J., Hornbrook, R. S., Kasibhatla, P. S., Kim, M. J., Luo, G., McKain, K., Millet, D. B., Moore, F. L., Peischl, J., Ryerson, T. B., Sherwen, T., Thames, A. B., Ullmann, K., Wang, X., Wennberg, P. O., Wolfe, G. M., and Yu, F.: Constraining remote oxidation capacity with ATom observations, Atmos. Chem. Phys., 20, 7753–7781, https://doi.org/10.5194/acp-20-7753-2020, 2020.
van der Werf, G. R., Randerson, J. T., Giglio, L., van Leeuwen, T. T., Chen, Y., Rogers, B. M., Mu, M., van Marle, M. J. E., Morton, D. C., Collatz, G. J., Yokelson, R. J., and Kasibhatla, P. S.: Global fire emissions estimates during 1997–2016, Earth Syst. Sci. Data, 9, 697–720, https://doi.org/10.5194/essd-9-697-2017, 2017.
Wang, H., Easter, R. C., Rasch, P. J., Wang, M., Liu, X., Ghan, S. J., Qian, Y., Yoon, J.-H., Ma, P.-L., and Vinoj, V.: Sensitivity of remote aerosol distributions to representation of cloud–aerosol interactions in a global climate model, Geosci. Model Dev., 6, 765–782, https://doi.org/10.5194/gmd-6-765-2013, 2013.
Wang, X., Jacob, D. J., Downs, W., Zhai, S., Zhu, L., Shah, V., Holmes, C. D., Sherwen, T., Alexander, B., Evans, M. J., Eastham, S. D., Neuman, J. A., Veres, P. R., Koenig, T. K., Volkamer, R., Huey, L. G., Bannan, T. J., Percival, C. J., Lee, B. H., and Thornton, J. A.: Global tropospheric halogen (Cl, Br, I) chemistry and its impact on oxidants, Atmos. Chem. Phys., 21, 13973–13996, https://doi.org/10.5194/acp-21-13973-2021, 2021.
Wells, K. C., Millet, D. B., Payne, V. H., Deventer, M. J., Bates, K. H., de Gouw, J. A., Graus, M., Warneke, C., Wisthaler, A., and Fuentes, J. D.: Satellite isoprene retrievals constrain emissions and atmospheric oxidation, Nature, 585, 225–233, https://doi.org/10.1038/s41586-020-2664-3, 2020.
Wofsy, S. C., Afshar, S., Allen, H. M., Apel, E., Asher, E. C., Barletta, B., Bent, J., Bian, H., Biggs, B. C., Blake, D. R., Blake, N., Bourgeois, I., Brock, C. A., Brune, W. H., Budney, J. W., Bui, T. P., Butler, A., Campuzano-Jost, P., Chang, C. S., Chin, M., Commane, R., Correa, G., Crounse, J. D., Cullis, P. D., Daube, B. C., Day, D. A., Dean-Day, J. M., Dibb, J. E., DiGangi, J. P., Diskin, G. S., Dollner, M., Elkins, J. W., Erdesz, F., Fiore, A. M., Flynn, C. M., Froyd, K., Gesler, D. W., Hall, S. R., Hanisco, T. F., Hannun, R. A., Hills, A. J., Hintsa, E. J., Hoffman, A., Hornbrook, R. S., Huey, L. G., Hughes, S., Jimenez, J. L., Johnson, B. J., Katich, J. M., Keeling, R. F., Kim, M. J., Kupc, A., Lait, L. R., Lamarque, J.-F., Liu, J., McKain, K., Mclaughlin, R. J., Meinardi, S., Miller, D. O., Montzka, S. A., Moore, F. L., Morgan, E. J., Murphy, D. M., Murray, L. T., Nault, B. A., Neuman, J. A., Newman, P. A., Nicely, J. M., Pan, X., Paplawsky, W., Peischl, J., Prather, M. J., Price, D. J., Ray, E., Reeves, J. M., Richardson, M., Rollins, A. W., Rosenlof, K. H., Ryerson, T. B., Scheuer, E., Schill, G. P., Schroder, J. C., Schwarz, J. P., St. Clair, J. M., Steenrod, S. D., Stephens, B. B., Strode, S. A., Sweeney, C., Tanner, D., Teng, A. P., Thames, A. B., Thompson, C. R., Ullmann, K., Veres, P. R., Vieznor, N., Wagner, N. L., Watt, A., Weber, R., Weinzierl, B., Wennberg, P., Williamson, C. J., Wilson, J. C., Wolfe, G. M., Woods, C. T., and Zeng, L. H.: ATom: Merged Atmospheric Chemistry, Trace Gases, and Aerosols, ORNL DAAC, Oak Ridge, Tennessee, USA, https://doi.org/10.3334/ORNLDAAC/1581, 2018.
Woo, J.-H., Kim, Y., Kim, H.-K., Choi, K.-C., Eum, J.-H., Lee, J.-B., Lim, J.-H., Kim, J., and Seong, M.: Development of the CREATE Inventory in Support of Integrated Climate and Air Quality Modeling for Asia, Sustainability, 12, 7930, https://doi.org/10.3390/su12197930, 2020.
Wu, S., Mickley, L. J., Jacob, D. J., Logan, J. A., Yantosca, R. M., and Rind, D.: Why are there large differences between models in global budgets of tropospheric ozone?, J. Geophys. Res.-Atmos., 112, D05302, https://doi.org/10.1029/2006JD007801, 2007.
Yang, L. H., Jacob, D. J., Colombi, N. K., Zhai, S., Bates, K. H., Shah, V., Beaudry, E., Yantosca, R. M., Lin, H., Brewer, J. F., Chong, H., Travis, K. R., Crawford, J. H., Lamsal, L. N., Koo, J.-H., and Kim, J.: Tropospheric NO2 vertical profiles over South Korea and their relation to oxidant chemistry: implications for geostationary satellite retrievals and the observation of NO2 diurnal variation from space, Atmos. Chem. Phys., 23, 2465–2481, https://doi.org/10.5194/acp-23-2465-2023, 2023.
Young, P. J., Archibald, A. T., Bowman, K. W., Lamarque, J.-F., Naik, V., Stevenson, D. S., Tilmes, S., Voulgarakis, A., Wild, O., Bergmann, D., Cameron-Smith, P., Cionni, I., Collins, W. J., Dalsøren, S. B., Doherty, R. M., Eyring, V., Faluvegi, G., Horowitz, L. W., Josse, B., Lee, Y. H., MacKenzie, I. A., Nagashima, T., Plummer, D. A., Righi, M., Rumbold, S. T., Skeie, R. B., Shindell, D. T., Strode, S. A., Sudo, K., Szopa, S., and Zeng, G.: Pre-industrial to end 21st century projections of tropospheric ozone from the Atmospheric Chemistry and Climate Model Intercomparison Project (ACCMIP), Atmos. Chem. Phys., 13, 2063–2090, https://doi.org/10.5194/acp-13-2063-2013, 2013.
Young, P. J., Naik, V., Fiore, A. M., Gaudel, A., Guo, J., Lin, M. Y., Neu, J. L., Parrish, D. D., Rieder, H. E., Schnell, J. L., Tilmes, S., Wild, O., Zhang, L., Ziemke, J. R., Brandt, J., Delcloo, A., Doherty, R. M., Geels, C., Hegglin, M. I., Hu, L., Im, U., Kumar, R., Luhar, A., Murray, L., Plummer, D., Rodriguez, J., Saiz-Lopez, A., Schultz, M. G., Woodhouse, M. T., and Zeng, G.: Tropospheric Ozone Assessment Report: Assessment of global-scale model performance for global and regional ozone distributions, variability, and trends, Elem. Sci. Anth., 6, 10, https://doi.org/10.1525/elementa.265, 2018.
Yu, F. and Luo, G.: Simulation of particle size distribution with a global aerosol model: contribution of nucleation to aerosol and CCN number concentrations, Atmos. Chem. Phys., 9, 7691–7710, https://doi.org/10.5194/acp-9-7691-2009, 2009.
Yu, K., Keller, C. A., Jacob, D. J., Molod, A. M., Eastham, S. D., and Long, M. S.: Errors and improvements in the use of archived meteorological data for chemical transport modeling: an analysis using GEOS-Chem v11-01 driven by GEOS-5 meteorology, Geosci. Model Dev., 11, 305–319, https://doi.org/10.5194/gmd-11-305-2018, 2018.
Zhao, Y., Saunois, M., Bousquet, P., Lin, X., Berchet, A., Hegglin, M. I., Canadell, J. G., Jackson, R. B., Hauglustaine, D. A., Szopa, S., Stavert, A. R., Abraham, N. L., Archibald, A. T., Bekki, S., Deushi, M., Jöckel, P., Josse, B., Kinnison, D., Kirner, O., Marécal, V., O'Connor, F. M., Plummer, D. A., Revell, L. E., Rozanov, E., Stenke, A., Strode, S., Tilmes, S., Dlugokencky, E. J., and Zheng, B.: Inter-model comparison of global hydroxyl radical (OH) distributions and their impact on atmospheric methane over the 2000–2016 period, Atmos. Chem. Phys., 19, 13701–13723, https://doi.org/10.5194/acp-19-13701-2019, 2019.
- Abstract
- Introduction
- Model description and methods
- Comparison of photolysis schemes: Fast-JX and TUV
- Global budgets and distributions of tropospheric oxidants
- Comparisons to global observations
- Comparison to KORUS-AQ aircraft campaign
- Conclusions
- Code availability
- Author contributions
- Competing interests
- Disclaimer
- Acknowledgements
- Financial support
- Review statement
- References
- Abstract
- Introduction
- Model description and methods
- Comparison of photolysis schemes: Fast-JX and TUV
- Global budgets and distributions of tropospheric oxidants
- Comparisons to global observations
- Comparison to KORUS-AQ aircraft campaign
- Conclusions
- Code availability
- Author contributions
- Competing interests
- Disclaimer
- Acknowledgements
- Financial support
- Review statement
- References