the Creative Commons Attribution 4.0 License.
the Creative Commons Attribution 4.0 License.
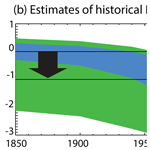
Opinion: The importance of historical and paleoclimate aerosol radiative effects
Longlei Li
Samuel Albani
Douglas S. Hamilton
Jasper F. Kok
Estimating past aerosol radiative effects and their uncertainties is an important topic in climate science. Aerosol radiative effects propagate into large uncertainties in estimates of how present and future climate evolves with changing greenhouse gas emissions. A deeper understanding of how aerosols affected the atmospheric energy budget under past climates is hindered in part by a lack of relevant paleo-observations and in part because less attention has been paid to the problem. Because of the lack of information we do not seek here to determine the change in the radiative forcing due to aerosol changes but rather to estimate the uncertainties in those changes. Here we argue that current uncertainties from emission uncertainties (90 % confidence interval range spanning 2.8 W m−2) are just as large as model spread uncertainties (2.8 W m−2) in calculating preindustrial to present-day aerosol radiative effects. There are no estimates of radiative forcing for important aerosols such as wildfire and dust aerosols in most paleoclimate time periods. However, qualitative analysis of paleoclimate proxies suggests that changes in aerosols between different past climates are similar in magnitude to changes in aerosols between the preindustrial and present day; plus, there is the added uncertainty from the variability in aerosols and fires in the preindustrial. From the limited literature we crudely estimate a paleoclimate aerosol uncertainty for the Last Glacial Maximum relative to preindustrial of 4.8 W m−2, and we estimate the uncertainty in the aerosol feedback in the natural Earth system over the paleoclimate (Last Glacial Maximum to preindustrial) to be about 3.2 W m−2 K−1. In order to more accurately assess the uncertainty in historical aerosol radiative effects, we propose a new model intercomparison project, which would include multiple plausible emission scenarios tested across a range of state-of-the-art climate models over the historical period. These emission scenarios would then be compared to the available independent aerosol observations to constrain which are most probable. In addition, future efforts should work to characterize and constrain paleo-aerosol forcings and uncertainties. Careful propagation of aerosol uncertainties in the literature is required to ensure an accurate quantification of uncertainties in projections of future climate changes.
- Article
(3271 KB) - Full-text XML
- BibTeX
- EndNote
While CO2 radiative forcing has been the most important driver of observed climate warming, aerosol interactions with radiation and cloud properties represent the largest source of uncertainty in the Intergovernmental Panel on Climate Change (IPCC) estimates of present-day to preindustrial radiative forcing (e.g., IPCC, 2021). This large uncertainty is driven by the heterogeneity of aerosols in space, time, composition, size and shape in the current climate, complexity in aerosol impacts on radiation and clouds, poor knowledge of aerosols in historical and past climate conditions, and how aerosol processes have changed over time (Albani et al., 2018; Bellouin et al., 2020; IPCC, 2021; Carslaw et al., 2017; Gulev et al., 2021; Szopa et al., 2021). Substantial research emphasis has been placed on understanding the direct changes in emissions from human activities (e.g., fossil fuel combustion); these emissions are estimated based on many processes, including emission factors for different industries, estimates of the changes in location and intensity of different industrial facilities, and time-dependent changes in technologies (e.g., Bond et al., 2007; Klimont et al., 2017). On the other hand, the uncertainty in aerosol emissions that are usually considered natural, such as from dust storms or wildfires, is likely larger and contributes to larger uncertainties in aerosol radiative forcing (Carslaw et al., 2010; Mahowald et al., 2011a; Regayre et al., 2018). Because of limited paleo-observations, we currently rely on emission models that were calibrated using current observations and apply them to past climates, not only for industrial sources but also for wildfire and dust aerosols (Van Marle et al., 2017; Turnock et al., 2020; Zhao et al., 2022).
However, the dominant mechanisms for natural emission processes are unlikely to have remained constant over time, and thus using present-day observations to constrain past model predictions is biased towards the present day and therefore anthropogenically influenced aerosol behavior (e.g., Hamilton et al., 2018). We therefore advocate that past model simulations should, as much as possible, be constrained using paleoenvironmental archives of past aerosol. Note that for IPCC estimates, most models use the same emission dataset(s) to drive emissions, meaning that aerosol radiative forcing uncertainty estimates based on Climate Model Intercomparison Project (CMIP6) estimates tend to accurately represent the uncertainty due to model spread using the same emission dataset but do not necessarily aim to include the uncertainty due to emission uncertainties (Hoesly et al., 2018; Bellouin et al., 2020; Thornhill et al., 2021; Pincus et al., 2016), which are especially large in preindustrial or paleoclimate climates (Li et al., 2019; Kok et al., 2023).
Here we argue that an improved characterization of the evolution of “natural” aerosols, especially dust and wildfire aerosols, is needed to improve our understanding of aerosol radiative effects over the historical and paleoclimate time periods. Before we can seek to constrain the uncertainties (e.g., Bellouin et al., 2020), we need to first characterize the different sources of uncertainties and their magnitude (Carslaw et al., 2017), especially emissions of natural aerosols (e.g., Hamilton et al., 2018; Kok et al., 2023), and propagate these uncertainties into the climate simulations. Additionally, we argue that radiative perturbations due to changes in natural aerosols that are affected by human actions, such as dust and wildfire aerosols, should be treated as a radiative forcing. Note that we use the IPCC glossary definition of radiative forcing as the change in the top-of-atmosphere radiative balance due to the addition of a species (IPCC, 2021; Gulev et al., 2021; Szopa et al., 2021), and we use the term radiative forcing instead of effective radiative forcing, for which the same arguments apply. In our discussion here, we include both direct effects and aerosol–cloud interactions, realizing that the largest uncertainties are often associated with aerosol–cloud interactions. We (1) discuss the limited available data constraining the changes in dust and wildfire aerosol emissions over the historical time period and how those compare to the CMIP6 emission datasets, (2) consider whether aerosols can be considered feedbacks or forcings, (3) propagate the uncertainty in emissions of natural aerosols to create more realistic estimates of aerosol radiative effect uncertainties, (4) estimate the uncertainties in aerosol radiative effects for paleoclimate time periods, and (5) point out other sources of aerosol uncertainties as well as (6) the importance of accurate aerosol radiative effect uncertainties for climate science. Finally, (7) we propose a path forward to improve the characterization of the uncertainties in aerosol radiative effects, which would then allow us to start constraining the uncertainties with observations.
Natural aerosols include dust, wildfire emissions, sea salts, and biogenic organic aerosols, among others (e.g., dimethyl sulfide, lightning NOx). Aerosols such as dust or carbonaceous and sulfur species emitted by wildfires can be generated under natural conditions. As such, these aerosols can produce important feedbacks in a changing climate (Allen et al., 2016; Kok et al., 2018; Thornhill et al., 2021). However, some of these aerosols, notably dust and wildfire aerosols, are also directly or indirectly affected by human actions. For instance, dust emissions can be increased substantially both by human disturbance of the natural landscape and by anthropogenic diversions of surface water flows (Lee et al., 2012; Ginoux et al., 2012; Xi and Sokolik, 2016). Similarly, wildfire emissions can be increased by open fires set by humans as part of deforestation or agricultural practices (van der Werf et al., 2010). Additionally, natural wildfire emissions can be suppressed by human activity, for example active firefighting or removal of forests and grasslands due to agricultural and urban development (Knorr et al., 2014; Bistinas et al., 2013). As such, the radiative perturbation due to historical changes in these natural aerosols can be partially due to human-induced land cover and land use changes (a forcing) as well as natural and anthropogenic climate changes (a feedback).
2.1 Historical changes in desert and agricultural dust
While the global concentration of long-lived and well-mixed species such as CO2 can be retrieved from ice cores, aerosols in the troposphere have lifetimes of only a few days to 2 weeks (Textor et al., 2006), meaning that aerosol observations need to be available across the globe in dozens or hundreds of locations at a high temporal frequency before we can begin to build a reasonable understanding of their distribution and behavior. Unfortunately, for most aerosol species we do not have that kind of coverage for most time periods, including the present day (see, e.g., the spatial coverage in Naik et al., 2021). Dust is an exception in some respects in that dust is preserved to some extent in several natural archives, and thus there are compilations of dust changes over different time periods. For paleoclimate conditions (e.g., the last glacial cycle, the Holocene) the main natural archives for dust include ice cores and marine and terrestrial (loess, paleosol) sediment records, for which paleodust compilations exist (e.g., Albani et al., 2015). Most of those natural archives do not have the potential to cover the last few centuries, including the late Holocene to preindustrial to modern transitions, due to issues with dating or disturbance of surface sediments (e.g., for many marine sediment records the core top is lost during retrieval and thus the last 100 or so years are not easily obtainable; loess provides the substrate for very fertile soils exploited for agriculture in the last millennia) (Maher et al., 2010). Other archives, such as firn cores from the polar areas, ice cores from mountain glaciers, and ombrotrophic peat bogs, have the potential to preserve dust deposition records over the last decades or centuries, although they are still affected by major uncertainties when it comes to retrieving accurate deposition records (Albani et al., 2015). With these caveats in mind, there are still paleo-data that can be compiled to infer the evolution of desert dust in different regions across the globe since the preindustrial, albeit with large uncertainties (Kok et al., 2023).
Desert dust is generated in dry, poorly vegetated regions with strong winds, and the generation of dust may be enhanced in regions with human land use (Ginoux et al., 2012). Between the 1960s and 1980s dust was observed to have changed by a factor of 4 over the North Atlantic region, perhaps due to expansion of land use, precipitation changes during Sahel drought, or changes in winds (Mahowald et al., 2002; Prospero and Lamb, 2003; Evan et al., 2016). Paleoclimate evidence also suggests that dust emissions are very sensitive to both climate change and land use (Lambert et al., 2008; Neff et al., 2008; Mulitza et al., 2010).
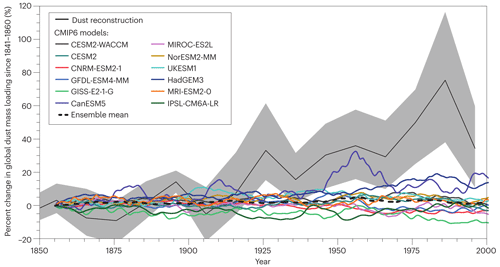
Figure 1Annual global mean dust aerosol loading from the observationally based reconstruction (Kok et al., 2023) in black (grey shading represents the 90 % confidence interval) compared against 10-year running means from historical runs of Climate Model Intercomparison Project (CMIP6) ensemble members. Reproduced with permission from Kok et al. (2023).
A recent synthesis of dust deposition observations suggests a 55 ± 30 % (90 % confidence interval) increase in dust globally since preindustrial times (Kok et al., 2023). While there are not sufficient data for each source to have complete confidence in such estimates, they still represent our best knowledge of the state of changes in dust since the 1850s. Unfortunately, the model simulations from the last Climate Model Intercomparison Project (CMIP6) that include prognostic dust do not match these changes, either because of the lack of correct inclusion of the impact of land use on dust or incorrect simulation of the feedbacks of natural and anthropogenic climate changes on dust (Fig. 1). Indeed, the CMIP6 models show relatively constant dust amounts in contrast to the large change that is observed (Kok et al., 2023).
2.2 Historical changes in wildfire and open fires
Paleoenvironmental archives for wildfires and other open fires include charcoal records near the source regions (Marlon et al., 2008), ice cores (McConnell et al., 2007b), tree ring scars, and speleothems. Wildfire is a natural ecosystem process but also susceptible to human influence (Bowman et al., 2009; Pechony and Shindell, 2010). For example, while humans are responsible for many fire ignitions today, the conversion of natural landscapes to managed lands reduces the area available for wildfire to spread before reaching a break in vegetation. In addition, humans may prematurely suppress or extinguish a fire once it starts, particularly in regions closer to urban development (Bowman et al., 2009; Kloster et al., 2010). Such contrasting influences on fire activity help highlight the complex interactions between humans and fire. Satellite observations over the last 20 years show a strong interannual variability in fire activity with 50 % of the observed change in emissions correlated with climate signals, although human contributions could have played a vital role (van der Werf et al., 2006). The open fire emission estimates used for CMIP6 use data from satellites to predict emissions from 1997 onwards (van Marle et al., 2017); however, prior to the satellite fire era the emissions are generated from several different fire models with some additional proxy evidence. These fire models include a range of representations of how fires have evolved since the Industrial Revolution but not all account for active or passive fire suppression by humans. Overall, this results in CMIP6 fire emissions increasing since 1850s (van Marle et al., 2017). However, there is ample evidence that the relationship between human population growth, land use change, and fire activity may be much more nuanced. Paleoclimate data from charcoal records suggest a maximum in open fires in the 1850s and a decrease since then (Marlon et al., 2008). Satellite data show a global decrease in burned area over the last decades, driven primarily by the conversion of natural lands to agricultural and pastoral lands (Andela et al., 2017; Jones et al., 2022). Indeed, fire models which include a more realistic representation of how fires and human population density are related simulate a much higher number of fires during the preindustrial (ca. 1850) than the CMIP6 (or AEROCOM) emission dataset suggests (Hamilton et al., 2018; Hoesly et al., 2018; Dentener et al., 2006). Including larger past fire emissions in aerosol models also improves the match of simulated data to the available ice core data on the deposition ratio between present-day and preindustrial black carbon (yellow and red symbols) than using the default CMIP6 datasets (blue symbols) (Hamilton et al., 2018; Liu et al., 2021) (Fig. 2). This suggests that it is probable that there were more open fire emissions during the preindustrial than accounted for in the emission inventories used for CMIP6 simulations (Hoesly et al., 2018; van Marle et al., 2017).
Is it plausible that emissions from wildfires and other open fires, such as agricultural fires, could have been so much larger in preindustrial times than is currently being accounted for in climate model emission datasets? It is difficult to know for certain of course, but a few examples show the possibility using different mechanisms. One study suggests that higher fire numbers in 1850 relative to today could be due to more land use change today than in 1850. In other words, today there is less natural area available for fires than in 1850 (van der Werf et al., 2013), which is consistent with the decreased fire burn area observed over the satellite era (Andela et al., 2017). Other studies have suggested that wildfire suppression has been important for reducing fires in North America, for example, over the last 50 years (Marlon et al., 2012). In addition, agricultural open burning could be important; today, northern India represents the region with some of the highest aerosol optical depths and worst air quality (Li et al., 2022; Burnett et al., 2018). Despite the area having large population centers and industrial emissions, one of the largest sources in that region is agricultural burning (Cusworth et al., 2018). Similarly, the ban on straw burning, a primary source of pollutants in central and eastern China (Wu et al., 2018), has become a national policy for air pollution control, as in many other countries. This suggests that, indeed, the high emission factors of open burning make it a very effective source of aerosols.
In addition, wildfires and open fires represent some of the most important aerosols for direct and aerosol–cloud radiative effects, with a total radiative effect in the current climate of −2 W m−2 (IPCC, 2021; Gulev et al., 2021; Szopa et al., 2021; Penner et al., 1992). Changes in wildfires and open fires represent about −1 W m−2 or 50 % of the anthropogenic aerosol radiative forcing since 1850, and all of these estimates have very large uncertainties (Unger et al., 2010; IPCC, 2019, 2021; Gulev et al., 2021; Szopa et al., 2021). The overall negative forcing results from the effect of an increasing aerosol burden increasing cloud albedo and from the prevailing effects of preferentially scattering sulfates and particulate organic matter (secondary aerosol from fire emission of precursors such as biogenic volatile organic compounds) over the preferentially absorbing black carbon emissions from fires (Hamilton et al., 2018; Carslaw et al., 2017; Penner et al., 1992; IPCC, 2021; Gulev et al., 2021; Szopa et al., 2021).
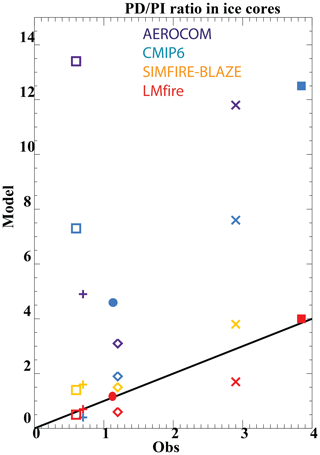
Figure 2Observed and modeled present-day to preindustrial ratios (PD PI) for black carbon in ice cores using different open fire emissions. Ice core sites are from Greenland (open square and plus signs), Wyoming (diamond), and France (x symbols) for four different model simulations: AEROCOM (purple), CMIP6 (blue), SIMFIRE-BLAZE (yellow), and LMfire (red) are taken from Hamilton et al. (2018). Ice core sites from Bolivia (solid circle) and Antarctica (solid square) using CMIP6 (blue) and LMfire (red) are taken from Liu et al. (2021). The solid black line shows the 1 : 1 line.
In summary, trade-offs between the effects of climate change and land use make it difficult to estimate past changes in the loading of dust and smoke from open fires. Changes in other aerosols (fossil fuels, biofuels, biogenic aerosols, sea spray) are difficult to estimate as well (e.g., Fig. 2.9a and b in Gulev et al., 2021). Without observations of these aerosols in past climates, it is difficult to have confidence in our existing emission models or their past and future emission projections.
The nomenclature that dust and wildfire aerosols are natural aerosols is perhaps misleading and might have caused the important changes that have occurred in these aerosols to receive insufficient consideration by climate scientists. For instance, in the Sixth Assessment Report, the radiative perturbation due to the ∼ 50 % increase in dust over the historical record (Hooper and Marx, 2018; Mahowald et al., 2010; Kok et al., 2023) is not explicitly accounted for as a radiative forcing of the climate system, although the dust–climate feedback was quantified (Forster et al., 2021; Naik et al., 2021) and the report does highlight the fact that there is substantial uncertainty in this feedback (Szopa et al., 2021).
There are several reasons why not explicitly accounting for the historical dust increase as a radiative forcing could be problematic. First, although the exact proportion of modern dust that can be considered anthropogenic is uncertain (Tegen et al., 2004; Mahowald, 2007; Ginoux et al., 2012; Stanelle et al., 2014), a large body of work indicates that human-induced land cover and land use changes in semi-arid and arid lands can produce a large increase in dust aerosol emissions (Neff et al., 2008; Webb and Pierre, 2018). Such land cover and land use changes have been widespread since the Industrial Revolution (Klein-Goldewijk, 2001), making it likely that a substantial part of the historical dust increase – perhaps even most of it – was driven by human-induced land cover and land use changes (Ginoux et al., 2012; Hooper and Marx, 2018; Kok et al., 2023), which thus constitutes a radiative forcing.
A second reason is that not accounting for the historical dust increase as a radiative forcing implicitly assumes that the historical dust increase was due to a climate feedback. However, the dust change per degree of global surface temperature warming is inconsistent between different time periods. Indeed, the dust increase during the planetary warming of the past century is opposite to what is seen in the paleo-record, for which cold periods like the Last Glacial Maximum (LGM) coincide with high dust loadings (Albani et al., 2014). Moreover, there is no model consensus on whether dust will increase or decrease under future climate warming, in part because of large uncertainties in how precipitation in arid regions will change (IPCC, 2019). This inconsistency in the dust change per unit of global surface temperature warming could be due to a number of factors: (1) the historical dust increase was primarily driven by human land use changes, not climate changes; (2) the dust feedback is highly dependent on the climate state; or (3) the dust feedback occurs over much longer timescales than the observed approximately century-scale dust increase. Whatever the reason, the fact that the dust change per unit of surface temperature change is not consistent between different time periods undermines both the plausibility and the usefulness of classifying historical dust changes as a feedback in the context of future climate predictions.
The final, and most important, reason why not explicitly accounting for the historical dust increase as a radiative forcing might be problematic is that the dust increase indicated by dust deposition records (McConnell et al., 2007a; Mulitza et al., 2010; Mahowald et al., 2010; Hooper and Marx, 2018) is not captured by climate models (Kok et al., 2023) (Fig. 1). These models therefore also predict a dust–climate feedback that is indistinguishable from zero (Thornhill et al., 2021; Kok et al., 2023). As such, not explicitly accounting for dust changes as a radiative forcing has the net effect of omitting this potentially important perturbation to Earth's energy balance. This can bias climate sensitivity constraints and projections of future climate changes (Kok et al., 2023).
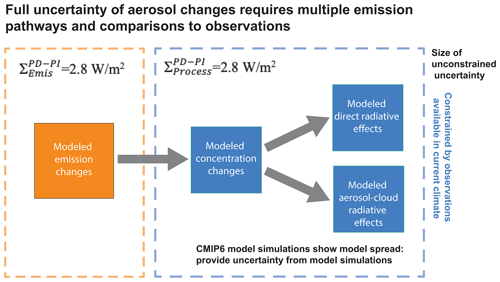
Figure 3Schematic of the sources of uncertainties in aerosol radiative effects from emission models to modeled concentration changes to modeled direct and aerosol–cloud radiative effects. The CMIP6 unconstrained uncertainties using a single emission scenario have a 90 % confidence interval range of 2.8 W m−2 (Bellouin et al., 2020; Sherwood et al., 2020). For the uncertainty summed over different aerosol species (Σ) using different emission scenarios for the past climate, the 90 % confidence interval ranges from wildfires of 2.8 W m−2 (Hamilton et al., 2018; Wan et al., 2021) is added in quadrature to uncertainties from dust of 0.4 W m−2 (Kok et al., 2023) and an estimate of industrial emission uncertainties (assuming 10 % error) of 0.2 W m−2, obtaining a 2.8 W m−2 uncertainty in emissions.
Considering these important issues discussed above, we argue that model simulations should include historical dust changes as an external forcing for two reasons: (1) because it is unlikely that the anthropogenic forcing and the climate feedback components of the historical dust loading change can be reliably separated in the near future and (2) because climate models currently cannot reproduce the historical dust changes (Kok et al., 2023; Mahowald et al., 2010). Since many models include prognostic schemes for dust, we propose that models add a temporally varying emissions factor obtained from constraints on the historical evolution of atmospheric dust deposition (e.g., Mahowald et al., 2010). This would enable models to both reproduce the historical change in dust and to also predict future changes in dust forced by climate and land use changes. A similar approach could be used for other natural aerosol emissions, such as from wildfires.
However, when models do treat changes in aerosols as feedbacks, the full uncertainty in the feedback should be included. For example, from this analysis, the feedback uncertainty should be ±1.6 W m−2 from the preindustrial to the present (see Sect. 4), over which time the global surface temperatures increased by about 1 K−1, so that means an uncertainty in the feedback of ±1.6 W m−2 K−1 (90 % confidence).
Since CMIP6 aerosol simulations are not consistent with available observations for dust and open fires, it is clear that additional uncertainty needs to be added to the aerosol radiative forcing estimates for the preindustrial to present day in order to make sure that the uncertainty ranges include available observations. This is a substantial undertaking, but here we schematically show a back-of-the-envelope calculation of how including the observations would affect estimates of aerosol radiative forcing uncertainty (Fig. 3).
We use a slightly different nomenclature here than Sherwood et al. (2020), for example, to emphasize the uncertainties in radiative forcing, without introducing too much nomenclature, and thus define ΔF as the change in radiative forcing between two different times and Σ as the uncertainty in that estimate using the 90 % confidence intervals.
Table 1Estimates of aerosol radiative forcing uncertainty, symbols, and citations. These represent the 90 % confidence intervals.
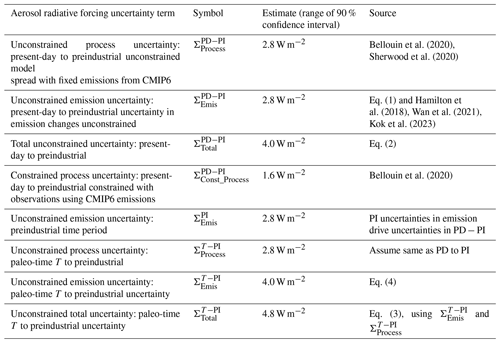
If we sum the sources of uncertainty currently available in the literature ( = 2.8 W m−2 from fires, = 0.4 W m−2 from dust – Hamilton et al., 2018; Wan et al., 2021; Kok et al., 2023) and assume a 10 % error for industrial emissions for = 0.2 W m−2 using Eq. (1), we obtain 2.8 W m−2, clearly dominated by fires (see Table 1 for terms). This is also consistent with attribution literature, which shows that much of the anthropogenic radiative forcing is from the biomass burning source (Fig. 1a in Unger et al., 2010).
The uncertainty in radiative forcing from uncertainty in preindustrial emissions ( = 2.8 W m−2) is similar in magnitude to the uncertainty from using one emission scenario for the historical time period (2.8 W m−2, which is the unconstrained model uncertainty using 90 % confidence intervals) (Bellouin et al., 2020; Sherwood et al., 2020), which we refer to here as the unconstrained aerosol process uncertainty for the present day to preindustrial (). The model spread in radiative forcing with the same emission scenario is due to differences in model simulations of concentration, radiation, and cloud interactions using the same emission change, which are large because these processes are poorly understood (Li et al., 2022; Pincus et al., 2016). Note that here we use the same term “scenario” for what happened in the past as what we use for choices in the future to emphasize that we do not know the emissions. The models make different assumptions about aerosol lifetime, size distribution, and aerosol microphysics, which results in different radiative forcings, so we assume this spread in models is the uncertainty in processes (), which is 2.8 W m−2. One could also think of this uncertainty in the process as coming from two sources: variability in present-day processes in simulating aerosols (e.g., that radiative forcing is sensitive not just to the total emissions, but also to where, what kind, and what else is in the region) (Li et al., 2022; Bellouin et al., 2020) and one part that is proportional to the strength of the change in aerosols, which could be proportional to the change in radiative forcing (ΔF) times some factor γ (Eq. 2).
We propose that future studies should identify the strength of the base uncertainty () and the portion of this process uncertainty that is proportional to the strength of the change in the radiative forcing (ΔF×γ)2.
The total unconstrained uncertainty due to aerosol changes could be estimated as being 4 W m−2 using Eq. (3), assuming the uncertainties are orthogonal (Eq. 3).
Emissions from industry (which are likely better known) have been increasing since 1850 in the CMIP6 simulations from which we estimated the aerosol radiative forcings. But aerosols from wildfires have also been increasing during this historical period in these simulations (van Marle et al., 2017). As discussed in Sect. 1, the paleoclimate data (and fire models which explicitly account for passive fire suppression effects of land use change) suggest that open fires have been decreasing since 1850, potentially offsetting the increase in industrial emissions (Fig. 4b). In contrast, the CMIP6 wildfire emissions assume large increases since 1850 in wildfires and open fires (van Marle et al., 2017). This produces a large uncertainty in the time series of aerosol forcing over the historical period. Notice that since wildfire emissions can vary strongly over a couple of years or decades (van der Werf et al., 2004), it should not be assumed (without observations) that the radiative forcing from wildfires follows either the top or the bottom of the error bar, but it could vary from one year to the other over the whole range.
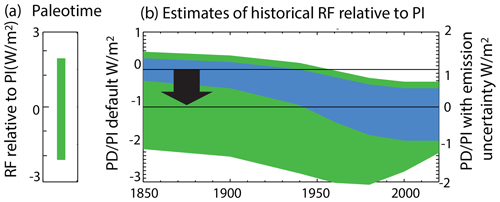
Figure 4Estimates of the unconstrained aerosol radiative forcing and its uncertainties for (a) different paleoclimate time periods relative to the preindustrial (PI; 1850) and (b) present day (PD) relative to 1850 based on CMIP6 model spread (schematic based on Smith et al., 2021, in blue) and including the emission uncertainties (90 % confidence intervals) from wildfires, dust, and anthropogenic aerosols as described in Fig. 3 (green) using the time series for wildfires from Marlon et al. (2008). The left vertical axis represents the present-day minus preindustrial radiative forcing (blue area) following Smith et al. (2021) and Sherwood et al. (2020), and the right axis adds in the emission uncertainties for the preindustrial (from Fig. 3; Hamilton et al., 2018), shifting the preindustrial baseline of aerosol radiative effects (black arrow; green area). Notice that the size of the black arrow and shift in the preindustrial state is not known, and this is a schematic to illustrate how the uncertainties in emissions in the preindustrial impact understanding of the radiative forcing.
Some of the difficulty of looking at preindustrial to present-day aerosol changes is simply understanding what natural aerosols would look like without humans. Unfortunately, there are strong fluctuations across the time period just before the industrial era (e.g., 1500–1850) in fires (Fig. 5), some potentially associated with humans (e.g., perhaps the increase in 1850 and decrease after this time period), but a large change during the Little Ice Age suggests that climate change can radically change fires (van der Werf et al., 2013). While the IPCC has used 1850 to 1900 as the preindustrial period for climate simulations (e.g., Allen et al., 2018), for aerosols this is not an ideal time period, as it is likely that aerosols are already elevated due to anthropogenic activities during this time period, while 1750 could be better, although still part of the Little Ice Age. The issue of what is the right baseline for preindustrial aerosols is also important for considering paleoclimates (Sect. 5). As shown in Figs. 3 and 5, the uncertainty in preindustrial to present-day emission changes in aerosols is driven by preindustrial emission uncertainties, which is partly associated with variability across the preindustrial time period.
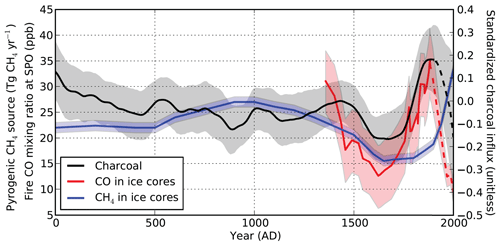
Figure 5Variability of biomass burning rates over the last centuries based on a worldwide compilation of charcoal records (Marlon et al., 2008), CO mixing ratios from fires using CO concentration measurements at the South Pole (SPO), its isotopic signature, and a mass balance model (Wang et al., 2010), as well s a similar approach but based on CH4 (Ferretti et al., 2005). The CO ice core data ended in 1897 but were extended (dashed line) by Wang et al. (2010) to the present day using firn samples (1968 and 1986) as well as modeling (year 2000). Shaded areas indicate reported uncertainty. Note that the datasets have different footprints and that absolute values cannot be compared directly. Reproduced with permission from van der Werf et al. (2013) under CCC3.0.
There are, of course, constraints on present-day radiative effects from aerosols from satellites and other tools, which can constrain the last 30–40 years (e.g., Bellouin et al., 2020). And there are energy constraints on the present-day to preindustrial change in aerosol radiative forcing using energy balance constraints (Smith et al., 2021; Sherwood et al., 2020), which result in a 57 % reduction in the uncertainty using fixed emissions ( = 1.6 W m−2). Unconstrained emission uncertainties and unconstrained process uncertainties have yet to be combined in a rigorous method like Bellouin et al. (2020) did for process uncertainties (in that study they assume that emissions are well known), but this should be done in the future. Adding in the uncertainties in emissions, especially from wildfires, would mean that while directly emitted anthropogenic aerosols are going up (as estimated in CMIP6), wildfire emissions may be going down. The wildfire aerosols resulting from these emissions would thus partially offset the radiative cooling from the increase in anthropogenic aerosols. Thus, if we take the case of high wildfires in the preindustrial (−2 W m−2 in Fig. 4), this could imply that estimates of aerosol radiative forcing from purely anthropogenic sources today which are large (−2 W m−2) would be more likely; the 1850 to 2000 aerosol radiative forcing would be the same as assumed now, but the 1850 aerosol radiative forcing would already be large. This would have important implications for climate warming over the next few decades, as anthropogenic emissions of aerosols are likely to decrease, leading to more warming than projected without including preindustrial aerosol emission uncertainties.
Unfortunately, except for dust or wildfires in certain time periods (Albani et al., 2015; Power et al., 2007; Zennaro et al., 2014; Marlon et al., 2008), there is very little information about the distribution or amount of aerosol in different climate regimes, and therefore we do not know the emissions well, nor the impact of those emissions on climate. We can envision these uncertainties as mostly unknown. We have some information that they are likely to be large (since aerosol uncertainties today are so large, and we know less about paleoclimate aerosols), but we cannot yet directly constrain these. We do know that there were large fluctuations: for example, dust was likely 2–4 times higher in the Last Glacial Maximum than it is today (Lambert et al., 2015; Mahowald et al., 1999; Albani et al., 2014; Albani and Mahowald, 2019), while between the preindustrial and present day, the change is smaller at only approximately 2 times (Kok et al., 2023) (Fig. 6). For dust, we have estimates at the LGM and 6000 years before present (Albani and Mahowald, 2019; Albani et al., 2014), which suggest that the changes in radiative forcing could be on the order of 0 to −2 W m−2 (Albani et al., 2018), although studies using carefully compared dust optics show smaller radiative forcings because dust both absorbs and reflects both shortwave and longwave radiation (Albani and Mahowald, 2019; Braconnot et al., 2021).
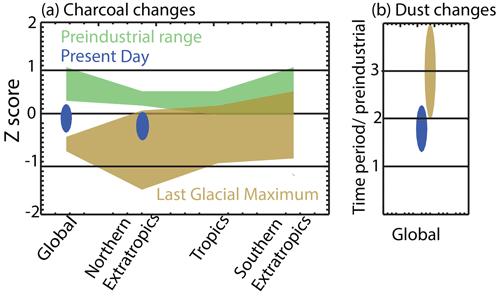
Figure 6Relative size of paleoclimate and historical changes in aerosols. (a) Based on z scores from charcoal records, the variability across preindustrial time periods (green), the present day (blue), and the Last Glacial Maximum is shown globally as well as for the northern extratropics, tropics, and southern extratropics based on data from Marlon et al. (2008, 2016). Charcoal reconstructions use z scores, which are normalized by the mean value at a site, divided by the variability, and thus a −2z score for LGM suggests significantly lower charcoal amounts. (b) Global dust change ratio of deposition between the present day and preindustrial (blue oval; Mahowald et al., 2010; Kok et al., 2023) and for the Last Glacial Maximum relative to the preindustrial (gold oval) (Mahowald et al., 1999; Albani et al., 2014, 2018; Lambert et al., 2015).
But changes between preindustrial and present-day aerosol radiative forcings are dominated by changes in fires (Sects. 1 and 2): are these changes as large in the paleodata as seen in the last 150 years? The limited paleodata suggest large changes in fires during different time periods in the past (Fischer et al., 2015; van der Werf et al., 2013; Zennaro et al., 2014; Arienzo et al., 2017). For example, in considering cold periods like the Last Glacial Maximum, there is likely a large reduction in fires at high latitudes due to the presence of the Laurentide and Fennoscandian ice sheets, which is consistent with fire proxies in Greenland ice cores (e.g., the ammonium record for the North Greenland Ice Core Project; Fischer et al., 2015). Generally, the charcoal record suggests lower fire frequency in the Last Glacial Maximum than preindustrial (Marlon et al., 2016), although ice sheets could have removed sediment records of wildfires (Fig. 6). For climate impacts, low- and middle-latitude fires tend to be more important today (Hamilton et al., 2018), so more information on the frequency and extent of wildfires in those regions is the most important and is difficult to retrieve from ice cores. The changes seen in wildfires between the preindustrial and, for example, Last Glacial Maximum are as large as if not larger than those seen between the preindustrial and present day (Fig. 6). These studies qualitatively suggest that the changes we have seen in fires over the preindustrial to present day are not unprecedented in size but rather are similar to paleoclimate changes.
In addition, paleoclimate data such as temperature changes or aerosol changes are relative to preindustrial changes, and as discussed in Sect. 2 and shown in Fig. 5, there is substantial variability in preindustrial fires. It is unclear what value to use for preindustrial aerosol emissions to compare to paleodata values: do we use 1850 values or values from the Little Ice Age? Or some average? One can think of the variability in changes in emissions between some time (T) and PI as being shown in Eq. (4). The uncertainties in emissions in PI are driving the uncertainties in PD–PI described above and are about 2.8 W m−2 (): since these uncertainties are due to variability not a lack of information, increased knowledge is unlikely to reduce these uncertainties (only if new studies show less variability). In addition, the changes in emissions between PI and any other time period are likely to be similarly large but may not be orthogonal (). Adding these together (using Eq. 4), we obtain = 4.0 W m−2. Note that the uncertainties in emissions proposed here for different time periods could be constrained to some extent (), but uncertainties due to the variability in PI emissions (Fig. 5) would be difficult to constrain, and there may be substantial variability as well as uncertainty in emissions during different time periods, so the values proposed here may actually underestimate the uncertainty.
Once we have paleo-proxies to provide data about changes in fire emissions, we can constrain the emission uncertainties for paleo-time periods relative to the present day, hopefully. Unfortunately knowing the emissions does not translate into knowing the radiative forcing in past times, as we known from our experience simulating preindustrial to present-day emission changes in existing models using the same emissions (Bellouin et al., 2020). There are uncertainties of translating these changes in emissions into changes in direct radiative and aerosol–cloud interactions or process uncertainties, which we assume here, since we do not have better information, are similar in size to present-day to preindustrial uncertainties ( = 2.8 W m−2). These uncertainties are due to differences in the modeling of aerosols as well as assumptions about size and how aerosols interact with clouds, which can be different depending on where the aerosols are emitted: this uncertainty will remain in paleoclimates and might even become larger, since the aerosol size, composition, and mixing state could be quite different and the very important impact of aerosols on clouds is sensitive to background conditions (Carslaw et al., 2017). Fires from different ecosystems, or even different types of fires in the same ecosystems, have very different emissions of black carbon, organic carbon, and sulfate and thus different effects, but we do not know how these will change in different time periods. Natural aerosols are the source of much of the uncertainty in today's climate compared to anthropogenic aerosols because of the difficulty of estimating the exact timing and distribution of emissions, as well as the fact that the sources are more complicated in composition and location (e.g., Mahowald et al., 2011b; Carslaw et al., 2017; Rathod et al., 2020). Similar to the present day relative to preindustrial, we can estimate the paleo-time to preindustrial radiative forcing uncertainty using Eq. (3) and obtain 4.8 W m−2 as the range of uncertainty for paleo-time periods (note that if we can constrain the change in radiative forcing from changing emissions of aerosols to be smaller than that between the preindustrial and present day using observations and Eq. 2, it is possible we could proportionately reduce the uncertainty in radiative forcing from aerosols from process uncertainties; see Eq. 2) Converting this radiative forcing uncertainty into a feedback uncertainty requires knowing the temperature change, which is also uncertain, but if we use 3∘C as a reasonable value, the aerosol feedback uncertainty derived from the Last Glacial Maximum to PI is ±1.6 W m−2 K−1, similar to the value derived from the PD–PI time period. If we add in the uncertainty in temperature change between the preindustrial and Last Glacial Maximum, this estimate would be even larger, of course.
It seems likely that aerosol emissions from fires during the Last Glacial Maximum are much smaller than preindustrial or present-day values, while estimates suggest that dust is ∼3 times larger in the Last Glacial Maximum than the present day. Will these changes in aerosols balance out? That is unlikely but vital to consider. Dust is by mass the most important aerosol in the atmosphere and contributes substantially to direct forcing and ice nucleation processes, but fire emissions are important for liquid aerosol–cloud processes (Mahowald et al., 2011a; Carslaw et al., 2010). Because of the nonlinearity in aerosol–cloud interactions, small changes in fire emissions in pristine environments, like the Last Glacial Maximum, might be even more important than estimated here (Carslaw et al., 2017). Understanding the aerosol interactions with clouds, especially for the Last Glacial Maximum, is both important and intriguing.
Since today the largest uncertainties in the radiative forcing come from aerosol uncertainties, estimates in past climates should ensure that aerosol uncertainties remain one of the largest uncertainties in those times as well: how could we know the change in aerosols from some paleoclimate to the preindustrial better than we know the change in aerosol forcing between the preindustrial and present day? If ice sheets, insolation, or continental distributions are different and causing large changes in top-of-atmosphere fluxes and thus climate regime, most likely aerosol changes are equally large, but we do not know in what direction. More analysis might result in even larger changes in radiative forcing and its uncertainty in some time periods, since here we are assuming, without prior information, that the radiative forcing of any paleo-time period relative to the preindustrial is around 0.0 W m−2. For the LGM, for example, if we only include dust, a more negative value should be chosen as the mean, since we have evidence of increased dust in the paleorecord (Albani et al., 2018). On the other hand, the limited data suggest that fires have substantially decreased relative to the preindustrial, which would warm the climate. It is beyond the scope of this opinion piece to characterize the central estimate, but rather here we just point out the many uncertainties in these estimates.
So far here we have focused on the more frequently studied processes of aerosol direct radiative effects and aerosol–cloud interactions with an emphasis on cloud condensation nuclei. However, substantial uncertainties remain in these aerosol radiative effects even in the current climate (Bellouin et al., 2020; Li et al., 2022) Aerosol is spatially and temporally heterogeneous in composition, size, and amount, leading to vastly different physical and chemical properties. They are in general poorly observed compared to meteorological phenomenon (e.g., Naik et al., 2021). It is not only the bulk composition that matters, but the details of the mixing state and size are also vital for radiative and cloud interactions (Matsui et al., 2018; Bond et al., 2013; Li et al., 2022, 2021). In addition, in preindustrial times, the impact of aerosols, for example on cloud properties, can be different than in the present day because of a lower background aerosol amount (Carslaw et al., 2017; Hamilton et al., 2014).
The impacts of large changes in important ice nuclei such as dust or primary biogenic particles is likely to be large but has yet to be fully assessed (Burrows et al., 2013; Murray et al., 2021; Storelvmo, 2017). Another important feedback that is relatively well known but not included in most climate models is due to nitrogen aerosols such as ammonium or nitrate (e.g., Bauer et al., 2007; Paulot et al., 2016). Future concentrations of aerosols derived from land use practices such as ammonia or nitrate are not likely to decrease as quickly as those from fossil fuels (Gidden et al., 2019). Indeed, as sulfate is phased out, more nitric acid will form nitrate aerosols (due to higher pH), partially buffering decreases in aerosol optical depth (AOD) (Paulot et al., 2016; Pye et al., 2009). Including better parameterizations of ice-nucleating particles and nitrogen aerosols is key to improving future aerosol projections.
In addition, aerosols can provide nutrients and pollutants to different ecosystems (Mahowald et al., 2017; Hamilton et al., 2021), linking aerosol changes to changes in biogeochemistry and the carbon cycle. These effects could potentially be quite large (0.5 ± 0.4 W m−2) (Mahowald, 2011) but are poorly constrained and do not explicitly appear in the standard radiative forcing diagram, since they reflect CO2 that is not in the atmosphere but could have been (Mahowald, 2011).
Another natural emission to which estimates of radiative forcing are sensitive is biogenic volatile organic compounds (BVOCs). BVOCs are a major source of new aerosol particles in the atmosphere (Guenther et al., 2006; Arneth et al., 2010). Furthermore, biogenic particle formation processes contributed more to the aerosol burden in the PI than the PD (Gordon et al., 2016). Estimates of the radiative forcing of BVOCs are sensitive to how well characterized new particle formation processes are in a model. The recent addition of an organic particle formation pathway, which occurs in the absence of SO2 (such the PI), results in a more increased aerosol burden in the past than the present. Once more increasing the PI aerosol burden reduces the estimate of the aerosol forcing over the historical period, this time by reducing the cloud forcing by ∼ 0.2 W m−2 (Zhu et al., 2019).
Aerosol radiative forcing and its uncertainty are used extensively in climate change science, including to constrain climate sensitivity (Sherwood et al., 2020) and thereby future climate changes (IPCC, 2021; Gulev et al., 2021; Szopa et al., 2021). Because the published aerosol uncertainties tend not to include poorly constrained uncertainties such as discussed here, this information is not effectively passed to physical climate scientists who use these estimates. For example, a recent review of climate sensitivity (Sherwood et al., 2020) focused on using independent methods to reduce uncertainty in climate sensitivity. In that paper, aerosol radiative forcing uncertainties for different time periods are mentioned in several different places. They use the unconstrained model range of the aerosol radiative forcing obtained by Bellouin et al. (2020), which as discussed above, does not account for emission uncertainties. Paleoclimate constraints are often used for constraining climate sensitivity, as discussed in Sherwood et al. (2020). Currently estimates exist for dust aerosol radiative forcing changes between the Last Glacial Maximum and the present, which is included in Sherwood et al. (2020) as −1.0 ± 1.6 W m−2 (90 % confidence intervals: they report 1σ values of ±1 W m−2 in Sherwood et al., 2020, which are converted to 90 % confidence here by multiplying by 1.6 as a first estimate), but no mention is made of the potential for changes in the more important wildfires. As discussed in Sect. 4, estimates for radiative forcing of aerosols for paleo-time periods, especially wildfires, are missing but should be estimated to be 0.0 ± 2.4 W m−2 (90 % confidence interval). In addition, the aerosol feedback within the system is assumed in Sherwood et al. (2020) to have an uncertainty of ±0.22 W m−2 (they report 1σ values of ±0.15 W m−2 in Sect. 3.2, which we convert to 90 % confidence intervals), whereas here we estimated the aerosol feedback uncertainty to be ±1.6 W m−2 (90 % confidence), substantially larger. Including more realistic aerosol uncertainties in estimates of climate sensitivity should be done to ensure adequate propagation of errors, although they are unlikely to change the central estimates (Sherwood et al., 2020).
In addition, some authors argue that there were not significant changes in aerosol radiative forcing during the 1970s and 1980s using standard CMIP6-type estimates and try to estimate climate sensitivity in this time period (Jiménez-de-la-Cuesta and Mauritsen, 2019). As noted above, however, the 1970s is a time period of Sahel drought, and dust radiative forcing between the 1960s and 1980s changed by perhaps −0.57 ± 0.46 W m−2 (Mahowald et al., 2010), suggesting that is not an ideal time period to target. One should add to this estimate the possibly important changes in wildfires which could have occurred over this time period but for which we do not have data.
In addition to climate sensitivity, some studies use the CMIP6 simulations to constrain past aerosol radiative forcing changes (e.g., Smith et al., 2021). Since the simulations do not include different spatial and temporal uncertainties in emissions, they do not include the real uncertainty in the aerosol forcing. Other studies use CMIP6 or similar simulations to attribute the change in temperatures or precipitation to different forcings (e.g., Biasutti and Giannini, 2006; Undorf et al., 2018; Hegerl et al., 2019), and these attempts to attribute changes could be based on a poor representation of the real uncertainty of the aerosol forcing in the preindustrial. Aerosol radiative forcing uncertainty cannot be constrained easily by global surface temperature time series, since other uncertainties can be difficult to disentangle from aerosol uncertainties (Kiehl, 2007; Lee et al., 2016). Attributing climate at a regional scale is the next frontier of detection and attribution, but this cannot be done without accurate aerosol histories (Lehner and Coats, 2021).
In summary, we argue that it is critical that the full uncertainty deduced in the aerosol literature, including due to changes in natural aerosols, be passed to physical climate scientists so that they can accurately account for these in constraints on climate sensitivity and in projections of future climate changes.
How can we address the systematic underestimate in the uncertainty of changes in aerosol radiative effects between different time periods? Here we propose some steps towards first characterizing the true uncertainties, including emission uncertainties, and then using observations to constrain these aerosol pathways to constrain the radiative forcing and uncertainties.
- a.
Characterize historical uncertainties in aerosol and aerosol precursor emissions using available knowledge of emissions and how they might have changed. These estimates should include some versions which are consistent with available paleodata (e.g., for dust Kok et al., 2023; and for wildfires Hamilton et al., 2018, and Liu et al., 2021). The uncertainties from emissions should be combined with uncertainties in aerosol processes to create a more robust uncertainty bound for different time periods. Note that many “natural” aerosols are prognostic in the models (e.g., dust), and therefore in order to match available scenarios, prognostic aerosol schemes may need to be corrected using a temporally varying emission factor to simulate the correct temporal trends (e.g., Mahowald et al., 2010).
- b.
Characterize paleoclimate emissions of aerosols and the resulting radiative forcing at important past climates, such as the Last Glacial Maximum and last interglacial. These estimates should be based as much as possible on observations and possible ranges.
- c.
We propose a new intercomparison project (AEROHISTMIP) which would include multiple emission pathways in the historical model simulations conducted for CMIP exercises. Note that developing these new emission pathways is likely to be beyond the capabilities of one or two groups and will thus require a workshop or other community activity. Several Earth system models should not only conduct ensemble members of one aerosol emission scenario, but also use multiple aerosol emission scenarios to better understand the uncertainty in aerosol radiative forcing and climate response due to the uncertainties in aerosol emissions in different time periods. The evolution of several related past model intercomparison projects under one umbrella (e.g., Composition, Air quality, Climate inTeractions Initiative: CACTI) provides the ideal opportunity to now include such simulations.
- d.
Constrain preindustrial to present-day aerosol radiative effects. From (c), combined with observations (a), the most likely past emission scenarios can be identified, and we can make the first steps towards constraining uncertainty, similar to the efforts underway to characterize which of the climate models are most reliable (e.g., IPCC, 2021). Any inability of existing models to simulate observations, as well as other remaining uncertainties, should be carefully assessed: it may not be possible for the models to simulate the observed changes.
- e.
Obtain more paleoclimate proxies for aerosol concentrations. Here we have focused mostly on wildfires and dust, since there are enough paleoclimate data to show that CMIP6 does not represent historical changes in these aerosols well, but indeed it is not currently possible to validate the changes in emissions for other natural (e.g., sea spray) and anthropogenic (e.g., sulfate) aerosols as well. We need the development of more proxies for historical and paleoclimate changes in aerosols to increase confidence in our estimates of aerosol radiative forcing.
- f.
Continue to improve aerosol measurement databases, including more in situ observations of the aerosol composition in more locations (Snider et al., 2016), as well as continued use of satellite observations to constrain the magnitude of aerosol radiative forcing (e.g., Smith et al., 2021). We encourage more observations for variables directly related to the radiative forcing (e.g., aerosol optical depth) and those that could help narrow uncertainties in crucial parameters that describe related physiochemical processes. Some of these data can also be used to assess the model performance, narrow the model spread, and validate satellite retrievals. As aerosol number concentration, the determinant of the change in cloud properties as a function of emission changes, cannot be retrieved from paleo-proxies (d) there needs to be a simultaneous effort in understanding natural aerosol processes and impacts on clouds under pristine “PI-like” present-day conditions (Hamilton et al., 2014; McCoy et al., 2020)
- g.
Characterize current model direct aerosol radiative effects and aerosol–cloud interactions using new tools. Currently meteorological models are tightly connected to the aerosol models they host, making it difficult to independently evaluate the structural differences between aerosol models. Recent efforts to develop generalized chemical and aerosol interfaces would allow more effective evaluation of chemical and aerosol schemes separate from their host models (Hodzic et al., 2023). A generalized framework could also allow artificial intelligence methods to be integrated into multiple models and model–data comparisons or assimilations to be used across models.
In summary, while there has been substantial progress in aerosol–climate science over the past 20 years, aerosols remain one of the most important uncertainties in climate change science and are likely to continue to be important to study for at least the next 20 years.
No data sets were used in this article.
NMM conceived of the idea and created the first draft, with substantial improvements from LL, SA, DSH, and JFK.
The contact author has declared that none of the authors has any competing interests.
Publisher's note: Copernicus Publications remains neutral with regard to jurisdictional claims made in the text, published maps, institutional affiliations, or any other geographical representation in this paper. While Copernicus Publications makes every effort to include appropriate place names, the final responsibility lies with the authors.
This article is part of the special issue “20 years of Atmospheric Chemistry and Physics”. It is not associated with a conference.
Natalie Mahowald and Longlei Li would like to acknowledge support from DOE DE-SC0021302. Jasper F. Kok acknowledges support from NSF grants 1856389 and 2151093. We acknowledge helpful discussions with Flavio Lehner and Steve Sherwood.
This research has been supported by the DOE (grant no. DE SC0021302) and NSF (grant nos. ATM 1856389 and 2151093).
This paper was edited by Ken Carslaw and James Allan and reviewed by two anonymous referees.
Albani, S. and Mahowald, N. M.: Paleodust insights onto dust impacts on climate, J. Climate, 32, 7897–7913, https://doi.org/10.1175/jcli-d-18-0742.1, 2019.
Albani, S., Mahowald, N. M., Perry, A. T., Scanza, R. A., Zender, C. S., Heavens, N. G., Maggi, V., Kok, J. F., and Otto-Bliesner, B. L.: Improved dust representation in the Community Atmosphere Model, J. Adv. Model. Earth Syst., 6, 541–570, https://doi.org/10.1002/2013MS000279, 2014.
Albani, S., Mahowald, N. M., Winckler, G., Anderson, R. F., Bradtmiller, L. I., Delmonte, B., François, R., Goman, M., Heavens, N. G., Hesse, P. P., Hovan, S. A., Kang, S. G., Kohfeld, K. E., Lu, H., Maggi, V., Mason, J. A., Mayewski, P. A., McGee, D., Miao, X., Otto-Bliesner, B. L., Perry, A. T., Pourmand, A., Roberts, H. M., Rosenbloom, N., Stevens, T., and Sun, J.: Twelve thousand years of dust: the Holocene global dust cycle constrained by natural archives, Clim. Past, 11, 869–903, https://doi.org/10.5194/cp-11-869-2015, 2015.
Albani, S., Balkanski, Y., Mahowald, N., Winckler, G., Maggi, V., and Delmonte, B.: Aerosol-Climate Interactions During the Last Glacial Maximum, Curr. Clim. Change Rep., 4, 99–114, https://doi.org/10.1007/s40641-018-0100-7, 2018.
Allen, M. R., Fuglestvedt, J. S., Shine, K. P., Reisinger, A., Pierrehumbert, R. T., and Forster, P. M.: New use of global warming potentials to compare cumulative and short-lived climate pollutants, Nat. Clim. Change, 6, 773–776, https://doi.org/10.1038/NCLIMATE2998, 2016.
Allen, M. R., Dube, O. P., Solecki, W., Aragón-Durand, F., Cramer, W., Humphreys, S., Kainuma, M., Kala, J., Mahowald, N., Mulugetta, Y., Perez, R., Wairiu, M., and Zickfeld, K.: Framing and Context, in: Global Warming of 1.5 ∘C. An IPCC Special Report on the impacts of global warming of 1.5 ∘C above pre-industrial levels and related global greenhouse gas emission pathways, in the context of strengthening the global response to the threat of climate change, edited by: Masson-Delmotte, V., Zhai, P., Pörtner, H.-O., Roberts, D., Skea, J., Shukla, P. R., Pirani, A., Moufouma-Okia, W., Péan, C., Pidcock, R., Connors, S., Matthews, J. B. R., Chen, Y., Zhou, X., Gomis, M. I., Lonnoy, E., Maycock, T., Tignor, M., and Waterfield, T.: World Meteorological Organization (WMO), Geneva, Switzerland, 45–91, https://doi.org/10.1017/9781009157940.003, 2018.
Andela, N., Morton, D. C., Giglio, L., Chen, Y., Van Der Werf, G. R., Kasibhatla, P. S., DeFries, R. S., Collatz, G. J., Hantson, S., Kloster, S., Bachelet, D., Forrest, M., Lasslop, G., Li, F., Mangeon, S., Melton, J. R., Yue, C., and Randerson, J. T.: A human-driven decline in global burned area, Science, 356, 1356–1362, https://doi.org/10.1126/science.aal4108, 2017.
Arienzo, M. M., McConnell, J. R., Murphy, L. N., Chellman, N., Das, S., Kipfstuhl, S., and Mulvaney, R.: Holocene black carbon in Antarctica paralleled Southern Hemisphere climate, J. Geophys. Res., 122, 6713–6728, https://doi.org/10.1002/2017JD026599, 2017.
Arneth, A., Harrison, S., Zaehle, S., Tsigaridis, K., Menon, S., Bartlein, P., Feichter, J., Korhola, A., Kulmala, M., O'Donnell, D., Schurgers, G., Sorvari, S., and Vesala, T.: Terrestrial biogeochemical feedbacks in the climate system, Nat. Geosci., 3, 525–532, https://doi.org/10.1038/NGEO905, 2010.
Bauer, S. E., Koch, D., Unger, N., Metzger, S. M., Shindell, D. T., and Streets, D. G.: Nitrate aerosols today and in 2030: a global simulation including aerosols and tropospheric ozone, Atmos. Chem. Phys., 7, 5043–5059, https://doi.org/10.5194/acp-7-5043-2007, 2007.
Bellouin, N., Quaas, J., Gryspeerdt, E., Kinne, S., Stier, P., Watson-Parris, D., Boucher, O., Carslaw, K. S., Christensen, M., Daniau, A. L., Dufresne, J. L., Feingold, G., Fiedler, S., Forster, P., Gettelman, A., Haywood, J. M., Lohmann, U., Malavelle, F., Mauritsen, T., McCoy, D. T., Myhre, G., Mülmenstädt, J., Neubauer, D., Possner, A., Rugenstein, M., Sato, Y., Schulz, M., Schwartz, S. E., Sourdeval, O., Storelvmo, T., Toll, V., Winker, D., and Stevens, B.: Bounding Global Aerosol Radiative Forcing of Climate Change, Rev. Geophys., 51, e2019RG000660, https://doi.org/10.1029/2019RG000660, 2020.
Biasutti, M. and Giannini, A.: Robust Sahel drying in response to late 20th century forcings, Geophys. Res. Lett., 33, L11706, https://doi.org/10.1029/2006GL026067, 2006.
Bistinas, I., Oom, D., Sá, A. C. L., Harrison, S. P., Prentice, I. C., and Pereira, J. M. C.: Relationships between human population density and burned area at continental and global scales, PLoS One, 8, 1–12, https://doi.org/10.1371/journal.pone.0081188, 2013.
Bond, T., Bhardwaj, E., Dong, R., Jogani, R., Jung, S., Roden, C., Streets, D., and Trautman, N.: Historical emissions of black and organic carbon aerosol from energy-related combustion, 1850–2000, Global Biogeochem. Cy., 21, GB2018, https://doi.org/10.1029/2006GB002840, 2007.
Bond, T., Doherty, S. J., Fahey, D., Forster, P., Bernsten, T., DeAngelo, B., Flanner, M., Ghan, S., Karcher, B., Koch, D., Kinne, S., Kondo, Y., Quinn, P. K., Sarofim, M., Schultz, M., Venkataraman, C., Zhang, H., Zhang, S., Bellouin, N., Guttikunda, S., Hopke, P., Jacobson, M., Kaiser, J. W., Klimont, Z., Lohman, U., Schwartz, J., Shindel, D., Storelvmo, T., Warren, S., and Zender, C.: Bounding the role of black carbon in the climate system: A scientific assessment, J. Geophys. Res., D118, 5380–5552, https://doi.org/10.1002/jgrd.50171, 2013.
Bowman, D. M. J. S., Artaxo, P., Bond, W. J., Carlson, J. M., Cochrane, M., D-Antoio, C., DeFries, R. S., Doyle, J. C., Harrison, S. P., Johnson, F., Keeley, J. E., Krawchuk, M. a, Kull, C. a, MArtson, J., Mortiz, M., Prentice, I. C., Roos, C. I., Scott, A. C., Swetnam, T. W., van der Werf, G., Pyne, S. J., Blach, J., Bowman, D. M. J. S., Balch, J. K., Artaxo, P., Bond, W. J., Carlson, J. M., Cochrane, M. a, D'Antonio, C. M., DeFries, R. S., Doyle, J. C., Harrison, S. P., Johnston, F. H., Keeley, J. E., Krawchuk, M. a, Kull, C. a, Marstone, J., Moritz, M. a, Prentice, C., Roos, C. I., Scott, A. C., Swetnam, T. W., van der Werf, G. R., Pyne, S. J., Marston, J. B., Moritz, M. a, Prentice, I. C., Roos, C. I., Scott, A. C., Swetnam, T. W., van der Werf, G. R., and Pyne, S. J.: Fire in the Earth System, Science, 324, 481–484, https://doi.org/10.1126/science.1163886, 2009.
Braconnot, P., Albani, S., Balkanski, Y., Cozic, A., Kageyama, M., Sima, A., Marti, O., and Peterschmitt, J.-Y.: Impact of dust in PMIP-CMIP6 mid-Holocene simulations with the IPSL model, Clim. Past, 17, 1091–1117, https://doi.org/10.5194/cp-17-1091-2021, 2021.
Burnett, R., Chen, H., Szyszkowicz, M., Fann, N., Hubbell, B., Pope, C. A., Apte, J. S., Brauer, M., Cohen, A., Weichenthal, S., Coggins, J., Di, Q., Brunekreef, B., Frostad, J., Lim, S. S., Kan, H., Walker, K. D., Thurston, G. D., Hayes, R. B., Lim, C. C., Turner, M. C., Jerrett, M., Krewski, D., Gapstur, S. M., Diver, W. R., Ostro, B., Goldberg, D., Crouse, D. L., Martin, R. V., Peters, P., Pinault, L., Tjepkema, M., Van Donkelaar, A., Villeneuve, P. J., Miller, A. B., Yin, P., Zhou, M., Wang, L., Janssen, N. A. H., Marra, M., Atkinson, R. W., Tsang, H., Thach, T. Q., Cannon, J. B., Allen, R. T., Hart, J. E., Laden, F., Cesaroni, G., Forastiere, F., Weinmayr, G., Jaensch, A., Nagel, G., Concin, H., and Spadaro, J. V.: Global estimates of mortality associated with longterm exposure to outdoor fine particulate matter, P. Natl. Acad. Sci. USA, 115, 9592–9597, https://doi.org/10.1073/pnas.1803222115, 2018.
Burrows, S. M., Hoose, C., Pöschl, U., and Lawrence, M. G.: Ice nuclei in marine air: biogenic particles or dust?, Atmos. Chem. Phys., 13, 245–267, https://doi.org/10.5194/acp-13-245-2013, 2013.
Carslaw, K. S., Boucher, O., Spracklen, D. V., Mann, G. W., Rae, J. G. L., Woodward, S., and Kulmala, M.: A review of natural aerosol interactions and feedbacks within the Earth system, Atmos. Chem. Phys., 10, 1701–1737, https://doi.org/10.5194/acp-10-1701-2010, 2010.
Carslaw, K. S., Gordon, H., Hamilton, D. S., Johnson, J. S., Regayre, L. A., Yoshioka, M., and Pringle, K. J.: Aerosols in the Pre-industrial Atmosphere, Curr. Clim. Change Rep., 3, 1–15, https://doi.org/10.1007/s40641-017-0061-2, 2017.
Cusworth, D. H., Mickley, L. J., Sulprizio, M. P., Liu, T., Marlier, M. E., Defries, R. S., Guttikunda, S. K., and Gupta, P.: Quantifying the influence of agricultural fires in northwest India on urban air pollution in Delhi, India, Environ. Res. Lett., 13, 044018, https://doi.org/10.1088/1748-9326/aab303, 2018.
Dentener, F., Kinne, S., Bond, T., Boucher, O., Cofala, J., Generoso, S., Ginoux, P., Gong, S., Hoelzemann, J. J., Ito, A., Marelli, L., Penner, J. E., Putaud, J.-P., Textor, C., Schulz, M., van der Werf, G. R., and Wilson, J.: Emissions of primary aerosol and precursor gases in the years 2000 and 1750 prescribed data-sets for AeroCom, Atmos. Chem. Phys., 6, 4321–4344, https://doi.org/10.5194/acp-6-4321-2006, 2006.
Evan, A., Flamant, C., Gaetani, M., and Guichard, F.: The past, present and future of African dust, Nature, 531, 493–495, https://doi.org/10.1038/nature17149, 2016.
Ferretti, D. F., Miller, J. B., White, J. W. C., Etheridge, D. M., Lassey, K. R., Lowe, D. C., Meure, C. M. M., Dreier, M. F., Trudinger, C. M., Van Ommen, T. D., and Langenfelds, R. L.: Unexpected Changes to the Global Methane Budget over the Past 2000 Years, Science, 309, 1714–1717, https://doi.org/10.1126/science.1115193, 2005.
Fischer, H., Schüpbach, S., Gfeller, G., Bigler, M., Röthlisberger, R., Erhardt, T., Stocker, T. F., Mulvaney, R., and Wolff, E. W.: Millennial changes in North American wildfire and soil activity over the last glacial cycle, Nat. Geosci., 8, 723–727, https://doi.org/10.1038/ngeo2495, 2015.
Forster, P., Alterskjaer, K., Smith, C., Colman, R., Damon Matthews, H., Ramaswamy, V., Storelvmo, T., Armour, K., Collins, W., Dufresne, J., Frame, D., Lunt, D., Mauritsen, T., Watanabe, M., Wild, M., Zhang, H., Pirani, A., Connors, S., Péan, C., Berger, S., Caud, N., Chen, Y., Goldfarb, L., Gomis, M., Huang, M., Leitzell, K., Lonnoy, E., Matthews, J., Maycock, T., Waterfield, T., Yelekçi, O., Yu, R., and Zhou, B.: Chapter 7: The Earth's Energy Budget, Climate Feedbacks and Climate Sensitivity, in: Climate Change 2021: The Physical Science Basis. Contribution of Working Group I to the Sixth Assessment Report of the Intergovernmental Panel on Climate Change, edited by: Masson-Delmotte, V., Zhai, P., Pirani, A., Connors, S. L., Péan, C., Berger, S., Caud, N., Chen, Y., Goldfarb, L., Gomis, M. I., Huang, M., Leitzell, K., Lonnoy, E., Matthews, J. B. R., Maycock, T. K., Waterfield, T., Yelekçi, O., Yu, R., and Zhou, B., Cambridge University Press, Cambridge, United Kingdom and New York, NY, USA, 923–1054, https://doi.org/10.1017/9781009157896.009, 2021.
Gidden, M. J., Riahi, K., Smith, S. J., Fujimori, S., Luderer, G., Kriegler, E., van Vuuren, D. P., van den Berg, M., Feng, L., Klein, D., Calvin, K., Doelman, J. C., Frank, S., Fricko, O., Harmsen, M., Hasegawa, T., Havlik, P., Hilaire, J., Hoesly, R., Horing, J., Popp, A., Stehfest, E., and Takahashi, K.: Global emissions pathways under different socioeconomic scenarios for use in CMIP6: a dataset of harmonized emissions trajectories through the end of the century, Geosci. Model Dev., 12, 1443–1475, https://doi.org/10.5194/gmd-12-1443-2019, 2019.
Ginoux, P., Prospero, J., Gill, T. E., Hsu, N. C., and Zhao, M.: Global scale attribution of anthropogenic and natural dust sources and their emission rates based on MODIS deep blue aerosol products, Rev. Geophys., 50, RG3005, https://doi.org/10.1029/2012RG000388, 2012.
Gordon, H., Sengupta, K., Rap, A., Duplissy, J., Frege, C., Williamson, C., Heinritzi, M., Simon, M., Yan, C., Almeida, J., Tröstl, J., Nieminen, T., Ortega, I. K., Wagner, R., Dunne, E. M., Adamov, A., Amorim, A., Bernhammer, A.-K., Bianchi, F., Breitenlechner, M., Brilke, S., Chen, X., Craven, J. S., Dias, A., Ehrhart, S., Fischer, L., Flagan, R. C., Franchin, A., Fuchs, C., Guida, R., Hakala, J., Hoyle, C. R., Jokinen, T., Junninen, H., Kangasluoma, J., Kim, J., Kirkby, J., Krapf, M., Kürten, A., Laaksonen, A., Lehtipalo, K., Makhmutov, V., Mathot, S., Molteni, U., Monks, S. A., Onnela, A., Peräkylä, O., Piel, F., Petäjä, T., Praplan, A. P., Pringle, K. J., Richards, N. A. D., Rissanen, M. P., Rondo, L., Sarnela, N., Schobesberger, S., Scott, C. E., Seinfeld, J. H., Sharma, S., Sipilä, M., Steiner, G., Stozhkov, Y., Stratmann, F., Tomé, A., Virtanen, A., Vogel, A. L., Wagner, A. C., Wagner, P. E., Weingartner, E., Wimmer, D., Winkler, P. M., Ye, P., Zhang, X., Hansel, A., Dommen, J., Donahue, N. M., Worsnop, D. R., Baltensperger, U., Kulmala, M., Curtius, J., and Carslaw, K. S.: Reduced anthropogenic aerosol radiative forcing caused by biogenic new particle formation, P. Natl. Acad. Sci. USA, 113, 12053–12058, https://doi.org/10.1073/pnas.1602360113, 2016.
Guenther, A., Karl, T., Harley, P., Wiedinmyer, C., Palmer, P. I., and Geron, C.: Estimates of global terrestrial isoprene emissions using MEGAN (Model of Emissions of Gases and Aerosols from Nature), Atmos. Chem. Phys., 6, 3181–3210, https://doi.org/10.5194/acp-6-3181-2006, 2006.
Gulev, S. K., Thorne, P. W., Ahn, J., Dentener, F. J., Domingues, C. M., Gerland, S., Gong, D., Kaufman, D. S., Nnamchi, H. C., Quaas, J., Rivera, J. A., Sathyendranath, S., Smith, S. L., Trewin, B., von Schuckmann, K., and Vose, R. S.: Changing State of the Climate System, in: Climate Change 2021: The Physical Science Basis. Contribution of Working Group I to the Sixth Assessment Report of the Intergovernmental Panel on Climate Change, edited by: Masson-Delmotte, V., Zhai, P., Pirani, A., Connors, S. L., Péan, C., Berger, S., Caud, N., Chen, Y., Goldfarb, L., Gomis, M. I., Huang, M., Leitzell, K., Lonnoy, E., Matthews, J. B. R., Maycock, T. K., Waterfield, T., Yelekçi, O., Yu, R., and Zhou, B., Cambridge University Press, Cambridge, United Kingdom and New York, NY, USA, 287–422, https://doi.org/10.1017/9781009157896.004, 2021.
Hamilton, D. S., Lee, L. A., Pringle, K. J., Reddington, C. L., Spracklen, D. V., and Carslaw, K. S.: Occurrence of pristine aerosol environments on a polluted planet, P. Natl. Acad. Sci. USA, 111, 18466–18471, https://doi.org/10.1073/pnas.1415440111, 2014.
Hamilton, D. S., Hantson, S., Scott, C. E., Kaplan, J. O., Pringle, K. J., Nieradzik, L. P., Rap, A., Folberth, G. A., Spracklen, D. V., and Carslaw, K. S.: Reassessment of pre-industrial fire emissions strongly affects anthropogenic aerosol forcing, Nat. Commun., 9, 3182, https://doi.org/10.1038/s41467-018-05592-9, 2018.
Hamilton, D. S., Perron, M. M. G., Bond, T. C., Bowie, A. R., Buchholz, R. R., Guieu, C., Ito, A., Maenhaut, W., Myriokefalitakis, S., Olgun, N., Rathod, S. D., Schepanski, K., Tagliabue, A., Wagner, R., and Mahowald, N. M.: Earth, Wind, Fire, and Pollution: Aerosol Nutrient Sources and Impacts on Ocean Biogeochemistry, 14, 303–330, https://doi.org/10.1146/annurev-marine-031921-013612, 2021.
Hegerl, G. C., Brönnimann, S., Cowan, T., Friedman, A. R., Hawkins, E., Iles, C., Müller, W., Schurer, A., and Undorf, S.: Causes of climate change over the historical record, Environ. Res., 14, 123006, https://doi.org/10.1088/1748-9326/ab4557, 2019.
Hodzic, A., Mahowald, N., Dawson, M., Johnson, J., Bernardet, L., Bosler, P. A., Clune, T., Fast, J. D., Fierce, L., Liu, X., Ma, P.-L., Murphy, B., Riemer, N., and Schulz, M.: GeneralIzed Aerosol/chemistry iNTerface (GIANT): a community effort to advance collaborative science across weather and climate models, B. Am. Meteorol. Soc., 104, E2065, https://doi.org/10.1175/BAMS-D-23-0013.1, 2023.
Hoesly, R. M., Smith, S. J., Feng, L., Klimont, Z., Janssens-Maenhout, G., Pitkanen, T., Seibert, J. J., Vu, L., Andres, R. J., Bolt, R. M., Bond, T. C., Dawidowski, L., Kholod, N., Kurokawa, J.-I., Li, M., Liu, L., Lu, Z., Moura, M. C. P., O'Rourke, P. R., and Zhang, Q.: Historical (1750–2014) anthropogenic emissions of reactive gases and aerosols from the Community Emissions Data System (CEDS), Geosci. Model Dev., 11, 369–408, https://doi.org/10.5194/gmd-11-369-2018, 2018.
Hooper, J. and Marx, S.: A global doubling of dust emissions during the Anthropocene?, Glob. Planet. Change, 169, 70–91, https://doi.org/10.1016/j.gloplacha.2018.07.003, 2018.
IPCC: Summary for Policymakers, in: IPCC Special Report on the Ocean and Cryosphere in a Changing Climate, edited by: Pörtner, H.-O., Roberts, D. C., Masson-Delmotte, V., Zhai, P., Tignor, M., Poloczanska, E., Mintenbeck, K., Alegría, A., Nicolai, M., Okem, A., Petzold, J., Rama, B., and Weyer, N. M., https://doi.org/10.1017/9781009157964.001, 2019.
IPCC: Summary for Policymakers, in: Climate Change 2021: The Physical Science Basis. Contribution of Working Group I to the Sixth Assessment Report of the Intergovernmental Panel on Climate Change, edited by: Masson-Delmotte, V., Zhai, P., Pirani, A., Connors, S. L., Péan, C., Berger, S., Caud, N., Chen, Y., Goldfarb, L., Gomis, M. I., Huang, M., Leitzell, K., Lonnoy, E., Matthews, J. B. R., Maycock, T. K., Waterfield, T., Yelekçi, O., Yu, R., and Zhou, B., Cambridge University Press, Cambridge, UK, 3–32, https://doi.org/10.1017/9781009157896.001, 2021.
Jiménez-de-la-Cuesta, D. and Mauritsen, T.: Emergent constraints on Earth's transient and equilibrium response to doubled CO2 from post-1970s global warming, Nat. Geosci., 12, 902–905, https://doi.org/10.1038/s41561-019-0463-y, 2019.
Jones, M. W., Abatzoglou, J. T., Veraverbeke, S., Andela, N., Lasslop, G., Forkel, M., Smith, A. J. P., Burton, C., Betts, R. A., van der Werf, G. R., Sitch, S., Canadell, J. G., Santín, C., Kolden, C., Doerr, S. H., and Le Quéré, C.: Global and regional trends and drivers of fire under climate change, Rev. Geophys., 60, e2020RG000726, https://doi.org/10.1029/2020RG000726, 2022.
Kiehl, J. T.: Twentieth century climate model response and climate sensitivity, Geophys. Res. Lett., 34, L22710, https://doi.org/10.1029/2007GL031383, 2007.
Klein-Goldewijk, K.: Estimating global land use change over the past 300 years: the HYDE database, Global Biogeochem. Cy., 15, 417–434, 2001.
Klimont, Z., Kupiainen, K., Heyes, C., Purohit, P., Cofala, J., Rafaj, P., Borken-Kleefeld, J., and Schöpp, W.: Global anthropogenic emissions of particulate matter including black carbon, Atmos. Chem. Phys., 17, 8681–8723, https://doi.org/10.5194/acp-17-8681-2017, 2017.
Kloster, S., Mahowald, N. M., Randerson, J. T., Thornton, P. E., Hoffman, F. M., Levis, S., Lawrence, P. J., Feddema, J. J., Oleson, K. W., and Lawrence, D. M.: Fire dynamics during the 20th century simulated by the Community Land Model, Biogeosciences, 7, 1877–1902, https://doi.org/10.5194/bg-7-1877-2010, 2010.
Knorr, W., Kaminski, T., Arneth, A., and Weber, U.: Impact of human population density on fire frequency at the global scale, Biogeosciences, 11, 1085–1102, https://doi.org/10.5194/bg-11-1085-2014, 2014.
Kok, J. F., Ward, D. S., Mahowald, N. M., and Evan, A. T.: Global and regional importance of the direct dust-climate feedback, Nat. Commun., 9, 241, https://doi.org/10.1038/s41467-017-02620-y, 2018.
Kok, J. F., Storelvmo, T., Karydis, V. A., Adebiyi, A., Mahowald, N., Evan, A., He, C., and Leung, D. M.: Mineral dust aerosol impacts on global climate and climate change, Nat. Rev. Earth Environ., 4, 71–86, https://doi.org/10.1038/s43017-022-00379-5, 2023.
Lambert, F., Delmonte, B., Petit, J. R., Bigler, M., Kaufmann, P. R., Hutterli, A. M. A., Stocker, T. F., Ruth, U., Steffensen, J. P., and Maggi, V.: Dust-climate couplings over the past 800 000 years from the EPICA Dome C ice core, Nature, 452, 616–619, 2008.
Lambert, F., Tagliabue, A., Shaffer, G., Lamy, F., Winckler, G., Farias, L., Gallardo, L., and De Pol-Holz, R.: Dust fluxes and iron fertilization in Holocene and Last Glacial Maximum climates, Geophys. Res. Lett., 42, 6014–6023, https://doi.org/10.1002/2015GL064250, 2015.
Lee, J. A., Baddock, M. C., Mbuh, M. J., and Gill, T. E.: Geomorphic and land cover characteristics of aeolian dust sources in West Texas and eastern New Mexico, USA, Aeolian Res., 3, 459–466, https://doi.org/10.1016/j.aeolia.2011.08.001, 2012.
Lee, L. A., Reddington, C. L., and Carslaw, K. S.: On the relationship between aerosol model uncertainty and radiative forcing uncertainty, P. Natl. Acad. Sci. USA, 113, 5820–5827, https://doi.org/10.1073/pnas.1507050113, 2016.
Lehner, F. and Coats, S.: Does Regional Hydroclimate Change Scale Linearly With Global Warming?, Geophys. Res. Lett., 48, e2021GL095127, https://doi.org/10.1029/2021GL095127, 2021.
Li, F., Val Martin, M., Andreae, M. O., Arneth, A., Hantson, S., Kaiser, J. W., Lasslop, G., Yue, C., Bachelet, D., Forrest, M., Kluzek, E., Liu, X., Mangeon, S., Melton, J. R., Ward, D. S., Darmenov, A., Hickler, T., Ichoku, C., Magi, B. I., Sitch, S., van der Werf, G. R., Wiedinmyer, C., and Rabin, S. S.: Historical (1700–2012) global multi-model estimates of the fire emissions from the Fire Modeling Intercomparison Project (FireMIP), Atmos. Chem. Phys., 19, 12545–12567, https://doi.org/10.5194/acp-19-12545-2019, 2019.
Li, J., Carlson, B. E., Yung, Y. L., Lv, D., Hansen, J., Penner, J. E., Liao, H., Ramaswamy, V., Kahn, R. A., Zhang, P., Dubovik, O., Ding, A., Lacis, A. A., Zhang, L., and Dong, Y.: Scattering and absorbing aerosols in the climate system, Nat. Rev.-Earth Environ., 3, 363–379, https://doi.org/10.1038/s43017-022-00296-7, 2022.
Li, L., Mahowald, N. M., Miller, R. L., Pérez García-Pando, C., Klose, M., Hamilton, D. S., Gonçalves Ageitos, M., Ginoux, P., Balkanski, Y., Green, R. O., Kalashnikova, O., Kok, J. F., Obiso, V., Paynter, D., and Thompson, D. R.: Quantifying the range of the dust direct radiative effect due to source mineralogy uncertainty, Atmos. Chem. Phys., 21, 3973–4005, https://doi.org/10.5194/acp-21-3973-2021, 2021.
Liu, P., Kaplan, J. O., Mickley, L. J., Li, Y., Chellman, N. J., Arienzo, M. M., Kodros, J. K., Pierce, J. R., Sigl, M., Freitag, J., Mulvaney, R., Curran, M. A. J., and Mcconnell, J. R.: Improved estimates of preindustrial biomass burning reduce the magnitude of aerosol climate forcing in the Southern Hemisphere, Sci. Adv., 7, eabc1379, https://doi.org/10.1126/sciadv.abc1379, 2021.
Maher, B., Prospero, J., Mackie, D., Gaiero, D., Hesse, P., and Balkanski, Y.: Global connections between aeolian dust, climate and ocean biogeochemistry at the present day and at the last glacial maximum, Earth Sci. Rev., 99, 61–97, 2010.
Mahowald, N.: Anthropocence changes in desert area: sensitivity to climate model predictions, Geophys. Res. Lett., 34, L18817, https://doi.org/10.1029/2007GL030472, 2007.
Mahowald, N.: Aerosol indirect effect on biogeochemical cycles and climate, Science, 334, 794, https://doi.org/10.1126/science.1207374, 2011.
Mahowald, N., Kohfeld, K., Hansson, M., Balkanski, Y., Harrison, S. P., Prentice, I. C., Schulz, M., and Rodhe, H.: Dust sources and deposition during the last glacial maximum and current climate: A comparison of model results with paleodata from ice cores and marine sediments, J. Geophys. Res.-Atmos., 104, 15895–15916, https://doi.org/10.1029/1999JD900084, 1999.
Mahowald, N., Ward, D. S., Kloster, S., Flanner, M. G., Heald, C. L., Heavens, N. G., Hess, P. G., Lamarque, J.-F., and Chuang, P. Y.: Aerosol impacts on climate and biogeochemistry, Annu. Rev. Environ. Resour., 36, 45–74, https://doi.org/10.1146/annurev-environ-042009-094507, 2011a.
Mahowald, N., Lindsay, K., Rothenberg, D., Doney, S. C., Moore, J. K., Thornton, P., Randerson, J. T., and Jones, C. D.: Desert dust and anthropogenic aerosol interactions in the Community Climate System Model coupled-carbon-climate model, Biogeosciences, 8, 387–414, https://doi.org/10.5194/bg-8-387-2011, 2011b.
Mahowald, N. M., Zender, C. S., Luo, C., Savoie, D., Torres, O., Corral, J. D., and Del Corral, J.: Understanding the 30-year Barbados desert dust record, J. Geophys. Res.-Atmos., 107, 4561, https://doi.org/10.1029/2002JD002097, 2002.
Mahowald, N. M., Kloster, S., Engelstaedter, S., Moore, J. K., Mukhopadhyay, S., McConnell, J. R., Albani, S., Doney, S. C., Bhattacharya, A., Curran, M. A. J., Flanner, M. G., Hoffman, F. M., Lawrence, D. M., Lindsay, K., Mayewski, P. A., Neff, J., Rothenberg, D., Thomas, E., Thornton, P. E., and Zender, C. S.: Observed 20th century desert dust variability: impact on climate and biogeochemistry, Atmos. Chem. Phys., 10, 10875–10893, https://doi.org/10.5194/acp-10-10875-2010, 2010.
Mahowald, N. M., Scanza, R., Brahney, J., Goodale, C. L., Hess, P. G., Moore, J. K., and Neff, J.: Aerosol Deposition Impacts on Land and Ocean Carbon Cycles, Curr. Clim. Change Rep., 3, 16–31, https://doi.org/10.1007/s40641-017-0056-z, 2017.
Marlon, J., Bartlein, P., Carcaillet, C., Gavin, D., Harrison, S., Higuera, P., Joos, F., Power, M., and Prentice, I. C.: Climate and human influences on global biomass burning over the past two millennia, Nat. Geosci., 1, 697–701, https://doi.org/10.1029/PA005i001p00001, 2008.
Marlon, J. R., Bartlein, P. J., Gavin, D. G., Long, C. J., Anderson, R. S., Briles, C. E., Brown, K. J., Colombaroli, D., Hallett, D. J., Power, M. J., Scharf, E. A., and Walsh, M. K.: Long-term perspective on wildfires in the western USA, P. Natl. Acad. Sci. USA, 109, E535–E543, https://doi.org/10.1073/pnas.1112839109, 2012.
Marlon, J. R., Kelly, R., Daniau, A.-L., Vannière, B., Power, M. J., Bartlein, P., Higuera, P., Blarquez, O., Brewer, S., Brücher, T., Feurdean, A., Romera, G. G., Iglesias, V., Maezumi, S. Y., Magi, B., Courtney Mustaphi, C. J., and Zhihai, T.: Reconstructions of biomass burning from sediment-charcoal records to improve data–model comparisons, Biogeosciences, 13, 3225–3244, https://doi.org/10.5194/bg-13-3225-2016, 2016.
Matsui, H., Hamilton, D. S., and Mahowald, N. M.: Black carbon radiative effects highly sensitive to emitted particle size when resolving mixing-state diversity, Nat. Commun., 9, 3446, https://doi.org/10.1038/s41467-018-05635-1, 2018.
McConnell, J. R., Aristarain, A., Banta, J., Edwards, P., and Simoes, J.: 20th Century doubling in dust archived in an Antarctic Penisula ice core parallels climate change and desertification in South America, P. Natl. Acad. Sci. USA, 104, 5743–5748, https://doi.org/10.1073/pnas.0607657104, 2007a.
McConnell, J. R., Edwards, R., Kok, G., Flanner, M., Zender, C. S., Saltzman, E., Banta, J. R., Pasteris, D. R., Carter, M. M., and Kahl, J. D. W.: 20th-century industrial black carbon emissions altered Arctic climate forcing, Science, 317, 1381–1384, 2007b.
McCoy, S. L., McCoy, D. T., Wood, R., Regayre, L., Watson-Parris, D., Grosvenor, D., Mulcahy, J., Hu, Y., Bender, F., Field, P., Carslaw, K., and Gordon, H.: The hemispheric contrast in cloud microphysical properties constrains aerosol forcing, P. Natl. Acad. Sci. USA, 117, 18998–19006, https://doi.org/10.1073/pnas.1922502117, 2020.
Mulitza, S., Heslop, D., Pittauerova, D., Fischer, H., Meyer, I., Stuut, J.-B., Zabel, M., Mollenhauer, G., Collins, J., Kuhnert, H., and Schulz, M.: Increase in African dust flux at the onset of commercial agriculture in the Sahel region, Nature, 466, 226–228, https://doi.org/10.1038/nature09213, 2010.
Murray, B. J., Carslaw, K. S., and Field, P. R.: Opinion: Cloud-phase climate feedback and the importance of ice-nucleating particles, Atmos. Chem. Phys., 21, 665–679, https://doi.org/10.5194/acp-21-665-2021, 2021.
Naik, V., Szopa, S., Adhikary, S., Artaxo, B. P., Berntsen, T., Collins, W. D., Fuzzi, S., Gallardo, L., Kiendler Scharr, A., Klimont, Z., Liao, H., Unger, N., and Zanis, P.: Chapter 6: Short-Lived Climate Forcers, in: Climate Change 2021: The Physical Science Basis. Contribution of Working Group I to the Sixth Assessment Report of the Intergovernmental Panel on Climate Change, edited by: Masson-Delmotte, V., Zhai, P., Pirani, A., Connors, S. L., Péan, C., Berger, S., Caud, N., Chen, Y., Goldfarb, L., Gomis, M. I., Huang, M., Leitzell, K., Lonnoy, E., Matthews, J. B. R., Maycock, T. K., Waterfield, T., Yelekçi, O., Yu, R., and Zhou, B., Cambridge University Press, Cambridge, United Kingdom and New York, NY, USA, https://doi.org/10.1017/9781009157896.008, 817–922, 2021.
Neff, J. C., Ballantyne, A. P., Farmer, G. L., Mahowald, N. M., Conroy, J. L., Landry, C. C., Overpeck, J. T., Painter, T. H., Lawrence, C. R., and Reynolds, R. L.: Increasing eolian dust deposition in the western United States linked to human activity, Nat. Geosci., 1, 189–195, https://doi.org/10.1038/ngeo133, 2008.
Paulot, F., Ginoux, P., Cooke, W. F., Donner, L. J., Fan, S., Lin, M.-Y., Mao, J., Naik, V., and Horowitz, L. W.: Sensitivity of nitrate aerosols to ammonia emissions and to nitrate chemistry: implications for present and future nitrate optical depth, Atmos. Chem. Phys., 16, 1459–1477, https://doi.org/10.5194/acp-16-1459-2016, 2016.
Pechony, O. and Shindell, D. T.: Driving forces of global wildfires over the past millennium and the forthcoming century, P. Natl. Acad. Sci. USA, 107, 19167–19170, https://doi.org/10.1073/pnas.1003669107, 2010.
Penner, J., Dickinson, R., and O'Neill, C. A.: Effects of Aerosol from Biomass Burning on the Global Radiation Budget, Science, 256, 1432–1434, 1992.
Pincus, R., Forster, P. M., and Stevens, B.: The Radiative Forcing Model Intercomparison Project (RFMIP): experimental protocol for CMIP6, Geosci. Model Dev., 9, 3447–3460, https://doi.org/10.5194/gmd-9-3447-2016, 2016.
Power, M. J., Marlon, J., Ortiz, N., Bartlein, P. J., Harrison, S. P., Mayle, F. E., Ballouche, A. Bradshaw, R. H. W., Carcaillet, C., Cordova, C., Mooney, S., Moreno, P., Prentice, I. C., Thonicke, K., Tinner, W., Whitlock, C., Zhang, Y., Zhao, Y., Ali, A. A., Anderson, R. S., Bee, R., Behling, H., Brile, C., Brown, K. J., Brunelle, A., Bush, M., Camill, P., Chu, G. Q., Clark, J., Colombaroli, D., Connor, S.. Daniau, A.-L, Daniels, M., Dodson, J., Doughty, E., Edwards, M. E., Finsinger, W., Foster, D., Frechette, J., Gaillard, M.-J., Gavin, D. G., Gobet, E., Haberle, S., Hallett, D. J., Higuera, P., Hope, G, Horn, S., Inoue, J., Kaltenrieder, P, Kennedy, L., Kong, Z. C., Larsen, C., Long, C. J., Lynch, J., Lynch, E. A., McGlone, M.. Meeks, S., Mensing, S., Meyer, G. Minckley, T., Mohr, J., Nelson, D. M., New, J., Newnham, R., Noti, R., Oswald, W., Pierce, J., Richard, P. J. H., Rowe, C, Sanchez Goni, M.F., Shuman, B. N., Takahara, H., Toney, J., Turney, C., Urrego-Sanchez, D. H., Umbanhowar, C., Vandergoes, M., Vanniere, B., Vescovi, E., Walsh, M., Wang, X.,.,Williams,, N., J. Wilmshurst, J., Zhang, J. H. : Changes in fire regimes since the Last Glacial Maximum: an assessment based on a global synthesis and analysis of charcoal data, Clim. Dynam., 30, 887–907, https://doi.org/10.1007/s00382-007-0334-x, 2007.
Prospero, J. and Lamb, P.: African droughts and dust transport to the Caribbean: climate change implications, Science, 302, 1024–1027, https://doi.org/10.1126/science.1089915, 2003.
Pye, H., Liao, H., Wu, S., Mickley, L., Jacob, D., Henze, D., and Seinfeld, J.: Effect of changes in climate and emissions on future sulfate-nitrate-ammonium aerosol levels in the United States, J. Geophys. Res., 114, D01205, https://doi.org/10.1029/2008JD010701, 2009.
Rathod, S., Hamilton, D. S., Mahowald, N., Klimont, Z., Corbett, J. J., and Bond, T.: A mineralogy-based anthropogenic combustion-iron emission inventory, J. Geophys. Res.-Atmos., 125, e2019JD032114, https://doi.org/10.1029/2019JD032114, 2020.
Regayre, L. A., Johnson, J. S., Yoshioka, M., Pringle, K. J., Sexton, D. M. H., Booth, B. B. B., Lee, L. A., Bellouin, N., and Carslaw, K. S.: Aerosol and physical atmosphere model parameters are both important sources of uncertainty in aerosol ERF, Atmos. Chem. Phys., 18, 9975–10006, https://doi.org/10.5194/acp-18-9975-2018, 2018.
Sherwood, S. C., Webb, M. J., Annan, J. D., Armour, K. C., Forster, P. M., Hargreaves, J. C., Hegerl, G., Klein, S. A., Marvel, K. D., Rohling, E. J., Watanabe, M., Andrews, T., Braconnot, P., Bretherton, C. S., Foster, G. L., Hausfather, Z., von der Heydt, A. S., Knutti, R., Mauritsen, T., Norris, J. R., Proistosescu, C., Rugenstein, M., Schmidt, G. A., Tokarska, K. B., and Zelinka, M. D.: An Assessment of Earth's Climate Sensitivity Using Multiple Lines of Evidence, Rev. Geophys., 58, 1–92, https://doi.org/10.1029/2019RG000678, 2020.
Smith, C. J., Harris, G. R., Palmer, M. D., Bellouin, N., Collins, W., Myhre, G., Schulz, M., Golaz, J. C., Ringer, M., Storelvmo, T., and Forster, P. M.: Energy Budget Constraints on the Time History of Aerosol Forcing and Climate Sensitivity, J. Geophys. Res.-Atmos., 126, e2020JD033622, https://doi.org/10.1029/2020JD033622, 2021.
Snider, G., Weagle, C. L., Murdymootoo, K. K., Ring, A., Ritchie, Y., Stone, E., Walsh, A., Akoshile, C., Anh, N. X., Balasubramanian, R., Brook, J., Qonitan, F. D., Dong, J., Griffith, D., He, K., Holben, B. N., Kahn, R., Lagrosas, N., Lestari, P., Ma, Z., Misra, A., Norford, L. K., Quel, E. J., Salam, A., Schichtel, B., Segev, L., Tripathi, S., Wang, C., Yu, C., Zhang, Q., Zhang, Y., Brauer, M., Cohen, A., Gibson, M. D., Liu, Y., Martins, J. V., Rudich, Y., and Martin, R. V.: Variation in global chemical composition of PM2.5: emerging results from SPARTAN, Atmos. Chem. Phys., 16, 9629–9653, https://doi.org/10.5194/acp-16-9629-2016, 2016.
Stanelle, T., Bey, I., Raddatz, T., Reick, C., and Tegen, I.: Anthropogenically induced changes in twentieth century mineral dust burden and the associated impact on radiative forcing, J. Geophys. Res., 119, 13526–13546, https://doi.org/10.1002/2014JD022062, 2014.
Storelvmo, T.: Aerosol Effects on Climate via Mixed-Phase and Ice Clouds, Annu. Rev. Earth Pl. Sc., 45, 199–222, https://doi.org/10.1146/annurev-earth-060115-012240, 2017.
Szopa, S., Naik, V., Adhikary, B., Artaxo, P., Berntsen, T., Collins, W. D., Fuzzi, S., Gallardo, L., Kiendler-Scharr, A., Klimont, Z., Liao, H., Unger, N., and Zanis, P.: Short-Lived Climate Forcers, in: Climate Change 2021: The Physical Science Basis. Contribution of Working Group I to the Sixth Assessment Report of the Intergovernmental Panel on Climate Change, edited by: Masson-Delmotte, V., Zhai, P., Pirani, A., Connors, S. L., Péan, C., Berger, S., Caud, N., Chen, Y., Goldfarb, L., Gomis, M. I., Huang, M., Leitzell, K., Lonnoy, E., Matthews, J. B. R., Maycock, T. K., Waterfield, T., Yelekçi, O., Yu, R., and Zhou, B., Cambridge University Press, Cambridge, United Kingdom and New York, NY, USA, 817–922, https://doi.org/10.1017/9781009157896.008, 2021.
Tegen, I., Werner, M., Harrison, S. P., Kohfeld, K. E., Mahowald, N. M., Rivera Rivera, G. D., and Luo, C.: Relative importance of climate and land use in determining present and future global soil dust emission, Geophys. Res. Lett., 31, L05105, https://doi.org/10.1029/2003GL019216, 2004.
Textor, C., Schulz, M., Guibert, S., Kinne, S., Balkanski, Y., Bauer, S., Berntsen, T., Berglen, T., Boucher, O., Chin, M., Dentener, F., Diehl, T., Easter, R., Feichter, H., Fillmore, D., Ghan, S., Ginoux, P., Gong, S., Grini, A., Hendricks, J., Horowitz, L., Huang, P., Isaksen, I., Iversen, I., Kloster, S., Koch, D., Kirkevåg, A., Kristjansson, J. E., Krol, M., Lauer, A., Lamarque, J. F., Liu, X., Montanaro, V., Myhre, G., Penner, J., Pitari, G., Reddy, S., Seland, Ø., Stier, P., Takemura, T., and Tie, X.: Analysis and quantification of the diversities of aerosol life cycles within AeroCom, Atmos. Chem. Phys., 6, 1777–1813, https://doi.org/10.5194/acp-6-1777-2006, 2006.
Thornhill, G., Collins, W., Olivié, D., Skeie, R. B., Archibald, A., Bauer, S., Checa-Garcia, R., Fiedler, S., Folberth, G., Gjermundsen, A., Horowitz, L., Lamarque, J.-F., Michou, M., Mulcahy, J., Nabat, P., Naik, V., O'Connor, F. M., Paulot, F., Schulz, M., Scott, C. E., Séférian, R., Smith, C., Takemura, T., Tilmes, S., Tsigaridis, K., and Weber, J.: Climate-driven chemistry and aerosol feedbacks in CMIP6 Earth system models, Atmos. Chem. Phys., 21, 1105–1126, https://doi.org/10.5194/acp-21-1105-2021, 2021.
Turnock, S. T., Allen, R. J., Andrews, M., Bauer, S. E., Deushi, M., Emmons, L., Good, P., Horowitz, L., John, J. G., Michou, M., Nabat, P., Naik, V., Neubauer, D., O'Connor, F. M., Olivié, D., Oshima, N., Schulz, M., Sellar, A., Shim, S., Takemura, T., Tilmes, S., Tsigaridis, K., Wu, T., and Zhang, J.: Historical and future changes in air pollutants from CMIP6 models, Atmos. Chem. Phys., 20, 14547–14579, https://doi.org/10.5194/acp-20-14547-2020, 2020.
Undorf, S., Polson, D., Bollasina, M. A., Ming, Y., Schurer, A., and Hegerl, G. C.: Detectable Impact of Local and Remote Anthropogenic Aerosols on the 20th Century Changes of West African and South Asian Monsoon Precipitation, J. Geophys. Res.-Atmos., 123, 4871–4889, https://doi.org/10.1029/2017JD027711, 2018.
Unger, N., Bond, T., Wang, J. S., Koch, D. M., Menon, S., Shindell, D., and Bauer, S.: Attribution of climate forcing to economic sectors, P. Natl. Acad. Sci. USA, 107, 3382–3387, 2010.
van der Werf, G. R., Randerson, J., Collatz, G. J., Giglio, L., Kasibhatla, P., Arrellano, A., Olson, S., and Kasischke, E.: Continental-scale partitioning of fire emissions during the 1997 to 2001 El Nino/La Nina Period, Science, 303, 73–76, 2004.
van der Werf, G. R., Randerson, J. T., Giglio, L., Collatz, G. J., Kasibhatla, P. S., and Arellano Jr., A. F.: Interannual variability in global biomass burning emissions from 1997 to 2004, Atmos. Chem. Phys., 6, 3423–3441, https://doi.org/10.5194/acp-6-3423-2006, 2006.
van der Werf, G. R., Randerson, J. T., Giglio, L., Collatz, G. J., Mu, M., Kasibhatla, P. S., Morton, D. C., DeFries, R. S., Jin, Y., and van Leeuwen, T. T.: Global fire emissions and the contribution of deforestation, savanna, forest, agricultural, and peat fires (1997–2009), Atmos. Chem. Phys., 10, 11707–11735, https://doi.org/10.5194/acp-10-11707-2010, 2010.
van der Werf, G. R., Peters, W., van Leeuwen, T. T., and Giglio, L.: What could have caused pre-industrial biomass burning emissions to exceed current rates?, Clim. Past, 9, 289–306, https://doi.org/10.5194/cp-9-289-2013, 2013.
van Marle, M. J. E., Kloster, S., Magi, B. I., Marlon, J. R., Daniau, A.-L., Field, R. D., Arneth, A., Forrest, M., Hantson, S., Kehrwald, N. M., Knorr, W., Lasslop, G., Li, F., Mangeon, S., Yue, C., Kaiser, J. W., and van der Werf, G. R.: Historic global biomass burning emissions for CMIP6 (BB4CMIP) based on merging satellite observations with proxies and fire models (1750–2015), Geosci. Model Dev., 10, 3329–3357, https://doi.org/10.5194/gmd-10-3329-2017, 2017.
Wan, J. S., Hamilton, D. S., and Mahowald, N. M.: Importance of Uncertainties in the Spatial Distribution of Preindustrial Wildfires for Estimating Aerosol Radiative Forcing, Geophys. Res. Lett., 48, e2020GL089758, https://doi.org/10.1029/2020GL089758, 2021.
Wang, Z., Chappellaz, J., Park, K., and Mak, J. E.: Large Variations in Southern, Science, 205, 1663–1666, https://doi.org/10.1126/science.1197257, 2010.
Webb, N. P. and Pierre, C.: Quantifying Anthropogenic Dust Emissions, Earths Future, 6, 286–295, https://doi.org/10.1002/2017EF000766, 2018.
Wu, J., Kong, S., Wu, F., Cheng, Y., Zheng, S., Yan, Q., Zheng, H., Yang, G., Zheng, M., Liu, D., Zhao, D., and Qi, S.: Estimating the open biomass burning emissions in central and eastern China from 2003 to 2015 based on satellite observation, Atmos. Chem. Phys., 18, 11623–11646, https://doi.org/10.5194/acp-18-11623-2018, 2018.
Xi, X. and Sokolik, I. N.: Quantifying the anthropogenic dust emission from agricultural land use and desiccation of the aral sea in central Asia, J. Geophys. Res., 121, 12270–12281, https://doi.org/10.1002/2016JD025556, 2016.
Zennaro, P., Kehrwald, N., McConnell, J. R., Schüpbach, S., Maselli, O. J., Marlon, J., Vallelonga, P., Leuenberger, D., Zangrando, R., Spolaor, A., Borrotti, M., Barbaro, E., Gambaro, A., and Barbante, C.: Fire in ice: two millennia of boreal forest fire history from the Greenland NEEM ice core, Clim. Past, 10, 1905–1924, https://doi.org/10.5194/cp-10-1905-2014, 2014.
Zhao, A., Ryder, C. L., and Wilcox, L. J.: How well do the CMIP6 models simulate dust aerosols?, Atmos. Chem. Phys., 22, 2095–2119, https://doi.org/10.5194/acp-22-2095-2022, 2022.
Zhu, J., Penner, J. E., Yu, F., Sillman, S., Andreae, M. O., and Coe, H.: Decrease in radiative forcing by organic aerosol nucleation, climate, and land use change, Nat. Commun., 10, 423, https://doi.org/10.1038/s41467-019-08407-7, 2019.
- Abstract
- Introduction
- Observations of natural aerosol changes since 1850
- Are dust and other natural aerosols forcers or feedbacks?
- Characterizing preindustrial to current aerosol forcing uncertainty
- Characterizing paleoclimate aerosol forcing uncertainty
- Aerosol processes and other sources of uncertainty
- Implications of including uncertainty in emissions in radiative forcing estimates
- Conclusions: pathway to improve historical and paleoclimate characterization of uncertainties
- Data availability
- Author contributions
- Competing interests
- Disclaimer
- Special issue statement
- Acknowledgements
- Financial support
- Review statement
- References
- Abstract
- Introduction
- Observations of natural aerosol changes since 1850
- Are dust and other natural aerosols forcers or feedbacks?
- Characterizing preindustrial to current aerosol forcing uncertainty
- Characterizing paleoclimate aerosol forcing uncertainty
- Aerosol processes and other sources of uncertainty
- Implications of including uncertainty in emissions in radiative forcing estimates
- Conclusions: pathway to improve historical and paleoclimate characterization of uncertainties
- Data availability
- Author contributions
- Competing interests
- Disclaimer
- Special issue statement
- Acknowledgements
- Financial support
- Review statement
- References