the Creative Commons Attribution 4.0 License.
the Creative Commons Attribution 4.0 License.
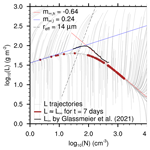
The impact of aerosol on cloud water: a heuristic perspective
Franziska Glassmeier
Graham Feingold
Aerosol–cloud interactions modulate the role of clouds in Earth's climate. We derive, evaluate, and apply a simple model to understand aerosol-mediated cloud water adjustments in stratocumulus based on only two prognostic equations for the integrated cloud water L and droplet number concentration N. The model is solved numerically and analytically and agrees well with documented large-eddy-simulation data and satellite retrievals. A tight relationship between adjustments at low and high N is found, revealing the influence of non-precipitation processes (primarily entrainment) on adjustments in precipitating clouds. Furthermore, it is shown that adjustments in non-precipitating clouds tend to be positively biased by external L or N perturbations, while adjustments in precipitating clouds are barely susceptible. By deliberately reducing the complexity of the underlying system, this study constitutes a way forward to facilitate process-level understanding of cloud water adjustments.
- Article
(2479 KB) - Full-text XML
-
Supplement
(1945 KB) - BibTeX
- EndNote
By constituting the nuclei on which cloud droplets form, aerosol substantially shapes the microphysical composition of clouds, their optical properties, and hence their role in Earth's climate. One important example is the ability of clouds to reflect incident solar radiation back to space, causing a negative (cooling) influence on Earth's radiation budget. While aerosol tends to increase cloud reflectance, this and other aerosol–cloud–climate interactions are only marginally understood (e.g., Boucher et al., 2013; Forster et al., 2021).
One metric to quantify aerosol–cloud–climate interactions is the susceptibility of the shortwave cloud albedo A to changes in the cloud droplet number concentration N (e.g., Platnick and Twomey, 1994). This susceptibility can be expressed as
where the term represents the fairly well understood increase in A with N at constant cloud water, commonly referred to as the Twomey effect (Twomey, 1974, 1977). This study will address the considerably less understood cloud water adjustments in the bracketed term. Depending on how the vertically integrated cloud water L changes with N, cloud water adjustments can increase, decrease, or even change the sign of S.
In earlier years, cloud water adjustments were mainly thought to be related to precipitation suppression, i.e., the increasingly less efficient production of precipitation by smaller cloud droplets, resulting in larger L for higher N, causing a larger S than anticipated from the Twomey effect alone (e.g., Albrecht, 1989). Later, it was recognized that the mixing of clouds with their environment (entrainment) increases for higher N, which causes L to decrease, resulting in a smaller or even negative S (e.g., Wang et al., 2003; Ackerman et al., 2004; Bretherton et al., 2007; Glassmeier et al., 2021). Together, these effects result in an increase in L for lower N, followed by a decrease for higher N. The commensurately more nuanced influence of cloud water adjustments on S has been retrieved from satellite observations of shallow cumulus and stratocumulus clouds (e.g., Gryspeerdt et al., 2019).
Large-eddy simulations (LESs) have become the primary tool to gain process-level understanding of cloud water adjustments. While LES estimates stem from the high-resolution representation of the underlying dynamics and cloud microphysics, they tend to be valid only for limited spatial domains and specific initial and boundary conditions (e.g., Ackerman et al., 2009; Glassmeier et al., 2021). On the other hand, satellite observations have become increasingly useful for an integrated view of aerosol–cloud–climate interactions, sampling a wealth of real-world data, but also for the inherent co-variability of aerosol and meteorology that confounds process-level understanding (e.g., Gryspeerdt et al., 2019; Mülmenstädt et al., 2024).
Aiming to combine the aforementioned integrated view with process-level insights, this paper will develop a heuristic model for cloud water adjustments in stratocumulus, a crucial cloud type in Earth's radiation budget (e.g., Wood, 2012). The foundations of this model will be laid out in Sect. 2, and it will be applied in Sect. 3. Basic sensitivities to model parameters are analyzed in Sect. 4. Section 5 addresses the variability of cloud water adjustments in externally perturbed systems, presenting a way forward to use this study's results when interpreting observed cloud water adjustment. The paper is summarized and concluded in Sect. 6.
The heuristic model is formulated using ideas that originated from satellite retrievals by Gryspeerdt et al. (2019) and the LES modeling by Hoffmann et al. (2020): their works showed that cloud water adjustments can be separated into two distinct regimes, which are dominated by precipitation at low N and thermodynamics at high N, respectively. Here, thermodynamics comprises the effects of entrainment, radiative cooling, and surface fluxes on L. Thus, we will refer to the underlying processes in those regimes as driven by precipitation or thermodynamics in the following. Moreover, we introduce the shorthand,
for the change in L with N. The optional subscripts h and l indicate limits for high and low N, respectively. The ∞ subscript marks (potentially prescribed) steady states.
The effects of precipitation and thermodynamics on the temporal change in L are represented as
whose terms will be described next. All variables, parameters, and derived parameters used in the heuristic model are summarized in Table 1.
The first term on the right-hand side of Eq. (3) represents a precipitation sink. The employed expression relates the cloud base rain rate to L and N. We express the precipitation sink by introducing a precipitation timescale
where the term in parentheses is a more common representation of the cloud base rain rate, which has been assessed observationally and theoretically (e.g., Van Zanten et al., 2005; Kostinski, 2008). It has been argued that the exponents of L and N depend on the assumed sedimentation velocity and hence droplet size (Feingold et al., 2013). For simplicity, these dependencies are neglected here, as is evaporation below the cloud base.
The second term on the right-hand side of Eq. (3) depicts the charge/discharge to the thermodynamic carrying capacity of the system L∞,h, which can also be interpreted as a steady-state L at high N, whose existence has been discussed by Hoffmann et al. (2020). For a given N, this term can be a sink to the L budget due to an excess in entrainment warming and drying, causing the cloud to evaporate (), or a source driven by longwave radiative cooling, leading to more condensation (), while the effect of surface fluxes is usually small (see Fig. 2 in Hoffmann et al., 2020). The timescale associated with this process is given by τt. The thermodynamic carrying capacity is derived from Eq. (2) and expressed as
where m∞,h determines how L∞,h changes with N, while L0 and N0 are constant parameters.
Many studies (Fig. 1 in Glassmeier et al., 2021) have determined m∞,h, and hence L∞,h, for high N, which excludes the effects of precipitation present at low N. Here, L∞,h is applied to all N with the same m∞,h. This idea is motivated by the insight that the temporal change in L due to thermodynamics (entrainment, radiative cooling, and surface fluxes) exhibits a sensitivity to N that seems independent of the presence of precipitation. This was initially shown in Fig. 3 of Hoffmann et al. (2020) but is recreated in a more useful way in the Supplement (Fig. S1). Thus, the same adjustment of thermodynamic processes at high N (i.e., m∞,h) is assumed to persist for low N. Specifically, due to the increase in entrainment with N (Wang et al., 2003; Ackerman et al., 2004; Bretherton et al., 2007).
The model parameters have been chosen to match the ensemble LES modeling of Glassmeier et al. (2021), who studied cloud water adjustments in stratocumulus clouds. They determined τt=9 h and using an emulator. Based on their Fig. 3a, we selected and to match their L∞ for high N and derived to match their L∞ for low N. Note that this set of parameters should be seen as one potential realization of cloud water adjustments. The sensitivity to these parameters will be analyzed in Sect. 4. Note that to fit the aforementioned ensemble LESs, c1 is about half the value observed by Van Zanten et al. (2005), necessary to account for the subadiabaticity of L naturally included in observations but not captured in Eq. (3). Further, note that the thermodynamic charge/discharge in Eq. (3) is driven by the linear difference , without further justification. A model driven by the logarithmic difference does not align well with the ensemble LES modeling of Glassmeier et al. (2021) but is briefly discussed in the Supplement (Sect. S1 and Fig. S2).
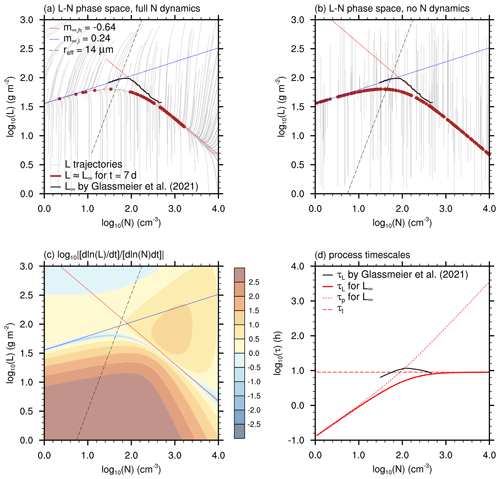
Figure 1Panel (a) and (b) show trajectories of individual simulations (gray lines) in an L–N phase space with and without N dynamics, respectively. Brown dots indicate the location of simulations after 7 d. The quotient of the relative motion in L and N directions is shown in panel (c). These panels are overlaid with the corresponding ensemble LES reference by Glassmeier et al. (2021) (thick black line), the slopes and (blue and red lines, respectively), and the 14 µm cloud top effective droplet radius (dashed black line). Panel (d) shows the process timescales τt, τp and τL (red lines), as well as ensemble LES reference by Glassmeier et al. (2021) (black line).
For completeness, a prognostic equation for N, loosely based on Baker and Charlson (1990), is solved. The expression
combines sinks of N by precipitation (first term on the right-hand side) and Brownian coagulation (second term), as well as a source SN (third term) that could represent, e.g., the emission of sea spray. Here, we choose (e.g., Seinfeld and Pandis, 2016) and , which can be considered the upper limit for c2 (Wood, 2006). Nonetheless, the effect of precipitation scavenging steered by c2 on the steady-state behavior of L is small, as we will show next.
Results from integrating Eqs. (3) and (6) for 7 d with a time step Δt=1 min are shown in Fig. 1a. The source SN has been neglected for simplicity. In total, 250 simulations are conducted, with initial L and N randomly placed between 1 and 1000 g m−2, as well as 1 and 100 000 cm−3, while only results for are shown. Note that while are frequently observed in stratocumulus, they tend to not exhibit (e.g., Wood, 2012). This discrepancy is irrelevant to this study that focuses on the change of L with N, i.e., the slope m, which is constant for such high N, as we will see below.
The individual simulations (gray lines in Fig. 1a) show substantial motion in the L direction, while motion in the N direction is only relevant at low due to precipitation scavenging and at high due to Brownian coagulation. Although SN=0 and hence everywhere in the phase space, a stable population of simulations persists between these limits for at least the 7 d of simulated time considered (brown dots). (Baker and Charlson (1990) showed how the consideration of a SN>0 could offset the losses in N, causing N∞ steady states.) In the L direction, these simulations approach a steady-state L∞ that agrees well with the ensemble LES reference by Glassmeier et al. (2021) (black line) and especially its slopes mh and ml toward high and low N. As we will show below, these slopes agree well with the heuristic model's steady-state slopes and (red and blue lines, respectively). The only notable difference to the LES reference is the more gentle transition between the two slopes, which might be due to the continuous representation of precipitation in Eq. (3), while the process of autoconversion, i.e., the initiation of precipitation, is a discontinuous process that only allows precipitation to form once a certain droplet size is exceeded (Kessler, 1969). This threshold is illustrated by the dashed black line indicating a cloud top effective droplet radius of 14 µm that is often used to discriminate precipitating from non-precipitating clouds and scales with (e.g., Gerber, 1996; Goren et al., 2019).
Solving only Eq. (3), i.e., without the N dynamics considered by Eq. (6), the steady-state L∞ exhibits very similar features to the previously discussed solution (Fig. 1b). Most importantly, the slopes and hence the cloud water adjustments agree, which is why N dynamics are neglected in the following. A reason for the apparent independence of cloud water adjustments from N dynamics is shown in Fig. 1c, which shows the relative motion of the system, . Relative changes in L exceed changes in N almost everywhere in the phase space (warm colors). Changes in N dominate primarily around the steady state (cold colors), where per definition. Brownian coagulation widens this region around the steady state for high N, while precipitation scavenging creates another region where N dynamics dominate at low N but for L≫L∞. Although precipitation scavenging is often reported for low N with potential implications for cloud water adjustments (e.g., Gryspeerdt et al., 2022), the L∞ values investigated here are too small to be affected by stronger N dynamics, thus allowing us to neglect them for now. Future work might want to include a prognostic equation for the cloud fraction, which tends to be smaller than unity for low N, resulting in higher in-cloud L than predicted by Eq. (3) and thus stronger precipitation scavenging by Eq. (6).
To further understand the steady-state behavior of L and its slope m, we investigate of Eq. (3) analytically. A few algebraic rearrangements yield
The term in parentheses describes the deviation of L∞ from L∞,h due to precipitation, and its strength depends on the ratio of the process timescales τt and τp. Figure 1d shows the N dependence of τt; τp; and the timescale of all L processes, , in the steady state. While τt (long-dashed red line) is constant as prescribed, a strong increase in τp (short-dashed red line) with N is shown, indicating that precipitation only affects L∞ for sufficiently small N. Thus, τL (continuous red line) follows τp for low N and τt for high N. τL from the ensemble of LESs of Glassmeier et al. (2021) (black line) captures this behavior only partially, which might be related to the difficulty in determining multiple derivatives from LES data. Note that we introduce as the boundary between the precipitation- and thermodynamics-dominated and hence low and high N parts of the phase space, as it corresponds to the L∞ inflection point in the heuristic model and LES ensemble data of Glassmeier et al. (2021) (Fig. 1a and b).
The logarithmic derivative of Eq. (7) with respect to ln (N) gives
with more details provided in the Supplement (Sect. S2). m∞ shows that for τt≪τp (), thermodynamics dominate cloud water adjustments via m∞,h. For τp≪τt (), m∞ approaches
which combines the effects of thermodynamic adjustments, m∞,h, with a precipitation adjustment of . This behavior is captured well in Fig. 1a and b, where the slopes (red line) and (blue line) overlap with the model data.
Additionally, the relationship (Eq. 9) constitutes a way to assess the consistency of cloud water adjustments derived for low and high N. Strictly speaking, the m∞,h derived from m∞,l via Eq. (9) only represents the thermodynamic adjustments at low N, while the thermodynamic adjustments at high N might differ. Nonetheless, the cloud water adjustments of ml=0.21 and determined from the ensemble of LESs by Glassmeier et al. (2021) agree well with Eq. (9). Deviations from Eq. (9) can indicate aerosol–meteorology co-variability commonly found in maritime and continental air masses (e.g., Brenguier et al., 2003) but absent in the LESs of Glassmeier et al. (2021) by design. Moreover, deviations can hint at changes in the sensitivity of thermodynamic processes to N, e.g., the stabilizing effect of evaporating precipitation on boundary-layer dynamics and hence entrainment at low N, which naturally vanishes for higher N due to decreasing precipitation (e.g., Nicholls, 1984; Caldwell et al., 2005; Wood, 2007; Hoffmann et al., 2023).
Now, the dependence of model (3) on the parameters τt, c1, L0, and m∞,h is tested in Fig. 2a to d, showing L after 7 d of integration as a function of N. The dependence on N0 is neglected here, as it is analogous to L0 via its influence on L∞,h. If τt, c1, L0 or m∞,h is not varied, their aforementioned default is used. The default case is indicated by gray dots, while setups with varied parameters are highlighted by colored dots. Time stepping and initialization follow the previously outlined procedure.
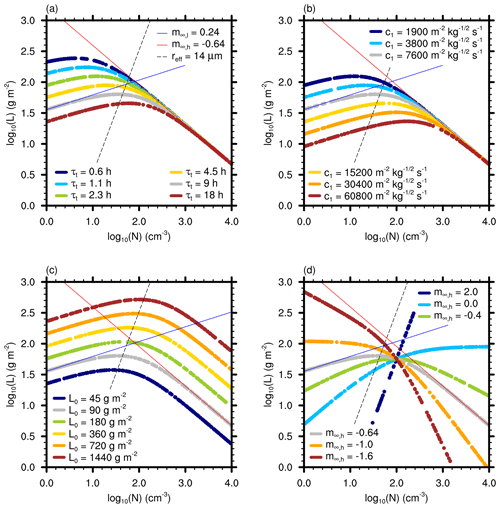
Figure 2L after 7 d as a function of N for variations in (a) τt, (b) c1, (c) L0, and (d) m∞,h (colored dots). The default configuration is differentiated by gray dots. Plots are overlaid with and (blue and red lines) and the 14 µm cloud top effective droplet radius (dashed black line).
Figure 2a shows that shorter τt force L to follow L∞,h for lower N compared to the default case. The commensurately higher L at low N is caused by a faster recharge of precipitation losses by thermodynamics, while L at high N is unchanged. As expected from Eq. (8), mh and ml approach the slopes m∞,h and m∞,l for all τt. However, the transition between mh and ml is shifted depending on the ratio . A similar influence is visible from variations in the precipitation constant c1, which determines the strength of precipitation losses at small N (Fig. 2b). Note that the value of c1 closest to the observations by Van Zanten et al. (2005) (yellow dots) results in stronger precipitation losses than in the ensemble LESs of Glassmeier et al. (2021) (gray dots).
L changes proportionally to L0 for all N, with its slopes matching m∞,l and m∞,h as before (Fig. 2c). For all L0, the maximum L agrees well with the cloud top effective droplet radius of 14 µm (dashed line), marking the transition between precipitating and non-precipitating clouds. Note that this is not the case for the previously discussed sensitivities on τt and c1 (Fig. 2a and b). This indicates that the usefulness of the cloud top effective radius threshold for separating the precipitating and non-precipitating branches of L depends on τt and c1.
The sensitivity to m∞,h is displayed in Fig. 2d. As indicated by Eq. (8), the slopes for high and low N are commensurate with the prescribed values m∞,h and the resultant m∞,l. Nonetheless, we would like to highlight a few interesting values that m∞,h may assume, even though is likely unphysical due to the negative impact of increased entrainment on L at higher N. For (dark-blue dots), cloud water adjustments are the same for all N (), while any will result in . Coincidentally, matches the slope of the effective radius (dashed line). If (orange dots), cloud water adjustments vanish at low N (), while they vanish at high N for (light-blue dots). Sufficiently strong negative cloud water adjustments can offset the Twomey effect and thus cause a decrease in cloud albedo with increasing N, i.e., S<0 according to Eq. (1). Obviously, (green dots) causes negative S for high N, but (brown dots) establishes negative S for all N by also guaranteeing that .
Building on the previous analysis of the unperturbed steady-state behavior of the model (Eq. 3), we would now like to understand its susceptibility to external perturbations in N and L. N perturbations exist at various temporal and spatial scales, covering highly localized aerosol emissions such as ship tracks to phenomena on regional scales like volcanic eruptions. At the same time, these perturbations might exhibit correlations with L.
In this study, perturbations are modeled as a Bernoulli process and are applied with the probability evaluated for every time step of the model. Here, τprt is the perturbation timescale, which is varied from 20 min to 2 weeks. If a perturbation takes place, ln (N) is modified by adding a Δln (N)prt=ξσprt, where ξ is a normally distributed random number with zero mean and unity standard deviation, modified by σprt=0.5, 1.0, or 2.0. At the same time, a Δln (L)=mprtΔln (N)prt is added to ln (L), with , 0.0, or 1.0 to introduce correlations in the perturbation. Note that τprt, σprt, and mprt are chosen to elucidate the general sensitivity of the system and not to match a realistic case. However, the resultant variability is similar to satellite retrievals (e.g., Gryspeerdt et al., 2019) if sufficiently slow perturbations are applied (Fig. S3). We use the default model parameters described above. Time stepping and initialization follow the previously outlined procedure. No N dynamics other than the perturbation are considered. Results are averaged over the last 2 d of the 7 d simulations. In total, 100 000 simulations are executed for each configuration.
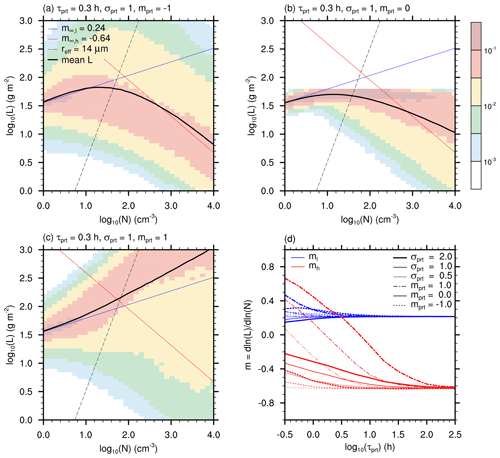
Figure 3Joint L–N histograms (opaque colors) and mean ln (L) (thick black line) for perturbations in L and N for τprt=0.3 h, σprt=1.0 with (a) , (b) 0.0, and (c) 1.0. Plots are overlaid with and (blue and red lines) and the 14 µm cloud top effective droplet radius (dashed black line). Note that the histograms are normalized such that the integral over each N column yields 1 (Gryspeerdt et al., 2019). Panel (d) shows the fitted slopes ml (blue lines) and mh (red lines) for σprt=0.5 (thin lines), 1.0 (medium lines), and 2.0 (thick lines) and (dashed lines), 0.0 (continuous lines), and 1.0 (dash-dotted lines).
Figure 3a to c show example distributions of L and N for , 0.0, and 1.0, respectively, with the same σprt=1.0 and τprt=0.3 h for all cases. The short τprt has been chosen to highlight some processes that are more subtle at larger τprt (see also Fig. S3 for τprt=10 h). For high N, one sees that the variability in L for a given N is proportional to the difference between mprt and m∞,h, which determines the time required for thermodynamics to counter a perturbation in L. Because thermodynamic charge/discharge is linear in L and the perturbations are applied in ln (L) space, more time is required to deplete a positive L perturbation than a negative. This asymmetric response results in slightly higher mean L (black lines) than in the unperturbed case, and adjustments appear less negative than the prescribed ().
A similar effect is also visible for low N. As long as L is sufficiently affected by the perturbation ( and 1.0, Fig. 3a and c), larger L values are possible due to the aforementioned asymmetric response by thermodynamics to the perturbation. However, precipitation removes positively perturbed L more efficiently for lower N than for larger, causing adjustments to appear more positive than in the unperturbed case (). For perturbations in N only (mprt=0.0, Fig. 3b), the mean L increases more gently than in the unperturbed cases, which results in . This is due to stronger precipitation for negative N perturbations, removing any excess in L more quickly than thermodynamics can increase L for positive N perturbations.
Figure 3d shows ml (blue lines) and mh (red lines) as a function of τp. The slopes have been determined by linear regression from the mean ln (L), using the respective ranges and , which have been chosen to minimize the influence of the transition zone between the slopes.
The strongest impact of perturbations on mh (red lines) is visible for mprt=1.0, and it scales with σprt as one would expect. Interestingly, all tested perturbations cause , but it is expected that more strongly negative perturbations () could cause a . Nonetheless, the influence of perturbations vanishes for τprt≫τt, i.e., when thermodynamic charge/discharge becomes faster than the perturbation. Similarly, ml (blue lines) is not affected when τprt≫τp. Because τp≪τt for low N (see Fig. 1d), ml is much less susceptible to perturbations than mh. Overall, ml is closer to than mh to for most tested configurations. Thus, ml might constitute a way to constrain the unperturbed m∞,h via Eq. (9), while mh might not necessarily enable conclusions on m∞,h as long as perturbations cannot be ruled out.
Understanding aerosol–cloud interactions is crucial for constraining the effects of aerosols on the climate. In this study, a heuristic model to understand aerosol-mediated cloud water adjustments in stratocumulus has been derived, evaluated, and applied. The model has been developed to predict the evolution of cloud water path L as a function of the cloud droplet number concentration N. Although the concurrent evolution in N can have an impact on the evolution of L (e.g., Gryspeerdt et al., 2022), it has been neglected for most of this study, and N has been considered a mere parameter. The reason for this is that the relatively small steady-state L to which the system converged does not enable substantial changes in N by precipitation scavenging.
For the evolution of L, two processes have been considered: (i) the removal of L by precipitation and (ii) changes in L by thermodynamics, i.e., the integrated effect of entrainment, radiation, and surface fluxes. The analytical and numerical analysis of the prognostic equation for L shows that it represents the development of two distinct slopes . One is dominated by precipitation at low N and the other by thermodynamics at high N, which is in agreement with previous studies using satellite retrievals (e.g., Gryspeerdt et al., 2019) and large-eddy simulations (LESs) (e.g., Hoffmann et al., 2020; Glassmeier et al., 2021). The study finds that these slopes are intimately related via
showing that precipitation adjustments at low N, i.e., ml, are partially controlled by the thermodynamic adjustments dominating at high N, i.e., mh. Thus, this relationship implicitly assumes the same thermodynamic adjustments mh for all N.
The slopes determined from an ensemble of LESs (Glassmeier et al., 2021) obey the aforementioned relationship between ml=0.21 and well. However, this LES ensemble did not include aerosol–meteorology co-variability by design and hence justifies the use of the same thermodynamic adjustments mh for all N. Observed values for ml are between 0.1 and 0.4 (e.g., Christensen and Stephens, 2011; Gryspeerdt et al., 2019; Possner et al., 2020), which would require mh to vary between −0.9 and −0.4 to follow the aforementioned relationship. But these values only barely overlap with the observed range for mh between −0.2 and −0.4 (e.g., Christensen and Stephens, 2011; Gryspeerdt et al., 2019; Possner et al., 2020). This discrepancy indicates stronger thermodynamic adjustments at low N that transition into weaker thermodynamic adjustments at high N, suggesting that mh should be a function of N. Note that any piecewise constant mh obeys the aforementioned relationship with ml, making it possible to use different mh for low and high N in the proposed framework.
Aerosol–meteorology co-variability could be an explanation for this N dependency. However, we would like to emphasize that this aerosol–meteorology co-variability does not have to be exogenous (e.g., differences in continental and maritime air, e.g., Brenguier et al., 2003) but could be created by the analyzed system endogenously (e.g., the stabilizing effect of evaporating precipitation on boundary-layer dynamics and hence entrainment, e.g., Nicholls, 1984; Caldwell et al., 2005; Wood, 2007; Hoffmann et al., 2023). Quantifying the influence of aerosol–meteorology co-variability on the relationship between ml and mh constitutes an interesting way to continue this study and to deepen process-level understanding of aerosol–cloud–climate interactions.
Another explanation for the weaker observed mh is external perturbations affecting N and L. Our results show that thermodynamic adjustments are sensitive to perturbations with timescales of a few tens of hours or less, causing mh to be weaker than in unperturbed simulations, i.e., to be closer to the aforementioned observations, while ml is barely affected (cf. Fig. 3d).
To constrain the role of aerosols and clouds in the climate system, these perturbations and their biases have to be given due consideration. At the same time, eliminating the effects of perturbations is similarly important for a deeper process-level understanding of cloud water adjustments. Simple models like the one developed here seem to be a useful approach to condense the wealth of theoretical, modeling, and observational knowledge gained so far. Together, this strengthens the need to combine top-down and bottom-up approaches to advance our understanding of aerosol–cloud–climate interactions (e.g., Mülmenstädt and Feingold, 2018; Glassmeier et al., 2019).
The data to reproduce Figs. 1 to 3 are archived in a repository (https://doi.org/10.5281/zenodo.10957468, Hoffmann et al., 2024).
The supplement related to this article is available online at: https://doi.org/10.5194/acp-24-13403-2024-supplement.
FH, FG, and GF conceived the study. FH carried out the study and wrote the initial manuscript. FG and GF contributed to revisions of the manuscript.
At least one of the (co-)authors is a member of the editorial board of Atmospheric Chemistry and Physics. The peer-review process was guided by an independent editor, and the authors also have no other competing interests to declare.
Views and opinions expressed are those of the author(s) only and do not necessarily reflect those of the European Union or the European Research Council Executive Agency. Neither the European Union nor the granting authority can be held responsible for them.
Publisher’s note: Copernicus Publications remains neutral with regard to jurisdictional claims made in the text, published maps, institutional affiliations, or any other geographical representation in this paper. While Copernicus Publications makes every effort to include appropriate place names, the final responsibility lies with the authors.
This research has been supported by the Deutsche Forschungsgemeinschaft (grant no. HO 6588/1-1); the Branco Weiss Fellowship – Society in Science, the European Commission, Horizon 2020 Framework program (grant no. 101117462); and the National Oceanic and Atmospheric Administration (grant no. 03-01-07-001).
This paper was edited by Minghuai Wang and reviewed by two anonymous referees.
Ackerman, A. S., Kirkpatrick, M. P., Stevens, D. E., and Toon, O. B.: The impact of humidity above stratiform clouds on indirect aerosol climate forcing, Nature, 432, 1014–1017, https://doi.org/10.1038/nature03174, 2004. a, b
Ackerman, A. S., VanZanten, M. C., Stevens, B., Savic-Jovcic, V., Bretherton, C. S., Chlond, A., Golaz, J.-C., Jiang, H., Khairoutdinov, M., Krueger, S. K., VanZanten, M. C., Stevens, B., Savic-Jovcic, V., Bretherton, C. S., Chlond, A., Golaz, J.-C., Jiang, H., Khairoutdinov, M., Krueger, S. K., Lewellen, D. C., Lock, A., Moeng, C.-H., Nakamura, K., Petters, M. D., Snider, J. R., Weinbrecht S., and Zulauf, M.: Large-eddy simulations of a drizzling, stratocumulus-topped marine boundary layer, Mon. Weather Rev., 137, 1083–1110, https://doi.org/10.1175/2008MWR2582.1, 2009. a
Albrecht, B. A.: Aerosols, cloud microphysics, and fractional cloudiness, Science, 245, 1227–1230, https://doi.org/10.1126/science.245.4923.1227, 1989. a
Baker, M. B. and Charlson, R. J.: Bistability of CCN concentrations and thermodynamics in the cloud-topped boundary layer, Nature, 345, 142–145, https://doi.org/10.1038/345142a0, 1990. a, b
Boucher, O., Randall, D., Artaxo, P., Bretherton, C., Feingold, G., Forster, P., Kerminen, V.-M., Kondo, Y., Liao, H., Lohmann, U., Rasch, P., Satheesh, S.K., Sherwood, S., Stevens, B., and Zhang, X.-Y.: Clouds and aerosols, in: Climate change 2013: the physical science basis, Contribution of Working Group I to the Fifth Assessment Report of the Intergovernmental Panel on Climate Change, Cambridge University Press, 571–657, https://doi.org/10.1017/CBO9781107415324.016, 2013. a
Brenguier, J.-L., Pawlowska, H., and Schüller, L.: Cloud microphysical and radiative properties for parameterization and satellite monitoring of the indirect effect of aerosol on climate, J. Geophys. Res., 108, D15, https://doi.org/10.1029/2002JD002682, 2003. a, b
Bretherton, C., Blossey, P. N., and Uchida, J.: Cloud droplet sedimentation, entrainment efficiency, and subtropical stratocumulus albedo, Geophys. Res. Lett., 34, L03813, https://doi.org/10.1029/2006GL027648, 2007. a, b
Caldwell, P., Bretherton, C. S., and Wood, R.: Mixed-layer budget analysis of the diurnal cycle of entrainment in southeast Pacific stratocumulus, J. Atmos. Sci., 62, 3775–3791, https://doi.org/10.1175/JAS3561.1, 2005. a, b
Christensen, M. W. and Stephens, G. L.: Microphysical and macrophysical responses of marine stratocumulus polluted by underlying ships: Evidence of cloud deepening, J. Geophys. Res., 116, D03201, https://doi.org/10.1029/2010JD014638, 2011. a, b
Feingold, G., McComiskey, A., Rosenfeld, D., and Sorooshian, A.: On the relationship between cloud contact time and precipitation susceptibility to aerosol, J. Geophys. Res., 118, 10–544, https://doi.org/10.1002/jgrd.50819, 2013. a
Forster, P., Storelvmo, T., Armour, K., Collins, W., Dufresne, J.-L., Frame, D., Lunt, D., Mauritsen, T., Palmer, M., Watanabe, M., Wild, M., and Zhang, H.: The Earth's Energy Budget, Climate Feedbacks, and Climate Sensitivity, Cambridge University Press, Cambridge, United Kingdom and New York, NY, USA, https://doi.org/10.1017/9781009157896.009, 2021. a
Gerber, H.: Microphysics of marine stratocumulus clouds with two drizzle modes, J. Atmos. Sci., 53, 1649–1662, https://doi.org/10.1175/1520-0469(1996)053<1649:MOMSCW>2.0.CO;2, 1996. a
Glassmeier, F., Hoffmann, F., Johnson, J. S., Yamaguchi, T., Carslaw, K. S., and Feingold, G.: An emulator approach to stratocumulus susceptibility, Atmos. Chem. Phys., 19, 10191–10203, https://doi.org/10.5194/acp-19-10191-2019, 2019. a
Glassmeier, F., Hoffmann, F., Johnson, J. S., Yamaguchi, T., Carslaw, K. S., and Feingold, G.: Aerosol-cloud-climate cooling overestimated by ship-track data, Science, 371, 485–489, https://doi.org/10.1126/science.abd3980, 2021. a, b, c, d, e, f, g, h, i, j, k, l, m, n, o
Goren, T., Kazil, J., Hoffmann, F., Yamaguchi, T., and Feingold, G.: Anthropogenic air pollution delays marine stratocumulus breakup to open cells, Geophys. Res. Lett., 46, 14135–14144, https://doi.org/10.1029/2019GL085412, 2019. a
Gryspeerdt, E., Goren, T., Sourdeval, O., Quaas, J., Mülmenstädt, J., Dipu, S., Unglaub, C., Gettelman, A., and Christensen, M.: Constraining the aerosol influence on cloud liquid water path, Atmos. Chem. Phys., 19, 5331–5347, https://doi.org/10.5194/acp-19-5331-2019, 2019. a, b, c, d, e, f, g, h
Gryspeerdt, E., Glassmeier, F., Feingold, G., Hoffmann, F., and Murray-Watson, R. J.: Observing short-timescale cloud development to constrain aerosol–cloud interactions, Atmos. Chem. Phys., 22, 11727–11738, https://doi.org/10.5194/acp-22-11727-2022, 2022. a, b
Hoffmann, F., Glassmeier, F., Yamaguchi, T., and Feingold, G.: Liquid water path steady states in stratocumulus: Insights from process-level emulation and mixed-layer theory, J. Atmos. Sci., 77, 2203–2215, https://doi.org/10.1175/JAS-D-19-0241.1, 2020. a, b, c, d, e
Hoffmann, F., Glassmeier, F., Yamaguchi, T., and Feingold, G.: On the Roles of Precipitation and Entrainment in Stratocumulus Transitions Between Mesoscale States, J. Atmos. Sci., 80, 2791–2803, https://doi.org/10.1175/JAS-D-22-0268.1, 2023. a, b
Hoffmann, F., Glassmeier, F., and Feingold, G.: The Impact of Aerosol on Cloud Water: A Heuristic Perspective, Zenodo [data set], https://doi.org/10.5281/zenodo.10957468, 2024. a
Kessler, E.: On distribution and continuity of water substance in atmospheric circulations, Meteorol. Monogr., 10, 1–84, https://doi.org/10.1007/978-1-935704-36-2_1, 1969. a
Kostinski, A.: Drizzle rates versus cloud depths for marine stratocumuli, Environ. Res. Lett., 3, 045019, https://doi.org/10.1088/1748-9326/3/4/045019, 2008. a
Mülmenstädt, J. and Feingold, G.: The radiative forcing of aerosol–cloud interactions in liquid clouds: Wrestling and embracing uncertainty, Curr. Clim. Change Rep., 4, 23–40, https://doi.org/10.1007/s40641-018-0089-y, 2018. a
Mülmenstädt, J., Gryspeerdt, E., Dipu, S., Quaas, J., Ackerman, A. S., Fridlind, A. M., Tornow, F., Bauer, S. E., Gettelman, A., Ming, Y., Zheng, Y., Ma, P.-L., Wang, H., Zhang, K., Christensen, M. W., Varble, A. C., Leung, L. R., Liu, X., Neubauer, D., Partridge, D. G., Stier, P., and Takemura, T.: General circulation models simulate negative liquid water path–droplet number correlations, but anthropogenic aerosols still increase simulated liquid water path, Atmos. Chem. Phys., 24, 7331–7345, https://doi.org/10.5194/acp-24-7331-2024, 2024. a
Nicholls, S.: The dynamics of stratocumulus: Aircraft observations and comparisons with a mixed layer model, Q. J. Roy. Meteor. Soc., 110, 783–820, https://doi.org/10.1002/qj.49711046603, 1984. a, b
Platnick, S. and Twomey, S.: Determining the susceptibility of cloud albedo to changes in droplet concentration with the Advanced Very High Resolution Radiometer, J. Appl. Meteorol. Clim., 33, 334–347, https://doi.org/10.1175/1520-0450(1994)033<0334:DTSOCA>2.0.CO;2, 1994. a
Possner, A., Eastman, R., Bender, F., and Glassmeier, F.: Deconvolution of boundary layer depth and aerosol constraints on cloud water path in subtropical stratocumulus decks, Atmos. Chem. Phys., 20, 3609–3621, https://doi.org/10.5194/acp-20-3609-2020, 2020. a, b
Seinfeld, J. H. and Pandis, S. N.: Atmospheric chemistry and physics: from air pollution to climate change, John Wiley & Sons, ISBN 978-1-118-94740-1, 2016. a
Twomey, S.: Pollution and the planetary albedo, Atmos. Environ., 8, 1251–1256, https://doi.org/10.1016/0004-6981(74)90004-3, 1974. a
Twomey, S.: The influence of pollution on the shortwave albedo of clouds, J. Atmos. Sci., 34, 1149–1152, https://doi.org/10.1175/1520-0469(1977)034<1149:TIOPOT>2.0.CO;2, 1977. a
Van Zanten, M., Stevens, B., Vali, G., and Lenschow, D.: Observations of drizzle in nocturnal marine stratocumulus, J. Atmos. Sci., 62, 88–106, https://doi.org/10.1175/JAS-3355.1, 2005. a, b, c
Wang, S., Wang, Q., and Feingold, G.: Turbulence, condensation, and liquid water transport in numerically simulated nonprecipitating stratocumulus clouds, J. Atmos. Sci., 60, 262–278, https://doi.org/10.1175/1520-0469(2003)060<0262:TCALWT>2.0.CO;2, 2003. a, b
Wood, R.: Rate of loss of cloud droplets by coalescence in warm clouds, J. Geophys. Res., 111, D21205, https://doi.org/10.1029/2006JD007553, 2006. a
Wood, R.: Cancellation of Aerosol Indirect Effects in Marine Stratocumulus through Cloud Thinning, J. Atmos. Sci., 64, 2657–2669, https://doi.org/10.1175/JAS3942.1, 2007. a, b
Wood, R.: Stratocumulus clouds, Mon. Weather Rev., 140, 2373–2423, https://doi.org/10.1175/MWR-D-11-00121.1, 2012. a, b