the Creative Commons Attribution 4.0 License.
the Creative Commons Attribution 4.0 License.
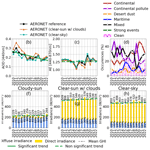
Influence of cloudy and clear-sky partitions, aerosols, and geometry on the recent variability in surface solar irradiance components in northern France
Gabriel Chesnoiu
Nicolas Ferlay
Isabelle Chiapello
Frédérique Auriol
Diane Catalfamo
Mathieu Compiègne
Thierry Elias
Isabelle Jankowiak
Surface solar irradiance (SSI) is a fundamental parameter whose components (direct and diffuse) and variabilities are highly influenced by changes in atmospheric content and scene parameters. The respective importance of cloudy-sky conditions and atmospheric aerosols on SSI evolutions is region dependent and only partially quantified. Here we provide a comprehensive analysis of SSI variabilities recorded in northern France, a region with extensive variability in sky conditions and aerosol loads. Through the application of automatic filtering methods to 1 min resolution SSI ground-based measurements over Lille, sky conditions are classified as clear-sky, 11 %; clear-sun-with-cloud, 22 %; and cloudy-sun situations, 67 % from 2010 to 2022, for which we analyze the statistics and variabilities in the global horizontal irradiance (GHI), beam horizontal irradiance (BHI), and diffuse horizontal irradiance (DHI). Coincident photometric measurements of aerosol properties and radiative-transfer simulations provide the means to conduct a multivariate analysis of the SSI observed trends and year-to-year evolutions and to estimate aerosol and cloud forcings under clear-sun conditions. The analysis of the record value of all-sky GHI in spring 2020 attributes 89 % of the changes to the exceptional sunlight conditions (57 % of clear-sun situations). It highlights also for that season the importance of solar zenith-angle changes, whose positive effects on clear-sun conditions surpass those due to aerosols. Our results show all-sky GHI and BHI positive trends of around +4.0 and +4.4 , respectively, in both spring and summer, which are explained by more than 60 % by an increase in clear-sun occurrences of +1 % yr−1. Additional significant BHI increases under clear-sun conditions are mainly explained in spring by the negative trend in aerosol optical depth (−0.011 yr−1) and partly by angular effects in summer. Moreover, we find that clear-sun-with-cloud situations are frequently marked by irradiance enhancement due to clouds, with 13 % more GHI on a monthly average and 10 % additional diffuse proportion than in clear-sky situations. Under such conditions, clouds add on average 25 W m−2 of diffuse irradiance that sets the GHI at the remarkable level of pristine (aerosol-and-cloud-free) conditions or even higher, by more than +10 W m−2 in summer and for low aerosol loads. Overall, our results highlight the dominant and complex influence of cloudy conditions on SSI, which precedes or combines with that of aerosols and geometrical effects, and leads to a remarkable global level of SSI in clear-sun-with-cloud situations.
- Article
(4863 KB) - Full-text XML
-
Supplement
(1415 KB) - BibTeX
- EndNote
The amount of solar energy that reaches the Earth's surface plays a critical role in the Earth's energy balance and governs a wide range of key physical processes, including evaporation and associated hydrological components, snow and glacier melt, and plant photosynthesis and related terrestrial carbon uptake, as well as the diurnal and seasonal course of surface temperatures. Furthermore, the amount of incident solar irradiance at the surface (SSI) has significant implications for solar energy production technologies and agricultural productivity.
Studies conducted in recent decades, through both observations and modeling, suggest that surface solar radiation is not necessarily constant on a decadal timescale. Instead, it exhibits significant decadal variations, with a worldwide decreasing (dimming) trend until the 1990s followed by an increasing (brightening) trend from then onwards (Wild et al., 2005; Wild, 2009; Liepert, 2002; Norris and Wild, 2007).
Determining the cause of the observed trends in SSI has proven to be a challenging task. However, an increasing number of studies indicate that the aerosol–radiation interaction is likely responsible for an increase in all-sky radiation since 1985 (Philipona et al., 2009; Manara et al., 2016; Ruckstuhl et al., 2008; Wild et al., 2021). It should be noted that the timing and intensity of the minimum cannot be accurately simulated using current aerosol emission inventories, as has been reported by Liepert and Tegen (2002), Romanou et al. (2007), Ruckstuhl and Norris (2009), and Turnock et al. (2015). This is due to the high temporal and spatial variability in clouds and aerosols, which is particularly difficult to represent in models.
Regional studies that record changes in surface radiation while taking into account atmospheric parameters that can impact such changes are crucial, as they provide more insight into the relationship between the variations in these parameters and the changes in surface radiation. A few studies were recently conducted in the Benelux region, especially over the Netherlands (Boers et al., 2017, 2019; van Heerwaarden et al., 2021), and over larger European areas (Pfeifroth et al., 2018; Schwarz et al., 2020) characterized by substantial proportions of clouds (Hahn and Warren, 2007) as well as a high population density that results in elevated levels of atmospheric particulate pollution. Most of these studies have so far primarily focused on the analysis of the impact of clouds and aerosols on the evolution of the global surface solar radiation and did not consider the variability in the direct and diffuse components. However, depending on their optical properties, aerosols and clouds influence incident radiation by reducing the direct component while enhancing the diffuse component. Although the impact on photovoltaic production depends mainly on the total amount of solar irradiation reaching the surface, the performance of all solar conversion systems is also sensitive to the partition between the diffuse and direct components (Kirn et al., 2015; Lindsay et al., 2020; Sengupta et al., 2021).
The study of incident direct and diffuse radiation is particularly important in the context of climate change, as significant changes in the composition of the atmosphere are expected by the end of the century, with varying magnitudes depending on the corresponding SSP (Shared Socioeconomic Pathway) and RCP (Representative Concentration Pathway) scenario (Moss et al., 2010; Hauglustaine et al., 2014; Drugé et al., 2021), leading to potentially very different solar environments. Moreover, mitigating the impact of greenhouse gas emissions on climate change requires the development of alternative methods of energy production. Currently, photovoltaic technologies represent one of the most important and promising technologies, in addition to energy produced by wind turbines. Several studies have investigated the future resilience of renewable installations (Tobin et al., 2018), especially photovoltaic ones (Gutiérrez et al., 2020; Hou et al., 2021; Jerez et al., 2015). These studies show that the future evolution of the solar environment and of the surface temperature will have a significant impact on the future development of these technologies and their production.
In the current study, we analyze the variability in the global surface solar irradiance and its direct and diffuse components in northern France over the years 2010–2022 as measured by the ATOLL (ATmospheric Observations in LiLle) platform, as well as the variability in coincident sub-hourly measurements of aerosol optical properties. Our analysis relies, in particular, on the development of a classification of the sky conditions based on automated processing of irradiance measurements by cloud filters and identification of aerosol class. Aside from obtaining climatologies at our site, our objective is to disentangle and quantify the influence of the different parameters that contribute to the year-to-year irradiance variabilities observed in Lille over the period of 2010–2022. These classifications allow us to distinguish the influence of the occurrence of cloudy and clear-sky situations from the influence of the atmospheric content (aerosols and gases) and of other parameters, such as the solar zenith angle, on the variability in the SSI and its direct and diffuse components. The effect of atmospheric parameter variability is quantified with radiative-transfer simulations in clear-sky conditions.
Section 2 provides a brief overview of the data and methods used to identify cloudy and clear-sky moments. A classification of the aerosol content based on the optical properties measured at the same time as part of the AERONET network is also introduced. The aim of this classification is twofold. On the one hand, it enables a more-precise characterization of the radiative impact of aerosols observed in Lille through the definition of new models of aerosol optical properties, which are used to perform simulations using the radiative-transfer code SOLARTDECO. On the other hand, it facilitates the study of the variability in the aerosol content in Lille. In particular, used in tandem with the classification of the sky conditions and radiative-transfer simulations, it enables the quantification of the contribution of the different atmospheric and geometric parameters to the variability in the surface solar irradiance over the period of 2010–2022, as described in Sect. 3. An analysis of the radiative effect of aerosols and clouds on the downwelling solar radiation at the surface over 2010–2022 is also presented in Sect. 4, with the objectives of quantifying the mean aerosol non-net direct radiative effect in clear-sky conditions and analyzing the combined effects of aerosols and clouds under irradiance enhancement conditions (Pecenak et al., 2016). Finally, Sect. 5 synthesizes the results of our study and outlines the possible directions for future work.
In this section, the measurement datasets, algorithms, and methods used in this study are described. Information on the data availability is given separately at the end of the paper.
2.1 Ground-based measurements from the ATOLL platform
2.1.1 Description of the site
This study relies on the coincident aerosol optical properties and surface solar irradiance measurements routinely performed at the ATOLL platform located in the north of France (50.61° N, 3.14° E; 70 m a.s.l.), 6 km southeast of the Lille downtown area on the rooftop of a building of the University of Lille in Villeneuve d'Ascq. As the metropolitan area of Lille is characterized by a high population density and more than 1.1 million inhabitants, the ATOLL site can be considered suburban. As shown in Fig. 1, with the Belgian border at a distance of roughly 15 km, the site is located very close to the Benelux region, one of the most densely urbanized and industrialized areas in northwestern Europe. It is thus influenced by many anthropogenic emission sources, both regional and trans-regional, with strong contributions from the residential and industrial sectors as well as transport and agriculture, which contribute to relatively frequent particulate pollution episodes (Potier et al., 2019; Favez et al., 2021; Velazquez-Garcia et al., 2023). It should be noted that the site is also subject to a significant maritime influence due to its proximity to the English Channel and the North Sea. According to the Köppen–Geiger climate classification (Beck et al., 2018), the climate is described as Cfb, meaning it is mostly temperate (C) with a warm summer (b) and the absence of a dry season (f). The typical precipitation amount is about 600 to 800 mm yr−1 and is related to a relatively high frequency of clouds throughout the year, with average cloud fraction values ranging between 60 % to 80 % depending on the time of year (Warren et al., 2007).
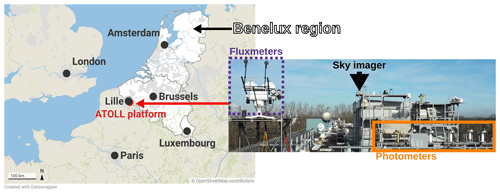
Figure 1Map representing the location of the ATOLL platform (red marker). The white area represents the Benelux region. Adjacent to the map is a picture of the platform including the main instruments used in this study, i.e., the pyronameter and pyrheliometer on a sun-tracking device (purple box), the AERONET sun photometers (orange box), and the sky imager (black arrow). Map created with Datawrapper. © OpenStreetMap contributors 2024. Distributed under the Open Data Commons Open Database License (ODbL) v1.0.
2.1.2 SSI measurements
A Kipp & Zonen CHP1 pyrheliometer mounted on a sun-tracking device measures the incident direct (or beam) normal irradiance (DNI) at the surface in the direction of the Sun with a field of view of 5 ± 0.2°. The beam horizontal irradiance (BHI) is then derived from these measurements using the cosine of the solar zenith angle (μ0=cos (SZA)), as . In addition to the pyrheliometer, a Kipp & Zonen CMP22 pyranometer is also placed on the sun tracker. The pyranometer is paired with a shading sphere that blocks the incoming direct irradiance, allowing for coincident measurements of the diffuse horizontal irradiance (DHI). The global horizontal irradiance (GHI) is then computed as the sum of the BHI and DHI. Such a procedure has the advantage of reducing cosine errors at low sun angles compared to measurements obtained with a single pyranometer (Michalsky et al., 1999). Both instruments measure radiation in the broadband range, between 200–3600 nm for the CMP22 and 200 to 4000 nm for the CHP1. Therefore, we have access to instantaneous measurements (50 ms) of the incoming surface solar irradiance and its direct and diffuse components on an horizontal plane sampled at a 1 min time resolution since February 2009. To guarantee the smooth operation of the system, regular maintenance is performed, including daily cleaning. Moreover, both instruments were calibrated in 2012, 2017, and 2022, and new calibration factors were applied after each calibration, with differences in calibration factors lower than 3 %. Under such operational conditions, the uncertainties in the measurements are expected to be on the order of 2 % to 3 % for the GHI and DHI and 1.5 % for the BHI, as typically reported in the literature (Derimian et al., 2008, 2012; Vuilleumier et al., 2014; Wild et al., 2021). It is worth noting that there are some missing values in the measurement series for winter months of several years, particularly in January and February. This gap occurs because the instruments are regularly sent to either M'Bour (Senegal), for calibration of local instruments, or Delft (the Netherlands), for a recalibration by the manufacturer.
2.1.3 Aerosol measurements
A CIMEL sun/sky photometer from the PHOTONS (PHOtométrie pour le Traitement Opérationnel de Normalisation Satellitaire) network, which is the French part of AERONET (Aerosol Robotic Network; Holben et al., 2001), is used to characterize and monitor aerosol optical and columnar microphysical properties in Lille. The photometer provides direct measurements of the aerosol optical depth (AOD) at six different wavelengths (340, 380, 440, 500, 870, and 1020 nm) along with their corresponding Ångström exponents (AE). In addition, precipitable water vapor content (PWV, in cm) is derived from measurements at 940 nm. AERONET almucantar inversions (Dubovik and King, 2000; Dubovik et al., 2002) of aerosol size distribution, complex refractive index (), and single-scattering albedo (SSA) were also used in this study. However, as they require a relatively large optical air mass (SZA>50°) and a perfectly cloudless sky, the number of available inversions is small (fewer than 500 data points). Their climatological weight is thus relatively limited. Therefore, they have been mainly used to compute site-specific aerosol optical properties through Mie calculations, as described in Sect. 2.3.1. In this study, mostly level 2.0 (cloud-screened and quality controlled) data have been used, except for the measurements from September to December 2022, for which only level 1.5 (cloud-screened only) data were available at the time of writing. The uncertainty in measured AOD is estimated to be approximately 0.02 for the ultraviolet (UV) channels (340 and 380 nm) and 0.01 otherwise (Giles et al., 2019). The reported uncertainty in the PWV measurements is around 10 %–15 % (Pérez-Ramírez et al., 2014; Smirnov et al., 2004), with a dry-bias of around 5 %–6 % (Pérez-Ramírez et al., 2014). The retrieval of the complex refractive index and volume size distribution is a challenging task, and the associated uncertainties are highly variable depending on the observation conditions. For example, the uncertainty in the volume size distribution ranges from 15 % to 100 %, depending on the size of the particles, while the imaginary part of the refractive index (k) varies between 30 % and 50 % for strongly and weakly absorbing aerosols, respectively (Dubovik et al., 2000; Dubovik and King, 2000; Nakajima et al., 1983, 1996).
2.1.4 Coincident aerosol and SSI measurements
For this study, we chose to analyze the period from 2010 to 2022, as it represents the longest period of continuous coincident aerosol and irradiance measurements available. The number of coincident data points is mainly determined by the frequency of AERONET direct observations. In the absence of clouds in the direction of the Sun, the standard time resolution of the photometric measurements was 15 min prior to 2016 and was later increased to 3 min due to a change in the instrument model. Overall, the complete dataset of synchronous aerosol and irradiance measurements comprises approximately 82 000 coincident observations in clear-sun conditions.
2.1.5 Other measurements
In addition to aerosol optical properties and irradiance measurements, other coincidental observations from the ATOLL platform have been used. Notably, sky images captured by a sky imager (CMS Schreder VIS-J1006) since 2009 with a time resolution of 3 min, have been visually examined to assess the cloud cover state over short periods of time. This manual evaluation of the sky images helped validate more systematic cloud-screening methods based on irradiance measurements, as described in Sect. 2.2.1. Meteorological observations of relative humidity (RH) have also been used to perform simulations of the surface solar irradiance in clear-sky conditions, as described in Sect. 2.3.1, while wind speed and wind direction measurements were used to complete the climatological study of the aerosol content and irradiance measurements in Sect. 3.1.
2.2 Classification of atmospheric conditions in Lille
2.2.1 Sky conditions
The presence of clouds in and out of the Sun's direction leads to situations with contrasting levels and characteristics of SSI, for which the sensitivity to atmospheric conditions varies widely. Most notably, the sensitivity of the SSI to aerosol properties is low when clouds are present, aside from some exceptions characterized by thin cirrus or isolated clouds. In order to describe and study the variabilities in the SSI and to analyze differences in its characteristics between different periods or identify the origin of observed temporal trends, we chose to divide sky conditions into three possible categories with contrasting levels of irradiance: clear-sky (CSKY), i.e., no clouds in the sky; clear-sun-with-cloud (CSWC), i.e., clouds are present but not in the Sun's direction; and cloudy-sun (CLOS) situations. The first two categories are characterized by high levels of surface solar irradiance and its direct component, but they differ in their diffuse contributions. In contrast, the third situation is characterized by much lower levels of SSI with a predominant contribution from diffuse radiation. Although AERONET measurements are cloud cleared, they only allow the distinction between clear-sun and cloudy-sun moments. Additionally, their time resolution is lower than that of our irradiance measurements. Therefore, two cloud-screening methods based on the available 1 min irradiance measurements were selected from the literature. The method of Batlles et al. (2000) is used to distinguish clear-sun moments from cloudy-sun situations, while a revised method based on the algorithm of García et al. (2014) is used to isolate clear skies (no clouds, i.e., CSKY) from situations with clouds. These two binary distinctions allow for the intended classification of the sky conditions into three categories: CLOS, CSKY, and CSWC. Both methods were evaluated in Gueymard et al. (2019) and were found to perform particularly well for these distinctions on a 1 min basis. Moreover, the method of García et al. (2014) relies on the popular detection scheme developed by Long and Ackerman (2000) and does not require parallel clear-sky simulations. It also relies on collocated AOD information, which should improve the detection of clear skies under higher aerosol loads compared to the initial method of Long and Ackerman (2000). Both methods used in this study are described in more detail in Appendix A.
In the present study, the performance of the cloud-screening methods has been evaluated by comparison with ground observations of the sky conditions based on the manual inspection of coincident sky images from ATOLL at a 3 min time resolution for the months of January and May 2018. The images were individually analyzed to determine the presence or absence of clouds in the sky and in the vicinity of the Sun. In the latter case, the assessment of clouds in the direction of the Sun was purposefully strict in order to account for the rather-large field of view of the pyrheliometer (5°), which is larger than the Sun's viewing angle (0.5°). The potential motion of clouds into or out of the pyrheliometer's field of view was also considered to account for any time delay between the irradiance measurements and the sky images. Since the output of both manual and irradiance-based estimates is binary, i.e., clear or cloudy, there are only four possible outcomes. This allows the definition of a confusion matrix as follows:
-
true positive (TP), the sky (Sun) is clear in both manual and filter-based estimates;
-
true negative (TN), the sky (Sun) is correctly identified as cloudy;
-
false positive (FP), the sky (Sun) is identified as clear by the cloud-screening method but is manually assessed as cloudy; and
-
false negative (FN), the sky (Sun) is wrongly assessed as cloudy by the algorithm.
The results are presented in Table 1 for both clear-sun and clear-sky conditions simultaneously. For the clear-sky detection, the revised method of García et al. (2014) gives satisfying results, with a low FP score (2.7 %) compared to the TP score (12.5 %), which leads to overall good precision () of 82 %. The latter score highlights that 82 % of the time the sky is correctly identified as clear by the algorithm. This method also presents a relatively low FN score (2.6 %), suggesting that it is also able to accurately isolate cloudy moments (either CSWC or CLOS). This leads to overall satisfactory performance for distinction between clear-sky and cloudy conditions, with a risk () of only 5 % of misidentifying sky conditions.
Table 1Confusion matrix for the estimation of the sky conditions between SSI-based filters and manual ground observations from sky images for January and May 2018 in Lille.
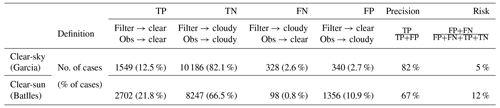
For the identification of clear-sun conditions, the method of Batlles et al. (2000) produces satisfactory but less-optimal results. The method shows strong ability to identify cloudy-sun moments (FN score of 0.8 %) and shows an overall satisfactory risk of only 12 % for the distinction between clear-sun and cloudy-sun conditions. However, its FP and TP scores are closer compared to the Garcia method, and thus its precision at correctly identifying clear-sun conditions is lower (67 %), which might lead to a slight overestimation of the proportion of clear-sun situations. Nonetheless, further analysis indicates that the Batlles FP cases are often due to the presence of cloud edges or clouds of low atmospheric optical depth in the Sun's direction, such as cirrus scenes or small isolated clouds, and that most of the time, 1352 out of 1356 FP cases, DNI values are greater than 120 W m−2. These FP cases, while not clear-sun cases, are thus almost entirely sunny moments (sunshine threshold defined by the WMO) and can be considered quasi clear-sun. In addition, a similar analysis of the performance of the AERONET cloud-screening method in January and May 2018 further confirms the results of the Batlles method, as it highlights comparable performance in distinguishing clear-sun and cloudy-sun conditions, with a satisfactory risk of 13 %.
It should be noted that both methods perform quite well under almost any aerosol load. Both algorithms properly transcribe the global probability density function (PDF) of AOD440 over 2010–2022 from the unfiltered AERONET observations. Comparisons were made with the initial algorithm of Long and Ackerman (2000). The overall precision of this method is higher (90 %), in connection with a much-lower FP score (1.0 %). However, the corresponding AOD probability density function suggests that this algorithm is less representative of the variability in the aerosol content in Lille, as it tends to misidentify clear skies for AOD values greater than 0.3.
Finally, note that irradiance measurements considered in this study were limited to times between sunrise+30 min and sunset−30 min in order to reduce the bias observed in Long and Ackerman (2000) for large solar-zenith angles, as such situations tend to be systematically classified as cloudy by the algorithm. This limitation was chosen to eliminate as many measurements in winter as in summer, which would not have been the case for a criterion based on the solar zenith angle. For the sake of simplification, in what follows, the method of García et al. (2014) will be labeled simply clear-sky (CSKY) and the method of Batlles et al. (2000) clear-sun (CSUN). Furthermore, situations qualified as CSUN but not CSKY will be referred to as CSWC (clear-sun-with-cloud), as they should correspond to moments when the sky is (partially) cloudy without clouds in the Sun aureole.
2.2.2 Aerosol conditions
For the present study, in addition to the classification of sky conditions, a specific classification of aerosol optical properties inspired by the work of Toledano et al. (2007) has been defined. The aim of this classification is twofold. On the one hand, it enables the definition of the new models of aerosol optical properties, which represent typical aerosol conditions encountered in northern France, and can then be used for radiative-transfer simulations as described in Sect. 2.3. On the other hand, it also serves a climatological purpose as it facilitates the characterization of the nature of and variability in the aerosol content and type present in the Lille area over the period of 2010–2022. This classification represents a rough categorization of the aerosol properties in Lille among six classes of measured AOD440 and AE440−870. The definition of each class, as well as the corresponding AOD440 and AE440−870 thresholds and their respective proportions, is summarized in Table 2. A graphical illustration of the classification is also available as Fig. S1 in the Supplement, which represents the scatterplot of AOD440 versus AE440−870 for all AERONET level 2.0 measurements acquired in Lille from 2010 to 2022.
2.3 Radiative-transfer simulations with SOLARTDECO
This section describes the SOLARTDECO radiative-transfer code used in this study. An evaluation of SOLARTDECO radiative-transfer simulations in clear-sky conditions over the period of 2010–2022 is also presented in Sect. 2.3.2 to ensure good transcription of the radiative effect of aerosols in Lille.
2.3.1 Description
The radiative-transfer code SOLARTDECO is a solar version of ARTDECO (Atmospheric Radiative Transfer Database for Earth Climate Observation), a toolbox comprising data of the properties of atmospheric components as well as several radiative-transfer models intended for the simulation of atmospheric radiances and radiative fluxes (Dubuisson et al., 2016). The scientific numerical core is written in Fortran90, while the configuration files and libraries are defined through Python and ASCII formats. It is thus flexible and highly portable, hence its selection for this study. The overall operating scheme of SOLARTDECO described below is also represented Fig. 2.
Initially, ARTDECO was designed for radiative-transfer simulations over the whole ultraviolet (UV) to thermal infrared (IR) range. It works for specific channels (monochromatic mode) as well as for more general spectra through the use of k distributions (Lacis and Oinas, 1991). SOLARTDECO, on the other hand, is dedicated to the study of the incoming surface solar radiation in clear-sky conditions. The spectral resolution of the simulations is defined using the k distribution of Kato et al. (1999), which is composed of 32 spectral bands spanning the solar range (0.24–4.0 µm) and is well suited for the computation of spectrally integrated irradiances. This k distribution is coupled with the gas concentration vertical profiles from Anderson et al. (1986) for a midlatitude summer to represent the gaseous absorption of the atmosphere. The profiles of O3 and H2O are scaled for each simulation with respect to the total ozone (Dobson units) and precipitable water vapor (cm) columnar contents available in the AERONET datasets. Note that SOLARTDECO also accounts for the absorption of CO2 and O2 with homogeneous concentrations of 407 and 209 000 ppmv, respectively.
Regarding the absorption and scattering of solar radiation by aerosols, SOLARTDECO uses a lookup table of extinction coefficients (Cextλ), single-scattering albedo (SSAλ), and components of the phase function (P11, P21, P34, and P44), which are used as inputs for radiative-transfer simulations. The idea is to limit time-consuming Mie calculations and to allow the definition of aerosol optical properties at a higher resolution than that of AERONET inversions, which are quite rare (less than 500 data points over 2010–2022) due to requirements regarding the optical air mass, AOD, and need for a perfectly cloudless sky (Dubovik et al., 2002). This lookup table relies on the AOD440 and AE440−870 classification defined in Sect. 2.2.2, as well as surface relative-humidity measurements from ATOLL, which are used to split AERONET inversions of volume size distribution and refractive index into several subsets. Note that level 2.0 inversions were used for classes that present AOD values greater than 0.4 (i.e., Continental polluted, Desert dust, Strong event, and Mixed), while level 1.5 data were used otherwise, provided that apart from the AOD threshold, all the other criteria were met. For each subset of the lookup table, the size distribution is divided into two modes (fine or coarse). Then, datasets of Cextλ, SSAλ, and phase function components are computed for each mode through Mie calculations based on the normalized mean number size distributions (i.e., number size distribution of each mode divided by the corresponding number concentration) and the total mean complex refractive index. Overall, the lookup table includes 120 datasets divided between the 2 modes, 6 classes, and 10 relative-humidity bins. These datasets are used to compute the total aerosol optical properties needed for each radiative-transfer simulation at the resolution of AERONET direct-sun measurements by mixing the pre-computed properties of the fine and coarse modes selected based on AOD440, AE440−870, and surface relative-humidity measurements. The methodology adopted in the present study to compute the total aerosol optical properties is described in detail in Appendix B. It involves solving a system of two equations with two unknowns for each simulation, based on the measured values of AOD440 and AE440−870 with the additional use of AOD550 data. The two coefficients derived from this system are then used, together with the mean number concentrations of the fine and coarse modes derived from AERONET size distributions, to mix the pre-computed optical properties of the two modes specific to each simulation. It is worth mentioning that the chosen system of two equations with two unknowns involving AOD440 and AE440−870 measurements was chosen as it shares inputs with the lookup table of aerosol optical properties. Furthermore, proxy simulations were also conducted with another parameterization based on a more common system involving the measured AOD440 and AOD870. Comparisons with ground-based measurements, similar to those presented for the system chosen in the following section, show very comparable results (Fig. S2 in the Supplement). Note that following the mixing of the two modes, the total columnar aerosol extinction for the simulation is scaled using the corresponding measured AERONET AOD550,meas as follows:
where AODλ,mix represents the total columnar aerosol optical depth at wavelength λ used for the simulation and Cextλ,mix the new extinction coefficients computed by mixing the corresponding fine and coarse modes. Although, this equation only represents the total columnar AOD, i.e., from the surface to the top of the atmosphere, AOD at other altitudes can be derived given the vertical profile of the aerosol layer. In this study, the aerosol layer was defined as an exponential decay of the aerosol density with a 2 km scale height (i.e., , with z the altitude in km). As only surface solar irradiance outputs were analyzed in this study, our results do not greatly depend on the vertical profile of the aerosol layer.
Finally, the remaining inputs of SOLARTDECO include the solar zenith angle and surface optical properties. For simplicity, this study assumes a Lambertian surface with a spectrally homogeneous albedo arbitrarily set at 0.15. The aforementioned properties of the atmosphere (gaseous absorption and aerosol extinction) and surface reflectivity then allow for computation of the incoming and outgoing spectral solar irradiances in clear-sky conditions between the top of the atmosphere and the surface through the use of the radiative-transfer model DISORT (Stamnes et al., 1988).
2.3.2 Validation of SSI simulations over 2010–2022
Figure 3a–c represent scatterplots of the differences between SOLARTDECO simulations and measurements of (a) GHI, (b) BHI, and (c) DHI over the period of 2010–2022 in Lille for all AERONET observations performed between sunrise plus 30 min and sunset minus 30 min in clear-sky situations (44 239 comparisons). Identification of clear-sky situations comes from the algorithm presented in Sect. 2.2.1. Simulated and measured mean flux values and associated standard deviations are plotted on the axes. Comparison statistics such as the mean bias (MBD), mean absolute bias (MAD), and root-mean-square deviation (RMSD) are also shown in each figure in a beige box.
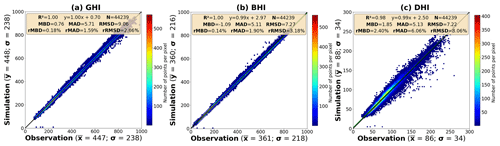
Figure 3Scatterplot of SOLARTDECO simulations based on level 2.0 AERONET inputs versus corresponding measurements of (a) GHI, (b) BHI, and (c) DHI performed in Lille over the period of 2010–2022. The black line represents the 1:1 line. Mean SSI values and associated standard deviations are shown on their respective axes for both simulations and observations. Absolute and relative values of MBD (mean bias difference), MAD (mean absolute difference), and RMSD (root-mean-square deviation) are also displayed in the beige boxes included in the different figures. Only observations that coincide with clear-sky flux measurements (identified by the algorithm presented in Sect. 2.2.1) and performed between sunrise plus 30 min and sunset minus 30 min are considered for these comparisons.
Overall, the performance of SOLARTDECO simulations is satisfactory for all irradiance components, with RMSD values lower than 10 % for the DHI (8.06 %) and 5 % for the BHI (3.18 %) and GHI (2.66 %), which are comparable to the results of the best-performing clear-sky irradiance models assessed in the latest studies of Sun et al. (2019) for the GHI and Sun et al. (2021) for the BHI and DHI. Note however that the Sun et al. (2019, 2021) validations were obtained using reanalysis atmospheric data from MERRA2 (instead of AERONET as was used here) and based on a larger number of worldwide stations of ground-based irradiance observations. Moreover, the performance of SOLARTDECO is well within the error margins expected for network-operational instruments (World Meteorological Organization, 2008), as mean absolute differences (MAD) are close to the resolution of network instruments (5 W m−2) for all irradiance components, and more than 95 % of the comparisons have mean differences lower than ±20 W m−2.
A focus on the years 2018 and 2019 shows that the performance of SOLARTDECO is also comparable to that of the radiative-transfer tool SOLARES under clear-sky conditions (also identified using the modified Garcia algorithm) in Lille over the same period (Elias et al., 2024).
However, SOLARTDECO does appear to slightly overestimate the DHI. This discrepancy could be linked to the circumsolar contribution, i.e., the diffuse part of the incident solar irradiance located in the vicinity of the direction of the Sun (0.6 to 8°). Indeed, the circumsolar contribution is measured by the instruments as part of the incident direct irradiance at the surface, while it is generally accounted for as part of the diffuse irradiance in radiative-transfer simulations. This difference between simulations and ground measurements, which represents at most 2 % to 3 % of the DNI in the majority of cloud-free events (Gueymard, 2001, 2010; Blanc et al., 2014), usually leads to an underestimation of the BHI and overestimation of the DHI in radiative-transfer simulations. This is in accordance with the overestimation of the diffuse irradiance by SOLARTDECO. However, no underestimation of the BHI is reflected by the mean biases, which could indicate the existence of other biases with opposite effects.
2.4 Multivariate analysis of SSI variability
In the present study, we undertake a multivariate analysis of the variability in the surface solar irradiance in Lille over the period of 2010–2022. The objective is to disentangle the contributions of the change in sky conditions (presence or absence of clouds, aerosol class and loading, and atmospheric parameters) and of geometrical conditions to the observed variabilities in the SSI. This section presents the methodology for the multivariate analysis (Sect. 2.4.1), as well as the sensitivity study of the SSI to input parameters that are used to perform the multivariate analysis (Sect. 2.4.2). For the purpose of this study, this methodology has been applied to analyze trends and year-to-year variability in the SSI over the period of 2010–2022 (Sect. 3.2) in spring (i.e., March–April–May) and summer (i.e., June–July–August). Nonetheless, it is worth mentioning that it could also be applied at other temporal resolutions, including monthly and intra-daily scales.
2.4.1 Methodology
The seasonal mean all-sky solar irradiance can be written as a weighted sum of the observed irradiances per sky condition:
where k represents the sky condition (CLOS, CSWC, or CSKY), Fk corresponds to the associated measured seasonal mean irradiances (GHI, BHI, or DHI), and freqk represents the yearly mean frequency of the kth sky condition for the corresponding season.
With this approach it is possible to isolate the contributions of the change in frequency of occurrence of sky conditions and of the change in irradiance under each sky condition to both inter-annual variability and trends in all-sky irradiances. Indeed, this decomposition allows the analysis of the observed differences between the year y and the overall mean over the period of 2010–2022:
where Fk,y and freqk,y represent the mean seasonal irradiances and frequencies of the various sky conditions for the year y, and the terms ΔFk,y and Δfreqk,y correspond, respectively, to the observed differences in irradiance and frequency of sky conditions between the seasonal averages for the year y and over the whole period of interest. With this framework, the term represents the contribution of the change in the sky's frequency of occurrence to the variability ΔFASKY,y, while the term corresponds to the contribution of the change in irradiance observed under each sky condition.
A similar approach can be used to decompose the eventual observed trends under all-sky conditions over the period of 2010–2022, following Eq. (4):
where Fk and freqk are the same as in Eq. (3), while and correspond, respectively, to the slopes of the observed seasonal trends in irradiances and frequencies of the various sky conditions. Again, the terms and represent the influence of the trends in the occurrences of the sky conditions and the trends in other atmospheric parameters, respectively.
This analysis of the different contributions to the observed irradiance variabilities can be extended by further decomposing the terms related to the variability in the irradiances observed under each sky condition that we note here: CSKY, CSWC, and CLOS. We describe here a second and third step, for which we decompose the irradiance variability only in the case of the CSKY and CSWC (i.e., CSUN) situations by accounting for changes in the occurrence of the five main aerosol classes and changes in solar irradiances per aerosol class. Note that for CSWC conditions, this is only valid for the BHI that is not influenced by clouds.
As in the previous paragraph, the seasonal mean solar irradiance for CSKY and CSWC conditions can be written as a weighted sum of the observed mean irradiances per aerosol class:
where Fclear represents the mean seasonal irradiances measured under clear-sky or clear-sun-with-cloud conditions, and the terms freqi and Fi correspond to the mean frequencies and irradiances of the ith aerosol class1 (i.e., Continental, Continental polluted, Mixed, Maritime, and Desert dust) for the same conditions (CSKY or CSWC). Then, similarly to the approach used under all-sky conditions, it is possible to isolate the contribution of the change in aerosol class frequency and the change in irradiance per aerosol class for both the inter-annual variability (Eq. 6) and the trends (Eq. 7):
where ΔFi,y and Δfreqi,y represent the observed differences in irradiances and frequencies of the different aerosol classes between the seasonal averages for the year y and over the period of 2010–2022, and the terms and correspond to the slopes of the observed seasonal linear trends in irradiances and frequencies of the various aerosol classes. In this form, ΔFi,y and result from the variabilities in the parameters of every scene (AOD, SSA, SZA, PWV, etc.) to which the irradiances are sensitive. In order to isolate and identify the impacts of each parameter, it is possible to further decompose these terms following Eqs. (8) and (9):
where corresponds to the seasonal sensitivity of the irradiances to the various x parameters (AOD, SZA, SSA, etc.), and the terms Δxi,y and represent the temporal variations (for a given season) of the considered parameter for the ith aerosol class. Note that the comparisons between SOLARTDECO simulations based on AERONET measurements and irradiance measurements from ATOLL under clear-sky conditions in Lille showed satisfactory results (Sect. 2.3.2). This validates the modeling of both the aerosol optical properties and the radiative-transfer processes. Hence, we use SOLARTDECO simulations with confidence to compute the sensitivities of irradiances to the various atmospheric parameters, as described in Sect. 2.4.2.
2.4.2 Sensitivity study of clear-sky SSI
The sensitivity of clear-sky irradiances, F, to each input of SOLARTDECO, x, is quantified by the computation of partial derivatives, which are obtained by imposing a small perturbation, δx, on the value of x as in Eq. (10):
where are the other parameters besides the variable of interest, x, needed to compute the clear-sky irradiances, F (i.e., GHI, BHI, or DHI). As in Thorsen et al. (2020), the perturbation of the parameter x is taken as an arbitrary increase of 1 % of the base value, which should be simultaneously small enough to cause a linear impact on the irradiances and large enough to avoid noise from numerical truncation errors. The analysis of these sensitivities is interesting in itself, as it provides an understanding of the importance of each parameter and how accurately it should be defined. It also shows how different the sensitivities of the direct and diffuse irradiances can be, and how they can lead to reduced sensitivities of GHI through compensation mechanisms. It also indicates the possible importance of aerosol's nature that interacts differently with solar radiation over the solar spectrum. As the values of the scene-related parameters have different magnitudes (e.g., SZA versus AOD), we chose to provide here values of the logarithmic sensitivity:
where F represents the irradiances (GHI, BHI, or DHI) and x the parameter of interest. The logarithmic sensitivities thus represent the response of the irradiances (in %) to a relative variation in the input parameter (here an increase) of 1 %.
Table 3a presents the logarithmic sensitivities of the clear-sky irradiances to the input parameters of SOLARTDECO (aerosol-related parameters are grouped) in the case of the most-frequent aerosol class in Lille, i.e., the Continental class. The sensitivities are calculated with a fixed set of properties that correspond to mean values observed in clear-sky conditions over the period of 2010–2022 (Table S1 in the Supplement). Note that for the aerosol layer height (Haer), we used the fixed input value (2 km) from SOLARTDECO described in Sect. 2.3.1 as the reference. The mean value of fine fraction, ff, used in this study comes from AERONET estimates based on the method of O'Neill et al. (2003). Moreover, as the number of available AERONET inversions of SSA is relatively small and thus less representative, the mean single-scattering albedo is based on the aerosol optical properties computed by mixing the fine and coarse modes (Eq. B9) for each clear-sky simulation over 2010–2022. This is valid, as the satisfactory results of SOLARTDECO simulations suggest a good transcription of aerosol optical properties derived for the mixing of the fine and coarse modes. Overall, our sensitivity results show that the most important parameter is the solar zenith angle, the 1 % increase (0.6°) of which induces a 2.5 % decrease in the GHI. The second-most-significant parameter is the single-scattering albedo that affects only the diffuse component. The third-most-important parameter is the aerosol optical depth, although the compensation between two opposite-in-sign effects on BHI and DHI leads to an overall effect on GHI on the order of the effects of the PWV variation. We noticed that an increase in the fine fraction for a constant AOD550 does increase the BHI and decrease DHI, which results from an overall decrease in the aerosol extinction over the solar spectrum, the consequence of a higher Ångström exponent. Sensitivity results in the case of larger particles, e.g., Maritime, not shown here, show sensitivities to AOD 5 % to 10 % higher in magnitude. It also shows a sensitivity to ff 2 or 3 times higher in magnitude. Both differences can be attributed to a modification of the aerosol optical properties over the whole solar spectrum.
Table 3Logarithmic sensitivities of global (GHI), direct (BHI), and diffuse (DHI) SSI to SOLARTDECO input parameters (the parameters related to aerosol properties are grouped) in the case of the Continental aerosol class. Results are given in percentages and equal the relative variation in irradiances for (a) a 1 % variation and (b) a variation on the order of the coefficient of variation for each input parameter. Computations were carried out for the Continental aerosol class using a fixed set of parameters based on average properties observed under clear-sky conditions over 2010–2022. The parameter ff represents the aerosol fine-mode fraction, RH the relative humidity, PWV the precipitable water vapor content, Haer the aerosol layer height (fixed at 2 km), and α the surface albedo.
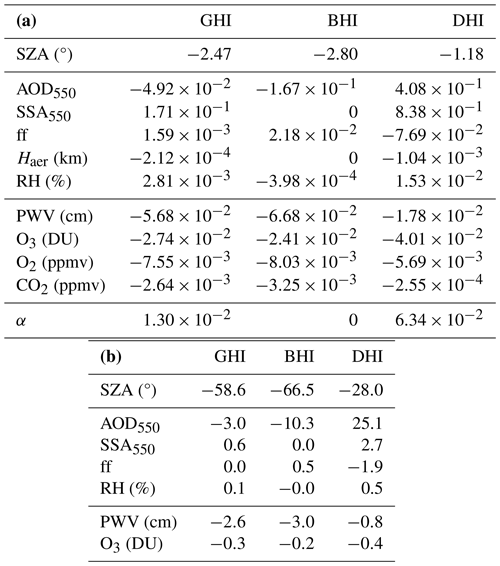
It is nevertheless biased to compare the irradiance sensitivities to all parameters as in Table 3a, as these parameters actually have different ranges of variation. It is thus more pertinent to account for these different ranges by multiplying each logarithmic sensitivity by the coefficient of variation in each parameter, defined as the ratio between the standard deviation and the associated mean value of this parameter observed in Lille. Table 3b provides the sensitivities of the irradiances (in %) to the various parameters for changes on the order of the coefficient of variation, still in the case of the Continental aerosol class. Note that the sensitivities to the surface albedo (α), oxygen (O2) and carbon dioxide (CO2) concentrations, and aerosol layer height (Haer) are not represented, as these parameters are constant in our simulations. Accounting for the variation in the input parameters, the clear sky irradiance sensitivities in Lille are thus, in decreasing order, related to the solar zenith angle, the aerosol optical thickness, the precipitable water vapor content, the single-scattering albedo, and the fine fraction, with relatively weak impacts (<1 % for all irradiance components) of the relative humidity and ozone content. The ordering of the sensitivities to SSA and PWV are inverted when considering only the diffuse irradiance. These results indicate the primary importance of the SZA: a temporal change in a clear-sky condition can lead to a significant change in the solar irradiance through modification of both the optical air mass and the horizontal projection of radiances, which affects the direct more than the diffuse horizontal irradiance. Also, a typical increase in aerosol loading () would lead to a 10 % decrease in BHI, a 25 % increase in DHI, and a 3 % decrease in GHI. A more-thorough analysis of the global non-net direct radiative impact of aerosols on the solar irradiance in Lille over 2010–2022, based on SOLARTDECO simulations as well as ATOLL irradiance measurements, is presented in Sect. 4 for clear-sun conditions, with seasonal and yearly averages and a distinction by aerosol class.
Finally, it is important to note that the sensitivities presented in this section are only for the Continental class and over the entire period of 2010–2022. Specific sensitivities, which vary according to the time period considered as well as the aerosol class, have been computed in order to perform the multivariate analysis of inter-annual variability and trends presented in Sects. 3.2.1 and 3.2.2.
In this section, the results of the joint analysis of the simultaneous measurements conducted in Lille over the period of 2010–2022 are presented. This analysis relies on classifications of both the sky conditions and aerosol optical properties introduced in Sect. 2, as well as on the radiative-transfer simulations (in clear-sky conditions) of SOLARTDECO. The objectives are to obtain a climatology of the solar environment in Lille over the past decade (Sect. 3.1) and examine the influence of clouds, aerosols, and gases on the variability in surface radiation in northern France (Sect. 3.2).
Firstly, in Sect. 3.1.1, the seasonal variability in solar irradiance, aerosol optical properties, and sky conditions is examined. Then we focus in Sect. 3.1.2 on the inter-annual variability in spring (i.e., March–April–May) and summer (i.e., June–July–August) over the period of 2010–2022. Finally, Sect. 3.2 presents the results of the multivariate analysis of the year-to-year variability and trends in surface solar irradiances in spring and summer, based on the methodology described in Sect. 2.4.
3.1 Recorded variabilities in sky conditions, aerosols, and SSI
3.1.1 Mean climatology and seasonal cycle
Figure 4a–h present the average monthly variations during daytime over the 13-year period of 2010–2022 in different measured and derived atmospheric quantities associated with the solar environment in Lille: Fig. 4a – sky conditions identified using the irradiance-based cloud-screening methods described in Sect. 2.2.1; Fig. 4b–d – aerosol properties from AERONET measurements; and Fig. 4e–h – irradiances measured for the different sky conditions.
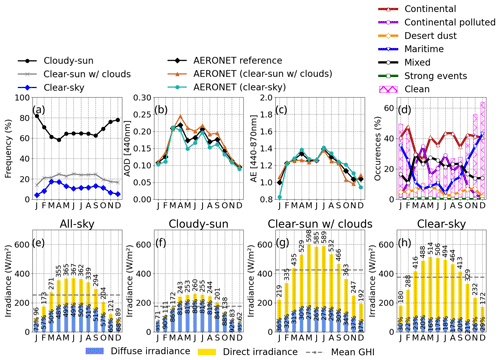
Figure 4Monthly variations in Lille over the period of 2010–2022 for several quantities derived from ATOLL measurements. (a) Frequencies of cloudy-sun (black line), clear-sun-with-cloud (grey line), and clear-sky moments (blue line) in percentages. (b) Monthly mean values of AOD440 for all AERONET measurements (i.e., AERONET reference; black line) and for the clear-sun-with-cloud (orange line) and clear-sky (green line) conditions. (c) Same as (b) but for the AE440−870. (d) Proportions of the different classes presented in Table 2: Continental (red line), Continental polluted (purple line), Desert dust (orange line), Maritime (blue line), Mixed (black line), and Strong event (green line) classes. The proportion of clean situations (AOD440≤0.1) for all coincident AERONET measurements is also represented as pink columns. (e–h) Mean monthly SSI for different cloud cover states: (e) all-sky, (f) cloudy-sun, (g) clear-sun-with-cloud, and (h) clear-sky conditions. Only values during daytime between sunrise+30 min and sunset−30 min are considered. The GHI is represented as columns with the lower, blue part corresponding to the DHI and the upper, yellow part to the BHI. The dashed grey lines represent the mean GHI over 2010–2022. Note that the percentages reflect the contribution of the DHI to the overall mean yearly GHI, the values of which are reported above each column.
The results of our cloud-screening approach confirm the significant influence of clouds in Lille observed by Warren et al. (2007). Over the 13-year period considered, we find that, on average, the Sun is obscured by clouds (CLOS situations) in nearly 67 % of cases, while completely clear skies are observed only 11 % of the time and intermediate conditions of partly cloudy skies with a visible Sun (CSWC conditions) represent only an average of 22 % of observed situations. Thus, the Sun is clear of clouds 33 % of the time (CSUN conditions). Note that CLOS situations also encompass instances characterized by thin clouds or clouds partially covering the Sun (cloud edges), which have a limited impact on incident solar radiation, with direct normal irradiance (DNI) values exceeding 120 W m−2 (sunshine criterion defined by the World Meteorological Organization; WMO, 2003). On average over the period of 2010–2022, these situations, which can be considered sunny, represent 9 % of observed conditions and account for about 13 % of cloudy-sun situations. The maximum (minimum) of CSKY (CLOS) occurrence happens in March–April. Clear-sky conditions are at a minimum in winter, with average occurrences of only around 5 %, associated with a maximum of cloudy-sun conditions of around 80 %. A relatively stable plateau of minimum (maximum) cloudy-sun (clear-sun) monthly proportions, with values consistently lower (greater) than 65 % (11 % and 24 % for clear-sky and clear-sun-with-cloud conditions), is also noticeable from March to September, i.e., spring (March–April–May) and summer (June–July–August).
Figure 4b–d illustrate the monthly variability in aerosol optical properties in Lille over the period of 2010–2022. Panels (b) and (c) represent the mean values of AOD and AE, respectively, for all AERONET measurements, as well as individually for situations coincident with clear-sky and clear-sun-with-cloud observations. In addition, panel (d) illustrates the monthly variability in the occurrence of the six aerosol classes defined in Sect. 2.2.2, as well as the monthly proportion of clean situations (AOD440≤0.1), considering all AERONET measurements. Overall, Lille appears to be a fairly polluted site, as only 27 % of AOD440 measurements show values lower than 0.1. Table 2 shows that the main contribution is from the Continental class (38 %), followed by Mixed cases (22 %) and Continental polluted events (20 %). Hence, aerosols observed in Lille are mostly related to anthropogenic activities, as fine particles prevail at least 58 % of the time. However, a non-negligible contribution of coarse particles is observed, as the Maritime and Desert dust classes represent 14 % and 5 % of the observations, respectively (Table 2). Note that the proportion of Desert dust influence obtained from our aerosol classification in clear-sun conditions in Lille is consistent with that previously provided by Putaud et al. (2010) based on particulate matter measurements in all-sky conditions, as these authors estimate a mean proportion of Desert dust particles in the range of 5 % to 12 % in the north of Europe. Moreover, Fig. 4b–d show that the period from March to September, characterized by a higher proportion of clear-sun conditions, is also significantly influenced by aerosols, with monthly mean AOD440 values greater than 0.15 (Fig. 4b). The stability of the associated Ångström exponents (values greater than 1.2; Fig. 4c) indicates a significant influence of fine particles, primarily from anthropogenic emissions, reaching a maximum in April (monthly mean values of AOD440 exceeding 0.2), with a minimum proportion of AOD measurements below 0.1 (clean conditions) of only 11 % (Fig. 4d). Spring is indeed characterized by a significant increase in agricultural activity, including fertilizer spreading in open fields that releases substantial amounts of ammonia into the atmosphere, which contributes to the formation of secondary aerosols (Hauglustaine et al., 2014). Moreover, the predominantly northeast wind flow observed in spring in Lille (Fig. S4b in the Supplement) could be the result of a higher frequency of anticyclonic conditions during this season. Overall, this would explain the lower frequency of cloudy moments and the accumulation of anthropogenic particles originating partly from the Benelux region. The accumulation of anthropogenic particles is consistent with the strong influence of the Continental polluted aerosol class, reaching a maximum monthly average of about 35 % around April (Fig. 4d). Conversely, in winter, when cloudy conditions are more frequent (Fig. 4a), the monthly mean values of AOD generally remain below 0.12, reaching a minimum of 0.09 in December (Fig. 4b). This winter drop in AOD is associated with a decrease in the monthly mean AE (Fig. 4c). These two decreases result from a higher proportion of the Maritime aerosol class, with monthly proportions exceeding 35 % for winter months (Fig. 4d). This finding aligns with surface wind direction and speed measurements from the ATOLL platform, which highlight prevailing westerly winds (Fig. S4a) that are less influenced by continental anthropogenic activities.
Figure 4e–h represent the monthly variability in the incident global surface flux in Lille over the period of 2010–2022, as well as the partitioning between direct and diffuse components for (e) all-sky (ASKY), (f) cloudy-sun (CLOS), (g) clear-sun-with-cloud (CSWC), and (h) clear-sky (CSKY) conditions. Note that only measurements performed between sunrise+30 min and sunset−30 min are considered, as the identification of the sky state performs better during this period.
Overall, the climatological yearly mean value of global irradiance is at a minimum in cloudy-sun conditions (192 W m−2), when the Sun is obscured by clouds. In comparison, the measured surface flux in clear-sky conditions is twice as high, with an average value around 375 W m−2. The maximum irradiance is observed for clear-sun-with-cloud conditions, which, due to the cloud side effects on the diffuse component, show a mean value around 424 W m−2 (13 % more than clear-sky). Thus, in conjunction with the frequency of each sky condition (Fig. 4a), the average solar radiation incident in Lille under all-sky conditions is approximately 253 W m−2. In addition to the discrepancies in GHI, it is worth mentioning that each sky condition is associated with varying proportions of direct and diffuse irradiance. In particular, the yearly mean diffuse ratio observed in Lille over the period of 2010–2022 varies between a minimum of 22 % in CSKY conditions and a maximum of 87 % in CLOS situations, with intermediate values of 32 % and 56 % in CSWC and ASKY conditions. This broad range of values highlights the predominant effects on the measured surface global irradiance of clouds in Lille and their position relative to the Sun and the observer.
Figure 4e–h show that the seasonal variations in the mean measured irradiances for all-sky states display almost symmetrical inverted U-shapes over the year that are linked to changes in solar zenith angle (Fig. S3a–d in the Supplement) and thus optical air mass, which are greater in winter (minimum GHI) than in summer (maximum GHI). Moreover, the variability in the optical air mass has a large influence on the proportion of diffuse irradiance, which varies under all-sky conditions from 51 % in summer to more than 65 % in winter. Note that in all-sky conditions, the influence of the optical air mass is enhanced by changes in occurrence of the sky conditions, as the frequency of cloudy-sun conditions (Fig. 4a) is greater (lower) in winter (summer), leading to overall lower (greater) mean ASKY GHI values. The influence of clouds is particularly important under CSWC conditions, as clouds, through 3D effects, enhance the amount of diffuse radiation reaching the surface. Most notably, our results highlight that due to the additional contribution of clouds, the monthly averages of global flux are, throughout the year, consistently higher for CSWC conditions (Fig. 4g) than for CSKY situations (Fig. 4h), with relative differences varying between +4 % in March and +21 % in January and with an absolute maximum difference of 95 W m−2 in July. Under CSWC conditions, the gain from clouds results in diffuse flux values around 170 W m−2 on average in summer, which are relatively comparable to those observed in CLOS conditions, reaching around 200 W m−2 on average for the same season. In relative terms, the contribution of diffuse radiation is, however, significantly lower in CSWC conditions, with an average proportion of DHI around 28 % in summer compared to 81 % in CLOS conditions. CLOS conditions thus typically offer very low direct radiation and a moderate amount of diffuse radiation, leading to global surface radiation levels that are 2 to 3 times lower, depending on the month, compared to other situations.
To conclude, our joint analysis of the seasonal variability in the solar environment suggests that the period covering spring and summer is characterized by relatively high amounts of incident radiation and surface energy, linked to smaller solar zenith angles and longer day lengths. Both seasons are thus particularly interesting in terms of solar energy exploitation, as the period from March to September represents nearly 80 % of the total energy accumulated over a year (approximately 1 MWh m−2; Fig. S3a). Hence, we chose to focus our study of the inter-annual variability in the solar environment in Lille over the period of 2010–2022 (Sect. 3.1.2) on spring and summer seasons. Note that as these two seasons are generally characterized by a lower influence of clouds and relatively high levels of AOD, they are of great interest for the study of the impact of aerosols on the SSI and its direct/diffuse partition.
3.1.2 Year-to-year evolution in spring and summer
Figures 5 and 6 represent the year-to-year evolution from 2010 to 2022 of daytime sky conditions (Figs. 5a and 6a), aerosol properties obtained from AERONET measurements (Figs. 5b–d and 6b–d), and surface solar irradiances measured for the different sky conditions (Figs. 5e–h and 6e–h) in Lille in spring and summer, respectively. Figures 5 and 6 show some local extrema of interest for these quantities, such as the maximum of irradiance and clear-sky occurrence for spring 2020. They show also some interesting trends over the past decade, some of which are statistically significant.
The 2010–2022 yearly averaged occurrence of clear-sky (CSKY) conditions represents 15 % of the observations in spring, whereas in summer it is substantially lower, corresponding to 11 %. The Sun is obscured by clouds (cloudy-sun, CLOS) 62 % of the time in spring and 65 % in summer, on average. The Sun is clear and surrounded by clouds (clear-sun-with-cloud, CSWC) 23 % and 24 % of the time in spring and summer, respectively. For both seasons, the year-to-year variabilities reported in Figs. 5a and 6a show decreasing linear trends in the frequency of CLOS conditions (of about −0.9 % yr−1) and inversely increasing trends in the frequency of CSWC and CSKY conditions (of about +0.4 % to 0.5 % yr−1). Precise values of the trends are given in Table 4. As highlighted in bold in Table 4 and with solid green lines in Figs. 5a and 6a, these seasonal trends are statistically significant (application of Mann–Kendall trend tests, Mann, 1945, and Kendall, 1990, with a significance level of 5 %) only in summer and only for the occurrences of CLOS and CSWC situations. These trends are issued from only 13 years of data and rely on the imperfect filtering of sky conditions. Their scope should thus be considered with caution, as they are quite sensitive to year-to-year variability and as uncertainty in the occurrence of sky conditions impacts their validity. However, it is worth noticing that the strongest (significantly decreasing) trend in CLOS situations was found to be associated with high confidence in the related occurrences (1.2 % of misidentifications) and that this observation is consistent with results from CM SAF data (C3S, 2024) that, since 2010, show repeated negative annual anomalies in cloud cover and positive ones in sunshine duration over European land areas and relative to the reference period of 1991–2020. It does encourage a multivariate analysis that includes the observed variabilities in the observed occurrence trends as in Eq. (4). In spring, the trends in sky conditions are not statistically significant (Table 4a), possibly due to the higher year-to-year variabilities recorded for this season. The spring of 2020 is particularly exceptional, with 35 % of CSKY occurrences and only 43 % of CLOS situations. An anomaly with very low cloud fraction was also observed in Cabauw (the Netherlands) in spring 2020 by van Heerwaarden et al. (2021). This study showed that the particular anticyclonic meteorological conditions of that spring were associated with record-breaking all-sky global irradiance measurements in Cabauw. These conditions were also characterized by a relatively low contribution of diffuse radiation, accounting for 38 % as opposed to over 50 % on average in spring in Cabauw. Similarly, in Lille, a high average global irradiance under all-sky conditions was observed in spring of 2020 (389 W m−2), representing the maximum GHI over the whole period of 2010–2022 for the spring season (Fig. 5e). This remarkable value is 55 W m−2 higher (+16 %) than the spring average observed over the period of 2010–2022 (334 W m−2). Additionally, the low contribution of the DHI (36 %), similar to that measured in Cabauw, is notably lower than the seasonal averages observed in spring in Lille, while the other years of 2010–2022 ranged between 43 % and 60 %. A detailed analysis of the contribution of the variability in the aerosol content and of the occurrence of sky conditions to the maximum solar radiation in the spring of 2020 is presented in Sect. 3.2.1.
Table 4Trends per year in frequency of sky conditions (a), aerosols (b), and SSI (c) recorded in Lille in spring and summer over the period of 2010–2022. Only parameters with at least one statistically significant trend (Mann–Kendall test, highlighted in bold text) in spring or summer are reported. Note that other parameters such as the solar zenith angle, precipitable water vapor content, and Ångström exponent were also investigated; however, no significant trends were observed.
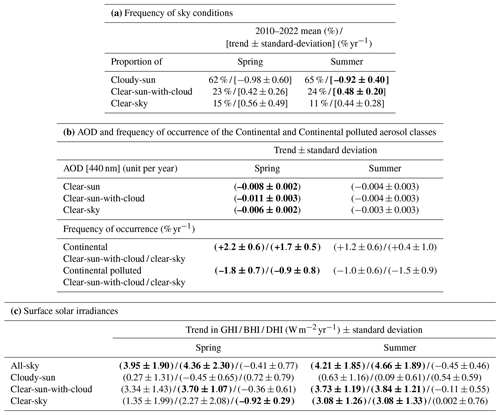
Figures 5b–d and 6b–d represent the variations in the yearly averaged aerosol content and properties in Lille over the period of 2010–2022 in spring and summer, respectively. For both seasons, strong fluctuations in AOD440 are observed (Figs. 5b and 6b) when considering all reference AERONET measurements (i.e., clear-sun measurements; black line) as well as only those coincident with clear-sun-with-cloud (orange line) and clear-sky (green line) observations. In spring, our measurements show a significant decreasing trend in AOD440 over the period of 2010–2022 (−0.008 ± 0.002 yr−1) from a yearly mean value of 0.26 in 2010 to around 0.20 in 2022. By comparison, the observed negative trend in summer (−0.004 ± 0.003 yr−1) appears to be much lower and statistically non-significant (Table 4). This decrease in AOD recorded in Lille agrees with the analysis of Ningombam et al. (2019), who identified a generalized decreasing trend in AOD for several European AERONET stations since 1995. The yearly mean Ångström exponent appears to be relatively stable for both seasons (Figs. 5c and 6c), with overall mean values of 1.27 and 1.33 in spring and summer, respectively. Interestingly, in spring, the stable AE and decrease in AOD translates to a balancing of trends in the occurrences of the Continental (+1.9 % ± 0.4 % yr−1) and Continental polluted classes (−1.2 % ± 0.7 % yr−1), while in summer no trends in the occurrences of these two aerosol classes are statistically significant (Figs. 5d and 6d).
Figures 5e–h and 6e–h depict the annual variations, for the different sky conditions, of the incident solar irradiance (colored columns) at the surface in Lille and the partition between the direct flux (yellow component) and diffuse flux (blue component) over the period of 2010–2022 in spring and summer, respectively. Under all-sky conditions, significant fluctuations in both the global irradiance and its direct component are observed, while the contribution of the diffuse flux remains relatively stable for both seasons (Figs. 5e and 6e). In summer in particular, measurements show a difference of 115 W m−2 between the minimum in 2011 (307 W m−2) and the maximum in 2022 (421 W m−2). In spring, a similar gap of around 105 W m−2 is also identified between the recorded minimum in 2013 (285 W m−2) and the maximum in 2020 (389 W m−2) mentioned earlier. These significant variations, which appear to be strongly linked to the year-to-year variability in meteorological conditions, suggest an increasing trend in all-sky incident solar irradiance at the surface in Lille in both spring and summer. Seasonal Mann–Kendall trend tests support this hypothesis, as similar increasing trends are indeed observed for both seasons, with a magnitude of approximately +4 ± 2 over the period of 2010–2022 (Table 4). As the observed trends in diffuse irradiance are relatively uncertain, the overall increase in global irradiance appears to be primarily linked to a rise in the direct component, which also displays significant trends in both spring (+4.4 ± 2.3 ) and summer (+4.7 ± 1.9 ).
Our findings are consistent with the conclusions of other studies (Boers et al., 2017; Mateos et al., 2014; Sanchez-Lorenzo et al., 2013), which also observed increasing trends in surface solar irradiance for various European measurement sites, in both the presence and absence of clouds. It is also coherent with the general increase in SSI and sunshine duration observed over Europe since 1991 based on CM SAF SARAH-3 data (C3S, 2024). However, it should be noted that trends of increasing all-sky surface solar irradiance reported in the literature, which often cover longer periods (several decades) or earlier periods, are less pronounced than those highlighted in this study in Lille over the past 13 years. Apart from differences related to geographic position of the measurement sites and periods considered in each study, a possible explanation for these discrepancies is the time range used for our analysis. As mentioned previously, our analysis is based exclusively on daytime measurements, performed between sunrise+30 min and sunset−30 min. In contrast, studies from the literature usually consider irradiance measurements over a 24 h period, which include nighttime measurements that are characterized by an absence of incident solar radiation. The average over 24 h is thus lower than when considering only daytime measurements. To put our results into perspective, we applied seasonal Mann–Kendall trend tests to the year-to-year means over the period of 2010–2022 with the addition of nighttime measurements in our datasets. In this configuration, an increasing trend in all-sky GHI is statistically significant for all seasons (even in winter), with maximum magnitudes in summer (+2.8 ± 1.1 ) and spring (+2.3 ± 1.0 ). Thus, on an annual average, a trend of about +1.5 is observed, which is more in line with the trends reported in the literature.
In Lille, significant increasing trends of both global and direct irradiances are also observed under CSWC (+3.8 ± 1.2 for both components) and CSKY (+3.1 ± 1.3 for both components) situations in summer (Table 4). In spring, the trends are more uncertain, apart from a significant increase in BHI of about +3.7 ± 1.1 under CSWC conditions. Our results also highlight a significant decreasing trend in diffuse irradiance for CSKY situations of around −0.9 ± 0.3 .
The results of van Heerwaarden et al. (2021) regarding spring 2020 in Cabauw showed that sky conditions had a significant influence on the variability in the measured all-sky global irradiance. It is also very likely that the GHI maximum of spring 2020 in Lille, as well as the increasing trends in both spring and summer observed under all-sky conditions, are strongly associated with the decrease in the frequency of CLOS conditions in favor of the CSWC and CSKY situations, which exhibit higher radiation values, especially for the direct component of the flux. Under clear-sun-with-cloud and clear-sky conditions, the observed trends in irradiances could be partly related to the observed declining trends in AOD440, especially the decrease in clear-sky DHI in spring. They could also be related to the evolution of water vapor content, ozone, or the solar zenith angle under clear-sun conditions, which are influenced by the frequency of occurrence of the sky conditions. However, an analysis of the inter-annual variability in these various atmospheric parameters (not shown) reveals no significant trends over the period of 2010–2022 in spring or summer, apart from the negative trend in springtime AOD mentioned previously.
To isolate the contribution of the different parameters (occurrence of sky conditions, AOD, SZA, PWV, etc.) to the observed variabilities in the SSI under the different sky conditions, a more-detailed analysis was conducted using irradiance measurements from ATOLL and radiative-transfer simulations from SOLARTDECO based on the methodology of the multivariate analysis presented in Sect. 2.4.
3.2 Investigating main factors of SSI variability
This section presents the results of the multivariate analysis of the variability in surface solar irradiance over the period of 2010–2022, following the methodology introduced in Sect. 2.4. Our analysis focuses on the specific trends and annual extrema observed over the period of 2010–2022 in spring and summer, as these seasons are the most significant in terms of surface solar energy, exhibiting robust trends and substantial yearly variations in SSI over the 13-year period. First, results of the multivariate analysis of the all-sky irradiance record of spring 2020 are presented in Sect. 3.2.1. Then, the analysis of the observed irradiance temporal trends in spring and summer is presented in Sect. 3.2.2.
3.2.1 Multivariate analysis of spring 2020
As mentioned previously, the approach presented in Sect. 2.4.1 can be applied to the analysis of the year-to-year variability in the solar irradiance measured at the surface for all seasons, as well as to the study of the observed seasonal trends in irradiances presented in Sect. 3.1.2.
We analyze here the record-high mean GHI value from spring 2020 measured at the ATOLL platform. Spring 2020, in connection with the particular conditions linked to COVID-19 and the associated confinement period, has been the subject of numerous studies about anthropogenic pollution and air quality in France and around the world (Ordóñez et al., 2020; Reifenberg et al., 2022; Voigt et al., 2022; Cuesta et al., 2022; Petit et al., 2021; Fu et al., 2020; Velders et al., 2021), as well as about the possible impact of the reduction in anthropogenic emissions on incident solar radiation (Shuvalova et al., 2022; Reifenberg et al., 2022; van Heerwaarden et al., 2021). In particular, the study of van Heerwaarden et al. (2021) showed similarly high values of all-sky global irradiance in Cabauw (the Netherlands), a site about 250 km northeast of Lille that experienced the same large-scale high-pressure meteorological situation. The mean GHI for that spring was 389 W m−2, 55 W m−2 (i.e., 16 %) higher than the average springtime GHI observed in Lille over the 2010–2022 period.
Figure 7a and b illustrate the results obtained from our methodology for spring 2020 in Lille. Figure 7a presents the results of the decomposition of all-sky irradiance measurements in terms of sky conditions, using Eq. (3), for all irradiance components. Figure 7b presents the additional decomposition of the direct irradiance component under clear-sun-with-cloud (ΔFCSWC) and clear-sky (ΔFCSKY) conditions in terms of changes in aerosol class partition and changes in scene parameters, as in Eqs. (6) and (8).
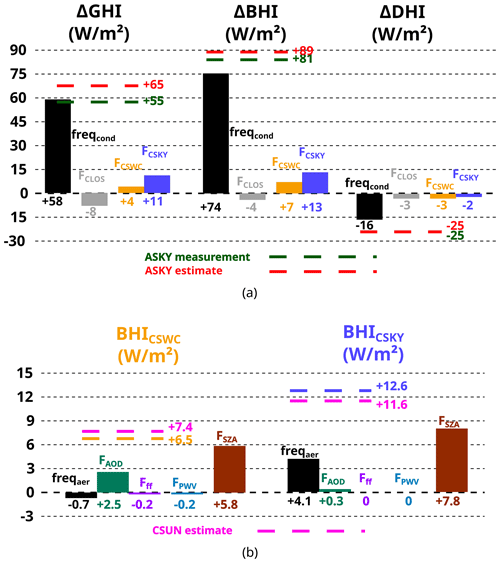
Figure 7Illustration of the decomposition for spring 2020: (a) the all-sky SSI components (GHI, BHI, and DHI) and (b) the direct component (BHI) in clear-sun-with-cloud and clear-sky conditions. In panel (a), the dashed green lines represent the observed deviation from the 2010–2022 spring average for spring 2020. The dashed red lines correspond to the deviation derived from the decomposition based on the sky conditions. The decomposition is represented by the black, gray, orange, and blue columns, which correspond to the contributions of the variability in the frequency of occurrence of sky conditions (freqcond) and the intrinsic variability in the irradiances in CLOS (FCLOS), CSWC (FCSWC), and CSKY (FCSKY) conditions, respectively. The additional decomposition of BHICSWC and BHICSKY with respect to the scene parameters is illustrated in panel (b). The orange and blue columns from panel (a), which represent the intrinsic variability in the BHI under CSWC and CSKY conditions, respectively, are represented as dashed lines of the same colors in panel (b). The pink dashed lines represent the values estimated from the decomposition of the BHI according to the scene parameters as in Eq. (6). The latter decomposition is also illustrated by the colored columns, which represent the contributions of the variability in the frequency of occurrence of aerosol classes (freqaer; black column), as well as the variability in the AOD (green column), ff (violet column), PWV (blue column), and SZA (brown column).
Overall, the decomposition of all-sky irradiances based on the different sky condition categories (cloudy-sun, clear-sun-with-cloud, and clear-sky) satisfactorily reproduces the measured differences in irradiances between the mean values of spring 2020 and the overall averages over the period of 2010–2022 for all components (Fig. 7a). Indeed, our approach estimates a variation of +65, +89, and −25 W m−2 for GHI, BHI, and DHI, respectively, versus the measured +55, +81, and −25 W m−2. As discussed in Sect. 3.1.2, spring 2020 in Lille was characterized by an exceptionally low frequency of cloudy-sun conditions (44 % compared to an average of 60 % in spring over 2010–2022; Fig. 5a). In contrast, clear-sky conditions were more than 2 times more frequent, representing 34 % of situations in spring 2020 compared to the spring average of 15 %. This change in sky conditions led, as shown in Fig. 7a, to an increase in BHI of +74 W m−2 (+83 % of the total increase in BHI) and a decrease in DHI of −16 W m−2 (−64 % of the total change in DHI). It did result in an increase in GHI of +58 W m−2, which represents 89 % of the total increase in GHI. Our approach also estimates that the remaining contribution (of only +7 W m−2, +11 % of the GHI change) comes mostly from changes in BHI resulting from effects that are partially opposite in sign, with fewer cloudy-sun situations being less bright at the surface ( W m−2), more clear-sky situations being brighter ( W m−2), and clear-sun-with-cloud being slightly brighter ( W m−2).
The results of the decomposition of the changes in BHI under clear-sun situations (CSUN, i.e., the sum of CSWC and CSKY) related to changes in aerosol classes and scene parameters, illustrated in Fig. 7b, provide more insight into the remaining +17 % increase in BHI that complements the effects of the change in sky situations. They suggest that most of the increase due to the change in scene parameters is due to a decrease in SZA for CSUN situations (−1.5 and −0.9° in the CSKY and CSWC situations, respectively) that by itself causes an increase of around +14.6 W m−2, which represents 71 % of the overall increase in BHI under clear-sun conditions. The remaining 29 % comes mostly from a change in aerosol properties. In CSWC situations, the increase in BHI due to SZA is 3 times higher than the increase due to a change in aerosol properties (linked with an average AOD of 0.16 in 2020 compared to the 2010–2022 average of 0.22). In the CSKY situation, the modification of aerosol class frequencies (a significant decrease in the occurrence of the Continental polluted class in favor of the Continental class; see Fig. 5d) is the main aerosol-related effect that results in a contribution of +4.1 W m−2, which is around slightly more than half of that due to changes in SZA (+7.8 W m−2).
Our estimates are consistent with the results of van Heerwaarden et al. (2021), who found that the observed irradiance record for spring 2020 in Cabauw is primarily due to the exceptionally low cloud fraction that year (a contribution of approximately 89 %), with modest additional contributions from changes in AOD (6 %) and water vapor content (5 %). Indeed, our analysis attributes an 89 % increase in all-sky GHI to the change in sky condition occurrences, which are also responsible for 83 % of the BHI increase and 64 % of the DHI increase. In addition, our results emphasize the predominant role of the SZA compared to aerosol changes for that season. We did not evaluate the CLOS situations that show a slight decrease in GHI, BHI, and DHI in the present study. However, we observe a slight increase in the SZA under CLOS situations, which might contribute to this general decrease in SSI in CLOS situations.
Our methodology was also used to explain the variability in irradiances for other years in spring and summer. Our analysis highlighted again the primary influence of the variability in the sky conditions, which also explains, for example, 69 % and 84 % of the variability in the all-sky GHI observed during the summer of 2022 (maximum of 421 W m−2) and spring 2013 (minimum of 285 W m−2), respectively. It also showed the primary importance of SZA associated with the variations in the occurrence of the sky conditions, which plays a key role in the variability in the irradiances for other years, while the influence of the variability in both aerosol and water vapor content is relatively limited. These results are consistent with the order of importance of the parameters determined by the sensitivity analysis in Sect. 2.4.2.
3.2.2 Multivariate analysis of spring and summer trends
As stated in Sect. 3.1.2 and Table 4, all-sky GHI irradiances show significant positive trends in spring and summer, with respective magnitudes of +4.0 ± 1.9 and +4.2 ± 1.9 due to an important increase in the direct component, with magnitudes of +4.4 ± 2.3 and +4.7 ± 1.9 in spring and summer, respectively. Figure 8a and b illustrate the results of these trend decompositions obtained from Eq. (4).
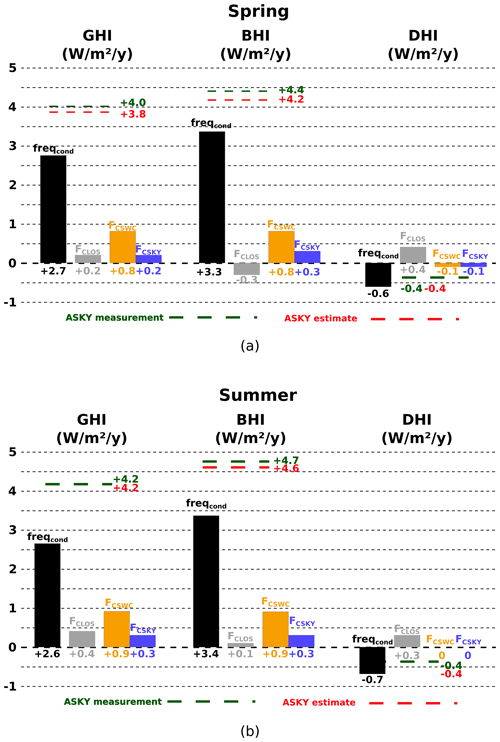
Figure 8Decomposition of the observed trends in all-sky SSI components (from left to right: global, direct, and diffuse) in (a) spring and (b) summer. The meanings of the colored lines and columns are similar to those described in Fig. 7a.
The decomposition of the trends shows similar features in spring and summer. The observed upward trends in all-sky GHI and BHI are largely linked to the trends in the occurrence of sky conditions, with contributions in spring of approximately +2.7 (71 %) and +3.3 (79 %) for the global and direct components, respectively, and +2.6 (62 %) and +3.4 (74 %) in summer. These results are consistent with the negative trends in the frequencies of CLOS situations observed almost equally for both seasons that lead to almost equal increases in the frequencies of CSWC and CSKY situations (Table 4). The remaining contributions in the GHI and BHI upward trends arise almost entirely from positive irradiance trends observed under the various sky conditions. The most important contribution comes from CSWC conditions (approx. +0.8–0.9 for both components), which align with the significant positive trends observed for these situations (Table 4) and their relatively high seasonal frequencies (25 % in spring, 22 % in summer). The statistical significance of these trends and the similarities between observed and derived all-sky GHI and BHI trends further validate these conclusions.
We further analyze the BHI variability in CSWC and CSKY conditions for spring and summer, decomposing their trends following Eqs. (7) and (9). Note that apart from the CSKY BHI trend in summer (where no parameter trends are significant), the computed trends generally yield results similar to the observed trends, with magnitudes within the uncertainty in the observations (±σ). The significant trends observed for CSWC BHI in spring (+3.7 ± 1.7 ) and summer (+3.8 ± 1.2 ), which contribute to the all-sky BHI trends by +0.85 and +0.91 , can be decomposed as in Fig. 9a and b. It shows that the main contribution to these trends in spring is related to changes in aerosol loading through variations in aerosol class frequencies (+0.21 W m−2) and increases in AOD (+0.35 W m−2) that explain 87 % of the CSWC BHI trend, while changes in SZA for those situations account for around 14 % of the CSWC BHI trend. These results align with the significant trends observed for both AOD and aerosol class frequencies in spring (Table 4). In summer, the contribution of aerosol properties is smaller, 49 %, while the contribution from changes in SZA reaches 44 %. Hence, although the trends in BHI under CSWC situations are very close in magnitude in spring and summer, the contribution of the different parameters is quite different for both seasons. It results from a decrease in AOD more than 2 times larger in spring compared to summer (see Table 4) and a decrease in SZA 3 times smaller in spring (−0.03 ± 0.09° yr−1; confidence level of 62 %) than in summer (−0.094 ± 0.09° yr−1; confidence level of 80 %). The contribution of the SZA variability is thus not to be neglected. Besides its effects on CSWC BHI, it also explains the limited CSKY BHI trend in spring, as the effects of the AOD decrease are compensated by an increase in SZA (+0.04 ± 0.14° yr−1; confidence level of 52 %). Furthermore, changes in SZA (−0.1 ± 0.09° yr−1; confidence level of 80 %) represent almost the sole contribution to the CSKY BHI trend in summer (Fig. 9).
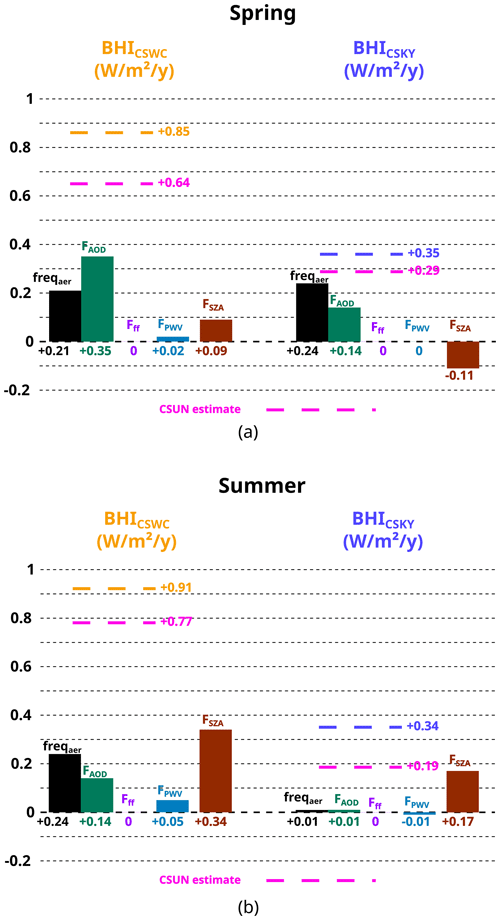
Figure 9Illustration of the decomposition of observed trends in the direct component of SSI (BHI) under clear-sun-with-cloud and clear-sky conditions in (a) spring and (b) summer. The meanings of the colored lines and columns are similar to those described in Fig. 7b.
Finally, it is worth noting that the influence of the other atmospheric parameters remains relatively limited, even for the water vapor content whose contribution is at a maximum for both seasons in clear-sun-with-cloud conditions but is limited to +3 % and +6 % in spring and summer, respectively.
In this section, we assess the radiative effects of atmospheric components, especially aerosols, on the downwelling surface solar irradiances (called REd, for radiative effect on the downwelling SSI) consistent with our ground-based dataset and relevent to surface-related applications (such as photovoltaic solar systems) and surface processes (e.g., photosynthesis). Note that only a few studies have assessed the direct radiative impact of aerosols specifically on downward surface solar radiation, as for example, was done in Papadimas et al. (2012) over the Mediterranean basin. Furthermore, our approach encompasses the shortwave radiative effects of atmospheric particles on the global, direct, and diffuse components of the downwelling SSI, as in Witthuhn et al. (2021). In Sect. 4.1 our analysis of the REd focuses on clear-sky conditions through a statistical investigation of the aerosol radiative effects on all downwelling SSI components (GHI, BHI, and DHI) over the whole period of 2010–2022. In addition, Sect. 4.2 provides an analysis of aerosol and cloud radiative effects in CSWC conditions. This approach relies on two sets of pristine (i.e., aerosol-and-cloud-free) and cloud-free simulations, which, by comparison with ground-based measurements that include the effect of clouds on SSI, allow the quantification of aerosol and cloud REd in CSWC conditions. Finally, the respective and cumulative REd of clouds (on DHI) and aerosols (on DHI and BHI) can be quantified over all CSUN (CSKY and CSWC) situations, which represent on average 33 % of sky conditions in Lille.
4.1 Clear-sky conditions
In clear-sky conditions, the radiative effect on the downwelling SSI (REd) of aerosols is defined as
or in relative terms as
where Fpristine and Fmeas correspond to the pristine (aerosol-and-cloud-free) simulations of SOLARTDECO and to the ATOLL measurements of the downwelling solar irradiance components (GHI, BHI, or DHI) in clear-sky conditions, respectively. The radiative effect efficiency of aerosols on the downwelling SSI (REEd) under clear-sky conditions, defined as
is also investigated in this section, as it allows the comparison of the REd for different aerosol loads (see for example Kok et al., 2017). Furthermore, as the REd is approximately linear with AOD (Satheesh and Ramanathan, 2000), REEd provides the sensitivity of REd to AOD.
The mean radiative effect of aerosols in Lille is investigated over the whole period of 2010–2022. Results are analyzed by season, by aerosol class, and by distinguishing between clean (AOD440≤0.1) and polluted (AOD440>0.1) situations. Table 5 provides the mean absolute and relative REd of aerosols along with the corresponding REEd for all coincident CSKY measurements and for the three components of the SSI.
Table 5Mean (absolute), [relative] aerosol REd, and associated <absolute efficiencies>, REEd, on SSI in clear-sky conditions in Lille over the period of 2010–2022 for coincident AERONET measurements only. The instantaneous values were computed using ATOLL irradiance measurements and SOLARTDECO pristine simulations for all irradiance components. The total mean corresponds to the overall mean REd and REEd for all coincident measurements in clear-sky conditions over the period of 2010–2022. Similar averages were made for each season as well as for the different classes and for clean (AOD440≤0.1) and polluted conditions.
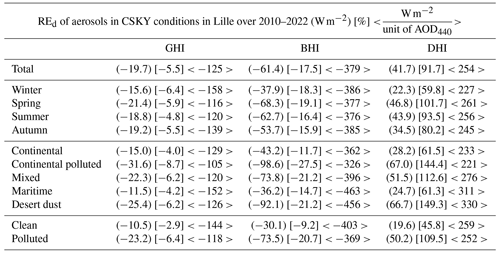
Our results show that in Lille over the period of 2010–2022, the overall yearly averaged radiative impact of aerosols constitutes a loss of around −61 W m−2 (−18 %) in direct irradiance and a gain of roughly +42 W m−2 (+92 %) in diffuse radiation, compared to pristine conditions. This leads to an important modification of the DHI contribution with a twofold (+105 %) increase in the average DHIGHI ratio, from 12 % in pristine conditions to an average of 24 % in CSKY conditions. The opposite-in-sign aerosol effects on BHI and DHI lead to a reduced effect of GHI, evidently always negative, which is on average reduced by about −20 W m−2 (−5.5 %). During polluted CSKY situations, which happen 73 % of the time, the aerosol REd exceeds −20 % and +100 % for BHI and DHI and −6 % for GHI. Inversely, for clean situations that mostly happen in fall and winter, the aerosol REd is weaker and smaller in magnitude than −10 % and +50 % for BHI and DHI and −3 % for GHI, i.e., 2 times smaller than in polluted situations. The compensation mechanism between the REd for the direct and diffuse irradiances shows, through the values of REEd, an aerosol-independent feature, with direct and diffuse components that are about 3 and 2 times more sensitive to the AOD than the GHI, respectively.
The analysis of the REd (GHI/BHI/DHI) indicates that the maximum radiative impact happens both in absolute (−21/−68/+47 W m−2) and relative (−6 %/−19 %/+102 %) terms in spring, with slightly higher values compared to summer (−19/−63/+44 W m−2; −5 %/−16 %/+94 %), and also for Continental polluted aerosols (−32/−99/+67 W m−2, −9 %/−28 %/144 %). This result is consistent with our analysis of the seasonal variability in the solar environment in Lille, which shows higher values of AOD in spring than in summer (Fig. 4b) and a high proportion of Continental polluted aerosols in spring. The minimum radiative impact occurs in absolute values in winter (−16/−38/+22 W m−2) and in Maritime aerosol conditions (−12/−36/+25 W m−2). This result is also consistent with the observed lower values of AOD in winter and higher proportion of Maritime aerosols during this season. It can also be related to higher solar-zenith angles that lead to overall lower surface irradiances in winter and thus lower aerosol REd in absolute values.
The REd efficiency (REEd) is higher in magnitude for Maritime and Desert dust aerosols, with values above −450 and +310 W m−2 for BHI and DHI, respectively. These results align with the conclusions of the sensitivity analysis (Sect. 2.4.2), which show higher sensitivities of the direct and diffuse components to Maritime and Desert dust aerosols. As already hypothesized, this result may be linked to the differences in the size of the particles, as the lower absolute value of the Ångström exponent for large particles implies higher extinction properties over the whole solar spectrum. Note that, on average, the radiative impact of the Maritime and Desert dust classes remains, however, relatively limited over the course of the year, as these two classes represent only 14 % and 5 % of observed situations, respectively. Moreover, although the Maritime class exhibits large REEd, the corresponding REd values remain relatively small due to low corresponding aerosol optical depths. Therefore, the radiative impact of aerosols in clear-sky conditions is largely dominated by the Continental, Continental polluted, and Mixed classes, which represent 38 %, 20 %, and 22 % of the situations in Lille, respectively.
Table 6Mean (absolute), [relative] aerosol REd, and <REEd> on SSI over the period of 2010–2022 in Lille under clear-sun-with-cloud conditions. Due to the presence of clouds in the sky, the instantaneous values are not based on ATOLL irradiance measurements but rather are computed using SOLARTDECO clear-sky simulations with aerosol properties issued from AERONET measurements as inputs.
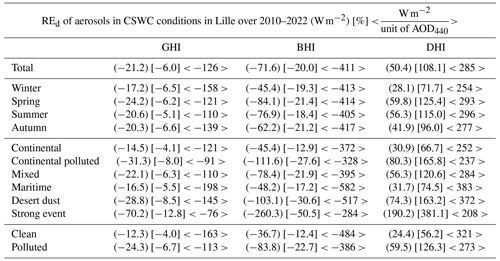
4.2 Clear-sun-with-cloud conditions
In clear-sun-with-cloud conditions, the overall particle REd on the direct SSI component is only related to aerosols. On the contrary, due to the presence of clouds out of the Sun's direction, their effects on the diffuse component are more complex, with contributions from both aerosols and clouds.
Their respective effects on the SSI diffuse component can generally not be separated. They are scene dependent and vary significantly with the cloud cover, notably the cloud fraction and the cloud opacities, even with the angular distance of the cloud cells to the Sun direction or the vertical profile of aerosols relative to clouds. Undoubtedly, aerosol REd on the diffuse SSI is minimal (maximal) when the cloud fraction is high (low). It is in any case out of the scope of the current study to find a complete distinction or disentangling of aerosol versus cloud REd in CSWC situations.
The radiative effects of all atmospheric particles, hydrometeors, and aerosols lead to an overall REd (REd, all) that can be decomposed as
Fmeas−Fcsky is by definition the cloud REd, i.e., the difference between the downwelling solar irradiance actually measured and due to the overall effects of all atmospheric components (gas, aerosol, and clouds) and the incoming radiation in the same conditions without clouds (i.e., clear-sky). In the solar direction, Fcsky−Fpristine equals the aerosol REd. However this is not the case for the diffuse SSI component. Fcsky−Fpristine is rather the radiation one would have compared to the pristine case if no clouds were present. Yet, in the rest of this section, Fcsky−Fpristine is named aerosol REd as in Sect. 4.1 and is written as Faer−Fpristine. Due to the presence of clouds, Faer is obtained from SOLARTDECO clear-sky simulations.
The motivation to analyze Faer−Fpristine is to appreciate the difference in aerosol REd between CSKY and CSWC situations and investigate the radiative compensation between cloud and aerosol REd in CSWC situations. Table 6 provides the mean absolute and relative values of Faer−Fpristine in CSWC situations over the period of 2010–2022. Values of REd are 15 % to 20 % higher than in CSKY, given in Table 5. This discrepancy is related to the slightly higher AOD440 values observed in CSWC conditions (0.19) compared to CSKY conditions (0.17), as well as to the slightly lower SZA values (60° on average in CSWC versus 62° in CSKY conditions), which lead to higher irradiance values and thus greater absolute radiative effects. Note that REEd are slightly greater than under clear-sky conditions, by about 30 W m−2 per unit of AOD for all irradiance components. This result is consistent with the effects of lower solar zenith angles and with the observation that aerosol Ångström exponents are on average slightly lower (by 0.06) in CSWC situations compared with the CSKY cases, leading to a higher REd efficiency.
Note that the study of CSWC situations allows us to analyze conditions characterized by the Strong event class (see Table 2), which were not identified as clear-sky because of either the presence of clouds or their high AOD values, as the clear-sky filter used in this study tends to erroneously misidentify CSKY conditions when the AOD is high (Liu et al., 2021). The aerosol REd for the Strong event class appears very substantial for both direct (−260 W m−2; i.e., −50 %) and diffuse (+190 W m−2; i.e., +381 %) components, leading to a relatively important reduction in the GHI of about −70 W m−2 (−13 %) on average. The climatological impact of the Strong event class is, however, relatively limited, as these situations only represent about 0.3 % of the situations observed in Lille over the period of 2010–2022.
As mentioned previously, the overall radiative effect of atmospheric components on CSWC conditions is obtained from the difference between measurements and pristine-like simulations and is the result of combined and compensating effects of both aerosols and clouds. Calculations of mean Faer−Fpristine, REd, cloud, CSWC, and REd, all CSWC over 2010–2022 according to the season and aerosol situation are synthesized in Fig. 10.
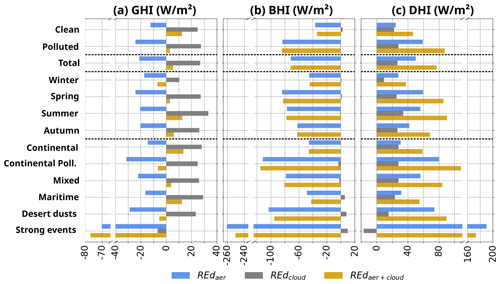
Figure 10Mean REd on SSI, under CSWC conditions, of aerosols and clouds combined (yellow), clouds (gray), and cloud-free REd of aerosols (blue) on SSI for the (a) global, (b) direct, and (c) diffuse components over the period of 2010–2022. Only points coincident with AERONET measurements are considered for the present computations. Instantaneous values are calculated using ATOLL SSI measurements and SOLARTDECO clear-sky simulations with and without aerosols. As in Table 5, the total averages correspond to the overall mean REd over the period of 2010–2022.
The radiative effects of all atmospheric particles (aerosols and clouds) are represented in yellow in Fig. 10 and those of clouds only are in grey. The REd of aerosols that would occur in cloud-free situations is in blue and corresponds to the values in Table 6.
As expected, REd, cloud, CSWC for the direct SSI component is close to zero (with an accuracy of ±1 W m−2), which is coherent with the absence of clouds in the Sun's direction. This result further validates the performance of both the CSWC filtering and our clear-sky radiative-transfer simulations (for most of the cases except for the Desert dust and Strong event situations, where small residuals exist).
In the case of clean situations (AOD<0.1), clouds contribute to an increase in DHI of around +22 W m−2 (gray column, Fig. 10c), comparable to the contribution of aerosols alone (+24 W m−2, blue column). In these conditions, the decrease in BHI due to aerosols (−37 W m−2, Fig. 10b) is overcompensated by the cumulative impact of both aerosols and clouds on the DHI, leading to GHI values greater than under pristine-like conditions (without aerosols and clouds) by about +13 W m−2 (yellow column, Fig. 10a). For polluted situations characterized by higher aerosol loads (AOD≥0.1), which are more frequent, the cumulative effect of clouds and aerosols results in a GHI greater than that in the pristine situations by +3 W m−2. The cloud contribution to DHI, +28 W m−2, is slightly higher than for clean situations, while the aerosol cloud-free contribution to BHI and DHI is significantly higher. If one considers all CSWC situations, clouds add, on average, 25 W m−2 compared to cloud-free atmospheres, i.e., half of the aerosol cloud-free REd of the diffuse component. Cloud REd is even higher than 50 W m−2 20 % of the time. As a consequence, the mean observed GHI in CSWC situations is close to pristine conditions or even slightly above, by +4 W m−2 (Fig. 10a). The corresponding proportions of direct and diffuse irradiances are, however, in this case very different, with an average decrease of about −20 % in BHI and an increase of +164 % in DHI compared to pristine-like conditions. The DHI proportion in the presence of clouds and aerosols under CSWC conditions (25 %) is thus on average much higher than under pristine conditions (10 %, i.e., cloud-free and aerosol-free situations).
The seasonal difference reveals that the global REd of particles is maximal in absolute (relative) values in summer (autumn) with +12.7 W m−2 (+0.9 %) above the pristine level. In spring, the global REd is lower due to higher aerosol REd but remains positive (+3 W m−2). In contrast, despite a weaker radiative impact of aerosols in winter, the corresponding effect of clouds is so small (+10 W m−2, i.e., 3 times less than the effect of aerosols; Fig. 10c) that the reduction in the BHI by aerosols is not fully compensated for, with average GHI values lower than under pristine-like conditions by about −7 W m−2 (Fig. 10a). Similar results are observed for the Continental polluted, Desert dust, and Strong event classes, with a decrease in GHI of about −6, −5, and −77 W m−2, respectively, compared to pristine-like conditions. In contrast, for the Maritime and Continental conditions characterized by lower aerosol loads, the combined REd of aerosols and clouds remains positive.
In summary, these results highlight the importance of clouds that cause a significant enhancement effect on the diffuse irradiance field in CSWC situations. For these situations, which occur 22 % of the time, the mean GHI level is comparable to pristine-like conditions (the difference is +4 W m−2). The SSI is even higher than in pristine conditions during clean, summer, or Continental aerosol moments, with an average increase in solar radiation of up to 13 W m−2. Clouds add on average between 20 and 30 W m−2, depending on the situation, and overall on average around 25 W m−2 compared to atmospheres that would be cloud-free. Hence, they not only substantially increase the level of GHI but also modify the direct/diffuse partition of the SSI, increasing the diffuse proportion by +4 % (21 % to 25 %) compared to the cloud-free atmosphere. Overall, the combined effects of aerosols and clouds lead to an increase in the DHI proportion of +15 % compared to the pristine atmosphere (from 10 % to 25 %).
Finally, mean radiative effects can also be computed considering all clear-sun situations, i.e., the sum of the CSKY and CSWC situations, which represent on average 33 % of the situations per year over 2010–2022. These REd are written as the sum of the REd per sky condition weighted by their respective occurrences, keeping in mind that the REd of aerosols in CSWC conditions is equal to Faer−Fpristine and is, as has already been said, the REd aerosols would have in the absence of clouds. Overall, the yearly clear-sun aerosol REd for measurements coincident with AERONET retrievals, is around −20 W m−2 (−66.5 and +46.6 of the direct and diffuse components, respectively), and the cloud REd is around +10 W m−2. As clouds act only on the diffuse component, the resulting mean measured CSUN GHI is about 10 W m−2 lower than under pristine conditions (no aerosols or clouds) but still 10 W m−2 above the cloud-free level.
We perform in this study an analysis of the variabilities in global horizontal irradiance (GHI) and its direct (BHI) and diffuse (DHI) components measured routinely at a 1 min resolution over the period of 2010–2022, as well as coincident aerosol optical properties measured by AERONET using ground-based measurements from the ATOLL platform (Villeneuve d'Ascq, France) in a region marked by extensive variabilities in sky conditions and aerosol loadings.
As the amount and characteristics of the SSI are quite different depending on sky situations, as well as its sensitivity to atmospheric parameters, we distinguish sky conditions by applying adapted filters based on irradiance components that lead to three categories: clear-sky (CSKY), clear-sun-with-cloud (CSWC), and cloudy-sun (CLOS) situations. Over 2010–2022, these sky condition occurrences represent 11 %, 22 %, and 67 % of the situations. The performance of this automatic filtering was validated with a visual analysis of around 12 000 sky imager observations. The risk of misinterpretation is 5 % and 12 % for the clear-sky and clear-sun (sum of CSKY and CSWC) situations, respectively, and higher for strong aerosol load situations, which are rare in our case. Climatologies of SSI and its components were obtained for these three situations between sunrise plus 30 min and sunset minus 30 min in order to minimize bias. CSWC situations, generally marked by irradiance enhancement effects due to clouds (Gueymard, 2017; Mol et al., 2023), are particularly interesting with, on a monthly average, 13 % more GHI than in CSKY situations (between +4 % to +21 %) and with diffuse proportions in CSWC situations on average 10 % above the mean proportion in CSKY situations (31 % versus 21 %).
A second classification of the sky condition concerns the atmospheric column loading of aerosols. A six class categorization of aerosols, inspired by the work of Toledano et al. (2007) and based on photometric measurements of the aerosol optical depth (AOD), serves a climatological purpose and allows the definition of new models of aerosol optical properties based on AERONET almucantar inversions. With this modeling, we perform radiative-transfer simulations under cloud-free and aerosol-and-cloud-free (pristine) assumptions that allow the computation of the radiative impact on the downwelling SSI (REd) of aerosols and clouds in Lille over 2010–2022, as well as allowing a sensitivity analysis of the solar irradiance to various input parameters, such as the aerosol optical depth and Ångström exponent, PWV (precipitable water vapor content), or even SZA (solar zenith angle). The computed sensitivities are used in conjunction with the above classifications to refine an analysis of the variabilities in irradiance components observed in clear-sun conditions.
We focus on the spring and summer seasons and analyze the coincident year-to-year variabilities in solar irradiances and sky conditions over the period of 2010–2022. The application of Mann–Kendall trend tests reveals (significance threshold of 5 %) statistically significant trends for those seasons for the occurrences of both clear and cloudy conditions, AOD, and the global and direct components of the solar irradiance. Indeed, the average proportion of CLOS moments shows a decreasing trend of around −1 % yr−1 for both seasons, in favor of a +0.5 % increased frequency of both CSWC and CSKY conditions. In addition, photometric measurements show a decrease in AOD over the period of 2010–2022 for both seasons, particularly in spring, with an average trend of −0.008 yr−1. Finally, measurements of the incident solar radiation indicate significant upward trends in all-sky GHI of around +4 ± 2 for both seasons, which are mainly driven by a rise in BHI of around +4.4 ± 2.3 and +4.7 ± 1.9 , respectively, in spring and summer. Additionally, in summer, significant positive trends are also observed for both GHI and BHI for clear-sky situations (+3.1 ), as well as under clear-sun-with-cloud situations (+3.7 and +3.8 , respectively for GHI and BHI). Note that although the statistical robustness of our trends may be affected by the performance of our classification of sky conditions and by the length of the study period (13 years), they appear consistent, especially under all-sky conditions, with longer-term results in Europe (C3S, 2024; Ningombam et al., 2019; Boers et al., 2017).
We conducted a multivariate analysis of the irradiance variabilities in order to quantify the different contributions to the variabilities. It was first employed to analyze the particular case of spring 2020, which exhibited record values for both clear-sky and clear-sun frequency (34 % and 57 % compared to the spring average of 15 % and 37 %) and radiation (389 W m−2 compared to the average of 334 W m−2). The analysis indicates that nearly 89 % and 83 % of the record increases in GHI and BHI, respectively, are attributable to exceptional sunlight conditions. This conclusion aligns with the results of van Heerwaarden et al. (2021) that also identified the peak in spring 2020 irradiance in Cabauw (the Netherlands) as being primarily driven (also an 89 % contribution) by a lower cloud fraction. In addition, our analysis reveals that most of the increase, 71 %, in BHI observed in spring 2020 in clear-sun conditions is due to a decrease in the SZA (−1.5 and −0.9° in CSKY and CSWC situations, respectively) rather than the observed change in aerosol properties (a decrease by 0.06 of AOD and a decrease in the Continental polluted class in the CSWC and CSKY situations, respectively). These findings are consistent with the results of the sensitivity analysis, which show that in the absence of clouds (clear-sky conditions), the SZA has the most impact on the global irradiance, followed by the AOD and PWV. The multivariate analysis of the temporal trends in global and direct irradiances reveals that the variability in sky conditions significantly influences the observed all-sky increases in spring and summer, with seasonal contributions for GHI of 71 % and 62 % and of 79 % and 74 % for BHI, respectively. Our analysis also indicates a significant contribution in all-sky seasonal BHI (around 20 %) from the variability in the BHI in CSWC conditions for both seasons. The sensitivity analysis of BHI to atmospheric parameters indicates that while the increase in spring is mainly linked to the variability in the aerosol content (+88 %) and most notably to a decrease in AOD (−0.011 yr−1), it is related in summer to comparable contributions from the solar zenith angle (44 %) and aerosol content (49 %) variabilities.
With radiative-transfer simulations of aerosol-and-cloud-free (pristine) conditions and of cloud-free conditions, the study of the radiative effects (REd) of aerosol and clouds is possible in clear-sun situations. Quantification of the aerosol REd in CSKY situations shows for our site an average effect on GHI of −20 W m−2 (−6 %), which results from the expected compensation between a negative effect on the direct (a decrease 3 times larger: −61 W m−2; −18 %) component and a positive forcing on the diffuse (an increase 2 times larger: +42 W m−2; +92 %) irradiance. The influence of aerosols on the solar environment is significant in CSKY situations, as the proportion of diffuse irradiance is on average 2 times larger (24 %) in the presence of aerosols than under pristine-like conditions (12 %). The statistical results show that the radiative impact of aerosols on irradiances is mainly driven by the AOD, so lower aerosol REd are found in winter and during clean situations, and conversely higher aerosol REd values are found in spring and for Continental polluted situations. This translates into REd efficiencies (REEd) that are more case independent than the REd is. In addition, results show that the REEd is larger for coarser particles, particularly on the diffuse irradiance.
The identification of CSWC situations, which represent 22 % of the cases, offers the opportunity to quantify important compensation mechanisms between the effects of aerosols (unambiguously on BHI) that tend to reduce the SSI and the effects of clouds (unambiguously on DHI) that tend to increase it. Our analysis reveals that clouds increase the diffuse and thus the global irradiances by about +27 W m−2 (6 %) on average. This cloud enhancement effect compensates or even overcompensates for the overall radiative impact of aerosols, especially in clean, summer, or Continental situations, resulting in GHI values comparable to or even higher than in pristine conditions (+13 and +4 W m−2 in clean and polluted situations), with a much-higher diffuse proportion (25 % versus 21 % in cloud-free situations; 10 % with the pristine hypothesis).
We present in this work a methodology and its potential for analyzing the contribution of the variability in cloudy and clear-sky conditions to changes in incident surface solar irradiances. A multivariate analysis reveals that the overall variability in the surface solar irradiance is influenced by a combination of factors whose impacts can sometimes accumulate (e.g., an increase in clear-sun occurrence, reduction in aerosol loading, and decrease in SZA) or compensate for one another and that simple geometrical effects are not to be neglected. Further investigations would concern clear-sun-with-cloud and cloudy-sun conditions, which are prevalent in Lille, in order to analyze the variability in the surface solar irradiance as a function of cloud cover parameters, including the fraction of the sky covered by clouds and the cloud type.
A1 Clear-sun detection
The method of Batlles et al. (2000) is a fairly straightforward method based on two criteria: (i) the clearness index (), which represents the transmittance of the atmosphere relative to the incoming extraterrestrial solar irradiance (I0), should be larger than a threshold value, Klim, while (ii) the fraction of diffuse radiation () should be smaller than a maximum value, Df,lim. Note that both thresholds vary with the Sun elevation angle as defined in Eqs. (A1) and (A2):
A2 Clear-sky detection
The method of García et al. (2014) is based on the algorithm originally presented in Long and Ackerman (2000), which relies on a series of four tests based on GHI and DHI measurements of high frequency (3 min or less). The first two tests remove obvious cloudy moments characterized by extreme values of the normalized GHI (test 1) and measured diffuse irradiance (test 2) through the definition of threshold values of both quantities. The third and fourth tests are more elaborate, allowing the more-subtle detection of cloud cover through the analysis of the temporal variability in the global irradiance and the normalized diffuse irradiance ratio, respectively. The normalization of the GHI and DHI in the first and fourth tests is based on a power law of the cosine of the solar zenith angle as in Eq. (A3):
where F corresponds to the measured global irradiance (GHI) or diffuse irradiance ratio (Df) and the coefficient bF represents the variations in the associated clear-sky quantities with the cosine of the solar zenith angle μ0. The subscript N represents the normalized quantities.
The four tests are applied in an iterative process to provide a new set of clear-sky moments each time, which are then used to calculate coefficients for the first and fourth tests for days with enough clear-sky observations, by fitting the GHI and Df 1 min measurements with respect to μ0, as in Eqs. (A4) and (A5):
where GHI and Df are the observed global irradiance and diffuse ratio and the parameter a represents the associated clear-sky quantities for a solar zenith angle of 0°. The coefficient b corresponds to the parameters used for the normalization of the GHI and Df, as in Eq. (A3). A new set of parameters is then derived as the mean of the daily values, and the iteration process continues until convergence is achieved within a tolerance of 5 %. This method is thus quite versatile and can theoretically be applied to any observational site equipped with measurements of both global and diffuse irradiances.
In their work, García et al. (2014) showed that for the particular conditions of the Izaña Observatory, a high-elevation arid site located in the Canary Islands, the parameters a and b from Eqs. (A4) and (A5) could be expressed as functions of the daily AOD at 500 nm. This possibly reduces the method's versatility, as it requires collocated aerosol optical properties. However, in this study, thanks to the availability of coincident photometric measurements, this method was selected as it gives more satisfactory results than the original algorithm of Long and Ackerman (2000), as shown in Sect. 2.2.1.
In the present study, as described in Elias et al. (2024), the variability in the parameters aGHI, bDHI, and bGHI with the AOD at 500 nm was adapted in order to better represent the conditions observed in Lille, as they are quite different from those observed at the Izaña Observatory.
For the present study, new models of aerosol optical properties were defined using the available AERONET inversions of the aerosol volume size distribution and complex refractive index in Lille over the period of 2010–2020. As mentioned in Sect. 2.3.1, the goal is to create a lookup table of pre-computed aerosol optical properties (extinction coefficients, single-scattering albedo, components of the phase function), which can be used as inputs by SOLARTDECO. Overall, the lookup table includes around 120 models of aerosol optical properties, which are divided between a fine and a coarse mode, the 6 aerosol classes from Sect. 2.2.2, and 10 bins of surface relative humidity. The properties of each model are obtained through Mie calculations based on average aerosol normalized number size distributions of each mode and on refractive indices derived from AERONET inversions in Lille over the period of 2010–2020. This methodology allows us to limit the use of Mie calculations and to compute specific aerosol optical properties at the resolution of AERONET direct-sun measurements by mixing pre-computed aerosol optical properties of the fine and coarse modes as follows.
-
First, the appropriate pair of modes is selected from the lookup table using the measured values of AOD440, AE440−870, and surface relative humidity.
-
Then, a system of two equations with two unknowns based on the measured AOD440 and AE440−870 values is solved to compute two coefficients, namely the fine- (ff) and coarse-mode (cf) fractions (Eqs. B1 and B2):
with AOD440,fine, AOD440,coarse, , and as the AOD values at 440 nm of the fine and coarse modes and AE between 440 and 870 nm. To determine the latter quantities, we assume that the observed aerosol layer corresponds to a mixture of two distinct observations, one characterized exclusively by fine-mode aerosols and the other one only by coarse-mode aerosols. Following this hypothesis, the measured aerosol optical depth at 550 nm (AOD550,meas) can be considered in each case to be the AOD at 550 nm of the corresponding mode. This allows us to determine the AOD of each mode (either fine or coarse) at 440 nm from the measurement at 550 nm, using the pre-computed extinction coefficients (Cextλ,mode) simulated by the Mie code at the wavelength λ (here, 440 and 550 nm), as in the following equation:
Note that the Ångström coefficients of each mode between 440 and 870 nm are computed directly from the associated extinction coefficients, using Eq. (B4):
-
The fractions ff and cf derived from the above system are then used, in conjunction with mean number concentrations NCfine and NCcoarse defined for each mode based on AERONET inversions, to estimate new mixing ratios specific to the simulation, Rfine and Rcoarse, through Eqs. (B5) and (B6):
-
Finally, these mixing ratios are used to compute, without additional Mie calculations, new mixed aerosol optical properties specific to the simulation by a combination of the properties of each mode at each wavelength, using Eqs. (B7) to (B10):
where is the scattering coefficient, SSAλ,mode the single-scattering albedo, and P represents the components of the phase function (P11, P21, P34 and P44). The subscript mix stands for the new optical properties computed from the combination of the fine and coarse modes.
Python code to analyze data and generate figures is available from the first author upon request. The AERONET datasets were downloaded from the NASA AERONET website, https://aeronet.gsfc.nasa.gov/ (NASA, 2024). The ARTDECO original radiative-transfer code was obtained from the ICARE Data Center in Lille, France (https://www.icare.univ-lille.fr/artdeco/, AERIS/ICARE, 2021). The dataset used in this study came from ground measurements from the ATOLL (Atmospheric Observations in LiLLE, https://www.loa.univ-lille.fr/observations/plateformes.html?p=lille, Université de Lille, 2015) platform in Villeneuve-d'Ascq, north of France, over the period of 2010–2022. It contains irradiance measurements, aerosol and gas column properties, and obtained clear-sky and clear-sun flags and is freely available from an EaSy Data repository at https://doi.org/10.57932/ca9c74c0-83f4-43f1-86c8-7f3ce517b03c (Chesnoiu et al., 2024).
The supplement related to this article is available online at: https://doi.org/10.5194/acp-24-12375-2024-supplement.
GC: formal analysis, methodology, data handling, and writing (original draft preparation). NF and IC: conceptualization, funding acquisition, supervision, methodology, and writing (review and editing). FA, DC, and IJ: help operating and maintaining the radiative sensors and the sky imager. MC and TE: collaboration on the design of SOLARTDECO, the solar version of the radiative-transfer code ARTDECO. All authors have contributed to the final paper.
The contact author has declared that none of the authors has any competing interests.
Publisher's note: Copernicus Publications remains neutral with regard to jurisdictional claims made in the text, published maps, institutional affiliations, or any other geographical representation in this paper. While Copernicus Publications makes every effort to include appropriate place names, the final responsibility lies with the authors.
The authors would like to thank Gérard Brogniez, who initiated the observational dataset of global, direct, and diffuse solar irradiances at a 1 min resolution in 2008 at LOA, and Colette Brogniez, who initiated the automatic acquisition of full hemispheric pictures of the sky with a sky imager in 2009. The authors would also like to thank Philippe Goloub, the PI of the AERONET site at Lille, and the personnel of the PHOTONS observation station for their efforts establishing and maintaining the AERONET Lille site. We also appreciate the comments of the two anonymous reviewers that have helped us to improve the paper.
This study comes from the work of Gabriel Chesnoiu during his PhD thesis, co-financed by the ADEME and Région Hauts-de-France. This research project has been granted under the LEFE (Les Enveloppes Fluides et l'Environnement)/IMAGO (Interactions Multiples dans l'Atmosphère, la Glace, et l'Océan) CNRS-INSU program over the period of 2021–2023.
This paper was edited by Stelios Kazadzis and reviewed by two anonymous referees.
AERIS/ICARE: Artdeco, (Atmospheric Radiative Transfer Database for Earth Climate Observation), https://www.icare.univ-lille.fr/artdeco/ (last access: 24 October 2024), 2021. a
Anderson, G., Clough, S., Kneizys, F., Chetwynd, J., and Shettle, E.: AFGL Atmospheric Constituent Profiles (0.120 km), Tech. rep., Air Force Geophys. Lab., Hanscom Air Force Base, Bedford, Mass., https://apps.dtic.mil/sti/citations/ADA175173 (last access: 24 October 2024), 1986. a
Batlles, F. J., Olmo, F. J., Tovar, J., and Alados-Arboledas, L.: Comparison of Cloudless Sky Parameterizations of Solar Irradianceat Various Spanish Midlatitude Locations, Theor. Appl. Climatol., 66, 81–93, https://doi.org/10.1007/s007040070034, 2000. a, b, c, d
Beck, H. E., Zimmermann, N. E., McVicar, T. R., Vergopolan, N., Berg, A., and Wood, E. F.: Present and future Köppen-Geiger climate classification maps at 1 km resolution, Scientific Data, 5, 180214, https://doi.org/10.1038/sdata.2018.214, 2018. a
Blanc, P., Espinar, B., Geuder, N., Gueymard, C., Meyer, R., Pitz-Paal, R., Reinhardt, B., Renné, D., Sengupta, M., Wald, L., and Wilbert, S.: Direct normal irradiance related definitions and applications: The circumsolar issue, Sol. Energy, 110, 561–577, https://doi.org/10.1016/j.solener.2014.10.001, 2014. a
Boers, R., Brandsma, T., and Siebesma, A. P.: Impact of aerosols and clouds on decadal trends in all-sky solar radiation over the Netherlands (1966–2015), Atmos. Chem. Phys., 17, 8081–8100, https://doi.org/10.5194/acp-17-8081-2017, 2017. a, b, c
Boers, R., Bosveld, F., Baltink, H. K., Knap, W., van Meijgaard, E., and Wauben, W.: Observing and Modelling the Surface Radiative Budget and Cloud Radiative Forcing at the Cabauw Experimental Site for Atmospheric Research (CESAR), the Netherlands, 2009–17, J. Climate, 32, 7209–7225, https://doi.org/10.1175/JCLI-D-18-0828.1, 2019. a
C3S: European State of the Climate 2023, Full report, https://climate.copernicus.eu/esotc/2023 (last access: 24 October 2024), 2024. a, b, c
Chesnoiu, G., Ferlay, N., and Chiapello, I.: Ground measurements of surface solar irradiance, aerosol optical properties and sky conditions estimates in Lille (North of France) over the period 2010–2022, EaSy Data [data set], https://doi.org/10.57932/ca9c74c0-83f4-43f1-86c8-7f3ce517b03c, 2024. a
Cuesta, J., Costantino, L., Beekmann, M., Siour, G., Menut, L., Bessagnet, B., Landi, T. C., Dufour, G., and Eremenko, M.: Ozone pollution during the COVID-19 lockdown in the spring of 2020 over Europe, analysed from satellite observations, in situ measurements, and models, Atmos. Chem. Phys., 22, 4471–4489, https://doi.org/10.5194/acp-22-4471-2022, 2022. a
Derimian, Y., Léon, J.-F., Dubovik, O., Chiapello, I., Tanré, D., Sinyuk, A., Auriol, F., Podvin, T., Brogniez, G., and Holben, B. N.: Radiative properties of aerosol mixture observed during the dry season 2006 over M'Bour, Senegal (African Monsoon Multidisciplinary Analysis campaign), J. Geophys. Res., 113, D00C09, https://doi.org/10.1029/2008jd009904, 2008. a
Derimian, Y., Dubovik, O., Tanre, D., Goloub, P., Lapyonok, T., and Mortier, A.: Optical properties and radiative forcing of the Eyjafjallajökull volcanic ash layer observed over Lille, France, in 2010, J. Geophys. Res.-Atmos., 117, D00U25, https://doi.org/10.1029/2011JD016815, 2012. a
Drugé, T., Nabat, P., Mallet, M., and Somot, S.: Future evolution of aerosols and implications for climate change in the Euro-Mediterranean region using the CNRM-ALADIN63 regional climate model, Atmos. Chem. Phys., 21, 7639–7669, https://doi.org/10.5194/acp-21-7639-2021, 2021. a
Dubovik, O. and King, M. D.: A flexible inversion algorithm for retrieval of aerosol optical properties from Sun and sky radiance measurements, J. Geophys. Res.-Atmos., 105, 20673–20696, https://doi.org/10.1029/2000JD900282, 2000. a, b
Dubovik, O., Smirnov, A., Holben, B. N., King, M. D., Kaufman, Y. J., Eck, T. F., and Slutsker, I.: Accuracy assessments of aerosol optical properties retrieved from Aerosol Robotic Network (AERONET) Sun and sky radiance measurements, J. Geophys. Res.-Atmos., 105, 9791–9806, https://doi.org/10.1029/2000JD900040, 2000. a
Dubovik, O., Holben, B., Eck, T. F., Smirnov, A., Kaufman, Y. J., King, M. D., Tanré, D., and Slutsker, I.: Variability of Absorption and Optical Properties of Key Aerosol Types Observed in Worldwide Locations, J. Atmos. Sci., 59, 590–608, https://doi.org/10.1175/1520-0469(2002)059<0590:VOAAOP>2.0.CO;2, 2002. a, b
Dubuisson, P., Labonnote, L., Riedi, J., Compiègne, M., and Winiarek, V.: ARTDECO: Atmospheric Radiative Transfer Database for Earth and Climate Observation, in: International Radiation Symposium (IRS), https://www.icare.univ-lille.fr/artdeco/ (last access: 24 October 2024), 2016. a
Elias, T., Ferlay, N., Chesnoiu, G., Chiapello, I., and Moulana, M.: Regional validation of the solar irradiance tool SolaRes in clear-sky conditions, with a focus on the aerosol module, Atmos. Meas. Tech., 17, 4041–4063, https://doi.org/10.5194/amt-17-4041-2024, 2024. a, b
Favez, O., Weber, S., Petit, J.-E., Alleman, L. Y., Albinet, A., Riffault, V., Chazeau, B., Amodeo, T., Salameh, D., Zhang, Y., Srivastava, D., Samaké, A., Aujay-Plouzeau, R., Papin, A., Bonnaire, N., Boullanger, C., Chatain, M., Chevrier, F., Detournay, A., Dominik-Sègue, M., Falhun, R., Garbin, C., Ghersi, V., Grignion, G., Levigoureux, G., Pontet, S., Rangognio, J., Zhang, S., Besombes, J.-L., Conil, S., Uzu, G., Savarino, J., Marchand, N., Gros, V., Marchand, C., Jaffrezo, J.-L., and Leoz-Garziandia, E.: Overview of the French Operational Network for In Situ Observation of PM Chemical Composition and Sources in Urban Environments (CARA Program), Atmosphere-Basel, 12, 207, https://doi.org/10.3390/atmos12020207, 2021. a
Fu, F., Purvis-Roberts, K. L., and Williams, B.: Impact of the COVID-19 Pandemic Lockdown on Air Pollution in 20 Major Cities around the World, Atmosphere-Basel, 11, 1189, https://doi.org/10.3390/atmos11111189, 2020. a
García, R. D., García, O. E., Cuevas, E., Cachorro, V. E., Romero-Campos, P. M., Ramos, R., and de Frutos, A. M.: Solar radiation measurements compared to simulations at the BSRN Izaña station. Mineral dust radiative forcing and efficiency study, J. Geophys. Res.-Atmos., 119, 179–194, https://doi.org/10.1002/2013JD020301, 2014. a, b, c, d, e, f
Giles, D. M., Sinyuk, A., Sorokin, M. G., Schafer, J. S., Smirnov, A., Slutsker, I., Eck, T. F., Holben, B. N., Lewis, J. R., Campbell, J. R., Welton, E. J., Korkin, S. V., and Lyapustin, A. I.: Advancements in the Aerosol Robotic Network (AERONET) Version 3 database – automated near-real-time quality control algorithm with improved cloud screening for Sun photometer aerosol optical depth (AOD) measurements, Atmos. Meas. Tech., 12, 169–209, https://doi.org/10.5194/amt-12-169-2019, 2019. a
Gueymard, C.: Parametrized transmittance model for direct beam and circumsolar spectral irradiance, Sol. Energy, 71, 325–346, https://doi.org/10.1016/S0038-092X(01)00054-8, 2001. a
Gueymard, C.: Spectral Circumsolar Radiation Contribution To CPV, AIP Conf. Proc., 1277, 316–319, https://doi.org/10.1063/1.3509220, 2010. a
Gueymard, C. A.: Cloud and albedo enhancement impacts on solar irradiance using high-frequency measurements from thermopile and photodiode radiometers. Part 1: Impacts on global horizontal irradiance., Sol. Energy, 153, 755–765, https://doi.org/10.1016/j.solener.2017.05.004, 2017. a
Gueymard, C. A., Bright, J. M., Lingfors, D., Habte, A., and Sengupta, M.: A posteriori clear-sky identification methods in solar irradiance time series: Review and preliminary validation using sky imagers, Renew. Sust. Energ. Rev., 109, 412–427, https://doi.org/10.1016/j.rser.2019.04.027, 2019. a
Gutiérrez, C., Somot, S., Nabat, P., Mallet, M., Corre, L., van Meijgaard, E., Perpiñán, O., and Gaertner, M. Á.: Future evolution of surface solar radiation and photovoltaic potential in Europe: investigating the role of aerosols, Environ. Res. Lett., 15, 034035, https://doi.org/10.1088/1748-9326/ab6666, 2020. a
Hahn, C. and Warren, S.: A Gridded Climatology of Clouds over Land (1971–1996) and Ocean (1954–2008) from Surface Observations Worldwide (NDP-026E)*, https://doi.org/10.3334/CDIAC/CLI.NDP026E, 2007. a
Hauglustaine, D. A., Balkanski, Y., and Schulz, M.: A global model simulation of present and future nitrate aerosols and their direct radiative forcing of climate, Atmos. Chem. Phys., 14, 11031–11063, https://doi.org/10.5194/acp-14-11031-2014, 2014. a, b
Holben, B. N., Tanré, D., Smirnov, A., Eck, T. F., Slutsker, I., Abuhassan, N., Newcomb, W. W., Schafer, J. S., Chatenet, B., Lavenu, F., Kaufman, Y. J., Castle, J. V., Setzer, A., Markham, B., Clark, D., Frouin, R., Halthore, R., Karneli, A., O'Neill, N. T., Pietras, C., Pinker, R. T., Voss, K., and Zibordi, G.: An emerging ground-based aerosol climatology: Aerosol optical depth from AERONET, J. Geophys. Res.-Atmos., 106, 12067–12097, https://doi.org/10.1029/2001JD900014, 2001. a
Hou, X., Wild, M., Folini, D., Kazadzis, S., and Wohland, J.: Climate change impacts on solar power generation and its spatial variability in Europe based on CMIP6, Earth Syst. Dynam., 12, 1099–1113, https://doi.org/10.5194/esd-12-1099-2021, 2021. a
Jerez, S., Tobin, I., Vautard, R., Montávez, J. P., López-Romero, J. M., Thais, F., Bartok, B., Christensen, O. B., Colette, A., Déqué, M., Nikulin, G., Kotlarski, S., van Meijgaard, E., Teichmann, C., and Wild, M.: The impact of climate change on photovoltaic power generation in Europe, Nat. Commun., 6, 10014, https://doi.org/10.1038/ncomms10014, 2015. a
Kato, S., Ackerman, T. P., Mather, J. H., and Clothiaux, E. E.: The k-distribution method and correlated-k approximation for a shortwave radiative transfer model, J. Quant. Spectrosc. Ra., 62, 109–121, https://doi.org/10.1016/S0022-4073(98)00075-2, 1999. a
Kendall, M.: Rank correlation methods, E. Arnold Oxford University Press, London New York, NY, ISBN10 0195208374, 1990. a
Kirn, B., Brecl, K., and Topic, M.: A new PV module performance model based on separation of diffuse and direct light, Sol. Energy, 113, 212–220, https://doi.org/10.1016/j.solener.2014.12.029, 2015. a
Kok, J. F., Ridley, D. A., Zhou, Q., Miller, R. L., Zhao, C., Heald, C. L., Ward, D. S., Albani, S., and Haustein, K.: Smaller desert dust cooling effect estimated from analysis of dust size and abundance, Nat. Geosci., 10, 274–278, https://doi.org/10.1038/ngeo2912, 2017. a
Lacis, A. A. and Oinas, V.: A description of the correlated k distribution method for modeling nongray gaseous absorption, thermal emission, and multiple scattering in vertically inhomogeneous atmospheres, J. Geophys. Res., 96, 9027, https://doi.org/10.1029/90jd01945, 1991. a
Liepert, B. and Tegen, I.: Multidecadal solar radiation trends in the United States and Germany and direct tropospheric aerosol forcing, J. Geophys. Res.-Atmos., 107, AAC 7-1–AAC 7-15, https://doi.org/10.1029/2001JD000760, 2002. a
Liepert, B. G.: Observed reductions of surface solar radiation at sites in the United States and worldwide from 1961 to 1990, Geophys. Res. Lett., 29, 61-1–61-4, https://doi.org/10.1029/2002GL014910, 2002. a
Lindsay, N., Libois, Q., Badosa, J., Migan-Dubois, A., and Bourdin, V.: Errors in PV power modelling due to the lack of spectral and angular details of solar irradiance inputs, Sol. Energy, 197, 266–278, https://doi.org/10.1016/j.solener.2019.12.042, 2020. a
Liu, M., Zhang, J., and Xia, X.: Evaluation of multiple surface irradiance-based clear sky detection methods at Xianghe – A heavy polluted site on the North China Plain, Atmospheric and Oceanic Science Letters, 14, 100016, https://doi.org/10.1016/j.aosl.2020.100016, 2021. a
Long, C. N. and Ackerman, T. P.: Identification of clear skies from broadband pyranometer measurements and calculation of downwelling shortwave cloud effects, J. Geophys. Res.-Atmos., 105, 15609–15626, https://doi.org/10.1029/2000JD900077, 2000. a, b, c, d, e, f
Manara, V., Brunetti, M., Celozzi, A., Maugeri, M., Sanchez-Lorenzo, A., and Wild, M.: Detection of dimming/brightening in Italy from homogenized all-sky and clear-sky surface solar radiation records and underlying causes (1959–2013), Atmos. Chem. Phys., 16, 11145–11161, https://doi.org/10.5194/acp-16-11145-2016, 2016. a
Mann, H. B.: Nonparametric Tests Against Trend, Econometrica, 13, 245, https://doi.org/10.2307/1907187, 1945. a
Mateos, D., Sanchez-Lorenzo, A., Antón, M., Cachorro, V. E., Calbó, J., Costa, M. J., Torres, B., and Wild, M.: Quantifying the respective roles of aerosols and clouds in the strong brightening since the early 2000s over the Iberian Peninsula, J. Geophys. Res.-Atmos., 119, 10382–10393, https://doi.org/10.1002/2014JD022076, 2014. a
Michalsky, J., Dutton, E., Rubes, M., Nelson, D., Stoffel, T., Wesley, M., Splitt, M., and DeLuisi, J.: Optimal Measurement of Surface Shortwave Irradiance Using Current Instrumentation, J. Atmos. Ocean. Tech., 16, 55–69, https://doi.org/10.1175/1520-0426(1999)016<0055:OMOSSI>2.0.CO;2, 1999. a
Mol, W. B., van Stratum, B. J. H., Knap, W. H., and van Heerwaarden, C.: Reconciling observations of solar irradiance variability with cloud size distributions, J. Geophys. Res.-Atmos., 128, e2022JD037894, https://doi.org/10.1029/2022JD037894, 2023. a
Moss, R. H., Edmonds, J. A., Hibbard, K. A., Manning, M. R., Rose, S. K., van Vuuren, D. P., Carter, T. R., Emori, S., Kainuma, M., Kram, T., Meehl, G. A., Mitchell, J. F. B., Nakicenovic, N., Riahi, K., Smith, S. J., Stouffer, R. J., Thomson, A. M., Weyant, J. P., and Wilbanks, T. J.: The next generation of scenarios for climate change research and assessment, Nature, 463, 747–756, https://doi.org/10.1038/nature08823, 2010. a
Nakajima, T., Tanaka, M., and Yamauchi, T.: Retrieval of the optical properties of aerosols from aureole and extinction data, Appl. Optics, 22, 2951–2959, https://doi.org/10.1364/AO.22.002951, 1983. a
Nakajima, T., Tonna, G., Rao, R., Boi, P., Kaufman, Y., and Holben, B.: Use of sky brightness measurements from ground for remote sensing of particulate polydispersions, Appl. Optics, 35, 2672–2686, https://doi.org/10.1364/AO.35.002672, 1996. a
NASA: Aeronet, Aerosol Robotic Network, https://aeronet.gsfc.nasa.gov/ (last access: 24 October 2024), 2024. a
Ningombam, S. S., Larson, E., Dumka, U., Estellés, V., Campanelli, M., and Steve, C.: Long-term (1995–2018) aerosol optical depth derived using ground based AERONET and SKYNET measurements from aerosol aged-background sites, Atmos. Pollut. Res., 10, 608–620, https://doi.org/10.1016/j.apr.2018.10.008, 2019. a, b
Norris, J. R. and Wild, M.: Trends in aerosol radiative effects over Europe inferred from observed cloud cover, solar “dimming,” and solar “brightening”, J. Geophys. Res.-Atmos., 112, D08214, https://doi.org/10.1029/2006JD007794, 2007. a
O'Neill, N. T., Eck, T. F., Smirnov, A., Holben, B. N., and Thulasiraman, S.: Spectral discrimination of coarse and fine mode optical depth, J. Geophys. Res.-Atmos., 108, 4559, https://doi.org/10.1029/2002JD002975, 2003. a
Ordóñez, C., Garrido-Perez, J. M., and García-Herrera, R.: Early spring near-surface ozone in Europe during the COVID-19 shutdown: Meteorological effects outweigh emission changes, Sci. Total Environ., 747, 141322, https://doi.org/10.1016/j.scitotenv.2020.141322, 2020. a
Papadimas, C. D., Hatzianastassiou, N., Matsoukas, C., Kanakidou, M., Mihalopoulos, N., and Vardavas, I.: The direct effect of aerosols on solar radiation over the broader Mediterranean basin, Atmos. Chem. Phys., 12, 7165–7185, https://doi.org/10.5194/acp-12-7165-2012, 2012. a
Pecenak, Z. K., Mejia, F. A., Kurtz, B., Evan, A., and Kleissl, J.: Simulating irradiance enhancement dependence on cloud optical depth and solar zenith angle, Sol. Energy, 136, 675–681, https://doi.org/10.1016/j.solener.2016.07.045, 2016. a
Pérez-Ramírez, D., Whiteman, D., Smirnov, A., Lyamani, H., Holben, B., Pinker, R., Andrade, M., and Arboledas, L.: Evaluation of AERONET precipitable water vapor versus microwave radiometry, GPS, and radiosondes at ARM sites, J. Geophys. Res.-Atmos., 119, 9596–9613, https://doi.org/10.1002/2014JD021730, 2014. a, b
Petit, J.-E., Dupont, J.-C., Favez, O., Gros, V., Zhang, Y., Sciare, J., Simon, L., Truong, F., Bonnaire, N., Amodeo, T., Vautard, R., and Haeffelin, M.: Response of atmospheric composition to COVID-19 lockdown measures during spring in the Paris region (France), Atmos. Chem. Phys., 21, 17167–17183, https://doi.org/10.5194/acp-21-17167-2021, 2021. a
Pfeifroth, U., Sanchez-Lorenzo, A., Manara, V., Trentmann, J., and Hollmann, R.: Trends and Variability of Surface Solar Radiation in Europe Based On Surface- and Satellite-Based Data Records, J. Geophys. Res.-Atmos., 123, 1735–1754, https://doi.org/10.1002/2017JD027418, 2018. a
Philipona, R., Behrens, K., and Ruckstuhl, C.: How declining aerosols and rising greenhouse gases forced rapid warming in Europe since the 1980s, Geophys. Res. Lett., 36, L02806, https://doi.org/10.1029/2008GL036350, 2009. a
Potier, E., Waked, A., Bourin, A., Minvielle, F., Péré, J., Perdrix, E., Michoud, V., Riffault, V., Alleman, L., and Sauvage, S.: Characterizing the regional contribution to PM10 pollution over northern France using two complementary approaches: Chemistry transport and trajectory-based receptor models, Atmos. Res., 223, 1–14, https://doi.org/10.1016/j.atmosres.2019.03.002, 2019. a
Putaud, J.-P., Van Dingenen, R., Alastuey, A., Bauer, H., Birmili, W., Cyrys, J., Flentje, H., Fuzzi, S., Gehrig, R., Hansson, H., Harrison, R., Herrmann, H., Hitzenberger, R., Hüglin, C., Jones, A., Kasper-Giebl, A., Kiss, G., Kousa, A., Kuhlbusch, T., Löschau, G., Maenhaut, W., Molnar, A., Moreno, T., Pekkanen, J., Perrino, C., Pitz, M., Puxbaum, H., Querol, X., Rodriguez, S., Salma, I., Schwarz, J., Smolik, J., Schneider, J., Spindler, G., ten Brink, H., Tursic, J., Viana, M., Wiedensohler, A., and Raes, F.: A European aerosol phenomenology – 3: Physical and chemical characteristics of particulate matter from 60 rural, urban, and kerbside sites across Europe, Atmos. Environ., 44, 1308–1320, https://doi.org/10.1016/j.atmosenv.2009.12.011, 2010. a
Reifenberg, S. F., Martin, A., Kohl, M., Bacer, S., Hamryszczak, Z., Tadic, I., Röder, L., Crowley, D. J., Fischer, H., Kaiser, K., Schneider, J., Dörich, R., Crowley, J. N., Tomsche, L., Marsing, A., Voigt, C., Zahn, A., Pöhlker, C., Holanda, B. A., Krüger, O., Pöschl, U., Pöhlker, M., Jöckel, P., Dorf, M., Schumann, U., Williams, J., Bohn, B., Curtius, J., Harder, H., Schlager, H., Lelieveld, J., and Pozzer, A.: Numerical simulation of the impact of COVID-19 lockdown on tropospheric composition and aerosol radiative forcing in Europe, Atmos. Chem. Phys., 22, 10901–10917, https://doi.org/10.5194/acp-22-10901-2022, 2022. a, b
Romanou, A., Liepert, B., Schmidt, G. A., Rossow, W. B., Ruedy, R. A., and Zhang, Y.: 20th century changes in surface solar irradiance in simulations and observations, Geophys. Res. Lett., 34, L05713, https://doi.org/10.1029/2006GL028356, 2007. a
Ruckstuhl, C. and Norris, J. R.: How do aerosol histories affect solar “dimming” and “brightening” over Europe?: IPCC-AR4 models versus observations, J. Geophys. Res.-Atmos., 114, D00D04, https://doi.org/10.1029/2008JD011066, 2009. a
Ruckstuhl, C., Philipona, R., Behrens, K., Collaud Coen, M., Dürr, B., Heimo, A., Mätzler, C., Nyeki, S., Ohmura, A., Vuilleumier, L., Weller, M., Wehrli, C., and Zelenka, A.: Aerosol and cloud effects on solar brightening and the recent rapid warming, Geophys. Res. Lett., 35, L12708, https://doi.org/10.1029/2008GL034228, 2008. a
Sanchez-Lorenzo, A., Calbó, J., and Wild, M.: Global and diffuse solar radiation in Spain: Building a homogeneous dataset and assessing their trends, Global Planet. Change, 100, 343–352, https://doi.org/10.1016/j.gloplacha.2012.11.010, 2013. a
Satheesh, S. and Ramanathan, V.: Large differences in tropical aerosol forcing at the top of the atmosphere and Earth's surface, Nature, 405, 60–63, 2000. a
Schwarz, M., Folini, D., Yang, S., Allan, R. P., and Wild, M.: Changes in atmospheric shortwave absorption as important driver of dimming and brightening, Nat. Geosci., 13, 110–115, https://doi.org/10.1038/s41561-019-0528-y, 2020. a
Sengupta, M., Habte, A., Wilbert, S., Gueymard, C., and Remund, J.: Best Practices Handbook for the Collection and Use of Solar Resource Data for Solar Energy Applications, 3rd edn., https://doi.org/10.2172/1778700, 2021. a
Shuvalova, J., Chubarova, N., and Shatunova, M.: Impact of Cloud Condensation Nuclei Reduction on Cloud Characteristics and Solar Radiation during COVID-19 Lockdown 2020 in Moscow, Atmosphere-Basel, 13, 1710, https://doi.org/10.3390/atmos13101710, 2022. a
Smirnov, A., Holben, B., Lyapustin, A., Slutsker, I., and Eck, T.: AERONET processing algorithms refinement, AERONET Workshop, https://www.researchgate.net/profile/Alexander-Smirnov-16/publication/291795812_AERONET_processing_algorithms_refinement/links/56afa70a08ae9ea7c3ad8856/AERONET-processing-algorithms-refinement.pdf (last access: 24 October 2024), 2004. a
Stamnes, K., Tsay, S. C., Wiscombe, W., and Jayaweera, K.: Numerically stable algorithm for discrete-ordinate-method radiative transfer in multiple scattering and emitting layered media, Appl. Opt, 27, 2502–2509, https://doi.org/10.1364/AO.27.002502, 1988. a
Sun, X., Bright, J., Gueymard, C., Acord, B., Wang, P., and Engerer, N.: Worldwide performance assessment of 75 global clear-sky irradiance models using Principal Component Analysis, Renew. Sust. Energ. Rev., 111, 550–570, https://doi.org/10.1016/j.rser.2019.04.006, 2019. a, b
Sun, X., Bright, J. M., Gueymard, C. A., Bai, X., Acord, B., and Wang, P.: Worldwide performance assessment of 95 direct and diffuse clear-sky irradiance models using principal component analysis, Renew. Sust. Energ. Rev., 135, 110087, https://doi.org/10.1016/j.rser.2020.110087, 2021. a, b
Thorsen, T. J., Ferrare, R. A., Kato, S., and Winker, D. M.: Aerosol Direct Radiative Effect Sensitivity Analysis, J. Climate, 33, 6119–6139, https://doi.org/10.1175/JCLI-D-19-0669.1, 2020. a
Tobin, I., Greuell, W., Jerez, S., Ludwig, F., Vautard, R., van Vliet, M. T. H., and Bréon, F.-M.: Vulnerabilities and resilience of European power generation to 1.5 °C, 2 °C and 3 °C warming, Environ. Res. Lett., 13, 044024, https://doi.org/10.1088/1748-9326/aab211, 2018. a
Toledano, C., Cachorro, V. E., Berjon, A., de Frutos, A. M., Sorribas, M., de la Morena, B. A., and Goloub, P.: Aerosol optical depth and Ångström exponent climatology at El Arenosillo AERONET site (Huelva, Spain), Q. J. Roy. Meteor. Soc., 133, 795–807, https://doi.org/10.1002/qj.54, 2007. a, b
Turnock, S. T., Spracklen, D. V., Carslaw, K. S., Mann, G. W., Woodhouse, M. T., Forster, P. M., Haywood, J., Johnson, C. E., Dalvi, M., Bellouin, N., and Sanchez-Lorenzo, A.: Modelled and observed changes in aerfosols and surface solar radiation over Europe between 1960 and 2009, Atmos. Chem. Phys., 15, 9477–9500, https://doi.org/10.5194/acp-15-9477-2015, 2015. a
Université de Lille: Plateformes d'Observation, Université de Lille [data set], https://www.loa.univ-lille.fr/observations/plateformes.html?p=lille (last access: 24 October 2024), 2015. a
van Heerwaarden, C. C., Mol, W. B., Veerman, M. A., Benedict, I., Heusinkveld, B. G., Knap, W. H., Kazadzis, S., Kouremeti, N., and Fiedlerq, S.: Record high solar irradiance in Western Europe during first COVID-19 lockdown largely due to unusual weather, Communications Earth & Environment, 2, 37, https://doi.org/10.1038/s43247-021-00110-0, 2021. a, b, c, d, e, f, g
Velazquez-Garcia, A., Crumeyrolle, S., de Brito, J. F., Tison, E., Bourrianne, E., Chiapello, I., and Riffault, V.: Deriving composition-dependent aerosol absorption, scattering and extinction mass efficiencies from multi-annual high time resolution observations in Northern France, Atmos. Environ., 298, 119613, https://doi.org/10.1016/j.atmosenv.2023.119613, 2023. a
Velders, G. J., Willers, S. M., Wesseling, J., van den Elshout, S., van der Swaluw, E., Mooibroek, D., and van Ratingen, S.: Improvements in air quality in the Netherlands during the corona lockdown based on observations and model simulations, Atmos. Environ., 247, 118158, https://doi.org/10.1016/j.atmosenv.2020.118158, 2021. a
Voigt, C., Lelieveld, J., Schlager, H., Schneider, J., Curtius, J., Meerkötter, R., Sauer, D., Bugliaro, L., Bohn, B., Crowley, J. N., Erbertseder, T., Groß, S., Hahn, V., Li, Q., Mertens, M., Pöhlker, M. L., Pozzer, A., Schumann, U., Tomsche, L., Williams, J., Zahn, A., Andreae, M., Borrmann, S., Bräuer, T., Dörich, R., Dörnbrack, A., Edtbauer, A., Ernle, L., Fischer, H., Giez, A., Granzin, M., Grewe, V., Harder, H., Heinritzi, M., Holanda, B. A., Jöckel, P., Kaiser, K., Krüger, O. O., Lucke, J., Marsing, A., Martin, A., Matthes, S., Pöhlker, C., Pöschl, U., Reifenberg, S., Ringsdorf, A., Scheibe, M., Tadic, I., Zauner-Wieczorek, M., Henke, R., and Rapp, M.: Cleaner Skies during the COVID-19 Lockdown, B. Am. Meteorol. Soc., 103, E1796–E1827, https://doi.org/10.1175/BAMS-D-21-0012.1, 2022. a
Vuilleumier, L., Hauser, M., Félix, C., Vignola, F., Blanc, P., Kazantzidis, A., and Calpini, B.: Accuracy of ground surface broadband shortwave radiation monitoring, J. Geophys. Res.-Atmos., 119, 13838–13860, https://doi.org/10.1002/2014JD022335, 2014. a
Warren, S., Eastman, R., and Hahn, C.: A Survey of Changes in Cloud Cover and Cloud Types over Land from Surface Observations, 1971–1996, J. Climate, 20, 717–738, https://doi.org/10.1175/JCLI4031.1, 2007. a, b
Wild, M.: Global dimming and brightening: A review, J. Geophys. Res.-Atmos., 114, D00D16, https://doi.org/10.1029/2008JD011470, 2009. a
Wild, M., Gilgen, H., Roesch, A., Ohmura, A., Long, C. N., Dutton, E. G., Forgan, B., Kallis, A., Russak, V., and Tsvetkov, A.: From Dimming to Brightening: Decadal Changes in Solar Radiation at Earth's Surface, Science, 308, 847–850, https://doi.org/10.1126/science.1103215, 2005. a
Wild, M., Wacker, S., Yang, S., and Sanchez-Lorenzo, A.: Evidence for Clear-Sky Dimming and Brightening in Central Europe, Geophys. Res. Lett., 48, e2020GL092216, https://doi.org/10.1029/2020GL092216, 2021. a, b
Witthuhn, J., Hünerbein, A., Filipitsch, F., Wacker, S., Meilinger, S., and Deneke, H.: Aerosol properties and aerosol–radiation interactions in clear-sky conditions over Germany, Atmos. Chem. Phys., 21, 14591–14630, https://doi.org/10.5194/acp-21-14591-2021, 2021. a
WMO: Manual on the Global Observing System, WMO, no. 544, Secretariat of the World Meteorological Organization, Geneva, Switzerland, ISBN 978-92-63-13544-5, 2003. a
World Meteorological Organization: WMO Guide To Meteorological Instruments And Methods Of Observation, vol. 8, 7th edn., World Meteorological Organization, 681, ISBN 78-92-63-10008-5, 2008. a
The Strong event class is not considered for the decomposition of the clear-sun irradiances, as it represents less than 1 % of observations in Lille.
- Abstract
- Introduction
- Data and methods
- Analysis of SSI variability over 2010–2022
- Radiative effects of atmospheric components on SSI
- Conclusions
- Appendix A: Description of the cloud-screening methods
- Appendix B: Definition of the models of aerosol optical properties
- Code and data availability
- Author contributions
- Competing interests
- Disclaimer
- Acknowledgements
- Financial support
- Review statement
- References
- Supplement
- Abstract
- Introduction
- Data and methods
- Analysis of SSI variability over 2010–2022
- Radiative effects of atmospheric components on SSI
- Conclusions
- Appendix A: Description of the cloud-screening methods
- Appendix B: Definition of the models of aerosol optical properties
- Code and data availability
- Author contributions
- Competing interests
- Disclaimer
- Acknowledgements
- Financial support
- Review statement
- References
- Supplement