the Creative Commons Attribution 4.0 License.
the Creative Commons Attribution 4.0 License.
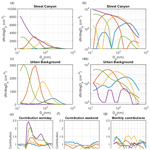
Source apportionment of particle number size distribution at the street canyon and urban background sites
Minna Aurela
Sanna Saarikoski
Jarkko V. Niemi
Harri Portin
Hanna Manninen
Ville Leinonen
Pasi Aalto
Phil K. Hopke
Tuukka Petäjä
Topi Rönkkö
Hilkka Timonen
Particle size is one of the key factors influencing how aerosol particles affect their climate and health effects. Therefore, a better understanding of particle size distributions from various sources is crucial. In urban environments, aerosols are produced in a large number of varying processes and conditions. This study intended to develop the source apportionment of urban aerosols by utilising a novel approach to positive matrix factorisation (PMF). The particle source profiles were detected in particle number size distribution data measured simultaneously in a street canyon and at a nearby urban background station between February 2015 and June 2019 in Helsinki, southern Finland. The novelty of the method is combining the data from both sites and finding profiles for the unified data. Five aerosol sources were found. Four of them were detected at both of the stations: slightly aged traffic (TRA2), secondary combustion aerosol (SCA), secondary aerosol (SecA), and long-range-transported aerosol (LRT). One of the sources, fresh traffic (TRA1) was only detected at a street canyon. The factors were identified based on available auxiliary data. Additionally, the trends of the found factors were studied, and statistically significant decreasing trends were found for TRA1 and SecA. A statistically significant increasing trend was found for TRA2. This work implies that traffic-related aerosols remain important in urban environments and that aerosol sources can be detected using only particle number size distribution data as input in the PMF method.
- Article
(1316 KB) - Full-text XML
-
Supplement
(1445 KB) - BibTeX
- EndNote
Urban aerosol is a complex mixture of particles of various sizes and compositions originating from multiple anthropogenic and natural sources, including sea salt; fuel combustion (e.g. in thermal power generation, incineration, domestic heating, and combustion engines); road, tire, and brake wear; dust; pollen; volcanic ash; forest fires; and industry (Almeida et al., 2006; Guerreiro et al., 2015; Karanasiou et al., 2009). Of these anthropogenic sources are predominant in urban areas (Guerreiro et al., 2015). The negative health effects related to particulate matter (PM) pollution (PM2.5 and PM10) are commonly accepted and well documented (i.e. Koenig, 2000; Wu et al., 2017), also leading to indirect financial consequences through increased mortality and treatment of respiratory and cardiovascular diseases (Johnston et al., 2021). The recent WHO good practice statement encourages the systematic measurement of particle number concentration (PNC) ≥ 10 nm, emphasising the significance of PNC in addition to PM mass (WHO, 2021).
Source apportionment of aerosols can be done in multiple ways. Commonly used source apportionment techniques in atmospheric sciences include k-means cluster analysis, principal component analysis (PCA), and receptor modelling methods. In this work, a receptor modelling method called positive matrix factorisation (PMF) was used. PMF is a mathematical multi-derivative method developed by Paatero (1997) that can be performed for many types of data, and it is currently the most widely used and established source apportion method for atmospheric aerosol particle data (Hopke et al., 2020, 2022). The decision to use PMF was made because PMF is a well-established source apportionment method in environmental sciences, and there was suitable software available. Additionally, as PMF is a factor analysis method, it is fundamentally suitable to this kind of study as it assumes that the observed data are a combination of latent underlying factors. In contrast, PCA, for example, attempts to linearly combine the underlying variables to reduce the size of the data. PMF has been used for chemical composition data (e.g. Li et al., 2003; Makkonen et al., 2023), mass spectra (e.g. Oduber et al., 2021; Teinilä et al., 2022), and particle number size distribution (PNSD) (Krecl et al., 2008; Zhou et al., 2005), as well as in combined matrixes with PNSD and auxiliary data (Rivas et al., 2020). However, conducting source apportionment solely based on PNSD data and using auxiliary data only to verify the sources seems to have some challenges as the source profiles might be mixed with multiple sources (Zhou et al., 2005; Jollife and Cadima, 2016; Krecl et al., 2008). This makes interpreting results using auxiliary data more difficult. To improve the separation between sources when using only PNSD data as input to PMF, PNSD data from two sites are combined into one data file in this study.
Urban aerosol size distributions have been studied in a comprehensive review of urban aerosols consisting of approximately 200 articles, including 114 cities in 43 countries (Wu and Boor, 2021). They stated that in urban environments, the majority of particles are in the size range of 10–100 nm and the PNC decreases approximately by a factor of 100 when the particle size increases from 100 to 1000 nm. In particular, PMF in particular has been applied to size distribution data in numerous studies in urban, suburban, urban background, or residential locations in Asia, Australia, the Middle East, Europe, and USA (Al-Dabbous and Kumar, 2015; Dai et al., 2021; Friend et al., 2012; Gu et al., 2011; Harrison et al., 2011; Kasumba et al., 2009; Kim et al., 2004; Krecl et al., 2008; Leoni et al., 2018; Liu et al., 2017; Ogulei et al., 2007; Pokorná et al., 2020; Rivas et al., 2020; Squizzato et al., 2019; Thimmaiah et al., 2009; Vu et al., 2016; Wang et al., 2013; Yue et al., 2008; Zong et al., 2019). Only one of the studies used data from Helsinki (Rivas et al., 2020). In this study, particle PNSD was investigated in urban background (UB) and street canyon (SC) sites in Helsinki, southern Finland. The simultaneous data from these two sites were analysed in previous studies. Okuljar et al. (2021) investigated the relative contribution of traffic and atmospheric new particle formation to the concentration of sub-3 nm particles. They utilised PNC data between 1 and 800 nm and auxiliary data from the stations. They found that the particle concentrations in the SC were higher over the whole size range. Additionally, they associated particles in the size range of 1–25 nm with local sources at the UB and found particles in the size range of 1–100 nm to have a dominant contribution from local sources in the SC. Rivas et al. (2020) used data from both sites in a study that applied PMF to PNSD data across four European cities. They identified five factors for both stations: nucleation, fresh traffic, urban background, biogenic, and secondary.
Earlier studies conducted in the Helsinki metropolitan area have shown that PNSDs vary based on the dominant source. Nucleation-produced particles are the smallest, with mode particle sizes of 7–11 nm; traffic-influenced emissions have varying mode particle sizes of 10–75 nm, with the smaller particles produced by nucleation and larger particles from soot (Harni et al., 2023; Pirjola et al., 2017; Rivas et al., 2020). Wood burning has been shown to produce slightly larger particles at a mode particle size of 46 nm and to have a wide particle size distribution (Harni et al., 2023; Pirjola et al., 2017). Biogenic emissions have been shown to produce particles with mode sizes between 69 and 100 nm (Harni et al., 2023; Rivas et al., 2020).
This study intended to improve the understanding of urban aerosol sources by applying statistical source apportionment methods such as PMF (EPA PMF 5) to long-term size distribution data. The factors were identified based on the diurnal cycles of PMF factors; available supporting data, including gases (NOx, CO2, O3); and particle chemistry. The data used in this study were measured at two sites, an SC and a UB from February 2015 to June 2019. These datasets, comprising more than 4 years, also allowed for indicative investigation of the trends in PNSDs and a discussion of the reasons for the changes observed in PNSDs. The PMF has been applied to the data from these two sites earlier in a study by Rivas et al. (2020). However, they used data from January 2007 to December 2016, whereas data in this study are from January 2015 to June 2019. Additionally, Rivas et al. (2020) used NO2, NO, SO2, CO, and O3 data in addition to PNSD data in the PMF input files to separate the sources. In contrast, in this study, only the PNSD data were included in the PMF input files, and the results were later compared to the other measurement data. In addition to this, the novelty of this study arises from how the data from the two nearby sites with strongly overlapping aerosol sources were handled by adding the data from the two sites to the same data matrix horizontally, as columns, instead of doing two separate PMF analyses.
2.1 Measurement sites
The data used in this study were measured at two atmospheric measurement stations. The first measurement station is an SC site in Mäkelänkatu in Helsinki, Finland (60°11′47.53′′ N, 24°57′6.41′′ E), and it is governed by the Helsinki Region Environmental Services Authority. The measurement site is situated beside one of the busiest main roads in Helsinki and is heavily influenced by traffic emissions. The SC measurement site is described in detail by Barreira et al. (2021). The second station, the UB station, is located at SMEAR III in Kumpula, Helsinki (60°12′10.41′′ N, 24°57′40.53′′ E). The site is situated near a park area more than 100 m from the nearest busy road. Järvi et al. (2009) describe the SMEAR III station in detail. The distance between the SC and UB stations is approximately 900 m.
2.2 Instruments
Particle PNSD data used in this work were measured between 13 February 2015 and 5 June 2019. The instruments used in the measurements are listed in Table 1. At the SC site, PNSDs were measured with a differential mobility particle sizer (DMPS) consisting of a condensation particle counter (CPC; A 20, Airmodus, Helsinki, Finland) and a Vienna-type differential mobility analyser (DMA). At the UB site, PNSDs were measured with a twin differential mobility particle sizer (Twin-DMPS). Hoppel (1978) describes the working principle of DMA and response functions in detail. The size spectra of DMPS at the SC site were measured in 26 size bins, with particle sizes ranging from 6 to 800 nm and a time resolution of approx. 8 min 40 s to 9 min 5 s. The DMPS at the UB site measured particles in 50 size bins, with particle sizes of 3–794 nm with a time resolution of approx. 9 min 50 s to 10 min 5 s. Both of the DMPS systems were made by the University of Helsinki and approved by the European Center for Aerosol Calibration and Characterization. Both of the systems had dryers in the inlet lines to keep the relative humidity (RH) below 40 %. The DMPS charger had difficulties charging the three smallest particle size bins (6.0, 7.3, and 9.0 nm) on the SC site; therefore, particles smaller than 10 nm were excluded from the analysis for both sites. Both DMPS systems participated in an intercomparison with a reference instrument from the Leibniz Institute for Tropospheric Research (TROPOS) DMPS in the UB station between 11 and 14 June 2021 and demonstrated comparable results. Non-refractory PM1 (organics, sulfate, nitrate, ammonium, and chloride) was measured with an aerosol chemical speciation monitor (Q-ACSM, Aerodyne Research Inc.; Ng et al., 2011) at the SC site. A mass-based Q-ACSM calibration was performed using dried selected-size (300 nm of mobility diameter) ammonium nitrate and ammonium sulfate aerosol particles. The effective nitrate-response factor (RFNO3) relative ionisation efficiencies of sulfate and ammonium (RIENH4) and relative ionisation efficiency of sulfate (RIESO4) were determined, and analyte signals were converted into nitrate-equivalent mass concentrations. IE(NO3) varied over the years, and the final correction of the NRPM1 mass was done against the mass concentrations derived from DMPS data as described by Barreira et al. (2021). The RIE for SO4 varied from 0.51–0.61 and for NH4 3.8–5.32. An effusive source of naphthalene, located in the detection region, was used as a reference for and ion transmission calibrations. A Nafion dryer was installed prior to the instrument inlet so that the RH of the sample flow was maintained below 40 %. A chemical-composition-dependent collection efficiency was used, having been calculated according to Middlebrook et al. (2012), with the exception that a collection efficiency of 0.45 was used for samples when ammonium was below the detection limit. More information can be found in Barreira et al. (2021).
PM2.5 and PM10 mass concentrations were measured using tapered element oscillating microbalance (TEOM; model 1405). The black carbon (BC) concentrations at the SC were measured using an aethalometer (AE33, Aerosol Magee Scientific). NOx and O3 concentrations were measured with a Horiba APNA-370 and a Horiba APOA-370. At the SC, CO2 concentrations were measured with the LICOR model LI-7000 and CO with a Horiba APMA-360. At the UB, NOx and O3 were measured with Thermo Environmental Instruments products 42S and 49. SO2 and CO were measured with a Horiba APMA-370 and a Horiba, APSA-360.
2.3 Meteorology
Helsinki is a northern city with four seasons. The total radiance (Itot) and RH were measured beside the UB at the Helsinki Kumpula weather station (60.203071° N; 24.961305° E, 24 m a.s.l.). The temperature (T) used in this study was measured at the Helsinki Kaisaniemi weather station (60.17523° N, 24.94459° E; 3 m a.s.l.), situated 2.4 km south of the SC and 3.2 km from the UB; the T data measured at the Helsinki Kumpula weather station had a large gap in late 2017, missing several months of data. The monthly average RH, T, and Itot are presented in Fig. 1. The RH reached maximum values during early winter (November–January), and the lowest values were measured during late spring (May). T and Itot reached maximum values during the summer months (June–August). Itot reached the maximum slightly earlier (June–July) than T (July–August). The highest monthly average T was measured during July 2018 (21.3 °C), and the lowest T was in January 2016 (−9.2 °C, Fig. 1c). In this paper, meteorological data were used in the interpretation of the results.
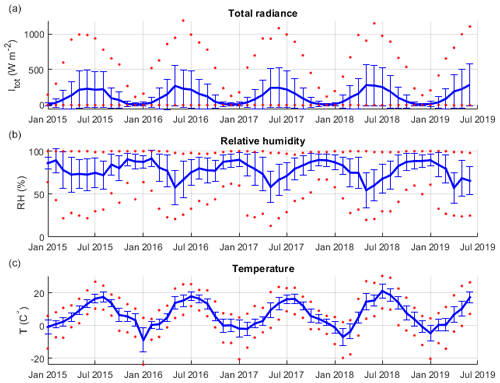
Figure 1Panel (a) presents monthly mean values for Itot, panel (b) for RH, and panel (c) for T over the measurement period (2015–2019) in Helsinki. The constant blue line represents the monthly mean value. The bars show the standard deviation, and the red dots show the maximum and minimum values counted from hourly values.
2.4 Data processing
The data were processed in the following manner before being input into the PMF: initially, outliers were identified and eliminated separately at both stations. Subsequently, the data were averaged on an hourly basis independently at each station. The data were then interpolated to 16 size bins at both locations. Finally, the data from the two sites were merged horizontally into a single matrix with 32 bins in total. In more detail, strong outliers were removed from the DMPS data by calculating the total concentration and removing the data points that had a concentration 10 times larger or smaller than the adjacent measurement points. These relatively relaxed outlier criteria were applied because the measurement site is less than a metre from the driving lanes, and, therefore, variations in the concentrations can be expected due to passing cars. After removing the outliers, the size distribution data from the DMPS were averaged over 1 h periods to minimise the effect of varying lengths of measurement cycles (approx. 8 min 40 s to 9 min 5 s at the SC and approx. 9 min 50 s to 10 min 5 s at the UB). Additionally, this allowed for matching time stamps on the SC and UB data. Only 1 h data points that had full coverage of data from both stations were included in the subsequent analyses. Averaging is not expected to affect the source profiles significantly, and average PNSD data have been used in PMF analysis in previous publications (e.g. Ogulei et al., 2006a).
The data analysis was done using a novel method of combing PNSD data from the two measurement stations horizontally so that PMF could solve factors for both stations simultaneously. To the authors' knowledge, this approach has not been used before. In this approach, a single common factor is calculated for both stations, comprising 32 size bins. The initial 16 size bins are associated with the SC and the remaining 16 with the UB. Given that there is only one set of factors, the time series are identical for both stations, whereas the size distribution profiles vary between the sites. If a factor has a substantial local contribution at one of the sites but not at the other, then its profile is pronounced at that station and near zero at the other. To be able to do this reliably and give an even weight to the data at both stations, the number and the particle size limits of bins need to be the same at both stations. If they are originally different they need to be modified to be identical. This was also necessary because growing the size of data files over a certain point would cause the EPA PMF 5.0 program to crash during the analysis because of the program running out of memory. The data were reduced so that SC and UB PNSDs presented in 16 size bins each. Data size bins have been modified for PMF in other studies. For example, Zhou et al. (2005), reduced 165 bins to 33 by averaging over five bins. In this study, bins were reduced so that a vector with an even lognormal bin width was created starting from 1 nm (lognormal width of 0.11 in this study). Then, the SC and UB data were interpolated linearly to this diameter vector so that the value for each new diameter point was given as a linear interpolation in a logarithmic x axis between the nearest original diameters. The new size bin midpoint diameters were 12.6, 16.2, 20.9, 26.9, 34.7, 44.7, 57.5, 74.1, 95.5, 123, 158, 204, 263, 339, 437, and 562 nm. The interpolation needs to be done on a logarithmic x axis; otherwise, the interpolated concentration is overestimated with a negative derivative and underestimated with a positive derivative of the PNSD curve. This effect is demonstrated in Fig. S1 in the Supplement. This approach enabled us to give the same diameters to both sites regardless of the original size bins. This procedure has two drawbacks. One is that the data are levelled slightly as the interpolated values are always between two original values. Therefore, the highest peaks are slightly lower, and the lowest bottoms are slightly higher than in the original data. The second drawback is that some of the smaller changes in the PNSD may be lost. An example of reduced data compared to the original data is presented in Fig. S2.
The decision to join the data horizontally instead of doing the PMF analysis separately for the SC and UB was made because the idea in this study was to use only PNSD data as input data in PMF analysis and to use only the auxiliary data for the identification of the factors. When the PMF analyses were done separately for the stations without additional data, the PMF was able to split the measured PNSD into factors at each site. However, seemingly any number of factors could be fitted, with PMF only fractioning the measured PNSD to more sub-modes. Additionally, when PMF analysis was performed separately, the attained factors had different modes and mode concentration in between the stations in all cases. This is not likely to resemble reality as the stations reside less than 1 km from each other, and, therefore, somewhat similar background and long-range transport factors would be expected. Adding the data from the two sites together horizontally forces the PMF to find a common time series between the stations. This is beneficial to finding the common factors for the UB and SC as the time series of the common factors can be expected to be similar because of the small distance between the stations. On the other hand, joining the data horizontally does not force the same factor profiles for both sites. An additional problem of doing the PMF separately for the stations was that all factors at the SC site seemed to correlate strongly with the traffic diurnal cycle, indicating that the traffic emissions are split between the different factors. The four-, five-, and six-factor solutions of the PMF analyses done separately for SC and UB are presented in Figs. S3, S4, and S5, respectively. The negative side of merging the data horizontally can be expected to lower the total explained a fraction of the PNSD, while the common time series are forced for both of the stations.
Source apportionment is typically conducted seasonally (winter, spring, summer, and autumn) among most long-term size distribution source apportionment analyses (Hopke et al., 2022). However, in this study, the data were analysed in one set to allow us to evaluate the changes in the contributions of various factors over the whole measurement period. Rose et al. (2021) stated that to maintain the representation of the total reliable concentration (Ntot), data coverage needs to exceed 50 % on the seasonal level and 60 % on the annual level; for the reliable evaluation of diurnal variation, yearly coverage of 75 % was required. The seasonal coverages of the overlapping data for both sites are presented in Table 2. Notably, the coverages for the first and last seasons (i.e. winter 2015 and summer 2019) were low as the measurement period started and ended in the middle of the seasons.
2.5 PMF
Developed by Paatero (1997), PMF is a multi-derivative method that is widely used in environmental sciences to apportion the sources of the measured data. PMF is a least-squares method based on the fact that the matrix X with dimensions n × m can be presented as a product of two matrices: A, with dimensions n × y, and B, with dimensions y × m. This can be used to make the matrix n × m the measured result matrix with n observations and with m species, or, in the case of PNSD, particle size bins and y can be set to be the number of independent sources.
In estimating error, the methodology was established by Ogulei et al. (2006b) and further developed by Rivas et al. (2020). The measurement uncertainties (σij) were calculated with the following equation:
where α is the arbitrary constant, similar to the work of Rivas et al. (2020) (0.02 for the SC and 0.022 for the UB), Nij is the concentration of sample i in size bin column j, and is the arithmetic mean of the concentration in size bin j. The overall uncertainty was calculated using the following equation:
where σij is measurement uncertainty, C3 is an arbitrary constant that was set in this study to 0.1 for both the UB and SC, and Nij is the concentration of bin j of sample i.
Wiedensohler et al. (2012) stated that concentration measurement errors seem to be approximately double for particles in the size range of 200–800 nm compared to those of 20–200 nm. Therefore, in this study, the measurement errors are corrected for these particle sizes by doubling the α factor.
The most reasonable solution to fit the data was a five-factor solution based on the testing to produce results with the most meaningful physical interpretation and reasonable residuals. The robustness of the solution was tested using five different random seeds as starting points. Performing analysis with a larger seed number sometimes caused the program to crash. In addition to using random seeds, a displacement analysis was performed on the solutions. The results of the displacement analysis showed no drop in Q values or swaps in any of the analyses. The factors were identified as fresh traffic (TRA1), slightly aged traffic (TRA2), secondary combustion aerosol (SCA), secondary aerosol (SecA), and long-range-transported aerosol (LRT). The dispersion-corrected PMF results were also calculated for the five factors for comparison, and the difference in the results calculated without the dispersion correction was found to be negligible (Dai et al., 2021). The differences between the normal and dispersion normalised PMF were evaluated based on the Pearson correlation coefficients between workday diurnals (> 0.98 for all factors), weekend diurnals (> 0.98 for all factors), monthly contributions (> 0.96 for all factors), and factor profiles (> 0.97 for all factors). In dispersion correction, the original measurement data are normalised by the ventilation coefficient, which is the height of the boundary layer multiplied by the average wind speed during the period. The goal of the dispersion correction is to reduce the inaccuracy in the source apportionment caused by the dispersion of aerosol in the atmosphere (Dai et al., 2021). The results calculated with dispersion normalisation are presented in Fig. S6. Figure 2 shows the mean residuals, mean scaled residuals, mean relative residuals, and values for the different number of factors between 2 and 10. At the chosen five-factor solution, the mean relative residual was only around 2.8 % on average. The residual and values decrease continuously as the number of factors increases. However, for scaled residuals, mean relative residuals, and values, the decrease is smaller after increasing the number of factors past five. This is an indication that five is an acceptable number of factors. Additionally, the neighbouring solutions of four and six are presented in Figs. S7 and S8, respectively. The four-factor solution merges the factors described later in this paper (SCA and SecA). In a five-factor solution, these two have notably different diurnal profiles, and, therefore, merging them is not sensible. The six-factor solution presented in Fig. S8 splits the SCA into two factors that have very similar diurnal profiles and contributions throughout the year, and, therefore, they are likely to be from the same source. In Fig. S9, the average relative residuals with the standard deviation are presented for each size bin. Also, the figures showing the regressions between the modelled and measured concentrations after interpolation are presented in Fig. S10. The average difference between these was 12.7 % at SC and 6.7 % at UB, and the temporal patterns for the difference are presented in Fig. S11.
2.6 Trend analysis
Time series of PMF factors were fitted with trends using the Theil–Sen estimator established by Theil (1950) and further developed by Sen (1968). The base-level concentrations at the beginning of the measurement period were calculated using the slope determined by the Theil–Sen estimator with each data point and counting the median. Because factors had clear seasonal variance, the Theil–Sen estimator was plotted in two ways. One involved using the seasonal Theil–Sen estimator, in which only data from the same months are compared when forming the estimate. The other way was first removing seasonality from data using the seasonal trend decomposition procedure presented by Cleveland et al. (1990). The seasonal trend removal was needed because the reliability of the results was evaluated using the Mann–Kendall test for monotonic trends (Mann, 1945), which can not be done with seasonal data. The results are presented in Table 4. Figures showing the trend decomposition for the factors and the fitted Theil–Sen estimators are presented in the Supplement. The trend decompositions for TRA1, TRA2, SCA, SecA, and LRT are presented in Figs. S12, S13, S14, S15, and S16, respectively. Additionally, the fitted Theil–Sen estimators are presented for TRA1, TRA2, SCA, SecA, and LRT in Figs. S17, S18, S19, S20, and S21, respectively. Notably, the trends calculated using the seasonal Theil–Sen estimator and the Theil–Sen estimator calculated from data without seasonal variability were almost identical, increasing the confidence in using seasonal trend decomposition for the data (Table 4).
3.1 General description of PNSD
Particle PNSD was found to be noticeably different between stations. The time series of the daily average PNSD in each year are presented in Fig. 3. Figure 3k presents the Pearson correlation coefficient as a function of particle size when the daily PNC in each of the 16 size bins are compared between the SC and UB. The observed PNSD at the SC in the size range from 12.6 to 562 nm contained significantly more nanosized (< 100 nm) particles on many occasions as well as higher overall particle concentrations compared to the UB. Notably, the correlation was higher for the larger particle sizes, indicating that if the smallest particles are disregarded, the time series would be relatively similar. The higher overall PNC at the SC, higher correlation for above-200 nm particles, and lower correlation for below-200 nm particles compared to the UB indicate that there was at least one local source producing a lot of nanosized particles at the SC that was missing at the UB.
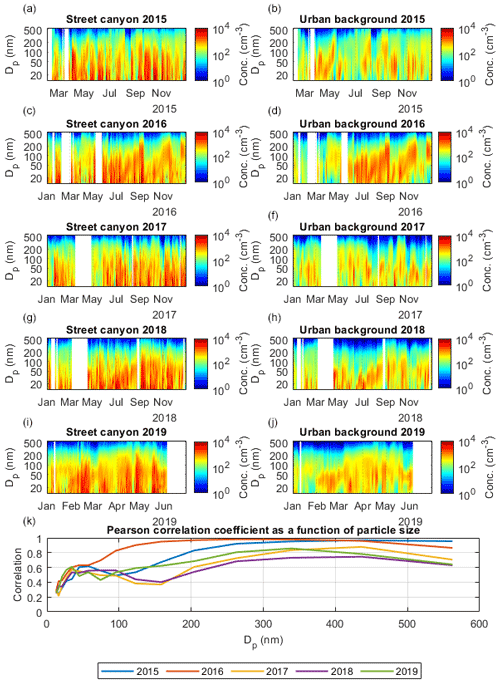
Figure 3Time series of daily average PNSD for SC and UB for each year: 2015 (a, b), 2016 (c, d), 2017 (e, f), 2018 (g, h), and 2019 (i, j). The data used are reduced to 16 size bins. The particle diameter (Dp) is presented on the y axis, the x axis presents the time, and PNC (cm3) is shown by the logarithmic colour scale. The yearly correlation between the UB and SC stations (Pearson correlation coefficient) is presented in the bottom plot for the various particle sizes and daily mean concentrations.
3.2 Identification of factors
The most reasonable solution in the PMF analysis was a five-factor solution. The factors were identified based on the diurnal profiles, annual variation in the factors, and comparison to the available auxiliary data measured at the UB and SC sites (trace gases, particle chemistry, PM mass concentrations, and meteorology). The factor profiles and identification variables, their diurnal contributions for workdays and weekends, and monthly contributions are presented in Fig. 4 and Table 3. The contributions depicted in Fig. 4 represent the scaling factors applied to each source profile at specific times. For instance, if the contribution at a certain time is 2, the corresponding source profile is scaled by a factor of 2 at that moment. The source profiles and their contributions are normalised so that the mean contribution from each source averages to 1 over the measurement period. These contributions were also calculated for each factor separately in Fig. S22 for different wind directions.
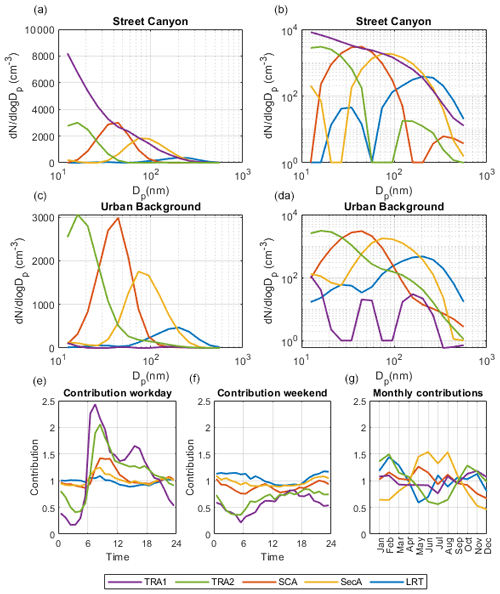
Figure 4PMF factors presented for both stations on linear (a for SC, c for UB) and logarithmic x axes. Panel (e) presents the hourly relative contributions during workdays, panel (f) during weekends, and panel (g) the average monthly contributions. Note that the linear scales for panels (a) and (b) are different. The value presented in contribution figures is the factor with which to multiply the factor profile at any current time to get the total contribution. The average for the contribution factor is 1 over the whole measurement period for all the factors.
TRA1 was interpreted to represent particles that originated from local traffic emissions in the immediate proximity of the measurement station. This factor was the dominant factor in the SC, while it was almost zero at the UB, which was located on a hill over 100 m from the nearest busy road. TRA1 had the highest number of particles in the smallest measured particle size (12.6 nm) and the second mode at around 50–60 nm. Possibly, a third mode at 100–200 nm can be seen as a tail in the log–log plot (Fig. 4b). Similar non-volatile modes at 10 and 70 nm particle diameters have been reported for laboratory measurements for modern petrol cars (Karjalainen et al., 2014). During weekdays, TRA1 had a distinctive diurnal profile, similar to BC and NOx; these are often related to traffic emissions, with the largest peak during the morning rush hour and the second, slightly lower peak during the afternoon rush hour (Fig. 5a). TRA1 had significantly lower contributions during weekends but a high correlation with NOx and BC (Fig. 5b). Overall, the linear relationship between the variables (Pearson correlation coefficient, R) for TRA1 with BC (AE33 with 880 nm) and NOx was 0.76 and 0.85 at the SC, respectively. TRA1 had also a high correlation with NO2 and NO at the SC (Fig. 7).
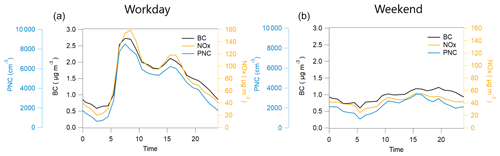
Figure 5Diurnal profiles for TRA1-factor-related PNC concentrations at the SC, along with NOx and BC880 concentrations presented separately for workdays (a) and weekends (b).
TRA2 was interpreted as a slightly aged traffic-related factor. Atmospheric ageing of aerosols is expected to increase the mode particle size of PNSD due to the oxidation of gaseous volatile organic compounds (VOCs) into compounds with lower volatilities. These oxidised compounds then condensate on existing particles, making them larger. Furthermore, smaller particles experience greater diffusion losses. Consequently, we can expect a shift in the mode particle size toward larger particles during ageing. The aged nature of the factor was concluded as shown in Fig. 4e; the morning rush-hour peak of TRA2 is observed 1 h later compared to TRA1. TRA2 also has similar contributions at the SC and UB, and, therefore, the TRA2 was considered to be slightly aged as the road is further away (100 m) from the UB station. Additionally, the mode particle size was larger for TRA2 compared to TRA1, with a maximum mode particle size of 16.2 nm at both stations. TRA2 also displayed a diurnal trend that matches the traffic pattern, with a peak during the morning rush hour and elevated concentrations for the rest of the working hours of the day (Fig. 4e). The morning peak was noticed approximately 1 h later than the TRA1 factor. Therefore, TRA2 can be considered slightly aged, regionally processed traffic emissions. The most significant correlations of TRA2 with auxiliary data were with NOx and NO measured at the UB (Fig. 7). Additionally, TRA2 correlated moderately with NOx and NO measured at the SC. This moderate correlation was expected as the NOx and NO measured at the UB are the background levels that are also measured at the SC. However, in addition, the NOx and NO concentrations at the SC are strongly influenced by the immediate traffic emissions, and, therefore, the correlation of TRA2 with the concentrations at the SC is lower than with the concentrations at the UB. This also supports the conclusion that slightly aged character of TRA2 as the SC site was dominated by immediate traffic-caused emissions (Fig. 4a). The TRA2 was more concentrated during colder months, which might be because at cold T values, VOCs condensate more efficiently on existing particles (Fig. 4g). In addition, the boundary layer is shallower during cold months, enhancing the accumulation of primary pollutants.
The SCA factor had a peak particle size of 44.7 nm at both sites and was interpreted as a secondary aerosol originating from combustion processes (i.e. of liquid fuel such as diesel, oil solid fuel such as biomass and coal, or gas). SCA had relatively weak correlations with the primary traffic emission (e.g. NOx, BC, CO, ) data as could be expected for atmospherically processed aerosol. Of these, (C4H9+, C3H5O+) is a part of the hydrocarbon-like organic aerosol (HOA) mass fraction that is linked to traffic exhaust emissions (Crilley et al., 2013; Crippa et al., 2013; Daellenbach et al., 2016; Mohr et al., 2012). The strongest Pearson correlation coefficient of 0.56 was observed between SCA and NOx at the UB site (Fig. 7). SCA and NOx at the UB site also had similar diurnal patterns on working days and weekends (Fig. 6). The highest SCA peak was seen approximately 3 h later than for the TRA1 factor, indicating that the SCA factor included traffic emissions that had been aged/processed for a couple of hours in the atmosphere. SCA was found to have an evening peak in addition to the morning rush-hour peak (Fig. 4e). The evening peak was more pronounced during weekends, which indicates possible contributions from biomass combustion (Fig. 4f). In an earlier study, BC originating from biomass combustion was shown to contribute 15 ± 14 % at the SC and between 41 ± 14 and 46 ± 15 % of the BC in residential/detached-house areas (Helin et al., 2018). To support this, the diurnal trends of SCA and organic fragments at (Q-ACSM) at the SC were plotted (Fig. 6). The fragments at , particularly its fraction of the total OA, have been widely used as a marker for primary wood combustion emissions (Alfarra et al., 2007). The shape of the diurnal profile was similar to the SCA diurnal profile during the workdays and weekends, strengthening the assumption of wood combustion contribution to SCA. An important thing to note is that the overall correlation with the was still relatively low (Fig. 7). The similar rush-hour peak of to that of SCA was slightly surprising as the is usually related to biomass combustion and not traffic. The annual variation in SCA is small (Fig. 4g), likely because although during the wintertime, the amount of biomass combustion increases the amount of sunlight is low, limiting secondary organic aerosol (SOA) formation, whereas during summer, the amount of biomass burning is lower but the amount of sunlight increases, thus enhancing SOA formation. In contrast, traffic emissions remain stable throughout the whole year.
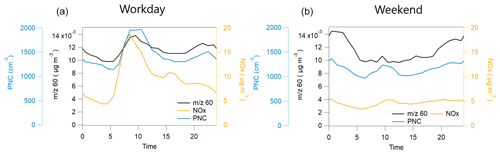
Figure 6Diurnal profiles for SCA-factor-related PNC concentrations at the SC, along with NOx concentration at the UB station and organic fragments at concentrations at the SC during workdays (a) and weekends (b).
The SecA factor had a peak particle size of 74.1 nm at both sites and was interpreted as an aged, photochemically formed secondary aerosol from biogenic and anthropogenic precursors. This assumption is based on the negligible difference in diurnal profiles between workdays and weekends and elevated contribution during the summer months with the highest total radiance (Figs. 1a and 4g). Additionally, the strongest correlations of the SecA factor were with total organics and (Fig. 7). The 43 has been associated with less oxidised secondary organic aerosol (Chen et al., 2022). Anthropogenic and biogenic VOCs are shown to be important SecA precursors in a traffic environment (Saarikoski et al., 2023). In addition, SecA had moderate correlations with and (Fig. 7). The reason for this is likely the high total amount of organics as the and do not refer to the relative fraction of the total organics but absolute concentrations of the mass fraction, and, therefore, they might be elevated with the higher total organic mass in particles. Surprisingly, the SecA factor also somewhat correlates with BC (Fig. 7), possibly indicating that BC particles that are ubiquitous in traffic environments might act as cores for or be mixed into these SecA particles.
The long-range-transported aerosol (LRT) factor had a peak particle size of 204 nm at both sites and is interpreted as a long-range transport because of its strong correlations with PM2.5, SO4, NO3, and organics (Fig. 7). The correlation was even higher (0.80) with the sum of NO3 and SO4 at the SC. Typically higher concentrations of accumulation mode particles have been observed during LRT events (Timonen et al., 2008). Furthermore, Niemi et al. (2009) showed that relatively high concentrations of inorganic ions, especially SO4, NH4, and BC, are typically observed during LRT events. The correlation of LRT with NH4 was relatively high, but the correlation with BC was quite low (Fig. 7). The reason for the low correlation with BC might be due to local sources of BC (e.g. traffic and the short atmospheric lifetime of BC; Cape et al., 2012). Additionally, Niemi et al. (2009) did not report high concentrations of NO3 during the LRT episodes, likely because of evaporation losses of ammonium nitrate from the Teflon filters. However, more recent studies with online analysis of NO3 have linked elevated NO3 concentrations to LRT episodes in the area (Harni et al., 2023; Barreira et al., 2021; Pirjola et al., 2017). Elevated PM1 and PM2.5 concentrations have also been related to the LRT episodes in the area (Harni et al., 2023; Barreira et al., 2021; Niemi et al., 2009; Pirjola et al., 2017). The LRT factor had a moderate correlation with 60. This is likely caused by the large PM of LRT particles and therefore higher total organic mass. Additionally, the correlation with 60 might indicate the contribution of remote biomass burning to the LRT factor.
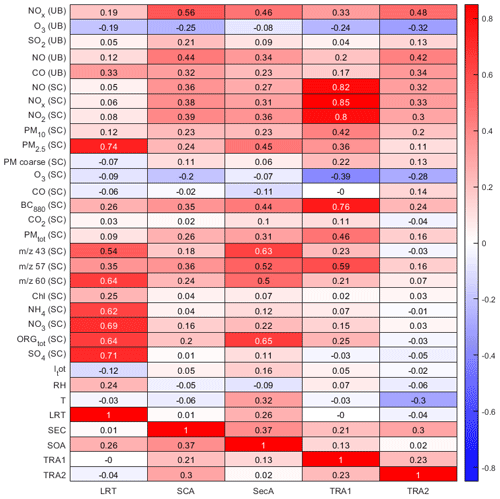
Figure 7Pearson correlation coefficients of the PMF solution factors (LRT, SCA, SecA TRA1, and TRA2) with other factors and other measured parameters (with NOx, O3, SO2, NO, and CO from the UB station; NO, NOx, NO2, PM10, PM2.5 PMcoarse, O3, CO, BC880(AE33), CO2, PMtot, 43, 57, 60 Chl, NH4, NO3, ORGtot, and SO4 from SC; Itot and RH from the Kumpula weather station; and T from the Kaisaniemi weather station.
3.3 Monthly average contributions and trends of factors
Figure 8 represents the time series for the contributions of the PMF factors to PNC at the SC and UB sites. The average monthly contributions at the SC site were 52 %, 15 %, 17 %, 13 %, and 3 % for TRA1, TRA2, SCA, SecA, and LRT, respectively. For the UB, the corresponding monthly average contributions were 1 %, 36 %, 34 %, 23 %, and 7 % for TRA1, TRA2, SCA, SecA, and LRT, respectively. TRA1 was seen to be the main contributor to PNC at the SC, while at the UB, the PNC was usually dominated by the slightly aged combustion-related factors, TRA2 and SCA, with quite similar contributions, as could be expected for stations situated next to the road and 100 m away from the road. During summertime, SecA also made a contribution that was roughly even with those of TRA2 and SCA at both stations, highlighting the importance of secondary aerosol formation even in urban environments. Barreira et al. (2021) described the increased contribution of organic aerosol mass during summertime in Helsinki. Similar contributions of traffic-related aerosols that are either fresh (46 %) like TRA1 in this study or aged (27 %) like TRA2 combined with SCA (sum of 28 %) have been reported in the roadside environment (Al-Dabbous and Kumar, 2015).
The contributions of factors at the SC and UB stations were also calculated in terms of particle volume. Notably, the contributions of the various factors to volume concentrations were different when compared to contributions to the PNC (Fig. 9). The average monthly contributions at the SC were 28 %, 1 %, 4 %, 26 %, and 41 % for TRA1, TRA2, SCA, SecA, and LRT, respectively. For the UB, the monthly average contributions were 1 %, 5 %, 7 %, 29 %, and 59 % for TRA1, TRA2, SCA, SecA, and LRT, respectively. Compared to PNC, the contributions of TRA1, TRA2, and SCA decreased, whereas those of SecA and especially LRT increased. The largest contributor to volume concentration was LRT followed by SecA at the UB. At the SC, the second-largest contributor to particle volume during summer months was also SecA, but during winter, the second-largest contributor was TRA1. The contributions of LRT to volume concentration varied greatly from month to month at both stations. The months of the highest concentrations varied between years, highlighting that the event nature of this factor as singular strong events can increase LRT contributions. This is in contrast to, for example, TRA1, which shows little month-to-month variation, and the concentrations stay relatively stable between the different months in Figs. 8 and 9.
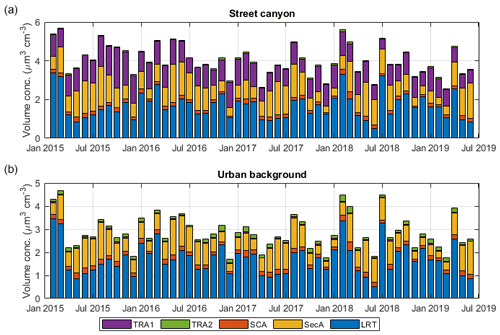
Figure 9Monthly average volume-based contributions of various factors at the SC (a) and UB (b) stations during the measurement period.
Three statistically significant trends in the factor concentrations were found: changing trends of −9.5 % and −6.5 % yearly for TRA1 and SecA, respectively, and an increasing trend of 6.4 % yearly for TRA2 (Table 4). The decreasing trend of TRA1 seems to imply that the primary emissions from traffic have been decreasing over the years, supported by the fact that the vehicle fleet renewed rapidly in Finland between 2015 and 2019. For instance, the proportions of low-emission (EURO 6/VI grade) vehicles in driven kilometres have increased in different vehicle classes in Finland as follows: cars from 6 % to 29 %, vans from 1 % to 25 %, and trucks from 7 % to 31 % (VTT's LIPASTO calculation system for traffic exhaust emission in Finland). The proportion of low-emission city buses has increased particularly quickly in the Helsinki metropolitan area from 17 % to 59 % over the course of 5 years (statistics from the Helsinki Regional Transport Authority, HSL). The impact of bus emissions is significant at the Mäkelänkatu SC site since there is a bus lane very close (0.5 m) to the air quality monitoring station. Notably, TRA2 has increased, possibly due to the change in the engine and after-treatment techniques of the vehicles, emphasising the significance of atmospheric processes interacting with traffic emissions.
Table 4Seasonal Theil–Sen estimators and Theil–Sen estimators calculated from data, with seasonality removed and with confidence levels and base-level concentrations at the beginning of the measurement period for TRA1, TRA2, SCA, SecA, and LRT. The trend in the table is significant if the significance level is above 95 % (p value < 0.05).
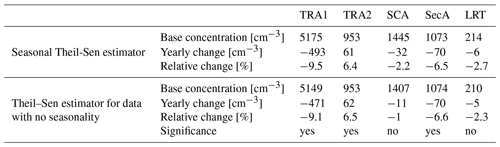
The decrease in the SecA factor is more complex to explain as it was speculated to have both anthropogenic and biogenic sources. The latter is closely connected to relatively stable biogenic sources and meteorology (T and Itot) and the former to the fast development of cleaner engine and after-treatment technologies driven by new emission limits. In the previous chapter, SecA concentrations were shown to indicate possible correlation with BC. One possible reason for this was suggested to be that the BC particles could act as cores for SecA. In fact, the decreasing trend was similar to earlier presented BC trends (decrease between −10 % yr−1 and −6 % yr−1) in different environments (traffic, urban background, and regional background) in Finland (Luoma et al., 2021). No statistically significant trend was found for SCA or LRT, although a slight decrease was indicated for both. There could be many reasons for this. Although traffic emissions have decreased (e.g. Barreira et al., 2021), biomass combustion for residential heating has increased lately. LRT emissions are mainly affected by meteorological conditions and can vary a lot between years. We note that more data (years) are needed to see if the trends found in this study were real as the larger the sample size , the better the hypothetical test. Table 4 presents all the results of the trend analysis.
Particle size is one of the most important parameters of atmospheric particles in terms of health and climate effects. In this study, the origin and characteristics of particle PNSD were investigated in Helsinki, southern Finland. The measurements were carried out at two sites, a UB and an SC site, between 2015 and 2019. The source apportionment based solely on the particle PNSD data was performed using PMF. A novel approach to analyse the data was used, as the particle PNSD data were combined from two nearby sites. As a result, the same factors with the same time series were obtained for both sites, only with different profiles. If a similar profile was seen at both sites, the source was considered regional.
Five factors were found in the data: TRA1, TRA2, SCA, SecA, and LRT. Three of the factors were related to traffic. TRA1 had a clear diurnal profile, with the highest peak during the morning rush hour and the second, slightly lower peak during the afternoon rush hour. TRA2 peaked approximately 1 h later than TRA1, indicating slight processing in the atmosphere. SCA reached a maximum 3 h later than TRA1, making it much more aged. SCA had an evening peak in addition to the morning rush-hour peak, which indicated that it might have originated from both liquid fuel (mainly traffic) and solid fuel (biomass) combustion. TRA1 was the main contributor to PNC at the SC, while at the UB, the PNC was usually dominated by the slightly aged combustion-related factors TRA2 and SCA. During summertime, SecA also made a significant contribution to aerosol PNC at both stations.
The trend analysis revealed that TRA1 and SecA have been decreasing by 9.1 % and 6.6 % yearly, respectively. For TRA2, an increasing trend of 6.5 % yearly was discovered. These findings indicate that the properties of particle emissions originating from traffic have changed in recent years, probably due to the changes in vehicle engines and after-treatment techniques. The significant decreasing trend for TRA1 implies that while the improved emission reduction techniques seem to be reducing freshly emitted particulate emissions of traffic, the slightly aged traffic emissions are even increasing as an increasing trend was observed for TRA2. This change in vehicle fleets is not related to direct emissions only; decreased SecA can be speculated to be linked to decreased core particle concentrations, such as that of BC.
The SCA factor seemed to be a mix of aged traffic particles and particles from biomass combustion. However, the contribution of biomass combustion to the PNC in the traffic environment entails high uncertainty. Additionally, all the factors had more than one mode. Therefore, in addition to the particle size bins, adding the auxiliary data to the PMF analysis might improve the separation between the factors. However, the novel method of attaching simultaneous data from two sites seems to improve the detection of various factors and could be useful in locations where PNSD data are available from more than one site.
In conclusion, traffic remains a large contributor to ambient PNC in urban environments despite the decreasing trend caused by the improvements in emission reduction technologies and electrification of the traffic fleet. Additionally, while the primary emissions have decreased, the effect on the secondary aerosols is more uncertain; in this study, the concentrations of slightly aged aerosols were increasing. Therefore, studying how emissions age in the atmosphere is important in the future. Additionally, the study demonstrated that detecting aerosol source factors purely based on PNSD data is possible, but attaching the factors to individual sources would be difficult without available auxiliary data.
Data are available upon request from the corresponding author, Sami D. Harni (sami.harni@fmi.fi).
The supplement related to this article is available online at: https://doi.org/10.5194/acp-24-12143-2024-supplement.
SDH created the formal analysis, software, and visualisation and wrote the original draft of the paper. MA, SS, and JVN contributed to the conceptualisation and writing by reviewing and editing the article. HP, PA, and HM contributed to the investigation. VL contributed to the formal analysis and reviewing and editing of the article. PKH contributed to the formal analysis. TP and TR contributed to editing and rewriting the article. HT acted as supervisor and contributed to the conceptualisation as well as reviewing and editing of the article.
At least one of the (co-)authors is a member of the editorial board of Atmospheric Chemistry and Physics. The peer-review process was guided by an independent editor, and the authors also have no other competing interests to declare.
Publisher's note: Copernicus Publications remains neutral with regard to jurisdictional claims made in the text, published maps, institutional affiliations, or any other geographical representation in this paper. While Copernicus Publications makes every effort to include appropriate place names, the final responsibility lies with the authors.
The authors acknowledge the financial support of the European Union's Horizon Europe 2020 research and innovation programme, Technology Industries of Finland Centennial Foundation, Business Finland, participating companies, and New York State Energy Research and Development Authority. AI tools were used to improve the language of the article.
This research has been supported by the European Union's Horizon 2020 research and innovation programme (grant nos. 101096133 (PAREMPI), 814978 (TUBE), and 101036245 (RI-URBANS); the Urban Air Quality 2.0 project, funded by Technology Industries of Finland Centennial Foundation; and the Black Carbon Footprint project, funded by Business Finland (grant no. 528/31/2019). The work in Rochester, NY, was funded by the New York State Energy Research and Development Authority under contract nos. 59802 and 125993.
This paper was edited by James Allan and reviewed by two anonymous referees.
Aalto, P., Hämeri, K., Becker, E. D. O., Weber, R., Salm, J., Mäkelä, J. M., Hoell, C., O'Dowd, C. D., Karlsson, H., Hansson, H.-C., Väkevä, M., Koponen, I. K., Buzorius, G., and Kulmala, M.: Physical characterization of aerosol particles during nucleation events, Tellus B, 53, 344–358, https://doi.org/10.1034/j.1600-0889.2001.530403.x, 2001.
Al-Dabbous, A. N. and Kumar, P.: Source apportionment of airborne nanoparticles in a Middle Eastern city using positive matrix factorization, Environ. Sci.-Proc. Imp., 17, 802–812, https://doi.org/10.1039/C5EM00027K, 2015.
Alfarra, M. R., Prevot, A. S. H., Szidat, S., Sandradewi, J., Weimer, S., Lanz, V. A., Schreiber, D., Mohr, M., and Baltensperger, U.: Identification of the mass spectral signature of organic aerosols from wood burning emissions, Environ. Sci. Technol., 41, 5770–5777, https://doi.org/10.1021/es062289b, 2007.
Almeida, S. M., Pio, C. A., Freitas, M. C., Reis, M. A., and Trancoso, M. A.: Source apportionment of atmospheric urban aerosol based on weekdays/weekend variability: Evaluation of road re-suspended dust contribution, Atmos. Environ., 40, 2058–2067, https://doi.org/10.1016/j.atmosenv.2005.11.046, 2006.
Barreira, L. M. F., Helin, A., Aurela, M., Teinilä, K., Friman, M., Kangas, L., Niemi, J. V., Portin, H., Kousa, A., Pirjola, L., Rönkkö, T., Saarikoski, S., and Timonen, H.: In-depth characterization of submicron particulate matter inter-annual variations at a street canyon site in northern Europe, Atmos. Chem. Phys., 21, 6297–6314, https://doi.org/10.5194/acp-21-6297-2021, 2021.
Cape, J. N., Coyle, M., and Dumitrean, P.: The atmospheric lifetime of black carbon, Atmos. Environ., 59, 256–263, https://doi.org/10.1016/j.atmosenv.2012.05.030, 2012.
Chen, G., Canonaco, F., Tobler, A., Aas, W., Alastuey, A., Allan, J., Atabakhsh, S., Aurela, M., Baltensperger, U., Bougiatioti, A., De Brito, J. F., Ceburnis, D., Chazeau, B., Chebaicheb, H., Daellenbach, K. R., Ehn, M., El Haddad, I., Eleftheriadis, K., Favez, O., Flentje, H., Font, A., Fossum, K., Freney, E., Gini, M., Green, D.C., Heikkinen, L., Herrmann, H., Kalogridis, A.-C., Keernik, H., Lhotka, R., Lin, C., Lunder, C., Maasikmets, M., Manousakas, M. I., Marchand, N., Marin, C., Marmureanu, L., Mihalopoulos, N., Močnik, G., Nęcki, J., O'Dowd, C., Ovadnevaite, J., Peter, T., Petit, J.-E., Pikridas, M., Platt, S. M., Pokorná, P., Poulain, L., Priestman, M., Riffault, V., Rinaldi, M., Różański, K., Schwarz, J., Sciare, J., Simon, L., Skiba, A., Slowik, J. G., Sosedova, Y., Stavroulas, I., Styszko, K., Teinemaa, E., Timonen, H., Tremper, A., Vasilescu, J., Via, M., Vodička, P., Wiedensohler, A., Zografou, O., Minguillón, M. C., and Prévôt, A. S. H.: European aerosol phenomenology – 8: Harmonised source apportionment of organic aerosol using 22 Year-long ACSM/AMS datasets, Environ. Int., 166, 107325, https://doi.org/10.1016/j.envint.2022.107325, 2022.
Cleveland, R. B., Cleveland, W. S., and Terpenning, I.: STL: A seasonal-trend decomposition procedure based on loess, J. Off. Stat., 6, 3–73, 1990.
Crilley, L. R, Ayoko, G. A., Jayaratne, E. R., Salimi, F., and Morawska, L.: Aerosol mass spectrometric analysis of the chemical composition of non-refractory PM1 samples from school environments in Brisbane, Australia, Sci. Total Environ., 458–460, 81–89, https://doi.org/10.1016/j.scitotenv.2013.04.007, 2013.
Crippa, M., DeCarlo, P. F., Slowik, J. G., Mohr, C., Heringa, M. F., Chirico, R., Poulain, L., Freutel, F., Sciare, J., Cozic, J., Di Marco, C. F., Elsasser, M., Nicolas, J. B., Marchand, N., Abidi, E., Wiedensohler, A., Drewnick, F., Schneider, J., Borrmann, S., Nemitz, E., Zimmermann, R., Jaffrezo, J.-L., Prévôt, A. S. H., and Baltensperger, U.: Wintertime aerosol chemical composition and source apportionment of the organic fraction in the metropolitan area of Paris, Atmos. Chem. Phys., 13, 961–981, https://doi.org/10.5194/acp-13-961-2013, 2013.
Daellenbach, K. R., Bozzetti, C., Křepelová, A., Canonaco, F., Wolf, R., Zotter, P., Fermo, P., Crippa, M., Slowik, J. G., Sosedova, Y., Zhang, Y., Huang, R.-J., Poulain, L., Szidat, S., Baltensperger, U., El Haddad, I., and Prévôt, A. S. H.: Characterization and source apportionment of organic aerosol using offline aerosol mass spectrometry, Atmos. Meas. Tech., 9, 23–39, https://doi.org/10.5194/amt-9-23-2016, 2016.
Dai, Q., Ding, J., Song, C., Liu, B., Bi, X., Wu, J., Zhang, Y., Feng, Y., and Hopke, P. K.: Changes in source contributions to particle number concentrations after the COVID-19 outbreak: Insights from a dispersion normalized PMF, Sci. Total Environ., 759, 143548, https://doi.org/10.1016/j.scitotenv.2020.143548, 2021.
Friend, A. J., Ayoko, G. A., Jayaratne, E. R., Jamriska, M., Hopke, P. K., and Morawska, L.: Source apportionment of ultrafine and fine particle concentrations in Brisbane, Australia, Environ. Sci. Pollut. R., 19, 2943–2950, https://doi.org/10.1007/s11356-012-0803-6, 2012.
Gu, J., Pitz, M., Schnelle-Kreis, J., Diemer, J., Reller, A., Zimmermann, R., Soentgen, J., Stoelzel, M., Wichmann, H. E., Peters, A., and Cyrys, J.: Source apportionment of ambient particles: Comparison of positive matrix factorization analysis applied to particle size distribution and chemical composition data, Atmos. Environ., 45, 1849–1857, https://doi.org/10.1016/j.atmosenv.2011.01.009, 2011.
Guerreiro, C., de Leeuw, F., Foltescu, V., Ortiz, A. G., and Horálek, J.: Air quality in Europe – 2015 report, EEA Report No 5/2015, https://doi.org/10.2800/62459, 2015.
Harni, S. D., Saarikoski, S., Kuula, J., Helin, A., Aurela, M., Niemi, J. V., Kousa, A., Rönkkö, T., and Timonen, H.: Effects of emission sources on the particle number size distribution of ambient air in the residential area, Atmos. Environ., 293, 119419, https://doi.org/10.1016/j.atmosenv.2022.119419, 2023.
Harrison, R. M., Beddows, D. C. S., and Dall'Osto, M.: PMF Analysis of Wide Particle Size Spectra Collected on a Major Highway, Environ. Sci. Technol., 45, 5522–5528, https://doi.org/10.1021/es2006622, 2011.
Helin, A., Niemi, J. v., Virkkula, A., Pirjola, L., Teinilä, K., Backman, J., Aurela, M., Saarikoski, S., Rönkkö, T., Asmi, E., and Timonen, H.: Characteristics and source apportionment of black carbon in the Helsinki metropolitan area, Finland, Atmos. Environ., 190, 87–98, https://doi.org/10.1016/j.atmosenv.2018.07.022, 2018.
Hopke, P. K., Dai, Q., Li, L., and Feng, Y.: Global review of recent source apportionments for airborne particulate matter, Sci. Total Environ., 740, 140091, https://doi.org/10.1016/j.scitotenv.2020.140091, 2020.
Hopke, P. K., Feng, Y., and Dai, Q.: Source apportionment of particle number concentrations: A global review, Sci. Total Environ., 819, 153104, https://doi.org/10.1016/j.scitotenv.2022.153104, 2022.
Hoppel, W.: Determination of the aerosol size distribution from the mobility distribution of the charged fraction of aerosols, J. Aerosol Sci., 9, 41–54, https://doi.org/10.1016/0021-8502(78)90062-9, 1977.
Järvi, L., Hannuniemi, H., Hussein, T., Junninen, H., Aalto, P. P., Hillamo, R., Mäkelä, T., Keronen, P., Siivola, E., Vesala, T., and Kulmala, M.: The urban measurement station SMEAR II: Continuous monitoring of air pollution and surface-atmosphere interactions in Helsinki, Finland, Boreal Environ. Res., 14, 86–109, 2009.
Johnston, F. H., Borchers-Arriagada, N., Morgan, G. G., Jalaludin, B., Palmer, A. J., Williamson, G. J., and Bowman, D. M. J. S.: Unprecedented health costs of smoke-related PM2.5 from the 2019–20 Australian megafires, Nature Sustainability, 4, 42–47, https://doi.org/10.1038/s41893-020-00610-5, 2021.
Jollife, I. T. and Cadima, J.: Principal component analysis: A review and recent developments, Philos. T. Roy. Soc. A, 374, 20150202, https://doi.org/10.1098/rsta.2015.0202, 2016.
Karanasiou, A. A., Siskos, P. A., and Eleftheriadis, K.: Assessment of source apportionment by Positive Matrix Factorization analysis on fine and coarse urban aerosol size fractions, Atmos. Environ., 43, 3385–3395, https://doi.org/10.1016/j.atmosenv.2009.03.051, 2009.
Karjalainen, P., Pirjola, L., Heikkilä, J., Lähde, T., Tzamkiozis, T., Ntziachristos, L., Keskinen, J., and Rönkkö, T.: Exhaust particles of modern gasoline vehicles: A laboratory and an on-road study, Atmos. Environ., 97, 262–270, https://doi.org/10.1016/j.atmosenv.2014.08.025, 2014.
Kasumba, J., Hopke, P. K., Chalupa, D. C., and Utell, M. J.: Comparison of sources of submicron particle number concentrations measured at two sites in Rochester, NY, Sci. Total Environ., 407, 5071–5084, https://doi.org/10.1016/j.scitotenv.2009.05.040, 2009.
Kim, E., Hopke, P. K., Larson, T. V., and Covert, D. S.: analysis of Ambient particle Size Distributions Using Unmix and Positive Matrix Factorization, Environ. Sci. Technol., 38, 202–209, https://doi.org/10.1021/es030310s, 2004.
Koenig, J. Q.: Health Effects of Particulate Matter, in: Health Effects of Ambient Air Pollution, Springer, Boston, MA, 115–137, https://doi.org/10.1007/978-1-4615-4569-9_10, 2000.
Krecl, P., Hedberg Larsson, E., Ström, J., and Johansson, C.: Contribution of residential wood combustion and other sources to hourly winter aerosol in Northern Sweden determined by positive matrix factorization, Atmos. Chem. Phys., 8, 3639–3653, https://doi.org/10.5194/acp-8-3639-2008, 2008.
Leoni, C., Pokorná, P., Hovorka, J., Masiol, M., Topinka, J., Zhao, Y., Křůmal, K., Cliff, S., Mikuška, P., and Hopke, P. K.: Source apportionment of aerosol particles at a European air pollution hot spot using particle number size distributions and chemical composition, Environ. Pollut., 234, 145–154, https://doi.org/10.1016/j.envpol.2017.10.097, 2018.
Li, A., Jang, J.-K., and Scheff, P. A.: Application of EPA CMB8.2 model for source apportionment of sediment PAHs in Lake Calumet, Chicago, Environ. Sci. Technol., 37, 2958–2965, https://doi.org/10.1021/es026309v, 2003.
Liu, Z., Hu, B., Zhang, J., Xin, J., Wu, F., Gao, W., Wang, M., and Wang, Y.: Characterization of fine particles during the 2014 Asia-Pacific economic cooperation summit: Number concentration, size distribution, and sources, Tellus B, 69, 1303228, https://doi.org/10.1080/16000889.2017.1303228, 2017.
Luoma, K., Niemi, J. V., Aurela, M., Fung, P. L., Helin, A., Hussein, T., Kangas, L., Kousa, A., Rönkkö, T., Timonen, H., Virkkula, A., and Petäjä, T.: Spatiotemporal variation and trends in equivalent black carbon in the Helsinki metropolitan area in Finland, Atmos. Chem. Phys., 21, 1173–1189, https://doi.org/10.5194/acp-21-1173-2021, 2021.
Makkonen, U., Vestenius, M., Huy, L. N., Anh, N. T. N., Linh, P. T. V., Thuy, P. T., Phuong, H. T. M., Nguye, H., Thuy, L. T., Aurela, M., Hellén, H., Love, K., Kouznetsov, R., Kyllönen, K., Teinilä, K., and Oanh, N. T. K.: Chemical composition and potential sources of PM2.5, in Hanoi, Atmos. Environ., 299, 119650, https://doi.org/10.1016/j.atmosenv.2023.119650, 2023.
Mann, H. B.: Non-Parametric Test against Trend, Econometrica, 13, 245–259, https://doi.org/10.2307/1907187, 1945.
Middlebrook, A. M., Bahreini, R., Jimenez, J. L., and Canagaratna, M. R.: Evaluation of Composition-Dependent Collection Efficiencies for the Aerodyne Aerosol Mass Spectrometer using Field Data, Aerosol Sci. Tech., 46, 258–271, https://doi.org/10.1080/02786826.2011.620041, 2012.
Mohr, C., DeCarlo, P. F., Heringa, M. F., Chirico, R., Slowik, J. G., Richter, R., Reche, C., Alastuey, A., Querol, X., Seco, R., Peñuelas, J., Jiménez, J. L., Crippa, M., Zimmermann, R., Baltensperger, U., and Prévôt, A. S. H.: Identification and quantification of organic aerosol from cooking and other sources in Barcelona using aerosol mass spectrometer data, Atmos. Chem. Phys., 12, 1649–1665, https://doi.org/10.5194/acp-12-1649-2012, 2012.
Ng, N. L., Herndon, S. C., Trimborn, A., Canagaratna, M. R., Croteau, P. L., Onasch, T. B., Sueper, D., Worsnop, D. R., Zhang, Q., Sun, Y. L., and Jayne, J. T.: An Aerosol Chemical Speciation Monitor (ACSM) for routine monitoring of the composition and mass concentrations of ambient aerosol, Aerosol Sci. Tech., 45, 780–794, 2011.
Niemi, J. V., Saarikoski, S., Aurela, M., Tervahattu, H., Hillamo, R., Westphal, D. L., Aarnio, P., Koskentalo, T., Makkonen, U., Vehkamäki, H., and Kulmala, M.: Long-range transport episodes of fine particles in southern Finland during 1999–2007, Atmos. Environ., 43, 1255–1264, https://doi.org/10.1016/j.atmosenv.2008.11.022, 2009.
Oduber, F., Calvo, A. I., Castro, A., Blanco-Alegre, C., Alves, C., Calzolai, G., Nava, S., Lucarelli, F., Nunes, T., Barata, J., and Fraile, R.: Characterization of aerosol sources in León (Spain) using Positive Matrix Factorization and weather types, Sci. Total Environ., 754, 142045, https://doi.org/10.1016/j.scitotenv.2020.142045, 2021.
Ogulei, D., Hopke, P. K., and Wallace, L. A.: Analysis of indoor particle size distributions in an occupied townhouse using positive matrix factorization, Indoor Air, 16, 204–215, https://doi.org/10.1111/j.1600-0668.2006.00418.x, 2006a.
Ogulei, D., Hopke, P. K., Zhou, L., Patrick Pancras, J., Nair, N., and Ondov, J. M.: Source apportionment of Baltimore aerosol from combined size distribution and chemical composition data, Atmos. Environ., 40, 396–410, https://doi.org/10.1016/j.atmosenv.2005.11.075, 2006b.
Ogulei, D., Hopke, P. K., Chalupa, D. C., and Utell, M. J.: Modeling Source Contributions to Submicron Particle Number Concentrations Measured in Rochester, New York, Aerosol Sci. Tech., 41, 179–201, https://doi.org/10.1080/02786820601116012, 2007.
Okuljar, M., Kuuluvainen, H., Kontkanen, J., Garmash, O., Olin, M., Niemi, J. V., Timonen, H., Kangasluoma, J., Tham, Y. J., Baalbaki, R., Sipilä, M., Salo, L., Lintusaari, H., Portin, H., Teinilä, K., Aurela, M., Dal Maso, M., Rönkkö, T., Petäjä, T., and Paasonen, P.: Measurement report: The influence of traffic and new particle formation on the size distribution of 1–800 nm particles in Helsinki – a street canyon and an urban background station comparison, Atmos. Chem. Phys., 21, 9931–9953, https://doi.org/10.5194/acp-21-9931-2021, 2021.
Paatero, P.: Least squares formulation of robust non-negative factor analysis, Chemometr. Intell. Lab., 37, 23–35, https://doi.org/10.1016/S0169-7439(96)00044-5, 1997.
Pirjola, L., Niemi, J. V., Saarikoski, S., Aurela, M., Enroth, J., Carbone, S., Saarnio, K., Kuuluvainen, H., Kousa, A., Rönkkö, T., and Hillamo, R.: Physical and chemical characterization of urban winter-time aerosols by mobile measurements in Helsinki, Finland, Atmos. Environ., 158, 60–75, https://doi.org/10.1016/j.atmosenv.2017.03.028, 2017.
Pokorná, P., Leoni, C., Schwarz, J., Ondráček, J., Ondráčková, L., Vodička, P., Zíková, N., Moravec, P., Bendl, J., Klán, M., Hovorka, J., Zhao, Y., Cliff, S. S., Ždímal, V., and Hopke, P. K.: Spatial-temporal variability of aerosol sources based on chemical composition and particle number size distributions in an urban settlement influenced by metallurgical industry, Environ. Sci. Pollut. R., 27, 38631–38643, https://doi.org/10.1007/s11356-020-09694-0, 2020.
Rivas, I., Beddows, D. C. S., Amato, F., Green, D. C., Järvi, L., Hueglin, C., Reche, C., Timonen, H., Fuller, G. W., Niemi, J. V., Pérez, N., Aurela, M., Hopke, P. K., Alastuey, A., Kulmala, M., Harrison, R. M., Querol, X., and Kelly, F. J.: Source apportionment of particle number size distribution in urban background and traffic stations in four European cities, Environ. Int., 135, 105345, https://doi.org/10.1016/j.envint.2019.105345, 2020.
Rose, C., Collaud Coen, M., Andrews, E., Lin, Y., Bossert, I., Lund Myhre, C., Tuch, T., Wiedensohler, A., Fiebig, M., Aalto, P., Alastuey, A., Alonso-Blanco, E., Andrade, M., Artíñano, B., Arsov, T., Baltensperger, U., Bastian, S., Bath, O., Beukes, J. P., Brem, B. T., Bukowiecki, N., Casquero-Vera, J. A., Conil, S., Eleftheriadis, K., Favez, O., Flentje, H., Gini, M. I., Gómez-Moreno, F. J., Gysel-Beer, M., Hallar, A. G., Kalapov, I., Kalivitis, N., Kasper-Giebl, A., Keywood, M., Kim, J. E., Kim, S.-W., Kristensson, A., Kulmala, M., Lihavainen, H., Lin, N.-H., Lyamani, H., Marinoni, A., Martins Dos Santos, S., Mayol-Bracero, O. L., Meinhardt, F., Merkel, M., Metzger, J.-M., Mihalopoulos, N., Ondracek, J., Pandolfi, M., Pérez, N., Petäjä, T., Petit, J.-E., Picard, D., Pichon, J.-M., Pont, V., Putaud, J.-P., Reisen, F., Sellegri, K., Sharma, S., Schauer, G., Sheridan, P., Sherman, J. P., Schwerin, A., Sohmer, R., Sorribas, M., Sun, J., Tulet, P., Vakkari, V., van Zyl, P. G., Velarde, F., Villani, P., Vratolis, S., Wagner, Z., Wang, S.-H., Weinhold, K., Weller, R., Yela, M., Zdimal, V., and Laj, P.: Seasonality of the particle number concentration and size distribution: a global analysis retrieved from the network of Global Atmosphere Watch (GAW) near-surface observatories, Atmos. Chem. Phys., 21, 17185–17223, https://doi.org/10.5194/acp-21-17185-2021, 2021.
Saarikoski, S., Hellén, H., Praplan, A. P., Schallhart, S., Clusius, P., Niemi, J. V., Kousa, A., Tykkä, T., Kouznetsov, R., Aurela, M., Salo, L., Rönkkö, T., Barreira, L. M. F., Pirjola, L., and Timonen, H.: Characterization of volatile organic compounds and submicron organic aerosol in a traffic environment, Atmos. Chem. Phys., 23, 2963–2982, https://doi.org/10.5194/acp-23-2963-2023, 2023.
Sen, P. K.: Estimates of the regression coefficient based on Kendall's tau, J. Am. Stat. Assoc., 63, 1379–1389, 1968.
Squizzato, S., Masiol, M., Emami, F., Chalupa, D. C., Utell, M. J., Rich, D. Q., and Hopke, P. K.: Long-Term Changes of Source Apportioned Particle Number Concentrations in a Metropolitan Area of the Northeastern United States, Atmosphere, 10, 27, https://doi.org/10.3390/atmos10010027, 2019.
Teinilä, K., Timonen, H., Aurela, M., Kuula, J., Rönkkö, T., Hellèn, H., Loukkola, K., Kousa, A., Niemi, J. V., and Saarikoski, S.: Characterization of particle sources and comparison of different particle metrics in an urban detached housing area, Finland, Atmos. Environ., 272, 118939, https://doi.org/10.1016/j.atmosenv.2022.118939, 2022.
Theil, H.: A rank-invariant method of linear and polynomial regression analysis, I. Proc. Kon. Ned. Akad. v. Wetensch., A53, 386–392, 1950.
Thimmaiah, D., Hovorka, J., and Hopke, P. K.: Source Apportionment of Winter Submicron Prague Aerosols from Combined Particle Number Size distribution and Gaseous Composition Data, Aerosol Air Qual. Res., 9, 209–236, https://doi.org/10.4209/aaqr.2008.11.0055, 2009.
Timonen, H., Saarikoski, S., Tolonen-Kivimäki, O., Aurela, M., Saarnio, K., Petäjä, T., Aalto, P. P., Kulmala, M., Pakkanen, T., and Hillamo, R.: Size distributions, sources and source areas of water-soluble organic carbon in urban background air, Atmos. Chem. Phys., 8, 5635–5647, https://doi.org/10.5194/acp-8-5635-2008, 2008.
Vu, T. V., Beddows, D. C. S., Delgado-Saborit, J. M., and Harrison, R. M.: Source apportionment of the Lung Dose of Ambient Submicrometre Particulate Matter, Aerosol Air Qual. Res., 16, 1548–15557, https://doi.org/10.4209/aaqr.2015.09.0553, 2016.
Wang, Z. B., Hu, M., Wu, Z. J., Yue, D. L., He, L. Y., Huang, X. F., Liu, X. G., and Wiedensohler, A.: Long-term measurements of particle number size distributions and the relationships with air mass history and source apportionment in the summer of Beijing, Atmos. Chem. Phys., 13, 10159–10170, https://doi.org/10.5194/acp-13-10159-2013, 2013.
WHO: WHO global air quality guidelines, Coastal And Estuarine Processes, 1–360, 2021.
Wiedensohler, A., Birmili, W., Nowak, A., Sonntag, A., Weinhold, K., Merkel, M., Wehner, B., Tuch, T., Pfeifer, S., Fiebig, M., Fjäraa, A. M., Asmi, E., Sellegri, K., Depuy, R., Venzac, H., Villani, P., Laj, P., Aalto, P., Ogren, J. A., Swietlicki, E., Williams, P., Roldin, P., Quincey, P., Hüglin, C., Fierz-Schmidhauser, R., Gysel, M., Weingartner, E., Riccobono, F., Santos, S., Grüning, C., Faloon, K., Beddows, D., Harrison, R., Monahan, C., Jennings, S. G., O'Dowd, C. D., Marinoni, A., Horn, H.-G., Keck, L., Jiang, J., Scheckman, J., McMurry, P. H., Deng, Z., Zhao, C. S., Moerman, M., Henzing, B., de Leeuw, G., Löschau, G., and Bastian, S.: Mobility particle size spectrometers: harmonization of technical standards and data structure to facilitate high quality long-term observations of atmospheric particle number size distributions, Atmos. Meas. Tech., 5, 657–685, https://doi.org/10.5194/amt-5-657-2012, 2012.
Wu, J., Zhu, J., Li, W., Xu, D., and Liu, J.: Estimation of the PM2.5 health effects in China during 2000–2011, Environ. Sci. Pollut. R., 24, 10695–10707, https://doi.org/10.1007/s11356-017-8673-6, 2017.
Wu, T. and Boor, B. E.: Urban aerosol size distributions: a global perspective, Atmos. Chem. Phys., 21, 8883–8914, https://doi.org/10.5194/acp-21-8883-2021, 2021.
Yue, W., Stölzel, M., Cyrys, J., Pitz, M., Heinrich, J., Kreyling, W. G., Wichmann, H. E., Peters, A., Wang, S., and Hopke, P. K.: Source apportionment of ambient fine particle size distribution using positive matrix factorization in Erfurt, Germany, Sci. Total Environ., 398, 133–144, 2008.
Zhou, L., Kim, E., Hopke, P. K., Stanier, C., and Pandis, S. N.: Mining airborne particulate size distribution data by positive matrix factorization, J. Geophys. Res.-Atmos., 110, D07S19, https://doi.org/10.1029/2004JD004707, 2005.
Zong, Y., Botero, M. L., Yu, L. E., and Kraft, M.: Size spectra and source apportionment of fine particulates in tropical urban environment during southwest monsoon season, Environ. Pollut., 244, 477–485, https://doi.org/10.1016/j.envpol.2018.09.124, 2019.