the Creative Commons Attribution 4.0 License.
the Creative Commons Attribution 4.0 License.
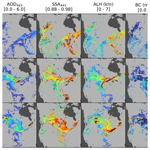
Light-absorbing black carbon and brown carbon components of smoke aerosol from DSCOVR EPIC measurements over North America and central Africa
Alexei Lyapustin
Gregory L. Schuster
Sujung Go
Yujie Wang
Sergey Korkin
Ralph Kahn
Jeffrey S. Reid
Edward J. Hyer
Thomas F. Eck
Mian Chin
David J. Diner
Olga Kalashnikova
Oleg Dubovik
Jhoon Kim
Hans Moosmüller
Wildfires and agricultural burning generate seemingly increasing smoke aerosol emissions, impacting societal and natural ecosystems. To understand smoke's effects on climate and public health, we analyzed the spatiotemporal distribution of smoke aerosols, focusing on two major light-absorbing components, namely black carbon (BC) and brown carbon (BrC) aerosols. Using NASA's Earth Polychromatic Imaging Camera (EPIC) instrument aboard NOAA's Deep Space Climate Observatory (DSCOVR) spacecraft, we inferred BC and BrC volume fractions and particle mass concentrations based on spectral absorption provided by the Multi-Angle Implementation of Atmospheric Correction (MAIAC) algorithm with 1–2 h temporal resolution and ∼ 10 km spatial resolution over North America and central Africa. Our analyses of regional smoke properties reveal distinct characteristics for aerosol optical depth (AOD) at 443 nm, spectral single-scattering albedo (SSA), aerosol layer height (ALH), and BC and BrC amounts. Smoke aerosols in North America showed extremely high AOD up to 6, with elevated ALH (6–7 km) and significant BrC components up to 250 mg m−2 along the transport paths, whereas the smoke aerosols in central Africa exhibited stronger light absorption (i.e., lower SSA) and lower AOD, resulting in higher-BC mass concentrations and similar BrC mass concentrations than the cases in North America. Seasonal burning source locations in central Africa, following the seasonal shift in the Intertropical Convergence Zone and diurnal variations in smoke amounts, were also captured. A comparison of retrieved AOD443, SSA443, SSA680, and ALH with collocated AERONET and CALIOP measurements shows agreement with RMSE values of 0.2, 0.03–0.04, 0.02–0.04, and 0.8–1.3 km, respectively. An analysis of the spatiotemporal average reveals distinct geographical characteristics in smoke properties closely linked to burning types and meteorological conditions. Forest wildfires over western North America generated smoke with a small-BC volume fraction of 0.011 and a high ALH with large variability (2.2 ± 1.2 km), whereas smoke from wildfires and agricultural burning over Mexico region shows more absorption and low ALH. Smoke from savanna fires over central Africa had the most absorption, with a high-BC volume fraction (0.015) and low ALH with a small variation (1.8 ± 0.6 km) among the analyzed regions. Tropical forest smoke was less absorbing and had a high variance in ALH. We also quantify the estimation uncertainties related to the assumptions of BC and BrC refractive indices. The MAIAC EPIC smoke properties with BC and BrC volume and mass fractions and assessment of the layer height provide observational constraints for radiative forcing modeling and air quality and health studies.
- Article
(13859 KB) - Full-text XML
- BibTeX
- EndNote
Natural and anthropogenic fires affect and shape nearly every terrestrial vegetated ecosystem on the planet (Pausas and Keeley, 2009; Bond and Keeley, 2005), and their emissions have long been known to affect the global atmospheric composition and radiative budget (Hobbs et al., 1997; Seiler and Crutzen, 1980). Recent climate changes and anthropogenic activities have affected wildfire and agricultural fire occurrence in many regions (Liu et al., 2010; Dennison et al., 2014). Global monitoring of the atmospheric smoke aerosol chemical, optical, and microphysical properties is important to quantify the impacts of increasing biomass burning on climate and air quality. However, the current understanding of smoke aerosol radiative forcing is still insufficient due to its high spatiotemporal variability in combination with the dynamic nature of smoke and variability in its physical and optical properties (IPCC, 2023).
One characteristic that distinguishes smoke particle components from other components is light absorption. Absorbing particle components converting incident electromagnetic energy into thermal energy results in heating of both the particles and the ambient surrounding atmosphere. Aerosol light absorption greatly affects the direct radiative forcing and atmospheric stability and convections (IPCC, 2023; Bellouin et al., 2005; Yu et al., 2002). Smoke particles emitted from biomass burning typically contain two major light-absorbing carbonaceous components: black carbon (BC) and brown carbon (BrC). The proportions of these light-absorbing components and their mixing ratios determine the spectral absorption characteristics (e.g., Jacobson, 2001; Chakrabarty et al., 2023).
BC is a byproduct of the incomplete combustion of carbonaceous materials. There is no specific chemical makeup of BC, and depending on measurement techniques, it is also called soot, elemental carbon, or light-absorbing carbon (Reid et al., 2005a; Moosmüller et al., 2009; Andreae and Gelencsér, 2006). BC is visibly black, resulting in a high and spectrally invariant imaginary refractive index (∼ 0.79) across UV-visible (UV-Vis) wavelengths (Bond and Bergstrom, 2006). During combustion, tiny BC spherules are aggregated with each other and grow by absorbing surrounding gas-phase molecules into large particles with a complex, generally fractal-like morphology (Moosmüller et al., 2009). Emitted atmospheric BC particles are generally hydrophobic (Petters et al., 2009) but can quickly evolve to hydrophilic if they acquire water-soluble coatings upon emission or during atmospheric aging (Tritscher et al., 2011). Atmospheric aging processes change BC's physical and chemical particle structure (Corbin et al., 2023; Bhandari et al., 2019; Sengupta et al., 2020), as well as optical properties (Gyawali et al., 2017; Kleinman et al., 2020; Reid et al., 2005b). Particle evolutions combine with the high spatiotemporal variability in the sources to make the net radiative effects of these particles highly uncertain (Bond et al., 2013; IPCC, 2023; Chakrabarty et al., 2023).
The largest carbonaceous aerosol component directly emitted from biomass burning is organic carbon (OC; e.g., Andreae and Merlet, 2001; Andreae, 2019, and references therein). This study defines the OC with a significant light-absorbing property in the tropospheric solar spectrum as brown carbon (BrC; e.g., Laskin et al., 2015). BrC exhibits spectral variability, absorbing more ultraviolet (UV) and short visible light than long visible light, resulting in a reddish or brownish appearance. Its imaginary refractive index varies spectrally, with generally higher values at shorter (i.e., UV) wavelengths and decreasing toward longer, visible, and infrared (IR) wavelengths (Kirchstetter et al., 2004). BrC emissions and the chemical processes responsible for BrC formation are complex and not yet fully understood. Some studies suggest that BrC consists primarily of water-soluble organic carbon compounds and humic-like substances (Sun et al., 2007; Phillips and Smith, 2014; Hoffer et al., 2006), whereas others suggest that non-polar compounds can absorb more light than polar compounds, especially in the UV- and short-wavelength visible range (Sengupta et al., 2018). BrC compounds can be released from smoldering biomass burning or be formed through secondary organic aerosol processes in the atmosphere (Chakrabarty et al., 2010; Laskin et al., 2015). BC coated with non-absorbing organic and inorganic compounds may exhibit a similar wavelength dependence of absorption, with higher values at shorter wavelengths (Wang et al., 2016). This similarity makes it challenging to differentiate between BrC and coated BC based on spectral absorption alone. Therefore, our BrC results may include contributions from coated BC.
According to the latest Intergovernmental Panel on Climate Change (IPCC) report (IPCC, 2023), the present-day global effective radiative forcing of black carbon from fossil fuel and biofuel is estimated at 0.107 W m−2, with a 5 %–95 % uncertainty range of −0.202 to 0.417 W m−2, with respect to the pre-industrial time of 1750. In contrast, primary organic aerosols from fossil fuel and biofuel, related to OC, exhibit a cooling effect of −0.209 W m−2, with an uncertainty range of −0.439 to −0.021 W m−2. Although BrC is not directly considered in this assessment, its radiative forcing is partially accounted for within primary organic aerosol, biomass burning, or secondary organic aerosols in some global aerosol models. Combining ground-based measurements and chemical transport modeling, Jo et al. (2016) attributed non-BC absorption to BrC and estimated the BrC fraction as 21 % of the global mean surface OC concentration, significantly impacting ozone photochemistry by altering the UV radiation field. Zhang et al. (2020) estimated that the global BrC direct radiative effect is 0.10 W m−2, suggesting that BrC can heat the tropical mid- and upper troposphere more than BC. Still, much uncertainty remains about BrC due to limited measurements and the complex processes involved, challenging accurate estimates of its radiative impact on climate (Liu et al., 2020).
Intensive in situ measurements have been instrumental in identifying the composition-related spectral light-absorption properties of smoke plumes, as summarized in Bond and Bergstrom (2006), Andreae and Gelencsér (2006), Moosmüller et al. (2009), and Samset et al. (2018). These measurements have enabled remote-sensing techniques to differentiate between various light-absorbing components in smoke plumes. For example, the Aerosol Robotic Network (AERONET) sun photometers routinely provide aerosol optical and microphysical properties, including spectral refractive indices from many sites worldwide (Holben et al., 1998; Dubovik and King, 2000). Using AERONET inversion data, Schuster et al. (2016) inferred aerosol components over smoke- and dust-dominated regions by matching the AERONET spectral refractive index to mixtures of components with different assumed optical properties. Specific absorbing components were assumed as inclusions, namely BC and BrC for smoke and iron oxides of hematite and goethite for dust aerosols. Wang et al. (2013) and Choi et al. (2020) applied a similar approach to East Asian sites. The synergy between visible/near-IR AERONET measurement and UV-visible Multifilter Rotating Shadowband Radiometer (MFRSR) measurements confirmed the sensitivity of spectral absorption consistent with a BrC component (Mok et al., 2016, 2018).
Inferring aerosol composition from satellites is more challenging than from ground-based remote sensing due to the need to account for the surface contribution to the top-of-atmosphere signal and the much greater range of conditions that a spaceborne instrument samples. Retrieving aerosol absorptions using multi-spectral bands in near-UV wavelengths has been applied to instruments such as the Total Ozone Mapping Spectrometer (TOMS) and the Ozone Monitoring Instrument (OMI), which have data records spanning decades, as well as more recently launched instruments like the TROPOspheric Monitoring Instrument (TROPOMI) and Earth Polychromatic Imaging Camera (EPIC; Torres et al., 1998, 2007, 2013, 2020; Ahn et al., 2021). The fraction of retrieved single-scattering albedo (SSA) within the expected error, defined as a fraction within ±0.03 from AERONET SSA, is approximately 50 %, based on long-term and global validation across these sensors (Ahn et al., 2021; Torres et al., 2020).
The Generalized Retrieval of Aerosol and Surface Properties (GRASP) algorithm (Dubovik et al., 2011, 2014) utilizes the multi-angle, multi-channel, and radiometric and polarimetric measurements from the POLarization and Directionality of the Earth's Reflectances (POLDER) instruments. With increased information incorporated by a multi-pixel multi-temporal smoothness constraint, the GRASP algorithm retrieves aerosol optical depth (AOD), particle size information, and absorption, showing robust agreement with global AERONET measurements (Chen et al., 2020). Recent improvement of the GRASP algorithm included the direct estimation of aerosol chemical composition concentrations without the need for intermediate steps such as retrieving refractive indices and particle size distributions (Li et al., 2019, 2020). The Multi-angle Imaging SpectroRadiometer (MISR) research algorithm also accounts for black-smoke and brown-smoke aerosol models (Limbacher et al., 2022), analogous to the BC and BrC components in this study, and is utilized to analyze fractional AODs along transport paths (Junghenn Noyes et al., 2020a, b, 2022). Still, it is worth noting that POLDER and MISR measurements are limited to visible and near-infrared (NIR) channels and do not include ultraviolet (UV) channels, where spectral absorption due to BC and, in particular, BrC is more pronounced.
The EPIC sensor aboard the Deep Space Climate Observatory (DSCOVR) spacecraft has provided UV to near-IR measurements of Earth since 2015 (Marshak et al., 2018). Recent studies by Lyapustin et al. (2021b) have utilized the Multi-Angle Implementation of Atmospheric Correction (MAIAC) processing of EPIC measurements to derive the AOD and spectral absorption. It enables inferring aerosol chemical compositional differences, such as BC and BrC in smoke aerosol plumes and iron oxides (e.g., hematite and goethite) in dust aerosol plumes. DSCOVR's orbit around Lagrange point 1, where the spacecraft remains stably positioned between the Sun and Earth, allows for global monitoring multiple times per day during the daylight hours with a temporal resolution of 1–2 h. In our study, we used EPIC measurements to infer BC and BrC volume fractions and mass concentrations in smoke plumes and identified distinct smoke properties over North America and central Africa. The estimation of iron oxides in dust aerosols using the MAIAC EPIC product was addressed in Go et al. (2022).
The structure of the paper is as follows. Section 2 introduces the MAIAC EPIC smoke aerosol retrieval algorithm and describes the methodology for inferring BC and BrC volume fractions and mass concentrations. It also includes descriptions of study regions and of AERONET and Cloud-Aerosol Lidar with Orthogonal Polarization (CALIOP) validation datasets. In Sect. 3, we analyzed individual smoke cases over North America and central Africa and provided a validation of AOD, spectral SSA, and the aerosol layer height (ALH). Additionally, time-integrated regional properties, including BrC BC ratios and uncertainty estimates based on different inclusion assumptions, are discussed. Finally, Sect. 4 offers the summary and concluding remarks.
2.1 MAIAC EPIC processing algorithm
EPIC measurements cover the entire sunlit hemisphere of Earth with 10 narrowband spectral channels from 317.5 to 779.5 nm. The spatial resolution of EPIC is ∼ 8–16 km at nadir, degrading toward the edge of the image. MAIAC EPIC algorithm grids and processes L1B data at 10 km resolution providing an oversampling. DSCOVR's Lagrange point 1 orbit between the Earth and the Sun (∼ 1.5×106 km) enables global multi-temporal daytime measurements, with 10–12 observations in boreal summer and 6–7 observations in winter at mid-latitudes and little seasonal change in tropical latitudes. Detailed information on EPIC measurements can be found in Marshak et al. (2018). Following the MAIAC Moderate Resolution Imaging Spectroradiometer (MODIS) algorithm (Lyapustin et al., 2018), the standard MAIAC processing offers cloud detection, atmospheric correction, and AOD with regionally specified background aerosol models (background AOD; Lyapustin et al., 2021a). In addition, a newly developed absorbing smoke or dust aerosol retrieval process was applied to both land and ocean pixels. Smoke/dust detection and separation are based on various tests including the UV aerosol index and spectral AOD shape. As EPIC band configuration does not allow us to distinguish between smoke and dust aerosols, the dust retrievals are only performed over pre-defined dust regions, whereas smoke retrievals are performed elsewhere globally (Lyapustin et al., 2021b).
The full algorithm description will be given elsewhere; here, we provide a very brief overview to facilitate understanding of our results. The novel version 3 (v3) MAIAC algorithm represents spectral aerosol absorption with two parameters, the imaginary refractive index at 680 nm (k0) and spectral absorption exponent (SAE), using a conventional power-law expression, , where λ0 = 680 nm. The real refractive index is assumed to have a spectrally invariant value of 1.51 (Lyapustin et al., 2021b). The particle log-normal volume size distribution is defined as
where i indicates each mode (fine and coarse), r is the particle radius, rv,i is the volume mean radius, σi is the geometric standard deviation, and Cv,i is the volumetric concentration. For smoke aerosols, we assumed a fine-mode volume mean radius (0.14 µm) and geometric standard deviation (0.4 µm), as well as a coarse-mode volumetric mean radius (2.8 µm) and geometric standard deviation (0.6 µm). In MAIAC v3, the Levenberg–Marquardt nonlinear optimal fitting algorithm (Levenberg, 1944; Marquardt, 1963) is used to simultaneously retrieve four parameters by matching EPIC measurements at UV to NIR wavelengths, including oxygen A and B bands. The algorithm uses pre-computed lookup tables (LUTs) covering the full range of expected variability in the above parameters. The maximum value of AOD at 443 nm in the algorithm is set to 6. Vertically, the aerosol is modeled by a single 2 km thick aerosol layer placed at different altitudes in the atmosphere, and the reported ALH is defined as the midpoint height of the layer. To avoid systemic biases in absorption, this retrieval is performed over detected absorbing smoke/dust pixels when the retrieved AOD, based on the background aerosol model with fixed regional properties, is greater than 0.4. Note that although smoke retrievals are limited with background AOD443 > 0.4, the retrieved smoke AOD443 could be lower than 0.4 due to different assumption of microphysical properties and the simultaneous retrieval of the spectral absorption and ALH.
2.2 MAIAC smoke composition inference
Given a very different spectral absorption of BC (high and spectrally fairly flat) and BrC (low and strongly increasing towards UV), the retrieved spectral absorption can be used to derive fractions of absorbing components. We assume that smoke aerosols consist of a non-absorbing host and two absorbing species, BC and BrC, with internal mixing based on the Maxwell–Garnett effective medium approximation (MG-EMA) (Bohren and Huffman, 1998; Schuster et al., 2005, 2016). The MG-EMA is suitable for characterizing smoke particles and is computationally efficient (Garnett, 1904; Bohren and Huffman, 1998; Schuster et al., 2005; Markel, 2016a, b). For that reason, it is widely used for inferring aerosol compositions from ground-based or satellite-based remote sensing (Li et al., 2019; Schuster et al., 2005, 2016; Choi et al., 2020; Go et al., 2022). Studies showed that different internal mixing rules, such as the Bruggeman approximation or volume averaging, yield similar results to the MG-EMA for inferring smoke components (Schuster et al., 2016; Li et al., 2019, and references therein). External mixing could be assumed, resulting in lower absorption than internal mixing (Lesins et al., 2002; Lack et al., 2012), but most BC particles exist in an internally mixed manner with other components in biomass-burning plumes (Schwarz et al., 2008). The non-absorbing host (or medium) represents a mixture of non-absorbing or low-absorbing components in smoke, such as non-absorbing OC, sulfate, nitrate, and/or ammonium. Although there are various ranges of refractive indices for both BC and BrC based on the literature and experiments, this study assumes a fixed refractive index to estimate their fractions from the limited information of the retrieved optical properties. The BC refractive index assumes the suggestion by Bond and Bergstrom (2006), which involves being spectrally flat with a real part (n) of 1.95 and an imaginary part (k) of 0.79 for the visible spectrum (i.e., 400–700 nm). A spectral dependence of k for BrC is based on Kirchstetter et al. (2004), whereas a constant real part of 1.54 was assumed based on Li et al. (2019). For a spectrally flat and non-absorbing host, we assume n = 1.51, consistent with the smoke aerosol model in the MAIAC EPIC algorithm, and k = 10−9, based on Kalashnikova et al. (2018). Table 1 summarizes the spectral refractive indices of BC, BrC, and the host. Please note that a sensitivity test for different assumptions regarding BC and BrC imaginary refractive indices affecting their volume fractions is detailed in Sect. 3.5.
The MG-EMA equation for smoke aerosol mixtures, as described in Bohren and Huffman (1998) and Schuster et al. (2005), is presented below.
Here, ϵm, ϵh, ϵBC, and ϵBrC represent the complex dielectric functions of the mixture, host, BC, and BrC, respectively. fBC and fBrC denote the volume fractions of BC and BrC, respectively. Note that identical BC and BrC components are assumed for both fine and coarse modes. Throughout plume evolution, different processes such as oxidation, hydration, deposition of volatile organics onto existing particles, or new particle formation may lead to larger particle sizes. Consequently, the fine-mode and coarse-mode components in smoke aerosols could exhibit differences. Schuster et al. (2016) also accounted for different component combinations between fine and coarse modes, considering dust particles for the coarse mode. It should be noted that biomass-burning aerosols are strongly dominated by the fine-mode component, with typically only a minor coarse-mode AOD. However, the MAIAC EPIC processing relies on a static particle size distribution, and the dynamic separation of fine and coarse modes is challenging with limited measurement information.
The refractive indices of the mixture can be determined using the following equations:
where ϵr and ϵi represent the real and imaginary parts of the mixture dielectric function ϵm. Given fixed spectral refractive indices of the host and inclusions (BC and BrC), the mixture refractive indices are determined by the volume fractions of two inclusions (fBC and fBrC).
Subsequently, we utilized the Levenberg–Marquardt nonlinear least square fitting method (Levenberg, 1944; Marquardt, 1963; Press et al., 2007) to derive the volume fractions of inclusions by comparing inferred and calculated refractive indices with the MG-EMA. Retrieved k0 and SAE were converted into spectral imaginary refractive indices (kλ for λ of 340, 380, 443, and 680 nm) and matched with theoretical values of a mixture to find solutions for fBC and fBrC.
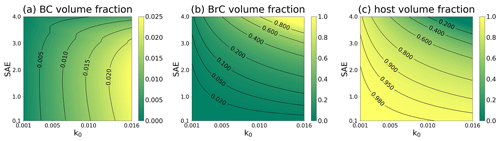
Figure 1The range of volume fractions for (a) BC, (b) BrC, and (c) the host across different values of k0 and SAE.
Figure 1 illustrates the derivable BC, BrC, and host volume fractions for assumed ranges of k0 (0.001–0.016) and SAE (0.1–4) in the MAIAC EPIC algorithm. Available fBC, fBrC, and fhost ranges are from 0 to 0.025, 0.994, and 0.998, respectively, where . The maximum fBC of 0.025 can be found in the condition of the maximum k0 of 0.016. A high fBrC near 1 can be retrieved when both k0 and SAE are high. The host volume fraction (fhost) shows an opposite tendency to fBrC and is low when both k0 and SAE are high. Conversion from retrieved k0 and SAE to volume fractions follows the presented distributions.
It should be mentioned that the upper limit of k0 = 0.016 was found empirically based on limited EPIC regional processing and then confirmed by the global processing of EPIC data. However, this limit may be increased in the future, based on detailed analysis of EPIC retrievals, in particular because AERONET inversion retrievals often show higher values, for example, in central and southern Africa savanna burning region (Eck et al., 2003).
The inferred volume fractions of BC and BrC can be converted to column-integrated volume concentrations as
where Cv is the column-integrated volume concentration (with a unit of µm3 µm−2), and the subscripts f and c indicate the fine mode and coarse mode, respectively. Despite the regional dependence of in the 4D-retrieval algorithm for smoke, we assume a static of 0.7 for BC and BrC processing to maintain consistency and reduce regional discrepancies arising from the ratio. Hygroscopicity was neglected using a static AOD per volume concentration, regardless of relative humidity. Given the size distribution and n, hf of 8.43 µm2 µm−3 is fine-mode AOD443 per unit volume concentration (µm3 µm−2) and hc of 0.72 µm2 µm−3 is coarse-mode AOD443 per unit volume concentration, as calculated based on Mie theory in the MAIAC EPIC smoke model (Lyapustin et al., 2021b). Given the complex refractive indices, size distribution with fine mode or coarse mode only, and non-sphericity, the h values, representing total column AOD per unit volume concentration, are computed using the DLS sphere and spheroid package (Dubovik et al., 2006) at a volume concentration of 1 µm3 µm−2. hf and hc are computed separately for the fine and coarse modes within the MAIAC LUT-generation package and can be used to assess mass extinction efficiency (MEE) with assumption of particle density. The column-integrated mass concentration of the chemical component is calculated as , where i indicates inclusions (BC and BrC), and ρ is the mass concentration per unit volume. We use ρBC of 1.8 g cm−3 and ρBrC of 1.2 g cm−3, following previous studies (Bond and Bergstrom, 2006; Turpin and Lim, 2001; Schuster et al., 2016; Li et al., 2020).
2.3 Study regions
We selected two major regions where smoke aerosols are dominant but exhibit different characteristics: North America (13–80° N and 170–50° W) and central Africa (5° N–17° S and 8–42° E). To avoid potential interference from dust aerosols on smoke analysis, we excluded the Sahel region bounding the Sahara desert from this study. The selected smoke aerosol analysis regions, along with detected fire counts from the Visible Infrared Imaging Radiometer Suite (VIIRS) instrument on board the Suomi National Polar-orbiting Partnership (NPP) satellite in 2018, are presented in Fig. 2. This study focused on the entire year of 2018, a year marked by one of highest monthly average AOD during the summer over North America (Eck et al., 2023). The EPIC dataset exhibited no temporal gaps, and ample AERONET and CALIOP data were accessible. Additionally, we included a single case study from 2017 to complement our analysis over North America.
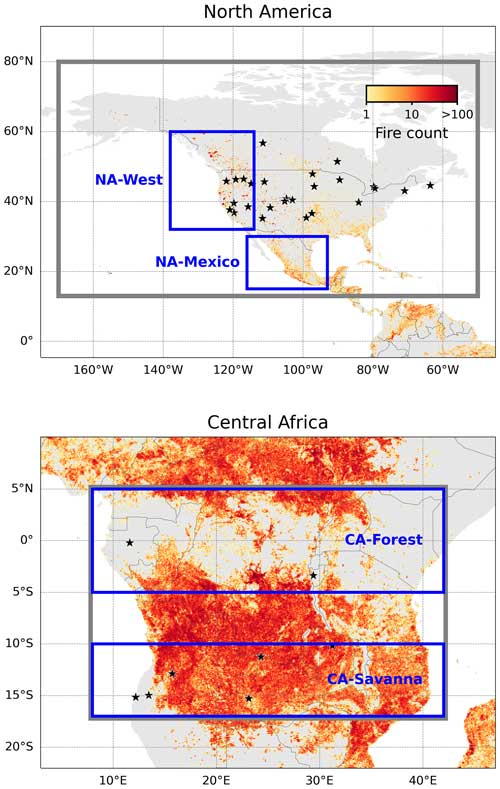
Figure 2Cumulative fire detection counts from VIIRS within a 0.1° by 0.1° latitude–longitude grid in 2018 over North America and central Africa. The study regions are denoted by gray rectangles, and AERONET locations are marked with black stars. Subregions, including western North America (NA-West) and Mexico (NA-Mexico) in North America, as well as the tropical forest (CA-Forest) and savanna (CA-Savanna) regions in central Africa, are denoted by blue rectangles.
2.4 AERONET
In order to evaluate the EPIC-retrieved AOD and spectral absorption, we utilized the version 3 level 2.0 AERONET Inversion dataset (Holben et al., 1998; Dubovik and King, 2000; Giles et al., 2019; Sinyuk et al., 2020). The EPIC-retrieved AOD443, SSA443, and SSA680 were compared with the AERONET counterpart derived from direct- and sky-radiance measurements. The AERONET measurements of spectral AOD have an accuracy of ∼ 0.01 to 0.02 at an optical air mass of 1, with higher uncertainty in the UV (Eck et al., 1999). The AERONET-retrieved SSA values at 440 nm have an uncertainty of ∼ 0.03 at AOD(440) = 0.4, with smaller uncertainties at larger AOD, decreasing to ∼ 0.015 at AOD(440) = 1.3 for biomass-burning aerosols at the Mongu, Zambia, site (Sinyuk et al., 2020). Spatiotemporal collocation between AERONET and EPIC measurements was conducted as follows: (1) averaging AERONET AOD within a ±30 min range and averaging SSA within a ±3 h range from the EPIC measurement time and (2) averaging EPIC 5 × 5 pixels (∼ 50 × 50 km2) collocated with the AERONET sites and limited to cosines of solar zenith angle and viewing zenith angle above 0.45 (i.e., solar zenith angle and viewing zenith angle < 63.3°). The EPIC pixels were spatially averaged when at least 50 % of the EPIC smoke products are valid in the spatial window. AERONET retrievals with an extinction Ångström exponent between 440 and 675 nm greater than 0.4 were selected to avoid possible dust contamination. SSA validation was conducted only when AERONET AOD at 440 nm was greater than 0.6. The AERONET sites with at least five measurements available were considered. Consequently, a total of 28 and 8 AERONET sites were chosen over North America and central Africa, respectively (see Fig. 2).
2.5 CALIOP
The Cloud-Aerosol Lidar with Orthogonal Polarization (CALIOP) on board the Cloud-Aerosol Lidar and Infrared Pathfinder Satellite Observations (CALIPSO) satellite has provided global measurements of aerosol vertical distribution. We collected profiles of total attenuated backscatter coefficients at 532 nm (β; units of km−1 sr−1) from the CALIPSO Lidar Level 2 Aerosol Profile version 4.51 dataset (CAL_LID_L2_05kmAPro-Standard-V4-51) in 2018. Subsequently, we calculated the backscatter-weighted aerosol layer height using the formula , where z represents the height of each layer. This definition is widely employed for validating aerosol layer height using CALIOP (Go et al., 2020; Xu et al., 2019). The ALHCALIOP data within a ±30 min range from EPIC acquisitions were spatially averaged within MAIAC EPIC grid. We used the same cutoff threshold for the Sun and viewing zenith angle as above. To mitigate the ALH uncertainty for weak-aerosol cases, the ALH comparison was conducted when CALIOP AOD at 532 nm exceeded 0.6.
3.1 Analysis of individual cases
3.1.1 North America
Western North America stands out as one of the most active wildfire regions globally. For our analysis, we selected an intense wildfire and associated smoke aerosol event occurring on 18 July 2017 at 20:52:19 coordinated universal time (UTC) over western Canada in Fig. 3. Note that all other analyses in this study are for 2018, except for this case. Utilizing the VIIRS/NPP Thermal Anomalies/Fire (Schroeder and Giglio, 2018), visualized as red dots within the true-color images, we identified wildfires in British Columbia. The true-color image and retrieved smoke particle properties illustrate the eastward transport of the smoke plume. Specifically, pixels near the wildfires (region “A” in Fig. 3) exhibited AOD443 nearing ∼ 4–6, alongside an SSA443 of ∼ 0.93. Pixels approximately 50∼ 100 km from the sources (region “B”) show decreased AOD443 (∼ 2) and less absorption (SSA443 of ∼ 0.96). Notably, the contrast in SSA is more pronounced at 388 nm than at 680 nm (not shown). Absorption changes within this distance are related to the aging process. Freshly emitted particles from wildfires exist in various mixing states and undergo multiple processes, such as coagulation, condensation/evaporation, oxidation, and secondary aerosol particles formed from chemical production (Reid et al., 2005a, b; Liu et al., 2020). Smoke aerosol mixtures become less absorbing in the UV- and shortwave-visible wavelengths when transported from sources through these aging processes, consistent with findings from other in situ and remote-sensing measurement studies (Junghenn Noyes et al., 2020a, b; Kleinman et al., 2020). The increased SSA443 from 0.93 to 0.96 (from regions A to B) corresponds to a decrease in the BrC fraction from 0.3 to 0.1. Aerosol plumes over Alberta, farther downwind to the east (region “C”), exhibited (a) high-AOD443 values (1–3), (b) SSA443 of ∼ 0.92–0.94, (c) increased BC volume fraction up to 0.01; and d) a similar BrC volume fraction (about 0.3 at the plume center) for pixels close to the fire sources. The eastern part of the plumes was located farther away from the source and could have undergone more extensive aging. Smoke aerosol near sources was located close to the surface (ALH above sea level of ∼ 1 km) and was elevated to about 5–6 km in the downwind area. It is important to consider that the fires could also undergo various stages of combustion intensity over time, which could also be a factor in BC and BrC production. The observed differences in ALH suggest that possibly some of these fires were more intense earlier, leading to the lofting of the plume to 5–6 km. Subsequently, the intensity may have decreased, resulting in a lower ALH as the plume transitioned to a more smoldering phase. This scenario, particularly applicable to long plume lengths, implies that fire intensity and the relative combustion fraction (flaming/smoldering) likely varied over the course of several hours during the transport of such a long plume distance.
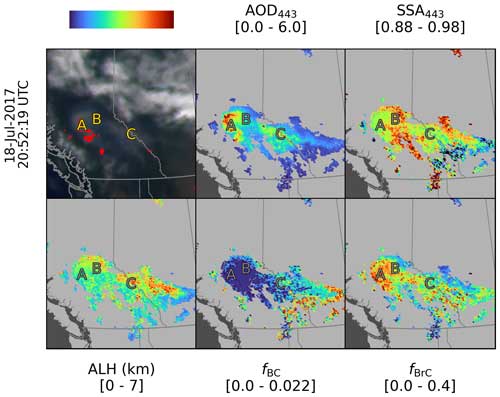
Figure 3Illustration of EPIC smoke aerosol optical properties over western North America on 18 July 2017. Red dots in the top-left panel are VIIRS/NPP thermal anomaly hotspots. The underlying image and analyses in subsequent panels correspond to EPIC true-color and MAIAC EPIC retrievals (AOD443, SSA443, and ALH) with inferred BC and BrC volume fractions. The color bar scale is indicated at the top of each panel.
Continental-scale smoke aerosol episodes in August 2018, derived from the analysis of Lyapustin et al. (2021b), are depicted in Fig. 4. On 13 August (top panels), smoke aerosol plumes along the west coast of North America, near the detected wildfire sources, exhibit a high AOD of nearly 3–4 and SSA443 of 0.93 in the plume center. Surrounding pixels of the plume generally show lower AOD and higher SSA than the pixels interior to the plume. Subsequently, westerly transported plumes with increased AOD (up to ∼ 6) and ALH (∼ 6–7 km) were detected on 16 and 17 August. Corresponding BC and BrC fractions ranged from 0.005 to 0.01 and 0.2 to 0.3 (not shown), with column mass concentrations reaching 15 and 250 mg m−2, respectively.
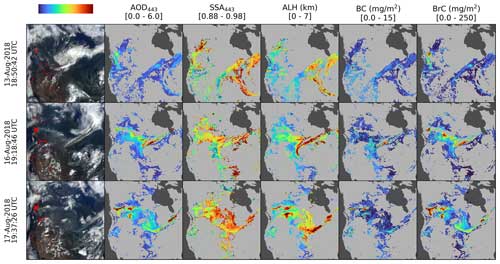
Figure 4Illustration of smoke aerosol optical properties (AOD443, SSA443, ALH, and BC and BrC mass concentrations) over North America on 13, 16, and 17 August 2018. The color bar scale is indicated at the top of each panel.
EPIC can effectively monitor the regional- to continental-scale variability in smoke optical properties at high temporal cadence. Meridional averages of AOD443, SSA443, ALH, and BC and BrC mass concentrations over the period from 13 to 17 August 2018 are represented as Hovmöller diagrams in Fig. 5. Plume evolution is clearly captured, with a temporal resolution of 1–2 h, from the initial smoke aerosol emission over western North America and the subsequent transport toward the east, with an increased ALH from ∼ 1 to 6–7 km, and eventually to dispersion.
3.1.2 Central Africa
Biomass burning over central Africa generates smoke aerosols with distinct optical properties. Long-term AERONET measurements over southern Africa savanna regions indicate the strongest absorption among global smoke regions, with SSA values at 440 and 680 nm of 0.87 and 0.86, respectively (Dubovik et al., 2002; Giles et al., 2012; Sayer et al., 2014). The biomass-burning emission pattern in Africa follows a clearly defined seasonal cycle, influenced by precipitation linked to the seasonal movement of the Intertropical Convergence Zone (ITCZ) (Swap et al., 2003). A strong temporal cycle of SSA exists there as well, with the lowest SSA values in June due to savanna burning, and increases through October as more forested areas burn (Eck et al., 2013). And yet, particle size distributions tend to remain unchanged (Reid et al., 2005b; Sayer et al., 2014). This makes the region an ideal test environment for absorption retrievals. We selected four cases (8 June, 26 July, 14 August, and 19 September 2018) to illustrate the seasonal changes in smoke regions from northeast to southwest; these align closely with the climatological patterns detected by other ground-based and satellite measurements (Eck et al., 2013; Duncan et al., 2003). The detected fires were subcontinent-wide (Fig. 6) and generated smoke with AOD reaching up to ∼ 2. The general particle properties were consistent across the four cases. The light absorption, corresponding to SSA443 as low as ∼ 0.84, was notably stronger than for the cases over North America. The ALH of pixels with high AOD remained relatively constant at 2–3 km. High-BC concentrations (e.g., > 5 mg m−2) were prevalent over the detected fire locations, despite a relatively lower-AOD condition (e.g., AOD443 < 2) than in the cases over North America, where similar BC concentrations were observed from the pixels with AOD443 ≳ 3.
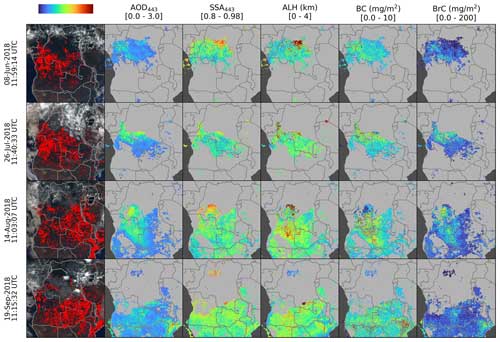
Figure 6Illustration of smoke optical properties (AOD443, SSA443, ALH, and BC and BrC mass concentrations) over central Africa on 8 June, 26 July, 14 August, and 19 September 2018.
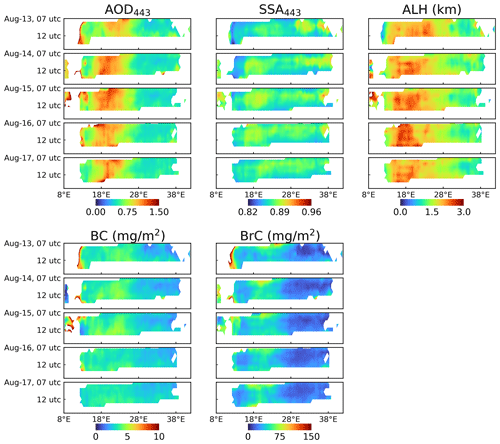
Figure 7Same with Fig. 5 except for the area over central Africa (5° N–17° S, 8–42° E; 0.5° longitudinal interval) from 13 to 17 August 2018.
The measurements taken over 5 consecutive days from 13–17 August over the central Africa study region detected weaker zonal smoke plume transport with less dynamic changes in particle properties (Fig. 7) compared to the North American cases (Fig. 5). The relatively low ALH of 2–3 km indicates that smoke aerosol mostly concentrated within the boundary layer and was less influenced by strong jets at higher altitudes. AOD was slightly enhanced during early morning and late afternoon by ∼ 10 %–20 % over the 20–25° E region. The afternoon pattern is consistent with long-term AERONET measurements shown in Eck et al. (2003), whereas the morning pattern should be further analyzed. From SEVIRI (Spinning Enhanced Visible and Infrared Imager) measurements, the peak of active fires is most frequently detected around noon (Wooster et al., 2021). Eck et al. (2003) concluded that elevated air temperatures, reduced relative humidity, and heightened wind speeds during the midday and afternoon periods often lead to more intense and rapidly spreading fires.
The observed difference between the two regions clearly correlates with the different fuel types, i.e., forests in North America and savannah grasses and bushes in central Africa. For instance, forest wildfires in North America with much higher thermal energy density result in elevated ALH, incomplete combustion, and higher-BrC concentrations, whereas fast-spreading grassland fires are known for high-BC concentration from flaming combustion emissions but lower-energy density, which keep generated smoke generally within the boundary layer over central Africa.
High fuel consumption can explain higher ALH from North America with more thermal energy. Fuel consumption is defined as the amount of biomass, coarse and fine litter, and soil organic matter consumed per unit area burned. It is the product of fuel load and combustion completeness, leading to regional differences. For instance, regions in the western USA, Canada, and Siberia categorized as boreal forests exhibit high fuel consumption (e.g., > 2 kg C m−2 burned), whereas the savanna region in central Africa has lower fuel consumption (e.g., 1−2 kg C m−2 burned; van der Werf et al., 2017). The energy released along the flame front is directly related to plume height, with plumes from these fires reaching altitudes between 2.2 and 13 km (Lavoué et al., 2000). MODIS-derived fire radiative power also shows significant differences between smoke plumes in the free troposphere (1620–1640 MW) and those within the boundary layer (174–465 MW; Val Martin et al., 2010).
3.2 Comparison of smoke properties derived from AERONET and CALIOP
The regional validation of AOD, spectral SSA, and ALH throughout 2018, using the AERONET and CALIOP datasets, is presented in Fig. 8. The AOD comparison over North America demonstrates a correlation coefficient (R) of 0.91 and a root mean squared error (RMSE) of 0.22. It is important to note that this comparison only covers smoke retrievals; it excludes low-AOD conditions (e.g., background AOD at 443 nm < 0.4) that may result in lower validation statistics compared to the previous analysis incorporating the combined “background and smoke” AOD (R of 0.85 and RMSE of 0.13 in Lyapustin et al., 2021b). Nonetheless, the mean bias error (MBE) of 0.02 in version 3 is smaller than the 0.05 reported by Lyapustin et al. (2021b), based on v2. In total, 74.9 % of results fall within the expected error envelope for AOD, defined as from AERONET AOD. Hereafter, “EE” refers to a percentage of retrievals within the expected error envelope. Central Africa AOD also exhibits similar validation statistics, except for a lower R (0.60), likely due to a narrow range of collocated AOD compared to North America. However, the MBE of −0.04 and EE of 74.8 % are comparable to the statistics for North America. Despite the absence of IR channels for cloud detection and the relatively coarse spatial resolution (> 10 km) of EPIC, which can lead to sub-pixel cloud contamination (Marshak et al., 2018), the achieved accuracy in AOD retrieval is very encouraging.
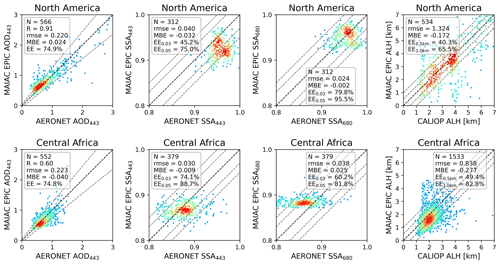
Figure 8Comparison of MAIAC EPIC smoke AOD443 (first column), SSA443 (second column), SSA680 (third column) with AERONET, and ALH with CALIOP (fourth column). Color represents the relative frequency of retrievals. The dashed black lines are the 1 : 1 reference line. The dashed gray lines are the ranges of expected error envelopes, with ) from AERONET AOD, ±0.03 or ±0.05 from AERONET SSA, and ±0.5 km or ±1.0 km from CALIOP ALH.
Regional comparisons of SSA with AERONET retrievals are more distinct than those of AOD. Overall, the SSA443 over North America from EPIC is lower than that from AERONET, with MBE of −0.03 and EE0.03 for SSA, and defined as a percentage of retrievals within ±0.03 from AERONET SSA, which is 45.2 %. The collocated range spans from about 0.88 to 0.97 from EPIC and 0.90–1.00 from AERONET. Comparisons over central Africa show a much smaller bias (MBE of −0.01) and higher EE0.03 of 74.1 %. The regional difference in accuracy could be attributed to uncertainty in our assumptions of regional smoke model properties (e.g., particle size and real refractive index). Nonetheless, the retrieved MAIAC EPIC SSA443 remains comparable to the Ozone Monitoring Instrument (OMI) Aerosol near-UV (OMAERUV) SSA440 retrievals (RMSE of 0.04 and EE0.03 of 57.5 % over North America; RMSE of 0.04 and EE0.03 of 66.4 % over South America and southern Africa in Jethva et al., 2014) and the TROPOMI aerosol algorithm (TropOMAER) SSA440 retrievals (RMSE from 0.04 to 0.04; EE0.03 from 48 to 51 % in Torres et al., 2020). Additionally, it is worth noting that the current AERONET algorithm has a strong spectral smoothness constraint for the imaginary part of refractive indices, resulting in less representation of BrC (Sinyuk et al., 2022; Eck et al., 2023). By employing the relaxed constraint, they found decreased SSA (e.g., more absorbing) with a smaller sky-radiance error from wildfire cases containing a large amount of BrC. However for the biomass-burning cases shown in Sinyuk et al. (2022) for both North American wildfire smoke and savanna-burning smoke in Zambia, the difference in spectral SSA at 443 nm were ∼ 0.01 or less for the relaxed versus standard V3 constraints, while some differences in SSA at 675 nm were ∼ 0.02 for North American smoke only. With this update from the AERONET side, we anticipate a potentially better agreement between EPIC and AERONET for SSA443 and a possibly better agreement for SSA680 in the future.
SSA680 retrievals from North America show better agreement with AERONET than SSA443, with a smaller MBE of −0.002, RMSE of 0.02, and a higher EE0.03 (79.8 %). However, central Africa shows slightly less agreement in SSA680 compared to SSA443, with a higher positive bias (MBE of 0.03) and smaller EE0.03 of 60.2 %. Additionally, the retrieved range of SSA680 is relatively narrower (∼ 0.87 to 0.92) than that of AERONET (∼ 0.80 to 0.99). Regardless, the statistical metrics are much closer to POLDER GRASP SSA680 retrievals (RMSE of 0.06; MBE from −0.04 to −0.02 in Chen et al., 2020).
The comparison of EPIC ALH with CALIOP also reveals strong regional dependence. Most collocated ALH retrievals are relatively high over North America (3–4 km) and sometimes reach 6–7 km. In central Africa, ALH ranges from 0 to 4–5 km, with most collocated retrievals falling within 1–3 km. The RMSE value is closely related to the range of ALH; thus, it is relatively high in North America (1.32 km). More favorable validation statistics were extracted from central Africa (RMSE of 0.84 km; EE0.5 km of 49.4 %; MBE of −0.28 km), where EE0.5 km is a percentage of retrievals within a range of ±0.5 km from CALIOP ALH. This level of accuracy, derived from a long-term validation rather than selected individual cases, is better than the operational TROPOMI ALH (MBE from −2.41 to −1.03 km and RMSE from 1.97 to 3.56 km in Nanda et al., 2020).
3.3 Regional climatology of smoke properties
We compiled all the smoke properties retrieved for 2018 and conducted a regional analysis to understand their climatology and relationships with environmental factors such as vegetation and fuel type, as well as meteorological conditions. Regional geographical distributions are illustrated in Fig. 9, and the corresponding statistical distributions are presented as box-and-whisker plots in Fig. 10.
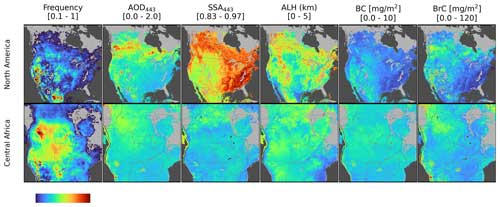
Figure 9Spatial distribution of relative retrieval frequency (i.e., relative number of retrievals) and smoke properties (AOD443, SSA443, ALH, and BC and BrC mass concentrations) for 2018 over North America (top panels) and central Africa (bottom panels). Pixels with retrieval frequencies lower than 10 % compared to the regional maximum are filtered out. The color bar scale is indicated at the top of each panel.
Active wildfires occur in late spring to fall over western North America, with expanded burned areas over the years (Dennison et al., 2014; Kalashnikova et al., 2018; Liu et al., 2010). Most smoke retrievals were detected over the western United States (e.g., California, Oregon, and Washington) and western Canada (e.g., British Columbia) (Fig. 9). The optical properties were quite distinct between source regions and downwind regions. The western USA and western Canada source regions show relatively low SSA and ALH, while central Canada, which is a source region but has mostly downwind regions for transported heavy smoke plume from western regions, also shows higher SSA and ALH. This difference is closely related to the smoke aging process discussed in Sect. 3.1.1. Spatiotemporally integrated spectral SSA over western North America (NA-West region in Fig. 2) of 0.86, 0.89, 0.92, 0.94, and 0.95 at 340, 388, 443, 554, and 680 nm, respectively, align with the range 0.915–0.935 at 443 nm and 0.95–0.97 at 680 nm derived from multiple AERONET measurements in September 2020 (Eck et al., 2023). The mean and standard deviation of ALH was 2.2 ± 1.2 km, with a wide range of values up to 4.6 km at the 95th percentile (Fig. 10b). The mean BC volume fraction of 0.011 ± 0.006 was the lowest among the selected regions. The number of smoke pixels was maximum in August, with the highest-BrC mass concentration (median value of 29 mg m−2) synchronized with seasonal wildfire activities over western North America. Although BC and BrC concentrations can reach up to more than 5 and 100 mg m−2, respectively, over some specific regions (Fig. 9), the averaged values were not as high due to high spatiotemporal variation. Another smoke-dominated region in North America is found over Mexico (NA-Mexico region in Fig. 2), where both natural wildfires and agricultural burns occur annually during the hot and dry season (March to May; Rios et al., 2023). This region exhibited smoke properties with more absorption and lower ALH with lower variation (1.6 ± 0.9 km) than western USA.
Central Africa is climatologically the largest global biomass-burning source, peaking during the austral winter. The region contributes approximately one-third of Earth's biomass-burning emissions from various sources, including wildfires, agricultural fires, and industrial activities (van der Werf et al., 2010). The distribution of smoke retrievals appears relatively homogeneous and similar to that of detected fires, with widespread retrieval frequency in Angola, the Democratic Republic of the Congo, and Zambia and more varied sources in Namibia (Fig. 9). During the August–October burning season in central Africa, aerosol light absorption is predominantly attributed to BC, a byproduct of savanna burning characterized by significant flaming-phase combustion (Ward et al., 1996). Although the retrieved smoke AOD is not as high as in North America, light absorption over savanna region in central Africa (CA-Savanna region in Fig. 2) was more substantial, leading to higher-BC and higher-BrC mass concentrations. Low SSA spanned from UV through the visible range (0.84, 0.86, 0.88, 0.89, and 0.89 at 340, 388, 443, 554, and 680 nm, respectively), with higher-BC and higher-BrC volume fractions of 0.015 and 0.178, respectively. The ALH is lower and has less variance (1.8 ± 0.6 km; 2.6 km for the 95th percentile) than that of western North America. The BC and BrC mass concentrations increased from July, peaked in September (median values of 3.3 and 28.4 mg m−2, respectively), and declined toward November; this aligns with long-term AERONET AOD measurements (Eck et al., 2003) and with AERONET-based BC and BrC estimations (Schuster et al., 2016). In contrast, smoke from tropical forest fires in central Africa (CA-Forest region in Fig. 2) shows slightly less absorption, with a lower-BC volume fraction (0.013) and larger variabilities in the BrC volume fraction (0.018 ± 0.11) and ALH (1.9 ± 1.1 km) than that of the savanna region. BC and BrC mass concentrations over the tropical forest region in central Africa peak in July (earlier than savanna region), with lower-BC (2.8 mg m−2) and higher-BrC (37.8 mg m−2) values than those of the savanna region.
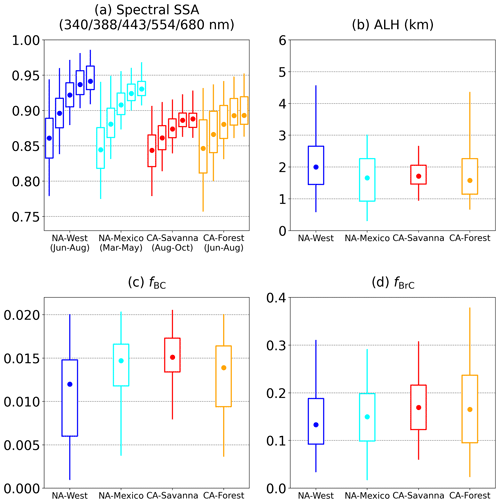
Figure 10Distribution of (a) spectral SSA, (b) ALH, (c) BC volume fraction, and (d) BrC volume fraction over western North America (NA-West) and Mexico (NA-Mexico) in North America and the savanna (CA-Savanna) and tropical forest (CA-Forest) in central Africa. Whiskers give the 5th and 95th percentiles, boxes represent the 25th and 75th percentiles, and dots denote the 50th percentile. In panel (a), five consecutive box-and-whisker plots for each region represent different wavelengths (i.e., 340, 388, 443, 554, and 680 nm from left to right).
4.1 Comparison of the BrC BC mass concentration ratio with other studies
The ratio between OC and elemental carbon (EC) (OC EC) is widely used to elucidate the apportionment of carbonaceous components in smoke particles as a proxy for assessing the dominance of primary emissions from flaming combustion (e.g., fossil fuel) versus smoldering combustion emissions and the secondary formation of OC (e.g., biomass burning, wildfires, and secondary organic aerosol (SOA) formation) (Lim and Turpin, 2002; Pokhrel et al., 2016). As BrC is an absorbing OC among total OC, we inferred regional BrC BC column mass concentration ratios (BrC BC) from EPIC and compared them with those from other studies providing BrC BC or OC EC.
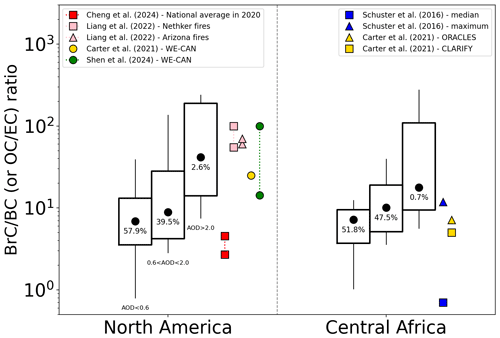
Figure 11Regional EPIC-derived BrC BC column mass concentration ratios across three AOD ranges (AOD < 0.6, 0.6 < AOD < 2.0, and AOD > 2.0). Each box-and-whisker plot comprises the range of percentiles (5, 15.9, 50, 84.1, and 95). The percentages of retrievals for each AOD range are denoted within the box. On the right-hand side of each region panel, the values (or range) of the BrC BC ratio (only for Schuster et al., 2016) or OC BC ratio (all others) from other studies are shown.
Results of the BrC BC ratio from this study in North America and central Africa are compared with other previous studies in Fig. 11. The absolute BC and BrC volume fractions in central Africa were higher than in North America, resulting in similar median values of the BrC BC mass concentration ratio (7.3 for North America and 8.0 for central Africa). When the ratios are categorized into different AOD ranges, the BrC BC increases with AOD from both regions. For two groups of AOD < 0.6 (low–moderate AOD) and 0.6 < AOD < 2.0 (high AOD), the median BrC BC is higher in central Africa (7.2 and 10.1) than in North America (6.9 and 8.9). The variance, represented as the range of estimations, is more significant in North America for the two groups, which could be ascribed to more diverse fuel types from natural, residential, and agricultural sources and related emission processes (Xiong et al., 2022). For the cases of AOD > 2.0 (extremely high AOD), which corresponds to 2.6 % and 0.7 % of the entire retrieval record in North America and central Africa, respectively, North America showed a higher BrC BC ratio (median value of 41.5) with a higher variance than central Africa (median value of 17.7). This higher BrC BC ratio in North America, compared to central Africa, may have its origin in more common smoldering combustion and/or more SOA formation during transport. Most extremely high-AOD cases were observed from transport plumes, where the increased BrC BC ratio is associated with their aging processes, including SOA formation. These results are consistent with POLDER/GRASP and MISR aerosol component analyses (Li et al., 2022; Junghenn Noyes et al., 2022).
Our estimates exhibit relatively high variance because they encompassed all pixels detected as smoke in the retrieval algorithm over the continents in 2018, rather than being limited to selected heavy plumes. The national average of the OC EC ratio (3.6 ± 0.9) obtained from the United States Environmental Protection Agency (U.S. EPA) ground-based chemical composition measurement networks (including the Chemical Speciation Network (CSN) and the Interagency Monitoring of Protected Visual Environments (IMPROVE)) for all sources, and not only for smoke sources (Cheng et al., 2024), falls within the estimates from EPIC's low–moderate AOD group. OC EC ratios obtained from specific wildfire samples including the WE-CAN (Western wildfire Experiment for Cloud chemistry, Aerosol absorption and Nitrogen) campaign during 2018 July–September over the western USA (Liang et al., 2022; Carter et al., 2021) range from approximately 14 to 100, corresponding to the extremely high-AOD group. It is important to note that although the BrC BC ratio is smaller than the OC EC ratio, obtaining an accurate BrC BC ratio is challenging without proper measurements separating BrC from OC, which is rarely done in experiments.
The ObseRvations of Aerosols above CLouds and their intEractionS (ORACLES; August–September 2016) and CLoud–Aerosol–Radiation Interaction and Forcing: Year 2017 (CLARIFY-2017, August) campaigns over the eastern South Atlantic Ocean (Carter et al., 2021) measured transported smoke aerosols from central Africa. The general level of AOD at 550 nm for both campaigns was ∼ 0.3 to ∼ 0.7 (Haywood et al., 2021; Sayer et al., 2019), and corresponding OC EC ratios were 5–7, which are consistent with the estimated EPIC ranges for low–moderate AOD and high AOD. Another comparison can be made with the BrC BC mass concentration ratio inferred from AERONET measurements (Schuster et al., 2016). Although the definition is similar to ours, using column-integrated and remote-sensing-based values, it shows relatively lower values than ours. This difference could be attributable to the different wavelengths (i.e., UV-Vis for EPIC and Vis-NIR for AERONET) used for the measurements and different assumptions in the components (e.g., dependence of composition on particle size in Schuster et al., 2016).
The EPIC BrC BC ratios increased with AOD, representing aging processes during transport over North America and central Africa. They are generally consistent with other studies despite different measurement characteristics, such as OC EC vs. BrC BC, and in situ versus remote sensing.
4.2 Uncertainty in the volume fractions due to assumed BC and BrC refractive indices
Assumed spectral imaginary refractive indices of BC and BrC determine their inferred volume fractions. Identical spectral absorption can result in lower-BC and lower-BrC fractions with higher-BC and higher-BrC imaginary refractive indices, and vice versa. As most satellite measurements, including EPIC, lack the sensitivity to infer both the imaginary refractive indices of inclusions and their volume fractions, we must assume the imaginary refractive indices of inclusions to infer their volume fractions. Here, we investigate the effect of this assumption on the inferred volume fractions and assess the resulting uncertainties.
A total of three different BC datasets were considered (Fig. 12a). BC1, which we used, and BC2 were derived from multiple measurements combined with the assumption that light-absorbing carbon has a single refractive index and that the variation can be expressed by the Bruggeman effective-medium theory (Bond and Bergstrom, 2006). BC3, utilized in aerosol modeling for the Airborne Multi-angle SpectroPolarimeter Imager (AirMSPI) analysis (Kalashnikova et al., 2018), was originally referred to as the “soot” component of the Optical Properties of Aerosols and Clouds (OPAC) dataset described in Hess et al. (1998). The value of k is between 0.4 and 0.8 and is spectrally invariant or nearly invariant.
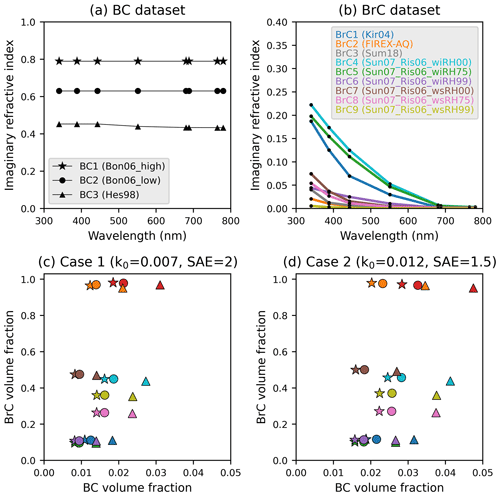
Figure 12Spectral imaginary refractive indices of (a) BC and (b) BrC. Range of BC and BrC volume fractions for (c) Case 1 (k0 of 0.007 and SAE of 2) and (d) Case 2 (k0 of 0.012 and SAE of 1.5). The star, circle, and triangle symbols in panels (c) and (d) refer to different BC assumptions of BC1, BC2, and BC3 in panel (a). The different colors in panels (c) and (d) refer to different BrC assumptions from BrC1 to BrC9 in panel (b).
We tested nine different BrC datasets (Fig. 12b). BrC1, which we used, was derived from organic carbon extracted from wood burning and Southern African Regional Science Initiative (SAFARI 2000) biomass smoke samples, as described in Kirchstetter et al. (2004). BrC2 is an AirMSPI-retrieved value during the Fire Influence on Regional to Global Environments and Air Quality (FIREX-AQ) campaign (Olga Kalashnikova, personal communication, 19 May 2020). BrC3 represents aerosols emitted from the smoldering combustion of boreal and Indonesian peatlands (Sumlin et al., 2018). BrC4, BrC5, and BrC6 represent water-insoluble BrC with a relative humidity of 0 %, 75 %, and 99 %, respectively, calculated by combining the upper curve of Sun et al. (2007) and hygroscopic properties in Rissler et al. (2006). BrC7, BrC8, and BrC9 are the same but represent water-soluble BrC. These datasets were obtained from the Table of Aerosol Optics (TAO) dataset within the framework of the Models, In situ, and Remote sensing of Aerosols (MIRA) working group projects (https://science.larc.nasa.gov/mira-wg/, last access: 5 September 2024).
Here, two smoke cases were analyzed: Case 1 (k0 of 0.007 and SAE of 2) and Case 2 (k0 of 0.012 and SAE of 1.5), representing the most populated EPIC retrievals in the AERONET validation over North America and central Africa, respectively. For Case 1, the fBC and fBrC, based on our current assumptions, are 0.011 and 0.112, respectively (marked with a star marker in dark blue in Fig. 12c). With different assumptions for inclusion properties, they have a range of 0.008–0.031 and 0.096–0.982, respectively. Less absorbing BC assumptions (i.e., smaller k) result in increased fBC to 0.012 (BC2 and BrC1) and 0.018 (BC3 and BrC2). The maximum difference for fBC is 0.013, with the lowest absorption in BrC (BrC9). The potential fBrC values exhibit greater variability. The fBrC value with the current assumption (0.112) is one of the lowest values among tested combinations and similar to those from BrC2 and BrC3, which have stronger absorption than others. The BrC assumptions with less absorbing properties show higher fBrC from 0.264 to 0.981. We also tested the spectral k for dark BrC obtained from the FIREX-AQ campaign in the western USA (Chakrabarty et al., 2023). They showed an estimated fBC close to zero because of the relatively high k of 0.1 at 680 nm. Case 2 is converted to higher fBC (0.019) and similar fBrC (0.117) when compared to Case 1 with the default assumption. The range of fBC and fBrC from the different combinations is 0.016–0.047 and 0.101–0.980, respectively. It is essential to acknowledge that inferring volume fractions and mass concentrations is based on assumed inclusion properties, which introduces some uncertainties. The assumed properties of BC and BrC will need to be refined in future studies (e.g., a suggested concept in Kahn et al., 2017) to enhance the accuracy of our findings.
This study introduced a technique for inferring the BC and BrC light-absorbing components of smoke aerosol by leveraging the spectral absorption retrieved in the MAIAC EPIC algorithm. Spectral absorption retrievals allowed us to quantify the BC and BrC fractions, which were then converted to column-integrated mass concentrations assuming the particle mass extinction efficiency. We assumed that BC and BrC are internally mixed, with a non-absorbing host representing non-absorbing OC, sulfate, nitrate, or ammonium components, using the MG-EMA.
We analyzed regional characteristics over North America and central Africa in 2018, utilizing all available MAIAC EPIC smoke property retrievals (AOD, spectral SSA, ALH, and BC and BrC volume fractions and mass concentrations). Selected cases showed that smoke aerosols emitted from wildfires over western North America exhibited extremely high AOD up to ∼ 6 with elevated ALH (6–7 km). Dynamic changes in spectral absorption and significant BrC components were observed during continental-scale transport. The MAIAC EPIC products successfully monitored the transport and evolution of smoke optical properties with a high temporal resolution during regional- to continental-scale transport. Biomass-burning smoke over central Africa displayed higher absorption with greater BC and BrC fractions than North America, showing seasonal changes in major source locations. They also showed less strong zonal transport with ALH closer to the surface and diurnal change in smoke amounts related to fire activities.
EPIC-retrieved AOD443, SSA443, SSA680, and ALH agreed with the collocated AERONET and CALIOP measurements, with RMSE values of 0.2, 0.03–0.04, 0.02–0.04, and 0.8–1.3 km, respectively, and the overall accuracies were comparable to other operational satellite products such as OMI, TROPOMI, and POLDER. Spatiotemporal integration of measurements revealed geographical characteristics and distinct differences in optical properties, ALH, and inferred BC and BrC that are closely linked to burning types and meteorological conditions. Smoke from forest fires in western North America shows SSA443 of 0.92, with a low-BC volume fraction of 0.011 and high ALH with larger standard deviation (2.2 ± 1.2 km). The wildfires and agricultural fires over the Mexico region generated smoke with more absorption and lower ALH. The savanna region in central Africa from August to October shows smoke properties with most absorbing with high-BC and high-BrC volume fractions (0.015 and 0.178, respectively) and lower ALH with a smaller variation. Smoke from tropical forests in central Africa exhibits absorption between that of western USA and savanna regions and high-ALH variability. The impact of assumed imaginary refractive indices of BC and BrC in estimating their volume fractions was analyzed based on a survey of the literature that presented the corresponding uncertainty ranges of our retrievals.
Although we focused on North America and central Africa, smoke aerosols have a significant impact on air quality and climate globally. Future studies will extend the analysis to other regions using almost a decade of EPIC measurements since 2015, with extensive validation and error analysis using multiple measurements, including AERONET, CALIOP, and in situ aerosol composition data.
The MAIAC EPIC smoke aerosol components presented here could serve as valuable a priori information for recent and upcoming satellite missions such as the Plankton, Aerosol, Cloud, ocean Ecosystem (PACE; https://pace.gsfc.nasa.gov/, last access: 5 September 2024) (Remer et al., 2019a, b); the Multi-Angle Imager for Aerosols (MAIA; https://maia.jpl.nasa.gov/, last access: 5 September 2024) (Diner et al., 2018); and EPS-SG Multi-Viewing Multi-Channel Multi-Polarization Imaging (3MI) (Fougnie et al., 2018) and Atmosphere Observing System (AOS; https://aos.gsfc.nasa.gov/, last access: 5 September 2024), focusing on retrieving aerosol microphysical and optical properties, and inferring chemical composition, with higher accuracy from multi-angle polarization measurements. Integration of our results with other in situ and remote-sensing measurements and models (e.g., Kahn et al., 2023) should enhance our understanding of smoke aerosol aging processes, improve air quality monitoring and forecasting, and refine the quantification of radiative forcing due to smoke aerosols on a global scale.
The retrievals can be requested directly from the corresponding author (myungje.choi@nasa.gov) or Alexei Lyapustin (alexei.i.lyapustin@nasa.gov).
MyC and AL designed the study with discussions with GLS and SG. GLS provided major guidance on developing the BC and BrC estimation algorithms. AL and YW provided the MAIAC EPIC products. AL and SK conducted RT calculations (LUTs for MAIAC). MyC, AL, YW, and SG developed the code and performed the retrievals. GLS and OK participated in the collection of refractive indices data. MyC, AL, GLS, and SG analyzed the results. MyC and AL wrote the paper with comments from all co-authors.
The contact author has declared that none of the authors has any competing interests.
Publisher’s note: Copernicus Publications remains neutral with regard to jurisdictional claims made in the text, published maps, institutional affiliations, or any other geographical representation in this paper. While Copernicus Publications makes every effort to include appropriate place names, the final responsibility lies with the authors.
The authors are grateful to the AERONET team for providing validation data and to the NASA Center for Climate Simulations providing resources for the EPIC data processing. The authors gratefully acknowledge the NASA Langley Research Center for providing the CALIOP data through the Atmospheric Science Data Center.
The work of Alexei Lyapustin, Myungje Choi, Sujung Go, Yujie Wang, and Sergey Korkin has been funded by the NASA DSCOVR program (grant no. 21-DSCOVR-21-0004; manager Richard Eckman) and in part by the NASA PACE program (grant no. 19-PACESAT19-0039). Jeffrey S. Reid and Edward J. Hyer have been funded by the Office of Naval Research (code 322). The work of Hans Moosmüller has been supported in part by the National Science Foundation (grant no. OIA-2148788) and by NASA (grant no. 80NSSC20M0205; PACE SAT Project: PACE UV ROAD). The part of this research was carried out at the Jet Propulsion Laboratory, California Institute of Technology, under contract with the National Aeronautics and Space Administration. Olga Kalashnikova contribution was supported by the NASA DSCOVR grant. The work of Jhoon Kim was supported by a grant from the National Research Foundation of Korea (NRF), funded by the Korean government (MSIT) (grant no. RS-2024-00346149).
This paper was edited by Allison C. Aiken and reviewed by two anonymous referees.
Ahn, C., Torres, O., Jethva, H., Tiruchirapalli, R., and Huang, L.-K.: Evaluation of Aerosol Properties Observed by DSCOVR/EPIC Instrument From the Earth-Sun Lagrange 1 Orbit, J. Geophys. Res.-Atmos., 126, e2020JD033651, https://doi.org/10.1029/2020JD033651, 2021.
Andreae, M. O.: Emission of trace gases and aerosols from biomass burning – an updated assessment, Atmos. Chem. Phys., 19, 8523–8546, https://doi.org/10.5194/acp-19-8523-2019, 2019.
Andreae, M. O. and Gelencsér, A.: Black carbon or brown carbon? The nature of light-absorbing carbonaceous aerosols, Atmos. Chem. Phys., 6, 3131–3148, https://doi.org/10.5194/acp-6-3131-2006, 2006.
Andreae, M. O. and Merlet, P.: Emission of trace gases and aerosols from biomass burning, Global Biogeochem. Cy., 15, 955–966, https://doi.org/10.1029/2000GB001382, 2001.
Bellouin, N., Boucher, O., Haywood, J., and Reddy, M. S.: Global estimate of aerosol direct radiative forcing from satellite measurements, Nature, 438, 1138–1141, https://doi.org/10.1038/nature04348, 2005.
Bhandari, J., China, S., Chandrakar, K. K., Kinney, G., Cantrell, W., Shaw, R. A., Mazzoleni, L. R., Girotto, G., Sharma, N., Gorkowski, K., Gilardoni, S., Decesari, S., Facchini, M. C., Zanca, N., Pavese, G., Esposito, F., Dubey, M. K., Aiken, A. C., Chakrabarty, R. K., Moosmüller, H., Onasch, T. B., Zaveri, R. A., Scarnato, B. V., Fialho, P., and Mazzoleni, C.: Extensive Soot Compaction by Cloud Processing from Laboratory and Field Observations, Sci. Rep., 9, 11824, https://doi.org/10.1038/s41598-019-48143-y, 2019.
Bohren, C. F. and Huffman, D. R.: Absorption and Scattering of Light by Small Particles, Wiley, https://doi.org/10.1002/9783527618156, 1998.
Bond, T. C. and Bergstrom, R. W.: Light Absorption by Carbonaceous Particles: An Investigative Review, Aerosol Sci. Tech., 40, 27–67, https://doi.org/10.1080/02786820500421521, 2006.
Bond, T. C., Doherty, S. J., Fahey, D. W., Forster, P. M., Berntsen, T., Deangelo, B. J., Flanner, M. G., Ghan, S., Kärcher, B., Koch, D., Kinne, S., Kondo, Y., Quinn, P. K., Sarofim, M. C., Schultz, M. G., Schulz, M., Venkataraman, C., Zhang, H., Zhang, S., Bellouin, N., Guttikunda, S. K., Hopke, P. K., Jacobson, M. Z., Kaiser, J. W., Klimont, Z., Lohmann, U., Schwarz, J. P., Shindell, D., Storelvmo, T., Warren, S. G., and Zender, C. S.: Bounding the role of black carbon in the climate system: A scientific assessment, J. Geophys. Res.-Atmos., 118, 5380–5552, https://doi.org/10.1002/jgrd.50171, 2013.
Bond, W. J. and Keeley, J. E.: Fire as a global “herbivore”: the ecology and evolution of flammable ecosystems, Trends Ecol. Evol., 20, 387–94, https://doi.org/10.1016/j.tree.2005.04.025, 2005.
Carter, T. S., Heald, C. L., Cappa, C. D., Kroll, J. H., Campos, T. L., Coe, H., Cotterell, M. I., Davies, N. W., Farmer, D. K., Fox, C., Garofalo, L. A., Hu, L., Langridge, J. M., Levin, E. J. T., Murphy, S. M., Pokhrel, R. P., Shen, Y., Szpek, K., Taylor, J. W., and Wu, H.: Investigating Carbonaceous Aerosol and Its Absorption Properties From Fires in the Western United States (WE-CAN) and Southern Africa (ORACLES and CLARIFY), J. Geophys. Res.-Atmos., 126, 647–650, https://doi.org/10.1029/2021JD034984, 2021.
Chakrabarty, R. K., Moosmüller, H., Chen, L.-W. A., Lewis, K., Arnott, W. P., Mazzoleni, C., Dubey, M. K., Wold, C. E., Hao, W. M., and Kreidenweis, S. M.: Brown carbon in tar balls from smoldering biomass combustion, Atmos. Chem. Phys., 10, 6363–6370, https://doi.org/10.5194/acp-10-6363-2010, 2010.
Chakrabarty, R. K., Shetty, N. J., Thind, A. S., Beeler, P., Sumlin, B. J., Zhang, C., Liu, P., Idrobo, J. C., Adachi, K., Wagner, N. L., Schwarz, J. P., Ahern, A., Sedlacek, A. J., Lambe, A., Daube, C., Lyu, M., Liu, C., Herndon, S., Onasch, T. B., and Mishra, R.: Shortwave absorption by wildfire smoke dominated by dark brown carbon, Nat. Geosci., 16, 683–688, https://doi.org/10.1038/s41561-023-01237-9, 2023.
Chen, C., Dubovik, O., Fuertes, D., Litvinov, P., Lapyonok, T., Lopatin, A., Ducos, F., Derimian, Y., Herman, M., Tanré, D., Remer, L. A., Lyapustin, A., Sayer, A. M., Levy, R. C., Hsu, N. C., Descloitres, J., Li, L., Torres, B., Karol, Y., Herrera, M., Herreras, M., Aspetsberger, M., Wanzenboeck, M., Bindreiter, L., Marth, D., Hangler, A., and Federspiel, C.: Validation of GRASP algorithm product from POLDER/PARASOL data and assessment of multi-angular polarimetry potential for aerosol monitoring, Earth Syst. Sci. Data, 12, 3573–3620, https://doi.org/10.5194/essd-12-3573-2020, 2020.
Cheng, B., Alapaty, K., and Arunachalam, S.: Spatiotemporal trends in PM2.5 chemical composition in the conterminous U.S. during 2006–2020, Atmos. Environ., 316, 120188, https://doi.org/10.1016/j.atmosenv.2023.120188, 2024.
Choi, Y., Ghim, Y. S., Zhang, Y., Park, S. M., and Song, I. H.: Estimation of surface concentrations of black carbon from long-term measurements at aeronet sites over korea, Remote Sens., 12, 1–24, https://doi.org/10.3390/rs12233904, 2020.
Corbin, J. C., Modini, R. L., and Gysel-Beer, M.: Mechanisms of soot-aggregate restructuring and compaction, Aerosol Sci. Tech., 57, 89–111, https://doi.org/10.1080/02786826.2022.2137385, 2023.
Dennison, P. E., Brewer, S. C., Arnold, J. D., and Moritz, M. A.: Large wildfire trends in the western United States, 1984–2011, Geophys. Res. Lett., 41, 2928–2933, https://doi.org/10.1002/2014GL059576, 2014.
Diner, D. J., Boland, S. W., Brauer, M., Bruegge, C., Burke, K. A., Chipman, R., Di Girolamo, L., Garay, M. J., Hasheminassab, S., and Hyer, E.: Advances in multiangle satellite remote sensing of speciated airborne particulate matter and association with adverse health effects: from MISR to MAIA, J. Appl. Remote Sens., 12, 042603, https://doi.org/10.1117/1.JRS.12.042603, 2018.
Dubovik, O. and King, M. D.: A flexible inversion algorithm for retrieval of aerosol optical properties from Sun and sky radiance measurements, J. Geophys. Res.-Atmos., 105, 20673–20696, https://doi.org/10.1029/2000JD900282, 2000.
Dubovik, O., Holben, B., Eck, T. F., Smirnov, A., Kaufman, Y. J., King, M. D., Tanré, D., and Slutsker, I.: Variability of Absorption and Optical Properties of Key Aerosol Types Observed in Worldwide Locations, J. Atmos. Sci., 59, 590–608, https://doi.org/10.1175/1520-0469(2002)059<0590:VOAAOP>2.0.CO;2, 2002.
Dubovik, O., Sinyuk, A., Lapyonok, T., Holben, B. N., Mishchenko, M., Yang, P., Eck, T. F., Volten, H., Muñoz, O., Veihelmann, B., van der Zande, W. J., Leon, J. F., Sorokin, M., and Slutsker, I.: Application of spheroid models to account for aerosol particle nonsphericity in remote sensing of desert dust, J. Geophys. Res.-Atmos., 111, 1–34, https://doi.org/10.1029/2005JD006619, 2006.
Dubovik, O., Herman, M., Holdak, A., Lapyonok, T., Tanré, D., Deuzé, J. L., Ducos, F., Sinyuk, A., and Lopatin, A.: Statistically optimized inversion algorithm for enhanced retrieval of aerosol properties from spectral multi-angle polarimetric satellite observations, Atmos. Meas. Tech., 4, 975–1018, https://doi.org/10.5194/amt-4-975-2011, 2011.
Dubovik, O., Lapyonok, T., Litvinov, P., Herman, M., Fuertes, D., Ducos, F., Torres, B., Derimian, Y., Huang, X., Lopatin, A., Chaikovsky, A., Aspetsberger, M., and Federspiel, C.: GRASP: a versatile algorithm for characterizing the atmosphere, SPIE Newsroom, https://doi.org/10.1117/2.1201408.005558, 2014.
Duncan, B. N., Martin, R. V., Staudt, A. C., Yevich, R., and Logan, J. A.: Interannual and seasonal variability of biomass burning emissions constrained by satellite observations, J. Geophys. Res.-Atmos., 108, 4040, https://doi.org/10.1029/2002JD002378, 2003.
Eck, T. F., Holben, B. N., Reid, J. S., Dubovik, O., Smirnov, A., O'Neill, N. T., Slutsker, I., and Kinne, S.: Wavelength dependence of the optical depth of biomass burning, urban, and desert dust aerosols, J. Geophys. Res., 104, 31333, https://doi.org/10.1029/1999JD900923, 1999.
Eck, T. F., Holben, B. N., Ward, D. E., Mukelabai, M. M., Dubovik, O., Smirnov, A., Schafer, J. S., Hsu, N. C., Piketh, S. J., Queface, A., Le Roux, J., Swap, R. J., and Slutsker, I.: Variability of biomass burning aerosol optical characteristics in southern Africa during the SAFARI 2000 dry season campaign and a comparison of single scattering albedo estimates from radiometric measurements, J. Geophys. Res.-Atmos., 108, 8477, https://doi.org/10.1029/2002jd002321, 2003.
Eck, T. F., Holben, B. N., Reid, J. S., Mukelabai, M. M., Piketh, S. J., Torres, O., Jethva, H. T., Hyer, E. J., Ward, D. E., Dubovik, O., Sinyuk, A., Schafer, J. S., Giles, D. M., Sorokin, M., Smirnov, A., and Slutsker, I.: A seasonal trend of single scattering albedo in southern African biomass-burning particles: Implications for satellite products and estimates of emissions for the world's largest biomass-burning source, J. Geophys. Res.-Atmos., 118, 6414–6432, https://doi.org/10.1002/jgrd.50500, 2013.
Eck, T. F., Holben, B. N., Reid, J. S., Sinyuk, A., Giles, D. M., Arola, A., Slutsker, I., Schafer, J. S., Sorokin, M. G., Smirnov, A., LaRosa, A. D., Kraft, J., Reid, E. A., O'Neill, N. T., Welton, E. J., and Menendez, A. R.: The extreme forest fires in California/Oregon in 2020: Aerosol optical and physical properties and comparisons of aged versus fresh smoke, Atmos. Environ., 305, 119798, https://doi.org/10.1016/j.atmosenv.2023.119798, 2023.
Fougnie, B., Marbach, T., Lacan, A., Lang, R., Schlüssel, P., Poli, G., Munro, R., and Couto, A. B.: The multi-viewing multi-channel multi-polarisation imager – Overview of the 3MI polarimetric mission for aerosol and cloud characterization, J. Quant. Spectrosc. Ra., 219, 23–32, https://doi.org/10.1016/j.jqsrt.2018.07.008, 2018.
Garnett, J. C. M.: XII. Colours in metal glasses and in metallic films, Philos. T. Roy. Soc. Lond. A, 203, 385–420, https://doi.org/10.1098/rsta.1904.0024, 1904.
Giles, D. M., Holben, B. N., Eck, T. F., Sinyuk, A., Smirnov, A., Slutsker, I., Dickerson, R. R., Thompson, A. M., and Schafer, J. S.: An analysis of AERONET aerosol absorption properties and classifications representative of aerosol source regions, J. Geophys. Res.-Atmos., 117, 1–16, https://doi.org/10.1029/2012JD018127, 2012.
Giles, D. M., Sinyuk, A., Sorokin, M. G., Schafer, J. S., Smirnov, A., Slutsker, I., Eck, T. F., Holben, B. N., Lewis, J. R., Campbell, J. R., Welton, E. J., Korkin, S. V., and Lyapustin, A. I.: Advancements in the Aerosol Robotic Network (AERONET) Version 3 database – automated near-real-time quality control algorithm with improved cloud screening for Sun photometer aerosol optical depth (AOD) measurements, Atmos. Meas. Tech., 12, 169–209, https://doi.org/10.5194/amt-12-169-2019, 2019.
Go, S., Kim, J., Park, S. S., Kim, M., Lim, H., Kim, J. Y., Lee, D. W., and Im, J.: Synergistic use of hyperspectral uv-visible omi and broadband meteorological imager modis data for a merged aerosol product, Remote Sens., 12, 1–34, https://doi.org/10.3390/rs12233987, 2020.
Go, S., Lyapustin, A., Schuster, G. L., Choi, M., Ginoux, P., Chin, M., Kalashnikova, O., Dubovik, O., Kim, J., da Silva, A., Holben, B., and Reid, J. S.: Inferring iron-oxide species content in atmospheric mineral dust from DSCOVR EPIC observations, Atmos. Chem. Phys., 22, 1395–1423, https://doi.org/10.5194/acp-22-1395-2022, 2022.
Gyawali, M., Arnott, W., Zaveri, R., Song, C., Flowers, B., Dubey, M., Setyan, A., Zhang, Q., China, S., Mazzoleni, C., Gorkowski, K., Subramanian, R., and Moosmüller, H.: Evolution of Multispectral Aerosol Absorption Properties in a Biogenically-Influenced Urban Environment during the CARES Campaign, Atmosphere, 8, 217, https://doi.org/10.3390/atmos8110217, 2017.
Haywood, J. M., Abel, S. J., Barrett, P. A., Bellouin, N., Blyth, A., Bower, K. N., Brooks, M., Carslaw, K., Che, H., Coe, H., Cotterell, M. I., Crawford, I., Cui, Z., Davies, N., Dingley, B., Field, P., Formenti, P., Gordon, H., de Graaf, M., Herbert, R., Johnson, B., Jones, A. C., Langridge, J. M., Malavelle, F., Partridge, D. G., Peers, F., Redemann, J., Stier, P., Szpek, K., Taylor, J. W., Watson-Parris, D., Wood, R., Wu, H., and Zuidema, P.: The CLoud–Aerosol–Radiation Interaction and Forcing: Year 2017 (CLARIFY-2017) measurement campaign, Atmos. Chem. Phys., 21, 1049–1084, https://doi.org/10.5194/acp-21-1049-2021, 2021.
Hess, M., Koepke, P., and Schult, I.: Optical Properties of Aerosols and Clouds: The Software Package OPAC, B. Am. Meteorol. Soc., 79, 831–844, https://doi.org/10.1175/1520-0477(1998)079<0831:OPOAAC>2.0.CO;2, 1998.
Hobbs, P. V., Reid, J. S., Kotchenruther, R. A., Ferek, R. J., and Weiss, R.: Direct Radiative Forcing by Smoke from Biomass Burning, Science, 275, 1777–1778, https://doi.org/10.1126/science.275.5307.1777, 1997.
Hoffer, A., Gelencsér, A., Guyon, P., Kiss, G., Schmid, O., Frank, G. P., Artaxo, P., and Andreae, M. O.: Optical properties of humic-like substances (HULIS) in biomass-burning aerosols, Atmos. Chem. Phys., 6, 3563–3570, https://doi.org/10.5194/acp-6-3563-2006, 2006.
Holben, B. N., Eck, T. F., Slutsker, I., Tanré, D., Buis, J. P., Setzer, A., Vermote, E., Reagan, J. A., Kaufman, Y. J., Nakajima, T., Lavenu, F., Jankowiak, I., and Smirnov, A.: AERONET – A Federated Instrument Network and Data Archive for Aerosol Characterization, Remote Sens. Environ., 66, 1–16, https://doi.org/10.1016/S0034-4257(98)00031-5, 1998.
IPCC: Climate Change 2021 – The Physical Science Basis, Cambridge University Press, edited by: Masson-Delmotte, V., Zhai, P., Pirani A., Connors, S. L., Péan, C., Chen, Y., Goldfarb, L., Gomis, M. I., Robin Matthews, J. B., Berger, S., Huang, M., Yelekçi, O., Yu, R., Zhou, B.,Lonnoy, E., Maycock, T. K., Waterfield, T., Leitzell, K., and Caud, N.: https://doi.org/10.1017/9781009157896, 2023.
Jacobson, M. Z.: Strong radiative heating due to the mixing state of black carbon in atmospheric aerosols, Nature, 409, 695–697, https://doi.org/10.1038/35055518, 2001.
Jethva, H., Torres, O., and Ahn, C.: Global assessment of OMI aerosol single-scattering albedo using ground-based AERONET inversion, J. Geophys. Res., 119, 9020–9040, https://doi.org/10.1002/2014JD021672, 2014.
Jo, D. S., Park, R. J., Lee, S., Kim, S.-W., and Zhang, X.: A global simulation of brown carbon: implications for photochemistry and direct radiative effect, Atmos. Chem. Phys., 16, 3413–3432, https://doi.org/10.5194/acp-16-3413-2016, 2016.
Junghenn Noyes, K., Kahn, R., Sedlacek, A., Kleinman, L., Limbacher, J., and Li, Z.: Wildfire Smoke Particle Properties and Evolution, from Space-Based Multi-Angle Imaging, Remote Sens., 12, 769, https://doi.org/10.3390/rs12050769, 2020a.
Junghenn Noyes, K. T., Kahn, R. A., Limbacher, J. A., Li, Z., Fenn, M. A., Giles, D. M., Hair, J. W., Katich, J. M., Moore, R. H., Robinson, C. E., Sanchez, K. J., Shingler, T. J., Thornhill, K. L., Wiggins, E. B., and Winstead, E. L.: Wildfire Smoke Particle Properties and Evolution, From Space-Based Multi-Angle Imaging II: The Williams Flats Fire during the FIREX-AQ Campaign, Remote Sens., 12, 3823, https://doi.org/10.3390/rs12223823, 2020b.
Junghenn Noyes, K. T., Kahn, R. A., Limbacher, J. A., and Li, Z.: Canadian and Alaskan wildfire smoke particle properties, their evolution, and controlling factors, from satellite observations, Atmos. Chem. Phys., 22, 10267–10290, https://doi.org/10.5194/acp-22-10267-2022, 2022.
Kahn, R. A., Berkoff, T. A., Brock, C., Chen, G., Ferrare, R. A., Ghan, S., Hansico, T. F., Hegg, D. A., Martins, J. V., McNaughton, C. S., Murphy, D. M., Ogren, J. A., Penner, J. E., Pilewskie, P., Seinfeld, J. H., and Worsnop, D. R.: SAM-CAAM: A Concept for Acquiring Systematic Aircraft Measurements to Characterize Aerosol Air Masses, B. Am. Meteorol. Soc., 98, 2215–2228, https://doi.org/10.1175/BAMS-D-16-0003.1, 2017.
Kahn, R. A., Andrews, E., Brock, C. A., Chin, M., Feingold, G., Gettelman, A., Levy, R. C., Murphy, D. M., Nenes, A., Pierce, J. R., Popp, T., Redemann, J., Sayer, A. M., da Silva, A. M., Sogacheva, L., and Stier, P.: Reducing Aerosol Forcing Uncertainty by Combining Models With Satellite and Within-The-Atmosphere Observations: A Three-Way Street, Rev. Geophys., 61, e2022RG000796, https://doi.org/10.1029/2022RG000796, 2023.
Kalashnikova, O. V., Garay, M. J., Bates, K. H., Kenseth, C. M., Kong, W., Cappa, C. D., Lyapustin, A. I., Jonsson, H. H., Seidel, F. C., Xu, F., Diner, D. J., and Seinfeld, J. H.: Photopolarimetric Sensitivity to Black Carbon Content of Wildfire Smoke: Results From the 2016 ImPACT-PM Field Campaign, J. Geophys. Res.-Atmos., 123, 5376–5396, https://doi.org/10.1029/2017JD028032, 2018.
Kirchstetter, T. W., Novakov, T., and Hobbs, P. V.: Evidence that the spectral dependence of light absorption by aerosols is affected by organic carbon, J. Geophys. Res.-Atmos., 109, 1–12, https://doi.org/10.1029/2004JD004999, 2004.
Kleinman, L. I., Sedlacek III, A. J., Adachi, K., Buseck, P. R., Collier, S., Dubey, M. K., Hodshire, A. L., Lewis, E., Onasch, T. B., Pierce, J. R., Shilling, J., Springston, S. R., Wang, J., Zhang, Q., Zhou, S., and Yokelson, R. J.: Rapid evolution of aerosol particles and their optical properties downwind of wildfires in the western US, Atmos. Chem. Phys., 20, 13319–13341, https://doi.org/10.5194/acp-20-13319-2020, 2020.
Lack, D. A., Langridge, J. M., Bahreini, R., Cappa, C. D., Middlebrook, A. M., and Schwarz, J. P.: Brown carbon and internal mixing in biomass burning particles, P. Natl. Acad. Sci. USA, 109, 14802–14807, https://doi.org/10.1073/pnas.1206575109, 2012.
Laskin, A., Laskin, J., and Nizkorodov, S. A.: Chemistry of Atmospheric Brown Carbon, Chem. Rev., 115, 4335–4382, https://doi.org/10.1021/cr5006167, 2015.
Lavoué, D., Liousse, C., Cachier, H., Stocks, B. J., and Goldammer, J. G.: Modeling of carbonaceous particles emitted by boreal and temperate wildfires at northern latitudes, J. Geophys. Res.-Atmos., 105, 26871–26890, https://doi.org/10.1029/2000JD900180, 2000.
Lesins, G., Chylek, P., and Lohmann, U.: A study of internal and external mixing scenarios and its effect on aerosol optical properties and direct radiative forcing, J. Geophys. Res.-Atmos., 107, AAC5-1–AAC5-12, https://doi.org/10.1029/2001jd000973, 2002.
Levenberg, K.: A method for the solution of certain non-linear problems in least squares, Q. Appl. Math., 2, 164–168, https://doi.org/10.1090/qam/10666, 1944.
Li, L., Dubovik, O., Derimian, Y., Schuster, G. L., Lapyonok, T., Litvinov, P., Ducos, F., Fuertes, D., Chen, C., Li, Z., Lopatin, A., Torres, B., and Che, H.: Retrieval of aerosol components directly from satellite and ground-based measurements, Atmos. Chem. Phys., 19, 13409–13443, https://doi.org/10.5194/acp-19-13409-2019, 2019.
Li, L., Che, H., Derimian, Y., Dubovik, O., Schuster, G. L., Chen, C., Li, Q., Wang, Y., Guo, B., and Zhang, X.: Retrievals of fine mode light-absorbing carbonaceous aerosols from POLDER/PARASOL observations over East and South Asia, Remote Sens. Environ., 247, 111913, https://doi.org/10.1016/j.rse.2020.111913, 2020.
Li, L., Derimian, Y., Chen, C., Zhang, X., Che, H., Schuster, G. L., Fuertes, D., Litvinov, P., Lapyonok, T., Lopatin, A., Matar, C., Ducos, F., Karol, Y., Torres, B., Gui, K., Zheng, Y., Liang, Y., Lei, Y., Zhu, J., Zhang, L., Zhong, J., Zhang, X., and Dubovik, O.: Climatology of aerosol component concentrations derived from multi-angular polarimetric POLDER-3 observations using GRASP algorithm, Earth Syst. Sci. Data, 14, 3439–3469, https://doi.org/10.5194/essd-14-3439-2022, 2022.
Liang, Y., Stamatis, C., Fortner, E. C., Wernis, R. A., Van Rooy, P., Majluf, F., Yacovitch, T. I., Daube, C., Herndon, S. C., Kreisberg, N. M., Barsanti, K. C., and Goldstein, A. H.: Emissions of organic compounds from western US wildfires and their near-fire transformations, Atmos. Chem. Phys., 22, 9877–9893, https://doi.org/10.5194/acp-22-9877-2022, 2022.
Lim, H.-J. and Turpin, B. J.: Origins of Primary and Secondary Organic Aerosol in Atlanta: Results of Time-Resolved Measurements during the Atlanta Supersite Experiment, Environ. Sci. Technol., 36, 4489–4496, https://doi.org/10.1021/es0206487, 2002.
Limbacher, J. A., Kahn, R. A., and Lee, J.: The new MISR research aerosol retrieval algorithm: a multi-angle, multi-spectral, bounded-variable least squares retrieval of aerosol particle properties over both land and water, Atmos. Meas. Tech., 15, 6865–6887, https://doi.org/10.5194/amt-15-6865-2022, 2022.
Liu, D., He, C., Schwarz, J. P., and Wang, X.: Lifecycle of light-absorbing carbonaceous aerosols in the atmosphere, npj Clim. Atmos. Sci., 3, 40, https://doi.org/10.1038/s41612-020-00145-8, 2020.
Liu, Y., Stanturf, J., and Goodrick, S.: Trends in global wildfire potential in a changing climate, For. Ecol. Manage., 259, 685–697, https://doi.org/10.1016/j.foreco.2009.09.002, 2010.
Lyapustin, A., Wang, Y., Korkin, S., and Huang, D.: MODIS Collection 6 MAIAC algorithm, Atmos. Meas. Tech., 11, 5741–5765, https://doi.org/10.5194/amt-11-5741-2018, 2018.
Lyapustin, A., Wang, Y., Go, S., Choi, M., Korkin, S., Huang, D., Knyazikhin, Y., Blank, K., and Marshak, A.: Atmospheric Correction of DSCOVR EPIC: Version 2 MAIAC Algorithm, Front. Remote Sens., 2, 1–10, https://doi.org/10.3389/frsen.2021.748362, 2021a.
Lyapustin, A., Go, S., Korkin, S., Wang, Y., Torres, O., Jethva, H., and Marshak, A.: Retrievals of Aerosol Optical Depth and Spectral Absorption From DSCOVR EPIC, Front. Remote Sens., 2, 1–14, https://doi.org/10.3389/frsen.2021.645794, 2021b.
Markel, V. A.: Introduction to the Maxwell Garnett approximation: tutorial, J. Opt. Soc. Am. A, 33, 1244, https://doi.org/10.1364/josaa.33.001244, 2016a.
Markel, V. A.: Maxwell Garnett approximation (advanced topics): tutorial, J. Opt. Soc. Am. A, 33, 2237, https://doi.org/10.1364/josaa.33.002237, 2016b.
Marquardt, D. W.: An Algorithm for Least-Squares Estimation of Nonlinear Parameters, J. Soc. Ind. Appl. Math., 11, 431–441, https://doi.org/10.1137/0111030, 1963.
Marshak, A., Herman, J., Szabo, A., Blank, K., Carn, S., Cede, A., Geogdzhayev, I., Huang, D., Huang, L. K., Knyazikhin, Y., Kowalewski, M., Krotkov, N., Lyapustin, A., McPeters, R., Meyer, K. G., Torres, O., and Yang, Y.: Earth observations from DSCOVR epic instrument, B. Am. Meteorol. Soc., 99, 1829–1850, https://doi.org/10.1175/BAMS-D-17-0223.1, 2018.
Mok, J., Krotkov, N. A., Arola, A., Torres, O., Jethva, H., Andrade, M., Labow, G., Eck, T. F., Li, Z., Dickerson, R. R., Stenchikov, G. L., Osipov, S., and Ren, X.: Impacts of brown carbon from biomass burning on surface UV and ozone photochemistry in the Amazon Basin, Sci. Rep., 6, 1–9, https://doi.org/10.1038/srep36940, 2016.
Mok, J., Krotkov, N. A., Torres, O., Jethva, H., Li, Z., Kim, J., Koo, J.-H., Go, S., Irie, H., Labow, G., Eck, T. F., Holben, B. N., Herman, J., Loughman, R. P., Spinei, E., Lee, S. S., Khatri, P., and Campanelli, M.: Comparisons of spectral aerosol single scattering albedo in Seoul, South Korea, Atmos. Meas. Tech., 11, 2295–2311, https://doi.org/10.5194/amt-11-2295-2018, 2018.
Moosmüller, H., Chakrabarty, R. K., and Arnott, W. P.: Aerosol light absorption and its measurement: A review, J. Quant. Spectrosc. Ra., 110, 844–878, https://doi.org/10.1016/j.jqsrt.2009.02.035, 2009.
Nanda, S., de Graaf, M., Veefkind, J. P., Sneep, M., ter Linden, M., Sun, J., and Levelt, P. F.: A first comparison of TROPOMI aerosol layer height (ALH) to CALIOP data, Atmos. Meas. Tech., 13, 3043–3059, https://doi.org/10.5194/amt-13-3043-2020, 2020.
Pausas, J. G. and Keeley, J. E.: A Burning Story: The Role of Fire in the History of Life, Bioscience, 59, 593–601, https://doi.org/10.1525/bio.2009.59.7.10, 2009.
Petters, M. D., Carrico, C. M., Kreidenweis, S. M., Prenni, A. J., DeMott, P. J., Collett, J. L., and Moosmüller, H.: Cloud condensation nucleation activity of biomass burning aerosol, J. Geophys. Res.-Atmos., 114, D22205, https://doi.org/10.1029/2009JD012353, 2009.
Phillips, S. M. and Smith, G. D.: Light Absorption by Charge Transfer Complexes in Brown Carbon Aerosols, Environ. Sci. Technol. Lett., 1, 382–386, https://doi.org/10.1021/ez500263j, 2014.
Pokhrel, R. P., Wagner, N. L., Langridge, J. M., Lack, D. A., Jayarathne, T., Stone, E. A., Stockwell, C. E., Yokelson, R. J., and Murphy, S. M.: Parameterization of single-scattering albedo (SSA) and absorption Ångström exponent (AAE) with EC / OC for aerosol emissions from biomass burning, Atmos. Chem. Phys., 16, 9549–9561, https://doi.org/10.5194/acp-16-9549-2016, 2016.
Press, W. H., Teukolsky, S. A., Vetterling, W. T., and Flannery, B. P.: Numerical Recipes 3rd Edition: The Art of Scientific Computing, 3rd edn., Cambridge University Press, USA, ISBN 9780521880688, 2007.
Reid, J. S., Koppmann, R., Eck, T. F., and Eleuterio, D. P.: A review of biomass burning emissions part II: intensive physical properties of biomass burning particles, Atmos. Chem. Phys., 5, 799–825, https://doi.org/10.5194/acp-5-799-2005, 2005a.
Reid, J. S., Eck, T. F., Christopher, S. A., Koppmann, R., Dubovik, O., Eleuterio, D. P., Holben, B. N., Reid, E. A., and Zhang, J.: A review of biomass burning emissions part III: intensive optical properties of biomass burning particles, Atmos. Chem. Phys., 5, 827–849, https://doi.org/10.5194/acp-5-827-2005, 2005b.
Remer, L. A., Davis, A. B., Mattoo, S., Levy, R. C., Kalashnikova, O. V, Coddington, O., Chowdhary, J., Knobelspiesse, K., Xu, X., Ahmad, Z., Boss, E., Cairns, B., Dierssen, H. M., Diner, D. J., Franz, B., Frouin, R., Gao, B., Ibrahim, A., Martins, J. V., Omar, A. H., Torres, O., Xu, F., and Zhai, P.-W.: Retrieving Aerosol Characteristics From the PACE Mission, Part 1: Ocean Color Instrument, Front. Earth Sci., 7, 1–20, https://doi.org/10.3389/feart.2019.00152, 2019a.
Remer, L. A., Knobelspiesse, K., Zhai, P., Xu, F., Kalashnikova, O. V., Chowdhary, J., Hasekamp, O., Dubovik, O., Wu, L., Ahmad, Z., Boss, E., Cairns, B., Coddington, O., Davis, A. B., Dierssen, H. M., Diner, D. J., Franz, B., Frouin, R., Gao, B., Ibrahim, A., Levy, R. C., Martins, J. V., Omar, A. H., and Torres, O.: Retrieving Aerosol Characteristics From the PACE Mission, Part 2: Multi-Angle and Polarimetry, Front. Environ. Sci., 7, 1–21, https://doi.org/10.3389/fenvs.2019.00094, 2019b.
Rios, B., Díaz-Esteban, Y., and Raga, G. B.: Smoke emissions from biomass burning in Central Mexico and their impact on air quality in Mexico City: May 2019 case study, Sci. Total Environ., 904, 166912, https://doi.org/10.1016/j.scitotenv.2023.166912, 2023.
Rissler, J., Vestin, A., Swietlicki, E., Fisch, G., Zhou, J., Artaxo, P., and Andreae, M. O.: Size distribution and hygroscopic properties of aerosol particles from dry-season biomass burning in Amazonia, Atmos. Chem. Phys., 6, 471–491, https://doi.org/10.5194/acp-6-471-2006, 2006.
Samset, B. H., Stjern, C. W., Andrews, E., Kahn, R. A., Myhre, G., Schulz, M., and Schuster, G. L.: Aerosol Absorption: Progress Towards Global and Regional Constraints, Curr. Clim. Chang. Reports, 4, 65–83, https://doi.org/10.1007/s40641-018-0091-4, 2018.
Sayer, A. M., Hsu, N. C., Eck, T. F., Smirnov, A., and Holben, B. N.: AERONET-based models of smoke-dominated aerosol near source regions and transported over oceans, and implications for satellite retrievals of aerosol optical depth, Atmos. Chem. Phys., 14, 11493–11523, https://doi.org/10.5194/acp-14-11493-2014, 2014.
Sayer, A. M., Hsu, N. C., Lee, J., Kim, W. V., Burton, S., Fenn, M. A., Ferrare, R. A., Kacenelenbogen, M., LeBlanc, S., Pistone, K., Redemann, J., Segal-Rozenhaimer, M., Shinozuka, Y., and Tsay, S.-C.: Two decades observing smoke above clouds in the south-eastern Atlantic Ocean: Deep Blue algorithm updates and validation with ORACLES field campaign data, Atmos. Meas. Tech., 12, 3595–3627, https://doi.org/10.5194/amt-12-3595-2019, 2019.
Schroeder, W. and Giglio, L.: VIIRS/NPP Thermal Anomalies/Fire Daily L3 Global 1km SIN Grid V001, NASA EOSDIS Land Processes DAAC, https://doi.org/10.5067/VIIRS/VNP14A1.001, 2018.
Schuster, G. L., Dubovik, O., Holben, B. N., and Clothiaux, E. E.: Inferring black carbon content and specific absorption from Aerosol Robotic Network (AERONET) aerosol retrievals, J. Geophys. Res.-Atmos., 110, 1–19, https://doi.org/10.1029/2004JD004548, 2005.
Schuster, G. L., Dubovik, O., and Arola, A.: Remote sensing of soot carbon – Part 1: Distinguishing different absorbing aerosol species, Atmos. Chem. Phys., 16, 1565–1585, https://doi.org/10.5194/acp-16-1565-2016, 2016.
Schwarz, J. P., Gao, R. S., Spackman, J. R., Watts, L. A., Thomson, D. S., Fahey, D. W., Ryerson, T. B., Peischl, J., Holloway, J. S., Trainer, M., Frost, G. J., Baynard, T., Lack, D. A., de Gouw, J. A., Warneke, C., and Del Negro, L. A.: Measurement of the mixing state, mass, and optical size of individual black carbon particles in urban and biomass burning emissions, Geophys. Res. Lett., 35, L13810, https://doi.org/10.1029/2008GL033968, 2008.
Seiler, W. and Crutzen, P. J.: Estimates of gross and net fluxes of carbon between the biosphere and the atmosphere from biomass burning, Clim. Change, 2, 207–247, https://doi.org/10.1007/BF00137988, 1980.
Sengupta, D., Samburova, V., Bhattarai, C., Kirillova, E., Mazzoleni, L., Iaukea-Lum, M., Watts, A., Moosmüller, H., and Khlystov, A.: Light absorption by polar and non-polar aerosol compounds from laboratory biomass combustion, Atmos. Chem. Phys., 18, 10849–10867, https://doi.org/10.5194/acp-18-10849-2018, 2018.
Sengupta, D., Samburova, V., Bhattarai, C., Watts, A. C., Moosmüller, H., and Khlystov, A. Y.: Polar semivolatile organic compounds in biomass-burning emissions and their chemical transformations during aging in an oxidation flow reactor, Atmos. Chem. Phys., 20, 8227–8250, https://doi.org/10.5194/acp-20-8227-2020, 2020.
Shen, Y., Pokhrel, R. P., Sullivan, A. P., Levin, E. J. T., Garofalo, L. A., Farmer, D. K., Permar, W., Hu, L., Toohey, D. W., Campos, T., Fischer, E. V., and Murphy, S. M.: Understanding Absorption by Black Versus Brown Carbon in Biomass Burning Plumes from the WE-CAN Campaign, EGUsphere [preprint], https://doi.org/10.5194/egusphere-2023-3114, 2024.
Sinyuk, A., Holben, B. N., Eck, T. F., Giles, D. M., Slutsker, I., Korkin, S., Schafer, J. S., Smirnov, A., Sorokin, M., and Lyapustin, A.: The AERONET Version 3 aerosol retrieval algorithm, associated uncertainties and comparisons to Version 2, Atmos. Meas. Tech., 13, 3375–3411, https://doi.org/10.5194/amt-13-3375-2020, 2020.
Sinyuk, A., Holben, B. N., Eck, T. F., Giles, D. M., Slutsker, I., Dubovik, O., Schafer, J. S., Smirnov, A., and Sorokin, M.: Employing relaxed smoothness constraints on imaginary part of refractive index in AERONET aerosol retrieval algorithm, Atmos. Meas. Tech., 15, 4135–4151, https://doi.org/10.5194/amt-15-4135-2022, 2022.
Sumlin, B. J., Heinson, Y. W., Shetty, N., Pandey, A., Pattison, R. S., Baker, S., Hao, W. M., and Chakrabarty, R. K.: UV–Vis–IR spectral complex refractive indices and optical properties of brown carbon aerosol from biomass burning, J. Quant. Spectrosc. Ra., 206, 392–398, https://doi.org/10.1016/j.jqsrt.2017.12.009, 2018.
Sun, H., Biedermann, L., and Bond, T. C.: Color of brown carbon: A model for ultraviolet and visible light absorption by organic carbon aerosol, Geophys. Res. Lett., 34, L17813, https://doi.org/10.1029/2007GL029797, 2007.
Swap, R. J., Annegarn, H. J., Suttles, J. T., King, M. D., Platnick, S., Privette, J. L., and Scholes, R. J.: Africa burning: A thematic analysis of the Southern African Regional Science Initiative (SAFARI 2000), J. Geophys. Res.-Atmos., 108, 8465, https://doi.org/10.1029/2003JD003747, 2003.
Torres, O., Bhartia, P. K., Herman, J. R., Ahmad, Z., and Gleason, J.: Derivation of aerosol properties from satellite measurements of backscattered ultraviolet radiation: Theoretical basis, J. Geophys. Res.-Atmos., 103, 17099–17110, https://doi.org/10.1029/98JD00900, 1998.
Torres, O., Tanskanen, A., Veihelmann, B., Ahn, C., Braak, R., Bhartia, P. K., Veefkind, P., and Levelt, P.: Aerosols and surface UV products form Ozone Monitoring Instrument observations: An overview, J. Geophys. Res.-Atmos., 112, 1–14, https://doi.org/10.1029/2007JD008809, 2007.
Torres, O., Ahn, C., and Chen, Z.: Improvements to the OMI near-UV aerosol algorithm using A-train CALIOP and AIRS observations, Atmos. Meas. Tech., 6, 3257–3270, https://doi.org/10.5194/amt-6-3257-2013, 2013.
Torres, O., Jethva, H., Ahn, C., Jaross, G., and Loyola, D. G.: TROPOMI aerosol products: evaluation and observations of synoptic-scale carbonaceous aerosol plumes during 2018–2020, Atmos. Meas. Tech., 13, 6789–6806, https://doi.org/10.5194/amt-13-6789-2020, 2020.
Tritscher, T., Jurányi, Z., Martin, M., Chirico, R., Gysel, M., Heringa, M. F., DeCarlo, P. F., Sierau, B., Prévôt, A. S. H., Weingartner, E., and Baltensperger, U.: Changes of hygroscopicity and morphology during ageing of diesel soot, Environ. Res. Lett., 6, 034026, https://doi.org/10.1088/1748-9326/6/3/034026, 2011.
Turpin, B. J. and Lim, H.-J.: Species Contributions to PM2.5 Mass Concentrations: Revisiting Common Assumptions for Estimating Organic Mass, Aerosol Sci. Tech., 35, 602–610, https://doi.org/10.1080/02786820119445, 2001.
Val Martin, M., Logan, J. A., Kahn, R. A., Leung, F.-Y., Nelson, D. L., and Diner, D. J.: Smoke injection heights from fires in North America: analysis of 5 years of satellite observations, Atmos. Chem. Phys., 10, 1491–1510, https://doi.org/10.5194/acp-10-1491-2010, 2010.
van der Werf, G. R., Randerson, J. T., Giglio, L., van Leeuwen, T. T., Chen, Y., Rogers, B. M., Mu, M., van Marle, M. J. E., Morton, D. C., Collatz, G. J., Yokelson, R. J., and Kasibhatla, P. S.: Global fire emissions estimates during 1997–2016, Earth Syst. Sci. Data, 9, 697–720, https://doi.org/10.5194/essd-9-697-2017, 2017.
Wang, L., Li, Z., Tian, Q., Ma, Y., Zhang, F., Zhang, Y., Li, D., Li, K., and Li, L.: Estimate of aerosol absorbing components of black carbon, brown carbon, and dust from ground-based remote sensing data of sun-sky radiometers, J. Geophys. Res.-Atmos., 118, 6534–6543, https://doi.org/10.1002/jgrd.50356, 2013.
Wang, X., Heald, C. L., Sedlacek, A. J., de Sá, S. S., Martin, S. T., Alexander, M. L., Watson, T. B., Aiken, A. C., Springston, S. R., and Artaxo, P.: Deriving brown carbon from multiwavelength absorption measurements: method and application to AERONET and Aethalometer observations, Atmos. Chem. Phys., 16, 12733–12752, https://doi.org/10.5194/acp-16-12733-2016, 2016.
Ward, D. E., Hao, W. M., Susott, R. A., Babbitt, R. E., Shea, R. W., Kauffman, J. B., and Justice, C. O.: Effect of fuel composition on combustion efficiency and emission factors for African savanna ecosystems, J. Geophys. Res.-Atmos., 101, 23569–23576, https://doi.org/10.1029/95JD02595, 1996.
Wooster, M. J., Roberts, G. J., Giglio, L., Roy, D., Freeborn, P., Boschetti, L., Justice, C., Ichoku, C., Schroeder, W., Davies, D., Smith, A., Setzer, A., Csiszar, I., Strydom, T., Frost, P., Zhang, T., Xu, W., de Jong, M., Johnston, J., Ellison, L., Vadrevu, K., McCarty, J., Tanpipat, V., Schmidt, C., and San-Miguel, J.: Satellite remote sensing of active fires: History and current status, applications and future requirements, Remote, Sent. Environ., 267, 112694 https://doi.org/10.1016/j.rse.2021.112694, 2021.
Xiong, R., Li, J., Zhang, Y., Zhang, L., Jiang, K., Zheng, H., Kong, S., Shen, H., Cheng, H., Shen, G., and Tao, S.: Global brown carbon emissions from combustion sources, Environ. Sci. Ecotechnology, 12, 100201, https://doi.org/10.1016/j.ese.2022.100201, 2022.
Xu, X., Wang, J., Wang, Y., Zeng, J., Torres, O., Reid, J. S., Miller, S. D., Martins, J. V., and Remer, L. A.: Detecting layer height of smoke aerosols over vegetated land and water surfaces via oxygen absorption bands: hourly results from EPIC/DSCOVR in deep space, Atmos. Meas. Tech., 12, 3269–3288, https://doi.org/10.5194/amt-12-3269-2019, 2019.
Yu, H., Liu, S. C., and Dickinson, R. E.: Radiative effects of aerosols on the evolution of the atmospheric boundary layer, J. Geophys. Res.-Atmos., 107, AAC3-1–AAC3-14, https://doi.org/10.1029/2001JD000754, 2002.
Zhang, A., Wang, Y., Zhang, Y., Weber, R. J., Song, Y., Ke, Z., and Zou, Y.: Modeling the global radiative effect of brown carbon: a potentially larger heating source in the tropical free troposphere than black carbon, Atmos. Chem. Phys., 20, 1901–1920, https://doi.org/10.5194/acp-20-1901-2020, 2020.