the Creative Commons Attribution 4.0 License.
the Creative Commons Attribution 4.0 License.
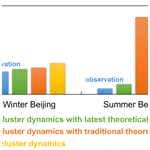
Cluster-dynamics-based parameterization for sulfuric acid–dimethylamine nucleation: comparison and selection through box and three-dimensional modeling
Jiewen Shen
Yuyang Li
Runlong Cai
Da Gao
Manish Shrivastava
Jingkun Jiang
Xiuhui Zhang
Clustering of gaseous sulfuric acid (SA) enhanced by dimethylamine (DMA) is a major mechanism for new particle formation (NPF) in polluted atmospheres. However, uncertainty remains regarding the SA–DMA nucleation parameterization that reasonably represents cluster dynamics and is applicable across various atmospheric conditions. This uncertainty hinders accurate three-dimensional (3-D) modeling of NPF and the subsequent assessment of its environmental and climatic impacts. Here we extensively compare different cluster-dynamics-based parameterizations for SA–DMA nucleation and identify the most reliable one through a combination of box model simulations, 3-D modeling, and in situ observations. Results show that the parameterization derived from Atmospheric Cluster Dynamic Code (ACDC) simulations, incorporating the latest theoretical insights (DLPNO-CCSD(T)/aug-cc-pVTZ//ωB97X-D/6-311G(3df,3pd) level of theory) and adequate representation of cluster dynamics, exhibits dependable performance in 3-D NPF simulation for both winter and summer conditions in Beijing and shows promise for application in diverse atmospheric conditions. Another ACDC-derived parameterization, replacing the level of theory with RI-CC2/aug-cc-pV(T+d)Z//M06-2X/6–311G(3df,3pd), also performs well in NPF modeling at relatively low temperatures around 280 K but exhibits limitations at higher temperatures due to inappropriate representation of SA–DMA cluster thermodynamics. Additionally, a previously reported parameterization incorporating simplifications is applicable for simulating NPF in polluted atmospheres but tends to overestimate particle formation rates under conditions of elevated temperature ( K) and low-condensation sink ( s−1). Our findings highlight the applicability of the new ACDC-derived parameterization, which couples the latest SA–DMA nucleation theory and holistic cluster dynamics, in 3-D NPF modeling. The ACDC-derived parameterization framework provides a valuable reference for developing parameterizations for other nucleation systems.
- Article
(4764 KB) - Full-text XML
-
Supplement
(2461 KB) - BibTeX
- EndNote
Atmospheric aerosols have significant impacts on visibility, human health, and global climate (Gordon et al., 2016; Gao et al., 2024). New particle formation (NPF) is the predominant source of global aerosol population, with nucleation being the key stage of the gas-to-particle transformation (Zhao et al., 2020; Almeida et al., 2013). In polluted regions such as urban China, compelling evidence indicates that sulfuric acid (SA)-driven nucleation enhanced by dimethylamine (DMA) can generate thermodynamically stable SA–DMA clusters and lead to high particle formation rates close to kinetic limit of SA clustering, which is responsible for the observed intensive NPF events (Cai et al., 2021; Yao et al., 2018). Meanwhile, it has been demonstrated that variations in atmospheric conditions, including condensation sinks (CSs) arising from background aerosols, along with temperature (T), can exert profound impacts on the cluster dynamics of SA–DMA nucleation by varying the particle formation rates across several orders of magnitude (Cai et al., 2021; Deng et al., 2020). Given that complex interactions exist among various gaseous precursors, molecular clusters, and pre-existing aerosols during nucleation, reasonable representation of the cluster dynamics of SA–DMA nucleation in three-dimensional (3-D) models is important for 3-D NPF modeling and the subsequent assessment of its impacts on the environment and climate.
Empirical models in the form of power-law functions have been extensively utilized to examine how particle formation rates respond to precursor concentrations (Semeniuk and Dastoor, 2018). Through parameter fitting, these empirical models can effectively reproduce the particle formation rates observed in both laboratory experiments and field measurements (Kulmala et al., 2006; Riccobono et al., 2014; Semeniuk and Dastoor, 2018). Subsequently, they can be integrated into 3-D models for regional or global NPF simulations. Bergman et al. (2015) and Dunne et al. (2016) have simulated SA–DMA nucleation utilizing global models, which incorporate empirical equations derived from experimental data obtained from CLOUD (Cosmics Leaving OUtdoor Droplets) chamber or flow tube experiments. These parameterization schemes successfully characterize the response of particle formation rates into precursor concentrations; however, they fail to account for dependencies on T and CS due to the ignorance of explicit cluster dynamics. As a result, they are identified to be inadequate for accurately reproducing NPF events in wintertime Beijing (Y. Li et al., 2023).
We recently developed an analytical equation for SA–DMA nucleation parameterization based on detailed cluster dynamics simulations (abbreviated as Dynamic_Sim) (Y. Li et al., 2023). Previous theoretical insights into the SA–DMA system (Olenius et al., 2013, 2017; Ortega et al., 2012; Myllys et al., 2019) indicate that (SA)k(DMA)k (k=1–4) and (SA)2(DMA)1 clusters are considered the key clusters along the cluster formation pathways in SA–DMA nucleation. Under the polluted conditions ( s−1), the evaporation rates of (SA)k(DMA)k (k=2–4) and (SA)2(DMA)1 clusters are negligible compared to their coagulation sink. Accordingly, several simplifications have been made in Dynamic_Sim, including the following: (1) only (SA)k(DMA)k (k=1–4) and (SA)2(DMA)1 clusters are considered, (2) clusters larger than (SA)1(DMA)1 are regarded as stable and with no evaporation, and (3) the (SA)4(DMA)4 cluster is the only terminal cluster in calculating particle formation rates. Subsequent applications in 3-D modeling have demonstrated the significantly improved performance of Dynamic_Sim compared to previous data-fitting parameterizations in simulating the particle formation rates, the evolution of particle number size distributions (PNSDs), and NPF events in wintertime Beijing. However, the efficacy of Dynamic_Sim in the NPF simulation has yet to be assessed under varying atmospheric conditions, such as the summer season characterized by relatively higher T and lower CS compared to winter. Moreover, the impacts of simplifications made in the derivation of Dynamic_Sim on 3-D NPF simulation under different atmospheric conditions remain unclear.
In addition to the form of explicit formulations, the integration of nucleation dynamics in 3-D models can also be realized using precomputed lookup tables generated by box models. Atmospheric Cluster Dynamics Code (ACDC) is a representative box model for simulating cluster dynamics and particle formation rates (McGrath et al., 2012; Olenius et al., 2013). In addition to representing T and CS dependencies for the particle formation rate as Dynamic_Sim, ACDC considers the source and sink terms of all given molecules/clusters within a nucleation system without simplifications of the clustering processes. By integrating quantum chemical calculations with ACDC, Almeida et al. (2013) discovered that the simulated SA–DMA nucleation provides valuable insights for interpreting the measurements from the CLOUD chamber experiments. Similarly, Lu et al. (2020) demonstrated that ACDC coupled with quantum chemistry calculations can effectively reproduce the particle formation rates observed in urban Shanghai. In addition to its extensive utilization in box modeling (Almeida et al., 2013; Lu et al., 2020; Yang et al., 2021), several studies have simulated nucleation pathways in chemical transport models using precomputed lookup tables generated by ACDC. For example, Baranizadeh et al. (2016) and Croft et al. (2016) used ACDC-derived lookup tables as nucleation parameterizations to probe the impacts of SA–NH3–H2O nucleation on aerosol number concentration, cloud properties, and radiation balance. Olin et al. (2022) and Julin et al. (2018) evaluated the impact of new particle formation on the aerosol number concentrations in Europe under historical and emission reduction scenarios, respectively, using ACDC-derived parameterizations involving both SA–NH3–H2O and SA–DMA nucleation. It should be noted that the ACDC program in modeling the nucleation process is highly reliant on specific thermodynamic data for the molecular clusters of interest, which are primarily obtained through quantum chemical calculations (Elm et al., 2020). A very recent study by Svenhag et al. (2024) compared the impact of two typical quantum calculation methods on 3-D modeling of SA–NH3 nucleation using ACDC-derived parameterizations. However, it is still unclear how different quantum chemical methods affect the 3-D modeling of SA–DMA nucleation.
This study aims to compare different cluster-dynamic-based parameterizations for SA–DMA nucleation and identify the robust one applicable for 3-D models. We introduced parameterizations developed using the ACDC program, incorporating various quantum chemical calculations. Different cluster-dynamic-based parameterizations, including ACDC-derived ones and Dynamic_Sim, are comprehensively compared and evaluated through a combination of box model simulations, 3-D modeling, and in situ observational data. Our findings reveal that by incorporating the latest theoretical understanding and complete representation of cluster dynamics, ACDC-derived parameterization demonstrates a reliable performance in the 3-D NPF simulation for both winter and summer conditions in Beijing and exhibits potential applicability in diverse atmospheric conditions. The study sheds light on the impacts of employing various simplifications in cluster dynamics and different theoretical approaches in deriving parameterizations on NPF simulation. In addition to contributing to the precise simulation of SA–DMA nucleation and the quantification of its environmental and climatic effects, this study provides valuable references for simulating other nucleation mechanisms in 3-D models.
2.1 Configurations of ACDC
Here, (SA)m(DMA)n clusters (, where m and n represent the number of SA and DMA molecules in a cluster) are used to build the ACDC-derived parameterizations for SA–DMA nucleation due to their reported much higher stability compared to those containing more DMA molecules than SA molecules (Xie et al., 2017). The ACDC code is available at https://github.com/tolenius/ACDC (Olenius, 2024). The conformations and thermodynamics of SA–DMA clusters are taken from our other study (Ning et al., 2024). Briefly, the conformations of selected clusters are taken from the reported global minima from Li et al. (2020), and the key thermodynamic data for ACDC, the Gibbs free-energy change (ΔG), are recalculated at the DLPNO-CCSD(T)/aug-cc-pVTZ//ωB97X-D/6-311G(3df,3pd) level of theory. Based on benchmark studies (Elm et al., 2020), this level of theory provides dependable thermodynamic insights into molecular clusters during nucleation and represents the latest theoretical approach. In addition, the rotational symmetry is consistently considered in quantum calculations, following Besel et al. (2020). Following most previous ACDC simulation studies (Xie et al., 2017; Elm et al., 2020; Ning et al., 2020), (SA)4(DMA)3 and (SA)4(DMA)4 clusters are defined as the boundary conditions, i.e., the clusters fluxing out the simulated system and participating in subsequent growth in ACDC simulations, considering their high stability. Since clusters containing SA tetramers are estimated to have an electrical mobility diameter of 1.4 nm (Cai et al., 2023; Jen et al., 2014; Thomas et al., 2016), the formation rates of (SA)4(DMA)3 and (SA)4(DMA)4 clusters are therefore deemed to be the particle formation rates at 1.4 nm (J1.4). The size-dependent coagulation sink (CoagS) is counted for each SA–DMA cluster, which is consistent with Dynamic_Sim (Y. Li et al., 2023) as follows:
where Vi and V1 (m3) represent the volume of cluster i and the SA molecule, respectively. The power-law exponent of −1.7 is selected according to typical range in the atmosphere (Lehtinen et al., 2007). In addition, an enhancement for collision processes from the Van de Waals force is also considered. We refer to the ACDC-derived parameterization in coupling the DLPNO-CCSD(T)/aug-cc-pVTZ//ωB97X-D/6-311G(3df,3pd) level of theory and adequate cluster dynamics as ACDC_DB, which is established as the base case for our discussion of other cluster-dynamics-based parameterizations.
In addition to the direct comparison of ACDC_DB to Dynamic_Sim, additional test parameterizations combining ACDC_DB and three simplifications within Dynamic_Sim are established and compared with ACDC_DB to further probe the impacts of these simplifications on NPF simulations. According to our previous study, altering the simplifications within Dynamic_Sim to explicit treatment would substantially escalate the computational demand by several orders of magnitude (Y. Li et al., 2023). Therefore, we utilize the ACDC-derived lookup tables to evaluate the impacts of the simplified treatments. The configurations of all parameterizations are detailed in Table 1. It should be noted that when all simplifications are applied on ACDC_DB, Dynamic_Sim still predicts higher J1.4 compared to ACDC_DB (Fig. S1A in the Supplement). This is because the ΔG value of the initial (SA)1(DMA)1 cluster at 298.15 K used in Dynamic_Sim, which is taken from Myllys et al. (2019), is slightly lower than that used in ACDC_DB (−13.5 kcal mol−1 for Dynamic_Sim and −12.9 kcal mol−1 for ACDC_DB) (Ning et al., 2024), even though both parameterizations employ the quantum chemical calculation method of DLPNO-CCSD(T). Possible reasons for the discrepancy include the utilization of a larger base set (3-zeta 6-311G(3df,3pd)) and higher convergence criteria (tight PNO and tight SCF) in this study compared to that in Myllys et al. (2019). Aligning the ΔG for (SA)1(DMA)1 cluster in Dynamic_Sim with that of ACDC leads to a high consistency in the predicted J1.4 between the two approaches (Fig. S1B). The uncertainty in ΔG used in Dynamic_Sim is discussed in our previous study (Y. Li et al., 2023), and here we mainly focus on the impacts of simplifications in Dynamic_Sim.
Table 1Summary of various cluster-dynamics-based parameterizations of SA–DMA nucleation in this study (main parameterizations are in bold, while test ones are roman).
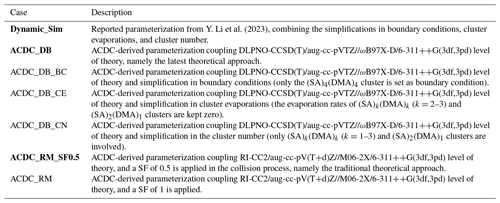
While the DLPNO-CCSD(T)/aug-cc-pVTZ//ωB97X-D/6-311G(3df,3pd) level of theory yields reasonable cluster thermodynamics, quantum chemistry calculations, employing the RI-CC2 method and predicting a lower ΔG for cluster formation (stronger binding between molecules within clusters), has been widely used in conjunction with ACDC to interpret experimental and observed particle formation rates in previous studies (Almeida et al., 2013; Kürten et al., 2018; Ning et al.,2020). The prevalent combination used with the RI-CC2 method is the RI-CC2/aug-cc-pV(T+d)Z//M06-2X/6-311G(3df,3pd) level of theory (Lu et al., 2020; Liu et al., 2021, 2019; Ning et al., 2022; Ning and Zhang, 2022). Based on the work by Elm et al. (2013, 2020), compared to DLPNO-CCSD(T)/aug-cc-pVTZ//ωB97X-D/6-311G(3df,3pd), the differences in the predicted cluster-binding energies primarily stem from discrepancies between DLPNO-CCSD(T) and RI-CC2 in single-point energy calculations, while the ωB97X-D and M06-2X functionals exhibit similar performance. Also, in previous studies, the RI-CC2 method combined with ACDC was consistently accompanied by the application of a sticking factor (SF) of 0.5 for treating collision processes (Almeida et al., 2013; Lu et al., 2020). However, it is noteworthy that, according to Stolzenburg et al. (2020), the SF of the neutral SA–DMA cluster system should be unity. Here, we refer to the traditional theoretical approach for employing the RI-CC2/aug-cc-pV(T+d)Z//M06-2X/6-311G(3df,3pd) level of theory and incorporating the SF of 0.5 in collision processes. An ACDC-derived parameterization coupling the traditional theoretical approach is established to assess the effectiveness of the traditional method in the NPF simulation (ACDC_RM_SF0.5). Except for the varied thermodynamic inputs and SF, the remaining configurations of ACDC_RM_SF0.5 are identical to ACDC_DB. Additionally, we establish a test parameterization coupling RI-CC2/aug-cc-pV(T+d)Z//M06-2X/6-311G(3df,3pd) level of theory with an SF of unity (ACDC_RM) to evaluate the impact solely arising from the quantum chemical calculation method. Note that an SF of unity is applied to all parameterizations in this study, except for the ACDC_RM_SF0.5.
To quantify the differences in simulating J1.4 among different cluster-dynamics-based parameterizations compared to our base case ACDC_DB, we introduce a parameter R as follows:
where ACDC_DBi and Xi denote the simulated J1.4 by the base case ACDC_DB and another specific parameterization X, respectively, given the input scenarios of i (a set of input values for T and CS and the concentration of SA ([SA]) and DMA ([DMA])), and n signifies the total number of input scenarios.
2.2 Incorporating the ACDC-derived parameterizations into WRF-Chem/R2D-VBS model
Various parameterizations are subsequently implemented in the Weather Research and Forecasting model coupled with Chemistry (WRF-Chem), integrating an experimentally constrained radical two-dimensional volatility basis set (2D-VBS) (denoted as WRF-Chem/R2D-VBS) (Zhao et al., 2020). Incorporating the box model ACDC into a 3-D model using the explicit mathematical formula, such as Dynamic_Sim, proves to be challenging. Here, we created a four-dimensional lookup table that delineates the response of J1.4 to four input variables (T, CS, [SA], and [DMA]) for each ACDC-derived parameterization (Yu, 2010). The table is derived based on multiple ACDC runs by varying input variables. The ranges for the input variables correspond to the typical conditions of the atmosphere. Except for T, the ranges of variation for all other variables exceed at least 1 order of magnitude. Therefore, the temperature is assumed to follow a uniform arithmetic distribution, while the other variables are assumed to follow a uniform geometric distribution. Details for the input variables are given in Table S1 in the Supplement. In WRF-Chem/R2D-VBS simulations, J1.4 values are calculated online by interpolating values from a lookup table based on real-time input parameters. In our previous study, we have developed an emission inventory for China and its surrounding regions (Y. Li et al., 2023). Here, [DMA] is calculated in WRF-Chem/R2D-VBS based on a comprehensive source–sink representation of DMA. More details of including DMA in WRF-Chem/R2D-VBS can be found in our previous study (Y. Li et al., 2023). In addition, a time-integrated average [DMA] and [SA] of each time step was used to drive SA–DMA nucleation, since SA–DMA nucleation is accompanied by the condensation of gaseous SA and DMA on pre-existing aerosols simultaneously in the atmosphere.
Besides SA–DMA nucleation, seven other nucleation mechanisms have already been incorporated in WRF-Chem/R2D-VBS (Zhao et al., 2020), including neutral/ion-induced SA–H2O nucleation, neutral/ion-induced SA–NH3-H2O nucleation, neutral/ion-induced pure organics nucleation, and SA–organics nucleation. The organics involved in nucleation are ultralow- and extremely low-volatility organic compounds (ULVOCs and ELVOCs) with . The formation chemistry of ULVOCs and ELVOCs from monoterpenes, including autoxidation and dimerization, is traced by the R2D-VBS framework (Zhao et al., 2020). Note that the impact of the other seven mechanisms on particle formation rates and particle number concentration is low compared to SA–DMA, as revealed by our previous study (Y. Li et al., 2023). In WRF-Chem/R2D-VBS, the evolution of PNSDs from 1 nm to 10 µm is treated by MOSAIC (Model for Simulating Aerosol Interactions and Chemistry). The newly formed 1.4 nm particles from SA–DMA nucleation are injected into the smallest size bin (1–1.5 nm) of MOSAIC.
2.3 Configurations of WRF-Chem/R2D-VBS model
The WRF-Chem/R2D-VBS model, incorporating various cluster-dynamics-based SA–DMA nucleation parameterizations, was employed in a simulation over a domain with a spatial resolution of 27 km. This domain covers eastern Asia, with Beijing situated close to the center of the simulation area. Details of model configurations can be found in our previous study (Y. Li et al., 2023). Briefly, we use the ABaCAS-EI 2017 dataset and the International Institute for Applied Systems Analysis (IIASA) 2015 emission inventories for mainland China and other areas in the domain, respectively, to represent the anthropogenic emissions (Zheng et al., 2019; Li et al., 2017; S. Li et al., 2023); we use Model of Emissions of Gases and Aerosols from Nature (MEGAN) v2.04 to calculate the biogenic emissions (Guenther et al., 2006). To accurately represent the variation and distribution of chemical species concentrations during the simulation period, the initial chemical conditions, which represent the concentration field of chemical species at the initial simulation time, and the boundary conditions, which represent the flux or concentration around the simulation domain during the simulation period (Brasseur et al., 2017), are used in our WRF-Chem/R2D-VBS simulations. The simulation results from the National Center for Atmospheric Research's Community Atmosphere Model with Chemistry (https://www.acom.ucar.edu/cam-chem/cam-chem.shtml, last access: 1 August 2024) is used for the chemical initial and boundary conditions in WRF-Chem/R2D-VBS simulations. In addition, we use a 5 d spin-up period to minimize the impact of chemical initial conditions on simulation results.
The simulation period consists of two parts: the winter period, which spans 14 to 31 January 2019, and the summer period, which is from 18 to 31 August 2019. Previous observational studies have shown that the particle formation rates reach their highest and lowest levels during winter and summer in China, respectively (Deng et al., 2020; Chu et al., 2019). Therefore, periods from these two seasons are selected as representative simulation periods in this study, and the specific time periods corresponded to those with relatively complete and continuous PNSDs and J1.4 observations. Since the observational data for DMA concentration are only available for the period from 1 to 23 January 2019, similar to our other study (Ning et al., 2024), we performed an additional simulation for this period to compare the observational and simulated DMA concentrations. For each season, all the SA–DMA parameterizations listed in Table 1 were employed for the simulation. Among them, ACDC_DB, Dynamic_Sim, and ACDC_RM_SF0.5 serve as three main parameterizations, while ACDC_DB_CE, ACDC_DB_BC, ACDC_DB_CN, and ACDC_RM are set as test cases to investigate the impact of individual simplification or theoretical approach on NPF simulations. In all comparisons, ACDC_DB is set as a reference.
2.4 Ambient measurements
In the 3-D simulations, we utilize the measured concentrations of nucleation precursors and PNSDs as a criterion to discuss the model performance with various parameterizations. The duration of the observational data matches that of the simulations mentioned above. Detailed descriptions of the observation site and instruments can be found in our previous research (Deng et al., 2020; Zhu et al., 2022). Briefly, the observation site is located on the west campus of the Beijing University of Chemical Technology. CI-TOF-MS (chemical ionization time-of-flight mass spectrometer; Aerodyne Research Inc.) devices were used to measure the concentrations of SA. Amine concentrations were measured with a modified TOF-MS using H3O+ or its clusters as the reagent ions (Zhu et al., 2022). PNSDs from 1 nm to 10 µm were measured using a PSD (particle size distribution) system and a DEG-SMPS (diethyl glycol scanning mobility particle spectrometer). J1.4 values derived from observation are calculated by employing an improved aerosol population balance formula (Cai and Jiang, 2017).
3.1 Comparison of different parameterizations based on box model simulations
3.1.1 Comparison between ACDC_DB and Dynamic_Sim
Figure 1 illustrates the comparison between the reported cluster-dynamics-based parameterization with simplifications, Dynamic_Sim, and the base case parameterization, ACDC_DB. The comparison is based on a comprehensive dataset that includes over 40 000 box model simulations for each parameterization by varying parameters such as [SA] (1×105–1×108 ), [DMA] (5×106–5×108 ), CS (– s−1), and T (250–320 K). In most scenarios, the J1.4 value predicted by ACDC_DB and Dynamic_Sim demonstrates deviations within 1 order of magnitude, with the majority falling within a factor of 3. However, Dynamic_Sim predicts notably higher J1.4 than ACDC_DB in scenarios where T exceeds ∼300 K, and CS is below s−1, characteristic of a clean atmosphere during summer. The discrepancy in these scenarios elevates the overall RDynamic_Sim up to 17.0. Furthermore, no clear correlation is observed between the differences in the two parameterizations and other input parameters such as [DMA] and [SA] (Fig. S2). The differences between the parameterizations are attributed to the combined effects of the three simplifications and the lower ΔG of (SA)1(DMA)1 cluster in Dynamic_Sim. However, the latter should not be the primary cause for the significant differences in the J1.4 prediction under high T and low CS conditions, as it typically results in an overestimation within an order of magnitude (R=3.3) (Fig. S1).
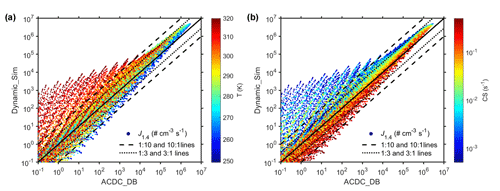
Figure 1Comparison of J1.4 predictions between ACDC_DB and Dynamic_Sim correlated with the T variation (a) and CS variation (b). Solid dots represent simulated J1.4 values, solid lines indicate a 1:1 line, dotted lines correspond to 1:3 and 3:1 lines, and dashed lines represent 1:10 and 10:1 lines.
The impacts of the three simplifications made in Dynamic_Sim are shown in Fig. 2. Specifically, the simplification in cluster evaporations tends to elevate the predicted J1.4, whereas the simplifications in boundary conditions and cluster number tend to lower them. When applying the simplification in cluster evaporations (clusters larger than (SA)1(DMA)1 are regarded stable with no evaporation) to ACDC_DB, the predicted J1.4 by ACDC_DB_CE only slightly exceed than that of ACDC_DB within a factor of 3 under conditions where K and s−1. However, the overestimation of J1.4 prediction by ACDC_DB_CE becomes much greater with increasing T and decreasing CS. The discrepancy between ACDC_DB_CE and ACDC_DB should be primarily attributed to the pivotal role of T in influencing cluster evaporation rates (Ortega et al., 2012; Deng et al., 2020). At low T, the evaporation rates of clusters are low enough to allow efficient nucleation; thus, setting the concerned SA–DMA clusters to evaporate based on the expected evaporation rates does not lead to a significant impact on the J1.4 prediction. However, at high T, the evaporation rates of clusters significantly increase; therefore, the simplification in cluster evaporations within ACDC_DB_CE is likely to predict higher J1.4 than those with no simplification. The impact of simplification in cluster evaporations across varying T is also found in a nonbranched SA–DMA nucleation scheme from 280 to 298 K reported by C. Li et al. (2023). Note also that the overestimation of ACDC_DB_CE diminishes as CS increases (Fig. 2d), with CS becoming the primary sink in the nucleation system and the impact of cluster evaporations becoming less pronounced. This underscores the connection between the specific deviation arising from simplification in cluster evaporations and the respective contributions of CS and cluster evaporations to the overall sink for clusters in nucleation. In addition, the relative independence of the differences between ACDC_DB_CE and ACDC_DB from variations in precursor concentrations ([SA] and [DMA]) is similar to that between Dynamic_Sim and ACDC_DB (Fig. S3). Overall, the scenarios in which ACDC_DB_CE predicts higher J1.4 than ACDC_DB only occur under conditions of both high T and low CS (Fig. 2a and d). The averaged discrepancy between ACDC_DB_CE and ACDC_DB RACDC_DB_CE is 22.3, closely resembling RDynamic_Sim, indicating that the simplification in cluster evaporations is a major factor contributing to the difference between Dynamic_Sim and ACDC_DB.
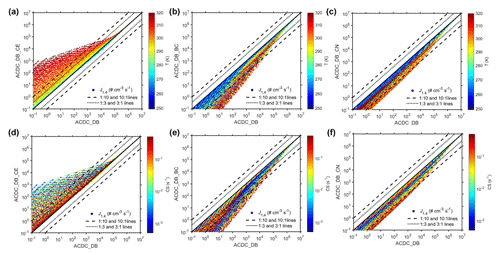
Figure 2Comparison of J1.4 predictions between ACDC_DB and test cases including ACDC_DB_CE (a, d), ACDC_DB_BC (b, e), and ACDC_DB_CN (c, f). The first row in panels (a)–(c) is correlated with T variation and the second row (d–f) is correlated with CS variation. Solid dots represent simulated J1.4 values, solid lines indicate a 1:1 line, dotted lines correspond to 1:3 and 3:1 lines, and dashed lines represent 1:10 and 10:1 lines.
The underestimations of ACDC_DB_BC and ACDC_DB_CN in the J1.4 prediction compared to base case ACDC_DB are related to the growth pathways of SA–DMA clusters. In the original scheme of ACDC_DB, precursor molecules have the flexibility to pass through any (SA)m(DMA)n clusters (), and terminal 1.4 nm particles are formed when the clusters grow to (SA)4(DMA)4 or (SA)4(DMA)3. As expected, ACDC_DB_BC, which assumes the (SA)4(DMA)4 cluster as the only boundary condition with an omission of the (SA)4(DMA)3 cluster, predicts lower J1.4 than ACDC_DB. (SA)4(DMA)3 and (SA)4(DMA)4 clusters are primarily formed from the (SA)3(DMA)3 cluster by colliding with a SA molecule and a (SA)1(DMA)1 cluster, respectively. As the concentration of the (SA)1(DMA)1 cluster is more sensitive to T, we further found that the discrepancy between ACDC_DB_BC and ACDC_DB becomes more pronounced with increasing T (Fig. 2b). Furthermore, we found no apparent correlation between the variation in CS and the disparity between ACDC_DB_BC and ACDC_DB (Fig. 2e).
In addition to ACDC_DB_BC, ACDC_DB_CN also underestimates J1.4 compared to ACDC_DB with a comparable value (∼0.5) of RACDC_DB_CN and RACDC_DB_BC. Under the simplification in cluster number, the formation of 1.4 nm clusters can only occur through specific pathways, including (SA)1(DMA)1 → (SA)2(DMA)2 → (SA)3(DMA)3 → (SA)4(DMA)4/(SA)4(DMA)3, (SA)1(DMA)1 → (SA)2(DMA)1 → (SA)2(DMA)2 → (SA)3(DMA)3 → (SA)4(DMA)4/(SA)4(DMA)3, or a combination thereof, while other pathways are restricted. Due to the variability in growth pathways and their contributions to J1.4 under different atmospheric conditions, the difference between ACDC_DB_CN and ACDC_DB is not strongly correlated with the variations in T and CS (Fig. 2c and f). Despite that, while the differences between the two tested parameterizations (ACDC_DB_BC and ACDC_DB_CN) involving cluster growth pathways and the original ACDC_DB are not highly correlated with [DMA], there is a more pronounced correlation with [SA], which implies a more important role of SA in cluster growth (Figs. S4 and S5).
In our previous study, we demonstrated improvements in computing the CS-dependent J1.4 of SA–DMA nucleation with the Dynamic_Sim compared to the previous power-law parameterizations under polluted atmospheric conditions (Y. Li et al., 2023). Here, we further show that, based on Dynamic_Sim, the new ACDC_DB with complete cluster dynamics can more reasonably simulate J1.4 under previously less studied conditions of high T ( K) and low CS ( s−1), where Dynamic_Sim tends to produce a significant overestimation of J1.4. This overestimation is primarily driven by the simplification in cluster evaporations within Dynamic_Sim. Even though a comparable performance in the J1.4 prediction between ACDC_DB and Dynamic_Sim could be achieved under other ambient conditions, caution should be taken to ensure that the mutual offsetting effect between overestimation and underestimation results from different simplifications in Dynamic_Sim when computing J1.4.
3.1.2 Comparison between ACDC_DB and ACDC_RM_SF0.5
In Fig. 3, ACDC_DB is compared with another main ACDC-derived parameterization, ACDC_RM_SF0.5, which uses the RI-CC2/aug-cc-pV(T+d)Z//M06-2X/6-311G(3df,3pd) level of theory and employs a SF of 0.5 in processing collision. It can be observed that at lower temperatures (∼280 K), ACDC_RM_SF0.5 and ACDC_DB exhibit a similar performance in predicting J1.4. However, with higher T (accompanied by lower CS with a slight dependency), J1.4 values predicted by ACDC_RM_SF0.5 become higher than that predicted by ACDC_DB, reaching even several orders of magnitude at the upper limit of the T range (320 K). Furthermore, we also observed that in scenarios close to the lower limit of the T range (250 K), the J1.4 values predicted by ACDC_RM_SF0.5 shift from being higher to lower compared to ACDC_DB.
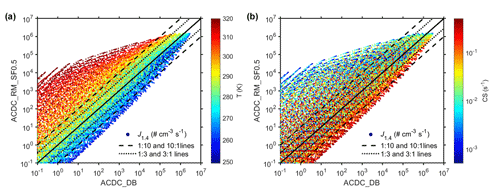
Figure 3Comparison of J1.4 predictions between ACDC_DB and ACDC_RM_SF0.5 correlated with T variation (a) and CS variation (b). Solid dots represent simulated J1.4 values, solid lines indicate a 1:1 line, dotted lines correspond to 1:3 and 3:1 lines, and dashed lines represent 1:10 and 10:1 lines.
The distinction between ACDC_RM_SF0.5 and ACDC_DB arises from the combined effects of the variation in the quantum chemical calculation method and the application of the 0.5 SF in collision processing. As depicted in Fig. 4, when the SF in ACDC_RM_SF0.5 is set to unity as in ACDC_DB, the resulting ACDC_RM parameterization predicts consistently higher J1.4 values than ACDC_DB. This implies that the modified quantum chemical calculation method, which results in lower evaporation rates for clusters within the system compared to ACDC_DB under the same condition, leads to higher J1.4 predictions. The impact from varying the quantum chemical calculation method is akin to that from simplification in cluster evaporations discussed earlier. The distinction between ACDC_RM and ACDC_DB_CE lies in the fact that the modified quantum chemical calculation method affects all clusters within the system, whereas the simplification in cluster evaporations is specific to the limited clusters. This contributes to a much higher RACDC_RM (614.5) compared to RACDC_DB_CE (22.3). Despite that, compared to ACDC_DB, the differences for both ACDC_DB_CE and ACDC_RM, as well as ACDC_RM_SF0.5, demonstrate similar sensitivity to T (Figs. 3a and 4a) and CS (Figs. 3b and 4b) but demonstrate independence on [SA] (Figs. S6A and S7A) and [DMA] (Figs. S6B and S7B). Comparing ACDC_RM_SF0.5 and ACDC_RM, it can be inferred that the application of a 0.5 SF in collision processes would result in an underestimation in the J1.4 prediction. It can be noted that in most previous studies (Almeida et al., 2013; Kürten et al., 2018; Elm et al., 2020), comparisons of ACDC simulations using the traditional method and measured particle formation rates are conducted at around 280 K. At this temperature, all three main parameterizations of ACDC_RM_SF0.5, ACDC_DB, and Dynamic_Sim tend to yield similar J1.4 predictions and should have consistent applicability in the NPF simulation.
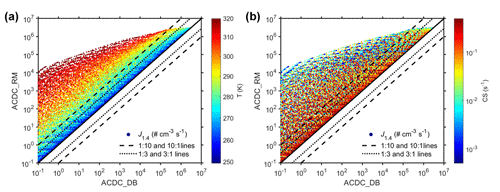
Figure 4Comparison of J1.4 predictions between ACDC_DB and ACDC_RM correlated with a T variation (a) and a CS variation (b). Solid dots represent simulated J1.4 values, solid lines indicate a 1:1 line, dotted lines correspond to 1:3 and 3:1 lines, and dashed lines represent 1:10 and 10:1 lines.
In summary, based on our base case parameterization ACDC_DB, the extensive box model simulations above demonstrate the characteristics of different parameterizations. Specifically, Dynamic_Sim shows general consistency with ACDC_DB in simulating J1.4 under most atmospheric conditions with K or s−1, while overestimating J1.4 with K and s−1 compared to ACDC_DB. ACDC_RM_SF0.5 performs similarly to ACDC_DB under conditions of ∼280 K but gives different J1.4 predictions at other temperatures. We further use reported measurements from well-controlled CLOUD chamber experiments to examine the characteristics and applicability of these parameterizations (Xiao et al., 2021). As shown in Fig. S8, simulated J1.4 values using three main parameterizations, ACDC_DB, ACDC_RM_SF0.5, and Dynamic_Sim, correspond well to measured J1.7 values at a low temperature (T=278 K), proving the applicability of all three parameterizations at this temperature. In the experiments with elevated temperature (T=293 K), ACDC_DB and Dynamic_Sim continue to exhibit a similar performance, with a slight overestimation by approximately 2 factors. This may be because the much lower cluster concentrations at high temperatures compared to those at low temperatures lead to slower cluster growth and thus an enlarged gap between J1.4 and J1.7 (Fig. S9). In contrast, ACDC_RM_SF0.5 only shows a slight T-dependence, which is a deviation from the measurements. The comparison between controlled experiments and box model simulations hence confirms our conclusions above and provides a solid basis for further discussions on 3-D simulations using these parameterizations with constraints from field observations.
3.2 Comparison of different parameterizations based on 3-D model simulations
Various cluster-dynamics-based parameterizations for SA–DMA nucleation were subsequently integrated into the WRF-Chem/R2D-VBS model. 3-D simulations using these parameterizations have been conducted for both wintertime and summertime conditions in Beijing. Given that the concentrations of precursors are crucial input variables for each parameterization, the simulated and observed concentrations of [DMA] and [SA] are compared. Figures S10 and S11 and Table S2 illustrates good consistencies in the temporal variations and the mean values between simulations and observations in Beijing. This validates the reliability of our representation of sources and sinks for nucleating precursors and serves as a foundation for our discussions on the performances of various parameterizations. In the following sections, we discuss the results of 3-D NPF simulations in Beijing during winter and summer by employing different parameterizations. The evaluation of various parameterizations focuses on their ability to reproduce in situ NPF measurements across different seasons.
3.2.1 Wintertime simulations
Figures 5a and S12A primarily compare the simulated J1.4 values from different parameterizations with those derived from wintertime observations in Beijing, as J1.4 is a key parameter describing NPF events. The performance of Dynamic_Sim in simulating J1.4 during wintertime Beijing has been discussed in our previous study (Y. Li et al., 2023). The averaged J1.4 simulated by three main parameterizations (Dynamic_Sim: 64.0 ; ACDC_DB: 51.6 ; ACDC_RM_SF0.5: 54.5 ) approximate the observation (46.7 ). For test cases, however, only ACDC_DB_CE (55.7 ) demonstrates a reasonable representation of J1.4. J1.4 values simulated from ACDC_DB_BC (20.5 ) and ACDC_DB_CN (20.8 ) are approximately 2 times lower than the observed values, while ACDC_RM (226.2 ) is approximately 5 times higher than the observations.
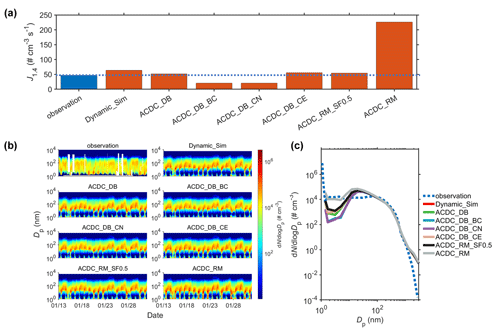
Figure 5Comparison of simulated particle formation rates and particle number size distributions (PNSDs) with observations during 13 to 31 January 2019 in Beijing. Panel (a) represents the averaged particle formation rates during the period; the blue bars and orange bars represent observations and simulations, respectively, while the dashed blue line represents the observed values. Daily maximum values of J1.4 are used, following Deng et al. (2020). (b) The time series of PNSDs. (c) The averaged PNSDs.
The performances of different parameterizations for depicting J1.4 subsequently influences their representations of PNSDs evolution and NPF events, which are shown in Fig. 5b. Generally, most parameterizations efficiently reproduce the observed time evolution of PNSDs and capture NPF events, such as those on 20, 21, 30, and 31 January, which are characterized by the burst of aerosol number concentrations in the nanometer-sized range. Simulations using ACDC_DB_BC and ACDC_DB_CN result in lower particle concentrations in the low size range (1–10 nm) during the NPF period compared to three main parameterizations and the observations, while simulations with ACDC_RM show higher concentrations. This is consistent with the comparison of J1.4 among different parameterizations and further evidenced by the comparison of averaged PNSDs in Fig. 5c. Notably, when compared to observations, all parameterizations consistently underestimate the averaged PNSDs within the 2–10 nm range but overestimate them in the 10–50 nm range. This discrepancy may stem from simplified assumptions in the particle growth simulation, as discussed in our previous study (Y. Li et al., 2023).
The results show the applicability of all three main parameterizations in NPF modeling during wintertime periods. Importantly, the reliability of the new ACDC-derived parameterization based on the latest theoretical approach (ACDC_DB) without simplifications in 3-D NPF simulation is affirmed. The differences among various parameterizations can be explained by the comprehensive box model simulations mentioned above at corresponding conditions. Compared to ACDC_DB, the J1.4 and PNSDs simulated by other two main parameterizations (Dynamic_Sim and ACDC_RM_SF0.5) agree similarly with the observations but for different reasons. In the case of Dynamic_Sim, the simplification in cluster evaporations has a minimal impact on the NPF simulation, since CS is the dominant sink for clusters under wintertime conditions (averaged T and CS values are 274.7 K and s−1, respectively). However, the simplifications in boundary conditions and cluster number lead to the underestimation of the J1.4, consequently lowering the simulated particle number concentrations in 1–100 nm size range due to the ignorance of clusters contributing to growth. As a result, the agreement of Dynamic_Sim to observations should result from a combination of the underestimation due to the simplifications in boundary conditions and cluster number, along with the compensatory effect of the overestimation caused by lower ΔG for (SA)1(DMA)1 cluster. For another main parameterization ACDC_RM_SF0.5, since the test parameterization ACDC_RM considerably overestimates J1.4 and PNSDs compared to the observations, the general agreement between ACDC_RM_SF0.5 and observations should be attributed to a balance between a reduced kinetic limit through the application of SF and the compensatory effect of the overestimation caused by inappropriate representation of cluster thermodynamics.
3.2.2 Summertime simulations
Figure 6 provides additional insight into the performance of various parameterizations in NPF simulation during summer. It can be noted that a significant difference exists in the particle formation rates between winter and summer in Beijing. As shown in Figs. 6 and S12B, ACDC_DB and Dynamic_Sim continues to demonstrate a consistent and effective performance in simulating J1.4 (within a factor of 2), PNSDs evolution, and NPF events. However, distinct differences emerge in the NPF simulation for other parameterizations, including another main parameterization ACDC_RM_SF0.5. Specifically, in contrast to the good performance of ACDC_DB and Dynamic_Sim, ACDC_RM_SF0.5, along with the test case ACDC_RM, exhibits a significant overestimation of J1.4, exceeding the observations by more than 15 times and over 2 orders of magnitude, respectively. This aligns with their overestimation of NPF occurrences and particle number concentration in the size range of 1–100 nm in comparison to observation, with a more pronounced overestimation for ACDC_RM. Conversely, the test cases of ACDC_DB_BC and ACDC_DB_CN show an underestimation of averaged J1.4 by approximately 4–5 times. They almost fail to depict NPF events, resulting in a significant underestimation of number concentrations in the 1–100 nm size range. Simulations using ACDC_DB_CE notably overestimate J1.4, especially on 28–31 August (Fig. S11B), resulting in an overestimation of averaged J1.4 by approximately 4 times compared to the observations. However, apart from a moderate overestimation in the initial particle size, we can observe a closer alignment of the particle number concentrations in the 2–50 nm range with observations for ACDC_DB_CE, which should result from a combination of surplus newly formed particles and fast particle growth from inadequate assumptions within the model. For the broader 2–100 nm size range, it can be observed that ACDC_DB and Dynamic_Sim are closer to the observations compared to ACDC_DB_CE and another major parameterization ACDC_RM_SF0.5 (Fig. S13). The latter two overestimate the average number concentrations during the simulation period by 1.6 times and 2.5 times, respectively. Given the more accurate representation of nucleation rates by ACDC_DB and Dynamic_Sim, the discrepancies in the 2–100 nm size range compared to the observed PNSDs should also stem from the simplified assumptions in particle growth simulations.
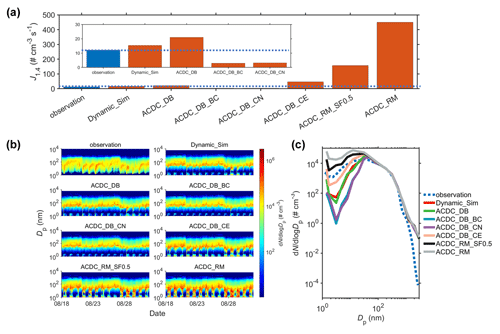
Figure 6Comparison of simulated particle formation rates and particle number size distributions (PNSDs) with observations during 18 to 31 August 2019 in Beijing. Panel (a) represents the averaged particle formation rates during the period; the blue bars and orange bars represent observations and simulations, respectively, while the dashed blue line represents the observed values. Daily maximum values of J1.4 are used following Deng et al. (2020). (b) The time series of PNSDs. (c) The averaged PNSDs.
Most previous NPF studies combining experiments/observations with simulations are conducted under conditions biased towards winter (∼280 K) (Almeida et al., 2013; Lu et al., 2020). Under summer conditions with elevated T, a deficiency in the parameterization evaluations exists for the simulation of NPF. The 3-D simulation results during the summer period provide additional validation for the reliability of ACDC_DB. For ACDC_RM_SF0.5, evidence from both box model simulations and 3-D simulations suggests that it can accurately reproduce real SA–DMA nucleation at temperatures around 280 K, while it has limitations in higher temperatures. Another main parameterization Dynamic_Sim consistently demonstrates a good performance in NPF simulation, akin to its efficacy in winter conditions. With the increased temperature in summer (averaged T is 298.2 K), the influence of simplifications in cluster evaporations, cluster number, and boundary conditions becomes more profound, mirroring the trends observed in box model simulations above. This leads to more significant overestimation for ACDC_DB_CE and underestimation for ACDC_DB_CN and ACDC_DB_BC compared to the observation, as well as the base case ACDC_DB. Note that CS during the summer period (averaged CS is s−1) decreases compared to winter but remains significantly higher than the typical values in clean regions ( s−1) (Dal Maso et al., 2008). According to the limited conditions for Dynamic_Sim described above, although the overestimation of the J1.4 prediction resulting from the simplification in the cluster evaporations is more pronounced in summer compared to that in winter, impacts from diverse overestimations and underestimations from different simplifications and varied thermodynamics for (SA)1(DMA)1 cluster can still offset each other, thereby allowing Dynamic_Sim to match observations. Based on previous comparisons using box models, significant differences in J1.4 predictions between Dynamic_Sim and ACDC_DB only exist under conditions of high K and low s−1; thus, a similar performance of Dynamic_Sim and ACDC_DB can be expected in the polluted atmosphere ( s−1). In a clean atmosphere with high temperature, however, caution is advised when using Dynamic_Sim for 3-D NPF simulations.
By integrating box modeling, 3-D simulations, also under the constraint from in situ measurements, this study conducts comprehensive comparison of different cluster-dynamics-based parameterizations for SA–DMA nucleation. Among them, the ACDC-derived parameterization grounded in the latest molecular-level understanding and complete representation of cluster dynamics (ACDC_DB) is identified to effectively model particle formation rates and PNSD evolution in both winter and summer in Beijing within 3-D simulations. While a previously proposed simplified cluster-dynamics-based parameterization (Dynamic_Sim) performs comparably in modeling NPF in Beijing, analysis reveals that their similarity arises from a delicate balance between overestimation and underestimation due to simplifications in cluster dynamics processes and the difference in thermodynamics of the initial cluster. Particularly, under specific conditions of high temperature ( K) and low CS ( s−1), Dynamic_Sim tends to make a significant overestimation of particle formation rates compared to the reality. Moreover, the study furnishes evidence that integrating ACDC-derived parameterizations with the traditional theoretical approach RI-CC2/aug-cc-pV(T+d)Z//M06-2X/6-311G(3df,3pd) (ACDC_RM_SF0.5) effectively captures particle formation rates and the evolution of PNSDs around 280 K, a temperature range frequently explored in prior experiments and simulations investigating NPF (Kirkby et al., 2011, 2016; Almeida et al., 2013; Xie et al., 2017; He et al., 2021; Ma et al., 2019). Therefore, ACDC_RM_SF0.5 exhibits consistent applicability as seen for the other two parameterizations at around ∼280 K. However, when attributed to an inappropriate representation of cluster thermodynamics, ACDC_RM_SF0.5 has limitations in predicting particle formation rates at elevated temperatures. Overall, considering all aspects, we recommend ACDC_DB as a more reliable parameterization for simulating NPF across various atmospheric environments.
In addition to contributing to a more reasonable 3-D modeling of NPF, our research further provides valuable references for the development of the parameterizations for other nucleation systems. First, we demonstrate the efficacy of the DLPNO-CCSD(T)/aug-cc-pVTZ//ωB97X-D/6-311G(3df,3pd) level of theory in describing the thermodynamic properties of SA–DMA clusters through comprehensive evidence. This approach can thus be referenced when using quantum chemical calculations to obtain thermodynamic data for other nucleation clusters, especially for other alkylamines such as methylamine-/trimethylamine-sulfuric acid clusters. Although the DLPNO method still has uncertainties with respect to accurately describing cluster thermodynamics (Besel et al., 2020), it is well recognized as the best method currently available (Elm et al., 2020). Besides, in some qualitative studies, e.g., comparing the enhancing potential or synergistic effects of different precursors in SA-driven nucleation, methods other than DLPNO-CCSD(T)/aug-cc-pVTZ//ωB97X-D/6-311G(3df,3pd), such as RI-CC2/aug-cc-pV(T+d)Z//M06-2X/6-311G(3df,3pd), are equally valid (Liu et al., 2019).
Comprehensive modeling evidence is provided in this study that certain simplifications or assumptions in cluster dynamics, such as reducing the number of expected clusters, modifying boundary conditions, and assuming certain clusters to be non-evaporative, can significantly impact the prediction of particle formation rates and hence alter the 3-D NPF simulation under certain conditions. While applying certain simplifications concurrently under specific ambient conditions can offset different influences against each other, leading to a satisfactory model-observation comparison, there is a risk that certain simplifications may drive the model's outcomes away from reality when environmental conditions change. Therefore, caution should be exercised when applying these simplifications in the derivation of nucleation parameterizations and subsequent application in 3-D models. In addition to the simplifications within the cluster dynamics regime, it should be noted that the current standard treatments in 3-D models that ignore detailed gas–cluster–aerosol interactions may also lead to biases under certain atmospheric conditions (Olenius and Roldin, 2022). This applies not only to parameterizations involving explicit mathematical expressions but also to those using ACDC-derived lookup tables. Additional evaluations for the SA–DMA system indicate that the impacts of these treatments may be highest under a combination of low temperature ( K), low CS ( s1), and low precursor concentrations, leading to elevated time to reach a steady state and a higher proportion of precursor consumption from cluster formation, as also indicated by the study of Olenius and Roldin (2022). Despite these impacts being generally limited under most atmospheric conditions in our modeling scenarios (see the Supplement), further research, especially using computationally lightweight models, should aim to circumvent the potential bias by linking the cluster and aerosol dynamics (Olenius and Roldin, 2022).
It is recognized that the development of cluster-dynamics-based nucleation parameterizations in the form of explicit mathematical expressions is subject to limitations, especially for systems involving multiple precursor species (Semeniuk and Dastoor, 2018). Given that the original ACDC has been extended to involve more than two precursor species, the ACDC-derived parameterization framework, in the form of a lookup table, is highly meaningful for establishing parameterizations for these multi-component nucleation systems. Given that multiple nucleation pathways may be simultaneously considered and simulated in 3-D modeling through ACDC-derived lookup tables, automatized incorporation of tables is needed through useful tools such as J-GAIN, which was developed recently (Yazgi and Olenius, 2023).
SA | Sulfuric acid |
DMA | Dimethylamine |
ACDC | Atmospheric Cluster Dynamic Code |
DB | DLPNO-CCSD(T)/aug-cc-pVTZ//ωB97X-D/6-311G(3df,3pd) level of theory |
RM | RI-CC2/aug-cc-pV(T+d)Z//M06-2X/6–311G(3df,3pd) level of theory |
CE | Simplification in cluster evaporations (only (SA)k(DMA)k (k=1–4) and (SA)2(DMA)1 clusters are considered) |
CN | Simplification in cluster number (clusters larger than (SA)1(DMA)1 are regarded stable with no evaporation) |
BC | Simplification in boundary conditions ((SA)4(DMA)4 cluster is set as the only terminal cluster in calculating particle formation rates) |
SF | Sticking factor used in collision process |
Dynamic_Sim | A reported cluster-dynamic-based parameterization incorporating simplifications of CE, CN, and BC. |
J1.4 | Particle formation rate at 1.4 nm |
R | A parameter to quantify the differences in simulating J1.4 among different cluster-dynamics-based parameterizations compared to the base case ACDC_DB |
The data and code used in this study are available upon request from the corresponding author. ACDC code can be accessed at https://github.com/tolenius/ACDC (Olenius, 2024).
The supplement related to this article is available online at: https://doi.org/10.5194/acp-24-10261-2024-supplement.
JS, BZ, and SW designed the research. AN and XZ collected the quantum chemistry calculation data. JS performed the ACDC and WRF-Chem/R2D-VBS simulations. YL, RC, and JJ collected the observational data. JS, BZ, and SW analyzed the data. RC, DG, JJ, YG, MS, BC, and HH presented important suggestions for the paper. JS, BZ, and SW wrote the paper with input from all co-authors.
At least one of the (co-)authors is a member of the editorial board of Atmospheric Chemistry and Physics. The peer-review process was guided by an independent editor, and the authors also have no other competing interests to declare.
Publisher's note: Copernicus Publications remains neutral with regard to jurisdictional claims made in the text, published maps, institutional affiliations, or any other geographical representation in this paper. While Copernicus Publications makes every effort to include appropriate place names, the final responsibility lies with the authors.
We are grateful for the helpful community comments from Tinja Olenius.
This study has been supported by the National Natural Science Foundation of China (grant nos. 22188102 and 42275110) and the Samsung Advanced Institute of Technology.
This paper was edited by Zhibin Wang and reviewed by two anonymous referees.
Almeida, J., Schobesberger, S., Kurten, A., Ortega, I. K., Kupiainen-Maatta, O., Praplan, A. P., Adamov, A., Amorim, A., Bianchi, F., Breitenlechner, M., David, A., Dommen, J., Donahue, N. M., Downard, A., Dunne, E., Duplissy, J., Ehrhart, S., Flagan, R. C., Franchin, A., Guida, R., Hakala, J., Hansel, A., Heinritzi, M., Henschel, H., Jokinen, T., Junninen, H., Kajos, M., Kangasluoma, J., Keskinen, H., Kupc, A., Kurten, T., Kvashin, A. N., Laaksonen, A., Lehtipalo, K., Leiminger, M., Leppa, J., Loukonen, V., Makhmutov, V., Mathot, S., McGrath, M. J., Nieminen, T., Olenius, T., Onnela, A., Petaja, T., Riccobono, F., Riipinen, I., Rissanen, M., Rondo, L., Ruuskanen, T., Santos, F. D., Sarnela, N., Schallhart, S., Schnitzhofer, R., Seinfeld, J. H., Simon, M., Sipila, M., Stozhkov, Y., Stratmann, F., Tome, A., Trostl, J., Tsagkogeorgas, G., Vaattovaara, P., Viisanen, Y., Virtanen, A., Vrtala, A., Wagner, P. E., Weingartner, E., Wex, H., Williamson, C., Wimmer, D., Ye, P., Yli-Juuti, T., Carslaw, K. S., Kulmala, M., Curtius, J., Baltensperger, U., Worsnop, D. R., Vehkamaki, H., and Kirkby, J.: Molecular understanding of sulphuric acid-amine particle nucleation in the atmosphere, Nature, 502, 359–363, https://doi.org/10.1038/nature12663, 2013.
Baranizadeh, E., Murphy, B. N., Julin, J., Falahat, S., Reddington, C. L., Arola, A., Ahlm, L., Mikkonen, S., Fountoukis, C., Patoulias, D., Minikin, A., Hamburger, T., Laaksonen, A., Pandis, S. N., Vehkamäki, H., Lehtinen, K. E. J., and Riipinen, I.: Implementation of state-of-the-art ternary new-particle formation scheme to the regional chemical transport model PMCAMx-UF in Europe, Geosci. Model Dev., 9, 2741–2754, https://doi.org/10.5194/gmd-9-2741-2016, 2016.
Bergman, T., Laaksonen, A., Korhonen, H., Malila, J., Dunne, E. M., Mielonen, T., Lehtinen, K. E. J., Kühn, T., Arola, A., and Kokkola, H.: Geographical and diurnal features of amine-enhanced boundary layer nucleation, J. Geophys. Res.-Atmos., 120, 9606–9624, https://doi.org/10.1002/2015jd023181, 2015.
Besel, V., Kubecka, J., Kurten, T., and Vehkamaki, H.: Impact of Quantum Chemistry Parameter Choices and Cluster Distribution Model Settings on Modeled Atmospheric Particle Formation Rates, J. Phys. Chem. A, 124, 5931–5943, https://doi.org/10.1021/acs.jpca.0c03984, 2020.
Brasseur, G. P. and Jacob, D. J.: Model Equations and Numerical Approaches, in: Modeling of Atmospheric Chemistry, Cambridge University Press, 84–204, ISBN 9781316544754, https://doi.org/10.1017/9781316544754, 2017.
Cai, R. and Jiang, J.: A new balance formula to estimate new particle formation rate: reevaluating the effect of coagulation scavenging, Atmos. Chem. Phys., 17, 12659–12675, https://doi.org/10.5194/acp-17-12659-2017, 2017.
Cai, R., Yan, C., Yang, D., Yin, R., Lu, Y., Deng, C., Fu, Y., Ruan, J., Li, X., Kontkanen, J., Zhang, Q., Kangasluoma, J., Ma, Y., Hao, J., Worsnop, D. R., Bianchi, F., Paasonen, P., Kerminen, V.-M., Liu, Y., Wang, L., Zheng, J., Kulmala, M., and Jiang, J.: Sulfuric acid–amine nucleation in urban Beijing, Atmos. Chem. Phys., 21, 2457–2468, https://doi.org/10.5194/acp-21-2457-2021, 2021.
Cai, R., Yin, R., Li, X., Xie, H.-B., Yang, D., Kerminen, V.-M., Smith, J. N., Ma, Y., Hao, J., Chen, J., Kulmala, M., Zheng, J., Jiang, J., and Elm, J.: Significant contributions of trimethylamine to sulfuric acid nucleation in polluted environments, npj Climate and Atmospheric Science, 6, 75, https://doi.org/10.1038/s41612-023-00405-3, 2023.
Chu, B., Kerminen, V.-M., Bianchi, F., Yan, C., Petäjä, T., and Kulmala, M.: Atmospheric new particle formation in China, Atmos. Chem. Phys., 19, 115–138, https://doi.org/10.5194/acp-19-115-2019, 2019.
Croft, B., Wentworth, G. R., Martin, R. V., Leaitch, W. R., Murphy, J. G., Murphy, B. N., Kodros, J. K., Abbatt, J. P. D., and Pierce, J. R.: Contribution of Arctic seabird-colony ammonia to atmospheric particles and cloud-albedo radiative effect, Nat. Commun., 7, 13444, https://doi.org/10.1038/ncomms13444, 2016.
Dal Maso, M., Hyvärinen, A., Komppula, M., Tunved, P., Kerminen, V.-M., Lihavainen, H., Viisanen, Y., Hansson, H.-C., and Kulmala, M.: Annual and interannual variation in boreal forest aerosol particle number and volume concentration and their connection to particle formation, Tellus B, 60, 495–508, https://doi.org/10.1111/j.1600-0889.2008.00366.x, 2008.
Deng, C., Fu, Y., Dada, L., Yan, C., Cai, R., Yang, D., Zhou, Y., Yin, R., Lu, Y., Li, X., Qiao, X., Fan, X., Nie, W., Kontkanen, J., Kangasluoma, J., Chu, B., Ding, A., Kerminen, V. M., Paasonen, P., Worsnop, D. R., Bianchi, F., Liu, Y., Zheng, J., Wang, L., Kulmala, M., and Jiang, J.: Seasonal Characteristics of New Particle Formation and Growth in Urban Beijing, Environ. Sci. Technol., 54, 8547–8557, https://doi.org/10.1021/acs.est.0c00808, 2020.
Dunne, E. M., Gordon, H., Kurten, A., Almeida, J., Duplissy, J., Williamson, C., Ortega, I. K., Pringle, K. J., Adamov, A., Baltensperger, U., Barmet, P., Benduhn, F., Bianchi, F., Breitenlechner, M., Clarke, A., Curtius, J., Dommen, J., Donahue, N. M., Ehrhart, S., Flagan, R. C., Franchin, A., Guida, R., Hakala, J., Hansel, A., Heinritzi, M., Jokinen, T., Kangasluoma, J., Kirkby, J., Kulmala, M., Kupc, A., Lawler, M. J., Lehtipalo, K., Makhmutov, V., Mann, G., Mathot, S., Merikanto, J., Miettinen, P., Nenes, A., Onnela, A., Rap, A., Reddington, C. L., Riccobono, F., Richards, N. A., Rissanen, M. P., Rondo, L., Sarnela, N., Schobesberger, S., Sengupta, K., Simon, M., Sipila, M., Smith, J. N., Stozkhov, Y., Tome, A., Trostl, J., Wagner, P. E., Wimmer, D., Winkler, P. M., Worsnop, D. R., and Carslaw, K. S.: Global atmospheric particle formation from CERN CLOUD measurements, Science, 354, 1119–1124, https://doi.org/10.1126/science.aaf2649, 2016.
Elm, J., Bilde, M., and Mikkelsen, K. V.: Assessment of binding energies of atmospherically relevant clusters, Phys. Chem. Chem. Phys., 15, 16442–16445, https://doi.org/10.1039/c3cp52616j, 2013.
Elm, J., Kubečka, J., Besel, V., Jääskeläinen, M. J., Halonen, R., Kurtén, T., and Vehkamäki, H.: Modeling the formation and growth of atmospheric molecular clusters: A review, J. Aerosol Sci., 149, 105621, https://doi.org/10.1016/j.jaerosci.2020.105621, 2020.
Gao, D., Zhao, B., Wang, S. X., Shen, J. W., Wang, Y., Zhou, C., Jiang, J. K., Wu, Q. R., Li, S. Y., Sun, Y. S., He, Y. C., Zhu, Y., and Jiang, Z.: Distinct PM2.5-Related Near-Term Climate Penalties Induced by Different Clean Air Measures in China, Geophys. Res. Lett., 51, e2024GL108204, https://doi.org/10.1029/2024gl108204, 2024.
Gordon, H., Sengupta, K., Rap, A., Duplissy, J., Frege, C., Williamson, C., Heinritzi, M., Simon, M., Yan, C., Almeida, J., Trostl, J., Nieminen, T., Ortega, I. K., Wagner, R., Dunne, E. M., Adamov, A., Amorim, A., Bernhammer, A. K., Bianchi, F., Breitenlechner, M., Brilke, S., Chen, X., Craven, J. S., Dias, A., Ehrhart, S., Fischer, L., Flagan, R. C., Franchin, A., Fuchs, C., Guida, R., Hakala, J., Hoyle, C. R., Jokinen, T., Junninen, H., Kangasluoma, J., Kim, J., Kirkby, J., Krapf, M., Kurten, A., Laaksonen, A., Lehtipalo, K., Makhmutov, V., Mathot, S., Molteni, U., Monks, S. A., Onnela, A., Perakyla, O., Piel, F., Petaja, T., Praplan, A. P., Pringle, K. J., Richards, N. A., Rissanen, M. P., Rondo, L., Sarnela, N., Schobesberger, S., Scott, C. E., Seinfeld, J. H., Sharma, S., Sipila, M., Steiner, G., Stozhkov, Y., Stratmann, F., Tome, A., Virtanen, A., Vogel, A. L., Wagner, A. C., Wagner, P. E., Weingartner, E., Wimmer, D., Winkler, P. M., Ye, P., Zhang, X., Hansel, A., Dommen, J., Donahue, N. M., Worsnop, D. R., Baltensperger, U., Kulmala, M., Curtius, J., and Carslaw, K. S.: Reduced anthropogenic aerosol radiative forcing caused by biogenic new particle formation, P. Natl. Acad. Sci. USA, 113, 12053–12058, https://doi.org/10.1073/pnas.1602360113, 2016.
Guenther, A., Karl, T., Harley, P., Wiedinmyer, C., Palmer, P. I., and Geron, C.: Estimates of global terrestrial isoprene emissions using MEGAN (Model of Emissions of Gases and Aerosols from Nature), Atmos. Chem. Phys., 6, 3181–3210, https://doi.org/10.5194/acp-6-3181-2006, 2006.
He, X. C., Tham, Y. J., Dada, L., Wang, M., Finkenzeller, H., Stolzenburg, D., Iyer, S., Simon, M., Kurten, A., Shen, J., Rorup, B., Rissanen, M., Schobesberger, S., Baalbaki, R., Wang, D. S., Koenig, T. K., Jokinen, T., Sarnela, N., Beck, L. J., Almeida, J., Amanatidis, S., Amorim, A., Ataei, F., Baccarini, A., Bertozzi, B., Bianchi, F., Brilke, S., Caudillo, L., Chen, D., Chiu, R., Chu, B., Dias, A., Ding, A., Dommen, J., Duplissy, J., El Haddad, I., Gonzalez Carracedo, L., Granzin, M., Hansel, A., Heinritzi, M., Hofbauer, V., Junninen, H., Kangasluoma, J., Kemppainen, D., Kim, C., Kong, W., Krechmer, J. E., Kvashin, A., Laitinen, T., Lamkaddam, H., Lee, C. P., Lehtipalo, K., Leiminger, M., Li, Z., Makhmutov, V., Manninen, H. E., Marie, G., Marten, R., Mathot, S., Mauldin, R. L., Mentler, B., Mohler, O., Muller, T., Nie, W., Onnela, A., Petaja, T., Pfeifer, J., Philippov, M., Ranjithkumar, A., Saiz-Lopez, A., Salma, I., Scholz, W., Schuchmann, S., Schulze, B., Steiner, G., Stozhkov, Y., Tauber, C., Tome, A., Thakur, R. C., Vaisanen, O., Vazquez-Pufleau, M., Wagner, A. C., Wang, Y., Weber, S. K., Winkler, P. M., Wu, Y., Xiao, M., Yan, C., Ye, Q., Ylisirnio, A., Zauner-Wieczorek, M., Zha, Q., Zhou, P., Flagan, R. C., Curtius, J., Baltensperger, U., Kulmala, M., Kerminen, V. M., Kurten, T., Donahue, N. M., Volkamer, R., Kirkby, J., Worsnop, D. R., and Sipila, M.: Role of iodine oxoacids in atmospheric aerosol nucleation, Science, 371, 589–595, https://doi.org/10.1126/science.abe0298, 2021.
Jen, C. N., Hanson, D. R., and McMurry, P. H.: Toward Reconciling Measurements of Atmospherically Relevant Clusters by Chemical Ionization Mass Spectrometry and Mobility Classification/Vapor Condensation, Aerosol Sci. Tech., 49, i–iii, https://doi.org/10.1080/02786826.2014.1002602, 2014.
Julin, J., Murphy, B. N., Patoulias, D., Fountoukis, C., Olenius, T., Pandis, S. N., and Riipinen, I.: Impacts of Future European Emission Reductions on Aerosol Particle Number Concentrations Accounting for Effects of Ammonia, Amines, and Organic Species, Environ. Sci. Technol., 52, 692–700, https://doi.org/10.1021/acs.est.7b05122, 2018.
Kirkby, J., Curtius, J., Almeida, J., Dunne, E., Duplissy, J., Ehrhart, S., Franchin, A., Gagne, S., Ickes, L., Kurten, A., Kupc, A., Metzger, A., Riccobono, F., Rondo, L., Schobesberger, S., Tsagkogeorgas, G., Wimmer, D., Amorim, A., Bianchi, F., Breitenlechner, M., David, A., Dommen, J., Downard, A., Ehn, M., Flagan, R. C., Haider, S., Hansel, A., Hauser, D., Jud, W., Junninen, H., Kreissl, F., Kvashin, A., Laaksonen, A., Lehtipalo, K., Lima, J., Lovejoy, E. R., Makhmutov, V., Mathot, S., Mikkila, J., Minginette, P., Mogo, S., Nieminen, T., Onnela, A., Pereira, P., Petaja, T., Schnitzhofer, R., Seinfeld, J. H., Sipila, M., Stozhkov, Y., Stratmann, F., Tome, A., Vanhanen, J., Viisanen, Y., Vrtala, A., Wagner, P. E., Walther, H., Weingartner, E., Wex, H., Winkler, P. M., Carslaw, K. S., Worsnop, D. R., Baltensperger, U., and Kulmala, M.: Role of sulphuric acid, ammonia and galactic cosmic rays in atmospheric aerosol nucleation, Nature, 476, 429–433, https://doi.org/10.1038/nature10343, 2011.
Kirkby, J., Duplissy, J., Sengupta, K., Frege, C., Gordon, H., Williamson, C., Heinritzi, M., Simon, M., Yan, C., Almeida, J., Trostl, J., Nieminen, T., Ortega, I. K., Wagner, R., Adamov, A., Amorim, A., Bernhammer, A. K., Bianchi, F., Breitenlechner, M., Brilke, S., Chen, X., Craven, J., Dias, A., Ehrhart, S., Flagan, R. C., Franchin, A., Fuchs, C., Guida, R., Hakala, J., Hoyle, C. R., Jokinen, T., Junninen, H., Kangasluoma, J., Kim, J., Krapf, M., Kurten, A., Laaksonen, A., Lehtipalo, K., Makhmutov, V., Mathot, S., Molteni, U., Onnela, A., Perakyla, O., Piel, F., Petaja, T., Praplan, A. P., Pringle, K., Rap, A., Richards, N. A., Riipinen, I., Rissanen, M. P., Rondo, L., Sarnela, N., Schobesberger, S., Scott, C. E., Seinfeld, J. H., Sipila, M., Steiner, G., Stozhkov, Y., Stratmann, F., Tome, A., Virtanen, A., Vogel, A. L., Wagner, A. C., Wagner, P. E., Weingartner, E., Wimmer, D., Winkler, P. M., Ye, P., Zhang, X., Hansel, A., Dommen, J., Donahue, N. M., Worsnop, D. R., Baltensperger, U., Kulmala, M., Carslaw, K. S., and Curtius, J.: Ion-induced nucleation of pure biogenic particles, Nature, 533, 521–526, https://doi.org/10.1038/nature17953, 2016.
Kulmala, M., Lehtinen, K. E. J., and Laaksonen, A.: Cluster activation theory as an explanation of the linear dependence between formation rate of 3 nm particles and sulphuric acid concentration, Atmos. Chem. Phys., 6, 787–793, https://doi.org/10.5194/acp-6-787-2006, 2006.
Kürten, A., Li, C., Bianchi, F., Curtius, J., Dias, A., Donahue, N. M., Duplissy, J., Flagan, R. C., Hakala, J., Jokinen, T., Kirkby, J., Kulmala, M., Laaksonen, A., Lehtipalo, K., Makhmutov, V., Onnela, A., Rissanen, M. P., Simon, M., Sipilä, M., Stozhkov, Y., Tröstl, J., Ye, P., and McMurry, P. H.: New particle formation in the sulfuric acid–dimethylamine–water system: reevaluation of CLOUD chamber measurements and comparison to an aerosol nucleation and growth model, Atmos. Chem. Phys., 18, 845–863, https://doi.org/10.5194/acp-18-845-2018, 2018.
Lehtinen, K. E. J., Dal Maso, M., Kulmala, M., and Kerminen, V. M.: Estimating nucleation rates from apparent particle formation rates and vice versa: Revised formulation of the Kerminen-Kulmala equation, J. Aerosol Sci., 38, 988–994, https://doi.org/10.1016/j.jaerosci.2007.06.009, 2007.
Li, C., Zhao, Y., Li, Z., Liu, L., Zhang, X., Zheng, J., Kerminen, V.-M., Kulmala, M., Jiang, J., Cai, R., and Xiao, H.: The dependence of new particle formation rates on the interaction between cluster growth, evaporation, and condensation sink, Environmental Science: Atmospheres, 3, 168–181, https://doi.org/10.1039/d2ea00066k, 2023.
Li, H., Ning, A., Zhong, J., Zhang, H., Liu, L., Zhang, Y., Zhang, X., Zeng, X. C., and He, H.: Influence of atmospheric conditions on sulfuric acid-dimethylamine-ammonia-based new particle formation, Chemosphere, 245, 125554, https://doi.org/10.1016/j.chemosphere.2019.125554, 2020.
Li, M., Zhang, Q., Kurokawa, J.-I., Woo, J.-H., He, K., Lu, Z., Ohara, T., Song, Y., Streets, D. G., Carmichael, G. R., Cheng, Y., Hong, C., Huo, H., Jiang, X., Kang, S., Liu, F., Su, H., and Zheng, B.: MIX: a mosaic Asian anthropogenic emission inventory under the international collaboration framework of the MICS-Asia and HTAP, Atmos. Chem. Phys., 17, 935–963, https://doi.org/10.5194/acp-17-935-2017, 2017.
Li, S., Wang, S., Wu, Q., Zhang, Y., Ouyang, D., Zheng, H., Han, L., Qiu, X., Wen, Y., Liu, M., Jiang, Y., Yin, D., Liu, K., Zhao, B., Zhang, S., Wu, Y., and Hao, J.: Emission trends of air pollutants and CO2 in China from 2005 to 2021, Earth Syst. Sci. Data, 15, 2279–2294, https://doi.org/10.5194/essd-15-2279-2023, 2023.
Li, Y., Shen, J., Zhao, B., Cai, R., Wang, S., Gao, Y., Shrivastava, M., Gao, D., Zheng, J., Kulmala, M., and Jiang, J.: A dynamic parameterization of sulfuric acid–dimethylamine nucleation and its application in three-dimensional modeling, Atmos. Chem. Phys., 23, 8789–8804, https://doi.org/10.5194/acp-23-8789-2023, 2023.
Liu, L., Zhong, J., Vehkamaki, H., Kurten, T., Du, L., Zhang, X., Francisco, J. S., and Zeng, X. C.: Unexpected quenching effect on new particle formation from the atmospheric reaction of methanol with SO3, P. Natl. Acad. Sci. USA, 116, 24966–24971, https://doi.org/10.1073/pnas.1915459116, 2019.
Liu, L., Yu, F., Tu, K., Yang, Z., and Zhang, X.: Influence of atmospheric conditions on the role of trifluoroacetic acid in atmospheric sulfuric acid–dimethylamine nucleation, Atmos. Chem. Phys., 21, 6221–6230, https://doi.org/10.5194/acp-21-6221-2021, 2021.
Lu, Y., Liu, L., Ning, A., Yang, G., Liu, Y., Kurtén, T., Vehkamäki, H., Zhang, X., and Wang, L.: Atmospheric Sulfuric Acid-Dimethylamine Nucleation Enhanced by Trifluoroacetic Acid, Geophys. Res. Lett., 47, e2019GL085627, https://doi.org/10.1029/2019gl085627, 2020.
Ma, F., Xie, H. B., Elm, J., Shen, J., Chen, J., and Vehkamaki, H.: Piperazine Enhancing Sulfuric Acid-Based New Particle Formation: Implications for the Atmospheric Fate of Piperazine, Environ. Sci. Technol., 53, 8785–8795, https://doi.org/10.1021/acs.est.9b02117, 2019.
McGrath, M. J., Olenius, T., Ortega, I. K., Loukonen, V., Paasonen, P., Kurtén, T., Kulmala, M., and Vehkamäki, H.: Atmospheric Cluster Dynamics Code: a flexible method for solution of the birth-death equations, Atmos. Chem. Phys., 12, 2345–2355, https://doi.org/10.5194/acp-12-2345-2012, 2012.
Myllys, N., Chee, S., Olenius, T., Lawler, M., and Smith, J.: Molecular-Level Understanding of Synergistic Effects in Sulfuric Acid-Amine-Ammonia Mixed Clusters, J. Phys. Chem. A, 123, 2420–2425, https://doi.org/10.1021/acs.jpca.9b00909, 2019.
Ning, A. and Zhang, X.: The synergistic effects of methanesulfonic acid (MSA) and methanesulfinic acid (MSIA) on marine new particle formation, Atmos. Environ., 269, 118826, https://doi.org/10.1016/j.atmosenv.2021.118826, 2022.
Ning, A., Zhang, H., Zhang, X., Li, Z., Zhang, Y., Xu, Y., and Ge, M.: A molecular-scale study on the role of methanesulfinic acid in marine new particle formation, Atmos. Environ., 227, 117378, https://doi.org/10.1016/j.atmosenv.2020.117378, 2020.
Ning, A., Liu, L., Ji, L., and Zhang, X.: Molecular-level nucleation mechanism of iodic acid and methanesulfonic acid, Atmos. Chem. Phys., 22, 6103–6114, https://doi.org/10.5194/acp-22-6103-2022, 2022.
Ning, A., Shen, J., Zhao, B., Wang, S., Cai, R., Jiang, J., Yan, C., Fu, X., Zhang, Y., Li, J., Ouyang, D., Sun, Y., Saiz-Lopez, A., Francisco, J. S., and Zhang, X.: Overlooked significance of iodic acid in new particle formation in the continental atmosphere, P. Natl. Acad. Sci. USA, 121, e2404595121, https://doi.org/10.1073/pnas.2404595121, 2024.
Olenius, T.: ACDC, GitHub [code], https://github.com/tolenius/ACDC, last access: 1 August 2024.
Olenius, T. and Roldin, P.: Role of gas-molecular cluster-aerosol dynamics in atmospheric new-particle formation, Sci. Rep.-UK, 12, 10135, https://doi.org/10.1038/s41598-022-14525-y, 2022.
Olenius, T., Kupiainen-Maatta, O., Ortega, I. K., Kurten, T., and Vehkamaki, H.: Free energy barrier in the growth of sulfuric acid-ammonia and sulfuric acid-dimethylamine clusters, J. Chem. Phys., 139, 084312, https://doi.org/10.1063/1.4819024, 2013.
Olenius, T., Halonen, R., Kurtén, T., Henschel, H., Kupiainen-Määttä, O., Ortega, I. K., Jen, C. N., Vehkamäki, H., and Riipinen, I.: New particle formation from sulfuric acid and amines: Comparison of monomethylamine, dimethylamine, and trimethylamine, J. Geophys. Res.-Atmos., 122, 7103–7118, https://doi.org/10.1002/2017jd026501, 2017.
Olin, M., Patoulias, D., Kuuluvainen, H., Niemi, J. V., Rönkkö, T., Pandis, S. N., Riipinen, I., and Dal Maso, M.: Contribution of traffic-originated nanoparticle emissions to regional and local aerosol levels, Atmos. Chem. Phys., 22, 1131–1148, https://doi.org/10.5194/acp-22-1131-2022, 2022.
Ortega, I. K., Kupiainen, O., Kurtén, T., Olenius, T., Wilkman, O., McGrath, M. J., Loukonen, V., and Vehkamäki, H.: From quantum chemical formation free energies to evaporation rates, Atmos. Chem. Phys., 12, 225–235, https://doi.org/10.5194/acp-12-225-2012, 2012.
Riccobono, F., Schobesberger, S., Scott, C. E., Dommen, J., Ortega, I. K., Rondo, L., Almeida, J., Amorim, A., Bianchi, F., Breitenlechner, M., David, A., Downard, A., Dunne, E. M., Duplissy, J., Ehrhart, S., Flagan, R. C., Franchin, A., Hansel, A., Junninen, H., Kajos, M., Keskinen, H., Kupc, A., Kurten, A., Kvashin, A. N., Laaksonen, A., Lehtipalo, K., Makhmutov, V., Mathot, S., Nieminen, T., Onnela, A., Petaja, T., Praplan, A. P., Santos, F. D., Schallhart, S., Seinfeld, J. H., Sipila, M., Spracklen, D. V., Stozhkov, Y., Stratmann, F., Tome, A., Tsagkogeorgas, G., Vaattovaara, P., Viisanen, Y., Vrtala, A., Wagner, P. E., Weingartner, E., Wex, H., Wimmer, D., Carslaw, K. S., Curtius, J., Donahue, N. M., Kirkby, J., Kulmala, M., Worsnop, D. R., and Baltensperger, U.: Oxidation Products of Biogenic Emissions Contribute to Nucleation of Atmospheric Particles, Science, 344, 717–721, https://doi.org/10.1126/science.1243527, 2014.
Semeniuk, K. and Dastoor, A.: Current state of aerosol nucleation parameterizations for air-quality and climate modeling, Atmos. Environ., 179, 77–106, https://doi.org/10.1016/j.atmosenv.2018.01.039, 2018.
Stolzenburg, D., Simon, M., Ranjithkumar, A., Kürten, A., Lehtipalo, K., Gordon, H., Ehrhart, S., Finkenzeller, H., Pichelstorfer, L., Nieminen, T., He, X.-C., Brilke, S., Xiao, M., Amorim, A., Baalbaki, R., Baccarini, A., Beck, L., Bräkling, S., Caudillo Murillo, L., Chen, D., Chu, B., Dada, L., Dias, A., Dommen, J., Duplissy, J., El Haddad, I., Fischer, L., Gonzalez Carracedo, L., Heinritzi, M., Kim, C., Koenig, T. K., Kong, W., Lamkaddam, H., Lee, C. P., Leiminger, M., Li, Z., Makhmutov, V., Manninen, H. E., Marie, G., Marten, R., Müller, T., Nie, W., Partoll, E., Petäjä, T., Pfeifer, J., Philippov, M., Rissanen, M. P., Rörup, B., Schobesberger, S., Schuchmann, S., Shen, J., Sipilä, M., Steiner, G., Stozhkov, Y., Tauber, C., Tham, Y. J., Tomé, A., Vazquez-Pufleau, M., Wagner, A. C., Wang, M., Wang, Y., Weber, S. K., Wimmer, D., Wlasits, P. J., Wu, Y., Ye, Q., Zauner-Wieczorek, M., Baltensperger, U., Carslaw, K. S., Curtius, J., Donahue, N. M., Flagan, R. C., Hansel, A., Kulmala, M., Lelieveld, J., Volkamer, R., Kirkby, J., and Winkler, P. M.: Enhanced growth rate of atmospheric particles from sulfuric acid, Atmos. Chem. Phys., 20, 7359–7372, https://doi.org/10.5194/acp-20-7359-2020, 2020.
Svenhag, C., Sporre, M. K., Olenius, T., Yazgi, D., Blichner, S. M., Nieradzik, L. P., and Roldin, P.: Implementing detailed nucleation predictions in the Earth system model EC-Earth3.3.4: sulfuric acid–ammonia nucleation, Geosci. Model Dev., 17, 4923–4942, https://doi.org/10.5194/gmd-17-4923-2024, 2024.
Thomas, J. M., He, S., Larriba-Andaluz, C., DePalma, J. W., Johnston, M. V., and Hogan Jr., C. J.: Ion mobility spectrometry-mass spectrometry examination of the structures, stabilities, and extents of hydration of dimethylamine-sulfuric acid clusters, Phys. Chem. Chem. Phys., 18, 22962–22972, https://doi.org/10.1039/c6cp03432b, 2016.
Xiao, M., Hoyle, C. R., Dada, L., Stolzenburg, D., Kürten, A., Wang, M., Lamkaddam, H., Garmash, O., Mentler, B., Molteni, U., Baccarini, A., Simon, M., He, X.-C., Lehtipalo, K., Ahonen, L. R., Baalbaki, R., Bauer, P. S., Beck, L., Bell, D., Bianchi, F., Brilke, S., Chen, D., Chiu, R., Dias, A., Duplissy, J., Finkenzeller, H., Gordon, H., Hofbauer, V., Kim, C., Koenig, T. K., Lampilahti, J., Lee, C. P., Li, Z., Mai, H., Makhmutov, V., Manninen, H. E., Marten, R., Mathot, S., Mauldin, R. L., Nie, W., Onnela, A., Partoll, E., Petäjä, T., Pfeifer, J., Pospisilova, V., Quéléver, L. L. J., Rissanen, M., Schobesberger, S., Schuchmann, S., Stozhkov, Y., Tauber, C., Tham, Y. J., Tomé, A., Vazquez-Pufleau, M., Wagner, A. C., Wagner, R., Wang, Y., Weitz, L., Wimmer, D., Wu, Y., Yan, C., Ye, P., Ye, Q., Zha, Q., Zhou, X., Amorim, A., Carslaw, K., Curtius, J., Hansel, A., Volkamer, R., Winkler, P. M., Flagan, R. C., Kulmala, M., Worsnop, D. R., Kirkby, J., Donahue, N. M., Baltensperger, U., El Haddad, I., and Dommen, J.: The driving factors of new particle formation and growth in the polluted boundary layer, Atmos. Chem. Phys., 21, 14275–14291, https://doi.org/10.5194/acp-21-14275-2021, 2021.
Xie, H. B., Elm, J., Halonen, R., Myllys, N., Kurten, T., Kulmala, M., and Vehkamaki, H.: Atmospheric Fate of Monoethanolamine: Enhancing New Particle Formation of Sulfuric Acid as an Important Removal Process, Environ. Sci. Technol., 51, 8422–8431, https://doi.org/10.1021/acs.est.7b02294, 2017.
Yang, S., Liu, Z., Clusius, P. S., Liu, Y., Zou, J., Yang, Y., Zhao, S., Zhang, G., Xu, Z., Ma, Z., Yang, Y., Sun, J., Pan, Y., Ji, D., Hu, B., Yan, C., Boy, M., Kulmala, M., and Wang, Y.: Chemistry of new particle formation and growth events during wintertime in suburban area of Beijing: Insights from highly polluted atmosphere, Atmos. Res., 255, https://doi.org/10.1016/j.atmosres.2021.105553, 2021.
Yao, L., Garmash, O., Bianchi, F., Zheng, J., Yan, C., Kontkanen, J., Junninen, H., Mazon, S. B., Ehn, M., Paasonen, P., Sipila, M., Wang, M., Wang, X., Xiao, S., Chen, H., Lu, Y., Zhang, B., Wang, D., Fu, Q., Geng, F., Li, L., Wang, H., Qiao, L., Yang, X., Chen, J., Kerminen, V. M., Petaja, T., Worsnop, D. R., Kulmala, M., and Wang, L.: Atmospheric new particle formation from sulfuric acid and amines in a Chinese megacity, Science, 361, 278–281, https://doi.org/10.1126/science.aao4839, 2018.
Yazgi, D. and Olenius, T.: J-GAIN v1.1: a flexible tool to incorporate aerosol formation rates obtained by molecular models into large-scale models, Geosci. Model Dev., 16, 5237–5249, https://doi.org/10.5194/gmd-16-5237-2023, 2023.
Yu, F.: Ion-mediated nucleation in the atmosphere: Key controlling parameters, implications, and look-up table, J. Geophys. Res., 115, D03206, https://doi.org/10.1029/2009jd012630, 2010.
Zhao, B., Shrivastava, M., Donahue, N. M., Gordon, H., Schervish, M., Shilling, J. E., Zaveri, R. A., Wang, J., Andreae, M. O., Zhao, C., Gaudet, B., Liu, Y., Fan, J., and Fast, J. D.: High concentration of ultrafine particles in the Amazon free troposphere produced by organic new particle formation, P. Natl. Acad. Sci. USA, 117, 25344–25351, https://doi.org/10.1073/pnas.2006716117, 2020.
Zheng, H., Cai, S., Wang, S., Zhao, B., Chang, X., and Hao, J.: Development of a unit-based industrial emission inventory in the Beijing–Tianjin–Hebei region and resulting improvement in air quality modeling, Atmos. Chem. Phys., 19, 3447–3462, https://doi.org/10.5194/acp-19-3447-2019, 2019.
Zhu, S., Yan, C., Zheng, J., Chen, C., Ning, H., Yang, D., Wang, M., Ma, Y., Zhan, J., Hua, C., Yin, R., Li, Y., Liu, Y., Jiang, J., Yao, L., Wang, L., Kulmala, M., and Worsnop, D. R.: Observation and Source Apportionment of Atmospheric Alkaline Gases in Urban Beijing, Environ. Sci. Technol., 56, 17545–17555, https://doi.org/10.1021/acs.est.2c03584, 2022.