the Creative Commons Attribution 4.0 License.
the Creative Commons Attribution 4.0 License.
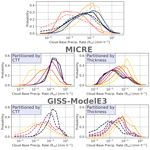
Earth-system-model evaluation of cloud and precipitation occurrence for supercooled and warm clouds over the Southern Ocean's Macquarie Island
Ann M. Fridlind
Israel Silber
Andrew S. Ackerman
Greg Cesana
Johannes Mülmenstädt
Alain Protat
Simon Alexander
Adrian McDonald
Over the remote Southern Ocean (SO), cloud feedbacks contribute substantially to Earth system model (ESM) radiative biases. The evolution of low Southern Ocean clouds (cloud-top heights < ∼ 3 km) is strongly modulated by precipitation and/or evaporation, which act as the primary sink of cloud condensate. Constraining precipitation processes in ESMs requires robust observations suitable for process-level evaluations. A year-long subset (April 2016–March 2017) of ground-based profiling instrumentation deployed during the Macquarie Island Cloud and Radiation Experiment (MICRE) field campaign (54.5∘ S, 158.9∘ E) combines a 95 GHz (W-band) Doppler cloud radar, two lidar ceilometers, and balloon-borne soundings to quantify the occurrence frequency of precipitation from the liquid-phase cloud base. Liquid-based clouds at Macquarie Island precipitate ∼ 70 % of the time, with deeper and colder clouds precipitating more frequently and at a higher intensity compared to thinner and warmer clouds. Supercooled cloud layers precipitate more readily than layers with cloud-top temperatures > 0 ∘C, regardless of the geometric thickness of the layer, and also evaporate more frequently. We further demonstrate an approach to employ these observational constraints for evaluation of a 9-year GISS-ModelE3 ESM simulation. Model output is processed through the Earth Model Column Collaboratory (EMC2) radar and lidar instrument simulator with the same instrument specifications as those deployed during MICRE, therefore accounting for instrument sensitivities and ensuring a coherent comparison. Relative to MICRE observations, the ESM produces a smaller cloud occurrence frequency, smaller precipitation occurrence frequency, and greater sub-cloud evaporation. The lower precipitation occurrence frequency by the ESM relative to MICRE contrasts with numerous studies that suggest a ubiquitous bias by ESMs to precipitate too frequently over the SO when compared with satellite-based observations, likely owing to sensitivity limitations of spaceborne instrumentation and different sampling methodologies for ground- versus space-based observations. Despite these deficiencies, the ESM reproduces the observed tendency for deeper and colder clouds to precipitate more frequently and at a higher intensity. The ESM also reproduces specific cloud regimes, including near-surface clouds that account for ∼ 25 % of liquid-based clouds during MICRE and optically thin, non-precipitating clouds that account for ∼ 27 % of clouds with bases higher than 250 m. We suggest that the demonstrated framework, which merges observations with appropriately constrained model output, is a valuable approach to evaluate processes responsible for cloud radiative feedbacks in ESMs.
- Article
(13112 KB) - Full-text XML
- BibTeX
- EndNote
Extratropical shortwave (SW) radiation cloud feedbacks are a significant source of uncertainty in Earth system model (ESM) projections of a perturbed climate (e.g., Caldwell et al., 2016; McCoy et al., 2020). In particular, ESMs in phase 5 of the Coupled Model Intercomparison Project (CMIP5; Taylor et al., 2012) exhibit high-biased SW absorption due to a deficit in low- and mid-level cloudiness over the Southern Ocean (SO) (Bodas-Salcedo et al., 2014, 2016; Naud et al., 2014). CMIP6 models improved this bias to some degree (e.g., Schuddeboom and McDonald, 2021; Cesana et al., 2022), but low- and mid-level clouds at latitudes higher than 55∘ S were found to still produce a low bias in reflected SW radiation compared to satellite observations (e.g., Mallet et al., 2023), likely due to poor phase representation in the dominant supercooled-liquid-cloud regime (Cesana et al., 2022). Furthermore, the equilibrium climate sensitivity (ECS) has increased from CMIP5 to CMIP6 generations, primarily due to stronger positive low-cloud feedbacks (Zelinka et al., 2020) that may contribute to increased high-biased sea surface temperatures in CMIP6 compared to CMIP5 (Zhang et al., 2023).
Low-level clouds (< ∼ 3 km) that form in the warm and cold sectors of extratropical cyclones account for up to 80 % of annual fractional cloud cover in observations (Mace et al., 2009). Cloud condensate amount and sustenance are heavily modulated by precipitation (Kay et al., 2016b; Tan et al., 2016), which is the dominant factor for moisture depletion (McCoy et al., 2020). In a warming climate, an expected shift to more liquid-bearing (“warm”) clouds has been shown to increase liquid-phase cloud amount, increase optical depth, and contribute to a larger negative cloud feedback (Mitchell et al., 1989; Tsushima et al., 2006; Mülmenstädt et al., 2021) following from findings that precipitation efficiency is generally weaker in warm clouds compared to supercooled clouds (Mitchell et al., 1989; Senior and Mitchell, 1993; Tsushima et al., 2006; Hoose et al., 2008). Properly predicting extratropical SW cloud feedbacks is thus dependent on an ESM's ability to faithfully represent both observed precipitation occurrence frequency and cloud phase, but these are common shortcomings of ESMs, especially over the SO (Kay et al., 2016b, 2018; Naud et al., 2020; Gettelman et al., 2020; Cesana et al., 2022).
Robust observational constraints are needed in order to understand precipitation occurrence frequency in ESMs. Spaceborne platforms offer the longest and most spatially expansive constraints but have some limitations. For example, the CloudSat Cloud Profiling Radar (CPR; Stephens et al., 2002) experiences contamination in the lowest 1 km due to ground clutter that hinders detection of low marine clouds, inducing a miss rate of up to 39 % over the global oceans (Liu et al., 2016; McErlich et al., 2021). Low CPR sensitivity also limits detection of optically thin clouds, and its relatively coarse horizontal resolution misses shallow cumulus clouds (Rodts et al., 2003; Zhang and Klein, 2013; Cesana et al., 2019a). Lamer et al. (2020a) found that CPR limitations impeded detection of warm marine-boundary-layer clouds over the eastern North Atlantic by 29 %–43 % and distorted cloud macroscopic properties compared to ground-based instrumentation. Over the Arctic and Antarctic, Silber et al. (2021a) found that differences in sensitivity and precipitation detection algorithms can reduce spaceborne estimates of cloud-base and surface precipitation occurrence frequency by more than 50 %. For the purpose of cloud-base precipitation evaluation, space-based lidars furthermore become attenuated in visibly opaque layers with optical depths > ∼ 3, preventing identification of a cloud layer throughout the entire column and thus leaving cloud-base height poorly defined (Vaughan et al., 2009).
Another approach used for characterizing precipitation frequency and intensity is the use of ground-based remote sensing deployments that allow for long-term (order of months to years) statistics to be compiled at high temporal and vertical spatial resolution (Illingworth et al., 2007; Bühl et al., 2016, 2019; Ansmann et al., 2019; Lamer et al., 2020b; Griesche et al., 2021; Ramelli et al., 2021; Silber et al., 2021a; McFarquhar et al., 2021). Such ground-based datasets usually include periodic balloon soundings that provide direct colocated measurements of atmospheric thermodynamic state, which are generally missing from satellite remote sensing. Although often horizontally limited (employing only zenith-viewing instruments), such methods provide a means to obtain characteristics of shallow, boundary-layer-limited clouds that are regionally ubiquitous and are complementary to satellite remote sensing. For instance, Silber et al. (2021a) used measurements from Utqiaġvik (formerly Barrow), North Slope of Alaska (NSA; Verlinde et al., 2016), and McMurdo Station, Antarctica (Lubin et al., 2020a), to establish the precipitation occurrence frequency in polar supercooled clouds. Using a combined sounding-radar approach, they found that supercooled cloud layers are precipitating from the liquid cloud base 75 % of the time at the NSA and 85 % of the time at McMurdo Station. Lamer et al. (2020b) similarly used a combined radar–lidar approach at the US Department of Energy (DOE) Atmospheric Radiation Measurement (ARM) program's eastern North Atlantic (ENA) site to determine that 80 % of warm clouds in subsidence regimes are precipitating from the cloud base. Ship-based deployments have also been extensively evaluated using these profiling measurement techniques. For example, Griesche et al. (2021) combined ship-based lidar, radar, and radiosondes during an Arctic summer voyage and found that for cloud-top temperatures > −15 ∘C, surface-coupled clouds were more likely to contain ice than were surface-decoupled clouds. These techniques have also been used to perform mixed-phase microphysical retrievals, such as ice- and liquid-mass flux (Bühl et al., 2016) and ice crystal number concentrations (Bühl et al., 2019).
Addressing ESM biases over the SO has recently motivated numerous airborne and ship-based field campaigns to characterize cloud, aerosol, and radiation properties across a latitudinal band from ∼ 45–75∘ S (Mace and Protat, 2018a, b; Kremser et al., 2021; McFarquhar et al., 2021). Ship-based campaigns equipped with lidar, radar, and radiosondes have yielded results on cloud processes and microphysics (Mace and Protat, 2018a, b; McFarquhar et al., 2021). For example, clouds near the Antarctic coast were found to have higher droplet number concentrations than those further north due to continental air masses with large cloud-condensation-nuclei concentrations and increased sulfate aerosol (Mace et al., 2021), and supercooled liquid drizzle is often observed beneath clouds in the same coastal Antarctic region (Alexander et al., 2021).
Complementary to these ship-based campaigns, the Macquarie Island Cloud and Radiation Experiment (MICRE) was organized by the DOE ARM program, the Australian Bureau of Meteorology (BoM), and the Australian Antarctic Division (AAD) from March 2016 to March 2018. MICRE is thus far the only stationary, ground-based campaign to provide an annual cycle of SO cloud measurements at a fixed site (where the SO is defined broadly as 45 to 75∘ S). Situated at 54.5∘ S and 158.9∘ E, Macquarie Island is well located in the middle of the SO midlatitude storm track, making it a valuable location to observe cloud regimes responsible for ESM biases, and has been subject to detailed study (e.g., Adams, 2009; Wang et al., 2015; Lang et al., 2018, 2020; Tansey et al., 2022). Tansey et al. (2022) combined data streams from a surface disdrometer, cloud radar, and tipping-bucket rain gauge during MICRE and found that surface precipitation occurs 44 ± 4 % of the time and is dominated by relatively small particles (< 1 mm in diameter). Wang et al. (2015) evaluated an 8-year record (2003–2011) of 3-hourly tipping-bucket rain gauge observations at Macquarie Island with a lower measurement limit of 0.2 mm h−1 and found that surface precipitation occurred 36 % of the time, with a large contribution from light-precipitation rates. Lang et al. (2020) used 18 years of hourly surface precipitation measurements to reveal a diurnal cycle in precipitation that peaks during night/early morning and is strongest during austral summer.
In this work we report a combined analysis of measurements from a 95 GHz (W-band) zenith-pointing Doppler cloud radar, two lidar ceilometers, and atmospheric soundings deployed at Macquarie Island that were coincident during a year of the MICRE campaign (April 2016 to May 2017; McFarquhar et al., 2021; Tansey et al., 2022). A leading objective is to merge instrument data streams to compute the precipitation occurrence frequency from the liquid cloud base (LCB). A focus on LCB precipitation, whether or not the precipitation reaches the surface, provides an important constraint for ESMs because it means that an active precipitation process is occurring that should be represented by a given model's physics parameterizations. In an observational analysis of coalescence scavenging over the SO, Kang et al. (2022) found that light-precipitation rates (< 0.1 mm h−1) have a significant impact on scavenging of cloud condensation nuclei and the resulting cloud droplet number concentration, demonstrating the relevance of precipitation rates at the low-intensity limit. Moreover, understanding the degree to which evaporation or sublimation is prevalent below the cloud base is important as it impacts sub-cloud precipitation accumulation, boundary layer structure, and cloud mesoscale organization. For example, Heymsfield et al. (2020) used satellite-based radar measurements to evaluate hydrometeor-phase contributions to the global precipitation budget and found a significant contribution from evaporation of melted and frozen precipitation in an ESM. Retrievals of LCB precipitation rates, cloud-top and cloud-base temperatures, and cloud geometric thickness are used here to investigate the degree to which LCB precipitation properties are sensitive to the cloud-top supercooling and the cloud geometric thickness. Retrievals of precipitation occurrence frequency are then projected onto sensitivities that emulate instrument and algorithm sensitivity, providing comparative uncertainties associated with space-based retrievals that can be used going forward to inform strategies for fusion of ground- and satellite-based data sources for model evaluation.
The merged MICRE dataset is finally used to evaluate a 9-year ESM simulation by means of the Earth Model Column Collaboratory (EMC2; Silber et al., 2022) radar and lidar instrument simulator and subcolumn generator. EMC2 was designed to enable robust comparisons between ground-based observations and ESM column physics in a manner that remains faithful to the model's physics assumptions. Using EMC2, forward simulations are performed on ESM output from the National Aeronautics and Space Administration (NASA) Goddard Institute for Space Studies (GISS) ModelE3 (GISS-ModelE3; Cesana et al., 2019b, 2021) ESM at 2.0 × 2.5∘ resolution as a free-running global simulation with prescribed sea surface temperatures and sea ice distributions. Vertical profiles of microphysical quantities required for forward simulation of remote-sensing observables are output at time-step frequency at Macquarie Island's geographic location and processed through EMC2 to produce radar and lidar calculations consistent with the specifications of instrumentation deployed during MICRE. In this manner, we demonstrate a framework for process-level evaluation of ESM column physics against long-term, ground-based observations over the SO using the MICRE measurements.
The remainder of the article is structured as follows: data and methods, including observational datasets and precipitation detection algorithm development, are described in Sect. 2. Observational results are presented in Sect. 3, and a demonstration of the GISS-ModelE3 evaluation against those results is provided in Sect. 4. Implications of findings for ESMs, satellite retrievals, and designing future SO missions are presented in Sect. 5, and conclusions are summarized in Sect. 6.
2.1 Data
Instruments used in this study include the BoM's Bistatic Radar System for Atmospheric Studies (BASTA; Delanoë et al., 2016) 95 GHz (W-band) zenith-pointing Doppler cloud radar, ARM's Vaisala CT25K 910 nm ceilometer (Morris et al., 2016; Morris, 2016), the University of Canterbury's Vaisala CL51 910 nm ceilometer (Alexander and McDonald, 2019), and 12-hourly atmospheric balloon soundings conducted by the Australian Bureau of Meteorology (Barnes-Keoghan, 2000a). A 2 h example of data from this instrumentation is shown in Fig. 1.
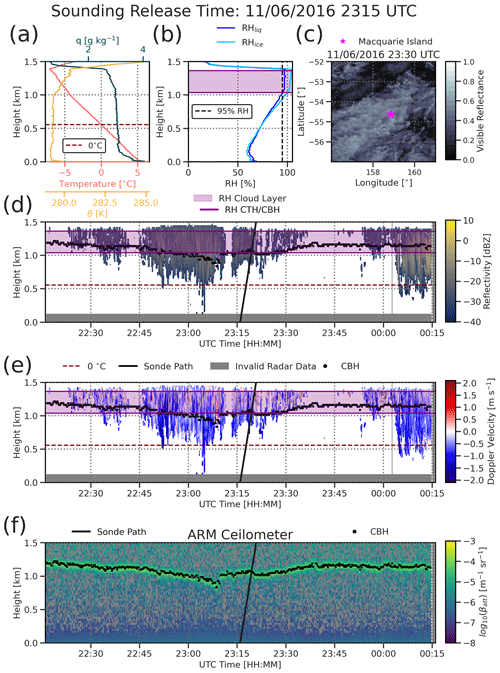
Figure 1A 2 h example of measurements at Macquarie Island: (a) sounding temperature, water vapor mixing ratio (q), and potential temperature (θ) with melting level indicated (dashed line); (b) relative humidity with respect to liquid water (RHliq) and ice (RHice) with 95 % RHliq indicated (dashed line); (c) satellite visible reflectance from the Himawari-8 satellite (ARM User Facility, 2016) and the location of Macquarie Island; (d) BASTA radar reflectivity; (e) BASTA mean Doppler velocity; and (f) ARM ceilometer apparent attenuated backscatter (βatt). In panels (d)–(f), the sounding path is shown as a black line from 23:15 UTC, and the cloud-base heights (CBHs) are shown as black dots. Purple shading in panels (b), (d), and (e) indicates the vertical extent where sounding RHliq > 95 %.
The BASTA radar operates in four 3 s modes with varying sensitivity and vertical resolution. Here, we use the 25 m mode most suitable for detecting low-level liquid cloud layers (Delanoë et al., 2016), for which the effective temporal resolution is 12 s, with a vertical range from 125 m to 12 km above ground level (a.g.l.). Although MICRE extended over 2 years (2016 to 2018), the BASTA radar's residence was limited to only approximately the first year of the campaign (April 2016 to March 2017). Calibration of BASTA is achieved using recent ship-based campaign data from BASTA, a 24 GHz Micro Rain Radar PRO, an optical disdrometer, and T-matrix calculations (Protat et al., 2019). BASTA has a sensitivity of −36 dBZ at 1 km a.g.l., and any bins with values below the theoretical minimum reflectivity (Ze,min; see Appendix B) are treated as free of hydrometeors.
The ARM ceilometer has native 16 s temporal resolution and 10 m vertical resolution extending from the surface to 7.7 km a.g.l. The cloud-base height (CBH) product (Morris, 2016) allows the detection of up to three CBHs, but only the lowest identified CBH is used here. CBH detections come from the vendor's proprietary software, which is generally associated with a peak signal in attenuated backscatter (βatt) with an uncertainty of ± 5 m for liquid clouds (Morris, 2016). The University of Canterbury ceilometer has native 6 s temporal resolution and 10 m vertical resolution with three CBHs retrieved up to 15.4 km a.g.l. at 10 m resolution. The ARM ceilometer is primarily used for CBH detection, though due to prolonged blackout periods, the University of Canterbury ceilometer is used to fill in gaps when the ARM ceilometer was not operational. Because the highest identifiable CBH by the ARM ceilometer is 7.7 km a.g.l., all CBHs higher than 7.7 km identified by the University of Canterbury ceilometer are discarded, though this limit is high enough to encapsulate the overwhelming majority of liquid layers. Collectively, the merged ceilometer dataset is referred to as CEIL. We note that attenuated backscatter was not calibrated in this study since CBH is provided by instrument firmware. Uncalibrated or “apparent” βatt is shown in Fig. 1f only for demonstration of peak βatt associated with the cloud base. However, attenuated backscatter is used to evaluate near-surface clouds in Sect. 3.4.2, where sensitivities to instrument calibration are considered and discussed.
Soundings were released nominally every 12 h and measured atmospheric pressure, temperature, and relative humidity with respect to liquid water (RHliq). Uncertainties in RHliq, temperature, and pressure are assumed to be 5 %, 0.5 ∘C, and 1 hPa, respectively (Holdridge, 2020). A surface meteorology station is also used contextually in our analysis (Howie and Protat, 2016).
2.2 Methods
All instruments are merged and gridded onto the BASTA time–height grid of 12 s and 25 m, and time periods with invalid radar and/or ceilometer data are discarded. Cloud-base heights are interpolated with a nearest-neighbor approach in time and space, where the nearest time cannot exceed 12 s from a BASTA time stamp, and the nearest heights lie within or on the edge of a valid BASTA range gate. Cloud-base precipitation occurrence frequency depends on the CEIL-identified CBH, the uncertainties for which are discussed next, along with calculations of cloud macrophysical and thermodynamic properties. Derivations of cloud-base and surface precipitation occurrence frequency (Pcb and Psfc, respectively) are then described, followed by retrievals of cloud-base precipitation rates (Rcb). Appendix A provides a list of abbreviations and notation used throughout the paper.
2.2.1 Cloud macrophysics and thermodynamics
All CBH detections by CEIL are assumed to be liquid cloud base (LCB) heights. Silber et al. (2018) compared various LCB height products for polar supercooled-liquid-cloud cases and found that the ARM ceilometer occasionally detects liquid cloud bases that are actually ice as identified by polarization lidar data, but these false detections remain below 2 % of the distribution for any given altitude, though we note the vastly different environments sampled between Macquarie Island and the polar sites they evaluated. We also note that although a polarization lidar was present during the MICRE campaign, the data have calibration stability and other problems that prevented their use in this study but are being corrected and will be released soon (Tansey et al., 2023).
Additionally, Silber et al. (2018) found based on a comparison with high-spectral-resolution lidar (HSRL) measurements that, on average, the ARM ceilometer detects the LCB 36–50 m in-cloud (site-dependent) but that it performs well in regions of heavy precipitation and exhibits low variability compared to other CBH detection algorithms. Sensitivity to biases in CBH are evaluated in Appendix C by decreasing the CBH by 25 to 50 m (i.e., one to two BASTA bins) for all retrievals. Herein, we also discard any CBH detections that have a cloud-base temperature (CBT) colder than the homogeneous freezing level (taken to be −38 ∘C).
In fog, CEIL signals attenuate completely near the surface, such that a CBH is identified near the surface and most often at altitudes below 250 m. Since Pcb is evaluated at a minimum height that is at least 200 m a.g.l. based on radar contamination and antenna coupling in the first few range bins, these fog-influenced backscatter profiles contribute minimally (<3 %) to profiles used for precipitation detection. For CBHs < 250 m, where they are relatively common, these CEIL backscatter profiles indicative of fog are flagged and discussed separately in Sect. 3.4.2 and Appendix F.
Independent evaluation of the CEIL LCB was made by using in situ RHliq thresholds from soundings. CEIL-recognized LCBs at sounding release times were colocated and are shown as a function of RHliq and temperature in Fig. D1, indicating that more than 66 (80) % of CEIL-recognized LCBs exhibit RHliq > 95 (90) %. Silber et al. (2020a) found that > 90 % of polar supercooled cloud bases identified by an HSRL had concurrent sounding RHliq > 95 %. The reduced percentage of CEIL-recognized LCBs with RHliq > 95 % in the MICRE dataset compared to polar supercooled cloud layers in Silber et al. (2020a) can be attributed at least in part to spatiotemporal discrepancies between the cloud environments sampled by the soundings and by CEIL. For example, Fig. 1b shows that RHliq drops quickly below the sounding-recognized LCB (i.e., where RHliq first exceeds 95 %; in purple shading). Therefore, variability in CBH by even 100 m (which is within the range of variability in CEIL CBHs for the 2 h time period in Fig. 1f) can lead to RHliq < 95 % at the CEIL-recognized LCB. In addition, there are frequently scenarios in which the sounding balloon passes in between horizontally inhomogeneous cloud layers, such that the sounding RHliq never reaches 95 % despite the identification of nearby clouds via the ceilometer. The approach taken by Silber et al. (2021a) in which cloud boundaries were identified by sounding RHliq thresholds rather than lidar and radar was motivated by the prevalence of overcast multi-layer supercooled clouds in their polar cloud regimes and also enabled a sufficiently long sounding dataset over ∼ 7 years in the Arctic. By contrast, the relatively short duration of MICRE and the greater heterogeneity of cloud boundaries over the SO relative to polar clouds in our case motivates LCB identification via remote sensing instrumentation with higher temporal resolution (i.e., CEIL and BASTA). Although there remains uncertainty in LCB height identification, particularly due to unknowns regarding CBH algorithms, we have attempted to mitigate these uncertainties by evaluating CEIL LCBs against sounding RHliq measurements (Appendix D), accounting for fog-influenced CEIL profiles (Appendix F), and accounting for uncertainty in Pcb due to errors in the height of the LCB identified by CEIL (Appendix C). Potential improvements to instrument strategies for LCB height determination in future campaigns are also discussed below.
Cloud-top height (CTH) is determined as the height at which a contiguous layer of reflectivity (Ze) above the CEIL-identified cloud base drops below (i.e., becomes free of hydrometeors). The difference between CTH and CBH defines the cloud geometric thickness. Cloud-top temperature (CTT) and cloud-base temperature (CBT) are determined by near-in-time atmospheric soundings. Soundings released nominally at 12 h intervals are linearly interpolated onto constant altitude levels in order to form a continuous curtain plausibly consistent with the radar and CEIL measurements. During periods when soundings were released more than 12 h apart, temperature is taken to be constant for 6 h on either side of the sounding release time, and time periods greater than 6 h from the sounding release time are discarded, though we note that the results here are not sensitive to the time period surrounding a given sounding (not shown). During periods of robust stratiform precipitation, the interpolated 0 ∘C isotherm is found to be consistent with a melting layer or “bright band” (i.e., a steep increase in Doppler velocity and an apparent jump in radar reflectivity; see Austin and Bemis, 1950), further indicating relatively robust measurements of tropospheric temperature despite the coarse time frequency of measurements. Using CBT and CTT, cloud layers are subdivided into supercooled layers (CBT and CTT < 0 ∘C), partially supercooled layers (CBT ≥ 0 ∘C and CTT < 0 ∘C), and warm layers (CBT and CTT ≥ 0 ∘C).
2.2.2 Precipitation occurrence frequency
Precipitation identification is determined by linearly averaging the reflectivity factor within a prescribed number of bins below the ceilometer-identified LCB height. The depth below LCB height used for precipitation detection is called Dmin. Precipitation occurrence requires that the linearly averaged reflectivity exceeds the theoretical reflectivity minimum as a function of height (; Fig. B1) and that the minimum mean Doppler velocity within the range of bins is negative (downward, thus excluding updrafts). We note instances in which there exists a CEIL-identified CBH without coincident reflectivity, where the higher sensitivity of the ceilometer to smaller hydrometeors produces detectable backscatter returns from small droplets unregistered by the radar. We consider these instances to be non-precipitating clouds, which are discussed in detail in Sect. 3.4.1.
Cloud-base precipitation occurrence frequency (Pcb) is calculated for varying minimum , which ranges from −55 to 15 dBZ, and for varying depths below the cloud base (Dmin) used for reflectivity averaging, ranging from 50 to 600 m. The minimum detectable height of the radar (hmin) is set to 150 m based on careful analysis of ground clutter contamination. The minimum allowable CBH is thus hmin + Dmin, ranging from 200 m to 750 m a.g.l. depending on Dmin (see Appendix E). Precipitation occurrence frequency at the surface (Psfc) is also derived by linearly averaging reflectivity within a prescribed number of bins above hmin.
2.2.3 Precipitation rates
Calculations of cloud-base precipitation rates (Rcb) are determined by first identifying the temperature of the LCB. For CBTs ≥ 0 ∘C, the drizzle reflectivity–rain rate relationship (Z–R) from Comstock et al. (2004) is used (Z = aRb, where a = 25, and b = 1.3). An examination of in situ aircraft data from the Southern Ocean Clouds, Radiation, Aerosol Transport Experimental Study (SOCRATES; McFarquhar et al., 2021) finds the Comstock et al. (2004) relationship holds well for drizzle falling from SO stratocumulus (Roger Marchand, personal communication, 2023). For CBTs < 0 ∘C, we follow the methodology of Silber et al. (2021a) and Bühl et al. (2016) and use the Hogan et al. (2006) parameterization for computing ice water content (IWC) via reflectivity and temperature and then compute ice water flux by multiplying IWC by the minimum mean Doppler velocity within a prescribed depth below the LCB (Dmin). This method assumes the column beneath the LCB is subsaturated (supersaturated) with respect to liquid (ice). The minimum mean (reflectivity-weighted) Doppler velocity is used as a central upper limit to the precipitation rate since preferential ice sublimation below the LCB can significantly reduce precipitation rates when averaged across Dmin. We note that there are significant uncertainties related to these precipitation rate retrievals, especially considering a lack of robust Z–R relationships derived for SO clouds available for this study and the inability to robustly determine hydrometeor phase with the available instrumentation (e.g., Silber et al., 2020b). Whereas Silber et al. (2021a) found that Ze below the LCB nearly universally increases downward in polar supercooled cloud layers, indicative of ice-phase precipitation that grows by vapor diffusion below the LCB (see their Appendix E), here we find that only ∼ 45 % to 60 % of supercooled layers exhibit Ze increasing below the LCB (not shown). This suggests that a relatively large fraction of supercooled LCBs are precipitating primarily in the liquid phase, with warmer CTTs showing a greater likelihood for decreasing Ze below the LCB (indicative of evaporation). The presence of liquid-phase precipitation below a supercooled LCB is consistent with Mace and Protat (2018a), who found that about half of supercooled liquid-based clouds contained liquid-phase precipitation during the month-long ship-based Clouds, Aerosols, Precipitation, Radiation, and Atmospheric Composition over the Southern Ocean (CAPRICORN I) campaign south of Tasmania (latitudinal range from ∼ 43 to 53 ∘S) from 13 March to 15 April 2016. Although there is uncertainty in the phase of precipitation and thus the retrieval used to derive Rcb, we accept these uncertainties as a starting point in this study and focus on quantifying trends as a function of cloud properties that are expected to be important modulating factors.
Liquid cloud bases are identified by CEIL in 76 % of valid profiles in the merged MICRE dataset spanning nearly 1 year, with month-to-month variability of ∼ 10 % (not shown). Given this variability and only a single annual cycle, we do not evaluate cloud and precipitation seasonal distributions but refer to Tansey et al. (2022) for a robust evaluation of MICRE's seasonal cycle of surface precipitation. However, we note that this total cloud occurrence frequency matches that determined by Mace and Protat (2018a) (76 %) during the CAPRICORN I voyage and by Protat et al. (2017) (77 %) during another ship-based SO campaign.
CEIL is obscured 2.5 % of the time, in which the ceilometer experienced attenuated backscatter, but a cloud base could not be determined. These profiles are omitted from further analysis, though we note that obscuration commonly occurs during heavy-precipitation or fog events, such that this 2.5 % may be considered an uncertainty in total cloud occurrence frequency.
When an LCB was identified, 26 % of identified LCBs are below 250 m a.g.l. and are discussed in Sect. 3.4.2. The remaining 74 % of LCBs are above 250 m a.g.l. and are used for precipitation detection. Of these, 61 % of LCBs are supercooled (i.e., CBT < 0 ∘C). Precipitation occurrence frequencies are discussed next.
3.1 Cloud-base precipitation occurrence frequency (Pcb)
Cloud-base precipitation occurrence frequency (Pcb) is first discussed in terms of the depth below the cloud base used for precipitation detection (Dmin, equivalent to the vertical resolution) and the minimum reflectivity threshold (; Fig. 2). As in Silber et al. (2021a), this approach simultaneously illustrates both the MICRE dataset characteristics (in the lower left-hand corners in Fig. 2 panels) and quantities roughly comparable to a wide range of current and future satellite instrument characteristics. For example, the and Dmin sensitivities of the CloudSat 2C-Precip-Column (2C-PC; Haynes et al., 2009) and 2C-Snow-Column (Wood et al., 2014) “possible” and the 2C-PC “certain” data products are shown as symbols in Fig. 2. At the BASTA sensitivity and Dmin = 50 m, 69 % of clouds are precipitating from the LCB (Fig. 2a) and decrease as a function of both Dmin and . We note that limiting profiles to those containing only one CEIL-recognized CBH (single layer clouds) changed Pcb by < 1 %, therefore likely mitigating significant influence of seeder–feeder mechanisms (e.g., He et al., 2022) to the extent that the ceilometer is not fully attenuated beyond the lowest cloud layer.
Supercooled-layer Pcb for BASTA is 61 % (Fig. 2c), and warm-layer Pcb is 66 % (Fig. 2g). While supercooled Pcb is not a strong function of Dmin, warm-layer Pcb decreases by a factor of 2 in the range of Dmin shown. In subsaturated air below the LCB, liquid-phase cloud drops are expected to evaporate. As Dmin increases, and Ze is averaged over a larger depth, evaporating drops become smaller such that the average Ze drops below the radar sensitivity. This is demonstrated at the surface (Fig. 2h), whereby the precipitation occurrence decreases by 12 percentage points relative to the cloud base. Conversely, the sub-cloud environment for supercooled layers precipitating in the ice phase is expected to be supersaturated with respect to ice (though temperature-dependent), allowing for ice growth via vapor deposition and thus increasing Ze below the LCB (Silber et al., 2021a). The neutral slope of supercooled Pcb as a function of Dmin indicates precipitation that is not strictly growing in the ice phase nor evaporating in the liquid phase. As described above, Ze below the LCB was found to often decrease below the LCB, indicating that a fraction of these supercooled cloud layers are precipitating primarily in the liquid phase, but the influence of precipitating ice is present. Indeed, near the surface, supercooled precipitation occurrence frequency (Psfc) decreases by 19 percentage points (Fig. 2d), suggesting the presence of evaporating liquid-phase precipitation from supercooled cloud layers, sublimation of ice, or evaporation of melted ice precipitation. Evaporation is discussed in more detail in Sect. 3.3.
Partially supercooled Pcb is 97 % for BASTA (Fig. 2e) and decreases by only 7 percentage points near the surface (Fig. 2f). These partially supercooled layers are shown below to generally be much thicker compared to purely supercooled or warm cloud layers and also to precipitate at a higher intensity, likely reasons for higher Pcb and less evaporation. Finally, we note that sensitivities of Pcb to potential biases in LCB height as discussed by Silber et al. (2018) are addressed in Appendix C and Fig. C1.
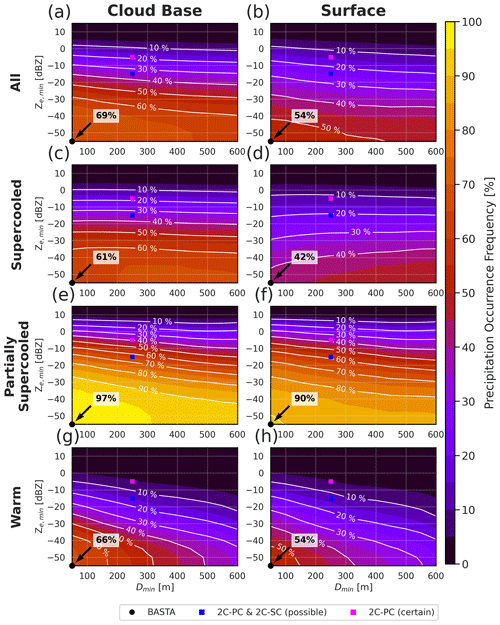
Figure 2Precipitation occurrence frequency (Pcb, contours and color fill) as a function of the minimum reflectivity threshold (, ordinate) and the depth below the cloud base used to detect precipitation (Dmin, abscissa). All cloud layers are shown in the top row, supercooled layers in the second row, partially supercooled layers in the third row, and warm layers in the bottom row. The first column is for precipitation from the cloud base (Pcb), and the second column is for precipitation at the surface (Psfc). The black circles in the bottom left-hand corner of each panel represent the BASTA and Dmin = 50 m (two range gates). Blue and magenta symbols in all plots represent the and Dmin (i.e., the vertical resolution) of the CloudSat 2C-PC/2C-SC “possible” and 2C-PC “certain” data products.
The projection of Pcb onto cloud thermodynamics and macrophysics is performed hereafter assuming a constant Dmin = 100 m (four range gates) to limit artifacts from false detections, and the native BASTA profile is retained. Occurrence frequencies and the precipitating fraction of cloud layers are shown as a function of cloud thickness, CBH, and CTT in Fig. 3, where occurrence frequencies are normalized by all cloud layers (pink) and by non-precipitating cloud layers (green), and the precipitating fraction is calculated for all samples in a given cloud property bin. Non-precipitating cloud layers are thinner (Fig. 3a–d), and CBHs are higher (Fig. 3e–h) relative to all cloud layers, and the precipitating fraction increases with increasing cloud thickness and decreases with increasing CBH. Partially supercooled cloud layers are generally thicker, and CBHs are lower relative to purely supercooled layers. Cloud thickness and CBH distributions for all layers closely follow the supercooled-layer distributions, consistent with Fig. E1, showing that the majority of cloud layers are supercooled.
Cloud layers with CTTs < −20 ∘C (Fig. 3i) are rare, and the distribution of CTTs peaks at slight supercoolings between 0 and −4 ∘C. The precipitating fraction as a function of CTT has a notable peak ∼ −15 ∘C, which may be due to temperatures ∼ −14 ∘C being the peak of vapor depositional growth rates on ice (e.g., Fukuta and Takahashi, 1999; Wallace and Hobbs, 2006), increasing the likelihood of radar detectability, as also seen in Silber et al. (2021a).
Alexander and Protat (2018) quantified the fraction of supercooled liquid-water clouds at Cape Grim, Tasmania (40.7 ∘S, 144.7∘ E) with ice virga below the LCB using a ground-based lidar. They found that for stratocumulus layers with CTTs < −15∘, the fraction of precipitating ice virga clouds was ∼ 70 %–80 %, but this fraction decreased to < 20 % for CTTs warmer than −15 ∘C. Radenz et al. (2021) found a similarly small percentage of ice virga clouds for CTTs warmer than −15 ∘C using a radar–lidar approach over Punta Arenas, Chile (53.1 ∘S, 70.9 ∘W). However, both of these studies limited their datasets to relatively optically and geometrically thin stratocumulus clouds. Here, the larger precipitating fraction at relatively warm supercooled CTTs (> −15 ∘C) may be due to the inclusion of optically and geometrically thicker layers (e.g., cumulus), particularly partially supercooled layers that precipitate in the liquid phase, are generally thicker, and precipitate quite frequently (Figs. 2e and 3c). Using soundings to calculate the estimated inversion strength (EIS; Wood and Bretherton, 2006), partially supercooled cloud layers were found to occur in environments associated with lower EIS values, indicating greater decoupling from the surface for this cloud type (not shown).
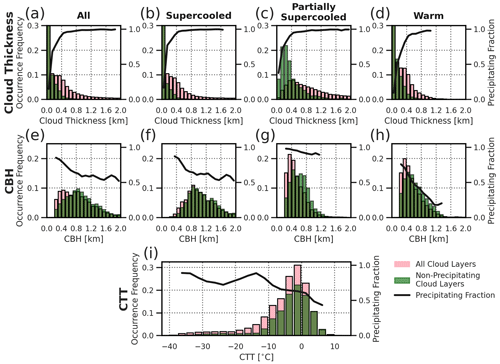
Figure 3Occurrence frequency distributions of cloud thickness (a–d), CBH (e–h), and CTT (i) for all cloud layers (a, e), supercooled layers (b, f), partially supercooled layers (c, g), and warm layers (d, h). All cloud layers are shown as pink bars, while non-precipitating cloud layers are shown as green bars. The precipitating fraction as a function of each cloud property bin is shown as a black line.
Figure 3 shows that thicker clouds and those with colder CTTs are more likely to precipitate, but the cloud thickness and CTT are highly correlated since thicker clouds have higher CTHs and thus colder CTTs. To discriminate between these two cloud properties, the cloud-base precipitating fraction is projected onto CTT and cloud thickness by means of joint histograms in Fig. 4. As expected, the distribution shows that cloud thickness generally increases with decreasing CTT. However, the precipitating fraction generally increases for colder CTTs for the same cloud thickness, indicating that supercooled cloud layers more readily precipitate than warm clouds (e.g., Mitchell et al., 1989; Senior and Mitchell, 1993; Tsushima et al., 2006; Hoose et al., 2008; Mülmenstädt et al., 2021). A stricter Ze threshold of −20 dBZ (Fig. 4b and as implied by Mace and Protat, 2018a, to indicate light precipitation) shows this more clearly, where the precipitating fraction increases by up to a factor of 2 between CTTs of 0 and −15 ∘C for even relatively thin clouds ( m). The exception to this is for cloud thicknesses < 200 m, where the majority of clouds do not precipitate, regardless of their CTT.
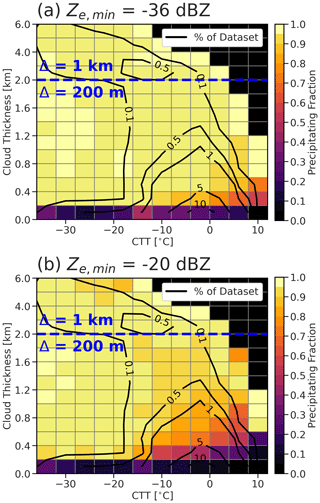
Figure 4Joint histogram of CTT (abscissa) and cloud thickness (ordinate) shown as the percentage of the dataset in black contours and color-filled with the precipitating fraction for all samples within a given CTT–cloud thickness bin. Panel (a) uses Ze,min = −36 dBZ for detecting precipitating layers, and panel (b) uses Ze,min = −20 dBZ. The bin width (Δ) for CTT is 4 ∘C. For cloud thickness, Δ is split between two ranges. For thicknesses <2 km, Δ = 200 m, while Δ = 1 km for thicknesses > 2 km, denoted by the horizontal dashed blue line.
3.2 Cloud-base precipitation rates (Rcb)
In total, 69 % of identified cloud layers with CBHs > 250 m are precipitating from the LCB. Of all precipitating cloud layers, ∼ 54 % are supercooled, 22 % are partially supercooled, and 24 % are warm (legend of Fig. 5a). Precipitation rates are derived as described in Sect. 2.2.3, and the probability distribution is shown in Fig. 5a. The Rcb distribution for all cloud layers peaks just under 10−1 mm h−1, where supercooled layers largely control the total Rcb distribution. Warm cloud layers produce the weakest Rcb and peak between rates of 10−4 and 10−3 mm h−1. The partially supercooled Rcb distribution is the narrowest, with a peak just above 10−1 mm h−1. Both supercooled and partially supercooled Rcb distributions are negatively skewed, while warm-cloud-layer Rcb distributions are positively skewed.
Rcb distributions are further partitioned by CTT (Fig. 5b) and cloud thickness (Fig. 5c). Rcb peak probabilities increase with decreasing CTT and increasing cloud thickness. Rcb was also found to increase for decreasing CTT while controlling for cloud thickness (not shown), implying that colder clouds, regardless of their thickness, have higher Rcb, likely owing to the presence of ice precipitation.
3.3 Evaporation/sublimation below the cloud base
Evaporation (or sublimation) below the cloud base is evaluated in terms of the evaporated fraction, which is the fraction of layers with detectable cloud-base precipitation that is not continuous down to hmin. The evaporated fraction is shown as a function of CTT and cloud thickness via a joint histogram in Fig. 6a and as a function of surface RH (RHsfc) and CBH in Fig. 6b. Evaporated fraction decreases with increasing cloud thickness. Thicker cloud layers are likely to have more vertically integrated condensate and have higher Rcb such that thicker layers are more resilient to complete desiccation (Fig. 5c). Unsurprisingly, evaporated fraction increases for decreasing CTT owing to the Clausius–Clapeyron relationship. This suggests that precipitation from supercooled cloud layers is more likely to evaporate/sublimate below the LCB than precipitation from warm layers. This trend is consistent with the larger decrease in supercooled-precipitation occurrence at the surface relative to the cloud base in supercooled layers compared to warm layers (Fig. 2c, d, g, h). In Fig. 6b, surface RH and CBH are expectedly correlated. The evaporated fraction increases for increasing CBH and decreasing RH, as cloud bases at higher altitudes have a larger depth of sub-cloud air for evaporation to take place and are likely to be colder (barring temperature inversions).
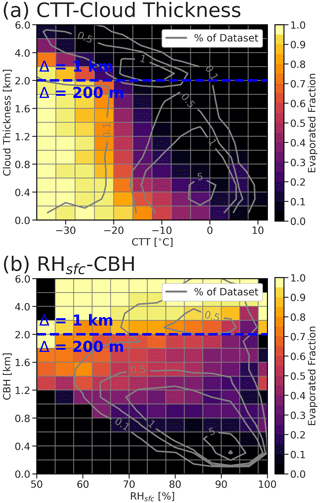
Figure 6Joint histograms of (a) CTT and cloud thickness and (b) RHsfc and CBH with percentage of the dataset contoured in gray, and the color fill is evaporated fraction. The bin width (Δ) for RHsfc is 5 %. Bin widths for CBH and cloud thickness are split between two ranges. For thicknesses < 2 km or CBHs < 2 km a.g.l., Δ = 200 m, while Δ = 1 km for thicknesses > 2 km or CBHs > 2 km a.g.l., denoted by the horizontal dashed blue line.
3.4 Special cases
3.4.1 Optically thin cloud layers
Cloud detection herein relies on the merged ceilometer dataset (CEIL), the CBHs for which are derived by the vendor's proprietary algorithm. Precipitation detection requires that reflectivity be coincident in the bin identified by CEIL, but a large proportion (27 %) of clouds with CBHs > 250 m were optically thin where the CEIL-identified cloud-base bins do not have coincident reflectivity. This is illustrated in Fig. 1, for example between ∼ 23:35 and 23:50 UTC, where the ARM ceilometer's apparent attenuated backscatter (βatt) has values > 10−4 m−1 sr−1 (indicative of liquid cloud bases; Fig. 1f), but radar reflectivity during this time period (Fig. 1d) does not reach BASTA's at that altitude. These layers are referred to as CEIL-only clouds.
Figure 7 shows a scatterplot between the CBH and CBT for these CEIL-only cloud bases. The color fill of each point on the scatterplot is the observation density, and a histogram is shown on each axis for the one-dimensional observation density for CBH and CBT, ignoring the other variable. The majority of these optically thin clouds have bases <2 km a.g.l. (peaking ∼ 1 km a.g.l.) and temperatures ranging from −10 to 5 ∘C. The median CBT for these clouds is −3 ∘C, indicating that many of these clouds are only very slightly supercooled.
Mace and Protat (2018a) determined that approximately 30 % of clouds during the SO CAPRICORN I voyage were detected only by a lidar with no coincident layer-averaged reflectivity (as opposed to just considering reflectivity at the cloud base as is done here). Here, the CEIL-only percentage reduces to ∼ 20 % when also considering profiles where radar reflectivities exceed the noise floor within 100 m above the LCB (not shown), which is evidence of cloud layers where droplets are too small to be recognizable by BASTA at the cloud base but become detectable as they grow above the cloud base. This indicates that 20 %–30 % of clouds from MICRE and CAPRICORN I are representative of optically thin liquid layers unregistered by BASTA. We note that these layers were also evaluated during times with colocated soundings, in which sounding RHliq values often showed a high peak (> 95 %) at the same level as enhanced βatt values where the LCB is detected without coincident reflectivity (not shown). Their structure is often persistent, with little vertical variability in the LCB height, and in some instances hydrometeors grow large enough to be intermittently detected by BASTA (for example in Fig. 1). Accounting for these optically thin clouds has important implications for defining Pcb since these non-precipitating cloud layers are a non-negligible fraction of the normalizing cloud population. Because many studies have required that a cloud layer have coincident reflectivity (e.g., Lamer et al., 2020b; Silber et al., 2021a), it is therefore possible that Pcb for warm cloud layers is overestimated in such studies due to elimination of these optically thin layers from the cloud population. However, for supercooled layers in which ice-phase precipitation can be “detached” from the cloud base as it grows below the LCB via vapor diffusion, Pcb may still be underestimated (e.g., Silber et al., 2021a). The prevalence of this cloud regime in other geographical regions is unclear, though Mace and Protat (2018a) also found this optically thin cloud type in ∼ 20 % of cloud layers over the ARM ENA site at Graciosa Island in the Azores (39 ∘N and 28 ∘W).
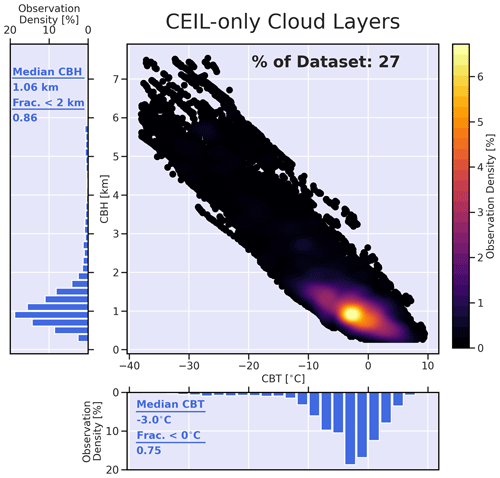
Figure 7Scatterplot of the 27 % of cloud bases above 250 m a.g.l. where a cloud base is detected only by CEIL (i.e., no coincident radar reflectivity) as a function of CBT (abscissa) and CBH (ordinate). Points are color-filled with the observation density. One-dimensional observation density histograms are also plotted on the respective axes.
3.4.2 Near-surface clouds and fog
Pcb calculations require the minimum CBH to be 250 m using Dmin = 100 m. Of all CEIL-identified layers, 26 % of cloud bases are <250 m, which collectively are called “”near-surface clouds”. The apparent βatt profiles for these periods show repeating patterns of specific cloud morphology. Two case studies for these morphologies are discussed in Appendix F. In particular, Figs. F1 and F2 show CBHs identified below 150 m (within the BASTA “blind zone”), and the apparent βatt profiles from CEIL show values > 10−4 m−1 sr−1 at the cloud base but with no significant reduction in apparent βatt below the cloud base towards the surface. We consider these cases to be fog, noting that this is a broad definition that may include deliquescent aerosols that produce haze or possibly sea spray.
A simple fog identification algorithm was developed to identify cases where the cloud-base apparent βatt > 10−4.5 m−1 sr−1 and does not decrease by at least an order of magnitude below the cloud base. There are several caveats to this detection method. First, only profiles with a valid CBH detection below 250 m a.g.l. are considered, therefore neglecting any profiles where fog may be detectable using βatt alone. Second, βatt is uncalibrated. To explore the sensitivity to this, calibration factors were applied to all near-surface CBH profiles (e.g., O'Connor et al., 2004; Hopkin et al., 2019; Kuma et al., 2021). Calibration factors were guided by literature (Kuma et al., 2021) and by applying the lidar autocalibration method described by O'Connor et al. (2004) for optically thick non-precipitating stratocumulus, though we note that few cases were found to be appropriate for calibration with this method in this dataset. For a cloud-base βatt threshold of 10−4.5 m−1 sr−1 used for fog identification, calibration factors ranging from 1–4 yielded fog occurrence frequencies relative to all near-surface clouds that ranged from 69 %–82 %. Sensitivity to calibration factors increased with increasing cloud-base βatt thresholds, and the fog occurrence frequency in general was more sensitive to this threshold than to calibration. Given these multiple uncertainties, we do not formally attempt to calibrate βatt in this study but note that future work concerning surface-based fog detection over the Southern Ocean should consider all profiles with valid βatt (regardless of valid CBH detection) and should pursue calibration methods appropriate for fog.
Profiles matching the fog identification algorithm using apparent βatt occurred 18 % of the time (accounting for 69 % of near-surface clouds). We examine distributions of surface measurements for all near-surface clouds and for those identified as fog in Fig. 8. RHsfc values exceed 90 % for almost the entirety of the distributions for near-surface clouds and fog, with some tendency for smaller values for non-fog profiles, supporting the possibility of haze in some instances. Surface temperatures are always above freezing during this time period, peaking around 7 ∘C, with no significant differences between the distributions of near-surface clouds and fog. Surface wind speeds also show no significant differences for fog relative to all near-surface clouds, but we note the persistence of rather strong surface wind speeds (distribution modes ∼ 20 m s−1), indicating that these fog events are likely of the advective type rather than radiative fog, which requires calm surface conditions. The fog formation processes may be analogous to those during Arctic air formation (Tjernström et al., 2019). Mace and Protat (2018a) reported that the air temperature was colder than the sea surface temperature except for a few days during the SO CAPRICORN I voyage spanning 43 to 53 ∘S, equatorward of Macquarie Island, which may explain the lack of similarly abundant near-surface clouds reported in their study. While other recent SO voyages reached the edge of Antarctica (e.g., Kremser et al., 2021; McFarquhar et al., 2021), none occurred during the coldest months of the year, and each was relatively short compared to the annual cycle observed during MICRE. Indeed, fog detections during MICRE were more frequent in austral winter and transition months than during austral summer (not shown).
Even though CBH is too low to establish precipitation below it, valid radar reflectivity was identified between 150 and 250 m in > 98 % of all near-surface clouds and fog layers. Figure 8d shows the layer-averaged Ze between 150 and 250 m a.g.l. (). Distributions of are largely similar between near-surface clouds and fog, although fog layers are shifted slightly toward larger values. Note that ∼ 60 % of the distributions have > −15 dBZ, suggesting that a non-negligible portion of these near-surface clouds experience precipitation from above, for example as demonstrated in Fig. F1.
The Arctic and Antarctic sites evaluated by Silber et al. (2021a) required an hmin of 300 m, such that near-surface clouds (including potential fog) were not considered, but we note that fog features were seen to some degree in the Arctic data from NSA. Because the radar “blind zone” (i.e., the surface through hmin) limits the detection of hydrometeors within this range, it is routine for studies to truncate cloud detection from ground-based instrumentation to above hmin. However, the large proportion of CBHs identified below 250 m (26 % of all clouds) in this study implies the need for more robust quantification of fog and near-surface clouds. Indeed, a 30-year climatology (1952–1981) of global cloud type distributions from ship-based observations showed a global peak in fog frequency of occurrence between a latitudinal band from 40 to 70 ∘S, including over Macquarie Island's longitude (Warren et al., 1988). They showed a seasonal cycle that appears to maximize during austral summer, suggesting that fog formation mechanisms are not limited to Arctic air formation during austral winter, as discussed above. In addition, Kuma et al. (2020) used ship-based ceilometer data from multiple SO voyages and found that occurrence frequencies of CBHs peak below 500 m a.g.l. and often very near the surface, indicative of fog, and that these low clouds were often associated with near-surface air temperatures <0 ∘C and warmer than the sea surface temperature (SST), analogous to Arctic air formation.
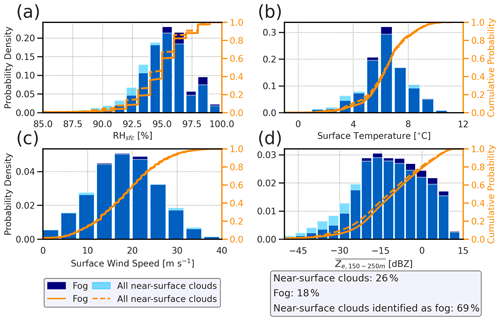
Figure 8Probability distributions of (a) RHsfc, (b) surface temperature, (c) surface wind speed, and (d) layer-averaged Ze between 150 and 250 m a.g.l. (). Light-blue bars are for all near-surface clouds, and dark-blue bars are for near-surface clouds identified as fog. The solid and dashed lines show the cumulative fraction for profiles identified as fog and for all near-surface clouds, respectively. The text box in the lower right shows the percentage of cloud profiles identified as near-surface clouds, the percentage of cloud profiles identified as fog, and the percentage of near-surface cloud profiles identified as fog.
4.1 Model setup
We next demonstrate use of the merged MICRE dataset to evaluate a 9-year (2012–2020), global free-running (i.e., no nudging) simulation using the NASA GISS-ModelE3 ESM. In brief, the simulation used here employs 2×2.5∘ resolution and 110 vertical levels. The model configuration is the same as that used by Cesana et al. (2021), also referred to as GISS-ModelE3-Phys in that study's supporting information, denoting a configuration that uses the default set of tuning parameters and an alternative entrainment closure for moist convection. Other aspects of the model are summarized by Cesana et al. (2021) and references therein. The simulation was initialized on 1 November 2011 for 2 months of model spin-up and prescribes sea surface temperatures using a climatology following the Atmospheric Model Intercomparison Project (AMIP) specifications (Gates, 1992; Gates et al., 1999). Aerosol profiles are prescribed as a single-mode log-normal size distribution with regionally and seasonally varying concentrations, and activation follows from Abdul-Razzak et al. (1998). For stratiform cloud microphysics, a modified version of the Gettelman and Morrison (2015) two-moment bulk microphysics scheme (MG2) is used that includes prognostic precipitation. Convective cloud microphysics are described in Cesana et al. (2019b). Both the stratiform and convective schemes include the following four hydrometeor classes: cloud liquid water, cloud ice, precipitating liquid water, and precipitating ice.
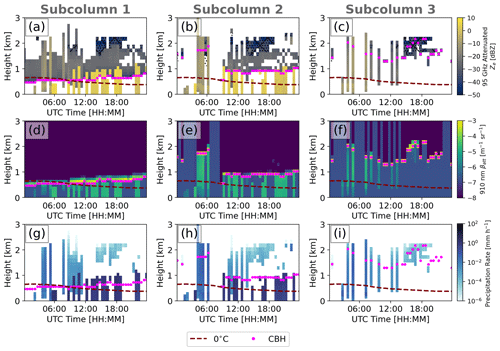
Figure 9Example 24 h time–height series of EMC2-simulated (a)–(c) 95 GHz attenuated Ze, (d)–(e) 910 nm βatt, and (g)–(i) GISS-ModelE3 precipitation rate (sum of convective and stratiform precipitation rates) for a slightly supercooled, precipitating stratocumulus case. The three columns represent three out of eight subcolumns generated using EMC2. CBH is denoted by magenta dots, and the 0 ∘C isotherm is shown by a dashed red line. Hatching in (a)–(c) represents hydrometeor-containing grid cells with reflectivity lower than the BASTA .
4.2 EMC2 instrument simulator application
For application of EMC2, microphysical variables required for the simulation of radar and lidar moments are output in the grid cell containing Macquarie Island at model physics time-step frequency (30 min) as instantaneous values for comparison with observations. EMC2 offers two approaches for remote sensing calculations, including a radiation scheme logic that generalizes hydrometeor fractions and uses bulk scattering calculations for specific size distributions and a microphysics logic that uses single-particle-scattering calculations with the model's predicted particle size distributions. Here, the microphysics scheme logic is used. After providing to EMC2 a user-defined number of subcolumns (taken here as eight), hydrometeors are allocated to the subcolumns by translating the volume fraction of the model's hydrometeor class to a number of hydrometeor-containing and hydrometeor-free subcolumn bins. The maximum–random overlap approach (Tian and Curry, 1989; Fan et al., 2011; Hillman et al., 2018) is then applied from the top down, which preferentially extends cloud layers vertically within a subcolumn and retains vertical continuity of cloud and precipitation features. Further details of subcolumn generation and forward simulation can be found in Silber et al. (2022).
A 24 h example of variables simulated by EMC2 is shown in Fig. 9 for a slightly supercooled, precipitating stratocumulus case. Three of the eight subcolumns are used to demonstrate simulated 95 GHz attenuated Ze, 910 nm βatt, and GISS-ModelE3 precipitation rates. Precipitation detection for GISS-ModelE3 is performed in a similar manner to MICRE observations with a few differences. Rather than performing a CBH identification algorithm via the simulated 910 nm ceilometer βatt, the LCB is identified explicitly as the lowest-altitude subcolumn pixel in time–height space that contains cloud liquid-water content (CLWC). This treatment implies an LCB for every column that contributes to total liquid-cloud fraction. We note that the LCB identified with this method is most often colocated with locally enhanced simulated βatt > 10−4 m−1 sr−1 (Fig. 9d–f). For comparison with the observational approach, we find that the cloud occurrence frequency is not sensitive to CLWC or βatt thresholding beyond an arbitrary value that is indicative of non-negligible liquid-cloud mass (see Appendix G and Fig. G1).
Precipitation detection is then performed at the same pixel as the LCB. While the GISS-ModelE3 convective and stratiform precipitation schemes inform whether or not the precipitation process is active immediately at the cloud base, precipitation is only considered detectable for comparison with MICRE observations where the simulated 95 GHz attenuated Ze is above the BASTA noise floor. If a column pixel has a Ze value above the noise floor coincident with hydrometeor mass from a precipitating hydrometeor species at the LCB, the cloud layer is diagnosed as precipitating. The explicit mass-weighted precipitation rate from the model at that pixel is then taken as the cloud-base precipitation rate (i.e., Rcb). We note that Pcb is not sensitive to an arbitrary minimum Rcb threshold (Appendix G). Finally, all algorithm limits applied to the MICRE dataset are applied to the GISS-ModelE3 simulation. Namely, LCBs are limited to altitudes below 7.7 km a.g.l., CBTs and CTTs are limited to being warmer than −38 ∘C, and noise floor restrictions from 95 GHz attenuated Ze emulating BASTA are applied to cloud and precipitation retrievals.
4.3 Comparison with MICRE
Table 1 provides a comparison of cloud and precipitation properties between MICRE and the GISS-ModelE3 simulation. All values are percentages relative to a normalizing population, given as the population one indentation level above. The top-level normalizing population for MICRE is ∼ 1 year of operational vertical profiles passing quality control, while the GISS-ModelE3 top-level normalizing population is 9 years of simulated profiles. Absolute differences between MICRE and GISS-ModelE3 statistics are denoted in parentheses. GISS-ModelE3 produces a total cloud occurrence frequency of 57% (interannual range of 55 %–61 %), which is 19 percentage points lower than the MICRE observations. Of all cloudy profiles, 74 % of GISS-ModelE3 CBHs are higher than 250 m, which is the same percentage as MICRE. Supercooled layers account for 78 % of all CBHs > 250 m a.g.l. in GISS-ModelE3 and 61 % in MICRE. For CBHs > 250 m a.g.l., 31 % of cloud bases in GISS-ModelE3 did not have coincident Ze above the noise floor compared to 27 % of MICRE cloud bases being identified only by CEIL.
Table 1Comparison of cloud and precipitation properties between the MICRE dataset and the 9-year GISS-ModelE3 ESM simulation. Indentations are used to represent percentages relative to the normalizing population given one indentation level above, where the top-level normalizing population for MICRE is ∼ 1 year of valid profiling instrument data and for GISS-ModelE3 is the 9 years of simulation data. Values in parentheses under the GISS-ModelE3 columns are absolute differences from MICRE observations. Brackets for the E3 total cloud occurrence frequency represent the interannual range of the 9-year simulation.
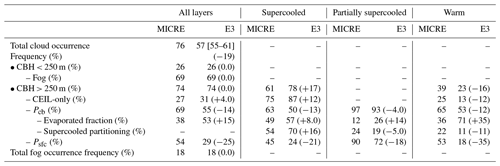
For CBHs > 250 m, 55 % are precipitating from the LCB in GISS-ModelE3 compared to 69 % in MICRE. Pcb as a function of is shown in Fig. 10 for GISS-ModelE3 and MICRE for all cloud layers and partitioned by supercooled, partially supercooled, and warm layers. This Pcb projection illustrates both the radar sensitivity and the contribution to Pcb by cloud bases precipitating at a given Ze threshold. All layers precipitate less frequently in GISS-ModelE3 compared to MICRE, which is constant regardless of . Partially supercooled cloud layers precipitate most frequently in GISS-ModelE3, with only a decrease by 4 percentage points relative to MICRE, while supercooled and warm layers precipitate less frequently in GISS-ModelE3 by 14 % and 12 %, respectively, at the BASTA sensitivity.
For supercooled and partially supercooled cloud layers, Pcb is relatively insensitive to < −36 dBZ (region to the left of the dashed light-blue line in Fig. 10), which occupies the lowest 1 km a.g.l. of BASTA's range. However, warm cloud layers populate this Ze range since warm CBH is generally < 1 km (see Fig. 3h). This Ze range accounts for a 10 % increase in warm-layer Pcb in GISS-ModelE3 and a 15 % increase in MICRE when decreasing from −36 to −50 dBZ. We emphasize that in both MICRE and GISS-ModelE3, although the Pcb values for supercooled and warm layers listed in Table 1 are similar, a large portion of warm-layer Pcb is attributable to cloud layers with a sub-cloud base dBZ. At higher thresholds (e.g., dBZ), supercooled cloud layers consistently precipitate more frequently than warm layers. Overall, GISS-ModelE3 produces a systematic low bias in Pcb relative to MICRE regardless of the cloud-top temperature or threshold. One potential cause for lower Pcb in GISS-ModelE3 is the lack of interactive aerosol, which is prescribed in the current runs and should be investigated in future studies.
Precipitating layers also evaporate more frequently in GISS-ModelE3 compared to MICRE. The evaporated fraction is 38 % in MICRE but 53 % in GISS-ModelE3. All levels of supercooling produce excessive evaporated fractions, but the largest bias occurs in warm clouds, where the evaporated fraction is 71 % in GISS-ModelE3 compared to 36 % in MICRE. This excessive evaporation results in a Psfc of only 18 % in GISS-ModelE3 relative to 53 % in MICRE.
Distributions of GISS-ModelE3 Rcb are shown in Fig. 11 and separated by supercooling, CTT, and cloud thickness, as in Fig. 5. The MICRE Rcb PDF is also shown in Fig. 11a. GISS-ModelE3 captures trends in Rcb that are present in the MICRE observations well, whereby supercooled layers have higher Rcb relative to warm layers, and partially supercooled layers have the highest Rcb. However, one distinct difference is lower supercooled Rcb and higher partially supercooled Rcb in GISS-ModelE3 relative to MICRE. This may be indicative of a transfer of relative rainfall production between cloud populations, whereby partially supercooled clouds produce more rainfall, and purely supercooled clouds produce less rainfall in GISS-ModelE3. Precipitation rates also increase with colder CTT and with larger cloud geometric thickness, as was seen in the MICRE dataset (Fig. 5b–c). The total Rcb distributions for both MICRE and GISS-ModelE3 are largely controlled by supercooled cloud layers, which account for 70 % of the distribution in GISS-ModelE3 compared to 54 % in MICRE (Table 1).
Finally, the same fog identification algorithm applied to the MICRE dataset in Sect. 3.4.2 is applied here. Fog is identified at the same frequency in GISS-ModelE3 as in MICRE. This agreement indicates that these near-surface cloud layers commonly observed during MICRE are to some degree represented in GISS-ModelE3.
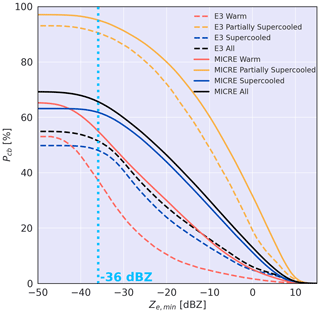
Figure 10Cloud-base precipitation occurrence frequency (Pcb) as a function of for the GISS-ModelE3 simulation (dashed lines) and for MICRE (solid lines) showing all cloud layers in black and partitioned by supercooling in colors.
5.1 Implications for ESMs
MICRE provides a unique year-long dataset for observing cloud and precipitation properties over the remote SO. A common shortcoming of CMIP5 ESMs over the SO is a lack of clouds in general, which results in excessive absorbed shortwave radiation at the surface relative to observations (e.g., Trenberth and Fasullo, 2010; Bodas-Salcedo et al., 2012, 2014; Flato et al., 2013; Cesana et al., 2022). Conversely, some CMIP6 models improved this bias and based on a classification of International Satellite Cloud Climatology Project (ISCCP) data now may simulate too many stratocumulus clouds that are not reflective enough (e.g., Schuddeboom and McDonald, 2021). In the current study, the occurrence frequency of liquid-based clouds is 57 % in GISS-ModelE3 compared to 76 % in MICRE (with month-to-month variability of ∼ 10 percentage points), implying that GISS-ModelE3 cloud occurrence frequency is lower than observed. The majority of LCBs in MICRE and in GISS-ModelE3 are supercooled, which is consistent with spaceborne documentation of ubiquitous supercooled low-level liquid clouds (e.g., Morrison et al., 2011; Huang et al., 2012; Cesana and Chepfer, 2013; Chubb et al., 2013; Bodas-Salcedo et al., 2016). Even though GISS-ModelE3 produces fewer liquid-based clouds relative to observations, the majority of these clouds are indeed supercooled. Kay et al. (2016a) found that the Community Earth System Model (CESM1; Hurrell et al., 2013) with the Community Atmosphere Model (CAM5) produced too few persistent supercooled liquid cloud layers and too much ice over the SO relative to satellite observations due to a preferential glaciation of simulated supercooled clouds. However, we note that here the supercooled Pcb in GISS-ModelE3 is weaker than observed, suggesting that a lack of simulated supercooled clouds in GISS-ModelE3 may not be caused by a tendency for supercooled liquid clouds to glaciate and precipitate quickly.
The finding that supercooled cloud layers precipitate more readily than warm cloud layers for the same geometric thickness has implications for precipitation behavior in a warming climate. Mülmenstädt et al. (2021) discuss a negative cloud radiative feedback (i.e., cooling effect) in which a shift from ice and mixed-phase clouds to mostly liquid clouds in a warming climate leads to more reflective clouds (optical feedback component) with a longer desiccation timescale (described as a so-called “lifetime” feedback component, where “lifetime” metaphorically refers to an increase in the horizontal extent and residence time of cloud condensate in the atmosphere). However, this negative cloud feedback is modulated by how readily warm clouds precipitate. Studies that compare ESM warm-rain precipitation probability to spaceborne active remote sensors show a relatively ubiquitous bias in which warm clouds precipitate too readily (e.g., Stephens et al., 2010; Suzuki et al., 2015; Jing et al., 2017; Kay et al., 2018). Indeed, Mülmenstädt et al. (2021) found in ESM simulations that a 4 K increase in surface temperature led to an increase in warm-rain fraction over the SO, increasing the optical feedback component. However, they found that warm-rain precipitation efficiency was high-biased relative to satellite observations, thereby reducing the efficiency of the lifetime feedback component. By reducing the warm-rain probability in the ESM to better agree with satellite observations, they found that the lifetime feedback component was 3 times larger than that in the default model owing to an increase in liquid-water path.
Here, we find that warm clouds precipitate less frequently in GISS-ModelE3 relative to ground-based observations, which is inconsistent with literature consensus based on satellite observations. Such differing conclusions could arise for several reasons. First, we demonstrated the likelihood that satellite observations underestimate precipitation occurrence frequency relative to colocated ground-based observations. Figure 2h shows Pcb for all liquid-based clouds using the sensitivity and vertical resolution of BASTA and for CloudSat 2C-PC “certain” and “possible” products, where Pcb decreased from ∼70 % for BASTA to ∼35 % (“possible”) and 20 % (“certain”) for 2C-PC. Although the sensitivity and vertical resolution of CloudSat suggested by Fig. 2h do not account for CloudSat's data characteristics below 750 m a.g.l., this is roughly consistent with Tansey et al. (2022, see their Fig. 10), who showed that liquid-phase surface precipitation frequency decreased by ∼ 30 % in their ground-based dataset compared to CloudSat. This comparison also implies that the GISS-ModelE3 Pcb of 55 % could be larger than CloudSat suggests, but confirming that would require applying EMC2 to GISS-ModelE3 outputs with CloudSat rather than BASTA radar characteristics. Related to this point, established model–observation comparisons may consider substantially different conditions owing to sampling or methodology in general. For example, the true cloud base is very difficult to observe from spaceborne instrumentation, making clouds and precipitation somewhat ambiguous. Moreover, satellite studies have often focused on warm-rain processes (e.g., Suzuki et al., 2015; Jing et al., 2017; Mülmenstädt et al., 2021). Clouds with CTTs > 0 ∘C during MICRE accounted for a smaller fraction of the cloud population than supercooled clouds, and most often warm cloud bases were below CloudSat's 750 m a.g.l. threshold. Despite this, Kay et al. (2018) found that Southern Ocean supercooled cloud layers also produced snow too often in CESM1 relative to satellite observations, in contrast to our results. This leaves open the possibility that GISS-ModelE3 behaves differently from other ESMs, which could be verified by evaluating supercooled Southern Ocean clouds across multiple models to determine the prevalence of this reasoning. Reconciling these differing conclusions regarding ESM precipitation occurrence to which model results are sensitive (Mülmenstädt et al., 2021) will motivate further work to robustly evaluate models simultaneously against both ground-based observations and satellite observations while directly comparing ground-based and space-based observations, as demonstrated by Tansey et al. (2022). Additionally, ESM evaluation methodology using ground-based versus space-based simulators is worthy of further investigation since the results and conclusions drawn can be sensitive to the representation of model physics (e.g., Cesana et al., 2021).
This study also found that GISS-ModelE3 precipitation evaporates too frequently before it reaches within 250 m of the surface, which can be expected to influence the cloud condensate budget in a number of competing ways. For example, sub-cloud evaporation can act as a condensate sink by stabilizing the boundary layer (decreasing vertical mixing and cloud amount) but can also act as a source of moisture in turbulent regions, where the condensate is not entirely lost to the surface through precipitation and therefore is a moisture source for condensation to later occur.
Although we do not seek to actively address the model biases presented herein, these findings stress the importance of understanding cloud and precipitation properties from a process-oriented perspective and using a simulator approach to account for both observational limitations and consistency with model physics. We leave further in-depth assessment of the model's physical mechanisms responsible for model–observation differences for future work. Ideally, future analyses should evaluate thermodynamic and cloud conditions simultaneously over multiple sites in order to more robustly establish process-based mechanisms and link them to leading biases. Indeed, Fiddes et al. (2022) evaluated nudged simulations by the Australian Community Climate and Earth System Simulator (ACCESS) atmosphere model against satellite observations over the SO and found that even when cloud radiative biases were small on average, cloud properties such as cloud fraction and vertically integrated condensate can remain large.
5.2 Related studies
Tansey et al. (2022) analyzed the same year of MICRE data and found that surface precipitation occurs 44 ± 4 % of the time during the campaign. In the current study, a cloud occurrence frequency of 76 % and a Psfc of 54 % (Table 1) imply a campaign-long surface precipitation occurrence frequency of ∼ 41 %, indicating good agreement with their study. Tansey et al. (2022) found precipitation to be primarily composed of small particles (< 1 mm in diameter) and found a significant contribution from light-rain rates (< 0.5 mm h−1) that accounted for 11 % of accumulated surface precipitation. Similar contributions by light-rain rates were documented by Wang et al. (2015).
Similar observational analyses have been performed at other geographic locations. For example, Silber et al. (2021a) documented the Pcb of supercooled liquid-bearing layers at an Antarctic site (McMurdo Station, Antarctica) during the ARM West Antarctic Radiation Experiment (AWARE; Lubin et al., 2020b) and at an Arctic site (NSA). They used soundings with an RHliq threshold to identify cloud boundaries combined with the ARM Ka-band zenith radar (KAZR; Widener et al., 2012) at both polar sites to detect sub-cloud precipitation. They found that 85 % (75 %) of supercooled clouds were precipitating from the LCB at the Arctic (Antarctic) site. McMurdo Station is located at 77.8 ∘S and 166.7 ∘E, roughly 22.5∘ south and 8∘ east of Macquarie Island. We note that KAZRs have sensitivities around −50 dBZ at 1 km a.g.l. (compared to −36 dBZ for BASTA during MICRE), although their hmin is typically higher (e.g., Silber et al., 2021a). When considering only Ze > −36 dBZ (below which supercooled clouds in this study are insensitive; see Fig. 10), the Pcb at McMurdo Station from supercooled cloud layers per Silber et al. (2021a, see their Fig. 1b) was ∼ 70 %, while in MICRE it was ∼ 61 % (see Fig. 2). Different cloud morphologies exist between Macquarie Island and McMurdo Station, even for supercooled layers, due to Macquarie Island's location north of the polar front, a shift towards more frequent supercooled clouds further south, and potential effects of terrain at McMurdo Station. The 9 % absolute difference in supercooled-cloud Pcb between the stations may also lie within their summed uncertainties owing to relatively short deployments for the purposes of a climatology.
Lamer et al. (2020b) used 3 years of data from the ARM ENA observatory to evaluate cloud and precipitation properties in post-cold frontal subsidence regimes using a ceilometer and a radar, also taking a similar approach. They found 80 % of cloud layers in subsidence regimes to be precipitating. The higher Pcb of 80 % over ENA compared to MICRE may be due to the requirement that their cloud layers produce detectable reflectivity above the lidar-identified cloud base, whereas here we also consider optically thin, non-precipitating clouds without coincident radar reflectivity above the noise floor, which increases the normalizing cloud population in our study. They also related cloud geometric thickness to Pcb and Rcb and found that Pcb increases with increasing cloud geometric thickness, which is consistent with this study and results in Silber et al. (2021a). Rcb also increased with increasing cloud thickness in Lamer et al. (2020b), agreeing with our study and following from other observational studies suggesting that Rcb scales with cloud thickness (e.g., Yang et al., 2018; vanZanten et al., 2005). Also similar to our study, Lamer et al. (2020b) found a higher likelihood for precipitation to reach the surface from deeper cloud layers.
5.3 Implications for satellites
Silber et al. (2021a) reconciled discrepancies between ground-based observations indicating that polar supercooled clouds are nearly continuously precipitating lightly from the LCB and have much lower precipitation frequencies derived from spaceborne instruments, based on differences in radar sensitivity and vertical resolution. Here we find a similar importance of radar sensitivity (Fig. 2) spanning clouds with both supercooled and warm CBTs, especially for Ze values that represent the weakest precipitation fluxes. Satellite observing platforms experience ground clutter near the surface and are thus unable to detect clouds within the lowest 0.75–1 km a.g.l. During MICRE, the majority of warm-based clouds and a large fraction of supercooled clouds have CBHs < 1 km (Fig. 3). This high frequency of CBHs < 1 km suggests severe limitations for detection of cloud-base precipitation from current spaceborne instrumentation. Indeed, CloudSat's 2C-PC “possible” = −15 dBZ and vertical resolution (Dmin) = 250 m would yield a Pcb = 40 %, nearly 30 percentage points lower than shown here from BASTA (see Fig. 2a). As discussed by Silber et al. (2021a), the future EarthCARE mission's Cloud Profiling Radar will be more sensitive and better at establishing light-precipitation processes (Kollias et al., 2014; Illingworth et al., 2015). However, given that all current and future ground-based and satellite instrument datasets will have limitations in terms of geographical and temporal coverage, instrument resolution and sensitivity, and factors such as attenuation and ground clutter, a simulator approach provides a robust pathway to enable fusion of spaceborne and ground-based platforms for reliable model evaluation, as pioneered by tools such as the second version of the Cloud Feedback Model Intercomparison Project Observational Simulator Package (COSP2; Swales et al., 2018).
5.4 Caveats and guidance for future Southern Ocean campaigns
Macquarie Island's latitude of 54.5 ∘S is not necessarily expected to be representative of the vast SO. For example, Fiddes et al. (2022) split the SO into three latitudinally bound regions and found that model biases in cloud phase and morphology were different among the three regions. Expansion of the results here to other latitudes should be the focus of future work. In addition, we note that Tansey et al. (2022) documented that MICRE summer surface precipitation was anomalously high relative to a long-term tipping-bucket record from Macquarie Island, indicating the need for more routine measurement platforms over the SO and robust satellite supplementation in order to place the results of this study within the context of the broader Macquarie Island and SO climatologies.
Finally, this study illustrates a number of needs for future ground-based missions over the SO. Longer deployments (order of years) are needed to robustly characterize the cloud climatology and seasonal variability over Macquarie Island. The cloud properties presented herein could be more robustly analyzed with higher-capability lidar instrumentation. Although polarization lidar capability was present during MICRE, it was not available for statistical evaluation. Verifying the phase of cloud-base detections via polarization lidar is needed since this is difficult to determine through ceilometer attenuated backscatter alone, though Guyot et al. (2022) demonstrated a data-driven approach to classify cloud phase based on ceilometer attenuated backscatter gradients. We also note that BASTA's sensitivity would have been higher during MICRE had the low-noise amplifier been operational (see Appendix B). In particular, determining the height of the LCB presented a leading uncertainty in this study, including that associated with the proprietary vendor algorithm used to detect LCB height. Low cloud bases within the radar “blind zone” should also be investigated further over the SO (e.g., Maahn et al., 2014; Kuma et al., 2020). For example, Alexander and Protat (2018) found that ∼ 15 % of lidar-identified cloud bases at Cape Grim, Tasmania (40.7 ∘S, 144.7 ∘E), were below 200 m a.g.l. During MICRE, about a quarter of ceilometer-identified CBHs were below 250 m. Over half of these surface-based clouds during MICRE were representative of fog, which with the exception of Kuma et al. (2020) has not been extensively studied over the SO and also deserves further investigation.
This study evaluated cloud and precipitation properties using ground-based profiling instrumentation at the Southern Ocean's Macquarie Island (54.5 ∘S, 158.9 ∘E) during ∼ 1 year of the MICRE field campaign. A merged dataset from a 95 GHz (W-band) cloud radar, ceilometers, and atmospheric soundings was constructed to analyze cloud and precipitation occurrence frequencies and their dependence on cloud thermodynamics and macrophysics. A 9-year simulation of the NASA GISS-ModelE3 ESM was then evaluated against the MICRE observations by extracting outputs at every time step in the grid cell containing Macquarie Island. Forward simulation of the GISS-ModelE3 ceilometer and radar variables was performed via the Earth Model Column Collaboratory (EMC2) radar and lidar instrument simulator, accounting for the sensitivities of the instrumentation deployed during MICRE. This approach yielded a comparison of observations and the ESM in a physically consistent framework. The main conclusions resulting from the observational analysis and the ESM evaluation are as follows:
-
Clouds precipitate frequently from the liquid cloud base over Macquarie Island (∼ 70 % of the time where cloud bases were identified).
-
Deeper and colder clouds precipitate more frequently and at a higher intensity than thinner and warmer clouds.
-
Clouds with colder CTTs precipitate more readily than at warm CTTs even for the same cloud geometric thickness.
-
Supercooled cloud layers experience more frequent evaporation/sublimation below the LCB compared to warm cloud layers.
-
The GISS-ModelE3 ESM simulation produced a smaller liquid-based cloud occurrence frequency, smaller precipitation occurrence frequency, and greater sub-cloud evaporation/sublimation compared to observations.
-
GISS-ModelE3 captures observed trends (shape and skewness) in cloud-base precipitation rate distributions whereby precipitation rates increase with decreasing CTT and increasing cloud thickness.
-
Geometrically and optically thin non-precipitating clouds and fog were similarly common in both observations and GISS-ModelE3.
The ESM evaluation demonstrated here followed a framework in which ESM column physics may be evaluated while remaining faithful to the model's physics parameterizations and accounting for instrument sensitivities. Systematic biases observed in GISS-ModelE3 (i.e, lower precipitation occurrence frequencies and more evaporation relative to MICRE observations) are unlikely to result from thresholding behavior for cloud-base precipitation detection since the biases are consistent for various minimum radar reflectivity thresholds used to qualify precipitation. Further work is needed in order to better understand these differences as they apply to GISS-ModelE3 and to other ESMs with different physics parameterizations. However, this study demonstrates that long-term, ground-based instrumentation can be used as a robust process-level constraint for ESM evaluation of precipitation occurrence when appropriate sensitivities are considered. Indeed, Mülmenstädt et al. (2021) argue that, for warm clouds, identifying the presence of precipitation can be a proxy for a simple binary estimate of the autoconversion process, which is parameterized in models in a manner that produces a process rate. Such process-driven studies are important to understand cloud and precipitation properties in the present-day atmosphere as well as for perturbed climates and how they may compensate, enhance, or reduce cloud radiative feedbacks in the extratropics.
βatt | 910 nm ceilometer attenuated backscatter (m−1 sr−1) |
BASTA | Bistatic Radar System for Atmospheric Sciences (Delanoë et al., 2016) |
CBH | Cloud-base height |
CTH | Cloud-top height |
CBT | Cloud-base temperature |
CTT | Cloud-top temperature |
CEIL | Merged ARM and University of Canterbury ceilometer datasets |
Dmin | Depth below the cloud base used for cloud-base precipitation detection, depth above hmin used for surface precipitation detection |
EMC2 | Earth Model Column Collaboratory instrument simulator (Silber et al., 2022) |
GISS-ModelE3 | US National Aeronautics and Space Administration (NASA) Goddard Institute for Space Studies ModelE3 |
hmin | Minimum detectable height of the BASTA radar, set to 150 m a.g.l. |
LCB | Liquid cloud base |
Pcb | Cloud-base precipitation occurrence frequency |
Psfc | Surface precipitation occurrence frequency (surface is hmin = 150 m a.g.l.) |
Rcb | Cloud-base precipitation rate |
RHliq | Relative humidity with respect to liquid water |
RHsfc | Relative humidity from surface meteorology station |
RHice | Relative humidity with respect to ice |
Ze | W-band (95 GHz) radar reflectivity (dBZ) |
Ze,min | Minimum detectable Ze |
Linearly averaged reflectivity between 150 and 250 m a.g.l. |
The BASTA radar reports a of −36 dBZ at 1 km a.g.l. Figure B1 shows the theoretical as a dashed black line, which is calculated assuming irradiance weakens inversely proportionally to the square of range, while the light-blue line shows the 0.01st percentile of BASTA Ze from the year during MICRE when BASTA was operational. Range gates where the reflectivity as a function of height is less than the theoretical are assumed to be free of hydrometeors. Importantly, we note that the BoM BASTA radar used here as reported by Delanoë et al. (2016) and also used by Mace and Protat (2018a, b) nominally reports a sensitivity of −49 dBZ at 1 km a.g.l. (−36 dBZ at 4 km a.g.l.). However, issues with the low-noise amplifier during MICRE degraded the BoM BASTA sensitivity to −36 dBZ at 1 km a.g.l. We emphasize that while BASTA detects Ze values down to −55 dBZ near hmin (150 m a.g.l.), the sensitivity below 1 km a.g.l. decreases rapidly with increasing range. Therefore, precipitation detection throughout the lowest 1 km a.g.l. is not the same as a more sensitive radar with a minimum detectable signal of ∼ −55 dBZ over the entire 1 km depth.
Following from the finding in Silber et al. (2018) that the ARM ceilometer tends to overestimate true LCB height by 36–50 m relative to other observing methods, Pcb is recalculated by offsetting the native CEIL-identified CBH downwards by 25 or 50 m (one to two BASTA range gates). Pcb is shown for these modified calculations in Fig. C1, where lowering the CBH by 25 (50) m decreases the total Pcb at the highest sensitivity by 2 (5) %. Sensitivities to and Dmin remain consistent with these offset CBHs. In general, offsetting the cloud base decreases the total Pcb, but the sensitivity is small.
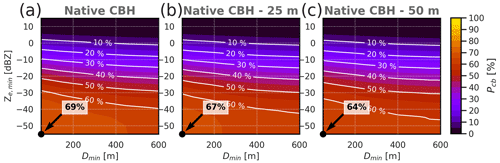
Figure C1Cloud-base precipitation occurrence frequency (Pcb, contours and color fill) as a function of threshold (ordinate) and vertical resolution (Dmin, abscissa) for all cloud layers using (a) the native CEIL-recognized CBH, (b) the native CBH offset by 25 m, and (c) the native CBH offset by 50 m. The black circles in the bottom left-hand corner of each panel represent the BASTA and Dmin = 50 m (two range gates).
Evaluation of ceilometer CBHs was performed by co-locating in time with soundings released nominally at 12 h intervals during MICRE. Figure D1 shows a joint histogram of RHliq and temperature at heights where the ceilometer recognized a CBH within 20 min after a sounding release time. There is a clear maximum in frequency for RHliq > 98 %. Following from Silber et al. (2021a) and assuming an RHliq uncertainty of 5 %, we consider a liquid-bearing cloud layer to have RHliq > 95 % in the sounding. In Fig. 1, altitude ranges where RHliq > 95 % are identified by transparent purple shading in the sounding profile and in the BASTA time–height series, with the sounding-based CBH and CTH shown as dark-purple lines. Figure 1d shows that this RHliq threshold appropriately identifies a sounding-based CBH where the ceilometer identifies a CBH and that the sounding-identified CTH is correctly located at the top of the radar reflectivity hydrometeor-containing layer. The low-frequency scatter of ceilometer CBHs with RHliq < 95 % in Fig. D1 is due to heterogeneity in the vertical placement of the liquid layer that causes spatiotemporal discrepancies between the cloud environment sampled by CEIL and by the sounding. Overall, 66 (80) % of soundings with colocated CEIL-identified CBHs obtained RHliq values > 95 (90) %.
The partitioning of supercooled versus warm-based (i.e., warm + partially supercooled) cloud layers is a strong function of Dmin (Fig. E1). At Dmin = 50 m, ∼ 55 % of detected clouds are supercooled, while ∼ 45 % of clouds have warm CBTs. At Dmin = 600 m, the fraction of cloud layers identified as supercooled increases (decreases) to ∼ 85 % (15 %) for supercooled (warm-based) clouds. This is due to the higher Dmin threshold limiting the number of clouds that can be detected below the minimum detectable CBH (i.e., Dmin + hmin). For a minimum CBH of 750 m, a large fraction of warm-based cloud layers are omitted from the analysis, and the total sample size of clouds capable of cloud-base precipitation detection decreases.
Generalized cloud morphologies are recognized during MICRE as representative of fog, where two primary cloud environments are demonstrated in Figs. F1 and F2. The first case (Fig. F1) is representative of a moderate- to heavy-precipitation event with intermittent periods of precipitation breaks. In these intermittent periods (e.g., ∼ 06:00 UTC), a shallow cloud layer is notable in the radar reflectivity that reaches 400 m a.g.l. (Fig. F1c, d). The RHsfc exceeds 95% during this time period (Fig. F1f), and the two soundings released during the event show completely saturated layers through at least 1 km a.g.l. (Fig. F1a, b). The University of Canterbury ceilometer apparent βatt (Fig. F1e) shows a persistent period from 00:00 to 14:00 UTC with CBHs very close to the surface (within the BASTA “blind zone”) where the signal is entirely attenuated above ∼ 125 m a.g.l.
The second example is a more traditional fog layer (Fig. F2). The CEIL apparent βatt profiles begin with enhanced values > 10−6 m−1 sr−1 without coincident radar reflectivity, which may be deliquescent aerosol (haze), before developing a surface-based cloud where apparent βatt values exceed 10−4 m−1 sr−1, and radar echoes develop (∼ 19:00 UTC on 22 May). RHsfc then exceeds 95 %, and the last sounding shows a liquid-saturated layer up through 800 m a.g.l. The CBHs in this case are not as consistent as in Fig. F1, with CBHs often being detected up to 400 m a.g.l. Also note instances (e.g., ∼ 09:00 UTC on 23 May) where shallow convection appears to rise out of the fog layer.
Regardless of the formation mechanism, these βatt profiles and their physical implications account for a large portion of cloud bases identified by CEIL (18 %; see Fig. 8). Although such profiles may be regarded as contamination of the ceilometer signal, they are coincident with RHsfc > 95 %, suggesting a prevalence of fog over this SO site with true cloud bases near the surface, and thus the relevant physical formation mechanisms should be represented by model physics.
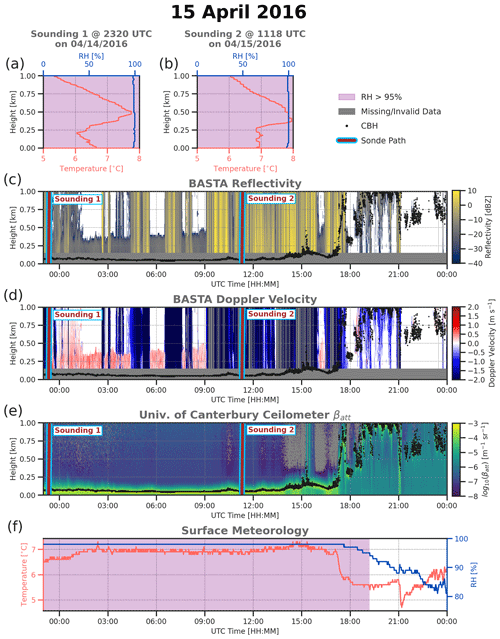
Figure F1Summary of a fog event that occurred during moderate to heavy precipitation on 15 April 2016: (a) temperature and RH for a sounding released at 23:20 UTC on 14 April 2016, (b) as in (a) but for a sounding released at 11:18 UTC on 15 April 2016, (c) 24 h time–height series of BASTA radar reflectivity, (d) as in (c) but for BASTA Doppler velocity, (e) as in (c) but for apparent βatt from the University of Canterbury ceilometer, and (f) a time series of RHsfc and Tsfc from the surface meteorological station. In (a), (b), and (f), times and heights where RH > 95 % are shaded in purple. CBHs from CEIL are given as black dots in panels (c)–(e). Sounding release times are marked in (c)–(e) via brown lines with a light-blue outline.
Cloud and precipitation occurrence frequencies may be sensitive to certain thresholding behavior in the model. LCB detection in GISS-ModelE3 is performed by identifying the lowest grid cell in altitude where CLWC exists. An arbitrary lower threshold for the statistics discussed here is found to be unnecessary for representing cloud occurrence frequency, which only decreases by a few percent between grid-cell mean CLWC values of 10−9 to 10−4 g m−3 (Fig. G1a). Similarly, the cloud occurrence frequency is shown to be insensitive for βatt < ∼ 10−5 m−1 sr−1 (top axis of Fig. G1a).
The detection of precipitation relies on the existence of a precipitating hydrometeor species within the grid cell identified as the cloud base, no matter how small the Rcb is in that grid cell. However, Fig. G1b shows that the precipitating fraction only decreases by ∼ 2.5 % for a range of Rcb from 10−12 to 10−6 mm h−1. This implies that the precipitation occurrence frequency is also not very sensitive to relevant minimum Rcb thresholds we expect to observe in nature.
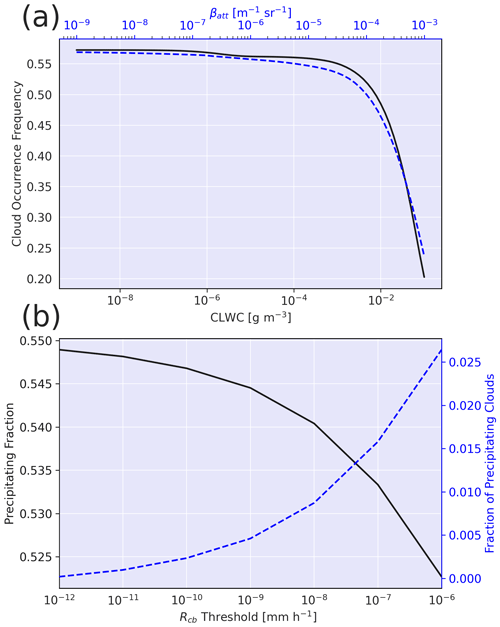
Figure G1Sensitivities of (a) cloud occurrence frequency to thresholding of grid-cell mean cloud liquid-water content (CLWC, solid black line) and ceilometer attenuated backscatter (βatt, dashed blue line) and (b) precipitating fraction to minimum Rcb threshold (black line). In (b), the fraction of precipitating clouds as a function of the Rcb threshold is shown as a dashed blue line.
Department of Energy (DOE) Atmospheric Radiation Measurement (ARM) program ceilometer data (https://doi.org/10.5439/1181954, Morris et al., 2016) and Australian BoM surface meteorology station data (https://doi.org/10.5439/1597382, Howie and Protat, 2016) are available through the DOE ARM data archive (https://adc.arm.gov/, last access: 1 February 2023). BASTA radar data (https://doi.org/10.26179/5d91836ca8fc3, Alexander, 2019) and the University of Canterbury ceilometer data (https://doi.org/10.26179/5d91835e2ccc3, Alexander and McDonald, 2020) are accessible through the Australian Antarctic Division’s Data Centre (https://data.aad.gov.au, last access: 1 February 2023). Upper-air soundings from the Australian BoM are available via online request at https://data.aad.gov.au/metadata/records/Antarctic_Meteorology (Barnes-Keoghan, 2000a). VISST-derived pixel-level products from the Himawari-8 satellite are available on the ARM Data Discovery website (https://adc.arm.gov/discovery/#/results/site_code::mcq, ARM User Facility, 2016). The Earth Model Column Collaboratory (EMC2) software package is available at https://doi.org/10.5281/zenodo.5115252 (Silber et al., 2021b). Code used for processing and scripts used to make all figures are available at https://doi.org/10.5281/zenodo.8231170 (Stanford, 2023).
MS, IS, and AF conceptualized the study. MS prepared and analyzed the observational datasets with assistance from IS. IS performed the GISS-ModelE3 simulations with assistance from AA and supported use of the EMC2 software. MS performed the model evaluation and drafted the manuscript, with subsequent input from all co-authors. AA contributed to interpretation of results. GC and JM aided in interpreting results as they relate to GISS-ModelE3 evaluation and cloud–climate feedbacks. AP provided the BASTA radar data. SA and AM provided the University of Canterbury ceilometer data. SA conceptualized the overall science goals for MICRE, secured funding and logistical support, and led the field deployment to Macquarie Island.
The contact author has declared that none of the authors has any competing interests.
Publisher’s note: Copernicus Publications remains neutral with regard to jurisdictional claims in published maps and institutional affiliations.
We thank Emily Tansey and Roger Marchand for their valuable discussions. Computing resources supporting this work were provided by the NASA Center for Climate Simulation (NCCS) at Goddard Space Flight Center. Technical and logistical support for MICRE was provided by the Australian Antarctic Division through Australian Antarctic Science projects 4292 and 4387, and we thank Andrew Klekociuk, John French, Peter de Vries, Terry Egan, Nick Cartwright, and Ken Barrett for all of their assistance.
This work was supported by the Office of Science (BER), US Department of Energy (DOE), under agreements DE-SC0016237 and 89243021SSC000078, and the NASA Modeling, Analysis, and Prediction Program. Israel Silber was supported by DOE grant DE-SC0021004. Johannes Mülmenstädt was supported by the Atmospheric System Research (ASR) program of DOE BER under Pacific Northwest National Laboratory (PNNL) project 57131; PNNL is operated for DOE by the Battelle Memorial Institute under contract DE-A06-76RLO 1830.
This paper was edited by Matthew Lebsock and reviewed by Mark Miller and Roger Marchand.
Abdul-Razzak, H., Ghan, S. J., and Rivera-Carpio, C.: A parameterization of aerosol activation: 1. Single aerosol type, J. Geophys. Res.-Atmos., 103, 6123–6131, https://doi.org/10.1029/97JD03735, 1998. a
Adams, N.: Climate trends at Macquarie Island and expectations of future climate change in the sub-Antarctic, Papers Proc. Roy. Soc. Tasmania, 143, 1–8, https://doi.org/10.26749/RSTPP.143.1.1, 2009. a
Alexander, S.: BASTA Cloud Radar data from Macquarie Island, 2016–2017, Ver. 1, Australian Antarctic Data Centre [data set], https://doi.org/10.26179/5d91836ca8fc3, 2019. a
Alexander, S. and McDonald, A.: University of Canterbury's Vaisala CL51 Ceilometer at Macquarie Island 2016–2018, Australian Antarctic Data Centre [data set], https://doi.org/10.26179/5d91835e2ccc3, 2019. a
Alexander, S. and McDonald, A.: University of Canterbury's Vaisala CL51 Ceilometer at Macquarie Island 2016–2018, Ver. 1, Australian Antarctic Data Centre [data set], https://doi.org/10.26179/5d91835e2ccc3, 2020. a
Alexander, S. P. and Protat, A.: Cloud Properties Observed From the Surface and by Satellite at the Northern Edge of the Southern Ocean, J. Geophys. Res.-Atmos., 123, 443–456, https://doi.org/10.1002/2017JD026552, 2018. a, b
Alexander, S. P., McFarquhar, G. M., Marchand, R., Protat, A., Vignon, Mace, G. G., and Klekociuk, A. R.: Mixed-Phase Clouds and Precipitation in Southern Ocean Cyclones and Cloud Systems Observed Poleward of 64∘S by Ship-Based Cloud Radar and Lidar, J. Geophys. Res.-Atmos., 126, e2020JD033626, https://doi.org/10.1029/2020JD033626, 2021. a
Ansmann, A., Mamouri, R.-E., Bühl, J., Seifert, P., Engelmann, R., Hofer, J., Nisantzi, A., Atkinson, J. D., Kanji, Z. A., Sierau, B., Vrekoussis, M., and Sciare, J.: Ice-nucleating particle versus ice crystal number concentrationin altocumulus and cirrus layers embedded in Saharan dust:a closure study, Atmos. Chem. Phys., 19, 15087–15115, https://doi.org/10.5194/acp-19-15087-2019, 2019. a
ARM User Facility: Minnis Cloud Products Using Visst Algorithm (VISSTPXHI8MINNIS), Atmospheric Radiation Measurement (ARM) user facility [data set], https://adc.arm.gov/discovery/#/results/site_code::mcq (last access: 1 February 2023), 2016. a, b
Austin, P. M. and Bemis, A. C.: A Quantitative Study Of The “Bright Band” In Radar Precipitation Echoes, J. Atmos. Sci., 7, 145–151, https://doi.org/10.1175/1520-0469(1950)007<0145:AQSOTB>2.0.CO;2, 1950. a
Barnes-Keoghan, I.: Antarctic Climate Data Collected by Australian Agencies, Ver. 1, Australian Antarctic Data Centre [data set], https://data.aad.gov.au/metadata/records/Antarctic_Meteorology (last access: 18 April 2021), 2000a. a, b
Bodas-Salcedo, A., Williams, K. D., Field, P. R., and Lock, A. P.: The Surface Downwelling Solar Radiation Surplus over the Southern Ocean in the Met Office Model: The Role of Midlatitude Cyclone Clouds, J. Climate, 25, 7467–7486, https://doi.org/10.1175/JCLI-D-11-00702.1, 2012. a
Bodas-Salcedo, A., Williams, K. D., Ringer, M. A., Beau, I., Cole, J. N., Dufresne, J. L., Koshiro, T., Stevens, B., Wang, Z., and Yokohata, T.: Origins of the solar radiation biases over the Southern Ocean in CFMIP2 models, J. Climate, 27, 41–56, https://doi.org/10.1175/JCLI-D-13-00169.1, 2014. a, b
Bodas-Salcedo, A., Andrews, T., Karmalkar, A. V., and Ringer, M. A.: Cloud liquid water path and radiative feedbacks over the Southern Ocean, Geophys. Res. Lett., 43, 938–10, https://doi.org/10.1002/2016GL070770, 2016. a, b
Bühl, J., Seifert, P., Myagkov, A., and Ansmann, A.: Measuring ice- and liquid-water properties in mixed-phase cloud layers at the Leipzig Cloudnet station, Atmos. Chem. Phys., 16, 10609–10620, https://doi.org/10.5194/acp-16-10609-2016, 2016. a, b, c
Bühl, J., Seifert, P., Radenz, M., Baars, H., and Ansmann, A.: Ice crystal number concentration from lidar, cloud radar and radar wind profiler measurements, Atmos. Meas. Tech., 12, 6601–6617, https://doi.org/10.5194/amt-12-6601-2019, 2019. a, b
Caldwell, P. M., Zelinka, M. D., Taylor, K. E., and Marvel, K.: Quantifying the Sources of Intermodel Spread in Equilibrium Climate Sensitivity, J. Climate, 29, 513–524, https://doi.org/10.1175/JCLI-D-15-0352.1, 2016. a
Cesana, G. and Chepfer, H.: Evaluation of the cloud thermodynamic phase in a climate model using CALIPSO-GOCCP, J. Geophys. Res.-Atmos., 118, 7922–7937, https://doi.org/10.1002/JGRD.50376, 2013. a
Cesana, G., Del Genio, A. D., and Chepfer, H.: The Cumulus And Stratocumulus CloudSat-CALIPSO Dataset (CASCCAD), Earth Syst. Sci. Data, 11, 1745–1764, https://doi.org/10.5194/essd-11-1745-2019, 2019a. a
Cesana, G., Del Genio, A. D., Ackerman, A. S., Kelley, M., Elsaesser, G., Fridlind, A. M., Cheng, Y., and Yao, M.-S.: Evaluating models' response of tropical low clouds to SST forcings using CALIPSO observations, Atmos. Chem. Phys., 19, 2813–2832, https://doi.org/10.5194/acp-19-2813-2019, 2019b. a, b
Cesana, G. V., Ackerman, A. S., Fridlind, A. M., Silber, I., and Kelley, M.: Snow Reconciles Observed and Simulated Phase Partitioning and Increases Cloud Feedback, Geophys. Res. Lett., 48, e2021GL094876, https://doi.org/10.1029/2021GL094876, 2021. a, b, c, d
Cesana, G. V., Khadir, T., Chepfer, H., and Chiriaco, M.: Southern Ocean Solar Reflection Biases in CMIP6 Models Linked to Cloud Phase and Vertical Structure Representations, Geophys. Res. Lett., 49, e2022GL099777, https://doi.org/10.1029/2022GL099777, 2022. a, b, c, d
Chubb, T. H., Jensen, J. B., Siems, S. T., and Manton, M. J.: In situ observations of supercooled liquid clouds over the Southern Ocean during the HIAPER Pole-to-Pole Observation campaigns, Geophys. Res. Lett., 40, 5280–5285, https://doi.org/10.1002/grl.50986, 2013. a
Comstock, K. K., Wood, R., Yuter, S. E., and Bretherton, C. S.: Reflectivity and rain rate in and below drizzling stratocumulus, Q. J. Roy. Meteorol. Soc., 130, 2891–2918, https://doi.org/10.1256/QJ.03.187, 2004. a, b
Delanoë, J., Protat, A., Vinson, J. P., Brett, W., Caudoux, C., Bertrand, F., du Chatelet, J. P., Hallali, R., Barthes, L., Haeffelin, M., and Dupont, J. C.: BASTA: A 95-GHz FMCW Doppler radar for cloud and fog studies, J. Atmos. Ocean. Technol., 33, 1023–1038, https://doi.org/10.1175/JTECH-D-15-0104.1, 2016. a, b, c, d
Fan, J., Ghan, S., Ovchinnikov, M., Liu, X., Rasch, P. J., and Korolev, A.: Representation of Arctic mixed-phase clouds and the Wegener-Bergeron-Findeisen process in climate models: Perspectives from a cloud-resolving study, J. Geophys. Res.-Atmos., 116, 0–07, https://doi.org/10.1029/2010JD015375, 2011. a
Fiddes, S. L., Protat, A., Mallet, M. D., Alexander, S. P., and Woodhouse, M. T.: Southern Ocean cloud and shortwave radiation biases in a nudged climate model simulation: does the model ever get it right?, Atmos. Chem. Phys., 22, 14603–14630, https://doi.org/10.5194/acp-22-14603-2022, 2022. a, b
Flato, G., Marotzke, J., Abiodun, B., Braconnot, P., Chou, S., Collins, W., Cox, P., Driouech, F., Emori, S., Eyring, V., Forest, C., Gleckler, P., Guilyardi, E., Jakob, C., Kattsov, V., Reason, C., and Rummukainen, M.: Evaluation of climate models, in: Climate Change 2013 the Physical Science Basis: Working Group I Contribution to the Fifth Assessment Report of the Intergovernmental Panel on Climate Change, vol. 9781107057999, 741–866, Cambridge University Press, https://doi.org/10.1017/CBO9781107415324.020, 2013. a
Fukuta, N. and Takahashi, T.: The Growth of Atmospheric Ice Crystals: A Summary of Findings in Vertical Supercooled Cloud Tunnel Studies, J. Atmos. Sci., 56, 1963–1979, https://doi.org/10.1175/1520-0469(1999)056<1963:TGOAIC>2.0.CO;2, 1999. a
Gates, W. L.: AN AMS CONTINUING SERIES: GLOBAL CHANGE–AMIP: The Atmospheric Model Intercomparison Project, B. Am. Meteorol. Soc., 73, 1962–1970, https://doi.org/10.1175/1520-0477(1992)073<1962:ATAMIP>2.0.CO;2, 1992. a
Gates, W. L., Boyle, J. S., Covey, C., Dease, C. G., Doutriaux, C. M., Drach, R. S., Fiorino, M., Gleckler, P. J., Hnilo, J. J., Marlais, S. M., Phillips, T. J., Potter, G. L., Santer, B. D., Sperber, K. R., Taylor, K. E., and Williams, D. N.: An Overview of the Results of the Atmospheric Model Intercomparison Project (AMIP I), B. Am. Meteorol. Soc., 80, 29–56, https://doi.org/10.1175/1520-0477(1999)080<0029:AOOTRO>2.0.CO;2, 1999. a
Gettelman, A. and Morrison, H.: Advanced Two-Moment Bulk Microphysics for Global Models. Part I: Off-Line Tests and Comparison with Other Schemes, J. Climate, 28, 1268–1287, https://doi.org/10.1175/JCLI-D-14-00102.1, 2015. a
Gettelman, A., Bardeen, C. G., McCluskey, C. S., Järvinen, E., Stith, J., Bretherton, C., McFarquhar, G., Twohy, C., D'Alessandro, J., and Wu, W.: Simulating Observations of Southern Ocean Clouds and Implications for Climate, J. Geophys. Res.-Atmos., 125, e2020JD032619, https://doi.org/10.1029/2020JD032619, 2020. a
Griesche, H. J., Ohneiser, K., Seifert, P., Radenz, M., Engelmann, R., and Ansmann, A.: Contrasting ice formation in Arctic clouds: surface-coupled vs. surface-decoupled clouds, Atmos. Chem. Phys., 21, 10357–10374, https://doi.org/10.5194/acp-21-10357-2021, 2021. a, b
Guyot, A., Protat, A., Alexander, S. P., Klekociuk, A. R., Kuma, P., and McDonald, A.: Detection of supercooled liquid water containing clouds with ceilometers: development and evaluation of deterministic and data-driven retrievals, Atmos. Meas. Tech., 15, 3663–3681, https://doi.org/10.5194/amt-15-3663-2022, 2022. a
Haynes, J. M., L'Ecuyer, T. S., Stephens, G. L., Miller, S. D., Mitrescu, C., Wood, N. B., and Tanelli, S.: Rainfall retrieval over the ocean with spaceborne W-band radar, J. Geophys. Res.-Atmos., 114, D00A22, https://doi.org/10.1029/2008JD009973, 2009. a
He, Y., Yi, F., Liu, F., Yin, Z., Yi, Y., Zhou, J., Yu, C., and Zhang, Y.: Natural Seeder-Feeder Process Originating From Mixed-Phase Clouds Observed With Polarization Lidar and Radiosonde at a Mid-Latitude Plain Site, J. Geophys. Res.-Atmos., 127, e2021JD036094, https://doi.org/10.1029/2021JD036094, 2022. a
Heymsfield, A. J., Schmitt, C., Chen, C. C. J., Bansemer, A., Gettelman, A., Field, P. R., and Liu, C.: Contributions of the Liquid and Ice Phases to Global Surface Precipitation: Observations and Global Climate Modeling, J. Atmos. Sci., 77, 2629–2648, https://doi.org/10.1175/JAS-D-19-0352.1, 2020. a
Hillman, B. R., Marchand, R. T., and Ackerman, T. P.: Sensitivities of Simulated Satellite Views of Clouds to Subgrid-Scale Overlap and Condensate Heterogeneity, J. Geophys. Res.-Atmos., 123, 7506–7529, https://doi.org/10.1029/2017JD027680, 2018. a
Hogan, R. J., Mittermaier, M. P., and Illingworth, A. J.: The Retrieval of Ice Water Content from Radar Reflectivity Factor and Temperature and Its Use in Evaluating a Mesoscale Model, J. Appl. Meteorol. Climatol., 45, 301–317, https://doi.org/10.1175/JAM2340.1, 2006. a
Holdridge, G.: Balloon-Borne Sounding System (SONDE) Instrument Handbook, ARM-TR-029, DOE Office of Science, Office of Biological and Environmental Research, United States, 38 pp., https://doi.org/10.2172/1020712, 2020. a
Hoose, C., Lohmann, U., Bennartz, R., Croft, B., and Lesins, G.: Global simulations of aerosol processing in clouds, Atmos. Chem. Phys., 8, 6939–6963, https://doi.org/10.5194/acp-8-6939-2008, 2008. a, b
Hopkin, E., Illingworth, A. J., Charlton-Perez, C., Westbrook, C. D., and Ballard, S.: A robust automated technique for operational calibration of ceilometers using the integrated backscatter from totally attenuating liquid clouds, Atmos. Meas. Tech., 12, 4131–4147, https://doi.org/10.5194/amt-12-4131-2019, 2019. a
Howie, J. and Protat, A.: Surface Meteorological Instrumentation (ABMMET), Atmospheric Radiation Measurement (ARM) user facility [data set], https://doi.org/10.5439/1597382, 2016. a, b
Huang, Y., Siems, S. T., Manton, M. J., Protat, A., and Delanoë, J.: A study on the low-altitude clouds over the Southern Ocean using the DARDAR-MASK, J. Geophys. Res.-Atmos., 117, D18204, https://doi.org/10.1029/2012JD017800, 2012. a
Hurrell, J. W., Holland, M. M., Gent, P. R., Ghan, S., Kay, J. E., Kushner, P. J., Lamarque, J. F., Large, W. G., Lawrence, D., Lindsay, K., Lipscomb, W. H., Long, M. C., Mahowald, N., Marsh, D. R., Neale, R. B., Rasch, P., Vavrus, S., Vertenstein, M., Bader, D., Collins, W. D., Hack, J. J., Kiehl, J., and Marshall, S.: The Community Earth System Model: A Framework for Collaborative Research, B. Am. Meteorol. Soc., 94, 1339–1360, https://doi.org/10.1175/BAMS-D-12-00121.1, 2013. a
Illingworth, A. J., Hogan, R. J., O'Connor, E. J., Bouniol, D., Brooks, M. E., Delanoë, J., Donovan, D. P., Eastment, J. D., Gaussiat, N., Goddard, J. W., Haeffelin, M., Klein Baltinik, H., Krasnov, O. A., Pelon, J., Piriou, J. M., Protat, A., Russchenberg, H. W., Seifert, A., Tompkins, A. M., van Zadelhoff, G. J., Vinit, F., Willen, U., Wilson, D. R., and Wrench, C. L.: Cloudnet: Continuous Evaluation of Cloud Profiles in Seven Operational Models Using Ground-Based Observations, B. Am. Meteorol. Soc., 88, 883–898, https://doi.org/10.1175/BAMS-88-6-883, 2007. a
Illingworth, A. J., Barker, H. W., Beljaars, A., Ceccaldi, M., Chepfer, H., Clerbaux, N., Cole, J., Delanoë, J., Domenech, C., Donovan, D. P., Fukuda, S., Hirakata, M., Hogan, R. J., Huenerbein, A., Kollias, P., Kubota, T., Nakajima, T., Nakajima, T. Y., Nishizawa, T., Ohno, Y., Okamoto, H., Oki, R., Sato, K., Satoh, M., Shephard, M. W., Velázquez-Blázquez, A., Wandinger, U., Wehr, T., and Van Zadelhoff, G. J.: The EarthCARE Satellite: The Next Step Forward in Global Measurements of Clouds, Aerosols, Precipitation, and Radiation, B. Am. Meteorol. Soc., 96, 1311–1332, https://doi.org/10.1175/BAMS-D-12-00227.1, 2015. a
Jing, X., Suzuki, K., Guo, H., Goto, D., Ogura, T., Koshiro, T., and Mülmenstädt, J.: A Multimodel Study on Warm Precipitation Biases in Global Models Compared to Satellite Observations, J. Geophys. Res.-Atmos., 122, 806–811, https://doi.org/10.1002/2017JD027310, 2017. a, b
Kang, L., Marchand, R. T., Wood, R., and McCoy, I. L.: Coalescence Scavenging Drives Droplet Number Concentration in Southern Ocean Low Clouds, Geophys. Res. Lett., 49, e2022GL097819, https://doi.org/10.1029/2022GL097819, 2022. a
Kay, J. E., Bourdages, L., Miller, N. B., Morrison, A., Yettella, V., Chepfer, H., and Eaton, B.: Evaluating and improving cloud phase in the Community Atmosphere Model version 5 using spaceborne lidar observations, J. Geophys. Res.-Atmos., 121, 4162–4176, https://doi.org/10.1002/2015JD024699, 2016a. a
Kay, J. E., Wall, C., Yettella, V., Medeiros, B., Hannay, C., Caldwell, P., and Bitz, C.: Global climate impacts of fixing the Southern Ocean shortwave radiation bias in the Community Earth System Model (CESM), J. Climate, 29, 4617–4636, https://doi.org/10.1175/JCLI-D-15-0358.1, 2016b. a, b
Kay, J. E., L'Ecuyer, T., Pendergrass, A., Chepfer, H., Guzman, R., and Yettella, V.: Scale-Aware and Definition-Aware Evaluation of Modeled Near-Surface Precipitation Frequency Using CloudSat Observations, J. Geophys. Res.-Atmos., 123, 4294–4309, https://doi.org/10.1002/2017JD028213, 2018. a, b, c
Kollias, P., Tanelli, S., Battaglia, A., and Tatarevic, A.: Evaluation of EarthCARE Cloud Profiling Radar Doppler Velocity Measurements in Particle Sedimentation Regimes, J. Atmos. Ocean. Technol., 31, 366–386, https://doi.org/10.1175/JTECH-D-11-00202.1, 2014. a
Kremser, S., Harvey, M., Kuma, P., Hartery, S., Saint-Macary, A., McGregor, J., Schuddeboom, A., von Hobe, M., Lennartz, S. T., Geddes, A., Querel, R., McDonald, A., Peltola, M., Sellegri, K., Silber, I., Law, C. S., Flynn, C. J., Marriner, A., Hill, T. C. J., DeMott, P. J., Hume, C. C., Plank, G., Graham, G., and Parsons, S.: Southern Ocean cloud and aerosol data: a compilation of measurements from the 2018 Southern Ocean Ross Sea Marine Ecosystems and Environment voyage, Earth Syst. Sci. Data, 13, 3115–3153, https://doi.org/10.5194/essd-13-3115-2021, 2021. a, b
Kuma, P., McDonald, A. J., Morgenstern, O., Alexander, S. P., Cassano, J. J., Garrett, S., Halla, J., Hartery, S., Harvey, M. J., Parsons, S., Plank, G., Varma, V., and Williams, J.: Evaluation of Southern Ocean cloud in the HadGEM3 general circulation model and MERRA-2 reanalysis using ship-based observations, Atmos. Chem. Phys., 20, 6607–6630, https://doi.org/10.5194/acp-20-6607-2020, 2020. a, b, c
Kuma, P., McDonald, A. J., Morgenstern, O., Querel, R., Silber, I., and Flynn, C. J.: Ground-based lidar processing and simulator framework for comparing models and observations (ALCF 1.0), Geosci. Model Dev., 14, 43–72, https://doi.org/10.5194/gmd-14-43-2021, 2021. a, b
Lamer, K., Kollias, P., Battaglia, A., and Preval, S.: Mind the gap – Part 1: Accurately locating warm marine boundary layer clouds and precipitation using spaceborne radars, Atmos. Meas. Tech., 13, 2363–2379, https://doi.org/10.5194/amt-13-2363-2020, 2020a. a
Lamer, K., Naud, C. M., and Booth, J. F.: Relationships Between Precipitation Properties and Large-Scale Conditions During Subsidence at the Eastern North Atlantic Observatory, J. Geophys. Res.-Atmos., 125, e2019JD031848, https://doi.org/10.1029/2019JD031848, 2020b. a, b, c, d, e, f
Lang, F., Huang, Y., Siems, S. T., and Manton, M. J.: Characteristics of the Marine Atmospheric Boundary Layer Over the Southern Ocean in Response to the Synoptic Forcing, J. Geophys. Res.-Atmos., 123, 7799–7820, https://doi.org/10.1029/2018JD028700, 2018. a
Lang, F., Huang, Y., Siems, S. T., and Manton, M. J.: Evidence of a Diurnal Cycle in Precipitation over the Southern Ocean as Observed at Macquarie Island, Atmosphere, 11, p. 181, https://doi.org/10.3390/ATMOS11020181, 2020. a, b
Liu, D., Liu, Q., Qi, L., and Fu, Y.: Oceanic single‐layer warm clouds missed by the Cloud Profiling Radar as inferred from MODIS and CALIOP measurements, J. Geophys. Res.-Atmos., 121, 947–12, https://doi.org/10.1002/2016JD025485, 2016. a
Lubin, D., Zhang, D., Silber, I., Scott, R. C., Kalogeras, P., Battaglia, A., Bromwich, D. H., Cadeddu, M., Eloranta, E., Fridlind, A., Frossard, A., Hines, K. M., Kneifel, S., Leaitch, W. R., Lin, W., Nicolas, J., Powers, H., Quinn, P. K., Rowe, P., Russell, L. M., Sharma, S., Verlinde, J., and Vogelmann, A. M.: The atmospheric radiation measurement (ARM) west antarctic radiation experiment, B. Am. Meteorol. Soc., 101, E1069–E1091, https://doi.org/10.1175/BAMS-D-18-0278.1, 2020a. a
Lubin, D., Zhang, D., Silber, I., Scott, R. C., Kalogeras, P., Battaglia, A., Bromwich, D. H., Cadeddu, M., Eloranta, E., Fridlind, A., Frossard, A., Hines, K. M., Kneifel, S., Leaitch, W. R., Lin, W., Nicolas, J., Powers, H., Quinn, P. K., Rowe, P., Russell, L. M., Sharma, S., Verlinde, J., and Vogelmann, A. M.: AWARE: The Atmospheric Radiation Measurement (ARM) West Antarctic Radiation Experiment, B. Am. Meteorol. Soc., 101, E1069–E1091, https://doi.org/10.1175/BAMS-D-18-0278.1, 2020b. a
Maahn, M., Burgard, C., Crewell, S., Gorodetskaya, I. V., Kneifel, S., Lhermitte, S., Van Tricht, K., and van Lipzig, N. P.: How does the spaceborne radar blind zone affect derived surface snowfall statistics in polar regions?, J. Geophys. Res.-Atmos., 119, 604–13, https://doi.org/10.1002/2014JD022079, 2014. a
Mace, G. G. and Protat, A.: Clouds over the Southern Ocean as Observed from the R/V Investigator during CAPRICORN. Part I: Cloud Occurrence and Phase Partitioning, J. Appl. Meteorol. Climatol., 57, 1783–1803, https://doi.org/10.1175/JAMC-D-17-0194.1, 2018a. a, b, c, d, e, f, g, h, i
Mace, G. G. and Protat, A.: Clouds over the Southern Ocean as Observed from the R/V Investigator during CAPRICORN. Part II: The Properties of Nonprecipitating Stratocumulus, J. Appl. Meteorol. Climatol., 57, 1805–1823, https://doi.org/10.1175/JAMC-D-17-0195.1, 2018b. a, b, c
Mace, G. G., Zhang, Q., Vaughan, M., Marchand, R., Stephens, G., Trepte, C., and Winker, D.: A description of hydrometeor layer occurrence statistics derived from the first year of merged Cloudsat and CALIPSO data, J. Geophys. Res.-Atmos., 114, D00A26, https://doi.org/10.1029/2007JD009755, 2009. a
Mace, G. G., Protat, A., Humphries, R. S., Alexander, S. P., McRobert, I. M., Ward, J., Selleck, P., Keywood, M., and McFarquhar, G. M.: Southern Ocean Cloud Properties Derived From CAPRICORN and MARCUS Data, J. Geophys. Res.-Atmos., 126, e2020JD033368, https://doi.org/10.1029/2020JD033368, 2021. a
Mallet, M. D., Humphries, R. S., Fiddes, S. L., Alexander, S. P., Altieri, K., Angot, H., Anilkumar, N., Bartels-Rausch, T., Creamean, J., Dall’Osto, M., Dommergue, A., Frey, M., Henning, S., Lannuzel, D., Lapere, R., Mace, G. G., Mahajan, A. S., McFarquhar, G. M., Meiners, K. M., Miljevic, B., Peeken, I., Protat, A., Schmale, J., Steiner, N., Sellegri, K., Simó, R., Thomas, J. L., Willis, M. D., Winton, V. H. L., and Woodhouse, M. T.: Untangling the influence of Antarctic and Southern Ocean life on clouds, Elementa: Sci. Anthropoc., 11, 1, https://doi.org/10.1525/ELEMENTA.2022.00130, 2023. a
McCoy, D. T., Field, P., Bodas-Salcedo, A., Elsaesser, G. S., and Zelinka, M. D.: A regime-oriented approach to observationally constraining extratropical shortwave cloud Feedbacks, J. Climate, 33, 9967–9983, https://doi.org/10.1175/JCLI-D-19-0987.1, 2020. a, b
McErlich, C., McDonald, A., Schuddeboom, A., and Silber, I.: Comparing Satellite- and Ground-Based Observations of Cloud Occurrence Over High Southern Latitudes, J. Geophys. Res.-Atmos., 126, e2020JD033607, https://doi.org/10.1029/2020JD033607, 2021. a
McFarquhar, G. M., Bretherton, C. S., Marchand, R., Protat, A., DeMott, P. J., Alexander, S. P., Roberts, G. C., Twohy, C. H., Toohey, D., Siems, S., Huang, Y., Wood, R., Rauber, R. M., Lasher-Trapp, S., Jensen, J., Stith, J. L., Mace, J., Um, J., Järvinen, E., Schnaiter, M., Gettelman, A., Sanchez, K. J., McCluskey, C. S., Russell, L. M., McCoy, I. L., Atlas, R. L., Bardeen, C. G., Moore, K. A., Hill, T. C., Humphries, R. S., Keywood, M. D., Ristovski, Z., Cravigan, L., Schofield, R., Fairall, C., Mallet, M. D., Kreidenweis, S. M., Rainwater, B., D'Alessandro, J., Wang, Y., Wu, W., Saliba, G., Levin, E. J., Ding, S., Lang, F., Truong, S. C., Wolff, C., Haggerty, J., Harvey, M. J., Klekociuk, A. R., and McDonald, A.: Observations of Clouds, Aerosols, Precipitation, and Surface Radiation over the Southern Ocean: An Overview of CAPRICORN, MARCUS, MICRE, and SOCRATES, B. Am. Meteorol. Soc., 102, E894–E928, https://doi.org/10.1175/BAMS-D-20-0132.1, 2021. a, b, c, d, e, f
Mitchell, J., Senior, C. A., and Ingram, W. J.: CO2 and climate: a missing feedback?, Nature, 341, 132–134, https://doi.org/10.1038/341132a0, 1989. a, b, c
Morris, V., Zhang, D., and Ermold, B.: Ceilometer (CEIL), Atmospheric Radiation Measurement (ARM) user facility [data set], https://doi.org/10.5439/1181954, 2016. a, b
Morris, V. R.: Ceilometer Instrument Handbook, ARM-TR-020, Tech. rep., DOE Office of Science, Office of Biological and Environmental Research, United States, 26 pp., https://doi.org/10.2172/1036530, 2016. a, b, c
Morrison, A. E., Siems, S. T., and Manton, M. J.: A three-year climatology of cloud-top phase over the Southern Ocean and North Pacific, J. Climate, 24, 2405–2418, https://doi.org/10.1175/2010JCLI3842.1, 2011. a
Mülmenstädt, J., Salzmann, M., Kay, J. E., Zelinka, M. D., Ma, P.-L., Nam, C., Kretzschmar, J., Hörnig, S., and Quaas, J.: An underestimated negative cloud feedback from cloud lifetime changes, Nat. Clim. Change, 11, 508–513, https://doi.org/10.1038/s41558-021-01038-1, 2021. a, b, c, d, e, f, g
Naud, C. M., Booth, J. F., and Del Genio, A. D.: Evaluation of ERA-Interim and MERRA cloudiness in the southern ocean, J. Climate, 27, 2109–2124, https://doi.org/10.1175/JCLI-D-13-00432.1, 2014. a
Naud, C. M., Booth, J. F., Lamer, K., Marchand, R., Protat, A., and McFarquhar, G. M.: On the Relationship Between the Marine Cold Air Outbreak M Parameter and Low‐Level Cloud Heights in the Midlatitudes, J. Geophys. Res.-Atmos., 125, e2020JD032465, https://doi.org/10.1029/2020JD032465, 2020. a
O'Connor, E. J., Illingworth, A. J., and Hogan, R. J.: A Technique for Autocalibration of Cloud Lidar, J. Atmos. Ocean. Technol., 21, 777–786, https://doi.org/10.1175/1520-0426(2004)021<0777:ATFAOC>2.0.CO;2, 2004. a, b
Protat, A., Schulz, E., Rikus, L., Sun, Z., Xiao, Y., and Keywood, M.: Shipborne observations of the radiative effect of Southern Ocean clouds, J. Geophys. Res.-Atmos., 122, 318–328, https://doi.org/10.1002/2016JD026061, 2017. a
Protat, A., Klepp, C., Louf, V., Petersen, W. A., Alexander, S. P., Barros, A., Leinonen, J., and Mace, G. G.: The Latitudinal Variability of Oceanic Rainfall Properties and Its Implication for Satellite Retrievals: 1. Drop Size Distribution Properties, J. Geophys. Res.-Atmos., 124, 13291–13311, https://doi.org/10.1029/2019JD031010, 2019. a
Radenz, M., Bühl, J., Seifert, P., Baars, H., Engelmann, R., Barja González, B., Mamouri, R.-E., Zamorano, F., and Ansmann, A.: Hemispheric contrasts in ice formation in stratiform mixed-phase clouds: disentangling the role of aerosol and dynamics with ground-based remote sensing, Atmos. Chem. Phys., 21, 17969–17994, https://doi.org/10.5194/acp-21-17969-2021, 2021. a
Ramelli, F., Henneberger, J., David, R. O., Bühl, J., Radenz, M., Seifert, P., Wieder, J., Lauber, A., Pasquier, J. T., Engelmann, R., Mignani, C., Hervo, M., and Lohmann, U.: Microphysical investigation of the seeder and feeder region of an Alpine mixed-phase cloud, Atmos. Chem. Phys., 21, 6681–6706, https://doi.org/10.5194/acp-21-6681-2021, 2021. a
Rodts, S. M., Duynkerke, P. G., and Jonker, H. J.: Size distributions and dynamical properties of shallow cumulus clouds from aircraft observations and satellite data, J. Atmos. Sci., 60, 1895–1912, https://doi.org/10.1175/1520-0469(2003)060<1895:SDADPO>2.0.CO;2, 2003. a
Schuddeboom, A. J. and McDonald, A. J.: The Southern Ocean Radiative Bias, Cloud Compensating Errors, and Equilibrium Climate Sensitivity in CMIP6 Models, J. Geophys. Res.-Atmos., 126, e2021JD035310, https://doi.org/10.1029/2021JD035310, 2021. a, b
Senior, C. and Mitchell, J.: Carbon Dioxide and Climate. The Impact of Cloud Parameterization, J. Climate, 6, 393–418, https://doi.org/10.1175/1520-0442(1993)006<0393:CDACTI>2.0.CO;2, 1993. a, b
Silber, I., Verlinde, J., Eloranta, E. W., Flynn, C. J., and Flynn, D. M.: Polar Liquid Cloud Base Detection Algorithms for High Spectral Resolution or Micropulse Lidar Data, J. Geophys. Res.-Atmos., 123, 4310–4322, https://doi.org/10.1029/2017JD027840, 2018. a, b, c, d
Silber, I., Fridlind, A. M., Verlinde, J., Russell, L. M., and Ackerman, A. S.: Nonturbulent Liquid-Bearing Polar Clouds: Observed Frequency of Occurrence and Simulated Sensitivity to Gravity Waves, Geophys. Res. Lett., 47, e2020GL087099, https://doi.org/10.1029/2020GL087099, 2020a. a, b
Silber, I., Verlinde, J., Wen, G., and Eloranta, E. W.: Can Embedded Liquid Cloud Layer Volumes Be Classified in Polar Clouds Using a Single-Frequency Zenith-Pointing Radar?, IEEE Geosci. Remote Sens. Lett., 17, 222–226, https://doi.org/10.1109/LGRS.2019.2918727, 2020b. a
Silber, I., Fridlind, A. M., Verlinde, J., Ackerman, A. S., Cesana, G. V., and Knopf, D. A.: The prevalence of precipitation from polar supercooled clouds, Atmos. Chem. Phys., 21, 3949–3971, https://doi.org/10.5194/acp-21-3949-2021, 2021a. a, b, c, d, e, f, g, h, i, j, k, l, m, n, o, p, q, r, s
Silber, I., Jackson, R. C., Fridlind, A. M., Ackerman, A. S., Collis, S., Verlinde, J., and Ding, J.: Silber_et_al_EMC2_gmd-2021-194_code_and_data, Zenodo [code], https://doi.org/10.5281/zenodo.5115252, 2021b a
Silber, I., Jackson, R. C., Fridlind, A. M., Ackerman, A. S., Collis, S., Verlinde, J., and Ding, J.: The Earth Model Column Collaboratory (EMC2) v1.1: an open-source ground-based lidar and radar instrument simulator and subcolumn generator for large-scale models, Geosci. Model Dev., 15, 901–927, https://doi.org/10.5194/gmd-15-901-2022, 2022. a, b, c
Stanford, M. W.: Stanford_et_al_ACP_MICRE_2023-170_code, Zenodo [code], https://doi.org/10.5281/zenodo.8231170, 2023. a
Stephens, G. L., Vane, D. G., Boain, R. J., Mace, G. G., Sassen, K., Wang, Z., Illingworth, A. J., O'Connor, E. J., Rossow, W. B., Durden, S. L., Miller, S. D., Austin, R. T., Benedetti, A., and Mitrescu, C.: The cloudsat mission and the A-Train: A new dimension of space-based observations of clouds and precipitation, B. Am. Meteorol. Soc., 83, 1771–1790, https://doi.org/10.1175/bams-83-12-1771, 2002. a
Stephens, G. L., L'Ecuyer, T., Forbes, R., Gettlemen, A., Golaz, J. C., Bodas-Salcedo, A., Suzuki, K., Gabriel, P., and Haynes, J.: Dreary state of precipitation in global models, J. Geophys. Res.-Atmos., 115, 24211, https://doi.org/10.1029/2010JD014532, 2010. a
Suzuki, K., Stephens, G., Bodas-Salcedo, A., Wang, M., Golaz, J. C., Yokohata, T., and Koshiro, T.: Evaluation of the Warm Rain Formation Process in Global Models with Satellite Observations, J. Atmos. Sci., 72, 3996–4014, https://doi.org/10.1175/JAS-D-14-0265.1, 2015. a, b
Swales, D. J., Pincus, R., and Bodas-Salcedo, A.: The Cloud Feedback Model Intercomparison Project Observational Simulator Package: Version 2, Geosci. Model Dev., 11, 77–81, https://doi.org/10.5194/gmd-11-77-2018, 2018. a
Tan, I., Storelvmo, T., and Zelinka, M. D.: Observational constraints on mixed-phase clouds imply higher climate sensitivity, Science, 352, 224–227, https://doi.org/10.1126/science.aad5300, 2016. a
Tansey, E., Marchand, R., Protat, A., Alexander, S. P., and Ding, S.: Southern Ocean Precipitation Characteristics Observed From CloudSat and Ground Instrumentation During the Macquarie Island Cloud & Radiation Experiment (MICRE): April 2016 to March 2017, J. Geophys. Res-.Atmos., 127, e2021JD035370, https://doi.org/10.1029/2021JD035370, 2022. a, b, c, d, e, f, g, h, i
Tansey, E., Marchand, R., Alexander, S. P., Klekociuk, A. R., and Protat, A. R: Southern Ocean low cloud and precipitation phase observed during the Macquarie Island Cloud and Radiation Experiment (MICRE), ESS Open Archive, https://doi.org/10.22541/essoar.168394768.89694625/v1, 2023. a
Taylor, K. E., Stouffer, R. J., and Meehl, G. A.: An Overview of CMIP5 and the Experiment Design, B. Am. Meteorol. Soc., 93, 485–498, https://doi.org/10.1175/BAMS-D-11-00094.1, 2012. a
Tian, L. and Curry, J. A.: Cloud overlap statistics, J. Geophy. Res.-Atmos., 94, 9925–9935, https://doi.org/10.1029/JD094ID07P09925, 1989. a
Tjernström, M., Shupe, M. D., Brooks, I. M., Achtert, P., Prytherch, J., and Sedlar, J.: Arctic Summer Airmass Transformation, Surface Inversions, and the Surface Energy Budget, J. Climate, 32, 769–789, https://doi.org/10.1175/JCLI-D-18-0216.1, 2019. a
Trenberth, K. E. and Fasullo, J. T.: Simulation of present-day and twenty-first-century energy budgets of the southern oceans, J. Climate, 23, 440–454, https://doi.org/10.1175/2009JCLI3152.1, 2010. a
Tsushima, Y., Emori, A. S., Ogura, A. T., Kimoto, M., Webb, A. M. J., Williams, A. K. D., Ringer, M. A., Soden, A. B. J., Li, A. B., and Andronova, A. N.: Importance of the mixed-phase cloud distribution in the control climate for assessing the response of clouds to carbon dioxide increase: a multi-model study, Clim. Dynam., 27, 113–126, https://doi.org/10.1007/s00382-006-0127-7, 2006. a, b, c
vanZanten, M. C., Stevens, B., Vali, G., and Lenschow, D. H.: Observations of Drizzle in Nocturnal Marine Stratocumulus, J. Atmos. Sci., 62, 88–106, https://doi.org/10.1175/JAS-3355.1, 2005. a
Vaughan, M. A., Powell, K. A., Kuehn, R. E., Young, S. A., Winker, D. M., Hostetler, C. A., Hunt, W. H., Liu, Z., Mcgill, M. J., and Getzewich, B. J.: Fully Automated Detection of Cloud and Aerosol Layers in the CALIPSO Lidar Measurements, J. Atmos. Ocean. Technol., 26, 2034–2050, https://doi.org/10.1175/2009JTECHA1228.1, 2009. a
Verlinde, J., Zak, B. D., Shupe, M. D., Ivey, M. D., and Stamnes, K.: The ARM North Slope of Alaska (NSA) Sites, Meteorol. Monogr., 57, 1–8, https://doi.org/10.1175/amsmonographs-d-15-0023.1, 2016. a
Wallace, J. M. and Hobbs, P. V.: Atmospheric Science: An Introductory Survey, International Geophysics Series, Elsevier Academic Press, 483 pp., ISBN 978-0-12-732951-2, 2006. a
Wang, Z., Siems, S. T., Belusic, D., Manton, M. J., and Huang, Y.: A Climatology of the Precipitation over the Southern Ocean as Observed at Macquarie Island, J. Appl. Meteorol. Climatol., 54, 2321–2337, https://doi.org/10.1175/JAMC-D-14-0211.1, 2015. a, b, c
Warren, G., Hahn, H., London, J., Chervin, M., and Jenne, L.: Global Distribution of Total Cloud Cover and Cloud Type Amounts Over the Ocean, (No. NCAR/TN-317+STR), University Corporation for Atmospheric Research, https://doi.org/10.5065/D6QC01D1, 1988. a
Widener, K., Bharadwaj, N., and Johnson, K.: Ka-Band ARM Zenith Radar (KAZR) Instrument Handbook, ARM-TR-106, Tech. rep., DOE Office of Science, Office of Biological and Environmental Research, United States, 25 pp., https://doi.org/10.2172/1035855, 2012. a
Wood, N. B., L’Ecuyer, T. S., Heymsfield, A. J., Stephen, G. L., Hudak, D. R., and Rodriguez, P.: Estimating snow microphysical properties using collocated multisensor observations, J. Geophys. Res.-Atmos., 119, 8941–8961, https://doi.org/10.1002/2013JD021303, 2014. a
Wood, R. and Bretherton, C. S.: On the Relationship between Stratiform Low Cloud Cover and Lower-Tropospheric Stability, J. Climate, 19, 6425–6432, https://doi.org/10.1175/JCLI3988.1, 2006. a
Yang, F., Luke, E. P., Kollias, P., Kostinski, A. B., and Vogelmann, A. M.: Scaling of Drizzle Virga Depth With Cloud Thickness for Marine Stratocumulus Clouds, Geophys. Res. Lett., 45, 3746–3753, https://doi.org/10.1029/2018GL077145, 2018. a
Zelinka, M. D., Myers, T. A., McCoy, D. T., Po-Chedley, S., Caldwell, P. M., Ceppi, P., Klein, S. A., and Taylor, K. E.: Causes of Higher Climate Sensitivity in CMIP6 Models, Geophys. Res. Lett., 47, e2019GL085782, https://doi.org/10.1029/2019GL085782, 2020. a
Zhang, Q., Liu, B., Li, S., and Zhou, T.: Understanding Models' Global Sea Surface Temperature Bias in Mean State: From CMIP5 to CMIP6, Geophys. Res. Lett., 50, e2022GL100888, https://doi.org/10.1029/2022GL100888, 2023. a
Zhang, Y. and Klein, S. A.: Factors controlling the vertical extent of fair-weather shallow cumulus clouds over land: Investigation of diurnal-cycle observations collected at the ARM southern great plains site, J. Atmos. Sci., 70, 1297–1315, https://doi.org/10.1175/JAS-D-12-0131.1, 2013. a
- Abstract
- Introduction
- Data and methods
- Results
- ESM evaluation
- Discussion
- Conclusions
- Appendix A: List of abbreviations and notation
- Appendix B: Minimum detectable BASTA Ze
- Appendix C: Addressing potential biases in LCB height detections
- Appendix D: Sounding RH and ceilometer CBH comparison
- Appendix E: Supercooled partitioning dependence on Dmin
- Appendix F: Fog case studies
- Appendix G: GISS-ModelE3 sensitivities of cloud and precipitation occurrence frequency to thresholding behavior
- Code and data availability
- Author contributions
- Competing interests
- Disclaimer
- Acknowledgements
- Financial support
- Review statement
- References
- Abstract
- Introduction
- Data and methods
- Results
- ESM evaluation
- Discussion
- Conclusions
- Appendix A: List of abbreviations and notation
- Appendix B: Minimum detectable BASTA Ze
- Appendix C: Addressing potential biases in LCB height detections
- Appendix D: Sounding RH and ceilometer CBH comparison
- Appendix E: Supercooled partitioning dependence on Dmin
- Appendix F: Fog case studies
- Appendix G: GISS-ModelE3 sensitivities of cloud and precipitation occurrence frequency to thresholding behavior
- Code and data availability
- Author contributions
- Competing interests
- Disclaimer
- Acknowledgements
- Financial support
- Review statement
- References