the Creative Commons Attribution 4.0 License.
the Creative Commons Attribution 4.0 License.
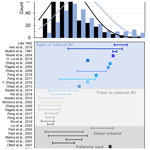
The density of ambient black carbon retrieved by a new method: implications for cloud condensation nuclei prediction
Jingye Ren
Lu Chen
Jieyao Liu
The effective density of black carbon (BC) is a crucial factor that is relevant to its aging degree and adds uncertainty in evaluating its climate effect. Here, we have developed a new method to retrieve the effective density of internally mixed BC in the atmosphere, combining field observations conducted from 15 November to 14 December 2016 in urban Beijing with the Köhler theory. The uncertainty in the retrieval method was evaluated to be within ±30 %, which was primarily caused by assumptions on both the hygroscopic parameter of organics and the proportional distribution of primary organic aerosols in different hygroscopic modes. Using the method, we find that the ambient internally mixed BC, accounting for 80 % ± 20 % of total BC aerosol particles, was retrieved with a campaign mean density of 1.1 ± 0.6 g cm−3 during the observed periods. The retrieved result was comparable with that reported in the literature. By applying a lower (0.14 g cm−3) and upper (2.1 g cm−3) limit of the retrieved BC density in the cloud condensation nuclei (CCN) number concentration (NCCN) estimation, we derived that the neglect of such variations in the BC density would lead to an uncertainty of −28 %–11 % in predicting NCCN at supersaturations of 0.23 % and 0.40 %. We also find that the NCCN was more sensitive to the variations in BC density when it was <1.0 g cm−3. This illustrates the necessity of accounting for the effect of BC density on CCN activity closer to source regions where the BC particles are mostly freshly emitted. The CCN closure was achieved when introducing the retrieved real-time BC density and mixing state. This study provides a unique way of utilizing field measurements to infer ambient BC density and highlights the importance of applying variable BC density values in models when predicting CCN and assessing its relevant climate effect.
- Article
(4177 KB) - Full-text XML
-
Supplement
(1118 KB) - BibTeX
- EndNote
Black carbon (BC) aerosols, as the major absorber of solar radiation, play a vital role in the energy budget and climate of the Earth–atmosphere system by affecting the radiative forcing and cloud properties (Flanner et al., 2007; Ramanathan and Carmichael, 2008). The light-absorbing capability induced by BC is related to its density and morphology (Zhang et al., 2008; Rissler et al., 2014), which can be modified after mixing with other atmospheric aerosol particles (Khalizov et al., 2009; Xue et al., 2009). Changes in its physicochemical properties or the aging process would also regulate its ability to serve as cloud condensation nuclei (CCN) and further affect the CCN number concentrations (F. Zhang et al., 2016, 2017; Ren et al., 2023) and the radiative balance by affecting the cloud processes (Yuan et al., 2008; Wang et al., 2011). Owing to the complex evolution of the mixing state, density, and morphology of BC, the contribution of BC particles to CCN budgets is still not well understood.
BC particles, with diesel vehicles, industrial coal combustion, and residential coal combustion as major sources, are ubiquitous in urban environments (Bond et al., 2013; Dameto et al., 2017; Li et al., 2017; D. Liu et al., 2019). The mixing state of BC describes the distribution of the bare BC and coating materials among the aerosol population. Typically, freshly generated BC exists in the form of chain aggregates and is initially uncoated, which is known as externally mixed BC (Ex-BC). When the BC particles were emitted, they generally mixed with other materials by condensation, coagulation, and other processes (Riemer et al., 2004; Zhang et al., 2008; Liu et al., 2013; F. Zhang et al., 2020), forming the internally mixed BC (In-BC) particles consisting of a BC core and other chemical components (Cheng et al., 2006; Y. Zhang et al., 2016). The BC structure would be more compact, with regular shapes (Pagels et al., 2009; Zhang et al., 2008; Wang et al., 2017), and the effective density of internally mixed BC is changed accordingly with the reconstruction (H. Liu et al., 2019). The density and morphology of BC particles are closely related to the sources, mobility size, coating thickness, coating material, and its chemical composition (Pagels et al., 2009; Zhang et al., 2022). A wide range of BC density has been reported in previous studies (Lide, 1992; McMurry et al., 2002; Park et al., 2004; Kiselev et al., 2010). Recent field measurement has indicated that the average BC density is ∼1.2 g cm−3 in the ambient atmosphere (Y. Zhang et al., 2016). Field measurements have also indicated that a considerable fraction of externally mixed/uncoated BC exists (Clarke et al., 2004; Cheng et al., 2012), although a higher proportion of internally mixed/aged BC particles were observed in the ambient atmosphere (Schwarz et al., 2008; Massoli et al., 2015; Chen et al., 2020). In climate models, the BC was generally assumed to be completely internally mixed and thus treated to have a void-free spherical structure and a density value of 1.8 g cm−3 (Bond et al., 2013). This may lead to bias in estimating the climate effect driven by BC.
A previous study, based on a case study, has shown that when the aging degree of ambient particles was low, then the BC density (∼1.8 g cm−3) under the spherical assumption would lead to the overestimation of particle hygroscopicity by 40 %–50 %, and the overestimation could be explained by almost 100 %, using the effective density of fresh BC (∼0.45 g cm−3; Fan et al., 2020). This indicates the importance of using reasonable BC density values in the calculation of particle hygroscopicity. In addition, when estimating the CCN number concentration, a significant bias of −35 %–+20 % was found due to the assumption of the particle mixing state (Ren et al., 2018). However, these studies have not yet accounted for such an impact of the BC density and mixing state on the CCN prediction due to a lack of real-time measurement data. Moreover, although the BC accounts for very small mass fractions (5 %–10 %) in total fine aerosols, according to our previous field observed results, the BC-containing particles could contribute 60 %–78 % toward the total number concentration in urban Beijing (Chen et al., 2020). This is comparable to the other results using the single-particle soot photometer (SP2) instrument, which measured the number fractions of the coated BC-containing aerosols and showed that they could be as high as about 50 %–80 % at the field sites in northern China (H. Liu et al., 2019; Zhao et al., 2022). Therefore, the effect of BC density on the uncertainty in CCN prediction should be concerned carefully.
The mixing state and the density of BC particles are usually directly measured by several techniques, such as an integrated system of a volatility tandem differential mobility analyzer and a SP2 instrument (VTDMA-SP2; Y. Zhang et al., 2016) or a differential mobility analyzer with a SP2 (DMA-SP2; Olfert et al., 2007; Rissler et al., 2014; Wu et al., 2019) and a differential mobility analyzer coupled with a centrifugal particle mass analyzer and a SP2 (DMA–CPMA–SP2) system (H. Liu et al., 2019; Yu et al., 2020). However, such techniques or measurements are not available in many previously conducted field campaigns. In this study, we develop a novel method for retrieving the mixing state and effective density of ambient BC particles by combining the field-measured hygroscopic growth factor and aerosol chemical composition with the Köhler theory (Petters and Kreidenweis, 2007). The uncertainty in the new retrieval method was evaluated. The retrieved results were also compared and validated with existing observations. In addition, the effect of the BC density and mixing state on the prediction of the CCN number concentrations was further evaluated through a sensitivity and closure test by accounting for the retrieved real-time variations in the BC density and mixing state.
2.1 Field measurements
Measurements in this study were conducted from 15 November to 14 December 2016 at a typical urban site of Beijing (39.97∘ N, 116.37∘ E; 49 m above sea level). The site is located at the Institute of Atmospheric Physics, Chinese Academy of Sciences, which is mainly influenced by the surrounding cooking, road traffic, and residential coal burning emissions during the home heating periods (Sun et al., 2016). Detailed information about the sampling site has been presented in previous studies (Sun et al., 2016; Zhang et al., 2019). The number concentration of the condensation nuclei (CN) at each size was measured by a scanning mobility particle sizer, which was equipped with a differential mobility analyzer (DMA; model 3081, TSI) and a condensation particle counter (CPC; model 3772, TSI). Subsequently, the monodispersed particles were introduced into a Droplet Measurement Technologies CCN counter (CCNc; DMT; Lance et al., 2006) to measure the CCN number concentration. A hygroscopic tandem differential mobility analyzer (HTDMA) system was used to measure the hygroscopic growth factor (Gf; Tan et al., 2013). Here, four diameters of 40, 80, 110, 150, and 200 nm were selected in the campaign. Gf is defined as the ratio of the mobility diameter at the given relative humidity (RH) to the dry diameter (Petters and Kreidenweis, 2007). The nonrefractory submicron aerosol chemical composition was measured by an Aerodyne high-resolution time-of-flight aerosol mass spectrometer (HR-ToF-AMS; Xu et al., 2019), including sulfate, nitrate, ammonium, chloride, and organics. Two factors, including a non-hygroscopic primary organic aerosol (POA) and hygroscopic secondary organic aerosol (SOA), were classified by positive matrix factorization (PMF) with the PMF algorithm (v4.2) method (Paatero and Tapper, 1994) and followed the procedures reported in Ulbrich et al. (2009). The refractory black carbon mass loading was measured by an Aethalometer (model AE33, Magee Scientific). Both the nonrefractory materials and BC mass concentration were measured with diameters <1.0 µm. A detailed description of the instrument operation and data process have been described in detail elsewhere (Ren et al., 2018; Xu et al., 2019; Zhang et al., 2019; Fan et al., 2020).
2.2 Retrieving the mixing state and density of BC
2.2.1 Retrieving the mixing state of BC
The Gf probability distribution function (Gf-PDF) for a specified diameter can be retrieved, first, based on the TDMAinv algorithm (Gysel et al., 2009). The κ-PDF can be further calculated based on the Gf-PDF (Fan et al., 2020). Size-resolved κ is derived using κ-Köhler theory, based on hygroscopic growth factor (Gf), as follows (Petters and Kreidenweis, 2007):
where Gf is hygroscopic growth factor, RH is the relative humidity in the HTDMA (90 %), Dd is the dry diameter, σs/a is assumed to be the surface tension of pure water, R is the universal gas constant, T is the temperature, and Mw and ρw are the molecular mass and the density of water, respectively.
The κ-PDF patterns of particles in different sizes always present two modes, namely the nearly hydrophobic (NH) mode, with κgf≤0.1, and more hygroscopic (MH) mode, with κgf>0.1 (Fig. S1 in the Supplement). First, based on the κ-PDF patterns, the number fraction (NF) of the total nearly hydrophobic group with the boundary of [0,0.1] was calculated according to the following equation:
Here, the κ-PDF, represented by c(κ,Dp), was normalized as , where κ can be replaced by κgf, Dp is the selected electrical mobility diameter in the campaign.
The nearly hydrophobic mode consists of both externally mixed POA (Ex-POA or bare POA) and externally mixed BC (Ex-BC). Since the number fraction of the nearly hydrophobic POA would change with the emission and aging processes, in this study, we have applied different values for the number fractions of hydrophobic POA (NH-POA) under clean (91 %), moderately polluted (70 %), and heavily polluted conditions (31 %) by referring to the literature (L. Liu et al., 2021), as shown in Fig. S2. The number concentration of Ex-BC was then calculated using the total number fraction of the NH mode minus the number of NH-POA.
where NPOA-containing and NFPOA-containing are the number concentration and fraction of POA-containing particles, Ntotal is the total number concentration, Nbare-POA and NFbare-POA are the number concentration and fraction of bare POA particles, and NNH is the number of the nearly hydrophobic group.
The number size distribution of the externally mixed BC (nEx-BC(log Dp)) can be calculated based on the particle number size distribution (PNSD) and the number fraction of the hydrophobic mode of BC (NFEx-BC) as follows:
where n(log Dp) is the function of the aerosol number size distribution, and Dp is the mobility diameter.
By assuming that the particles were spherical (Rader and McMurry, 1986), the mass size distribution of Ex-BC (MEx-BC) was obtained as follows
where Dp is the mobility diameter, ρ is the effective density of Ex-BC, and nEx-BC(log Dp) is the function of the number size distribution of Ex-BC, respectively. By reviewing and summarizing the existing results, we show that the typical values of density for the freshly emitted or externally mixed BC observed in the winter of urban Beijing or the North China Plain span over 0.14–0.50 g cm−3, with a mean of ∼0.40 ± 0.10 g cm−3 (Fig. S3), in the size range of 100 to 300 nm, which is where the mass concentration of externally mixed BC mostly concentrated (Geller et al., 2006; Wu et al., 2019; Liu et al., 2020; Zhao et al., 2022). Therefore, an average ρEx-BC of 0.4 g cm−3 was used to calculate the mass concentration of externally mixed BC in our study. Uncertainty analyses due to the variations in ρEx-BC are given in Sect. 2.3.
The mass size distribution of Ex-BC was fitted using the lognormal distribution, as shown in Fig. S4 (Wu et al., 2017; D. Liu et al., 2019; Zhao et al., 2022). Thus, the bulk mass concentration of Ex-BC can be calculated from the integration of the mass size distribution as follows:
where Dstart and Dend are the lower and upper size limit, and MEx-BC(log Dp) is the function of the Ex-BC mass size distribution. We then obtained the bulk mass concentration of internally mixed BC (mIn-BC) by subtracting mEx-BC from the bulk BC mass concentration measured by the AE33 in Eq. (7). It should be noted that the mass concentration of BC obtained from the AE33 based on aerosol light absorption may lead to some uncertainties, as has been further addressed in Sect. 2.3.
2.2.2 Retrieving the density of BC
For the retrieval of the density of BC, the principal idea is to use the measured κgf to calculate the density of BC based on the Zdanovskii–Stokes–Robinson (ZSR) mixing rule (Stokes and Robinson, 1966; Zdanovskii, 1948) with the chemical composition measured by AMS (Petters and Kreidenweis, 2007). In the retrieval, several aspects are concerned. First, since the ZSR rule assumes that the aerosol particles are internally mixed, the κgf value of the more MH mode (κgf-MH) is thus applied for retrieving the density of the internally mixed BC. Second, since the size distribution of BC number concentration usually has peaks between 100 and 200 nm (D. Liu et al., 2019; Yu et al., 2020; Zhao et al., 2022), the κgf-MH value of the particles in accumulation mode was averaged and applied for the retrieval. Previous studies showed an independence of κgf-MH for the particle size when the Dp>100 nm during the campaign period (Fan et al., 2020). Therefore, the average of κgf-MH in the accumulation mode is reasonable for the determination of the In-BC density. Third, since only one hydrophobic and/or one hygroscopic mode was observed by the HTDMA in most cases during the campaign (Figs. S1 and S5), the chemical components of the more hygroscopic (MH) mode at a given diameter should contain both the hygroscopic non-BC and the coating on BC-containing particles, which would be measured together by the HR-ToF-AMS instrument. Therefore, by subtracting the externally mixed POA in the non-hygroscopic mode (see Sect. 2.3), the concentration and mass fraction of each component measured by HR-ToF-AMS can represent the overall chemical composition of MH modes, and thus, this was applied in the ZSR mixing rule for the retrieval of the density of internally mixed BC in this study. In addition, because of the inversion including measurements from HTDMA and HR-ToF-AMS, a total mass closure of the measured aerosol particles was conducted between the two techniques by comparing the mass concentration of PM1, and the results are in good agreement (Fig. S6). The density of the internally mixed BC (In-BC), ρIn-BC is then derived from the following equations:
where κgf-MH is the hygroscopic parameter of the more hygroscopic (MH) mode, κchem is the hygroscopic parameter of the aerosol particles in the mixed composition and can be calculated based on chemical volume fractions using a simple rule (Stokes and Robinson, 1966; Petters and Kreidenweis, 2007), κi is the hygroscopic parameter of each pure composition, and εi is the volume faction of the individual components in the internally mixed particle. vinorg, vSOA, and vIn-POA are the volumes of the inorganic, SOA, and internally mixed POA species and can be calculated as follows: , , and . vtotal is the total volume of all the species and can be written as . In Eq. (8), κBC and κPOA are assumed to be 0. So, the total volume vtotal can be further written as . The volume of internally mixed vIn-BC can be calculated as follows:
Then, the ρIn-BC can be calculated based on its mass concentration and volume as follows:
where mIn-BC is the mass concentration of internally mixed BC, and minorg and mSOA are the mass concentrations of the inorganic species and SOA, which are measured by the AMS. mIn-POA is the mass concentration of the internally mixed POA and can be calculated through subtracting the mass fraction of the NH-POA from the total mass concentrations of the POA. ρinorg, ρSOA and ρPOA are the density of the inorganic species, i.e., SOA and POA, since the AMS measures the concentrations of the organic and inorganic ions, including , , , and Cl−. Here, inorganic species were derived by applying a simplified ion pairing scheme (Gysel et al., 2007) to convert the mass concentrations of the ions to the inorganic salts as follows:
where n represents the number of moles, and the mass concentrations were obtained by the number of moles times the molar mass of each inorganic salt. Because the value of the was zero in this campaign, three inorganic salts including NH4HSO4, (NH4)2SO4, and NH4NO3 were applied in our study. The densities for inorganic salts were taken from previous studies (Gysel et al., 2007; Wu et al., 2016). Here the densities for the three inorganic salts are 1.78, 1.77, and 1.72 g cm−3, respectively. By summarizing the previous studies (Gysel et al., 2007; Dinar et al., 2006), 1.4 g cm−3 was selected as the density of SOA (ρSOA). The density of POA (ρPOA) is assumed to be 1.0 g cm−3 for urban environments, which is similar to the that of the lubricating oil (Wu et al., 2016). Since the cooking organic aerosols represent a high contribution to POA in urban environments, we choose the mean density of the rapeseed oil and oleic acid (∼0.85 g cm−3; Reyes-Villegas et al., 2018) to evaluate the result, as shown in Sect. 2.3. The values of κ for inorganic components are 0.56 for NH4HSO4, 0.48 for (NH4)2SO4, and 0.58 for NH4NO3, along with the best-fit values for the three inorganic salts (Petters and Kreidenweis, 2007; Gunthe et al., 2009). The κSOA is assumed to be 0.15, according to the field studies in urban areas (Chang et al., 2010; Kawana et al., 2016).
Note that the method fails to retrieve the BC density when organic aerosol (OA) accounts for a large fraction (>60 %). This is because a higher fraction of OA usually corresponds to lower total volume of all the species (Fig. S7), yielding negative values for vIn-BC introduced in Eq. (9). As a result, 61 % of the data observed during the campaign were valid for calculating the BC density.
Similarly, the bulk density of BC (ρbulk-BC) is calculated with the same method as for the calculation of the ρIn-BC. When calculating the ρbulk-BC, the bulk κgf value measured by HTDMA is applied, assuming that all the aerosol particles are internally mixed.
2.3 Uncertainties and limitations
For the retrieval, the assumptions on the values of κSOA, ρPOA, ρSOA, and ρEx-BC, as well as the fraction of primary organic aerosols in non-hygroscopic mode, would add uncertainty to the inferred values of ambient internally mixed BC density. For example, the freshly emitted POA particles might be consistently coated with secondary particles during the aging process, resulting in changes in the NFNH-POA. However, a real-time variation in the NFNH-POA is not yet available due to the lack of such measurement data.
Applying only rough fractions of hydrophobic POA for three different atmospheric conditions could still cause uncertainties. Also, the densities of POA and SOA may differ due to their precursors, emission sources, and the formation mechanisms in ambient atmosphere (Alfarra et al., 2006; Reyes-Villegas et al., 2018). The density of Ex-BC is generally characterized by the morphology and size (Wu et al., 2019). In addition, the value of κSOA spans largely due to the variations in the emissions of gas precursors and formation processes under different atmospheric conditions (Zhang et al., 2015; J. Liu et al., 2021). Therefore, we examined the sensitivities of In-BC density to the variations in these factors, as exhibited in Figs. 1 and 2.
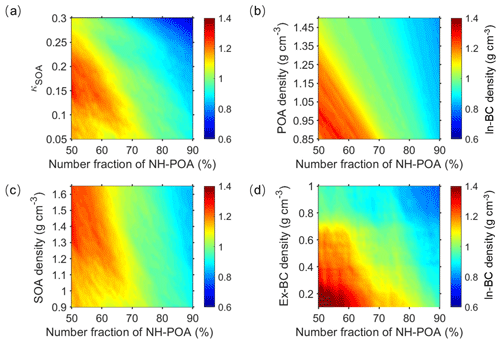
Figure 1Sensitivities of In-BC density to the variations in the number fraction of the nearly hydrophobic (NH) POA and hygroscopic parameter of OA (kSOA) (a), POA density (b), SOA density (c), and the externally mixed BC density (d).
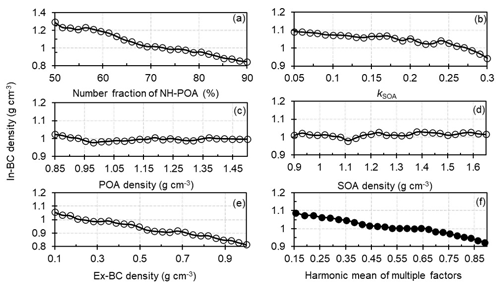
Figure 2Sensitivity of the In-BC density to variations in the number fraction of nearly hydrophobic (NH) POA (a), the hygroscopic parameter of SOA (b), the POA density (c), the SOA density (d), the externally mixed BC density (e), and the harmonic mean of multiple factors (f).
The figures show that the In-BC density gradually decreased with the increment of the NFNH-POA, implying that the high fraction of bare POA particles corresponds to the early aging stage of aerosol particles. With the increase in κSOA, the In-BC density was generally reduced but with small fluctuations (Figs. 1a and 2b). This suggests a complex impact of the assumptions of κSOA on the retrieved BC density. In addition, the In-BC density decreased slightly as ρEx-BC increased (Fig. 2e), suggesting that applying a larger ρEx-BC would derive smaller values for In-BC density. The In-BC density was insensitive to the changes in the density of POA and SOA, showing an almost negligible effect on the retrieved results (Fig. 2c and d).
The uncertainty analysis shows that, by comparing the results based on the mean fraction of the NFNH-POA with a typical atmospheric observed range of 50 %–90 % for the NFNH-POA (L. Liu et al., 2021), the assumption on NFNH-POA can lead to relative deviations (uncertainty) of −17 %–+27 % for the retrieved BC density (Fig. 3a).
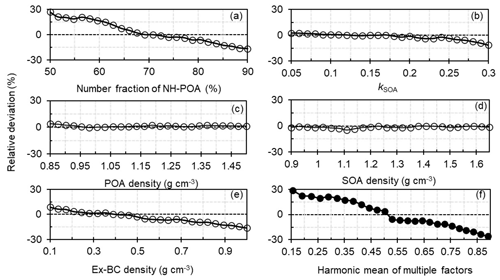
Figure 3Relative deviations of the number fraction of nearly hydrophobic (NH) POA to the In-BC density (a), the hygroscopic parameter of OA to the In-BC density (b), the POA density to the In-BC density (c), the SOA density to the In-BC density (d), the externally mixed BC density to the In-BC density (e), and the combined deviations based on multiple factors mentioned above (f).
In addition, unlike inorganics (e.g., NH4HSO4, (NH4)2SO4, and NH4NO3), for which the hygroscopicity is already well understood (Petters and Kreidenweis, 2007), the hygroscopicity of organic species varies largely due to the complexity in organic aerosol constituents. Therefore, the assumption of the values of κSOA will add to the uncertainty in the calculation of BC density. Previous studies have suggested that the organics have a wide range of κ values ranging from 0.05 to 0.3 (Jimenez et al., 2009; Mei et al., 2013). Thus, a sensitivity test has also been done to examine the effect due to changes in κSOA on calculating the density of BC (Fig. 1a). The result shows that the assumption of κSOA values can cause an average relative deviation of −10 %–+3 % when calculating the density of In-BC (Fig. 3b).
However, the sensitivity test shows that the impact of both the ρPOA and ρSOA variations on the BC density estimation was very small or even negligible (Fig. 1b and c). By varying the ρPOA from 0.85 to 1.5 g cm−3 and the ρSOA from 0.9 to 1.65 g cm−3, according to the literature (Noureddini et al., 1992; Alfarra et al., 2006; Reyes-Villegas et al., 2018; Kostenidou et al., 2007), the retrieval uncertainties in the BC density were both within ±5 % (Fig. 3c and d). For ρEx-BC, it exhibited that the evolution of the ρEx-BC could lead to an average deviation of −16 %–+9 % in calculating the In-BC density (Fig. 3e) when increasing the values of ρEx-BC from 0.1 to 1.0 g cm−3, which represents a typical range in the ambient atmosphere (Wu et al., 2019; Liu et al., 2020). A combined uncertainty (δ) caused by the multiple factors (δi), which was calculated by Eq. (12), was −26 %–+29 %, as shown in Fig. 3f.
In addition, it should be noted that the mass concentration of BC obtained from AE33, based on aerosol light absorption, may lead to some uncertainties. However, the comparison of the simultaneously measured data by SP2 with those of AE33 during the campaign shows that the temporal variations in BC mass concentrations measured by the two techniques were consistent (Fig. S8). Note that the BC mass measured by SP2 is occasionally low, probably because of the low detection efficiency for small sizes (McMeeking et al., 2010; Schwarz et al., 2006). In addition, the SP2 is unable to quantify the BC mass beyond a certain limit because of the saturation of electronic devices recording signals (Pileci et al., 2021). We show that, compared the results that were retrieved when applying the BC mass measured by SP2, the BC density retrieved based on AE33 can be 18 % higher. Given the measurement bias from SP2, this overestimation indicates an upper limit of the uncertainty.
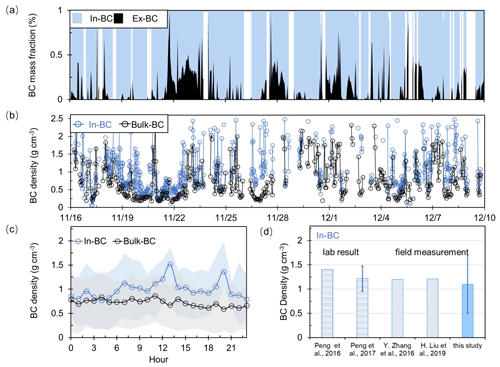
Figure 4(a) Time series of the mass fraction of the retrieved internal and external mixed BC. (b) Time series of the retrieved density of the bulk and internal mixed BC (In-BC). (c) Diurnal variation in the retrieved density of bulk and In-BC. (d) Comparison of the results of the derived In-BC density in this study with that reported in the literature.
3.1 A comparison and validation of retrieved mixing state and density of BC
Figure 4a shows the retrieved time series of the mixing state of ambient BC during the campaign. Large temporal variations in the mass fraction of internally and externally mixed BC are present during the observed period at the sites. The temporal changes should be related to the atmospheric aging process or diurnal variations in emissions (D. Liu et al., 2019; Fan et al., 2020). Statistically, the average mass fractions of externally and internally mixed BC were 20 % ± 18 % and 80 % ± 20 %, respectively, showing that most of the BC particles were aged and internally mixed with other components. Previous studies at urban sites have shown the co-existence of the externally mixed BC in the ambient atmosphere (Schwarz et al., 2008; Cheng et al., 2012; Chen et al., 2020) due to continuous combustion processes (e.g., vehicle exhaust and residential sector; Wang et al., 2017; D. Liu et al., 2019). Our results are basically comparable with those previously reported, which are directly measured or indirectly retrieved. For example, Chen et al. (2020) found that the mass fraction of internally mixed BC particles was nearly ∼80 %–90 % in summer in Beijing, based on VTDMA measurements. Liu et al. (2020), using a tandem system with an aerodynamic aerosol classifier and SP2, reported that the mass fraction of internal BC-containing particles would increase with increasing size and reach ∼70 % in Beijing. Overall, the mass fraction obtained in our study was comparable with that reported in urban Beijing. Previous studies also showed the significant diversity of the BC mixing state among emission conditions and coating processes (Shiraiwa et al., 2008; Pan et al., 2017; Y. Zhang et al., 2020). Accordingly, the densities of the bulk and internally mixed BC present apparent fluctuations (as shown in Fig. 4b), which is significantly affected by the variations in BC emission sources and BC aging processes. The density of the In-BC during daytime was generally higher than that at night (Fig. 4c). The elevated BC density during daytime was likely due to the strong photochemical processes promoting the aging of BC particles, which resulted in a conversion from an uncompacted structure to the compact and regular spherical shapes of BC (Qiao et al., 2018; H. Liu et al., 2019). The increase in BC density at around 20:00 LT (local time) might indicate that the BC particles would be rapidly coated with secondary inorganic aerosol (SIA) particles and continuously aged in the polluted period due to the heterogeneous reactions of SIA in urban regions (Y. Zhang et al., 2016; Peng et al., 2016, 2017). Actually, following the haze evolution, the fraction of the nearly hydrophobic group reduced rapidly (Fig. S9). Consequently, the average density of In-BC showed an obvious increase from the clean conditions to the polluted periods (Fig. S5). A slight decrease was observed in the bulk BC density during traffic hours. This is likely associated with the continuous emissions (e.g., vehicle exhaust) that lead to uncoated or uncompacted BC particles in this period. The diurnal cycle in In-BC density was consistent with the coating thickness measured by a tandem CPMA-SP2-DMA-SP2 (Liu et al., 2020), demonstrating that the new method can derive the density of ambient BC particles reasonably. On average, the campaign average values of the bulk and internally mixed BC densities were 0.7 ± 0.5 and 1.1 ± 0.6 g cm−3, respectively, which were much less than 1.8 g cm−3, implying that the BC particles are not void-free spheres in the urban atmosphere. The results of In-BC density were comparable with those observed at other sites in North China Plain (NCP; as shown in Fig. 4d), illustrating that the BC effective density retrieved by this method was within the range of density from field measurements.
Based on both field measurements (e.g., Lide, 1992; Y. Zhang et al., 2016; Wu et al., 2019; H. Liu et al., 2019) and laboratory studies (e.g., McMurry et al., 2002; Park et al., 2003, 2004; Olfert et al., 2007; Kiselev et al., 2010; Gysel et al., 2011, 2012), the BC density from diverse combustion sources or representing different aging degrees has been obtained and ranges widely from 0.14 to 2.1 g cm−3, as summarized and shown in Fig. 5. The mean probability distribution function (PDF) of the density of bulk and In-BC retrieved by this study is also presented in Fig. 5. It shows that the retrieved density of bulk BC exhibited a dominant mode, with a peak value at 0.7 g cm−3, which was situated between the typical density range of those externally mixed and internally mixed BC measured previously. For the In-BC, the PDF had a peak value at 1.1 g cm−3 but ranged widely from ∼0.5 to 2.5 g cm−3, which indicated various morphologies and different aging degrees and compositions of ambient BC particles due to the complex impact of multiple local sources and aging processes during the observed period in urban Beijing. Overall, the retrieved values for In-BC density fall within the range of the typical internally mixed BC values reported in the literature, verifying the reliability of our inversion results.
3.2 Sensitivity of predicted NCCN to changes in BC density
A previous study showed that the use of an inaccurate density value of BC particles would result in large biases in estimating κ of ambient aerosol particles with the ZSR mixing rule (Fan et al., 2020) and would further lead to uncertainties in the prediction of NCCN and relevant climate effects. Considering the large variation range of BC density during the campaign, which is closely associated with BC morphology or the degree of BC aging, we further examine the sensitivity of critical supersaturation (Sc), critical diameter (Dcut), and predicted NCCN to variations in BC density (Fig. 6). Here, we use the critical diameter and particle number size distribution to calculate NCCN. The method to derive the critical diameter is based on the Köhler theory and ZSR rule. Three CCN closure studies were assumed to evaluate the effect of BC density and mixing state on the prediction of the CCN number concentrations. Closure studies provide a useful way to investigate the importance of aerosol properties for CCN concentration prediction. If the closure study is achieved, then it means that the bias between the predicted and measured CCN concentrations is within ±15 % (Chang et al., 2007). Detailed calculation methods are presented in the Supplement (see the Methods section) or in Ren et al. (2018). The results show that, by varying the value of the density that represents the range of BC density in the atmosphere from 0.14 to 2.1 g cm−3, the Dcut is apparently reduced at a given supersaturation (S; Fig. 6a), or similarly, the Sc is decreased rapidly for a given particle size (Fig. 6b). The results show that the changes in the Dcut and Sc were more sensitive when the BC density was below 1.0 g cm−3. The effects on the Dcut and Sc both gradually weakened with the increase in BC density. This shows that it is critical to apply a more accurate BC density for the aerosol particles with a low aging degree for predicting CCN and its climate effect. Accordingly, the ratios of predicted-to-measured NCCN ranged from 0.72 to 1.11 by varying the BC density from 0.14 to 2.1 g cm−3 at the typical S of 0.23 % and 0.40 % (Fig. 6c and d), showing an estimation uncertainty of −28 %–11 % in the NCCN prediction.
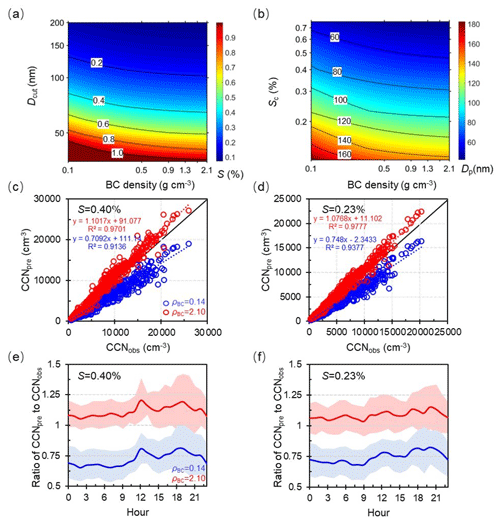
Figure 6Sensitivity of critical supersaturation (Sc) (a) and diameter (Dcut) (b) to the variations in BC density. Predicted NCCN as a function of measured NCCN by varying the density from 0.14 to 2.1 g cm−3 at S=0.40 % (c) and S=0.23 % (d). The solid black line is the 1:1 line. Diurnal variations in the ratio of the predicted-to-measured NCCN at S=0.40 % (e) and S=0.23 % (f) are shown.
The diurnal variations in the ratio of predicted-to-measured NCCN at S=0.40 % and 0.23 % are shown to examine the response of the BC density on NCCN prediction at different time periods (Fig. 6e and f). By applying the lower limit of the density value of 0.14 g cm−3, the prediction was much worse than when using the density of 2.1 g cm−3 at night (00:00–06:00 LT), which is when the latter was much closer to the real density of ambient BC (Fig. 4c). The prediction was improved substantially by applying the value of 0.14 g cm−3 during evening rush hours (18:00–20:00 LT), during which the ambient BC particles were disturbed by the traffic emissions (Fig. 4c). The prediction became worse by applying the value of 2.1 g cm−3, and an obvious overestimation by up to ∼40 % was shown. The results further illustrate that it is critical to account for the real-time mixing state and density of BC particles in NCCN prediction, particularly in regions with heavy traffic and residential coal emissions.
It should be noted that the assumption of the surface tension of water would overestimate the critical diameter and underpredict CCN number concentration, while the surface tension depression might be more obvious for small-sized particles (<60 nm), as the fraction of organics is higher at small particle sizes (Meng et al., 2014; Cai et al., 2018). Here, in this study, we calculated the critical diameters at supersaturations of 0.40 % and 0.23 %, typical values in cloud, corresponding to larger sizes (>70 and 90 nm) of aerosols. Therefore, the uncertainties from the application of the surface tension of pure water should be negligible (<10 %).
3.3 NCCN prediction based on the real-time variations in BC density and mixing state
Figure 7 exhibits the comparisons between predicted and measured NCCN at S of 0.23 % and 0.40 % by accounting for the retrieved real-time variations in the BC density and mixing state. It shows that the NCCN can be well predicted, with a slope of 1.01 and 1.02 at S of 0.23 % and 0.40 %, respectively (Fig. 7a and b), and only presents a slight deviation. The slight deviation is primarily due to the fixed value of the density for the externally mixed BC caused by the retrieval method, especially during noontime and evening rush periods (Fig. 7c and d).
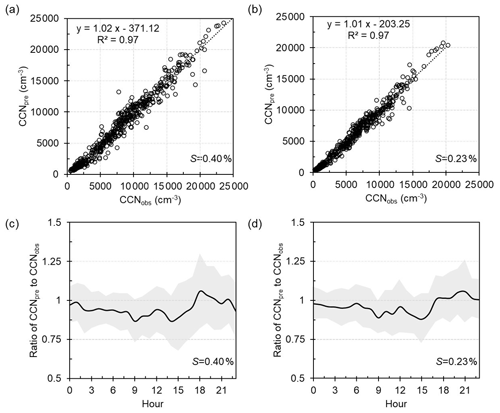
Figure 7Predicted CCN number concentration using the mixing state and In-BC density derived from HTDMAs at S=0.40 % (a) and S=0.23 % (b). Diurnal variations in the ratio of predicted-to-measured NCCN at S=0.40 % (c) and S=0.23 % (d) are shown.
The diurnal variations in the ratio of predicted-to-measured NCCN show that the NCCN can be underestimated by up to 15 % at S=0.40 % during noontime, while being slightly overrated during the evening traffic hours and nighttime, possibly due to the underestimation of the number fraction of Ex-BC. Overall, the dependence of the CCN prediction on S is due to the size dependence of κ and the mixing state (Zhang et al., 2017; Liu et al., 2020; Ren et al., 2018). A better closure at S=0.23 % is because the bulk κ of the particles is closer to that at the critical diameter of 100–150 nm. Similarly, the effect on the CCN prediction induced by the bulk mixing state would be more critical for smaller particles, corresponding to the critical diameter at high S.
Overall, when considering the effective density of BC relevant to its mixing state, the CCN closure is achieved. Previous studies have shown that the freshly emitted BC particles may convert from fractal-like aggregates to a compact structure, and their density would increase with the aging process (H. Liu et al., 2019; F. Zhang et al., 2020, 2022), but the actual density of In-BC may be lower than 1.8 g cm−3 in the ambient atmosphere, according to this study. Therefore, the currently applied value represents a density of the void-free structure of BC particles and may cause an overestimation in CCN prediction. In addition, although the BC accounts for small mass fractions in ambient fine aerosols, according to the measurements simultaneously conducted at the site, the BC-containing particles could contribute 60 %–78 % to the total number concentration in urban Beijing (Chen et al., 2020). Our results further highlight that the effect of BC density on the uncertainty in CCN prediction should be concerned carefully.
The mixing state and effective density of BC changed through the heterogenous chemistry process and thus would cause uncertainty in evaluating its CCN activity. In this study, we develop a new method to retrieve the mixing state and effective density of ambient BC using field measurements and the Köhler theory. The uncertainty in the new retrieval method was evaluated within ±30 %, which was primarily caused by assuming the value of κSOA and the fraction of primary organic aerosols in the non-hygroscopic mode. The retrieved results show that most of the BC particles were aged and internally mixed with other components, with the mean mass fraction of 80 % ± 20 %. Averagely, the retrieved densities of the bulk and internally mixed BC were 0.7 ± 0.5 and 1.1 ± 0.6 g cm−3, respectively, but ranged widely from ∼0.1 to 2.5 g cm−3, indicating various morphologies and different aging degrees and compositions of ambient BC particles, due to the complex impact of multiple local sources and aging processes during the observed period. The retrieved result was basically comparable with previous observations in the North China Plain.
A further examination shows that the uncertainties in the NCCN prediction were −28 %–11 % at the typical S of 0.23 % and 0.40 %, by varying the BC density from 0.14 to 2.1 g cm−3 that represented the range of ambient BC particles. Moreover, the prediction was found to be more sensitive to the variations in the BC density when it was <1.0 g cm−3, suggesting a great significance of accounting for the effect of BC density on the aerosol particles with a low aging degree when evaluating the climate effect. The CCN closure was achieved when introducing the retrieved real-time BC density relevant to its mixing state. This work provides a unique way of utilizing field observations to infer ambient BC density and highlights that the current assumption of a void-free structure of BC particles in models would cause large uncertainties in CCN prediction and in the relevant climate effect evaluation.
The method used to derive the ambient BC density has limitations, since the assumptions of the values of κSOA, ρPOA, ρSOA, and ρEx-BC, as well as the fraction of primary organic aerosols in non-hygroscopic mode, would add uncertainty to the inferred values of ambient internally mixed BC density. It is thus necessary to examine observational data to verify this methodology in future studies. However, the method and results of this study could provide an avenue for a more comprehensive understanding of the variations in the BC density in Beijing. Additionally, it has the potential to reveal the uncertainties in the usage of a void-free structure of the BC density when assessing the climate effects.
All data needed to evaluate the conclusions in the paper are presented in the paper and/or the Supplement. All data used in the study are also available from the corresponding author upon request (zhangfang2021@hit.edu.cn).
The supplement related to this article is available online at: https://doi.org/10.5194/acp-23-4327-2023-supplement.
FZ and JR conceived the conceptual development of the paper. JR directed and performed of the experiments with JL, LC, and FZ. JR conducted the data analysis and wrote the draft. All authors edited and commented on the various sections of the paper.
The contact author has declared that none of the authors has any competing interests.
Publisher's note: Copernicus Publications remains neutral with regard to jurisdictional claims in published maps and institutional affiliations.
This work has been funded by the National Natural Science Foundation of China (NSFC) research project (grant nos. 41975174 and 41675141) and the Shenzhen Science and Technology Plan Project (grant no. GXWD20220811174022002). We thank all participants in the field campaigns for their tireless work and cooperation. We also thank Yele Sun and his group for providing the data of the nonrefractory submicron aerosol chemical composition.
This research has been supported by the National Natural Science Foundation of China (grant nos. 41975174 and 41675141) and the Shenzhen Science and Technology Plan Project (grant no. GXWD20220811174022002).
This paper was edited by Zhibin Wang and reviewed by four anonymous referees.
Alfarra, M. R., Paulsen, D., Gysel, M., Garforth, A. A., Dommen, J., Prévôt, A. S. H., Worsnop, D. R., Baltensperger, U., and Coe, H.: A mass spectrometric study of secondary organic aerosols formed from the photooxidation of anthropogenic and biogenic precursors in a reaction chamber, Atmos. Chem. Phys., 6, 5279–5293, https://doi.org/10.5194/acp-6-5279-2006, 2006.
Bond, T. C., Doherty, S. J., Fahey, D., Forster, P., Berntsen, T., DeAngelo, B., Flanner, M., Ghan, S., Kärcher, B., and Koch, D.: Bounding the role of black carbon in the climate system: A scientific assessment, J. Geophys. Res.-Atmos., 118, 5380–5552, https://doi.org/10.1002/jgrd.50171, 2013.
Cai, M., Tan, H., Chan, C. K., Qin, Y., Xu, H., Li, F., Schurman, M. I., Liu, L., and Zhao, J.: The size-resolved cloud condensation nuclei (CCN) activity and its prediction based on aerosol hygroscopicity and composition in the Pearl Delta River (PRD) region during wintertime 2014, Atmos. Chem. Phys., 18, 16419–16437, https://doi.org/10.5194/acp-18-16419-2018, 2018.
Chang, R. Y. W., Liu, P. S. K., Leaitch, W. R., and Abbatt, J. P. D.: Comparison between measured and predicted CCN concentrations at Egbert, Ontario: Focus on the organic aerosol fraction at a semirural site, Atmos. Environ., 41, 8172–8182, https://doi.org/10.1016/j.atmosenv.2007.06.039, 2007.
Chang, R. Y.-W., Slowik, J. G., Shantz, N. C., Vlasenko, A., Liggio, J., Sjostedt, S. J., Leaitch, W. R., and Abbatt, J. P. D.: The hygroscopicity parameter (κ) of ambient organic aerosol at a field site subject to biogenic and anthropogenic influences: relationship to degree of aerosol oxidation, Atmos. Chem. Phys., 10, 5047–5064, https://doi.org/10.5194/acp-10-5047-2010, 2010.
Chen, L., Zhang, F., Yan, P., Wang, X., Sun, L., Li, Y., Zhang, X., Sun, Y., and Li, Z.: The large proportion of black carbon (BC)-containing aerosols in the urban atmosphere, Environ. Pollut., 263, 114507, https://doi.org/10.1016/j.envpol.2020.114507, 2020.
Cheng, Y. F., Eichler, H., Wiedensohler, A., Heintzenberg, J., Zhang, Y. H., Hu, M., Herrmann, H., Zeng, L. M., Liu, S., Gnauk, T., Brüggemann, E., and He, L. Y.: Mixing state of elemental carbon and non-light-absorbing aerosol components derived from in situ particle optical properties at Xinken in Pearl River Delta of China, J. Geophys. Res., 111, D20204, https://doi.org/10.1029/2005JD006929, 2006.
Cheng, Y. F., Su, H., Rose, D., Gunthe, S. S., Berghof, M., Wehner, B., Achtert, P., Nowak, A., Takegawa, N., Kondo, Y., Shiraiwa, M., Gong, Y. G., Shao, M., Hu, M., Zhu, T., Zhang, Y. H., Carmichael, G. R., Wiedensohler, A., Andreae, M. O., and Pöschl, U.: Size-resolved measurement of the mixing state of soot in the megacity Beijing, China: diurnal cycle, aging and parameterization, Atmos. Chem. Phys., 12, 4477–4491, https://doi.org/10.5194/acp-12-4477-2012, 2012.
Clarke, A. D., Shinozuka, Y., Kapustin, V. N., Howell, S., Huebert, B., Doherty, S., Anderson, T., Covert, D., Anderson, J., Hua, X., Moore II, K. G., McNaughton, C., Carmichael, G., and Weber, R.: Size distributions and mixtures of dust and black carbon aerosol in Asian outflow: physiochemistry and optical properties, J. Geophys. Res.-Atmos., 109, D15S09, https://doi.org/10.1029/2003JD004378, 2004.
Dameto, C., Wonaschütz, A., Steiner, G., Rosati, B., Demattio, A., Schuh, H., and Hitzenberger, R.: Long-term quantitative field study of New Particle Formation (NPF) events as a source of Cloud Condensation Nuclei (CCN) in the urban background of Vienna, Atmos. Environ., 164, 289–298, https://doi.org/10.1016/j.atmosenv.2017.06.001, 2017.
Dinar, E., Mentel, T. F., and Rudich, Y.: The density of humic acids and humic like substances (HULIS) from fresh and aged wood burning and pollution aerosol particles, Atmos. Chem. Phys., 6, 5213–5224, https://doi.org/10.5194/acp-6-5213-2006, 2006.
Fan, X., Liu, J., Zhang, F., Chen, L., Collins, D., Xu, W., Jin, X., Ren, J., Wang, Y., Wu, H., Li, S., Sun, Y., and Li, Z.: Contrasting size-resolved hygroscopicity of fine particles derived by HTDMA and HR-ToF-AMS measurements between summer and winter in Beijing: the impacts of aerosol aging and local emissions, Atmos. Chem. Phys., 20, 915–929, https://doi.org/10.5194/acp-20-915-2020, 2020.
Flanner, M. G., Zender, C. S., Randerson, J. T., and Rasch, P. J.: Present-day climate forcing and response from black carbon in snow, J. Geophys. Res.-Atmos., 112, D11202, https://doi.org/10.1029/2006JD008003, 2007.
Geller, M., Biswas, S., and Sioutas, C.: Determination of particle effective density in urban environments with a differential mobility analyzer and aerosol particle mass analyzer, Aerosol Sci. Tech., 40, 709–723, https://doi.org/10.1080/02786820600803925, 2006.
Gunthe, S. S., King, S. M., Rose, D., Chen, Q., Roldin, P., Farmer, D. K., Jimenez, J. L., Artaxo, P., Andreae, M. O., Martin, S. T., and Pöschl, U.: Cloud condensation nuclei in pristine tropical rainforest air of Amazonia: size-resolved measurements and modeling of atmospheric aerosol composition and CCN activity, Atmos. Chem. Phys., 9, 7551–7575, https://doi.org/10.5194/acp-9-7551-2009, 2009.
Gysel, M., Crosier, J., Topping, D. O., Whitehead, J. D., Bower, K. N., Cubison, M. J., Williams, P. I., Flynn, M. J., McFiggans, G. B., and Coe, H.: Closure study between chemical composition and hygroscopic growth of aerosol particles during TORCH2, Atmos. Chem. Phys., 7, 6131–6144, https://doi.org/10.5194/acp-7-6131-2007, 2007.
Gysel, M., McFiggans, G. B., and Coe, H.: Inversion of tandem differential mobility analyser (TDMA) measurements, J. Aerosol Sci., 40, 134–151, https://doi.org/10.1016/j.jaerosci.2008.07.013, 2009.
Gysel, M., Laborde, M., Olfert, J. S., Subramanian, R., and Gröhn, A. J.: Effective density of Aquadag and fullerene soot black carbon reference materials used for SP2 calibration, Atmos. Meas. Tech., 4, 2851–2858, https://doi.org/10.5194/amt-4-2851-2011, 2011.
Gysel, M., Laborde, M., Mensah, A. A., Corbin, J. C., Keller, A., Kim, J., Petzold, A., and Sierau, B.: Technical Note: The single particle soot photometer fails to reliably detect PALAS soot nanoparticles, Atmos. Meas. Tech., 5, 3099–3107, https://doi.org/10.5194/amt-5-3099-2012, 2012.
Jimenez, J. L., Canagaratna, M. R., Donahue, N. M., Prevot, A. S. H., Zhang, Q., Kroll, J. H., DeCarlo, P. F., Allan, J. D., Coe, H., Ng, N. L., Aiken, A. C., Docherty, K. S., Ulbrich, I. M., Grieshop, A. P., Robinson, A. L., Duplissy, J., Smith, J. D., Wilson, K. R., Lanz, V. A., Hueglin, C., Sun, Y. L., Tian, J., Laaksonen, A., Raatikainen, T., Rautiainen, J., Vaattovaara, P., Ehn, M., Kulmala, M., Tomlinson, J. M., Collins, D. R., Cubison, M. J., Dunlea, E. J., Huffman, J. A., Onasch, T. B., Alfarra, M. R., Williams, P. I., Bower, K., Kondo, Y., Schneider, J., Drewnick, F., Borrmann, S., Weimer, S., Demerjian, K., Salcedo, D., Cottrell, L., Griffin, R., Takami, A., Miyoshi, T., Hatakeyama, S., Shimono, A., Sun, J. Y., Zhang, Y. M., Dzepina, K., Kimmel, J. R., Sueper, D., Jayne, J. T., Herndon, S. C., Trimborn, A. M., Williams, L. R., Wood, E. C., Middlebrook, A. M., Kolb, C. E., Baltensperger, U., and Worsnop, D. R.: Evolution of Organic Aerosols in the Atmosphere, Science, 326, 1525–1529, https://doi.org/10.1126/science.1180353, 2009.
Kawana, K., Nakayama, T., and Mochida, M.: Hygroscopicity and CCN activity of atmospheric aerosol particles and their relation to organics: Characteristics of urban aerosols in Nagoya, Japan, J. Geophys. Res.-Atmos., 121, 4100–4121, https://doi.org/10.1002/2015JD023213, 2016.
Khalizov, A. F., Zhang, R., Zhang, D., Xue, H., Pagels, J., and McMurry, P. H.: Formation of highly hygroscopic soot aerosols upon internal mixing with sulfuric acid vapor, J. Geophys. Res.-Atmos., 114, D05208, https://doi.org/10.1029/2008jd010595, 2009.
Kiselev, A., Wennrich, C., Stratmann, F., Wex, H., Henning, S., Mentel, T. F., Kiendler-Scharr, A., Schneider, J., Walter, S., and Lieberwirth, I.: Morphological characterization of soot aerosol particles during LACIS Experiment in November (LExNo), J. Geophys. Res.-Atmos., 115, D11204, https://doi.org/10.1029/2009jd012635, 2010.
Kostenidou, E., Pathak, R. K., and Pandis, S. N.: An Algorithm for the Calculation of Secondary Organic Aerosol Density Combining AMS and SMPS Data, Aerosol Sci. Tech., 41, 1002–1010, https://doi.org/10.1080/02786820701666270, 2007.
Lance, S., Medina, J., Smith, J., and Nenes, A.: Mapping the operation of the DMT continuous flow CCN counter, Aerosol Sci. Tech., 40, 242–254, https://doi.org/10.1080/02786820500543290, 2006.
Li, M., Zhang, Q., Kurokawa, J.-I., Woo, J.-H., He, K., Lu, Z., Ohara, T., Song, Y., Streets, D. G., Carmichael, G. R., Cheng, Y., Hong, C., Huo, H., Jiang, X., Kang, S., Liu, F., Su, H., and Zheng, B.: MIX: a mosaic Asian anthropogenic emission inventory under the international collaboration framework of the MICS-Asia and HTAP, Atmos. Chem. Phys., 17, 935–963, https://doi.org/10.5194/acp-17-935-2017, 2017.
Lide, D. R. (Ed.): CRC Handbook of Chemistry and Physics, CRC Press Inc., Boca Raton, Florida, 1992.
Liu, D., Allan, J., Whitehead, J., Young, D., Flynn, M., Coe, H., McFiggans, G., Fleming, Z. L., and Bandy, B.: Ambient black carbon particle hygroscopic properties controlled by mixing state and composition, Atmos. Chem. Phys., 13, 2015–2029, https://doi.org/10.5194/acp-13-2015-2013, 2013.
Liu, D., Joshi, R., Wang, J., Yu, C., Allan, J. D., Coe, H., Flynn, M. J., Xie, C., Lee, J., Squires, F., Kotthaus, S., Grimmond, S., Ge, X., Sun, Y., and Fu, P.: Contrasting physical properties of black carbon in urban Beijing between winter and summer, Atmos. Chem. Phys., 19, 6749–6769, https://doi.org/10.5194/acp-19-6749-2019, 2019.
Liu, H., Pan, X., Wu, Y., Wang, D., Tian, Y., Liu, X., Lei, L., Sun, Y., Fu, P., and Wang, Z.: Effective densities of soot particles and their relationships with the mixing state at an urban site in the Beijing megacity in the winter of 2018, Atmos. Chem. Phys., 19, 14791–14804, https://doi.org/10.5194/acp-19-14791-2019, 2019.
Liu, H., Pan, X., Liu, D., Liu, X., Chen, X., Tian, Y., Sun, Y., Fu, P., and Wang, Z.: Mixing characteristics of refractory black carbon aerosols at an urban site in Beijing, Atmos. Chem. Phys., 20, 5771–5785, https://doi.org/10.5194/acp-20-5771-2020, 2020.
Liu, J., Zhang, F., Xu, W., Sun, Y., Chen, L., and Li, S.: Hygroscopicity of organic aerosols linked to formation mechanisms, Geophys. Res. Lett., 48, e2020GL091683, https://doi.org/10.1029/2020gl091683, 2021.
Liu, L., Zhang, J., Zhang, Y., Wang, Y., Xu, L., Yuan, Q., Liu, D., Sun, Y., Fu, P., Shi, Z., and Li, W.: Persistent residential burning-related primary organic particles during wintertime hazes in North China: insights into their aging and optical changes, Atmos. Chem. Phys., 21, 2251–2265, https://doi.org/10.5194/acp-21-2251-2021, 2021.
Massoli, P., Onasch, T. B., Cappa, C. D., Nuamaan, I., Hakala, J., Hayden, K., Li, S. M., Sueper, D. T., Bates, T. S., Quinn, P. K., Jayne, J. T., and Worsnop, D. R.: Characterization of black carbon-containing particles from soot particle aerosol mass spectrometer measurements on the R/V Atlantis during CalNex 2010, J. Geophys. Res.-Atmos., 120, 2575–2593, https://doi.org/10.1002/2014JD022834, 2015.
McMeeking, G. R., Hamburger, T., Liu, D., Flynn, M., Morgan, W. T., Northway, M., Highwood, E. J., Krejci, R., Allan, J. D., Minikin, A., and Coe, H.: Black carbon measurements in the boundary layer over western and northern Europe, Atmos. Chem. Phys., 10, 9393–9414, https://doi.org/10.5194/acp-10-9393-2010, 2010.
McMurry, H. P., Wang, X., Park, K., and Ehara, K.: The Relationship between Mass and Mobility for Atmospheric Particles: A New Technique for Measuring Particle Density, Aerosol Sci. Tech., 36, 227–238, https://doi.org/10.1080/027868202753504083, 2002.
Mei, F., Setyan, A., Zhang, Q., and Wang, J.: CCN activity of organic aerosols observed downwind of urban emissions during CARES, Atmos. Chem. Phys., 13, 12155–12169, https://doi.org/10.5194/acp-13-12155-2013, 2013.
Meng, J. W., Yeung, M. C., Li, Y. J., Lee, B. Y. L., and Chan, C. K.: Size-resolved cloud condensation nuclei (CCN) activity and closure analysis at the HKUST Supersite in Hong Kong, Atmos. Chem. Phys., 14, 10267–10282, https://doi.org/10.5194/acp-14-10267-2014, 2014.
Noureddini, H., Teoh, B. C., and Davis Clements, L.: Densities of vegetable oils and fatty acids, J. Am. Oil Chem. Soc., 69, 1184–1188, 1992.
Olfert, J. S., Symonds, J. P. R., and Collings, N.: The effective density and fractal dimension of particles emitted from a light-duty diesel vehicle with a diesel oxidation catalyst, J. Aerosol Sci., 38, 69–82, https://doi.org/10.1016/j.jaerosci.2006.10.002, 2007.
Paatero, P. and Tapper, U.: Positive matrix factorization: A nonnegative factormodel with optimal utilization of error estimates of data values, Environmetrics, 5, 111–126, 1994.
Pagels, J., Khalizov, A. F., McMurry, P. H., and Zhang, R. Y.: Processing of soot by controlled sulphuric acid and water condensation-mass and mobility relationship, Aerosol Sci. Tech., 43, 629–640, https://doi.org/10.1080/02786820902810685, 2009.
Pan, X., Kanaya, Y., Taketani, F., Miyakawa, T., Inomata, S., Komazaki, Y., Tanimoto, H., Wang, Z., Uno, I., and Wang, Z.: Emission characteristics of refractory black carbon aerosols from fresh biomass burning: a perspective from laboratory experiments, Atmos. Chem. Phys., 17, 13001–13016, https://doi.org/10.5194/acp-17-13001-2017, 2017.
Park, K., Cao, F., Kittelson, D. B., and McMurry, P. H.: Relationship between particle mass and mobility for diesel exhaust particles, Environ. Sci. Technol., 37, 577–583, https://doi.org/10.1021/es025960v, 2003.
Park, K., Kittelson, D. B., and McMurry, P. H.: Structural properties of diesel exhaust particles measured by transmission electron microscopy (TEM): Relationships to particle mass and mobility, Aerosol Sci. Tech., 38, 881–889, https://doi.org/10.1080/027868290505189, 2004.
Peng, J., Hu, M., Guo, S., Du, Z., Shang, D., Zheng, J., Zheng, J., Zeng, L., Shao, M., Wu, Y., Collins, D., and Zhang, R.: Ageing and hygroscopicity variation of black carbon particles in Beijing measured by a quasi-atmospheric aerosol evolution study (QUALITY) chamber, Atmos. Chem. Phys., 17, 10333–10348, https://doi.org/10.5194/acp-17-10333-2017, 2017.
Peng, J. F., Hu, M., Guo, S., Du, Z. F., Zheng, J., Shang, D. J., Zamora, M., Zeng, L. M., Shao, M., Wu, Y. S., Zheng, J., Wang, Y., Glen, C., Collins, D., Molina, M., and Zhang, R. Y.: Markedly enhanced absorption, and direct radiative forcing of black carbon under polluted urban environments, P. Natl. Acad. Sci. USA, 113, 4266–4271, https://doi.org/10.1073/pnas.1602310113, 2016.
Petters, M. D. and Kreidenweis, S. M.: A single parameter representation of hygroscopic growth and cloud condensation nucleus activity, Atmos. Chem. Phys., 7, 1961–1971, https://doi.org/10.5194/acp-7-1961-2007, 2007.
Pileci, R. E., Modini, R. L., Bertò, M., Yuan, J., Corbin, J. C., Marinoni, A., Henzing, B., Moerman, M. M., Putaud, J. P., Spindler, G., Wehner, B., Müller, T., Tuch, T., Trentini, A., Zanatta, M., Baltensperger, U., and Gysel-Beer, M.: Comparison of co-located refractory black carbon (rBC) and elemental carbon (EC) mass concentration measurements during field campaigns at several European sites, Atmos. Meas. Tech., 14, 1379–1403, https://doi.org/10.5194/amt-14-1379-2021, 2021.
Qiao, K., Wu, Z., Pei, X., Liu, Q., Shang, D., Zheng, J., Du, Z., Zhu, W., Wu, Y., Lou, S., Guo, S., Chan, C. K., Pathak, R. K., Hallquist, M., and Hu, M.: Size-resolved effective density of submicron particles during summertime in the rural atmosphere of Beijing, China, J. Environ. Sci.-China, 73, 69–77, https://doi.org/10.1016/j.jes.2018.01.012, 2018.
Rader, D. J. and McMurry, P. H.: Application of the tandem differential mobility analyzer to studies of droplet growth or evaporation, J. Geophys. Res.-Atmos., 17, 771–787, https://doi.org/10.1016/0021-8502(86)90031-5, 1986.
Ramanathan, V. and Carmichael, G.: Global and regional climate changes due to black carbon, Nat. Geosci., 36, 221–227, https://doi.org/10.1038/ngeo156, 2008.
Ren, J., Zhang, F., Wang, Y., Collins, D., Fan, X., Jin, X., Xu, W., Sun, Y., Cribb, M., and Li, Z.: Using different assumptions of aerosol mixing state and chemical composition to predict CCN concentrations based on field measurements in urban Beijing, Atmos. Chem. Phys., 18, 6907–6921, https://doi.org/10.5194/acp-18-6907-2018, 2018.
Ren, J., Zhang, F., Chen, L., Cao, G., Liu, M., Li, X., Wu, H., Cheng, Y., and Li, Z.: Identifying the hygroscopic properties of fine aerosol particles from diverse sources in urban atmosphere and the applicability in prediction of cloud nuclei, Atmos. Environ., 298, 119615, https://doi.org/10.1016/j.atmosenv.2023.119615, 2023.
Reyes-Villegas, E., Bannan, T., Le Breton, M., Mehra, A., Priestley, M., Percival, C., Coe, H., and Allan, J. D.: Online Chemical Characterization of Food-Cooking Organic Aerosols: Implications for Source Apportionment, Environ. Sci. Technol., 52, 5308–5318, https://doi.org/10.1021/acs.est.7b06278, 2018.
Riemer, N., Vogel, H., and Vogel, B.: Soot aging time scales in polluted regions during day and night, Atmos. Chem. Phys., 4, 1885–1893, https://doi.org/10.5194/acp-4-1885-2004, 2004.
Rissler, J., Nordin, E. Z., Eriksson, A. C., Nilsson, P. T., Frosch, M., Sporre, M. K., Wierzbicka, A., Svenningsson, B., Londahl, J., Messing, M. E., Sjogren, S., Hemmingsen, J. G., Loft, S., Pagels, J. H., and Swietlicki, E.: Effective Density and Mixing State of Aerosol Particles in a Near-Traffic Urban Environment, Environ. Sci. Technol., 48, 6300–6308, https://doi.org/10.1021/es5000353, 2014.
Schwarz, J. P., Gao, R. S., Fahey, D. W., Thomson, D. S., Watts, L. A., Wilson, J. C., Reeves, J. M., Darbeheshti, M., Baumgardner, D. G., Kok, G. L., Chung, S. H., Schulz, M., Hendricks, J., Lauer, A., Kärcher, B., Slowik, J. G., Rosenlof, K. H., Thompson, T. L., Langford, A. O., Loewenstein, M., and Aikin, K. C.: Single-particle measurements of midlatitude black carbon and light-scattering aerosols from the boundary layer to the lower stratosphere, J. Geophys. Res.-Atmos., 111, D16207, https://doi.org/10.1029/2006JD007076, 2006.
Schwarz, J. P., Gao, R. S., Spackman, J. R., Watts, L. A., Thomson, D. S., Fahey, D. W., Ryerson, T. B., Peischl, J., Holloway, J. S., Trainer, M., Frost, G. J., Baynard, T., Lack, D. A., de Gouw, J. A., Warneke, C., and Del Negro, L. A.: Measurement of the mixing state, mass, and optical size of individual black carbon particles in urban and biomass burning emissions, Geophys. Res. Lett., 35, L13810, https://doi.org/10.1029/2008GL033968, 2008.
Shiraiwa, M., Kondo, Y., Moteki, N., Takegawa, N., Sahu, L., Takami, A., Hatakeyama, S., Yonemura, S., and Blake, D. R.: Radiative impact of mixing state of black carbon aerosol in Asian outflow, J. Geophys. Res.-Atmos., 113, D24210, https://doi.org/10.1029/2008JD010546, 2008.
Stokes, R. and Robinson, R.: Interactions in aqueous nonelectrolyte solutions, I. Solute-solvent equilibria, J. Phys. Chem.-US, 70, 2126–2131, 1966.
Sun, Y., Du, W., Fu, P., Wang, Q., Li, J., Ge, X., Zhang, Q., Zhu, C., Ren, L., Xu, W., Zhao, J., Han, T., Worsnop, D. R., and Wang, Z.: Primary and secondary aerosols in Beijing in winter: sources, variations and processes, Atmos. Chem. Phys., 16, 8309–8329, https://doi.org/10.5194/acp-16-8309-2016, 2016.
Tan, H., Xu, H., Wan, Q., Li, F., Deng, X., Chan, P. W., Xia, D., and Yin, Y.: Design and application of an unattended multifunctional H-TDMA system, J. Atmos. Ocean. Tech., 30, 1136–1148, https://doi.org/10.1175/JTECH-D-12-00129.1, 2013.
Ulbrich, I. M., Canagaratna, M. R., Zhang, Q., Worsnop, D. R., and Jimenez, J. L.: Interpretation of organic components from Positive Matrix Factorization of aerosol mass spectrometric data, Atmos. Chem. Phys., 9, 2891–2918, https://doi.org/10.5194/acp-9-2891-2009, 2009.
Wang, Y., Wan, Q., Meng, W., Liao, F., Tan, H., and Zhang, R.: Long-term impacts of aerosols on precipitation and lightning over the Pearl River Delta megacity area in China, Atmos. Chem. Phys., 11, 12421–12436, https://doi.org/10.5194/acp-11-12421-2011, 2011.
Wang, Y. Y., Liu, F. S., He, C. L., Bi, L., Cheng, T. H., Wang, Z. L., Zhang, H., Zhang, X. Y., Shi, Z. B., and Li, W. J.: Fractal dimensions and mixing structures of soot particles during atmospheric processing, Environ. Sci. Tech. Let., 4, 487–493, https://doi.org/10.1021/acs.estlett.7b00418, 2017.
Wu, Y., Wang, X., Tao, J., Huang, R., Tian, P., Cao, J., Zhang, L., Ho, K.-F., Han, Z., and Zhang, R.: Size distribution and source of black carbon aerosol in urban Beijing during winter haze episodes, Atmos. Chem. Phys., 17, 7965–7975, https://doi.org/10.5194/acp-17-7965-2017, 2017.
Wu, Y., Xia, Y., Huang, R., Deng, Z., Tian, P., Xia, X., and Zhang, R.: A study of the morphology and effective density of externally mixed black carbon aerosols in ambient air using a size-resolved single-particle soot photometer (SP2), Atmos. Meas. Tech., 12, 4347–4359, https://doi.org/10.5194/amt-12-4347-2019, 2019.
Wu, Z. J., Zheng, J., Shang, D. J., Du, Z. F., Wu, Y. S., Zeng, L. M., Wiedensohler, A., and Hu, M.: Particle hygroscopicity and its link to chemical composition in the urban atmosphere of Beijing, China, during summertime, Atmos. Chem. Phys., 16, 1123–1138, https://doi.org/10.5194/acp-16-1123-2016, 2016.
Xu, W., Sun, Y., Wang, Q., Zhao, J., Wang, J., Ge, X., Xie, C., Zhou, W., Du, W., Li, J., Fu, P., Wang, Z., Worsnop, D. R., and Coe, H.: Changes in aerosol chemistry from 2014 to 2016 in winter in beijing: Insights from high-resolution aerosol mass spectrometry, J. Geophys. Res.-Atmos., 124, 1132–1147, https://doi.org/10.1029/2018jd029245, 2019.
Xue, H., Khalizov, A. F., Wang, L., Zheng, J., and Zhang, R.: Effects of dicarboxylic acid coating on the optical properties of soot, Phys. Chem. Chem. Phys., 11, 7869–7875, https://doi.org/10.1039/b904129j, 2009.
Yu, C., Liu, D., Broda, K., Joshi, R., Olfert, J., Sun, Y., Fu, P., Coe, H., and Allan, J. D.: Characterising mass-resolved mixing state of black carbon in Beijing using a morphology-independent measurement method, Atmos. Chem. Phys., 20, 3645–3661, https://doi.org/10.5194/acp-20-3645-2020, 2020.
Yuan, T., Li, Z., Zhang, R., and Fan, J.: Increase of cloud droplet size with aerosol optical depth: An observation and modeling study, J. Geophys. Res.-Atmos., 113, D04201, https://doi.org/10.1029/2007JD008632, 2008.
Zdanovskii, A.: New methods for calculating solubilities of electrolytes in multicomponent systems, Zh. Fiz. Khim. C, 22, 1475–1485, 1948.
Zhang, F., Li, Z., Li, Y., Sun, Y., Wang, Z., Li, P., Sun, L., Wang, P., Cribb, M., Zhao, C., Fan, T., Yang, X., and Wang, Q.: Impacts of organic aerosols and its oxidation level on CCN activity from measurement at a suburban site in China, Atmos. Chem. Phys., 16, 5413–5425, https://doi.org/10.5194/acp-16-5413-2016, 2016.
Zhang, F., Wang, Y., Peng, J., Ren, J., Collins, D., Zhang, R., Sun, Y., Yang, X., and Li, Z.: Uncertainty in predicting CCN activity of aged and primary aerosols, J. Geophys. Res.-Atmos., 122, 11723–11736, https://doi.org/10.1002/2017jd027058, 2017.
Zhang, F., Ren, J., Fan, T., Chen, L., Xu, W., Sun, Y., Zhang, R., Liu, J., Jiang, S., Jin, X., Wu, H., Li, S., Cribb, M. C., and Li, Z.: Significantly enhanced aerosol CCN activity and number, J. Geophys. Res.-Atmos., 124, 14102–14113, https://doi.org/10.1029/2019jd031457, 2019.
Zhang, F., Wang, Y., Peng, J., Chen, L., Sun, Y., Duan, L., Ge, X., Li, Y., Zhao, J., Liu, C., Zhang, X., Zhang, G., Pan, Y., Wang, Y., Zhang, A. L., Ji, Y., Wang, G., Hu, M., Molina, M. J., and Zhang, R.: An unexpected catalyst dominates formation and radiative forcing of regional haze, P. Natl. Acad. Sci. USA, 117, 3960–3966, https://doi.org/10.1073/pnas.1919343117, 2020.
Zhang, F., Peng, J., Chen, L., Collins, D., Li, Y., Jiang, S., Liu, J., and Zhang, R.: The effect of Black carbon aging from NO2 oxidation of SO2 on its morphology, optical and hygroscopic properties, Environ. Res., 212, 113238, https://doi.org/10.1016/j.envres.2022.113238, 2022.
Zhang, R., Wang, G., Guo, S., Zamora, M. L., Ying, Q., and Lin, Y.: Formation of urban fine particulate matter, Chem. Rev., 115, 3803–3855, https://doi.org/10.1021/acs.chemrev.5b00067, 2015.
Zhang, R. Y., Khalizov, A. F., Pagels, J., Zhang, D., Xue, H. X., and McMurry, P. H.: Variability in morphology, hygroscopicity, and optical properties of soot aerosols during atmospheric processing, P. Natl. Acad. Sci. USA, 105, 10291–10296, https://doi.org/10.1073/pnas.0804860105, 2008.
Zhang, Y., Zhang, Q., Cheng, Y., Su, H., Kecorius, S., Wang, Z., Wu, Z., Hu, M., Zhu, T., Wiedensohler, A., and He, K.: Measuring the morphology and density of internally mixed black carbon with SP2 and VTDMA: new insight into the absorption enhancement of black carbon in the atmosphere, Atmos. Meas. Tech., 9, 1833–1843, https://doi.org/10.5194/amt-9-1833-2016, 2016.
Zhang, Y., Zhang, Q., Yao, Z., and Li, H.: Particle Size and Mixing State of Freshly Emitted Black Carbon from Different Combustion Sources in China, Environ. Sci. Technol., 54, 7766–7774, https://doi.org/10.1021/acs.est.9b07373, 2020.
Zhao, G., Tan, T., Hu, S., Du, Z., Shang, D., Wu, Z., Guo, S., Zheng, J., Zhu, W., Li, M., Zeng, L., and Hu, M.: Mixing state of black carbon at different atmospheres in north and southwest China, Atmos. Chem. Phys., 22, 10861–10873, https://doi.org/10.5194/acp-22-10861-2022, 2022.