the Creative Commons Attribution 4.0 License.
the Creative Commons Attribution 4.0 License.
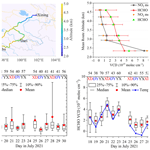
Mobile MAX-DOAS observations of tropospheric NO2 and HCHO during summer over the Three Rivers' Source region in China
Siyang Cheng
Xinghong Cheng
Xiangde Xu
Wenqian Zhang
Jinguang Lv
Gang Bai
Bing Chen
Siying Ma
Steffen Ziegler
Sebastian Donner
Thomas Wagner
The tropospheric concentrations of nitrogen dioxide (NO2) and formaldehyde (HCHO) have high spatio-temporal variability, and in situ observations of these trace gases are still scarce, especially in remote background areas. We made four similar circling journeys of mobile multi-axis differential optical absorption spectroscopy (MAX-DOAS) measurements in the Three Rivers' Source region over the Tibetan Plateau in summer (18–30 July) 2021 for the first time. The differential slant column densities (DSCDs) of NO2 and HCHO were retrieved from the measured spectra, with very weak absorptions along the driving routes. The tropospheric NO2 and HCHO vertical column densities (VCDs) were calculated from their DSCDs by the geometric approximation method, and they were further filtered to form reliable data sets by eliminating the influences of sunlight shelters and the vehicle's vibration and bumpiness. The observational data show that the tropospheric NO2 and HCHO VCDs decreased with the increasing altitude of the driving route, whose background levels ± standard deviations were 0.40 ± 1.13×1015 molec. cm−2 for NO2 and molec. cm−2 for HCHO in July 2021 over the Three Rivers' Source region. The NO2 VCDs show similar geographical distribution patterns between the different circling journeys, but the levels of the HCHO VCDs are different between the different circling journeys. The elevated NO2 VCDs along the driving routes usually corresponded to enhanced transport emissions from the towns crossed. However, the spatial distributions of the HCHO VCDs depended significantly on natural and meteorological conditions, such as surface temperature. By comparing TROPOMI satellite products and mobile MAX-DOAS results, we found that TROPOMI NO2 and HCHO VCDs have large positive offsets in the background atmosphere over the main area of the Three Rivers' Source. Our study provides valuable data sets and information of NO2 and HCHO over the Tibetan Plateau, benefitting the scientific community in investigating the spatio-temporal evolution of atmospheric composition in the background atmosphere at high altitudes, validating and improving the satellite products over mountain terrains, and evaluating the model's ability to simulate atmospheric chemistry over the Tibetan Plateau.
- Article
(11870 KB) - Full-text XML
-
Supplement
(879 KB) - BibTeX
- EndNote
The Tibetan Plateau, also known as the Qinghai–Tibet Plateau in China, is usually referred to as “the Third Pole” (or “the roof of the world”) with an average surface altitude of 4000–5000 m, covering a vast region located at 26–40∘ N latitude and 73–105∘ E longitude (Qiu, 2008). Due to thermal and dynamic processes in conditions of high altitude and large terrain, the Tibetan Plateau has an important influence on the atmospheric circulation (such as the Asian Summer Monsoon), the Asian climate and even the global climate, and the hydrological cycle (Bolin, 1950; Boos and Kuang, 2010; Dong et al., 2017; Duan et al., 2007; Liu et al., 2007; Yanai et al., 1992; Zhou et al., 2009). As the “Asian water towers”, there are many water resources in the forms of glaciers, snowpack, lakes, and rivers over the Tibetan Plateau, which are the headwaters of major rivers in Asia (such as the Indus River, Ganges River, Yangtze River, Yellow River, and Lancang River) and influence economic development and billions of peoples' survival in the downstream region (Xu et al., 2008; Gao et al., 2019). Therefore, the area of “Three Rivers' Source” (i.e. Yangtze River, Yellow River, and Lancang River) was established as one of the first five national parks in China in 2021 to better protect the ecological environment. However, we still know very little about the ecological environment, including the atmospheric environment, over this region at present. Almost no observations focus on the abundances and variations of atmospheric composition over the Three Rivers' Source region, limited by the extremely high altitude, topographical heterogeneity, variable weather, and effective techniques and methods. As one of the remote regions in Eurasia, the Tibetan Plateau, with low anthropogenic activities and a low population density, can be considered to be a natural laboratory to investigate the background atmospheric chemistry of the inner Eurasian continent (Ma et al., 2021). With increasing emissions of air pollution over the Tibetan Plateau and its surrounding areas (such as tourism in summer), measurements of the background atmosphere with a high spatio-temporal resolution are urgent to improve the understanding of the spatio-temporal evolution of the atmospheric composition (Singh, 2021; Yang et al., 2019; Kang et al., 2022).
Nitrogen dioxide (NO2) and formaldehyde (HCHO) are two important trace gases in the troposphere, participating in the control of the strong atmospheric oxidant of ozone (O3; Seinfeld and Pandis, 2016). Nitrogen oxides (NOx), i.e. the sum of NO2 and nitric oxide (NO), can be not only released by various anthropogenic emission sources, such as the burning of fossil fuels and biomass, but also emitted by natural processes, including microbial activities in soils and lightning in the atmosphere (Lee et al., 1997; Granier et al., 2011; Kurokawa et al., 2013). HCHO is produced not only by primary sources (e.g. emissions of industry and transportation in the city and biomass burning) but also by photochemical oxidation of methane and non-methane volatile organic compounds (e.g. isoprene emitted from natural vegetation) in the remote atmosphere (Stavrakou et al., 2009). High-accuracy measurements of NO2 and HCHO with high spatial and temporal resolution are beneficial to understanding their various characteristics in the background atmosphere, which is quite useful for validating the satellite products and is very valuable for exploring the processes of atmospheric chemistry.
The ground-based observations of NO2 and HCHO concentrations in the background atmosphere at high altitude are relatively scarce at present. Under the frameworks of the Global Atmosphere Watch programme of the World Meteorological Organization (WMO-GAW) and the Network for the Detection of Atmospheric Composition Change (NDACC), long-term observations of atmospheric composition have been carried out at some high mountain stations, such as the Waliguan (WLG; 3816 m above sea level) global atmosphere background observatory, located in the northeastern part of the Tibetan Plateau (Xu et al., 2020; Ma et al., 2021). With respect to NO2 at WLG, previous studies found different levels (5–600 pmol mol−1) of NO2 during different periods, leading to a positive or negative sign of net ozone production in the remote troposphere (Xue et al., 2011; Meng et al., 2010; Ma et al., 2020, 2002). Short-term HCHO observations at WLG in 2005 indicated that the possible sources for HCHO were photo-oxidation of biogenic emission of isoprene, animal excrement, and long-distance transportation from polluted air (Mu et al., 2007). The two stations of Qinghai Lake and Menyuan are adjacent to WLG, but the diurnal variations of NOx (NO2) are different and possibly influenced by traffic and residential emissions, complex terrain, boundary layer processes, and transport from city air masses (Wang et al., 2015; Zhao et al., 2020). According to the measurements at the Qomolangma Atmospheric and Environmental Observation and Research Station (QOMS; 4276 m above sea level) of the south-central Tibetan Plateau from December 2017 to March 2019, the levels of NO2 and HCHO were significantly higher than those at WLG station, related to local emissions (e.g. tourism, biomass burning, vegetation) and air pollution transport from South Asia (Xing et al., 2021; Ma et al., 2020). Increased concentrations of tropospheric NO2 at QOMS are concentrated in the lower layers, with obvious seasonal variations (peak of 1.28 nmol mol−1 in autumn) and diurnal variations (two peaks at 11:00–13:00 BJT and after 16:00 BJT; BJT denotes Beijing time and is equal to coordinated universal time plus 8 h; Xing et al., 2021). The tropospheric HCHO vertical distribution showed an exponential shape at QOMS, with a seasonal peak of 5.20 nmol mol−1 in autumn, and the peaks of HCHO appeared between 10:00–16:00 BJT in winter and spring and after 16:00 BJT in summer and autumn, respectively (Xing et al., 2021). In recent years, the China National Environmental Monitoring Center (CNEMC) also established several atmospheric composition monitoring stations over the Tibetan Plateau, but they mainly focused on the continuous monitoring of the surface particulate matter with aerodynamic diameter less than 2.5 and 10 µm (PM2.5 and PM10), NO2, sulfur dioxide (SO2), O3, and carbon oxide (CO) in cities, such as Lhasa and Xining (Chen et al., 2019; He et al., 2017; Yang et al., 2019). As a whole, these station observations cannot meet the demand of detecting the NO2 and HCHO variations with high spatial resolution over the Tibetan Plateau, which are also crucial for the validation of satellite products over areas with complex terrain. To the best of our knowledge, there are no reports about mobile measurements of NO2 and HCHO in the background atmosphere over the Tibetan Plateau.
The measurements of NO2 and HCHO with high spatial and temporal resolution are challenging over the Tibetan Plateau. In the early days, some studies on NO2 and HCHO were based on the time-consuming air-sampling method (Mu et al., 2007; Meng et al., 2010; Ma et al., 2002). The air samplers were analysed by ion chromatography or a spectrophotometer for NO2 and by high-performance liquid chromatography and mass spectrometry or gas chromatography for HCHO in the laboratory. With the development of measurement techniques, in situ methods started to be applied to measure surface concentrations of NOx (NO2) and volatile organic compounds (VOCs) at a few stations (Wang et al., 2006; Xue et al., 2011; Wang et al., 2015; Zhao et al., 2020; Ran et al., 2014; Chen et al., 2019; Yang et al., 2019; Xue et al., 2013; Duo et al., 2018). These in situ measurements at fixed stations were usually achieved by the chemiluminescence analyser for NOx (NO2) and by the gas chromatograph for VOCs, respectively. However, there are limitations in the spatio-temporal representation for the sampling and in situ measurement methods. As an alternative, satellite remote sensing can perform long-term observations of NO2 and HCHO and can cover large areas with sparse spatio-temporal resolution, but the uncertainties of satellite NO2 and HCHO products are rather large owing to complex terrain and weather over the Tibetan Plateau (Guo et al., 2016; Zhang et al., 2021). As a kind of advanced ground-based remote-sensing technique, Multi-axis differential optical absorption spectroscopy (MAX-DOAS) has been certified in the measurement techniques of NDACC (De Mazière et al., 2018). The successful observations of trace gases with very low abundances by MAX-DOAS depend on multi-factors, such as long optical paths, a high signal-to-noise ratio of the instrument, and characteristic spectral absorption features of the target species. According to previous studies, MAX-DOAS has the potential to measure tropospheric trace gases with very low level mixing ratios (pmol mol−1 order for NO2 and sub-nmol mol−1 order for HCHO) in the background atmosphere at high-altitude stations (Franco et al., 2015; Gil-Ojeda et al., 2015; Gomez et al., 2014; Marais et al., 2021; Schreier et al., 2016). Also, this technique has been used to measure the levels and monthly variations of NO2 and HCHO in the global pristine atmosphere at WLG station (Ma et al., 2020). Stratospheric O3 and its depleting substances (including NO2) have been successfully retrieved from zenith DOAS spectra at a clean suburb station in the northern Tibetan Plateau (Cheng et al., 2021). Moreover, ground-based MAX-DOAS has been applied to monitor vertical distributions of NO2 and HCHO in the southern Tibetan Plateau (Xing et al., 2021). Compared with MAX-DOAS observations at a fixed site, mobile MAX-DOAS measurements in the background atmosphere over the Tibetan Plateau are a greater challenge for the following reasons: (1) the vehicle's violent vibration and bumpiness reduce the stability of the signal acquisition and even introduce unknown interference signals; (2) the measured signals can be strongly reduced by shelters due to complex terrain, such as tunnels, bridges, signposts, and mountains (usually such measurements have to be filtered out); and (3) the observations in practice are also controlled by various factors, e.g. variable weather, hypoxic environment in the plateau, geospatial signal loss, and problems with the power supply. Although there are challenges in measuring NO2 and HCHO concentrations by mobile MAX-DOAS over the Tibetan Plateau, they are useful for studies on the spatio-temporal evolution of the atmospheric composition in the background atmosphere, validation and improvement of satellite products over mountain terrain, and evaluation of the simulation results of atmospheric chemistry models over the Tibetan Plateau.
We made the mobile MAX-DOAS measurements in July 2021 over the plateau terrain for the first time. In this study, the primary objective is to analyse the spectra of scattered sunlight collected in the Three Rivers' Source region over the Tibetan Plateau, to obtain the data sets of tropospheric NO2 and HCHO vertical column densities (VCDs) in the background atmosphere at high altitudes, and to investigate the abundances and spatio-temporal variations of tropospheric NO2 and HCHO VCDs during the field campaign. Large effort was made on the spectral analysis and data filtering to obtain reliable tropospheric NO2 and HCHO VCDs because of the very weak spectral absorptions of the respective trace gases in the background atmosphere at high altitude, as well as the influences of shelters and the vehicle's vibration and bumpiness along the driving routes. In Sect. 2, we describe the field experiment in July 2021 over the Tibetan Plateau, including the observation vehicle, MAX-DOAS instrument, experiment region, and deployment strategies. Section 3 introduces the spectral analysis, as well as the calculation and filtering of the NO2 and HCHO VCDs. In Sect. 4, we present the abundances and the spatio-temporal variation of the tropospheric NO2 and HCHO VCDs during the field campaign, as well as the comparison with TROPOMI products. A summary and conclusions are given in Sect. 5.
2.1 Description of vehicle and instrumentation
A mobile vehicle has been designed and assembled for measurements of atmospheric composition over the Tibetan Plateau (Fig. 1a). The mobile vehicle has been operated by the Chinese Academy of Meteorological Sciences (CAMS) since February 2021. The outside parts of the instrumentation are fixed on the roof of the vehicle, which is about 3.5 m above the ground. The outside parts of the instrumentation contain the sensors for spatial position (longitude, latitude, altitude) and attitude (yaw, pitch, and roll angles) of the mobile vehicle. The units of the system control, data collection, screen display, and uninterruptible power supply (UPS) are mounted in the interior of the mobile vehicle. The UPS's battery pack, recharged after the mobile vehicle reaches the destination of the observation route, can offer operation time of around 16 h with a power of 2000 W. All instrumentation has been specially reinforced to allow the mobile vehicle to travel over the difficult road conditions of the Tibetan Plateau. The mobile vehicle usually runs at a speed of ∼ 60 km h−1 for motorways and ∼ 40 km h−1 for ordinary roads, respectively, during our field experiment.
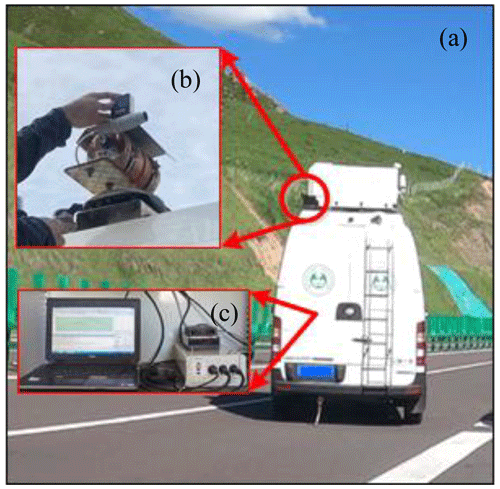
Figure 1(a) Mobile observation vehicle for atmospheric composition and meteorological parameters. Two parts of the Tube MAX-DOAS instrument are installed (b) on the rear of the vehicle's roof and (c) inside the vehicle, respectively.
For the field campaign of mobile observations of the atmospheric environment over the Tibetan Plateau, the aforementioned vehicle was equipped with an instrument called Tube MAX-DOAS (Donner, 2016; Cheng et al., 2021), developed by the Max Planck Institute for Chemistry (MPIC), Mainz, Germany. The Tube MAX-DOAS instrument contained two parts, one outside (Fig. 1b) and another inside (Fig. 1c) the vehicle, respectively. (1) The outside part was fixed on the rear of the vehicle's roof and is mainly composed of the telescope, optical fibre, stepper motor, tubular shell, and protective cover. The telescope, pointing to the back of the vehicle, rotated in the vertical plane to achieve the measurement at seven different elevation angles (3, 6, 10, 15, 20, 30, 90∘ relative to the mobile vehicle) driven by the stepper motor. The scattered sunlight was collected by the telescope and transferred to a spectrograph inside the vehicle via the optical fibre. (2) The inside part was made up of the spectrograph, data collection unit, temperature control unit, and a laptop which controls the instrument operation and data collection. For each elevation angle, the Tube MAX-DOAS instrument collected one spectrum at a stable detector temperature of 15 ± 0.1∘ with the integration time of ∼ 1 min. The AvaSpec-ULS2048x64-USB2 spectrograph was built by the AVANTES company and covered the wavelength range of 300–466 nm with ∼ 0.6 nm spectral resolution. The Tube MAX-DOAS instrument not only automatically collected the scattered sunlight spectra for the cyclic elevation angle sequences during daytime but also recorded spectra of dark current (DC) and electronic offset (OS) at night for correcting the daytime spectra of scattered sunlight. The laptop coordinated the operation of each module during the measurement procedure. The MPIC Tube MAX-DOAS system has been successfully applied to the ground-based observations of atmospheric composition at the Golmud station over the northern Tibetan Plateau (Cheng et al., 2021).
2.2 Description of the measurement location and deployment strategies
The mobile field observation campaign was performed over the Three Rivers' Source region on the northeast of the Tibetan Plateau in western China (Fig. 2). The main vegetation types are alpine steppe and meadows in the region along the observation route, belonging to a unique and typical alpine ecosystem. The main landform is the mountain plain. The Three Rivers' Source region has a typical plateau continental climate, characterised by a large diurnal temperature difference, long sunshine time, and strong solar radiation. There are also rapid spatial and temporal variations of the local climate over the Three Rivers' Source region. Yak and sheep grazing in summer is the main industry over the Three Rivers' Source region, isolated from industrial and population centres.
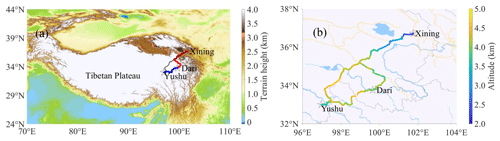
Figure 2Driving routes of the mobile observation vehicle. The driving routes are added to (a) the terrain height map over the Tibetan Plateau (red, blue and black lines) and (b) the street map (https://map.baidu.com/, last access: 16 June 2022) in the experiment region as an overlay, respectively. The altitudes along the driving routes are marked by dotted coloured curves in (b). Light blue lines and areas in (b) indicate rivers and lakes.
In order to reveal the background abundance and spatio-temporal variation of the atmospheric composition over the Three Rivers' Source region, we took various factors into consideration during the design of the deployment strategies, such as the regional representativeness of the driving routes, the technical requirement of the passive MAX-DOAS measurement, the sunlight shelter and the bumpy conditions along the driving route, the reliable electric power safeguard, and first aid for sudden altitude sickness. Finally, the mobile MAX-DOAS field experiment was carried out in the southeast of Qinghai Province, China (Fig. 2b). The driving routes traverse the Yangtze River and the Yellow River and are close to the Lancang River. It took 3 d for one circling journey. Four circling journeys were made during the mobile MAX-DOAS field experiment period in July 2021 (Table 1). We drove from the meteorological bureau of the city of Xining, the capital of Qinghai Province, to the meteorological bureau of Dari County of the Guoluo Tibetan Autonomous Prefecture, southeastern Qinghai Province, on the first day of each circling journey (Fig. 2). We travelled from the meteorological bureau of Dari County to the meteorological bureau of the Yushu Tibetan Autonomous Prefecture on the middle day (Fig. 2). We returned to Xining city from the Yushu Tibetan Autonomous Prefecture on the third day (Fig. 2). Hereafter, the three segments of the closed-loop journey are referred to as XD, DY, and YX, respectively. The durations were about 12, 8, and 13 h for the XD, DY, and YX segments, respectively. Most of the driving routes are motorways, except parts of the national roads in the YX segment. More sunlight shelters occurred in the XD segment because of the tunnels, bridges, signposts, and mountains. The observed MAX-DOAS data were saved on the laptop and backed up when arriving at the terminus of each segment of the journey. In addition to troubleshooting by field observers, our MAX-DOAS team also provided the technical support via remote wireless network during the campaign.
3.1 Spectral analysis
Based on the Beer–Lambert law, the column densities of trace gases can be retrieved from the scattered sunlight spectra by the widely used method of differential optical absorption spectroscopy (DOAS; Platt and Stutz, 2008). The basic idea of DOAS is to decompose the atmospheric spectral extinction into two terms, i.e. terms with slow spectral variation (such as atmospheric scattering) and fast variation (mainly trace-gas absorptions) with wavelength. The slant column density (SCD) of a trace gas is defined as its concentration integrated along the effective light path (Cheng et al., 2019). The total (from the instrument to the top of atmosphere) SCD can be split into two parts, i.e. so-called tropospheric SCD (SCDTrop) and stratospheric SCD (SCDStra). For species concentrated in the troposphere or for light traversing the same path in the stratosphere for different elevation angles (α), the SCDStra can be neglected or cancels out, respectively, which means SCD (Ma et al., 2013). In the practice of the MAX-DOAS spectral analysis, a Fraunhofer reference spectrum (FRS) needs to be selected to correct the strong solar Fraunhofer lines. Thus, the result of the spectral analysis is the so-called differential slant column density (DSCD) of the target species (such as NO2 and HCHO in this study), which represents the difference in trace-gas absorption between the measured atmospheric spectrum and the FRS (Hönninger et al., 2004). There are two schemes for the FRS selection from measured spectra (Wang et al., 2018): one is using a fixed spectrum (hereafter named “fixed FRS”), usually at the 90∘ elevation angle during noon to minimise the tropospheric and stratospheric contributions, for all measured spectra; the other is using sequential spectra (hereafter named “sequential FRS”), which are defined as the time-interpolated spectra between two zenith spectra measured before and after the measurement time of the current off-zenith elevation angle. Due to more similar atmospheric conditions and instrument properties between a specific measured spectrum and the corresponding sequential FRS, higher signal-to-noise ratios and smaller fitting errors are achieved by using a sequential FRS rather than a fixed FRS. Figure 3 shows the root mean square (rms) of the spectral-fitting residuals using a fixed FRS and a sequential FRS for NO2 and HCHO, respectively. It is clear that the rms medians are smaller for a sequential FRS than those for a fixed FRS. Thus, we prefer to use the sequential FRS for the mobile MAX-DOAS measurements in this study. For NO2, we can retrieve the DSCD not only in the ultraviolet (UV) region (351–390 nm) but also in the visible region (400–434 nm; Cheng et al., 2022, 2019). Figure 4 compares the NO2 DSCDs and the rms's of the spectral-fitting residuals for using either the visible or UV spectral interval. The overall trends of the NO2 DSCDs are consistent between both spectral intervals, with a correlation coefficient of R=0.75, but the averaged rms's of the spectral-fitting residuals in the visible wavelength region, i.e. (6.26 ± 6.92) × 10−4, are smaller than those in the UV wavelength interval, i.e. (7.62 ± 9.17) × 10−4. The final settings of the NO2 and HCHO spectral retrieval parameters, such as cross sections of the target and interference species, ring spectra, polynomial degree, and intensity offset, are similar to those in previous studies (Cheng et al., 2022, 2019) – see Table 2. The spectral analysis, including DC and OS corrections of the measurement spectra and the spectral calibration of the FRS, was implemented by the QDOAS software based on a non-linear least-squares fitting method developed by the Royal Belgian Institute for Space Aeronomy (BIRA-IASB; Danckaert et al., 2017). Figure 5 shows an example of the spectral fitting for the NO2 and HCHO DSCDs from a spectrum measured at the elevation angle of 15∘ at 11:02 BJT on 18 July 2021 (SZA = 34.11∘). In the post-processing of NO2 and HCHO DSCDs, we applied the following filters: rms < 0.005; offset (constant) should be between ±0.03; SZA < 80∘. These filters were selected because they provide a good balance between quality of the results and not skipping too many data. These filters almost filtered out all “bad measurements”, which were caused by sunlight shelters and bumpy conditions. Finally, relative to measurements with SZA < 90∘, the percentages of remaining DSCD data were 69 % for NO2 and 74 % for HCHO, respectively. During DOAS measurements, the instrument detection limit can be conveniently estimated by the spectral-fit errors (Cheng et al., 2021; Coburn et al., 2011; Stutz and Platt, 1996). The instrument detection limits of NO2 and HCHO DSCDs were defined as twice the medians of the spectral-fit errors in this study, i.e. 0.68×1015 and 2.11×1015 molec. cm−2 at 15∘ elevation angle, respectively. According to the DSCD detection limits divided by the differential air mass factor (DAMF) for a 15∘ elevation angle, the VCD detection limits were estimated to be about 0.24 × 1015 molec. cm−2 for NO2 and 0.74 × 1015 molec. cm−2 for HCHO, respectively. Note that, for individual measurements, the VCD detection limits might be lower or higher by about ±30 % because of the uncertainties of the geometric approximation (up to about 20 %) and the effect of varying ground slope (also up to about 20 %). There are 17 % and 15 % of the retrieved NO2 and HCHO DSCDs below the detection limits, respectively. Based on the spectral-fit errors, we can also calculate the relative errors for each NO2 and HCHO DSCD. Then the mean relative errors of NO2 and HCHO DSCDs were about 21 % and 12 % at a 15∘ elevation angle, respectively.
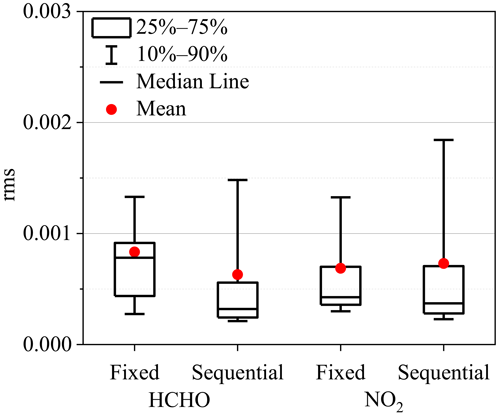
Figure 3Statistics of the root mean square (rms) of the NO2 and HCHO spectral-fitting residuals using a sequential FRS or fixed FRS (for rms < 0.005 and SZA < 80∘) during the field campaign. Lower (upper) error bars and boxes are the 10th (90th) and 25th (75th) percentiles, respectively. Lines inside the boxes and dots denote the medians and the mean values.
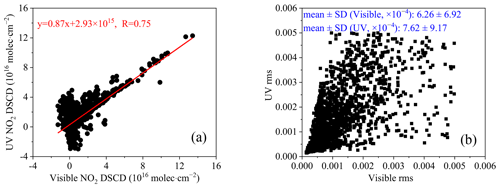
Figure 4Comparison of NO2 spectral-fitting results using the visible and UV wavelength intervals (for rms < 0.005 and SZA < 80∘) during the field campaign. (a) Linear fit of corresponding NO2 DSCDs between visible and UV spectral intervals. (b) Corresponding NO2 rms between visible and UV spectral intervals. The red lines denote the results of the regression analyses, and the corresponding equations and correlation coefficients are displayed in (a). The numbers in (b) indicate the mean ± standard deviation (SD) in the visible and UV spectral intervals.
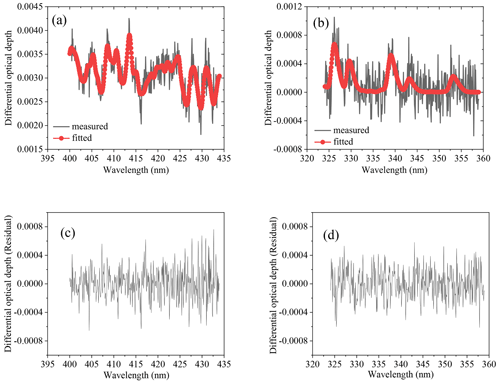
Figure 5Examples of DOAS spectral analyses for NO2 and HCHO. Black curves and red curves with dots indicate the measured and fitted differential optical depth for (a) NO2 and (b) HCHO, respectively. The NO2 and HCHO DSCDs are 5.27 × 1015 and 9.36 × 1015 molec. cm−2, respectively. The rms's of the spectral-fitting residuals between measured and fitted spectra are 2.17 × 10−4 for (c) NO2 and 2.09 × 10−4 for (d) HCHO, respectively.
3.2 NO2 and HCHO VCDs
Based on the aforementioned filtered NO2 and HCHO DSCDs retrieved from the spectra, we need to firstly obtain the tropospheric DSCDs at the elevation angle α (i.e. ), which are used to calculate the NO2 and HCHO vertical column densities (VCDs) in the troposphere. In the situation of fixed FRS, the DSCDTrop values are produced by the DSCDs of off-zenith viewing direction minus those at a 90∘ elevation angle of the same elevation sequence. In the case of sequential FRS in this study, the DSCDs from spectral inversion can be regarded as DSCDTrop (Hönninger et al., 2004).
The SCDs (or DSCDs) depend on the concentration profile of the target species, effective light path length, measurement geometry, and solar position. Using the air mass factor (AMF), the SCDs (or DSCDs) can be converted to the VCDs, which are independent of the light path and the observation geometry and are thus convenient for comparison between different measurements. The tropospheric AMF at the elevation angle α (AMFα,Trop) is given by the ratio of the SCD to VCD in the troposphere:
If ,
We define the DAMFα,Trop as the tropospheric differential AMF, i.e.
By Eq. (1) minus Eq. (2), VCDTrop can be deduced:
where the AMF can be simulated by an atmospheric radiative transfer model or estimated by the method of geometric approximation. The former method is more accurate but requires information on various input parameters, such as the profiles of trace gas and aerosol, which are usually not known. The latter method is simpler and assumes trace gases are uniformly distributed in the lower troposphere. Due to the lack of necessary data over the Tibetan Plateau to simulate the correct NO2 and HCHO AMFs, we adopted the geometric approximation method in this study. Here, it should be noted that the errors caused by the geometric approximation method are much smaller for measurements at high altitudes because the scattering probability is much smaller compared to measurements at sea level. Thus, the direct-viewing-path length becomes longer and is in better agreement with the assumptions of the geometric approximation method. We explored the applicability of the geometric approximation method by radiative transfer simulations with the full spherical Monte Carlo radiative transfer model, MCARTIM (Deutschmann et al., 2011; see Sect. S1 in the Supplement). The main findings are as follows: (1) the typical errors of the geometric approximation are < 20 % for NO2 and HCHO (Fig. S1). (2) The retrieved HCHO VCDs using the geometric approximation will represent well the part of the HCHO profile located below 2 km, but the use of the geometric approximation systematically underestimates (∼ 60 %) the background HCHO above 2 km (Figs. S2, S3). However, from model simulations over the Tibetan Plateau (Fig. S2; Ma et al., 2019), we find that the corresponding vertical HCHO column density is rather small: about 1.3 × 1015 molec. cm−2. Thus, the retrieved HCHO VCDs underestimate the true total VCD by about 0.6 × 1.3 × 1015 molec. cm molec. cm−2. (3) In the presence of clouds, the sensitivity for trace gases below the clouds is slightly enhanced compared to the geometric approximation, while it is strongly reduced for trace gases above the clouds (Fig. S4). The AMFα,Trop in the condition of geometric approximation in a polluted environment can be expressed as follows:
Therefore, Eq. (4) becomes
Ideally, the elevation angles should be corrected by the attitude angles of the mobile vehicle when applying the geometric approximation. However, the partial system of the attitude angles of the mobile vehicle did not work well, which may be connected with the special environment of the Tibetan Plateau (such as low atmospheric pressure) and the bumpiness of the mobile observation platform (leading to instabilities of the data collection). Thus, we use the uncorrected elevation angles during the conversion of DSCD to VCD in Eq. (6). Of course, the uncorrected elevation angles will cause some errors if the mobile observation vehicle is not on a horizontal surface, but these errors are typically small for the larger elevation angles (for example, 15, 20, and 30∘). Based on the mobile platform attitude angles, the elevation angle error is estimated to be about 2.3∘. The corresponding error of an individual measurement will be up to about 21 %, but over the full loop, these errors will at least partially cancel. To further judge how good the geometric approximation is, the resulting VCDs derived for different elevation angles have been compared (Brinksma et al., 2008). Table 3 shows the NO2 (HCHO) VCDs between the three elevation angles (15, 20, and 30∘). The VCDs are rather consistent at the three elevation angles, with correlation coefficients of R=0.91–0.95 for NO2 and R=0.66–0.80 for HCHO, respectively (Table 3). This implies that the geometric approximation method is self-consistent. The standard deviation of the NO2 (HCHO) VCDs is small at 15∘ elevation angles (Table 3), implying the high reliability of VCDs at a 15∘ elevation angle (VCD). Therefore, to compromise between accuracy of the geometric approximation and signal to noise, the VCD were treated as the reliable results based on a selection criterion (for NO2, the absolute difference of VCDs between 15 and 20∘ is <1 × 1015 molec. cm−2, or the relative difference is < 5 %; for HCHO, the absolute difference of VCDs between 15 and 20∘ is < 2 × 1015 molec. cm−2, or the relative difference is < 5 %). The filtered NO2 and HCHO VCD values during the mobile measurement period were kept as the final results to explore the background abundance and spatio-temporal variation of NO2 and HCHO over the Three Rivers' Source region of the Tibetan Plateau. It took about 8 min to obtain measurements at two adjacent 15∘ elevation angles. Therefore, the corresponding spatial resolution was approximately 8 km at a mobile-vehicle speed of ∼ 60 km h−1. Assuming that the trace gas is located in the lowest 1000 m above the surface, we can also estimate the horizontal extent of the line of sight through that layer. For measurements at a 15∘ elevation angle, this extent is about 4 km.
From our experience during the measurements, we also suggest that the telescope scans at 15, 20, and 90∘ elevation angles in future mobile MAX-DOAS measurements of the background atmosphere over mountain terrain. There are at least two reasons: (1) the relatively large elevation angles are less influenced by the road tilt and obstructions; (2) the measurements at 15 and 20∘ elevation angles still have an enhanced sensitivity to tropospheric trace gases (increase of sensitivity compared to 90∘ elevation angle is by about a factor of 3.8 and 2.9, respectively).
4.1 Abundance
The background levels of the filtered final NO2 and HCHO VCDs can be estimated by the maximum-frequency method (Cheng et al., 2017). According to the Lorentz fitted curves of the relative frequency distribution of the NO2 and HCHO VCDs during the field campaign (Fig. 6a), the background levels were 0.40 ± 1.13 × 1015 molec. cm−2 for NO2 and 2.27 ± 1.66 × 1015 molec. cm−2 for HCHO in summer on the northeast side of the Tibetan Plateau, wherein the uncertainties of the background levels were estimated by the standard deviations of NO2 and HCHO VCDs. The background levels are smaller than those observed in summer 2018 at the Qomolangma Atmospheric and Environmental Observation and Research Station of the Chinese Academy of Sciences, located in the south-central Tibetan Plateau (medians of 0.80 × 1015 molec. cm−2 for NO2 and 3.13 × 1015 molec. cm−2 for HCHO, respectively; Xing et al., 2021). To explore the dependence of the NO2 and HCHO VCDs on the route altitude (in the range of 2280–4830 m), we divided the mobile-route altitudes into vertical bins with intervals of 500 m. Figure 6b shows the means, medians, and standard deviations of the NO2 and HCHO VCDs in each vertical grid cell. There are generally decreasing trends with increasing altitude. This is consistent with our knowledge of the natural background atmosphere, i.e. the higher the altitude, the lower the air density. Different from the nearly constant decreasing rate of the HCHO VCDs with the route altitude, there are at least two segments with significantly different decreasing rates above and below 2750 m altitude. The NO2 VCDs in the 2000–2500 m grid cell (8.17 × 1015 molec. cm−2) were substantially larger because the mobile route was close to the city of Xining (about 2260 m altitude), where there are stronger anthropogenic emission sources of air pollutants, such as increased urban transport emissions leading to higher NO2 levels. The NO2 VCDs were quite low at altitudes above 3500 m, partly related to almost no human activities at this altitude. Due to very limited emissions of anthropogenic VOCs over the Tibetan Plateau, the changes of the HCHO VCDs with altitude were likely to be primarily connected with the natural process, such as the oxidation of methane and non-methane volatile organic compounds (Stavrakou et al., 2009). Combining the hourly surface air pressure and temperature at 2 m above the land surface with the 0.25∘ × 0.25∘ resolution from ERA5, the profiles of NO2 and HCHO mixing ratios were also derived from the corresponding mean and median VCDs along driving routes, respectively (Fig. S5). As a whole, the measurements (except close to the cities) at the higher altitudes in summer are able to reflect the background atmosphere with rather low NO2 and HCHO levels over the Three Rivers' Source region.
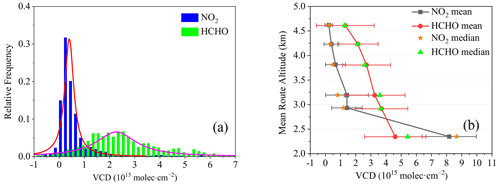
Figure 6Overall characteristics of NO2 and HCHO VCDs during the field campaign. (a) Frequency distributions of NO2 (blue column) and HCHO (green column) VCDs, as well as their Lorentz distribution curves for NO2 (red curve) and HCHO (magenta curve), respectively. (b) Dependence of the NO2 and HCHO VCDs on mean altitude of driving route from 2000 to 5000 m at vertical intervals of 500 m. The black (red) lines with squares (dots), stars (triangles), and error bars denote the means, medians, and standard deviations of the NO2 (HCHO) VCDs for each altitude range.
4.2 Spatio-temporal variation
4.2.1 NO2
The day-to-day variations of NO2 VCDs are similar between different circling journeys, characterised by the larger means and 90th percentiles on the first and the third days (i.e. on the days of the XD and YX driving routes) and correspondingly lower values on the second day (i.e. on the day of the DY driving route) of each circling journey (Fig. 7a). The NO2 means are always larger than the medians on each day, especially in the situation of the XD driving route, partly because the driving route covers small areas with very high NO2 abundances, such as Xining city, and large background areas with relatively low NO2 abundances. For the same driving route of the four circling journeys, the daily NO2 levels are close to each other, with the NO2 medians in the range of 0.19–0.63 × 1015 molec. cm−2 during the field campaign.
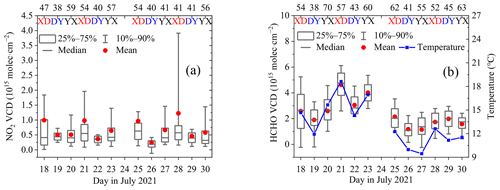
Figure 7Day-to-day variations of the daily averaged (a) NO2 and (b) HCHO VCDs over the mobile observation routes (XD, DY, YX). Lower (upper) error bars and boxes are the 10th (90th) and 25th (75th) percentiles of the data. Lines inside the boxes and red dots denote the medians and the mean values, respectively. The integrated sampling numbers for specific day are labelled at the top axis. The blue curves with squares in (b) denote the daily air temperature at 2 m above the land surface.
Figure 8 shows the spatial distributions of the tropospheric NO2 VCDs along the XD, DY, and YX driving routes in July 2021. For the same segment of four circling journeys (i.e. XD, DY, or YX), the tropospheric NO2 VCDs present a nearly consistent spatial distribution. It is also clear that the tropospheric NO2 VCDs were elevated when the mobile observation vehicle passed through counties or cities, such as Xining and Yushu. This can be attributed to increased anthropogenic activities in cities or counties, such as traffic and residential emissions. There are significantly larger NO2 VCDs on the driving routes of southeastern Qinghai Lake, which is a famous tourist destination. Moreover, as one of the arterial roads to Tibet, there are many diesel vehicles passing through the basin of Qinghai Lake via national motorways surrounding the lake. The touring buses or cars, as well as the cargo transport vehicles, could lead to the higher NO2 abundances in summer around Qinghai Lake. According to previous studies at the northwest section of Qinghai Lake shore in October of 2010 and 2011, the emissions from diesel vehicles around Qinghai Lake were likely the main source of nitrogen oxides (NOx; Wang et al., 2015). The enhanced NO2 levels could even be found at the motorway junction (such as the location of 35.20∘ N, 98.97∘ E) and the tunnel exit (such as the location of 34.92∘ N, 99.40∘ E; note that the telescope of the MAX-DOAS pointed to the reverse of the driving direction; Fig. 8a1, d1). This situation would not appear once traffic flow was lower at these special locations (Fig. 8b1, c1). The NO2 spatial distributions over the main area of the Three Rivers' Source, such as around the counties of Dari, Shiqu, Chenduo, and Maduo during the DY driving route and the first half of the YX driving route, were relatively uniform with very low levels (<1 × 1015 molec. cm−2). Previous investigations of the tropospheric ozone chemical budget, simulated and constrained by measured NO2 concentration at the Waliguan background station located in the northeastern Tibetan Plateau, showed that the NOx levels play a vital role in the net sign of ozone production from formation and loss reaction for the tropospheric background atmosphere (Ma et al., 2002, 2020; Xue et al., 2013). Therefore, with more and more anthropogenic activities, the effects of increasing NO2 levels on the photochemistry and oxidation capacity of the background atmosphere should be paid more attention to better build an ecological civilisation over the remote Three Rivers' Source region in the future.
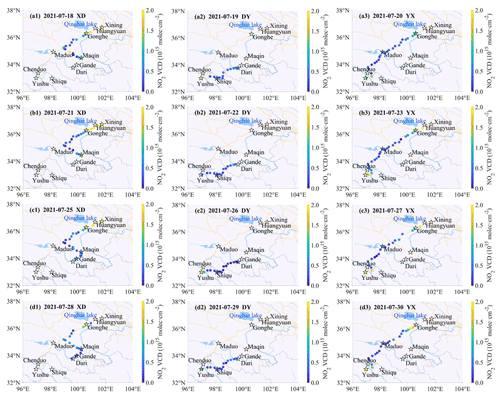
Figure 8Spatial distributions of gridded NO2 VCDs with 0.25∘ × 0.25∘ resolution. The observed NO2 VCDs in each spatial grid cell are averaged for three segments (1, 2, 3) of four circling journeys (a, b, c, d). The main cities and counties on the driving routes are marked by the black stars. On the background map, the light-blue lines and areas represent rivers and lakes (such as Qinghai Lake), the yellow lines denote the roads, and the grey lines indicate the administrative boundaries.
The available time period, confined by the sunshine duration and the distance of the driving routes, is the shortest for the DY driving route. The diurnal cycle of the NO2 VCD means or medians presents high values in the morning and evening and shows lower levels of ∼ 0.38 × 1015 molec. cm−2 from 12:00 to 17:00 BJT (Fig. 9a). The means of the NO2 VCD are also significantly higher than the corresponding medians before 11:00 BJT, with larger standard deviations. The NO2 diurnal variation patterns of the XD, DY, and YX driving routes are different, although the diurnal patterns are rather consistent for different days of the same driving route (Fig. 9b–d). The NO2 VCDs sharply decreased in the morning during the XD driving route, with larger standard deviations around 16:00 BJT, when the mobile observation vehicle was close to the toll station. For the DY driving route, the NO2 VCDs stayed at the lower level and then slightly increased in the late afternoon. In the situation of the YX driving route, the diurnal pattern of NO2 VCDs was a symmetric “U” shape. It should be noted that the mobile observation vehicle reached the destination of the YX driving route around 22:00 BJT, and the lacking NO2 VCDs were due to SZA > 80∘ after 20:00 BJT. The amplitudes of the NO2 diurnal variation and the maxima NO2 levels among different driving routes decreased in the order of the segments XD, YX, and DY. Previous studies at the background station of lower altitude showed that the NO2 diurnal variation could be affected by the higher photolysis rate owing to stronger solar irradiance at noon and for a site location far away from emission sources (Cheng et al., 2019). We also checked whether the enhanced NO2 VCDs in the morning and evening might be an artefact caused by the effect of stratospheric NO2 on the derived tropospheric NO2 VCD. In our data analysis (see Sect. 3.1), it is assumed that the stratospheric NO2 absorption is independent of the elevation angle. While this is not exactly true, it is a valid assumption for typical measurement situations in polluted or slightly polluted environments. If, however, the tropospheric NO2 absorption is very weak, the remaining stratospheric influence might be substantial. We tested this potential influence of the stratospheric NO2 absorption on the retrieved tropospheric NO2 VCD for our measurements by performing radiative transfer simulations using a stratospheric NO2 profile with a stratospheric NO2 VCD of 4 × 1015 molec. cm−2. As a result, we found that, for SZA < 80∘, the introduced NO2 DSCD for an elevation angle of 15∘ is < 1 × 1015 molec. cm−2 (see Fig. S6), thus leading to a maximum artificial NO2 VCD of 3.5 × 1014 molec. cm−2. Moreover, for SZA < 80∘, the artificial NO2 VCD shows almost no SZA dependence. Thus, the potential influence of the stratospheric NO2 absorption cannot explain the observed diurnal cycle of the tropospheric NO2 VCD. From these findings, we conclude that the NO2 diurnal variations were primarily caused by enhanced pollution in the morning and evening, when the mobile observation vehicle was located in or close to the cities or county town; i.e. the NO2 diurnal patterns reflected the differences of the NO2 spatial distribution. An additional effect on the diurnal variation is probably caused by the enhanced NO2 photolysis around noon.
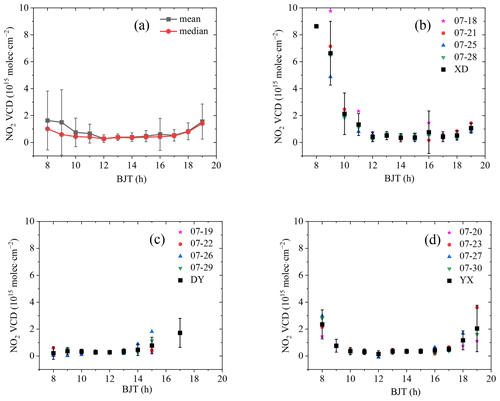
Figure 9Diurnal variations of the NO2 VCDs over the mobile observation routes. (a) Diurnal variations of the overall means (black curve with squares), medians (red curves with dots), and standard deviations (error bars) of the NO2 VCDs. (b) Diurnal variations of the mean NO2 VCDs on selected days (18, 21, 25, and 28 July 2021), as well as the means and standard deviations of the NO2 VCDs on the XD driving route. (c, d) Same as (b) but for the DY and YX driving routes during the field campaign.
4.2.2 HCHO
The means and medians of the daily HCHO VCDs are basically consistent on all days, with the maximum mean of 4.63 × 1015 molec. cm−2 on 21 July 2021 and the minimum mean of 1.15 × 1015 molec. cm−2 on 27 July 2021 (Fig. 7b). There are obvious differences in the levels of HCHO VCDs between the different circling journeys. The higher and lower HCHO VCDs appeared during the second circling journey (i.e. 21–23 July 2021) and the third circling journey (i.e. 25–27 July 2021), respectively. HCHO has large natural vegetation sources, with the emission strength depending strongly on weather conditions such as temperature and solar radiation at the Earth's surface (Borovski et al., 2014). We looked at air temperature at 2 m above the land surface and the downward solar radiation at the surface (SSRD), which are derived from hourly ERA5 reanalysis data with 0.25∘ × 0.25∘ resolution. According to the ERA5 grid cell and hour to which each HCHO measurement belongs, the air temperature and SSRD are extracted and then averaged for each day (Fig. 10a). It is shown that the daily variations between air temperature and HCHO VCDs are highly correlated, with the correlation coefficient of R=0.95 (Figs. 7b, 10b). It is probable that higher temperatures are connected with more VOCs emitted by vegetation, leading to higher HCHO VCDs. The daily HCHO VCDs are also related to surface solar radiation but with a smaller correlation coefficient of R=0.27 (Fig. 10b), which is probably caused by the higher variability of local solar radiation over the Tibetan Plateau compared to the temperature. Therefore, the remarkable HCHO daily variations are mainly connected with the variable weather over the Tibetan Plateau, which affects the natural emissions of HCHO precursors significantly.
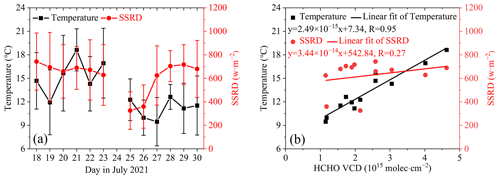
Figure 10Comparison of the HCHO VCDs with other data sets. (a) Day-to-day variations of the mean air temperature at 2 m above the land surface (black curves with squares) and the downward solar radiation at the surface (SSRD; red curves with dots), as well as (b) linear fits between the two parameters and the daily averaged HCHO VCDs over the mobile observation routes. The error bars denote the standard deviations of the air temperature and SSRD in (a). The lines denote the results of the regression analyses, and the corresponding equations and correlation coefficients are displayed in (b).
Figure 11 shows the spatial distributions of the HCHO VCDs during the field campaign in July 2021. For the specific driving routes (XD, DY, or YX), the HCHO spatial distributions were similar on different days. Normally, the HCHO VCDs were larger at the starting points and ending points of the driving routes (if reaching the ending points under the condition of SZA < 80∘), which matched with the larger HCHO values in the morning and evening (Fig. 12). However, the HCHO levels were significantly different at the same location on different days. For example, the HCHO VCDs on the second circling journey (Fig. 11b1–b3) were obviously larger than those on the other three circling journeys, most probably due to higher surface temperatures on the second circling journey (Fig. S7). From the northeast to the southwest in the region of the mobile-observation field experiment, the HCHO VCDs present a decreasing trend. These lower HCHO levels in the main area of Three Rivers' Source reflect the overall conditions of atmospheric HCHO background. The spatial distributions of the HCHO column observed by the OMI satellite from 2009 to 2019 over the Tibetan Plateau also found that the regions with sparse population and fewer human activities were frequently affected by natural factors, such as air temperature and precipitation (Zhang et al., 2021). The elevated HCHO VCDs around Maqin County of the XD driving route were partly related to anthropogenic HCHO emissions, such as biomass burning and fossil fuel combustion (Fig. 11a1, b1, c1, d1; Zhang et al., 2021). Comparing the HCHO VCDs before and after Maduo County on the YX driving route, the former were larger than the latter, corresponding to the jump of the HCHO diurnal variation before and after 13:00 BJT (Fig. 12d). Besides the differences in human activities, the spatial step changes in the HCHO VCDs were also partly connected with the decreasing altitudes on the YX driving route (Fig. 2b).
With respect to the total means and medians of the HCHO VCDs in the range of 1.92–4.36 × 1015 molec. cm−2 (Fig. 12a), their diurnal variations are rather consistent throughout the whole day. They decrease slightly before 10:00 BJT and increase after 18:00 BJT, and they also have no significant differences in their standard deviations. However, the diurnal variations of the HCHO VCDs are obviously different, both for different days of the same driving route and among different driving routes (Fig. 12b–d). On average, the diurnal pattern of the HCHO VCDs during the XD driving route presents a weak “U” shape, i.e. slightly higher HCHO levels in the morning and evening. For the DY driving route, the total averaged HCHO VCDs almost maintain the level around 2 × 1015 molec. cm−2 before 14:00 BJT and then gradually increase until the end of the DY journey. The diurnal pattern of the HCHO VCDs for the YX driving route presents a “W” shape; i.e. higher HCHO VCDs occur around 13:00 BJT, in the morning, and in the evening. The variable diurnal cycles of HCHO VCDs were also found by ship-based MAX-DOAS measurements over the middle and lower Yangtze River in winter, where both primary sources and photochemical secondary formation have large influences (Hong et al., 2018). Even at the starting and ending points of the driving route, there were almost no strong HCHO primary sources caused by anthropogenic activities over the Three Rivers' Source region. Thus, we infer that the variable diurnal patterns of HCHO were mainly connected with the secondary photochemical formation of active VOCs emitted from vegetation (Mu et al., 2007). Meanwhile, due to the varying local microclimates over the Tibetan Plateau, as well as the different types and amounts of vegetation at different altitudes, the diurnal variations of secondary HCHO production are quite changeable and closely related to the specific property of a location. More comprehensive observations are needed over the Tibetan Plateau in the future to deeply understand the HCHO spatio-temporal evolution.
4.3 Comparison with TROPOMI observations
The TROPOspheric Monitoring Instrument (TROPOMI) is the sole payload on the Copernicus Sentinel-5 Precursor (Sentinel-5P or S5P) satellite, which provides measurements of multiple atmospheric trace species, including NO2 and HCHO, at high spatial and temporal resolutions (Veefkind et al., 2012). The S5P reference orbit is a near-polar sun-synchronous orbit with a mean local solar time of 13:30 at the ascending node. TROPOMI covers the wavelength ranges of ultraviolet–visible (270–495 nm), near infrared (675–775 nm), and shortwave infrared (2305–2385 nm) with a 108∘ field of view in nadir view. TROPOMI achieves daily global coverage with a spatial resolution of 5.5 × 3.5 km2 at nadir since the along-track pixel size reduction on 6 August 2019. The NO2 retrieval consists of a three-step procedure: (1) the total NO2 SCDs are retrieved from the Level 1b spectra measured by TROPOMI using the DOAS method; (2) the total NO2 SCDs are separated into stratospheric SCDs and tropospheric SCDs on the basis of information coming from a data assimilation system; (3) the tropospheric NO2 SCDs are converted into VCDs through a lookup table of tropospheric AMFs. The first and third steps also apply to HCHO, but in addition, a bias of the HCHO SCDs needs to be corrected before the conversion of the HCHO SCDs to VCDs. In this study, we use the TROPOMI Level 2 NO2 and HCHO products (i.e. S5P_L2__ NO2____HiR and S5P_L2__HCHO___HiR) downloaded from the NASA Goddard Earth Sciences Data and Information Services Center (GES-DISC; ESA and KNMI, 2021; ESA and DLR, 2020). For comparison between the mobile MAX-DOAS and TROPOMI observations, their NO2 and HCHO VCDs are gridded into 0.25∘ × 0.25∘ cells (Figs. 13, 14). The reason for averaging two data sets into a 0.25∘ × 0.25∘ grid is to balance the spatial resolution and the number of observed NO2 and HCHO VCDs at a specific grid cell. The TROPOMI relative precisions in the “ΔT1.5” situation (explained below) are estimated to be 72 % and 113 % for tropospheric NO2 and HCHO VCDs, derived from the products of S5P_L2__NO2____HiR and S5P_L2__HCHO___HiR, respectively.
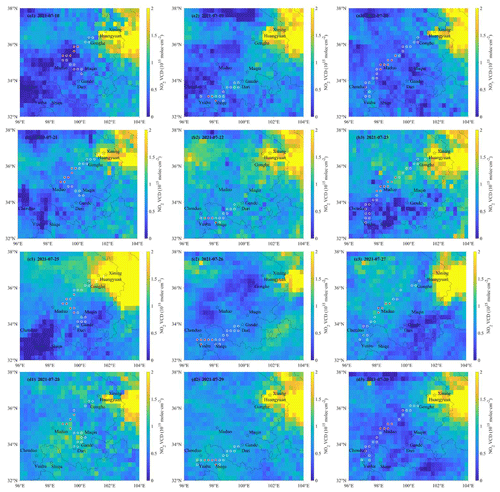
Figure 13Spatial distributions of the tropospheric NO2 VCDs observed by TROPOMI on each day of the field campaign. The TROPOMI S5P_L2__NO2____HiR product has been gridded to 0.25∘ × 0.25∘ cells. The main cities and counties on the driving routes of the field campaign are marked by the black stars. The black curves indicate the administrative boundaries. The white circles and red plus symbols show the grid cell where the data of both TROPOMI and MAX-DOAS are available on the same day or within a 1.5 h time difference, respectively.
Figure 13 shows the spatial distributions of the tropospheric gridded NO2 VCDs from TROPOMI on each day of the field campaign. The spatial distributions of the tropospheric NO2 VCDs are basically consistent on different days; i.e. higher values are found in the northeast, and lower values are found in the southwest. Similarly as for the mobile MAX-DOAS, the TROPOMI NO2 VCDs are larger around Xining city than in the main area of the Three Rivers' Source region. However, the elevated trends of the tropospheric NO2 VCDs around the counties, which are clearly observed by the mobile MAX-DOAS, are nearly not captured by TROPOMI. To validate the fine-scale (0.25∘ × 0.25∘) spatial variability in tropospheric NO2 VCDs, we made a linear regression analysis between both data sets (Fig. 15a). When using all tropospheric NO2 VCDs at the same grid cell on the same day during the field campaign (referred to as “All” in Fig. 15a, corresponding to the white circles in Fig. 13), the consistency is good, with a correlation coefficient of R=0.67 between the two data sets. However, the slope is much lower than unity, indicating that the NO2 VCDs from TROPOMI are systematically lower than those from mobile MAX-DOAS over the polluted areas. Besides the probable underestimation of TROPOMI, the lower TROPOMI NO2 VCDs are also connected with the time differences between the two observation methods at the same grid cell. In contrast, there is almost no correlation of the two data sets if we only use the tropospheric NO2 VCDs within the 1.5 h time difference between mobile MAX-DOAS and TROPOMI at the same grid (referred to as “ΔT1.5” in Fig. 15a, corresponding to the red pluses in Fig. 13). The weak correlation is understandable, because (1) the level and the range variation of the NO2 VCDs are very small in the background atmosphere over the Tibetan Plateau; and (2) the signal-to-noise ratio is reduced due to the measurement errors for both MAX-DOAS and TROPOMI, introduced by the spectral analysis, ground slope, and the applied tropospheric AMF. Comparing the situations of All and ΔT1.5, significant differences in the correlation are connected with the former, including the larger NO2 VCDs close to the cities, inferred by the locations of the grid cell in Fig. 13. For the ΔT1.5 comparison, mostly the low background values are included. These results indicate that the TROPOMI can distinguish the differences in tropospheric NO2 VCDs between city and background atmosphere over the Tibetan Plateau. Relative to the NO2 VCDs by mobile MAX-DOAS during the field campaign, the relative (absolute) differences of the NO2 VCDs by TROPOMI are −12 % (−9.47 × 1013 molec. cm−2) and 40 % (1.77 × 1014 molec. cm−2) for All and ΔT1.5 on average, respectively. The positive bias for ΔT1.5 is probably related to the horizontal NO2 inhomogeneity, caused by mountain terrains over the main area of the Three Rivers' Source. However, without detailed knowledge about the true three-dimensional NO2 distribution, this bias cannot be fully understood in direction and magnitude. As a whole, in contrast to routine TROPOMI validation based on site observations (Verhoelst et al., 2021), the mobile MAX-DOAS observations can serve as a supplement to quantify the impact of the fine-scale NO2 horizontal variability on satellite products.
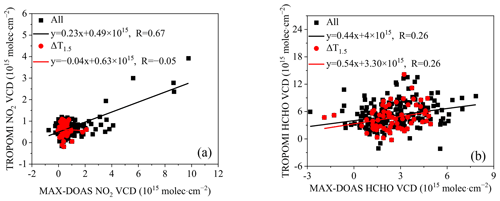
Figure 15Linear fit between the tropospheric (a) NO2 and (b) HCHO VCDs measured by the mobile MAX-DOAS and TROPOMI. The black squares and red dots represent the available VCDs of both data sets at the same grid cell on the same day or within a 1.5 h time difference, respectively. The black (red) lines denote the results of the regression analyses, and the corresponding equations and correlation coefficients are displayed in the panels.
In contrast to NO2, the spatial distributions of the tropospheric gridded HCHO VCDs from TROPOMI are not uniform among different days of the field campaign (Fig. 14). The higher HCHO VCDs appear more in the second circling journey, and the lower HCHO VCDs appear more in the third and fourth circling journeys, consistent with the aforementioned results derived from mobile MAX-DOAS. The HCHO levels around the city of Xining are also not significantly enhanced and are even lower than those in the main area of the Three Rivers' Source region on some days, such as 25 July 2021. We also perform a linear regression analysis of tropospheric HCHO VCDs derived from mobile MAX-DOAS and TROPOMI, respectively. Whether for the All (corresponding to the white circles in Fig. 14) situation or for the ΔT1.5 (corresponding to the red pluses in Fig. 14) situation, the correlation coefficients are the same (R=0.26 in Fig. 15b), indicating that there are no strong anthropogenic HCHO sources along the driving routes, even in the city of Xining. The rather small correlation coefficient between the two data sets is also related to the rather small variability of the HCHO VCDs and the relatively low signal-to-noise ratio of the TROPOMI satellite product in the background atmosphere over the Tibetan Plateau. Comparing the “ΔT1.5” situation between NO2 and HCHO, the correlation of the tropospheric HCHO VCDs is higher than that of NO2, which is probably related to the stronger HCHO daily variations in the background atmosphere influenced by natural factors, such as air temperature and precipitation (Zhang et al., 2021). Similar to the validations of TROPOMI at remote sites by ground-based solar-absorption Fourier transform infrared (FTIR) measurements (Vigouroux et al., 2020), an overestimation of the true HCHO VCD by TROPOMI is also found during the field campaign, with significantly larger relative (absolute) differences of 104 % (2.60 × 1015 molec. cm−2) and 87 % (2.16 × 1015 molec. cm−2) for “All” and “ΔT1.5” on average, respectively (Fig. 15b). This large positive offset of the TROPOMI HCHO VCDs is probably connected with the horizontal HCHO inhomogeneity caused by mountain terrain and varying local microclimates over the Tibetan Plateau. Therefore, although TROPOMI significantly improves the precision of the HCHO observations at short temporal scales and for low HCHO columns (De Smedt et al., 2021), it is still a challenge for satellite instruments to detect the spatio-temporal variations of HCHO over the Tibetan Plateau.
In this study, we performed mobile MAX-DOAS measurements over the Tibetan Plateau in summer (18–30 July) 2021 for the first time. We analysed spectra of scattered sunlight collected in the Three Rivers' Source region over the Tibetan Plateau and obtained the data sets of tropospheric NO2 and HCHO VCDs in the background atmosphere. We further investigated the abundances and spatio-temporal variations of the tropospheric NO2 and HCHO VCDs and validated the TROPOMI satellite products during the field campaign.
We tested the influences of different Fraunhofer reference spectra (FRSs) and different spectral intervals on the spectral retrieval and found that the fitting residuals are smaller when using the sequential FRSs in the NO2 visible-wavelength region for mobile MAX-DOAS measurements in the background atmosphere over mountain terrain. After investigating the optimal filters to eliminate the “bad measurements” caused by sunlight shelters and the vehicle's vibration and bumpiness, the NO2 and HCHO DSCDs were retained with the conditions of (1) rms < 0.005, (2) offset (constant) between ±0.03, and (3) SZA < 80∘. The qualified NO2 and HCHO DSCDs were converted to the corresponding VCDs based on the air mass factor (AMF) estimated by the geometric approximation method. Through comparing the resulting NO2 and HCHO VCDs at three different elevation angles (15, 20, 30∘), the VCD values were further filtered and kept as the final data sets of tropospheric NO2 and HCHO VCDs when absolute and relative VCD differences (ΔVCD) between 15 and 20∘ are < 1015 molec. cm−2 or < 5 % for NO2 and < 2 × 1015 molec. cm−2 or < 5 % for HCHO, respectively.
The background levels ± standard deviations of tropospheric NO2 and HCHO VCDs, estimated by the maximum frequency method, were 0.40 ± 1.13 × 1015 molec. cm−2 for NO2 and 2.27 ± 1.66 × 1015 molec. cm−2 for HCHO in July 2021 over the Three Rivers' Source region. We also determined the dependence of the tropospheric NO2 and HCHO VCDs on altitude, which generally presents a decreasing trend with increasing altitude. This characteristic for natural background atmosphere is probably mainly related to the lower air density at higher altitudes. However, different from the nearly constant decreasing rate of HCHO VCDs with increasing altitude, the differences of decreasing rate above and below the 2750 m altitude for NO2 VCDs are significant, which is highly connected with different contributions of anthropogenic sources and natural sources for NO2 and HCHO.
With respect to the spatio-temporal distributions, the day-to-day variations of the NO2 VCDs between different circling journeys were similar; i.e. similar geographical distributions of the NO2 VCDs were observed for each circling journey. The tropospheric NO2 VCDs over the main area of the Three Rivers' Source were relatively uniform with very low levels (<1 × 1015 molec. cm−2), but they were usually elevated in cities or counties, around Qinghai Lake, and even occasionally at the motorway junction and the tunnel exit, where there were enhanced transport emissions. The daytime diurnal patterns of NO2 VCDs, i.e. higher values in the morning and evening, could also reflect the differences of the NO2 spatial distribution. Based on radiative transfer simulations, we can rule out that the stratospheric NO2 absorption can explain the observed diurnal cycle of the tropospheric NO2 VCDs. Besides the enhanced NO2 photolysis around noon, the enhanced NO2 VCDs in the morning and evening were primarily caused by enhanced pollution levels when the mobile observation vehicle was located in or close to the cities or county towns. However, the day-to-day variations of the HCHO VCDs were highly correlated to the air temperature and were significantly different between different circling journeys. Overall, the HCHO VCDs presented a decreasing trend from the northeast to the southwest in the region of the field experiment. The HCHO VCDs were elevated at the starting points and ending points of the driving routes, corresponding to larger HCHO VCDs in the morning and evening. The levels of the HCHO VCDs were variable on different days at the same location, implying that natural factors, such as air temperature, significantly influenced the atmospheric HCHO photochemical formation.
TROPOMI NO2 clearly presents the obvious influences of anthropogenic sources on enhanced NO2 VCDs around Xining city; i.e. it can distinguish the differences in tropospheric NO2 VCDs between the city and the background atmosphere over the region of the field campaign. However, the elevated trends of the tropospheric NO2 VCDs around the counties over the main area of the Three Rivers' Source region, which are clearly observed by the mobile MAX-DOAS, are nearly not captured by TROPOMI. In contrast, the stronger influences of natural factors on HCHO lead to larger daily variation of HCHO, which causes inconsistent and variable spatial distributions of TROPOMI HCHO VCDs on different days but also a higher correlation between mobile MAX-DOAS and TROPOMI than for NO2 for the background atmosphere. The positive offsets of TROPOMI NO2 and HCHO VCDs are 40 % and 87 % on average, respectively. This is probably caused by mountain terrains and varying local microclimates over the main area of the Three Rivers' Source region.
As a whole, we obtained valuable data sets and information of the spatio-temporal variation of NO2 and HCHO over the Tibetan Plateau, which have great potential for investigating the evolution of the atmospheric composition in the background atmosphere at high altitude, validating and improving the satellite products over mountain terrain, and evaluating atmospheric chemistry model over the Tibetan Plateau.
The filtered final NO2 and HCHO VCDs for the field campaign by mobile MAX-DOAS observations on 18–30 July 2021 over the Three Rivers' Source region of the Tibetan Plateau in China are available upon request.
The supplement related to this article is available online at: https://doi.org/10.5194/acp-23-3655-2023-supplement.
XC and XX designed the field experiment. SC and JM set up the mobile MAX-DOAS measurement platform under discussions with XC, JL, SZ, SD, and TW. WZ, GB, BC, and SM contributed to the field measurements. SC performed the spectra retrieval and data analysis with contributions from TW, SZ, SD, and JM. SC, JM, and TW prepared the paper with consent from all co-authors.
At least one of the (co-)authors is a member of the editorial board of Atmospheric Chemistry and Physics. The peer-review process was guided by an independent editor, and the authors also have no other competing interests to declare.
Publisher's note: Copernicus Publications remains neutral with regard to jurisdictional claims in published maps and institutional affiliations.
This article is part of the special issue “In-depth study of the atmospheric chemistry over the Tibetan Plateau: measurement, processing, and the impacts on climate and air quality (ACP/AMT inter-journal SI)”. It is not associated with a conference.
We thank the staff at the Qinghai Meteorological Administration for supporting the measurements. We thank BIRA-IASB for QDOAS spectral analysis software. We also thank ESA, KNMI, DLR, and NASA for the TROPOMI satellite products.
This research is supported by grants from the Fundamental Research Funds for Central Public-interest Scientific Institution from the Chinese Academy of Meteorological Sciences (grant no. 2021Z013), the National Natural Science Foundation of China (grant no. 41875146), and the Fund of State Key Laboratory of Applied Optics (grant no. SKLAO2021001A02).
This paper was edited by Steven Brown and reviewed by two anonymous referees.
Bolin, B.: On the Influence of the Earth's Orography on the General Character of the Westerlies, Tellus, 2, 184–195, https://doi.org/10.3402/tellusa.v2i3.8547, 1950.
Boos, W. R. and Kuang, Z.: Dominant control of the South Asian monsoon by orographic insulation versus plateau heating, Nature, 463, 218–222, https://doi.org/10.1038/nature08707, 2010.
Borovski, A. N., Dzhola, A. V., Elokhov, A. S., Grechko, E. I., Kanaya, Y., and Postylyakov, O. V.: First measurements of formaldehyde integral content in the atmosphere using MAX-DOAS in the Moscow Region, Int. J. Remote Sens., 35, 5609–5627, 2014.
Brinksma, E. J., Pinardi, G., Volten, H., Braak, R., Richter, A., Schönhardt, A., van Roozendael, M., Fayt, C., Hermans, C., Dirksen, R. J., Vlemmix, T., Berkhout, A. J. C., Swart, D. P. J., Oetjen, H., Wittrock, F., Wagner, T., Ibrahim, O. W., de Leeuw, G., Moerman, M., Curier, R. L., Celarier, E. A., Cede, A., Knap, W. H., Veefkind, J. P., Eskes, H. J., Allaart, M., Rothe, R., Piters, A. J. M., and Levelt, P. F.: The 2005 and 2006 DANDELIONS NO2 and aerosol intercomparison campaigns, J. Geophys. Res., 113, D16S46, https://doi.org/10.1029/2007jd008808, 2008.
Chen, P., Kang, S., Yang, J., Pu, T., Li, C., Guo, J., and Tripathee, L.: Spatial and Temporal Variations of Gaseous and Particulate Pollutants in Six Sites in Tibet, China, during 2016–2017, Aerosol Air Qual. Res., 19, 516–527, https://doi.org/10.4209/aaqr.2018.10.0360, 2019.
Cheng, S., Wang, Y., and An, X.: Temporal Variation and Source Identification of Black Carbon at Lin'an and Longfengshan Regional Background Stations in China, J. Meteorol. Res., 31, 1070–1084, https://doi.org/10.1007/s13351-017-7030-5, 2017.
Cheng, S., Ma, J., Cheng, W., Yan, P., Zhou, H., Zhou, L., and Yang, P.: Tropospheric NO2 vertical column densities retrieved from ground-based MAX-DOAS measurements at Shangdianzi regional atmospheric background station in China, J. Environ. Sci. (China), 80, 186–196, https://doi.org/10.1016/j.jes.2018.12.012, 2019.
Cheng, S., Ma, J., Zheng, X., Gu, M., Donner, S., Dörner, S., Zhang, W., Du, J., Li, X., Liang, Z., Lv, J., and Wagner, T.: Retrieval of O3, NO2, BrO and OClO Columns from Ground-Based Zenith Scattered Light DOAS Measurements in Summer and Autumn over the Northern Tibetan Plateau, Remote Sens., 13, 4242, https://doi.org/10.3390/rs13214242, 2021.
Cheng, S., Jin, J., Ma, J., Lv, J., Liu, S., and Xu, X.: Temporal Variation of NO2 and HCHO Vertical Profiles Derived from MAX-DOAS Observation in Summer at a Rural Site of the North China Plain and Ozone Production in Relation to HCHO/NO2 Ratio, Atmosphere, 13, 860, https://doi.org/10.3390/atmos13060860, 2022.
Coburn, S., Dix, B., Sinreich, R., and Volkamer, R.: The CU ground MAX-DOAS instrument: characterization of RMS noise limitations and first measurements near Pensacola, FL of BrO, IO, and CHOCHO, Atmos. Meas. Tech., 4, 2421–2439, https://doi.org/10.5194/amt-4-2421-2011, 2011.
Danckaert, T., Fayt, C., Roozendael, M. V., Smedt, I. D., Letocart, V., Merlaud, A., and Pinardi, G.: QDOAS Software user manual, Belgian Institute for Space Aeronomy, Brussels, Belgium, https://uv-vis.aeronomie.be/software/QDOAS/ (last access: 12 April 2022), 2017.
De Mazière, M., Thompson, A. M., Kurylo, M. J., Wild, J. D., Bernhard, G., Blumenstock, T., Braathen, G. O., Hannigan, J. W., Lambert, J.-C., Leblanc, T., McGee, T. J., Nedoluha, G., Petropavlovskikh, I., Seckmeyer, G., Simon, P. C., Steinbrecht, W., and Strahan, S. E.: The Network for the Detection of Atmospheric Composition Change (NDACC): history, status and perspectives, Atmos. Chem. Phys., 18, 4935–4964, https://doi.org/10.5194/acp-18-4935-2018, 2018.
De Smedt, I., Pinardi, G., Vigouroux, C., Compernolle, S., Bais, A., Benavent, N., Boersma, F., Chan, K.-L., Donner, S., Eichmann, K.-U., Hedelt, P., Hendrick, F., Irie, H., Kumar, V., Lambert, J.-C., Langerock, B., Lerot, C., Liu, C., Loyola, D., Piters, A., Richter, A., Rivera Cárdenas, C., Romahn, F., Ryan, R. G., Sinha, V., Theys, N., Vlietinck, J., Wagner, T., Wang, T., Yu, H., and Van Roozendael, M.: Comparative assessment of TROPOMI and OMI formaldehyde observations and validation against MAX-DOAS network column measurements, Atmos. Chem. Phys., 21, 12561–12593, https://doi.org/10.5194/acp-21-12561-2021, 2021.
Deutschmann, T., Beirle, S., Frieß, U., Grzegorski, M., Kern, C., Kritten, L., Platt, U., Prados-Román, C., Puķīte, J., Wagner, T., Werner, B., and Pfeilsticker, K.: The Monte Carlo atmospheric radiative transfer model McArtim: Introduction and validation of Jacobians and 3D features, J. Quant. Spectrosc. Ra., 112, 1119–1137, https://doi.org/10.1016/j.jqsrt.2010.12.009, 2011.
Dong, W., Lin, Y., Wright, J. S., Xie, Y., Xu, F., Xu, W., and Wang, Y.: Indian Monsoon Low-Pressure Systems Feed Up-and-Over Moisture Transport to the Southwestern Tibetan Plateau, J. Geophys. Res.-Atmos., 122, 12140–12151, https://doi.org/10.1002/2017jd027296, 2017.
Donner, S.: Mobile MAX-DOAS measurements of the tropospheric formaldehyde column in the Rhein-Main region, Master thesis, University of Mainz, Mainz, http://hdl.handle.net/11858/00-001M-0000-002C-EB17-2 (last acess: 3 April 2021), 2016.
Duan, A., Zhang, Q., Liu, Y., Wu, G., Wang, T., Wan, R., Liu, X., Li, W., Wang, Z., and Liang, X.: The Influence of Mechanical and Thermal Forcing by the Tibetan Plateau on Asian Climate, J. Hydrometeorol., 8, 770–789, https://doi.org/10.1175/jhm609.1, 2007.
Duo, B., Cui, L., Wang, Z., Li, R., Zhang, L., Fu, H., Chen, J., Zhang, H., and Qiong, A.: Observations of atmospheric pollutants at Lhasa during 2014–2015: Pollution status and the influence of meteorological factors, J. Environ. Sci. (China), 63, 28–42, https://doi.org/10.1016/j.jes.2017.03.010, 2018.
ESA and DLR: Sentinel-5P TROPOMI Tropospheric Formaldehyde HCHO 1-Orbit L2 5.5km x 3.5km, Goddard Earth Sciences Data and Information Services Center (GES DISC) [data set], https://doi.org/10.5270/S5P-vg1i7t0, 2020.
ESA and KNMI: Sentinel-5P TROPOMI Tropospheric NO2 1-Orbit L2 5.5km x 3.5km, Goddard Earth Sciences Data and Information Services Center (GES DISC) [data set], https://doi.org/10.5270/S5P-9bnp8q8, 2021.
Franco, B., Hendrick, F., Van Roozendael, M., Müller, J.-F., Stavrakou, T., Marais, E. A., Bovy, B., Bader, W., Fayt, C., Hermans, C., Lejeune, B., Pinardi, G., Servais, C., and Mahieu, E.: Retrievals of formaldehyde from ground-based FTIR and MAX-DOAS observations at the Jungfraujoch station and comparisons with GEOS-Chem and IMAGES model simulations, Atmos. Meas. Tech., 8, 1733–1756, https://doi.org/10.5194/amt-8-1733-2015, 2015.
Gao, J., Yao, T., Masson-Delmotte, V., Steen-Larsen, H. C., and Wang, W.: Collapsing glaciers threaten Asia's water supplies, Nature, 565, 19–21, https://doi.org/10.1038/d41586-018-07838-4, 2019.
Gil-Ojeda, M., Navarro-Comas, M., Gómez-Martín, L., Adame, J. A., Saiz-Lopez, A., Cuevas, C. A., González, Y., Puentedura, O., Cuevas, E., Lamarque, J.-F., Kinninson, D., and Tilmes, S.: NO2 seasonal evolution in the north subtropical free troposphere, Atmos. Chem. Phys., 15, 10567–10579, https://doi.org/10.5194/acp-15-10567-2015, 2015.
Gomez, L., Navarro-Comas, M., Puentedura, O., Gonzalez, Y., Cuevas, E., and Gil-Ojeda, M.: Long-path averaged mixing ratios of O3 and NO2 in the free troposphere from mountain MAX-DOAS, Atmos. Meas. Tech., 7, 3373–3386, https://doi.org/10.5194/amt-7-3373-2014, 2014.
Granier, C., Bessagnet, B., Bond, T., D'Angiola, A., Denier van der Gon, H., Frost, G. J., Heil, A., Kaiser, J. W., Kinne, S., Klimont, Z., Kloster, S., Lamarque, J.-F., Liousse, C., Masui, T., Meleux, F., Mieville, A., Ohara, T., Raut, J.-C., Riahi, K., Schultz, M. G., Smith, S. J., Thompson, A., van Aardenne, J., van der Werf, G. R., and van Vuuren, D. P.: Evolution of anthropogenic and biomass burning emissions of air pollutants at global and regional scales during the 1980–2010 period, Climatic Change, 109, 163–190, https://doi.org/10.1007/s10584-011-0154-1, 2011.
Guo, F., Bao, M., Mu, Y., Liu, Z., Li, Y., and Shi, H.: Temporal and spatial characteristics of lightning-produced nitrogen oxides in China, J. Atmos. Sol.-Terr. Phys., 149, 100–107, https://doi.org/10.1016/j.jastp.2016.10.007, 2016.
He, J., Gong, S., Yu, Y., Yu, L., Wu, L., Mao, H., Song, C., Zhao, S., Liu, H., Li, X., and Li, R.: Air pollution characteristics and their relation to meteorological conditions during 2014–2015 in major Chinese cities, Environ. Pollut., 223, 484–496, https://doi.org/10.1016/j.envpol.2017.01.050, 2017.
Hong, Q., Liu, C., Chan, K. L., Hu, Q., Xie, Z., Liu, H., Si, F., and Liu, J.: Ship-based MAX-DOAS measurements of tropospheric NO2, SO2, and HCHO distribution along the Yangtze River, Atmos. Chem. Phys., 18, 5931–5951, https://doi.org/10.5194/acp-18-5931-2018, 2018.
Hönninger, G., von Friedeburg, C., and Platt, U.: Multi axis differential optical absorption spectroscopy (MAX-DOAS), Atmos. Chem. Phys., 4, 231–254, https://doi.org/10.5194/acp-4-231-2004, 2004.
Kang, S., Zhang, Q., Zhang, Y., Guo, W., Ji, Z., Shen, M., Wang, S., Wang, X., Tripathee, L., Liu, Y., Gao, T., Xu, G., Gao, Y., Kaspari, S., Luo, X., and Mayewski, P.: Warming and thawing in the Mt. Everest region: A review of climate and environmental changes, Earth-Sci. Rev., 225, 103911, https://doi.org/10.1016/j.earscirev.2021.103911, 2022.
Kurokawa, J., Ohara, T., Morikawa, T., Hanayama, S., Janssens-Maenhout, G., Fukui, T., Kawashima, K., and Akimoto, H.: Emissions of air pollutants and greenhouse gases over Asian regions during 2000–2008: Regional Emission inventory in ASia (REAS) version 2, Atmos. Chem. Phys., 13, 11019–11058, https://doi.org/10.5194/acp-13-11019-2013, 2013.
Lee, D. S., Köhler, I., Grobler, E., Rohrer, F., Sausen, R., Gallardo-Klenner, L., Olivier, J. G. J., Dentener, F. J., and Bouwman, A. F.: Estimations of global NOx emissions and their uncertainties, Atmos. Environ., 31, 1735–1749, https://doi.org/10.1016/s1352-2310(96)00327-5, 1997.
Liu, Y., Hoskins, B., and Blackburn, M.: Impact of Tibetan Orography and Heating on the Summer Flow over Asia, J. Meteorol. Soc. Japan. Ser. II, 85B, 1–19, https://doi.org/10.2151/jmsj.85B.1, 2007.
Ma, J. Z., Tang, J., Zhou, X. J., and Zhang, X. S.: Estimates of the chemical budget for ozone at Waliguan Observatory, J. Atmos. Chem., 41, 21–48, https://doi.org/10.1023/A:1013892308983, 2002.
Ma, J. Z., Beirle, S., Jin, J. L., Shaiganfar, R., Yan, P., and Wagner, T.: Tropospheric NO2 vertical column densities over Beijing: results of the first three years of ground-based MAX-DOAS measurements (2008–2011) and satellite validation, Atmos. Chem. Phys., 13, 1547–1567, https://doi.org/10.5194/acp-13-1547-2013, 2013.
Ma, J., Brühl, C., He, Q., Steil, B., Karydis, V. A., Klingmüller, K., Tost, H., Chen, B., Jin, Y., Liu, N., Xu, X., Yan, P., Zhou, X., Abdelrahman, K., Pozzer, A., and Lelieveld, J.: Modeling the aerosol chemical composition of the tropopause over the Tibetan Plateau during the Asian summer monsoon , Atmos. Chem. Phys., 19, 11587–11612, https://doi.org/10.5194/acp-19-11587-2019, 2019.
Ma, J., Dörner, S., Donner, S., Jin, J., Cheng, S., Guo, J., Zhang, Z., Wang, J., Liu, P., Zhang, G., Pukite, J., Lampel, J., and Wagner, T.: MAX-DOAS measurements of NO2, SO2, HCHO, and BrO at the Mt. Waliguan WMO GAW global baseline station in the Tibetan Plateau, Atmos. Chem. Phys., 20, 6973–6990, https://doi.org/10.5194/acp-20-6973-2020, 2020.
Ma, J., Zhou, X., Xu, X., Xu, X., Gromov, S., and Lelieveld, J.: Ozone and aerosols over the Tibetan Plateau, in: Asian Atmospheric Pollution: Sources, Characteristics and Impacts, edited by: Singh, R. P., Elsevier, Amsterdam, Netherlands, 287–302, ISBN 9780128166932, 2021.
Marais, E. A., Roberts, J. F., Ryan, R. G., Eskes, H., Boersma, K. F., Choi, S., Joiner, J., Abuhassan, N., Redondas, A., Grutter, M., Cede, A., Gomez, L., and Navarro-Comas, M.: New observations of NO2 in the upper troposphere from TROPOMI, Atmos. Meas. Tech., 14, 2389–2408, https://doi.org/10.5194/amt-14-2389-2021, 2021.
Meller, R. and Moortgat, G. K.: Temperature dependence of the absorption cross sections of formaldehyde between 223 and 323 K in the wavelength range 225–375 nm, J. Geophys. Res.-Atmos., 105, 7089–7101, https://doi.org/10.1029/1999jd901074, 2000.
Meng, Z.-Y., Xu, X.-B., Wang, T., Zhang, X.-Y., Yu, X.-L., Wang, S.-F., Lin, W.-L., Chen, Y.-Z., Jiang, Y.-A., and An, X.-Q.: Ambient sulfur dioxide, nitrogen dioxide, and ammonia at ten background and rural sites in China during 2007–2008, Atmos. Environ., 44, 2625–2631, https://doi.org/10.1016/j.atmosenv.2010.04.008, 2010.
Mu, Y., Pang, X., Quan, J., and Zhang, X.: Atmospheric carbonyl compounds in Chinese background area: A remote mountain of the Qinghai-Tibetan Plateau, J. Geophys. Res., 112, D22302, https://doi.org/10.1029/2006jd008211, 2007.
Platt, U. and Stutz, J.: Differential Optical absorption spectroscopy, Principles and Applications, Springer, Berlin, ISBN 9783540211938, 2008.
Polyansky, O. L., Kyuberis, A. A., Zobov, N. F., Tennyson, J., Yurchenko, S. N., and Lodi, L.: ExoMol molecular line lists XXX: a complete high-accuracy line list for water, Mon. Not. R. Astron. Soc., 480, 2597–2608, https://doi.org/10.1093/mnras/sty1877, 2018.
Qiu, J.: China: The third pole, Nature, 454, 393–396, https://doi.org/10.1038/454393a, 2008.
Ran, L., Lin, W. L., Deji, Y. Z., La, B., Tsering, P. M., Xu, X. B., and Wang, W.: Surface gas pollutants in Lhasa, a highland city of Tibet – current levels and pollution implications, Atmos. Chem. Phys., 14, 10721–10730, https://doi.org/10.5194/acp-14-10721-2014, 2014.
Schreier, S. F., Richter, A., Wittrock, F., and Burrows, J. P.: Estimates of free-tropospheric NO2 and HCHO mixing ratios derived from high-altitude mountain MAX-DOAS observations at midlatitudes and in the tropics, Atmos. Chem. Phys., 16, 2803–2817, https://doi.org/10.5194/acp-16-2803-2016, 2016.
Seinfeld, J. H. and Pandis, S. N.: Atmospheric Chemistry and Physics: From Air Pollution to Climate Change, 3rd Edn., John Wiley & Sons, Inc., Hoboken, New Jersey, USA, ISBN 9780471178163, 2016.
Serdyuchenko, A., Gorshelev, V., Weber, M., Chehade, W., and Burrows, J. P.: High spectral resolution ozone absorption cross-sections – Part 2: Temperature dependence, Atmos. Meas. Tech., 7, 625–636, https://doi.org/10.5194/amt-7-625-2014, 2014.
Singh, R. P.: Asian Atmospheric Pollution: Sources, Characteristics and Impacts, Elsevier, Amsterdam, Netherlands, ISBN 9780128166932, 2021.
Stavrakou, T., Müller, J.-F., De Smedt, I., Van Roozendael, M., van der Werf, G. R., Giglio, L., and Guenther, A.: Evaluating the performance of pyrogenic and biogenic emission inventories against one decade of space-based formaldehyde columns, Atmos. Chem. Phys., 9, 1037–1060, https://doi.org/10.5194/acp-9-1037-2009, 2009.
Stutz, J. and Platt, U.: Numerical analysis and estimation of the statistical error of differential optical absorption spectroscopy measurements with least-squares methods, Appl. Opt., 35, 6041–6053, https://doi.org/10.1364/AO.35.006041, 1996.
Thalman, R. and Volkamer, R.: Temperature dependent absorption cross-sections of O2-O2 collision pairs between 340 and 630 nm and at atmospherically relevant pressure, Phys. Chem. Chem. Phys., 15, 15371–15381, https://doi.org/10.1039/c3cp50968k, 2013.
Vandaele, A. C., Hermans, C., Simon, P. C., Carleer, M., Colin, R., Fally, S., Mérienne, M. F., Jenouvrier, A., and Coquart, B.: Measurements of the NO2 absorption cross-section from 42 000 cm−1 to 10 000 cm−1 (238–1000 nm) at 220 K and 294 K, J. Quant. Spectrosc. Ra., 59, 171–184, https://doi.org/10.1016/s0022-4073(97)00168-4, 1998.
Veefkind, J. P., Aben, I., McMullan, K., Förster, H., Vries, J. d., Otter, G., Claas, J., Eskes, H. J., Haan, J. F. d., Kleipool, Q., Weele, M. v., Hasekamp, O., Hoogeveen, R., Landgraf, J., Snel, R., Tol, P., Ingmann, P., Voors, R., Kruizinga, B., Vink, R., Visser, H., and Levelt, P. F.: TROPOMI on the ESA Sentinel-5 Precursor: A GMES mission for global observations of the atmospheric composition for climate, air quality and ozone layer applications, Remote Sens. Environ., 120, 70–83, https://doi.org/10.1016/j.rse.2011.09.027, 2012.
Verhoelst, T., Compernolle, S., Pinardi, G., Lambert, J.-C., Eskes, H. J., Eichmann, K.-U., Fjæraa, A. M., Granville, J., Niemeijer, S., Cede, A., Tiefengraber, M., Hendrick, F., Pazmiño, A., Bais, A., Bazureau, A., Boersma, K. F., Bognar, K., Dehn, A., Donner, S., Elokhov, A., Gebetsberger, M., Goutail, F., Grutter de la Mora, M., Gruzdev, A., Gratsea, M., Hansen, G. H., Irie, H., Jepsen, N., Kanaya, Y., Karagkiozidis, D., Kivi, R., Kreher, K., Levelt, P. F., Liu, C., Müller, M., Navarro Comas, M., Piters, A. J. M., Pommereau, J.-P., Portafaix, T., Prados-Roman, C., Puentedura, O., Querel, R., Remmers, J., Richter, A., Rimmer, J., Rivera Cárdenas, C., Saavedra de Miguel, L., Sinyakov, V. P., Stremme, W., Strong, K., Van Roozendael, M., Veefkind, J. P., Wagner, T., Wittrock, F., Yela González, M., and Zehner, C.: Ground-based validation of the Copernicus Sentinel-5P TROPOMI NO2 measurements with the NDACC ZSL-DOAS, MAX-DOAS and Pandonia global networks, Atmos. Meas. Tech., 14, 481–510, https://doi.org/10.5194/amt-14-481-2021, 2021.
Vigouroux, C., Langerock, B., Bauer Aquino, C. A., Blumenstock, T., Cheng, Z., De Mazière, M., De Smedt, I., Grutter, M., Hannigan, J. W., Jones, N., Kivi, R., Loyola, D., Lutsch, E., Mahieu, E., Makarova, M., Metzger, J.-M., Morino, I., Murata, I., Nagahama, T., Notholt, J., Ortega, I., Palm, M., Pinardi, G., Röhling, A., Smale, D., Stremme, W., Strong, K., Sussmann, R., Té, Y., van Roozendael, M., Wang, P., and Winkler, H.: TROPOMI–Sentinel-5 Precursor formaldehyde validation using an extensive network of ground-based Fourier-transform infrared stations, Atmos. Meas. Tech., 13, 3751–3767, https://doi.org/10.5194/amt-13-3751-2020, 2020.
Wang, Q. Y., Gao, R. S., Cao, J. J., Schwarz, J. P., Fahey, D. W., Shen, Z. X., Hu, T. F., Wang, P., Xu, X. B., and Huang, R. J.: Observations of high level of ozone at Qinghai Lake basin in the northeastern Qinghai-Tibetan Plateau, western China, J. Atmos. Chem., 72, 19–26, https://doi.org/10.1007/s10874-015-9301-9, 2015.
Wang, T., Wong, H. L. A., Tang, J., Ding, A., Wu, W. S., and Zhang, X. C.: On the origin of surface ozone and reactive nitrogen observed at a remote mountain site in the northeastern Qinghai-Tibetan Plateau, western China, J. Geophys. Res., 111, D08303, https://doi.org/10.1029/2005jd006527, 2006.
Wang, Y., Puíīte, J., Wagner, T., Donner, S., Beirle, S., Hilboll, A., Vrekoussis, M., Richter, A., Apituley, A., Piters, A., Allaart, M., Eskes, H., Frumau, A., Van Roozendael, M., Lampel, J., Platt, U., Schmitt, S., Swart, D., and Vonk, J.: Vertical Profiles of Tropospheric Ozone From MAX-DOAS Measurements During the CINDI-2 Campaign: Part 1-Development of a New Retrieval Algorithm, J. Geophys. Res.-Atmos., 123, 10637–10670, https://doi.org/10.1029/2018jd028647, 2018.
Xing, C., Liu, C., Wu, H., Lin, J., Wang, F., Wang, S., and Gao, M.: Ground-based vertical profile observations of atmospheric composition on the Tibetan Plateau (2017–2019), Earth Syst. Sci. Data, 13, 4897–4912, https://doi.org/10.5194/essd-13-4897-2021, 2021.
Xu, X., Lu, C., Shi, X., and Gao, S.: World water tower: An atmospheric perspective, Geophys. Res. Lett., 35, L20815, https://doi.org/10.1029/2008gl035867, 2008.
Xu, X., Lin, W., Xu, W., Jin, J., Wang, Y., Zhang, G., Zhang, X., Ma, Z., Dong, Y., Ma, Q., Yu, D., Li, Z., Wang, D., and Zhao, H.: Long-term changes of regional ozone in China: implications for human health and ecosystem impacts, Elementa: Science of the Anthropocene, 8, 13, https://doi.org/10.1525/elementa.409, 2020.
Xue, L. K., Wang, T., Zhang, J. M., Zhang, X. C., Deliger, Poon, C. N., Ding, A. J., Zhou, X. H., Wu, W. S., Tang, J., Zhang, Q. Z., and Wang, W. X.: Source of surface ozone and reactive nitrogen speciation at Mount Waliguan in western China: New insights from the 2006 summer study, J. Geophys. Res., 116, D07306, https://doi.org/10.1029/2010jd014735, 2011.
Xue, L. K., Wang, T., Guo, H., Blake, D. R., Tang, J., Zhang, X. C., Saunders, S. M., and Wang, W. X.: Sources and photochemistry of volatile organic compounds in the remote atmosphere of western China: results from the Mt. Waliguan Observatory, Atmos. Chem. Phys., 13, 8551–8567, https://doi.org/10.5194/acp-13-8551-2013, 2013.
Yanai, M., Li, C., and Song, Z.: Seasonal Heating of the Tibetan Plateau and Its Effects on the Evolution of the Asian Summer Monsoon, J. Meteorol. Soc. Japan. Ser. II, 70, 319–351, https://doi.org/10.2151/jmsj1965.70.1B_319, 1992.
Yang, J., Ji, Z., Kang, S., Zhang, Q., Chen, X., and Lee, S.-Y.: Spatiotemporal variations of air pollutants in western China and their relationship to meteorological factors and emission sources, Environ. Pollut., 254, 112952, https://doi.org/10.1016/j.envpol.2019.07.120, 2019.
Zhang, Y., Ju, T., Shi, Y., Wang, Q., Li, F., and Zhang, G.: Analysis of spatiotemporal variation of formaldehyde column concentration in Qinghai-Tibet Plateau and its influencing factors, Environ. Sci. Pollut. Res. Int., 28, 55233–55251, https://doi.org/10.1007/s11356-021-14719-3, 2021.
Zhao, R., Dou, X., Zhang, N., Zhao, X., Yang, W., Han, B., Yu, H., Azzi, M., Wang, Y., and Bai, Z.: The characteristics of inorganic gases and volatile organic compounds at a remote site in the Tibetan Plateau, Atmos. Res., 234, 104740, https://doi.org/10.1016/j.atmosres.2019.104740, 2020.
Zhou, X., Zhao, P., Chen, J., Chen, L., and Li, W.: Impacts of thermodynamic processes over the Tibetan Plateau on the Northern Hemispheric climate, Science in China Series D: Earth Sciences, 52, 1679–1693, https://doi.org/10.1007/s11430-009-0194-9, 2009.
- Abstract
- Introduction
- Field experiment
- Spectral retrieval and data filtering
- Interpretation of the results
- Summary and conclusions
- Code and data availability
- Author contributions
- Competing interests
- Disclaimer
- Special issue statement
- Acknowledgements
- Financial support
- Review statement
- References
- Supplement
- Abstract
- Introduction
- Field experiment
- Spectral retrieval and data filtering
- Interpretation of the results
- Summary and conclusions
- Code and data availability
- Author contributions
- Competing interests
- Disclaimer
- Special issue statement
- Acknowledgements
- Financial support
- Review statement
- References
- Supplement