the Creative Commons Attribution 4.0 License.
the Creative Commons Attribution 4.0 License.
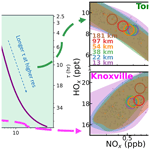
Variable effects of spatial resolution on modeling of nitrogen oxides
Randall V. Martin
Ronald C. Cohen
Liam Bindle
Dandan Zhang
Deepangsu Chatterjee
Hongjian Weng
Jintai Lin
The lifetime and concentration of nitrogen oxides (NOx) are susceptible to nonlinear production and loss and to the resolution of a chemical transport model (CTM). This is due to the strong spatial gradients of NOx and the dependence of its own chemical loss on such gradients. In this study, we use the GEOS-Chem CTM in its high-performance implementation (GCHP) to investigate NOx simulations over the eastern United States across a wide range of spatial model resolutions (six different horizontal grids from 13 to 181 km). Following increasing grid size, afternoon surface NOx mixing ratios over July 2015 generally decrease over the Great Lakes region (GL) and increase over the southern states of the US region (SS), yielding regional differences (181 km vs. 13 km) of −16 % (in the GL) to 7 % (in the SS); meanwhile, hydrogen oxide radicals (HOx) increase over both regions, consistent with their different chemical regimes (i.e., NOx-saturated in the GL and NOx-limited in the SS). Nighttime titration of ozone by surface nitric oxide (NO) was found to be more efficient at coarser resolutions, leading to longer NOx lifetimes and higher surface mixing ratios of nitrogen dioxide (NO2) over the GL in January 2015. The tropospheric NO2 column density at typical afternoon satellite overpass time has spatially more coherent negative biases (e.g., −8 % over the GL) at coarser resolutions in July, which reversed the positive biases of surface NOx over the SS. The reduced NOx aloft (>1 km altitude) at coarser resolutions was attributable to the enhanced HOx that intrudes into the upper troposphere. Application of coarse-resolution simulations for interpreting satellite NO2 columns will generally underestimate surface NO2 over the GL and overestimate surface NO2 over the SS in summer, but it will uniformly overestimate NOx emissions over both regions. This study significantly broadens understanding of factors contributing to NOx resolution effects and the role of fine-resolution data in accurately simulating and interpreting NOx and its relevance to air quality.
- Article
(20471 KB) - Full-text XML
-
Supplement
(1016 KB) - BibTeX
- EndNote
Nitrogen oxides (NOx≡ NO + NO2) have major roles in tropospheric chemistry and air quality. During daytime, NOx interacts with hydrogen oxide radicals (HOx≡ OH + HO2) and volatile organic compounds (VOCs) via photochemical reactions to affect formation of ozone and nitrate aerosols (e.g., Sillman, 1999; Thornton et al., 2002; Pusede et al., 2015; Zhu et al., 2022). During nighttime, persistent NOx emissions are the main chemical sink of ozone in urban areas (Zhang et al., 2004; Brown et al., 2006; Zakoura and Pandis, 2018); meanwhile, sequential formation of the nitrate radical (NO3) significantly alters nocturnal atmospheric oxidation capacity and secondary aerosols (Evans and Jacob, 2005; Brown and Stutz, 2012; Rollins et al., 2012). NOx has strongly localized emissions (Miyazaki et al., 2017; Crippa et al., 2018; Beirle et al., 2019) and relatively short lifetimes (Kenagy et al., 2018; Laughner and Cohen, 2019), which determine its strong spatial heterogeneity, with a short e-folding distance of 30 km and less (Heue et al., 2008; Beirle et al., 2011; Valin et al., 2011). While such localization is advantageous for identifying and quantifying NOx emissions using observations such as satellite nitrogen dioxide (NO2) column density (e.g., Martin et al., 2006; Cooper et al., 2017; Goldberg et al., 2019; Laughner and Cohen, 2019; Wang et al., 2022), it poses a challenge for chemical transport models (CTMs) attempting to accurately represent the relevant production and loss processes at interurban scales (on the order of 10 km) due to limited computational resources.
One major issue is the resolution dependence of simulated NOx lifetime (τ) (Charlton-Perez et al., 2009; Valin et al., 2011), which is sensitive to NOx abundance itself due to the interaction of NOx with its own chemical loss (Laughner and Cohen, 2019; Shah et al., 2020), such as hydroxyl radical (OH) and ozone (e.g., Fig. A1a). Systematic differences in the simulated τ and NOx concentration at different resolutions were reported from simulated NOx plumes of power plants, cities and ship emissions (Sillman et al., 1990; Charlton-Perez et al., 2009; Valin et al., 2011) due to the stronger NOx localization at higher resolution. Regional chemical transport modeling with more realistically distributed emissions was also performed to examine how NOx abundance changes with varying resolutions (Valin et al., 2011; Yamaji et al., 2014; Yan et al., 2016; Yu et al., 2016). The majority of these studies indicated increased τ and NOx concentration at higher resolutions and attributed it to the titration of OH by NOx over sources in the NOx-saturated regime (e.g., the right part of Fig. A1a). However, evidence (Laughner and Cohen, 2019; Jin et al., 2020; Zhu et al., 2022) has emerged that many cities across the United States (US) recently experienced the transition into the NOx-limited regime following emission regulations, where stronger NOx emissions actually promote HOx production and decrease τ (e.g., the left part of Fig. A1a). It is unclear how this change will update our understanding of the spatial resolution dependency of simulated NOx. Furthermore, nighttime effects have rarely been studied, possibly due to the prolonged τ localization and reduced NOx localization, although the significant spatial heterogeneity of nocturnal NOx and ozone in urban environments has still been noted (Zhang et al., 2004; Pan et al., 2017; Zakoura and Pandis, 2018), and long-term effects of changing NOx on nighttime τ have also been found (Shah et al., 2020). Finally, retrieval of tropospheric NO2 column density (Ω) from a growing constellation of satellite instruments (e.g., OMI, TROPOMI, TEMPO, Sentinel-4, GEMS) offers observational information to constrain NOx emissions across vast continental and global regions (Veefkind et al., 2012; Streets et al., 2013; Zoogman et al., 2017; Levelt et al., 2018; Timmermans et al., 2019; Kim et al., 2020). Existing studies have not separately discussed the resolution effects on Ω and surface NOx, which can potentially differ due to the vertically nonuniform spatial heterogeneity and species abundances.
Given that numerous existing studies depicted an evident yet potentially incomplete mechanistic understanding, this study uses a CTM across a wide range of spatial resolutions to significantly enrich the current understanding of the resolution dependency of NOx simulations. We find evidence over the eastern US that changes in simulated NOx abundances at different resolutions are by no means uniform but instead depend on factors such as chemical regimes, dominant processes and vertical layering. This information urges future studies to apply an adequate resolution that captures the spatial scale of NOx sources and sinks to simulate and interpret NOx-relevant atmospheric chemistry and air quality issues.
We use the GEOS-Chem model in its high-performance implementation (GCHP, http://www.geos-chem.org, last access: February 2022, version 13.2.1, https://doi.org/10.5281/zenodo.5500718; The International GEOS-Chem User Community, 2021) to simulate NOx and its relevant components over the eastern US.
GCHP is a grid-independent implementation of GEOS-Chem operating in a distributed-memory framework for massive parallelization (Long et al., 2015; Eastham et al., 2018). Chemical transport is simulated using a finite-volume advection code (FV3) on a cubed-sphere grid (Putman and Lin, 2007). GCHP uses identical chemistry and physics modules as the traditional GEOS-Chem implementation (GEOS-Chem Classic). A stretched-grid capability offers finer resolution over a user-specified domain of interest (Bindle et al., 2021). The model version used here (v13.2.1) features significant advances for performance and ease of use (Martin et al., 2022). The model is driven by the Goddard Earth Observation System Forward Processing (GEOS-FP) assimilated meteorological data, with a native resolution of 0.25∘ × 0.3125∘, from the NASA Global Modeling and Assimilation Office (GMAO). The GEOS-FP data are currently the finest-resolution meteorology available for GCHP simulations for the simulation year and were regridded to each simulation resolution, including a resolution (of 13 km) that is finer than 0.25∘ × 0.3125∘. Although nonideal, this capability, as demonstrated by Bindle et al. (2021), will not significantly alter our interpretations, which focus on discussing the redistribution of NOx emissions and chemical feedbacks rather than effects from meteorology. Yan et al. (2016) showed that sub-coarse-grid emission–chemical variability dominantly contributed to the differences in simulated tropospheric chemistry between resolutions, overwhelming the effects from resolution of nonchemical factors such as meteorological data. Consistent with GEOS-FP, the atmosphere is vertically distributed into 72 layers (from surface to 0.01 hPa) following the hybrid sigma-pressure grid definition during the simulation. Boundary layer mixing is simulated with a nonlocal scheme (Lin and McElroy, 2010). The lowest layer is roughly 120 m thick, with mixing ratios of NOx, HOx and ozone that we refer to as the “surface concentrations” or “surface mixing ratios” interchangeably.
We use the standard full-chemistry scheme of the GEOS-Chem model, which is widely used to study air quality (Koplitz et al., 2016; Li et al., 2017; Shah et al., 2020; Gu et al., 2021). The scheme includes detailed gas-phase mechanisms of HOx–NOx–VOC–ozone chemistry (Bey et al., 2001; Mao et al., 2013; Sherwen et al., 2016), including heterogeneous uptake of reactive gases (McDuffie et al., 2018; Holmes et al., 2019) by the simultaneously simulated aerosols. Anthropogenic NOx emissions are from EDGAR v5.0 at 0.1∘ resolution (Crippa et al., 2021), and speciated anthropogenic non-methane VOC emissions are from CEDS v2 (Hoesly et al., 2018). Open-burning emissions are from GFED v4.1 (van der Werf et al., 2017). Although the latter two inventories have nonideal (0.5∘ and 0.25∘) resolutions due to availability, they are acceptable for the purpose of identifying the resolution dependence of NOx. One favorable capability of the simulation is the pre-calculated offline dust, lightning NOx, biogenic VOCs (BVOCs), soil NOx and sea salt aerosol emissions (Murray et al., 2012; Weng et al., 2020; Meng et al., 2021), which all avoid possible regional emission biases due to online calculations using meteorological fields at different resolutions and the consequent interference with the interpretation of the results. All of the emissions are handled by the HEMCO 3.0 module (Keller et al., 2014; Lin et al., 2021).
We conduct GCHP simulations for January (winter) and July (summer) of 2015 at six resolutions spanning the range of conventional global model capabilities (Table 1). The highest resolution (13 km) is close to the currently finest information from emission inventories (0.1∘) in global CTMs without downscaling, and the lowest resolution (181 km) remains widely used in global and regional air quality studies (i.e., similar to 2∘ × 2.5∘ resolution). For the three relatively coarse resolutions, we conduct global cubed-sphere simulations, while for resolutions <50 km we exploited the recently developed grid-stretching capability (Bindle et al., 2021) to greatly reduce the computational resource requirements. The stretched-grid configuration smoothly decreases the grid cell size towards the refined region of interest. We follow recommendations by Bindle et al. (2021) to choose moderate stretch factors (Table 1), ensuring that the lowest resolutions (i.e., on the antipode of the refined region) remain finer than 300 km while achieving substantially refined resolutions over the eastern US (Table 1, right).
Table 1Description of the simulations with six resolutions over the eastern US.
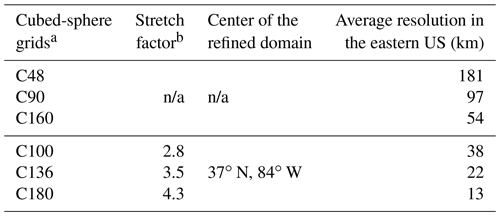
a A cubed-sphere grid contains a mosaic of six grids (faces). Each face is regularly spaced with N×N grid cells, and the notation of each resolution (CN) here identifies the size N. b Stretch factor (S) defines the strength of the grid-stretching. The resolution is about S times higher than the regular cubed sphere over the refined region (eastern US), while it is about of the original resolution over the antipode (n/a: not applicable).
In this work, we apply chemical operator durations of 20 min and transport operator durations of 10 min (i.e., C20T10) (Philip et al., 2016) to all of the simulations, largely in accordance with the GCHP default for cubed-sphere simulations, while avoiding interferences with our interpretation from inconsistent operator duration settings.
Initial conditions on 1 December 2014 and 1 June 2015 are obtained from a 1-year spin-up run of the C360 global cubed-sphere simulation using identical model version and inputs. These initial conditions resemble a realistic global high-resolution (∼ 25 km) distribution of NOx-relevant species and their oxidants driven by the consistent emissions and chemistry used in this study and were then regridded to drive the 2-month simulations at each resolution. The second month (January and July 2015) from each simulation is used for the analysis to allow for a further spin-up at each resolution. We archive the hourly average model outputs to enable the detailed discussion in Sect. 3.
Figure 1a shows the study domain of interest (26–48∘ N and 70–98∘ W) and the emission ratio of isoprene to NOx. The northern part of the domain comprises some of the most populous cities on the continent (e.g., New York City, Toronto and Chicago), with strong and localized NOx emissions as observed from space (Russell et al., 2012; Lu et al., 2015; Laughner and Cohen, 2019), ideal for investigating NOx resolution dependence. The southern regime is characteristic of the strongest biogenic VOC (e.g., isoprene) emission rates across the US (Romer et al., 2016; Yu et al., 2016; Jin et al., 2020) that could affect NOx lifetime and its response to resolution.
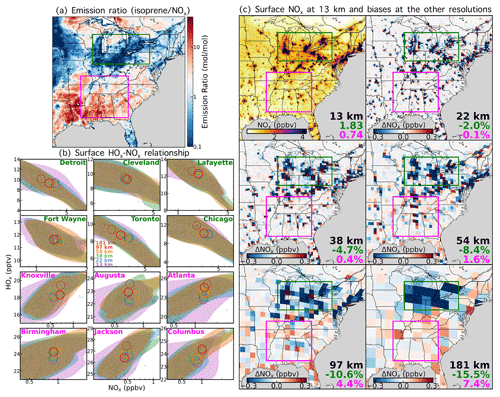
Figure 1Afternoon (UTC 19–24 or CST 13–18) resolution effects of surface NOx mixing ratio driven by NOx–HOx feedbacks (e.g., Fig. A1a) over July 2015. (a) Emission ratio of isoprene vs. NOx. (b) Simulated HOx–NOx relationship at each resolution (color coded). The contour lines (for resolutions <60 km) include 90 % of the scatter (points) of data within each 2∘ × 2∘ window centered over each city (based on kernel density estimation), and the circles indicated the means for all resolutions. (c) Surface NOx mixing ratio at 13 km (top left) and the differences (minus 13 km) at the other resolutions (other subpanels). The regional mean NOx (for 13 km, in ppbv) and percentage biases (for the other resolutions) are indicated at the bottom right of each subpanel. Green and magenta (rectangles, city names and numbers) label the Great Lakes region and the southern states of the US as defined in this study, respectively.
To assess the implications for interpreting satellite observations of NO2, we also apply monthly mean scattering weights (w) (Palmer et al., 2001) from the TROPOspheric Monitoring Instrument (TROPOMI) at its overpass time (19:00–21:00 UTC, i.e., 13:00–15:00 CST, central standard time) to calculate NO2 line-of-sight (slant) column density (Ωs). Comparing Ωs with Ω enables investigation of effects of satellite sensitivity on the resolution dependency of NO2 columnar abundances. TROPOMI is currently the only instrument with sufficient fine resolution to provide w information for all the investigated resolutions over the study domain. We assign every clear-sky (i.e., geometric cloud fraction <0.2) TROPOMI w in January or July 2019 to the colocated grid cells of each resolution to derive the monthly mean w distribution, which is then applied to the afternoon mean NO2 profiles in the same month of 2015 for each grid cell to calculate Ωs. These mean scattering weights are dependent primarily on observing geometry and relative vertical profiles of molecular and aerosol scattering (Palmer et al., 2001; Cooper et al., 2020), which are expected to be similar in the same month among proximal years.
In this study, all of the regridding procedures between different resolutions are conducted using the conservative (surface-area-weighted) algorithm (https://earthsystemmodeling.org/regrid/, last access: February 2022) in the Earth System Modeling Framework.
3.1 Daytime resolution effects at the surface in summer
Figure 1c shows the afternoon (19:00–24:00 UTC, corresponding to 13:00–18:00 CST) resolution effects of simulated surface NOx mixing ratios over the eastern US in July 2015. At the finest resolution of 13 km (Fig. 1c, upper left), NOx exhibits notable local enhancements over cities and major industrial corridors due to its short lifetime (τ, several hours). Overall, the stronger emissions and agglomerate sources in the Great Lakes region (GL, green box) lead to higher NOx levels than in the southern states of the US region (SS, magenta box) where NOx sources are relatively weak and sparse. As the grid cell size increases to 22 km resolution, the NOx level shows an overall decrease over emission centers and increase over nearby grids (by up to 1 ppbv) relative to 13 km, an expected consequence due to dilution of emissions. However, systematic biases in predicted NOx relative to 13 km resolution start to emerge, especially further downwind of urban NOx sources and over the SS, reflecting the effects from the resolution-dependent τ. The biases relative to 13 km resolution become increasingly pronounced and regionally coherent as grid cells further enlarge. At the two lowest resolutions (>90 km), a clear dipole of negative biases over the GL and positive biases over the SS becomes visible.
The opposite resolution effects of simulated NOx over the GL and SS are summarized as regional mean biases in each subpanel of Fig. 1c (e.g., −15.5 % at 181 km over the GL), which gradually increase in magnitude following increasing grid cell sizes, and these effects are attributable to their corresponding chemical regimes. The GL in summer is characterized by stronger NOx emissions and lower VOC emissions, while the opposite scenario prevails over the SS with strong BVOC sources, partially characterized by the distribution of the isopreneNOx emission ratio in Fig. 1a. Consequently, the NOx sources in the GL tend to be located in the NOx-saturated regime (Fig. A1a, right), where concentrated NOx levels at higher resolutions consume more OH and increase τ. Meanwhile, over the SS the relatively low NOx, together with the enhanced VOCs, can in contrast promote HOx production at higher NOx levels (NOx-limited regime, Fig. A1a, left), and thus the higher resolution introduces higher OH and lower τ. The lower τ can be a result of directly scavenging NO2 via the enhanced OH and of indirectly reducing nitric oxide (NO) via the OH-promoted organic peroxy radicals (RO2), an important NOx sink pathway under low-NOx and high-VOC environments (i.e., the SS) (Browne and Cohen, 2012; Perring et al., 2013; Romer et al., 2016; Romer Present et al., 2020). As NOx emissions continue to decrease, multiple lines of evidence suggest that NOx sources across the United States have recently entered or are approaching the NOx-limited regime (Laughner and Cohen, 2019; Jin et al., 2020; Koplitz et al., 2021; Jung et al., 2022; Zhu et al., 2022), especially over the SS since the 2010s (Jin et al., 2020; Koplitz et al., 2021; Zhu et al., 2022).
Figure 1b shows examples of afternoon HOx–NOx relationships for 12 cities. We use HOx to identify the regime representation since OH is unstable and has a very short lifetime (<1 s). HOx and NOx are anti-correlated over the GL (green label) and positively correlated over the SS (magenta label), consistently indicative of their different chemical regimes. Additionally, Figs. 1c and A2b show that (at 13 km resolution) locations with strong NOx enhancements coincide with locally lower HOx in the GL and generally associate with enhanced HOx in the SS. The mean NOx concentrations (circles) in Fig. 1b thus roughly decrease over the GL and increase over the SS following the opposite changes in τ as grid cell size increases (i.e., from purple to brown circles). The mean HOx biases within the 2∘ × 2∘ windows above the cities are uniformly positive due to the opposite HOx–NOx associations over the GL and SS. Figure A2b further identifies the broadly uniformly positive simulation biases of HOx in response to the opposite changes in NOx in the two regions, consistently verifying the two chemical regimes.
The spatial extent of chemical regimes and their effects on the NOx biases vary during the course of the day and contribute to relatively weak sensitivity of simulated NOx to resolution in the SS. Figure A3 shows the resolution dependence of simulated surface NOx for morning hours. Relative to the overall effects during afternoon (Fig. 1c), the NOx-saturated regime (with negative NOx biases at coarser resolution) has a broader extent (e.g., intruding further into the south) in the morning hours, whereas locations with positive biases (NOx-limited regime) are substantially reduced. The magnitudes of NOx bias (e.g., 181 km vs. 13 km) are also enhanced over the GL in the morning (−27.8 %). These differences are consistent with the relative diel evolution of NOx (decreasing since sunrise) and HOx (accumulating and peaking after noon) abundances and the consequence on the dominant HOx loss pathway (e.g., Ren et al., 2003; Ma et al., 2022).
Another potential cause of the relatively weak sensitivity of simulated NOx to resolution in the SS is the impact from BVOCs in addition to NOx heterogeneity. Figure A1b shows that changing VOC reactivity mainly affects OH concentration and τ over the NOx-limited regime but has little effect on the NOx-saturated regime, consistent with previous studies (Edwards et al., 2014; Laughner and Cohen, 2019; Zhu et al., 2022). Apart from increasing OH, which decreases τ (Fig. A1b), decreasing VOCs can also decrease the strength of the NO–RO2 loss pathway and increase τ (Browne and Cohen, 2012). Nonetheless, both processes indicate an increasing sensitivity of τ to VOCs in low-NOx environments (Romer et al., 2016; Laughner and Cohen, 2019; Romer Present et al., 2020). The SS features strong BVOC emissions and strong spatial segregation of NOx and VOC sources (Yu et al., 2016; Travis et al., 2016), as reflected by lower isopreneNOx emission ratios in urban centers relative to its neighborhood in Fig. 1a. Such segregation is reduced as the resolution coarsens (e.g., Fig. S1). The concurrent and usually opposite changes in NOx (increases) and BVOC (decreases) emissions around NOx sources at higher resolutions can jointly lead to the overall small changes among resolutions in the SS relative to in the GL. In summary, our simulations reveal that the predictability of actual resolution dependency of NOx in the NOx-limited regime is reduced due to the joint sensitivities to VOCs.
3.2 Nighttime resolution effects at surface in winter
Figure 2a shows the surface NOx mixing ratio and its resolution dependence at nighttime (03:00–10:00 UTC or 21:00–04:00 CST) in January 2015. With prolonged τ (∼ 20 h) as photochemistry ceases and OH becomes negligible, the wintertime and nighttime NOx exhibits reduced spatial heterogeneity (e.g., Fig. S2) relative to summertime and daytime in Fig. 1c, and thus the resolution effects are also less pronounced (i.e., ≤5 %). However, one characteristic phenomenon at nighttime that depends on resolution is the titration between NO and ozone (O3), which are the main nighttime sinks to each other in urban environments (Brown et al., 2006; Wang et al., 2006; Brown and Stutz, 2012; Kenagy et al., 2018; Shah et al., 2020). Figure 2b and 2c indicate that the titration between NO and O3 at the surface is enhanced by enlarged grid cells, as both concentrations were nearly uniformly reduced (by up to ∼ 50 % and ∼ 10 %, respectively) across the domain. At coarser resolutions, the faster O3 titration produces more NO2, complemented by less efficient scavenging of NO2 by the more titrated O3 (Fig. 2d). The resolution effect on surface NOx (Fig. 2a) is thus jointly contributed by the opposite changes in NO (Fig. 2b) and NO2 (Fig. 2d), the latter being more determinant due to its stronger contribution to total NOx.
The faster titration efficiency at coarser resolution is driven by the spatial anti-correlation of NO and O3, as demonstrated by Fig. A4. Typical nighttime high-NO regions are coincident with low-O3 locations at 13 km resolution (first row), a result of daytime O3 formation suppression and nighttime O3 titration (Jacob et al., 1995; Zhang et al., 2004; Jin et al., 2017; Yan et al., 2018; Sicard et al., 2020; Li et al., 2022) over strong NOx sources. This anti-correlation at fine resolution leads to inefficient NO–O3 reaction, which is to first-order proportional to their products, shown in the third column of Fig. A4. By simply diluting their concentrations to larger grid cells (second–sixth rows), the products of NO–O3 from less anti-correlated concentrations are enhanced systematically. Consequently, there would also be faster production of N2O5 and nitrates, which have been proposed by Zakoura and Pandis (2018) as an explanation for systematic overprediction of nitrate aerosols by CTMs at coarse resolution.
As the GL region has greater NO mixing ratios than the SS (Fig. 2b), surface O3 is more effectively titrated (Fig. 2c), leading to increased NO2 and NOx concentrations (Fig. 2d). Meanwhile, over the SS the NO2 response is less pronounced due to the lower NO levels, and NO2 exhibits reductions over certain locations at lower resolutions (Fig. 2d), indicating that the excess O3 can consume more NO2 after titrating NO over these grids.
3.3 Seasonal and diel variation in relevant processes
Figure 3 summarizes the resolution effects of regional mean surface NOx, HOx and ozone at different hours of the day. Over the GL, the strongest percentage biases of regional mean NOx (mainly NO2, Fig. S3) at resolutions >13 km occur at nighttime in January (up to 5 %) and during daytime in July (up to −30 %), revealing a pronounced seasonality of dominant mechanisms driving the resolution effects. This seasonality is driven by the stronger intensity and duration of daytime oxidant production in July (i.e., purple lines for HOx and O3 in Fig. 3); meanwhile, the greater nighttime O3 titration at coarser resolution partially counteracts the daytime effects in July (i.e., reduces the percentage biases) and dominates in January.
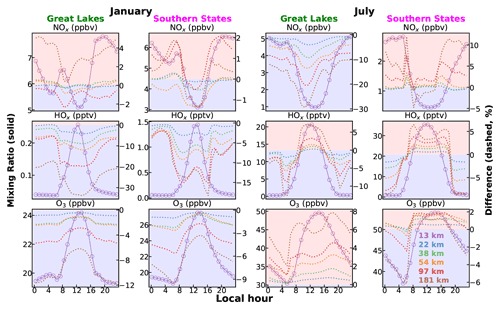
Figure 3Resolution effects of surface NOx dominated by nighttime biases in January and by daytime biases in July 2015. The absolute monthly mixing ratios (left y axis) of regional mean NOx, HOx and O3 are shown as circles and solid lines at 13 km resolution, and the differences vs. 13 km resolution (right y axis) are shown as dashed lines for the other resolutions (see legend in the lower-right subpanel). The positive (light red) and negative (light blue) difference regimes are divided by color.
The SS region has relatively strong daytime resolution sensitivity that compensates for the opposite nighttime effects on NO2 in January (Fig. S3), resulting in overall small changes in NOx. In July, the resolution effects exhibit both the daytime photochemical processes and titration-driven nighttime effects, especially the unique positive biases of both NOx and HOx (NOx-limited regime), during afternoon hours. Compared to the GL, July and daytime biases over the SS are not strong enough (e.g., discussions in Sect. 3.1) to mask the nighttime effects.
Overall, the daytime resolution effects driven by the involvement of NOx in HOx and O3 production (Sect. 3.1) compete with the nighttime effects driven by NOx-O3 titration (Sect. 3.2). The changing dominance of each mechanism during summer vs. winter, as well as during daytime and nighttime, leads to the characteristic seasonal and diel variation in Fig. 3.
3.4 Vertically variable resolution effects
Figure 4 shows the resolution-dependent changes in regional mean afternoon NOx vertical profiles in the lower troposphere (below 4 km). Uniform decreases in the simulated afternoon NOx following larger grid cells are apparent at >1 km altitude in July, despite opposite changes over the two regions near the surface being present (Fig. 1). These vertically dependent responses are caused by the different vertical profiles of NOx and HOx (i.e., purple lines). As NOx mixing ratio decreases exponentially aloft while HOx increases (in the GL) or remains relatively uniform (in the SS), HOx becomes more abundant relative to NOx at higher altitudes, meaning that τ is less sensitive to NOx local mixing ratios even above strong NOx sources. The enhanced oxidants (ozone and HOx) due to surface NOx emission heterogeneity (Sect. 3.1) then vertically mix to systematically enhance the HOx profile (Fig. 4, right) and reduce τ and NOx in these aloft layers. Therefore, both regions exhibit negative NOx biases due to coarse resolution above 1 km, regardless of chemical regime.
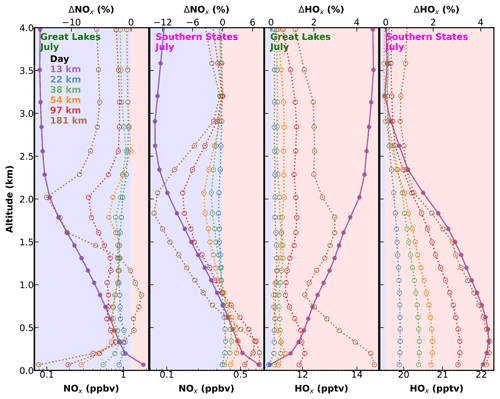
Figure 4Change in afternoon NOx vertical distribution in the lower troposphere at different resolutions in July. The absolute monthly and regional mean mixing ratios (lower x axis) of NOx and HOx are shown as filled symbols and solid lines at 13 km resolution, respectively, and the differences vs. 13 km resolution (upper x axis) are shown as empty symbols and dashed lines for the other resolutions, respectively (see legend in the left column). The positive (light red) and negative (light blue) difference regimes are color-divided.
Figure 5 shows the changes in the nighttime vertical profiles of NOx and O3 in January. These again show opposite vertical distributions of NOx and its nighttime sink (ozone). Over the GL, although the surface NOx lifetime can be possibly prolonged at coarse resolution due to the faster titration of O3 by NO (Sect. 3.2), NO quickly becomes insufficient to titrate the increasing ozone at higher altitudes. Therefore, both NOx species ultimately become affected by the resolution-dependent titration efficiency above 1 km (similar to the surface responses over the SS), leading to the negative biases in simulated NOx regardless of surface NOx emission strength.
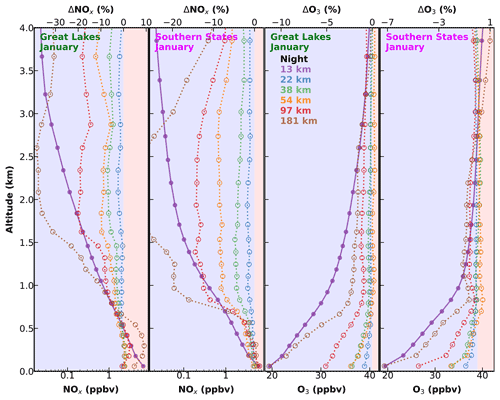
Figure 5Change in nighttime NOx vertical distribution in the lower troposphere at different resolutions in January. Similar to Fig. 4 but for nighttime NOx and O3 in January.
In summary, Figs. 4 and 5 reveal that the resolution effects of τ at the surface can differ from those at elevated altitudes, even over source regions. Such altitude-dependent responses will further affect interpretation of satellite-retrieved NO2 columnar properties, using model simulations at these resolutions.
3.5 Implications for satellite remote sensing applications
Satellite retrievals of NO2 vertical column density (Ω) have been widely used to quantify and characterize spatiotemporal variation in NOx abundances and sources. Here we evaluate the implications of the NOx resolution dependency on two major applications – estimating surface NO2 concentration and deriving NOx emissions.
Figure 6 shows the simulated fraction of NO2 abundance within the surface layer of GEOS-Chem relative to the whole troposphere during low Earth orbit (LEO) afternoon satellite overpass time. This surface fraction (Fs) is lower in summer than in winter, driven by stronger convection, lightning NOx and an elevated boundary layer. Fs is also lower over the SS than the GL in July due to relatively weak NOx emissions at the surface and stronger lightning NOx emissions in the upper troposphere (Murray et al., 2012; Silvern et al., 2019; Zhu et al., 2019). The changes in Fs following varying resolutions in general qualitatively resemble those of surface NOx (e.g., when comparing Fig. 6 with Figs. 1c and 2a). Overall, Fs has stronger biases in July than in January, lowered by 10 % over the GL and enhanced by 4 % over the SS at 181 km, relative to 13 km resolution. As Fs is a key parameter in estimating surface NO2 concentration from satellite-retrieved Ω (Lamsal et al., 2008; Cooper et al., 2020), directly applying the simulated NO2 vertical profiles will propagate such resolution-dependent biases that also vary regionally and seasonally, as indicated in Fig. 6.
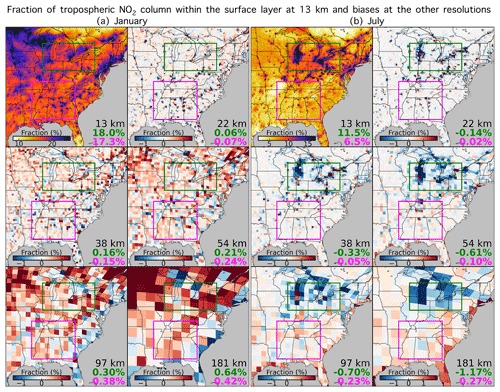
Figure 6Coarse-resolution simulations yield variable biases in satellite-based estimations of surface NO2 concentration. Panels (a) and (b) are both similar to Fig. 1c (for January and July 2015, respectively) but for the fraction (%) of NO2 tropospheric column within the surface layer during afternoon satellite overpass time (19:00–21:00 UTC). The numbers for the other resolutions vs. 13 km resolution are absolute differences in percentage number.
Figure 7 shows the simulated tropospheric NO2 vertical column density (Ω) and its resolution dependence during LEO afternoon overpass time, and Fig. A5 shows the corresponding slant column density (Ωs) after applying TROPOMI scattering weights. Relative to the surface biases (Fig. 1c), the Ω and Ωs differences show stronger regional uniformity, revealing overall increasingly negative biases with increasing grid size over both seasons and regions. For July and over the SS, the afternoon columnar biases are reversed to be negative compared to the positive surface biases (e.g., Fig. 7 vs. Fig. 1c), driven by the reversed responses at higher altitudes (Fig. 4). This remarkable reversal of Ω biases (negative) vs. surface biases (positive) reinforces the need to include vertical profile information to correctly simulate NOx columnar properties. Ω and Ωs exhibit quantitatively consistent resolution dependence and spatial distribution (Fig. 7 vs. Fig. A5), indicating that the changes in vertical profile do not significantly alter the resolution dependence of satellite-observed columnar abundances compared to the direct effects from changing τ. Assuming NOx emissions are locally related to NO2 columns at coarse resolution will result in similar magnitudes of overprediction (up to 8 % and 4 % over the GL and SS, respectively, in July) of derived NOx emissions to compensate the underestimated Ω to emission relationship under an inverse modeling framework configured at each resolution, as shown in Figs. 7 and A5.
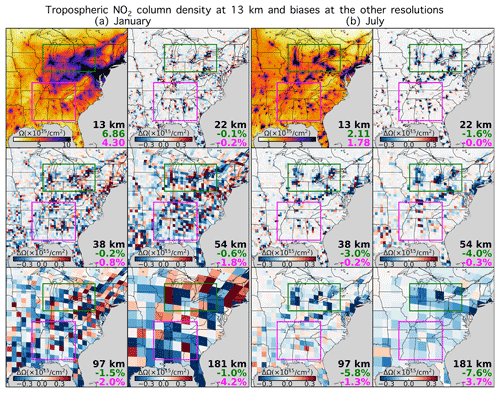
Figure 7Coarse-resolution simulations yield positive biases in spaceborne inverse modeling of NOx emissions. Panels (a) and (b) are both similar to Fig. 1c (for January and July 2015, respectively) but for the mean GEOS-Chem tropospheric NO2 column density (in molec. cm−2) during afternoon satellite overpass time (19:00–21:00 UTC).
The strong, and more importantly regionally variable, NOx resolution dependences that we find in the lower troposphere over the contemporary eastern US warrant care in interpreting coarse-resolution NOx simulations. We find that resolution-dependent NOx biases are particularly pronounced at the surface in summer, with variable effects seasonally, regionally, diurnally and vertically, which also affect remote sensing observations interpreted with low-resolution simulations. At daytime with strong photochemistry, higher-resolution modeling more realistically concentrates NOx emissions near sources, thus decreasing τ in the NOx-limited regime and increasing τ in the NOx-saturated regime (Fig. A1). Existing literature about NOx resolution dependencies in box models (Valin et al., 2011), at power plants (Sillman et al., 1990), in ship plumes (Charlton-Perez et al., 2009) and in CTMs (Wild and Prather, 2006; Yamaji et al., 2014; Yan et al., 2016) primarily discusses the NOx-saturated regimes. We find limited prior literature about the positive biases of NOx over weak sources (i.e., in the NOx-limited regime over the SS) in CTM simulations. The lack of similar prior reports reflects the chemical regime transition occurring in the last ∼ 10 years, while typical point sources were previously predominantly in the NOx-saturated regime. Attention to the NOx-limited regime and its corresponding resolution effects is timely given declining NOx emissions across the US with NOx emission regulations. At the same time, the joint sensitivity to NOx heterogeneity and concurrent VOC level (Sect. 3.1) in the NOx-limited regime will continue complicating its predictability, since NOx and VOCs can have various spatial co-variabilities (e.g., positively correlated where transportation-relevant VOCs and NOx both dominate) and regime-dependent effects on τ. Therefore, accurately capturing such regime differences and transitions from CTM requires not only accurate emission inventories of NOx and VOC but also simulations at representative spatial scales (e.g., 10 km or finer) that correctly distribute these emissions (Valin et al., 2011).
We found systematic resolution effects of nighttime NO–O3 titration efficiency that can drive the NOx biases over winter (Fig. 2 and Sect. 3.2), as the anti-correlation between NO and O3 implies faster reaction rates at coarser resolutions. In air quality modeling, many key reactions involve spatially correlated (e.g., co-emitted SO2 and NO2 cause severe urban haze (Wang et al., 2020)) or segregated species (e.g., agricultural NH3 and NOx-formed HNO3 for nitrate aerosol partitioning (Gu et al., 2021)). Like in this study, the segregated species will consume precursors and produce products more efficiently at coarser resolutions, while colocated sources will experience opposite effects. Interpreting the evolution of relevant species and air pollution processes using a CTM is therefore also preferable at the spatial scales that are representative of these sources. This effect should also be taken into account when CTMs are simulated on coarser scales.
Our detailed simulation of resolution effects at different altitudes (Figs. 4 and 5) revealed vertically variable sensitivity of NOx to its chemical loss at different spatial resolutions. These findings significantly enriched the understanding of resolution dependency of satellite columnar observations, which is in contrast to previous studies that neglected vertical layering. The example of the opposite resolution effect of Ω and surface NOx (Fig. 7 vs. Fig. 1c) over the SS in July highlights the necessity of realistic vertical profiling of NOx and its chemical sinks. For two conventional applications of satellite-retrieved Ω, namely estimation of surface NO2 concentration and constraining NOx emissions, we found that regionally and seasonally varying biases at the level of ∼ 10 % due to adopting coarse model simulations (∼ 200 km) are inevitable.
Overall, we conducted a comprehensive novel evaluation of NOx resolution dependence using a CTM across a wide range of resolutions (13–181 km) and scenarios (including nighttime, winter and higher altitudes). We found the strongest resolution effects in the summer and during daytime (e.g., −16 % for surface NOx and −8 % for columnar NO2 over the GL) where and when the NOx spatial heterogeneity is the strongest and its lifetime is the shortest (e.g., Fig. S2). We attribute these systematic simulation biases mainly to the strong localization of NOx emission and chemistry at a spatial scale of ∼ 10 km and below. Additional modulations from other factors across resolutions, such as sub-grid meteorology-relevant processes (e.g., transport) are also possible but are fully coupled with the feedbacks revealed in this paper and are non-trivial to disentangle. These systematic resolution dependences should be considered when constraining model parameters (e.g., emissions, reaction yields, removal rates) using ground- or satellite-based observations. In other words, relevant interpretations and conclusions by coarse-model simulations in previous studies are worth revisiting. For example, NOx sources and sinks constrained by matching inadequately coarse simulations with observations could be biased to compensate for the intrinsic model errors discussed here.
Although this study exploited state-of-science capabilities, biases with respect to resolutions finer than 13 km resolution likely exist considering the highly localized NOx, especially in summer (Valin et al., 2011; Larkin et al., 2017; Beirle et al., 2019). Following NOx regulations in the US, the magnitudes of resolution effects are expected to continue decreasing as the enhancements over sources reduce relative to the background NOx level (Russell et al., 2012; Jin et al., 2020; Qu et al., 2021), and the requirements regarding resolution may diminish (e.g., partially reflected by the smaller effects over the SS relative to over the GL). Nonetheless, over developing areas where current NOx emissions are stronger or are projected to increase, the resolution effects will be exacerbated, and applying finer-resolution simulations to accurately capture NOx lifetime and budgets will be increasingly critical for air quality modeling applications. Optimization of an appropriate resolution that can capture the relevant processes accurately for specific applications given computational resource constraints is also of great interest. GCHP offers this global high-resolution simulation capability and also the opportunity to expand this analysis into a more comprehensive understanding of the global resolution dependence of NOx and its nonlinear chemistry.
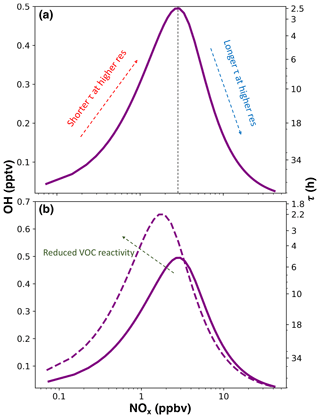
Figure A1(a) Illustration of the daytime NOx–OH lifetime feedback. Higher-resolution modeling tends to concentrate the most NOx emissions near sources, thus will decrease NOx lifetime in the NOx-limited regime (left) and increase NOx lifetime in the NOx-saturated regime (right). The steady-state concentrations were calculated assuming a ratio of 4, an alkyl nitrate branching ratio of 0.04, a VOC reactivity of 3 s−1 and an initial ozone concentration of 25 ppb, and the production of HOx is proportional to ozone. Panel (b) is the same as (a) but including an additional scenario where the VOC reactivity is reduced by 50 % (dashed).
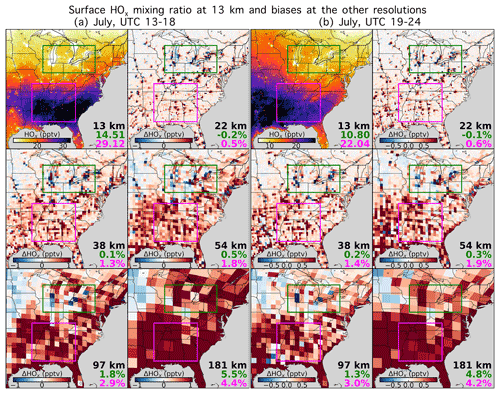
Figure A2Similar to Fig. 1c but for HOx in the morning (a 13:00–18:00 UTC or 07:00–12:00 CST) and afternoon (b 19:00–24:00 UTC or 13:00–18:00 CST), respectively.
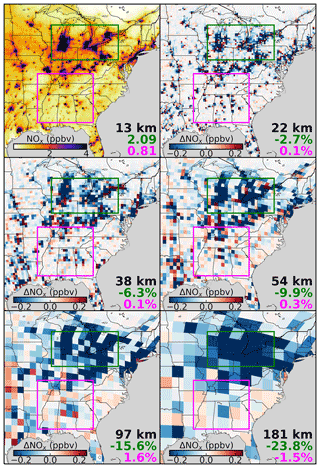
Figure A3Similar to Fig. 1c but for morning (13:00–18:00 UTC or 07:00–12:00 CST) NOx.
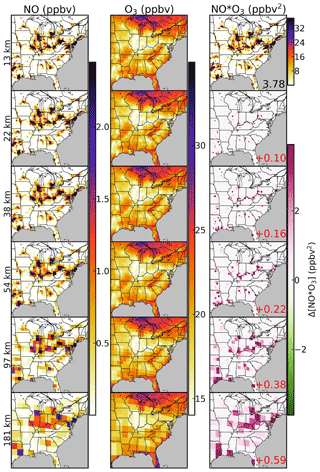
Figure A4Illustration of the resolution-dependent nighttime NO–O3 titration efficiency. The left and middle columns are mean surface NO and O3 concentration at 00:00 UTC+6 in January 2015 at 13 km (top row) and regridded to the other resolutions. The right column shows their products at 13 km resolution and their differences if calculated at the other coarser resolutions. Domain mean products and differences are shown as an inset.
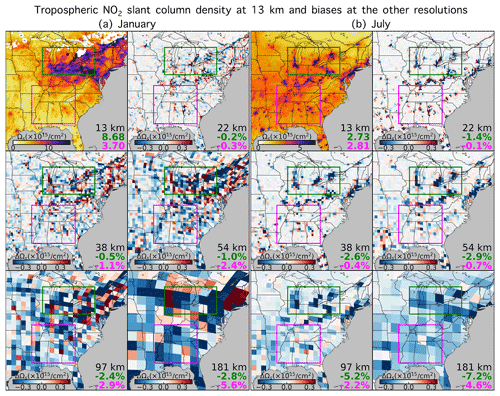
Figure A5Similar to Fig. 7 but for slant column density (Ωs, molec. cm−2) calculated from GEOS-Chem NO2 mixing ratios and scattering weights corresponding to TROPOMI observations. The small blank (white) areas in January have no available TROPOMI retrievals under clear-sky and snow-free conditions.
GEOS-Chem 13.2.1, including GCHP, is available for download at https://doi.org/10.5281/zenodo.5500718 (The International GEOS-Chem User Community, 2021). TROPOMI NO2 data are available from https://tropomi.gesdisc.eosdis.nasa.gov/data/S5P_TROPOMI_Level2 (NASA Goddard Earth Sciences Data and Information Services Center, 2020). The hourly model output for the 2 months and six resolutions used in this study are available upon request to the corresponding author (chili@wustl.edu; lynchlee90@gmail.com).
The supplement related to this article is available online at: https://doi.org/10.5194/acp-23-3031-2023-supplement.
The manuscript was written using contributions from all authors. The conceptualization was initialized by CL, RVM and RCC. The methodology was developed by CL, LB and DZ. DC processed the satellite scattering weights. HW and JL conducted the offline emission calculation. CL performed the model simulations, visualization and analysis of the results. CL wrote the original draft. All authors have reviewed, edited and given approval to the final version of the manuscript.
At least one of the (co-)authors is a member of the editorial board of Atmospheric Chemistry and Physics. The peer-review process was guided by an independent editor, and the authors also have no other competing interests to declare.
Publisher’s note: Copernicus Publications remains neutral with regard to jurisdictional claims in published maps and institutional affiliations.
This work has been supported by the NASA AIST (80NSSC20K0281) and ACCDAM (80NSSC21K1343) programs. We thank the GEOS-Chem support team for maintaining the model used in this work.
This research has been supported by the National Aeronautics and Space Administration (grant nos. 80NSSC20K0281 and 80NSSC21K1343).
This paper was edited by Thomas von Clarmann and reviewed by two anonymous referees.
Beirle, S., Boersma, K. F., Platt, U., Lawrence, M. G., and Wagner, T.: Megacity Emissions and Lifetimes of Nitrogen Oxides Probed from Space, Science, 333, 1737–1739, https://doi.org/10.1126/science.1207824, 2011. a
Beirle, S., Borger, C., Dörner, S., Li, A., Hu, Z., Liu, F., Wang, Y., and Wagner, T.: Pinpointing nitrogen oxide emissions from space, Science Advances, 5, eaax9800, https://doi.org/10.1126/sciadv.aax9800, 2019. a, b
Bey, I., Jacob, D. J., Yantosca, R. M., Logan, J. A., Field, B. D., Fiore, A. M., Li, Q., Liu, H. Y., Mickley, L. J., and Schultz, M. G.: Global modeling of tropospheric chemistry with assimilated meteorology: Model description and evaluation, J. Geophys. Res.-Atmos., 106, 23073–23095, 2001. a
Bindle, L., Martin, R. V., Cooper, M. J., Lundgren, E. W., Eastham, S. D., Auer, B. M., Clune, T. L., Weng, H., Lin, J., Murray, L. T., Meng, J., Keller, C. A., Putman, W. M., Pawson, S., and Jacob, D. J.: Grid-stretching capability for the GEOS-Chem 13.0.0 atmospheric chemistry model, Geosci. Model Dev., 14, 5977–5997, https://doi.org/10.5194/gmd-14-5977-2021, 2021. a, b, c, d
Brown, S. S. and Stutz, J.: Nighttime radical observations and chemistry, Chem. Soc. Rev., 41, 6405–6447, https://doi.org/10.1039/C2CS35181A, 2012. a, b
Brown, S. S., Neuman, J., Ryerson, T., Trainer, M., Dubé, W., Holloway, J., Warneke, C., De Gouw, J., Donnelly, S., and Atlas, E.: Nocturnal odd‐oxygen budget and its implications for ozone loss in the lower troposphere, Geophys. Res. Lett., 33, L08801, https://doi.org/10.1029/2006GL025900, 2006. a, b
Browne, E. C. and Cohen, R. C.: Effects of biogenic nitrate chemistry on the NOx lifetime in remote continental regions, Atmos. Chem. Phys., 12, 11917–11932, https://doi.org/10.5194/acp-12-11917-2012, 2012. a, b
Charlton-Perez, C. L., Evans, M. J., Marsham, J. H., and Esler, J. G.: The impact of resolution on ship plume simulations with NOx chemistry, Atmos. Chem. Phys., 9, 7505–7518, https://doi.org/10.5194/acp-9-7505-2009, 2009. a, b, c
Cooper, M., Martin, R. V., Padmanabhan, A., and Henze, D. K.: Comparing mass balance and adjoint methods for inverse modeling of nitrogen dioxide columns for global nitrogen oxide emissions, J. Geophys. Res.-Atmos., 122, 4718–4734, 2017. a
Cooper, M. J., Martin, R. V., McLinden, C. A., and Brook, J. R.: Inferring ground-level nitrogen dioxide concentrations at fine spatial resolution applied to the TROPOMI satellite instrument, Environ. Res. Lett., 15, 104013, https://doi.org/10.1088/1748-9326/aba3a5, 2020. a, b
Crippa, M., Guizzardi, D., Muntean, M., Schaaf, E., Dentener, F., van Aardenne, J. A., Monni, S., Doering, U., Olivier, J. G. J., Pagliari, V., and Janssens-Maenhout, G.: Gridded emissions of air pollutants for the period 1970–2012 within EDGAR v4.3.2, Earth Syst. Sci. Data, 10, 1987–2013, https://doi.org/10.5194/essd-10-1987-2018, 2018. a
Crippa, M., Guizzardi, D., Muntean, M., and Schaaf, E.: EDGAR v5.0 Global Air Pollutant Emissions, Joint Research Centre (JRC) [data set], http://data.europa.eu/89h/377801af-b094-4943-8fdc-f79a7c0c2d19, last access: December 2021. a
Eastham, S. D., Long, M. S., Keller, C. A., Lundgren, E., Yantosca, R. M., Zhuang, J., Li, C., Lee, C. J., Yannetti, M., Auer, B. M., Clune, T. L., Kouatchou, J., Putman, W. M., Thompson, M. A., Trayanov, A. L., Molod, A. M., Martin, R. V., and Jacob, D. J.: GEOS-Chem High Performance (GCHP v11-02c): a next-generation implementation of the GEOS-Chem chemical transport model for massively parallel applications, Geosci. Model Dev., 11, 2941–2953, https://doi.org/10.5194/gmd-11-2941-2018, 2018. a
Edwards, P. M., Brown, S. S., Roberts, J. M., Ahmadov, R., Banta, R. M., deGouw, J. A., Dubé, W. P., Field, R. A., Flynn, J. H., Gilman, J. B., Graus, M., Helmig, D., Koss, A., Langford, A. O., Lefer, B. L., Lerner, B. M., Li, R., Li, S.-M., McKeen, S. A., Murphy, S. M., Parrish, D. D., Senff, C. J., Soltis, J., Stutz, J., Sweeney, C., Thompson, C. R., Trainer, M. K., Tsai, C., Veres, P. R., Washenfelder, R. A., Warneke, C., Wild, R. J., Young, C. J., Yuan, B., and Zamora, R.: High winter ozone pollution from carbonyl photolysis in an oil and gas basin, Nature, 514, 351–354, https://doi.org/10.1038/nature13767, 2014. a
Evans, M. and Jacob, D. J.: Impact of new laboratory studies of N2O5 hydrolysis on global model budgets of tropospheric nitrogen oxides, ozone, and OH, Geophys. Res. Lett., 32, L09813, https://doi.org/10.1029/2005GL022469, 2005. a
Goldberg, D. L., Lu, Z., Streets, D. G., de Foy, B., Griffin, D., McLinden, C. A., Lamsal, L. N., Krotkov, N. A., and Eskes, H.: Enhanced Capabilities of TROPOMI NO2: Estimating NOx from North American Cities and Power Plants, Environ. Sci. Technol., 53, 12594–12601, https://doi.org/10.1021/acs.est.9b04488, 2019. a
Gu, B., Zhang, L., Van Dingenen, R., Vieno, M., Van Grinsven, H. J., Zhang, X., Zhang, S., Chen, Y., Wang, S., Ren, C., Rao, S., Holland, M., Winiwarter, W., Chen, D., Xu, J., and Sutton, M. A.: Abating ammonia is more cost-effective than nitrogen oxides for mitigating PM2.5 air pollution, Science, 374, 758–762, https://doi.org/10.1126/science.abf8623, 2021. a, b
Heue, K.-P., Wagner, T., Broccardo, S. P., Walter, D., Piketh, S. J., Ross, K. E., Beirle, S., and Platt, U.: Direct observation of two dimensional trace gas distributions with an airborne Imaging DOAS instrument, Atmos. Chem. Phys., 8, 6707–6717, https://doi.org/10.5194/acp-8-6707-2008, 2008. a
Hoesly, R. M., Smith, S. J., Feng, L., Klimont, Z., Janssens-Maenhout, G., Pitkanen, T., Seibert, J. J., Vu, L., Andres, R. J., Bolt, R. M., Bond, T. C., Dawidowski, L., Kholod, N., Kurokawa, J.-I., Li, M., Liu, L., Lu, Z., Moura, M. C. P., O'Rourke, P. R., and Zhang, Q.: Historical (1750–2014) anthropogenic emissions of reactive gases and aerosols from the Community Emissions Data System (CEDS), Geosci. Model Dev., 11, 369–408, https://doi.org/10.5194/gmd-11-369-2018, 2018. a
Holmes, C. D., Bertram, T. H., Confer, K. L., Graham, K. A., Ronan, A. C., Wirks, C. K., and Shah, V.: The role of clouds in the tropospheric NOx cycle: A new modeling approach for cloud chemistry and its global implications, Geophys. Res. Lett., 46, 4980–4990, 2019. a
Jacob, D. J., Horowitz, L. W., Munger, J. W., Heikes, B. G., Dickerson, R. R., Artz, R. S., and Keene, W. C.: Seasonal transition from NOx‐to hydrocarbon‐limited conditions for ozone production over the eastern United States in September, J. Geophys. Res.-Atmos., 100, 9315–9324, 1995. a
Jin, X., Fiore, A. M., Murray, L. T., Valin, L. C., Lamsal, L. N., Duncan, B., Folkert Boersma, K., De Smedt, I., Abad, G. G., and Chance, K.: Evaluating a space‐based indicator of surface ozone‐NOx‐VOC sensitivity over midlatitude source regions and application to decadal trends, J. Geophys. Res.-Atmos., 122, 10–439, 2017. a
Jin, X., Fiore, A., Boersma, K. F., Smedt, I. D., and Valin, L.: Inferring Changes in Summertime Surface Ozone–NOx–VOC Chemistry over U.S. Urban Areas from Two Decades of Satellite and Ground-Based Observations, Environ. Sci. Technol., 54, 6518–6529, https://doi.org/10.1021/acs.est.9b07785, 2020. a, b, c, d, e
Jung, J., Choi, Y., Mousavinezhad, S., Kang, D., Park, J., Pouyaei, A., Ghahremanloo, M., Momeni, M., and Kim, H.: Changes in the ozone chemical regime over the contiguous United States inferred by the inversion of NOx and VOC emissions using satellite observation, Atmos. Res., 270, 106076, https://doi.org/10.1016/j.atmosres.2022.106076, 2022. a
Keller, C. A., Long, M. S., Yantosca, R. M., Da Silva, A. M., Pawson, S., and Jacob, D. J.: HEMCO v1.0: a versatile, ESMF-compliant component for calculating emissions in atmospheric models, Geosci. Model Dev., 7, 1409–1417, https://doi.org/10.5194/gmd-7-1409-2014, 2014. a
Kenagy, H. S., Sparks, T. L., Ebben, C. J., Wooldrige, P. J., Lopez‐Hilfiker, F. D., Lee, B. H., Thornton, J. A., McDuffie, E. E., Fibiger, D. L., and Brown, S. S.: NOx lifetime and NOy partitioning during WINTER, J. Geophys. Res.-Atmos., 123, 9813–9827, 2018. a, b
Kim, J., Jeong, U., Ahn, M.-H., Kim, J. H., Park, R. J., Lee, H., Song, C. H., Choi, Y.-S., Lee, K.-H., Yoo, J.-M., Jeong, M.-J., Park, S. K., Lee, K.-M., Song, C.-K., Kim, S.-W., Kim, Y. J., Kim, S.-W., Kim, M., Go, S., Liu, X., Chance, K., Chan Miller, C., Al-Saadi, J., Veihelmann, B., Bhartia, P. K., Torres, O., Abad, G. G., Haffner, D. P., Ko, D. H., Lee, S. H., Woo, J.-H., Chong, H., Park, S. S., Nicks, D., Choi, W. J., Moon, K.-J., Cho, A., Yoon, J., Kim, S.-k., Hong, H., Lee, K., Lee, H., Lee, S., Choi, M., Veefkind, P., Levelt, P. F., Edwards, D. P., Kang, M., Eo, M., Bak, J., Baek, K., Kwon, H.-A., Yang, J., Park, J., Han, K. M., Kim, B.-R., Shin, H.-W., Choi, H., Lee, E., Chong, J., Cha, Y., Koo, J.-H., Irie, H., Hayashida, S., Kasai, Y., Kanaya, Y., Liu, C., Lin, J., Crawford, J. H., Carmichael, G. R., Newchurch, M. J., Lefer, B. L., Herman, J. R., Swap, R. J., Lau, A. K. H., Kurosu, T. P., Jaross, G., Ahlers, B., Dobber, M., McElroy, C. T., and Choi, Y.: New Era of Air Quality Monitoring from Space: Geostationary Environment Monitoring Spectrometer (GEMS), B. Am. Meteorol. Soc., 101, E1–E22, https://doi.org/10.1175/BAMS-D-18-0013.1, 2020. a
Koplitz, S., Simon, H., Henderson, B., Liljegren, J., Tonnesen, G., Whitehill, A., and Wells, B.: Changes in Ozone Chemical Sensitivity in the United States from 2007 to 2016, ACS Environ. Au, 2, 206–222, https://doi.org/10.1021/acsenvironau.1c00029, 2021. a, b
Koplitz, S. N., Mickley, L. J., Marlier, M. E., Buonocore, J. J., Kim, P. S., Liu, T., Sulprizio, M. P., DeFries, R. S., Jacob, D. J., Schwartz, J., Pongsiri, M., and Myers, S. S.: Public health impacts of the severe haze in Equatorial Asia in September–October 2015: demonstration of a new framework for informing fire management strategies to reduce downwind smoke exposure, Environ. Res. Lett., 11, 094023, https://doi.org/10.1088/1748-9326/11/9/094023, 2016. a
Lamsal, L., Martin, R., Van Donkelaar, A., Steinbacher, M., Celarier, E., Bucsela, E., Dunlea, E., and Pinto, J.: Ground‐level nitrogen dioxide concentrations inferred from the satellite‐borne Ozone Monitoring Instrument, J. Geophys. Res.-Atmos., 113, D16308, https://doi.org/10.1029/2007JD009235, 2008. a
Larkin, A., Geddes, J. A., Martin, R. V., Xiao, Q., Liu, Y., Marshall, J. D., Brauer, M., and Hystad, P.: Global Land Use Regression Model for Nitrogen Dioxide Air Pollution, Environ. Sci. Technol., 51, 6957–6964, https://doi.org/10.1021/acs.est.7b01148, 2017. a
Laughner, J. L. and Cohen, R. C.: Direct observation of changing NOx lifetime in North American cities, Science, 366, 723–727, https://doi.org/10.1126/science.aax6832, 2019. a, b, c, d, e, f, g, h
Levelt, P. F., Joiner, J., Tamminen, J., Veefkind, J. P., Bhartia, P. K., Stein Zweers, D. C., Duncan, B. N., Streets, D. G., Eskes, H., van der A, R., McLinden, C., Fioletov, V., Carn, S., de Laat, J., DeLand, M., Marchenko, S., McPeters, R., Ziemke, J., Fu, D., Liu, X., Pickering, K., Apituley, A., González Abad, G., Arola, A., Boersma, F., Chan Miller, C., Chance, K., de Graaf, M., Hakkarainen, J., Hassinen, S., Ialongo, I., Kleipool, Q., Krotkov, N., Li, C., Lamsal, L., Newman, P., Nowlan, C., Suleiman, R., Tilstra, L. G., Torres, O., Wang, H., and Wargan, K.: The Ozone Monitoring Instrument: overview of 14 years in space, Atmos. Chem. Phys., 18, 5699–5745, https://doi.org/10.5194/acp-18-5699-2018, 2018. a
Li, C., Martin, R. V., van Donkelaar, A., Boys, B. L., Hammer, M. S., Xu, J.-W., Marais, E. A., Reff, A., Strum, M., Ridley, D. A., Crippa, M., Brauer, M., and Zhang, Q.: Trends in Chemical Composition of Global and Regional Population-Weighted Fine Particulate Matter Estimated for 25 Years, Environ. Sci. Technol., 51, 11185–11195, https://doi.org/10.1021/acs.est.7b02530, 2017. a
Li, C., Zhu, Q., Jin, X., and Cohen, R. C.: Elucidating Contributions of Anthropogenic Volatile Organic Compounds and Particulate Matter to Ozone Trends over China, Environ. Sci. Technol., 56, 12906–12916, https://doi.org/10.1021/acs.est.2c03315, 2022. a
Lin, H., Jacob, D. J., Lundgren, E. W., Sulprizio, M. P., Keller, C. A., Fritz, T. M., Eastham, S. D., Emmons, L. K., Campbell, P. C., Baker, B., Saylor, R. D., and Montuoro, R.: Harmonized Emissions Component (HEMCO) 3.0 as a versatile emissions component for atmospheric models: application in the GEOS-Chem, NASA GEOS, WRF-GC, CESM2, NOAA GEFS-Aerosol, and NOAA UFS models, Geosci. Model Dev., 14, 5487–5506, https://doi.org/10.5194/gmd-14-5487-2021, 2021. a
Lin, J.-T. and McElroy, M. B.: Impacts of boundary layer mixing on pollutant vertical profiles in the lower troposphere: Implications to satellite remote sensing, Atmos. Environ., 44, 1726–1739, https://doi.org/10.1016/j.atmosenv.2010.02.009, 2010. a
Long, M. S., Yantosca, R., Nielsen, J. E., Keller, C. A., da Silva, A., Sulprizio, M. P., Pawson, S., and Jacob, D. J.: Development of a grid-independent GEOS-Chem chemical transport model (v9-02) as an atmospheric chemistry module for Earth system models, Geosci. Model Dev., 8, 595–602, https://doi.org/10.5194/gmd-8-595-2015, 2015. a
Lu, Z., Streets, D. G., de Foy, B., Lamsal, L. N., Duncan, B. N., and Xing, J.: Emissions of nitrogen oxides from US urban areas: estimation from Ozone Monitoring Instrument retrievals for 2005–2014, Atmos. Chem. Phys., 15, 10367–10383, https://doi.org/10.5194/acp-15-10367-2015, 2015. a
Ma, X., Tan, Z., Lu, K., Yang, X., Chen, X., Wang, H., Chen, S., Fang, X., Li, S., Li, X., Liu, J., Liu, Y., Lou, S., Qiu, W., Wang, H., Zeng, L., and Zhang, Y.: OH and HO2 radical chemistry at a suburban site during the EXPLORE-YRD campaign in 2018, Atmos. Chem. Phys., 22, 7005–7028, https://doi.org/10.5194/acp-22-7005-2022, 2022. a
Mao, J., Paulot, F., Jacob, D. J., Cohen, R. C., Crounse, J. D., Wennberg, P. O., Keller, C. A., Hudman, R. C., Barkley, M. P., and Horowitz, L. W.: Ozone and organic nitrates over the eastern United States: Sensitivity to isoprene chemistry, J. Geophys. Res.-Atmos., 118, 11256–11268, https://doi.org/10.1002/jgrd.50817, 2013. a
Martin, R. V., Sioris, C. E., Chance, K., Ryerson, T. B., Bertram, T. H., Wooldridge, P. J., Cohen, R. C., Neuman, J. A., Swanson, A., and Flocke, F. M.: Evaluation of space‐based constraints on global nitrogen oxide emissions with regional aircraft measurements over and downwind of eastern North America, J. Geophys. Res.-Atmos., 111, D15308, https://doi.org/10.1029/2005JD006680, 2006. a
Martin, R. V., Eastham, S. D., Bindle, L., Lundgren, E. W., Clune, T. L., Keller, C. A., Downs, W., Zhang, D., Lucchesi, R. A., Sulprizio, M. P., Yantosca, R. M., Li, Y., Estrada, L., Putman, W. M., Auer, B. M., Trayanov, A. L., Pawson, S., and Jacob, D. J.: Improved advection, resolution, performance, and community access in the new generation (version 13) of the high-performance GEOS-Chem global atmospheric chemistry model (GCHP), Geosci. Model Dev., 15, 8731–8748, https://doi.org/10.5194/gmd-15-8731-2022, 2022. a
McDuffie, E. E., Fibiger, D. L., Dubé, W. P., Lopez‐Hilfiker, F., Lee, B. H., Thornton, J. A., Shah, V., Jaeglé, L., Guo, H., and Weber, R. J.: Heterogeneous N2O5 uptake during winter: Aircraft measurements during the 2015 WINTER campaign and critical evaluation of current parameterizations, J. Geophys. Res.-Atmos., 123, 4345–4372, 2018. a
Meng, J., Martin, R. V., Ginoux, P., Hammer, M., Sulprizio, M. P., Ridley, D. A., and van Donkelaar, A.: Grid-independent high-resolution dust emissions (v1.0) for chemical transport models: application to GEOS-Chem (12.5.0), Geosci. Model Dev., 14, 4249–4260, https://doi.org/10.5194/gmd-14-4249-2021, 2021. a
Miyazaki, K., Eskes, H., Sudo, K., Boersma, K. F., Bowman, K., and Kanaya, Y.: Decadal changes in global surface NOx emissions from multi-constituent satellite data assimilation, Atmos. Chem. Phys., 17, 807–837, https://doi.org/10.5194/acp-17-807-2017, 2017. a
Murray, L. T., Jacob, D. J., Logan, J. A., Hudman, R. C., and Koshak, W. J.: Optimized regional and interannual variability of lightning in a global chemical transport model constrained by LIS/OTD satellite data, J. Geophys. Res.-Atmos., 117, D20307, https://doi.org/10.1029/2012JD017934, 2012. a, b
NASA Goddard Earth Sciences (GES) Data and Information Services Center (DISC): Copernicus Sentinel-5P TROPOMI Version 2 Level-2 Products, NASA [data set], https://tropomi.gesdisc.eosdis.nasa.gov/data/S5P_TROPOMI_Level2 (last access: February 2022), 2020. a
Palmer, P. I., Jacob, D. J., Chance, K., Martin, R. V., Spurr, R. J. D., Kurosu, T. P., Bey, I., Yantosca, R., Fiore, A., and Li, Q.: Air mass factor formulation for spectroscopic measurements from satellites: Application to formaldehyde retrievals from the Global Ozone Monitoring Experiment, J. Geophys. Res.-Atmos., 106, 14539–14550, https://doi.org/10.1029/2000JD900772, 2001. a, b
Pan, S., Choi, Y., Roy, A., and Jeon, W.: Allocating emissions to 4 km and 1 km horizontal spatial resolutions and its impact on simulated NOx and O3 in Houston, TX, Atmos. Environ., 164, 398–415, https://doi.org/10.1016/j.atmosenv.2017.06.026, 2017. a
Perring, A. E., Pusede, S. E., and Cohen, R. C.: An Observational Perspective on the Atmospheric Impacts of Alkyl and Multifunctional Nitrates on Ozone and Secondary Organic Aerosol, Chem. Rev., 113, 5848–5870, https://doi.org/10.1021/cr300520x, 2013. a
Philip, S., Martin, R. V., and Keller, C. A.: Sensitivity of chemistry-transport model simulations to the duration of chemical and transport operators: a case study with GEOS-Chem v10-01, Geosci. Model Dev., 9, 1683–1695, https://doi.org/10.5194/gmd-9-1683-2016, 2016. a
Pusede, S. E., Steiner, A. L., and Cohen, R. C.: Temperature and Recent Trends in the Chemistry of Continental Surface Ozone, Chem. Rev., 115, 3898–3918, https://doi.org/10.1021/cr5006815, 2015. a
Putman, W. M. and Lin, S.-J.: Finite-volume transport on various cubed-sphere grids, J. Comput. Phys., 227, 55–78, https://doi.org/10.1016/j.jcp.2007.07.022, 2007. a
Qu, Z., Jacob, D. J., Silvern, R. F., Shah, V., Campbell, P. C., Valin, L. C., and Murray, L. T.: US COVID‐19 shutdown demonstrates importance of background NO2 in inferring NOx emissions from satellite NO2 observations, Geophys. Res. Lett., 48, e2021GL092783, https://doi.org/10.1029/2021GL092783, 2021. a
Ren, X., Harder, H., Martinez, M., Lesher, R. L., Oliger, A., Simpas, J. B., Brune, W. H., Schwab, J. J., Demerjian, K. L., He, Y., Zhou, X., and Gao, H.: OH and HO2 Chemistry in the urban atmosphere of New York City, Atmos. Environ., 37, 3639–3651, https://doi.org/10.1016/S1352-2310(03)00459-X, 2003. a
Rollins, A. W., Browne, E. C., Min, K.-E., Pusede, S. E., Wooldridge, P. J., Gentner, D. R., Goldstein, A. H., Liu, S., Day, D. A., Russell, L. M., and Cohen, R. C.: Evidence for NOx Control over Nighttime SOA Formation, Science, 337, 1210–1212, https://doi.org/10.1126/science.1221520, 2012. a
Romer, P. S., Duffey, K. C., Wooldridge, P. J., Allen, H. M., Ayres, B. R., Brown, S. S., Brune, W. H., Crounse, J. D., de Gouw, J., Draper, D. C., Feiner, P. A., Fry, J. L., Goldstein, A. H., Koss, A., Misztal, P. K., Nguyen, T. B., Olson, K., Teng, A. P., Wennberg, P. O., Wild, R. J., Zhang, L., and Cohen, R. C.: The lifetime of nitrogen oxides in an isoprene-dominated forest, Atmos. Chem. Phys., 16, 7623–7637, https://doi.org/10.5194/acp-16-7623-2016, 2016. a, b, c
Romer Present, P. S., Zare, A., and Cohen, R. C.: The changing role of organic nitrates in the removal and transport of NOx, Atmos. Chem. Phys., 20, 267–279, https://doi.org/10.5194/acp-20-267-2020, 2020. a, b
Russell, A. R., Valin, L. C., and Cohen, R. C.: Trends in OMI NO2 observations over the United States: effects of emission control technology and the economic recession, Atmos. Chem. Phys., 12, 12197–12209, https://doi.org/10.5194/acp-12-12197-2012, 2012. a, b
Shah, V., Jacob, D. J., Li, K., Silvern, R. F., Zhai, S., Liu, M., Lin, J., and Zhang, Q.: Effect of changing NOx lifetime on the seasonality and long-term trends of satellite-observed tropospheric NO2 columns over China, Atmos. Chem. Phys., 20, 1483–1495, https://doi.org/10.5194/acp-20-1483-2020, 2020. a, b, c, d
Sherwen, T., Schmidt, J. A., Evans, M. J., Carpenter, L. J., Großmann, K., Eastham, S. D., Jacob, D. J., Dix, B., Koenig, T. K., Sinreich, R., Ortega, I., Volkamer, R., Saiz-Lopez, A., Prados-Roman, C., Mahajan, A. S., and Ordóñez, C.: Global impacts of tropospheric halogens (Cl, Br, I) on oxidants and composition in GEOS-Chem, Atmos. Chem. Phys., 16, 12239–12271, https://doi.org/10.5194/acp-16-12239-2016, 2016. a
Sicard, P., De Marco, A., Agathokleous, E., Feng, Z., Xu, X., Paoletti, E., Rodriguez, J. J. D., and Calatayud, V.: Amplified ozone pollution in cities during the COVID-19 lockdown, Sci. Total Environ., 735, 139542, https://doi.org/10.1016/j.scitotenv.2020.139542, 2020. a
Sillman, S.: The relation between ozone, NOx and hydrocarbons in urban and polluted rural environments, Atmos. Environ., 33, 1821–1845, https://doi.org/10.1016/S1352-2310(98)00345-8, 1999. a
Sillman, S., Logan, J. A., and Wofsy, S. C.: A regional scale model for ozone in the United States with subgrid representation of urban and power plant plumes, J. Geophys. Res.-Atmos., 95, 5731–5748, 1990. a, b
Silvern, R. F., Jacob, D. J., Mickley, L. J., Sulprizio, M. P., Travis, K. R., Marais, E. A., Cohen, R. C., Laughner, J. L., Choi, S., Joiner, J., and Lamsal, L. N.: Using satellite observations of tropospheric NO2 columns to infer long-term trends in US NOx emissions: the importance of accounting for the free tropospheric NO2 background, Atmos. Chem. Phys., 19, 8863–8878, https://doi.org/10.5194/acp-19-8863-2019, 2019. a
Streets, D. G., Canty, T., Carmichael, G. R., de Foy, B., Dickerson, R. R., Duncan, B. N., Edwards, D. P., Haynes, J. A., Henze, D. K., Houyoux, M. R., Jacob, D. J., Krotkov, N. A., Lamsal, L. N., Liu, Y., Lu, Z., Martin, R. V., Pfister, G. G., Pinder, R. W., Salawitch, R. J., and Wecht, K. J.: Emissions estimation from satellite retrievals: A review of current capability, Atmos. Environ., 77, 1011–1042, https://doi.org/10.1016/j.atmosenv.2013.05.051, 2013. a
Thornton, J., Wooldridge, P., Cohen, R., Martinez, M., Harder, H., Brune, W., Williams, E., Roberts, J., Fehsenfeld, F., and Hall, S.: Ozone production rates as a function of NOx abundances and HOx production rates in the Nashville urban plume, J. Geophys. Res.-Atmos., 107, 4146, https://doi.org/10.1029/2001JD000932, 2002. a
Timmermans, R., Segers, A., Curier, L., Abida, R., Attié, J.-L., El Amraoui, L., Eskes, H., de Haan, J., Kujanpää, J., Lahoz, W., Oude Nijhuis, A., Quesada-Ruiz, S., Ricaud, P., Veefkind, P., and Schaap, M.: Impact of synthetic space-borne NO2 observations from the Sentinel-4 and Sentinel-5P missions on tropospheric NO2 analyses , Atmos. Chem. Phys., 19, 12811–12833, https://doi.org/10.5194/acp-19-12811-2019, 2019. a
The International GEOS-Chem User Community: geoschem/GCHP: GCHP 13.2.1 (13.2.1), Zenodo [data set], https://doi.org/10.5281/zenodo.5500718, 2021. a, b
Travis, K. R., Jacob, D. J., Fisher, J. A., Kim, P. S., Marais, E. A., Zhu, L., Yu, K., Miller, C. C., Yantosca, R. M., Sulprizio, M. P., Thompson, A. M., Wennberg, P. O., Crounse, J. D., St. Clair, J. M., Cohen, R. C., Laughner, J. L., Dibb, J. E., Hall, S. R., Ullmann, K., Wolfe, G. M., Pollack, I. B., Peischl, J., Neuman, J. A., and Zhou, X.: Why do models overestimate surface ozone in the Southeast United States?, Atmos. Chem. Phys., 16, 13561–13577, https://doi.org/10.5194/acp-16-13561-2016, 2016. a
Valin, L. C., Russell, A. R., Hudman, R. C., and Cohen, R. C.: Effects of model resolution on the interpretation of satellite NO2 observations, Atmos. Chem. Phys., 11, 11647–11655, https://doi.org/10.5194/acp-11-11647-2011, 2011. a, b, c, d, e, f, g
van der Werf, G. R., Randerson, J. T., Giglio, L., van Leeuwen, T. T., Chen, Y., Rogers, B. M., Mu, M., van Marle, M. J. E., Morton, D. C., Collatz, G. J., Yokelson, R. J., and Kasibhatla, P. S.: Global fire emissions estimates during 1997–2016, Earth Syst. Sci. Data, 9, 697–720, https://doi.org/10.5194/essd-9-697-2017, 2017. a
Veefkind, J., Aben, I., McMullan, K., Förster, H., de Vries, J., Otter, G., Claas, J., Eskes, H., de Haan, J., Kleipool, Q., van Weele, M., Hasekamp, O., Hoogeveen, R., Landgraf, J., Snel, R., Tol, P., Ingmann, P., Voors, R., Kruizinga, B., Vink, R., Visser, H., and Levelt, P.: TROPOMI on the ESA Sentinel-5 Precursor: A GMES mission for global observations of the atmospheric composition for climate, air quality and ozone layer applications, Remote Sens. Environ., 120, 70–83, https://doi.org/10.1016/j.rse.2011.09.027, 2012. a
Wang, J., Li, J., Ye, J., Zhao, J., Wu, Y., Hu, J., Liu, D., Nie, D., Shen, F., Huang, X., Huang, D. D., Ji, D., Sun, X., Xu, W., Guo, J., Song, S., Qin, Y., Liu, P., Turner, J. R., Lee, H. C., Hwang, S., Liao, H., Martin, S. T., Zhang, Q., Chen, M., Sun, Y., Ge, X., and Jacob, D. J.: Fast sulfate formation from oxidation of SO2 by NO2 and HONO observed in Beijing haze, Nat. Commun., 11, 2844, https://doi.org/10.1038/s41467-020-16683-x, 2020. a
Wang, K., Wu, K., Wang, C., Tong, Y., Gao, J., Zuo, P., Zhang, X., and Yue, T.: Identification of NOx hotspots from oversampled TROPOMI NO2 column based on image segmentation method, Sci. Total Environ., 803, 150007, https://doi.org/10.1016/j.scitotenv.2021.150007, 2022. a
Wang, S., Ackermann, R., and Stutz, J.: Vertical profiles of O3 and NOx chemistry in the polluted nocturnal boundary layer in Phoenix, AZ: I. Field observations by long-path DOAS, Atmos. Chem. Phys., 6, 2671–2693, https://doi.org/10.5194/acp-6-2671-2006, 2006. a
Weng, H., Lin, J., Martin, R., Millet, D. B., Jaeglé, L., Ridley, D., Keller, C., Li, C., Du, M., and Meng, J.: Global high-resolution emissions of soil NOx, sea salt aerosols, and biogenic volatile organic compounds, Scientific Data, 7, 148, https://doi.org/10.1038/s41597-020-0488-5, 2020. a
Wild, O. and Prather, M. J.: Global tropospheric ozone modeling: Quantifying errors due to grid resolution, J. Geophys. Res.-Atmos., 111, D11305, https://doi.org/10.1029/2005JD006605, 2006. a
Yamaji, K., Ikeda, K., Irie, H., Kurokawa, J., and Ohara, T.: Influence of model grid resolution on NO2 vertical column densities over East Asia, J. Air Waste Manag. Assoc., 64, 436–444, https://doi.org/10.1080/10962247.2013.827603, 2014. a, b
Yan, Y., Lin, J., Chen, J., and Hu, L.: Improved simulation of tropospheric ozone by a global-multi-regional two-way coupling model system, Atmos. Chem. Phys., 16, 2381–2400, https://doi.org/10.5194/acp-16-2381-2016, 2016. a, b, c
Yan, Y., Lin, J., and He, C.: Ozone trends over the United States at different times of day, Atmos. Chem. Phys., 18, 1185–1202, https://doi.org/10.5194/acp-18-1185-2018, 2018. a
Yu, K., Jacob, D. J., Fisher, J. A., Kim, P. S., Marais, E. A., Miller, C. C., Travis, K. R., Zhu, L., Yantosca, R. M., Sulprizio, M. P., Cohen, R. C., Dibb, J. E., Fried, A., Mikoviny, T., Ryerson, T. B., Wennberg, P. O., and Wisthaler, A.: Sensitivity to grid resolution in the ability of a chemical transport model to simulate observed oxidant chemistry under high-isoprene conditions, Atmos. Chem. Phys., 16, 4369–4378, https://doi.org/10.5194/acp-16-4369-2016, 2016. a, b, c
Zakoura, M. and Pandis, S.: Overprediction of aerosol nitrate by chemical transport models: The role of grid resolution, Atmos. Environ., 187, 390–400, https://doi.org/10.1016/j.atmosenv.2018.05.066, 2018. a, b, c
Zhang, R., Lei, W., Tie, X., and Hess, P.: Industrial emissions cause extreme urban ozone diurnal variability, P. Natl. Acad. Sci. USA, 101, 6346–6350, https://doi.org/10.1073/pnas.0401484101, 2004. a, b, c
Zhu, Q., Laughner, J. L., and Cohen, R. C.: Lightning NO2 simulation over the contiguous US and its effects on satellite NO2 retrievals, Atmos. Chem. Phys., 19, 13067–13078, https://doi.org/10.5194/acp-19-13067-2019, 2019. a
Zhu, Q., Laughner, J. L., and Cohen, R. C.: Estimate of OH trends over one decade in North American cities, P. Natl. Acad. Sci. USA, 119, e2117399119, https://doi.org/10.1073/pnas.2117399119, 2022. a, b, c, d, e
Zoogman, P., Liu, X., Suleiman, R., Pennington, W., Flittner, D., Al-Saadi, J., Hilton, B., Nicks, D., Newchurch, M., Carr, J., Janz, S., Andraschko, M., Arola, A., Baker, B., Canova, B., Chan Miller, C., Cohen, R., Davis, J., Dussault, M., Edwards, D., Fishman, J., Ghulam, A., González Abad, G., Grutter, M., Herman, J., Houck, J., Jacob, D., Joiner, J., Kerridge, B., Kim, J., Krotkov, N., Lamsal, L., Li, C., Lindfors, A., Martin, R., McElroy, C., McLinden, C., Natraj, V., Neil, D., Nowlan, C., O'Sullivan, E., Palmer, P., Pierce, R., Pippin, M., Saiz-Lopez, A., Spurr, R., Szykman, J., Torres, O., Veefkind, J., Veihelmann, B., Wang, H., Wang, J., and Chance, K.: Tropospheric emissions: Monitoring of pollution (TEMPO), J. Quant. Spectrosc. Ra., 186, 17–39, https://doi.org/10.1016/j.jqsrt.2016.05.008, 2017. a