the Creative Commons Attribution 4.0 License.
the Creative Commons Attribution 4.0 License.
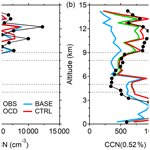
Strong particle production and condensational growth in the upper troposphere sustained by biogenic VOCs from the canopy of the Amazon Basin
Yunfan Liu
Siwen Wang
Chao Wei
Wei Tao
Mira L. Pöhlker
Christopher Pöhlker
Bruna A. Holanda
Ovid O. Krüger
Thorsten Hoffmann
Manfred Wendisch
Paulo Artaxo
Ulrich Pöschl
Meinrat O. Andreae
Nucleation and condensation associated with biogenic volatile organic compounds (BVOCs) are important aerosol formation pathways, yet their contribution to the upper-tropospheric aerosols remains inconclusive, hindering the understanding of aerosol climate effects. Here, we develop new schemes describing these organic aerosol formation processes in the WRF-Chem model and investigate their impact on the abundance of cloud condensation nuclei (CCN) in the upper troposphere (UT) over the Amazon Basin. We find that the new schemes significantly increase the simulated CCN number concentrations in the UT (e.g., up to ∼ 400 cm−3 at 0.52 % supersaturation) and greatly improve the agreement with the aircraft observations. Organic condensation enhances the simulated CCN concentration by 90 % through promoting particle growth, while organic nucleation, by replenishing new particles, contributes an additional 14 %. Deep convection determines the rate of these organic aerosol formation processes in the UT through controlling the upward transport of biogenic precursors (i.e., BVOCs). This finding emphasizes the importance of the biosphere–atmosphere coupling in regulating upper-tropospheric aerosol concentrations over the tropical forest and calls for attention to its potential role in anthropogenic climate change.
- Article
(8728 KB) - Full-text XML
- BibTeX
- EndNote
Atmospheric aerosol particles can influence the Earth's climate by acting as cloud condensation nuclei (CCN), among other pathways. The CCN residing in the upper troposphere (UT), which have been repeatedly observed in a substantial amount over the globe (e.g., Minikin et al., 2003; Andreae et al., 2018, and references therein; Williamson et al., 2019), not only constitute an important aerosol source for the lower troposphere (J. Wang et al., 2016; Williamson et al., 2019), but also can directly be activated into cloud droplets through in-cloud activation and thus alter the cloud properties (Paluch and Knight, 1984; Fan et al., 2018). However, the formation mechanisms for the upper-tropospheric CCN are poorly understood, which impedes their representation in models and the assessment of their climate effects (Heald et al., 2011; Watson-Parris et al., 2019).
Large concentrations of cloud active aerosol particles were detected in the UT over the Amazon by aircraft observations during the ACRIDICON-CHUVA campaign (Wendisch et al., 2016; Andreae et al., 2018). Chemical analysis demonstrated that their composition is dominated by organic compounds with signatures of secondary organic aerosol (SOA) related to the oxidation of biogenic volatile organic compounds (BVOCs; Schulz et al., 2018); yet, detailed processes driving the biogenic SOA formation remain inconclusive. Generally, two mechanisms may promote the CCN production from biogenic SOA in the UT. The first relates to organic new particle formation (NPF), where aerosol particles can form out of nucleation of highly oxygenated molecules (HOMs) oxidized from biogenic organic vapors such as α-pinene and β-pinene (Burkholder et al., 2007; Kirkby et al., 2016) and subsequently grow to larger sizes. The pure organic NPF can notably affect the atmospheric CCN budget in the planetary boundary layer (PBL; Gordon et al., 2016). Alternatively, if there are enough preexisting fine particles in the UT from transport or inorganic nucleation, the condensation of low volatile organic compounds (LVOCs) produced by BVOC oxidation onto the preexisting particles can also increase the CCN number (D'Andrea et al., 2013). However, a quantitative assessment of the BVOC-driven nucleation and condensation processes is lacking (Tröstl et al., 2016; Williamson et al., 2019). To what extent these two processes account for the CCN production in the Amazon UT, and whether these processes and CCN formation proceed in the UT or if CCN form in the lower troposphere and then are transported upwards, is not known.
Motivated by these questions, this study implements the laboratory-based organic nucleation (HOM-induced nucleation) and condensation processes into the WRF-Chem model and conducts simulations to quantify the CCN production from these BVOC-driven SOA formation pathways in the Amazon UT. We explore the upper-tropospheric biogenic SOA formation mechanisms in terms of the involved atmospheric physical and chemical processes and on a diurnal variation scale.
2.1 Simulation of number concentration of CCN and total aerosol particles in the upper troposphere
The organic nucleation mechanism in this study focuses on pure organic nucleation, including neutral and ion-induced processes, triggered by HOMs from α-pinene and β-pinene oxidation (Kirkby et al., 2016), as it was found dominant among organic nucleation pathways in the Amazon (Zhu and Penner, 2019). The organic condensation mechanism addresses the dynamic condensation of LVOCs oxidized from α-pinene, β-pinene, and isoprene (Mann et al., 2010) as well as HOMs. For an accurate representation of HOM concentrations, we adopted the common representative intermediates mechanism (CRIMECH) scheme (Jenkin et al., 2008) with an explicit description of α-pinene and β-pinene oxidation and calculated the HOM concentration dynamically. Such processing methods circumvented the uncertainties related to species approximation in other chemical schemes (Riccobono et al., 2014; Zhu et al., 2019) and the bulk assumption of an equilibrium state of HOMs (Gordon et al., 2016; Tröstl et al., 2016), respectively. The temperature effects on nucleation rate and LVOC yields were included in the model according to a combination of nucleation theory (Yu et al., 2017) and experimental results (Sahhaf et al., 2008). Details of the model description can be found in Appendix Sect. A1.1 and A1.2.
To disentangle the organic nucleation effect from the organic condensation influence, we performed the following sensitivity simulations:
-
BASE, the default WRF-Chem simulation with H2SO4-H2O binary nucleation (Wexler et al., 1994) and without biogenic nucleation or condensation;
-
CTRL, in which both the newly developed nucleation and condensation modules were added;
-
OCD, which only added the organic condensation;
-
BNUnoT and OCDnoT, which excluded the temperature effect on the nucleation rate and the LVOC yields, respectively;
-
NoOH, which was based on CTRL but without the HOM formation from OH oxidation.
Settings for all scenarios are summarized in Table A3. The simulations were conducted for two nested domains covering the Amazon with a horizontal resolution of 75 km and 15 km, respectively (Fig. A1) from 1 September to 1 October 2014. Aircraft measurements of aerosol number concentration profiles reaching up to 15 km altitude, close to the tropopause (18 km, Wendisch et al., 2016), sampled during the ACRIDICON-CHUVA campaign (Wendisch et al., 2016; Andreae et al., 2018), were used to evaluate the model results. Details of the model configuration and observation dataset are documented in Appendix Sect. A1.3–A2.
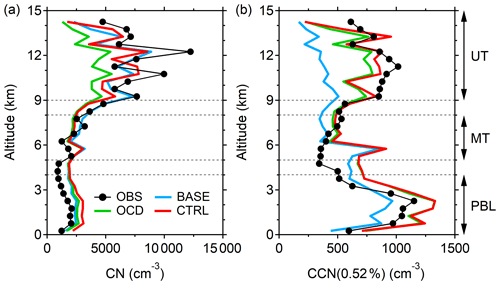
Figure 1Comparison of observed and simulated number concentrations of (a) condensation nuclei (CN, total aerosol population) with diameters above 20 nm and (b) cloud condensation nuclei (CCN) at 0.52 % supersaturation. The aerosol concentrations are at standard temperature and pressure (STP; 273.15 K and 1000 hPa). Planetary boundary layer (PBL), middle troposphere (MT), and upper troposphere (UT) are defined as the altitude range of 0–4, 5–8, and 9–15 km, respectively. The standard deviations of the observations are provided in Table A4.
Figure 1 shows the average profiles of the number concentration of aerosol particles (also called condensation nuclei, CN) with a diameter above 20 nm and of the CCN at a supersaturation of 0.52 % from aircraft observation (Fig. A1) and model simulations. The size of the CCN is approximately 90 nm in diameter, calculated according to the algorithm of Su et al. (2010; Sect. A3). Compared with the observations, the BASE case appears to reproduce the vertical distribution of CN in general (Sect. A3), but the CCN concentration in this simulation shows noticeable biases, especially in the UT (9–15 km) where the model underestimates the observed CCN number by up to 500 cm−3 (58 %). Considering the different size ranges in which CN and CCN reside, the large model underestimation in CCN may suggest insufficient growth of the smaller particles in the UT.
When adding the particle growth from the LVOC condensation into the model (i.e., the OCD case), the simulated CCN number in the UT rises notably, with an increase of 310 cm−3 (about 90 % relative to the BASE case; Table A4). However, the larger particles from the condensation growth meanwhile deplete the nano-sized particles, causing a dramatic drop in CN number concentrations from BASE to OCD. Though the non-cloud-resolving resolution of the simulations may cause an excessive mixing of ultrafine particle-laden fresh cloud outflows and their surrounding air masses (Andreae et al., 2018), and thus aggravate the particle scavenging, the considerable underestimation of the averaged CN number under a reasonable condensation growth in OCD is strongly indicative of some missing NPF mechanisms (Zhu et al., 2019; Zhao et al., 2020). As expected, by further taking into account the organic nucleation (i.e., the CTRL case), the simulated CN number concentrations are enhanced substantially (2100 cm−3, over 50 %; Table A4) relative to the OCD case and in markedly better agreement with the observation, while the CCN number concentrations in the model show a relatively weaker increase (90 cm−3, about 14 %; Table A4). Thus, in total, both the BVOC-driven organic nucleation and condensation play important roles in maintaining the particle population and size distribution in the UT (Fig. A7). The HOM nucleation effectively increases the CN number by replenishing new nano-sized particles, yet its contribution to the CCN, which are mainly in accumulation mode, is relatively limited. In contrast, the organic condensation causes efficient particle growth and, therefore, greatly enhances the CCN population.
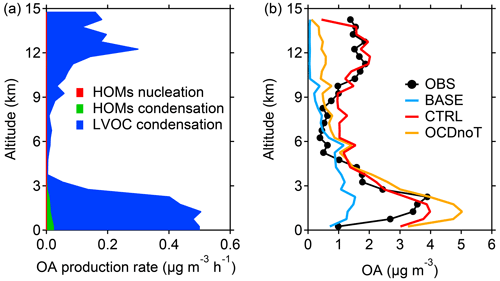
Figure 2Profiles of (a) contribution to organic aerosol (OA) mass from secondary organic aerosol production processes and (b) observed and simulated OA mass averaged along all the flight tracks. The OA production rate and aerosol mass concentrations are at STP.
Consistent with the CCN behavior, organic aerosol (OA), the dominant aerosol component over the simulated region and period (Andreae et al., 2018), is also underestimated in the UT in default WRF-Chem (BASE) but improved close to observation when the biogenic SOA formation is included (Fig. 2b). The condensation of LVOCs plays a predominant role in the OA mass production among all processes, while the other two formation pathways, especially the HOM nucleation, contribute little (Fig. 2a). This also explains why the organic condensation can cause profound particle growth while the HOM nucleation works mainly to increase the number of small particles. The OA production from the SOA processes in the UT shows a similar vertical pattern to that of the OA mass (Fig. 2b), implying local origins of the upper-tropospheric CCN.
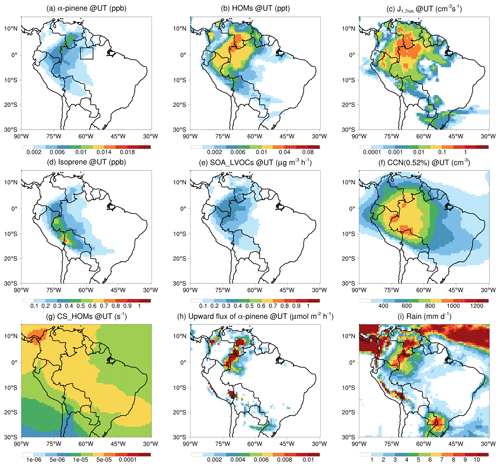
Figure 3Spatial distribution of (a) α-pinene, (b) HOMs, (c) organic nucleation rate, (d) isoprene, (e) SOA production rate by LVOCs, (f) CCN at 0.52 % supersaturation, and (g) condensation sink of HOMs in the upper troposphere (UT) averaged over 1 September–1 October 2014, all at STP. Also shown are (h) upward α-pinene flux to the UT and (i) precipitation rate averaged over the same period. The black rectangle in (a) denotes the region of the Central Amazon for further analysis in this study.
2.2 Factors influencing organic-driven particle formation and growth in the upper troposphere
Figure 3 shows the horizontal distributions of the monthly mean biogenic organic precursors (α-pinene and isoprene; α-pinene is used here as a surrogate for the organic nucleation precursors) and the HOM nucleation (Jorg) and LVOC condensation rates (SOA_LVOCs) in the upper troposphere. The organic nucleation and condensation distributions closely follow that of the biogenic precursors (Fig. 3c, e, a, and d) but not the oxidants (O3 and OH; Fig. A8), suggesting the upper-tropospheric BVOC concentration as the limiting factor for the organic nucleation/condensation in the Amazon UT. The region with high BVOC concentrations in the UT is different from the α-pinene distribution in the PBL (Fig. A9) but identical to the precipitation pattern as well as the large upward α-pinene flux (Fig. 3h–i), showing a necessary role of deep convection transport in the BVOC availability in the UT.
The Jorg can reach over 0.1 cm−3 s−1 in the UT (Fig. 3), whereas the PBL (0–4 km) and middle troposphere (MT; 5–8 km) show low values (Figs. A9–A10), even though the α-pinene concentration in PBL is a magnitude larger than in the UT. Such high upper-tropospheric Jorg is favored by not only the low sink of HOMs (CS; Fig. 3g) but also the low temperature in the UT (Fig. A2a). When the temperature dependence of Jorg (Sect. A1.2) is not considered, the Jorg in the UT is much lower than in the model run with the temperature effect (Fig. A11). The overall magnitude of Jorg is lower than simulated previously in the Amazon (Zhu et al., 2019), possibly due to the consideration of the ion sink in this study. For the SOA production from LVOC condensation, the bulk assumption of the LVOC yields used previously in the boreal forest (Scott et al., 2014) fails to reproduce the observed OA mass due to different conditions in the tropics (Sect. A4). A temperature-dependent correction of LVOC yields based on laboratory experiments (Saathoff et al., 2009) is necessary for correcting the OA simulation bias associated with the bulk LVOC yields assumption (Fig. 2b). The low temperature in the UT also serves as a favorable condition for the SOA production from LVOC condensation.
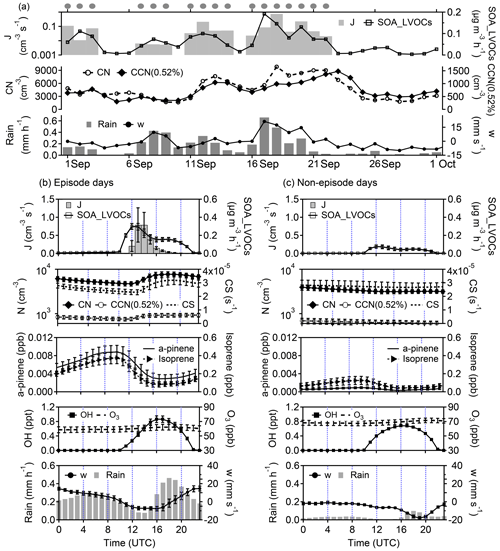
Figure 4Modeled temporal variation of the rate and precursors of organic nucleation and condensation as well as meteorological variables. (a) Daily variation of regionally averaged nucleation and condensation rates, number concentration of CN and CCN at 0.52 % supersaturation (CCN(0.52 %)), vertical wind at 8 km (w), and precipitation. The dots above the figure mark the upper-tropospheric biogenic SOA episodes. The diurnal patterns of nucleation and condensation rate, number concentration of CN and CCN(0.52 %), condensation sink of HOMs, and mixing ratio of α-pinene, isoprene, O3, and OH average in the upper troposphere as well as the vertical wind at 8 km and precipitation for the (b) upper-tropospheric biogenic SOA episode days and (c) non-episode days. The bars denote the standard error. The concentrations of gases and aerosols, the production rates, and the condensation sink are normalized to STP. The analyzed region is indicated by the black rectangle in Fig. 3a.
As the biogenic SOA formation in the UT exhibits prominent daily variation, we defined days with Jorg greater than cm−3 s−1 and simultaneous condensational SOA production rate larger than 0.05 µg m−3 h−1 as UT biogenic SOA episodes (Fig. 4a) to identify their characteristics. The biogenic SOA episodes in the UT emerge with stronger convection, where strong vertical wind effectively transports biogenic precursors to the UT and fosters SOA formation (Fig. 4b). In contrast, the biogenic SOA formation in the PBL tends to be suppressed by deep convection (Fig. A14) due to the decreased emission and oxidation of BVOCs by reduced incident solar radiation in cloudy weather (Fig. A13) as well as the BVOC dilution near the surface by low-BVOC air from the MT.
The organic nucleation and condensation rates demonstrate a clear diurnal cycle, with significant occurrence in the daytime. As the oxidant in the HOM and LVOC production, OH has reaction rates several orders faster than O3 (Sect. A1.2; Atkinson et al., 2006), making it the dominant oxidant, which is also evident as the sensitivity study switching off the OH oxidation (NoOH) shows significantly reduced HOM concentrations (Fig. A15). Thus, the photolysis origin of OH (Fig. 4b–c) explains the daytime burst of the Jorg and condensation rate. A detailed mechanism tracking the diurnal variation of the upper-tropospheric CCN production can be drawn. The nighttime convective activity as an extension of the former daytime convection contributes to the upper-tropospheric accumulation of biogenic precursors. With the onset of solar radiation, the photochemical reactions start to produce OH, which efficiently oxidizes BVOCs to form HOMs and LVOCs and then triggers the organic nucleation and condensation. The CN and CCN concentrations increase accordingly and reach high levels in the afternoon, which is also the typical time for the vigorous development of local convective clouds and thereby favors potential interactions between upper-tropospheric CCN and clouds.
In this work, we developed a new organic nucleation and condensation scheme for the WRF-Chem model to investigate the CN and CCN production in the UT (upper troposphere) by BVOC-driven SOA formation over a forest canopy region, the Amazon Basin.
The model evaluation against aircraft measurements shows that including the BVOC-driven SOA formation significantly improves the model agreement with the measured upper-tropospheric CCN (at 0.52 % supersaturation) number concentrations by elevating the simulated values up to ∼ 400 cm−3. Individually, the organic condensation drives efficient particle growth and enhances the CCN concentration in the UT by about 90 %. With the nano-sized particles from H2SO4-H2O binary nucleation scavenged under sufficient particle growth, the organic nucleation serves to replenish nano-particles and enhances the upper-tropospheric CN and CCN number concentration by over 50 % and 14 %, respectively. Note that although pure organic nucleation contributes importantly to the aerosol population in the UT, the relative roles of pure organic nucleation and other nucleation mechanisms, such as ternary and ion-induced inorganic nucleation (Napari et al., 2002; Yu et al., 2008), in the UT aerosol production remain to be investigated with a comprehensive consideration of nucleation parametrizations, e.g., those in Dunne et al. (2016) and Riccobono et al. (2014). The rates of SOA processes in the UT depend on deep convection for its vertical transport and are favored by low condensation sink and temperature at high altitudes.
The considerable CCN production in the UT by BVOC-driven organic processes underlines the important regulation of biospheric BVOCs on the high-altitude aerosol concentrations. Considering the climate significance of these upper-tropospheric aerosols, the biosphere–atmosphere coupling should be emphasized in the context of climate change, not only for its possible impact on the preindustrial reference state (Gordon et al., 2016), but also for its feedback to climate under the future anthropogenic influence (e.g., deforestation) and climate change.
A1 Model development
A1.1 Model description
The Weather Research and Forecasting model coupled with chemistry (WRF-Chem), version 3.9.1, was utilized in this study to investigate the biogenic organic nucleation and condensation over the Amazon. WRF-Chem is a meteorology–chemistry online coupled regional model, which integrates meteorological, gas-phase, and aerosol fields by calculating transport of chemical species under the same dynamical system as meteorological elements at each time step and considering complicated feedbacks between meteorological field and trace gases and aerosols (Grell et al., 2005).
The WRF-Chem configurations used are listed in Table A1. We chose the common representative intermediates mechanism (CRIMECH) scheme (Jenkin et al., 2008; Watson et al., 2008) with the kinetic preprocessor (KPP) interface to simulate the gas-phase chemistry. CRIMECH contains up to 112 non-methane volatile organic compounds (VOCs), including α-pinene, β-pinene, and isoprene (Archer-Nicholls et al., 2014; Watson et al., 2008), and adopts 652 chemical reactions involving inorganic species, organic vapors, and their oxidation intermediates, based on the Master Chemical Mechanism (MCM). These chemical settings enable it to directly provide the gas-phase precursors, i.e., α-pinene, β-pinene, and isoprene, and chemical reactions for the biogenic SOA formation. The Model for Simulating Aerosol Interactions and Chemistry (MOSAIC; Zaveri et al., 2008) was utilized to simulate aerosols, which uses discrete size bins to represent the aerosol size distribution. This study employed the four-bin version with the size bins distributed as listed in Table A2. Aerosol species in MOSAIC include five inorganic ions, i.e., sulfate, nitrate, ammonium, sodium, and chloride, and three unreactive primary aerosol species, i.e., black carbon (BC), particulate organic matter (POM), and other inorganics (OIN; Fast et al., 2006; Zaveri et al., 2008). In the WRF-Chem version 3.9.1 MOSAIC aerosol module, the binary nucleation of H2SO4-H2O is included (Wexler et al., 1994), while the organic nucleation and condensation are not accounted for. The coagulation process of particles and gas-particle partitioning were parameterized as described in Zaveri et al. (2008). The dry deposition of aerosol is parameterized by the updated resistance-in-series approach of Wesely (1989). The in- and below-cloud wet deposition take place by activating aerosol from an interstitial state into cloud-borne particles and calculating the washout rate due to precipitation, respectively (Chapman et al., 2009; Easter et al., 2004). The WRF-Chem model configurations used in this study allow aerosol–cloud interactions, following the way described by Fast et al. (2006) and Chapman et al. (2009), while the aerosol–radiation interactions were not included in the model simulations.
A1.2 Model improvement
Based on the aforementioned CRIMECH gas-phase chemistry scheme and MOSAIC aerosol scheme, a new module resolving the purely organic nucleation and condensation associated with BVOCs has been added to WRF-Chem, which provides a modeling tool to investigate biogenic SOA formation and its contribution to the upper-tropospheric CCN.
The implementation of the new organic nucleation includes integrating the production of HOMs in the CRIMECH gas-phase chemistry scheme, resetting the sectional bins in the aerosol model, and adding parameterizations of pure organic nucleation mechanisms by HOMs. Specifically, four reactions regarding HOM production from the oxidation of α-pinene and β-pinene by O3 and OH were added to the CRIMECH mechanism based on reaction coefficients and yields suggested by laboratory experiments (Atkinson et al., 2006). Then, the condensation sink of HOMs was represented according to the algorithm of Kerminen et al. (2004). Instead of parameterizing the HOM concentration as the ratio of its production and condensation sink with the assumption that the HOMs were in a thermal equilibrium state (Kirkby et al., 2016; Gordon et al., 2016), the kinetic calculation of the HOM production and condensation sink in this study enables a more accurate representation of the HOM concentration.
The four-bin MOSAIC scheme in WRF-Chem addresses aerosols with diameters from 39 nm to 10 µm, which does not cover the size range of newly formed particles whose diameters are in the nanometer range (Kulmala, 2003). To explicitly represent the nucleation of vapor into particles, we extended the lower end of the aerosol size range in the MOSAIC scheme from 39 to 0.6 nm by introducing three additional size bins whose boundaries are set following the same lognormal size distribution law as the original four bins (Table A2). Thus, the newly developed seven-bin MOSAIC scheme can resolve the formation and initial growth of new particles and assures a high computation efficiency. The four-bin MOSAIC scheme includes the H2SO4-H2O binary nucleation using a thermodynamic equilibrium parameterization where a critical concentration of H2SO4 is calculated based on air temperature and relative humidity and then the extra H2SO4 beyond this threshold is parameterized into aerosols centered at 78 nm (i.e., the 39–156 nm bin). The equilibrium method for describing H2SO4-H2O binary nucleation is validated for the aerosol size above 10 nm. With extended aerosol size bins in the seven-bin MOSAIC scheme, we now applied the H2SO4-H2O binary nucleation parameterization to the third bin (i.e., 10–39 nm) to not only assure the practical application of this nucleation parameterization (Wexler et al., 1994) but also keep the aerosol size range in agreement with the observations (i.e., starting from 20 nm).
Then, in addition to the existing H2SO4-H2O binary nucleation, pure biogenic nucleation mechanisms induced by HOMs were integrated into the MOSAIC module. The mechanisms of organic nucleation were investigated in CLOUD (Cosmics Leaving OUtdoors Droplets) chamber experiments (Kirkby et al., 2016), which suggested that the HOM-induced pure organic nucleation rate (Jorg, unit: cm−3 s−1) can be represented by the combination of the neutral (Jn) and the ion-induced (Jiin) nucleation rate. The detailed parameterization of the Jn and Jiin is as follows:
where HOM concentrations are in units of 107 molecules per cubic centimeter and obtained by chemical kinetic calculations as described above; the ai represent free parameters whose values were suggested by Kirkby et al. (2016) where a1, a2, a3, a4, and a5 equaled to 0.04001, 1.848, 0.001366, 1.566, and 0.1863, respectively.
n± is the ion concentration produced from radon and galactic cosmic rays and is parameterized as
where q (in cm−3 s−1) represents the ion-pair production rate and adopts the value of 10 cm−3 s−1 (Hõrrak et al., 2008). α is the ion–ion recombination coefficient (in cm3 s−1) and was set to cm3 s−1 here. The ion loss rate, ki, is due to the ion condensation sink (CS) onto aerosols and the ion-induced nucleation
In this study, the condensation sink term was calculated according to the empirical parameterization proposed by Tammet (1991). The HOM nucleation rate, Jorg, is then modulated by temperature. Unlike the approximated temperature correction suggested in Dunne et al. (2016), a temperature dependence associated with the Gibbs free energy for forming the critical cluster based on the classical homogeneous nucleation theory (Yu et al., 2017) is used here. We applied temperature corrections to Jn and Jiin by multiplying them by a correction factor, exp() and exp(), respectively, and the ΔGn and ΔGiin are based on smog chamber results (Kirkby et al., 2016). After organic nucleation, the newly formed particles were added into the smallest bin and underwent subsequent processes such as coagulation, transport, and deposition.
Additionally, a new module addressing the condensation of LVOCs was integrated into WRF-Chem. The LVOCs were oxidation products of α-pinene, β-pinene, and isoprene by O3, OH, and NO3. A yield of 13 % for monoterpene oxidation products and 3 % for isoprene oxidation products were used in Scott et al. (2014). Laboratory chamber experiments found a temperature dependence of the SOA yield from α-pinene oxidation (Saathoff et al., 2009). Therefore, instead of constant LVOC yields, temperature-corrected yields based on these laboratory experiment results (Saathoff et al., 2009) were applied in the model here.
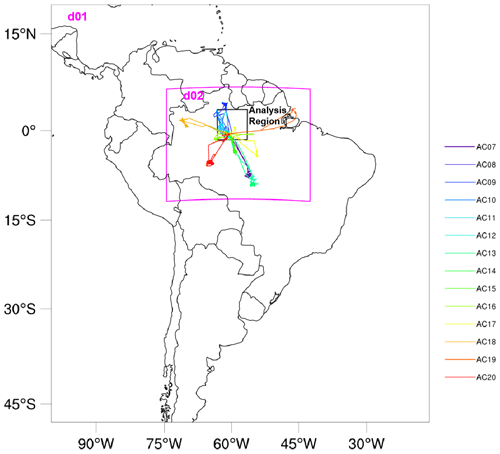
Figure A1Model domain and tracks of flight AC07 to AC20 from the ACRIDICON-CHUVA campaign. The outer map represents the parent domain with 75 km horizontal grid spacing, and the embedded square shows the extent of the 15 km resolution (d02) domain. The black rectangle is the same as the one marked in Fig. 3a, denoting the region of the Central Amazon for further analysis in this study.
A1.3 Numerical experiment design
In this study, two nested domains with a horizontal spacing of 75 and 15 km were set up over South America (Fig. A1), with Domain1 covering most of the South American continent, while Domain2 is over the Amazon Basin area. Vertical layers of 29 levels extending from the ground surface to a height of 50 hPa were applied for all domains. The initial and boundary meteorological and chemical conditions were from the 6 h National Centers for Environmental Prediction (NCEP) final analysis (FNL) data and model for ozone and related chemical tracers, version 4 (MOZART-4) global chemical transport model output (Emmons et al., 2010), respectively. The anthropogenic emissions with a resolution of were obtained from the global air pollution emission dataset, EDGAR-HTAPv2 (https://edgar.jrc.ec.europa.eu/dataset_htap_v2, last access: 23 December 2022; Janssens-Maenhout et al., 2015). The Fire INventory from NCAR version 1.5 (FINNv1.5; Wiedinmyer et al., 2011) provided the biomass burning emission, which is updated daily at 1 km resolution. The rise of fire plumes after emission was represented by a plume-rise parameterization (Freitas et al., 2007). The primary organic matter (POM) emission rate was calculated based on the OC emission by applying a ratio of 1.6 between the mass of POM and OC (Andreae, 2019). The biogenic emissions of NO and volatile organic compounds (VOCs) were generated online by the Model of Emissions of Gases and Aerosols from Nature (MEGAN; Guenther et al., 2012). Among the biogenic VOCs are the precursors, i.e., α-pinene, β-pinene, and isoprene, for the organic nucleation and condensation which are investigated in this study. Based on the United States Geological Survey (USGS) land use category, temperature, and radiation, MEGAN calculated the emission of biogenic gases, which were subsequently put into the corresponding chemical species in the CRIMECH gas-phase scheme as a source term. The simulation was conducted from 24 August to 1 October 2014, and the first 8 d of the simulation were used as spin up. The comparisons between model outputs and aircraft measurements in Sect. 2.1 are made with the results from Domain2. A rectangular area focusing on the Central Amazon, as shown in Fig. A1, was used in the analysis in Sect. 2.2.
To characterize the pure organic nucleation and condensation and investigate the controlling factors, a series of sensitivity simulations were performed as listed in Table A3. A baseline simulation (BASE) was conducted based on the default WRF-Chem, except the binary nucleation-generated aerosols were put into the third bin as described above. Simulation using the improved version of WRF-Chem, CTRL, was conducted, where new particles can be formed by organic nucleation in addition to the default H2SO4-H2O binary nucleation scheme in BASE and where the organic condensation process was also taken into consideration. In order to examine the effect of atmospheric vertical temperature variation on the organic nucleation and condensation growth, sensitivity simulations were performed using the modified WRF-Chem model but without temperature influence on the nucleation rate and the yields of LVOCs, namely BNUnoT and OCDnoT, respectively. For the purpose of distinguishing the influence from the organic nucleation and the condensation of organics, an additional sensitivity simulation was made where only the condensation of organics was included in the BASE case, which was termed OCD. To examine the relative importance of O3 and OH in the HOM-generating oxidation reactions, NoOH was conducted based on CTRL but with the HOM formation from OH oxidation turned off.
A2 Data
The ACRIDICON-CHUVA (ACRIDICON stands for “Aerosol, Cloud, Precipitation, and Radiation Interactions and Dynamics of Convective Cloud Systems”, and CHUVA is the acronym for “Cloud Processes of the Main Precipitation Systems in Brazil: A Contribution to Cloud Resolving Modeling and to the GPM (Global Precipitation Measurement)”; Wendisch et al., 2016) campaign was conducted in the Amazon region in 2014. It aimed at in-depth investigations of the properties of the aerosols and clouds in this area and the explorations of interactions between aerosols, radiation, clouds, and precipitation. Fourteen flights were operated between 6 September and 1 October 2014, encompassing comprehensive measurements of meteorology, trace gases, and aerosols with ceiling heights up to 15 km, close to the top of the troposphere. The measurements of meteorological parameters (air temperature, relative humidity, and wind speed), O3, total aerosol number concentration, CCN number concentration, and black carbon and organic aerosol mass conducted on the 14 flights were used in this study. The gas and aerosol concentrations have been normalized to standard temperature and pressure (STP). The total aerosol particles, also called condensation nuclei (CN) focus on aerosols with a diameter above 20 nm. The observed CCN are the CCN at a supersaturation of 0.52 % (CCN(0.52 %); Andreae et al., 2018). The flight tracks are shown in Fig. A1. Overviews of the ACRIDICON-CHUVA campaign and observation are documented by Wendisch et al. (2016) and Andreae et al. (2018).
A3 Model evaluation
To compare against the flight observation, the hourly model outputs at the corresponding location of the observed data within the hour were used. The modeled gas and aerosol concentrations are values at STP, consistent with the observed data. The model reasonably reproduced the meteorological conditions (Fig. A2), the O3 vertical distributions, and the black carbon concentrations (Fig. A3), showing its ability to capture the meteorological processes, basic atmospheric chemical processes, and primary aerosol emission and transport processes. The simulated concentrations of the biogenic organic vapors, α-pinene, β-pinene, and isoprene (Fig. A4) are of comparable magnitude to previous observations (Kuhn et al., 2010), demonstrating a reasonable model simulation of the biogenic emissions.
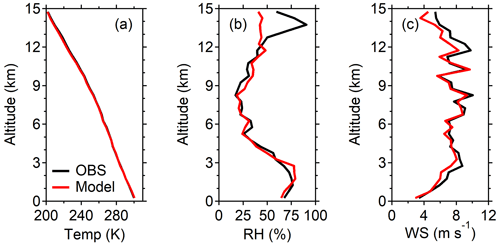
Figure A2Comparison of (a) air temperature (Temp), (b) relative humidity (RH), and (c) horizontal wind speed (WS) averaged from all flight measurements (OBS) and WRF-Chem simulations (Model).
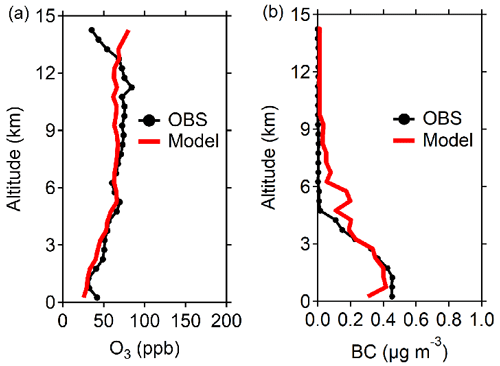
Figure A3Comparison of (a) O3 mixing ratio and (b) black carbon (BC) mass concentration averaged from all flight measurements (OBS) and WRF-Chem simulations (Model), all at STP.
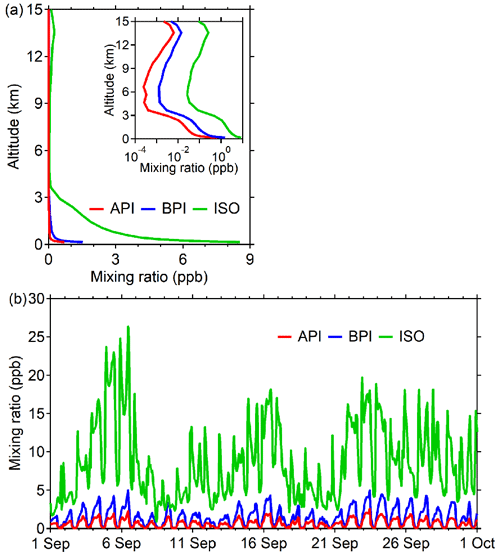
Figure A4Simulated (a) vertical profiles and (b) time series of α-pinene (API), β-pinene (BPI), and isoprene (ISO) mixing ratios (STP) at the location of ATTO. The embedded figure in (a) is the same as the outer figure but on a log scale.
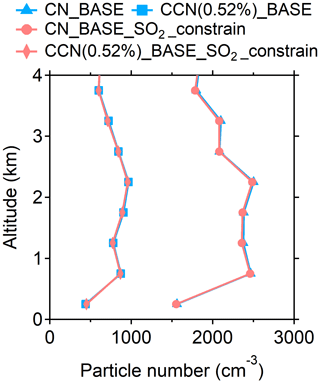
Figure A6Vertical profiles of the simulated number concentrations (STP) of CN and CCN at 0.52 % supersaturation averaged along the observation trajectories within the planetary boundary layer (PBL).
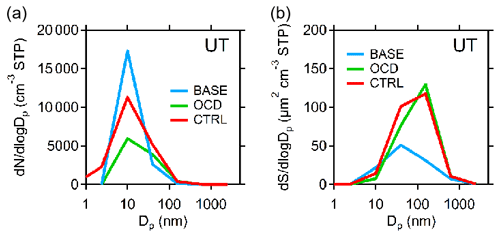
Figure A7Simulated size distributions of (a) particle number concentration, and (b) surface area in the upper troposphere (UT) along the flight tracks. The particle size distributions are normalized to STP.
The simulated SO2 concentration of around 21 ppt throughout most of the free troposphere (FT) at the location of the ATTO site (Fig. A5) is in fair agreement with an observed background SO2 concentration of 18 ppt in the FT over the Central Amazon (Andreae and Andreae, 1988), which is an important prerequisite for an accurate simulation of the H2SO4-H2O binary nucleation in the FT (Wexler et al., 1994). Compared to the observed SO2 concentration of 27 ppt in the PBL (Andreae and Andreae, 1988) and 80 ppt near the ground surface (Ramsay et al., 2020), the modeled SO2 concentration in the PBL, especially near the ground surface, was relatively higher (Fig. A5). This could be related to several factors, e.g., overestimated SO2 emission (Andreae, 2019) and/or inadequate scavenging (Hardacre et al., 2021), and requires further investigations. The influence of the SO2 overestimation in the PBL on the simulated aerosol concentration was examined by conducting a sensitivity study, namely BASE_SO2_constrain, where all the settings were the same as the BASE case except that the SO2 concentration in the PBL was fixed to 30 ppt in accordance with the lower end of the range of published measurements during the dry season. The simulation results show that the difference in the aerosol concentration within the PBL between BASE and BASE_SO2_constrain is minor (Fig. A6), which indicates an insignificant influence of the SO2 overestimation in the PBL on the simulated aerosol concentration.
In this study, the H2SO4-H2O binary nucleation mechanism was simulated as it has been widely used for describing inorganic nucleation in the free troposphere (Cui et al., 2014; Gordon et al., 2016; Zhu and Penner, 2019). As shown from the nucleation rate in Fig. A12, H2SO4-H2O binary nucleation mainly occurs in the free troposphere, which is consistent with the vertical distribution of binary nucleation simulated for the Amazon region in Zhao et al. (2020). The H2SO4-H2O binary nucleation causes a CN increase of over 3000 cm−3 in the UT under sufficient particle condensational growth as approximately estimated from the difference between OCD and BASEnoNUC (Table A4). It is of a comparable magnitude to the CN increase of 2100 cm−3 by organic nucleation. A higher rate of the H2SO4-H2O binary nucleation over the organic nucleation was also found by Zhao et al. (2020) in the Amazon from 9 to 13 km altitude but the overall H2SO4-H2O binary nucleation in the UT was insignificant in Zhao et al. (2020), which is different from the result in this study. This is expected as the result in Zhao et al. (2020) was for a low-SO2 area and there was competition for H2SO4 by other H2SO4-involving nucleation processes in Zhao et al. (2020). In a global simulation where the inorganic nucleation was represented only by the H2SO4-H2O binary nucleation, the column-integrated H2SO4-H2O binary nucleation in the Amazon is of the same magnitude as but somewhat lower than the organic nucleation (Zhu and Penner, 2019). Considering the H2SO4-H2O binary nucleation occurs mainly in the upper troposphere and the organic nucleation in Zhu and Penner (2019) includes the hetero-molecular organic nucleation, the relative importance of H2SO4-H2O binary nucleation to pure organic nucleation in the UT should be greater than shown in the column-integrated results. Therefore the simulated H2SO4-H2O binary nucleation in this study should be generally reasonable.
The model can generally reproduce the vertical distribution of CN (Fig. 1) as described in Sect. 2.1; yet, a systematic overestimation of CN exists in model simulations below 5 km. This could be associated with uncertainties in the fire emission inventories (Andreae, 2019), as aerosols near the ground surface during the Amazon dry season are overwhelmingly influenced by persistent biomass burning (Andreae et al., 2015). In addition, the comparison of a grid-average value in the model with an observation on a spot may also contribute to the discrepancy. To make the CCN comparison, the modeled aerosols of a size consistent with that of the observed CCN(0.52 %) were used. The cut-off size of the CCN(0.52 %) was calculated to be approximately 90 nm in diameter (Su et al., 2010), based on an observed average hygroscopicity value of 0.12 derived from the aerosol component observations. This hygroscopicity level is close to those of organic aerosols (Petters and Kreidenweis, 2007), which is expected since organic aerosols dominate the aerosol components in this area (Andreae et al., 2018). As the main aerosol component (i.e., organic aerosols) can be well reproduced by the developed model version (Fig. 2b), it is justifiable to use this observed hygroscopicity to calculate the CCN size in models. The comparison shows that the CCN number was underestimated by the BASE case. The model underestimation of CCN number in the UT reaches −58 % as shown in Sect. 2.1. Compared to the UT, the biases in CCN below 4 km are much smaller and lie around −9 %. The negative biases in CCN number in both the UT and PBL are however corrected in the CTRL case, suggesting the lack of SOA production and inadequate particle growth in the BASE case as the reason for the CCN underestimation.
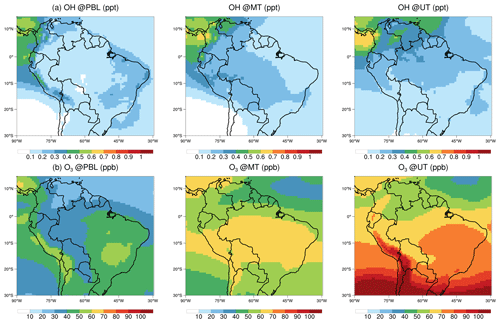
Figure A8Horizontal distribution of (a) OH and (b) O3 averaged over 1 September–1 October 2014 from the CTRL case. The concentrations are at STP.
Table A4Averaged values of modeled and observed aerosol particle number concentration.
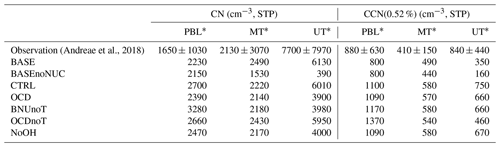
* PBL, MT, and UT are defined as the altitude range of 0–4, 5–8, and 9–15 km, respectively.
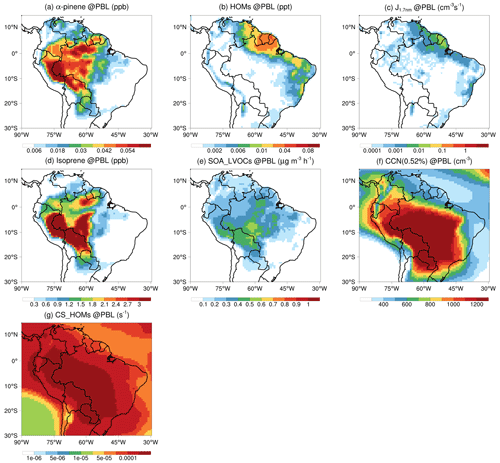
Figure A9Same as Fig. 3 (a–g) but for the planetary boundary layer (PBL). Note that the scales in (a) and (d) are 3 times those in Fig. 3a and d, respectively.
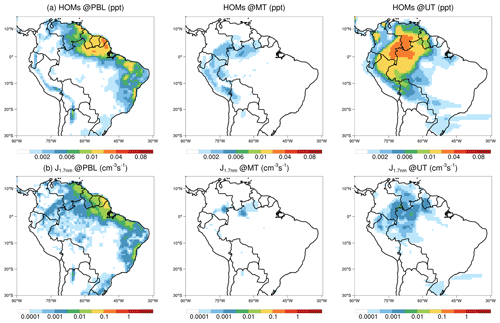
Figure A11Horizontal distribution of (a) HOMs and (b) organic nucleation rate averaged over 1 September–1 October 2014 from the BNUnoT case, all at STP.
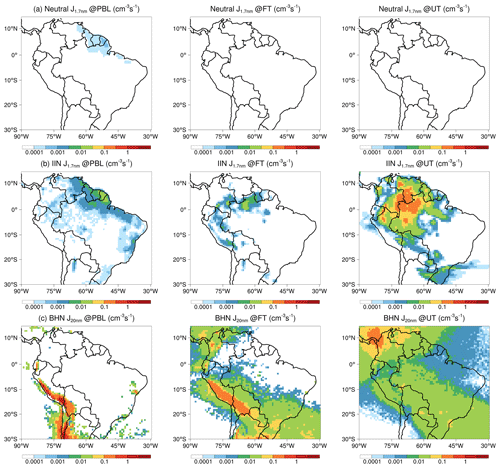
Figure A12Horizontal distribution of (a) neutral organic nucleation rate, (b) ion-induced organic nucleation rate, and (c) H2SO4–H2O binary nucleation rate at the planetary boundary layer (PBL, left panel), middle troposphere (MT, middle panel), and upper troposphere (UT, right panel) averaged over 1 September–1 October 2014 from the CTRL case. The nucleation rates are for STP.
A4 Sensitivity simulations of LVOC condensation
Figure 2b compares the simulated OA mass from CTRL and BASE with the observed data. The BASE case using the MOSAIC aerosol module and FINN biomass burning emission inventory shows a reasonable performance of OA representation in the PBL, which was also confirmed by previous evaluations for this region (Archer-Nicholls et al., 2014; Q. Wang et al., 2016); however, the OA in the UT is significantly underestimated. This negative bias of OA mass in the UT in the BASE case is greatly improved in the CTRL case by considering the organic aerosol processes driven by the biogenic precursors, among which the LVOC condensation plays a dominant role (Fig. 2a).
To further examine the uncertainty of the LVOC condensation in terms of the LVOC yields, sensitivity simulations regarding the temperature dependence of the LVOC yields were performed. The OCDnoT case adopted a bulk assumption of a yield of 13 % from monoterpene oxidation and 3 % from isoprene oxidation (OCDnoT), as suggested by Scott et al. (2014); while in the CTRL case, the temperature dependence of LVOC yields based on an α-pinene oxidation experiment (Saathoff et al., 2009) was applied to the LVOC yields. The OCDnoT case produces a larger amount of boundary layer OA than the CTRL case, causing a higher bias in the model compared with the observations and suggesting an excessive SOA production. A significant difference between the environment where the LVOC yields were originally based (Kroll et al., 2005) and the region investigated here may be the reason for the poor performance of the bulk yields in the studied area, as the temperature in previous applications is much lower than the tropical forest boundary layer conditions. On the other end, the temperature difference could also explain the underestimation of OA mass with bulk yields in the OCDnoT for the UT (Fig. 2b), where the temperature is far below the freezing point (Fig. A2). With the temperature dependence correction, i.e., LVOC yields increasing with colder temperature, the OA underestimation in the UT in the OCDnoT case can be effectively corrected in the CTRL case.
The model results presented in this work are available at https://doi.org/10.17617/3.3ISOYC (Liu, 2022).
YC designed and led the study. YL and YC conducted the model development. YL performed the model simulation and analyzed the data. YL, YC, and HS interpreted the results. MOA and UP contributed to discussing the results. SW, CW, and WT supported model simulation and data visualization. MOA, UP, PA, and MW coordinated the ACRIDICON-CHUVA observation field campaign. MLP, CP, OOK, and BAH provided the observation data from HALO for model comparison. YL wrote the paper with input from all co-authors.
At least one of the (co-)authors is a member of the editorial board of Atmospheric Chemistry and Physics. The peer-review process was guided by an independent editor, and the authors also have no other competing interests to declare.
Publisher's note: Copernicus Publications remains neutral with regard to jurisdictional claims in published maps and institutional affiliations.
This study is supported by the Max Planck Society (MPG). Yafang Cheng and Yunfan Liu acknowledge the Minerva Program of MPG. We also gratefully acknowledge the observational data and data providers from the ACRIDICON-CHUVA campaign and a wide range of other institutional partners.
This research was supported by the Max Planck Society (MPG) through the Minerva
Program. The ACRIDICON-CHUVA campaign was supported by the MPG, the German Science Foundation
(DFG Priority Program SPP 1294), the German Aerospace Center (DLR), and the FAPESP (São Paulo Research
Foundation) (grant nos. 2009/15235-8, 2013/05014-0, and 2017/17047-0).
The article processing charges for this open-access publication were covered by the Max Planck Society.
This paper was edited by Martina Krämer and James Allan and reviewed by Eimear Dunne and one anonymous referee.
Andreae, M. O.: Emission of trace gases and aerosols from biomass burning – an updated assessment, Atmos. Chem. Phys., 19, 8523–8546, https://doi.org/10.5194/acp-19-8523-2019, 2019.
Andreae, M. O. and Andreae, T. W.: The cycle of biogenic sulfur compounds over the Amazon Basin: 1. Dry season, J. Geophys. Res.-Atmos., 93, 1487–1497, https://doi.org/10.1029/JD093iD02p01487, 1988.
Andreae, M. O., Acevedo, O. C., Araùjo, A., Artaxo, P., Barbosa, C. G. G., Barbosa, H. M. J., Brito, J., Carbone, S., Chi, X., Cintra, B. B. L., da Silva, N. F., Dias, N. L., Dias-Júnior, C. Q., Ditas, F., Ditz, R., Godoi, A. F. L., Godoi, R. H. M., Heimann, M., Hoffmann, T., Kesselmeier, J., Könemann, T., Krüger, M. L., Lavric, J. V., Manzi, A. O., Lopes, A. P., Martins, D. L., Mikhailov, E. F., Moran-Zuloaga, D., Nelson, B. W., Nölscher, A. C., Santos Nogueira, D., Piedade, M. T. F., Pöhlker, C., Pöschl, U., Quesada, C. A., Rizzo, L. V., Ro, C.-U., Ruckteschler, N., Sá, L. D. A., de Oliveira Sá, M., Sales, C. B., dos Santos, R. M. N., Saturno, J., Schöngart, J., Sörgel, M., de Souza, C. M., de Souza, R. A. F., Su, H., Targhetta, N., Tóta, J., Trebs, I., Trumbore, S., van Eijck, A., Walter, D., Wang, Z., Weber, B., Williams, J., Winderlich, J., Wittmann, F., Wolff, S., and Yáñez-Serrano, A. M.: The Amazon Tall Tower Observatory (ATTO): overview of pilot measurements on ecosystem ecology, meteorology, trace gases, and aerosols, Atmos. Chem. Phys., 15, 10723–10776, https://doi.org/10.5194/acp-15-10723-2015, 2015.
Andreae, M. O., Afchine, A., Albrecht, R., Holanda, B. A., Artaxo, P., Barbosa, H. M. J., Borrmann, S., Cecchini, M. A., Costa, A., Dollner, M., Fütterer, D., Järvinen, E., Jurkat, T., Klimach, T., Konemann, T., Knote, C., Krämer, M., Krisna, T., Machado, L. A. T., Mertes, S., Minikin, A., Pöhlker, C., Pöhlker, M. L., Pöschl, U., Rosenfeld, D., Sauer, D., Schlager, H., Schnaiter, M., Schneider, J., Schulz, C., Spanu, A., Sperling, V. B., Voigt, C., Walser, A., Wang, J., Weinzierl, B., Wendisch, M., and Ziereis, H.: Aerosol characteristics and particle production in the upper troposphere over the Amazon Basin, Atmos. Chem. Phys., 18, 921–961, https://doi.org/10.5194/acp-18-921-2018, 2018.
Archer-Nicholls, S., Lowe, D., Utembe, S., Allan, J., Zaveri, R. A., Fast, J. D., Hodnebrog, Ø., Denier van der Gon, H., and McFiggans, G.: Gaseous chemistry and aerosol mechanism developments for version 3.5.1 of the online regional model, WRF-Chem, Geosci. Model Dev., 7, 2557–2579, https://doi.org/10.5194/gmd-7-2557-2014, 2014.
Atkinson, R., Baulch, D. L., Cox, R. A., Crowley, J. N., Hampson, R. F., Hynes, R. G., Jenkin, M. E., Rossi, M. J., Troe, J., and IUPAC Subcommittee: Evaluated kinetic and photochemical data for atmospheric chemistry: Volume II – gas phase reactions of organic species, Atmos. Chem. Phys., 6, 3625–4055, https://doi.org/10.5194/acp-6-3625-2006, 2006.
Burkholder, J. B., Baynard, T., Ravishankara, A. R., and Lovejoy, E. R.: Particle nucleation following the O3 and OH initiated oxidation of α-pinene and β-pinene between 278 and 320 K, J. Geophys. Res.-Atmos., 112, D10216, https://doi.org/10.1029/2006jd007783, 2007.
Chapman, E. G., Gustafson Jr., W. I., Easter, R. C., Barnard, J. C., Ghan, S. J., Pekour, M. S., and Fast, J. D.: Coupling aerosol-cloud-radiative processes in the WRF-Chem model: Investigating the radiative impact of elevated point sources, Atmos. Chem. Phys., 9, 945–964, https://doi.org/10.5194/acp-9-945-2009, 2009.
Cui, Y. Y., Hodzic, A., Smith, J. N., Ortega, J., Brioude, J., Matsui, H., Levin, E. J. T., Turnipseed, A., Winkler, P., and de Foy, B.: Modeling ultrafine particle growth at a pine forest site influenced by anthropogenic pollution during BEACHON-RoMBAS 2011, Atmos. Chem. Phys., 14, 11011–11029, https://doi.org/10.5194/acp-14-11011-2014, 2014.
D'Andrea, S. D., Häkkinen, S. A. K., Westervelt, D. M., Kuang, C., Levin, E. J. T., Kanawade, V. P., Leaitch, W. R., Spracklen, D. V., Riipinen, I., and Pierce, J. R.: Understanding global secondary organic aerosol amount and size-resolved condensational behavior, Atmos. Chem. Phys., 13, 11519–11534, https://doi.org/10.5194/acp-13-11519-2013, 2013.
Dunne, E. M., Gordon, H., Kurten, A., Almeida, J., Duplissy, J., Williamson, C., Ortega, I. K., Pringle, K. J., Adamov, A., Baltensperger, U., Barmet, P., Benduhn, F., Bianchi, F., Breitenlechner, M., Clarke, A., Curtius, J., Dommen, J., Donahue, N. M., Ehrhart, S., Flagan, R. C., Franchin, A., Guida, R., Hakala, J., Hansel, A., Heinritzi, M., Jokinen, T., Kangasluoma, J., Kirkby, J., Kulmala, M., Kupc, A., Lawler, M. J., Lehtipalo, K., Makhmutov, V., Mann, G., Mathot, S., Merikanto, J., Miettinen, P., Nenes, A., Onnela, A., Rap, A., Reddington, C. L., Riccobono, F., Richards, N. A., Rissanen, M. P., Rondo, L., Sarnela, N., Schobesberger, S., Sengupta, K., Simon, M., Sipila, M., Smith, J. N., Stozkhov, Y., Tome, A., Trostl, J., Wagner, P. E., Wimmer, D., Winkler, P. M., Worsnop, D. R., and Carslaw, K. S.: Global atmospheric particle formation from CERN CLOUD measurements, Science, 354, 1119–1124, https://doi.org/10.1126/science.aaf2649, 2016.
Easter, R. C., Ghan, S. J., Zhang, Y., Saylor, R. D., Chapman, E. G., Laulainen, N. S., Abdul-Razzak, H., Leung, L. R., Bian, X. D., and Zaveri, R. A.: MIRAGE: Model description and evaluation of aerosols and trace gases, J. Geophys. Res.-Atmos., 109, D20210, https://doi.org/10.1029/2004jd004571, 2004.
Emmons, L. K., Walters, S., Hess, P. G., Lamarque, J.-F., Pfister, G. G., Fillmore, D., Granier, C., Guenther, A., Kinnison, D., Laepple, T., Orlando, J., Tie, X., Tyndall, G., Wiedinmyer, C., Baughcum, S. L., and Kloster, S.: Description and evaluation of the Model for Ozone and Related chemical Tracers, version 4 (MOZART-4), Geosci. Model Dev., 3, 43–67, https://doi.org/10.5194/gmd-3-43-2010, 2010.
Fan, J., Rosenfeld, D., Zhang, Y., Giangrande, S. E., Li, Z., Machado, L. A. T., Martin, S. T., Yang, Y., Wang, J., Artaxo, P., Barbosa, H. M. J., Braga, R. C., Comstock, J. M., Feng, Z., Gao, W., Gomes, H. B., Mei, F., Pöhlker, C., Pöhlker, M. L., Pöschl, U., and de Souza, R. A. F.: Substantial convection and precipitation enhancements by ultrafine aerosol particles, Science, 359, 411–418, https://doi.org/10.1126/science.aan8461, 2018.
Fast, J. D., Gustafson, W. I., Easter, R. C., Zaveri, R. A., Barnard, J. C., Chapman, E. G., Grell, G. A., and Peckham, S. E.: Evolution of ozone, particulates, and aerosol direct radiative forcing in the vicinity of Houston using a fully coupled meteorology-chemistry-aerosol model, J. Geophys. Res., 111, D21305, https://doi.org/10.1029/2005jd006721, 2006.
Freitas, S. R., Longo, K. M., Chatfield, R., Latham, D., Silva Dias, M. A. F., Andreae, M. O., Prins, E., Santos, J. C., Gielow, R., and Carvalho Jr., J. A.: Including the sub-grid scale plume rise of vegetation fires in low resolution atmospheric transport models, Atmos. Chem. Phys., 7, 3385–3398, https://doi.org/10.5194/acp-7-3385-2007, 2007.
Gordon, H., Sengupta, K., Rap, A., Duplissy, J., Frege, C., Williamson, C., Heinritzi, M., Simon, M., Yan, C., Almeida, J., Trostl, J., Nieminen, T., Ortega, I. K., Wagner, R., Dunne, E. M., Adamov, A., Amorim, A., Bernhammer, A. K., Bianchi, F., Breitenlechner, M., Brilke, S., Chen, X., Craven, J. S., Dias, A., Ehrhart, S., Fischer, L., Flagan, R. C., Franchin, A., Fuchs, C., Guida, R., Hakala, J., Hoyle, C. R., Jokinen, T., Junninen, H., Kangasluoma, J., Kim, J., Kirkby, J., Krapf, M., Kurten, A., Laaksonen, A., Lehtipalo, K., Makhmutov, V., Mathot, S., Molteni, U., Monks, S. A., Onnela, A., Perakyla, O., Piel, F., Petaja, T., Praplan, A. P., Pringle, K. J., Richards, N. A., Rissanen, M. P., Rondo, L., Sarnela, N., Schobesberger, S., Scott, C. E., Seinfeld, J. H., Sharma, S., Sipila, M., Steiner, G., Stozhkov, Y., Stratmann, F., Tome, A., Virtanen, A., Vogel, A. L., Wagner, A. C., Wagner, P. E., Weingartner, E., Wimmer, D., Winkler, P. M., Ye, P., Zhang, X., Hansel, A., Dommen, J., Donahue, N. M., Worsnop, D. R., Baltensperger, U., Kulmala, M., Curtius, J., and Carslaw, K. S.: Reduced anthropogenic aerosol radiative forcing caused by biogenic new particle formation, P. Natl. Acad. Sci. USA, 113, 12053–12058, https://doi.org/10.1073/pnas.1602360113, 2016.
Grell, G. A., Peckham, S. E., Schmitz, R., McKeen, S. A., Frost, G., Skamarock, W. C., and Eder, B.: Fully coupled “online” chemistry within the WRF model, Atmos. Environ., 39, 6957–6975, https://doi.org/10.1016/j.atmosenv.2005.04.027, 2005.
Guenther, A. B., Jiang, X., Heald, C. L., Sakulyanontvittaya, T., Duhl, T., Emmons, L. K., and Wang, X.: The Model of Emissions of Gases and Aerosols from Nature version 2.1 (MEGAN2.1): an extended and updated framework for modeling biogenic emissions, Geosci. Model Dev., 5, 1471–1492, https://doi.org/10.5194/gmd-5-1471-2012, 2012.
Hardacre, C., Mulcahy, J. P., Pope, R. J., Jones, C. G., Rumbold, S. T., Li, C., Johnson, C., and Turnock, S. T.: Evaluation of SO2, SO and an updated SO2 dry deposition parameterization in the United Kingdom Earth System Model, Atmos. Chem. Phys., 21, 18465–18497, https://doi.org/10.5194/acp-21-18465-2021, 2021.
Heald, C. L., Coe, H., Jimenez, J. L., Weber, R. J., Bahreini, R., Middlebrook, A. M., Russell, L. M., Jolleys, M., Fu, T.-M., Allan, J. D., Bower, K. N., Capes, G., Crosier, J., Morgan, W. T., Robinson, N. H., Williams, P. I., Cubison, M. J., DeCarlo, P. F., and Dunlea, E. J.: Exploring the vertical profile of atmospheric organic aerosol: comparing 17 aircraft field campaigns with a global model, Atmos. Chem. Phys., 11, 12673–12696, https://doi.org/10.5194/acp-11-12673-2011, 2011.
Hõrrak, U., Aalto, P. P., Salm, J., Komsaare, K., Tammet, H., Mäkelä, J. M., Laakso, L., and Kulmala, M.: Variation and balance of positive air ion concentrations in a boreal forest, Atmos. Chem. Phys., 8, 655–675, https://doi.org/10.5194/acp-8-655-2008, 2008.
Janssens-Maenhout, G., Crippa, M., Guizzardi, D., Dentener, F., Muntean, M., Pouliot, G., Keating, T., Zhang, Q., Kurokawa, J., Wankmüller, R., Denier van der Gon, H., Kuenen, J. J. P., Klimont, Z., Frost, G., Darras, S., Koffi, B., and Li, M.: HTAP_v2.2: a mosaic of regional and global emission grid maps for 2008 and 2010 to study hemispheric transport of air pollution, Atmos. Chem. Phys., 15, 11411–11432, https://doi.org/10.5194/acp-15-11411-2015, 2015.
Jenkin, M. E., Watson, L. A., Utembe, S. R., and Shallcross, D. E.: A Common Representative Intermediates (CRI) mechanism for VOC degradation. Part 1: Gas phase mechanism development, Atmos. Environ., 42, 7185–7195, https://doi.org/10.1016/j.atmosenv.2008.07.028, 2008.
Kerminen, V.-M., Anttila, T., Lehtinen, K., and Kulmala, M.: Parameterization for Atmospheric New-Particle Formation: Application to a System Involving Sulfuric Acid and Condensable Water-Soluble Organic Vapors, Aerosol Sci. Tech., 38, 1001–1008, https://doi.org/10.1080/027868290519085, 2004.
Kirkby, J., Duplissy, J., Sengupta, K., Frege, C., Gordon, H., Williamson, C., Heinritzi, M., Simon, M., Yan, C., Almeida, J., Trostl, J., Nieminen, T., Ortega, I. K., Wagner, R., Adamov, A., Amorim, A., Bernhammer, A. K., Bianchi, F., Breitenlechner, M., Brilke, S., Chen, X., Craven, J., Dias, A., Ehrhart, S., Flagan, R. C., Franchin, A., Fuchs, C., Guida, R., Hakala, J., Hoyle, C. R., Jokinen, T., Junninen, H., Kangasluoma, J., Kim, J., Krapf, M., Kurten, A., Laaksonen, A., Lehtipalo, K., Makhmutov, V., Mathot, S., Molteni, U., Onnela, A., Perakyla, O., Piel, F., Petaja, T., Praplan, A. P., Pringle, K., Rap, A., Richards, N. A., Riipinen, I., Rissanen, M. P., Rondo, L., Sarnela, N., Schobesberger, S., Scott, C. E., Seinfeld, J. H., Sipila, M., Steiner, G., Stozhkov, Y., Stratmann, F., Tome, A., Virtanen, A., Vogel, A. L., Wagner, A. C., Wagner, P. E., Weingartner, E., Wimmer, D., Winkler, P. M., Ye, P., Zhang, X., Hansel, A., Dommen, J., Donahue, N. M., Worsnop, D. R., Baltensperger, U., Kulmala, M., Carslaw, K. S., and Curtius, J.: Ion-induced nucleation of pure biogenic particles, Nature, 533, 521–526, https://doi.org/10.1038/nature17953, 2016.
Kroll, J. H., Ng, N. L., Murphy, S. M., Flagan, R. C., and Seinfeld, J. H.: Secondary organic aerosol formation from isoprene photooxidation under high-NOx conditions, Geophys. Res. Lett., 32, L18808, https://doi.org/10.1029/2005gl023637, 2005.
Kuhn, U., Ganzeveld, L., Thielmann, A., Dindorf, T., Schebeske, G., Welling, M., Sciare, J., Roberts, G., Meixner, F. X., Kesselmeier, J., Lelieveld, J., Kolle, O., Ciccioli, P., Lloyd, J., Trentmann, J., Artaxo, P., and Andreae, M. O.: Impact of Manaus City on the Amazon Green Ocean atmosphere: ozone production, precursor sensitivity and aerosol load, Atmos. Chem. Phys., 10, 9251–9282, https://doi.org/10.5194/acp-10-9251-2010, 2010.
Kulmala, M.: How particles nucleate and grow, Science, 302, 1000–1001, https://doi.org/10.1126/science.1090848, 2003.
Liu, Y.: Data for “Strong particle production and condensational growth in the upper troposphere sustained by biogenic VOCs from the canopy of the Amazon Basin”, Edmond [data set], https://doi.org/10.17617/3.3ISOYC, 2022.
Mann, G. W., Carslaw, K. S., Spracklen, D. V., Ridley, D. A., Manktelow, P. T., Chipperfield, M. P., Pickering, S. J., and Johnson, C. E.: Description and evaluation of GLOMAP-mode: a modal global aerosol microphysics model for the UKCA composition-climate model, Geosci. Model Dev., 3, 519–551, https://doi.org/10.5194/gmd-3-519-2010, 2010.
Minikin, A., Petzold, A., Ström, J., Krejci, R., Seifert, M., van Velthoven, P., Schlager, H., and Schumann, U.: Aircraft observations of the upper tropospheric fine particle aerosol in the Northern and Southern Hemispheres at midlatitudes, Geophys. Res. Lett., 30, 1503, https://doi.org/10.1029/2002gl016458, 2003.
Napari, I., Noppel, M., Vehkamaki, H., and Kulmala, M.: Parametrization of ternary nucleation rates for H2SO4-NH3-H2O vapors, J. Geophys. Res., 107, 4381, https://doi.org/10.1029/2002JD002132, 2002.
Paluch, I. R. and Knight, C. A.: Mixing and the evolution of cloud droplet size spectra in a vigorous continental cumulus, J. Atmos. Sci., 41, 1801–1815, 1984.
Petters, M. D. and Kreidenweis, S. M.: A single parameter representation of hygroscopic growth and cloud condensation nucleus activity, Atmos. Chem. Phys., 7, 1961–1971, https://doi.org/10.5194/acp-7-1961-2007, 2007.
Ramsay, R., Di Marco, C. F., Sörgel, M., Heal, M. R., Carbone, S., Artaxo, P., de Araùjo, A. C., Sá, M., Pöhlker, C., Lavric, J., Andreae, M. O., and Nemitz, E.: Concentrations and biosphere–atmosphere fluxes of inorganic trace gases and associated ionic aerosol counterparts over the Amazon rainforest, Atmos. Chem. Phys., 20, 15551–15584, https://doi.org/10.5194/acp-20-15551-2020, 2020.
Riccobono, F., Schobesberger, S., Scott, C. E., Dommen, J., Ortega, I. K., Rondo, L., Almeida, J., Amorim, A., Bianchi, F., Breitenlechner, M., David, A., Downard, A., Dunne, E. M., Duplissy, J., Ehrhart, S., Flagan, R. C., Franchin, A., Hansel, A., Junninen, H., Kajos, M., Keskinen, H., Kupc, A., Kurten, A., Kvashin, A. N., Laaksonen, A., Lehtipalo, K., Makhmutov, V., Mathot, S., Nieminen, T., Onnela, A., Petaja, T., Praplan, A. P., Santos, F. D., Schallhart, S., Seinfeld, J. H., Sipila, M., Spracklen, D. V., Stozhkov, Y., Stratmann, F., Tome, A., Tsagkogeorgas, G., Vaattovaara, P., Viisanen, Y., Vrtala, A., Wagner, P. E., Weingartner, E., Wex, H., Wimmer, D., Carslaw, K. S., Curtius, J., Donahue, N. M., Kirkby, J., Kulmala, M., Worsnop, D. R., and Baltensperger, U.: Oxidation products of biogenic emissions contribute to nucleation of atmospheric particles, Science, 344, 717–721, https://doi.org/10.1126/science.1243527, 2014.
Saathoff, H., Naumann, K.-H., Möhler, O., Jonsson, Å. M., Hallquist, M., Kiendler-Scharr, A., Mentel, Th. F., Tillmann, R., and Schurath, U.: Temperature dependence of yields of secondary organic aerosols from the ozonolysis of α-pinene and limonene, Atmos. Chem. Phys., 9, 1551–1577, https://doi.org/10.5194/acp-9-1551-2009, 2009.
Schulz, C., Schneider, J., Amorim Holanda, B., Appel, O., Costa, A., de Sá, S. S., Dreiling, V., Fütterer, D., Jurkat-Witschas, T., Klimach, T., Knote, C., Krämer, M., Martin, S. T., Mertes, S., Pöhlker, M. L., Sauer, D., Voigt, C., Walser, A., Weinzierl, B., Ziereis, H., Zöger, M., Andreae, M. O., Artaxo, P., Machado, L. A. T., Pöschl, U., Wendisch, M., and Borrmann, S.: Aircraft-based observations of isoprene-epoxydiol-derived secondary organic aerosol (IEPOX-SOA) in the tropical upper troposphere over the Amazon region, Atmos. Chem. Phys., 18, 14979–15001, https://doi.org/10.5194/acp-18-14979-2018, 2018.
Scott, C. E., Rap, A., Spracklen, D. V., Forster, P. M., Carslaw, K. S., Mann, G. W., Pringle, K. J., Kivekäs, N., Kulmala, M., Lihavainen, H., and Tunved, P.: The direct and indirect radiative effects of biogenic secondary organic aerosol, Atmos. Chem. Phys., 14, 447–470, https://doi.org/10.5194/acp-14-447-2014, 2014.
Su, H., Rose, D., Cheng, Y. F., Gunthe, S. S., Massling, A., Stock, M., Wiedensohler, A., Andreae, M. O., and Pöschl, U.: Hygroscopicity distribution concept for measurement data analysis and modeling of aerosol particle mixing state with regard to hygroscopic growth and CCN activation, Atmos. Chem. Phys., 10, 7489–7503, https://doi.org/10.5194/acp-10-7489-2010, 2010.
Tröstl, J., Chuang, W. K., Gordon, H., Heinritzi, M., Yan, C., Molteni, U., Ahlm, L., Frege, C., Bianchi, F., Wagner, R., Simon, M., Lehtipalo, K., Williamson, C., Craven, J. S., Duplissy, J., Adamov, A., Almeida, J., Bernhammer, A. K., Breitenlechner, M., Brilke, S., Dias, A., Ehrhart, S., Flagan, R. C., Franchin, A., Fuchs, C., Guida, R., Gysel, M., Hansel, A., Hoyle, C. R., Jokinen, T., Junninen, H., Kangasluoma, J., Keskinen, H., Kim, J., Krapf, M., Kurten, A., Laaksonen, A., Lawler, M., Leiminger, M., Mathot, S., Mohler, O., Nieminen, T., Onnela, A., Petaja, T., Piel, F. M., Miettinen, P., Rissanen, M. P., Rondo, L., Sarnela, N., Schobesberger, S., Sengupta, K., Sipila, M., Smith, J. N., Steiner, G., Tome, A., Virtanen, A., Wagner, A. C., Weingartner, E., Wimmer, D., Winkler, P. M., Ye, P., Carslaw, K. S., Curtius, J., Dommen, J., Kirkby, J., Kulmala, M., Riipinen, I., Worsnop, D. R., Donahue, N. M., and Baltensperger, U.: The role of low-volatility organic compounds in initial particle growth in the atmosphere, Nature, 533, 527–531, https://doi.org/10.1038/nature18271, 2016.
Wang, J., Krejci, R., Giangrande, S., Kuang, C., Barbosa, H. M., Brito, J., Carbone, S., Chi, X., Comstock, J., Ditas, F., Lavric, J., Manninen, H. E., Mei, F., Moran-Zuloaga, D., Pohlker, C., Pohlker, M. L., Saturno, J., Schmid, B., Souza, R. A., Springston, S. R., Tomlinson, J. M., Toto, T., Walter, D., Wimmer, D., Smith, J. N., Kulmala, M., Machado, L. A., Artaxo, P., Andreae, M. O., Petaja, T., and Martin, S. T.: Amazon boundary layer aerosol concentration sustained by vertical transport during rainfall, Nature, 539, 416–419, https://doi.org/10.1038/nature19819, 2016.
Wang, Q., Saturno, J., Chi, X., Walter, D., Lavric, J. V., Moran-Zuloaga, D., Ditas, F., Pöhlker, C., Brito, J., Carbone, S., Artaxo, P., and Andreae, M. O.: Modeling investigation of light-absorbing aerosols in the Amazon Basin during the wet season, Atmos. Chem. Phys., 16, 14775–14794, https://doi.org/10.5194/acp-16-14775-2016, 2016.
Watson, L. A., Shallcross, D. E., Utembe, S. R., and Jenkin, M. E.: A Common Representative Intermediates (CRI) mechanism for VOC degradation. Part 2: Gas phase mechanism reduction, Atmos. Environ., 42, 7196–7204, https://doi.org/10.1016/j.atmosenv.2008.07.034, 2008.
Watson-Parris, D., Schutgens, N., Reddington, C., Pringle, K. J., Liu, D., Allan, J. D., Coe, H., Carslaw, K. S., and Stier, P.: In situ constraints on the vertical distribution of global aerosol, Atmos. Chem. Phys., 19, 11765–11790, https://doi.org/10.5194/acp-19-11765-2019, 2019.
Wendisch, M., Pöschl, U., Andreae, M. O., Machado, L. A. T., Albrecht, R., Schlager, H., Rosenfeld, D., Martin, S. T., Abdelmonem, A., Afchine, A., Araùjo, A. C., Artaxo, P., Aufmhoff, H., Barbosa, H. M. J., Borrmann, S., Braga, R., Buchholz, B., Cecchini, M. A., Costa, A., Curtius, J., Dollner, M., Dorf, M., Dreiling, V., Ebert, V., Ehrlich, A., Ewald, F., Fisch, G., Fix, A., Frank, F., Fütterer, D., Heckl, C., Heidelberg, F., Hüneke, T., Jäkel, E., Järvinen, E., Jurkat, T., Kanter, S., Kästner, U., Kenntner, M., Kesselmeier, J., Klimach, T., Knecht, M., Kohl, R., Kölling, T., Krämer, M., Krüger, M., Krisna, T. C., Lavric, J. V., Longo, K., Mahnke, C., Manzi, A. O., Mayer, B., Mertes, S., Minikin, A., Molleker, S., Münch, S., Nillius, B., Pfeilsticker, K., Pöhlker, C., Roiger, A., Rose, D., Rosenow, D., Sauer, D., Schnaiter, M., Schneider, J., Schulz, C., de Souza, R. A. F., Spanu, A., Stock, P., Vila, D., Voigt, C., Walser, A., Walter, D., Weigel, R., Weinzierl, B., Werner, F., Yamasoe, M. A., Ziereis, H., Zinner, T., and Zöger, M.: ACRIDICON–CHUVA Campaign: Studying Tropical Deep Convective Clouds and Precipitation over Amazonia Using the New German Research Aircraft HALO, B. Am. Meteorol. Soc., 97, 1885–1908, https://doi.org/10.1175/bams-d-14-00255.1, 2016.
Wesely, M. L.: Parameterization of surface resistances to gaseous dry deposition in regional-scale numerical models, Atmos. Environ., 23, 1293–1304, https://doi.org/10.1016/0004-6981(89)90153-4, 1989.
Wexler, A. S., Lurmann, F. W., and Seinfeld, J. H.: Modelling urban and regional aerosols – I. model development, Atmos. Environ., 28, 531–546, https://doi.org/10.1016/1352-2310(94)90129-5, 1994.
Wiedinmyer, C., Akagi, S. K., Yokelson, R. J., Emmons, L. K., Al-Saadi, J. A., Orlando, J. J., and Soja, A. J.: The Fire INventory from NCAR (FINN): a high resolution global model to estimate the emissions from open burning, Geosci. Model Dev., 4, 625–641, https://doi.org/10.5194/gmd-4-625-2011, 2011.
Williamson, C. J., Kupc, A., Axisa, D., Bilsback, K. R., Bui, T., Campuzano-Jost, P., Dollner, M., Froyd, K. D., Hodshire, A. L., Jimenez, J. L., Kodros, J. K., Luo, G., Murphy, D. M., Nault, B. A., Ray, E. A., Weinzierl, B., Wilson, J. C., Yu, F., Yu, P., Pierce, J. R., and Brock, C. A.: A large source of cloud condensation nuclei from new particle formation in the tropics, Nature, 574, 399–403, https://doi.org/10.1038/s41586-019-1638-9, 2019.
Yu, F., Wang, Z., Luo, G., and Turco, R.: Ion-mediated nucleation as an important global source of tropospheric aerosols, Atmos. Chem. Phys., 8, 2537–2554, https://doi.org/10.5194/acp-8-2537-2008, 2008.
Yu, F., Luo, G., Nadykto, A. B., and Herb, J.: Impact of temperature dependence on the possible contribution of organics to new particle formation in the atmosphere, Atmos. Chem. Phys., 17, 4997–5005, https://doi.org/10.5194/acp-17-4997-2017, 2017.
Zaveri, R. A., Easter, R. C., Fast, J. D., and Peters, L. K.: Model for Simulating Aerosol Interactions and Chemistry (MOSAIC), J. Geophys. Res.-Atmos., 113, D13204, https://doi.org/10.1029/2007jd008782, 2008.
Zhao, B., Shrivastava, M., Donahue, N. M., Gordon, H., Schervish, M., Shilling, J. E., Zaveri, R. A., Wang, J., Andreae, M. O., Zhao, C., Gaudet, B., Liu, Y., Fan, J., and Fast, J. D.: High concentration of ultrafine particles in the Amazon free troposphere produced by organic new particle formation, P. Natl. Acad. Sci. USA, 117, 25344, https://doi.org/10.1073/pnas.2006716117, 2020.
Zhu, J. L. and Penner, J. E.: Global Modeling of Secondary Organic Aerosol With Organic Nucleation, J. Geophys. Res.-Atmos., 124, 8260–8286, https://doi.org/10.1029/2019jd030414, 2019.
Zhu, J. L., Penner, J. E., Yu, F. Q., Sillman, S., Andreae, M. O., and Coe, H.: Decrease in radiative forcing by organic aerosol nucleation, climate, and land use change, Nat. Commun., 10, 423, https://doi.org/10.1038/s41467-019-08407-7, 2019.