the Creative Commons Attribution 4.0 License.
the Creative Commons Attribution 4.0 License.
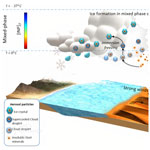
Role of K-feldspar and quartz in global ice nucleation by mineral dust in mixed-phase clouds
Marios Chatziparaschos
Nikos Daskalakis
Stelios Myriokefalitakis
Nikos Kalivitis
Athanasios Nenes
María Gonçalves Ageitos
Montserrat Costa-Surós
Carlos Pérez García-Pando
Medea Zanoli
Mihalis Vrekoussis
Ice-nucleating particles (INPs) enable ice formation, profoundly affecting the microphysical and radiative properties, lifetimes, and precipitation rates of clouds. Mineral dust emitted from arid regions, particularly potassium-containing feldspar (K-feldspar), has been shown to be a very effective INP through immersion freezing in mixed-phase clouds. However, despite the fact that quartz has a significantly lower ice nucleation activity, it is more abundant than K-feldspar in atmospheric desert dust and therefore may be a significant source of INPs. In this contribution, we test this hypothesis by investigating the global and regional importance of quartz as a contributor to INPs in the atmosphere relative to K-feldspar. We have extended a global 3-D chemistry transport model (TM4-ECPL) to predict INP concentrations from both K-feldspar and quartz mineral dust particles with state-of-the-art parameterizations using the ice-active surface-site approach for immersion freezing. Our results show that, although K-feldspar remains the most important contributor to INP concentrations globally, affecting mid-level mixed-phase clouds, the contribution of quartz can also be significant. Quartz dominates the lowest and the highest altitudes of dust-derived INPs, affecting mainly low-level and high-level mixed-phase clouds. The consideration of quartz INPs also improves the comparison between simulations and observations at low temperatures. Our simulated INP concentrations predict ∼ 51 % of the observations gathered from different campaigns within 1 order of magnitude and ∼ 69 % within 1.5 orders of magnitude, despite the omission of other potentially important INP aerosol precursors like marine bioaerosols. Our findings support the inclusion of quartz in addition to K-feldspar as an INP in climate models and highlight the need for further constraining their abundance in arid soil surfaces along with their abundance, size distribution, and mixing state in the emitted dust atmospheric particles.
- Article
(6225 KB) -
Supplement
(1337 KB) - BibTeX
- EndNote
In the atmosphere, aerosol particles are emitted from various natural and anthropogenic sources or formed from precursor gases. Aerosols are important for climate due to their interactions with radiation by scattering and absorbing light (direct climate effect) (Miller et al., 2014) and their ability to act as precursors of clouds, modulating clouds' global distribution and radiative forcing (indirect effect) (IPCC, 2021) along with the hydrological cycle (Seinfeld et al., 2016). The radiative properties and microphysics of clouds are particularly sensitive to aerosol concentration, composition, and size (Boucher, 2015). Aerosols serve as cloud condensation nuclei (CCN) to form liquid cloud droplets (Seinfeld et al., 2016) or as ice nucleating particles (INPs) to form ice crystals (Murray et al., 2012). Ice formation in clouds proceeds for supersaturation with regard to relative humidity with respect to ice (RHi) (Kanji et al., 2019) by both heterogeneous and homogeneous freezing at temperatures lower than −38 ∘C (Liu et al., 2012) and by heterogeneous freezing at warmer temperatures (DeMott et al., 2010; Murray et al., 2012; O'Sullivan et al., 2016; Price et al., 2018). INPs can dramatically reduce the lifetime of shallow clouds (Vergara-Temprado et al., 2018). Also, the presence of INPs may alter the development of deep convective clouds through convective invigoration (Rosenfeld et al., 2008), where enhanced glaciation of clouds increases the release of latent heat and buoyancy, which in turn increases up-draft and vertical development, thus modifying the cloud structure (Lohmann and Gasparini, 2017).
In mixed-phased clouds (MPCs), adding CCN with low INP concentrations suppresses precipitation, and the supercooled clouds are mostly composed of liquid droplets, especially in warm mixed-phase orographic clouds (Fan et al., 2017), so that CCN variations directly modulate the clouds' microphysical properties and radiative forcing (Vergara-Temprado et al., 2018). Increases in INP concentrations tend to glaciate clouds, as water vapor preferentially partitions to ice owing to its equilibrium vapor pressure being lower than that of water (Bergeron–Fiendesein process; Pruppacher et al., 1998b). Given that INPs tend to be orders of magnitude lower in concentration than CCN (Seinfeld et al., 2016), a cloud that glaciates can rapidly redistribute its water from many cloud droplets down to much fewer ice crystals, leading to the formation of precipitation (Pruppacher et al., 1998b; Seinfeld et al., 2016). This entails a shorter cloud lifetime, lower albedo, less reflected radiation, and thus less cooling of the atmosphere than by liquid clouds (DeMott et al., 2010). Secondary processes (ice–ice collisions, droplet riming, and droplet shattering) can further modulate these phenomena (e.g., Georgakaki et al., 2022; Sotiropoulou et al., 2021; Field et al., 2017) so that ice production is promoted even at extremely low INP concentrations (e.g., Morales Betancourt et al., 2012; Sullivan et al., 2018). High concentrations of CCN can further modulate these processes by reducing the efficiency of riming and droplet shattering (e.g., Lance, 2012). Although global aerosol models consider the aerosol–cloud droplet link (Ghan et al., 2011), much work remains to improve the description of ice formation (e.g., Vergara-Temprado et al., 2018), since the dynamics and properties of mixed-phase clouds are among the largest uncertainties in climate models (Fan et al., 2016). Murray et al. (2021) suggest that, in a warmer future climate, ice in mixed-phase clouds will be replaced by liquid water, potentially leading to strong negative feedback. However, it is uncertain whether clouds dampen or amplify warming, and a key factor of this uncertainty is how models estimate the concentration of INPs. Many thermodynamic and microphysical feedbacks determine the glaciation state and properties of mixed-phase clouds, all of which can be affected by perturbations in CCN and INP concentrations (Solomon et al., 2018). Representing mixed-phase cloud properties in models requires an accurate description of INP along with the dynamical drivers of ice and cloud droplet formation. To achieve this, parameterizations of ice nucleating properties of the aerosol components, which are species specific, need to be considered in climate models (Murray et al., 2012).
While several aerosol types, like bioaerosol, black carbon, and dust, have been identified to act as INPs, the most important INP in the global atmosphere is thought to be mineral dust emitted into the atmosphere from deserts and other arid and semi-arid regions (Murray et al., 2012; Seinfeld et al., 2016). This is supported by a plethora of observational and modeling studies; the most direct evidence, perhaps, is measurements showing that ice crystal residuals in mixed-phase clouds are strongly enhanced in mineral dust (Pratt et al., 2009). Initially, most ice nucleation studies focused on clay minerals (Hoose and Möhler, 2012; Roberts and Hallett, 1969). Clays tend to be hydrophilic and, therefore, can act as a CCN, which is a prerequisite for acting as an immersion mode INP (Kumar et al., 2011), and are transported over longer distances from the source regions due to their higher abundance in the smallest dust sizes and hence their longer lifetime (Ginoux, 2017; Kok et al., 2017). More recently, it was shown that, among dust minerals, alkali feldspar, in particular the potassium feldspar (K-feldspar), nucleate ice more efficiently than plagioclase (Ca-feldspar) (Harrison et al., 2016). Also, Na-rich feldspar is very ice active but was found to lose its ice activity with time compared to K-feldspar (Harrison et al., 2016); thus, K-feldspar is the most efficient INP. Note, however, that little is known about the processes governing the IN capability of these minerals, so more work is needed to unravel their effect and contribution for all possible ice formation conditions (Burrows et al., 2022). To explain the enhanced K-feldspar ice activity, it has been suggested that surface −OH groups on feldspars exposed to surface defects (i.e., steps, cracks, and cavities) are responsible for its high ice nucleation efficiency as a result of allowing ice-like structures to form (Kiselev et al., 2017). However, other minerals in the airborne dust remain insufficiently characterized regarding their ice-nucleating activity. Quartz is a major component of airborne mineral dust but has lower ice nucleation ability than K-feldspar, with approximately 2 orders of magnitude less active site density (Harrison et al., 2019). Several studies have shown that quartz can also be a significant source of INPs (Kumar et al., 2019; Harrison et al., 2019). Boose et al. (2016) investigated the representativeness of surface-collected dust for the airborne dust particles and showed a correlation between the INP activity of nine desert dust types and the combined concentration of quartz and K-feldspar, emphasizing the importance of taking into account the presence of both quartz and K-feldspar in the atmosphere for INP modelling.
Several approaches have been proposed to parameterize INPs in climate models (e.g., Lohmann and Diehl, 2006; Spracklen and Heald, 2014; Wang et al., 2014). The first empirical parameterizations to calculate heterogeneous ice nucleation assumed that INP concentrations depend on (i) temperature only (Meyers et al., 1992) or (ii) temperature and the concentration of aerosol particles with a diameter larger than 0.5 µm (DeMott et al., 2010). These parameterizations did not consider additional important complexities such as chemical composition variations over time and space. The simplest and most performant parameterization is based on the ice-nucleating active surface sites concept (Vali et al., 2015). This approach adopts the so-called singular hypothesis that assumes that each droplet contains a single ice-nucleating particle that is active in a given temperature interval and that the time dependence (stochasticity) of nucleation is of secondary importance (Vali et al., 2015). This hypothesis allows for the formulation of a very simple link between aerosol chemical composition (surface structure), size distribution, and an ice nucleation spectrum constrained by experimental data (e.g., Barahona and Nenes, 2009), from which one can predict INPs as a function of supersaturation (in the deposition mode) or temperature (in the immersion mode). Immersion freezing occurs when ice nucleation is initiated by an INP that becomes immersed in an aqueous solution or water droplet via activation of CCN during liquid cloud formation, and it is thought to be the most dominant freezing mechanism for mixed-phase clouds (Kanji et al., 2017). This is the ice formation pathway we focus on in this study. Different materials are assumed to have a different ice active density (ns) per surface unit and a critical temperature below which an INP with multiple nucleation sites activates in the immersion mode to form ice (Hoose et al., 2010; Murray et al., 2012). Only a few INP parameterizations for immersion-freezing mode explicitly consider ns, and it is only recently that models that adopt this level of compositional complexity have emerged, mainly considering one type of INP from the most active mineral K-feldspar (Atkinson et al., 2013; Vergara-Temprado et al., 2017).
Harrison et al. (2019) proposed a new parameterization based on experimental work in immersion-freezing mode by testing 10 naturally occurring quartz samples that highlighted the variability in their ice-nucleating ability. It was observed that the quartz group of minerals is generally less active than K-feldspars, with the most active quartz samples being of similar activity than some K-feldspars. It was also found that the ice-nucleating activity of some quartz samples is very sensitive to aging when exposed to air and water, contrary to other quartz and K-feldspar samples. This variability indicates that mineral dust particles do not nucleate ice through a lattice-matching mechanism. The latter is consistent with recent observations that ice nucleation occurs at active sites (Holden et al., 2019). However, once in the atmosphere, mineral dust particles undergo aging through the condensation of sulfates, nitrates, and secondary organic aerosol material, becoming soluble and thus promoting wet scavenging (Abdelkader et al., 2017). The occurrence of such atmospheric aging of INPs via coating by acids or water-soluble organics that modifies the ice-nucleating efficiency of aerosols is supported by the detection of sulfate and/or nitrate peaks of the IN-active particles using single-particle analysis by micro-Raman spectroscopy (Iwata and Matsuki, 2018). In addition, the solution environment may substantially impact the ice nucleation efficiency of both quartz and feldspar (Whale et al., 2018; Kumar et al., 2019), but the study of the impact of this process on INPs is beyond the scope of the present study.
Here we present, to our knowledge, the first global modelling study of the role of airborne mineral dust as a precursor of INPs, accounting for both dust's quartz and feldspar contents. We simulate the three-dimensional global distribution of INP concentrations, evaluate the model results against observations, and investigate (a) the importance of quartz relative to feldspar in INP formation and (b) potentially missing INP sources other than dust minerals. To achieve this, we use a well-documented global 3-D chemistry transport model to simulate the global temporally and spatially varying immersion-mode distributions of INPs from feldspar and quartz minerals.
2.1 Global modeling
For the present study, we use the global 3-D chemistry transport model TM4-ECPL (Daskalakis et al., 2016, 2022; Myriokefalitakis et al., 2016, 2015; Kanakidou et al., 2020), driven by ERA-interim reanalysis meteorological fields produced with the European Centre for Medium-Range Weather Forecasts (ECMWF) meteorological model (Dee et al., 2011). The specific model version has a horizontal resolution of 3∘ longitude by 2∘ latitude with 25 hybrid pressure vertical levels from surface up to 0.1 hPa (about 65 km) and uses a model time step of 30 min. The model simulates tropospheric chemistry accounting for non-methane volatile organics, all major aerosol components (including dust), and the atmospheric cycles of nitrogen, iron, and phosphorus (Tsigaridis et al., 2014; Tsigaridis and Kanakidou, 2007; Kanakidou et al., 2016, 2020; Myriokefalitakis et al., 2015, 2016). Uncertainties in the simulations resulting from the use of different emission databases have been investigated by Daskalakis et al. (2015). The model considers lognormal aerosol distributions in fine and coarse modes and allows hygroscopic growth of particles, as well as removal by large-scale and convective precipitation and gravitational settling. In-cloud and below-cloud scavenging are parameterized in TM4-ECPL, as described in detail by Jeuken et al. (2001). In-cloud scavenging of water-soluble gases accounts for the solubility of the gases (effective Henry's law coefficients; Myriokefalitakis et al., 2011; Tsigaridis et al., 2006 and references therein). Details on the emissions used in the model are provided in Myriokefalitakis et al. (2015, 2016) and Kanakidou et al. (2020). Dust emissions are calculated online, as described by Tegen et al. (2002), and implemented as in van Noije et al. (2014). Dust aerosol (including feldspar and quartz) emissions are represented by lognormal distributions in fine and coarse modes with dry-mass median radii (lognormal standard deviation) of 0.34 (1.59) and 1.75 µm (2.00), respectively, and particle density is equal to 2650 kg m−3 (Ginoux et al., 2004; Tegen et al., 2002). We assume that all aerosols are externally mixed. Typical lognormal distribution equations are used to convert mass to number concentrations of aerosols in each grid box of the model.
2.2 K-feldspar and quartz emissions
To calculate the quartz and K-feldspar emissions, we use the global soil mineralogy atlas of Claquin et al. (1999), including the updates proposed in Nickovic et al. (2012). The atlas of Claquin et al. (1999) provides mineral fractions for eight major minerals, including quartz and feldspar, in arid and semi-arid regions for two soil size fractions: clay (up to 2 µm) and silt (from 2 to 50 µm). Claquin et al. (1999) exclusively apportion feldspar to the silt size fraction, but feldspar is also observed in the smaller sizes, both in the soil (e.g., Journet et al., 2014) and the airborne dust (e.g., Kandler et al., 2009). Following Atkinson et al. (2013), we added feldspar in the clay size fraction of the soil by scaling the feldspar fraction in the silt size fraction of the soil with the clay-to-silt ratio of quartz. Since Claquin et al. (1999) only estimated total feldspar, based on observations, we also assumed that K-feldspar represents 35 % of feldspar (Atkinson et al., 2013).
Atkinson et al. (2013) calculated the emission of K-feldspar as the product of dust emission flux and the soil fractions of K-feldspar. Here, we additionally account for the known differences between soil and emitted mineral fractions. The soil samples that constitute the basis of soil mineralogical atlases are subject to destructive analytical techniques. As a result, the aggregates originally present in the soil are more effectively disturbed than through wind erosion processes, hence overemphasizing the fine fraction of clay minerals compared to their actual abundance at emission (Perlwitz et al., 2015a). We calculated the emitted mass fractions of quartz and K-feldspar in the fine and coarse modes based on brittle fragmentation theory (BFT) from Kok (2011). BFT restores soil clay-sized minerals (mainly illite, kaolinite, and smectite), created by wet sieving, into emitted coarse aggregates. As a consequence, the fractional contributions of quartz and K-feldspar in the emitted coarse dust are reduced relative to their fractions in the silt size of the soil (Pérez García-Pando et al., 2016; Perlwitz et al., 2015a, b). Finally, the corresponding accumulation and coarse-mode emission of each mineral is calculated by applying the respective mineral-emitted mass fractions to the dust emission flux.
2.3 Calculation of INP concentrations
The influence of mineralogy on INP concentrations is parameterized in TM4-ECPL using the singular approximation of ice-nucleating activities of aerosols and based on laboratory-derived active site parameterizations provided in Harrison et al. (2019). These are good approximations for the majority of feldspars and quartz studied in the laboratory. The parameterization for K-feldspar is valid between −3.5 and −37.5 ∘C and captures the observed plateau in active-sites density (ns(T)) below −30 ∘C, reproducing higher ns(T) values at temperatures warmer than −10 ∘C. In addition, Harrison et al. (2019) provide a new parameterization for quartz minerals to constrain their role as INPs that is valid for a temperature range from −10.5 to −37.5∘C. In this study, the parameterizations are exclusively applied within the valid temperature limits, since outside these limits, the parameterizations yield non-realistic results. These parameterizations have an uncertainty, expressed as the standard deviation for log (ns(T)), of about 0.8 for both quartz and K-feldspar INPs that corresponds to 1 order of magnitude in their concentrations. Part of this uncertainty is derived from impurities that influence how individual quartz samples fracture, impacting the presence of active sites (Harrison et al., 2019). As discussed in the introduction, the ice-nucleating ability of the different minerals is determined from laboratory experiments using artificial mineral samples (Kumar et al., 2019), which may not be representative of realistic atmospheric conditions. Also, data analysis provided in Atkinson et al. (2013) suggests that aggregation of feldspar particles resulting in reduced surface area is, at most, a minor effect in the ice nucleation efficiency compared with the differences in the active-sites density of different types of K-feldspar (factor of 6) (Harrison et al., 2016). Additionally, considerable differences in simulated INP numbers using laboratory-based and field-based INP parameterizations are attributed to biological nanoscale fragments attached to mineral dust particles (O'Sullivan et al., 2016). These effects are not considered in the present study.
The ice nucleation active surface site density that is derived by the spectrum of ice-nucleating properties and for a polydisperse aerosol sample is given by the Poisson distribution:
where IN is the ice number concentration formed on the aerosol based on parameterizations provided in Harrison et al. (2019), and ni,j is the total aerosol number concentration, where indexes i, j correspond to aerosol type (quartz or K-feldspar) and size mode (accumulation or coarse mode), respectively. Si,j is the individual aerosol particle mean surface area in size mode j (see Niemand et al., 2012, for details).
In order to estimate aerosol surface area, we assume that each mineral dust particle is spherical and externally mixed (Atkinson et al., 2013; Vergara-Temprado et al., 2017). As discussed in Harrison et al. (2019), Atkinson et al. (2013) found that fully internally mixed feldspar particles produce the same INP concentrations as externally mixed particles at temperatures above −25 ∘C but lead to higher INP concentrations by 1 to 2 orders of magnitude at the lowest temperatures of validity of the parameterization. In addition, in the present study, removal mechanisms by physical processes, such as sedimentation, deposition, and scavenging, are applied directly to all dust particles and not preferentially to INPs.
There are two different ways to represent simulated INP concentrations by the model, either as potential INPs that can activate to ice at a fixed temperature ([INP]T) or as ambient INPs that are calculated to be active in the model's ambient local atmospheric temperature. [INP]ambient is a useful way of looking at the INP concentration relevant to non-deep convective mixed-phase clouds, while [INP]T can be an indicator for the distribution of INP concentrations at temperatures that will affect clouds with vertical extent like deep-convective systems (Vergara-Temprado et al., 2017), as illustrated in Fig. 1. Additionally, because most measurements are made by exposing particles to specific temperatures, depending on instrumentation, [INP]T is a pertinent quantity when comparing modeled and observed INP concentrations.
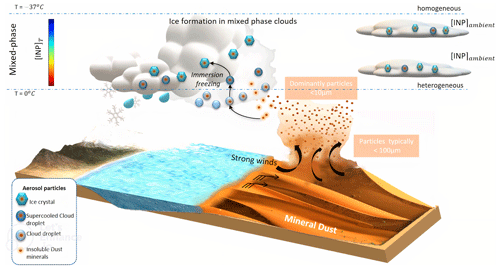
Figure 1Illustration of the formation of INPs from mineral dust aerosols and the two ways in which we display INP concentrations in the present study: [INP]ambient, calculated at ambient model temperature relevant to non-deep convective mixed-phase clouds using the ambient temperature in the model temperature level (right part of the figure), and [INP]T, calculated at a fixed temperature relevant for vertically extended clouds such as deep-convective systems (left part of the figure). The temperature color scale from 0 to −37 ∘C is provided to the left (see text). The figure is modified by combining the ones in Kanji et al. (2017) (Fig. 1), Harrison et al. (2019) (Fig. 1), and Vergara-Temprado et al. (2017) (Fig. 3).
3.1 Dust
Airborne-dust concentration is of paramount importance to this work, since dust directly affects the amount of INPs in the atmosphere. Consequently, we evaluate modeled dust by comparing it with monthly averaged dust observations from stations that are in the outflow of the Sahara and with worldwide climatological observations. The climatological annual-means comparison allows us to validate the geographical distribution of sources and transported dust regions, while the monthly means comparison reveals if the intra-annual variability can be well captured by the model.
We first evaluate the dust mass simulations against monthly averaged dust observations at nine ground-based stations between 2000 and 2017. Fig. S1 in the Supplement shows the location of these stations plotted over the simulated dust concentration for summer 2015. All stations are in areas suitable for studying emissions of Saharan dust and its atmospheric transport. Four stations (M'Bour, Bambey, Cinzana, and Banizoumbou) are located at the edge of the Sahel, the major natural dust source of the region (Lebel et al., 2010); three stations (Miami, Barbados, and Cayenne) are on the American continent, downwind of the dust Atlantic transport (Zuidema et al., 2019; Prospero et al., 2020); and two stations, Finokalia (Crete, Greece) (Mihalopoulos et al., 1997; Kalivitis et al., 2007) and Agia Marina (Cyprus) (Kleanthous et al., 2014; Pikridas et al., 2018), lie on the dust transport route crossing the Mediterranean. Figure 2 depicts the comparison of model dust aerosol concentrations with observations on a monthly mean basis (correlation coefficient R=0.81). The methodology to derive dust concentrations from the aerosol observations at these sites is discussed in the Supplement. The mean bias between model and observations is 8.3 µg m−3. The total average dust concentration observed at the selected locations and during the studied period 2000–2017 is approximately 43.3 µg m−3. Thus, the mean bias corresponds to 5 % of the total concentration. The relative error increases with decreasing concentrations, and only a few outliers are not within 1 order of magnitude of the observations in Fig. 2, mainly corresponding to observations at the Caribbean and American stations.
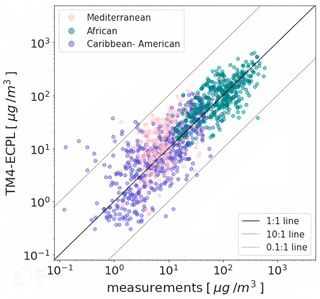
Figure 2Monthly averaged model-simulated versus observed dust concentrations at the selected locations in µg m−3. The continuous black line is the 1:1 line, while the dashed lines are the 10:1 and 1:10, top and bottom lines, respectively. The stations are geographically grouped: African stations in green (M'bour, Bambey, Cinzana, Banizoumbou), American stations in violet (Miami, Barbados, Cayenne), and Mediterranean stations in pink (Agia Marina and Finokalia). Simulations were run with a 3∘ × 2∘ spatial resolution.
We further evaluate modeled dust by comparing with climatological, globally distributed observations of dust surface concentration and deposition for the years from 2009 to 2016, when we also perform the comparison between observed and modeled INP concentrations (see below, Sect. 3.2). Climatological annual means of dust surface from the Rosenstiel School of Marine and Atmospheric Science (RSMAS) of the University of Miami (Arimoto et al., 1995; Prospero, 1996, 1999) and the African Aerosol Multidisciplinary Analysis (AMMA) (Marticorena et al., 2010) are compared with the multi-annual model mean surface dust concentration (Fig. 3a). We also compare the modeled deposition dust fluxes with the observations for modern climate compiled by Albani et al. (2014). Measurements from 110 different locations and the constrained mass fraction for particles with a diameter lower than 10 µm to conform to the range of simulated sizes are compared to modeled deposition dust fluxes (Fig. 3b). Table S1 summarizes the statistical parameters used to validate the model, and the locations (and regions) of the various observations are depicted in Fig. S2. The correlation coefficient (R) reveals the linear relationship between model results and observations, while the normalized mean bias (nMB) and the normalized root mean square error (nRMSE) are the measures of the mean deviation of the model from the observations due to random and systematic errors. All the equations that are used for the statistical analysis of model results are provided in the Supplement (Eqs. S1–S3).
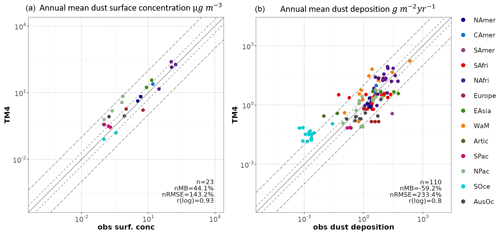
Figure 3Comparison of (a) the modeled annual mean dust surface concentration for the years 2009–2016 against climatological mean values from RSMAS sites and AMMA campaign, and (b) modeled annual dust deposition flux averaged for the same period against observations compiled in Albani et al. (2014) from several sources. The solid line represents the 1:1 correspondence, the long dashed lines show the 10:1 and 1:10 relationships, and short dashed lines correspond to a factor of 2, respectively. Colors indicate measurement locations listed in the figure legend.
The geographical distribution of the available concentration stations (23 in total) in Fig. 3a covers locations close to emission sources (AMMA stations over the Sahelian dust transect) and in both transport and remote regions (RSMAS). The overestimation of the dust surface concentration already shown at the monthly scale is also observed in the comparison with annual mean climatological observations, with an overall normalized mean bias of 44.1 %. Errors are generally larger after transport to remote regions, particularly over the North Pacific, than over source regions (Fig. 3a). When it comes to deposition, the errors are larger than in the representation of the surface concentration fields – in particular, more overestimations are found over the Southern Ocean and the Arctic than over downwind sources (Fig. 3b), while concentrations over Europe and South America are largely underestimated. They are, however, kept within the 1-order-of-magnitude differences, except for those points located over the Southern Ocean, where overestimations are significant. The aerosol concentrations and deposition fluxes in such remote regions are usually low; thus, small differences are emphasized in relative terms. There may be multiple causes for the discrepancy between modeled and observed fluxes (e.g., an excessive modeled fine fraction of aerosols, differences in the wet deposition fluxes caused by differences in precipitation, differences in the observed and modeled period, or uncertainties associated with the observations themselves). The main purpose of this comparison is to assess the ability of the model to reproduce the geographical distribution of dust in the atmosphere, which is found to be satisfactory; exploring further the issues behind the deviation in a particular remote region is out of the scope of this work.
3.2 INPs
In Fig. 4, we compare the INP concentrations calculated by the TM4-ECPL model with available INP observations from the databases of BACCHUS (Impact of Biogenic versus Anthropogenic emissions on Clouds and Climate: towards a Holistic UnderStanding) (http://www.bacchus-env.eu/in/index.php, last access: 24 March 2019) and Wex et al. (2019) (https://doi.pangaea.de/10.1594/PANGAEA.899701, last access: 14 February 2022). These data span the years 2009 to 2016 and originate from different campaigns (locations are shown in Fig. S3 in the Supplement). For this comparison, [INP]T concentrations are calculated at the temperature at which measurements were performed. All model results are compared to observations for the specific month and year of the observations, except the observed dataset by Yin et al. (2012), which covers temporally scattered measurements (between 1963 to 2003). This dataset is compared to the modeled multi-annual monthly mean INP concentration considering the years 2009 to 2016. The color bar shows the corresponding temperature at which the measurement is performed (Fig. 4a) or the modeled dust aerosol mass concentration (Fig. 4b). These different color bars allow us to determine if the bias of the model can be correlated with the temperature of the observed INPs (e.g., highly active INPs, reddish symbols in Fig. 4a) or with modeled dust mass (e.g., low modeled dust mass, blueish symbols in Fig. 4b). The model agrees reasonably well with observations of INPs (R=0.84, Table S2) at the full temperature range of the measurements when dust is present in significant amounts (dust concentrations larger than 10−1 µg m−3) (Fig. 4b) and at temperatures lower than −10 ∘C (Fig. 4a). Overall, the model reproduces about 51 % of the observations within 1 order of magnitude and about 69 % within 1.5 orders of magnitude (Table S2).
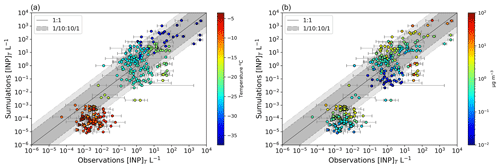
Figure 4Comparison of [INP]T concentrations calculated at the temperature of the measurements against observations (dates and locations provided in the Table S3 in the Supplement). For each observation, the INP concentration is calculated at the temperature corresponding to the instrument temperature of the measurement. Circle markers correspond to the BACCHUS database, and triangles correspond to the Wex et al. (2019a) database. The dark gray dashed lines represent 1 order of magnitude of difference between modeled and observed, and the light-gray dashed lines represent 1.5 orders of magnitude of difference. The simulated values correspond to monthly mean concentration, and the error bars correspond to the observed error of monthly mean INP values. The color bars show the corresponding instrument temperature of the measurement in Celsius (a) and the modeled dust aerosol mass concentration in µg m−3 (b).
The model is not efficient enough in simulating highly ice-active INPs observed in both the Wex (triangles) and BACCHUS (circles) databases (Fig. 4a, red spots corresponding to high temperatures). The temperature range of these measurements is very close to the temperature limits of the Harrison et al. (2019) parameterization. Since the quartz parameterization temperature limit is at −10.5 ∘C, INP observations measured between −3.5 and −10.5 ∘C are compared with simulated INPs derived from K-feldspar only. Fig. S4 in the Supplement depicts the comparison to observations using quartz and K-feldspar separately. This figure reveals that INPs from quartz can improve the comparison with observations at low temperatures, which is consistent with the suggestion of Boose et al. (2016) that, for temperatures between −33 to −37 ∘C, quartz can be a significant contributor to INP concentration. Figure S4d clearly shows that consideration of quartz together with K-feldspar INPs improves the comparison with observations by moving the respective points closer to the 1:1 line. This is particularly true for high INP concentrations at low temperatures (below −30∘C) and for relatively low INP concentrations at temperatures around −20∘C. Overall, about 3 % more data points are within 1.5 orders of magnitude from the observations when accounting for quartz INPs in addition to feldspar INPs (Table S2). Additionally, modeled INP concentrations are strongly underestimated compared to observations for temperatures above −25 ∘C. Si et al. (2019) pointed out that mineral dust was a major contributor to the INP population at temperatures lower than −25 ∘C. They also found that, for three coastal sites, modeled INP concentrations based on K-feldspar as the only INP precursor agreed well with INP measurements at −25 ∘C, but measurements at −15 ∘C were underestimated, indicating a missing source of INPs that activates at temperatures higher than −25 ∘C. These results on the importance of dust as an INP for different temperature ranges agree with our study, as can also be seen in Fig. S4c in the Supplement.
Figure 4b reveals that the observed high concentrations of INPs correspond to high dust mass concentrations. Additionally, the observed low concentrations of INPs correspond to particles that activate at relatively high temperatures (higher than −10 ∘C; reddish symbols in Fig. 4a) and low mass dust concentrations (blueish symbols in Fig. 4b). Thus, our model underestimates measurements corresponding to low dust mass concentrations (blueish symbols in Fig. 4b). As shown in Fig. 4a, these low dust mass points correspond to measurements performed at temperatures around −20 and −25 ∘C. Therefore, these INP observations not reproduced by our model when accounting only for dust-originating INPs are probably not solely affected by airborne mineral dust, pointing to other aerosol sources contributing to INPs, as discussed below.
Fig. S5 in the Supplement depicts, globally, the regions and months where the model overestimates (blueish) and underestimates (reddish) INP observations. Improving the representation of the dust cycle could reduce the bias of INP simulations. The model underestimate of the dust surface concentration observations over Europe and the Southern Ocean and the dust deposition fluxes over South Africa and West Asia, discussed in Sect. 3.1, may at least partly explain the underestimation by the model of the observed INP concentrations (close to 2 orders of magnitude, reddish points) in these regions, as depicted in Fig. S5 in the Supplement. Additionally, model deviation from measurements could be attributed to potential inaccuracies in the simulated content of quartz and K-feldspar due to uncertainties in the dust cycle and in the emitted mineral fractions (Perlwitz et al., 2015a; Pérez García-Pando et al., 2016). Furthermore, the boxes in Fig. S5 in the Supplement depict the climatological monthly mean bias (observations – model) at specific regions (Arctic, Europe, China, North America, Central America, North Africa, South Africa, and West Asia–Middle East). This analysis shows that observed INP concentrations are underestimated by 2 orders of magnitude over Europe during autumn and over West Asia–Middle East during autumn and winter.
The overall agreement between the model results and observations is reasonable (see also Table S2 in the Supplement), but there are significant discrepancies. These differences between model results and observations could be attributed to several factors, such as the omission of the contribution of marine organic (Wilson et al., 2015) and terrestrial biogenic aerosols (Myriokefalitakis et al., 2017; Spracklen and Heald, 2014). Ice nucleation at temperatures in the vicinity of zero is typically related to macromolecules from biogenic entities such as bacteria, fungal spores, pollen, and marine biota. These ice-active macromolecules nucleate ice from just below 0 down to roughly −20 ∘C (O'Sullivan et al., 2018; Kanji et al., 2017; Murray et al., 2012). Indeed, based on air mass back-trajectories analysis, Wex et al. (2019) (triangles in Fig. 4a) suggested that terrestrial locations in both the Arctic and the adjacent sea were possible source areas for highly active INPs derived from material of biogenic origin. In addition, mineral dust particles likely only become ice active at low temperatures (see Sect. 2.3), but they may be carriers of biogenic ice-active macromolecules (O'Sullivan et al., 2014; Hill et al., 2016; O'Sullivan et al., 2016), which enhance dust nucleation activity at higher temperatures. Thus, although some biological nanoscale fragments could be attached to mineral dust particles (Froehlich-Nowoisky et al., 2015; Violaki et al., 2021; O'Sullivan et al., 2016), their effect on the ice-nucleating activity of dust was not considered here. Furthermore, errors may be partly due to atmospheric processes such as aging, resulting in ice ability degradation (leading to positive model bias) or a possible underestimation of the concentration of these transported aerosol species (leading to negative model bias). These processes are currently neglected in our model, but the sensitivity of the INP simulations to these uncertainties is the subject of an ongoing complementary study.
Figure 5a presents the simulated distributions of K-feldspar and quartz particles present at an atmospheric pressure level of 600 hPa that are able to freeze in the immersion mode at −20 ∘C and form ice crystals, hereafter called [INP]−20. These conditions are representative of mixed-phase clouds' glaciation and allow both quartz and K-feldspar to activate and act as INPs. [INP]−20 distributions are of interest for their impact on clouds formed in deep-convective systems experiencing large vertical velocities (Fig. 1). They are mainly observed in subtropical and tropical regions. [INP]−20 concentrations derived from K-feldspar and quartz (Fig. 5a) contribute much more to mid-latitude, mid-level mixed-phase clouds in the Northern Hemisphere (NH) than in the Southern Hemisphere (SH) due to the location of dust sources and long-range atmospheric transport patterns that favor dust concentration in the NH. However, there are also dust-related INP sources in the SH, such as those from Patagonia, South Africa, and west Australia, that yield considerable [INP]−20 concentrations (larger than 10−2 L−1).
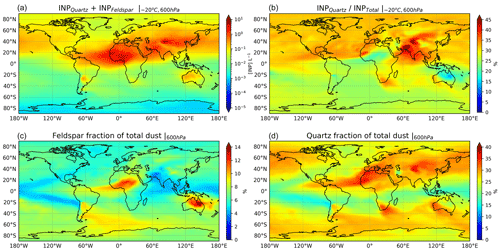
Figure 5Annual-mean distributions calculated by TM4-ECPL for the year 2015 of (a) INP concentrations for an activation temperature of −20 ∘C based on K-feldspar and quartz at a pressure level of 600 hPa, (b) ratio of INPs from quartz over total INPs from dust (K-feldspar and quartz) at the same conditions, (c and d) mass ratios of feldspars (c) and quartz (d) in percentages over total dust mass at 600 hPa.
To investigate the spatial variability of the contribution of each mineral to potential primary ice crystals concentration at −20 ∘C, we also present the ratio of INPs from quartz ([INP]quartz) to the INPs derived from both quartz and K-feldspar ([INP]total) at that temperature (Fig. 5b). This analysis shows regions at the pressure level of 600 hPa and the temperature of −20 ∘C, where [INP]quartz has the potential to significantly contribute to the total ice crystals concentration derived by immersion freezing on dust particles – in particular, we attribute to quartz 30 % of the total [INP]total over the North Atlantic and southern USA and more than 40 % of that over India and Eurasia.
The areas most affected by ice crystals derived from quartz are the regions of the southern Saudi Arabian Peninsula, India, northern Indonesia, and Eurasia (Fig. 5b), where high ratios of quartz minerals to dust mass (Fig. 5d) and high concentrations of derived ice crystals of about 0.5 L−1 are simulated (Fig. 5a). The mass contribution of quartz to the dust particles originating from Saudi Arabia and the Gobi Deserts exceeds 35 % (Fig. 5d). At the same time, the quartz contribution to ice crystals formed by immersion freezing on dust particles is 35 %–40 % (Fig. 5b). When the mass of quartz is 6 times that of feldspars (Fig. 6), the quartz-derived ice crystal concentration ([INP]quartz) constitutes more than 35 %–45 % of total ice crystals concentration derived from dust (Fig. 5b). Furthermore, the North Atlantic and, generally, the NH are likely affected by ice formation from both quartz and K-feldspar. In contrast, the South Atlantic is affected by K-feldspar INPs originating from the Patagonia desert, since 75 % of ice crystals potentially formed at −20 ∘C are derived from K-feldspar, and the remaining 25 % is from quartz (Fig. 5b). Additionally, high percentages of quartz minerals and low feldspars (about 6 %) in terms of mass are calculated over South Africa, originating from the Kalahari Desert. There, 35 % of the total INP concentrations (around 10−2L−1) originate from quartz particles (Fig. 5c–d).
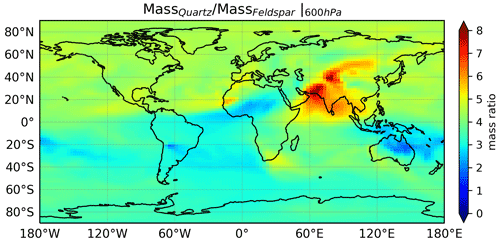
Figure 6Annual mean ratio of quartz to feldspar mineral masses in dust, as calculated by dividing quartz mass by feldspar mass at 600 hPa.
As expected, concentrations of primary ice crystals derived from dust at the model temperature, [INP]ambient, follow the air temperature distribution, showing the highest modeled values roughly poleward of 50∘ in both hemispheres (Fig. 7 for model dust concentrations and temperature at 600 hPa). The contributions of both minerals to primary ice crystals are comparable (Fig. 7c–d); [INP]quartz appears as important as [INP]feldspar around 40∘ and poleward of 70∘. Overall, the number concentration of primary ice formed by immersion freezing on dust particles strongly depends on the ambient temperature and the mass concentrations of these minerals, with positive dependence on mass and negative dependence on temperature.
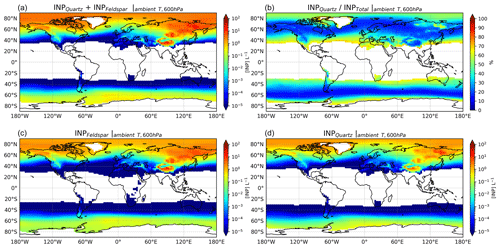
Figure 7Annual-mean distributions of ice-nucleating particles number concentrations [INP]ambient, calculated by TM4-ECPL at ambient (model's) temperature and 600 hPa based on the presence both of K-feldspar and quartz (a). Ratio of INPs from quartz over total INPs from K-feldspar and quartz at the same conditions (b). Panels (c)–(d) show the corresponding INP concentrations derived individually from each mineral: K-feldspar (c) and quartz (d).
Figure 8a shows the annual zonal-mean distribution of primary ice crystals derived from both minerals. Total primary ice crystals ([INP]total) maximize at approximately 600–500 hPa below −25 ∘C in mid-latitudes at local model temperature (Fig. 8a), with K-feldspar-derived ice crystals accounting for the largest fraction (Fig. 8b). This behavior is attributed to the high ice activity of K-feldspar and its temperature dependence. However, at lower altitudes and below −12 ∘C, low [INP]total concentrations (<10−2 L−1) are calculated and are mainly derived from quartz dust particles (60 %) (Fig. 8b). This outcome is attributed to quartz's high number concentration between 30–40∘ N at and below 700 hPa, partially associated with higher local quartz than feldspar emissions from Asian dust sources (Claquin et al., 1999; Nickovic et al., 2012). At temperatures below −25 ∘C, the quartz contribution becomes increasingly important, with increases in INP concentration when the temperature decreases, reaching up to 50 % at −35 ∘C (Fig. 8b). These findings agree well with Ilić et al. (2022) and Boose et al. (2016), who showed that quartz could significantly contribute to INPs at temperatures between the homogeneous freezing limit and −33 ∘C. Overall, [INP]quartz dominates at the lowest and the highest altitudes of dust-derived INPs (Fig. 8b; reddish colors). This is clearly demonstrated in Fig. S6 in the Supplement for Eurasia, where at the range of 700–900 and around 450 hPa model pressure levels the contribution of [INP]quartz to total ice crystals from immersion freezing on dust particles exceeds 60 %. Figure S7 in the Supplement shows that, in the Southern Hemisphere, quartz contribution to total INPs is about 40 %. Consequently, [INP]feldspar is expected to affect mid-altitude clouds, while [INP]quartz is expected to affect both the low-altitude clouds and the high-altitude cold clouds. The present study emphasizes that INP concentrations could be significantly affected not only by K-feldspar, as generally thought, but also by quartz. The evaluation of the climate impact of these INPs is a subject of ongoing research. It requires the incorporation of parameterizations, such as those used here, into a climate model able to simulate ice formation and its interactions within the climate system.
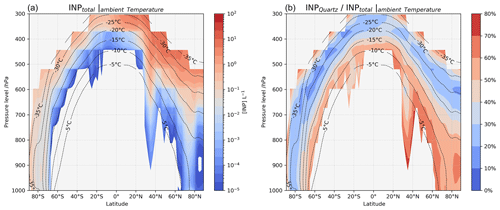
Figure 8Annual zonal-mean profiles of [INP]ambient number concentration for 2015, calculated by TM4-ECPL and accounting for K-feldspar and quartz. The black contour dashed lines show the annual mean temperature of the model. The color map shows the INP concentration derived from feldspar and quartz dust aerosols [INP]total (a) and the ratio of INPs from quartz to the total INPs from both minerals (b).
The contribution of K-feldspar and quartz dust minerals as ice-nucleating particles has been investigated in this study using a three-dimensional global chemistry transport model as a first step towards their incorporation in a climate model. Laboratory-derived parameterizations based on the singular description and aerosol chemical composition have been incorporated into the model together with an explicit representation of the dust mineralogy that considers regional variations in the composition of the dust sources and relies on the brittle fragmentation theory to derive the size-distributed mineral abundances in the atmosphere. To our knowledge, this is the first time a global chemistry transport model considers the contribution of both quartz and K-feldspar to predict INP concentrations.
Quartz particles have lower ice-active density (Harrison et al., 2019) but are more abundant in airborne dust than K-feldspar, thus partly compensating for their lower ice-nucleating activity with respect to K-feldspar. We find that [INP]quartz dominates at lower altitudes over locations with abundant dust emissions but low INP concentrations. There are regions of the atmosphere, in particular over middle and high latitudes of Asia, where quartz contributes over 60 % (at ambient model temperature) to the total INP concentration derived from dust aerosols represented by quartz and K-feldspar. The quartz contribution increases when temperature decreases, resulting in high INP concentrations at temperatures below about −30 ∘C and at high altitudes. Consideration of quartz-derived INPs improves the comparison with observations for high INP concentrations at low temperatures (below about −30 ∘C) and relatively low INP concentrations at temperatures around −20 ∘C. These results highlight the important role of quartz in increasing INP concentrations, mainly over the mid-to-high latitudes of both hemispheres.
Overall, the simulated concentrations of INPs from two dust components agree with the observational data from the BACCHUS and Wex et al. (2019) databases within 1.5 orders of magnitude. Statistical analysis of the comparison of the modeled annual mean dust surface concentration has shown that the model underestimates dust concentration over Europe and the Southern Ocean, potentially explaining part of the bias between modeled and observed INP concentrations. Furthermore, the omission of marine and terrestrial biological INP sources, dust mineralogy uncertainties, and atmospheric processes such as aging and in-cloud removal could also explain the model's discrepancies. The evaluation of the importance of these factors on INP simulated number concentrations is the topic of ongoing studies. As importantly, the heterogeneously formed ice crystals could trigger secondary ice production processes, such as the Hallett–Mossop process, which usually takes place around −5 ∘C and could probably improve our comparison at high temperatures. These processes are not considered in our study. However, they are known to further increase the number concentration of ice crystals (Sotiropoulou et al., 2020) and their subsequent climate impact.
We conclude that dust particles appear to be important precursors of INPs, able to account for most of the observed INP number concentration globally. K-feldspar contributes, globally, most of the INPs associated with desert dust because it is more ice active and less sensitive than quartz to aging processes that reduce the ice-nucleating activity of the particles (Harrison et al., 2019). However, we highlight that INP concentrations could also be significantly affected by quartz particles, which are abundant in airborne dust. Improving the representation of INPs thus requires further constraining the abundance of quartz and K-feldspar in arid soil surfaces along with their abundance, size distribution, and mixing state in the emitted dust atmospheric particles at a global scale. Also, additional experimental studies on the ice-nucleating ability of mineral dust particles must be performed to investigate the impact of atmospheric aging and the presence of biological fragments attached to dust on the ice-nucleating activity of dust particles.
The INP data used in this study can be accessed through BACCHUS (http://www.bacchus-env.eu/in/info.php? id=71, last access: 24 March 2019) and (frozen fractions and INP concentrations) on Pangaea (https://doi.pangaea.de/10.1594/PANGAEA.899701; Wex et al., 2019a, b). Model output is available on Zenodo https://doi.org/10.5281/zenodo.7512255 (Chatziparaschos et al., 2023).
The supplement related to this article is available online at: https://doi.org/10.5194/acp-23-1785-2023-supplement.
MK and MC conceived the study. MC modified the model to account for INPs and performed the simulations, visualized and analyzed data, and wrote the initial version of the paper. MK supervised the work with feedback from AN and CPGP and re-edited the paper. SM implemented the dust emissions module and the initial dataset of dust mineralogy in the model. ND, MZ, MV, and MGA evaluated the dust simulations. NK provided characterized dust aerosol observations from Finokalia station. MCS provided key feedback on the implementation of the ice nucleation active-surface site density parameterization. CPGP and MGA provided the emitted mineral fractions of quartz and feldspar. CPGP re-edited parts of the paper. All authors read and commented the paper.
At least one of the (co-)authors is a member of the editorial board of Atmospheric Chemistry and Physics. The peer-review process was guided by an independent editor, and the authors also have no other competing interests to declare.
Publisher's note: Copernicus Publications remains neutral with regard to jurisdictional claims in published maps and institutional affiliations.
This work has been supported by the project “PANhellenic infrastructure for Atmospheric Composition and climatE change” (PANACEA; MIS 5021516), which is implemented under the Action “Reinforcement of the Research and Innovation Infrastructure”, funded by the Operational Programme “Competitiveness, Entrepreneurship and Innovation” (NSRF 2014-2020) and co-financed by Greece and the European Union (European Regional Development Fund). Nikos Daskalakis, Maria Kanakidou, Mihalis Vrekousis acknowledge support from the Deutsche Forschungsgemeinschaft (DFG, German Research Foundation) under Germany's Excellence Strategy (University Allowance, EXC 2077, University of Bremen). Maria Kanakidou, Marios Chatziparaschos, Athanasios Nenes, Montserrat Costa-Surós, María Gonçalves Ageitos, and Carlos Pérez García-Pando acknowledge support by the European Union Horizon 2020 project FORCeS under grant agreement no. 821205. Carlos Pérez García-Pando, Montserrat Costa-Surós, and María Gonçalves Ageitos acknowledge support from the European Research Council under the European Union's Horizon 2020 research and innovation programme (grant no. 773051; FRAGMENT) and from the AXA Research Fund. Montserrat Costa-Surós has received funding from the European Union's Horizon 2020 research and innovation programme, under the Marie Skłodowska-Curie grant agreements, grant no. 754433, from the call H2020-MSCA-COFUND-2016. We thank Joseph M. Prospero for PM10 measurements in Banizoumbou (Niger), Cinzana (Mali), M'Bour (Senegal), which were performed in the framework of the French National Observatory Service INDAAF (International Network to study Deposition and Atmospheric composition in Africa; https://indaaf.obs-mip.fr/, last access: 27 January 2021), piloted by the LISA and LAERO and supported by the INSU/CNRS, the IRD, and the Observatoire Midi-Pyrénées and the Observatoire des Sciences de l'Univers EFLUVE and Michael Pikridas from the Climate and Atmosphere Research Center of The Cyprus Institute
This research has been supported by the Operational Programme “Competitiveness, Entrepreneurship and Innovation” (NSRF 2014-2020) and co-financed by Greece and the European Regional Development Fund (grant no. MIS 5021516) and EU-Horizon 2020 project FORCeS (grant no. 821205).
This paper was edited by Susannah Burrows and reviewed by two anonymous referees.
Abdelkader, M., Metzger, S., Steil, B., Klingmüller, K., Tost, H., Pozzer, A., Stenchikov, G., Barrie, L., and Lelieveld, J.: Sensitivity of transatlantic dust transport to chemical aging and related atmospheric processes, Atmos. Chem. Phys., 17, 3799–3821, https://doi.org/10.5194/acp-17-3799-2017, 2017.
Albani, S., Mahowald, N. M., Perry, A. T., Scanza, R. A., Zender, C. S., G.Heavens, N., V. Maggi, J. F. K., and L.Otto-Bliesner, and B.: Improved dust representation in the CommunityAtmosphere Model, J. Adv. Model. Earth Sy, 6, 92–95, https://doi.org/10.1002/2013MS000279, 2014.
Arimoto, R., Duce, R. A., Ray, B. J., Ellis, W. G., Cullen, J. D., and Merrill, J. T.: Trace elements in the atmosphere over the North Atlantic, J. Geophys. Res., 100, 1199–1213, https://doi.org/10.1029/94JD02618, 1995.
Atkinson, J. D., Murray, B. J., Woodhouse, M. T., Whale, T. F., Baustian, K. J., Carslaw, K. S., Dobbie, S., O'Sullivan, D., and Malkin, T. L.: The importance of feldspar for ice nucleation by mineral dust in mixed-phase clouds, Nature, 498, 355–358, https://doi.org/10.1038/nature12278, 2013.
Barahona, D. and Nenes, A.: Parameterizing the competition between homogeneous and heterogeneous freezing in ice cloud formation – polydisperse ice nuclei, Atmos. Chem. Phys., 9, 5933–5948, https://doi.org/10.5194/acp-9-5933-2009, 2009.
Boose, Y., Welti, A., Atkinson, J., Ramelli, F., Danielczok, A., Bingemer, H. G., Plötze, M., Sierau, B., Kanji, Z. A., and Lohmann, U.: Heterogeneous ice nucleation on dust particles sourced from nine deserts worldwide – Part 1: Immersion freezing, Atmos. Chem. Phys., 16, 15075–15095, https://doi.org/10.5194/acp-16-15075-2016, 2016.
Boucher, O.: Atmospheric Aerosols. Properties and Climate Impacts, Springer Netherlands, Dordrecht, Heidelberg, New York, London, 311 pp., https://doi.org/10.1007/978-94-017-9649-1, 2015.
Burrows, S. M., McCluskey, C. S., Cornwell, G., Steinke, I., Zhang, K., Zhao, B., Zawadowicz, M., Raman, A., Kulkarni, G., China, S., Zelenyuk, A., and DeMott, P. J.: Ice-Nucleating Particles That Impact Clouds and Climate: Observational and Modeling Research Needs, Rev. Geophys., 60, e2021RG000745, https://doi.org/10.1029/2021RG000745, 2022.
Chatziparaschos, M., Daskalakis, N., Myriokefalitakis, S., and Kanakidou, M.: 3-D model data used to investigate the role of K-feldspar and quartz in global ice nucleation by mineral dust in mixed-phase clouds, Version 1, Zenodo [data set], https://doi.org/10.5281/zenodo.7512255, 2023.
Claquin, T., Schulz, M., and Balkanski, Y. J.: Modeling the mineralogy of atmospheric dust sources, J. Geophys. Res.-Atmos., 104, 22243–22256, https://doi.org/10.1029/1999JD900416, 1999.
Daskalakis, N., Myriokefalitakis, S., and Kanakidou, M.: Sensitivity of tropospheric loads and lifetimes of short lived pollutants to fire emissions, Atmos. Chem. Phys., 15, 3543–3563, https://doi.org/10.5194/acp-15-3543-2015, 2015.
Daskalakis, N., Tsigaridis, K., Myriokefalitakis, S., Fanourgakis, G. S., and Kanakidou, M.: Large gain in air quality compared to an alternative anthropogenic emissions scenario, Atmos. Chem. Phys., 16, 9771–9784, https://doi.org/10.5194/acp-16-9771-2016, 2016.
Daskalakis, N., Gallardo, L., Kanakidou, M., Nüß, J. R., Menares, C., Rondanelli, R., Thompson, A. M., and Vrekoussis, M.: Impact of biomass burning and stratospheric intrusions in the remote South Pacific Ocean troposphere, Atmos. Chem. Phys., 22, 4075–4099, https://doi.org/10.5194/acp-22-4075-2022, 2022.
Dee, D. P., Uppala, S. M., Simmons, A. J., Berrisford, P., Poli, P., Kobayashi, S., Andrae, U., Balmaseda, M. A., Balsamo, G., Bauer, P., Bechtold, P., Beljaars, A. C. M., van de Berg, L., Bidlot, J., Bormann, N., Delsol, C., Dragani, R., Fuentes, M., Geer, A. J., Haimberger, L., Healy, S. B., Hersbach, H., Hólm, E. V., Isaksen, L., Kållberg, P., Köhler, M., Matricardi, M., Mcnally, A. P., Monge-Sanz, B. M., Morcrette, J. J., Park, B. K., Peubey, C., de Rosnay, P., Tavolato, C., Thépaut, J. N., and Vitart, F.: The ERA-Interim reanalysis: Configuration and performance of the data assimilation system, Q. J. Roy. Meteor. Soc., 137, 553–597, https://doi.org/10.1002/qj.828, 2011.
DeMott, P. J., Prenni, A. J., Liu, X., Kreidenweis, S. M., Petters, M. D., Twohy, C. H., Richardson, M. S., Eidhammer, T., and Rogers, D. C.: Predicting global atmospheric ice nuclei distributions and their impacts on climate, P. Natl. Acad. Sci. USA, 107, 11217–11222, https://doi.org/10.1073/pnas.0910818107, 2010.
Fan, J., Wang, Y., Rosenfeld, D., and Liu, X.: Review of aerosol-cloud interactions: Mechanisms, significance, and challenges, J. Atmos. Sci., 73, 4221–4252, https://doi.org/10.1175/JAS-D-16-0037.1, 2016.
Fan, J., Leung, L. R., Rosenfeld, D., and DeMott, P. J.: Effects of cloud condensation nuclei and ice nucleating particles on precipitation processes and supercooled liquid in mixed-phase orographic clouds, Atmos. Chem. Phys., 17, 1017–1035, https://doi.org/10.5194/acp-17-1017-2017, 2017.
Field, P. R., Lawson, R. P., Brown, P. R. A., Lloyd, G., Westbrook, C., Moisseev, D., Miltenberger, A., Nenes, A., Blyth, A., Choularton, T., Connolly, P., Buehl, J., Crosier, J., Cui, Z., Dearden, C., DeMott, P., Flossmann, A., Heymsfield, A., Huang, Y., Kalesse, H., Kanji, Z. A., Korolev, A., Kirchgaessner, A., Lasher-Trapp, S., Leisner, T., McFarquhar, G., Phillips, V., Stith, J., and Sullivan, S.: Chapter 7. Secondary Ice Production – current state of the science and recommendations for the future, Meteorol. Monogr., 58, 7.1–7.20, https://doi.org/10.1175/amsmonographs-d-16-0014.1, 2017.
Fröhlich-Nowoisky, J., Hill, T. C. J., Pummer, B. G., Yordanova, P., Franc, G. D., and Pöschl, U.: Ice nucleation activity in the widespread soil fungus Mortierella alpina, Biogeosciences, 12, 1057–1071, https://doi.org/10.5194/bg-12-1057-2015, 2015.
Georgakaki, P., Sotiropoulou, G., Vignon, É., Billault-Roux, A.-C., Berne, A., and Nenes, A.: Secondary ice production processes in wintertime alpine mixed-phase clouds, Atmos. Chem. Phys., 22, 1965–1988, https://doi.org/10.5194/acp-22-1965-2022, 2022.
Ghan, S. J., Abdul-Razzak, H., Nenes, A., Ming, Y., Liu, X., Ovchinnikov, M., Shipway, B., Meskhidze, N., Xu, J., and Shi, X.: Droplet nucleation: Physically-based parameterizations and comparative evaluation, J. Adv. Model. Earth Sy., 3, M10001, https://doi.org/10.1029/2011ms000074, 2011.
Ginoux, P.: Atmospheric chemistry: Warming or cooling dust?, Nat. Geosci., 10, 246–247, https://doi.org/10.1038/ngeo2923, 2017.
Ginoux, P., Prospero, J. M., Torres, O., and Chin, M.: Long-term simulation of global dust distribution with the GOCART model: Correlation with North Atlantic Oscillation, Environ. Model. Softw., 19, 113–128, https://doi.org/10.1016/S1364-8152(03)00114-2, 2004.
Harrison, A. D., Whale, T. F., Carpenter, M. A., Holden, M. A., Neve, L., O'Sullivan, D., Vergara Temprado, J., and Murray, B. J.: Not all feldspars are equal: a survey of ice nucleating properties across the feldspar group of minerals, Atmos. Chem. Phys., 16, 10927–10940, https://doi.org/10.5194/acp-16-10927-2016, 2016.
Harrison, A. D., Lever, K., Sanchez-Marroquin, A., Holden, M. A., Whale, T. F., Tarn, M. D., McQuaid, J. B., and Murray, B. J.: The ice-nucleating ability of quartz immersed in water and its atmospheric importance compared to K-feldspar, Atmos. Chem. Phys., 19, 11343–11361, https://doi.org/10.5194/acp-19-11343-2019, 2019.
Hill, T. C. J., DeMott, P. J., Tobo, Y., Fröhlich-Nowoisky, J., Moffett, B. F., Franc, G. D., and Kreidenweis, S. M.: Sources of organic ice nucleating particles in soils, Atmos. Chem. Phys., 16, 7195–7211, https://doi.org/10.5194/acp-16-7195-2016, 2016.
Holden, M. A., Whale, T. F., Tarn, M. D., O'Sullivan, D., Walshaw, R. D., Murray, B. J., Meldrum, F. C., and Christenson, H. K.: High-speed imaging of ice nucleation in water proves the existence of active sites, Sci. Adv., 5, eaav4316, https://doi.org/10.1126/sciadv.aav4316, 2019.
Hoose, C. and Möhler, O.: Heterogeneous ice nucleation on atmospheric aerosols: a review of results from laboratory experiments, Atmos. Chem. Phys., 12, 9817–9854, https://doi.org/10.5194/acp-12-9817-2012, 2012.
Hoose, C., Kristjánsson, J. E., and Burrows, S. M.: How important is biological ice nucleation in clouds on a global scale?, Environ. Res. Lett., 5, 024009, https://doi.org/10.1088/1748-9326/5/2/024009, 2010.
Ilić, L., Jovanović, A., Kuzmanoski, M., Lazić, L., Madonna, F., Rosoldi, M., Mytilinaios, M., Marinou, E., and Ničković, S.: Mineralogy Sensitive Immersion Freezing Parameterization in DREAM, J. Geophys. Res.-Atmos., 127, e2021JD035093, https://doi.org/10.1029/2021JD035093, 2022.
IPCC: Summary for Policymakers, in: Climate Change 2021. The Physical Science Basis, Contribution of Working Group I to the Sixth Assessment Report of the Intergovernmental Panel on Climate Change, edited by: Masson-Delmotte, V., Zhai, P., Pirani, A., Connors, S. L., Péan, C., Berger, S., Caud, N., Chen, Y., Goldfarb, L., Gomis, M. I., Huang, M., Leitzell, K., Lonnoy, E., Matthews, J. B. R., Maycock, T. K., Waterfield, T., Yelekçi, O., Yu, R., and Zhou, B., Masson-Delmotte, V., R. Y. and B. Z., Cambridge University Press, Cambridge, United Kingdom and New York, NY, USA, 3–32, ISBN 978-92-9169-158-6, 2021.
Iwata, A. and Matsuki, A.: Characterization of individual ice residual particles by the single droplet freezing method: a case study in the Asian dust outflow region, Atmos. Chem. Phys., 18, 1785–1804, https://doi.org/10.5194/acp-18-1785-2018, 2018.
Jeuken, M., Van Wijk, R., Peleman, J., and Lindhout, P.: An integrated interspecific AFLP map of lettuce (Lactuca) based on two L. sativa × L. saligna F2 populations, Theor. Appl. Genet., 103, 638–647, https://doi.org/10.1007/s001220100657, 2001.
Journet, E., Balkanski, Y., and Harrison, S. P.: A new data set of soil mineralogy for dust-cycle modeling, Atmos. Chem. Phys., 14, 3801–3816, https://doi.org/10.5194/acp-14-3801-2014, 2014.
Kalivitis, N., Gerasopoulos, E., Vrekoussis, M., Kouvarakis, G., Kubilay, N., Hatzianastassiou, N., Vardavas, I., and Mihalopoulos, N.: Dust transport over the eastern mediterranean derived from total ozone mapping spectrometer, aerosol robotic network, and surface measurements, J. Geophys. Res.-Atmos., 112, D03202, https://doi.org/10.1029/2006JD007510, 2007.
Kanakidou, M., Myriokefalitakis, S., Daskalakis, N., Fanourgakis, G., Nenes, A., Baker, A. R., Tsigaridis, K., and Mihalopoulos, N.: Past, present, and future atmospheric nitrogen deposition, J. Atmos. Sci., 73, 2039–2047, https://doi.org/10.1175/JAS-D-15-0278.1, 2016.
Kanakidou, M., Myriokefalitakis, S., and Tsagkaraki, M.: Atmospheric inputs of nutrients to the Mediterranean Sea, Deep-Sea Res. Pt. II, 171, 104606, https://doi.org/10.1016/j.dsr2.2019.06.014, 2020.
Kandler, K., Schütz, L., Deutscher, C., Ebert, M., Hofmann, H., Jäckel, S., Jaenicke, R., Knippertz, P., Lieke, K., Massling, A., Petzold, A., Schladitz, A., Weinzierl, B., Wiedensohler, A., Zorn, S., and Weinbruch, S.: Size distribution, mass concentration, chemical and mineralogical composition and derived optical parameters of the boundary layer aerosol at Tinfou, Morocco, during SAMUM 2006, Tellus B, 61, 32–50, https://doi.org/10.3402/tellusb.v61i1.16798, 2009.
Kanji, Z. A., Ladino, L. A., Wex, H., Boose, Y., Burkert-Kohn, M., Cziczo, D. J., and Krämer, M.: Overview of Ice Nucleating Particles, Meteorol. Monogr., 58, 1.1–1.33, https://doi.org/10.1175/AMSMONOGRAPHS-D-16-0006.1, 2017.
Kanji, Z. A., Sullivan, R. C., Niemand, M., DeMott, P. J., Prenni, A. J., Chou, C., Saathoff, H., and Möhler, O.: Heterogeneous ice nucleation properties of natural desert dust particles coated with a surrogate of secondary organic aerosol, Atmos. Chem. Phys., 19, 5091–5110, https://doi.org/10.5194/acp-19-5091-2019, 2019.
Kiselev, A., Bachmann, F., Pedevilla, P., Cox, S. J., Michaelides, A., Gerthsen, D., and Leisner, T.: Active sites in heterogeneous ice nucleation-the example of K-rich feldspars, Science, 355, 367–371, https://doi.org/10.1126/science.aai8034, 2017.
Kleanthous, S., Vrekoussis, M., Mihalopoulos, N., Kalabokas, P., and Lelieveld, J.: On the temporal and spatial variation of ozone in Cyprus, Sci. Total Environ., 476–477, 677–687, https://doi.org/10.1016/j.scitotenv.2013.12.101, 2014.
Kok, J. F.: A scaling theory for the size distribution of emitted dust aerosols suggests climate models underestimate the size of the global dust cycle, P. Natl. Acad. Sci. USA, 108, 1016–1021, https://doi.org/10.1073/pnas.1014798108, 2011.
Kok, J. F., Ridley, D. A., Zhou, Q., Miller, R. L., Zhao, C., Heald, C. L., Ward, D. S., Albani, S., and Haustein, K.: Smaller desert dust cooling effect estimated from analysis of dust size and abundance, Nat. Geosci., 10, 274–278, https://doi.org/10.1038/ngeo2912, 2017.
Kumar, A., Marcolli, C., and Peter, T.: Ice nucleation activity of silicates and aluminosilicates in pure water and aqueous solutions – Part 2: Quartz and amorphous silica, Atmos. Chem. Phys., 19, 6035–6058, https://doi.org/10.5194/acp-19-6035-2019, 2019.
Kumar, P., Sokolik, I. N., and Nenes, A.: Cloud condensation nuclei activity and droplet activation kinetics of wet processed regional dust samples and minerals, Atmos. Chem. Phys., 11, 8661–8676, https://doi.org/10.5194/acp-11-8661-2011, 2011.
Lance, S.: Coincidence errors in a cloud droplet probe (CDP) and a cloud and aerosol spectrometer (CAS), and the improved performance of a modified CDP, J. Atmos. Ocean. Tech., 29, 1532–1541, https://doi.org/10.1175/JTECH-D-11-00208.1, 2012.
Lebel, T., Parker, D. J., Flamant, C., Bourlès, B., Marticorena, B., Mougin, E., Peugeot, C., Diedhiou, A., Haywood, J. M., Ngamini, J. B., Polcher, J., Redelsperger, J. L., and Thorncroft, C. D.: The AMMA field campaigns: Multiscale and multidisciplinary observations in the West African region, Q. J. Roy. Meteor. Soc., 136, 8–33, https://doi.org/10.1002/qj.486, 2010.
Liu, X., Shi, X., Zhang, K., Jensen, E. J., Gettelman, A., Barahona, D., Nenes, A., and Lawson, P.: Sensitivity studies of dust ice nuclei effect on cirrus clouds with the Community Atmosphere Model CAM5, Atmos. Chem. Phys., 12, 12061–12079, https://doi.org/10.5194/acp-12-12061-2012, 2012.
Lohmann, U. and Diehl, K.: Sensitivity studies of the importance of dust ice nuclei for the indirect aerosol effect on stratiform mixed-phase clouds, J. Atmos. Sci., 63, 968–982, https://doi.org/10.1175/JAS3662.1, 2006.
Lohmann, U. and Gasparini, B.: A cirrus cloud climate dial?, Science, 357, 248–249, https://doi.org/10.1126/science.aan3325, 2017.
Marticorena, B., Chatenet, B., Rajot, J. L., Traoré, S., Coulibaly, M., Diallo, A., Koné, I., Maman, A., NDiaye, T., and Zakou, A.: Temporal variability of mineral dust concentrations over West Africa: analyses of a pluriannual monitoring from the AMMA Sahelian Dust Transect, Atmos. Chem. Phys., 10, 8899–8915, https://doi.org/10.5194/acp-10-8899-2010, 2010.
Meyers, Michael P., Paul DeMott, and W. C.: New Primary Ice-Nucleation Parameterizations in an Explicit Cloud Model, J. Appl. Meteorol. Clim., 31, 708–721, https://doi.org/10.1175/1520-0450(1992)031<0708:NPINPI>2.0.CO;2, 1992.
Mihalopoulos, N., Stephanou, E., Kanakidou, M., Pilitsidis, S., and Bousquet, P.: Tropospheric aerosol ionic composition in the Eastern Mediterranean region, Tellus B, 49B, 314–326, https://doi.org/10.3402/tellusb.v49i3.15970, 1997.
Miller, R. L., Knippertz, P., Pérez García-Pando, C., Perlwitz, J. P., and Tegen, I.: Impact of dust radiative forcing upon climate, in: Mineral Dust: A Key Player in the Earth System, Springer Netherlands, Dordrecht, 327–357, https://doi.org/10.1007/978-94-017-8978-3_13, 2014.
Morales Betancourt, R., Lee, D., Oreopoulos, L., Sud, Y. C., Barahona, D., and Nenes, A.: Sensitivity of cirrus and mixed-phase clouds to the ice nuclei spectra in McRAS-AC: single column model simulations, Atmos. Chem. Phys., 12, 10679–10692, https://doi.org/10.5194/acp-12-10679-2012, 2012.
Murray, B. J., O'Sullivan, D., Atkinson, J. D., and Webb, M. E.: Ice nucleation by particles immersed in supercooled cloud droplets, Chem. Soc. Rev., 41, 6519–54, https://doi.org/10.1039/c2cs35200a, 2012.
Murray, B. J., Carslaw, K. S., and Field, P. R.: Opinion: Cloud-phase climate feedback and the importance of ice-nucleating particles, Atmos. Chem. Phys., 21, 665–679, https://doi.org/10.5194/acp-21-665-2021, 2021.
Myriokefalitakis, S., Tsigaridis, K., Mihalopoulos, N., Sciare, J., Nenes, A., Kawamura, K., Segers, A., and Kanakidou, M.: In-cloud oxalate formation in the global troposphere: a 3-D modeling study, Atmos. Chem. Phys., 11, 5761–5782, https://doi.org/10.5194/acp-11-5761-2011, 2011.
Myriokefalitakis, S., Daskalakis, N., Mihalopoulos, N., Baker, A. R., Nenes, A., and Kanakidou, M.: Changes in dissolved iron deposition to the oceans driven by human activity: a 3-D global modelling study, Biogeosciences, 12, 3973–3992, https://doi.org/10.5194/bg-12-3973-2015, 2015.
Myriokefalitakis, S., Nenes, A., Baker, A. R., Mihalopoulos, N., and Kanakidou, M.: Bioavailable atmospheric phosphorous supply to the global ocean: a 3-D global modeling study, Biogeosciences, 13, 6519–6543, https://doi.org/10.5194/bg-13-6519-2016, 2016.
Myriokefalitakis, S., Fanourgakis, G., Kanakidou, M.: The Contribution of Bioaerosols to the Organic Carbon Budget of the Atmosphere, in: Perspectives on Atmospheric Sciences, edited by: Karacostas, T., Bais, A., and Nastos, P., Springer Atmospheric Sciences, Springer, Cham, https://doi.org/10.1007/978-3-319-35095-0_121, 2017.
Nickovic, S., Vukovic, A., Vujadinovic, M., Djurdjevic, V., and Pejanovic, G.: Technical Note: High-resolution mineralogical database of dust-productive soils for atmospheric dust modeling, Atmos. Chem. Phys., 12, 845–855, https://doi.org/10.5194/acp-12-845-2012, 2012.
Niemand, M., Möhler, O., Vogel, B., Vogel, H., Hoose, C., Connolly, P., Klein, H., Bingemer, H., Demott, P., Skrotzki, J., and Leisner, T.: A particle-surface-area-based parameterization of immersion freezing on desert dust particles, J. Atmos. Sci., 69, 3077–3092, https://doi.org/10.1175/JAS-D-11-0249.1, 2012.
O'Sullivan, D., Murray, B. J., Malkin, T. L., Whale, T. F., Umo, N. S., Atkinson, J. D., Price, H. C., Baustian, K. J., Browse, J., and Webb, M. E.: Ice nucleation by fertile soil dusts: relative importance of mineral and biogenic components, Atmos. Chem. Phys., 14, 1853–1867, https://doi.org/10.5194/acp-14-1853-2014, 2014.
O'Sullivan, D., Murray, B. J., Ross, J. F., and Webb, M. E.: The adsorption of fungal ice-nucleating proteins on mineral dusts: a terrestrial reservoir of atmospheric ice-nucleating particles, Atmos. Chem. Phys., 16, 7879–7887, https://doi.org/10.5194/acp-16-7879-2016, 2016.
O'Sullivan, D., Adams, M. P., Tarn, M. D., Harrison, A. D., Vergara-Temprado, J., Porter, G. C. E., Holden, M. A., Sanchez-Marroquin, A., Carotenuto, F., Whale, T. F., McQuaid, J. B., Walshaw, R., Hedges, D. H. P., Burke, I. T., Cui, Z., and Murray, B. J.: Contributions of biogenic material to the atmospheric ice-nucleating particle population in North Western Europe, Sci. Rep., 8, 13821, https://doi.org/10.1038/s41598-018-31981-7, 2018.
Pérez García-Pando, C., Miller, R. L., Perlwitz, J. P., Rodríguez, S., and Prospero, J. M.: Predicting the mineral composition of dust aerosols: Insights from elemental composition measured at the Izaña Observatory, Geophys. Res. Lett., 43, 10520–10529, https://doi.org/10.1002/2016GL069873, 2016.
Perlwitz, J. P., Pérez García-Pando, C., and Miller, R. L.: Predicting the mineral composition of dust aerosols – Part 1: Representing key processes, Atmos. Chem. Phys., 15, 11593–11627, https://doi.org/10.5194/acp-15-11593-2015, 2015a.
Perlwitz, J. P., Pérez García-Pando, C., and Miller, R. L.: Predicting the mineral composition of dust aerosols – Part 2: Model evaluation and identification of key processes with observations, Atmos. Chem. Phys., 15, 11629–11652, https://doi.org/10.5194/acp-15-11629-2015, 2015b.
Pikridas, M., Vrekoussis, M., Sciare, J., Kleanthous, S., Vasiliadou, E., Kizas, C., Savvides, C., and Mihalopoulos, N.: Spatial and temporal (short and long-term) variability of submicron, fine and sub-10 µm particulate matter (PM1, PM2.5, PM10) in Cyprus, Atmos. Environ., 191, 79–93, https://doi.org/10.1016/j.atmosenv.2018.07.048, 2018.
Pratt, K. A., DeMott, P. J., French, J. R., Wang, Z., Westphal, D. L., Heymsfield, A. J., Twohy, C. H., Prenni, A. J., and Prather, K. A.: In situ detection of biological particles in cloud ice-crystals, Nat. Geosci., 2, 398–401, https://doi.org/10.1038/ngeo521, 2009.
Price, H. C., Baustian, K. J., McQuaid, J. B., Blyth, A., Bower, K. N., Choularton, T., Cotton, R. J., Cui, Z., Field, P. R., Gallagher, M., Hawker, R., Merrington, A., Miltenberger, A., Neely, R. R., Parker, S. T., Rosenberg, P. D., Taylor, J. W., Trembath, J., Vergara-Temprado, J., Whale, T. F., Wilson, T. W., Young, G., and Murray, B. J.: Atmospheric Ice-Nucleating Particles in the Dusty Tropical Atlantic, J. Geophys. Res.-Atmos., 123, 2175–2193, https://doi.org/10.1002/2017JD027560, 2018.
Prospero, J. M.: The Atmospheric Transport of Particles to the Ocean, in: Particle Flux in the Ocean, edited by: Ittekkot, V., Schafer, P., Honjo, S., and Depetris, P.J., Wiley, New York, 19–52, 1996.
Prospero, J. M.: Long-term measurements of the transport of African mineral dust to the southeastern United States: Implications for regional air quality, J. Geophys. Res. Atmos., 104, 15917–15927, https://doi.org/10.1029/1999JD900072, 1999.
Prospero, J. M., Barkley, A. E., Gaston, C. J., Gatineau, A., Campos y Sansano, A., and Panechou, K.: Characterizing and Quantifying African Dust Transport and Deposition to South America: Implications for the Phosphorus Budget in the Amazon Basin, Global Biogeochem. Cy., 34, e2020GB006536, https://doi.org/10.1029/2020GB006536, 2020.
Pruppacher, H. R., Klett, J. D., and K., W. P.: Microphysics of Clouds and Precipitation, Aerosol Sci. Technol., 28, 381–382, https://doi.org/10.1080/02786829808965531, 1998a.
Pruppacher, H. R., Klett, J. D., and Wang, P. K.: Microphysics of Clouds and Precipitation, Aerosol Sci. Technol., 28, 381–382, https://doi.org/10.1080/02786829808965531, 1998b.
Roberts, P. and Hallett, J.: A laboratory study of the ice nucleating properties of some mineral particulates, Q. J. Roy. Meteor. Soc., 95, 204–205, https://doi.org/10.1002/qj.49709540317, 1969.
Rosenfeld, D., Lohmann, U., Raga, G. B., O'Dowd, C. D., Kulmala, M., Fuzzi, S., Reissell, A., and Andreae, M. O.: Flood or drought: How do aerosols affect precipitation?, Science, 321, 1309–1313, https://doi.org/10.1126/science.1160606, 2008.
Seinfeld, J. H., Bretherton, C., Carslaw, K. S., Coe, H., DeMott, P. J., Dunlea, E. J., Feingold, G., Ghan, S., Guenther, A. B., Kahn, R., Kraucunas, I., Kreidenweis, S. M., Molina, M. J., Nenes, A., Penner, J. E., Prather, K. A., Ramanathan, V., Ramaswamy, V., Rasch, P. J., Ravishankara, A. R., Rosenfeld, D., Stephens, G., and Wood, R.: Improving our fundamental understanding of the role of aerosol-cloud interactions in the climate system, P. Natl. Acad. Sci. USA, 113, 5781–5790, https://doi.org/10.1073/pnas.1514043113, 2016.
Si, M., Evoy, E., Yun, J., Xi, Y., Hanna, S. J., Chivulescu, A., Rawlings, K., Veber, D., Platt, A., Kunkel, D., Hoor, P., Sharma, S., Leaitch, W. R., and Bertram, A. K.: Concentrations, composition, and sources of ice-nucleating particles in the Canadian High Arctic during spring 2016, Atmos. Chem. Phys., 19, 3007–3024, https://doi.org/10.5194/acp-19-3007-2019, 2019.
Solomon, A., de Boer, G., Creamean, J. M., McComiskey, A., Shupe, M. D., Maahn, M., and Cox, C.: The relative impact of cloud condensation nuclei and ice nucleating particle concentrations on phase partitioning in Arctic mixed-phase stratocumulus clouds, Atmos. Chem. Phys., 18, 17047–17059, https://doi.org/10.5194/acp-18-17047-2018, 2018.
Sotiropoulou, G., Sullivan, S., Savre, J., Lloyd, G., Lachlan-Cope, T., Ekman, A. M. L., and Nenes, A.: The impact of secondary ice production on Arctic stratocumulus, Atmos. Chem. Phys., 20, 1301–1316, https://doi.org/10.5194/acp-20-1301-2020, 2020.
Sotiropoulou, G., Vignon, É., Young, G., Morrison, H., O'Shea, S. J., Lachlan-Cope, T., Berne, A., and Nenes, A.: Secondary ice production in summer clouds over the Antarctic coast: an underappreciated process in atmospheric models, Atmos. Chem. Phys., 21, 755–771, https://doi.org/10.5194/acp-21-755-2021, 2021.
Spracklen, D. V. and Heald, C. L.: The contribution of fungal spores and bacteria to regional and global aerosol number and ice nucleation immersion freezing rates, Atmos. Chem. Phys., 14, 9051–9059, https://doi.org/10.5194/acp-14-9051-2014, 2014.
Sullivan, S. C., Hoose, C., Kiselev, A., Leisner, T., and Nenes, A.: Initiation of secondary ice production in clouds, Atmos. Chem. Phys., 18, 1593–1610, https://doi.org/10.5194/acp-18-1593-2018, 2018.
Tegen, I., Harrison, S. P., Kohfeld, K., Prentice, I. C., Coe, M., and Heimann, M.: Impact of vegetation and preferential source areas on global dust aerosol: Results from a model study, J. Geophys. Res.-Atmos., 107, 4576, https://doi.org/10.1029/2001JD000963, 2002.
Tsigaridis, K. and Kanakidou, M.: Secondary organic aerosol importance in the future atmosphere, Atmos. Environ., 41, 4682–4692, https://doi.org/10.1016/j.atmosenv.2007.03.045, 2007.
Tsigaridis, K., Krol, M., Dentener, F. J., Balkanski, Y., Lathière, J., Metzger, S., Hauglustaine, D. A., and Kanakidou, M.: Change in global aerosol composition since preindustrial times, Atmos. Chem. Phys., 6, 5143–5162, https://doi.org/10.5194/acp-6-5143-2006, 2006.
Tsigaridis, K., Daskalakis, N., Kanakidou, M., Adams, P. J., Artaxo, P., Bahadur, R., Balkanski, Y., Bauer, S. E., Bellouin, N., Benedetti, A., Bergman, T., Berntsen, T. K., Beukes, J. P., Bian, H., Carslaw, K. S., Chin, M., Curci, G., Diehl, T., Easter, R. C., Ghan, S. J., Gong, S. L., Hodzic, A., Hoyle, C. R., Iversen, T., Jathar, S., Jimenez, J. L., Kaiser, J. W., Kirkevåg, A., Koch, D., Kokkola, H., Lee, Y. H., Lin, G., Liu, X., Luo, G., Ma, X., Mann, G. W., Mihalopoulos, N., Morcrette, J.-J., Müller, J.-F., Myhre, G., Myriokefalitakis, S., Ng, N. L., O'Donnell, D., Penner, J. E., Pozzoli, L., Pringle, K. J., Russell, L. M., Schulz, M., Sciare, J., Seland, Ø., Shindell, D. T., Sillman, S., Skeie, R. B., Spracklen, D., Stavrakou, T., Steenrod, S. D., Takemura, T., Tiitta, P., Tilmes, S., Tost, H., van Noije, T., van Zyl, P. G., von Salzen, K., Yu, F., Wang, Z., Wang, Z., Zaveri, R. A., Zhang, H., Zhang, K., Zhang, Q., and Zhang, X.: The AeroCom evaluation and intercomparison of organic aerosol in global models, Atmos. Chem. Phys., 14, 10845–10895, https://doi.org/10.5194/acp-14-10845-2014, 2014.
Vali, G., DeMott, P. J., Möhler, O., and Whale, T. F.: Technical Note: A proposal for ice nucleation terminology, Atmos. Chem. Phys., 15, 10263–10270, https://doi.org/10.5194/acp-15-10263-2015, 2015.
van Noije, T. P. C., Le Sager, P., Segers, A. J., van Velthoven, P. F. J., Krol, M. C., Hazeleger, W., Williams, A. G., and Chambers, S. D.: Simulation of tropospheric chemistry and aerosols with the climate model EC-Earth, Geosci. Model Dev., 7, 2435–2475, https://doi.org/10.5194/gmd-7-2435-2014, 2014.
Vergara-Temprado, J., Murray, B. J., Wilson, T. W., O'Sullivan, D., Browse, J., Pringle, K. J., Ardon-Dryer, K., Bertram, A. K., Burrows, S. M., Ceburnis, D., DeMott, P. J., Mason, R. H., O'Dowd, C. D., Rinaldi, M., and Carslaw, K. S.: Contribution of feldspar and marine organic aerosols to global ice nucleating particle concentrations, Atmos. Chem. Phys., 17, 3637–3658, https://doi.org/10.5194/acp-17-3637-2017, 2017.
Vergara-Temprado, J., Miltenberger, A. K., Furtado, K., Grosvenor, D. P., Shipway, B. J., Hill, A. A., Wilkinson, J. M., Field, P. R., Murray, B. J., and Carslaw, K. S.: Strong control of Southern Ocean cloud reflectivity by ice-nucleating particles, P. Natl. Acad. Sci. USA, 115, 2687–2692, https://doi.org/10.1073/pnas.1721627115, 2018.
Violaki, K., Nenes, A., Tsagkaraki, M., Paglione, M., Jacquet, S., Sempéré, R., and Panagiotopoulos, C.: Bioaerosols and dust are the dominant sources of organic P in atmospheric particles, npj Clim. Atmos. Sci., 4, 63, https://doi.org/10.1038/s41612-021-00215-5, 2021.
Wang, Y., Liu, X., Hoose, C., and Wang, B.: Different contact angle distributions for heterogeneous ice nucleation in the Community Atmospheric Model version 5, Atmos. Chem. Phys., 14, 10411–10430, https://doi.org/10.5194/acp-14-10411-2014, 2014.
Wex, H., Huang, L., Zhang, W., Hung, H., Traversi, R., Becagli, S., Sheesley, R. J., Moffett, C. E., Barrett, T. E., Bossi, R., Skov, H., Hünerbein, A., Lubitz, J., Löffler, M., Linke, O., Hartmann, M., Herenz, P., and Stratmann, F.: Annual variability of ice-nucleating particle concentrations at different Arctic locations, Atmos. Chem. Phys., 19, 5293–5311, https://doi.org/10.5194/acp-19-5293-2019, 2019a.
Wex, H., Huang, L., Sheesley, R., Bossi, R., and Traversi, R.: Annual concentrations of ice nucleating particles at different Arctic stations, PANGAEA [data set], https://doi.org/10.1594/PANGAEA.899701, 2019b.
Whale, T. F., Holden, M. A., Wilson, T. W., O'Sullivan, D., and Murray, B. J.: The enhancement and suppression of immersion mode heterogeneous ice-nucleation by solutes, Chem. Sci., 9, 4142–4151, https://doi.org/10.1039/c7sc05421a, 2018.
Wilson, T. W., Ladino, L. A., Alpert, P. A., Breckels, M. N., Brooks, I. M., Browse, J., Burrows, S. M., Carslaw, K. S., Huffman, J. A., Judd, C., Kilthau, W. P., Mason, R. H., McFiggans, G., Miller, L. A., Nájera, J. J., Polishchuk, E., Rae, S., Schiller, C. L., Si, M., Temprado, J. V., Whale, T. F., Wong, J. P. S., Wurl, O., Yakobi-Hancock, J. D., Abbatt, J. P. D., Aller, J. Y., Bertram, A. K., Knopf, D. A., and Murray, B. J.: A marine biogenic source of atmospheric ice-nucleating particles, Nature, 525, 234–238, https://doi.org/10.1038/nature14986, 2015.
Yin, J., Wang, D., and Guoqing, Z.: An Evaluation of Ice Nuclei Characteristics from the Long-term Measurement Data over North China An Evaluation of Ice Nuclei Characteristics from the Long-term Measurement Data over North China, J. Atmos. Sci., 48, 197–204 https://doi.org/10.1007/s13143-012-0020-8, 2012.
Zuidema, P., Alvarez, C., Kramer, S. J., Custals, L., Izaguirre, M., Sealy, P., Prospero, J. M., and Blades, E.: Is summer African dust arriving earlier to Barbados?, B. Am. Meteorol. Soc., 100, 1981–1986, https://doi.org/10.1175/BAMS-D-18-0083.1, 2019.