the Creative Commons Attribution 4.0 License.
the Creative Commons Attribution 4.0 License.
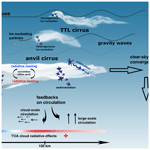
Opinion: Tropical cirrus – from micro-scale processes to climate-scale impacts
Blaž Gasparini
Sylvia C. Sullivan
Adam B. Sokol
Bernd Kärcher
Eric Jensen
Dennis L. Hartmann
Tropical cirrus clouds, i.e., any type of ice cloud with tops above 400 hPa, play a critical role in the climate system and are a major source of uncertainty in our understanding of global warming. Tropical cirrus clouds involve processes spanning a wide range of spatial and temporal scales, from ice microphysics on cloud scales to mesoscale convective organization and planetary wave dynamics. This complexity makes tropical cirrus clouds notoriously difficult to model and has left many important questions stubbornly unanswered. At the same time, their multi-scale nature makes them well-positioned to benefit from the rise of global, high-resolution simulations of Earth's atmosphere and a growing abundance of remotely sensed and in situ observations. Rapid progress on our understanding of tropical cirrus requires coordinated efforts to take advantage of these modern computational and observational abilities.
In this opinion paper, we review recent progress in cirrus studies, highlight important unanswered questions, and discuss promising paths forward. Significant progress has been made in understanding the life cycle of convectively generated “anvil” cirrus and the response of their macrophysical properties to large-scale controls. On the other hand, much work remains to be done to fully understand how small-scale anvil processes and the climatological anvil radiative effect will respond to global warming. Thin, in situ formed cirrus clouds are now known to be closely tied to the thermal structure and humidity of the tropical tropopause layer, but microphysical uncertainties prevent a full understanding of this link, as well as the precise amount of water vapor entering the stratosphere. Model representation of ice-nucleating particles, water vapor supersaturation, and ice depositional growth continue to pose great challenges to cirrus modeling. We believe that major advances in the understanding of tropical cirrus can be made through a combination of cross-tool synthesis and cross-scale studies conducted by cross-disciplinary research teams.
- Article
(3785 KB) - Full-text XML
- BibTeX
- EndNote
The tropical upper troposphere is the cloudiest place on Earth due to widespread cirrus cloud coverage (Fig. 1). These cirrus clouds arise from two main sources: deep convection and in situ ice formation outside of convective clouds. Anvil cirrus clouds form when deep convection deposits moist and ice-laden air in the upper troposphere. Often outliving the deep convective systems that generate them, anvils cover up to one-third of convectively active regions such as the Indo-Pacific Warm Pool and the Intertropical Convergence Zone (ITCZ) (Yuan and Houze, 2010). Above the typical level of deep convective outflow, in the tropical tropopause layer (TTL; ≃ 14–19 km), cirrus clouds formed in situ are ubiquitous (Fig. 2). Here, the large-scale ascent characteristic of the TTL drives adiabatic cooling and high relative humidity, creating a favorable environment for ice formation (Fueglistaler et al., 2009; Randel and Jensen, 2013).
Tropical cirrus clouds encompass both anvil and TTL cirrus and are of critical importance to many aspects of Earth's climate system. The climatological significance of anvil cirrus begins with their influence on radiative fluxes, which leads not only to large, local changes in the top-of-atmosphere energy budget, but also to substantial diabatic heating rates within the atmosphere itself (Robert A Houze, 1982; Ackerman et al., 1988; Ramanathan et al., 1989). These cloud radiative effects (CREs) play an important role in many aspects of global circulation and climate (Fig. 2). By decreasing the net radiative cooling rate of the atmosphere below, anvil cirrus clouds reduce the demand for latent heat in the troposphere, which slows the hydrologic cycle (Su et al., 2017; Albern et al., 2018). Anvils are thought to contribute to the narrowing of the ITCZ, broadening of regions of subtropical descent, and strengthening of the Hadley cell (Harrop and Hartmann, 2016; Popp and Silvers, 2017; Albern et al., 2018). Their radiative impacts have been identified as a critical component of the Madden–Julian oscillation (Raymond, 2001) and of convective self-aggregation in high-resolution numerical models (Wing and Emanuel, 2014; Holloway and Woolnough, 2016).
While thin in situ cirrus clouds in the TTL also modify local radiative fluxes (Haladay and Stephens, 2009), their global importance arises primarily from their effects on the transport of water vapor into the stratosphere. Ascent across the tropical tropopause is the primary source of tropospheric air to the stratosphere (Holton et al., 1995). As air ascends through the TTL (Fig. 2), its water content is depleted as ice crystals nucleate, grow via vapor deposition, and sediment downward, removing water to lower levels (Jensen et al., 1996). Following this freeze-drying, tropical stratospheric air is transported poleward by the Brewer–Dobson circulation. Cirrus microphysical processes thus regulate global stratospheric water vapor content, with important implications for stratospheric chemistry and Earth's radiation budget (Solomon et al., 2010).
Because of these global impacts, accurate understanding and characterization of tropical cirrus are critical to model climate and project its changes. While we have expanded satellite and high-altitude aircraft datasets and improved ice microphysics parameterizations over the last 2 decades, our understanding of tropical cirrus remains incomplete. Indeed, changes in anvil cirrus areal coverage with warming were the least certain climate feedback in a recent assessment of Earth's climate sensitivity (Sherwood et al., 2020). Much of this uncertainty stems from the fact that cirrus clouds are notoriously difficult to model (Sect. 5) and to observe for both in situ and satellite-based instruments. From ice microphysics to planetary-scale circulations, cirrus clouds are sensitive to processes occurring across a wide range of spatiotemporal scales, as discussed below (Fig. 3).
This opinion paper has several goals. One is to synthesize recent progress in cirrus studies in terms of both methodologies and important findings. We also articulate the questions that present the most urgent challenges to our understanding of tropical cirrus and their role in global climate and climate change. Finally, we identify promising ways forward.
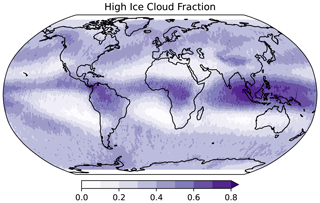
Figure 1Cirrus cloud fraction observed by CALIOP from 2007–2015. Cirrus clouds are defined here as those with cloud-top pressure below 400 hPa, including deep convective clouds. Data were obtained from the CALIPSO Level 3 GEWEX cloud product, v1-00 NASA/LARC/SD/ASDC (2019).
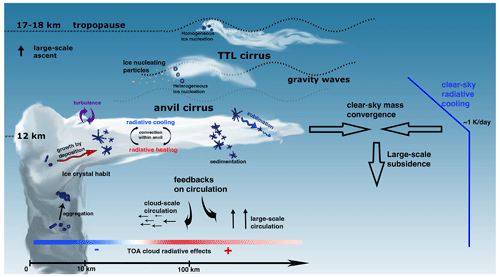
Figure 2Illustration of key processes controlling tropical anvil cirrus and TTL cirrus. These include microphysical processes such as ice nucleation, depositional growth and sublimation, aggregation, and sedimentation; interaction of ice crystals with radiative fluxes; and large-scale mass convergence and subsidence driven primarily by clear-sky radiative cooling, large-scale ascent in the TTL, and gravity waves. The interaction of small-scale with large-scale processes in anvils can drive circulations and generate turbulence. Processes with a significant influence on the diabatic temperature heating budget are colored in red (warming) or blue (cooling).
2.1 Observing cirrus from space
Satellite measurements provide a unique global perspective on tropical cirrus clouds, adding to the information from ground-based and aircraft measurements. We divide these remote sensing measurements into polar-orbiting active, polar-orbiting passive, geostationary, tracking, and synergistic datasets.
Several spaceborne active sensors provide advanced understanding of tropical cirrus properties, evolution, and radiative effects. Launched in 2006, the CloudSat Cloud Profiling Radar (Stephens et al., 2008) and the Cloud–Aerosol Lidar with Orthogonal Polarization (CALIOP) (Winker et al., 2010) represented a substantial increase in the observational capacity for high clouds and their properties (Stephens et al., 2018). This combination of active instruments provides global observations of cloud fraction and condensate mixing ratio profiles for both optically thick and thin clouds. Using the merged CloudSat radar reflectivity and CALIOP attenuated backscatter signals, the CloudSat level 2C Ice Cloud Property Product (2C-ICE, Deng et al., 2010) and the Radar–Lidar Product (DARDAR, Delanoë and Hogan, 2008, 2010) retrieve ice water content, effective ice crystal size, and extinction coefficient. Sourdeval et al. (2018) extended the DARDAR algorithm to globally compute ice crystal number concentration, a quantity that can vary by orders of magnitude, and thus better constrain ice nucleation pathways (Gryspeerdt et al., 2018), aerosol–cirrus interactions (Gryspeerdt et al., 2018; Mitchell et al., 2018; Zhu et al., 2022), and anvil radiative feedbacks (Sokol and Hartmann, 2020; Wall et al., 2020). CloudSat–CALIPSO-derived cirrus properties were also used as inputs to estimate cloud radiative heating rates within the atmosphere (L'Ecuyer et al., 2008; Henderson et al., 2013; Matus and L'Ecuyer, 2017; Hang et al., 2019) and cloud radiative effects (CREs) at the top and bottom of the atmosphere (Hong et al., 2016; Matus and L'Ecuyer, 2017; L’Ecuyer et al., 2019). The high sensitivity of CALIOP retrievals to small particles (Winker et al., 2010; Avery et al., 2012) has greatly enhanced knowledge of the occurrence and radiative effects of TTL cirrus (Sassen et al., 2009; Massie et al., 2010; Haladay and Stephens, 2009; Yang et al., 2010; Fu et al., 2018), their interannual variability (Virts et al., 2010; Tseng and Fu, 2017), and their relationship to gravity waves (Chang and L'Ecuyer, 2020).
Despite supplying vertical profiles of cloud properties, active instruments suffer from poor statistical sampling: they only provide a curtain of measurements along the satellite path. Passive instruments overcome the sampling problem with their wide swathes but often sacrifice spatial resolution as a result. Hence, passive instruments, such as the Atmospheric Infrared Sounder (AIRS, Chahine et al., 2006) or the Infrared Atmospheric Sounding Interferometer (IASI, Hilton et al., 2012), give statistically robust estimates of cloud fraction and other macrophysical properties over the full globe (Stubenrauch et al., 2017; Leonarski et al., 2020). These macrophysical observations have previously been combined with meteorological data to study the response of tropical cirrus to changes in surface temperatures (Zelinka and Hartmann, 2011; Stubenrauch et al., 2017; Protopapadaki et al., 2017) and to constrain global climate model (GCM) ice microphysics (Stubenrauch et al., 2019). Recently, a combination of multi-angle polarization and shortwave infrared measurements from Polarization and Directionality of the Earth's Reflectances (POLDER) and Moderate Resolution Imaging Spectroradiometer (MODIS) provided information on ice crystal properties at the tops of thick ice clouds (van Diedenhoven et al., 2016, 2020).
Geostationary satellites make up another class of remote sensing data, particularly useful in estimating high-cloud evolution due to their continuous, high-frequency sampling. Cirrus clouds have been observed for decades as part of the International Satellite Cloud Climatology Project (ISCCP) (Rossow and Schiffer, 1999), which combines visible and infrared measurements from multiple geostationary and polar-orbiting satellites with sub-daily resolution. These measurements provide information on cloud amounts, cloud particle effective size, cloud optical depth, and cloud-top temperatures (Parol et al., 1991; Rossow and Schiffer, 1999; Stubenrauch et al., 2013). ISCCP data have been used to validate high clouds in model simulations (Pincus et al., 2012; Tselioudis et al., 2021) and to study the life cycle and diurnal cycle of tropical cirrus (Luo and Rossow, 2004; Wylie and Woolf, 2002; Yin and Porporato, 2017). The multidecadal duration of ISCCP data provided the first evidence of altitude shifts of high clouds with warming (Norris et al., 2016).
Geostationary observations are also used to track mesoscale convective systems (MCSs), organized storms whose outflow is the source of tropical anvil cirrus (e.g., Fiolleau and Roca, 2013; Roca et al., 2017). From visible and infrared channels, a transition can be inferred from isolated convective cores, to optically thick anvil outflow, to optically thin residual outflow in the growing, mature, and dissipating stages of an MCS life cycle (Jones et al., 2023). This inference is generally based upon brightness temperature thresholds or changes in cloud-top radiative cooling rates. Combining geostationary infrared imagery with environmental and radiative properties from low-Earth orbiting satellites or from satellite-based precipitation measurements has also provided insight into the MCS and anvil life cycles (e.g., Feng et al., 2011; Fiolleau and Roca, 2013). Importantly, Feng et al. (2021) have developed a global MCS tracking database at hourly and 10 km resolution using a fusion of geostationary satellite data and Global Precipitation Measurement Mission rainfall observations. An important challenge for the community will be standardizing various tracking databases.
Finally, remote sensing datasets that combine multiple satellite observations and reanalysis fields are of particular utility for careful studies of tropical cirrus properties and their radiative effects (e.g., Wall et al., 2018). The CALIPSO–CloudSat–CERES–MODIS merged product (CCCM, Kato et al., 2011) represents such a dataset, frequently used for model evaluation purposes (Xie et al., 2018; Caldwell et al., 2021).
2.2 In situ measurements
Relative to satellite measurements, in situ data have better spatiotemporal sampling and more precise measurements of cirrus microphysical evolution and vertical distribution. In situ measurements are the only way to obtain detailed information about the size distributions and habits of cirrus ice crystals and ice-nucleating particle (INP) size and chemistry. The in situ measurements not only provide information about cirrus micro-scale properties and physical processes; they also allow evaluation and improvement of the satellite remote sensing retrieval algorithms. On the other hand, in situ aircraft data are limited by the inability to sample at close proximity to convective cores and a far smaller sample size than satellites.
Several field campaigns have probed tropical cirrus over the past few decades. In the early 2000s, the Cirrus Regional Study of Tropical Anvils and Cirrus Layers Florida Area Cirrus Experiment (CRYSTAL-FACE; Jensen et al., 2004) took place out of Florida, providing ice crystal, radiative, and meteorological measurements. These measurements provided the basis for early aerosol-dependent ice nucleation parameterizations (Phillips et al., 2008). In 2006, the Tropical Warm Pool–International Cloud Experiment (TWP-ICE) again focused on providing observational constraints for ice cloud parameterizations with flights out of Darwin, Australia (May et al., 2008). During the same year, in situ measurements were made in anvil cirrus generated by MCSs over western Africa during the Stratospheric–Climate Links with Emphasis on the UTLS – African Monsoon Multidisciplinary Analysis campaign (SCOUT-AMMA; Frey et al., 2011). The 2006 Costa Rica Aura Validation Experiment (CR-AVE) and the 2007 Tropical Composition, Cloud and Climate Coupling Experiment (TC4) extensively sampled anvil cirrus over the eastern tropical Pacific (Jensen et al., 2009). Four years later, the Ice In Clouds Experiment-Tropical (ICE-T), operating out of the US Virgin Islands, studied ice nucleation pathways in tropical cirrus (Heymsfield and Willis, 2014). In 2014 and 2015, the High Altitude Ice Crystals–High Ice Water Content (HAIC–HIWC) campaign sampled oceanic and continental deep convection as well as cirrus outflow near Darwin (Australia), Cayenne (French Guiana), and Fort Lauderdale (FL, USA) (Hu et al., 2021a; Strapp et al., 2020). The Airborne Tropical TRopopause EXperiment (ATTREX; Jensen et al., 2017) and POSIDON (Pacific Oxidants, Sulfur, Ice, Dehydration, and cONvection experiment; Jensen et al., 2018a) campaigns provided extensive measurements of TTL cirrus, as well as anvil cirrus generated by typhoons. Both the Stratospheric and Upper Tropospheric Processes for Better Climate Predictions (StratoClim) and Asian Summer Monsoon Chemical and Climate Impact Project (ACCLIP) campaigns yielded new sources of upper-tropospheric ice crystal size distributions, water vapor isotopic composition (a proxy for ice crystal origin), and specific humidity during the Asian monsoon time frame. Airborne campaign measurements have also been used to develop the vast majority of parameterizations used in satellite retrieval algorithms.
A good summary of these tropical cirrus-focused field campaigns exists in the “Cirrus Guide” of Krämer et al. (2016) and Krämer et al. (2020). These guides aggregate the in situ measurement statistics to construct ice water path–temperature and ice crystal number–temperature spaces from 24 field campaigns conducted between 1999 and 2017 with a total sampling time in cirrus of 168 h, two-thirds of which took place in the tropics. These climatologies of ice cloud properties versus temperature find that tropical cirrus clouds tend to be optically thicker more often than their midlatitude counterparts. A large number of in situ observations of tropical anvil clouds is also available in the HAIC–HIWC campaign dataset (Strapp et al., 2020).
Weather balloons and quasi-Lagrangian super-pressure balloons are additional platforms to collect in situ data on cirrus and the upper troposphere (Cirisan et al., 2014; Khaykin et al., 2016; Wolf et al., 2018) as well as upper-tropospheric waves (Podglajen et al., 2016; Corcos et al., 2021). Balloons circulating the tropics at an altitude of 18.5 and 20.5 km were deployed as part of the Stratéole 2 field campaign (Haase et al., 2018; Kalnajs et al., 2021). These balloons carry a number of lightweight instruments, such as a reel-down instrument for in situ measurements of TTL cirrus down to 2 km below the balloon flight level, providing information on the interaction of equatorial waves with TTL cirrus (Bramberger et al., 2022). Some of the balloons carry a microlidar system that can detect even the thinnest TTL cirrus (Ravetta et al., 2020). Dynamical information inferred from balloons has already been used in several studies of TTL cirrus (Jensen et al., 2016; Corcos et al., 2023) and offers further insight into the tropical cirrus life cycle Sect. 6.3.
2.3 Laboratory measurements
Laboratory studies are critical for advancing fundamental understanding of cloud microphysical processes (Morrison et al., 2020). Insights from theory, models, or field observations can be validated against well-designed laboratory measurements. To develop process-level understanding and to identify key parameters of the processes under study, experiments under well-controlled conditions are critical.
Examples of laboratory work related to cirrus include the study of thresholds and rate constants for homogeneous ice nucleation from solution droplets (Koop et al., 2000; Schneider et al., 2021), as well as the environmental conditions under which various INPs contribute to ice formation (see Kanji et al., 2017, for a recent review). As opposed to the macrophysical cloud scales (order of kilometers) retrieved by satellites and simulated by models, cloud microphysical processes occur at the molecular scale. Recent ice nucleation studies highlight the importance of the nanometer scale with direct observation of initial ice germ formation along inhomogeneities in the INP surface (Kiselev et al., 2017). Nanometer-sized pores were also found to be an important factor driving ice nucleation in cirrus clouds (Marcolli, 2014; David et al., 2019).
Laboratory studies have also been critical in developing a fundamental understanding of ice crystal growth (Libbrecht, 2005). Ice crystal growth from water vapor is known to be sensitive to crystal surface properties (Magee et al., 2014) as well as the ambient temperature and supersaturation. Many laboratory studies have tried to quantify the water vapor deposition coefficient (Skrotzki et al., 2013; Lamb et al., 2023), which influences cirrus cloud microphysical and radiative properties. Moreover, changes in the preferential partitioning of water isotopes by non-equilibrium kinematic effects during ice deposition gives insight into ice crystal surface kinetics and deposition coefficients (Nelson, 2011; Lamb et al., 2017).
While studies of INPs and depositional growth can be performed with benchtop setups, larger cloud chamber facilities are able to better replicate real-world conditions for cirrus, in part by minimizing wall effects and strong thermodynamic gradients and in part by providing more robust statistics from larger sample sizes. Because of significant installation and maintenance costs, relatively few large cloud chambers exist in the world. The Aerosol Interaction and Dynamics in the Atmosphere (AIDA) chamber at the Karlsruhe Institute of Technology and the Pi Cloud Chamber at Michigan Tech are two such facilities that have increased our understanding of (1) the deposition coefficient of water vapor molecules, (2) the effect of organic coatings on ice-nucleating efficiencies, and (3) secondary ice nucleation in the wake of sedimenting crystals, among other processes (Skrotzki et al., 2013; Kanji et al., 2019; Prabhakaran et al., 2020).
However, even for larger-scale chamber experiments, it often remains unclear to what extent results can be directly compared to atmospheric conditions. For example, while laboratory studies use ice crystals produced in a controlled pure-water-vapor environment, atmospheric aging processes can alter both ice crystal nucleation and growth mechanisms. These limitations suggest that laboratory experiments should be combined with satellite and in situ observations to improve our knowledge of tropical cirrus.
2.4 Modeling cirrus
Numerical models represent another class of tools used to study tropical cirrus. The wide range of spatial and temporal scales involved in tropical cirrus (Figs. 2 and 3) means that no single modeling tool can describe all processes and interactions. Complicating this situation further is the need to account for the small-scale dynamical forcings that drive ice formation and for detailed aerosol information, e.g., size distributions and chemical composition of INPs (Sect. 5.1), to enable the calculation of aerosol–cirrus interactions.
Parameterizations are simplified representations of physical processes that focus on a limited number of interactions and require validation with detailed numerical models. Microphysical parameterizations with varying degrees of complexity have been developed for ice nucleation, depositional growth, and other subgrid-scale processes (e.g., Hubbard, 1977; Barahona and Nenes, 2008, 2009; Connolly et al., 2012; Phillips et al., 2008; Kärcher and Lohmann, 2002, 2003). Radiation parameterizations are another example, wherein the representation of unresolved variability in cirrus properties on resolved mean fluxes and heating rates remains challenging. An important but often overlooked factor is the need for microphysical and radiation parameterizations to have similar degrees of complexity and consistent formulations, i.e., the same definitions of commonly used variables and same degrees of approximation (Baran et al., 2014, 2016; Sullivan and Voigt, 2021).
These parameterizations are at the heart of GCMs that run at the coarsest spatial and temporal scales. With increasing computational power, grid spacings can be greatly refined, and global storm-resolving models (GSRMs) now operate at kilometer-scale resolution, reducing the number of subgrid processes that must be parameterized. In particular, GSRMs resolve deep convection explicitly, removing numerous long-standing biases caused by convective parameterization (Stevens et al., 2020; Judt et al., 2021; Feng et al., 2023). They bridge the gap between GCMs and classic cloud-resolving models (CRMs) and allow improved simulation of cirrus evolution in the presence of realistic large-scale circulations.
High-resolution CRMs that are capable of simulating anvil life cycles are used for validation and advanced process studies (Saleeby and van den Heever, 2013; Gasparini et al., 2019; Prein et al., 2021). Regional-scale CRMs capture more scale-dependent interactions and some large-scale controls but still rely on simplified microphysical schemes representing bulk cloud properties only (Powell et al., 2012; Weverberg et al., 2013). Along with full-field simulations that represent an Eulerian perspective of ice clouds, trajectory models can also be used for a Lagrangian perspective (Sect. 6.3). They may discretize ice crystal growth over a fixed-size grid or track individual ice particles.
Anvil clouds are unique among Earth's clouds because they can exert both positive and negative CRE over their life cycle. Anvil clouds form at the top of the convectively mixed troposphere, near the 220 K ( ∘C) isotherm, where the water vapor radiative cooling rapidly declines (Fig. 2 and Sect. 3.2.1). They can be optically thick and precipitating at the time of their formation, but over their life cycle various dynamical, radiative, and microphysical processes cause them to thin and spread out over a large area (Lilly, 1988; Ackerman et al., 1988; Hartmann et al., 2018; Schmidt and Garrett, 2013; Gasparini et al., 2019, 2021; Wall et al., 2020; Garrett et al., 2005). Cloud ice is slow to sublimate at the frigid temperatures of the tropical upper troposphere (Seeley et al., 2019), and diabatically driven circulations can sustain cloud ice against sedimentation (Hartmann et al., 2018; Gasparini et al., 2022). These processes, among others, allow anvil cirrus to cover a much greater area and persist for much longer periods of time than deep convective towers themselves (Luo and Rossow, 2004).
Because of their convective origins, anvil cirrus may be affected by any factor that affects the distribution, intensity, and character of deep convection. As a result, anvils are linked to processes across a wide range of spatial and temporal scales. Large-scale tropical oscillations such as the El Niño–Southern Oscillation (ENSO), the Madden–Julian oscillation, and the quasi-biennial oscillation may impact the distribution, properties, and overall abundance of anvil cirrus (Riley et al., 2011; Sullivan et al., 2019; Sweeney et al., 2023). At the same time, the evolution of individual anvil cloud systems is shaped by mesoscale and cloud-scale circulations. These circulations are driven by radiative and latent heating, both of which depend critically on microphysical processes (Gasparini et al., 2019). All of these scales must be considered to fully understand the role of anvil cirrus in global climate. This section discusses progress and challenges in this important area of work. We first address small-scale processes affecting anvil cirrus evolution before turning to large-scale controls and anvil radiative feedbacks.
3.1 Small-scale processes in anvils and their implications
3.1.1 What microphysical processes occur in anvil cirrus life cycle?
Deep convective clouds form at the top of the boundary layer, and the first ice crystals begin to form by heterogeneous nucleation between 6 and 9 km altitude, roughly between −10 and −30 ∘C. Some liquid droplets, however, may persist up to the homogeneous freezing temperature of water (−38 ∘C) at about 10 km (Rosenfeld and Woodley, 2000). In recent years, much effort has been devoted to identifying which aerosols serve as INPs during convective ascent (Sect. 5.1), and several other reviews are devoted to summarizing this work (Kanji et al., 2017; Murray et al., 2021). As they ascend, ice crystals grow by deposition, a process whose rate is affected by crystal non-sphericity and remains challenging to represent (Sect. 5.3). A fraction of the large ice crystals formed via heterogeneous nucleation is likely to break up and produce a large number of smaller ice crystals – a process known as secondary ice formation (Ladino et al., 2017; Qu et al., 2022). Remote sensing observations and exploratory simulations generally indicate that secondary ice production can enhance ice crystal numbers 1000-fold but with infrequent occurrence (< 10 %) (Luke et al., 2021). The relative importance of primary nucleation versus secondary production as ice crystal sources in anvil clouds is still subject to large uncertainties (E. Hawker et al., 2021; Sullivan et al., 2017, 2018).
When convecting air parcels full of ice reach the level of neutral buoyancy, the air is injected into a stably stratified environment, which leads to flattening and spreading of this air and formation of a thick anvil cirrus cloud (Lilly, 1988). Within a few hours of detrainment, the largest ice crystals quickly precipitate out of the cloud and sublimate in the subsaturated layer below, reducing the ice content and optical depth of the anvil (Jensen et al., 2018b; Gasparini et al., 2021). The cloud loses additional mass by forming larger, rapidly falling snowflakes in a process called aggregation, which is relevant at temperatures warmer than −40 ∘C. Falling snowflakes can collect ice crystals through accretion, further decreasing the anvil ice water path (Heikenfeld et al., 2019).
As the anvil reaches its mature stage, cloud–radiation interactions as well as latent heating and cooling begin to play an important role in modulating its morphology and climatic effects (Sect. 3.1.2). These effects frequently manifest at the cloud scale and mesoscale (Dinh et al., 2024) but can also impact large-scale circulation in the tropics and extratropics (Schumacher et al., 2004; Voigt and Shaw, 2016; Voigt et al., 2019) (Sect. 3.1.3). Finally, sedimentation and sublimation dominate the decaying stage of the cloud. The decay timescale is therefore strongly dependent on the temperature (colder temperatures imply slower sublimation, Seeley et al., 2019), environmental relative humidity and size of ice crystals (Jensen et al., 2018b), and the mesoscale and synoptic-scale dynamical support.
3.1.2 How do microphysical processes determine diabatic heating?
The processes detailed in the previous section influence atmospheric temperature and pressure gradients through their associated latent and radiative heating. Micro-scale and mesoscale cloud radiative effects play an important role in the anvil cirrus life cycle. Latent heating dominates the contribution to cloud-scale circulation over radiative heating, especially early in the cloud life cycle and in the lower part of the cirrus outflow (Gasparini et al., 2019).
As noted before, tropical cirrus can generate positive or negative radiative effects at the top of atmosphere or surface based on their optical thickness and age. Microphysical processes and parameters play an important role in this modulation (Zhang et al., 1999). Modeling studies show that ice microphysics schemes can alter the top-of-the-atmosphere outgoing longwave radiation by as much as 30 W m−2, while the consideration of different ice habits can alter ice crystal number concentrations and resultant radiative effects drastically (Sullivan and Voigt, 2021; Lamraoui et al., 2023). Along with the small-scale physics and shape of ice crystals, their complexity – a term encompassing factors such as surface roughness and hollowness – can generate additional shortwave (SW) cooling on the order of 1 W m−2 (Järvinen et al., 2018). Cirrus optical properties can also alter top-of-the-atmosphere and surface fluxes by as much as tens of watts per square meter (W m−2) (Zhao et al., 2018; Yi, 2022).
Microphysical processes also influence – and are influenced by – the diurnal cycle of these fluxes. SW absorption by crystals produces a mesoscale circulation that buoys the cirrus and prolongs its lifetime (Sect. 3.1.3). Polarimetric difference measurements of the Global Precipitation Measurement Microwave Imager indicate that ice crystal size and shape have strong diurnal variations over land and are related to the cycles in macroscopic variables, such as vertical or horizontal extent (Gong et al., 2017). Flux divergences, or atmospheric cloud radiative heating rates, have been relatively less studied than the top-of-the-atmosphere or surface fluxes. But cloud radiative heating, especially in the upper troposphere, has a more direct influence on circulation and is modulated strongly by ice microphysical factors such as the aerosol dependence of the nucleation scheme or the consistency between the microphysical and optical parameterizations (Sullivan and Voigt, 2021; Sullivan et al., 2022). Cloud radiative heating climatologies and ensembles, both observational and model-based, indicate the largest differences in the tropical upper troposphere (Voigt et al., 2019; Cesana et al., 2019), and the associated ice microphysical controls warrant further study.
3.1.3 How are anvil cirrus circulations affected by diabatic heating?
The interaction of ice crystals with radiation in anvil cirrus leads to changes in upper-tropospheric temperature gradients, which drive large-scale and mesoscale circulations. For brevity, we omit the cirrus impacts on large-scale circulations in this opinion paper (interested readers can find more in Voigt et al., 2019, 2021). Typically, mesoscale circulations consist of an updraft within the radiatively heated cloud with horizontal outflow near cloud top and compensating sinking motion in nearby clear-sky areas. The circulation is closed by inflow near cloud base, where the maximum radiative heating is located (Ackerman et al., 1988; Durran et al., 2009; Dinh et al., 2010; Gasparini et al., 2019; Dinh et al., 2024). Such circulations are boosted by SW heating during daytime; in-cloud updrafts around the middle of the day, when the insolation is high, can be strong enough to counteract the sedimentation of small ice crystals, prolonging cloud lifetime (Deng and Mace, 2008; Wall et al., 2020; Gasparini et al., 2022). Circulation patterns can be more complex in anvil cirrus of optical depths larger than approximately 3 with strong cloud-top cooling due to emission of longwave radiation, which induces an additional circulation cell. This consists of a sinking inflow of drier environmental air that erodes the cloud top and decreases the lifetime of the cloud (Gasparini et al., 2022).
Prolonged exposure to intense cloud heating can cause isentropic surfaces to warp, leading to development of unstable, turbulent, convective layers in high clouds (Dobbie and Jonas, 2001; Dinh et al., 2010; Ferlay et al., 2014; Schmidt and Garrett, 2013). Radiatively driven turbulence within anvils can occasionally trigger new ice crystal nucleation, prolonging the lifetime of the cloud and influencing the optical depth distribution of anvils (Hartmann et al., 2018; Sokol and Hartmann, 2020).
It is currently not known whether and how the interactions between microphysics, radiation, latent heating, and the resulting circulations may change in a warmer world and whether this contributes substantially to the uncertainty in anvil cirrus feedbacks (as outlined in Sect. 3.2).
3.2 Large-scale anvil cloud climate feedbacks
3.2.1 What controls the altitude of anvil cirrus?
It can be argued on the basis of radiative–convective equilibrium physics that the tops of convective clouds should remain at about the same temperature as the climate changes (Hartmann and Larson, 2002). To obtain this result it must be assumed that water vapor is the dominant agent for cooling the atmosphere, in which case the ability of the atmosphere to cool and thereby balance convective heating declines rapidly at a temperature of about 220 K ( ∘C). This marks the top of the actively convecting layer of the atmosphere (Fig. 2) and is where the strongest net detrainment of cloud ice occurs (Kuang and Hartmann, 2007; Harrop and Hartmann, 2012; Hartmann et al., 2019; Jeevanjee and Fueglistaler, 2020). If the highest cloud tops do remain at a constant temperature as the climate changes, this fixed anvil temperature (FAT) produces a climate with a more positive (global warming intensifying) radiative feedback than if the clouds remained at a fixed pressure because the emission from an optically thick cloud top remains unchanged as the surface temperature warms, decoupling the upward longwave cloud emission from the surface temperature (Zelinka and Hartmann, 2010). The assignment of a positive feedback to this FAT mechanism is complicated by the fact that the emission temperature of the clear atmosphere in which the clouds are embedded also tends to remain constant as the climate changes for the same physical reasons on which the FAT hypothesis is based (Koll and Cronin, 2018; Stevens and Kluft, 2023).
The FAT hypothesis of fixed cloud-top temperatures assumes the dominance of water vapor for atmospheric radiative cooling and equilibration of clouds and climate, as over the warm oceans. If these assumptions are violated as the climate warms, the average cloud-top temperature can vary. For example, if the 220 K isotherm extends upward into the region of the atmosphere where absorption of solar radiation by ozone becomes important, then the cloud tops will warm as the surface temperature increases (Thuburn and Craig, 2002; Kuang and Hartmann, 2007; Harrop and Hartmann, 2012; Seidel and Yang, 2022). We know that individual convective cells do penetrate above the 220 K level and occasionally overshoot beyond the tropopause. Over land with a strong diurnal cycle, the convection may be out of equilibrium with the global tropics and cloud tops may penetrate beyond the temperature threshold predicted by the FAT hypothesis. Average cloud-top temperatures are different over the western and eastern Pacific Ocean, although the relationship between cloud abundance and radiatively driven large-scale divergence seems to hold (Kubar et al., 2007). The radiative cooling rate at a fixed temperature can vary depending on the lapse rate (Hartmann et al., 2022), so if lapse rates change with the climate, then we might expect cloud-top temperature to also change. Tropical cloud properties over land areas are different from those over ocean areas, with updraft velocities over land exceeding those over the oceans (Liu et al., 2007). As the climate changes, the distribution of sea surface temperature and the contrast between land and sea surface temperature may shift, in turn altering the average cloud-top temperature and Earth's energy balance. The response of anvil cirrus temperature and amount to changes in the geographical distribution of surface temperature should be a key topic for ongoing research.
3.2.2 What controls the amount of anvil cirrus on large scales?
Changes in anvil cirrus cloud amount (ACA) and their associated climate implications have been a subject of much interest since the publication of the iris hypothesis over 20 years ago (Lindzen et al., 2001). Based on 20 months of satellite observations, this original study found ACA to be inversely related to sea surface temperature (SST). While controversial at the time of its publication (Hartmann and Michelsen, 2002; Fu et al., 2002), the iris hypothesis raised the important questions of whether changes in ACA could act as a significant feedback on global warming. Today, this question remains relevant and without consensus.
The study by Lindzen et al. was the first of many seeking to understand the ACA–SST relationship by leveraging interannual climate variability over the satellite record (Su et al., 2008; Zelinka and Hartmann, 2011; Behrangi et al., 2012; Igel et al., 2014; Zhou et al., 2014; Choi et al., 2017; Liu et al., 2017; Kubar and Jiang, 2019; Saint-Lu et al., 2020, 2022; Höjgård-Olsen et al., 2022). These studies have produced varied results, which may be expected due to differences in study region and period, satellite instrumentation, how ACA is quantified, and how, if at all, anvil cirrus clouds are distinguished from TTL cirrus and deep convective cores. Nevertheless, on the whole, these observational assessments suggest an inverse relationship between tropical SST and ACA, although the strength of this relationship likely varies from region to region and as a function of anvil cirrus thickness.
Most CRMs and GCMs also suggest that ACA decreases with warming (Zelinka and Hartmann, 2010; Wing et al., 2020; Stauffer and Wing, 2022), albeit with less consistency than observations of interannual variability. Increases in ACA with warming were found in one-third of the models participating in the Radiative–Convective Equilibrium Model Intercomparison Project (RCEMIP; Wing et al., 2018, 2020) and have long been a salient feature of NICAM, a GSRM (Tsushima et al., 2014). These inconclusive results, along with the known sensitivity of simulated anvil cirrus to factors such as horizontal resolution (Jeevanjee and Zhou, 2022) and ice crystal habit assumptions (Lamraoui et al., 2023), show just how difficult anvil cirrus clouds are to model (see Sect. 5).
In addition to these assessments, significant progress has been made in understanding the physical mechanisms underlying the ACA response to warming. The original iris hypothesis speculated that the decline in ACA with increasing SST was caused by the enhancement of precipitation efficiency (PE) within convective updrafts (Lindzen et al., 2001). While this mechanism has been demonstrated in models (Mauritsen and Stevens, 2015; Jeevanjee and Zhou, 2022; Li et al., 2019), it is difficult to assess in the real world because PE, as is it traditionally defined, is a difficult quantity to observe on a large scale. Studies using modified definitions of PE suited for observational analyses have yielded conflicting results (Ito and Masunaga, 2022; Choi et al., 2017). Moreover, whether or not PE actually increases in response to warming remains uncertain.
An alternative explanation relates ACA to the overall strength of the convective overturning circulation in the tropics, which has long been expected to weaken with warming (Betts and Ridgway, 1989; Knutson and Manabe, 1995; Held and Soden, 2006). Several explanations have been provided for this weakening (see Jenney et al., 2020 for a thorough discussion), but one – the stability iris mechanism outlined in Bony et al. (2016) – has come to dominate the discussion of ACA (Fig. 4). In brief, the stability iris hypothesis states that surface warming causes the tropical troposphere to become more stable, which leads to a reduction in the radiatively driven, clear-sky convergence at the anvil cloud level (Zelinka and Hartmann, 2010). Because clear-sky convergence must be balanced by the detrainment of mass from deep convection, a reduction in clear-sky convergence leads to reduced detrainment and reduced ACA. Observations (Zelinka and Hartmann, 2011; Saint-Lu et al., 2020, 2022) and models (Stauffer and Wing, 2022) are generally consistent with the stability iris hypothesis, although a small number of the RCEMIP models produce simultaneous reductions in clear-sky convergence and increases in ACA in apparent violation of stability iris physics (Stauffer and Wing, 2022). While the stability iris hypothesis represents significant progress and may very well describe the first-order impact of warming on ACA, other work suggests that there is more to the story (Beydoun et al., 2021; Jeevanjee, 2022). This is hardly surprising considering that anvil cirrus dynamics are affected by processes occurring on much smaller scales than those addressed by stability iris physics (Sect. 3.1.1).
Another factor that is known to affect ACA is the large-scale aggregation of convection. Observations (Yuan and Houze, 2010; Tobin et al., 2012, 2013; Stein et al., 2017) and models (Bony et al., 2016; Wing and Cronin, 2016; Wing et al., 2020) both show that higher degrees of convective aggregation are associated with reduced ACA. This link appears to be robust, suggesting that changes in aggregation could modulate the ACA response to warming. Unfortunately, convection-resolving models do not provide a consensus on how warming impacts aggregation (Wing et al., 2020); even if they did, model-simulated aggregation is not exactly the same as the forms of convective organization observed in the tropics (Holloway et al., 2017). Changes in aggregation would have very important impacts on Earth's energy budget independent of anvil cirrus (Bony et al., 2020), and continued study of aggregation may thus prove a doubly beneficial endeavor, as progress would benefit not only our understanding of the ACA feedback but also of Earth's climate sensitivity on the whole.
3.2.3 What controls the radiative effect of anvil cirrus on climatological scales?
Even if we were to take for granted that ACA declines with warming, that alone does not tell us whether changes in ACA are a positive, negative, or neutral feedback. Unlike tropical marine low clouds, which have a uniformly negative CRE, anvil cirrus can act both as positive and negative forcing agents depending on their optical thickness and altitude, which vary over the course of their life cycle (Jensen et al., 1994; Hartmann et al., 2001). Fresh, optically thick anvils have a negative CRE, while thinner, aged anvils have a positive CRE. The sign and magnitude of the ACA feedback will therefore depend on how changes in ACA are distributed across the wide spectrum of anvil cirrus observed in the tropics. A preferential reduction in thick anvil coverage, for example, may constitute a positive feedback, while a preferential reduction in thin anvil coverage would be a negative feedback. Because nonuniform changes in the area of thick and thin clouds would alter the mean anvil optical depth, the anvil area and optical feedbacks are closely intertwined; for simplicity, we use the term “ACA feedback” to refer to both changes in cloud area and the resulting changes in mean optical depth.
Constraining the ACA feedback has proved difficult, as evidenced by the fact that it was the least certain of all cloud feedbacks in a recent assessment of equilibrium climate sensitivity by Sherwood et al. (2020). In that paper, ACA feedback was estimated to be −0.2 W m−2 K−1 with a likely range of −0.4–0 W m−2 K−1. In apparent disagreement with that assessment, it is our opinion that a positive ACA feedback cannot be ruled out at this time. As we discuss below, attempts to constrain the ACA feedback from interannual variability have produced mixed results, and potential changes in anvil optical depth have not been yet been adequately studied.
Attempts to constrain the ACA feedback have so far produced mixed results. Model-based assessments are inconclusive on the whole (Mauritsen and Stevens, 2015; Li et al., 2019; Zelinka et al., 2022) and have relied primarily on GCMs, which, in our opinion, do not reliably simulate anvil processes and parameterize cloud fraction. Assessments relying on observations of interannual climate variability have taken varied methodological approaches and have produced estimates ranging from strongly negative to slightly positive (Ramanathan and Collins, 1991; Lindzen et al., 2001; Lin et al., 2002; Chambers et al., 2002; Williams and Pierrehumbert, 2017; Choi et al., 2017; Ito and Masunaga, 2022; McKim et al., 2023). These assessments are complicated by the fact that variability in observed CRE is related not only to ACA but also to in situ formed cirrus, low- and mid-level cloud properties, anvil altitude (FAT), and clear-sky radiative fluxes. Moreover, interannual temperature variability, which is primarily ENSO-driven, is associated with a spatial reorganization of convection that is not necessarily representative of long-term warming. While dealing with these factors is not always straightforward, continued empirical assessment may be worthwhile in the absence of robust theory relating ACA and CRE.
Changes in the climatological CRE of anvil cirrus may result not only from changes in ACA, but also from changes in anvil cloud optical depth. Such changes may arise if fresh, optically thick anvils and aged, thin anvils are affected differently by warming. This seems likely, since thick and thin anvils are affected by different processes and have been shown to be affected differently by changes in convective organization (Stein et al., 2017). Anvil optical depth feedback has not, to our knowledge, been systematically assessed, so its importance relative to changes in ACA is unknown. However, observational work generally suggests that the cirrus population in convective regions thins with warming (Liu et al., 2017; Kubar and Jiang, 2019; Höjgård-Olsen et al., 2022), although it is unclear how much of this thinning comes from changes in anvil optical depth as opposed to changes in the relative numbers of anvil and in situ cirrus clouds. If it is indeed anvil-related to some degree, such thinning would have important implications for climatological anvil CRE. For this reason, among others, we believe that the anvil optical depth question should be urgently pursued in future work.
The tropical tropopause layer (TTL; ≃ 14–19 km) is a region with ubiquitous optically thin cirrus clouds (Fig. 2). The tropical mean cloud cover in this layer indicated by CALIOP measurements is approximately 30 % (compare with Fig. 1), and in situ aircraft measurements with better sensitivity indicate even higher cloud frequencies (Davis et al., 2010). Given the relatively low optical depths of these clouds, their impact on the top-of-the-atmosphere radiative flux is much smaller than for lower-lying convectively generated anvil cirrus: TTL cirrus clouds exert a small but non-negligible tropical average net top-of-the-atmosphere radiative effect of about 4 W m−2 (Haladay and Stephens, 2009; Lee et al., 2009). TTL cirrus radiative heating within the atmosphere is an important component of the TTL thermal budget, generating a tropical average heating of about 0.1 K d−1, with peak values of up to 0.5–1 K d−1 (Yang et al., 2010; Fu et al., 2018).
4.1 What controls TTL cirrus occurrence and microphysical properties?
Much of the interest in the TTL in general, and TTL cirrus in particular, stems from the fact that upward motion across the tropopause as part of the Brewer–Dobson circulation provides the primary source of air to the stratosphere. TTL cirrus clouds regulate the humidity of the stratosphere by freeze-drying the air to approximately the saturation mixing ratio corresponding to the cold tropical tropopause temperature. This mechanism provides an indirect climate impact of TTL cirrus since small changes in stratospheric humidity can have important climate (Forster and Shine, 2002; Solomon et al., 2010; Huang et al., 2020) and stratospheric ozone chemistry impacts (Shindell, 2001).
Upward vertical motion and very low temperatures maintain high relative humidity in the TTL, which leads to higher zonal cloud occurrence frequencies than other regions in the atmosphere. The regional distribution of TTL cirrus is controlled by the TTL thermal structure; rapid horizontal motions transport air into cold tropical tropopause regions, such as the western Pacific, where TTL cirrus clouds form (Holton and Gettelman, 2001). The temporal variability of TTL cirrus occurrence is largely controlled by temperature variability driven by atmospheric waves, including gravity, Kelvin, and mixed Rossby–gravity waves (Boehm and Verlinde, 2000; Immler et al., 2008; Kim et al., 2016; Sweeney et al., 2023). TTL cirrus structure and lifetimes will be affected by wind shear, wave-driven temperature variability, and possibly small-scale dynamics driven by cloud radiative heating (Dinh et al., 2010). However, TTL cirrus may typically be too short-lived for cloud radiative heating to drive thermal instability (Jensen et al., 2011).
TTL cirrus clouds generally form above the primary detrainment level for tropical deep convection (≃ 12–13 km, Fig. 2). However, extreme deep convective systems that overshoot their level of neutral buoyancy and extend up to near the tropical tropopause provide the primary source of water vapor for the TTL (Ueyama et al., 2018). Hence, TTL cirrus clouds do tend to occur frequently in convectively active tropical regions. In some cases, TTL cirrus clouds form above deep convective systems (i.e., pileus clouds), presumably driven by convective lifting and cooling (Garrett et al., 2004). However, it is challenging to disentangle the relationships between convection and TTL thermal variability since convective regions in the tropics tend to coincide with cold tropopause regions.
Nucleation of ice crystals under cold TTL conditions can occur via either homogeneous freezing of aqueous aerosols or heterogeneous nucleation on insoluble solid particles. Numerous laboratory experiments have shown that homogeneous freezing at low temperatures requires very large supersaturation with respect to ice (60 %–100 %; Schneider et al., 2021 and references therein), and substantial supersaturation is prevalent in the TTL (Rollins et al., 2016). In situ measurements showing bursts of numerous small ice crystals forming at high supersaturations provide direct evidence of homogeneous freezing in the TTL (Jensen et al., 2013, 2022). Heterogeneous nucleation at low temperatures is less well-understood, but particle types such as mineral dust, crystalline ammonium sulfate, and glassy organic aerosols may serve as INPs in the TTL (Sect. 5.1). If effective INPs are abundant enough, they could increase TTL cirrus occurrence frequency, affect TTL cirrus microphysics, and possibly impact dehydration efficiency (Jensen et al., 2018a). If homogeneous freezing dominates, then TTL cirrus ice crystal number concentration will be primarily controlled by cooling rate. On the other hand, if heterogeneous nucleation dominates, then the ice crystal number concentration will be limited by the abundance of INPs.
4.2 How well are TTL cirrus represented in climate models?
Representing TTL cirrus in global climate models presents some of the same challenges as for anvil cirrus, including the mismatch between global model grid box sizes and cloud-scale structures and physical processes. A particular issue for TTL cirrus is the importance of small-scale, high-frequency gravity waves for TTL cirrus microphysical processes. Stochastic approaches have been developed recently whereby temperature perturbations at each model time step are randomly selected from Laplace distributions based on quasi-Lagrangian super-pressure balloon measurements in the lowermost stratosphere (Podglajen et al., 2016; Kärcher and Podglajen, 2019) (Sect. 2.2). Most global models use bulk microphysics schemes to simulate cirrus clouds; bulk microphysics schemes assume a functional form for the ice crystal size distribution. Since these schemes were developed based on measurements in warmer clouds, it is not clear how well they work for cold TTL cirrus. Maloney et al. (2019) recently used a bin microphysics scheme coupled to the CAM5 global model to simulate TTL cirrus and compare with aircraft observations. In contrast to bulk schemes, bin schemes discretize the size distribution into many size bins. This study highlighted the difficulty of properly simulating TTL humidity, particularly its small-scale variability caused by gravity waves, and cloud properties with a relatively coarse-resolution global model. Further, such a detailed, computationally expensive microphysical scheme is not appropriate for long-duration simulations of a changing climate.
4.3 How will TTL cirrus clouds change in the future?
The issue of how TTL cirrus (and, correspondingly, the stratospheric-entry water vapor mixing ratio) will evolve in a changing climate potentially involves a number of processes and feedbacks. TTL cirrus occurrence is tightly coupled to tropopause temperatures, as indicated by the observed covariance between quasi-biennial-oscillation- and ENSO-driven interannual variability in tropopause temperature and TTL cirrus occurrence (Davis et al., 2013). They also follow the seasonal cycle in the tropopause temperatures, largely driven by varying strength of the Brewer–Dobson circulation (Li and Thompson, 2013; Fu, 2013; Tseng and Fu, 2017). As noted above, extreme deep convective systems extending into the TTL are the primary source of water vapor to the region; hence, increases or decreases in future occurrence of overshooting convection would presumably result in corresponding increases or decreases in TTL cirrus occurrence.
Trends in tropopause temperature, TTL humidity, and deep convection occurrence are difficult to discern from the available measurement records. Ladstädter et al. (2023) and Scherllin-Pirscher et al. (2021) have used GPS radio occultation measurements to show a positive trend in TTL temperature, but given the large interannual variability, it is not clear whether the relatively short data record indicates a long-term trend. Assessing TTL humidity trends is even more difficult given the extreme temporal variability and limited observational records.
A lack of proper representation of cirrus clouds in global models compromises the fidelity of climate projections (e.g., Sherwood et al., 2020 and Sect. 3.2). The last few decades have seen major improvements in the model representation of cirrus and in the understanding of observed cirrus cloud properties (Sect. 2). Among the areas of research that have received increased attention is the representation of cirrus cloud fraction and ice water content in GCMs and numerical weather prediction models (NWPs). Additionally, there is a growing recognition of the importance of aerosol–cirrus interactions and their impact on climate.
In the past, cirrus cloud representation in models suffered due to the use of thermodynamic equilibrium assumptions, which are more suited for liquid water clouds (Tiedtke, 1993), since vapor pressure adjustment is much faster over liquid water than over ice (Korolev and Mazin, 2003). These assumptions affect both the macroscopic fractional cloud coverage and the microphysics of cloud ice formation, preventing ice supersaturation (i.e., relative humidity exceeding ice saturation) from occurring (Kärcher and Burkhardt, 2008). A prognostic fractional cloud coverage was implemented in one GCM along with an advanced representation of the impact of aerosols in cirrus formation to simulate cirrus microphysical and macrophysical properties consistently (Muench and Lohmann, 2020). The consistency between the simulated cirrus cloud cover and ice-supersaturated areas is crucial for accurately representing anthropogenic effects on cirrus. To date, many global cloud microphysical schemes employ a parameterization of ice nucleation as an explicit source of ice crystals from the atmospheric aerosol and solve diffusion equations describing the uptake of water vapor on ice crystals, allowing ice supersaturation to occur over multiple time steps.
The formulation of microphysical two-moment schemes predicting both bulk cloud particle number and mass in GCMs enabled modelers to simulate how cirrus clouds respond to changes in the abundance of liquid solution droplets and solid INPs, considered better ice-forming agents. While cirrus properties are relatively insensitive to changes in homogeneous solution droplet freezing, adding INPs leads to a decrease in total cirrus ice crystal number concentrations. Competing ice nucleation appears to occur frequently during the formation of cirrus clouds (DeMott et al., 2003; Froyd et al., 2022). While significant progress has been achieved in studying aerosol–cirrus interactions by coupling cloud and aerosol schemes within global models, parameterized cirrus and aerosol microphysics must be supplemented by parameterizations of the underlying small-scale dynamical forcing of ice supersaturation. As a result, global model estimates of radiative forcing from aerosol cirrus interactions remain highly uncertain.
In our view, the study of small-scale processes needs to be prioritized to improve our ability to predict future cirrus evolution and associated climate feedbacks more reliably: ice activity and number concentrations of INPs, magnitude and variability of small-scale vertical wind speeds in ice-supersaturated areas, and vapor growth of cirrus ice crystals. These interconnected factors influence the life-cycle-integrated radiative impact of cirrus. The list of topics offered here is not all-inclusive but follows directly from the research themes delineated above.
5.1 What are the properties of ice-nucleating particles?
In an ice formation event, solution droplets freeze homogeneously once ice supersaturation exceeds 70 % in the TTL. INPs become ice-active and thus nucleate ice crystals within a range of ice supersaturations. Typically, efficient INPs such as mineral dust particles require ice supersaturation values exceeding ≃ 30 % to activate. Early forming ice crystals take up supersaturated vapor and thereby change conditions for further ice nucleation. In this way, INPs, if present, weaken or prevent homogeneous freezing, thus lowering ice crystal number concentrations in cirrus. Mineral dust is widely recognized to be the most important INP in the upper troposphere, well-characterized in terms of both ice activity (Atkinson et al., 2013; Ullrich et al., 2017) and number concentrations (Froyd et al., 2022). However, properties of other INP types remain poorly constrained by observations, especially in the TTL (Sect. 4). For example, ice nucleation properties of black carbon (BC) particles depend strongly on the combustion source and whether they have been processed within clouds (Marcolli et al., 2021). Simulating mineral dust and BC particles in global models also presents a significant challenge due to uncertainties associated with source specification, transport, and scavenging by clouds. Comparisons between global model simulations and observations of dust and BC particles have shown significant discrepancies (Zhang et al., 2020; Lian et al., 2022).
Aerosols in the TTL consist of aqueous, sulfate-containing particles with other (largely unspecified) organic compounds. When present in sufficiently low number concentrations, INPs have a minimal impact on cirrus formation so that crystal numbers are instead dictated by cooling rates and temperature (Kärcher, 2022). A detailed model study, constrained by observations, suggests that only unusually large number concentrations of mineral dust or ammonium sulfate INPs have a notable effect on TTL cirrus properties and probability of occurrence (Jensen et al., 2018a). Moreover, the lack of information about the TTL INP properties translates into large uncertainties in aerosol–TTL cirrus interactions.
Very little is known about ice-forming aerosol particles in anvils and the small-scale thermodynamic environment promoting the transition of anvils into long-lived, large-scale cirrus. INPs may either be entrained from the ambient air surrounding the anvil or left over from detrained convective outflow (Twohy et al., 2017; Sauter et al., 2019). Ice formation within existing cirrus can only take place in regions with very few ice crystals, as vapor deposition on pre-existing ice crystals generally consumes the available vapor, preventing supersaturation high enough to allow ice nucleation (Kärcher et al., 2006). Nevertheless, in situ homogeneous or heterogeneous ice nucleation may play a role in the maintenance of long-lived anvil cirrus (Jensen et al., 2009; Hartmann et al., 2018; Sokol and Hartmann, 2020).
5.2 How well is ice supersaturation and its dynamical forcing represented in models?
Ice supersaturation determines the properties of cirrus clouds. Water vapor injected by deep convection moistens the upper troposphere and produces ice-supersaturated regions. Adiabatic cooling can generate high supersaturation and cirrus ice crystal number concentrations in localized regions. Cirrus ice is removed by sublimation in warmer or drier air, often caused by large-scale subsidence. Another important sink of ice is sedimentation into lower, subsaturated layers of the atmosphere where the ice will sublimate.
The extent and longevity of large-scale ice-supersaturated regions in global models depend on the synoptic situation and the model's ability to simulate the upper-tropospheric water budget and temperature fields accurately. Occurrence frequencies and seasonal variations of upper-tropospheric ice supersaturation are reasonably well-reproduced in the ERA-Interim reanalyses over the North Atlantic (Reutter et al., 2020) compared to satellite observations (Lamquin et al., 2012). However, the fine structure and magnitudes of ice supersaturation within such regions are not well-reproduced. This deficiency is tied mainly to the representation of cloud-scale dynamical forcing and cloud ice microphysics in high-resolution models. Often, poor vertical resolution contributes to the inability to reproduce thin observed ice-supersaturated layers. The spatial distribution of cirrus ice is particularly strongly affected by temperature fluctuations generated by mesoscale gravity waves (GWs) (Kim et al., 2016; Podglajen et al., 2018). Cirrus ice crystals form as a result of GW activity and may be affected by turbulence. Tropical GW activity is closely associated with deep convective systems (Alexander and Pfister, 1995; Corcos et al., 2021). Mean GW-induced updrafts are at least 10 times faster than the slow, large-scale uplift and are therefore the main driver of homogeneous freezing and aerosol–cirrus interactions (Dinh et al., 2016; Kärcher et al., 2022).
Both climate and cloud-resolving models struggle to realistically represent the spectrum of upper-tropospheric updrafts (Nugent et al., 2022; Atlas and Bretherton, 2023). Failure to represent the high-frequency variability of vertical wind speeds and associated mesoscale temperature fluctuations caused by GWs prevent us from accurately representing in situ ice cloud formation. Only a combination of high horizontal (0.5–1 km) and high vertical resolution (100–200 m) in the upper troposphere allows models to resolve GWs and tropospheric updraft variability (Kuang and Bretherton, 2004; Kim et al., 2016; Skamarock et al., 2019). These resolutions are beyond the reach of GSRMs for now.
In the tropics, small-scale turbulence is mainly associated with deep convection (Lane et al., 2003; Podglajen et al., 2017; Barber et al., 2018; Atlas and Bretherton, 2023) and in-cloud radiative heating (Gasparini et al., 2019). In the case of anvil cirrus, radiatively driven dissipation rates of turbulent kinetic energy (>10−3 m2 s−3) are much larger than typical upper-tropospheric values based on cloud-resolving model simulations (Hartmann et al., 2018).
The effect of micro-scale turbulence in cirrus ice formation has not yet been understood. In fact, ice nucleation and growth take place below the viscous dissipation scale, at length scales <10 mm. Yet, in all cloud models, cirrus ice formation is forced by homogeneous moisture and temperature fields with magnitudes of ice supersaturation set by the much larger resolved (model grid) scales. Thus, turbulence, inhomogeneous mixing, and molecular diffusion of moisture and heat affect nucleated ice crystal numbers in unknown ways.
5.3 What controls cloud ice growth from vapor?
Depositional growth is the primary source of cloud ice mass and determines cirrus ice crystal habits, such as columns, plates, or bullet rosettes. Deposition growth rates are determined by vapor and heat diffusion through the air and attachment processes at the ice surface that control the incorporation of adsorbed molecules into the crystalline lattice (Libbrecht, 2005; Lamb and Verlinde, 2011). In cirrus models, surface kinetic processes are treated based on deposition coefficients. These coefficients generally depend on ambient ice supersaturation, temperature, pressure, and ice crystal size and decline during ice growth, as facets develop (Pokrifka et al., 2020). As depositional growth rates by diffusion of water vapor decrease with increasing particle size, aggregation takes over as the dominant growth mechanism for crystals with maximum dimensions larger than a few hundred micrometers (Sölch and Kärcher, 2011).
Microphysical models are almost exclusively based on assuming ice crystals to be perfect spheres characterized by a single constant deposition coefficient. Cirrus models resort to this crude approximation due to the lack of a complete theory of vapor growth encompassing the full cirrus temperature regime and to prevent adding additional tracers to represent different ice crystal habits. While bulk water uptake can be modeled reliably with this approximation, it does not allow the prediction of habits, which importantly modulate ice cloud radiative effects and ice crystal fall speeds.
More research is needed to advance deposition coefficient models at cirrus temperatures. Measurements of ice deposition coefficients need to be extended to cirrus temperatures. Theory development will most likely be based on controlled laboratory experiments. Measurements in large aerosol chambers may provide a link between laboratory and real-world conditions (Lamb et al., 2017). Any progress in this direction will improve estimates of ice crystal fall speeds and aggregation efficiencies and thus the simulation of anvil life cycle (Sect. 3.1.1).
This section explores several strategies and perspectives for improving our understanding of tropical cirrus clouds. We discuss the need for continued development and updating of cirrus cloud parameterizations, particularly with respect to ice nucleation, growth, and micro-scale turbulence. Discrepancies between coarse climate models, basic physical understanding, and observations of aerosol–cirrus interactions and anvil life cycle are a cause of concern that will be hard to resolve, as long as cloud-scale processes remain parameterized. We therefore emphasize the benefits of high-resolution models, while acknowledging the remaining challenges associated with small-scale parameterized processes, especially microphysics. In addition, we highlight the potential of various flow-following approaches and both in situ and satellite observations to improve the understanding of cirrus processes. Overall, these approaches, coupled with advances in big data analytics and computing power, offer promising avenues for advancing our knowledge of tropical cirrus clouds.
6.1 Representation of ice microphysics in models
While increasing resolution helps to improve the representation of cirrus clouds in models, processes at the molecular level, such as ice nucleation and growth, still need to be parameterized. Cirrus parameterizations will therefore need to be continually developed and updated in light of new knowledge about the types and ice-forming properties of INPs that are, or might become, increasingly present in the upper troposphere. If future research identifies a significant impact of turbulent mixing on cirrus ice formation (Sect. 5.2), the impact of micro-scale turbulence on ice nucleation and growth will also need to be considered in parameterizations of cirrus microphysics. Another case of concern is the proper representation of the ice crystal aggregation process and the subsequent generation of ice precipitation (Sölch and Kärcher, 2010).
An interesting recent development in this field is the Lagrangian microphysical schemes coupled to weather and climate models, which promise breakthroughs in the process understanding of cloud and precipitation formation (Jensen and Pfister, 2004; Sölch and Kärcher, 2010; Grabowski et al., 2019; Morrison et al., 2020). The particle-following approach offers several advantages over more traditional Eulerian approaches; e.g., the microphysical evolution of the cloud and the sources of ice crystals become easier to track, while unresolved turbulence can be considered in ice crystal evolution. Such approaches also eliminate artificial numerical diffusion, which cannot be completely avoided in more traditional modeling approaches. On the downside, particle-based microphysical approaches require large computational resources and a good fundamental understanding of the underlying microphysical properties, such as ice particle shapes (Shima et al., 2020). Nevertheless, once available for climate modeling, Lagrangian microphysics could lead to substantial progress in the understanding of cirrus clouds.
A second strategy in improving model representation of cirrus clouds has been the removal of artificial distinctions and thresholds within microphysics schemes. Eight years ago, a particle property paradigm was first proposed by Morrison and Milbrandt (2015) in which frozen hydrometeor categories of ice versus snow versus hail versus graupel were removed in favor of tracking a rime fraction. Wang et al. (2021) have documented the improved performance of the predicted particle property (P3) scheme relative to a traditional two-moment schemes for deep convective scenes over the US. Other artificial thresholds exist in microphysics schemes, most notably the minimum and maximum bounds imposed on ice crystal number concentrations. Indeed, in a global analysis of ice microphysical process rates, Bacer et al. (2021) found that artificial numerical tendencies contribute to a third of the overall process budget.
However, the careful budget analysis of the underlying microphysical source and sink processes is often neglected (refer to Sect. 5.1 for a discussion on nucleation). Modern microphysical schemes allow the prognostic numerical computation of ice crystal number and mass concentrations; however, most often, only the resulting total cloud ice mass, number, or effective radius is analyzed. Tracking process rates and constructing ice microphysical process budgets are potential avenues to interpret model biases in ice water content, ice crystal number concentration, or cirrus cloud lifetime.
6.2 High-resolution models
The realistic representation of ice cloud microphysics (Morrison et al., 2020) and their coupling with macrophysics (e.g., Dietlicher et al., 2019), radiation, and climate are persistent challenges in climate modeling. A first step to simplify and partially resolve this challenge is to operate at the cloud-resolving scale, circumventing the need for separate convective ice microphysics. Multiple studies highlight the influence of updrafts on tropical cirrus clouds (e.g., Sullivan et al., 2016; Donner et al., 2016; Barahona et al., 2017), and convection-permitting resolutions will better capture the distribution of vertical velocities. GSRM simulations therefore represent a step forward in the representation of tropical high-cloud properties and their evolution.
In addition, GSRMs explicitly resolve deep convection and the development of anvil cirrus. Since convection is the main source of GWs in the tropics, the number and realism of resolved GWs should increase in such simulations (Stephan et al., 2019). The same is true for waves from orographic sources and perturbations of the large-scale flow field, which are more common in the extratropics. However, while most low-frequency GW activity is simulated by GSRMs, vertical wind speed variances are not yet fully captured at high intrinsic wave frequencies (Podglajen et al., 2020; Atlas and Bretherton, 2023), which are important for in situ cirrus formation. Current GSRMs do not fully capture vertical wind speed variance as observed by balloons (Köhler et al., 2023), at least in part because the current generation of GSRMs lacks the required horizontal and vertical resolution.
The explicit simulation of deep convection allows GSRMs to realistically represent the diurnal cycle of convection (Nugent et al., 2022), the lifetime of deep convective systems, and their anvil cirrus cloud shield (Feng et al., 2023). GSRMs are capable of simulating cirrus initiated by (overshooting) convection within the TTL (Nugent et al., 2022). Despite their increasing realism and explicit transport of water vapor and frozen water mass fluxes in the upper troposphere, the current generation of models exhibit large intermodel spread and significant biases in, for example, frozen water path, cirrus radiative properties, or upper-tropospheric vertical velocity distribution (Roh et al., 2021; Nugent et al., 2022; Turbeville et al., 2022).
Large intermodel spread in high-resolution simulations is not a surprise, since cloud microphysical processes still need to be parameterized. In fact, most of the intermodel spread in GSRMs can be attributed to the parameterization of cloud microphysics and their coupling to the dynamical forcing (Nugent et al., 2022; Turbeville et al., 2022). This point emphasizes the need for continuous development and updating of ice microphysical schemes and cirrus parameterizations. Unfortunately, the microphysical schemes used in the current generation of GSRMs are often inferior to those used in state-of-the-art GCMs and are therefore inadequate for addressing questions related to, for example, tropical anvil cirrus cloud–climate feedbacks. Fortunately, some of these biases can be addressed through relatively simple modifications to the ice microphysical schemes, e.g., by removing unphysical limiters on microphysical process rates (particularly ice crystal number) that are often present in microphysical codes (Atlas et al., 2022).
6.3 Evolution perspective
Both observational and modeling data typically provide only snapshots or temporal averages of the current state of clouds in the atmosphere. While these insights help us understand the microphysical and macrophysical properties of anvil cirrus, they may not provide enough detail to understand the underlying processes, such as ice crystal formation. A deeper understanding of such processes would be of particular significance for anvils, whose rapid evolution has substantial effects on both precipitation and radiative fluxes. As a more natural perspective on the evolution of cirrus clouds, Lagrangian studies provide a level of process understanding that is impossible to achieve with Eulerian analysis. Three such approaches have been used to date to advance knowledge of tropical cirrus.
-
Storm tracking. Storm tracking is valuable for understanding the evolution of anvil cirrus because it helps track the development and movement of the parent storm that is the source of anvil cirrus (Menzel, 2001; Jones et al., 2023). This is a relatively simple approach, often relying on a single 2D variable such as brightness temperature or outgoing longwave radiation obtained from geostationary satellites or model output (Fiolleau and Roca, 2013). Storm tracking provides information on the temporal and size evolution of mesoscale convective systems, thick anvil cirrus cloud shields, and microphysical and radiative properties of cirrus clouds (Protopapadaki et al., 2017; Wall et al., 2018; Roca et al., 2017). It can also help explain the response of anvil cirrus properties to interannual climatic variability (Sullivan et al., 2019). However, storm tracking algorithms can only provide information about the developing and mature stages of anvil evolution, as the detection of thinner anvils (below a cloud optical depth of 3) can be biased by signals from lower-lying clouds (Bouniol et al., 2016).
-
Trajectories. Tracking air parcel trajectories can provide valuable information about the processes governing cloud formation, evolution, and decay and can also improve atmospheric models and the interpretation of airborne measurements or remote sensing data (Sullivan et al., 2022). Tracking detrained clouds and water vapor has provided new insights into the life cycle of tropical cirrus, revealing that their lifetimes are longer than expected from ice crystal sedimentation rates (Luo and Rossow, 2004; Mace et al., 2006). Similar methods have been used to study cirrus cloud microphysical origin in both tropical and extratropical regions (Luo and Rossow, 2004; Wernli et al., 2016). Jensen et al. (2018b) used a Lagrangian particle approach to study the evolution of ice crystals transported by subtropical convection, highlighting the importance of sedimentational size sorting and growth by deposition. Gasparini et al. (2021) used offline trajectories coupled to high-resolution GCM data to study changes in anvil evolution between the present and a warmer climate. Meanwhile, a study using trajectories coupled with a CRM and an offline microphysical scheme highlighted the large uncertainties associated with the description of cirrus microphysical processes (Sullivan et al., 2022). Innovative uses of trajectories coupled with simple microphysical modeling can also significantly enhance the value of airborne as well as satellite aerosol and cloud measurements, providing important insights into the sources of in situ cirrus clouds, such as dust INPs (Froyd et al., 2022).
-
Passive tracers. While storm tracking and trajectory analysis offer many benefits, they can be challenging to implement. Passive tracers offer an alternative approach, often used in the analysis of stratospheric circulation (Waugh and Hall, 2002) or as a transport diagnostic for climate models (Krol et al., 2018). Though they cannot track individual cloud parcels and can only be used in a model framework, tracers can provide valuable aggregate information about cloud evolution and microphysics. Because atmospheric models generally allow for easy implementation of tracers, they come with a modest computational cost. In previous studies, cloudy updraft tracers were used to investigate changes in anvil temperature in different climate states (Hartmann et al., 2019) and to study the impact of insolation on cloud lifetime (Gasparini et al., 2022). Tracers can also be adapted to track the time after the onset of a particular microphysical process, such as ice nucleation, making them useful for studying ice crystal evolution pathways. The relative amount of heavier deuterium compared to hydrogen and oxygen-18 compared to oxygen-16 in water is strongly influenced by phase transitions. These ratios can be measured in the atmosphere by both in situ and remote sensing data (Galewsky et al., 2016, and references therein). Stable water isotopes can therefore be used as real-world tracers of the atmospheric water cycle and ice evolution pathways as they contain information on air parcel evaporation and sublimation as well as condensation and deposition history. Isotopes proved particularly useful in studies of the role of deep convective transport in the formation of tropical cirrus of both convective and in situ origin (Blossey et al., 2010; de Vries et al., 2022).
We believe that more frequent use of the evolution perspective in both model studies and satellite or in situ observations coupled to reanalysis data is a way to increase knowledge of cirrus cloud processes, especially in the coming era of high-resolution cloud-resolving model simulations.
6.4 Airborne and satellite observations
Several aircraft campaigns have recently collected data on tropical cirrus clouds, allowing direct measurements of ice crystal properties (Sect. 2.2). However, most of these measurements have been taken in cirrus clouds located away from convective sources, providing limited information about the microphysics within deep convection or freshly formed anvil cirrus. To better understand how ice crystal properties evolve in aging anvil cirrus, it is crucial to conduct measurements at various stages of their life cycle, including the tops of deep convective systems.
Moreover, aircraft field campaign data are key to reducing the large uncertainties that remain regarding the role of heterogeneous nucleation in cirrus clouds and the associated aerosol cirrus indirect effects (Sect. 5.1). Despite extensive laboratory studies, relatively few direct measurements of INPs have been made in the upper troposphere. We thus believe INP measurements at temperatures colder than −35 ∘C are urgently needed to address the important open questions about the roles of heterogeneous ice nuclei in both TTL cirrus and evolving anvil cirrus.
Satellite observations are valuable for studying tropical cirrus clouds and providing context for in situ measurements. However, there is a need for further progress in developing satellite products that directly contribute to understanding cirrus formation processes, such as ice crystal number concentration. While such products have recently emerged (Sect. 2.1), they still show several biases that require attention and resolution (Sourdeval et al., 2018; Krämer et al., 2020). It is crucial to improve the representation of particle size distribution (PSD) in satellite remote sensing algorithms, especially for small particles, based on recent in situ observations (Bartolomé García et al., 2023). Additionally, comparing and evaluating satellite observations with in situ measurements, either directly or in relation to environmental metrics (e.g., the temperature), are essential. Moreover, long-term unified datasets are a key to detecting trends in cloud properties. While the first evidence of such trends has been detected, the recent decommissioning of CALIOP creates a gap in thin cloud measurements that may complicate the creation of long-term datasets with future active instruments (e.g., on board the EarthCARE satellite mission, Illingworth et al., 2015).
Comparing satellite products with models can be challenging due to inconsistencies in physical assumptions between satellite algorithms and models. In particular, differences in assumptions regarding PSDs or the mass–diameter relationship can lead to important differences that should be characterized. Transparent reporting of these assumptions or using different sets of assumptions to provide an ensemble of retrievals can address this issue. Developing adequate satellite simulators will facilitate efficient comparisons between space-based retrievals and modeling results.
Geostationary radiometers provide valuable temporal resolution that low-Earth orbiting satellites lack but have limited capabilities for cirrus cloud retrievals. However, recent advancements in neural network approaches applied to geostationary observations and learning from active sensors (e.g., Strandgren et al., 2017) show promise and should be explored further.
Dynamical parameters lack observational constraints from satellites, such as vertical velocities, which is crucial for understanding cirrus formation and accurately estimating convective mass flux. This is particularly relevant for thick anvil cirrus and can help clarify their relationship with convective intensity, precipitation, and circulation as well as expected changes in their optical depth in a warmer world. Fortunately, three planned satellite missions will address this gap by combining satellite-derived microphysical parameters with coincident vertical velocities. The Investigation of Convective Updrafts (INCUS; van den Heever et al., 2022) mission will infer convective mass fluxes using microwave radiometers and radars flying in close proximity, while the Earth Clouds, Aerosols and Radiation Explorer (EarthCARE; Illingworth et al., 2015) and the Atmosphere Observing System (AOS; Braun et al., 2022) missions will both use Doppler radar to derive the vertical velocity of cloud particles.
Other future missions have great potential to significantly improve our understanding of cirrus clouds and drive new product developments. For example, sub-millimeter passive microwave scanning can give independent information on ice mass, particle radius, and atmospheric thermodynamic variables, particularly when combined with lidar remote sensing of cloud vertical structure (Jiang et al., 2019). High-resolution lidars will enable more accurate and sensitive cirrus retrievals by directly measuring the lidar ratio (defined as the ratio of extinction to backscatter coefficients) rather than assuming a value. Polarized multi-angle radiometers, like the EPS-SG Multi-Viewing Multi-Channel Multi-Polarization Imaging (3MI; Fougnie et al., 2018) instrument, can provide valuable insights into phase detection and characterization of ice crystal habits. Overall, synergistic approaches are encouraged to ensure consistency and improve our representation and understanding of cirrus radiative effects.
In addition, microwave radiances sensitive to cloud ice are becoming important for weather forecasting, with a potential similar to that of microwave temperature soundings (Geer et al., 2017). The Ice Cloud Imager (Eriksson et al., 2020) aboard MetOP-SG will be the first sensor dedicated to ice observations aboard an operational satellite, offering semi-global coverage on a daily basis. The climate and operational weather forecasting communities are increasingly interested in similar observations, as illustrated by recent investigation into the added value of assimilating lidar and radar observations (Janisková, 2015), which could create synergies and lead to advances in the understanding of tropical cirrus.
6.5 Taking advantage of big data and modern computing
Computing power doubles about once every 1.2 years, and NASA alone produces 4 TB of new remotely sensed Earth science data per day (Porterfield, 2021). This outflux of data represents both an opportunity and a challenge. The ease of generating more data is tempting relative to the difficulty of carefully analyzing existing data. We may also sacrifice interpretability at the expense of comprehensiveness (e.g., Beucler et al., 2021; Proske et al., 2022).
We propose a few strategies to make progress on tropical cirrus in harmony with big data. First, machine learning (ML) has become pervasive as a tool to extract patterns from large quantities of data. The number of clouds and climate research articles mentioning ML has exploded from almost none in 2012 to 200 publications throughout 2019 (Sullivan and Hoose, 2022). Cirrus clouds emerge from certain ML-based cloud classifications (Kurihana et al., 2022; Zantedeschi et al., 2022). Artificial neural networks have been used for cloud-top height and ice water path retrievals (Kox et al., 2014; Strandgren et al., 2017; Amell et al., 2022) and to derive a 3D perspective on tropical cloud systems (Stubenrauch et al., 2023), while the explainable ML technique was used for the analysis of retrieved cirrus properties related to meteorological and aerosol conditions (Jeggle et al., 2023). But much room remains to explore ML for tropical cirrus, for example in satellite image analysis of anvil optical depth over time.
Standardization of datasets and metrics provides a second opportunity for progress. As an example, the MCS tracking datasets discussed in Sect. 2.1 often employ different thresholds or variables to identify convective systems over time, complicating any intercomparisons. A contrasting example of successful intercomparison comes from Krämer et al. (2016) and Krämer et al. (2020) in which ice water content-temperature spaces were constructed to aggregate in situ data from various field campaigns and identify cirrus origin. Such phase spaces or consistent metrics define a common language for the research community. The outgoing longwave radiation–albedo space, originally proposed by Hartmann and Short (1980), is another example, used most recently by Turbeville et al. (2022) to compare GSRM representations of tropical cirrus radiative properties. A final example is the use of distributions of cloud-controlling factors (CCFs), or environmental conditions promoting cloudiness. CCFs were initially suggested as a means of understanding the relative influence of flow and aerosol on low clouds and have been primarily used to understand low-cloud feedbacks with warming (Stevens and Brenguier, 2009; Klein et al., 2017). Decompositions of tropical cirrus properties with respect to such CCFs could provide further insight into what drives the life cycle or why results on anvil coverage differ.
A third strategy is improved communication of results. Computational power has been used disproportionately for running high-resolution model simulations and could instead be directed toward sophisticated visualization or videos that provide a more intuitive grasp of output. Innovations in graphics “allow the emergence of a richer relationship between the visualization and the reader, who is now less of a spectator and more of an explorer” (, ). Renderings from groups like the NCAR VisLab and the Climate Visualization Laboratory of the University of Hamburg illustrate how powerful these tools can be to grasp information in large datasets.
A separate modeling-specific consideration for tropical cirrus is our approach to complexity. Increasing computational power facilitates models of increasing sophistication. Simpler, less expensive models will not become obsolete but can instead complement the more sophisticated models. This notion has been termed the model hierarchy, the utility and dangers of which have been discussed by Held (2005), Jeevanjee et al. (2017), and Maher et al. (2019).
What could such a model hierarchy look like for tropical cirrus? We suggest that a useful three-rung hierarchy consists of (1) limited-area or long-channel radiative–convective equilibrium simulations, (2) Mock–Walker simulations with a prescribed SST gradient and TTL ascent rate, and (3) GSRMs (if possible coupled with an interactive ocean). The more idealized models with no or prescribed circulation are probably sufficient to estimate the total water budget from various cloud microphysics schemes, whereas full-complexity models are needed to assess anvil feedbacks. As outlined in Jeevanjee et al. (2017), an array of models is key to determining the robustness of our results: we can turn processes on or off in mechanism denial experiments and test the sensitivity of our results to domain size, boundary conditions, and physical assumptions and parameters.
6.6 A holistic approach
Big data and the model hierarchy both feed into a third question: given these powerful tools, should our dominant approach be to seek more detailed understanding at a process level and at the smallest scales? Indeed, as we have argued in Sect. 6.1, the balance of ice microphysical sources and sinks is often overlooked in understanding model biases in cirrus cloud representation. However, given the range of scales involved in tropical cirrus, we argue that it would be a mistake to overly zoom in with our new tools. More sophisticated ice microphysics schemes do not always have an influence on globally averaged radiation and precipitation fluxes (Proske et al., 2022), and the issue of equifinality implies that the more degrees of freedom our model has, the likelier we are to get a reasonable output for the wrong reason (Mülmenstädt et al., 2020).
We advocate instead for three other strategies that we call cross-tool synthesis, cross-scale studies, and cross-discipline efforts. Cross-tool synthesis refers to combinations of or comparisons across the tools outlined in Sect. 2. Satellite simulators allow like-to-like comparison of remote sensing data and model output. Microphysical outputs could especially stand to gain in accuracy from these, for example in assessing simulated ice crystal numbers relative to DARDAR. Fusion remote sensing products, like CCCM, help circumvent issues of spatiotemporal insufficiency or offset. And sampling of GSRM output in a subdomain around the flight track facilitates more meaningful comparison to in situ data (e.g., Kretzschmar et al., 2020).
Cross-scale studies emphasize the need to link work on micro-scale processes to macroscale phenomena and vice versa. Those in the microphysics community have not always prioritized putting their work into a broader context such that microphysics can be perceived by other scientific communities as in the weeds. There is, however, evidence that uncertain description of microphysics is decisive for large-scale factors, including cloud radiative heating rates and equilibrium climate sensitivity (e.g., Zhao et al., 2016; Hu et al., 2021b; Sullivan and Voigt, 2021). Propagating ice microphysical uncertainties to macroscale ones should be a focus to motivate further work on small-scale processes and parameters. Likewise, anvil feedback studies could benefit from consideration of microphysics. How might ice microphysical sources and sinks modulate the anvil cirrus cloud amount, stability iris, or fixed-anvil-temperature feedbacks? The lack of consensus in RCEMIP simulations on the ACA feedback strength, as well as theoretical work suggesting lack of robustness in the stability iris hypothesis, highlights a role for small-scale processes in constraining these climate feedbacks (Wing et al., 2020; Jeevanjee, 2022).
Following on more studies across scales, cross-discipline efforts again acknowledge the importance of better communicating our results – from those more observationally focused to those more theoretically focused and from those working at process scales to those working at climate scales. Dialogue across these groups is key at a time when our models and observations are more powerful than ever, and several initiatives have already tried to bridge the disciplinary divide (e.g., the PIRE project, https://www.pire-cirrus.org, last access: 14 December 2023; projects initiated by the GEWEX Global Atmospheric System Studies Panel, such as UTCC PROES https://www.gewex.org/UTCC-PROES/, last access: 14 December 2023). We look forward to advances on tropical cirrus over the next years, fueled by big data, the model hierarchy, improved communication, and an urgent need for understanding of cirrus cloud–climate interactions.
The plotting scripts and processed data used to produce Fig. 1 are accessible at https://github.com/adambsokol/gasparini_et_al_2023 (Sokol, 2023).
BG initiated and coordinated the writing of this paper and wrote part of the paper. SCS, ABS, BK, EJ, and DLH all contributed to the writing and editing of the paper.
The contact author has declared that none of the authors has any competing interests.
Publisher's note: Copernicus Publications remains neutral with regard to jurisdictional claims made in the text, published maps, institutional affiliations, or any other geographical representation in this paper. While Copernicus Publications makes every effort to include appropriate place names, the final responsibility lies with the authors.
This article is part of the special issue “20 years of Atmospheric Chemistry and Physics”. It is not associated with a conference.
The PIRE project (NSF grant OISE-1743753) stimulated a lot of discussions on the content summarized in this opinion paper. We appreciate the insightful reviews by Maximilien Bolot and Aurélien Podglajen and the comment by Claudia Stubenrauch. We thank Odran Sourdeval, Fabian Mahrt, Brett McKim, and Maximilien Bolot for their comments on early versions of the paper.
This research has been supported by the European Union's Horizon 2020 research and innovation program under a Marie Skłodowska-Curie grant (agreement no. 101025473 to Blaž Gasparini), the University of Arizona start-up grant (Sylvia C. Sullivan), Division of Atmospheric and Geospace Sciences grant no. 2124496 (Dennis L. Hartmann and Adam B. Sokol), and the Division of Earth Sciences (FINESST grant no. 80NSSC20K1613 to Adam B. Sokol).
This paper was edited by Martina Krämer and Timothy Garrett and reviewed by Maximilien Bolot and Aurélien Podglajen.
Ackerman, T. P., Liou, K.-N., Valero, P. J. F., and Pfister, L.: Heating Rates in Tropical Anvils, J. Atmos. Sci., 45, 1606–1623, https://doi.org/10.1175/1520-0469(1988)045<1606:HRITA>2.0.CO;2, 1988. a, b, c
Albern, N., Voigt, A., Buehler, S. A., and Grützun, V.: Robust and Nonrobust Impacts of Atmospheric Cloud-Radiative Interactions on the Tropical Circulation and Its Response to Surface Warming, Geophys. Res. Lett., 45, 8577–8585, https://doi.org/10.1029/2018GL079599, 2018. a, b
Alexander, M. J. and Pfister, L.: Gravity wave momentum flux in the lower stratosphere over convection, Geophys. Res. Lett., 22, 2029–2032, https://doi.org/10.1029/95GL01984, 1995. a
Amell, A., Eriksson, P., and Pfreundschuh, S.: Ice water path retrievals from Meteosat-9 using quantile regression neural networks, Atmos. Meas. Tech., 15, 5701–5717, https://doi.org/10.5194/amt-15-5701-2022, 2022. a
Atkinson, J. D., Murray, B. J., Woodhouse, M. T., Whale, T. F., Baustian, K. J., Carslaw, K. S., Dobbie, S., O'Sullivan, D., and Malkin, T. L.: The importance of feldspar for ice nucleation by mineral dust in mixed-phase clouds., Nature, 498, 355–358, https://doi.org/10.1038/nature12278, 2013. a
Atlas, R. and Bretherton, C. S.: Aircraft observations of gravity wave activity and turbulence in the tropical tropopause layer: prevalence, influence on cirrus clouds, and comparison with global storm-resolving models, Atmos. Chem. Phys., 23, 4009–4030, https://doi.org/10.5194/acp-23-4009-2023, 2023. a, b, c
Atlas,R., Bretherton, C.S., Sokol., A., Blossey, P., and Khairoutdinov, M.: What are the causes of tropical cirrus longwave biases in global storm resolving simulations?, Earth Space Sci. Open Arch., [preprint], https://doi.org/10.1002/essoar.10511104.1, 13 April 2022. a
Avery, M., Winker, D., Heymsfield, A., Vaughan, M., Young, S., Hu, Y., and Trepte, C.: Cloud ice water content retrieved from the CALIOP space-based lidar, Geophys. Res. Lett., 39, 2–7, https://doi.org/10.1029/2011GL050545, 2012. a
Bacer, S., Sullivan, S. C., Sourdeval, O., Tost, H., Lelieveld, J., and Pozzer, A.: Cold cloud microphysical process rates in a global chemistry–climate model, Atmos. Chem. Phys., 21, 1485–1505, https://doi.org/10.5194/acp-21-1485-2021, 2021. a
Barahona, D. and Nenes, A.: Parameterization of cirrus cloud formation in large-scale models: Homogeneous nucleation, J. Geophys. Res.-Atmos., 113, D11211, https://doi.org/10.1029/2007JD009355, 2008. a
Barahona, D. and Nenes, A.: Parameterizing the competition between homogeneous and heterogeneous freezing in cirrus cloud formation – monodisperse ice nuclei, Atmos. Chem. Phys., 9, 369–381, https://doi.org/10.5194/acp-9-369-2009, 2009. a
Barahona, D., Molod, A., and Kalesse, H.: Direct estimation of the global distribution of vertical velocity within cirrus clouds, Sci. Rep., 7, 6840, https://doi.org/10.1038/s41598-017-07038-6, 2017. a
Baran, A. J., Hill, P., Furtado, K., Field, P., and Manners, J.: A Coupled Cloud Physics–radiation Parameterization of the Bulk Optical Properties of Cirrus and Its Impact on the Met Office Unified Model Global Atmosphere 5.0 Configuration, J. Climate, 27, 7725–7752, https://doi.org/10.1175/JCLI-D-13-00700.1, 2014. a
Baran, A. J., Hill, P., Walters, D., Hardiman, S. C., Furtado, K., Field, P. R., and Manners, J.: The Impact of Two Coupled Cirrus Microphysics–Radiation Parameterizations on the Temperature and Specific Humidity Biases in the Tropical Tropopause Layer in a Climate Model, J. Climate, 29, 5299–5316, https://doi.org/10.1175/JCLI-D-15-0821.1, 2016. a
Barber, K. A., Mullendore, G. L., and Alexander, M. J.: Out-of-Cloud Convective Turbulence: Estimation Method and Impacts of Model Resolution, J. Appl. Meteorol. Climatol., 57, 121–136, https://doi.org/10.1175/JAMC-D-17-0174.1, 2018. a
Bartolomé García, I., Sourdeval, O., Spang, R., and Krämer, M.: Technical note: Bimodal Parameterizations of in situ Ice Cloud Particle Size Distributions, EGUsphere [preprint], https://doi.org/10.5194/egusphere-2023-754, 2023. a
Behrangi, A., Kubar, T., and Lambrigtsen, B.: Phenomenological Description of Tropical Clouds Using CloudSat Cloud Classification, Mon. Weather Rev., 140, 3235–3249, https://doi.org/10.1175/MWR-D-11-00247.1, 2012. a
Betts, A. K. and Ridgway, W.: Climatic Equilibrium of the Atmospheric Convective Boundary Layer over a Tropical Ocean, J. Atmos. Sci., 46, 2621–2641, https://doi.org/10.1175/1520-0469(1989)046<2621:CEOTAC>2.0.CO;2, 1989. a
Beucler, T., Ebert-Uphoff, I., Rasp, S., Pritchard, M., and Gentine, P.: Machine Learing for Clouds and Climate, in: Clouds and Their Climatic Impact: Radiation, Circulation, and Precipitation, edited by: Sullivan, S. C. and Hoose, C., Wiley–American Geophysical Union, 327–346, ISBN 978-1-119-70031-9, 2024. a
Beydoun, H., Caldwell, P. M., Hannah, W. M., and Donahue, A. S.: Dissecting Anvil Cloud Response to Sea Surface Warming, Geophys. Res. Lett., 48, e2021GL094049, https://doi.org/10.1029/2021GL094049, 2021. a
Blossey, P. N., Kuang, Z., and Romps, D. M.: Isotopic composition of water in the tropical tropopause layer in cloud-resolving simulations of an idealized tropical circulation, J. Geophys. Res.-Atmos., 115, 1–23, https://doi.org/10.1029/2010JD014554, 2010. a
Boehm, M. T. and Verlinde, J.: Stratospheric influence on upper tropospheric tropical cirrus, Geophys. Res. Lett., 27, 3209–3212, https://doi.org/10.1029/2000GL011678, 2000. a
Bony, S., Stevens, B., Coppin, D., Becker, T., Reed, K. A., Voigt, A., and Medeiros, B.: Thermodynamic control of anvil cloud amount, P. Natl. Acad. Sci. USA, 113, 8927–8932, https://doi.org/10.1073/pnas.1601472113, 2016. a, b
Bony, S., Semie, A., Kramer, R. J., Soden, B., Tompkins, A. M., and Emanuel, K. A.: Observed Modulation of the Tropical Radiation Budget by Deep Convective Organization and Lower-Tropospheric Stability, AGU Advances, 1, e2019AV000155, https://doi.org/10.1029/2019AV000155, 2020. a
Bouniol, D., Roca, R., Fiolleau, T., and Poan, D. E.: Macrophysical, Microphysical, and Radiative Properties of Tropical Mesoscale Convective Systems over Their Life Cycle, J. Climate, 29, 3353–3371, https://doi.org/10.1175/JCLI-D-15-0551.1, 2016. a
Bramberger, M., Alexander, M. J., Davis, S., Podglajen, A., Hertzog, A., Kalnajs, L., Deshler, T., Goetz, J. D., and Khaykin, S.: First Super-Pressure Balloon-Borne Fine-Vertical-Scale Profiles in the Upper TTL: Impacts of Atmospheric Waves on Cirrus Clouds and the QBO, Geophys. Res. Lett., 49, e2021GL097596, https://doi.org/10.1029/2021GL097596, 2022. a
Braun, S. A., Yorks, J., Thorsen, T., Cecil, D., and Kirschbaum, D.: NASA'S Earth System Observatory-Atmosphere Observing System, in: IGARSS 2022 – 2022 IEEE International Geoscience and Remote Sensing Symposium, 7391–7393, https://doi.org/10.1109/igarss46834.2022.9884029, 2022. a
Caldwell, P. M., Terai, C. R., Hillman, B., Keen, N. D., Bogenschutz, P., Lin, W., Beydoun, H., Taylor, M., Bertagna, L., Bradley, A. M., Clevenger, T. C., Donahue, A. S., Eldred, C., Foucar, J., Golaz, J.-C., Guba, O., Jacob, R., Johnson, J., Krishna, J., Liu, W., Pressel, K., Salinger, A. G., Singh, B., Steyer, A., Ullrich, P., Wu, D., Yuan, X., Shpund, J., Ma, H.-Y., and Zender, C. S.: Convection-Permitting Simulations With the E3SM Global Atmosphere Model, J. Adv. Model. Earth Syst., 13, e2021MS002544, https://doi.org/10.1029/2021MS002544, 2021. a
Cesana, G., Waliser, D. E., Henderson, D., L'Ecuyer, T. S., Jiang, X., and Li, J.-L. F.: The Vertical Structure of Radiative Heating Rates: A Multimodel Evaluation Using A-Train Satellite Observations, J. Climate, 32, 1573–1590, https://doi.org/10.1175/JCLI-D-17-0136.1, 2019. a
Chahine, M. T., Pagano, T. S., Aumann, H. H., Atlas, R., Barnet, C., Blaisdell, J., Chen, L., Divakarla, M., Fetzer, E. J., Goldberg, M., Gautier, C., Granger, S., Hannon, S., Irion, F. W., Kakar, R., Kalnay, E., Lambrigtsen, B. H., Lee, S.-Y., Marshall, J. L., McMillan, W. W., McMillin, L., Olsen, E. T., Revercomb, H., Rosenkranz, P., Smith, W. L., Staelin, D., Strow, L. L., Susskind, J., Tobin, D., Wolf, W., and Zhou, L.: AIRS: Improving Weather Forecasting and Providing New Data on Greenhouse Gases, B. Am. Meteorol. Soc., 87, 911–926, https://doi.org/10.1175/BAMS-87-7-911, 2006. a
Chambers, L. H., Lin, B., and Young, D. F.: Examination of New CERES Data for Evidence of Tropical Iris Feedback, J. Climate, 15, 3719–3726, https://doi.org/10.1175/1520-0442(2002)015<3719:EONCDF>2.0.CO;2, 2002. a
Chang, K.-W. and L'Ecuyer, T.: Influence of gravity wave temperature anomalies and their vertical gradients on cirrus clouds in the tropical tropopause layer – a satellite-based view, Atmos. Chem. Phys., 20, 12499–12514, https://doi.org/10.5194/acp-20-12499-2020, 2020. a
Choi, Y.-S., Kim, W., Yeh, S.-W., Masunaga, H., Kwon, M.-J., Jo, H.-S., and Huang, L.: Revisiting the iris effect of tropical cirrus clouds with TRMM and A-Train satellite data, J. Geophys. Res.-Atmos., 122, 5917–5931, https://doi.org/10.1002/2016JD025827, 2017. a, b, c
Cirisan, A., Luo, B. P., Engel, I., Wienhold, F. G., Sprenger, M., Krieger, U. K., Weers, U., Romanens, G., Levrat, G., Jeannet, P., Ruffieux, D., Philipona, R., Calpini, B., Spichtinger, P., and Peter, T.: Balloon-borne match measurements of midlatitude cirrus clouds, Atmos. Chem. Phys., 14, 7341–7365, https://doi.org/10.5194/acp-14-7341-2014, 2014. a
Connolly, P. J., Emersic, C., and Field, P. R.: A laboratory investigation into the aggregation efficiency of small ice crystals, Atmos. Chem. Phys., 12, 2055–2076, https://doi.org/10.5194/acp-12-2055-2012, 2012. a
Corcos, M., Hertzog, A., Plougonven, R., and Podglajen, A.: Observation of gravity waves at the tropical tropopause using superpressure balloons., J. Geophys. Res., 126, e2021JD035165, https://doi.org/10.1029/2021JD035165, 2021. a, b
Corcos, M., Hertzog, A., Plougonven, R., and Podglajen, A.: A simple model to assess the impact of gravity waves on ice-crystal populations in the tropical tropopause layer, Atmos. Chem. Phys., 23, 6923–6939, https://doi.org/10.5194/acp-23-6923-2023, 2023. a
David, R. O., Marcolli, C., Fahrni, J., Qiu, Y., Sirkin, Y. A. P., Molinero, V., Mahrt, F., Brühwiler, D., Lohmann, U., and Kanji, Z. A.: Pore condensation and freezing is responsible for ice formation below water saturation for porous particles, P. Natl. Acad. Sci. USA, 116, 8184–8189, https://doi.org/10.1073/pnas.1813647116, 2019. a
Davis, S. M., Hlavka, D., Jensen, E., Rosenlof, K., Yang, Q., Schmidt, S., Borrmann, S., Frey, W., Lawson, P., Vömel, H., and Bui, T.-P.: In situ and lidar observations of tropopause subvisible cirrus clouds during TC4, J. Geophys. Res., 115, D00J17, https://doi.org/10.1029/2009JD013093, 2010. a
Davis, S. M., Liang, C. K., and Rosenlof, K.: Interannual variability of tropical tropopause layer clouds, Geophys. Res. Lett., 40, 2862–2866, https://doi.org/10.1002/grl.50512, 2013. a
Delanoë, J. and Hogan, R. J.: Combined CloudSat-CALIPSO-MODIS retrievals of the properties of ice clouds, J. Geophys. Res., 115, 1–17, https://doi.org/10.1029/2009JD012346, 2010. a
Delanoë, J. M. and Hogan, R. J.: A variational scheme for retrieving ice cloud properties from combined radar, lidar, and infrared radiometer, J. Geophys. Res.-Atmos., 113, 1–21, https://doi.org/10.1029/2007JD009000, 2008. a
DeMott, P. J., Cziczo, D. J., Prenni, A. J., Murphy, D. M., Kreidenweis, S. M., Thomson, D. S., Borys, R., and Rogers, D. C.: Measurements of the concentration and composition of nuclei for cirrus formation, P. Natl. Acad. Sci. USA, 100, 14655–14660, https://doi.org/10.1073/pnas.2532677100, 2003. a
Deng, M. and Mace, G. G.: Cirrus microphysical properties and air motion statistics using cloud radar doppler moments. Part II: Climatology, J. Appl. Meteorol. Climatol., 47, 3221–3235, https://doi.org/10.1175/2008JAMC1949.1, 2008. a
Deng, M., Mace, G. G., Wang, Z., and Okamoto, H.: Tropical composition, cloud and climate coupling experiment validation for cirrus cloud profiling retrieval using cloudsat radar and CALIPSO lidar, J. Geophys. Res.-Atmos., 115, 1–18, https://doi.org/10.1029/2009JD013104, 2010. a
de Vries, A. J., Aemisegger, F., Pfahl, S., and Wernli, H.: Stable water isotope signals in tropical ice clouds in the West African monsoon simulated with a regional convection-permitting model, Atmos. Chem. Phys., 22, 8863–8895, https://doi.org/10.5194/acp-22-8863-2022, 2022. a
Dietlicher, R., Neubauer, D., and Lohmann, U.: Elucidating ice formation pathways in the aerosol–climate model ECHAM6-HAM2, Atmos. Chem. Phys., 19, 9061–9080, https://doi.org/10.5194/acp-19-9061-2019, 2019. a
Dinh, T., Podglajen, A., Hertzog, A., Legras, B., and Plougonven, R.: Effect of gravity wave temperature fluctuations on homogeneous ice nucleation in the tropical tropopause layer, Atmos. Chem. Phys., 16, 35–46, https://doi.org/10.5194/acp-16-35-2016, 2016. a
Dinh, T., Gasparini, B., and Bellon, G.: Clouds and radiatively induced circulations, in: Clouds and Their Climatic Impact: Radiation, Circulation, and Precipitation, edited by: Sullivan, S. C. and Hoose, C., Wiley–American Geophysical Union, 239–254, ISBN 978-1-119-70031-9, 2024. a, b
Dinh, T. P., Durran, D. R., and Ackerman, T. P.: Maintenance of tropical tropopause layer cirrus, J. Geophys. Res.-Atmos., 115, 1–15, https://doi.org/10.1029/2009JD012735, 2010. a, b, c
Dobbie, S. and Jonas, P.: Radiative influences on the structure and lifetime of cirrus clouds, Q. J. Roy. Meteorol. Soc., 127, 2663–2682, https://doi.org/10.1002/qj.49712757808, 2001. a
Donner, L. J., O'Brien, T. A., Rieger, D., Vogel, B., and Cooke, W. F.: Are atmospheric updrafts a key to unlocking climate forcing and sensitivity?, Atmos. Chem. Phys., 16, 12983–12992, https://doi.org/10.5194/acp-16-12983-2016, 2016. a
Durran, D. R., Dinh, T., Ammerman, M., and Ackerman, T.: The Mesoscale Dynamics of Thin Tropical Tropopause Cirrus, J. Atmos. Sci., 66, 2859–2873, https://doi.org/10.1175/2009jas3046.1, 2009. a
Hawker, R. E., Miltenberger, A. K., Wilkinson, J. M., Hill, A. A., Shipway, B. J., Cui, Z., Cotton, R. J., Carslaw, K. S., Field, P. R., and Murray, B. J.: The temperature dependence of ice-nucleating particle concentrations affects the radiative properties of tropical convective cloud systems, Atmos. Chem. Phys., 21, 5439–5461, https://doi.org/10.5194/acp-21-5439-2021, 2021. a
Eriksson, P., Rydberg, B., Mattioli, V., Thoss, A., Accadia, C., Klein, U., and Buehler, S. A.: Towards an operational Ice Cloud Imager (ICI) retrieval product, Atmos. Meas. Tech., 13, 53–71, https://doi.org/10.5194/amt-13-53-2020, 2020. a
Feng, Z., Dong, X., Xi, B., Schumacher, C., Minnis, P., and Khaiyer, M.: Top-of-atmosphere radiation budget of convective core/stratiform rain and anvil clouds from deep convective systems, J. Geophys. Res., D23202,, https://doi.org/10.1029/2011JD016451, 2011. a
Feng, Z., Leung, L. R., Liu, N., Wang, J., Houze Jr, R. A., Li, J., Hardin, J. C., Chen, D., and Guo, J.: A Global High-Resolution Mesoscale Convective System Database Using Satellite-Derived Cloud Tops, Surface Precipitation, and Tracking, J. Geophys. Res.-Atmos., 126, e2020JD034202, https://doi.org/10.1029/2020JD034202, 2021. a
Feng, Z., Leung, L. R., Hardin, J., Terai, C. R., Song, F., and Caldwell, P.: Mesoscale Convective Systems in DYAMOND Global Convection-Permitting Simulations, Geophys. Res. Lett., 50, e2022GL102603, https://doi.org/10.1029/2022GL102603, 2023. a, b
Ferlay, N., Garrett, T. J., and Minvielle, F.: Satellite Observations of an Unusual Cloud Formation near the Tropopause, J. Atmos. Sci., 71, 3801–3815, https://doi.org/10.1175/jas-d-13-0361.1, 2014. a
Fiolleau, T. and Roca, R.: An algorithm for the detection and tracking of tropical mesoscale convective systems using infrared images from geostationary satellite, IEEE Trans. Geosci. Remote Sens., 51, 4302–4315, https://doi.org/10.1109/TGRS.2012.2227762, 2013. a, b, c
Forster, P. M. F. and Shine, K. P.: Assessing the climate impact of trends in stratospheric water vapor, Geophys. Res. Lett., 29, 10–1/4, https://doi.org/10.1029/2001GL013909, 2002. a
Fougnie, B., Marbach, T., Lacan, A., Lang, R., Schlüssel, P., Poli, G., Munro, R., and Couto, A. B.: The Multi-Viewing Multi-Channel Multi-Polarisation Imager – Overview of the 3mi Polarimetric Mission for Aerosol and Cloud Characterization, J. Quant. Spectrosc. Ra., 219, 23–32, https://doi.org/10.1016/j.jqsrt.2018.07.008, 2018. a
Frey, W., Borrmann, S., Kunkel, D., Weigel, R., de Reus, M., Schlager, H., Roiger, A., Voigt, C., Hoor, P., Curtius, J., Krämer, M., Schiller, C., Volk, C. M., Homan, C. D., Fierli, F., Di Donfrancesco, G., Ulanovsky, A., Ravegnani, F., Sitnikov, N. M., Viciani, S., D'Amato, F., Shur, G. N., Belyaev, G. V., Law, K. S., and Cairo, F.: In situ measurements of tropical cloud properties in the West African Monsoon: upper tropospheric ice clouds, Mesoscale Convective System outflow, and subvisual cirrus, Atmos. Chem. Phys., 11, 5569–5590, https://doi.org/10.5194/acp-11-5569-2011, 2011. a
Froyd, K. D., Yu, P., Schill, G. P., Brock, C. A., Kupc, A., Williamson, C. J., Jensen, E. J., Ray, E., Rosenlof, K. H., Bian, H., Darmenov, A. S., Colarco, P. R., Diskin, G. S., Bui, T. P., and Murphy, D. M.: Dominant role of mineral dust in cirrus cloud formation revealed by global-scale measurements, Nat. Geosci., 15, 177–183, https://doi.org/10.1038/s41561-022-00901-w, 2022. a, b, c
Fu, Q.: Bottom up in the tropics, Nat. Clim. Change, 3, 957–958, https://doi.org/10.1038/nclimate2039, 2013. a
Fu, Q., Baker, M., and Hartmann, D. L.: Tropical cirrus and water vapor: an effective Earth infrared iris feedback?, Atmos. Chem. Phys., 2, 31–37, https://doi.org/10.5194/acp-2-31-2002, 2002. a
Fu, Q., Smith, M., and Yang, Q.: The impact of cloud radiative effects on the tropical tropopause layer temperatures, Atmosphere, 9, 1–13, https://doi.org/10.3390/atmos9100377, 2018. a, b
Fueglistaler, S., Dessler, A. E., Dunkerton, T. J., Folkins, I., Fu, Q., and Mote, P. W.: Tropical tropopause layer, Rev. Geophys., 47, 1–31, https://doi.org/10.1029/2008RG000267, 2009. a
Galewsky, J., Steen-Larsen, H. C., Field, R. D., Worden, J., Risi, C., and Schneider, M.: Stable isotopes in atmospheric water vapor and applications to the hydrologic cycle, Rev. Geophys., 54, 809–865, https://doi.org/10.1002/2015RG000512, 2016. a
Garrett, T. J., Heymsfield, A. J., McGill, M. J., Ridley, B. A., Baumgardner, D. G., Bui, T. P., and Webster, C. R.: Convective generation of cirrus near the tropopause, J. Geophys. Res.-Atmos., 109, D21203, https://doi.org/10.1029/2004JD004952, 2004. a
Garrett, T. J., Navarro, B. C., Twohy, C. H., Jensen, E. J., Baumgardner, D. G., Bui, P. T., Gerber, H., Herman, R. L., Heymsfield, A. J., Lawson, P., Minnis, P., Nguyen, L., Poellot, M., Pope, S. K., Valero, F. P., and Weinstock, E. M.: Evolution of a Florida cirrus anvil, J. Atmos. Sci., 62, 2352–2372, https://doi.org/10.1175/JAS3495.1, 2005. a
Gasparini, B., Blossey, P. N., Hartmann, D. L., Lin, G., and Fan, J.: What Drives the Life Cycle of Tropical Anvil Clouds?, J. Adv. Model. Earth Syst., 11, 2586–2605, https://doi.org/10.1029/2019MS001736, 2019. a, b, c, d, e, f
Gasparini, B., Rasch, P. J., Hartmann, D. L., Wall, C. J., and Dütsch, M.: A Lagrangian perspective on tropical anvil cloud lifecycle in present and future climate, J. Geophys. Res.-Atmos., 126, 1–26, https://doi.org/10.1029/2020jd033487, 2021. a, b, c
Gasparini, B., Sokol, A. B., Wall, C. J., Hartmann, D. L., and Blossey, P. N.: Diurnal Differences in Tropical Maritime Anvil Cloud Evolution, J. Climate, 35, 1655–1677, https://doi.org/10.1175/jcli-d-21-0211.1, 2022. a, b, c, d
Geer, A. J., Baordo, F., Bormann, N., Chambon, P., English, S. J., Kazumori, M., Lawrence, H., Lean, P., Lonitz, K., and Lupu, C.: The growing impact of satellite observations sensitive to humidity, cloud and precipitation, Q. J. Roy. Meteorol. Soc., 143, 3189–3206, https://doi.org/10.1002/qj.3172, 2017. a
Gong, J., Zeng, X., Wu, D. L., and Li, X.: Diurnal Variation of Tropical Ice Cloud Microphysics: Evidence from Global Precipitation Measurement Microwave Imager Polarimetric Measurements, J. Geophys. Res., 45, 1185–1193, 2017. a
Grabowski, W. W., Morrison, H., Shima, S.-I., Abade, G. C., Dziekan, P., and Pawlowska, H.: Modeling of Cloud Microphysics: Can We Do Better?, B. Am. Meteorol. Soc., 100, 655–672, https://doi.org/10.1175/bams-d-18-0005.1, 2019. a
Gryspeerdt, E., Sourdeval, O., Quaas, J., Delanoë, J., Krämer, M., and Kühne, P.: Ice crystal number concentration estimates from lidar–radar satellite remote sensing – Part 2: Controls on the ice crystal number concentration, Atmos. Chem. Phys., 18, 14351–14370, https://doi.org/10.5194/acp-18-14351-2018, 2018. a, b
Haase, J. S., Alexander, M. J., Hertzog, A., Kalnajs, L., Deshler, T., Davis, S. M., Plougonven, R., Cocquerez, P., , and Venel, S.: Around the world in 84 days, Eos, Transactions American Geophysical Union, https://doi.org/10.1029/2018EO091907, 2018. a
Haladay, T. and Stephens, G.: Characteristics of tropical thin cirrus clouds deduced from joint CloudSat and CALIPSO observations, J. Geophys. Res., 114, 1–13, https://doi.org/10.1029/2008JD010675, 2009. a, b, c
Hang, Y., L’Ecuyer, T. S., Henderson, D. S., Matus, A. V., and Wang, Z.: Reassessing the Effect of Cloud Type on Earth’s Energy Balance in the Age of Active Spaceborne Observations. Part II: Atmospheric Heating, J. Climate, 32, 6219–6236, https://doi.org/10.1175/JCLI-D-18-0754.1, 2019. a
Harrop, B. E. and Hartmann, D. L.: Testing the Role of Radiation in Determining Tropical Cloud-Top Temperature, J. Climate, 25, 5731–5747, https://doi.org/10.1175/jcli-d-11-00445.1, 2012. a, b
Harrop, B. E. and Hartmann, D. L.: The Role of Cloud Radiative Heating in Determining the Location of the ITCZ in Aquaplanet Simulations, J. Climate, 29, 2741–2763, https://doi.org/10.1175/JCLI-D-15-0521.1, 2016. a
Hartmann, D., Dygert, B. D., Blossey, P. N., Fu, Q., and Sokol, A. B.: The Vertical Profile of Radiative Cooling and Lapse Rate in aWarming Climate, J. Climate, 35, 2653–2665, https://doi.org/10.1175/JCLI-D-21-0861.1, 2022. a
Hartmann, D. L. and Larson, K.: An important constraint on tropical cloud-climate feedback, Geophys. Res Lett., 29, 1951–1954, https://doi.org/10.1029/2002GL015835, 2002. a
Hartmann, D. L. and Michelsen, M. L.: No evidence for Iris, B. Am. Meteorol. Soc., 83, 249–254, https://doi.org/10.1175/1520-0477(2002)083<0249:NEFI>2.3.CO;2, 2002. a
Hartmann, D. L. and Short, D. A.: On the Use of Earth Radiation Budget Statistics for Studies of Clouds and Climate, J. Atmos. Sci., 6, 1233–1250, 1980. a
Hartmann, D. L., Moy, L. A., and Fu, Q.: Tropical convection and the energy balance at the top of the atmosphere, J. Climate, 14, 4495–4511, https://doi.org/10.1175/1520-0442(2001)014<4495:TCATEB>2.0.CO;2, 2001. a
Hartmann, D. L., Gasparini, B., Berry, S. E., and Blossey, P. N.: The Life Cycle and Net Radiative Effect of Tropical Anvil Clouds, J. Adv. Model. Earth Syst., 10, 3012–3029, https://doi.org/10.1029/2018MS001484, 2018. a, b, c, d, e
Hartmann, D. L., Blossey, P. N., and Dygert, B. D.: Convection and Climate: What Have We Learned from Simple Models and Simplified Settings?, Current Clim. Change Rep., 5, 196–206, https://doi.org/10.1007/s40641-019-00136-9, 2019. a, b
Heikenfeld, M., White, B., Labbouz, L., and Stier, P.: Aerosol effects on deep convection: the propagation of aerosol perturbations through convective cloud microphysics, Atmos. Chem. Phys., 19, 2601–2627, https://doi.org/10.5194/acp-19-2601-2019, 2019. a
Held, I. M.: The Gap between Simulation and Understanding in Climate Modeling, B. Am. Meteorol. Soc., 86, 1609–1614, https://doi.org/10.1175/BAMS-86-11-1609, 2005. a
Held, I. M. and Soden, B. J.: Robust Responses of the Hydrological Cycle to Global Warming, J. Climate, 19, 5686–5699, https://doi.org/10.1175/JCLI3990.1, 2006. a
Henderson, D. S., L'Ecuyer, T., Stephens, G., Partain, P., and Sekiguchi, M.: A Multisensor Perspective on the Radiative Impacts of Clouds and Aerosols, J. Appl. Meteorol. Climatol., 52, 853–871, https://doi.org/10.1175/JAMC-D-12-025.1, 2013. a
Heymsfield, A. and Willis, P.: Cloud Conditions Favoring Secondary Ice Particle Production in Tropical Maritime Convection, J. Atmos. Sci., 71, 4500 – 4526, https://doi.org/10.1175/JAS-D-14-0093.1, 2014. a
Hidalgo, C. A. and Almossawi, A.: The data-visualization revolution, Sci. Am., Vol. 310, 104–113, March 2014. a
Hilton, F., Armante, R., August, T., Barnet, C., Bouchard, A., Camy-Peyret, C., Capelle, V., Clarisse, L., Clerbaux, C., Coheur, P.-F., Collard, A., Crevoisier, C., Dufour, G., Edwards, D., Faijan, F., Fourrié, N., Gambacorta, A., Goldberg, M., Guidard, V., Hurtmans, D., Illingworth, S., Jacquinet-Husson, N., Kerzenmacher, T., Klaes, D., Lavanant, L., Masiello, G., Matricardi, M., McNally, A., Newman, S., Pavelin, E., Payan, S., Péquignot, E., Peyridieu, S., Phulpin, T., Remedios, J., Schlüssel, P., Serio, C., Strow, L., Stubenrauch, C., Taylor, J., Tobin, D., Wolf, W., and Zhou, D.: Hyperspectral Earth Observation from IASI: Five Years of Accomplishments, B. Am. Meteorol. Soc., 93, 347–370, https://doi.org/10.1175/BAMS-D-11-00027.1, 2012. a
Holloway, C. E. and Woolnough, S. J.: The sensitivity of convective aggregation to diabatic processes in idealized radiative-convective equilibrium simulations, J. Adv. Model. Earth Syst., 8, 166–195, https://doi.org/10.1002/2015MS000511, 2016. a
Holloway, C. E., Wing, A. A., Bony, S., Muller, C., Masunaga, H., L’Ecuyer, T. S., Turner, D. D., and Zuidema, P.: Observing Convective Aggregation, Surv. Geophys., 38, 1199–1236, https://doi.org/10.1007/s10712-017-9419-1, 2017. a
Holton, J. R. and Gettelman, A.: Horizontal transport and the dehydration of the stratosphere, Geophys. Res. Lett., 28, 2799–2802, https://doi.org/10.1029/2001GL013148, 2001. a
Holton, J. R., Haynes, P. H., McIntyre, M. E., Douglass, A. R., Rood, R. B., and Pfister, L.: Stratosphere-troposphere exchange, Rev. Geophys., 33, 403–439, https://doi.org/10.1029/95RG02097, 1995. a
Hong, Y., Liu, G., and Li, J.-L. F.: Assessing the Radiative Effects of Global Ice Clouds Based on CloudSat and CALIPSO Measurements, J. Climate, 29, 7651–7673, https://doi.org/10.1175/JCLI-D-15-0799.1, 2016. a
Hu, Y., McFarquhar, G. M., Wu, W., Huang, Y., Schwarzenboeck, A., Protat, A., Korolev, A., Rauber, R. M., and Wang, H.: Dependence of Ice Microphysical Properties on Environmental Parameters: Results from HAIC-HIWC Cayenne Field Campaign, J. Atmos. Sci., 78, 2957 – 2981, https://doi.org/10.1175/JAS-D-21-0015.1, 2021a. a
Hu, Z., Lamraoui, F., and Kuang, Z.: Influence of Upper-Troposphere Stratification and Cloud–Radiation Interaction on Convective Overshoots in the Tropical Tropopause Layer, Journal of the Atmospheric Sciences, 78, 2493–2509, https://doi.org/10.1175/JAS-D-20-0241.1, 2021b. a
Huang, Y., Wang, Y., and Huang, H.: Stratospheric water vapor feedback disclosed by a locking experiment, Geophys. Res. Lett., 47, e2020GL087987, https://doi.org/10.1029/2020GL087987, 2020. a
Hubbard, K. G.: Parameterization of Depositional Ice Growth, J. Appl. Meteorol. Climatol., 16, 177–182, https://doi.org/10.1175/1520-0450(1977)016<0177:PODIG>2.0.CO;2, 1977. a
Höjgård-Olsen, E., Chepfer, H., and Brogniez, H.: Satellite Observed Sensitivity of Tropical Clouds and Moisture to Sea Surface Temperature on Various Time and Space Scales: 1. Focus on High Level Cloud Situations Over Ocean, J. Geophys. Res.-Atmos., 127, e2021JD035438, https://doi.org/10.1029/2021JD035438, 2022. a, b
Igel, M. R., Drager, A. J., and van den Heever, S. C.: A CloudSat cloud object partitioning technique and assessment and integration of deep convective anvil sensitivities to sea surface temperature, J. Geophys. Res.-Atmos., 119, 10515–10535, https://doi.org/10.1002/2014JD021717, 2014. a
Illingworth, A. J., Barker, H. W., Beljaars, A., Ceccaldi, M., Chepfer, H., Clerbaux, N., Cole, J., Delanoë, J., Domenech, C., Donovan, D. P., Fukuda, S., Hirakata, M., Hogan, R. J., Huenerbein, A., Kollias, P., Kubota, T., Nakajima, T., Nakajima, T. Y., Nishizawa, T., Ohno, Y., Okamoto, H., Oki, R., Sato, K., Satoh, M., Shephard, M. W., Velázquez-Blázquez, A., Wandinger, U., Wehr, T., and van Zadelhoff, G. J.: The EarthCARE Satellite: The Next Step Forward in Global Measurements of Clouds, Aerosols, Precipitation, and Radiation, B. Am. Meteorol. Soc, 96, 1311–1332, https://doi.org/10.1175/BAMS-D-12-00227.1, 2015. a, b
Immler, F., Krüger, K., Fujiwara, M., Verver, G., Rex, M., and Schrems, O.: Correlation between equatorial Kelvin waves and the occurrence of extremely thin ice clouds at the tropical tropopause, Atmos. Chem. Phys., 8, 4019–4026, https://doi.org/10.5194/acp-8-4019-2008, 2008. a
Ito, M. and Masunaga, H.: Process-Level Assessment of the Iris Effect Over Tropical Oceans, Geophys. Res. Lett., 49, e2022GL097997, https://doi.org/10.1029/2022GL097997, 2022. a, b
Janisková, M.: Assimilation of Cloud Information From Space‐borne Radar and Lidar: Experimental Study Using a 1d+4d‐var Technique, Quarterly J. Roy. Meteorol. Soc., 141, 2708–2725, https://doi.org/10.1002/qj.2558, 2015. a
Järvinen, E., Wernli, H., and Schnaiter, M.: Investigations of Mesoscopic Complexity of Small Ice Crystals in Midlatitude Cirrus, Geophys. Res. Lett., 45, 11,465–11,472, https://doi.org/10.1029/2018GL079079, 2018. a
Jeevanjee, N.: Three Rules for the Decrease of Tropical Convection With Global Warming, J. Adv. Model. Earth Syst., 14, e2022MS003285, https://doi.org/10.1029/2022MS003285, 2022. a, b
Jeevanjee, N. and Fueglistaler, S.: Simple Spectral Models for Atmospheric Radiative Cooling, J. Atmos. Sci., 77, 479–497, https://doi.org/10.1175/jas-d-18-0347.1, 2020. a
Jeevanjee, N. and Zhou, L.: On the Resolution-Dependence of Anvil Cloud Fraction and Precipitation Efficiency in Radiative-Convective Equilibrium, J. Adv. Model. Earth Syst., 14, e2021MS002759, https://doi.org/10.1029/2021MS002759, 2022. a, b
Jeevanjee, N., Hassanzadeh, P., Hill, S., and Sheshadri, A.: A perspective on climate model hierarchies, J. Adv. Model. Earth Syst., 9, 1760–1771, https://doi.org/10.1002/2017MS001038, 2017. a, b
Jeggle, K., Neubauer, D., Camps-Valls, G., and Lohmann, U.: Understanding cirrus clouds using explainable machine learning, Environ. Data Sci., 2, e19, https://doi.org/10.1017/eds.2023.14, 2023. a
Jenney, A. M., Randall, D. A., and Branson, M. D.: Understanding the Response of Tropical Ascent to Warming Using an Energy Balance Framework, J. Adv. Model. Earth Syst., 12, e2020MS002056, https://doi.org/10.1029/2020MS002056, 2020. a
Jensen, E. and Pfister, L.: Transport and freeze-drying in the tropical tropopause layer, J. Geophys. Res-.Atmos., 109, https://doi.org/10.1029/2003JD004022, 2004. a
Jensen, E., Starr, D., and Toon, O. B.: Mission investigates tropical cirrus clouds, Eos, Transactions American Geophysical Union, 85, 45–50, https://doi.org/10.1029/2004EO050002, 2004. a
Jensen, E. J., Kinne, S., and Toon, O. B.: Tropical cirrus cloud radiative forcing: Sensitivity studies, Geophys. Res. Lett., 21, 2023–2026, https://doi.org/10.1029/94GL01358, 1994. a
Jensen, E. J., Toon, O. B., Pfister, L., and Selkirk, H. B.: Dehydration of the upper troposphere and lower stratosphere by subvisible cirrus clouds near the tropical tropopause, Geophys. Res. Lett., 23, 825–828, https://doi.org/10.1029/96GL00722, 1996. a
Jensen, E. J., Lawson, P., Baker, B., Pilson, B., Mo, Q., Heymsfield, A. J., Bansemer, A., Bui, T. P., McGill, M., Hlavka, D., Heymsfield, G., Platnick, S., Arnold, G. T., and Tanelli, S.: On the importance of small ice crystals in tropical anvil cirrus, Atmos. Chem. Phys., 9, 5519–5537, https://doi.org/10.5194/acp-9-5519-2009, 2009. a, b
Jensen, E. J., Pfister, L., and Toon, O. B.: Impact of radiative heating, wind shear, temperature variability, and microphysical processes on the structure and evolution of thin cirrus in the tropical tropopause layer, J. Geophys. Res.-Atmos., 116, D12209, https://doi.org/10.1029/2010JD015417, 2011. a
Jensen, E. J., Diskin, G., Lawson, R. P., Lance, S., Bui, T. P., Hlavka, D., McGill, M., Pfister, L., Toon, O. B., and Gao, R.-S.: Ice nucleation and dehydration in the Tropical Tropopause Layer, P. Nat. Acad. Sci. USA, 110, 2041–2046, https://doi.org/10.1073/pnas.1217104110, 2013. a
Jensen, E. J., Ueyama, R., Pfister, L., Bui, T. V., Alexander, M. J., Podglajen, A., Hertzog, A., Woods, S., Lawson, R. P., Kim, J.-E., and Schoeberl, M. R.: High-frequency gravity waves and homogeneous ice nucleation in tropical tropopause layer cirrus, Geophys. Res. Lett., 43, 6629–6635, https://doi.org/10.1002/2016GL069426, 2016. a
Jensen, E. J., Pfister, L., Jordan, D. E., Bui, T. V., Ueyama, R., Singh, H. B., Thornberry, T. D., Rollins, A. W., Gao, R.-S., Fahey, D. W., Rosenlof, K. H., Elkins, J. W., Diskin, G. S., DiGangi, J. P., Lawson, R. P., Woods, S., Atlas, E. L., Rodriguez, M. A. N., Wofsy, S. C., Pittman, J., Bardeen, C. G., Toon, O. B., Kindel, B. C., Newman, P. A., McGill, M. J., Hlavka, D. L., Lait, L. R., Schoeberl, M. R., Bergman, J. W., Selkirk, H. B., Alexander, M. J., Kim, J.-E., Lim, B. H., Stutz, J., and Pfeilsticker, K.: The NASA Airborne Tropical Tropopause Experiment: High-Altitude Aircraft Measurements in the Tropical Western Pacific, B. Am. Meteorol. Soc., 98, 129–143, https://doi.org/10.1175/BAMS-D-14-00263.1, 2017. a
Jensen, E. J., Kärcher, B., Ueyama, R., Pfister, L., Bui, T. V., Diskin, G. S., DiGangi, J. P., Woods, S., Lawson, R. P., Froyd, K. D., and Murphy, D. M.: Heterogeneous ice nucleation in the tropical tropopause layer, J. Geophys. Res., 123, 12210–12227, https://doi.org/10.1029/2018JD028949, 2018a. a, b, c
Jensen, E. J., van den Heever, S. C., and Grant, L. D.: The lifecycles of ice crystals detrained from the tops of deep convection, J. Geophys. Res.-Atmos., 123, 9624– 9634, https://doi.org/10.1029/2018JD028832, 2018b. a, b, c
Jensen, E. J., Diskin, G., DiGangi, J., Woods, S., Lawson, R. P., and Bui, T. V.: Homogeneous freezing events sampled in the Tropical Tropopause Layer, J. Geophys. Res., 127, e2022JD036535, https://doi.org/10.1029/2022JD036535, 2022. a
Jiang, J. H., Yue, Q., Su, H., Kangaslahti, P., Lebsock, M., Reising, S., Schoeberl, M., Wu, L., and Herman, R. L.: Simulation of Remote Sensing of Clouds and Humidity From Space Using a Combined Platform of Radar and Multifrequency Microwave Radiometers, Earth Space Sci., 6, 1234–1243, https://doi.org/10.1029/2019EA000580, 2019. a
Jones, W. K., Christensen, M. W., and Stier, P.: A semi-Lagrangian method for detecting and tracking deep convective clouds in geostationary satellite observations, Atmos. Meas. Tech., 16, 1043–1059, https://doi.org/10.5194/amt-16-1043-2023, 2023. a, b
Judt, F., Klocke, D., Rios-Berrios, R., Vanniere, B., Ziemen, F., Auger, L., Biercamp, J., Bretherton, C., Chen, X., Düben, P., Hohenegger, C., Khairoutdinov, M., Kodama, C., Kornblueh, L., Lin, S.-J., Nakano, M., Neumann, P., Putman, W., Röber, N., Roberts, M., Satoh, M., Shibuya, R., Stevens, B., Vidale, P. L., Wedi, N., and Zhou, L.: Tropical Cyclones in Global Storm-Resolving Models, J. Meteorol. Soc. JPN II, 99, 579–602, https://doi.org/10.2151/jmsj.2021-029, 2021. a
Kalnajs, L. E., Davis, S. M., Goetz, J. D., Deshler, T., Khaykin, S., St. Clair, A., Hertzog, A., Bordereau, J., and Lykov, A.: A reel-down instrument system for profile measurements of water vapor, temperature, clouds, and aerosol beneath constant-altitude scientific balloons, Atmos. Meas. Tech., 14, 2635–2648, https://doi.org/10.5194/amt-14-2635-2021, 2021. a
Kanji, Z. A., Ladino, L. A., Wex, H., Boose, Y., Burkert-Kohn, M., Cziczo, D. J., and Krämer, M.: Chapter 1: Overview of Ice Nucleating Particles, Meteor. Mon., 58, 1–1, https://doi.org/10.1175/AMSMONOGRAPHS-D-16-0006.1, 2017. a, b
Kanji, Z. A., Sullivan, R. C., Niemand, M., DeMott, P. J., Prenni, A. J., Chou, C., Saathoff, H., and Möhler, O.: Heterogeneous ice nucleation properties of natural desert dust particles coated with a surrogate of secondary organic aerosol, Atmos. Chem. Phys., 19, 5091–5110, https://doi.org/10.5194/acp-19-5091-2019, 2019. a
Kärcher, B.: A parameterization of cirrus cloud formation: Revisiting competing ice nucleation, J. Geophys. Res., 127, e2022JD036907, https://doi.org/10.1029/2022JD036907, 2022. a
Kärcher, B. and Burkhardt, U.: A cirrus cloud scheme for general circulation models, Q. J. Roy. Meteorol. Soc., 134, 1439–1461, https://doi.org/10.1002/qj.301, 2008. a
Kärcher, B. and Lohmann, U.: A parameterization of cirrus cloud formation: Homogeneous freezing of supercooled aerosols, J. Geophys. Res.-Atmos., 107, AAC4-1–AAC4-10, https://doi.org/10.1029/2001JD000470, 2002. a
Kärcher, B. and Lohmann, U.: A parameterization of cirrus cloud formation: Heterogeneous freezing, J. Geophys. Res.-Atmos., 108, 4402, https://doi.org/10.1029/2002JD003220, 2003. a
Kärcher, B. and Podglajen, A.: A stochastic representation of temperature fluctuations induced by mesoscale gravity waves, J. Geophys. Res., 124, 11506–11529, https://doi.org/10.10292019JD030680, 2019. a
Kärcher, B., Hendricks, J., and Lohmann, U.: Physically based parameterization of cirrus cloud formation for use in global atmospheric models, J. Geophys. Res., 111, D01205, https://doi.org/10.1029/2005JD006219, 2006. a
Kärcher, B., DeMott, P. J., Jensen, E. J., and Harrington, J. Y.: Studies on the competition between homogeneous and heterogeneous ice nucleation in cirrus formation, J. Geophys. Res., 127, e2021JD035805, https://doi.org/10.1029/2021JD035805, 2022. a
Kato, S., Rose, F. G., Sun-Mack, S., Miller, W. F., Chen, Y., Rutan, D. A., Stephens, G. L., Loeb, N. G., Minnis, P., Wielicki, B. A., Winker, D. M., Charlock, T. P., Stackhouse, P. W., Xu, K. M., and Collins, W. D.: Improvements of top-of-atmosphere and surface irradiance computations with CALIPSO-, CloudSat-, and MODIS-derived cloud and aerosol properties, J. Geophys. Res.-Atmos., 116, 1–21, https://doi.org/10.1029/2011JD016050, 2011. a
Khaykin, S. M., Pommereau, J.-P., Riviere, E. D., Held, G., Ploeger, F., Ghysels, M., Amarouche, N., Vernier, J.-P., Wienhold, F. G., and Ionov, D.: Evidence of horizontal and vertical transport of water in the Southern Hemisphere tropical tropopause layer (TTL) from high-resolution balloon observations, Atmos. Chem. Phys., 16, 12273–12286, https://doi.org/10.5194/acp-16-12273-2016, 2016. a
Kim, J.-E., Alexander, M. J., Bui, T. P., Dean-Day, J. M., Lawson, R. P., Woods, S., Hlavka, D., Pfister, L., and Jensen, E. J.: Ubiquitous influence of waves on tropical high cirrus cloud, Geophys. Res. Lett., 43, 5895–5901, https://doi.org/10.1002/2016GL069293, 2016. a, b, c
Kiselev, A., Bachmann, F., Pedevilla, P., Cox, S. J., Michaelides, A., Gerthsen, D., and Leisner, T.: Active sites in heterogeneous ice nucleation—the example of K-rich feldspars, Science, 355, 367–371, https://doi.org/10.1126/science.aai8034, 2017. a
Klein, S. A., Hall, A., Norris, J. R., and Pincus, R.: Low-Cloud Feedbacks from Cloud-Controlling Factors: A Review, Surv. Geophys., 38, 1307–1329, https://doi.org/10.1007/s10712-017-9433-3, 2017. a
Knutson, T. R. and Manabe, S.: Time-Mean Response over the Tropical Pacific to Increased C02 in a Coupled Ocean-Atmosphere Model, J. Climate, 8, 2181–2199, https://doi.org/10.1175/1520-0442(1995)008<2181:TMROTT>2.0.CO;2, 1995. a
Koll, D. D. B. and Cronin, T. W.: Earth’s outgoing longwave radiation linear due to H2O greenhouse effect, P. Nat. Acad. Sci. USA, 115, 10293–10298, https://doi.org/10.1073/pnas.1809868115, 2018. a
Koop, T., Luo, B., Tsias, A., and Peter, T.: Water activity as the determinant for homogeneous ice nucleation in aqueous solutions, Nature, 406, 611–614, 2000. a
Korolev, A. V. and Mazin, I. P.: Supersaturation of Water Vapor in Clouds, J. Atmos. Sci., 60, 2957–2974, https://doi.org/10.1175/1520-0469(2003)060<2957:SOWVIC>2.0.CO;2, 2003. a
Kox, S., Bugliaro, L., and Ostler, A.: Retrieval of cirrus cloud optical thickness and top altitude from geostationary remote sensing, Atmos. Meas. Tech., 7, 3233–3246, https://doi.org/10.5194/amt-7-3233-2014, 2014. a
Krämer, M., Rolf, C., Luebke, A., Afchine, A., Spelten, N., Costa, A., Meyer, J., Zöger, M., Smith, J., Herman, R. L., Buchholz, B., Ebert, V., Baumgardner, D., Borrmann, S., Klingebiel, M., and Avallone, L.: A microphysics guide to cirrus clouds – Part 1: Cirrus types, Atmos. Chem. Phys., 16, 3463–3483, https://doi.org/10.5194/acp-16-3463-2016, 2016. a, b
Krämer, M., Rolf, C., Spelten, N., Afchine, A., Fahey, D., Jensen, E., Khaykin, S., Kuhn, T., Lawson, P., Lykov, A., Pan, L. L., Riese, M., Rollins, A., Stroh, F., Thornberry, T., Wolf, V., Woods, S., Spichtinger, P., Quaas, J., and Sourdeval, O.: A microphysics guide to cirrus – Part 2: Climatologies of clouds and humidity from observations, Atmos. Chem. Phys., 20, 12569–12608, https://doi.org/10.5194/acp-20-12569-2020, 2020. a, b, c
Kretzschmar, J., Stapf, J., Klocke, D., Wendisch, M., and Quaas, J.: Employing airborne radiation and cloud microphysics observations to improve cloud representation in ICON at kilometer-scale resolution in the Arctic, Atmos. Chem. Phys., 20, 13145–13165, https://doi.org/10.5194/acp-20-13145-2020, 2020. a
Krol, M., de Bruine, M., Killaars, L., Ouwersloot, H., Pozzer, A., Yin, Y., Chevallier, F., Bousquet, P., Patra, P., Belikov, D., Maksyutov, S., Dhomse, S., Feng, W., and Chipperfield, M. P.: Age of air as a diagnostic for transport timescales in global models, Geosci. Model Dev., 11, 3109–3130, https://doi.org/10.5194/gmd-11-3109-2018, 2018. a
Kuang, Z. and Bretherton, C. S.: Convective Influence on the Heat Balance of the Tropical Tropopause Layer: A Cloud-Resolving Model Study, J. Atmos. Sci., 61, 2919–2927, https://doi.org/10.1175/jas-3306.1, 2004. a
Kuang, Z. and Hartmann, D. L.: Testing the Fixed Anvil Temperature Hypothesis in a Cloud-Resolving Model, J. Climate, 20, https://doi.org/10.1175/JCLI4124.1, 2051–2057, https://doi.org/10.1175/JCLI4124.1, 2007. a, b
Kubar, T. L. and Jiang, J. H.: Net Cloud Thinning, Low-Level Cloud Diminishment, and Hadley Circulation Weakening of Precipitating Clouds with Tropical West Pacific SST Using MISR and Other Satellite and Reanalysis Data, Remote Sens., 11, 1250, https://doi.org/10.3390/rs11101250, 2019. a, b
Kubar, T. L., Hartmann, D. L., and Wood, R.: Radiative and Convective Driving of Tropical High Clouds, J. Climate, 20, 5510–5526, https://doi.org/10.1175/2007JCLI1628.1, 2007. a
Kurihana, T., Moyer, E. J., and Foster, I. T.: AICCA: AI-Driven Cloud Classification Atlas, Remote Sens., 14, 5690, https://doi.org/10.3390/rs14225690, 2022. a
Köhler, L., Green, B., and Stephan, C. C.: Comparing Loon Superpressure Balloon Observations of Gravity Waves in the Tropics With Global Storm-Resolving Models, J. Geophys. Res.-Atmos., 128, e2023JD038549, https://doi.org/10.1029/2023JD038549, 2023. a
Ladino, L. A., Korolev, A., Heckman, I., Wolde, M., Fridlind, A. M., and Ackerman, A. S.: On the role of ice-nucleating aerosol in the formation of ice particles in tropical mesoscale convective systems, Geophys. Res. Lett., 44, 1574–1582, https://doi.org/10.1002/2016GL072455, 2017. a
Ladstädter, Steiner, F. A. K., and Gleisner, H.: Resolving the 21st century temperature trends of the upper troposphere-lower stratosphere with satellite observations, Nature, 13, 2023, https://doi.org/10.1038/s41598-023-28222-x, 2023. a
Lamb, D. and Verlinde, J.: Physics and Chemistry of Clouds, University Press, Cambridge, NY, https://doi.org/10.1017/CBO9780511976377, 2011. a
Lamb, K. D., Clouser, B. W., Bolot, M., Sarkozy, L., Ebert, V., Saathoff, H., Möhler, O., and Moyer, E. J.: Laboratory measurements of HDO/H2O isotopic fractionation during ice deposition in simulated cirrus clouds, P. Natl. Acad. Sci. USA, 114, 5612–5617, https://doi.org/10.1073/pnas.1618374114, 2017. a, b
Lamb, K. D., Harrington, J. Y., Clouser, B. W., Moyer, E. J., Sarkozy, L., Ebert, V., Möhler, O., and Saathoff, H.: Re-evaluating cloud chamber constraints on depositional ice growth in cirrus clouds – Part 1: Model description and sensitivity tests, Atmos. Chem. Phys., 23, 6043–6064, https://doi.org/10.5194/acp-23-6043-2023, 2023. a
Lamquin, N., Stubenrauch, C. J., Gierens, K., Burkhardt, U., and Smit, H.: A global climatology of upper-tropospheric ice supersaturation occurrence inferred from the Atmospheric Infrared Sounder calibrated by MOZAIC, Atmos. Chem. Phys., 12, 381–405, https://doi.org/10.5194/acp-12-381-2012, 2012. a
Lamraoui, F., Krämer, M., Afchine, A., Sokol, A. B., Khaykin, S., Pandey, A., and Kuang, Z.: Sensitivity of convectively driven tropical tropopause cirrus properties to ice habits in high-resolution simulations, Atmos. Chem. Phys., 23, 2393–2419, https://doi.org/10.5194/acp-23-2393-2023, 2023. a, b
Lane, T. P., Sharman, R. D., Clark, T. L., and Hsu, H.-M.: An Investigation of Turbulence Generation Mechanisms above Deep Convection, J. Atmos. Sci., 60, 1297–1321, https://doi.org/10.1175/1520-0469(2003)60<1297:AIOTGM>2.0.CO;2, 2003. a
L'Ecuyer, T. S., Wood, N. B., Haladay, T., Stephens, G. L., and Stackhouse, P. W.: Impact of clouds on atmospheric heating based on the R04 CloudSat fluxes and heating rates data set, J. Geophys. Res., 113, D00A15, https://doi.org/10.1029/2008JD009951, 2008. a
Lee, J., Yang, P., Dessler, A. E., Gao, B.-C., and Platnick, S.: Distribution and Radiative Forcing of Tropical Thin Cirrus Clouds, J. Atmos. Sci., 66, 3721–3731, https://doi.org/10.1175/2009JAS3183.1, 2009. a
Leonarski, L., C.-Labonnote, L., Compiègne, M., Vidot, J., Baran, A. J., and Dubuisson, P.: Potential of Hyperspectral Thermal Infrared Spaceborne Measurements To Retrieve Ice Cloud Physical Properties: Case Study of Iasi and Iasi-Ng, Remote Sens., 13, 116, https://doi.org/10.3390/rs13010116, 2020. a
Li, R. L., Storelvmo, T., Fedorov, A. V., and Choi, Y.-S.: A Positive Iris Feedback: Insights from Climate Simulations with Temperature-Sensitive Cloud–Rain Conversion, J. Climate, 32, 5305–5324, https://doi.org/10.1175/JCLI-D-18-0845.1, 2019. a, b
Li, Y. and Thompson, D. W. J.: The signature of the stratospheric Brewer‒Dobson circulation in tropospheric clouds, J. Geophys. Res.-Atmos., 118, 3486–3494, https://doi.org/10.1002/jgrd.50339, 2013. a
Lian, S., Zhou, L., Murphy, D. M., Froyd, K. D., Toon, O. B., and Yu, P.: Global distribution of Asian, Middle Eastern, and North African dust simulated by CESM1/CARMA, Atmos. Chem. Phys., 22, 13659–13676, https://doi.org/10.5194/acp-22-13659-2022, 2022. a
Libbrecht, K. G.: The physics of snow crystals, Rep. Prog. Phys., 68, 855, https://doi.org/10.1088/0034-4885/68/4/R03, 2005. a, b
Lilly, D. K.: Cirrus outflow dynamics, J. Atmos. Sci., 45, 1594–1605, https://doi.org/10.1175/1520-0469(1988)045<1594:COD>2.0.CO;2, 1988. a, b
Lin, B., Wielicki, B. A., Chambers, L. H., Hu, Y., and Xu, K.-M.: The Iris Hypothesis: A Negative or Positive Cloud Feedback?, J. Climate, 15, 3–7, https://doi.org/10.1175/1520-0442(2002)015<0003:TIHANO>2.0.CO;2, 2002. a
Lindzen, R. S., Chou, M. D., and Hou, A. Y.: Does the Earth Have an Adaptive Infrared Iris?, B. Am. Meteorol. Soc., 82, 417–432, https://doi.org/10.1175/1520-0477(2001)082<0417:DTEHAA>2.3.CO;2, 2001. a, b, c, d
Liu, C., Zipser, E. J., and Nesbitt, S. W.: Global Distribution of Tropical Deep Convection: Different Perspectives from TRMM Infrared and Radar Data, J. Climate, 20, 489–503, https://doi.org/10.1175/JCLI4023.1, 2007. a
Liu, R., Liou, K.-N., Su, H., Gu, Y., Zhao, B., Jiang, J. H., and Liu, S. C.: High cloud variations with surface temperature from 2002 to 2015: Contributions to atmospheric radiative cooling rate and precipitation changes, J. Geophys. Res.-Atmos., 122, 5457–5471, https://doi.org/10.1002/2016JD026303, 2017. a, b
Luke, E. P., Yang, F., Kollias, P., Vogelmann, A. M., and Maahn, M.: New insights into ice multiplication using remote-sensing observations of slightly supercooled mixed-phase clouds in the Arctic, P. Natl. Acad. Sci. USA, 118, e2021387118, https://doi.org/10.1073/pnas.2021387118, 2021. a
Luo, Z. and Rossow, W. B.: Characterizing Tropical Cirrus Life Cycle, Evolution, and Interaction with Upper-Tropospheric Water Vapor Using Lagrangian Trajectory Analysis of Satellite Observations, J. Climate, 17, 4541–4563, https://doi.org/10.1175/3222.1, 2004. a, b, c, d
L’Ecuyer, T. S., Hang, Y., Matus, A. V., and Wang, Z.: Reassessing the Effect of Cloud Type on Earth’s Energy Balance in the Age of Active Spaceborne Observations. Part I: Top of Atmosphere and Surface, J. Climate, 32, 6197–6217, https://doi.org/10.1175/JCLI-D-18-0753.1, 2019. a
Mace, G. G., Deng, M., Soden, B., and Zipser, E.: Association of Tropical Cirrus in the 10–15-km Layer with Deep Convective Sources: An Observational Study Combining Millimeter Radar Data and Satellite-Derived Trajectories, J. Atmos. Sci., 63, 480–503, https://doi.org/10.1175/JAS3627.1, 2006. a
Magee, N. B., Miller, A., Amaral, M., and Cumiskey, A.: Mesoscopic surface roughness of ice crystals pervasive across a wide range of ice crystal conditions, Atmos. Chem. Phys., 14, 12357–12371, https://doi.org/10.5194/acp-14-12357-2014, 2014. a
Maher, P., Gerber, E. P., Medeiros, B., Merlis, T. M., Sherwood, S., Sheshadri, A., Sobel, A. H., Vallis, G. K., Voigt, A., and Zurita-Gotor, P.: Model Hierarchies for Understanding Atmospheric Circulation, Rev. Geophys., 57, 250–280, https://doi.org/10.1029/2018RG000607, 2019. a
Maloney, C., Bardeen, C., Toon, O. B., Jensen, E., Woods, S., Thornberry, T., Pfister, L., Diskin, G., and Bui, T. P.: An evaluation of the representation of tropical tropopause cirrus in the CESM/CARMA model using satellite and aircraft observations, J. Geophys. Res., 124, 8659–8687, https://doi.org/10.1029/2018JD029720, 2019. a
Marcolli, C.: Deposition nucleation viewed as homogeneous or immersion freezing in pores and cavities, Atmos. Chem. Phys., 14, 2071–2104, https://doi.org/10.5194/acp-14-2071-2014, 2014. a
Marcolli, C., Mahrt, F., and Kärcher, B.: Soot PCF: pore condensation and freezing framework for soot aggregates, Atmos. Chem. Phys., 21, 7791–7843, https://doi.org/10.5194/acp-21-7791-2021, 2021. a
Massie, S. T., Gille, J., Craig, C., Khosravi, R., Barnett, J., Read, W., and Winker, D.: HIRDLS and CALIPSO observations of tropical cirrus, J. Geophys. Res.-Atmos., 115, D00H11, https://doi.org/10.1029/2009JD012100, 2010. a
Matus, A. V. and L'Ecuyer, T. S.: The role of cloud phase in Earth's radiation budget, J. Geophys. Res.-Atmos., 122, 2559– 2578, https://doi.org/10.1002/2016JD025951, 2017. a, b
Mauritsen, T. and Stevens, B.: Missing iris effect as a possible cause of muted hydrological change and high climate sensitivity in models, Nat. Geosci., 8, 346–351, https://doi.org/10.1038/ngeo2414, 2015. a, b
May, P. T., Mather, J. H., Vaughan, G., Jakob, C., McFarquhar, G. M., Bower, K. N., and Mace, G. G.: The Tropical Warm Pool International Cloud Experiment, B. Am. Meteorol.l Soc., 89, 629–646, https://doi.org/10.1175/BAMS-89-5-629, 2008. a
McKim, B., Bony, S., and Dufresne, J.-L.: Physical and observational constraints on the anvil cloud feedback, ESS Open Archive [preprint], https://doi.org/10.22541/au.167769953.39966398/v2, 11 June 2023. a
Menzel, W. P.: Cloud Tracking with Satellite Imagery: From the Pioneering Work of Ted Fujita to the Present, B. Am. Meteorol. Soc., 82, 33–48, https://doi.org/10.1175/1520-0477(2001)082<0033:CTWSIF>2.3.CO;2, 2001. a
Mitchell, D. L., Garnier, A., Pelon, J., and Erfani, E.: CALIPSO (IIR–CALIOP) retrievals of cirrus cloud ice-particle concentrations, Atmos. Chem. Phys., 18, 17325–17354, https://doi.org/10.5194/acp-18-17325-2018, 2018. a
Morrison, H. and Milbrandt, J. A.: Parameterization of Cloud Microphysics Based on the Prediction of Bulk Ice Particle Properties. Part I: Scheme Description and Idealized Tests, J. Atmos. Sci., 72, 287–311, https://doi.org/10.1175/JAS-D-14-0065.1, 2015. a
Morrison, H., van Lier-Walqui, M., Fridlind, A. M., Grabowski, W. W., Harrington, J. Y., Hoose, C., Korolev, A., Kumjian, M. R., Milbrandt, J. A., Pawlowska, H., Posselt, D. J., Prat, O. P., Reimel, K. J., Shima, S. I., van Diedenhoven, B., and Xue, L.: Confronting the Challenge of Modeling Cloud and Precipitation Microphysics, J. Adv. Model. Earth Systems, 12, e2019MS001689, https://doi.org/10.1029/2019MS001689, 2020. a, b, c
Muench, S. and Lohmann, U.: Developing a cloud scheme with prognostic cloud fraction and two moment microphysics for ECHAM-HAM, J. Adv. Model. Earth Sys., 12, e2019MS001824, https://doi.org/10.1029/2019MS001824, 2020. a
Mülmenstädt, J., Nam, C., Salzmann, M., Kretzschmar, J., L’Ecuyer, T. S., Lohmann, U., Ma, P.-L., Myhre, G., Neubauer, D., Stier, P., Suzuki, K., Wang, M., and Quaas, J.: Reducing the aerosol forcing uncertainty using observational constraints on warm rain processes, Sci. Adv., 6, eaaz6433, https://doi.org/10.1126/sciadv.aaz6433, 2020. a
Murray, B. J., Carslaw, K. S., and Field, P. R.: Opinion: Cloud-phase climate feedback and the importance of ice-nucleating particles, Atmos. Chem. Phys., 21, 665–679, https://doi.org/10.5194/acp-21-665-2021, 2021. a
NASA/LARC/SD/ASDC: CALIPSO Lidar Level 3 Global Energy and Water Cycle Experiment (GEWEX) Cloud, Standard V1-00, https://doi/org/10.5067/CALIOP/CALIPSO/LID_L3_GEWEX, 2019. a
Nelson, J.: Theory of isotopic fractionation on facetted ice crystals, Atmos. Chem. Phys., 11, 11351–11360, https://doi.org/10.5194/acp-11-11351-2011, 2011. a
Norris, J. R., Allen, R. J., Evan, A. T., Zelinka, M. D., O'Dell, C. W., and Klein, S. A.: Evidence for climate change in the satellite cloud record, Nature, 536, 72–75, https://doi.org/10.1038/NATURE18273, 2016. a
Nugent, J. M., Turbeville, S. M., Bretherton, C. S., Blossey, P. N., and Ackerman, T. P.: Tropical Cirrus in Global Storm-Resolving Models. Part I: Role of Deep Convection, Earth Space Sci., 9, e2021EA001965, https://doi.org/10.1029/2021EA001965, 2022. a, b, c, d, e
Parol, F., Buriez, J. C., Brogniez, G., and Fouquart, Y.: Information content of AVHRR channel 4 and 5 with respect to the effective radius of cirrus cloud particles, J. Appl. Meteor., 30, 973–984, 1991. a
Phillips, V. T. J., DeMott, P. J., and Andronache, C.: An Empirical Parameterization of Heterogeneous Ice Nucleation for Multiple Chemical Species of Aerosol, J. Atmos. Sci., 65, 2757–2783, https://doi.org/10.1175/2007JAS2546.1, 2008. a, b
Pincus, R., Platnick, S., Ackerman, S. A., Hemler, R. S., and Patrick Hofmann, R. J.: Reconciling simulated and observed views of clouds: MODIS, ISCCP, and the limits of instrument simulators, J. Climate, 25, 4699–4720, https://doi.org/10.1175/JCLI-D-11-00267.1, 2012. a
Podglajen, A., Hertzog, A., Plougonven, R., and Legras, B.: Lagrangian temperature and vertical velocity fluctuations due to gravity waves in the lower stratosphere, Geophys. Res. Lett., 43, 3543–3553, https://doi.org/10.1002/2016GL068148, 2016. a, b
Podglajen, A., Bui, T. P., Dean-Day, J. M., Pfister, L., Jensen, E. J., Alexander, M. J., Hertzog, A., Kärcher, B., Plougonven, R., and Randel, W. J.: Small-scale wind fluctuations in the tropical tropopause layer from aircraft measurements: Occurrence, nature and impact on vertical mixing, J. Atmos. Sci., 74, 3847–3869, https://doi.org/10.1175/JAS-D-17-0010.1, 2017. a
Podglajen, A., Plougonven, R., Hertzog, A., and Jensen, E.: Impact of gravity waves on the motion and distribution of atmospheric ice particles, Atmos. Chem. Phys., 18, 10799–10823, https://doi.org/10.5194/acp-18-10799-2018, 2018. a
Podglajen, A., Hertzog, A., Plougonven, R., and Legras, B.: Lagrangian gravity wave spectra in the lower stratosphere of current (re)analyses, Atmos. Chem. Phys., 20, 9331–9350, https://doi.org/10.5194/acp-20-9331-2020, 2020. a
Pokrifka, G. F., Moyle, A. M., Hanson, L. E., and Harrington, J. Y.: Estimating surface attachment kinetic and growth transition influences on vapor-grown ice crystals, J. Atmos. Sci., 70, 2393–2410, https://doi.org/10.1175/JAS-D-19-0303.1, 2020. a
Popp, M. and Silvers, L. G.: Double and Single ITCZs with and without Clouds, J. Climate, 30, 9147–9166, https://doi.org/10.1175/JCLI-D-17-0062.1, 2017. a
Porterfield, M.: Data from NASA's Missions, Research, and Activities, https://www.nasa.gov/open/data.html (last access: 31 March 2023), 2021. a
Powell, S. W., Houze, R. A. J., Kumar, A., and McFarlane, S. A.: Comparison of Simulated and Observed Continental Tropical Anvil Clouds and Their Radiative Heating Profiles, J. Atmos. Sci., 69, 2662–2681, https://doi.org/10.1175/jas-d-11-0251.1, 2012. a
Prabhakaran, P., Kinney, G., Cantrell, W., Shaw, R. A., and Bodenschatz, E.: High supersaturation in the wake of falling hydrometeors: Implications for cloud invigoration and ice nucleation, Geophys. Res. Lett., 47, e2020GL088055, https://doi.org/10.1029/2020GL088055, 2020. a
Prein, A. F., Rasmussen, R. M., Wang, D., and Giangrande, S. E.: Sensitivity of organized convective storms to model grid spacing in current and future climates, Philos. T. R. Soc. A, 379, 20190546, https://doi.org/10.1098/rsta.2019.0546, 2021. a
Proske, U., Ferrachat, S., Neubauer, D., Staab, M., and Lohmann, U.: Assessing the potential for simplification in global climate model cloud microphysics, Atmos. Chem. Phys., 22, 4737–4762, https://doi.org/10.5194/acp-22-4737-2022, 2022. a, b
Protopapadaki, S. E., Stubenrauch, C. J., and Feofilov, A. G.: Upper tropospheric cloud systems derived from IR sounders: properties of cirrus anvils in the tropics, Atmos. Chem. Phys., 17, 3845–3859, https://doi.org/10.5194/acp-17-3845-2017, 2017. a, b
Qu, Z., Korolev, A., Milbrandt, J. A., Heckman, I., Huang, Y., McFarquhar, G. M., Morrison, H., Wolde, M., and Nguyen, C.: The impacts of secondary ice production on microphysics and dynamics in tropical convection, Atmos. Chem. Phys., 22, 12287–12310, https://doi.org/10.5194/acp-22-12287-2022, 2022. a
Ramanathan, V. and Collins, W.: Thermodynamic regulation of ocean warming by cirrus clouds deduced from observations of the 1987 El Niño, Nature, 351, 27–32, https://doi.org/10.1038/351027a0, 1991. a
Ramanathan, V., Cess, R. D., Harrison, E. F., Minnis, P., Barkstrom, B. R., Ahmad, E., and Hartmann, D.: Cloud-radiative forcing and climate: Results from the earth radiation budget experiment, Science, 243, 57–63, https://doi.org/10.1126/science.243.4887.57, 1989. a
Randel, W. J. and Jensen, E. J.: Physical processes in the tropical tropopause layer and their roles in a changing climate, Nat. Geosci., 6, 169–176, https://doi.org/10.1038/ngeo1733, 2013. a
Ravetta, F., Mariage, V., Brousse, E., DÁlmeida, E., Ferreira, F., Pelon, J., and Victori, S.: BeCOOL: A Balloon-Borne Microlidar System Designed for Cirrus and Convective Overshoot Monitoring, EPJ Web Conf., 237, 7003, https://doi.org/10.1051/epjconf/202023707003, 2020. a
Raymond, D. J.: A New Model of the Madden–Julian Oscillation, J. Atmos. Sci., 58, 2807–2819, https://doi.org/10.1175/1520-0469(2001)058<2807:ANMOTM>2.0.CO;2, 2001. a
Reutter, P., Neis, P., Rohs, S., and Sauvage, B.: Ice supersaturated regions: properties and validation of ERA-Interim reanalysis with IAGOS in situ water vapour measurements, Atmos. Chem. Phys., 20, 787–804, https://doi.org/10.5194/acp-20-787-2020, 2020. a
Riley, E. M., Mapes, B. E., and Tulich, S. N.: Clouds Associated with the Madden–Julian Oscillation: A New Perspective from CloudSat, J. Atmos. Sci., 68, 3032–3051, https://doi.org/10.1175/JAS-D-11-030.1, 2011. a
Robert A Houze, J.: Cloud Clusters and Large-Scale Vertical Motions in the Tropics, J. Meteorol. Soc. Jap, 60, 396–410, https://doi.org/10.2151/jmsj1965.60.1_396, 1982. a
Roca, R., Fiolleau, T., and Bouniol, D.: A simple model of the life cycle of mesoscale convective systems cloud shield in the tropics, J. Climate, 30, 4283–4298, https://doi.org/10.1175/JCLI-D-16-0556.1, 2017. a, b
Roh, W., Satoh, M., and Hohenegger, C.: Intercomparison of Cloud Properties in DYAMOND Simulations over the Atlantic Ocean, J. Meteorol. Soc. Jpn, 99, 1439–1451, https://doi.org/10.2151/jmsj.2021-070, 2021. a
Rollins, A. W., Thornberry, T. D., Gao, R. S., Woods, S., Lawson, R. P., Bui, T. P., Jensen, E. J., and Fahey, D. W.: Observational constraints on the efficiency of dehydration mechanisms in the tropical tropopause layer, Geophys. Res. Lett., 43, 2912–2918, https://doi.org/10.1002/2016GL067972, 2016. a
Rosenfeld, D. and Woodley, W. L.: Deep convective clouds with sustained supercooled liquid water down to −37.5 ∘C, Nature, 405, 440–442, https://doi.org/10.1038/35013030, 2000. a
Rossow, W. B. and Schiffer, R. A.: Advances in Understanding Clouds from ISCCP, B. Am. Meteorol. Soc., 80, 2261–2287, https://doi.org/10.1175/1520-0477(1999)080<2261:AIUCFI>2.0.CO;2, 1999. a, b
Saint-Lu, M., Bony, S., and Dufresne, J.-L.: Observational Evidence for a Stability Iris Effect in the Tropics, Geophys. Res. Lett., 47, e2020GL089059, https://doi.org/10.1029/2020GL089059, 2020. a, b
Saint-Lu, M., Bony, S., and Dufresne, J.-L.: Clear-sky control of anvils in response to increased CO2 or surface warming or volcanic eruptions, npj Clim. Atmos. Sci., 5, 1–8, https://doi.org/10.1038/s41612-022-00304-z, number: 1 Publisher: Nature Publishing Group, 2022. a, b
Saleeby, S. M. and van den Heever, S. C.: Developments in the CSU-RAMS Aerosol Model: Emissions, Nucleation, Regeneration, Deposition, and Radiation, J. Appl. Meteorol. Climatol., 52, 2601–2622, https://doi.org/10.1175/JAMC-D-12-0312.1, 2013. a
Sassen, K., Wang, Z., and Liu, D.: Cirrus clouds and deep convection in the tropics: Insights from CALIPSO and CloudSat, J. Geophys. Res.-Atmos., 114, 1–11, https://doi.org/10.1029/2009JD011916, 2009. a
Sauter, K., L'Ecuyer, T. S., van den Heever, S. C., Twohy, C., Heidinger, A., Wanzong, S., and Wood, N.: The Observed Influence of Tropical Convection on the Saharan Dust Layer, J. Geophys. Res.-Atmos., 124, 10896–10912, https://doi.org/10.1029/2019JD031365, 2019. a
Scherllin-Pirscher, B. ., Steiner, A. K., Anthes, R. A., Alexander, M. J., Alexander, S. P., Biondi, R., Birner, T., Kim, J., Randel, W. J., Son, S.-W., Tsuda, T., and Zeng, Z.: Tropical temperature variability in the UTLS: New insights from GPS radio occultation observations, J. Climate, 34, 2813–2838, https://doi.org/10.1175/JCLI-D-20-0385.1, 2021. a
Schmidt, C. T. and Garrett, T. J.: A Simple Framework for the Dynamic Response of Cirrus Clouds to Local Diabatic Radiative Heating, J. Atmos. Sci., 70, 1409–1422, https://doi.org/10.1175/JAS-D-12-056.1, 2013. a, b
Schneider, J., Höhler, K., Wagner, R., Saathoff, H., Schnaiter, M., Schorr, T., Steinke, I., Benz, S., Baumgartner, M., Rolf, C., Krämer, M., Leisner, T., and Möhler, O.: High homogeneous freezing onsets of sulfuric acid aerosol at cirrus temperatures, Atmos. Chem. Phys., 21, 14403–14425, https://doi.org/10.5194/acp-21-14403-2021, 2021. a, b
Schumacher, C., Houze, R. A. J., and Kraucunas, I.: The Tropical Dynamical Response to Latent Heating Estimates Derived from the TRMM Precipitation Radar, J. Atmos. Sci., 61, 1341–1358, https://doi.org/10.1175/1520-0469(2004)061<1341:TTDRTL>2.0.CO;2, 2004. a
Seeley, J. T., Jeevanjee, N., Langhans, W., and Romps, D. M.: Formation of Tropical Anvil Clouds by Slow Evaporation, Geophys. Res. Lett., 46, 492–501, https://doi.org/10.1029/2018GL080747, 2019. a, b
Seidel, S. D. and Yang, D.: Temperatures of Anvil Clouds and Radiative Tropopause in a Wide Array of Cloud-Resolving Simulations, J. Climate, 35, 8065–8078, https://doi.org/10.1175/JCLI-D-21-0962.1, 2022. a
Sherwood, S. C., Webb, M. J., Annan, J. D., Armour, K. C., Forster, P. M., Hargreaves, J. C., Hegerl, G., Klein, S. A., Marvel, K. D., Rohling, E. J., Watanabe, M., Andrews, T., Braconnot, P., Bretherton, C. S., Foster, G. L., Hausfather, Z., Heydt, A. S. v. d., Knutti, R., Mauritsen, T., Norris, J. R., Proistosescu, C., Rugenstein, M., Schmidt, G. A., Tokarska, K. B., and Zelinka, M. D.: An Assessment of Earth's Climate Sensitivity Using Multiple Lines of Evidence, Rev. Geophys., 58, e2019RG000678, https://doi.org/10.1029/2019RG000678, 2020. a, b, c
Shima, S., Sato, Y., Hashimoto, A., and Misumi, R.: Predicting the morphology of ice particles in deep convection using the super-droplet method: development and evaluation of SCALE-SDM 0.2.5–2.2.0, −2.2.1, and −2.2.2, Geosci. Model Dev., 13, 4107–4157, https://doi.org/10.5194/gmd-13-4107-2020, 2020. a
Shindell, D. T.: Climate and ozone response to increased stratospheric water vapor, Geophys. Res. Lett., 28, 1551–1554, https://doi.org/10.1029/1999GL011197, 2001. a
Skamarock, W. C., Snyder, C., Klemp, J. B., and Park, S.-H.: Vertical Resolution Requirements in Atmospheric Simulation, Mon. Weather Rev., 147, 2641–2656, https://doi.org/10.1175/MWR-D-19-0043.1, 2019. a
Skrotzki, J., Connolly, P., Schnaiter, M., Saathoff, H., Möhler, O., Wagner, R., Niemand, M., Ebert, V., and Leisner, T.: The accommodation coefficient of water molecules on ice – cirrus cloud studies at the AIDA simulation chamber, Atmos. Chem. Phys., 13, 4451–4466, https://doi.org/10.5194/acp-13-4451-2013, 2013. a, b
Sokol, A. B.: gasparini_et_al_2023, Github [code], https://github.com/adambsokol/gasparini_et_al_2023 (last access: last access: 14 December 2023), 2023. a
Sokol, A. B. and Hartmann, D. L.: Tropical Anvil Clouds: Radiative Driving Toward a Preferred State, J. Geophys. Res.-Atmos., 125, e2020JD033107, https://doi.org/10.1029/2020JD033107, 2020. a, b, c
Sölch, I. and Kärcher, B.: A large-eddy model for cirrus cloudsvwith explicit aerosol and ice microphysics and Lagrangian ice particle tracking, Q. J. Roy. Meteorol. Soc., 136, 2074–2093, https://doi.org/10.1002/qj.689, 2010. a, b
Sölch, I. and Kärcher, B.: Process-oriented large-eddy simulations of a midlatitude cirrus cloud system based on observations, Q. J. Roy. Meteorol. Soc., 137, 374–393, https://doi.org/10.1002/qj.764, 2011. a
Solomon, S., Rosenlof, K., Portmann, R., Daniel, J., Davis, S., Sanford, T., and Plattner, G.-K.: Contributions of stratospheric water vapor changes to decadal variations in the rate of global warming, Science, 327, 1219–1223, https://doi.org/10.1126/science.1182488, 2010. a, b
Sourdeval, O., Gryspeerdt, E., Krämer, M., Goren, T., Delanoë, J., Afchine, A., Hemmer, F., and Quaas, J.: Ice crystal number concentration estimates from lidar–radar satellite remote sensing – Part 1: Method and evaluation, Atmos. Chem. Phys., 18, 14327–14350, https://doi.org/10.5194/acp-18-14327-2018, 2018. a, b
Stauffer, C. L. and Wing, A. A.: Properties, Changes, and Controls of Deep-Convecting Clouds in Radiative-Convective Equilibrium, J. Adv. Model. Earth Syst., 14, e2021MS002917, https://doi.org/10.1029/2021MS002917, 2022. a, b, c
Stein, T. H. M., Holloway, C. E., Tobin, I., and Bony, S.: Observed Relationships between Cloud Vertical Structure and Convective Aggregation over Tropical Ocean, J. Climate, 30, 2187–2207, https://doi.org/10.1175/JCLI-D-16-0125.1, 2017. a, b
Stephan, C. C., Strube, C., Klocke, D., Ern, M., Hoffmann, L., Preusse, P., and Schmidt, H.: Gravity waves in global high-resolution simulations with explicit and parameterized convection, J. Geophys. Res., 124, 4446–4459, https://doi.org/10.1029/2018JD030073, 2019. a
Stephens, G., Winker, D., Pelon, J., Trepte, C., Vane, D., Yuhas, C., L'Ecuyer, T., and Lebsock, M.: CloudSat and CALIPSO within the A-Train: Ten Years of Actively Observing the Earth System, B. Am. Meteorol. Soc., 99, 569–581, https://doi.org/10.1175/BAMS-D-16-0324.1, 2018. a
Stephens, G. L., Vane, D. G., Tanelli, S., Im, E., Durden, S., Rokey, M., Reinke, D., Partain, P., Mace, G. G., Austin, R., L'Ecuyer, T. S., Haynes, J., Lebsock, M., Suzuki, K., Waliser, D., Wu, D., Kay, J., Gettelman, A., Wang, Z., and Marchand, R.: CloudSat mission: Performance and early science after the first year of operation, J. Geophys. Res.-Atmos., 114, 1–18, https://doi.org/10.1029/2008JD009982, 2008. a
Stevens, B. and Brenguier, J.-L.: Cloud-controlling Factors: Low Clouds, in: Clouds in the Perturbed Climate System: Their Relationship to Energy Balance, Atmospheric Dynamics, and Precipitation, The MIT Press, ISBN 9780262012874, https://doi.org/10.7551/mitpress/9780262012874.003.0008, 2009. a
Stevens, B. and Kluft, L.: A Colorful look at Climate Sensitivity, EGUsphere [preprint], https://doi.org/10.5194/egusphere-2022-1460, 2023. a
Stevens, B., Acquistapace, C., Hansen, A., Heinze, R., Klinger, C., Klocke, D., Rybka, H., Schubotz, W., Windmiller, J., Adamidis, P., Arka, I., Barlakas, V., Biercamp, J., Brueck, M., Brune, S., Buehler, S. A., Burkhardt, U., Cioni, G., Costa-Surós, M., Crewell, S., Crüger, T., Deneke, H., Friederichs, P., Henken, C. C., Hohenegger, C., Jacob, M., Jakub, F., Kalthoff, N., Köhler, M., van LAAR, T. W., Li, P., Löhnert, U., Macke, A., Madenach, N., Mayer, B., Nam, C., Naumann, A. K., Peters, K., Poll, S., Quaas, J., Röber, N., Rochetin, N., Scheck, L., Schemann, V., Schnitt, S., Seifert, A., Senf, F., Shapkalijevski, M., Simmer, C., Singh, S., Sourdeval, O., Spickermann, D., Strandgren, J., Tessiot, O., Vercauteren, N., Vial, J., Voigt, A., and Zängl, G.: The added value of large-eddy and storm-resolving models for simulating clouds and precipitation, J. Meteorol. Soc. Jpn, 98, 395–435, https://doi.org/10.2151/jmsj.2020-021, 2020. a
Strandgren, J., Fricker, J., and Bugliaro, L.: Characterisation of the artificial neural network CiPS for cirrus cloud remote sensing with MSG/SEVIRI, Atmos. Meas. Tech., 10, 4317–4339, https://doi.org/10.5194/amt-10-4317-2017, 2017. a, b
Strapp, J., Schwarzenboeck, A., Bedka, K., Bond, T., Calmels, A., Delanoë, J., Dezitter, F., Grzych, M., Harrah, S., Korolev, A., Leroy, D., Lilie, L., Mason, J., Potts, R., Protat, A., Ratvasky, T., Riley, J., and Wolde, M.: An Assessment of Cloud Total Water Content and Particle Size from Flight Test Campaign Measurements in High Ice Water Content, Mixed Phase/Ice Crystal Icing Conditions: Primary In-Situ Measurements, Tech. rep., Federal Aviation Administration, https://rosap.ntl.bts.gov/view/dot/57746 (last access: 14 December 2023), 2020. a, b
Stubenrauch, C. J., Rossow, W. B., Kinne, S., Ackerman, S., Cesana, G., Chepfer, H., Di Girolamo, L., Getzewich, B., Guignard, A., Heidinger, A., Maddux, B. C., Menzel, W. P., Minnis, P., Pearl, C., Platnick, S., Poulsen, C., Riedi, J., Sun-Mack, S., Walther, A., Winker, D., Zeng, S., and Zhao, G.: Assessment of global cloud datasets from satellites: Project and database initiated by the GEWEX radiation panel, B. Am. Meteorol. Soc., 94, 1031–1049, https://doi.org/10.1175/BAMS-D-12-00117.1, 2013. a
Stubenrauch, C. J., Feofilov, A. G., Protopapadaki, S. E., and Armante, R.: Cloud climatologies from the infrared sounders AIRS and IASI: strengths and applications, Atmos. Chem. Phys., 17, 13625–13644, https://doi.org/10.5194/acp-17-13625-2017, 2017. a, b
Stubenrauch, C. J., Bonazzola, M., Protopapadaki, S. E., and Musat, I.: New Cloud System Metrics to Assess Bulk Ice Cloud Schemes in a GCM, J. Adv. Model. Earth Syst., 11, 3212–3234, https://doi.org/10.1029/2019MS001642, 2019. a
Stubenrauch, C. J., Mandorli, G., and Lemaitre, E.: Convective organization and 3D structure of tropical cloud systems deduced from synergistic A-Train observations and machine learning, Atmos. Chem. Phys., 23, 5867–5884, https://doi.org/10.5194/acp-23-5867-2023, 2023. a
Su, H., Jiang, J. H., Gu, Y., Neelin, J. D., Kahn, B. H., Feldman, D., Yung, Y. L., Waters, J. W., Livesey, N. J., Santee, M. L., and Read, W. G.: Variations of tropical upper tropospheric clouds with sea surface temperature and implications for radiative effects, J. Geophys. Res.-Atmos., 113, D10211, https://doi.org/10.1029/2007JD009624, 2008. a
Su, H., Jiang, J. H., Neelin, J. D., Shen, T. J., Zhai, C., Yue, Q., Wang, Z., Huang, L., Choi, Y.-S., Stephens, G. L., and Yung, Y. L.: Tightening of tropical ascent and high clouds key to precipitation change in a warmer climate, Nat. Commun., 8, 15771, https://doi.org/10.1038/ncomms15771, 2017. a
Sullivan, S. C. and Hoose, C.: Science of Cloud and Climate Science: An Analysis of the Literature Over the Past 50 Years, in: Clouds and Their Climatic Impact: Radiation, Circulation, and Precipitation, edited by: Sullivan, S. C. and Hoose, C., Wiley–American Geophysical Union, 1-14, ISBN 978-1-119-70031-9, 2024. a
Sullivan, S., Voigt, A., Miltenberger, A., Rolf, C., and Krämer, M.: A Lagrangian Perspective of Microphysical Impact on Ice Cloud Evolution and Radiative Heating, J. Adv. Model. Earth Syst., 14, e2022MS003226, https://doi.org/10.1029/2022MS003226, 2022. a, b, c
Sullivan, S. C. and Voigt, A.: Ice microphysical processes exert a strong control on the simulated radiative energy budget in the tropics, Commun. Earth Environ., 2, 137, https://doi.org/10.1038/s43247-021-00206-7, 2021. a, b, c, d
Sullivan, S. C., Lee, D., Oreopoulos, L., and Nenes, A.: Role of updraft velocity in temporal variability of global cloud hydrometeor number, P. Natl. Acad. Sci. USA, 113, 5791–5796, https://doi.org/10.1073/pnas.1514039113, 2016. a
Sullivan, S. C., Hoose, C., and Nenes, A.: Investigating the contribution of secondary ice production to in-cloud ice crystal numbers, J. Geophys. Res.-Atmos., 122, 9391–9412, https://doi.org/10.1002/2017JD026546, 2017. a
Sullivan, S. C., Hoose, C., Kiselev, A., Leisner, T., and Nenes, A.: Initiation of secondary ice production in clouds, Atmos. Chem. Phys., 18, 1593–1610, https://doi.org/10.5194/acp-18-1593-2018, 2018. a
Sullivan, S. C., Schiro, K. A., Stubenrauch, C., and Gentine, P.: The Response of Tropical Organized Convection to El Niño Warming, J. Geophys. Res.-Atmos., 124, 8481–8500, https://doi.org/10.1029/2019JD031026, 2019. a, b
Sweeney, A., Fu, Q., Pahlavan, H. A., and Haynes, P.: Seasonality of the QBO Impact on Equatorial Clouds, J. Geophys. Res.-Atmos., 128, e2022JD037737, https://doi.org/10.1029/2022JD037737, 2023. a, b
Thuburn, J. and Craig, G. C.: On the temperature structure of the tropical substratosphere, J. Geophys. Res., 107, 4017, https://doi.org/10.1029/2001JD000448, 2002. a
Tiedtke, M.: Representation of Clouds in Large-Scale Models, Mon. Weather Rev., 121, 3040–3061, https://doi.org/10.1175/1520-0493(1993)121<3040:ROCILS>2.0.CO;2, 1993. a
Tobin, I., Bony, S., and Roca, R.: Observational Evidence for Relationships between the Degree of Aggregation of Deep Convection, Water Vapor, Surface Fluxes, and Radiation, J. Climate, 25, 6885–6904, https://doi.org/10.1175/JCLI-D-11-00258.1, 2012. a
Tobin, I., Bony, S., Holloway, C. E., Grandpeix, J.-Y., Sèze, G., Coppin, D., Woolnough, S. J., and Roca, R.: Does convective aggregation need to be represented in cumulus parameterizations?, J. Adv. Model. Earth Syst., 5, 692–703, https://doi.org/10.1002/jame.20047, 2013. a
Tselioudis, G., Rossow, W. B., Jakob, C., Remillard, J., Tropf, D., and Zhang, Y.: Evaluation of Clouds, Radiation, and Precipitation in CMIP6 Models Using Global Weather States Derived from ISCCP-H Cloud Property Data, J. Climate, 34, 7311–7324, https://doi.org/10.1175/JCLI-D-21-0076.1, 2021. a
Tseng, H. H. and Fu, Q.: Temperature Control of the Variability of Tropical Tropopause Layer Cirrus Clouds, J. Geophys. Res.-Atmos., 122, 11,062–11,075, https://doi.org/10.1002/2017JD027093, 2017. a, b
Tsushima, Y., Iga, S.-i., Tomita, H., Satoh, M., Noda, A. T., and Webb, M. J.: High cloud increase in a perturbed SST experiment with a global nonhydrostatic model including explicit convective processes, J. Adv. Model. Earth Syst., 6, 571–585, https://doi.org/10.1002/2013MS000301, 2014. a
Turbeville, S. M., Nugent, J. M., Ackerman, T. P., Bretherton, C. S., and Blossey, P. N.: Tropical Cirrus in Global Storm-Resolving Models: 2. Cirrus Life Cycle and Top-of-Atmosphere Radiative Fluxes, Earth Space Sci., 9, e2021EA001978, https://doi.org/10.1029/2021EA001978, 2022. a, b, c
Twohy, C. H., Anderson, B. E., Ferrare, R. A., Sauter, K. E., L'Ecuyer, T. S., van den Heever, S. C., Heymsfield, A. J., Ismail, S., and Diskin, G. S.: Saharan Dust, Convective Lofting, Aerosol Enhancement Zones and Potential Impacts on Ice Nucleation in the Tropical Upper Troposphere, J. Geophys. Res.-Atmos., 122, 8833–8851, https://doi.org/10.1002/2017JD026933, 2017. a
Ueyama, R., Jensen, E. J., and Pfister, L.: Convective Influence on the Humidity and Clouds in the Tropical Tropopause Layer During Boreal Summer, J. Geophys. Res.-Atmos., 123, 7576–7593, https://doi.org/10.1029/2018JD028674, 2018. a
Ullrich, R., Hoose, C., Möhler, O., Niemand, M., Wagner, R., Höhler, K., Hiranuma, N., Saathoff, H., and Leisner, T.: A New Ice Nucleation Active Site Parameterization for Desert Dust and Soot, J. Atmos. Sci., 74, 699–717, https://doi.org/10.1175/JAS-D-16-0074.1, 2017. a
van den Heever, S., Haddad, Z., Tanelli, S., Stephens, G., Posselt, D., Kim, Y., Brown, S., Braun, S., Grant, L., Kollias, P., Luo, Z. J., Mace, G., Marinescu, P., Padmanabhan, S., Partain, P., Petersent, W., Prasanth, S., Rasmussen, K., Reising, S., and Schumacher, C. and the INCUS Mission team: The INCUS Mission, EGU General Assembly 2022, Vienna, Austria, 23–27 May 2022, EGU22-9021, https://doi.org/10.5194/egusphere-egu22-9021, 2022. a
van Diedenhoven, B., Fridlind, A. M., Cairns, B., Ackerman, A. S., and Yorks, J. E.: Vertical variation of ice particle size in convective cloud tops, Geophys. Res. Lett., 43, 4586–4593, https://doi.org/10.1002/2016GL068548, 2016. a
van Diedenhoven, B., Ackerman, A. S., Fridlind, A. M., Cairns, B., and Riedi, J.: Global Statistics of Ice Microphysical and Optical Properties at Tops of Optically Thick Ice Clouds, J. Geophys. Res.-Atmos., 125, 1–21, https://doi.org/10.1029/2019JD031811, 2020. a
Virts, K. S., Wallace, J. M., Fu, Q., and Ackerman, T. P.: Tropical Tropopause Transition Layer Cirrus as Represented by CALIPSO Lidar Observations, J. Atmos. Sci., 67, 3113–3129, https://doi.org/10.1175/2010JAS3412.1, 2010. a
Voigt, A. and Shaw, T. A.: Impact of regional atmospheric cloud radiative changes on shifts of the extratropical jet stream in response to global warming, J. Climate, 29, 8399–8421, https://doi.org/10.1175/JCLI-D-16-0140.1, 2016. a
Voigt, A., Albern, N., and Papavasileiou, G.: The atmospheric pathway of the cloud-radiative impact on the circulation response to global warming: Important and uncertain, J. Climate, 32, 3051–3067, https://doi.org/10.1175/JCLI-D-18-0810.1, 2019. a, b, c
Voigt, A., Albern, N., Ceppi, P., Grise, K., Li, Y., and Medeiros, B.: Clouds, radiation, and atmospheric circulation in the present-day climate and under climate change, Wiley Interdisciplinary Reviews: Climate Change, 12, 1–22, https://doi.org/10.1002/wcc.694, 2021. a
Wall, C. J., Hartmann, D. L., Thieman, M. M., Smith, W. L., and Minnis, P.: The Life Cycle of Anvil Clouds and the Top-of-Atmosphere Radiation Balance over the Tropical West Pacific, J. Climate, 31, 10059–10080, https://doi.org/10.1175/JCLI-D-18-0154.1, 2018. a, b
Wall, C. J., Norris, J. R., Gasparini, B., Smith Jr., W. L., Thieman, M. M., and Sourdeval, O.: Observational Evidence that Radiative Heating Modifies the Life Cycle of Tropical Anvil Clouds, J. Climate, 33, 8621–8640, https://doi.org/10.1175/JCLI-D-20-0204.1, 2020. a, b, c
Wang, J., Fan, J., Feng, Z., Zhang, K., Roesler, E., Hillman, B., Shpund, J., Lin, W., and Xie, S.: Impact of a New Cloud Microphysics Parameterization on the Simulations of Mesoscale Convective Systems in E3SM, J. Adv. Model. Earth Syst., 13, e2021MS002628, https://doi.org/10.1029/2021MS002628, 2021. a
Waugh, D. and Hall, T.: Age of stratospheric air: theory, observations, and models, Rev. Geophys., 40, 1–26, https://doi.org/10.1029/2000RG000101, 2002. a
Wernli, H., Boettcher, M., Joos, H., Miltenberger, A. K., and Spichtinger, P.: A trajectory-based classification of ERA-Interim ice clouds in the region of the North Atlantic storm track, Geophys. Res. Lett., 43, 1–8, https://doi.org/10.1002/2016GL068922., 2016. a
Weverberg, K. V., Vogelmann, A. M., Lin, W., Luke, E. P., Cialella, A., Minnis, P., Khaiyer, M., Boer, E. R., and Jensen, M. P.: The Role of Cloud Microphysics Parameterization in the Simulation of Mesoscale Convective System Clouds and Precipitation in the Tropical Western Pacific, J. Atmos. Sci., 70, 1104–1128, https://doi.org/10.1175/JAS-D-12-0104.1, 2013. a
Williams, I. N. and Pierrehumbert, R. T.: Observational evidence against strongly stabilizing tropical cloud feedbacks, Geophys. Res. Lett., 44, 1503–1510, https://doi.org/10.1002/2016GL072202, 2017. a
Wing, A. A. and Cronin, T. W.: Self-aggregation of convection in long channel geometry, Q. J. Roy. Meteorol. Soc., 142, 1–15, https://doi.org/10.1002/qj.2628, 2016. a
Wing, A. A. and Emanuel, K. A.: Physical mechanisms controlling self-aggregation of convection in idealized numerical modeling simulations, J. Adv. Model. Earth Syst., 6, 59–74, https://doi.org/10.1002/2013MS000269, 2014. a
Wing, A. A., Reed, K. A., Satoh, M., Stevens, B., Bony, S., and Ohno, T.: Radiative–convective equilibrium model intercomparison project, Geosci. Model Dev., 11, 793–813, https://doi.org/10.5194/gmd-11-793-2018, 2018. a
Wing, A. A., Stauffer, C. L., Becker, T., Reed, K. A., Ahn, M., Arnold, N. P., Bony, S., Branson, M., Bryan, G. H., Chaboureau, J., Roode, S. R., Gayatri, K., Hohenegger, C., Hu, I., Jansson, F., Jones, T. R., Khairoutdinov, M., Kim, D., Martin, Z. K., Matsugishi, S., Medeiros, B., Miura, H., Moon, Y., Müller, S. K., Ohno, T., Popp, M., Prabhakaran, T., Randall, D., Rios‐Berrios, R., Rochetin, N., Roehrig, R., Romps, D. M., Ruppert, J. H., Satoh, M., Silvers, L. G., Singh, M. S., Stevens, B., Tomassini, L., Heerwaarden, C. C., Wang, S., and Zhao, M.: Clouds and Convective Self‐Aggregation in a Multi‐Model Ensemble of Radiative‐Convective Equilibrium Simulations, J. Adv. Model. Earth Syst., 12, e2020MS00213, https://doi.org/10.1029/2020MS002138, 2020. a, b, c, d, e
Winker, D. M., Pelon, J., Coakley, J. A., Ackerman, S. A., Charlson, R. J., Colarco, P. R., Flamant, P., Fu, Q., Hoff, R. M., Kittaka, C., Kubar, T. L., Le Treut, H., McCormick, M. P., Mégie, G., Poole, L., Powell, K., Trepte, K., Vaughan, M. A., and Wielicki, B. A.: The Calipso Mission: A Global 3D View of Aerosols and Clouds, B. Am. Meteorol. Soc., 91, 1211–1229, https://doi.org/10.1175/2010BAMS3009.1, 2010. a, b
Wolf, V., Kuhn, T., Milz, M., Voelger, P., Krämer, M., and Rolf, C.: Arctic ice clouds over northern Sweden: microphysical properties studied with the Balloon-borne Ice Cloud particle Imager B-ICI, Atmos. Chem. Phys., 18, 17371–17386, https://doi.org/10.5194/acp-18-17371-2018, 2018. a
Wylie, D. P. and Woolf, H. M.: The Diurnal Cycle of Upper-Tropospheric Clouds Measured by GOES-VAS and the ISCCP, Mon. Weather Rev., 130, 171–179, https://doi.org/10.1175/1520-0493(2002)130<0171:TDCOUT>2.0.CO;2, 2002. a
Xie, S., Lin, W., Rasch, P. J., Ma, P.-L., Neale, R., Larson, V. E., Qian, Y., Bogenschutz, P. A., Caldwell, P., Cameron-Smith, P., Golaz, J.-C., Mahajan, S., Singh, B., Tang, Q., Wang, H., Yoon, J.-H., Zhang, K., and Zhang, Y.: Understanding Cloud and Convective Characteristics in Version 1 of the E3SM Atmosphere Model, J. Adv. Model. Earth Syst., 10, 1–27, https://doi.org/10.1029/2018MS001350, 2018. a
Yang, Q., Fu, Q., and Hu, Y.: Radiative impacts of clouds in the tropical tropopause layer, J. Geophys. Res.-Atmos., 115, 1–21, https://doi.org/10.1029/2009JD012393, 2010. a, b
Yi, B.: Diverse cloud radiative effects and global surface temperature simulations induced by different ice cloud optical property parameterizations, Sci. Rep., 12, 10539, https://doi.org/10.1038/s41598-022-14608-w, 2022. a
Yin, J. and Porporato, A.: Diurnal cloud cycle biases in climate models, Nat. Commun., 8, 2269, https://doi.org/10.1038/s41467-017-02369-4, 2017. a
Yuan, J. and Houze, R. A.: Global variability of mesoscale convective system anvil structure from A-train satellite data, J. Climate, 23, 5864–5888, https://doi.org/10.1175/2010JCLI3671.1, 2010. a, b
Zantedeschi, V., Falasca, F., Douglas, A., Strange, R., Kusner, M. J., and Watson-Parris, D.: Cumulo: A Dataset for Learning Cloud Classes, arxive, https://doi.org/10.48550/arXiv.1911.04227, 2022. a
Zelinka, M. D. and Hartmann, D. L.: Why is longwave cloud feedback positive?, J. Geophys. Res-.Atmos., 115, D16117, https://doi.org/10.1029/2010JD013817, 2010. a, b, c
Zelinka, M. D. and Hartmann, D. L.: The observed sensitivity of high clouds to mean surface temperature anomalies in the tropics, J. Geophys. Res.-Atmos., 116, 1–16, https://doi.org/10.1029/2011JD016459, 2011. a, b, c
Zelinka, M. D., Klein, S. A., Qin, Y., and Myers, T. A.: Evaluating Climate Models’ Cloud Feedbacks Against Expert Judgment, J. Geophys. Res.-Atmos., 127, e2021JD035198, https://doi.org/10.1029/2021JD035198, 2022. a
Zhang, A., Wang, Y., Zhang, Y., Weber, R. J., Song, Y., Ke, Z., and Zou, Y.: Modeling the global radiative effect of brown carbon: a potentially larger heating source in the tropical free troposphere than black carbon, Atmos. Chem. Phys., 20, 1901–1920, https://doi.org/10.5194/acp-20-1901-2020, 2020. a
Zhang, Y., Macke, A., and Albers, F.: Effect of crystal size spectrum and crystal shape on stratiform cirrus radiative forcing, Atmos. Res., 52, 59–75, https://doi.org/10.1016/S0169-8095(99)00026-5, 1999. a
Zhao, M., Golaz, J.-C., Held, I. M., Ramaswamy, V., Lin, S.-J., Ming, Y., Ginoux, P., Wyman, B., Donner, L. J., Paynter, D., and Guo, H.: Uncertainty in Model Climate Sensitivity Traced to Representations of Cumulus Precipitation Microphysics, J. Climate, 29, 543–560, https://doi.org/10.1175/JCLI-D-15-0191.1, 2016. a
Zhao, W., Peng, Y., Wang, B., Yi, B., Lin, Y., and Li, J.: Comparison of three ice cloud optical schemes in climate simulations with community atmospheric model version 5, Atmos. Res., 204, 37–53, https://doi.org/10.1016/J.ATMOSRES.2018.01.004, 2018. a
Zhou, C., Dessler, A. E., Zelinka, M. D., Yang, P., and Wang, T.: Cirrus feedback on interannual climate fluctuations, Geophys. Res. Lett., 41, 9166–9173, https://doi.org/10.1002/2014GL062095, 2014. a
Zhu, J., Penner, J. E., Garnier, A., Boucher, O., Gao, M., Song, L., Deng, J., Liu, C.-q., and Fu, P.: Decreased Aviation Leads to Increased Ice Crystal Number and a Positive Radiative Effect in Cirrus Clouds, AGU Advances, 3, e2021AV000546, https://doi.org/10.1029/2021AV000546, 2022. a
- Abstract
- Introduction
- How cirrus clouds are studied
- Anvil cirrus
- TTL cirrus
- Key challenges for the model representation of cirrus and aerosol–cirrus interactions
- The way forward
- Code and data availability
- Author contributions
- Competing interests
- Disclaimer
- Special issue statement
- Acknowledgements
- Financial support
- Review statement
- References
- Abstract
- Introduction
- How cirrus clouds are studied
- Anvil cirrus
- TTL cirrus
- Key challenges for the model representation of cirrus and aerosol–cirrus interactions
- The way forward
- Code and data availability
- Author contributions
- Competing interests
- Disclaimer
- Special issue statement
- Acknowledgements
- Financial support
- Review statement
- References