the Creative Commons Attribution 4.0 License.
the Creative Commons Attribution 4.0 License.
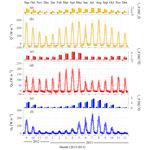
Surface energy balance fluxes in a suburban area of Beijing: energy partitioning variability
Junxia Dou
Sue Grimmond
Shiguang Miao
Bei Huang
Huimin Lei
Mingshui Liao
Measurements of radiative and turbulent heat fluxes for 16 months in suburban Miyun with a mix of buildings and agriculture allows the changing role of these fluxes to be assessed. Daytime turbulent latent heat fluxes (QE) are largest in summer and smaller in winter, consistent with the net all-wave radiation (Q*), whereas the daytime sensible heat flux (QH) is greatest in spring but smallest in summer rather than in winter, as commonly observed in suburban areas. The results have larger seasonal variability in energy partitioning compared to previous suburban studies. Daytime energy partitioning is between 0.15–0.57 for (mean summer = 0.16; winter = 0.46), 0.06–0.56 for (mean summer = 0.52; winter = 0.10), and 0.26–7.40 for (mean summer = 0.32; winter = 4.60). Compared to the literature for suburban areas, these are amongst the lowest and highest values. Results indicate that precipitation, irrigation, vegetation growth activity, and land use and land cover all play critical roles in the energy partitioning. These results will help to enhance our understanding of surface–atmosphere energy exchanges over cities and are critical to improving and evaluating urban canopy models needed to support integrated urban services that include urban planning to mitigate the adverse effects of urban climate change.
- Article
(12170 KB) - Full-text XML
-
Supplement
(1049 KB) - BibTeX
- EndNote
In the atmospheric boundary layer, turbulent flows are fundamental to mass and energy transport and are regarded as a basic part of comprehensive observation studies of the urban boundary layer, such as the UBL/CLU-ESCOMPTE project in Marseille, France (Mestayer et al., 2005); the BUBBLE project in Basel, Switzerland (Rotach et al., 2005); and the SURF experiment in Beijing, China (Liang et al., 2018). The eddy covariance (EC) method allows the direct measurement of heat and water vapor exchange between the surface and the atmosphere and is considered the best method to obtain turbulent fluxes (Baldocchi, 2003). Since the 1990s, EC methods are gradually undertaken in cities around the world. These measurements have provided information about surface energy exchanges and helped the development of parameterizations (Grimmond and Oke, 1999b, 2002; Järvi et al., 2019), and evaluation and application of land surface models (Grimmond et al., 2010; Järvi et al., 2011, 2014; Karsisto et al., 2015; Ward et al., 2016; Liu et al., 2017; Kim et al., 2019) and remote-sensing products (Kim and Kwon, 2019).
The EC method sites cover most of the local climate zones (LCZs) (Stewart and Oke, 2012), with building types from compact high rise, midrise, and low rise (LCZ1–LCZ3) to open high rise, midrise, and low rise (LCZ4–LCZ6) and large low rise (LCZ8) (Oke et al., 2017). The land use of these sites is mainly residential and commercial (Vesala et al., 2008; Bergeron and Strachan, 2012; Ao et al., 2016a; Roth et al., 2017; Hong et al., 2020) as well as including sites with institutions and universities (Guo et al., 2016), and industrial areas (Grimmond and Oke, 1999b; Offerle et al., 2006b). The ratio of impervious (e.g., buildings, parking lots, roads) to pervious (e.g., trees, grass) land cover in the EC source area spans a large range from 22 % / 78 % in a suburban residential site in Łódź, Poland (Offerle et al., 2006b) to 100 % / 0 % in a car park site in Basel, Switzerland (Christen and Vogt, 2004). The earliest observations were generally for short periods (a few days to several months) (e.g., Grimmond and Oke, 1995; Spronken-Smith, 2002). With more observational experience and recognition of the benefits of longer periods, funders have supported longer measurement periods. Measurements that cover a season, a year, or even many years (e.g., Christen and Vogt, 2004; Moriwaki and Kanda, 2004; Offerle et al., 2006a, b; Vesala et al., 2008; Ward et al., 2013; Kotthaus and Grimmond, 2014; Ao et al., 2016a; Roth et al., 2017; Tomoya and Masahito, 2017) provide many new insights. At a suburban site in the UK, for example, energy partitioning favors turbulent sensible heat during summer but latent heat in winter and is strongly dependent on land cover fractions (Ward et al., 2013). However, the seasonal variability in energy fluxes normalized by net radiation is relatively small in a residential neighborhood of Singapore, as the measurement site is in the equatorial region with a very small variability in the background climate (Roth et al., 2017).
According to the LCZs classification (Stewart and Oke, 2012), LCZ 1–4 sites are defined as “urban” and LCZ 5–9 sites as “suburban” in this study. If the surface is covered by more than 80 % vegetation (including trees, grass, crops, etc.), this observation station is referred to as a rural site. In Beijing, observations been taken in a densely built-up commercial and residential area (Liu et al., 2012; Miao et al., 2012; Wang et al., 2015), an open midrise residential to large agricultural area (Dou et al., 2019), and a rural area (Wang et al., 2015). These include observations for 1 year at the urban site individually and at the urban and rural sites concurrently. However, there is still a need to investigate seasonal variations in the suburban area, to understand the key factors impacting the surface energy balance at different timescales as, to date, only summertime observations have been analyzed (Dou et al., 2019).
In this study, daily, monthly, and seasonal variations in surface energy fluxes for a suburban site (Miyun) in Beijing are analyzed using 16 months of observations. We focus on the impact of site characteristics and precipitation on energy partitioning.
2.1 Site description
Miyun, located about 80 km northeast of the center of Beijing City (Tian'anmen Square) (Fig. 1a), has an area of 50 km2 and population of ∼500 000 (in 2019) (Miyun District Bureau of Statistics, 2020). The Miyun Meteorological Station (MY; 40∘22′39′′ N, 116∘51′51′′ E), on the southeast edge of Miyun City is in a transition zone between the city and the countryside (Fig. 1b and c). Using the Stewart and Oke (2012) local climate zone classification, the site is LCZ5 – the “Open midrise” class.
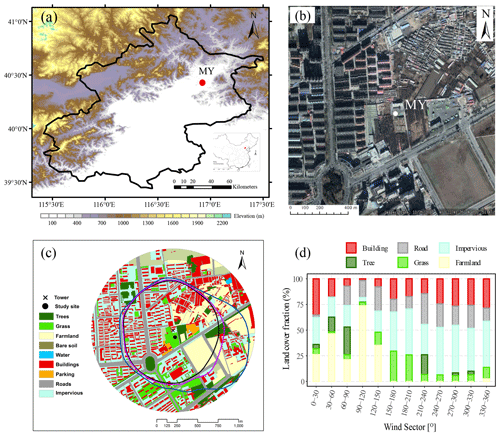
Figure 1Study area: (a) Beijing topography with Miyun (MY) (inset; location in China, red dot); (b) aerial view around MY flux tower (© Google Earth 2017); (c) land cover within 1 km radius around the MY flux tower (black cross) and 90 % eddy covariance source area for daytime ( W m−2, black line), night (blue), and daily (pink) averages; (d) daily mean land cover derived from a Gaofen-2 high-resolution image (CCRSDA, 2016) for the 30∘ wind sectors of the 90 % source area (shown in c).
Within a 1 km radius of the Miyun instrumented tower, the surface is 69.6 % impervious (20.6 % buildings, 14.6 % roads, and 34.3 % parking lots and pavement) and 30.3 % vegetation (15 % wheat–maize rotation farmland, 7.2 % trees, 8.1 % grass) based on an analysis of a Gaofen-2 high-resolution image (CCRSDA, 2016) with a spatial resolution of 1 m (Fig. 1c). To the east and southeast of the tower, there is farmland. The mainly residential six-story (∼18 m) buildings are at varying distances (west: from 170 m; northwest: >200 m; north: >70 m) of the tower. Other buildings include the farmer's one- to two-story house (3.5–7.3 m), which is about 300 m northeast of the tower. Since 2016, to the southwest (500-1000 m), newer, taller (18–34 m) residential buildings have been built. To the south (150–500 m away) are office (height: 8–50 m) and light commercial buildings (small shops, 8–12 m). Southwest of the tower, there is a roundabout that connects the two main roads in the study area: the east–west Jingmi Road (170 m to south) and the north–south Tanxi Road (420 m to west).
2.2 Instruments and data processing
Both the eddy covariance (EC) system and radiometer are mounted 36 m above ground level (agl) on a 38 m triangular lattice tower, with the EC system pointing in the prevailing wind direction (east) and the radiometer pointing south to avoid shadows. The EC system's three-dimensional sonic anemometer–thermometer (CSAT3, Campbell Scientific Inc., USA) measures vertical, along-wind, and crosswind velocity and virtual temperature; and the open-path infrared gas analyzer (LI-7500, LI-COR, Inc, USA) measures water vapor and carbon dioxide molar densities. The 10 Hz data are logged on a CR3000 data logger (Campbell Scientific Inc., USA). The 30 min turbulent sensible and latent heat fluxes are obtained using the EddyPro Advanced (v6.1.0 beta, LI-COR) software with standard correction procedures (Moncrieff et al., 1997) applied to ensure data quality (e.g., de-spiking raw data, tilt correction, time-lag compensation, double coordinate rotation, spectral corrections, and Webb et al. (1980) density corrections). Any 30 min period with a poor-quality flag (i.e., 2; LI-COR, 2023; N=1552 (6.6 %) of QH and 1987 (8.5 %) of QE) is excluded from this analysis. Data are excluded during rain and 2 h after rain (N=615 (1.4 %)). Wind direction data are corrected for changes in magnetic declination as the anemometer was installed with respect to magnetic north.
The radiation fluxes, measured with a CNR4 radiometer (Kipp & Zonen, the Netherlands), are 1 min samples by the CR3000 data logger, from which the 30 min means are calculated. The incoming and outgoing longwave (L↓ and L↑) and shortwave (K↓ and K↑) radiation and net all-wave radiation (Q*) data are restricted to physically reasonable thresholds, with nocturnal shortwave radiation forced to 0 W m−2 (Michel et al., 2008).
On the MY tower four levels (10.0, 17.2, 24.2, 36.0 m) of air temperature and relative humidity (HMP45C, Vaisala, Finland) and wind speed (010C, Met One Instruments, Inc., USA) and two levels (10.0, 36.0 m) of wind direction (020C, Met One Instruments, Inc., USA) are measured.
During the 16-month period analyzed (September 2012–December 2013), after instrument failures (14 January to 18 February 2013), 91.6 % (N=21 416; 30 min periods) of the radiation, 78.9 % (N=18 453) of sensible heat (QH), and 77.3 % (N=18 059) of the latent heat flux (QE) data are available.
Additional observations analyzed are from an automatic weather station located 30 m from the EC tower. The variables include air temperature (HMP155, Vaisala, Finland) measured at 1.5 m a.g.l.; precipitation from a tipping bucket rain gauge (SL3-1, Shanghai Meteorological Instrument Factory, China) mounted at 0.7 m a.g.l (April to October) and from a weighing bucket rain gauge (DSC1, Aerospace Newsky Technology, China) mounted at 1.5 m a.g.l. (November to March); wind speed and direction (ZQZ-TF, Aerospace Newsky Technology, China) measured at 10 m a.g.l.; and soil temperature (ZQZ-TW, Aerospace Newsky Technology, China) measured at 0, 0.05, 0.10, 0.20, 0.40, 0.80, 1.6, and 3.2 m below ground. These data are sampled at 1 s, except for wind speed and direction (0.25 s), and averaged to 1 min (WUSH-BH, Aerospace Newsky Technology, China). Hourly means (or accumulated totals for precipitation) are used here. Quality control checks include plausible range, internal consistency, temporal and spatial consistency, and other standard China Meteorological Administration network checks (Ren et al., 2015).
Soil moisture content is measured from gravimetric samples collected with a straight-shank drill with a scale. Measurements are taken on the 8th, 18th, and 28th of each month within 300 m of the site between 8 March and 28 November each year at five equally spaced depths (0.1 m interval to 0.5 m) in irrigated cropland and for the natural bare soil at five additional levels (i.e., 10 levels of 0.1 to 1 m).
2.3 Meteorological conditions during the study period
The study period (1 September 2012 to 31 December 2013) is compared to normal (1991–2020) MY weather station data. The range in mean 2 m air temperature is between −13.3 ∘C (23 December 2012) and 29.4 ∘C (17 August 2013) at the daily scale and between −7.0 ∘C (January 2013) to 26.1 ∘C (July 2013) for monthly scale (Fig. 2a). There are only small deviations from the norm in the monthly mean air temperatures, with the biggest differences (December 2012, April 2013) both 3.1 ∘C cooler than normal.
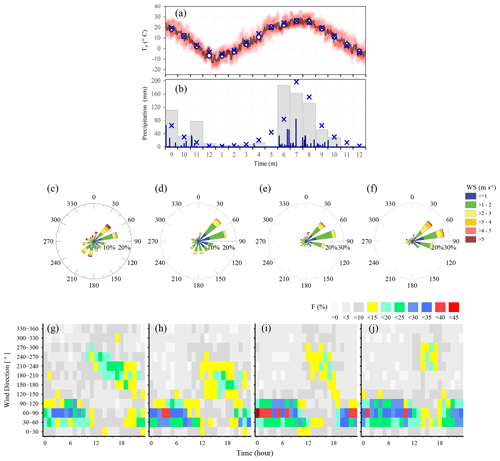
Figure 2Monthly normal (1991–2020, blue crosses) and automated weather station data (September 2012 to December 2013): (a) 2 m air temperature (Ta ) as 1 h (dots), daily (solid line), monthly averages (white circles); (b) daily (blue), monthly (grey) precipitation; wind roses (30∘ bins, 1 h data) stratified by wind speed frequency for (c) spring (MAM), (d) summer (JJA), (e) autumn (SON), (f) winter (DJF); frequency distribution of wind direction (30∘, 1 h data) by time of day for (g) MAM, (h) JJA, (i) SON, and (j) DJF.
The study period precipitation differed significantly from normal conditions in the region (Fig. 2b). The year 2012 was extremely wet, especially on a monthly basis with November (77.4 mm) and December (10.6 mm) about 580 % and 400 % greater than the norm (13.4 mm, November; 2.7 mm, December), respectively. In contrast, most of 2013 had below-average rainfall, except for June, which was wetter (186.7 mm, 223 % of the norm). Notably, dry spells occurred in May, November, and December 2013. Only 0.7 mm (1.6 % of the norm) of rain fell in May, and there was no rain in November and December 2013 at all.
Easterly winds (30–120∘) prevailed at night and the whole day for every season in MY, with a frequency of 50 % and 38 % in spring, 54 % and 43 % in summer, 69 % and 54 % in autumn, and 72 % and 62 % in winter, respectively (Fig. 2c–j). During the daytime, wind also came from the southwest direction (180–270∘) because of the mountain–valley breeze, despite the southwest wind differing at the start and end times of the seasons (Fig. 2g–j). In addition, the strongest winds (wind speed > 5 m s−1) mainly came from the northeast (30–60∘), with a higher relative frequency in spring and winter (Fig. 2c–f).
2.4 EC footprint analyses
To calculate the turbulent flux source areas for each 30 min period, we use the Kljun et al. (2004) EC footprint model. The roughness length for momentum (z0) and zero plane displacement height (zd) input parameters is based on the height of roughness elements within a 1 km radius of the EC tower. The buildings vary from 3.0 to 50.4 m. The farmers' houses range from 3.5–7.3 m to the northeast of the tower. The residential buildings (six floors) are to the west, northwest, and north of the tower, with consistent heights of 16.0–18.5 m. The height of buildings to the south, southwest, and north of the tower varies greatly, but most of the buildings exceed 20 m. The tallest building (50.4 m) is 380 m south of the tower. The mean, weighted by the plan area fraction of building height (zh), is 13.1 m. The trees are along roadsides, within the median strips of roundabouts, public gardens, and orchards (Fig. 1c). The average tree height is 8.5 m (trees > 1.5 m), varying between 5.0–21.0 m with tree species differences. The fruit trees in the orchards (northeast and east of the tower) are a relatively consistent 7.2 to 9.3 m (average = 8.6 m). The mature wheat (June) is 1.1 m and maize (September) is 2.7 m. Using a rule of thumb (Grimmond and Oke, 1999a) with a mean building height of 13.1 m, z0 is estimated to be 1.3 m and zd is 9.2 m, whereas using Kanda et al. (2013) for the 36–10∘ sectors gives values between 0 and 3.26 m (z0) and between 0 and 36.4 m (zd). Anemometric estimates are 2.9 m (z0) and 4.1 m (zd) (Dou et al., 2019). Given that prevailing wind is from the east (30–150∘) where vegetation fraction (λv) occupies 60 % of the plan area (≤1 km radius of EC tower), anemometric values are used. By using the iterative method suggested by Kent et al. (2017), the impact of these initial values on the probable turbulent flux source dimensions should become insignificant.
The atmospheric stability parameter () is a function of the Obukhov length (L) obtained from the EC observations, the sensor height (z) and zd. Here the variation in turbulence is classified as unstable (), neutral (), and stable conditions (ζ>0.1). During the observation period, after quality control (N=17 142; 30 min periods), the stability is predominately either unstable (40.9 %) or stable (42.4 %), with neutral conditions for 16.7 % of the time.
Footprints during unstable and neutral conditions are analyzed together by wind direction (5∘ sectors) but split into day ( W m−2) and night. The median 90 % source area extends to 582–677 m (by direction) during the daytime and 596–908 m at night (Fig. 1c), which corresponds to a daily median of 559–676 m. Within these source areas, the land use and land cover vary by sector from being more highly vegetated (30–150∘) to more built-up (210–360∘) (Fig. 1d).
2.5 Anthropogenic heat flux
The LQF version (Lindberg et al., 2018; Gabey et al., 2019) of the Large-scale Urban Consumption of energY (LUCY) model (Allen et al., 2011; Lindberg et al., 2013) is used to calculate anthropogenic heat flux (QF). The temperature response coefficients are calculated for MY using local air temperature and electricity consumption, vehicle, and population data (Appendix A).
2.6 Storage heat flux
Given the difficulty of measuring the storage heat flux (ΔQs) directly in urban areas (e.g., Offerle et al., 2006b; Roberts et al., 2006), we use two methods to estimate it.
-
Objective Hysteresis Model ΔQs,ohm: see Appendix B for details.
-
Energy balance residual : includes the uncertainty in other terms in the equation. On the one hand, the EC fluxes (QH and QE) underestimated by 10 %–20 % (Wilson et al., 2002; Foken, 2008), and on the other hand, given the prevailing easterly winds (30–150∘) (Fig. 2c–j), vegetation accounts for 60 % of the area of which 44 % is cropland (Fig. 1c and d) if QF is included, the ΔQs,res values should be regarded as the upper limit of heat storage flux (Ward et al., 2013).
2.7 Data availability and analysis of fluxes
Analysis is done by season (spring: March–May (MAM); summer: JJA; autumn: SON; and winter: DJF) and by time of day (daytime: W m−2, night: other times).
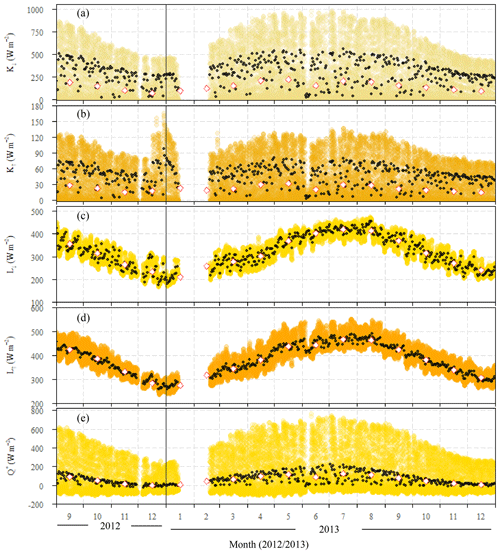
Figure 3Observed 30 min (color), daily (black), and monthly (white diamonds) means at Miyun (September 2012 to December 2013) of (a) incoming shortwave radiation (K↓), (b) outgoing shortwave radiation (K↑), (c) incoming longwave radiation (L↓), (d) outgoing longwave radiation (L↑), and (e) net all-wave radiation (Q*). The data gap in January and February 2013 is due to instrument failure. For the daily fluxes, daytime values ( W m−2) are given for the shortwave fluxes, but 24 h values are given for the others.
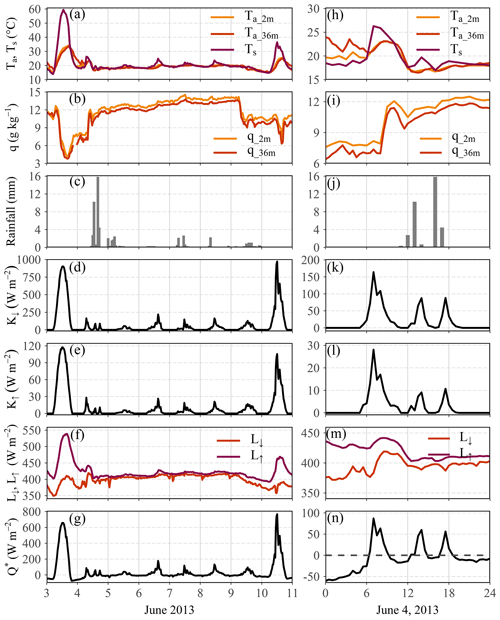
Figure 4Time series of (a, h) air temperature at 2 and 36 m and surface temperature, (b, i) specific humidity at 2 and 36 m, (c, j) rainfall, (d, k) incoming shortwave radiation, (e, l) outgoing shortwave radiation, (f, m) incoming and outgoing longwave radiation, and (g, n) net radiation for (a–g) 3–10 June 2013 and (h–n) 4 June 2013 at Miyun. Rainfall has a 1 h resolution, and the other data have a 30 min resolution.
Missing data are not gap-filled. The mean values of radiation and turbulent fluxes for each half hour during a day in the month (season) are first calculated to get their mean diurnal patterns. Then the daytime ( W m−2) or daily (24 h) mean values are averaged from corresponding periods within the mean diurnal patterns. The daytime (daily) mean ratios are the ratios of daytime (daily) mean values of corresponding radiation and energy fluxes.
3.1 Surface radiation budget
At the MY site, daytime maxima of incoming shortwave radiation (K↓) range from about 500 W m−2 in winter to 1000 W m−2 in summer (Fig. 3a). As expected, daytime maxima of K↓ in winter are greater than those for higher-latitude cities (e.g., London, 52∘ N, ∼200 W m−2; Kotthaus and Grimmond, 2014) and smaller than for lower-latitude cities (e.g., Shanghai, 31.19∘ N, ∼600 W m−2; Ao et al., 2016b), owing to differences in the solar elevation angle. In summer at MY, the daytime maxima of K↓ is similar to London (Kotthaus and Grimmond, 2014) but slightly higher than for Shanghai (∼800 W m−2; Ao et al., 2016b). This may be attributable to the higher humidity reducing K↓, exceeding the latitudinal differences. The extremely low K↓ values are associated with precipitation, such as recorded in early June 2013 (Fig. 3a). With rain everyday from 4 to 9 June, the K↓ maxima were <220 W m−2 (Fig. 4c and d). K↓ was 0 W m−2 between 11:00-12:00 and 16:00 LT on 4 June during rainfall (Fig. 4j and k).
The outgoing reflected shortwave radiation (K↑) daytime maxima vary between 65 W m−2 (December) and 120 W m−2 (July and August). However, some observations in December 2012 and January 2013 exceed 120 W m−2 and even 150 W m−2 (Fig. 3b) when snow occurred causing albedo to increase to 0.6 and then decrease with the days since snowfall (Fig. 5). When there is no snow on the ground, an asymmetry in the albedo still exists. With smaller solar elevation, the asymmetry is more pronounced (Fig. S1 in the Supplement). Surface heterogeneity is thought to explain this as there is a basketball court in the field of view of the radiometer (southeast, Fig. S1a), and albedo will differ from impervious and vegetation surfaces in other parts of the field of view (Fig. S1a), including specular reflection from glass. The building with windows is ∼9 m north of the tower, increasing the albedo before noon (cf. afternoon) (Fig. S1b and c). During the spring, summer, and autumn, daily midday (10:00–14:00 LT) albedo was between 0.128 and 0.209 in 2012 (0.113–0.209, 2013), while in winter in 2012 (2013) it reached 0.155–0.478 (0.134–0.293) with the variations in snow cover. Overall, the representative daytime (sunrise to sunset) local-scale albedo was 0.148, which is slightly larger than the midday (10:00–14:00 LT) value (0.145).
Incoming longwave radiation (L↓) is primarily influenced by near-surface air temperature and water vapor content (Flerchinger et al., 2009; Kotthaus and Grimmond, 2014). Thus, the larger L↓ values are observed during warm and humid times of the year (i.e., summer, June–August) (Fig. 3c), and smaller values are observed in the colder winter. The daily maxima of L↓ vary between ∼320 W m−2 (winter) and ∼470 W m−2 (summer). The monthly mean L↓ varies between 420 W m−2 (July) and 212 W m−2 (January 2013). The latter was the coldest month of the observation period (Sect. 2.3).
Outgoing longwave radiation (L↑) depends on the surface temperature and emissivity. The former is highly influenced by the total amount of incoming radiative energy. Thus, the larger L↑ is observed in summer (Fig. 3d), consistent with the larger K↓ and L↓. And vice versa, the lowest L↑, along with smaller K↓ and L↓, is measured in winter. The highest monthly mean L↑ of 467 W m−2 occurred in July and the smallest (275 W m−2) again in January 2013.
As the net all-wave radiation (Q*) is the net balance of the four radiation budget components, it is affected by sun elevation angle, sky conditions, and surface characteristics. The variation in Q* is similar to that in K↓, with daytime maxima of Q* being greater in summer and smaller in winter. Daily mean Q* values vary between −20 W m−2 (winter) and 222 W m−2 (summer), with large scatter seen from May to September (Fig. 3e). Hence, the largest monthly mean Q* is in summer, with the maximum value (126 W m−2) in July 2013. The smaller monthly mean Q* in June is attributed to its smaller K↓ caused by frequent rainfall. Notably, the 4 June's extremely low K↓ results in a daily mean Q* that is <0 W m−2 (Fig. 4n). With longer winter nights the monthly mean Q* is small or even negative. Notably, the minimum value during the observation period is in December 2012 when snow is present (i.e., decreasing K↓) resulting in a monthly mean of only −0.5 W m−2 (Fig. 3e).
3.2 Anthropogenic heat (QF) and storage heat (ΔQS) fluxes
The median QF values vary between 5 and 39 W m−2 diurnally across all of the months (Fig. 6). Heat released from buildings (QF,B) dominated QF, with its diurnal median value ranging from 4.6 to 36.4 W m−2 and accounting for 85 %–95 % of QF (Fig. S2a). The contribution of vehicle emission (QF,V) is small (1 %–9 % of QF). The maximum QF,V value during the morning rush hour (08:00–09:00 LT) is only 1.5 W m−2, since the house is usually close to the work place or school and residents do not rely on cars for daily travel or commuting at MY (Fig. S2b). The diurnal variation in the human metabolism (QF,M) is constant throughout a year because of the fixed population density (5657 people km−2 in 2012 and 5702 people km−2 in 2013). Like in other studies, QF,M is small, with values ranging between 0.4–1 W m−2 and contributions being 5 %–8 %.
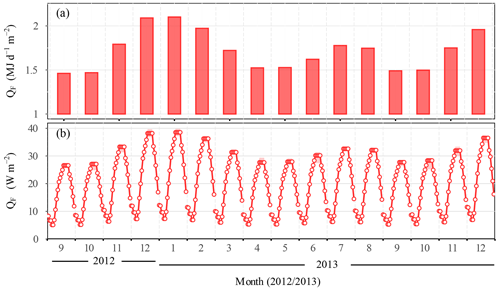
Figure 6Monthly anthropogenic heat flux (QF) at Miyun (September 2012 to December 2013) calculated as described in Sect. 2.2: (a) mean total for 24 h and (b) median diurnal patterns with inter-quartile range (IQR) (shading).
The fluxes are larger in summer and winter associated with cooling and heating needs, respectively. Our QF,B values are larger than estimated at other suburban sites, such as Montréal during winter (Bergeron and Strachan, 2012) and Swindon (Ward et al., 2013); even the air temperature in Montréal is lower in winter. The reason is that Miyun has a greater mean building height (13.1 m) and building cover (21 %). Moreover, buildings include hospitals and office buildings, which usually have greater energy consumption and emissions than residential buildings. However, our QF,V values are lower than those reported in Montréal and Swindon, due to the small number of motor vehicles and various travel modes at Miyun.
The monthly mean QF values vary between 17 and 24 W m−2 (daily totals = 1.46–2.10 MJ d−1 m−2). These suburban values are larger than those reported in other suburban residential areas, such as 10–12 W m−2 in Montréal during winter (Bergeron and Strachan, 2012) and 6–10 W m−2 in Swindon (Ward et al., 2013). However, these suburban Beijing values are much less than in Beijing city center (∼130 W m−2, Wang et al., 2020). Hence, the MY values appear to be reasonable.
The storage heat fluxes are determined using two methods (Sect. 2.6). Generally, storage heat flux is expected to have a net gain in summer and net loss in winter, giving almost zero net heat gain/release over the annual period (Grimmond et al., 1991). From this point of view, ΔQs,ohm has a credible annual variation in daily total values (Fig. 7a), but the annual total value of ΔQs,ohm was 352.8 MJ m−2 in 2013, indicating that ΔQs,ohm is overestimated.
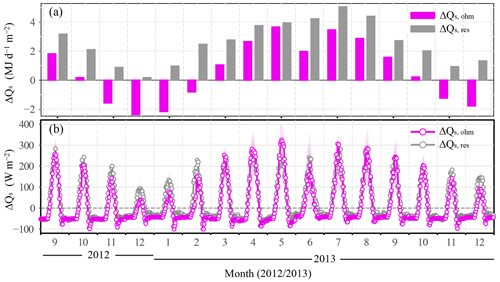
Figure 7Miyun (September 2012 to December 2013) monthly storage heat flux (ΔQS) determined using two methods (color, Sect. 2.5): (a) 24 h mean and (b) diurnal patterns median with inter-quartile range (IQR) (shading).
In spring and summer, there is little difference in daytime values of ΔQs,ohm and ΔQs,res except for May 2013 (Fig. 7b), indicating an overestimation of ΔQs,ohm. The May high ΔQs,ohm is the result of both fewer rain events and more radiative input (Sects. 2.3 and 3.1; Figs. 2b and 3a), as it depends on the net radiation value according to the objective hysteresis model (OHM) equation. Meanwhile, farmland irrigation prompts more energy to be used for evaporation (Sect. 3.4 and 3.5; Figs. 14 and 15), resulting in a decrease in ΔQs,res. This makes daytime ΔQs,ohm obviously higher than ΔQs,res in May. In addition, at MY, it is common to see sun after rain in spring and summer, which is similar to pavement/road watering on sunny days. Pavement heat flux (G) is reduced after watering, and the maximum value of G is only half of that without pavement watering under the same sunny conditions (Hendel et al., 2015). However, the OHM coefficients of road/pavement/impervious surface used in this study were fitted from dry surfaces under clear-sky conditions (Anandakumar, 1999; Doll et al., 1985; Wang et al., 2008), so it inevitably leads to an overestimation of ΔQs,ohm when it is sunny after rain. The comparison results of a MY daytime ΔQs,ohm value greater than ΔQs,res within 24 h after rain in spring and summer confirm this point (Fig. S3).
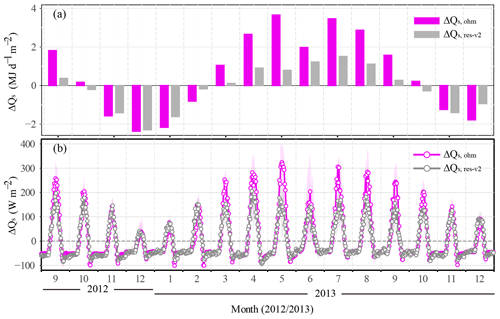
Figure 8Monthly storage heat flux (ΔQS,ohm and ΔQS,res-v2) at Miyun (September 2012 to December 2013) (Sect. 3.2): (a) mean flux for 24 h and (b) median diurnal patterns with inter-quartile range (IQR) (shading).
In autumn and winter (November–February), ΔQs,ohm is smaller than ΔQs,res during the day but larger (more negative) at night (Fig. 7b), similar to residential Swindon results (Ward et al., 2013). As the MY site has greater wintertime QF emissions, which directly impact ΔQs,res we make a second estimate which omits QF and assumes that the turbulent heat fluxes are underestimated by 20 % (Wilson et al., 2002; Foken, 2008) (). This gives a lower limit, with ΔQs,ohm smaller than ΔQs,res-v2 in winter (Fig. 8). This indicates that the winter ΔQs,ohm underestimates are related to OHM coefficients for the prevailing wind directions. As cropland covers a greater proportion in the easterly wind direction, it suggests that the wheat coefficients are poor in winter (Fig. B2d). This is because smaller fluxes are obtained from using the soil heat flux (QG) data. These relations to Q* do not account for those components of heat storage fluxes above the soil heat flux plate, such as ground heat storage and biomass heat storage (Meyers and Hollinger, 2004; Oliphant et al., 2004). They constitute an important proportion of the total storage heat flux. Except for cropland and roof surfaces, OHM coefficients of other land cover types are fitted from the spring or summer observation dataset, while these coefficients may not be applicable to autumn and winter and cause the ΔQs,ohm to be underestimated in autumn and winter. Long-term datasets covering seasonal changes and surface conditions, especially winter, can provide more OHM coefficients for use (Anandakumar, 1999; Ward et al., 2013).
In addition to the OHM coefficients, mismatching the source area between radiation and turbulent fluxes may also cause the uncertainty in the ΔQs,ohm estimation (Fig. S4). ΔQs,res is used in the following analyses, but it is clearly biased, as monthly mean daily total values are all positive throughout the year, even in winter (Fig. 7a).
3.3 Monthly diurnal variation in directly observed fluxes
Typically, Q*, turbulent sensible (QH), and latent heat fluxes (QE) each have a unimodal distribution in the year but with different seasonal peaks (Fig. 9). Monthly median diurnal maxima of Q* are higher from April to September, with the maximum value occurring in August, reaching 600 W m−2. The daytime median maximum Q* (450 W m−2) in June is slightly less than other months during this high-frequency rainfall period. The median diurnal Q* in December and January is obviously lower than other months, with a peak value of 180 W m−2 in December 2012 being the minimum value during the observation period. Nighttime Q* is around −20 W m−2 during summer and mostly lies between −80 and −60 W m−2 in other months. Overall, daytime values are greater than nighttime ones during the investigation period except for December 2012 (−0.04 MJ m−2 d−1), which results in monthly mean daily Q* having net positive values. These vary between 0.62 and 10.88 MJ m−2 d−1 (Fig. 9a and b).
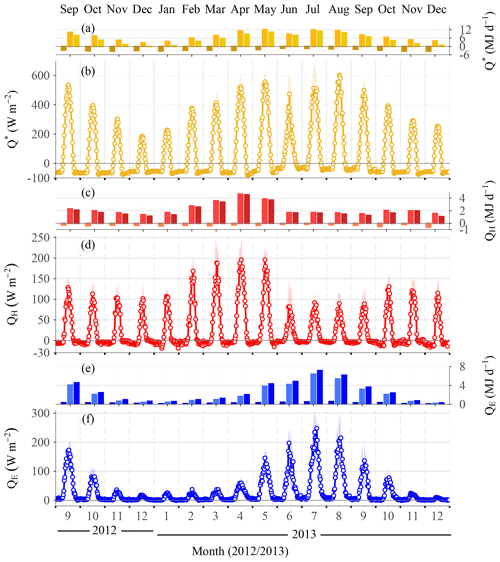
Figure 9Miyun (September 2012–December 2013) monthly fluxes of (a, c, e) total energy for night, day, and 24 h (left to right) and (b, d, f) diurnal median (line) and IQR (shading) for (a, b) net all-wave radiation Q*, (c, d) sensible heat flux QH, and (e, f) latent heat flux QE.
Sensible heat flux QH is greatest in spring (March–May) and smallest in June–September 2013 (Fig. 9c and d). The daytime median peak values for these two periods are close to 200 and ∼90 W m−2, respectively. In other months, daytime QH maximum values tend to be of between 100–130 W m−2, but in February 2013 they approach 170 W m−2. In previous suburban/urban studies of the East Asian monsoon region, daytime QH values are highest in spring (Institute of Atmospheric Physics – IAP, Beijing; Miao et al., 2012; Wang et al., 2015; Xianghe, Beijing, Wang et al., 2015; residential area in Seoul, Hong et al., 2020; Seoul Forest Park, Seoul, Lee et al., 2021) or summer (Tokyo, Moriwaki and Kanda, 2004; Shanghai, Ao et al., 2016a; Osaka, Ando and Ueyama, 2017), depending on whether spring is dry with little rain or warm humidity. Meanwhile, daytime QH values are lowest in winter when the least solar radiation in the year occurs, except for Xianghe; Beijing; and Seoul Forest Park, Seoul. Similar to MY, daytime QH values of these two sites are lowest in summer, with irrigated cropland/forest within the flux source area. Hence at MY, the summer daytime QH minima are attributed to the extensive irrigated cropland in the prevailing wind direction (Figs. 1c, d and 2d, h). This enhances available energy to support evaporation and transpiration and leads to smaller QH values (Dou et al., 2019). Notably, these characteristics are different from wheat–maize rotation farmland under the same climate background. The daytime QH values in farmland are lowest in spring, owing to sufficient irrigation and high water consumption for wheat growth (Fig. S5). Nocturnal QH is negative throughout the year, from a mean of −10.5 W m−2 in December (2013) to −4.3 W m−2 in July, which is similar to the values observed at more open suburban sites (Loridan and Grimmond 2012, their Table 2; Oke et al., 2017, their Fig. 6.25). At MY, daily total QH values on a monthly basis are smallest in winter, 1.07 MJ m−2 d−1 in December 2013, and largest in spring (4.53 MJ m−2 d−1, April 2013) (Fig. 9c). Although the daytime QH maxima are smallest in summer, the absolute values at night are smaller than in winter; thus the minimum daily total value occurs in winter.
The seasonal pattern of QE is driven by available energy, water supply (including precipitation and irrigation), and phenological variations. Along with the largest Q* and rainfall, as well as the strongest crop growth in summer, daytime QE peaks in July, with the maximum reaching 248 W m−2 (Fig. 9f). In winter, QE is lowest, with median diurnal values < 40 W m−2. In December 2013, during the long dry spell, with no precipitation from 20 October 2013 (Fig. 2b) and no irrigation (as crops are dormant, typically the last irrigation is before 15 November), the maximum value is only 10 W m−2. Previous suburban studies report summer and winter daytime QE maxima within this range, but at the higher and lower end, respectively (e.g., Grimmond and Oke, 2002; Spronken-Smith, 2002; Christen and Vogt, 2004; Moriwaki and Kanda, 2004; Goldbach and Kuttler, 2012; Dou et al., 2019). Similar to other urban and suburban studies, throughout the day QE is positive (Grimmond and Oke, 1995; Balogun et al., 2009; Ward et al., 2013; Ao et al., 2016b). At night QE is close to 0, being slightly larger during June–August (between 2–19 W m−2; mean 7–26 W m−2 during 20:00–00:00 LT).
Monthly totals of QE are between 0.32 and 7.16 MJ d−1 m−2 (Fig. 9e), with corresponding maximum and minimum monthly totals occurring in two seasons (summer, i.e., July, and winter, i.e., December 2013, respectively).
3.4 Surface energy balance partitioning
To facilitate the comparison of surface energy balance (SEB) partitioning between sites a series of average daily and daytime ( W m−2) flux ratios are analyzed. These include fluxes normalized by net all-wave radiation (Q*) and the Bowen ratio () (Figs. 10 and 11; Table 1). The ratios are calculated for the 30 min time period, allowing the distributions to be analyzed (Fig. 10), but also using monthly mean fluxes.
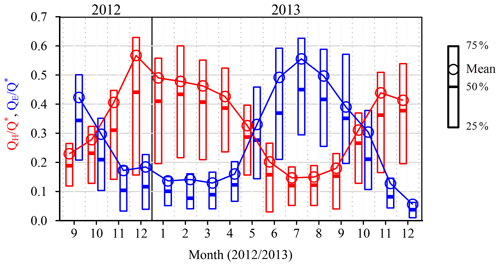
Figure 10Monthly median, inter-quartile range (IQR) and mean (30 min) of daytime ( W m−2) sensible heat flux QH and latent heat fluxes QE normalized by net all-wave radiation (Q*) at MY (September 2012 to December 2013).
Table 1Seasonal and annual mean fluxes and ratios for daytime ( W m−2) and daily (24 h) periods at the Miyun site. The table shows radiation fluxes, net all-wave radiation (Q*), incoming radiation (Q↓; ), incoming and outgoing shortwave radiation (K↓, K↑), incoming and outgoing longwave radiation (L↓ and L↑), albedo (, turbulent sensible and latent heat fluxes (QH, QE) and their ratios (radiative ratios; and Bowen ratio (), and storage heat flux calculated as . Anthropogenic heat flux QF calculated by the LQF version (Gabey et al., 2019; Lindberg et al., 2018) of the LUCY model (Allen et al., 2011; Lindberg et al., 2013).
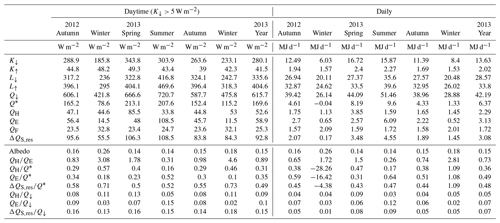
During the observation period, daytime QH is 15 %–57 % of Q* (Fig. 10). Unlike the absolute values (Sect. 3.3), is largest in winter and smallest in summer, whereas has the opposite shape with an annual peak in July (Fig. 10). It varies between 6 %–56 % during our study period.
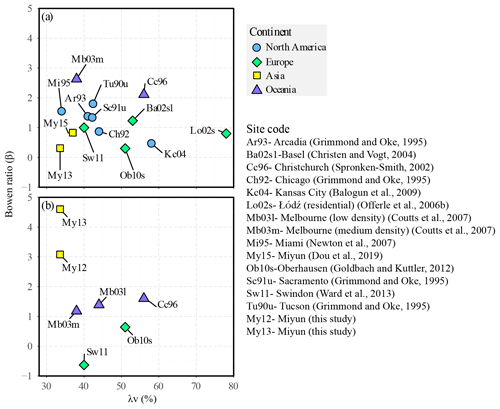
Figure 12Daytime (the definition of daytime is given in the corresponding publication) Bowen ratio during summer (a) and winter (b) versus vegetation fraction (λν) for MY and for various suburban sites in the literature (see the legend for references).
At MY, from November to April (Fig. 10), QH dominates the Q* ratio, whereas from June to September QE dominates the Q* ratio. For May and October, they have a similar ratio to Q* (Fig. 10). This partitioning pattern is different from that observed in dense residential Tokyo, where is always greater than throughout the year (Moriwaki and Kanda, 2004); it is also unlike the pattern reported at a suburban site (Oberhausen), where QE is almost always dominant (Goldbach and Kuttler, 2012). These pattern differences could be partly attributed to the different vegetation cover; i.e., λV=34 % at MY, 21 % at Tokyo, and 61 % at Oberhausen.
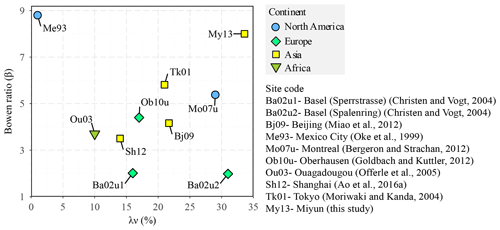
Figure 13As Fig. 12 but winter daytime Bowen ratio for MY and for various urban sites with a vegetation cover of 1 %–31 %. At Bi09, Ba02u1, Ba02u2, and Sh12, the Bowen ratio is the mean value of December–February. At My13, Me93, and Mo07u, it is the mean value of December. At Ob10u, the value is for January. At Ou03 and TK01, the value is for February. All Bowen ratios are accurately provided in the paper, except for Sh12 extracted from the plot and Mo07u extracted from ratios of and .
The MY June–August daytime ratios between 0.49–0.56 (mean summer = 0.52) are greater than at most suburban sites, including those with more vegetation coverage than MY, such as Chicago (; λV=44 %; Grimmond and Oke, 1995) and Basel (; λV=53 %; Christen and Vogt, 2004). Therefore, MY June–August daytime ratios (0.15–0.20; summer mean = 0.16) are lower in comparison to previous studies (Grimmond and Oke, 2002; Balogun et al., 2009; Goldbach and Kuttler, 2012; Dou et al., 2019). Frequent rainfall and irrigation, prevailing winds from farmland, and rapid crop growth are all believed to enhance the latent heat flux, leading to higher and smaller Bowen ratio values (Sect. 3.5), and they are consistent with a previous study at the MY (Dou et al., 2019).
In winter, MY daytime QH was 41 %–57 % of Q*, which is greater than at most suburban sites and some urban sites, including sites with larger plan area of buildings (λb), such as Tokyo ( %–40 %; λb=33 %; Moriwaki and Kanda, 2004), IAP Beijing ( %; λb=68.3 %; Miao et al., 2012), and Mexico City ( %; λb=32 %; Oke et al., 1999). However, the ratios (0.08–0.18) are comparable to those in Tokyo (–0.11; Moriwaki and Kanda, 2004) and slightly larger than that of IAP Beijing (; Miao et al., 2012). This suggests that the higher winter MY ratio could be attributed to the relatively lower amount of heat absorbed and stored by buildings (here ) due to smaller λb (18.9 %). The ΔQS is a small proportion of the Q* energy partitioning (–0.53).
The partitioning between QH and QE has clear seasonal variations, with a similar tendency to that of (Fig. 11, cf. 10). The daytime β varies between 0.26 and 7.40 through the year (Fig. 11). This range is much greater than at many sites, such as 1.38–5.81 at Tokyo (Moriwaki and Kanda, 2004) and 0.36–1.23 at Oberhausen (Goldbach and Kuttler, 2012). The MY summer and winter (2012 and 2013) daytime mean β values (summer 0.32 and winter 3.08 and 4.60) are at the lower and higher end of those at other suburban sites (Fig. 12). Precipitation plays a significant role in the magnitude and amplitude of β (see Sect. 3.5).
At the annual timescale, QH and QE are 31 % and 35 % of mean daytime Q* and 36 % and 49 % of the daily Q* (i.e., QE dominates) (Table 1). Similarly annual, daytime β is 0.89, and daily β is 0.73 (i.e., QE dominates).
3.5 Factors influencing energy balance fluxes
At MY, the impacts of precipitation on energy partitioning are obvious. As monthly rainfall from September to December in 2012 and 2013 is higher and lower than the norm (1991–2020), respectively, monthly daytime mean β values of September–December 2012 are correspondingly lower than those of the same period in 2013. Notably with no precipitation in November and December 2013, the β in December 2013 reaches 7.40. This is 2.4 times greater than the December 2012 (β=3.08). To our knowledge, this large β value is one of the highest observed not only in suburban areas (Fig. 12b), but also in the central part of cities (Fig. 13), but it is still less than the values observed in central London (β=5–10; Kotthaus and Grimmond, 2014) or Mexico City (β=8.8; Oke et al., 1999).
Irrigation supplements precipitation and plays an important role in energy partitioning (Grimmond and Oke, 1986; Grimmond et al., 1996; Kokkonen et al., 2018). At MY, winter wheat and summer maize are the two predominant crops and are cultivated in rotation. The growing season of winter wheat is from October to mid-June, while maize is planted in late June and harvested at the end of September every year. June and October are the intermittent months for the two crops. Irrigation is frequent during wheat growth in spring because of the drought and climatic conditions with little rain. As shown in Fig. 14, the soil moisture of natural conditions and cropland was almost the same at the end of April (28 April). With only 3 d (26, 27, and 28 May with 0.1, 0.4, and 0.2 mm, respectively) having rain in May 2013 (Fig. 2b), the soil moisture due to natural conditions decreases dramatically in May, whereas, by contrast, that of cropland increases. This indicates that cropland has irrigation to replenish water. For this reason, the May β value clearly presents a decreasing tendency even if there are more than 20 d of no rain (Fig. 15), as external water provided by cropland irrigation makes the available energy favor the latent heat flux. The monthly mean β of 0.50 in May (Fig. 11) clearly indicates the importance of this additional source of water.
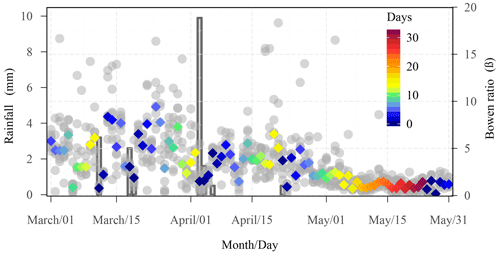
Figure 15Daytime (circles) and median 30 min daytime (diamonds) Bowen ratio by number of days since rainfall (colors) (right-hand axis) and daily rainfall (bars, left-hand axis) for 1 March to 31 May 2013.
Crop growth and phenology are also important influences. Transpiration during crop growth releases a large amount of water into the atmosphere observed as QE (e.g., Dou et al., 2019). The soil moisture in the irrigated cropland is higher than in the natural rainfed areas (Fig. 14). The gravimetric sample dates are critical relative to the timing of both irrigation and rainfall (e.g., 8 July 2013 compared to a few days later) as the soil moisture values can be inverted, as cropland utilizes stored soil water via transpiration and evaporation.
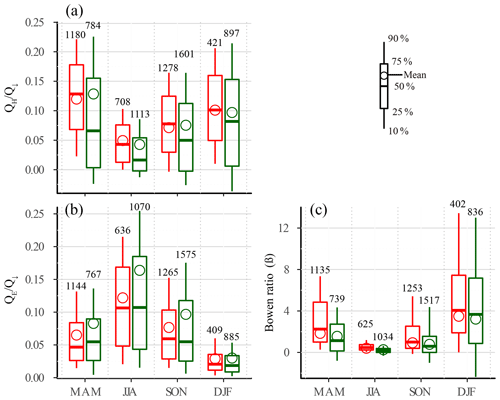
Figure 16Median, inter-quartile range (IQR), and mean flux ratio (30 min, number of periods indicated) by season when the wind is from the building- (red) and farmland-dominated (dark green) directions. (a) , (b) , and (c) β (see Table 1 for definitions).
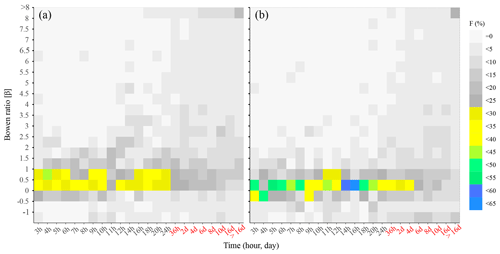
Figure 17Frequency (F, %) of Bowen ratio β values by time since the last rainfall for (a) building-dominated (210–360∘) and (b) farmland-dominated (30–150∘) directions.
In addition to these factors, land use and land cover also play a critical role in the energy budget. As the daily averaged source area is mainly covered by vegetation (60 %) and impervious surface (87 %) in the 30–150 and 210–360∘ sectors of the tower, respectively, and farmland and buildings are separately the most representative land use and land cover types in the two sectors, observed data from these two sectors are selected to compare energy flux ratios and are accordingly referred to as from farmland- and building-dominated directions. At MY, normalizing measurements by the incoming radiation () the and β () are always more (less) when the wind is from directions with more buildings (cf. cropland-dominated) irrespective of season (Fig. 16). Compared to impervious surfaces, the cropland surface obviously changes between “dry” and “wet” more frequently and with a longer transition time, due to cropland irrigation and water storage by soil. This results in a greater amplitude of and from the cropland-dominated direction. The inter-quartile range and the differences between the mean and median of ratios are clearly greater from the cropland direction (Fig. 16). The temporal variations in β after rainfall differ between these two direction types (Fig. 17). Water can drain relatively quickly after rain in the building-dominated direction because of the high proportion of impervious surface, whereas water can infiltrate into the soil (i.e., it is stored), so β can remain near 1 for longer periods after rainfall (Fig. 17).
In this analysis of surface energy flux measurements for a suburb (MY) of Beijing over 16 months we gain a better understanding of surface–atmosphere dynamics.
All components of the radiation balance and net radiation (K↓, K↑, L↓, L↑, Q*) have unimodal annual patterns, with higher values in summer (lower in winter) and with the maximum monthly means in July (minima December/January).
At MY, the daytime sensible heat flux QH is greatest in spring. However, it is smallest in summer rather than winter, unlike in previous suburban studies. This is because, in the prevailing wind direction, there are extensive cropland, irrigation, and frequent rainfall in summer. All of these factors are thought to play a role. Nocturnal QH is negative throughout the year and smaller in winter, so the minimum daily total QH still occurs in winter.
The latent heat flux QE is positive throughout the day. The daytime maximum QE is greatest in summer (July; lowest in winter December) because of the influence of rainfall, irrigation, plant growth activity, and available energy. Monthly median diurnal maxima of QE vary from 10–248 W m−2 during measurement period. These are close to both the smallest and largest values reported for suburban areas. The maximum monthly mean daily total QE is in summer (July 2013), and the minimum is in winter (December 2013).
Daytime is lower in summer (higher in winter). Across the year it varies from 15 %–57 %. has the opposite seasonal trends but a similar annual range (6 %–56 %). Summer daytime means of QHQ* (0.16) are lower and those of (0.52) higher than that reported in most suburban areas. In winter daytime (0.41–0.57) is greater than reported for most suburban sites, but (0.08 to 0.18) is similar to other suburban sites. Thus the storage heat flux ΔQS at MY is a small fraction of Q* because of the low building fraction. At an annual timescale, daytime (31 %) and (35 %) are very similar, but for the whole day the proportions are 36% and 49 %, respectively.
The large seasonal differences in precipitation and irrigation lead to a wide range in Bowen ratio (β) values across the year (0.26–7.40). Daytime mean β values in summer (0.32) are lower than in winter (4.60) and are at the lower and higher end of the range in the literature for suburban sites. The annual mean daytime β is 0.89 and the daily value is 0.73; thus QE dominates available energy.
The results confirm the combined importance of precipitation, irrigation, vegetation growth, and land cover as being key factors affecting energy partitioning in MY. These findings will help to enhance the understanding of the surface–atmosphere energy exchange over Chinese suburban areas, provide observational data for model verification and parameterization scheme improvement, and be a reference for formulating policies to mitigate the adverse effects of urban climate and climate change.
LQF provides a method to calculate anthropogenic heat flux QF (Gabey et al., 2019) based on population, vehicle, energy consumption, and air temperature data. It estimates heat released from buildings (QF,B), traffic (QF,V), and the human metabolism (QF,M) (Allen et al., 2011; Lindberg et al., 2013). In this study, we determine an appropriate temperature response coefficient for the MY area.
A1 Temperature response coefficient
In LQF, the mathematical expression of QF,B is as follows:
where ρpop is the population density (people ha−1) and EB,b is daily building energy consumption (kWh d−1 per capita). The latter, from daily electricity consumption data, accounts for 16 % of the total energy consumption in MY (Table A1).
The coefficient, fb captures the air temperature response to energy consumption. Given large differences in energy consumption between countries and between cities (e.g., Lindberg et al., 2013), it is preferable to determine the appropriate local fb as it plays a critical role in building anthropogenic heat emissions. Here fb varies with air temperature Ta:
Several key parameters to determine fb are shown in Fig. A1. Here Tb is the air temperature when the energy consumption is the lowest. If the air temperature is higher (lower) than Tb, more energy will be consumed due to cooling (heating). Ac (Ah) is the building energy consumption thermal response slope for cooling when air temperature is above (below) Tb, also known as a cooling (heating) coefficient. C is the minimum energy consumption. Tmax and Tmin are threshold values for air temperature. When air temperature is beyond this range, energy consumption has reached saturation and no longer increases with air temperature changes.
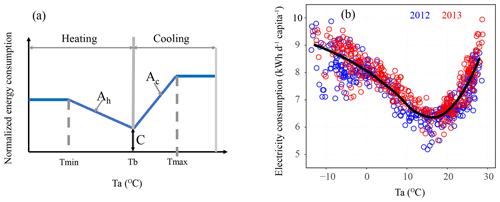
Figure A1Energy consumption response to air temperature: (a) general response function (see text for definitions) and (b) data for Miyun District (Miyun District daily electricity consumption; kWh d−1 per capita; State Grid Beijing Electric Power Company, http://www.bj.sgcc.com.cn/, last access: 10 October 2023), normalized by population (245 people km−2 in 2012 and 246 people km−2 in 2013; Miyun District Bureau of Statistics, 2013, 2014), and MY daily mean air temperature (Ta) for 2012–2013 with the general trend (black, local polynomial regression fitting – LOESS).
Table A1Energy consumption in Miyun (Miyun District Bureau of Statistics, 2013, 2014) in tons of coal equivalent (TCE) is converted to kilowatt hours assuming 1 TCE = 8141 kWh (Kyle's Converter, 2017).
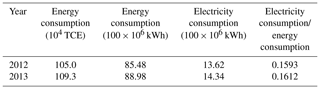
A new set of temperature response parameters applicable to this region is obtained by using daily air temperature and electricity consumption data for the Miyun district in 2012–2013 (Table A1). The resulting parameters are given in Table A2. The energy consumption response to temperature changes is a V-shaped curve in Miyun rather than a U shape as seen in Shanghai City (Ao et al., 2018). The cooling coefficient Ac in MY is lower than that in Shanghai City (0.04 ∘C−1), which is attributed to relatively short-period air conditioning in MY given the cooler summer nights caused by topography and the continental monsoon climate. However, in winter with colder air temperatures and a longer heating period, the heating coefficient Ah for MY is higher than for Shanghai (Ah=0.01 ∘C−1) (Ao et al., 2018).
A2 Vehicle numbers
In LQF, QF,V is calculated as a function of vehicle numbers, traffic speed, and time (Ao et al., 2018). The average Beijing vehicle numbers per 1000 capita in 2012 and 2013 (245 people km−2 in 2012 and 246 people km−2 in 2013; Miyun District Bureau of Statistics, 2013; 2014) are 196.9 and 201.7 cars, 11.7 and 11.7 motorcycles, and 42.6 and 43.7 freight vehicles. For all vehicles the following is assumed: an average vehicle speed of 48 km h−1 and petrol as fuel. Diurnal variation in vehicle numbers for weekdays, weekends, and holidays for Shanghai (Ao et al., 2018) is used as no such data are available for Miyun or Beijing. This will cause additional uncertainties. Given that the QF,V values are small (Sect. 3.2 and Fig. S2b), these additional uncertainties could be ignored.
A3 Population density
The Miyun Meteorological Station is located within the Gulou Street administrative division of Miyun District. The population density of the Gulou Street area was 5657 and 5702 people km−2 in 2012 and 2013, respectively (Miyun District Bureau of Statistics, 2013, 2014). This population density is much greater than in the Gridded Population of the World, version 4 (GPWv4; CIESIN, 2016) of about 1007 people km−2 around the study site. This is why Lindeberg et al. (2013) and Gabey et al. (2019) indicate the importance of updating data locally wherever possible.
One of the two methods (Sect. 2.5) used in this study to determine the storage heat flux is the objective hysteresis model (OHM), which uses coefficients by land cover type (a1,i–a3,i) with net all-wave radiation Q* (Grimmond et al., 1991; Meyn and Oke, 2009):
where t is time and fi is the area fraction covered by the ith land cover type. Given the differences in land cover around the MY flux tower, the plan area fractions of 30∘ wind sector footprint areas are used to calculate ΔQs,ohm by direction (Fig. B1).
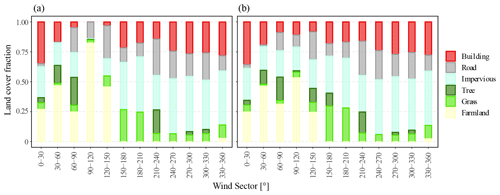
Figure B1Land cover fraction for 30∘ wind sectors but varying footprint length (Sect. 2.4) around the MY flux tower for (a) day ( W m−2) and (b) night.
The coefficients used (Table B1) include values obtained from 30 min measured data of net radiation (Q*) and soil heat flux (QG) at the Weishan Experimental Station (36∘39′ N, 116∘09′ E). Weishan and MY have a similar climate background, the same wheat–maize rotation pattern, and a consistent growth cycle (Lei and Yang, 2010; Lei et al., 2018).
The Weishan station has a 10 m tower with a four-component radiometer (CNR1, Kipp & Zonen, the Netherlands) installed at 3.5 m a.g.l. and two soil heat flux plates (HFP01SC, Hukseflux, Netherlands) installed at a depth of −0.03 m. The instrument data are recorded at 10 min intervals using a CR10X data logger (Campbell Scientific, Inc., USA). The soil heat flux (QG) data are the average of two measurement sites to the east and west of the tower.
For application convenience, the cropland coefficients are determined by season. A random but an arbitrary 70 % of data are selected in each season to fit the coefficients. The remaining 30 % is used to evaluate the simulation results. The results are good but poorest in winter (Fig. B2). These values are used for the farmland (Table B1). Some large observed QG values are not reproduced by the OHM model, as they are the maximum values and appear simultaneously with the peak of Q* on that day. Generally, the maximum QG appears later than that of Q*, with the fitted a2 of farmland being negative in all seasons (Table B1). This phenomenon has been reported in previous studies; i.e., OHM estimates perform satisfactorily in the mean but miss short-term variability (Grimmond and Oke, 1999b; Roberts et al., 2006).
Building coefficients also vary with season (Table B1). Here we use data from Nanjing City (Wang et al., 2008), with an average of summer and winter values used in spring and autumn. Other coefficients are used year round. For the road/impervious areas, an average of the literature values is used (Table B1).
The MY site coefficients vary with season and time of day (Fig. B3). The a1 and a2 values are larger in the afternoon, as the wind comes from the southwest direction where artificial underlying surfaces dominate (Figs. 2g–j and B1). The a1 and a2 values of various impervious surfaces are relatively high (Table B1). The winter a1 and a2 values are smaller than other seasons throughout the day. This is because the prevailing wind in winter comes from the farmland-dominated direction, while the a1 and a2 values of farmland in winter are the smallest (Table B1). The a3 value is exactly the opposite of a1 and a2.
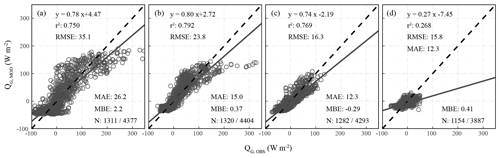
Figure B2Weishan station modeled and observed soil heat flux, with statistical evaluation metrics: root mean square error (RMSE), coefficient of determination (r2), mean absolute error (MAE), and mean bias error (MBE). N: evaluation data/total observations available for (a) spring, (b) summer, (c) autumn, and (d) winter.
The observation data used in the study are available from the corresponding author (e-mail: jxdou@ium.cn). We downloaded the flux data of the Shandong Yucheng Agro-Ecosystem National Observation and Research Station from the National Ecosystem Science Data Center at the National Science & Technology Infrastructure of China website: http://www.nesdc.org.cn; https://doi.org/10.12199/nesdc.ecodb.chinaflux2003-2010.2021.yca.005 (National Ecosystem Science Data Center, 2023).
The supplement related to this article is available online at: https://doi.org/10.5194/acp-23-13143-2023-supplement.
JD conducted the eddy covariance measurement, carried out the data analyses, and wrote the draft. SG supervised the scientific interpretation of the results and polished the writing. SM, BH, HL, and ML provided key datasets and contributed to the anthropogenic heat flux estimation and OHM coefficient fitting.
The contact author has declared that none of the authors has any competing interests.
Publisher's note: Copernicus Publications remains neutral with regard to jurisdictional claims in published maps and institutional affiliations.
This research was supported by the Youth Beijing Scholars Program (grant no. 2018-007), the National Natural Science Foundation of China (grant no. 41505102), the Key Innovation Team of the China Meteorological Administration (no. CMA2022ZD09), the Beijing Natural Science Foundation (grant no. 8212026), and the China Scholarship Council (CSC no. 201805330002). We are especially grateful to the Weishan Experiment Station for providing observation data of net radiation and soil heat flux in farmland used to fit the OHM parameters in the paper. We thank all those who have supported the observations analyzed in this project. Sue Grimmond thanks the Newton Fund/Met Office CSSP-China project and ERC urbisphere (855005).
This research has been supported by the Youth Beijing Scholars Program (grant no. 2018-007), the National Natural Science Foundation of China (grant no. 41505102), the Newton Fund/Met Office CSSP-China project and the ERC urbisphere (grant no. 855005), and the China Scholarship Council (grant no. 201805330002).
This paper was edited by Thomas Karl and reviewed by two anonymous referees.
Allen, L., Lindberg, F., and Grimmond, C. S. B.: Global to city scale urban anthropogenic heat flux: Model and variability, Int. J. Climatol., 31, 1990–2005, https://doi.org/10.1002/joc.2210, 2011.
Anandakumar, K.: A study on the partition of net radiation into heat fluxes on a dry asphalt surface, Atmos. Environ., 33, 3911–3918, https://doi.org/10.1016/S1352-2310(99)00133-8, 1999.
Ando, T. and Ueyama, M.: Surface energy exchange in a dense urban built-up area based on two-year eddy covariance measurements in Sakai, Japan, Urban Climate, 19, 155–169, https://doi.org/10.1016/j.uclim.2017.01.005, 2017.
Ao, X. Y., Grimmond, C. S. B., Chang, Y. Y., Liu, D. W., Tang, Y. Q., Hu, P., Wang, Y. D., Zou, J., and Tan, J. G.: Heat, water and carbon exchanges in the tall megacity of Shanghai: challenges and results, Int. J. Climatol., 36, 4608–4624, https://doi.org/10.1002/joc.4657, 2016a.
Ao, X. Y., Grimmond, C. S. B., Liu, D. W., Han, Z. H., Hu, P., Wang, Y. D., Zhen, X. R., and Tan, J. G.: Radiation fluxes in a business district of Shanghai, China, J. Appl. Meteorol. Clim., 55, 2451–2468, https://doi.org/10.1175/JAMC-D-16-0082.1, 2016b.
Ao, X. Y., Grimmond, C. S. B., Ward, H. C., Gabey, A. M., Tan, J. G., Yang, X. Q., Liu, D. W., Zhi, X., Liu, H. Y., and Zhang, N.: Evaluation of the surface urban energy and water balance scheme (SUEWS) at a dense urban site in Shanghai: Sensitivity to anthropogenic heat and irrigation, J. Hydrometeorol., 19, 1983–2005, https://doi.org/10.1175/JHM-D-18-0057.1, 2018.
Asaeda, T. and Ca, V. T.: The subsurface transport of heat and moisture and its act on the environment: a numerical model, Bound.-Lay. Meteorol., 65, 159–178, https://doi.org/10.1007/BF00708822, 1993.
Baldocchi, D. D: Assessing the eddy covariance technique for evaluating carbon dioxide exchange rates of ecosystems: Past, present and future, Global Change Biol., 9, 479–492, https://doi.org/10.1046/j.1365-2486.2003.00629.x, 2003.
Balogun, A. A., Adegoke, J. O., Vezhapparambu, S., Mauder, M., McFadden, J., and Gallo, K.: Surface energy balance measurements above an exurban residential neighbourhood of Kansas City, Missouri, Bound.-Lay. Meteorol., 133, 299–321, https://doi.org/10.1007/s10546-009-9421-3, 2009.
Bergeron, O. and Strachan, I. B.: Wintertime radiation and energy budget along an urbanization gradient in Montreal, Canada, Int. J. Climatol., 32, 137–152, https://doi.org/10.1002/joc.2246, 2012.
CCRSDA – China Centre for Resources Satellite Data and Application: GF-2 (Gaofen-2) High-resolution Image, China Aerospace Science and Technology Corporation, https://data.cresda.cn/#/home (last access: 13 September 2023), 2016.
Christen, A. and Vogt, R.: Energy and radiation balance of a central European city, Int. J. Climatol., 24, 1395–1421, https://doi.org/10.1002/joc.1074, 2004.
CIESIN – Center for International Earth Science Information Network, Gridded Population of the World, Version 4 (GPWv4): Population Count Grid, NASA SEDAC – Socioeconomic Data and Applications Center, Columbia University, Palisades, NY, https://sedac.ciesin.columbia.edu/data/set/gpw-v4-population-density-rev11/data-download (last access: 13 September 2023), 2016.
Coutts, A. M., Beringer, J., and Tapper, N. J.: Impact of increasing urban density on local climate: Spatial and temporal variations in the surface energy balance in Melbourne, Australia, J. Appl. Meteorol. Clim., 46, 477–493, https://doi.org/10.1175/jam2462.1, 2007.
Doll, D., Ching, J. K. S., and Kaneshiro, J.: Parameterization of subsurface heating for soil and concrete using net radiation data, Bound.-Lay. Meteorol., 32, 351–372, https://doi.org/10.1007/BF00122000, 1985.
Dou, J. X., Grimmond, C. S. B., Cheng, Z. G., Miao, S. G., Feng, D. Y., and Liao, M. S.: Summertime surface energy balance fluxes at two Beijing sites, Int. J. Climatol., 39, 2793–2810, https://doi.org/10.1002/joc.5989, 2019.
Flerchinger, G. N., Xiao, W., Marks, D., Sauer, T. J., and Yu, Q.: Comparison of algorithms for incoming atmospheric longwave radiation, Water Resour. Res., 45, W03423, https://doi.org/10.1029/2008WR007394, 2009.
Foken, T.: The energy balance closure problem: An overview, Ecol. Appl., 18, 1351–1367, https://doi.org/10.1890/06-0922.1, 2008.
Gabey, A., Grimmond, C. S. B., and Capel-Timms, I.: Anthropogenic heat flux: advisable spatial resolutions when input data are scarce. Theor. Appl. Climatol., 135, 791–807, https://doi.org/10.1007/s00704-018-2367-y, 2019.
Goldbach, A, and Kuttler, W.: Quantification of turbulent heat fluxes for adaptation strategies within urban planning, Int. J. Climatol., 33, 143–159, https://doi.org/10.1002/joc.3437, 2012.
Grimmond, C. S. B. and Oke, T. R.: Urban water balance II: Results from a suburb of Vancouver, BC, Water Resour. Res., 22, 1404–1412, https://doi.org/10.1029/WR022i010p01404, 1986.
Grimmond, C. S. B. and Oke, T. R.: Comparison of heat fluxes from summertime observations in the suburbs of four North American cities, J. Appl. Meteorol., 34, 873–889, https://doi.org/10.1175/1520-0450(1995)034<0873:COHFFS>2.0.CO;2, 1995.
Grimmond, C. S. B. and Oke, T. R.: Aerodynamic properties of urban areas derived, from analysis of surface form, J. Appl. Meteorol., 38, 1262–1292, 1999a.
Grimmond, C. S. B. and Oke, T. R.: Heat storage in urban areas: Local-scale observations and evaluation of a simple model, J. Appl. Meteorol., 38, 922–940, 1999b.
Grimmond, C. S. B. and Oke, T. R.: Turbulent heat fluxes in urban areas: Observations and a local-scale urban meteorological parameterization scheme (LUMPS), J. Appl. Meteorol., 41, 792–810, 2002.
Grimmond, C. S. B., Cleugh, H. A., and Oke, T. R.: An objective urban heat storage model and its comparison with other schemes, Atmos. Environ., 25, 311–326, https://doi.org/10.1016/0957-1272(91)90003-W, 1991.
Grimmond, C. S. B., Souch, C., Hubble, M.: Influence of tree cover on summertime energy balance fluxes, San Gabriel Valley, Los Angeles, Clim. Res., 6, 45–57, 1996.
Grimmond, C. S. B., Blackett, M., Best, M. J., Barlow, J., Baik, J. J., Belcher, S. E., Bohnenstengel, S. I., Calmet, I., Chen, F., Dandou, A., Fortuniak, K., Gouvea, M. L., Hamdi, R., Hendry, M., Kawai, T., Kawamoto, Y., Kondo, H., Krayenhoff, E. S., Lee, S. H., Loridan, T., Martilli, A., Masson, V., Miao, S., Oleson, K., Pigeon, G., Porson, A., Ryu, Y. H., Salamanca, F., Shashua-Bar, L., Steeneveld, G. J., Tombrou, M., Voogt, J., Young, D., and Zhang, N.: The international urban energy balance models comparison project: First results from Phase 1, J. Appl. Meteorol. Clim., 49, 1268–1292, https://doi.org/10.1175/2010JAMC2354.1, 2010.
Guo, W. D., Wang, X. Q., Sun, J. N., Ding, A. J., and Zou, J.: Comparison of land–atmosphere interaction at different surface types in the mid- to lower reaches of the Yangtze River valley, Atmos. Chem. Phys., 16, 9875–9890, https://doi.org/10.5194/acp-16-9875-2016, 2016.
Hendel, M., Colombert, M., Diab, Y., and Royon, L.: An analysis of pavement heat flux to optimize the water efficiency of a pavement-watering method, Appl. Therm. Eng., 78, 658–669, https://doi.org/10.1016/j.applthermaleng.2014.11.060, 2015.
Hong, J. W., Lee, S. D., Lee, K., and Hong, J.: Seasonal variations in the surface energy and CO2 flux over a high-rise, high-population, residential urban area in the East Asian monsoon region, Int. J. Climatol., 40, 4384–4407, https://doi.org/10.1002/joc.6463, 2020.
Järvi, L., Grimmond, C. S. B., and Christen, A.: The Surface Urban Energy and Water Balance Scheme (SUEWS): evaluation in Los Angeles and Vancouver, J. Hydrometeorol., 411, 219–237, https://doi.org/10.1016/j.jhydrol.2011.10.001, 2011.
Järvi, L., Grimmond, C. S. B., Taka, M., Nordbo, A., Setälä, H., and Strachan, I. B.: Development of the surface Urban Energy and Water Balance Scheme (SUEWS) for cold climate cities, Geosci. Model. Dev., 7, 1691–1711, https://doi.org/10.5194/gmd-7-1691-2014, 2014.
Järvi, L., Havu, M., Ward, H. C., Bellucco, V., McFadden, J. P., Toivonen, T., Heikinheimo, V., Kolari, P., Riikonen, A., Grimmond, C. S. B.: Spatial modeling of local-scale biogenic and anthropogenic carbon dioxide emissions in Helsinki, J. Geophys. Res.-Atmos., 124, 8363–8384, https://doi.org/10.1029/2018JD029576, 2019.
Kanda, M., Inagaki, A., Miyamoto, T., Gryschka, M., and Raasch, S.: A new aerodynamic parametrization for real urban surfaces, Bound.-Lay. Meteorol., 148, 357–377, https://doi.org/10.1007/s10546-013-9818-x, 2013.
Karsisto, P., Fortelius, C., Demuzere, M., Grimmond, C. S. B., Oleson, K. W., Kouznetsov, R., Masson, V., and Järvi, L.: Seasonal surface urban energy balance and wintertime stability simulated using three land-surface models in the high-latitude city Helsinki, Q. J. Roy. Meteorol. Soc., 142, 401–417, https://doi.org/10.1002/qj.2659, 2015.
Kent, C. W., Grimmond, C. S. B., Barlow, J., Gatey, D., Kotthaus, S., Lindberg, F., Halios, C. H.: Evaluation of urban local-scale aerodynamic parameters: implications for the vertical profile of wind and source areas, Bound.-Lay. Meteorol., 164, 183–213, https://doi.org/10.1007/s10546-017-0248-z, 2017.
Kim, H., Hong, J. W., Lim, Y. J., Hong, J., Shin, S. S., and Kim, Y. J.: Evaluation of JULES land surface model based on in-situ data of NIMS flux sites, Atmosphere, 29, 355–365, https://doi.org/10.14191/Atmos.2019.29.4.355, 2019.
Kim, M. S. and Kwon, B. H.: Estimation of sensible heat flux and atmospheric boundary layer height using an unmanned aerial vehicle, Atmosphere, 10, 363, https://doi.org/10.3390/atmos10070363, 2019.
Kljun, N., Calanca, P., Rotach, M. W., and Schmid, H. P.: A simple parameterization for flux footprint predictions, Bound.-Lay. Meteorol., 112, 503–523, https://doi.org/10.1023/B:BOUN.0000030653.71031.96, 2004.
Kokkonen, T. V., Grimmond, C. S. B., Christen, A., Oke, T. R., and Järvi, L.: Changes to the water balance over a century of urban development in two neighborhoods: Vancouver, Canada, Water Resour. Res., 54, 6625–6642, https://doi.org/10.1029/2017WR022445, 2018.
Kotthaus, S. and Grimmond, C. S. B.: Energy exchange in a dense urban environment – Part I: temporal variability of long-term observations in central London, Urban Climate, 10, 261–280, https://doi.org/10.1016/j.uclim.2013.10.002, 2014.
Kyle's Converter: Convert tons of coal equivalent to kilowatt-hours, Kyle's Converter, http://www.kylesconverter.com/energy,-work,-and-heat/tons-of-coal-equivalent-to-kilowatt--hours (last access: 1 December 2021), 2017.
Lee, K. M., Hong, J. W., Kim, J. W., Jo, S. S., and Hong, J. Y.: Traces of urban forest in temperature and CO2 signals in monsoon East Asia, Atmos. Chem. Phys., 21, 17833–17853, https://doi.org/10.5194/acp-21-17833-2021, 2021.
Lei, H. M. and Yang, D. W.: Interannual and seasonal variability in evapotranspiration and energy partitioning over an irrigated cropland in the North China Plain, Agr. Forest Meteorol., 150, 581–589, https://doi.org/10.1016/j.agrformet.2010.01.022, 2010.
Lei, H. M., Gong, T. T., Zhang, Y. C., and Yang, D. W.: Biological factors dominate the interannual variability of evapotranspiration in an irrigated cropland in the North China Plain, Agr. Forest Meteorol., 250–251, 262–276, https://doi.org/10.1016/j.agrformet.2018.01.007, 2018.
Liang, X. D., Miao, S. G., Li, J., Bornstein, R., Zhang, X., Gao, Y., Chen, F., Cao, X., Cheng, Z., Clements, C., Dabberdt, W., Ding, A., Ding, D.,Dou, J. J., Dou, J. X., Grimmond, C. S. B., González-Cruz, J. E., He, J., Huang, M., Huang, X., Ju, S., Li, Q., Niyogi, D., Quan, J., Sun, J., Sun, J. Z., Yu, M., Zhang, J., Zhang, Y., Zhao, X., Zheng, Z., and Zhou, M.: SURF – Understanding and predicting urban convection and haze, B. Am. Meteorol. Soc., 99, 1391–1413, https://doi.org/10.1175/BAMS-D-16-0178.1, 2018.
LI-COR, Inc: EddyPro software instruction manual, https://www.licor.com/env/support/EddyPro/manuals.html (last access: 13 September 2023), 2023.
Lindberg, F., Grimmond, C. S. B., Yogeswaran, N., Kotthaus, S., and Allen, L.: Impact of city changes and weather on anthropogenic heat flux in Europe 1995–2015, Urban Climate, 4, 1–15, https://doi.org/10.1016/j.uclim.2013.03.002, 2013.
Lindberg, F., Grimmond, C. S. B., Gabey, A., Huang, B., Kent, C. W., Sun, T., Theeuwes, N. E., Järvi, L., Ward, H. C., Capel-Timms, I., Chang, Y. Y., Jonsson, P., Krave, N., Liu, D. W., Meyer, D., Olofson, K. F. G., Tan, J. G., Wästberg, D., Xue, L., and Zhang, Z.: Urban multiscale environmental predictor (UMEP) – An integrated tool for city-based climate services, Environ. Model. Softw., 99, 70–87, https://doi.org/10.1016/j.envsoft.2017.09.020, 2018.
Liu, H. Z., Feng, J. W., Järvi, L., and Vesala, T.: Four-year (2006–2009) eddy covariance measurements of CO2 flux over an urban area in Beijing, Atmos. Chem. Phys., 12, 7881–7892, https://doi.org/10.5194/acp-12-7881-2012, 2012.
Liu, X., Li, X. X., Harshan, S., Roth, M., and Velasco, E.: Evaluation of an urban canopy model in a tropical city: the role of tree evapotranspiration, Environ. Res. Lett., 12, 094008, https://doi.org/10.1088/1748-9326/aa7ee7, 2017.
Loridan, T. and Grimmond, C. S. B.: Characterization of energy flux partitioning in urban environments: links with surface seasonal properties, J. Appl. Meteorol. Clim., 51, 219–241, https://doi.org/10.1175/JAMC-D-11-038.1, 2012.
McCaughey, J. H.: Energy balance storage terms in a mature mixed forest at Petawawa Ontario: A case study, Bound.-Lay. Meteorol., 31, 89–101, https://doi.org/10.1007/BF00120036, 1985.
Mestayer, P. G., Durand, P., Augustin, P., Bastin, S., Bonnefond J. M., Bénech, B., Campistron, B., Coppalle, A., Delbarre, H., Dousset, B., Drobinski, P., Druilhet, A., Fréjafon, E., Grimmond, C. S. B., Groleau, D., Irvine, M., Kergomard, C., Kermadi, S., Lagouarde, J. -P., Lemonsu, A., Lohou, F., Long, N., Masson, V., Moppert, C., Noilhan, J., Offerle, B., Oke, T. R., Pigeon, G., Puygrenier, V., Roberts, S., Rosant, J. -M., Sanïd, F., Salmond, J., Talbaut, M., and Voogt, J.: The urban boundary-layer field campaign in Marseille (UBL/CLU-ESCOMPTE): Set-up and first results, Bound.-Lay. Meteorol., 114, 315–365, https://doi.org/10.1007/s10546-004-9241-4, 2005.
Meyers, T. P. and Hollinger, S. E.: An assessment of storage terms in the surface energy balance of maize and soybean, Agr. Forest Meteorol., 125, 105–115, https://doi.org/10.1016/j.agrformet.2004.03.001, 2004.
Meyn, S. K. and Oke, T. R.: Heat fluxes through roofs and their relevance to estimates of urban heat storage, Energ. Build., 41, 745–52, https://doi.org/10.1016/j.enbuild.2009.02.005, 2009.
Miao, S. G., Dou, J. X., Chen, F., Li, J., and Li, A. G.: Analysis of observations on the urban surface energy balance in Beijing, Sci. China Earth Sci., 55, 1881–1890, https://doi.org/10.1007/s11430-012-4411-6, 2012.
Michel, D., Philipona, R., Ruckstuhl, C., Vogt, R., and Vuilleumier, L.: Performance and uncertainty of CNR1 net radiometers during a one-year field comparison, J. Atmos. Ocean. Tech., 25, 442–451, https://doi.org/10.1175/2007JTECHA973.1, 2008.
Miyun District Bureau of Statistics: Beijing Miyun Statistical Yearbook-2013, http://www.bjmy.gov.cn/art/2013/12/12/art_76_116971.html (last access: 13 September 2023), 2013.
Miyun District Bureau of Statistics: Beijing Miyun Statistical Yearbook-2014, http://www.bjmy.gov.cn/art/2014/12/4/art_76_116972.html (last access: 13 September 2023), 2014.
Miyun District Bureau of Statistics: Beijing Miyun Statistical Yearbook-2020, http://www.bjmy.gov.cn/art/2020/11/19/art_76_339489.html (last access: 13 September 2023), 2020.
Moncrieff, J. B., Massheder, J. M., de Bruin, H., Elbers, J., Friborg, T., Heusinkveld, B., Kabat, P., Scott, S., Soegaard, H., and Verhoef, A.: A system to measure surface fluxes of momentum, sensible heat, water vapour and carbon dioxide, J. Hydrol., 188–199, 589–611, https://doi.org/10.1016/S0022-1694(96)03194-0, 1997.
Moriwaki, R. and Kanda, M.: Seasonal and diurnal fluxes of radiation, heat, water vapor, and carbon dioxide over a suburban area, J. Appl. Meteorol. Clim., 43, 1700–1710, https://doi.org/10.1175/JAM2153.1, 2004.
Narita, K., Sekine, T., and Tokuoka, T.: Thermal properties of urban surface materials: study on heat balance at asphalt pavement, Geogr. Rev. pn. Ser. A, 57, 639–651, https://doi.org/10.4157/grj1984a.57.9_639, 1984.
National Ecosystem Science Data Center: Half-hour flux data of Shandong Yucheng Agro-Ecosystem National Observation and Research Station from 2003 to 2010, National Ecosystem Science Data Center [data set], https://doi.org/10.12199/nesdc.ecodb.chinaflux2003-2010.2021.yca.005, last access: 3 August 2023.
Newton, T., Oke, T. R., Grimmond, C. S. B., Roth, M: The suburban energy balance in Miami, Florida, Geogr. Ann. A, 89, 331-347, https://doi.org/10.1111/j.1468-0459.2007.00329.x, 2007.
Offerle, B., Jonsson, P., Eliasson, I., Grimmond, C. S. B.: Urban modification of the surface energy balance in the West African Sahel: Ouagadougou, Burkina Faso, J. Climate., 18, 3983–3995, https://doi.org/10.1175/JCLI3520.1, 2005.
Offerle, B., Grimmond, C. S. B., Fortuniak, K., Kłysik, K., and Oke, T. R.: Temporal variations in heat fluxes over a central European city centre, Theor. Appl. Climatol., 84, 103–115, https://doi.org/10.1007/s00704-005-0148-x, 2006a.
Offerle, B., Grimmond, C. S. B., Fortuniak, K., Pawlak, W.: Intraurban differences of surface energy fluxes in a central European city, J. Appl. Meteorol. Clim., 45, 125–136, https://doi.org/10.1175/JAM2319.1, 2006b.
Oke, T. R., Spronken-Smith, R. A., Jáuregui, E., Grimmond, C. S. B.: The energy balance of central Mexico City during the dry season, Atmos. Environ., 33, 3919–3930, https://doi.org/10.1016/S1352-2310(99)00134-X, 1999.
Oke, T. R., Mills, G., Christen, A., and Voogt, J. A.: Urban Climates, Cambridge University Press, Cambridge, 525 pp., https://doi.org/10.1017/9781139016476, 2017.
Oliphant, A. J., Grimmond, C. S. B., Zutter, H. N, Schmid, H. P., Su, H. B., Scott, S. L., Offerle, B., Randolph, J. C., and Ehman, J.: Heat storage and energy balance fluxes for a temperate deciduous forest, Agr. Forest Meteorol., 126, 185–201, https://doi.org/10.1016/j.agrformet.2004.07.003, 2004.
Ren, Z. H., Zhang, Z. F., Sun, C., Liu, Y. M., Li, J., Ju, X. H., Zhao, Y. F., Li, Z. P., Zhang, W., Li, H. K., Zeng, X. J., Ren, X. W., Liu, Y., and Wang, H. J.: Development of three-step quality control system of real-time observation data from AWS in China, Meteorol. Month., 41, 1268–1277, https://doi.org/10.7519/j.issn.1000-0526.2015.10.010, 2015.
Roberts, S. M., Oke, T. R., and Grimmond, C. S. B.: Comparison of four methods to estimate urban heat storage, J. Appl. Meteorol. Clim., 45, 1766–1781, https://doi.org/10.1175/JAM2432.1, 2006.
Rotach, M. W., Vogt, R., Bernhofer, C., Batchvarova, E., Christen, A., Clappier, A., Feddersen, B., Gryning, S. E., Martucci, G., Mayer, H., Mitev, V., Oke, T. R., Parlow, E., Richner, H., Roth, M., Roulet, Y. A., Ruffieux, D., Salmond, J. A., Schatzmann, M., and Voogt, J. A.: BUBBLE-an urban boundary layer meteorology project, Theor. Appl. Climatol., 81, 231–261, https://doi.org/10.1007/s00704-004-0117-9, 2005.
Roth, M., Jansson, C., and Velasco, E.: Multi-year energy balance and carbon dioxide fluxes over a residential neighbourhood in a tropical city, Int. J. Climatol., 37, 2679–2698, https://doi.org/10.1002/joc.4873, 2017.
Spronken-Smith, R. A.: Comparison of summer- and winter-time suburban energy fluxes in Christchurch, New Zealand, Int. J. Climatol., 22, 979–992, https://doi.org/10.1002/joc.767, 2002.
Stewart, I. D. and Oke, T. R.: Local climate zones for urban temperature studies, B. Am. Meteorol. Soc., 93, 1879–1900, https://doi.org/10.1175/BAMS-D-11-00019.1, 2012.
Tomoya, A. and Masahito, U.: Surface energy exchange in a dense urban built-up area based on two-year eddy covariance measurements in Sakai, Japan, Urban Climate, 19, 155–169, https://doi.org/10.1016/j.uclim.2017.01.005, 2017.
Vesala, T., Järvi, L., Launiainen, S., Sogachev, A., Rannik, Ü., Mammarella, I., Siivola, E., Keronen, P., Rinne, J., Riikonen, A., and Nikinmaa, E.: Surface-atmosphere interactions over complex urban terrain in Helsinki, Finland, Tellus B, 60, 188–199, https://doi.org/10.1111/j.1600-0889.2007.00312.x, 2008.
Wang, C. G., Sun, J. N., and Jiang, W. M.: Observation and analysis on thermodynamic characteristics of heat storage of urban roof, Acta Energiae Solaris Sinica, 6, 694–699, https://doi.org/10.3321/j.issn:0254-0096.2008.06.011, 2008.
Wang, L. L., Gao, Z. Q., Miao, S. G., Guo, X. F., Sun, T., Liu, M. F., and Li, D.: Contrasting characteristics of the surface energy balance between the urban and rural areas of Beijing, Adv. Atmos. Sci., 32, 505–514, https://doi.org/10.1007/s00376-014-3222-4, 2015.
Wang, L. L., Fan, S. H., Hu, F., Miao, S. G., Yang, A. Q., Li, Y. B., Liu, J. K., Liu, C. W., Chen, S. S., Ho, H. C., Duan, Z. X., Gao, Z. Q., and Yang, Y. J.: Vertical gradient variations in radiation budget and heat fluxes in the urban boundary layer: A comparison study between polluted and clean air episodes in Beijing during winter, J. Geophys. Res.-Atmos., 125, e2020JD032478, https://doi.org/10.1029/2020JD032478, 2020.
Ward, H. C., Evans, J. G., and Grimmond, C. S. B.: Multi-season eddy covariance observations of energy, water and carbon fluxes over a suburban area in Swindon, UK, Atmos. Chem. Phys., 13, 4645–4666, https://doi.org/10.5194/acp-13-4645-2013, 2013.
Ward, H. C., Kotthaus, S., Järvi, and Grimmond, C. S. B.: Surface Urban Energy and Water Balance Scheme (SUEWS): Development and evaluation at two UK sites, Urban Climate, 18, 1–32, https://doi.org/10.1016/j.uclim.2016.05.001, 2016.
Webb, E., Pearman, G., and Leuning, R.: Correction of the flux measurements for density effects due to heat and water vapor transfer, Q. J. Roy. Meteorol. Soc., 106, 85–100, https://doi.org/10.1002/qj.49710644707, 1980.
Wilson, K., Goldstein, A., Falge, E., Aubinet, M., Baldocchi, D., Berbigier, P., Bernhofer, C., Ceulemans, R., Dolman, H., Field, C., Grelle, A., Ibrom, A., Law, B. E., Kowalski, A., Meyers, T., Moncrieff, J., Monson, R., Oechel, W., Tenhunen, J., Valentini, R., and Verma, S.: Energy balance closure at FLUXNET sites, Agr. Forest Meteorol., 113, 223–243, https://doi.org/10.1016/S0168-1923(02)00109-0, 2002.
- Abstract
- Introduction
- Methods
- Results and discussion
- Conclusions
- Appendix A: Anthropogenic heat flux estimation
- Appendix B: Storage heat flux estimation using OHM
- Data availability
- Author contributions
- Competing interests
- Disclaimer
- Acknowledgements
- Financial support
- Review statement
- References
- Supplement
- Abstract
- Introduction
- Methods
- Results and discussion
- Conclusions
- Appendix A: Anthropogenic heat flux estimation
- Appendix B: Storage heat flux estimation using OHM
- Data availability
- Author contributions
- Competing interests
- Disclaimer
- Acknowledgements
- Financial support
- Review statement
- References
- Supplement