the Creative Commons Attribution 4.0 License.
the Creative Commons Attribution 4.0 License.
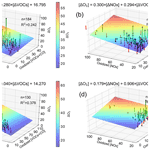
Observation-based analysis of ozone production sensitivity for two persistent ozone episodes in Guangdong, China
Kaixiang Song
Yu Wang
Tao Liu
Liyan Wei
Yanxing Wu
Junyu Zheng
Boguang Wang
Shaw Chen Liu
An observation-based method (OBM) is developed to investigate the sensitivity of ozone formation to precursors during two persistent elevated ozone episodes observed at 77 stations in Guangdong. Average OH concentrations derived at the 77 stations between 08:00 and 13:00 local time stay within a narrow range of 2.5×106 to 5.5×106 cm−3 with a weak dependence on the NOx. These values are in good agreement with OH values observed at a rural station in the Pearl River Delta (PRD). They also agree well with a box model constrained by the ambient conditions observed during the two episodes. The OBM has been used to evaluate the ozone production efficiency, ε(NOx or volatile organic compound, VOC), defined as the number of O3 molecules produced per molecule of NOx (or VOC) oxidized. Average values of ε(NOx) and ε(VOC) determined by the OBM are 3.0 and 2.1 ppb ppb−1, respectively, and both compared well with values in previous studies. Approximately 67 % of the station days exhibit ozone formation sensitivity to NOx, and approximately 20 % of the station days are in the transitional regime sensitive to both NOx and VOC, and only approximately 13 % of the station days are sensitive to VOC. These results are in semi-quantitative agreement with the ozone formation sensitivity calculated by the box model constrained by ambient conditions observed during the two episodes. However, our OBM results differ from those of most previous investigations, which suggested that limiting the emission of VOC rather than NOx would be more effective in reducing ozone reduction in Guangdong.
- Article
(4218 KB) - Full-text XML
-
Supplement
(616 KB) - BibTeX
- EndNote
Increases in surface ozone (O3) can have serious adverse impacts on human health and ecological systems (Wang et al., 2005; Song et al., 2017; Lin et al., 2018). In addition, tropospheric ozone is a significant greenhouse gas (IPCC, 2013). With a high rate of urbanization and industrialization, and the increasing use of motor vehicles, Guangdong has been suffering from severe O3 pollution (Zhang et al., 2011). The primary pollutant in Guangdong has switched from particulate matter to O3 since 2015, thanks to a stringent emission control policy that has effectively reduced other air pollutants (Department of Ecology and Environment of Guangdong Province, 2016). In fact, the number of days with O3 as the primary pollutant is 68.7 %, far exceeding that of PM2.5 (15.8 %) and PM10 (8.3 %) in 2020 (Department of Ecology and Environment of Guangdong Province, 2021).
O3 is a secondary pollutant produced from photochemical reactions involving nitrogen oxides (NOx) and volatile organic compounds (VOCs) (Trainer et al., 2000; Zhang et al., 2014; T. Wang et al., 2017). The sensitivity of O3 production is nonlinearly dependent on precursor concentrations and is usually categorized into photochemical regimes such as NOx-limited or VOC-limited (Kleinman et al., 1994; Sillman et al., 1998). There have been a number of studies on the sensitivity of O3 production to NOx and VOC by photochemical air quality models (Sillman et al., 2003; Lei et al., 2004; Tang et al., 2010), as well as observation-based methods (OBMs) (Thielmann et al., 2002; Zaveri et al., 2003; Shiu et al., 2007). Several modeling approaches have been used to evaluate the O3 production sensitivity, including the method, where LN is the radical loss via the reactions with NOx and Q is the total primary radical production (Kleinman et al., 2001; Kleinman, 2005; Mao et al., 2010); the relative incremental reactivity method (RIR) (Shao et al., 2009; Cheng et al., 2010; Lu et al., 2010a; Xue et al., 2014; Li et al., 2017); and the Empirical Kinetics Modeling Approach (EKMA) (Dodge, 1977). These model-based studies usually have large uncertainties in their input parameters, particularly in the emission inventories and photochemistry of VOC (Chang et al., 2020). Observation-based methods can avoid some of the uncertainties by using observations to constrain the analysis (Thielmann et al., 2002; Zaveri et al., 2003; Shiu et al., 2007).
In this study, we adopt the approach proposed by Shiu et al. (2007) and develop an OBM to evaluate the O3 production sensitivity during two multi-day O3 pollution episodes in Guangdong. In this OBM, the concentration of OH is derived from observed NOx and CO in a new approach as described in the methodology section. The OBM is then used to evaluate the ozone production efficiency, ε(NOx or VOC), defined as the number of O3 molecules produced per molecule of NOx (or VOC) oxidized. Finally, 3D-EKMA plots are generated basing on the OBM. The rest of the paper is organized as follows: Sect. 2 describes the data sources and analysis methods, Sect. 3 presents the results and discussions, and Sect. 4 presents a summary and conclusions.
2.1 Data
Hourly surface O3, PM2.5, CO and NO2 concentration data at 77 out of a total 102 stations in Guangdong operated by the China National Environmental Monitoring Centre (CNEMC) during the period 2018–2019 are used in this study (available at http://www.cnemc.cn/en/, last access: 10 November 2021). The 77 stations (Fig. 1a) are chosen for their completeness of data. It can be seen in Fig. 1a that polluted stations are mainly located in the PRD, while clean stations are located in the northeast of Guangdong. In this study, we choose two persistent O3 pollution episodes to perform the OBM analysis, specifically 2 to 8 October 2018 and 24 September to 1 October 2019. Figure 1b is the same as Fig. 1a except it shows the average ozone concentrations of all ozone-exceeding days in Guangdong in 2018 and 2019. One can see clearly that the ozone distribution during the two episodes in autumn is representative of and even slightly higher than the ozone concentrations during ozone pollution days in Guangdong in the entire 2 years. In fact, the monthly peak ozone concentrations in Guangdong tend to occur in September and October because Guangdong is usually under heavily overcast conditions with southerly winds bringing clean moist air from the South China Sea in the summer which tends to suppress the ozone formation.
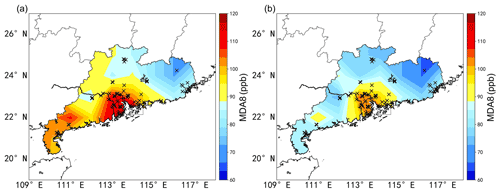
Figure 1(a) Spatial distribution of the average maximum daily 8 h average ozone concentration (MDA8) in Guangdong during the study period, black crosses mark the locations of observation sites. (b) Same as (a) but for MDA8 of all ozone exceeding days in Guangdong in 2018 and 2019.
Hourly meteorological data are obtained from the European Centre for Medium-Range Weather Forecasts ERA5 reanalysis, including relative humidity (RH), 2 m temperature (T), 10 m zonal wind (U10), 10 m meridional wind (V10), geopotential height, cloud cover, surface net solar radiation, boundary layer height and K index, with a resolution of 0.25∘ × 0.25∘ (available at https://www.ecmwf.int/, last access: 10 November 2021).
2.2 Methods
2.2.1 Derivation of nitric oxide (NO) concentration
Since the observation of NO concentration ([NO]) is severely limited in the CNEMC data set, we calculate [NO] from the assumption of photochemical equilibrium between [NO2] and [O3] according to the following equation:
where k1 represents the reaction rate constant for the reaction of NO with O3. This equation neglects the reactions of NO reactions with HO2 and RO2. The uncertainty due to this neglection is around 20 %, which is acceptable as discussed in Sect. 3.5. The value of k1 is taken from Seinfeld and Pandis (1998):
is the photolysis rate of NO2. Its value depends on the solar zenith angle (χ) and total shortwave radiation (TSR) (Wiegand and Bofinger, 2000):
2.2.2 Derivation of VOC
In this study, we use CO as a tracer to estimate VOC. This tracer method has been widely used in previous studies (Heald et al., 2003; Hsu et al., 2010; Shao et al., 2011; Yao et al., 2012; Tang et al., 2013). Individual VOCs at 08:00 local time (LT) are calculated by multiplying the freshly emitted CO at 08:00 LT with the ratio of in the emission inventories of Huang et al. (2021), according to the equations listed in the Supplement. The freshly emitted CO is assumed to be the difference in CO between 08:00 and 13:00 LT as shown in Fig. 2 (Eq. S1 in the Supplement). The CO at 13:00 LT is considered to be the leftover CO for the following day and is used to evaluate the leftover VOCs (Eq. S2). Oxidized VOCs (OVOCs) are estimated from the observed ratios of CH2O, CH3CHO and ketone to CO (Wang et al., 2016; Wu et al., 2020). Other OVOCs are not included. The VOCs and OVOCs derived this way can be validated by comparing with observed values in terms of the OH reactivity. Tan et al. (2019) reported that observed NOx, CO, HCHO and VOCs in PRD in autumn 2014 contributed, respectively, 14 %, 10 %, 5 %–8 % and 20 %, for a total of about 50 %, to the observed OH reactivity, which scale to 28 %, 20 %, 10 %–16 % and 40 %, respectively, when normalized to 100 %. In comparison, in our study the average NOx, CO, OVOCs and VOCs contribute 33 %, 17 %, 24 % and 26 %, respectively, to the OH reactivity. There is a reasonable agreement between our results and those of Tan et al. (2019) except for OVOCs and VOCs. The disagreement on OVOCs can be easily explained by the fact that HCHO accounts for about two-thirds of the OVOCs in our case. Nevertheless, the underestimate of the VOC contribution in our study remains unresolved and suggests significant uncertainty in the VOCs derived by our method.
2.2.3 Derivation of OH concentrations
The ratio ethylbenzene m,p-xylene has been suggested to be a good measure of the photochemical processing by OH (Calvert, 1976; Singh, 1977; Shiu et al., 2007). Following a Lagrangian trajectory, the ratio can be shown as
where E and X represent concentrations of ethylbenzene and m,p-xylene at time t, respectively. E0 and X0 are their corresponding initial concentrations, kx and ke are their reaction rate constants with OH, and kx and ke equal to and cm3 s−1, respectively (Atkinson, 1990). With a known value of , [OH × t] can be evaluated from observed at time t. This provides an OBM-derived density of OH.
In the real atmosphere, the Lagrangian condition rarely exists due to turbulence mixing as well as atmospheric advection. Nevertheless, Eq. (4) tends to hold because atmospheric transport affects the two species similarly. This is a key advantage of the OBM. In this study, due to limited measurements of VOC, we use CO and NOx to replace ethylbenzene and m,p-xylene, respectively.
2.2.4 Calculation of oxidized VOC and NOx
In this study, we consider the reaction of NO2 with OH as the only removal process for NOx and assume the removal of NOx is pseudo-first order as shown below. In this case, following the Lagrangian trajectory, we have the following:
where k is the reaction rate constant of NOx with OH. The reaction rate constant for NO2 and OH is cm3 s−1 at 25 ∘C and 1 atm pressure according to Sander et al. (2003). Since NO2 is part of NOx, the value of k should be scaled down by the ratio . The average of the ratio is about 0.6, thus k for NOx is prescribed at cm3 s−1.
Similarly, we have the following:
where kVOC and kco are the reaction rate constants of VOCs and CO with OH, respectively. KVOC values of individual VOCs are listed in Table S1 in the Supplement, and kco is prescribed at cm3 s−1 (Atkinson et al., 2006).
Since the Lagrangian condition is sometimes not observed, it is necessary to select the time periods during which the quasi-Lagrangian condition as shown in Fig. 2 is approximately valid. The selection criterion is that the ratio of CO concentrations between 08:00 and 13:00 LT lies within 50 % of 1 standard deviation (vertical bars) of the ratio of CO shown in Fig. 2, which is assumed to be in the Lagrangian condition. This criterion usually filters out about 60 % of data; i.e., about 40 % of the days satisfy approximately the Lagrangian condition. We have tested this selection criterion by parameterizing it between 30 % and 80 % of 1 standard deviation and found our major results are robust within this range.
2.2.5 Dilution effect
Diurnal variations of pollutants averaged over all stations and the two episodes are shown in Fig. 2. Previous studies have shown that part of the early-morning rise in O3 is due to O3 entrained from the residual layer above the boundary layer during the development of the boundary layer in the morning (Shiu et al., 2007; Zhao et al., 2019). We adopt the approach proposed by Shiu et al. (2007) to account for the dilution effects. Specifically, the reduction of CO concentrations from 08:00 to 13:00 LT (approximately 20 %) is assumed to be the dilution effect and used for all other species. The uncertainty due to this assumption is discussed in Sect. 3.5.
2.2.6 Emissions of NOx, CO and VOCs between 08:00 and 13:00 LT
Equations (5), (6) and (7) do not account for the emissions of NOx, CO or VOC during the period of 08:00–13:00 LT. Inclusion of these emissions would affect the value of OH derived from Eq. (5) as well as the dilution effect. We estimate the emission of NOx by taking advantage of the fact that NOx reaches a quasi-steady state around 13:00–16:00 LT as evident in Fig. 2. We believe that the quasi-steady state is maintained by the balance between the oxidation of NOx and its emission. This is based on the notion that oxidation of NO2 by OH is the predominant sink of NOx in 13:00–16:00 LT, of which the integration over the mixed or boundary layer should be balanced by the emission flux of NOx according to the continuous equation of NOx. Assuming the oxidation loss rate of NOx in the mixed layer is uniform with height, we obtain that the divergence of the hourly NOx emission rate is equal to the oxidation loss rate of NOx at 13:00–16:00 LT. Using the average OH of 5×106 cm−3 at noon derived from Eq. (5) (Fig. 4) and mean NOx at 13:00–16:00 LT (Fig. 2), a value of approximately 1.8 ppb h−1 can be obtained. This value is assumed to be the hourly NOx emission rate between 08:00 and 13:00 LT. The emissions of CO and VOC are calculated using their ratios to NOx in the emission inventories of Huang et al. (2021).
2.2.7 Box model
A photochemical box model with a carbon bond mechanism (PBM-CB05) (Yarwood et al., 2005; Coates and Butler, 2015; Y. Wang et al., 2017) is used to simulate the O3 production rate and OH radical. Unlike emission-based models, the PBM-CB05 used in this study is based on observed concentrations of air pollutants and meteorological parameters (Y. Wang et al., 2017). In the CB05 module, VOCs are grouped according to carbon bond type and the reactions of individual VOCs are condensed using the lumped structure technique (Yarwood et al., 2005; Coates and Butler, 2015). In this study, the pollution indicators (O3, NO, NO2, CO and VOC) and meteorological parameters (temperature, relative humidity, pressure) observed during the two episodes are utilized as input parameters for the model. There are 37 VOC species considered in our case. The model simulation starts from 07:00 and ends at 18:00 LT with hourly input data based on observed concentrations of air pollutants and meteorological parameters during the two episodes.
3.1 Air quality and meteorological conditions
Figure 3 shows the time series of hourly concentrations of air pollutants. The time period covers the two ozone episodes and extends to 2 d before and 2 d after. Mean maximum daily 8 h average (MDA8) O3 concentrations in episode 1 was 88.7 ppb, and in episode 2 was 99.6 ppb. The average daily concentration of CO of the two episodes was 0.74 and 0.85 ppm, respectively. The corresponding time series of key meteorological parameters are shown in Fig. S1 in the Supplement. As O3 is formed through photochemical reactions involving precursors NOx and VOC, strong solar radiation, high temperature and low wind speed have been identified to be common conditions conducive to the formation of ozone (Liu et al., 2017; L. Wang et al., 2018). During both O3 episodes, the weather in Guangdong was dominated by high-pressure systems with warm and cloudless conditions, and northeasterly winds. In particular, the average maximum temperature for episode 1 was 28∘ and for episode 2 was 30∘.
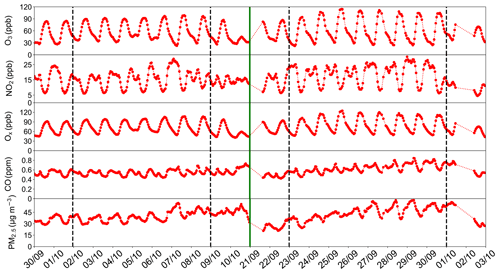
Figure 3Hourly surface concentrations of air pollutants during the study period. The green line is added to separate the two episodes; the black dashed lines indicate 2 d before and 2 d after the episodes.
The general patterns of O3 concentrations of the two episodes were similar. Relatively high O3 concentrations with northerly or northeasterly winds appeared at least 2 d before the episode in both episodes. Afterward, the high O3 kept increasing or stayed at a high level until the prevailing northeasterly wind shifted away and the surface pressure dropped. Starting on 22 September 2018, a precipitation event occurred which obviously ended the first episode. The heavier cloud cover greatly reduced the intensity of solar radiation and O3 photochemical formation reactions. The disappearance of high O3 in the second episode is believed to be related to a shift to southerly winds that brought in clean moist air from the South China Sea.
3.2 OH concentrations derived from OBM
Figure 4 shows the hourly OH concentrations between 08:00 and 13:00 LT derived from Eq. (4) based on the concentrations of NOx and CO observed at the 77 stations. Average OH concentrations derived at the 77 stations between 08:00 and 13:00 LT stay within a narrow range of 2.5×106 cm−3 to 5.5×106 cm−3 with a weak dependence on the NOx. The mean OH concentrations and their 1 standard deviations derived by the OBM (black dots and black vertical bars, respectively) are approximately 30 % higher than the mean OH concentrations and 1 standard deviations observed at a rural station in PRD in October–November 2014 (blue line and blue shade, respectively) (Tan et al., 2019). Nevertheless, there is a complete overlap of the 1 standard deviations of the two data sets (blue shade and black vertical bars), which indicates a good agreement between our OBM OH values and those observed by Tan et al. (2019). In another comparison with a previous investigation, our OH concentrations are approximately 40 % lower than the OH calculated by a box model constrained by observed air pollutants during an experiment at a remote island site in the PRD from August to November 2013 (red line and red shade) (Y. Wang et al., 2018). There is also a nearly complete overlap of the 1 standard deviations of the two data sets (red shade and black vertical bars). Figure 4 also includes the noon OH concentrations calculated by the box model described above. The box model is constrained by the ambient conditions observed during the two episodes. The average modeled OH concentration is approximately 3.2×106 cm−3 with a 1 standard deviation of 0.6×106 cm−3 (red cross and red vertical bar, respectively). This value of OH is approximately 40 % less than the OH values of cm−3 derived by the OBM at noon. Again, there is a good overlap of the 1 standard deviations of the two data sets. The agreement among the OH concentrations derived by the OBM, the box model and field observations gives credence to our observation-based analysis, at least in terms of the derived OH concentration which plays a critical role in the O3 formation.
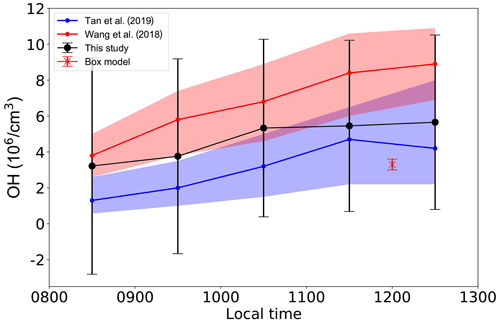
Figure 4Hourly average OH concentrations between 08:00 and 13:00 LT derived from the OBM are shown in the black line with black dots; observed OH concentrations by Tan et al. (2019) are shown in the blue line and blue shade; calculated OH concentrations by Y. Wang et al. (2018) are shown in the red line and red shade. The blue shade denotes the 25 % and 75 % percentiles of the data; the red shade indicates the 95 % confidence interval of the data. The red cross with red vertical whiskers denotes the mean OH concentration and 1 standard deviation, respectively, calculated by a box model constrained by observed ambient conditions during the two episodes.
Nevertheless, we acknowledge that the OH concentrations derived here are approximately a factor of 3 to 5 lower than the OH concentrations observed at Backgarden (a suburban site about 70 km downwind of Guangzhou) during an intensive campaign in 2006, in which the OH reached daily peak values of 15–26 × 106 cm−3 (Lu et al., 2012). This discrepancy remains unresolved.
3.3 Ozone production efficiency
Ozone production efficiency (ε) is defined as the number of O3 molecules produced per molecule of NOx (or VOC) oxidized photochemically (Liu et al., 1987; Trainer et al., 2000). ε can be calculated by the following equations:
where Δ[O3] represents the amount of ozone generated from 08:00 to 13:00 LT which is equal to the observed difference in O3 between 08:00 and 13:00 LT, after adjustment to the dilution factor. Δ[NOx] (Δ[VOC]) represents the consumption and oxidation of NOx (VOC) between 08:00 and 13:00 LT.
Figure 5a shows the relationship of ε as a function of the average NOx concentration between 08:00 and 13:00 LT. As expected ε is greater at lower NOx; i.e., the O3 production efficiency is greater in rural and suburban environments than urban conditions, in agreement with previous findings (Liu et al., 1987; Kleinman et al., 2002). The value of ε(NOx) converges to a narrow range of about 1.0±0.5 when NOx is greater than 70 ppb. This range of ε(NOx) in Fig. 5a is consistent with previous investigations in urban environments (Sillman et al., 1998; Daum et al., 2000) as well as in rural environments (Chin et al., 1994; Trainer et al., 1995). Compared to previous investigations in PRD areas, values in Fig. 5a at NOx higher than 20 ppb are in good agreement with the ε(NOx) values of 2.1–2.5 found at urban stations in PRD by Yu et al. (2020) and Lu et al. (2010b). However, ε(NOx) values of 6.0–13.3 were found at rural stations in PRD (Lu et al., 2010b; Wei et al., 2012; Xu et al., 2015; Yang et al., 2017), which are about a factor of 2 higher than our values at low NOx. Considering that our values are derived for two ozone pollution episodes in which the ε(NOx) should be higher than non-episode periods, this discrepancy is puzzling. Figure 5b is the same as Fig. 5a except that the x axis is changed to Δ[NOx] or the oxidized NOx. Figure 5b shows a relatively smoother distribution compared to Fig. 5a, most likely because the oxidized NOx, rather than NOx itself, is more closely related photochemically to Δ[O3]. As Δ[NOx] increases beyond 30 ppb, ε[NOx] levels off linearly to a nearly constant value around 1.0 when Δ[NOx] approaches 80 ppb (Fig. 5b). ε[VOC] is also greater at lower Δ[VOC] and has an asymptotic value of about 1.0±0.5 when Δ[VOC] becomes greater than 50 ppb (Fig. 5c).
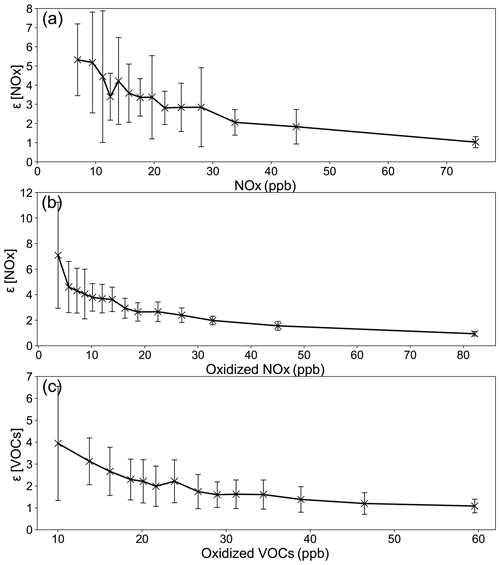
Figure 5Ozone production efficiency plotted as a function of NOx (a), oxidized NOx (b) and oxidized VOC (c).
Figure 5b and c have some useful implications for the ozone control strategy. For instance, ε[NOx]=1.7 when Δ[NOx]=50 ppb can be interpreted as in a highly polluted ambient environment in Guangdong where Δ[NOx] equals 50 ppb, approximately 1.7 ppb of ozone is produced for each ppb of NOx oxidized. The overall average value of ε[NOx] is about 3.0 (Fig. 5b), which implies on average 3.0 ozone molecules are produced for each NOx molecule oxidized. The overall average value of ε[VOC] is approximately 2.1 (Fig. 5c), which implies 2.1 ozone molecules are produced for each VOC molecule oxidized, which is about 50 % less efficient than that of NOx.
Photochemical oxidation of a VOC molecule under common ambient urban conditions produces approximately two or more peroxyl radicals – one HO2 and more than one RO2 (Seinfeld and Pandis, 1998; Jacob, 1999). Because there is abundant NOx in the ambient atmosphere in Guangdong, nearly all peroxyl radicals are expected to react with NO to produce NO2 and then O3. Jacob (1999) suggested an ozone formation rate of 2Δ[VOC] in the urban atmosphere. This is in excellent agreement with the overall value of 2.1Δ[VOC] found here by the OBM. This agreement, as well as the consistency with previous investigations on the ε[NOx], provides credence again to the observation-based analysis of this study.
3.4 Ozone sensitivity to precursors
The sensitivity of ozone formation (ΔO3) to ozone precursor NOx is examined in Fig. 6a, in which ΔO3 (right-hand side in red) and the oxidized VOC (left-hand side in black) are plotted as a function of the oxidized NOx. Similarly in Fig. 6b ΔO3 (right-hand side in red) and the oxidized NOx (left-hand side in black) are plotted as a function of the oxidized VOC. It can be seen in Fig. 6a that ΔO3 increases with the value of oxidized NOx. The increase first has a very sharp slope of about 2.0 ppb ppb−1 when oxidized NOx is below 30 ppb, indicating a strong sensitivity of ozone formation to oxidized NOx. The slope flattens out quickly to around 0.2 ppb ppb−1 when oxidized NOx gets greater than 30 ppb, suggesting other factors such as VOC and the ratio may become more important in controlling the ozone formation rate. Figure 6b shows that ΔO3 increases with the value of oxidized VOC with a slope of about 0.4 ppb ppb−1. However, this slope is much smaller than that of NOx, especially in the low oxidized NOx regime (<30 ppb). In a brief summary for Fig. 6a and b, the ozone formation is most sensitive to the oxidized NOx in relatively clean regimes of oxidized NOx < 30 ppb. In more polluted regimes, other factors such as the initial VOC and/or the ratio appear to have a significant impact on the ozone formation. Additional evidence in support of these points is elaborated below.
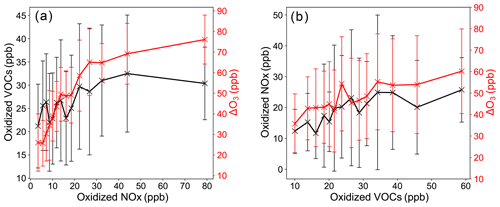
Figure 6Ozone formation rate (ΔO3, right-hand side in red) and the oxidized VOC (left-hand side in black) plotted as a function of oxidized NOx (a). Ozone formation rate (ΔO3, right-hand side in red) and oxidized NOx (left-hand side in black) plotted as a function of the oxidized VOC (b).
Figure S2 presents a three-dimensional EKMA-like depiction of ozone formation rates (ΔO3, black dots, 471 points) plotted as a function of the oxidized NOx (x axis) and oxidized (VOCs + CO) (y axis). The colored plane is a linear regression to the ozone formation rates (black dots), and the green and red bars denote positive and negative deviations of individual dots from the plane, respectively. Different color shades from blue to red denote different concentrations of ΔO3 in ppb. The equation for [ΔO3] represents the plane as a function of the oxidized NOx (ΔNOx) and oxidized VOC (ΔVOC). The coefficients in front of ΔNOx and ΔVOC in the equation are the ozone sensitivities to ΔNOx and ΔVOC, respectively. The plane fits the black dots (ozone formation rates) reasonably well with an R2 value of 0.423. The coefficient of ΔNOx is 0.755 which is about 3 times of that of ΔVOC (0.247), indicating the ozone formation rate is about 3 times more sensitive to ΔNOx than ΔVOC when considering all data at the 77 stations in Guangdong during the two episodes. This is consistent with the findings from Fig. 6a and b.
Some uneven congregations of red and green bars appear; e.g., a large number of red bars have low values of ΔNOx, while many green bars tend to have moderate values of ΔNOx and high values of ΔVOC. This suggests that there is a need to divide Fig. S2 into different congregations or regimes. Figure 7 is the same as Fig. S2 except it is divided into four quadrants of different levels of oxidized ozone precursors: panel (a) shows low ΔNOx and low ΔVOC (ΔNOx<20 ppb, ΔVOC<25 ppb), panel (b) shows high ΔNOx and high ΔVOC (ΔNOx>20 ppb, ΔVOC>25 ppb), panel (c) shows low ΔNOx and high ΔVOC (ΔNOx<20 ppb, ΔVOC>25 ppb), panel (d) shows high ΔNOx and low ΔVOC (ΔNOx>20 ppb, ΔVOC<25 ppb).
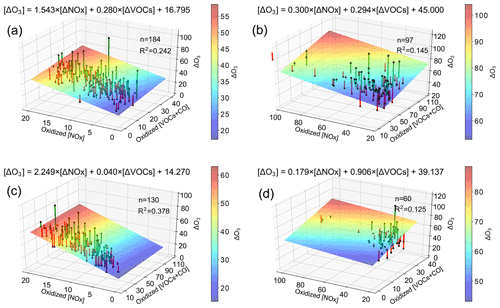
Figure 7Three-dimensional depiction of ozone formation rate (ΔO3, z axis) plotted as a function of oxidized NOx (x axis) and oxidized VOC (y axis). The black dots denote values of ΔO3, the colored plane is the best linear fit to the black dots, and the green and red bars denote positive and negative deviations from the plane, respectively. The equation listed represents the surface as a function of oxidized NOx and oxidized VOC. R2 is the square of correlation coefficient of the linear regression. Four quadrants: (a) low NOx and low VOC (NOx < 20 ppb, VOC < 25 ppb), (b) high NOx and high VOC (NOx > 20 ppb, VOC > 25 ppb), (c) low NOx and high VOC (NOx < 20 ppb, VOC > 25 ppb), (d) high NOx and low VOC (NOx > 20 ppb, VOC < 25 ppb).
In total, 39 % of all data points (184 out of 471 points) lie in panel (a), the slope of ΔO3 against ΔNOx (coefficient of ΔNOx in the equation) is approximately 1.54 ppb ppb−1 (p value < 0.01), while the slope of ΔO3 against ΔVOC (coefficient of ΔVOC) has a value of 0.28 ppb ppb−1 (p value = 0.021). These values of slopes imply that the ozone formation at stations in panel (a), a relatively clean environment, is about 5 times more sensitive to ΔNOx than ΔVOC; i.e., the ozone formation is NOx-limited. This is in good agreement with the conclusion reached based on Figs. 6a, b and S2. Panel (b) contains about 20 % of the data points. The coefficient of ΔNOx is 0.3 ppb ppb−1 (p value < 0.01), while the coefficient of ΔVOC is 0.29 ppb ppb−1 (p value = 0.043), suggesting that the ozone formation is sensitive to both ΔVOC and ΔNOx. This quadrant belongs to the transitional regime. Panel (c) has 28 % of the data points, and the coefficients of ΔNOx and ΔVOC are 2.25 ppb ppb−1 (p value < 0.01) and 0.04 ppb ppb−1 (p value = 0.785), respectively. Here again the ozone formation is NOx-limited. Panel (d) has 13 % of the data points, the coefficients of ΔNOx and ΔVOC are 0.18 ppb ppb−1 (p value = 0.126) and 0.91 ppb ppb−1 (p value = 0.037), respectively. These values of coefficients indicate that the ozone formation is more sensitive to ΔVOC than ΔNOx; i.e., the ozone formation is VOC-limited.
The analysis above provides an observation-based method for evaluating the ozone-precursor sensitivity. This method has the potential to provide quantitative information on the ozone control strategy for individual regions. In theory, the quadrants can be further divided into, for example, a specific region represented by individual stations, such that an ozone control strategy suitable to the region could be developed. In practice, this is limited by the data available for making the three-dimensional plot like Fig. 7.
We have compared the OBM results to those of the box model constrained by the observed ambient environment in this study. Figure 8 shows the traditional 2D-EKMA plot calculated by the model. To facilitate the comparison, the x axis and y axis in Fig. 8 are changed to hourly oxidized NOx and oxidized VOC, respectively, rather than the usual early-morning concentrations of NOx and VOC. The modeled results are shown in colored isopleths of ozone increments between 06:00 and 16:00 LT, while results of the OBM are shown in colored dots for ozone increment or formation between 08:00 and 13:00 LT. The difference in the length of time has a negligible effect on the ozone increment as evident in Fig. 2. The OBM values agree with the model results semi-quantitatively. For instance, the colored dots of OBM shift from blue (20 ppb) to green (60–80 ppb) consistently with the colored isopleths, but the OBM dots rarely turn yellow when modeled isopleths become greater than 90 ppb. Two red lines (left red and right red) are added to Fig. 8 to facilitate the assessment of the sensitivity of ozone formation. There are 127 points located to the left of left red line, which clearly belongs to the NOx-limited regime according to the modeled ozone isopleths. There are 141 points located to the right of right red line, which clearly belongs to the VOC-limited regime according to the modeled ozone isopleths. In between the two red lines there are 203 points, which are in the transitional regime sensitive to both NOx and VOC. These three regimes overlap and agree in ozone formation sensitivity with panels (a) and (c), panel (d) and panel (b) of the OBM results, respectively. However, the numbers of points in the three regimes deviate significantly from those of the four OBM quadrants. For example, panel (b) has only 97 points compared to the 203 points in the transitional regime of Fig. 8; panel (d) has only 60 points compared to the 141 points in the VOC-limited regime of Fig. 8; while panels (a) and (c) have 314 points compared to the 127 points in an NOx-limited regime of Fig. 8. In terms of ozone sensitivity, the modeled results show a nearly equal number of points in the NOx-limited regime as the VOC-limited regime, while the OBM results show five to one in favor of the NOx-limited regime. A quantitative agreement between the OBM results (dots) and the modeling results (isopleths) would require shifting the dots in Fig. 8 leftward by approximately 0.5–1 ppbv h−1, which would mean a reduction of OH by approximately 30 %–50 %. Interestingly this requirement matches well with the fact that modeled OH is approximately 40 % less than the OH value derived by the OBM at noon as shown in Fig. 4.
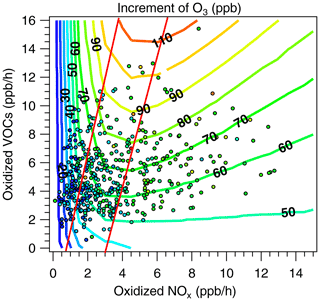
Figure 8Ozone isopleths (in ppbv) of traditional 2D-EKMA plot for the two episodes calculated by the box model are shown in colored lines, ozone concentrations at 13:00 LT derived by the OBM are shown in colored dots, and the x axis and y axis are hourly oxidized NOx and oxidized VOC, respectively.
Comparing with previous studies, we notice that almost all previous researches suggested that limiting the emission of VOCs in Guangdong would have a positive role in reducing ozone reduction (Zhang et al., 2008; T. Wang et al., 2017; Jiang et al., 2018), but different results may appear in different places and time. Yu et al. (2020) found that NOx reduction in Shenzhen has led to higher ozone production from 2015 to 2018 given the nearly constant VOCs. However, the ozone mitigation would be benefit from further NOx reduction under the conditions of 2018. Yang et al. (2019) analyzed the relationship between ozone and precursors in PRD from 2007 to 2017 and found that the northeastern PRD was NOx-limited and the southwest VOC-limited. Obviously, these findings are in general different from our results except in a highly polluted environment like panel (b). Some of the difference can be explained by the fact that most of the previous studies were focused on urban regions, while many rural stations are included in our OBM analysis. Finally, we acknowledge that our results are based on the analysis of only two multi-day ozone episodes which maybe not representative of the general ambient environment in Guangdong. A comprehensive regional and temporal OBM analysis is needed to make a definitive comparison with previous findings.
In summary of Sect. 3.4, the sensitivity of ozone formation to its precursors is complex and highly dependent on the ambient conditions of the station day. Our OBM shows that approximately 67 % of the station days exhibit ozone formation sensitivity to NOx, approximately 20 % of the station days are in the transitional regime sensitive to both NOx and VOC; only approximately 13 % of the station days are sensitive to VOC. These findings are different from results of most previous studies, which favor ozone formation sensitivity to VOC.
3.5 Uncertainty analysis
Significant uncertainties and limitations exist in our OBM analysis. First and foremost is the uncertainty involved with the Lagrangian air mass assumption, which does not take into account mixing, entrainment or surface deposition effects. Omitting the mixing of NOx emitted between 08:00 and 13:00 LT into the Lagrangian air mass can lead to an underestimate of the OH concentration, while omitting the mixing of CO emission can underestimate the dilution effect. We account for the mixing of NOx emission by assuming that NOx reached a quasi-steady state around 13:00–16:00 LT (Sect. 2.2.6), and in turn the mixing of CO and VOC emissions are calculated using their ratios to NOx in the emission inventories of Huang et al. (2021). However, no surface deposition effect is included. The selection criterion defined by 50 % of 1 standard deviation (1.0±0.5σ) from the mean CO distribution works well in filtering out those data deviating significantly from the Lagrangian condition. However, the criterion filters out about 60 % of the data, thus limiting the representativeness of the OBM analysis. This limitation has been evaluated by relaxing the selection criterion to 1.0±0.8σ, which filters out only about 30 % of the data. No significant difference has been detected, suggesting the results of the OBM analysis are representative of the majority of the data. Another source of uncertainty is that one single dilution factor is adopted for all air pollutants, including O3, CO, PM2.5 and NOx. In this context, it is reassuring to find out that the dilution factors derived independently from CO and PM2.5 agree within 10 % with each other. In a brief summary, we estimate the uncertainty involved with the Lagrangian assumption to be in the range of 20 %–40 %.
The second largest source of uncertainty is the evaluation of VOCs. Individual VOCs, including OVOCs, are calculated based on the observed concentration of CO and the ratio of in the emission inventories as discussed in Sect. 2.2.2. We have evaluated the VOCs and OVOCs derived this way by comparing their contributions to the OH reactivity observed by Tan et al. (2019) in PRD in autumn 2014. There is a reasonable agreement between our estimates of the contributions of NOx, CO, OVOCs and VOCs to the OH reactivity and those of Tan et al. (2019) except for a 35 % underestimation of VOCs. Hence we estimate the uncertainty in the evaluation of VOCs to be in the range of 30 %–50 %.
Another source of uncertainty may come from the neglection of heterogeneous reactions in this study. The largest impact of neglecting heterogeneous reactions is most likely to involve NOx between 08:00 and 13:00 LT, during which the OH is derived. Since the effect of heterogeneous reactions is included in the observations, the neglection of any heterogeneous removal of NOx (e.g., deposition of NOx on aerosols in the humid conditions in Guangdong) can lead to an overestimate of OH concentrations by the OBM. This would have a significant impact on the outcome of this study, as OH plays a critical role in the photochemistry of NOx, VOCs and ozone. On the other hand, presence of significant natural sources of NOx such as biogenic emission and/or lightning source in 08:00–13:00 LT would lead to an underestimate of OH concentration.
Finally, another source of uncertainty is attributable to the coarse resolution of CO measurements which is reported at 0.1 ppm intervals. As a result, many hourly CO data would show identical values and lose their value as a tracer.
In this study, two persistent elevated ozone episodes in Guangdong (77 stations) that occurred on 2–8 October 2018 and 24 September–1 October 2019 were analyzed to investigate the sensitivity of ozone generation to precursor concentrations at the 77 stations. An OBM is developed by modifying the approach suggested by Shiu et al. (2007). Specifically, NOx and CO are used in this OBM to substitute for the two hydrocarbon species utilized in Shiu et al. (2007).
Major outputs from the OBM include the OH concentrations, O3 production efficiency and the sensitivity of ozone formation to the precursors at the 77 stations during the two ozone episodes. The average OH concentrations between 08:00 and 13:00 LT agree well with the OH values observed at a rural station in PRD in October–November 2014 by Tan et al. (2019). The OH values derived from the OBM are also in good agreement with a box model constrained by the ambient conditions observed during the two episodes. On the other hand, the OH concentrations derived here are approximately a factor of 2 to 4 lower than the OH concentrations observed at Backgarden, a suburban site about 70 km downwind of Guangzhou (Lu et al., 2012).
The O3 production efficiency against NOx, , is greater at lower NOx (Fig. 5a), in agreement with previous findings (Liu et al., 1987; Kleinman et al., 2002). The value of ε converges to a narrow range of about 1.0±0.5 when NOx is greater than 70 ppb. This range of ε(NOx) is consistent with previous investigations in urban environments (Sillman et al., 1998; Daum et al., 2000) as well as in rural environments (Chin et al., 1994; Trainer et al., 1995). Compared to previous investigations in PRD areas, our values of ε(NOx) at NOx higher than 20 ppb are in good agreement with the values of 2.1–2.5 found at urban stations in PRD by Yu et al. (2020) and Lu et al. (2010b). However, ε(NOx) values of 6.0–13.3 were found at rural stations in PRD (Lu et al., 2010b; Wei et al., 2012; Xu et al., 2015; Yang et al., 2017), which are about a factor of 2 higher than our values at low NOx. Considering that our values are derived for two ozone pollution episodes in which the ε(NOx) should be higher than non-episode periods, this discrepancy is puzzling. The overall average value of ε[NOx] is about 3.0 (Fig. 5b), which implies on average three ozone molecules are produced for each NOx molecule oxidized. The overall average value of ε[VOC] is approximately 2.1 (Fig. 5c), which implies 2.1 ozone molecules are produced for each VOC molecule oxidized, about 50 % less efficient than that of NOx. Jacob (1999) suggested an ozone formation rate of 2Δ[VOC] in the urban atmosphere. This is in excellent agreement with the value of 2.1Δ[VOC] found here by the OBM. This agreement, as well as the consistency with previous investigations on the ε[NOx] and OH concentrations, provides credence to the observation-based analysis (OBM) of this study.
The sensitivity of ozone formation to its precursors is complex and highly dependent on the ambient conditions of the station day. Our OBM shows that approximately 67 % of the station days exhibit ozone formation sensitivity to NOx, approximately 20 % of the station days are in the transitional regime sensitive to both NOx and VOC, and only approximately 13 % of the station days are sensitive to VOC. These findings are different from results of most previous studies, which favor ozone formation sensitivity to VOC. Some of the difference can be explained by the fact that most of the previous studies were focused on urban regions, while many rural stations are included in our OBM analysis. Finally, we acknowledge that our results are based on the analysis of only two multi-day ozone episodes which may not be representative of the general ambient environment in Guangdong. A comprehensive spatial and temporal OBM analysis is needed to make a definitive comparison with previous findings.
Hourly surface O3, PM2.5, CO and NO2 data were obtained from China National Environmental Centre (http://www.cnemc.cn/en/, CNEMC, 2021). Hourly meteorological data were obtained from European Centre for Medium-Range Weather Forecasts ERA5 reanalysis (https://doi.org/10.24381/cds.adbb2d47, Hersbach et al., 2018). The data presented in this publication are available at the following DOI: https://doi.org/10.6084/m9.figshare.20055221 (Song et al., 2022).
The supplement related to this article is available online at: https://doi.org/10.5194/acp-22-8403-2022-supplement.
SCL proposed the essential research idea. KS performed the analysis. KS, RL and SCL drafted the manuscript. YuW, TL, LW, YaW, JZ and BW helped with the analysis and offered valuable comments. All authors have read and agreed to the published version of the paper.
The contact author has declared that neither they nor their co-authors have any competing interests.
Publisher's note: Copernicus Publications remains neutral with regard to jurisdictional claims in published maps and institutional affiliations.
The authors thank the China National Environmental Centre and European Centre for Medium-Range Weather Forecasts for providing data sets that made this work possible. We also acknowledge the support of the Institute for Environmental and Climate Research and Guangdong-Hongkong-Macau Joint Laboratory of Collaborative Innovation for Environmental Quality in Jinan University. We are grateful to the two anonymous reviewers for their thoughtful reviews, which led to an improved revised manuscript.
This research was supported by the National Natural Science Foundation of China (grant nos. 92044302 and 41805115); the Guangzhou Municipal Science and Technology Project, China (grant no. 202002020065); the Special Fund Project for Science and Technology Innovation Strategy of Guangdong Province (grant no. 2019B121205004); the Guangdong Innovative and Entrepreneurial Research Team Program (grant no. 2016ZT06N263); and the National Key Research and Development Program of China (grant no. 2018YFC0213906).
This paper was edited by Andreas Hofzumahaus and reviewed by two anonymous referees.
Atkinson, R.: Gas-phase tropospheric chemistry of organic compounds: a review, Atmos. Environ., 24, 1–41, https://doi.org/10.1016/0960-1686(90)90438-S, 1990.
Atkinson, R., Baulch, D. L., Cox, R. A., Crowley, J. N., Hampson, R. F., Hynes, R. G., Jenkin, M. E., Rossi, M. J., Troe, J., and IUPAC Subcommittee: Evaluated kinetic and photochemical data for atmospheric chemistry: Volume II – gas phase reactions of organic species, Atmos. Chem. Phys., 6, 3625–4055, https://doi.org/10.5194/acp-6-3625-2006, 2006.
Calvert, J. G.: Test of the theory of ozone generation in Los Angeles atmosphere, Environ. Sci. Technol., 10, 248–256, https://doi.org/10.1021/es60114a002, 1976.
Chang, C.-C., Yak, H.-K., and Wang, J.-L.: Consumption of hydrocarbons and its relationship with ozone formation in two Chinese megacities, Atmosphere, 11, 326, https://doi.org/10.3390/atmos11040326, 2020.
Cheng, H., Guo, H., Wang, X., Saunders, S. M., Lam, S. H., Jiang, F., Wang, T., Ding, A., Lee, S., and Ho, K. F.: On the relationship between ozone and its precursors in the Pearl River Delta: application of an observation-based model (OBM), Environ. Sci. Pollut. R., 17, 547–560, https://doi.org/10.1007/s11356-009-0247-9, 2010.
Chin, M., Jacob, D. J., Munger, J. W., Parrish, D. D., and Doddridge, B. G.: Relationship of ozone and carbon monoxide over North America, J. Geophys. Res.-Atmos., 99, 14565–14573, https://doi.org/10.1029/94JD00907, 1994.
CNEMC: China National Environmental Centre, http://www.cnemc.cn/en/, last access: 10 November 2021.
Coates, J. and Butler, T. M.: A comparison of chemical mechanisms using tagged ozone production potential (TOPP) analysis, Atmos. Chem. Phys., 15, 8795–8808, https://doi.org/10.5194/acp-15-8795-2015, 2015.
Daum, P. H., Kleinman, L., Imre, D. G., Nunnermacker, L. J., Lee, Y. N., Springston, S. R., Newman, L., and Weinstein-Lloyd, J.: Analysis of the processing of Nashville urban emissions on July 3 and July 18, 1995, J. Geophys. Res.-Atmos., 105, 9155–9164, https://doi.org/10.1029/1999jd900997, 2000.
Department of Ecology and Environment of Guangdong Province: 2015 Report on the state of Guangdong provincial environment, 38 pp., http://gdee.gd.gov.cn/attachment/0/484/484303/2335528.pdf (last access: 29 June 2022), 2016 (in Chinese).
Department of Ecology and Environment of Guangdong Province: 2020 Report on the state of Guangdong provincial ecology and environment, 56 pp., http://gdee.gd.gov.cn/attachment/0/483/483983/3266052.pdf (last access: 29 June 2022), 2021 (in Chinese).
Dodge, M. C.: Combined use of modeling techniques and smog chamber data to derive ozone precursor relationships, in: Proceedings of the International Conference on Photochemical Oxidant Pollution and its Control, edited by: Dimitriades, B., Vol. II, EPA-600/3-77-0016, Health & Environmental Research Online ID 38646, US EPA, RTP, NC, 881–889, 1977.
Heald, C. L., Jacob, D. J., Fiore, A. M., Emmons, L. K., Gille, J. C., Deeter, M. N., Warner, J., Edwards, D. P., Crawford, J. H., Hamlin, A. J., Sachse, G. W., Browell, E. V., Avery, M. A., Vay, S. A., Westberg, D. J., Blake, D. R., Singh, H. B., Sandholm, S. T., Talbot, R. W., and Fuelberg, H. E.: Asian outflow and trans-Pacific transport of carbon monoxide and ozone pollution: An integrated satellite, aircraft, and model perspective, J. Geophys. Res.-Atmos., 108, 4804, https://doi.org/10.1029/2003jd003507, 2003.
Hersbach, H., Bell, B., Berrisford, P., Biavati, G., Horányi, A., Muñoz Sabater, J., Nicolas, J., Peubey, C., Radu, R., Rozum, I., Schepers, D., Simmons, A., Soci, C., Dee, D., Thépaut, J.-N.: ERA5 hourly data on single levels from 1959 to present, Copernicus Climate Change Service (C3S) Climate Data Store (CDS) [data set], https://doi.org/10.24381/cds.adbb2d47, 2018.
Hsu, Y.-K., VanCuren, T., Park, S., Jakober, C., Herner, J., FitzGibbon, M., Blake, D. R., and Parrish, D. D.: Methane emissions inventory verification in southern California, Atmos. Environ., 44, 1–7, https://doi.org/10.1016/j.atmosenv.2009.10.002, 2010.
Huang, Z., Zhong, Z., Sha, Q., Xu, Y., Zhang, Z., Wu, L., Wang, Y., Zhang, L., Cui, X., Tang, M., Shi, B., Zheng, C., Li, Z., Hu, M., Bi, L., Zheng, J., and Yan, M.: An updated model-ready emission inventory for Guangdong Province by incorporating big data and mapping onto multiple chemical mechanisms, Sci. Total Environ., 769, 144535, https://doi.org/10.1016/j.scitotenv.2020.144535, 2021.
IPCC: Climate Change 2013: The Physical Science Basis. Contribution of Working Group I to the Fifth Assessment Report of the Intergovernmental Panel on Climate Change, edited by: Stocker, T. F., Qin, D., Plattner, G.-K., Tignor, M., Allen, S. K., Boschung, J., Nauels, A., Xia, Y., Bex, V., and Midgley, P. M., Cambridge University Press, Cambridge, United Kingdom and New York, NY, USA, 1535 pp., https://www.ipcc.ch/site/assets/uploads/2018/02/WG1AR5_all_final.pdf (last access: 29 June 2022), 2013.
Jacob, D. J.: Introduction to Atmospheric Chemistry, Princeton University Press, Princeton, NJ, http://www.jstor.org/stable/j.ctt7t8hg (last access: 29 June 2022), 1999.
Jiang, M., Lu, K., Su, R., Tan, Z., Wang, H., Li, L., Fu, Q., Zhai, C., Tan, Q., Yue, D., Chen, D., Wang, Z., Xie, S., Zeng, L., and Zhang, Y.: Ozone formation and key VOCs in typical Chinese city clusters, Chinese Sci. Bull., 63, 1130–1141, 2018 (in Chinese).
Kleinman, L. I.: The dependence of tropospheric ozone production rate on ozone precursors, Atmos. Environ., 39, 575–586, https://doi.org/10.1016/j.atmosenv.2004.08.047, 2005.
Kleinman, L. I., Lee, Y.-N., Springston, S. R., Nunnermacker, L., Zhou, X., Brown, R., Hallock, K., Klotz, P., Leahy, D., Lee, J. H., and Newman, L.: Ozone formation at a rural site in the southeastern United States, J. Geophys. Res.-Atmos., 99, 3469–3482, https://doi.org/10.1029/93JD02991, 1994.
Kleinman, L. I., Daum, P. H., Lee, Y.-N., Nunnermacker, L. J., Springston, S. R., Weinstein-Lloyd, J., and Rudolph, J.: Sensitivity of ozone production rate to ozone precursors, Geophys. Res. Lett., 28, 2903–2906, https://doi.org/10.1029/2000gl012597, 2001.
Kleinman, L. I., Daum, P. H., Lee, Y.-N., Nunnermacker, L. J., Springston, S. R., Weinstein-Lloyd, J., and Rudolph, J.: Ozone production efficiency in an urban area, J. Geophys. Res.-Atmos., 107, ACH 23-1–ACH 23-12, https://doi.org/10.1029/2002jd002529, 2002.
Lei, W., Zhang, R., Tie, X., and Hess, P.: Chemical characterization of ozone formation in the Houston-Galveston area: A chemical transport model study, J. Geophys. Res.-Atmos., 109, D12301, https://doi.org/10.1029/2003jd004219, 2004.
Li, K., Chen, L., Ying, F., White, S. J., Jang, C., Wu, X., Gao, X., Hong, S., Shen, J., Azzi, M., and Cen, K.: Meteorological and chemical impacts on ozone formation: A case study in Hangzhou, China, Atmos. Res., 196, 40–52, https://doi.org/10.1016/j.atmosres.2017.06.003, 2017.
Lin, Y., Jiang, F., Zhao, J., Zhu, G., He, X., Ma, X., Li, S., Sabel, C. E., and Wang, H.: Impacts of O3 on premature mortality and crop yield loss across China, Atmos. Environ., 194, 41–47, https://doi.org/10.1016/j.atmosenv.2018.09.024, 2018.
Liu, J., Wu, D., Fan, S., Liao, Z., and Deng, T.: Impacts of precursors and meteorological factors on ozone pollution in Pearl River Delta, China Environ. Sci., 37, 813–820, 2017 (in Chinese).
Liu, S. C., Trainer, M., Fehsenfeld, F. C., Parrish, D. D., Williams, E. J., Fahey, D. W., Hübler, G., and Murphy, P. C.: Ozone production in the rural troposphere and the implications for regional and global ozone distributions, J. Geophys. Res.-Atmos., 92, 4191–4207, https://doi.org/10.1029/JD092iD04p04191, 1987.
Lu, K. D., Zhang, Y., Su, H., Brauers, T., Chou, C. C., Hofzumahaus, A., Liu, S. C., Kita, K., Kondo, Y., Shao, M., Wahner, A., Wang, J., Wang, X., and Zhu, T.: Oxidant (O3 + NO2) production processes and formation regimes in Beijing, J. Geophys. Res.-Atmos., 115, D07303, https://doi.org/10.1029/2009jd012714, 2010a.
Lu, K., Zhang, Y., Su, H., Shao, M., Zeng, L., Zhong, L., Xiang, Y., Chang, C. C., Chou, C. K. C., and Andreas, W.: Regional ozone pollution and key controlling factors of photochemical ozone production in Pearl River Delta during summer time, Sci. China Chem., 53, 651–663, https://doi.org/10.1007/s11426-010-0055-6, 2010b.
Lu, K. D., Rohrer, F., Holland, F., Fuchs, H., Bohn, B., Brauers, T., Chang, C. C., Häseler, R., Hu, M., Kita, K., Kondo, Y., Li, X., Lou, S. R., Nehr, S., Shao, M., Zeng, L. M., Wahner, A., Zhang, Y. H., and Hofzumahaus, A.: Observation and modelling of OH and HO2 concentrations in the Pearl River Delta 2006: a missing OH source in a VOC rich atmosphere, Atmos. Chem. Phys., 12, 1541–1569, https://doi.org/10.5194/acp-12-1541-2012, 2012.
Mao, J., Ren, X., Chen, S., Brune, W. H., Chen, Z., Martinez, M., Harder, H., Lefer, B., Rappenglück, B., Flynn, J., and Leuchner, M.: Atmospheric oxidation capacity in the summer of Houston 2006: Comparison with summer measurements in other metropolitan studies, Atmos. Environ., 44, 4107–4115, https://doi.org/10.1016/j.atmosenv.2009.01.013, 2010.
Sander, S. P., Friedl, R. R., Ravishankara, A. R., Golden, D. M., Kolb, C. E., Kurylo, M. J., Huie, R. E., Orkin, V. L., Molina, M. J., Moortgat, G. K., and Finlayson-Pitts, B. J.: Chemical Kinetics and Photochemical Data for Use in Atmospheric Studies Evaluation Number 14, JPL Publication 02-25, 2003.
Seinfeld, J. H. and Pandis, S. N.: Atmospheric Chemistry and Physics: From Air Pollution to Climate Change, John Wiley & Sons, Inc., 1998.
Shao, M., Zhang, Y., Zeng, L., Tang, X., Zhang, J., Zhong, L., and Wang, B.: Ground-level ozone in the Pearl River Delta and the roles of VOC and NOx in its production, J. Environ. Manage., 90, 512–518, https://doi.org/10.1016/j.jenvman.2007.12.008, 2009.
Shao, M., Huang, D., Gu, D., Lu, S., Chang, C., and Wang, J.: Estimate of anthropogenic halocarbon emission based on measured ratio relative to CO in the Pearl River Delta region, China, Atmos. Chem. Phys., 11, 5011–5025, https://doi.org/10.5194/acp-11-5011-2011, 2011.
Shiu, C.-J., Liu, S. C., Chang, C.-C., Chen, J.-P., Chou, C. C. K., Lin, C.-Y., and Young, C.-Y.: Photochemical production of ozone and control strategy for Southern Taiwan, Atmos. Environ., 41, 9324–9340, https://doi.org/10.1016/j.atmosenv.2007.09.014, 2007.
Sillman, S., He, D., Pippin, M. R., Daum, P. H., Imre, D. G., Kleinman, L. I., Lee, J. H., and Weinstein-Lloyd, J.: Model correlations for ozone, reactive nitrogen, and peroxides for Nashville in comparison with measurements: Implications for O3-NOx-hydrocarbon chemistry, J. Geophys. Res.-Atmos., 103, 22629–22644, https://doi.org/10.1029/98jd00349, 1998.
Sillman, S., Vautard, R., Menut, L., and Kley, D.: O3-NOx-VOC sensitivity and NOx-VOC indicators in Paris: Results from models and atmospheric pollution over the Paris area (ESQUIF) measurements, J. Geophys. Res.-Atmos., 108, 8563, https://doi.org/10.1029/2002jd001561, 2003.
Singh, H. B.: Atmospheric halocarbons: evidence in favor of reduced average hydroxyl radical concentration in the troposphere, Geophys. Res. Lett., 4, 101–104, https://doi.org/10.1029/GL004i003p00101, 1977.
Song, C., Wu, L., Xie, Y., He, J., Chen, X., Wang, T., Lin, Y., Jin, T., Wang, A., Liu, Y., Dai, Q., Liu, B., Wang, Y.-N., and Mao, H.: Air pollution in China: Status and spatiotemporal variations, Environ. Pollut., 227, 334–347, https://doi.org/10.1016/j.envpol.2017.04.075, 2017.
Song, K., Liu, R., Wang, Y., Liu, T., Wei, L., Wu, Y., Zheng, J., Wang, B., and Liu, S. C.: Ozone, Figshare [data set], https://doi.org/10.6084/m9.figshare.20055221, 2022.
Tan, Z., Lu, K., Hofzumahaus, A., Fuchs, H., Bohn, B., Holland, F., Liu, Y., Rohrer, F., Shao, M., Sun, K., Wu, Y., Zeng, L., Zhang, Y., Zou, Q., Kiendler-Scharr, A., Wahner, A., and Zhang, Y.: Experimental budgets of OH, HO2, and RO2 radicals and implications for ozone formation in the Pearl River Delta in China 2014, Atmos. Chem. Phys., 19, 7129–7150, https://doi.org/10.5194/acp-19-7129-2019, 2019.
Tang, X., Wang, Z., Zhu, J., Gbaguidi, A. E., Wu, Q., Li, J., and Zhu, T.: Sensitivity of ozone to precursor emissions in urban Beijing with a Monte Carlo scheme, Atmos. Environ., 44, 3833–3842, https://doi.org/10.1016/j.atmosenv.2010.06.026, 2010.
Tang, X., Zhu, J., Wang, Z. F., Wang, M., Gbaguidi, A., Li, J., Shao, M., Tang, G. Q., and Ji, D. S.: Inversion of CO emissions over Beijing and its surrounding areas with ensemble Kalman filter, Atmos. Environ., 81, 676–686, https://doi.org/10.1016/j.atmosenv.2013.08.051, 2013.
Thielmann, A., Prévôt, A. S., and Staehelin, J.: Sensitivity of ozone production derived from field measurements in the Italian Po basin, J. Geophys. Res.-Atmos., 107, LOP 7-1–LOP 7-10, https://doi.org/10.1029/2000jd000119, 2002.
Trainer, M., Ridley, B. A., Buhr, M. P., Kok, G., Walega, J., Hübler, G., Parrish, D. D., and Fehsenfeld, F. C.: Regional ozone and urban plumes in the southeastern United States: Birmingham, A case study, J. Geophys. Res.-Atmos., 100, 18823–18834, https://doi.org/10.1029/95JD01641, 1995.
Trainer, M., Parrish, D. D., Goldan, P. D., Roberts, J., and Fehsenfeld, F. C.: Review of observation-based analysis of the regional factors influencing ozone concentrations, Atmos. Environ., 34, 2045–2061, https://doi.org/10.1016/S1352-2310(99)00459-8, 2000.
Wang, B., Liu, Y., Shao, M., Lu, S., Wang, M., Yuan, B., Gong, Z., He, L., Zeng, L., Hu, M., and Zhang, Y.: The contributions of biomass burning to primary and secondary organics: A case study in Pearl River Delta (PRD), China, Sci. Total Environ., 569–570, 548–556, https://doi.org/10.1016/j.scitotenv.2016.06.153, 2016.
Wang, L., Liu, D., Han, G., Wang, Y., Qing, T., and Jiang, L.: Study on the relationship between surface ozone concentrations and meteorological conditions in Nanjing, China, Acta Scientiae Circumstantiae, 38, 1285–1296, 2018 (in Chinese).
Wang, T., Xue, L., Brimblecombe, P., Lam, Y. F., Li, L., and Zhang, L.: Ozone pollution in China: A review of concentrations, meteorological influences, chemical precursors, and effects, Sci. Total Environ., 575, 1582–1596, https://doi.org/10.1016/j.scitotenv.2016.10.081, 2017.
Wang, X., Carmichael, G., Chen, D., Tang, Y., and Wang, T.: Impacts of different emission sources on air quality during March 2001 in the Pearl River Delta (PRD) region, Atmos. Environ., 39, 5227–5241, https://doi.org/10.1016/j.atmosenv.2005.04.035, 2005.
Wang, Y., Wang, H., Guo, H., Lyu, X., Cheng, H., Ling, Z., Louie, P. K. K., Simpson, I. J., Meinardi, S., and Blake, D. R.: Long-term O3–precursor relationships in Hong Kong: field observation and model simulation, Atmos. Chem. Phys., 17, 10919–10935, https://doi.org/10.5194/acp-17-10919-2017, 2017.
Wang, Y., Guo, H., Zou, S., Lyu, X., Ling, Z., Cheng, H., and Zeren, Y.: Surface O3 photochemistry over the South China Sea: Application of a near-explicit chemical mechanism box model, Environ. Pollut., 234, 155–166, https://doi.org/10.1016/j.envpol.2017.11.001, 2018.
Wiegand, A. N. and Bofinger, N. D.: Review of empirical methods for the calculation of the diurnal NO2 photolysis rate coefficient, Atmos. Environ., 34, 99–108, https://doi.org/10.1016/S1352-2310(99)00294-0, 2000.
Wei, X., Liu, Q., Lam, K. S., and Wang, T.: Impact of precursor levels and global warming on peak ozone concentration in the Pearl River Delta region of China, Adv. Atmos. Sci., 29, 635–645, https://doi.org/10.1007/s00376-011-1167-4, 2012.
Wu, C., Wang, C., Wang, S., Wang, W., Yuan, B., Qi, J., Wang, B., Wang, H., Wang, C., Song, W., Wang, X., Hu, W., Lou, S., Ye, C., Peng, Y., Wang, Z., Huangfu, Y., Xie, Y., Zhu, M., Zheng, J., Wang, X., Jiang, B., Zhang, Z., and Shao, M.: Measurement report: Important contributions of oxygenated compounds to emissions and chemistry of volatile organic compounds in urban air, Atmos. Chem. Phys., 20, 14769–14785, https://doi.org/10.5194/acp-20-14769-2020, 2020.
Xu, Z., Xue, L., Wang, T., Xia, T., Gao, Y., Louie, P. K. K., and Luk, C. W. Y.: Measurements of peroxyacetyl nitrate at a background site in the Pearl River Delta region: Production efficiency and regional transport, Aerosol Air Qual. Res., 15, 833–841, https://doi.org/10.4209/aaqr.2014.11.0275, 2015.
Xue, L., Wang, T., Wang, X., Blake, D. R., Gao, J., Nie, W., Gao, R., Gao, X., Xu, Z., Ding, A., Huang, Y., Lee, S., Chen, Y., Wang, S., Chai, F., Zhang, Q., and Wang, W.: On the use of an explicit chemical mechanism to dissect peroxy acetyl nitrate formation, Environ. Pollut., 195, 39–47, https://doi.org/10.1016/j.envpol.2014.08.005, 2014.
Yang, L., Luo, H., Yuan, Z., Zheng, J., Huang, Z., Li, C., Lin, X., Louie, P. K. K., Chen, D., and Bian, Y.: Quantitative impacts of meteorology and precursor emission changes on the long-term trend of ambient ozone over the Pearl River Delta, China, and implications for ozone control strategy, Atmos. Chem. Phys., 19, 12901–12916, https://doi.org/10.5194/acp-19-12901-2019, 2019.
Yang, Y., Shao, M., Keßel, S., Li, Y., Lu, K., Lu, S., Williams, J., Zhang, Y., Zeng, L., Nölscher, A. C., Wu, Y., Wang, X., and Zheng, J.: How the OH reactivity affects the ozone production efficiency: case studies in Beijing and Heshan, China, Atmos. Chem. Phys., 17, 7127–7142, https://doi.org/10.5194/acp-17-7127-2017, 2017.
Yao, B., Vollmer, M. K., Zhou, L. X., Henne, S., Reimann, S., Li, P. C., Wenger, A., and Hill, M.: In-situ measurements of atmospheric hydrofluorocarbons (HFCs) and perfluorocarbons (PFCs) at the Shangdianzi regional background station, China, Atmos. Chem. Phys., 12, 10181–10193, https://doi.org/10.5194/acp-12-10181-2012, 2012.
Yarwood, G., Rao, S., Yocke, M., and Whitten, G.: Updates to the Carbon Bond Chemical Mechanism: CB05, Technical Report, Final Report to US EPA, RT-0400675, https://camx-wp.azurewebsites.net/Files/CB05_Final_Report_120805.pdf (last access: 29 June 2022), 2005.
Yu, D., Tan, Z., Lu, K., Ma, X., Li, X., Chen, S., Zhu, B., Lin, L., Li, Y., Qiu, P., Yang, X., Liu, Y., Wang, H., He, L., Huang, X., and Zhang, Y.: An explicit study of local ozone budget and NOx-VOCs sensitivity in Shenzhen China, Atmos. Environ., 224, 117304, https://doi.org/10.1016/j.atmosenv.2020.117304, 2020.
Zaveri, R. A., Berkowitz, C. M., Kleinman, L. I., Springtson, S. R., Doskey, P. V., Lonneman, W. A., and Spicer, C. W.: Ozone production efficiency and NOx depletion in an urban plume: Interpretation of field observations and implications for evaluating O3-NOx-VOC sensitivity, J. Geophys. Res.-Atmos., 108, 4436, https://doi.org/10.1029/2002jd003144, 2003.
Zhang, Q., Yuan, B., Shao, M., Wang, X., Lu, S., Lu, K., Wang, M., Chen, L., Chang, C.-C., and Liu, S. C.: Variations of ground-level O3 and its precursors in Beijing in summertime between 2005 and 2011, Atmos. Chem. Phys., 14, 6089–6101, https://doi.org/10.5194/acp-14-6089-2014, 2014.
Zhang, Y. H., Su, H., Zhong, L. J., Cheng, Y. F., Zeng, L. M., Wang, X. S., Xiang, Y. R., Wang, J. L., Gao, D. F., Shao, M., Fan, S. J., and Liu, S. C.: Regional ozone pollution and observation-based approach for analyzing ozone–precursor relationship during the PRIDE-PRD2004 campaign, Atmos. Environ., 42, 6203–6218, https://doi.org/10.1016/j.atmosenv.2008.05.002, 2008.
Zhang, Y. N., Xiang, Y. R., Chan, L. Y., Chan, C. Y., Sang, X. F., Wang, R., and Fu, H. X.: Procuring the regional urbanization and industrialization effect on ozone pollution in Pearl River Delta of Guangdong, China, Atmos. Environ., 45, 4898–4906, https://doi.org/10.1016/j.atmosenv.2011.06.013, 2011.
Zhao, W., Tang, G., Yu, H., Yang, Y., Wang, Y., Wang, L., An, J., Gao, W., Hu, B., Cheng, M., An, X., Li, X., and Wang, Y.: Evolution of boundary layer ozone in Shijiazhuang, a suburban site on the North China Plain, J. Environ. Sci., 83, 152–160, https://doi.org/10.1016/j.jes.2019.02.016, 2019.