the Creative Commons Attribution 4.0 License.
the Creative Commons Attribution 4.0 License.
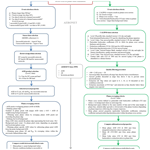
A novel method of identifying and analysing oil smoke plumes based on MODIS and CALIPSO satellite data
Alexandru Mereuţă
Andrei T. Radovici
Nikolaos Papagiannopoulos
Lucia T. Deaconu
Camelia S. Botezan
Horaţiu I. Ştefănie
Doina Nicolae
Alexandru Ozunu
Black carbon aerosols are the second largest contributor to global warming while also being linked to respiratory and cardiovascular disease. These particles are generally found in smoke plumes originating from biomass burning and fossil fuel combustion. They are also heavily concentrated in smoke plumes originating from oil fires, exhibiting the largest ratio of black carbon to organic carbon. In this study, we identified and analysed oil smoke plumes derived from 30 major industrial events within a 12-year timeframe. To our knowledge, this is the first study of its kind that utilized a synergetic approach based on satellite remote sensing techniques. Satellite data offer access to these events, which, as seen in this study, are mainly located in war-prone or hazardous areas. This study focuses on the use of MODIS (Moderate Resolution Imaging Spectroradiometer) and CALIPSO (Cloud-Aerosol Lidar and Infrared Pathfinder Satellite Observations) products regarding these types of aerosol while also highlighting their intrinsic limitations. By using data from both MODIS instruments on board Terra and Aqua satellites, we addressed the temporal evolution of the smoke plume while assessing lidar-specific properties and plume elevation using CALIPSO data. The analysis method in this study was developed to better differentiate between oil smoke aerosols and the local atmospheric scene. We present several aerosol properties in the form of plume-specific averaged values. We believe that MODIS values are a conservative estimation of plume aerosol optical depth (AOD) since MODIS algorithms rely on general aerosol models and various atmospheric conditions within the look-up tables, which do not reflect the highly absorbing nature of these smoke plumes. Based on this study we conclude that the MODIS land algorithms are not yet suited for retrieving aerosol properties for these types of smoke plumes due to the strong absorbing properties of these aerosols. CALIPSO retrievals rely heavily on the type of lidar solutions showing discrepancy between constrained and unconstrained retrievals. Smoke plumes identified within a larger aerosol layer were treated as unconstrained retrievals and resulted in conservative AOD estimates. Conversely, smoke plumes surrounded by clear air were identified as opaque aerosol layers and resulted in higher lidar ratios and AOD values. Measured lidar ratios and particulate depolarization ratios showed values similar to the upper ranges of biomass burning smoke. Results agree with studies that utilized ground-based retrievals, in particular for Ångström exponent (AE) and effective radius (Reff) values. MODIS and CALIPSO retrieval algorithms disagree on AOD ranges, for the most part, due to the extreme light-absorbing nature of these types of aerosols. We believe that these types of studies are a strong indicator for the need of improved aerosol models and retrieval algorithms.
- Article
(17536 KB) - Full-text XML
- BibTeX
- EndNote
Atmospheric aerosols are chemically complex mixtures of solid and liquid particles dynamically suspended in air. They originate from both natural and anthropogenic emissions. More common naturally occurring aerosols can be observed in the form of fog, dust, sea salt spray, biological exudates and grey smoke (biomass burning). Haze, smog and black smoke are typically a result of industrial and transportation activities (Stocker et al., 2014; Wei et al., 2020). Distinct species such as black carbon (BC), organic carbon (OC), sulfates, nitrates, trace elements, sea salt, mineral dust and biological matter suffer atmospheric alteration, resulting in different combinations of compounds. Defining aerosol types is a difficult task as they possess a large degree of variance in composition and concentration due to different atmospheric residence times, dry deposition and wet scavenging, emission rates and sources, transport trajectories, and seasonal variability (Dutkiewicz et al., 2009; Li et al., 2015; Samset et al., 2018).
Health effects associated with both short- and long-term exposure to aerosol have been widely documented in scientific literature (Brauer et al., 2015; Laumbach and Kipen, 2012; Zhang and Batterman, 2013; Pascal et al., 2013; Lee et al., 2014; Guarnieri and Balmes, 2014; Zhang et al., 2017). Aerosols have been linked to respiratory and cardiovascular diseases due to fine particulate matter (PM2.5;<2.5 µm in diameter) that can penetrate the lungs, resulting in increased rates of morbidity and mortality (Brunekreef and Holgate, 2002; Pope III et al., 2002; Lim et al., 2012; Beelen et al., 2014; Hoek et al., 2013).
Global circulation of aerosols is a known transport vector of minerals and nutrients to the biosphere (McTainsh and Strong, 2007; Maher et al., 2010; Lequy et al., 2012). Aerosols have a direct effect on radiation distribution by scattering, absorbing and emitting light through the atmosphere. In addition, they can affect the climate system through indirect effects acting as cloud condensation nuclei, impacting cloud lifetime and properties, atmospheric stability and precipitation factors (Popp et al., 2016; Samset et al., 2018; Stocker et al., 2014). They can disrupt circulation patterns, impact air temperatures and severe weather systems (Fan et al., 2016), reduce visibility (Deng et al., 2012; Wang et al., 2015), and lead to ozone depletion (Popp et al., 2016).
Because of their complex influence on the environment and climate system, assessing aerosol key parameters is essential for any atmospheric study. Aerosol optical depth (AOD), the extinction vertically integrated throughout the atmospheric column, is strongly correlated to PM concentrations. Together with other properties such as Ångström exponent (AE), single-scattering albedo (SSA), size distribution and vertical distribution, we can better describe their atmospheric impacts. Between ground stations and spaceborne observations, satellite remote sensing offers a more comprehensive global view of aerosols. Since the 1970s onwards, there has been a significant number of satellite sensors used successfully for retrieving AOD and other aerosol properties (Li et al., 2015, 2016; Dubovik et al., 2019; Schutgens et al., 2020; Wei et al., 2020; Sayer et al., 2020). When choosing between different aerosol products, one must take into account the wide variety of sensors and their characteristics such as spatial, temporal and spectral resolutions; single or multi-view retrieval methods; intensity or polarimetric design; and different retrieval algorithms (Wei et al., 2020; Fan and Qu, 2019; Sogacheva et al., 2020; Li et al., 2020). In addition to sensor characteristics, other factors such as cloud coverage, surface type, aerosol models and retrieval algorithms contribute to overall retrieval uncertainties (Wei et al., 2020; Li et al., 2015; Virtanen et al., 2018). Most sensors can retrieve a wide variety of aerosol properties; however they rely on inversion techniques and complex radiative transfer computations (Schutgens et al., 2020).
Smoke aerosols are primarily composed of two distinct carbonaceous species: BC, highly absorbent in all visible wavelengths, and OC, highly scattering of solar radiation (Ramanathan and Carmichael, 2008; Dutkiewicz et al., 2009). Recent studies suggest black carbon (BC) is the second largest contributor to global warming after CO2; however their impact on global radiative budget is still a subject of debate posing significant uncertainties (Bond et al., 2013; Andreae and Ramanathan, 2013; Stocker et al., 2014; Boucher et al., 2016). BC (or soot) is generated from incomplete combustion processes, with 59 % from biomass burning while the rest is attributed to fossil fuel combustion (Bond et al., 2013). Particle morphology of BC is significantly affected by multi-phase processes shortly after being emitted (Kokhanovsky, 2019; Noyes et al., 2020). Ageing/coating processes further change optical and microphysical properties of BC with direct impacts on their radiative effects (Riemer et al., 2010; He et al., 2015; Fierce et al., 2015; Peng et al., 2016). In practice, many remote sensing studies use rough estimations of particle shapes for fresh and aged BC, further inducing uncertainties (Kokhanovsky, 2019). Other considerations such as fuel type and emission sources need to be addressed as smoke plumes largely differ in composition and thus may exhibit different absorption and scattering efficiencies. This study will focus mainly on oil fire smoke plumes, which are far less debated in scientific studies as opposed to biomass burning smoke.
The most abundant source of atmospheric data on oil smoke plumes was gathered from the Kuwait oil fires in 1991. An estimated 700 oil wells were set on fire while smoke plumes engulfed large areas of the Gulf of Kuwait region (Cahalan, 1992). The amount of burned oil was estimated between 1.2 and 7.5 million barrels per day (Sadiq and McCain, 1993). Satellite images of visible smoke were first acquired on 9 February spanning until November when the last fires were extinguished (Draxler et al., 1994; Limaye et al., 1992). Several international research teams conducted extensive field campaigns, concentrating their efforts on atmospheric, environmental and health-related issues focusing on the potential impacts on global climate (Sadiq and McCain, 1993; Husain, 1995; World Meteorological Organization, 1993). Studies on health effects related to the Kuwait oil fires suggest that smoke exposure led to acute respiratory illnesses with some suspecting long-term effects (Etzel and Ashley, 1994; Brain et al., 1998; Smith, 2002; Lange et al., 2002; Kelsall, 2004; Heller, 2011; Barth et al., 2016). Valuable atmospheric data were also collected from smaller events such as oil depot fires, most notably the Buncefield incident on 11 December 2005. A number of explosions led to a large fire engulfing 20 storage tanks until 15 December. The fire burned 58 000 t of fuel while injecting a large smoke plume above the boundary layer at 3000 m (Vautard et al., 2007; Health and Safety Executive and Buncefield Major Incident Investigation Board (Great Britain), 2008). An initial report on air quality concluded that the smoke plume remained aloft over cold and stable atmospheric layers, thus reducing the potential impacts at ground level (Targa et al., 2006). Health studies related to the event concluded no long-term impacts on people exposed to the smoke; however acute respiratory symptoms were reported (Hoek et al., 2007; Morgan et al., 2008).
Oil fire and biomass burning (BB) smoke plumes significantly differ in ratios. Studies show values ranging from 3 to 20 for BB (Andreae and Merlet, 2001; Ichoku et al., 2012; Konovalov et al., 2018; Andreae, 2019; Akagi et al., 2011), largely dependent on fuel types. This ratio also relates to higher single-scattering albedo (SSA) values of 0.7–0.96 for light grey visible smoke plumes (Radke et al., 1991; Dubovik et al., 1998; Eck et al., 1998; Leahy et al., 2007; Pokhrel et al., 2016). However ratios are much lower for oil fires: 0.83–1.05 (Laursen et al., 1992; Daum et al., 1993; Ferek et al., 1992), also depending on fuel types, and SSA values are lower, 0.3–0.65 (Johnson et al., 1991; Hobbs and Radke, 1992; Mather et al., 2007; Mikhailov et al., 2006; Gullett et al., 2017), for dark black visible smoke plumes. Studies also report large BC content in smoke plumes with PM (particulate matter), ranging from 46 %–50 % for Kuwait pool fires (Hobbs and Radke, 1992; Laursen et al., 1992; Stevens et al., 1993) to 50 %–75 % for the Buncefield plume (Mather et al., 2007; Targa et al., 2006) and 75 %–82 % for plumes generated by burning oil on the ocean (Gullett et al., 2016, 2017; Ross et al., 1996). These findings suggest that oil smoke plumes heavily absorb light in visible wavelengths and thus require adequate adjustments to any models used for retrieving optical and microphysical properties via remote sensing techniques.
1.1 Objectives
One objective of this study is to highlight the importance of satellite remote sensing techniques in identifying these types of events. As opposed to ground-based data, satellite data offer access to remote areas all over the globe, which would otherwise be very difficult to achieve. As seen in Table 1, oil installations may be situated in desert areas, at sea or in secluded locations far away from air quality monitoring stations or AERONET (AErosol RObotic NETwork) sites (Holben et al., 1998). In addition to this advantage, a synergistic approach using different types of satellite instruments can offer three-dimensional space coverage. While in situ ground stations and modelling tools are viable options for smoke plume research, these methods have limitations in areas prone to armed conflicts or posing high health risks. Out of the aforementioned events, only event 10 was analysed by different techniques, as seen in local AERONET data (Sect. 3.4). It goes without saying that retrieving optical and microphysical properties of petrochemical burnings may be challenging in most cases even with this approach. This study will focus on the use of MODIS and CALIPSO aerosol products regarding these types of aerosol while also highlighting their limitations. By using data from both MODIS instruments on board Terra and Aqua satellites, we addressed the temporal evolution of the smoke plume while assessing lidar-specific properties and plume elevation using CALIPSO data. The low number of studies on petrochemical smoke plumes, especially in the last decade, further encourages us to address these issues. While biomass burning and industrial haze are abundantly discussed in scientific literature, the same cannot be said for petrochemical smoke plumes resulting from major technological accidents. To our knowledge, we have not identified any similar studies focused specifically on retrieving aerosol properties from major petrochemical accidents by using synergistic satellite techniques.
1.2 Event synopsis
This section summarizes a collection of events ranging from 2008 to 2019 that were successfully identified by satellite remote sensing techniques. Table 1 also provides the coordinates and the number of MODIS observation for each of the events covered in this study.
Events similar in nature to the Kuwait oil fires took place in northern Iraq as oil fields at Qayyarah and Najma were intentionally set ablaze by Islamic State of Iraq and Syria (ISIS) militants in an attempt to deter coalition air strikes. The first fires were detected east of Baiji in early January 2016. Other oil wells burned intermittently from May to June close to Mosul and Kirkuk. The bulk of smoke plumes were observed largely between June and November; however smoke plumes were continuously detected from the Qayyarah oil fields for a total of 225 d ranging from 13 June 2016 to 27 March 2017. As a result of these events, an estimated 1.33 million barrels of oil were burned (Bulmer, 2018; Tichý and Eichler, 2018). Residents south of the Qayyarah oil fields were exposed for 103 d to smoke plumes. Short-term health effects were reported, especially for patients with pre-existing respiratory conditions (Bulmer, 2018).
The Gulf of Sidra has seen extensive episodes of smoke plumes as oil terminals at As Sidr and Ra's Lanuf, Libya, have been repeatedly set on fire over the course of a decade. These events have been captured by MODIS sensors through the last decade all the way since 2008. All events were characterized by dark plumes, suggesting high contents of BC. On 19 August 2008 a tank fire erupted in Fiba tank farm at Ra's Lanuf after workers failed a maintenance operation (Piafom, 2018; The Telegraph, 2011). The fire lasted 9 d, during which smoke plumes could be detected from 19 to 22 August. In March 2011 the terminal was struck by air artillery in the battle of Ra's Lanuf as the country was engaged in civil war (BBC, 2011; The Christian Science Monitor, 2011; The Guardian, 2011). Smoke plumes were visible on 12 and 14 March. In December 2014 the tank farm at As Sidr oil terminal was struck by rockets as rebels fought to seize control of the city port. Seven storage tanks where engulfed in flames, burning 1.8 million barrels of crude oil (Reuters, 2014; BBC, 2014; Al Jazeera, 2014). Smoke plumes covered large areas of the Gulf of Sidra between 26 and 30 December and could be seen as far east as Timimi and Crete. In January 2016 both tank farms at As Sidr and Ra's Lanuf were struck by Islamic State militants. On 5 January As Sidr suffered five tank fires, while two tanks were hit at Ra's Lanuf, amounting to 850 000 barrels of oil. A week later IS militants struck oil infrastructure connecting Ra's Lanuf terminal to other installations in the area (Tichý and Eichler, 2018; Tichý, 2019). A second attack at Ra's Lanuf tank farm was conducted on 21 January (Business Insider, 2016). Throughout the month, extremely dense smoke plumes could be seen in the region from 5 January all the way to 23 January. The most recent incident at Ra's Lanuf took place in June 2018 when rival armed groups clashed. The fire which started on 14 June was contained at two storage tanks before it was extinguished several days later (Reuters, 2018). Visible smoke plumes were detected on 17 and 18 June (Bellingcat, 2018).
2.1 MODIS aerosol data
The MODerate resolution Imaging Spectroradiometer (MODIS) is a passive remote sensing instrument on board NASA's Earth Observing Satellites (EOS). The instrument has been collecting climate-related data, including aerosol products, since 2000 from Terra and 2002 from Aqua satellite platforms. To achieve a vast catalogue of products, MODIS uses its wide spectral range, 36 channels between 0.41 and 14.5 µm, fine spatial resolution (250 to 1000 m) broad swath width (2330 km), and daily temporal resolution.
Herein we will summarize the aerosol retrieval algorithms Dark Target (DT) over land and ocean (Kaufman et al., 1997; Tanré et al., 1997; Levy et al., 2007a, b, c, 2013; Remer et al., 2005, 2008, 2013) and Deep Blue (DB) over land (Hsu et al., 2004, 2006, 2013), with some emphasis on the atmospheric parameters used in the construction of look-up tables (LUTs) and aerosol model selection as these properties/assumptions are crucial for proper AOD retrieval in oil smoke events.
The DT land algorithm is used over dark vegetated surfaces with low surface reflectance. DT makes use of the “VIS to 2.1” relationship to distinguish surface contributions to the top-of-atmosphere (TOA) reflectance, as aerosols have a low absorbing and scattering effect in the shortwave infrared (2.12 µm) band compared to the visible blue (0.47 µm) and red (0.66 µm) bands. After applying several pixel screening and selection techniques, the algorithm chooses specific aerosol models and types based on seasonal and geographical characteristics. From here it determines aerosol-related atmospheric parameters as a function of TOA, surface and gas contributions to the apparent reflectance. To achieve AOD inversion, these parameters are matched to values within predetermined LUTs as an attempt to describe the most likely aerosol conditions. The land algorithm uses five aerosol types composed of two or more models (fine or coarse), each with its specific aerosol optical properties. Primary products retrieved are AOD at 0.55 µm, fine model aerosol type (FMF) and spectral fitting error (ε).
The DT ocean algorithm works in much the same way as the land algorithm, although it requires masking sediments and filtering out strong glint areas. It uses the spectral dependencies of six bands, 0.55, 0.65, 0.87, 1.24, 1.63 and 2.12 µm, for retrieving surface reflectance while it finds the exact match between the observed and the predetermined LUT reflectance values for the 0.87 µm band. It then attempts the best fit for the remaining bands. The 0.87 µm band is a good indicator of aerosol loading as it is less impacted by water radiance. Preliminary data such as AOD 0.55 µm, reflectance weighting parameter (η) at 0.55 µm and aerosol effective radius (Reff) are determined before the LUT inversion. DT ocean retrieves the same products as DT land; however it assumes aerosol properties based on a combination of two models, one fine model (four possible modes) and one coarse model (five possible modes). These models are combined by the weighting factor (η) such that the solution yields the lowest fitting error.
Aerosol properties within the LUT such as size distribution parameters, refractive indexes and SSA are crucial for proper aerosol typing and subsequent AOD retrieval. LUT information and model selection are critical for deriving other optical properties, such as Ångström exponent (AE), which can also be used to describe size distribution.
The DB algorithm was developed to retrieve AOD over arid, semi-arid and urban areas where surface reflectance values are higher than those over Dark Target regions. The principle behind the algorithm suggests that surface reflectance in these areas shows higher values in red and near-infrared bands and lower values in the blue band. The algorithm uses reflectance values from nine bands through each step of the retrieval. After screening and pixel selection, surface reflectance values are determined based on three bands (0.412, 0.490 and 0.67 µm) using either a database or a dynamic approach. The approach is selected based on the normalized difference vegetation index (NDVI) and may be a function of region, season, scattering angle and land type. DB matches observed to LUT radiance values using a maximum likelihood method to determine the mixing ratio for a dust and a smoke model. The method retrieves two types of aerosol products: AOD and SSA from the dust model and AOD and AE from the smoke model.
2.2 CALIPSO aerosol data
The Cloud Aerosol Lidar with Orthogonal Polarization (CALIOP) on board the CALIPSO satellite has been observing vertically distributed aerosol and cloud properties since 2006. CALIOP is an elastic backscatter lidar operating at two wavelengths (532 and 1064 nm) equipped with a polarization channel at 532 nm (Hunt et al., 2009; Winker et al., 2009). Calibration is achieved through a molecular normalization technique for night-time measurements at 532 nm, which is subsequently the basis for daytime calibrations at both channels (Powell et al., 2009; Kar et al., 2018). The latest CALIOP Version 4 data are significantly improved thanks to the refined calibration algorithms (Getzewich et al., 2018; Kar et al., 2018; Vaughan et al., 2019).
CALIOP data require several processing sequences, handled by different algorithms, to achieve the desired aerosol and cloud properties (Winker et al., 2009). The first algorithm (selective iterative boundary locator – SIBYL) starts by analysing calibrated level 1 data averaged horizontally (at resolutions from 0.33 to 80 km) through the use of an adaptive threshold scheme establishing layer boundary limits (Vaughan et al., 2009). The next steps require the use of scene classification algorithms (SCAs). Firstly, the cloud-aerosol discrimination (CAD) algorithm uses multidimensional probability density functions (PDFs) to distinguish clouds from aerosol layers (Liu et al., 2005, 2009). The primary inputs from the CAD algorithm are later used for subtyping aerosol species throughout the troposphere and stratosphere (Omar et al., 2009; Kim et al., 2018). Finally, SCA uses the attenuated backscatter and volume depolarization ratios (both layer-integrated) to distinguish between water and ice clouds (Hu et al., 2009; Avery et al., 2020). To extract aerosol properties (particulate backscatter and extinction coefficients, optical depth), the classified layer data are fed through several hybrid extinction retrieval algorithms (HERAs) (Young and Vaughan, 2009; Young et al., 2013, 2018).
Lidar ratios are essential for calculating extinction coefficients, and throughout these sequences of algorithms lidar ratios are selected in one of two ways. For unconstrained retrievals, lidar ratios are selected based on the aerosol subtype classification, which is a function of surface type, location, particulate depolarization ratio and integrated attenuated backscatter (Omar et al., 2009; Kim et al., 2018). For each aerosol subtype, a lidar ratio is assigned based on AERONET data, direct measurements and theoretical scattering calculations (Omar et al., 2009; Tackett et al., 2018). The second approach, known as constrained retrievals, is based on measured layer two-way transmittance (Young and Vaughan, 2009; Young et al., 2018). Selection between these two approaches is done based on scene complexity and feature classification (Young and Vaughan, 2009; Young et al., 2018). In most cases aerosol lidar ratios are determined using unconstrained retrievals (e.g. layers in contact with the surface); however constrained solutions are possible in certain situations (Young and Vaughan, 2009; Young et al., 2018).
2.3 Synergic approach
Figure 1 summarizes the steps of the analysis in detail. Events reported in scientific literature as well as events that drew significant media attention within a period of 12 years (2008–2019) were selected, a period for which both MODIS and CALIPSO were operational. MODIS (aboard Aqua and Terra satellites) RGB composite images are used to visually identify the plume. Plumes larger than 500 km2 were only studied to ensure sufficient pixel count. Subsequently, cloudy scenes, with over 50 % cloud coverage, were discarded. Next, aerosol retrievals were grouped into successful and unsuccessful based on the AOD values. Successful retrieval is considered when the AOD values of the pixels that are flagged as smoke yield some degree of variation (for at least 50 % of pixels, the AOD differences should vary at least by 0.01), whereas unsuccessful retrieval is considered when AOD values are either below 0.1 or constant throughout the plume (over 90 % of plume pixels with a fixed AOD value of 0.09 as seen in Fig. 3). We used successful and unsuccessful retrievals to highlight the capabilities and limitation of MODIS. The MODIS 6.1 collection was used in this study (MODIS Atmosphere Science Team, 2017a, b), and the algorithm for the AOD was selected based on surface type (DT over ocean and land) and locations (DB over desert and arid areas) for both successful and unsuccessful retrievals. We took advantage of the higher-resolution 3×3 km2 level 2 AOD products for statistical relevance in successful retrievals over ocean. For unsuccessful retrievals we used the 10×10 km2 level 2 AOD products (DB over desert and arid areas) and the 3×3 km2 level 2 AOD products over land.
Aerosol properties were only analysed for successful retrievals. The following aerosol properties were used in our analysis: AOD at 0.55 µm, AE and Reff. For successful retrievals, we developed an averaging technique to remove background aerosol from the identified smoke plume. Since both RGB and AOD images show a clear transition from background aerosol to oil smoke areas, as seen in Fig. 2a and b, we identify the plume edge based on the AOD pixel gradient. Conversely, the plume edge pixels have AOD values different from the neighbouring background pixels by a value of at least 0.03, a value that has been decided with a simultaneous inspection of RGB and AOD maps. The averaged AOD within the plume edge is called “total plume AOD” and comprises oil smoke and background aerosols. The “plume-specific AOD” is a result of subtracting the local background AOD from the total plume AOD. The local background AOD is defined as the average AOD from a smoke-free area. This area needs to contain between 3 and 10 times the pixels of the smoke plume. This decision stems from the local geography and meteorological conditions (see Fig. 7a, event 13, on 29 December 2014, for high pixel count and Fig. 7a, event 16, on 21 October 2016, for low pixel count). In Sect. 3.2, detailed discussions of successful MODIS retrievals are presented.
CALIPSO is used complementarily as it provides important insight into the plume monitoring, being an active sensor. Moreover, CALIPSO flies as part of the A-Train constellation and follows MODIS Aqua observations by 2 min; thus similar atmospheric volume is sampled. The particulate backscatter coefficient (532 nm) is used to define the extent of the plume cross section. Smoke plumes have higher backscatter values than the background aerosol and are easily identifiable in the backscatter profiles. The minimum plume horizontal extent is set to 5 km as this is the standard level 2 data output (Winker, 2018). For daytime, MODIS Aqua RGB images prior to the CALIPSO overpass are used for visual confirmation. Conversely, for night-time, one MODIS image before and one after the CALIPSO overpass are used to assess the plume spatial continuity.
To retrieve detailed information on the aerosol optical properties, we use CALIPSO Level 2 data – 5 km Aerosol Profile (532 and 1064 nm), standard version 4.20 (Winker, 2018). The methodology to quality assure the CALIPSO profiles is mostly similar to the rubric used by Tackett et al. (2018). For cloud-free scenes, only aerosol profiles with a cloud-aerosol-discrimination (CAD) score of −100< CAD score <−20 are selected. Furthermore, aerosol profiles directly below any type of clouds are discarded as these may be affected. Smoke plumes above 4 km (mean surface level) in contact with ice clouds were discarded to prevent misclassifications as cirrus fringes. For the extinction coefficient filtering procedure, QC flag values not equal to 0, 1, 16 or 18 are discarded as low-confidence retrievals. Extinction coefficients where the uncertainty is equal to 99.9 km−1 are rejected as well as the values in bins directly below this range.
In this analysis, the particle backscatter coefficient is used to identify the geometrical properties of the smoke plume. The plume is defined as the area where the values are at least 2 times higher than the background, which is considered an area of identical thickness located either above or below the plume. The plume AOD (532 and 1064 nm) is calculated by integrating the particle extinction coefficient in the plume region, and the plume mean AOD is the average of the individual (i.e. 5 km) plume AODs that comprise the plume. Additionally, the plume extinction-to-backscatter ratio (i.e. lidar ratio), Ångström (532 1064 nm) exponent and particle depolarization ratio are assessed to investigate the type-dependent characteristics of the plume and whether oil smoke presents distinctive intensive properties.
AERONET observations, when available, are also investigated and compared with the satellite measurements. Lastly, in case of events that have already been investigated by means of ground-based or airborne observations, we compared the published results with our methodology, reflecting the impacts oil smoke plumes have on current satellite retrieval capabilities.
3.1 Case study illustration
Based on the information given in Table 1, we filtered a total of 375 d in which oil smoke plumes were observed by the MODIS sensors. After applying the selection criteria for the MODIS sensor, we obtained a total of 10 d with successful retrievals. The majority of oil plumes resulted in unsuccessful retrievals, 70.7 % while 26.7 % of plumes were screened out due to high percentage of cloud coverage. When applying the selection criteria for CALIPSO, we obtained six plume sections suitable for analysis. Table 2 shows the dates for both MODIS and CALIPSO retrievals suitable for analysis.
We selected a successful retrieval to better describe the method used for our analysis. Figure 2 shows event 14, the case at Ra's Lanuf and As Sidr tank farms which caught fire on 5 January 2016 and burned throughout 6 and 7 January. The retrieval in the images was taken on 6 January by MODIS Aqua at 12:05 UTC. Figure 2a represents a true-colour composite image showing the smoke plumes emerging from both sites and travelling ENE over the Gulf of Sidra. Judging by this image alone, we can only distinguish parts of the smoke plume which appear to be less dispersed and thus present a smaller mixing ratio with the local background aerosols. In this study, we focused our attention on the plume areas where heavy concentrations of aerosol are present while discarding retrievals done at the edges of the plume where background aerosol may have a large influence on the retrieved values. Thus Fig. 2b was constructed based on the AOD (0.55 µm) retrieval and a plume edge selection technique. To determine the plume edge, we constructed isolines of AOD values from the retrieval pixels. The 3 km × 3 km product is better suited for determining the AOD gradients and thus was selected over the standard 10 km × 10 km product. Figure 2b shows higher values of AOD in the selected plume area as opposed to the local background levels. At the time of the retrieval, we can observe two distinct plumes of smoke, a thin plume originating from the As Sidr and the main plume (within the black contour, Fig. 2b) originating from Ra's Lanuf. Since the As Sidr plume did not meet the selection criteria, the analysis is made for the main Ra's Lanuf plume. To further discriminate between plume and background AOD values, we averaged all non-plume pixel values, over water, within the Gulf of Sidra region without considering the pixels of the plume. Then, the averaged AOD values were subtracted from each pixel of the plume AOD values to determine the overall plume contribution. Consequently, Fig. 2c illustrates the plume-specific AOD gradient. Figure 2d and e show the AE (0.55 0.86 µm) and the Reff (µm), which were selected for aerosol typing. For AE and Reff we used the same edge selection technique as described above without the background subtraction. The AE is shown to have very low values, indicating a dominant coarse mode which is further evidenced by the large Reff chosen from the LUT. It is also evident from both figures that both plumes extend further from the edge selection. In this case the mean plume-specific AOD was 0.13, while the background values averaged 0.08. Figure 2c shows AOD values as high as 0.24 over the average AOD background level for the plume originating at Ra's Lanuf. The AOD gradient, in Fig. 2c, shows the largest values at the centre of the plume where aerosol mixing is expected to be lower. Mean plume AE was −0.18 as opposed to the background value of 0.45, and plume Reff showed 1.45 µm versus 0.51 µm for background values.
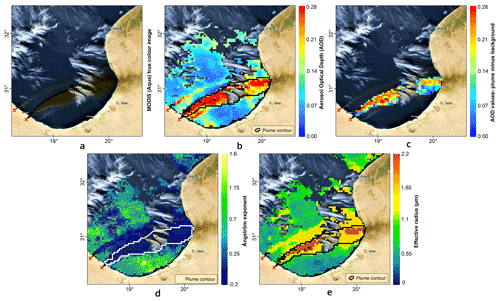
Figure 2Visual representation of the analysis method for MODIS data: (a) plume captured in true colour; (b) AOD retrieval over the plume area and background (Gulf of Sidra); (c) AOD retrieval as a result of plume minus background values; (d) Ångström exponent for plume and background area; (e) effective radius for plume and background area. The red coloured “x” indicates the event origin (satellite imagery from the NASA Worldview application, https://worldview.earthdata.nasa.gov, last access: 5 January 2022).
Figure 3 shows an example of an unsuccessful retrieval of the land algorithm for the event 13 plume on 30 December 2014. We can distinguish the plume from the RGB image over the Gulf of Sidra while also observing AOD values over land where the smoke plume drifted ENE towards the island of Crete. However, there seems to be no distinguishable AOD gradient, over land, in the plume section. A further inspection suggested that all pixels showed values of 0.095, which suggests that the lower radiance values did not match well with pre-existing LUT values. Consequently, the region is classified as “clean atmosphere”, and thus, a unique AOD value is assigned to all the pixels. Conversely, the ocean algorithm retrieved AOD that varied between 0.1 and 0.37. Since these heavy smoke plumes are the result of extreme scenarios, they are rarely observed and may not end up being a subject of research. Thus, we believe there are no cases within the LUT values describing extremely low atmospheric transmission and radiance values, highly absorbent aerosol, low SSA and low reflectance values over a large spectral range including MODIS bands 1 through 7.
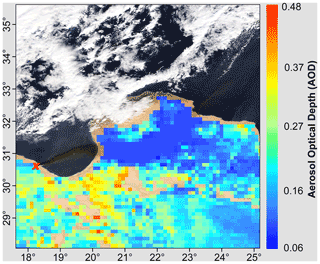
Figure 3Retrieval of plume (unsuccessful) and background AOD values: event 13, 30 December 2014. The red coloured “x” indicates the event origin (satellite imagery from the NASA Worldview application, https://worldview.earthdata.nasa.gov, last access: 5 January 2022).
Event 14 at Ra's Lanuf and As Sidr, 6 January 2016, was also captured by CALIPSO lidar measurements as CALIPSO overpass matched a cross section of the plume area. Figure 4a shows this overlap in near-real time as CALIPSO succeeds Aqua within a 2 min time frame. Within the 15 km plume cross section, we selected a particulate backscatter coefficient profile for reference, Fig. 4b, and based on this parameter we determined plume elevation and thickness. The average plume thickness was approximately 920 m. The layer base was situated between 2600 and 3100 m above the Gulf of Sidra while the top was measured between 3300 and 4200 m. The entire plume cross section is presented in Fig. 5a. We observe the main plume from Ra's Lanuf elevated between 2600 and 4200 m. Figure 5a also shows the secondary plume from As Sidr, 0.2∘ north of the main plume situated around 2000 m. Based on CALIPSO measurements of the main plume, average particulate backscatter (532 nm) values measured 0.015 km−1 sr−1 while values at 1064 nm measured 0.017 km−1 sr−1. Average extinction coefficient values at 532 nm were measured at 1.65 km−1 while the 1064 nm channel yielded a value of 1.55 km−1.
This event is an example of an opaque aerosol layer, where the lidar did not penetrate the plume up to the sea surface over the Gulf of Sidra. This event recorded a lidar ratio of 109 ± 47 sr at 532 nm and 86 ± sr at 1064 nm. These values are larger than the CALIPSO V4 aerosol subtype values for elevated smoke 70 ± 16 (532 nm) and 30 ± 18 (1064 nm) and polluted continental/smoke 70 ± 25 (532 nm) and 30 ± 18 (1064 nm) (Kim et al., 2018). The initial lidar ratios were reduced by 5 % based on the scheme described by Young et al. (2018) for opaque aerosol layers. These events are described as occurring infrequently (1 % of all unique aerosol layers, detected in 2012; Young et al., 2018) and may be subjected to further uncertainties. The initial value of the lidar ratio (SP) is described by Young et al. (2018) in Eq. (1). This assumes a zero value of the two-way transmittance () and a multiple scattering factor value of 1 (η=1). Young et al. (2018) also suggest that η=1 assumption may not be valid for opaque aerosol layers and may introduce bias errors. These errors can be propagated through the extinction and AOD retrievals and result in more conservative estimates. The particulate depolarization ratio for the Ra's Lanuf plume was 0.11 ± 0.43, which corresponds to moderately depolarizing smoke (Kim et al., 2018). Figure 5c shows the CALIPSO feature classification while Fig. 5b shows the aerosol typing results.
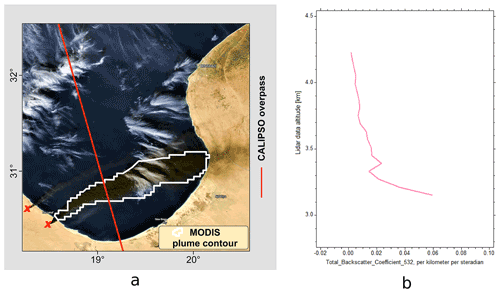
Figure 4(a) CALIPSO overpass and MODIS plume contour 7. (b) Particulate backscatter coefficient profile CALIPSO level 2 (532 nm) (satellite imagery from the NASA Worldview application, https://worldview.earthdata.nasa.gov, last access: 5 January 2022).
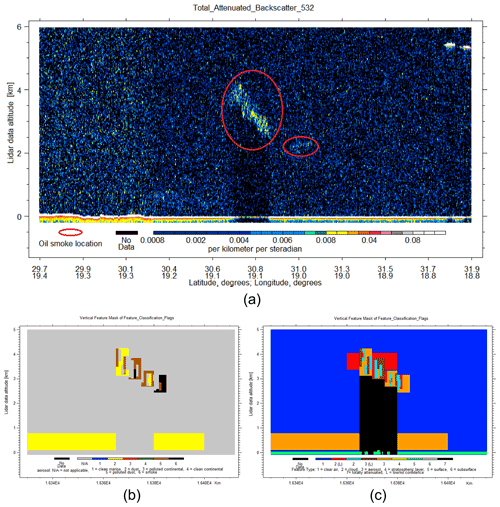
Figure 5(a) Image of event 14 plume based on CALIPSO total attenuated backscatter (532 nm) vs. lidar data altitude data. (b) Aerosol feature classification. (c) Cloud feature classification.
Judging from these images and from the average CAD score of −48, the smoke plume represented a mixed feature of cloud and aerosols. This is to be expected as water vapour and particulate matter are primary components in emissions of petrochemical burnings (Johnson et al., 1991; Ferek et al., 1992; Daum et al., 1993). Cloud formations on top of oil smoke, plumes, such as pyrocumulonimbus, have been observed in other instances (Johnson et al., 1991), as seen in Fig. 6, a phenomenon which hinders AOD retrievals in both passive and active sensors.
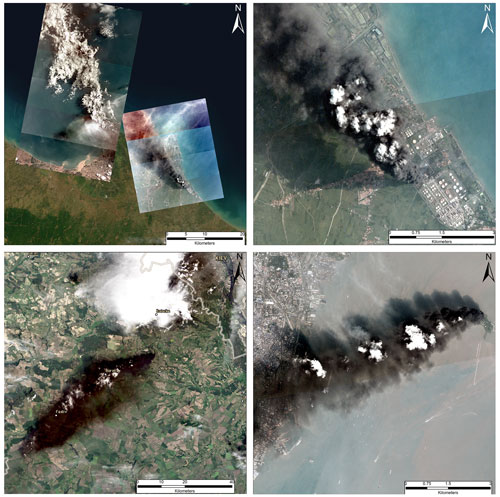
Figure 6Cloud formation on top of oil smoke plumes. Upper images depicting the fire at Balongan, Indonesia, 29 March 2021; lower left image depicting the fire at Vasylkiv, Ukraine, on 9 June, 2015; lower right image depicting the fire at Butcher Island, India, on 7 October 2017 (satellite imagery from OpenStreetMap©OpenStreetMap contributors, 2021. Distributed under the Open Data Commons Open Database License (ODbL) v1.0 and Planet Team, https://www.planet.com/, last access: 5 January 2022).
The current version of the vertical feature mask gives a mixed result for aerosol typing comprised of dust, polluted dust and smoke aerosols for this oil smoke plume. The average values for plume AOD ranged between 1.52 ± 0.8 (532 nm) and 1.43 ± 0.47 (1064 nm). We also computed the plume AE (532 1064), which resulted in 0.09, indicating the presence of coarse particles.
3.2 Successful MODIS retrievals
The results of the successful MODIS retrievals are presented in Tables 3 and 4 in the form of mean and standard deviation values. The MODIS Aqua retrieval presented in Sect. 3.1 was found to be in good agreement with the Terra retrieval. Event 14 showed a larger difference in plume-specific AOD values between Terra and Aqua retrievals. However this was to be expected since the fire spread to several oil tanks between the two retrievals. Based on these results, we identified no large discrepancies between the two sensors. For plume and plume-specific AOD, the majority of values fall within the expected uncertainty interval of the retrieval algorithm, ± (0.05 + 0.20 × AOD) when comparing between the two sensors (Gupta et al., 2018). Small changes in AOD values can also be attributed to plume dispersion. For the majority of cases the plume-specific AOD is the main contributor to the total AOD in the atmospheric column; however all AOD values are generally low. In cases where background AOD is already low, a thin layer of black smoke can reduce atmospheric transmission and radiance values. This effect would result in lower TOA reflectance and plume-specific AOD values.
Table 3Mean and standard deviation values of aerosol properties (AOD, AE, Reff) based on MODIS Terra successful retrievals.
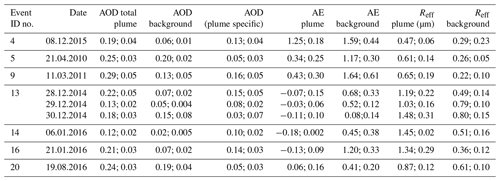
Table 4Mean and standard deviation values of aerosol properties (AOD, AE, Reff) based on MODIS Aqua successful retrievals.
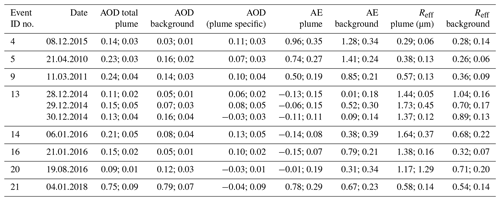
Except for event 4, all the plumes exhibit AE values lower than 1. The larger AE values from event 4 may be attributed to the different fuel type since the event surrounding SOCAR's platform no. 10 also involved four gas wells (Business-humanrights, 2015). While AE plume values are generally low, these extremely low values may not be primarily a direct result of particle size distribution. MODIS uses spectral reflectance relations to determine AOD and subsequently AE levels. While other types of aerosols have a varying spectral reflectance signature, heavy concentrated black carbon exhibits a flat and linear signature that results in low spectral reflectance values (Johnson et al., 1991; King, 1992; Pilewskie and Valero, 1992; Soulen et al., 2000). To further distinguish between these events and the atmospheric background, we selected the effective radius based on MODIS LUT. For the ocean algorithm, Reff values range from 0.10 to 2.50 µm, with lower values being a good indicator of fine dominant aerosols, whereas higher values indicate coarse dominant aerosol type. The results show plume values up to 3 times higher than background values. Both AE and Reff values show the presence of large particles in the plume areas.
Following event 14 in Fig. 2, Fig. 7 shows a visual representation of successful MODIS retrievals from events 13 and 16. We choose to describe in detail the events from Libya as they are also analysed based on CALIPSO retrievals. Moreover, the plumes resulting from these events share the same locations (As Sidr and Ra's Lanuf). Figure 7a shows plume specific AOD values ranging from 0 to 0.28. Plumes from As Sidr, event 13, are visible in the first three rows of Fig. 7. This event was captured over multiple days while the fire engulfed several oil tanks and subsequently injected higher amounts of aerosols in the region. Depending on the local background levels, average plume-specific AOD ranged from −0.03 to 0.15. Negative values can be explained by the presence of dust and marine aerosols in the atmospheric background. This is especially evident for event 13 on 30 December 2014 when high background levels were registered in the Gulf of Sidra while lower levels were seen off the shores of At Tamimi, 600 km NE of As Sidr. The fourth row in Fig. 7 shows the plume from event 16, marking the second attack on the Ra's Lanuf tank farm in 2016. The plume section over the Gulf of Sidra recorded AOD values twice as high as the background level; however the net contribution amounted, on average, to a value of 0.10. The AE values below 0 seen in Fig. 7b suggest a coarse dominant scene. Figure 7b also shows low AE values identified further from the plume's edge, showing the spatial extent of these types of aerosols. The Gulf of Sidra is situated in one of the main pathways of long-range-transported dust (Kallos et al., 2007), thus affecting AE local background values, as seen in Tables 3 and 4. In Fig. 7c we identify high Reff values consistently over 1 µm while, in some cases, values close to the fire and within the centre of the plume area reached the maximum 2.50 µm. These large values are consistent with the observed AE trend observed, indicating larger particles and coarse-mode-dominant aerosol type. Background values for these events fluctuated between 0.32 and 1.04 µm due to regional dust-like aerosols.
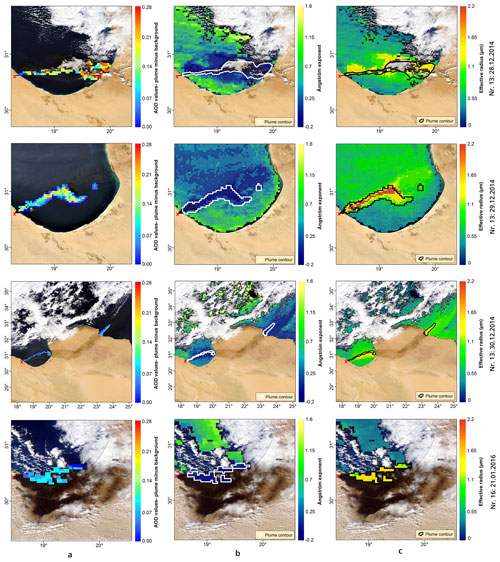
Figure 7(a) Successful retrievals of aerosol properties for events 13 and 16. Plume-specific AOD: (b) AE values for plume and the local background; (c) Reff values for plume and the local background. The red coloured “x” indicates the event origin (satellite imagery from the NASA Worldview application, https://worldview.earthdata.nasa.gov, last access: 5 January 2022).
3.3 CALIPSO retrievals
Within the 12-year period we identified three events in the Gulf of Sidra, events 11, 13 and 14. Apart from event 14, previously described in Sect. 3.1, all the remaining CALIPSO retrievals were unconstrained retrievals. Event 13, at As Sidra on 29 December 2014, was detected by a CALIPSO night-time overpass which fell approximately 12 h between the successful MODIS retrievals of 28 and 29 December 2014. The plume at As Sidr can be observed in Fig. 8a. Off the coast of As Sidr a distinct feature above the sea surface reaching 650 m in altitude is observed. Figure 8b shows a particulate backscatter profile where we can distinguish a plume thickness of approximately 240 m. This event was smaller in magnitude with respect to event 14 where multiple storage tank fires contributed to the same plume mass. In this case, CALIPSO overflew much closer to the tank farm, also resulting in a narrower plume cross section. The SIBYL algorithm level 2 products were averaged over a larger 20 km area, as opposed to the 5 km averaging resolution; thus plume values are harder to distinguish from background aerosol levels. Backscatter and extinction values can be seen in Table 5. The plume cross section measured approximately 3 km, as seen in Fig. 8c and d. Consequently, CALIPSO identified dusty marine aerosols within the Gulf of Sidra region as evident from the plume lidar ratio of 37 ± 15 sr (both 532 and 1064 nm) within the atmospheric layer (Kim et al., 2018). The plume particulate depolarization ratio value, 0.12 ± 0.14, was similar to the values detected from event 14; however the plume and background values were indistinguishable, in part due to the larger averaging scheme. The local scene over the Gulf of Sidra region was fairly clean judging by the low AOD values seen in Table 7. One reason for low AOD retrievals was proposed by Jethva et al. (2014) and was shown in Deaconu et al. (2017). In case of an optically thick aerosol layer, the sensitivity of the backscattered signal would be reduced or lost because of the strongly attenuated two-way transmission. As a result, the operational algorithm may position the base of the aerosol layer higher in altitude, thus underestimating the geometrical thickness of the aerosol layer and consequently the AOD. The selection of an inappropriate aerosol lidar ratio might also contribute to the underestimation of the AOD. AE values were also relatively low, 0.12; however these are again not indicative of plume optical properties as they are for the larger background layer. In any case, computing AE based on low AOD values may not be a good estimate for local aerosol particle size. Figure 8c shows a plume composition similar to the event 14 plume. The vertical feature mask shows a mixed feature of clouds, aerosols and low-confidence aerosol. Figure 8d shows the aerosol classification within the aerosol layer. Judging by these results the aerosol layer reaching up to 1.5 km above mean sea level was classified as a mixture of dust, polluted dust and smoke. The plume cross section was successfully identified as smoke aerosols; however the background layer situated north of the plume was not typed as dusty marine, as seen from the lidar ratio of 37 ± 15 sr.
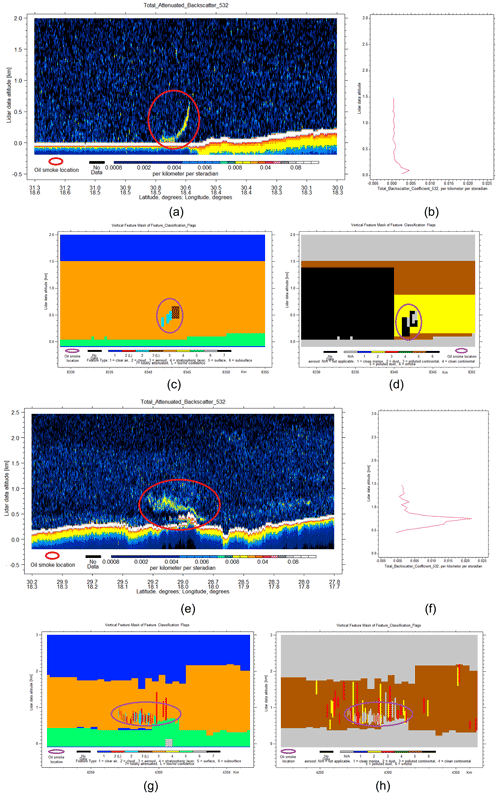
Figure 8Image of event 13 (a) based on CALIPSO total attenuated backscatter (532 nm) vs. lidar data altitude data. (b) Particulate backscatter coefficient profile CALIPSO level 2 (532 nm) at As Sidr. (c) Cloud feature classification and (d) aerosol feature classification at As Sidr. (e) Image of event 11, based on CALIPSO total attenuated backscatter (532 nm) vs. lidar data altitude data. (f) Particulate backscatter coefficient profile CALIPSO level 2 (532 nm) at Ra's Lanuf. (g) Cloud feature classification and (h) aerosol feature classification at Ra's Lanuf.
Table 6Mean plume values for lidar-specific aerosol properties (PDR – particulate depolarization ratio; lidar ratio) and uncertainty estimates based on CALIPSO measurements.
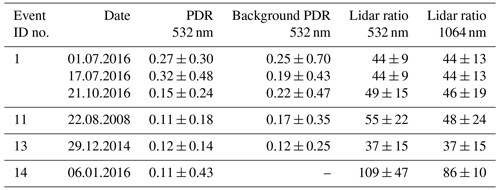
Event 11 was captured inland as the plume cross section was identified 170 km south of the Ra's Lanuf tank depot. Figure 8f shows a particulate backscatter profile through the plume centre, describing a fairly inhomogeneous mass of smoke particles. The main plume was concentrated between 500 and 900 m; however lower concentration may have been mixed with local dust particles all the way up to 1500 m. Figure 8e shows the extent of the plume as it travelled southwards inland. As was the case of the previous event, plume lidar ratios were determined by an unconstrained solution. Thus values of 55 ± 22 and 48 ± 24 sr from 532 and 1064 nm channels were determined within the plume feature as well as for the entire layer within Fig. 8e representing the local planetary boundary layer (PBL). These values suggest a mixture of polluted dust within the local PBL (Kim et al., 2018). Plume particulate depolarization ratio values of 0.11 ± 0.18 are similar to the previous events while background values of 0.17 ± 0.35 are indicative of a polluted dust mixture (Omar et al., 2009). The average plume particulate backscatter (532 nm) measured 0.007 km−1 sr−1 while the 1064 nm channel measured 0.008 km−1 sr−1, which showed an increase of 6 to 9 times larger than the local background values. This large difference is also evident from the plume and background AOD. These plume values were directly influenced by the lidar ratio solution. A constrained solution may have resulted in larger values since smoke LR values are generally higher than polluted dust values (Kim et al., 2018). AE values remain low (0.05) and similar to the previous event at Ra's Lanuf, suggesting a coarse-mode-dominant aerosol mixture, while background values were more indicative of polluted dust, averaging 0.71. Unlike event 13, this plume was averaged at 5 km resolution, resulting in plume AOD values higher than background values. Similar to the previous events, the feature classification algorithm shows a mixture of clouds and aerosols in the plume bins. This composition is evident in Fig. 8g and may affect the retrieval of smoke optical properties. Figure 8h shows the local aerosol layer up to approximately 2 km above local ground level. A significant portion of plume bins were discarded as cloud features even though the CAD score of −99 indicated high-confidence aerosols.
Event 1, at the Qayyarah oil fields in northern Iraq, was captured by CALIPSO in three distinct cases. In all three cases CALIPSO overpassed within less than 35 km southwest from the well fires. The plume particulate backscatter and extinction coefficients ranged from 2 to 5 times higher than local background values. The plumes were identified within the PBL, and as a result, the lidar ratios between 44 ± 9 and 49 ± 15 suggested the presence of dust aerosols. Particulate depolarization ratio values were higher than the previous cases, ranging from 0.15 ± 0.24 to 0.32 ± 0.48, also suggesting dust dominance. AOD values observed that in plume the bins remained generally low, <0.1. However these values were still up to 5 times higher than background values. The AE values remain consistently low, ranging between −0.03 and 0.39, indicating the presence of a coarse-mode-dominant aerosol mixture. The local atmospheric scene on 21 October 2016 was marked by a mixture of oil smoke and SO2 plume as a result of the Islamic State setting fire to the Al-Mishraq sulfur plant situated NNE of the burning oil fields. This mixed layer was also suggested by Kahn et al. (2019), who used MISR Active Aerosol Plume-Height (AAP) to establish the SO2 and oil smoke plume elevation on the same day (Khan and Zhaoying, 2020). Judging from the lidar ratios 49 ± 15 (532 nm) and 46 ± 19 (1064 nm) and the particulate depolarization ratio of 0.15 ± 0.24, the CALIPSO subtyping algorithm identified a mix of polluted dust aerosols.
Based on CALIPSO measurements, the smoke backscatter and extinction coefficient ranged from 2 to 9 times higher than background levels. In four out of six cases, particulate depolarization ratio revealed values between 0.11 and 0.15, resembling moderately depolarizing smoke, while larger values in two cases were mostly due to the presence of dust particles in the local atmospheric scene. Apart from one case, all lidar ratios were obtained by unconstrained retrievals as the plume resided in the PBL. The opaque feature measured high lidar ratios of 109 ± 47 sr (532 nm) and 86 ± 10 sr (1064 nm) that resemble the smoke lidar ratio found in literature (Giannakaki et al., 2016; Haarig et al., 2018). We suspect these values are a strong indicator for the heavy light-absorbing nature of the smoke plume. Average CAD scores ranged from −46 to −99, which would indicate a strong confidence for the presence of aerosols. The feature classification algorithm indicated the presence of small clouds in three out of six cases, suggesting mixed cloud–aerosol features. AE values were consistently low in all cases, suggesting the presence of larger smoke particles in the plume's cross sections. AOD values measured between 0.02 and 1.52 and were directly influenced by fuel burning rates, local background aerosol loading and especially lidar ratio solutions. The event 14 plume was identified above the PBL up to 4200 m. This is a good indicator for the magnitude of the event as it involved several tank fires with higher burning rates simultaneously injecting larger concentrations of aerosol at higher elevations in the troposphere. Based on this small number of events, it is difficult to assign a separate aerosol type for these oil smoke plumes. However valuable information regarding size distributions, particulate depolarization ratio and to some extent lidar ratio can be retained from this study. It should be mentioned that these values reflect smoke plumes located very close to the fire sources and thus present low mixing ratios with other local aerosols.
3.4 AERONET case study
As discussed in the introduction section, oil smoke plumes have been rarely observed using ground-based remote sensing instruments such as AERONET sun photometers. We used AERONET version 3 direct sun data to assess the presence of oil smoke plumes. Only one study was found in scientific literature (Mather et al., 2007), which measured aerosol properties of the Buncefield plume at two distinct locations. Here we identified the smoke plume, at event 10, resulting from naphtha tank fires in Vasylkiv, Kyiv Oblast, Ukraine, on 9 June 2015. The smoke plume was also captured in RGB images as seen in Fig. 6, lower left image. Figure 9a shows the distinct signature of the oil smoke plume as AOD values increased significantly in all wavelengths. Figure 9c is a good indication of the increasing particle size with respect to the other days observed in MODIS and CALIPSO data as well. Figure 9d shows the daily evolution of AE with values between 0.45 and 0.9 for the time frame in which the plume was observed. Figure 9b shows AOD values rising as the plume was travelling NE over Kiev. The AERONET station in Kiev is situated approximately 35 km NE of the Vasylkiv tank farm. The peak of the plume was detected at 09:45 UTC when the AOD was 0.68 at 500 nm. Unfortunately, no inversion products coinciding with direct sun measurements were available as the Kiev sky was partially cloudy at the time.
3.5 Data comparison between methods and other similar studies
The results presented in this study show a wide range of values that are attributed to a multitude of local factors such as background aerosols, burning rates, weather conditions, fuel type, time of retrieval and local geography. Other factors can be attributed to the different types of methods and algorithms used to retrieve aerosol-specific data. MODIS data showed relatively low values of plume-specific AOD ranging from −0.04 ± 0.04 to 0.16 ± 0.08. The only event which was captured by both MODIS and CALIPSO retrievals, within 2 min apart, showed a large level of discrepancy. In particular, event 14 showed average column AOD values of 0.21 ± 0.09 over the plume area with a maximum pixel value of 0.32 ± 0.11 (550 nm). In contrast, CALIOP measurements revealed an average plume AOD value of 1.52 ± 0.8 (532 nm), which was plume specific as no other extinction values were detected beneath or above the plume through the troposphere and stratosphere in the local scene. In the remaining five cases, CALIPSO retrieved AOD values ranging from 0.02 ± 0.01 to 0.16 ± 0.06 for average plume thickness ranging from 0.120 to 0.375 km. While these values more closely resemble the successful MODIS retrievals, one should restrain from a forward comparison. A reason is that MODIS did not successfully retrieve AOD values over land; thus no direct comparison can be made with CALIPSO for events 1 and 11. MODIS retrievals from event 13 on 28 and 29 December 2014 were approximately 12 h apart from the CALIPSO retrievals. Both sensors agreed to low AOD values for the plumes in question. The high levels of uncertainty surrounding MODIS LUT values and CALIPSO unconstrained lidar solutions suggest the need for a more in-depth analysis. The only case seen by an AERONET sun photometer (event 10) indicates AOD values ranging from 0.28 to 0.68 ± 0.01 (500 nm); however the satellite images suggest that these values were not indicative for the main plume, which most likely did not reach Kiev. Nevertheless, MODIS did not successfully retrieve any AOD values from this event or any other over land while for other events over ocean it did not yield such high AOD values. It is safe to say that MODIS AOD retrievals for oil smoke plumes may not produce satisfactory results since the predetermined LUT values may not contain events similar to the ones described in this study. The CALIPSO AOD measurements are directly influenced by the lidar ratio. For the events retrieved by CALIPSO, a correct estimate of lidar ratio is very difficult to achieve based on unconstrained solution. On one hand, these lidar ratios are not directly measured. On the other hand, lidar ratio for oil smoke plumes may exhibit a different behaviour, considering the high BC content, different from biomass smoke or smoke/polluted continental aerosols. In cases of “clean” atmospheric conditions a constrained solution may result in better AOD estimates. However these conditions are rarely achieved, with less than 0.01 % of all aerosol layers detected (Tackett et al., 2018). In the one case where the lidar ratios were directly estimated (event no. 14) the uncertainties regarding opaque aerosol layers make it difficult to assess if the AOD values are overestimated or underestimated. AE values seem to be more consistent between MODIS, CALIPSO and AERONET as all techniques suggest the presence of coarse aerosol mixtures; however in conditions with low AOD values, one should restrain from direct comparisons.
Table 8 lists the oil smoke optical properties from different studies that utilized similar ground-based or airborne measuring techniques. Overall, the MODIS AOD estimates are very low compared to the reference studies given in Table 8. It should be mentioned that AOD values from the Gulf war smoke plumes are larger for the most part due to the magnitude of the event. These measurements describe super composite plumes resulting from a large number of well fires and pool fires. An event that more closely resembles the events in this study was analysed by Mather et al. (2007), who retrieved AOD values of 0.28 to 0.68 ± 0.01 (500 nm) at 50 km away from the oil depot (Mather et al., 2007). These values are similar to the AERONET values from Kiev (event 10) presented in this study as in both cases AOD was retrieved using sun photometers, and the Kiev AERONET station is located approximately 20 km from the oil depot. However, the Buncefield event was significantly larger than the Vasylkiv event. Larger AOD values were measured by Pilewskie and Valero (1992) and Nakajima et al. (1996), in both cases describing much larger smoke plumes than event 10. AE values from and Nakajima et al. (1996) and Mather et al. (2007) are in general in good agreement with our case studies. Reff values from MODIS also show the presence of larger particles analogous to Mather et al. (2007).
Table 8Oil smoke optical properties from ground-based and flight measurements along with the scientific reference.
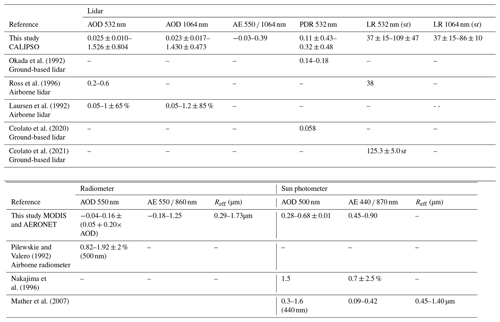
CALIPSO AOD measurements from event 14 are similar to the upper ranges measured by Laursen et al. (1992) while the unconstrained retrievals from this study more closely resemble the lower bounds from Laursen et al. (1992). The AOD values registered by Ross et al. (1996) fall out of the range of this current study. This may be a result of plume dimensions as the Ra's Lanuf plume cross section was much larger and thicker than the plume described in Ross et al. (1996). In any case, event 14 is expected to measure the largest AOD values out of all smoke plumes solely based on the magnitude of the event. Judging by the results seen in Fig. 8a, an aerosol feature exhibiting such heavy attenuation in the layers directly beneath would most likely yield higher AOD values. Particulate depolarization ratio for four out of six cases reflects the values shown by Okada et al. (1992), indicating that oil smoke particles are moderately depolarizing. Ceolato et al. (2020) showed PDR of 0.058 for super aggregates. However these values reflect early combustion particles unaltered by the effects of coating. The effects of coating on soot particles can result in PDR larger than 0.1 for oil smoke as suggested by Okada et al. (1992). The same effects are also visible in PDR of biomass burning evident in Kanngießer and Kahnert (2018), Haarig et al. (2018), and references therein. The opaque feature indicated high lidar ratio values (109 ± 47 sr at 532 nm), even after a 5 % reduction, much larger than Ross et al. (1996), who measured only 38 sr. High LR values, 125.3 ± 5.0 sr at 550 nm, are also suggested in Ceolato et al. (2021) and closely resemble the values estimated from event 14. One would expect large lidar ratio values to be the result of the highly absorbent nature of these smoke plumes (high percentage of black carbon, high plume homogeneity and low mixing ratio), leading to larger extinction values.
In this study, we examined oil smoke plumes derived from 30 major industrial events within a 12-year period. To our knowledge this is the first study that utilized a synergetic approach based on satellite remote sensing techniques. The MODIS ocean algorithm successfully retrieved aerosol properties in 10 cases, ranging on average from −0.06 to 0.16 for plume-specific AOD, −0.18 to 1.25 for Ångström exponent and 0.29 to 1.73 µm for effective radius. Apart from event 4, all the remaining smoke plumes exhibited AE values lower than 0.74, suggesting that the smoke plumes were coarse-mode dominant. CALIPSO measurements showed values of plume AOD ranging from 0.02 to 0.16 (532 nm) and 0.02 to 0.15 (1064 nm) except for one event where AOD values reached 1.52 (532 nm) and 1.43 (1064 nm). AE values ranged from −0.03 to 0.39, which agree with MODIS. A large discrepancy was found in event 14 where CALIPSO AOD values were 5 times higher than MODIS. For this specific event, CALIPSO retrieved high lidar ratios of 109 ± 47 (532 nm) and 86 ± 10 sr (1064 nm) based on a constrained retrieval scheme for opaque aerosol layers. The high concentration of water vapour emitted by the oil fire may have contributed to instances of small cloud formation above the smoke plume and thus contaminated the retrievals. Typically, lidar ratio ranged from 37 to 55 sr (532 nm) and 37 to 48 sr (1064 nm); however these unconstrained solutions were indicative of the local aerosol scene and not directly measured. Particulate backscatter coefficient values ranged from 0.002 to 0.015 km−1 sr−1 (532 nm) and 0.002 to 0.017 km−1 sr−1 (1064 nm). Particulate extinction coefficient values ranged from 0.10 to 1.65 km−1 (532 nm) and 0.10 to 1.55 km−1 (1064 nm). On average backscatter and extinction coefficient values were 2 to 9 times higher than the local background. Particulate depolarization ratios ranged from 0.11 to 0.15 for four out of six cases while the remaining cases were 0.27 and 0.32. We suspect that this discrepancy in the two cases at Qayyarah are a result of dust aerosols presented in the smoke plumes. The values presented agree with similar studies that used ground-based and airborne measurements. We believe that MODIS gives a conservative estimate of the plume AOD since MODIS algorithms rely on general aerosol models and various atmospheric conditions within the look-up tables which do not reflect the highly light-absorbent nature of these smoke plumes. Furthermore, the spectral reflectance relationship used by MODIS algorithms may hinder most retrieval attempts as thick black plumes exhibit a distinct spectral signature. CALIPSO measurements are heavily dependent on unconstrained solutions, which in turn do not reflect the oil smoke plumes. Thus, we also believe that the AOD values based on CALIPSO measurements are conservative in nature since strong absorbing smoke would yield larger lidar ratios and AOD values. In general, constrained retrievals would better reflect the actual smoke properties because they do not rely on an ad hoc assignment of lidar ratio. However, assigning a constrained retrieval to oil smoke plumes requires (1) for the plume to be surrounded by clear air and (2) smoke concentrations not to exceed a threshold where total attenuation is achieved. The lidar ratios generated from event 14 represent an extremely rare occasion where the smoke plume was treated as an opaque aerosol layer. As such, it was difficult to assess whether the lidar ratios where over- or underestimated, although we believe that this current solution is still preferable to unconstrained solutions. We stress the need for further lidar measurements of oil smoke plumes since based on this study we cannot conclude whether these aerosols belong to a different smoke subtype. Future space-borne lidar missions such as EarthCare (Illingworth et al., 2015) will provide direct measurements of lidar ratios and the possibility of better AOD estimations with regard to these types of events. Based on this study we concluded that the MODIS land algorithms are not yet suited for retrieving aerosol properties for these types of smoke plumes due to the highly light-absorbing properties of these aerosols. This study has shown a novel method of oil smoke plume identification and analysis, which does not require, in some cases, perilous fieldwork. We believe that these types of studies are a strong indication for the need of improved aerosol models and retrieval algorithms. For these types of aerosols, better AOD estimates are important for both air quality and climate change implications.
Not applicable.
CALIPSO data are available at https://asdc.larc.nasa.gov/project/CALIPSO/CAL_LID_L1-Standard-V4-10_V4-10, https://asdc.larc.nasa.gov/project/CALIPSO/CAL_LID_L2_05kmALay-Standard-V4-20_V4-20, https://asdc.larc.nasa.gov/project/CALIPSO/CAL_LID_L2_05kmAPro-Standard-V4-20_V4-20 and https://asdc.larc.nasa.gov/project/CALIPSO/CAL_LID_L2_VFM-Standard-V4-20_V4-20 (Winker, 2016, 2018a, b and c). MODIS data are available: https://ladsweb.modaps.eosdis.nasa.gov/missions-and-measurements/products/MOD04_L2 and https://ladsweb.modaps.eosdis.nasa.gov/missions-and-measurements/products/MYD04_L2 (MODIS Atmosphere Science Team, 2017a and b).
AM, NA and AO carried out the conceptualization and methodology. AM, ATR and HIS carried out the formal analysis. AM, ATR and HIS provided visuals for the paper. AM, NA and CSB wrote the initial draft. NP, LTD and DN reviewed and edited the initial draft. NA and CSB acquired funding for the current research. AO provided supervision for the PhD students AM and ATR.
The contact author has declared that neither they nor their co-authors have any competing interests.
Publisher's note: Copernicus Publications remains neutral with regard to jurisdictional claims in published maps and institutional affiliations.
This work was supported by the project entitled “Development of ACTRIS-UBB infrastructure with the aim of contributing to pan-European research on atmospheric composition and climate change” SMIS CODE 126436, co-financed by the European Union through the Competitiveness Operational Programme 2014–2020.
This work was supported by the project entitled “Strengthening the participation of the ACTRIS-RO consortium in the pan-European research infrastructure ACTRIS” SMIS CODE 107596, co-financed by the European Union through the Competitiveness Operational Programme 2014–2020.
This research has been supported by the Ministerul Cercetării şi Inovării (grant nos. SMIS CODE 126436 and SMIS CODE 107596).
This paper was edited by Evangelos Gerasopoulos and reviewed by two anonymous referees.
Ahmadi, O., Mortazavi, S. B., and Mahabadi, H. A.: Review of Atmospheric Storage Tank Fire Scenarios: Costs and Causes, J. Fail. Anal. Preven., 20, 384–405, https://doi.org/10.1007/s11668-020-00846-5, 2020.
Akagi, S. K., Yokelson, R. J., Wiedinmyer, C., Alvarado, M. J., Reid, J. S., Karl, T., Crounse, J. D., and Wennberg, P. O.: Emission factors for open and domestic biomass burning for use in atmospheric models, Atmos. Chem. Phys., 11, 4039–4072, https://doi.org/10.5194/acp-11-4039-2011, 2011.
Al Jazeera: Soldiers die in attack on Libya oil terminals, https://www.aljazeera.com/news/middleeast/2014/12/soldiers-killed-attack-benghazi-port-2014122673856971767.html (last access: 12 September 2019), 2014.
Andreae, M. O.: Emission of trace gases and aerosols from biomass burning – an updated assessment, Atmos. Chem. Phys., 19, 8523–8546, https://doi.org/10.5194/acp-19-8523-2019, 2019.
Andreae, M. O. and Merlet, P.: Emission of trace gases and aerosols from biomass burning, Global Biogeochem. Cycles, 15, 955–966, https://doi.org/10.1029/2000GB001382, 2001.
Andreae, M. O. and Ramanathan, V.: Climate's dark forcings, Science, 340, 280–281, 2013.
An Han, H., Han, I., McCurdy, S., Whitworth, K., Delclos, G., Rammah, A., and Symanski, E.: The Intercontinental Terminals Chemical Fire Study: A Rapid Response to an Industrial Disaster to Address Resident Concerns in Deer Park, Texas, Int. J. Environ. Res. Public Health, 17, 986, https://doi.org/10.3390/ijerph17030986, 2020.
Avery, M. A., Ryan, R. A., Getzewich, B. J., Vaughan, M. A., Winker, D. M., Hu, Y., Garnier, A., Pelon, J., and Verhappen, C. A.: CALIOP V4 cloud thermodynamic phase assignment and the impact of near-nadir viewing angles, Atmos. Meas. Tech., 13, 4539–4563, https://doi.org/10.5194/amt-13-4539-2020, 2020.
Barth, S. K., Dursa, E. K., Bossarte, R., and Schneiderman, A.: Lifetime Prevalence of Respiratory Diseases and Exposures Among Veterans of Operation Enduring Freedom and Operation Iraqi Freedom Veterans: Results From the National Health Study for a New Generation of U.S. Veterans, J. Occup. Environ. Med., 58, 1175–1180, https://doi.org/10.1097/JOM.0000000000000885, 2016.
BBC: Libya: Gaddafi troops take rebel oil port of Ras Lanuf, https://www.bbc.com/news/world-africa-12721908, (last access: 12 September 2019), 2011.
BBC: Libya airstrikes hit Misrata militants for first time, https://www.bbc.com/news/world-africa-30616817, (last access: 12 September 2019), 2014.
BBC: Gulf of Oman tanker attacks: What we know, https://www.bbc.com/news/world-middle-east-48627014, (last access: 10 October 2019), 2019.
Beelen, R., Raaschou-Nielsen, O., Stafoggia, M., Andersen, Z. J., Weinmayr, G., Hoffmann, B., Wolf, K., Samoli, E., Fischer, P., Nieuwenhuijsen, M., Vineis, P., Xun, W. W., Katsouyanni, K., Dimakopoulou, K., Oudin, A., Forsberg, B., Modig, L., Havulinna, A. S., Lanki, T., Turunen, A., Oftedal, B., Nystad, W., Nafstad, P., De Faire, U., Pedersen, N. L., Östenson, C.-G., Fratiglioni, L., Penell, J., Korek, M., Pershagen, G., Eriksen, K. T., Overvad, K., Ellermann, T., Eeftens, M., Peeters, P. H., Meliefste, K., Wang, M., Bueno-de-Mesquita, B., Sugiri, D., Krämer, U., Heinrich, J., de Hoogh, K., Key, T., Peters, A., Hampel, R., Concin, H., Nagel, G., Ineichen, A., Schaffner, E., Probst-Hensch, N., Künzli, N., Schindler, C., Schikowski, T., Adam, M., Phuleria, H., Vilier, A., Clavel-Chapelon, F., Declercq, C., Grioni, S., Krogh, V., Tsai, M.-Y., Ricceri, F., Sacerdote, C., Galassi, C., Migliore, E., Ranzi, A., Cesaroni, G., Badaloni, C., Forastiere, F., Tamayo, I., Amiano, P., Dorronsoro, M., Katsoulis, M., Trichopoulou, A., Brunekreef, B., and Hoek, G.: Effects of long-term exposure to air pollution on natural-cause mortality: an analysis of 22 European cohorts within the multicentre ESCAPE project, The Lancet, 383, 785–795, https://doi.org/10.1016/S0140-6736(13)62158-3, 2014.
Bellingcat: Fuel to the Fire: Satellite Imagery Captures Burning Oil Tanks Libya, https://www.bellingcat.com/news/mena/2018/06/18/fuel-fire-satellite-imagery-captures-burning-oil-tanks-libya/, (last access: 12 September 2019), 2018.
Biezma, M. V., Andrés, M. A., Agudo, D., and Briz, E.: Most fatal oil & gas pipeline accidents through history: A lessons learned approach, Engineering Failure Analysis, 110, 104446, https://doi.org/10.1016/j.engfailanal.2020.104446, 2020.
Bloomberg: Nigeria Loses Almost Half Its Power Output After Pipeline Fire, https://www.bloomberg.com/news/articles/2018-01-03/nigeria-loses-almost-half-its-power-output-after-pipeline-fire, (last access: 6 May 2019), 2018.
Bond, T. C., Doherty, S. J., Fahey, D. W., Forster, P. M., Berntsen, T., DeAngelo, B. J., Flanner, M. G., Ghan, S., Kärcher, B., Koch, D., Kinne, S., Kondo, Y., Quinn, P. K., Sarofim, M. C., Schultz, M. G., Schulz, M., Venkataraman, C., Zhang, H., Zhang, S., Bellouin, N., Guttikunda, S. K., Hopke, P. K., Jacobson, M. Z., Kaiser, J. W., Klimont, Z., Lohmann, U., Schwarz, J. P., Shindell, D., Storelvmo, T., Warren, S. G., and Zender, C. S.: Bounding the role of black carbon in the climate system: A scientific assessment: Black Carbon in the Climate System, J. Geophys. Res.-Atmos., 118, 5380–5552, https://doi.org/10.1002/jgrd.50171, 2013.
Boucher, O., Balkanski, Y., Hodnebrog, Ø., Myhre, C. L., Myhre, G., Quaas, J., Samset, B. H., Schutgens, N., Stier, P., and Wang, R.: Jury is still out on the radiative forcing by black carbon, P. Natl. Acad. Sci. USA, 113, E5092–E5093, https://doi.org/10.1073/pnas.1607005113, 2016.
Brain, J. D., Long, N. C., Wolfthal, S. F., Dumyahn, T., and Dockery, D. W.: Pulmonary toxicity in hamsters of smoke particles from Kuwaiti oil fires, Environ. Health Persp., 106, 141–146, https://doi.org/10.1289/ehp.98106141, 1998.
Brauer, M., Freedman, G., Frostad, J., van Donkelaar, A., Martin, R. V., Dentener, F., Dingenen, R. van, Estep, K., Amini, H., Apte, J. S., Balakrishnan, K., Barregard, L., Broday, D., Feigin, V., Ghosh, S., Hopke, P. K., Knibbs, L. D., Kokubo, Y., Liu, Y., Ma, S., Morawska, L., Sangrador, J. L. T., Shaddick, G., Anderson, H. R., Vos, T., Forouzanfar, M. H., Burnett, R. T., and Cohen, A.: Ambient Air Pollution Exposure Estimation for the Global Burden of Disease 2013, Environ. Sci. Technol., 50, 79–88, https://doi.org/10.1021/acs.est.5b03709, 2015.
Brunekreef, B. and Holgate, S. T.: Air pollution and health, The Lancet, 360, 1233–1242, https://doi.org/10.1016/S0140-6736(02)11274-8, 2002.
Bulmer, M. H.: Military Use Of Environmental Degradation by Islamic State, Northern Iraq, SCIM, 46, 123–147, https://doi.org/10.5787/46-1-1228, 2018.
Business-humanrights: Actual conditions On the assessment of fire accident happened on December 4, 2015 in Deep Sea Platform No. 10 of Guneshli Oilfield owned by SOCAR, https://www.business-humanrights.org/sites/default/files/documents/SOCAR-response-re-Guneshli-oil-field-accident.pdf, (last access: 12 September 2019), 2015.
Business Insider: Militants attack storage tanks near Libya's Ras Lanuf oil terminal, https://www.businessinsider.com/militants-attack-near-libyas-ras-lanuf-oil-terminal-2016-1, (last access: 12 September 2019), 2016.
Cahalan, R. F.: The Kuwait oil fires as seen by Landsat, J. Geophys. Res.-Amos., 97, 14565, https://doi.org/10.1029/92JD00799, 1992.
Ceolato, R., Paulien, L., Maughan, J. B., Sorensen, C. M., and Berg, M. J.: Radiative properties of soot fractal superaggregates including backscattering and depolarization, J. Quant. Spectrosc. Ra., 247, 106940, https://doi.org/10.1016/j.jqsrt.2020.106940, 2020.
Ceolato, R., Bedoya-Velasquez, A., Fossard, F., Mouysset, V., Paulien, L., Lefebvre, S., Mazzoleni, C., Sorensen, C., Berg, M., and Yon, J.: Black carbon aerosol number and mass concentration measurements by picosecond short-range elastic backscatter lidar, https://doi.org/10.21203/rs.3.rs-806433/v1, in review, 2021.
Daum, P. H., Al-Sunaid, A., Busness, K. M., Hales, J. M., and Mazurek, M.: Studies of the Kuwait oil fire plume during midsummer 1991, J. Geophys. Res., 98, 16809, https://doi.org/10.1029/93JD01204, 1993.
Deaconu, L. T., Waquet, F., Josset, D., Ferlay, N., Peers, F., Thieuleux, F., Ducos, F., Pascal, N., Tanré, D., Pelon, J., and Goloub, P.: Consistency of aerosols above clouds characterization from A-Train active and passive measurements, Atmos. Meas. Tech., 10, 3499–3523, https://doi.org/10.5194/amt-10-3499-2017, 2017.
Deng, X., Shi, C., Wu, B., Chen, Z., Nie, S., He, D., and Zhang, H.: Analysis of aerosol characteristics and their relationships with meteorological parameters over Anhui province in China, Atmos. Res., 109–110, 52–63, https://doi.org/10.1016/j.atmosres.2012.02.011, 2012.
Draxler, R. R., McQueen, J. T., and Stunder, B. J.: An evaluation of air pollutant exposures due to the 1991 Kuwait oil fires using a Lagrangian model, Atmos. Environ., 28, 2197–2210, 1994.
Dubovik, O., Holben, B. N., Kaufman, Y. J., Yamasoe, M., Smirnov, A., Tanré, D., and Slutsker, I.: Single-scattering albedo of smoke retrieved from the sky radiance and solar transmittance measured from ground, J. Geophys. Res., 103, 31903–31923, https://doi.org/10.1029/98JD02276, 1998.
Dubovik, O., Li, Z., Mishchenko, M. I., Tanre, D., Karol, Y., Bojkov, B., Cairns, B., Diner, D. J., Espinosa, W. R., and Goloub, P.: Polarimetric remote sensing of atmospheric aerosols: Instruments, methodologies, results, and perspectives, J. Quant. Spectrosc. Ra., 224, 474–511, 2019.
Dutkiewicz, V. A., Alvi, S., Ghauri, B. M., Choudhary, M. I., and Husain, L.: Black carbon aerosols in urban air in South Asia, Atmos. Environ., 43, 1737–1744, https://doi.org/10.1016/j.atmosenv.2008.12.043, 2009.
Eck, T. F., Holben, B. N., Slutsker, I., and Setzer, A.: Measurements of irradiance attenuation and estimation of aerosol single scattering albedo for biomass burning aerosols in Amazonia, J. Geophys. Res., 103, 31865–31878, https://doi.org/10.1029/98JD00399, 1998.
Etzel, R. A. and Ashley, D. L.: Volatile organic compounds in the blood of persons in Kuwait during the oil fires, Int. Arch. Occ. Env. Hea., 66, 125–129, https://doi.org/10.1007/BF00383368, 1994.
Fan, J., Wang, Y., Rosenfeld, D., and Liu, X.: Review of Aerosol – Cloud Interactions: Mechanisms, Significance, and Challenges, J. Atmos. Sci., 73, 4221–4252, https://doi.org/10.1175/JAS-D-16-0037.1, 2016.
Fan, X. and Qu, Y.: Retrieval of High Spatial Resolution Aerosol Optical Depth from HJ-1 A/B CCD Data, Remote Sensing, 11, 832, https://doi.org/10.3390/rs11070832, 2019.
Ferek, R. J., Hobbs, P. V., Herring, J. A., Laursen, K. K., Weiss, R. E., and Rasmussen, R. A.: Chemical composition of emissions from the Kuwait oil fires, J. Geophys. Res., 97, 14483, https://doi.org/10.1029/92JD01247, 1992.
Fierce, L., Riemer, N., and Bond, T. C.: Explaining variance in black carbon's aging timescale, Atmos. Chem. Phys., 15, 3173–3191, https://doi.org/10.5194/acp-15-3173-2015, 2015.
Financial Tribune: Oil, LNG Pipeline Blast Prompts Evacuation in Khuzestan Village, https://financialtribune.com/articles/energy/97831/oil-lng-pipeline-blast-prompts-evacuation-in-khuzestan-village, last access: 9 October 2019.
Getzewich, B. J., Vaughan, M. A., Hunt, W. H., Avery, M. A., Powell, K. A., Tackett, J. L., Winker, D. M., Kar, J., Lee, K.-P., and Toth, T. D.: CALIPSO lidar calibration at 532 nm: version 4 daytime algorithm, Atmos. Meas. Tech., 11, 6309–6326, https://doi.org/10.5194/amt-11-6309-2018, 2018.
Giannakaki, E., van Zyl, P. G., Müller, D., Balis, D., and Komppula, M.: Optical and microphysical characterization of aerosol layers over South Africa by means of multi-wavelength depolarization and Raman lidar measurements, Atmos. Chem. Phys., 16, 8109–8123, https://doi.org/10.5194/acp-16-8109-2016, 2016.
Guarnieri, M. and Balmes, J. R.: Outdoor air pollution and asthma, The Lancet, 383, 1581–1592, https://doi.org/10.1016/S0140-6736(14)60617-6, 2014.
Gullett, B. K., Hays, M. D., Tabor, D., and Wal, R. V.: Characterization of the particulate emissions from the BP Deepwater Horizon surface oil burns, Mar. Pollut. Bull., 107, 216–223, https://doi.org/10.1016/j.marpolbul.2016.03.069, 2016.
Gullett, B. K., Aurell, J., Holder, A., Mitchell, W., Greenwell, D., Hays, M., Conmy, R., Tabor, D., Preston, W., George, I., Abrahamson, J. P., Vander Wal, R., and Holder, E.: Characterization of emissions and residues from simulations of the Deepwater Horizon surface oil burns, Mar. Pollut. Bull., 117, 392–405, https://doi.org/10.1016/j.marpolbul.2017.01.083, 2017.
Gupta, P., Remer, L. A., Levy, R. C., and Mattoo, S.: Validation of MODIS 3 km land aerosol optical depth from NASA's EOS Terra and Aqua missions, Atmos. Meas. Tech., 11, 3145–3159, https://doi.org/10.5194/amt-11-3145-2018, 2018.
Haarig, M., Ansmann, A., Baars, H., Jimenez, C., Veselovskii, I., Engelmann, R., and Althausen, D.: Depolarization and lidar ratios at 355, 532, and 1064 nm and microphysical properties of aged tropospheric and stratospheric Canadian wildfire smoke, Atmos. Chem. Phys., 18, 11847–11861, https://doi.org/10.5194/acp-18-11847-2018, 2018.
Health and Safety Executive and Buncefield Major Incident Investigation Board (Great Britain): The Buncefield incident, 11 December 2005: the final report of the Major Incident Investigation Board, Vol. 1., Health and Safety Executive, Sudbury, ISBN: 978 0 7176 6270 8, 2008.
He, C., Liou, K.-N., Takano, Y., Zhang, R., Levy Zamora, M., Yang, P., Li, Q., and Leung, L. R.: Variation of the radiative properties during black carbon aging: theoretical and experimental intercomparison, Atmos. Chem. Phys., 15, 11967–11980, https://doi.org/10.5194/acp-15-11967-2015, 2015.
Heller, J. M.: Oil Well Fires of Operation Desert Storm – Defining Troop Exposures and Determining Health Risks, Mil. Med., 176, 46–51, https://doi.org/10.7205/MILMED-D-11-00079, 2011.
Hobbs, P. V. and Radke, L. F.: Airborne Studies of the Smoke from the Kuwait Oil Fires, Science, 256, 987–991, https://doi.org/10.1126/science.256.5059.987, 1992.
Hoek, G., Krishnan, R. M., Beelen, R., Peters, A., Ostro, B., Brunekreef, B., and Kaufman, J. D.: Long-term air pollution exposure and cardio-respiratory mortality: a review, J. Environ. Health, 12, 1–16, 2013.
Hoek, M. R., Bracebridge, S., and Oliver, I.: Health impact of the Buncefield oil depot fire, December 2005: Study of accident and emergency case records, J. Public Health., 29, 298–302, https://doi.org/10.1093/pubmed/fdm036, 2007.
Holben, B. N., Eck, T. F., Slutsker, I., al Tanre, D., Buis, J. P., Setzer, A., Vermote, E., Reagan, J. A., Kaufman, Y. J., and Nakajima, T.: AERONET – A federated instrument network and data archive for aerosol characterization, Remote Sens. Environ., 66, 1–16, 1998.
Hsu, N. C., Tsay, S.-C., King, M. D., and Herman, J. R.: Aerosol Properties Over Bright-Reflecting Source Regions, IEEE T. Geosci. Remote, 42, 557–569, https://doi.org/10.1109/TGRS.2004.824067, 2004.
Hsu, N. C., Tsay, S.-C., King, M. D., and Herman, J. R.: Deep Blue Retrievals of Asian Aerosol Properties During ACE-Asia, IEEE T. Geosci. Remote, 44, 3180–3195, https://doi.org/10.1109/TGRS.2006.879540, 2006.
Hsu, N. C., Jeong, M.-J., Bettenhausen, C., Sayer, A. M., Hansell, R., Seftor, C. S., Huang, J., and Tsay, S.-C.: Enhanced Deep Blue aerosol retrieval algorithm: The second generation, J. Geophys. Res.-Atmos., 118, 9296–9315, https://doi.org/10.1002/jgrd.50712, 2013.
Hunt, W. H., Winker, D. M., Vaughan, M. A., Powell, K. A., Lucker, P. L., and Weimer, C.: CALIPSO Lidar Description and Performance Assessment, J. Atmos. Ocean. Technol., 26, 1214–1228, https://doi.org/10.1175/2009JTECHA1223.1, 2009.
Husain, Tahir: Kuwaiti Oil Fires, Elsevier, https://doi.org/10.1016/B978-0-08-042418-7.X5000-2, 1995.
Hu, Y., Winker, D., Vaughan, M., Lin, B., Omar, A., Trepte, C., Flittner, D., Yang, P., Nasiri, S. L., Baum, B., Holz, R., Sun, W., Liu, Z., Wang, Z., Young, S., Stamnes, K., Huang, J., and Kuehn, R.: CALIPSO/CALIOP Cloud Phase Discrimination Algorithm, J. Atmos. Ocean. Technol., 26, 2293–2309, https://doi.org/10.1175/2009JTECHA1280.1, 2009.
Ichoku, C., Kahn, R., and Chin, M.: Satellite contributions to the quantitative characterization of biomass burning for climate modeling, Atmos. Res., 111, 1–28, https://doi.org/10.1016/j.atmosres.2012.03.007, 2012.
Illingworth, A. J., Barker, H. W., Beljaars, A., Ceccaldi, M., Chepfer, H., Clerbaux, N., Cole, J., Delanoë, J., Domenech, C., Donovan, D. P., Fukuda, S., Hirakata, M., Hogan, R. J., Huenerbein, A., Kollias, P., Kubota, T., Nakajima, T., Nakajima, T. Y., Nishizawa, T., Ohno, Y., Okamoto, H., Oki, R., Sato, K., Satoh, M., Shephard, M. W., Velázquez-Blázquez, A., Wandinger, U., Wehr, T., and van Zadelhoff, G.-J.: The EarthCARE Satellite: The Next Step Forward in Global Measurements of Clouds, Aerosols, Precipitation, and Radiation, B. Am. Meteorol. Soc., 96, 1311–1332, https://doi.org/10.1175/BAMS-D-12-00227.1, 2015.
Jethva, H., Torres, O., Waquet, F., Chand, D., and Hu, Y.: How do A-train sensors intercompare in the retrieval of above-cloud aerosol optical depth? A case study-based assessment: A-TRAIN ABOVE-CLOUD AEROSOL OPTICAL DEPTH, Geophys. Res. Lett., 41, 186–192, https://doi.org/10.1002/2013GL058405, 2014.
Johnson, D. W., Kilsby, C. G., McKenna, D. S., Saunders, R. W., Jenkins, G. J., Smith, F. B., and Foot, J. S.: Airborne observations of the physical and chemical characteristics of the Kuwait oil smoke plume, Nature, 353, 617–621, https://doi.org/10.1038/353617a0, 1991.
Kahn, R., Limbacher, J., Flower, V., and Val Martin, M.: Learning About Earth from Space-Based, Multi-Angle Imaging, in: UMBC Earth Day Symposium, Baltimore, MD, https://ntrs.nasa.gov/api/citations/20190025311/downloads/20190025311.pdf, (last access: 05 January 2022), 2019.
Kallos, G., Astitha, M., Katsafados, P., and Spyrou, C.: Long-Range Transport of Anthropogenically and Naturally Produced Particulate Matter in the Mediterranean and North Atlantic: Current State of Knowledge, J. Appl. Meteorol. Clim., 46, 1230–1251, https://doi.org/10.1175/JAM2530.1, 2007.
Kanngießer, F. and Kahnert, M.: Calculation of optical properties of light-absorbing carbon with weakly absorbing coating: A model with tunable transition from film-coating to spherical-shell coating, J. Quant. Spectrosc. Ra., 216, 17–36, https://doi.org/10.1016/j.jqsrt.2018.05.014, 2018.
Kar, J., Vaughan, M. A., Lee, K.-P., Tackett, J. L., Avery, M. A., Garnier, A., Getzewich, B. J., Hunt, W. H., Josset, D., Liu, Z., Lucker, P. L., Magill, B., Omar, A. H., Pelon, J., Rogers, R. R., Toth, T. D., Trepte, C. R., Vernier, J.-P., Winker, D. M., and Young, S. A.: CALIPSO lidar calibration at 532 nm: version 4 nighttime algorithm, Atmos. Meas. Tech., 11, 1459–1479, https://doi.org/10.5194/amt-11-1459-2018, 2018.
Kaufman, Y. J., Tanré, D., Remer, L. A., Vermote, E. F., Chu, A., and Holben, B. N.: Operational remote sensing of tropospheric aerosol over land from EOS moderate resolution imaging spectroradiometer, J. Geophys. Res., 102, 17051–17067, https://doi.org/10.1029/96JD03988, 1997.
Kelsall, H. L.: Respiratory health status of Australian veterans of the 1991 Gulf War and the effects of exposure to oil fire smoke and dust storms, Thorax, 59, 897–903, https://doi.org/10.1136/thx.2003.017103, 2004.
Khan, A. and Zhaoying, H.: Conflict escalation in the Middle East revisited: thinking through interstate rivalries and state-sponsored terrorism, Isr. Aff., 26, 242–256, https://doi.org/10.1080/13537121.2020.1720115, 2020.
Kim, M.-H., Omar, A. H., Tackett, J. L., Vaughan, M. A., Winker, D. M., Trepte, C. R., Hu, Y., Liu, Z., Poole, L. R., Pitts, M. C., Kar, J., and Magill, B. E.: The CALIPSO version 4 automated aerosol classification and lidar ratio selection algorithm, Atmos. Meas. Tech., 11, 6107–6135, https://doi.org/10.5194/amt-11-6107-2018, 2018.
King, M. D.: Directional and spectral reflectance of the Kuwait oil-fire smoke, J. Geophys. Res. Atmos., 97, 14545–14549, 1992.
Kokhanovsky, A. (Ed.): Springer Series in Light Scattering: Volume 4: Light Scattering and Radiative Transfer, Springer International Publishing, Cham, https://doi.org/10.1007/978-3-030-20587-4, 2019.
Konovalov, I. B., Lvova, D. A., Beekmann, M., Jethva, H., Mikhailov, E. F., Paris, J.-D., Belan, B. D., Kozlov, V. S., Ciais, P., and Andreae, M. O.: Estimation of black carbon emissions from Siberian fires using satellite observations of absorption and extinction optical depths, Atmos. Chem. Phys., 18, 14889–14924, https://doi.org/10.5194/acp-18-14889-2018, 2018.
Kovalets, I. V., Maistrenko, S. Yà, Khalchenkov, O. V., Zagreba, T. O., Khurtsilava, K. V., Anulich, S. M., Bespalov, V. P., and Udovenko, O. I.: “Povitrya” Web-Based Software System for Operational Forecasting Atmospheric Pollution after Manmade Accidents in Ukraine, Nauka Innov., 13, 13–24, https://doi.org/10.15407/scin13.06.013, 2017.
Krausmann, E. and Cruz, A. M.: Impact of the 11 March 2011, Great East Japan earthquake and tsunami on the chemical industry, Nat. Hazards, 67, 811–828, https://doi.org/10.1007/s11069-013-0607-0, 2013.
Lange, J. L., Schwartz, D. A., Doebbeling, B. N., Heller, J. M., and Thorne, P. S.: Exposures to the Kuwait oil fires and their association with asthma and bronchitis among gulf war veterans, Environ. Health Persp., 110, 1141–1146, 2002.
Laumbach, R. J. and Kipen, H. M.: Respiratory health effects of air pollution: Update on biomass smoke and traffic pollution, J. Aller. Cl. Immun., 129, 3–11, https://doi.org/10.1016/j.jaci.2011.11.021, 2012.
Laursen, K. K., Ferek, R. J., Hobbs, P. V., and Rasmussen, R. A.: Emission factors for particles, elemental carbon, and trace gases from the Kuwait oil fires, J. Geophys. Res., 97, 14491, https://doi.org/10.1029/92JD01370, 1992.
Leahy, L. V., Anderson, T. L., Eck, T. F., and Bergstrom, R. W.: A synthesis of single scattering albedo of biomass burning aerosol over southern Africa during SAFARI 2000, Geophys. Res. Lett., 34, L12814, https://doi.org/10.1029/2007GL029697, 2007.
Lee, B.-J., Kim, B., and Lee, K.: Air Pollution Exposure and Cardiovascular Disease, Toxicological Research, 30, 71–75, https://doi.org/10.5487/TR.2014.30.2.071, 2014.
Lequy, É., Conil, S., and Turpault, M.-P.: Impacts of Aeolian dust deposition on European forest sustainability: A review, Forest Ecology and Management, For. Ecol. Manag., 267, 240–252, https://doi.org/10.1016/j.foreco.2011.12.005, 2012.
Levy, R. C., Remer, L. A., and Dubovik, O.: Global aerosol optical properties and application to Moderate Resolution Imaging Spectroradiometer aerosol retrieval over land, J. Geophys. Res., 112, 2006JD007815, https://doi.org/10.1029/2006JD007815, 2007a.
Levy, R. C., Remer, L. A., Mattoo, S., Vermote, E. F., and Kaufman, Y. J.: Second-generation operational algorithm: Retrieval of aerosol properties over land from inversion of Moderate Resolution Imaging Spectroradiometer spectral reflectance, J. Geophys. Res., 112, 2006JD007811, https://doi.org/10.1029/2006JD007811, 2007b.
Levy, R. C., Remer, L. A., Mattoo, S., Vermote, E. F., and Kaufman, Y. J.: Second-generation operational algorithm: Retrieval of aerosol properties over land from inversion of Moderate Resolution Imaging Spectroradiometer spectral reflectance, J. Geophys. Res.-Atmos., 112, D13211, https://doi.org/10.1029/2006JD007811, 2007c.
Levy, R. C., Mattoo, S., Munchak, L. A., Remer, L. A., Sayer, A. M., Patadia, F., and Hsu, N. C.: The Collection 6 MODIS aerosol products over land and ocean, Atmos. Meas. Tech., 6, 2989–3034, https://doi.org/10.5194/amt-6-2989-2013, 2013.
Li, J., Li, X., Carlson, B. E., Kahn, R. A., Lacis, A. A., Dubovik, O., and Nakajima, T.: Reducing multisensor satellite monthly mean aerosol optical depth uncertainty: 1. Objective assessment of current AERONET locations, J. Geophys. Res. Atmos., 121, 13609–13627, 2016.
Li, J., Kahn, R. A., Wei, J., Carlson, B. E., Lacis, A. A., Li, Z., Li, X., Dubovik, O., and Nakajima, T.: Synergy of Satellite- and Ground-Based Aerosol Optical Depth Measurements Using an Ensemble Kalman Filter Approach, J. Geophys. Res.-Atmos., 125, 1–17, https://doi.org/10.1029/2019JD031884, 2020.
Limaye, S. S., Ackerman, S. A., Fry, P. M., Isa, M., Ali, H., Ali, G., Wright, A., and Rangno, A.: Satellite monitoring of smoke from the Kuwait oil fires, J. Geophys. Res. Atmos., 97, 14551–14563, 1992.
Lim, S. S., Vos, T., Flaxman, A. D., et al.: A comparative risk assessment of burden of disease and injury attributable to 67 risk factors and risk factor clusters in 21 regions, 1990–2010: a systematic analysis for the Global Burden of Disease Study 2010, The Lancet, 380, 2224–2260, https://doi.org/10.1016/S0140-6736(12)61766-8, 2012.
Li, S., Kahn, R., Chin, M., Garay, M. J., and Liu, Y.: Improving satellite-retrieved aerosol microphysical properties using GOCART data, Atmos. Meas. Tech., 8, 1157–1171, https://doi.org/10.5194/amt-8-1157-2015, 2015.
Liu, Z., Omar, A. H., Hu, Y., Vaughan, M. A., and Winker, D. M.: CALIOP algorithm theoretical basis document – Part 3: Scene classification algorithms, Release 1.0, PC-SCI-202 [preprint], http://www-calipso.larc.nasa.gov/resources/pdfs/PC-SCI-202_Part3_v1.0.pdf, (last access: 5 January 2022), 2005.
Liu, Z., Vaughan, M., Winker, D., Kittaka, C., Getzewich, B., Kuehn, R., Omar, A., Powell, K., Trepte, C., and Hostetler, C.: The CALIPSO Lidar Cloud and Aerosol Discrimination: Version 2 Algorithm and Initial Assessment of Performance, J. Atmos. Ocean. Tech., 26, 1198–1213, https://doi.org/10.1175/2009JTECHA1229.1, 2009.
Li, Y., Yu, H., Wang, Z., Li, Y., Pan, Q., Meng, S., Yang, Y., Lu, W., and Guo, K.: The forecasting and analysis of oil spill drift trajectory during the Sanchi collision accident, East China Sea, Ocean Eng., 187, 106231, https://doi.org/10.1016/j.oceaneng.2019.106231, 2019.
Maher, B. A., Prospero, J. M., Mackie, D., Gaiero, D., Hesse, P. P., and Balkanski, Y.: Global connections between aeolian dust, climate and ocean biogeochemistry at the present day and at the last glacial maximum, Earth-Sci. Rev., 99, 61–97, https://doi.org/10.1016/j.earscirev.2009.12.001, 2010.
Mather, T. A., Harrison, R. G., Tsanev, V. I., Pyle, D. M., Karumudi, M. L., Bennett, A. J., Sawyer, G. M., and Highwood, E. J.: Observations of the plume generated by the December 2005 oil depot explosions and prolonged fire at Buncefield (Hertfordshire, UK) and associated atmospheric changes, Proc. R. Soc. A., 463, 1153–1177, https://doi.org/10.1098/rspa.2006.1810, 2007.
McTainsh, G. and Strong, C.: The role of aeolian dust in ecosystems, Geomorphology, 89, 39–54, 2007.
Mikhailov, E. F., Vlasenko, S. S., Podgorny, I. A., Ramanathan, V., and Corrigan, C. E.: Optical properties of soot–water drop agglomerates: An experimental study, J. Geophys. Res., 111, D07209, https://doi.org/10.1029/2005JD006389, 2006.
MODIS Atmosphere Science Team: MODIS/Terra Aerosol 5-Min L2 Swath 10 km, Earth data [data set], https://doi.org/10.5067/MODIS/MOD04_L2.061, 2017a.
MODIS Atmosphere Science Team: MYD04_L2 MODIS/Aqua Aerosol 5-Min L2 Swath 10 km, Earth data [data set], https://doi.org/10.5067/MODIS/MYD04_L2.061, 2017b.
Morgan, O., Verlander, N. Q., Kennedy, F., Moore, M., Birch, S., Kearney, J., Lewthwaite, P., Lewis, R., O'Brian, S., Osman, J., and Reacher, M.: Exposures and reported symptoms associated with occupational deployment to the Buncefield fuel depot fire, England 2005, Occup. Environ. Med., 65, 404–411, https://doi.org/10.1136/oem.2007.035303, 2008.
Nakajima, T., Hayasaka, T., Higurashi, A., Hashida, G., Moharram-Nejad, N., Najafi, Y., and Valavi, H.: Aerosol optical properties in the Iranian region obtained by ground-based solar radiation measurements in the summer of 1991, J. Appl. Meteorol., 35, 1265–1278, 1996.
Necci, A., Tarantola, S., Vamanu, B., Krausmann, E., and Ponte, L.: Lessons learned from offshore oil and gas incidents in the Arctic and other ice-prone seas, Ocean Eng., 185, 12–26, https://doi.org/10.1016/j.oceaneng.2019.05.021, 2019.
New York Times: Two Major Saudi Oil Installations Hit by Drone Strike, and U.S. Blames Iran, https://www.nytimes.com/2019/09/14/world/middleeast/saudi-arabia-refineries-drone-attack.html, last access: 12 November 2019.
Noyes, K. J., Kahn, R., Sedlacek, A., Kleinman, L., Limbacher, J., and Li, Z.: Wildfire Smoke Particle Properties and Evolution, from Space-Based Multi-Angle Imaging, Remote Sensing, 12, 769, https://doi.org/10.3390/rs12050769, 2020.
Okada, K., Ikegami, M., Uchino, O., Nikaidou, Y., Zaizen, Y., Tsutsumi, Y., and Makino, Y.: Extremely high proportions of soot particles in the upper troposphere over Japan, Geophys. Res. Lett., 19, 921–924, https://doi.org/10.1029/92GL00487, 1992.
Omar, A. H., Winker, D. M., Vaughan, M. A., Hu, Y., Trepte, C. R., Ferrare, R. A., Lee, K.-P., Hostetler, C. A., Kittaka, C., Rogers, R. R., Kuehn, R. E., and Liu, Z.: The CALIPSO Automated Aerosol Classification and Lidar Ratio Selection Algorithm, J. Atmos. Ocean. Tech., 26, 1994–2014, https://doi.org/10.1175/2009JTECHA1231.1, 2009.
Pascal, M., Corso, M., Chanel, O., Declercq, C., Badaloni, C., Cesaroni, G., Henschel, S., Meister, K., Haluza, D., Martin-Olmedo, P., and Medina, S.: Assessing the public health impacts of urban air pollution in 25 European cities: Results of the Aphekom project, Sci. Total Environ., 449, 390–400, https://doi.org/10.1016/j.scitotenv.2013.01.077, 2013.
Peng, J., Hu, M., Guo, S., Du, Z., Zheng, J., Shang, D., Levy Zamora, M., Zeng, L., Shao, M., Wu, Y.-S., Zheng, J., Wang, Y., Glen, C. R., Collins, D. R., Molina, M. J., and Zhang, R.: Markedly enhanced absorption and direct radiative forcing of black carbon under polluted urban environments, P. Natl. Acad. Sci. USA, 113, 4266–4271, https://doi.org/10.1073/pnas.1602310113, 2016.
Piafom: Ras Lanuf Oil Complex – Ras Lanuf, Libya 2008, https://www.pifoam.ch/incidents (last access: 12 September 2019), 2018.
Pilewskie, P. and Valero, F. P. J.: Radiative effects of the smoke clouds from the Kuwait oil fires, J. Geophys. Res., 97, 14541, https://doi.org/10.1029/92JD01371, 1992.
Pokhrel, R. P., Wagner, N. L., Langridge, J. M., Lack, D. A., Jayarathne, T., Stone, E. A., Stockwell, C. E., Yokelson, R. J., and Murphy, S. M.: Parameterization of single-scattering albedo (SSA) and absorption Ångström exponent (AAE) with for aerosol emissions from biomass burning, Atmos. Chem. Phys., 16, 9549–9561, https://doi.org/10.5194/acp-16-9549-2016, 2016.
Pope III, C. A., Burnett, R. T., Thun, M., J., Calle, E., E., Krewski, D., Ito, K., and Thurston, G. D.: Lung Cancer, Cardiopulmonary Mortality, and Long-term Exposure to Fine Particulate Air Pollution, JAMA, 287, 1132, https://doi.org/10.1001/jama.287.9.1132, 2002.
Popp, T., De Leeuw, G., Bingen, C., Brühl, C., Capelle, V., Chedin, A., Clarisse, L., Dubovik, O., Grainger, R., and Griesfeller, J.: Development, production and evaluation of aerosol climate data records from European satellite observations (Aerosol_cci), Remote Sensing, 8, 421, https://doi.org/10.3390/rs8050421, 2016.
Powell, K. A., Hostetler, C. A., Vaughan, M. A., Lee, K.-P., Trepte, C. R., Rogers, R. R., Winker, D. M., Liu, Z., Kuehn, R. E., and Hunt, W. H.: CALIPSO lidar calibration algorithms. Part I: Nighttime 532-nm parallel channel and 532 nm perpendicular channel, J. Atmos. Ocean. Tech., 26, 2015–2033, 2009.
Qiao, F., Wang, G., Yin, L., Zeng, K., Zhang, Y., Zhang, M., Xiao, B., Jiang, S., Chen, H., and Chen, G.: Modelling oil trajectories and potentially contaminated areas from the Sanchi oil spill, Sci. Total Environ., 685, 856–866, https://doi.org/10.1016/j.scitotenv.2019.06.255, 2019.
Radke, L. F., Hegg, D. A., Hobbs, P. V., Nance, J. D., Lyons, J. H., Laursen, K. K., Weiss, R. E., Riggan, P. J., and Ward, D. E.: Particulate and trace gas emissions from large biomass fire in North America, in: Global Biomass Burning: Atmospheric, Climatic, and Biospheric Implications, edited by: Levine, J. S, The MIT Press, Cambridge, Massachusetts, 209–216, https://doi.org/10.7551/mitpress/3286.003.0032 1991.
Ramanathan, V. and Carmichael, G.: Global and regional climate changes due to black carbon, Nat. Geosci., 1, 221–227, https://doi.org/10.1038/ngeo156, 2008.
Remer, L. A., Kaufman, Y. J., Tanré, D., Mattoo, S., Chu, D. A., Martins, J. V., Li, R.-R., Ichoku, C., Levy, R. C., Kleidman, R. G., Eck, T. F., Vermote, E., and Holben, B. N.: The MODIS Aerosol Algorithm, Products, and Validation, J. Atmos. Sci., 62, 947–973, https://doi.org/10.1175/JAS3385.1, 2005.
Remer, L. A., Kleidman, R. G., Levy, R. C., Kaufman, Y. J., Tanré, D., Mattoo, S., Martins, J. V., Ichoku, C., Koren, I., Yu, H., and Holben, B. N.: Global aerosol climatology from the MODIS satellite sensors, J. Geophys. Res., 113, D14S07, https://doi.org/10.1029/2007JD009661, 2008.
Remer, L. A., Mattoo, S., Levy, R. C., and Munchak, L. A.: MODIS 3 km aerosol product: algorithm and global perspective, Atmos. Meas. Tech., 6, 1829–1844, https://doi.org/10.5194/amt-6-1829-2013, 2013.
Reuters: Fire at Libyan oil port destroys up to 1.8 million barrels of crude, https://www.reuters.com/article/us-libya-security-oil/fire-at-libyan-oil-port-destroys-up-to-1-8-million-barrels-of-crude-idUSKBN0K810S20141230, (last access: 12 September 2019), 2014.
Reuters: Fuel depot blaze in Ukraine kills five, https://www.reuters.com/article/us-ukraine-crisis-fire-idUSKBN0OP0HB20150609, (last access: 22 May 2019), 2015.
Reuters: Storage tank at Libya's Ras Lanuf oil port has collapsed: oil workers, https://www.reuters.com/article/us-libya-oil-security-tank/storage-tank-at-libyas-ras-lanuf-oil-port-has-collapsed-oil-workers-idUSKBN1JF1XS, (last access: 12 September 2019), 2018.
Reuters: Costly Saudi defenses prove no match for drones, cruise missiles, https://www.reuters.com/article/us-saudi-aramco-security/costly-saudi-defenses-prove-no-match-for-drones-cruise-missiles-idUSKBN1W22FR, (last access: 12 November 2019), 2019.
Riemer, N., West, M., Zaveri, R., and Easter, R.: Estimating black carbon aging time-scales with a particle-resolved aerosol model, J. Aerosol Sci., 41, 143–158, https://doi.org/10.1016/j.jaerosci.2009.08.009, 2010.
Ross, J. L., Waggoner, A. P., Hobbs, P. V., and Ferek, R. J.: Airborne lidar measurements of a smoke plume produced by a controlled burn of crude oil on the ocean, J. Air Waste Manag. Assoc., 46, 327–334, 1996.
Sadiq, M. and McCain, J. C.: The Gulf War Aftermath: an Environmental Tragedy, Springer Netherlands, Imprint, Springer, Dordrecht, https://doi.org/10.1007/978-94-011-1685-5, 1993.
Samset, B. H., Stjern, C. W., Andrews, E., Kahn, R. A., Myhre, G., Schulz, M., and Schuster, G. L.: Aerosol Absorption: Progress Towards Global and Regional Constraints, Curr. Clim. Change Rep., 4, 65–83, https://doi.org/10.1007/s40641-018-0091-4, 2018.
Sayer, A. M., Govaerts, Y., Kolmonen, P., Lipponen, A., Luffarelli, M., Mielonen, T., Patadia, F., Popp, T., Povey, A. C., Stebel, K., and Witek, M. L.: A review and framework for the evaluation of pixel-level uncertainty estimates in satellite aerosol remote sensing, Atmos. Meas. Tech., 13, 373–404, https://doi.org/10.5194/amt-13-373-2020, 2020.
Schmidt, S., Mishra, B. K., and Wehrstedt, K.-D.: Cfd based reproduction of amuay refinery accident 2012, Chem. Eng. Trans., 48, 7–12, https://doi.org/10.3303/CET1648002, 2016.
Schutgens, N., Sayer, A. M., Heckel, A., Hsu, C., Jethva, H., de Leeuw, G., Leonard, P. J. T., Levy, R. C., Lipponen, A., Lyapustin, A., North, P., Popp, T., Poulsen, C., Sawyer, V., Sogacheva, L., Thomas, G., Torres, O., Wang, Y., Kinne, S., Schulz, M., and Stier, P.: An AeroCom–AeroSat study: intercomparison of satellite AOD datasets for aerosol model evaluation, Atmos. Chem. Phys., 20, 12431–12457, https://doi.org/10.5194/acp-20-12431-2020, 2020.
Smith, T. C.: Are Gulf War Veterans Experiencing Illness due to Exposure to Smoke from Kuwaiti Oil Well Fires? Examination of Department of Defense Hospitalization Data, Am. J. Epidemiol., 155, 908–917, https://doi.org/10.1093/aje/155.10.908, 2002.
Sogacheva, L., Popp, T., Sayer, A. M., Dubovik, O., Garay, M. J., Heckel, A., Hsu, N. C., Jethva, H., Kahn, R. A., Kolmonen, P., Kosmale, M., de Leeuw, G., Levy, R. C., Litvinov, P., Lyapustin, A., North, P., Torres, O., and Arola, A.: Merging regional and global aerosol optical depth records from major available satellite products, Atmos. Chem. Phys., 20, 2031–2056, https://doi.org/10.5194/acp-20-2031-2020, 2020.
Soulen, P. F., King, M. D., Tsay, S.-C., Arnold, G. T., and Li, J. Y.: Airborne spectral measurements of surface-atmosphere anisotropy during the SCAR-A, Kuwait oil fire, and TARFOX experiments, J. Geophys. Res., 105, 10203–10218, https://doi.org/10.1029/1999JD901115, 2000.
Stevens, R., Pinto, J., Mamane, Y., Ondov, J., Abdulraheem, M., Al-Majed, N., Sadek, M., Cofer, W., Ellenson, W., and Kellogg, R.: Chemical and Physical Properties of Emissions from Kuwaiti Oil Fires, Water Sci. Technol., 27, 223–233, https://doi.org/10.2166/wst.1993.0555, 1993.
Stocker, T., Qin, D., Plattner, G.-K., Tignor, M., Allen, S. K., Boschung, J., Nauels, A., Xia, Y., Bex, V., and Midgley, P. M. (Eds.): Climate change 2013: the physical science basis: Working Group I contribution to the Fifth assessment report of the Intergovernmental Panel on Climate Change, Cambridge university press, Cambridge, United Kingdom, New York, NY, USA, 1535 pp., ISBN: 1-107-05799-X, 2014.
Tackett, J. L., Winker, D. M., Getzewich, B. J., Vaughan, M. A., Young, S. A., and Kar, J.: CALIPSO lidar level 3 aerosol profile product: version 3 algorithm design, Atmos. Meas. Tech., 11, 4129–4152, https://doi.org/10.5194/amt-11-4129-2018, 2018.
Tanré, D., Kaufman, Y. J., Herman, M., and Mattoo, S.: Remote sensing of aerosol properties over oceans using the MODIS/EOS spectral radiances, J. Geophys. Res.-Atmos., 102, 16971–16988, 1997.
Targa, J., Kent, A., Stewart, R., Coleman, P., Bower, J., Webster, H., Taylor, J., Murray, V., Mohan, R., and Aus, C.: Initial review of Air Quality aspects of the Buncefield Oil Depot Explosion, ED 48692, https://uk-air.defra.gov.uk/assets/documents/reports/cat05/0606201126_Buncefield_report_vF3_text2.pdf (last access: 5 January 2022), 2006.
The Christian Science Monitor: Qaddafi bombs oil facility in blow to Libya's oil infrastructure, https://www.csmonitor.com/World/Middle-East/2011/0309/Qaddafi-bombs-oil-facility-in-blow-to-Libya-s-oil-infrastructure, (last access: 12 September 2019), 2011.
The Guardian: Gaddafi troops pound Libya rebels out of Ras Lanuf, https://www.theguardian.com/world/2011/mar/10/ras-lanuf-rebel-retreat-libya, (last access: 12 September 2019), 2011.
The Indian Express: Butcher Island fire put out after four days, https://indianexpress.com/article/cities/mumbai/butcher-island-fire-put-out-after-four-days-4884375/, (last acess: 6 July 2019), 2017.
The Telegraph: Environmental disaster averted: how Libya (mis)handled recent oil tank blaze, https://www.telegraph.co.uk/news/wikileaks-files/libya-wikileaks/8294856/ENVIRONMENTAL-DISASTER-AVERTED-HOW-LIBYA-MISHANDLED-RECENT-OIL-TANK-BLAZE.html, (last access: 12 September 2019), 2011.
Tichý, L.: The Islamic State oil and gas strategy in North Africa, Energy Strateg. Rev., 24, 254–260, 2019.
Tichý, L. and Eichler, J.: Terrorist Attacks on the Energy Sector: The Case of Al Qaeda and the Islamic State, Stud. Confl. Terror., 41, 450–473, https://doi.org/10.1080/1057610X.2017.1323469, 2018.
Vasanth, S., Tauseef, S. M., Abbasi, T., and Abbasi, S. A.: Multiple pool fires: Occurrence, simulation, modeling and management, J. Loss Prevent. Proc., 29, 103–121, https://doi.org/10.1016/j.jlp.2014.01.005, 2014.
Vaughan, M. A., Powell, K. A., Winker, D. M., Hostetler, C. A., Kuehn, R. E., Hunt, W. H., Getzewich, B. J., Young, S. A., Liu, Z., and McGill, M. J.: Fully Automated Detection of Cloud and Aerosol Layers in the CALIPSO Lidar Measurements, J. Atmos. Ocean. Tech., 26, 2034–2050, https://doi.org/10.1175/2009JTECHA1228.1, 2009.
Vaughan, M., Garnier, A., Josset, D., Avery, M., Lee, K.-P., Liu, Z., Hunt, W., Pelon, J., Hu, Y., Burton, S., Hair, J., Tackett, J. L., Getzewich, B., Kar, J., and Rodier, S.: CALIPSO lidar calibration at 1064 nm: version 4 algorithm, Atmos. Meas. Tech., 12, 51–82, https://doi.org/10.5194/amt-12-51-2019, 2019.
Vautard, R., Ciais, P., Fisher, R., Lowry, D., Bréon, F. M., Vogel, F., Levin, I., Miglietta, F., and Nisbet, E.: The dispersion of the Buncefield oil fire plume: An extreme accident without air quality consequences, Atmos. Environ., 41, 9506–9517, https://doi.org/10.1016/j.atmosenv.2007.08.055, 2007.
Virtanen, T. H., Kolmonen, P., Sogacheva, L., Rodríguez, E., Saponaro, G., and de Leeuw, G.: Collocation mismatch uncertainties in satellite aerosol retrieval validation, Atmos. Meas. Tech., 11, 925–938, https://doi.org/10.5194/amt-11-925-2018, 2018.
Wang, Y., Jiang, J. H., and Su, H.: Atmospheric responses to the redistribution of anthropogenic aerosols, J. Geophys. Res.-Atmos., 120, 9625–9641, https://doi.org/10.1002/2015JD023665, 2015.
Wei, X., Chang, N.-B., Bai, K., and Gao, W.: Satellite remote sensing of aerosol optical depth: advances, challenges, and perspectives, Crit. Rev. Env. Sci. Tec., 50, 1640–1725, https://doi.org/10.1080/10643389.2019.1665944, 2020.
Winker, D.: CALIPSO LID L1 Standard HDF File – Version 4.10, Earth data [data set], https://doi.org/10.5067/CALIOP/CALIPSO/LID_L1-STANDARD-V4-10, 2016.
Winker, D.: CALIPSO Lidar Level 2 5 km Aerosol Layer Data V4-20, Earth data [data set], https://doi.org/10.5067/CALIOP/CALIPSO/LID_L2_05KMALAY-STANDARD-V4-20, 2018a.
Winker, D.: CALIPSO Lidar Level 2 Aerosol Profile Data V4-20, Earth data [data set], https://doi.org/10.5067/CALIOP/CALIPSO/LID_L2_05KMAPRO-STANDARD-V4-20, 2018b.
Winker, D.: CALIPSO Lidar Level 2 Vertical Feature Mask Data V4-20, Earth data [data set], https://doi.org/10.5067/CALIOP/CALIPSO/LID_L2_VFM-STANDARD-V4-20, 2018c.
Winker, D. M., Vaughan, M. A., Omar, A., Hu, Y., Powell, K. A., Liu, Z., Hunt, W. H., and Young, S. A.: Overview of the CALIPSO Mission and CALIOP Data Processing Algorithms, J. Atmos. Ocean. Tech., 26, 2310–2323, https://doi.org/10.1175/2009JTECHA1281.1, 2009.
World Meteorological Organization: Report of the second WMO meeting of experts to assess the response to the atmospheric effects of the Kuwait oil fires, WMO/TD No. 512, 1993.
Young, S. A. and Vaughan, M. A.: The Retrieval of Profiles of Particulate Extinction from Cloud-Aerosol Lidar Infrared Pathfinder Satellite Observations (CALIPSO) Data: Algorithm Description, J. Atmos. Ocean. Tech., 26, 1105–1119, https://doi.org/10.1175/2008JTECHA1221.1, 2009.
Young, S. A., Vaughan, M. A., Kuehn, R. E., and Winker, D. M.: The Retrieval of Profiles of Particulate Extinction from Cloud–Aerosol Lidar and Infrared Pathfinder Satellite Observations (CALIPSO) Data: Uncertainty and Error Sensitivity Analyses, J. Atmos. Ocean. Tech., 30, 395–428, https://doi.org/10.1175/JTECH-D-12-00046.1, 2013.
Young, S. A., Vaughan, M. A., Garnier, A., Tackett, J. L., Lambeth, J. D., and Powell, K. A.: Extinction and optical depth retrievals for CALIPSO's Version 4 data release, Atmos. Meas. Tech., 11, 5701–5727, https://doi.org/10.5194/amt-11-5701-2018, 2018.
Zhang, K. and Batterman, S.: Air pollution and health risks due to vehicle traffic, Sci. Total Environ., 450, 307–316, 2013.
Zhang, Q., Jiang, X., Tong, D., Davis, S. J., Zhao, H., Geng, G., Feng, T., Zheng, B., Lu, Z., Streets, D. G., Ni, R., Brauer, M., van Donkelaar, A., Martin, R. V., Huo, H., Liu, Z., Pan, D., Kan, H., Yan, Y., Lin, J., He, K., and Guan, D.: Transboundary health impacts of transported global air pollution and international trade, Nature, 543, 705–709, https://doi.org/10.1038/nature21712, 2017.