the Creative Commons Attribution 4.0 License.
the Creative Commons Attribution 4.0 License.
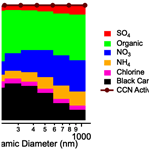
Aerodynamic size-resolved composition and cloud condensation nuclei properties of aerosols in a Beijing suburban region
Chenjie Yu
Kang Hu
Ping Tian
Yangzhou Wu
Delong Zhao
Huihui Wu
Dawei Hu
Wenbo Guo
Qiang Li
Mengyu Huang
Deping Ding
James D. Allan
The size-resolved physiochemical properties of aerosols determine their atmospheric lifetime, cloud interactions and the deposition rate on the human respiratory system; however most atmospheric composition studies tend to evaluate these properties in bulk. This study investigated size-resolved constituents of aerosols on mass and number basis, and their droplet activation properties, by coupling a suite of online measurements with an aerosol aerodynamic classifier (AAC) based on aerodynamic diameter (Da) in Pinggu, a suburb of Beijing. While organic matter accounted for a large fraction of mass, a higher contribution of particulate nitrate at larger sizes (Da>300 nm) was found under polluted cases. By considering the mixing state of refractory-black-carbon-containing particles (rBCc) and composition-dependent densities, aerosols including rBCc were confirmed to be nearly spherical at Da>300 nm. Importantly, the number fraction of rBCc was found to increase with Da at all pollution levels. The number fraction of refractory black carbon (rBC) is found to increase from ∼3 % at ∼90 nm to ∼15 % at ∼1000 nm, and this increasing rBC number fraction may be caused by the coagulation during atmospheric ageing. The droplet activation diameter at a water supersaturation of 0.2 % was 112±6 and 193±41 nm for all particles with Da smaller than 1 µm (PM1) and rBCc respectively. As high as 52±6 % of rBCc and 50±4 % of all PM1 particles in number could be activated under heavy pollution due to enlarged particle size, which could be predicted by applying the volume mixing of substance hygroscopicity within rBCc. As rBCc contribute to the quantity of aerosols at larger particle size, these thickly coated rBCc may contribute to the radiation absorption significantly or act as an important source of cloud condensation nuclei (CCN). This size regime may also exert important health effects due to their higher deposition rate.
- Article
(3333 KB) - Full-text XML
-
Supplement
(750 KB) - BibTeX
- EndNote
Atmospheric aerosols make a significant contribution to a number of atmospheric chemical and physical processes (Riemer et al., 2019). Aerosols from anthropogenic emissions have a negative impact on air quality and human health (West et al., 2016). As a major megacity, the air pollution in Beijing and its surrounding regions has raised much attention in the past years (Shi et al., 2019). The rapid urbanization and the continued increase in vehicle numbers have contributed to a complicated air pollution situation in Beijing (Squires et al., 2020). A number of in situ measurements have characterized the submicron aerosol compositions in urban Beijing (Wang et al., 2020, 2019; Hu et al., 2016). However, few studies have characterized the detailed composition or cloud condensation nuclei (CCN) abilities of particles at Beijing rural sites (Chen et al., 2020a). The relocation of industry from the urban Beijing area has led the surrounding cities around Beijing to be highly industrialized in recent years (Wang et al., 2018), and the rural sites of Beijing are significantly impacted by the air pollutants transported from the surrounding industrial regions in the North China Plain (NCP) (Wu et al., 2011). Furthermore, controls targeting pollution from residential solid fuel use and diesel vehicles do not apply outside of the main metropolitan area of Beijing. The detailed characterization of fine aerosol physiochemical properties in a variety of different environments is essential to understand the evolution of atmospheric particulate matter.
Fine particulate matter can also cause damage to human health via the respiratory system (Xing et al., 2016; Xu et al., 2016). The aerodynamic size of aerosols crucially determines the area of deposition (Sturm, 2010, 2017; Vu et al., 2018); for example, particles with an aerodynamic diameter (Da) below 2.5 µm can reach the alveoli in the lungs and possibly pass into the blood (Lipworth et al., 2014). Through particle-resolving model simulation, Ching and Kajino (2018) found that the deposition efficiency in human alveoli also depended on the mixing state. The toxicity of aerosols is composition-dependent (Kwon et al., 2020) and influenced by the complex morphology (Sturm, 2010; Vu et al., 2018); therefore the aerodynamic size-resolved properties of aerosols are important when understanding their influences on human health.
Atmospheric aerosols also play important roles in the climate through scattering and absorbing solar radiation directly or indirectly through altering cloud properties (Liu et al., 2020; Ravishankara et al., 2015) or causing snowmelt after deposition. Black carbon (BC) is produced from incomplete combustion and is the dominant optically absorbing component in aerosols (Liu et al., 2020; Bond et al., 2013). By mixing with other compounds, the absorption ability of coated BC can be enhanced through the “lensing effect” (Lack and Cappa, 2010). However, detailed simulation and characterization of optical properties of BC remain uncertain since they are influenced by factors such as shape and mixing state (Cappa et al., 2012; Liu et al., 2017; Fierce et al., 2020), which can be modified through atmospheric processing. Thus, better characterization of light absorbing carbonaceous particles is essential.
Coated BC is also known as an important form of CCN, and wet removal is its main atmospheric loss mechanism, so its in-cloud scavenging efficiency and thus lifetime is influenced by its size, mixing state and hygroscopic properties (Taylor et al., 2014), but this is subject to large uncertainties (Myhre and Samset, 2015). Studies have confirmed that the hygroscopicity of refractory-black-carbon-containing particles (rBCc) is largely dictated by the coating material, and rBCc will transform from hydrophobic to hydrophilic after emission by acquiring more non-BC material and increasing in size (Hu et al., 2020a; Wu et al., 2019; Liu et al., 2013). Previous studies (Levin et al., 2014; Broekhuizen et al., 2006; Gunthe et al., 2011; Fan et al., 2020; Wu et al., 2017) have provided both the measured size-resolved CCN ability and aerosol physiochemical properties. However, the CCN ability of rBCc based on atmospheric data remains poorly constrained. Previously, size-resolved composition has been widely investigated using size-segregated offline analysis of cascade impactor samples (Marple et al., 1991). This technique offers great advantages in obtaining detailed information about composition in combination with advanced offline measurements; however it often requires large amounts of material and may not be able to provide sufficient time resolution. Information about particle mixing state and CCN activity is also not available through this technique. The aerosol mass spectrometer (AMS) is also capable of delivering size-resolved aerosol compositions; however the poor accuracy of the AMS in the size range important for CCN (typically 50–100 nm) has hampered quantitative work for the application of CCN concentration derivation based on κ-Köhler theory (Petters and Kreidenweis, 2007).
A previous study (Yu et al., 2020) has characterized the size-resolved mixing state of rBCc in Beijing using a tandem aerosol classifier system. To explore the size-resolved physiochemical properties and CCN ability for bulk aerosol compositions, here we performed a new online measurement method by coupling an aerodynamic aerosol classifier (AAC) with different aerosol measurement techniques including a single-particle soot photometer (SP2) and an aerosol mass spectrometer (AMS). Compared to the previous studies performed with a differential mobility analyser (DMA), the AAC classifies particles without multiple charging artefacts in a wide size range and with better transmission efficiency (Johnson et al., 2018). The simultaneous measurement of size-resolved chemical composition and CCN activation enables a detailed analysis of the hygroscopicity of rBCc and its size-dependent contribution to the CCN activation in a polluted environment. This information will deliver a better understanding of the BC deposition properties for the climate and air pollution impacts on human health studies.
2.1 Experiment location and instruments
The experiment was performed between 5 and 20 January 2020 in the Beijing Weather Modification Office field experiment base located in Pinggu (the red star shown in Fig. 4a), a northeastern suburb of Beijing (Shi et al., 2019). With agriculture dominating its local economy, Pinggu is surrounded by small villages and farmlands (Han et al., 2020). Figure 1 describes the schematic of the instruments used for the size-resolved aerosol measurements. An aerodynamic aerosol classifier (AAC; Cambustion) was placed upstream of the aerosol measurement instruments. The operation and validation of the AAC were described in previous studies (Tavakoli and Olfert, 2013, 2014). Unlike the DMA or centrifugal particle mass analyser (CPMA), the AAC selects particles based on aerodynamic sizes according to particle relaxation time without needing charging for electrostatic or mass sizing. A suite of online measurements was introduced downstream of the AAC, including a high-resolution time-of-flight aerosol mass spectrometer (HR-ToF-AMS; Aerodyne) (Decarlo et al., 2006), which was operated in V mode to characterize the non-refractory aerosol composition, and a single-particle soot photometer (SP2, DMT) (Schwarz et al., 2010) for the measurement of the concentrations of rBCc. The minimum mass-equivalent diameter of a refractory black carbon (rBC) core that can be detected by the SP2 is 70 nm using a rBC material density of 1.8 g cm−3. The volume properties of non-refractory material within rBCc (hence referred to as “coating thickness”) were derived by the SP2 leading-edge-only (LEO) method and is described as the ratio between the optical volume-equivalent diameter of total rBCc and the mass-equivalent diameter of rBC core () (Liu et al., 2019). A previous morphology-independent measurement study validated that the uncertainty of the SP2 LEO fitting method in determining the coating thickness of rBCc is within 20 % (Yu et al., 2020). A cloud condensation nuclei counter (CCNc; DMT) was used to sample the potential CCN activation ability at a constant supersaturation (SS) of 0.2 %, and a condensation particle counter (CPC; TSI model 3776) was used to measure the condensation nuclei (CN) number concentration. The SP2 incandescence signal was calibrated using nebulized Aquadag black carbon particle standards, while the scattering channel was calibrated by 200 nm polystyrene latex spheres before the measurement, and the standard correction factor of 0.75 for ambient rBC measurement was applied (Laborde et al., 2012). The ionization efficiency of the AMS was calibrated using monodisperse ammonium nitrate particles following the standard protocols (Xu et al., 2017), and a constant collection efficiency (CE) of 0.5 was applied (Middlebrook et al., 2012). More details of the calibration and operation of this AMS instrument can be seen in previous publications based on field measurement studies (D. Hu et al., 2021; Liu et al., 2021). The term “all particles” in this study is referred as the PM1 compositions including organic compounds (Org), sulfate (SO4), ammonia (NH4), nitrate (NO3), chloride (Cl) and rBC from AMS and SP2. The AAC was set to classify dry aerosol particles from 90 to 1100 nm in aerodynamic diameter (Da) to cover the detection range of the SP2 and AMS. It took around 15 min to complete one scan using the AAC step scanning mode, and a timed valve was placed upstream of the AAC for switching between monodisperse and polydisperse every 30 min. An example of a running cycle is presented in the Supplement.
2.2 Calculation of size-resolved aerosol morphology parameters
The dynamic shape factor (χ) describes the shape of particles (Decarlo et al., 2004). χ=1 denotes a perfectly spherical particle, and χ>1 means more non-sphericity. Based on the size-resolved measurement here, χ can be calculated by
where ρp is the particle material density, Cc represents the slip correction factor at a given diameter and is calculated following the description in Kim et al. (2005), Dv is the particle volume-equivalent diameter and Da is the aerodynamic diameter classified by the AAC. This calculation is performed for all particles (including rBCc) and rBCc at each size bin, using their respective parameters (ρp and Dv). For all particles, ρp is the mean density weighted by the PM1 results measured by the AMS and SP2. Mineral dust particles are not measured during the experiment because of the instrument upper detection limit. Mineral dust particles are mainly externally mixed with other aerosol compositions and mostly in coarse mode (with Da>1 µm) (Seinfeld and Pandis, 2016), and there was no dust event during the experimental periods. Previous measurements showed that mineral dust particles only accounted for ∼10 % of total PM1 mass concentration in Beijing (Zhang et al., 2018), and most of them were at Da>500 nm (Li et al., 2014). Therefore, our results may slightly underestimate the ρall and total particle mass (Mall) in bins with Da>500 nm if dust is present, although we consider this effect to be minor. To compute the particle volume results based on the AMS-measured ion and Org concentrations, a simplified ion pairing scheme presented in Gysel et al. (2007) was applied, and the solutions are described in the Supplement. The ρp of rBCc is calculated as the weighted density within rBCc including rBC and coatings, where the coating material of rBCc is assumed to constitute the same volume fractions of ambient non-refractory compositions (Liu et al., 2015; D. Hu et al., 2021):
where MrBCc and VrBCc are the mass and volume of the rBCc respectively, and ρNR is the particle density of non-refractory compositions. The rBC core diameter (Dc) and the total diameter of rBCc (Dp,rBCc) are derived through the SP2 LEO method.
For all particles, mean single-particle mass is derived from the total mass (Mall) obtained by AMS and SP2 divided by the total number (Ntotal) obtained by the CPC; hereinafter the mean Dv of particle is assumed to equal to the mass-equivalent diameter (Dm) and is obtained by applying the mean ρp above:
where ρall is the particle density of all aerosol particles. The parameters used for the calculation are also listed in Appendix A.
2.3 Hygroscopicity parameter calculation
The hygroscopicity parameter (κ) (Petters and Kreidenweis, 2007) of measured aerosols is predicted based on the measured aerosol compositions and invoking the Zdanovskii–Stokes–Robinson (ZSR) mixing rule (Stokes and Robinson, 1966). The κ for all particles (κall) is calculated as
where εi and κi are the volume fraction and hygroscopicity parameter of each chemical composition respectively. The κ values based on the AMS-measured concentrations were calculated based on the same simplified ion pairing scheme described above. The detailed information for each parameter used for κ calculation is listed in Table S1 in the Supplement. For rBCc, the κrBCc is calculated by
where εcoating,i and εrBC are the volume fraction coating and rBC respectively; κcoating,i represents the hygroscopicity parameter for each coating composition of rBCc and is assumed to be equal to the κ of ambient non-refractory compositions (Motos et al., 2019b; D. Hu et al., 2021); κrBC represents the hygroscopicity parameter for rBC; and the last term can be ignored since pure rBC is assumed to be hydrophobic. Due to the coating material of rBCc not being included in the calculation process of κall here, κall may be slightly underestimated when rBCc are thickly coated at larger particle size.
2.4 CCN ability of all particles and rBCc
The CCN activation fraction is determined as the ratio between CCN number concentration at SS=0.2 % and the CN number concentration measured by the CPC. The size-resolved CCN activation fraction (AF) is fitted with a sigmoid function:
where E and C are fitting coefficients which represent the asymptote and the slope respectively. Dp is the particle dry diameter, and D50 represents the critical particle diameter where 50 % of particles in number can be activated as CCN (Petters and Kreidenweis, 2007).
The number concentration of rBCc which act as CCN is derived from the concurrent measurements of rBC number concentration, CCN and CN. The method described by D. Hu et al. (2021) has been applied to determine the activation of rBCc. Firstly, the un-activated particle number concentration is derived from the difference between CN and CCN, as the red line in Fig. 2a shows. For particles with Da>300 nm in the example, the un-activated particles are nil; thus all rBCc are also activated. Here particles are considered to be well mixed, and rBCc are less hydrophilic than any other non-refractory particles at the same particle size. Thus, the rBCc are more difficult to be activated as CCN than the other particles. For particles with Da<300 nm, the rBCc are therefore considered to be the first in contributing the un-activated particles, and the activated number concentration of rBC is the number concentration of rBC that is higher than the un-activated particle number concentration. In this way, the size-dependent number concentration of activated rBCc can be obtained (black line in Fig. 2b). D50,rBCc can then be derived through Eq. (6) based on the activation fraction curve of rBCc. The activation fraction of rBCc derived through this method is further referred to as “measured AFrBCc”. There may be some occasions when rBCc could exhibit a higher hygroscopicity, if coated with sufficient hygroscopic substances, even higher than a particle without containing rBC. This means the scenario here may underestimate some fractions of activated rBCc. The method here may therefore serve as a minimum estimation of droplet activation of rBCc from this aspect.
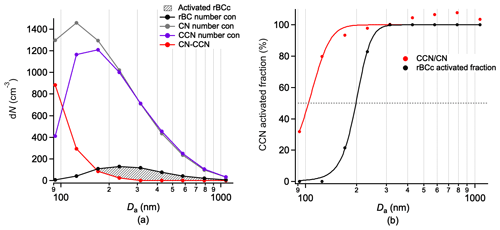
Figure 2An example of all particles and activation of rBCc. The dashed grey line in (b) indicates 50 % of all particles or rBCc activated.
The activation of rBCc is also estimated through the calculated size-resolved critical supersaturation (SSc) (Wu et al., 2019; D. Hu et al., 2021) for comparison, which is derived based on the κrBCc described before from the κ-Köhler theory:
where D is the diameter of the droplet, DrBCc is the dry diameter of rBCc, Mw and ρw are the molecular weight and density of water respectively, T is temperature, R is the ideal gas constant and is the surface tension of the solution–air interface. A decreased SS with increasing Da can be obtained (Fig. 3), so the D50,rBCc at SSc=0.2 % is the cross point above which a diameter of only SS<0.2 % is required to activate the targeting rBCc. The number concentration of activated rBCc is the concentration of rBCc with size larger than D50,rBCc. The activation fraction estimated through this method is further referred to as “modelled AFrBCc”.
2.5 NAME dispersion model
The air mass classification results used to identify potential source regions are generated by the UK Met Office Numerical Atmospheric dispersion Modelling Environment (NAME) dispersion model (Jones et al., 2007). The model presented the 48 h backward dispersion results on a grid using the three-dimensional gridded meteorological field generated from the UK Met Office's Unified Model (Brown et al., 2012). Beijing and its surrounding areas have been classified into five regions as shown in Fig. 4a in order to attribute the air mass histories: Local Beijing (39–41.5∘ N, 115–117∘ E), the North (41.5–45∘ N, 104–121∘ E), the South (32–39∘ N, 115–121∘ E), the West (32–41.5∘ N, 104–115∘ E) and the East region (39–41.5∘ N, 117–121∘ E).
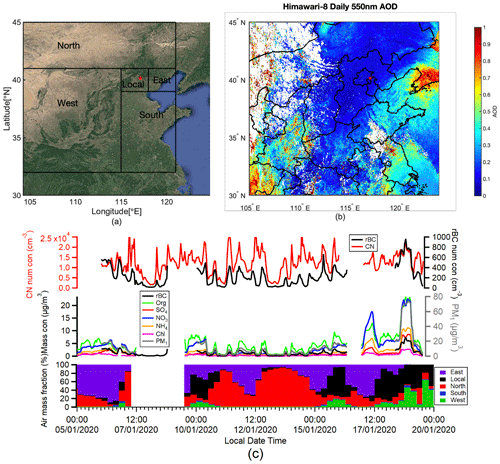
Figure 4Overview of the experiment. (a) Location of the measurement site (marked by red star) and regions classified for air mass (from © Google Maps). (b) The mean aerosol optical depth (AOD) distribution during the experiment period. (c) Aerosol mass and number concentrations and classified air mass types.
3.1 Overview of the whole campaign period
Figure 4c presents the overview of aerosol total number and mass concentrations during the experimental period. Beijing and its suburban regions experience large contrasts in pollution conditions depending on the wind direction (Liu et al., 2019; Chen et al., 2020b). To test whether the aerosol physiochemical properties vary according to ambient pollution concentrations, the pollution is classified into three levels according to the frequency distribution of PM1 concentrations during the whole measurement period: heavy pollution (PM1≥30 µg m−3), moderate pollution () and light pollution (). Combining the air mass history results with the aerosol optical depth (AOD) spatial distribution results from the Himawari-8 Level 2 aerosol product (Bessho et al., 2016; Fukuda et al., 2013) (Fig. 4b), the heavy and moderate pollution periods were mostly attributed to air masses from the East and West regions, respectively. While the contribution from the Local air mass cannot be ignored in some pollution cases, relatively clean northerly air masses were associated with the light pollution periods.
3.2 Size-resolved aerosol mass compositions and mixing state of rBCc
Figure 5a–e present the size-resolved average mass concentrations for PM1, rBC, organic compounds (Org), sulfate (SO4) and nitrate (NO3) under each pollution condition. Though the heavy pollution period has the highest aerosol mass concentrations among three cases, the mass concentrations for total PM1 and non-refractory compositions were close at Da<200 nm under different pollution levels (Fig. 5a). Notable contributions to the total PM1 from non-refractory material were observed for Da>300 nm, especially for the heavy pollution condition in Fig. 5i–k. Unlike the more polluted conditions, the non-refractory aerosol mass concentrations during light pollution periods show size-dependent variation in a much lower absolute value range. Figure 5b shows that the size distribution of rBC mass concentration reached the peak at 400 nm under heavy pollution, while the peak for the moderate and light pollution was at a smaller Da, which was between 300 and 400 nm. Figure 5c–e show that the peak diameter of non-refractory submicron particulate matter (NR-PM1) observed in Pinggu was at around 700 nm and is higher than the peak diameter of NR-PM1 reported at the urban site of Beijing, which is between 400 and 500 nm in winter (Hu et al., 2016). Due to the higher primary organic aerosol (POA) emissions, the results at the Beijing urban site have a higher contribution of Org at smaller size (<500 nm) (Zhang et al., 2014). The Org peak diameter in Pinggu is at around 700 nm, which is close to the peak diameter of secondary inorganic compositions. The average oxygen to carbon ratio ( ratio) for the total Org aerosol in Pinggu is 0.5 and is higher than the ratio in the Beijing urban region in winter, which is 0.32, reported by Hu et al. (2016). This suggests the higher oxidization of Org in the Beijing suburban region than the urban region. The higher peak diameter of secondary inorganic compound also indicates well-mixed aerosol components in the suburban regions (Liu et al., 2016). This size-resolved composition result reported in Pinggu is consistent with the previous measurement in another suburban region in the NCP (Li et al., 2021). Comparing the composition mass fractions under three different pollution cases shown in Fig. 5i–k, one of the remarkable differences is that particulate nitrate accounted for a larger mass fraction during the heavy and moderate pollution periods than during the light pollution period. Previous studies showed that this rapid particulate nitrate formation in Beijing area is mainly associated with the heterogeneous hydrolysis of N2O5 at night (Li et al., 2018). Particulate nitrate has become one of the major secondary inorganic aerosol pollutants in urban environment recently (Zhang et al., 2015), and NO3 also contributed to the aerosol hygroscopicity significantly during the haze pollution periods (Sun et al., 2018). Due to the significant reduction of SOx emissions in China in recent years (Zhang et al., 2012), the mass fractions of SO4 remained low in pollution cases. The Org contributed to the aerosol mass compositions significantly, and the capping of rBC mass fraction was around 25 % among all three cases.
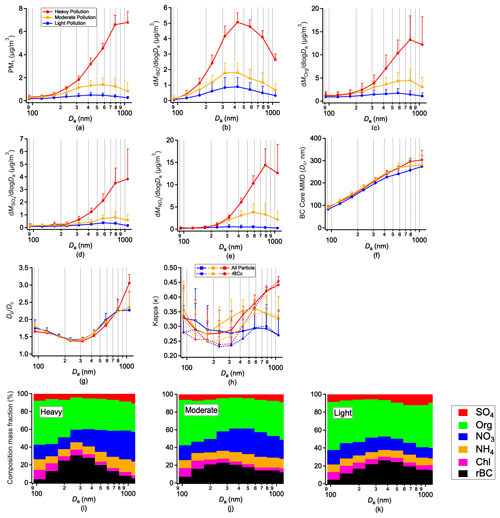
Figure 5Size-resolved (a) PM1 (mean ± standard deviation), (b) rBC mass concentration, (c) Org mass concentration, (d) SO4 mass concentration, (e) NO3 mass concentration, (f) size-resolved rBC core mass median diameter (MMD), (g) size-resolved coating thickness () of rBCc, (h) hygroscopicity parameter (κ) and (i–k) aerosol composition mass fractions under three different pollution levels.
Figure 5f and g present the size distribution of rBC core mass median diameter (MMD) and the coating thickness. This indicates larger Da had selected rBCc with larger rBC core and higher coatings. The MMD of rBC core increased from below 100 nm to around 300 nm with an increase in particle size. The rBC core for the light pollution condition was a little smaller than the other two periods, indicating a possible coagulation process in more polluted cases with higher rBC concentrations. The coating thickness of rBCc decreased slightly when Da increased from 90 to 300 nm. This decreasing trend of rBC coating thickness may be caused by the local traffic emissions. Studies have shown that fresh traffic diesel engines that emitted rBCc importantly contribute to the condensation-mode particles with diameters of 100–300 nm (Seinfeld and Pandis, 2016; Gong et al., 2016). Joshi et al. (2021) demonstrated that traffic emissions dominated the rBC fluxes in Beijing, and previous studies also showed a similar decreasing trend of rBC coatings for engine emissions within this particle size range (Han et al., 2019; Zhang et al., 2020). According to the diffusion-controlled particle growth law, smaller particles diffuse more quickly and hence grow more effectively than the larger particles (Seinfeld and Pandis, 2016). Therefore, smaller rBC acquires more coatings within particle diameters of 100–300 nm. Limited differences were observed for the size-resolved among the three pollution levels. The average for all rBCc was 2.1±0.2, 1.6±0.1 and 1.5±0.04 for heavy, moderate and light pollution respectively. More heavily coated rBCc were present in the heavy pollution condition, and this was consistent with more secondary particle formation than the other periods.
Figure 5h shows the distribution of the hygroscopicity parameter (κ). The lowest κall between 150 and 300 nm at heavy and moderate pollution condition was mainly caused by the increase of rBC fractions. Due to the increase of more hydroscopic inorganic compositions for larger particles under heavy and moderate pollution conditions, κall increased considerably for particles Da>200 and 300 nm. In contrast to the more polluted cases, κall under the light pollution period varied slightly with the increase of Da. Caused by the absence of more soluble inorganic compositions, κall for particles with Da>300 nm during the light pollution period was lower than the other conditions. For rBCc, κrBCc was more influenced by the coating volume fractions rather than the coating compositions, as the variation of κrBCc generally followed the trend of the coating thickness of rBCc (Fig. 5g). κrBCc for particles with Da<300 nm was close under three different pollution levels, and the decreasing trend of κrBCc between 90 and 300 nm was caused by the reduction of coating material fraction.
3.3 Size-resolved particle morphology
Figure 6 shows the distribution of particle density, average single-particle size and mass, and morphology parameters for all particles (left) and rBCc (right). The average particle density for all particles (ρall) varied slightly between 1.55 and 1.6 g cm−3, and the particle density of rBCc (ρrBCc) within the measurement size range was generally higher than the ρall due to the higher density of rBC. The peak ρrBCc reached between 200 and 300 nm in Da due to the rBCc was least coated within this size range. Dv was larger than Da and deviated more at smaller size but was close to Da for all particles and rBCc larger than 200 nm. The dynamic shape factor (χ) of all particles declined from around 1.8 to 1.2, while χ of rBCc declined from around 2 to 1.2. All particles with Da above 400 nm and rBCc with Da above 500 nm tended to have lower χ, which was around 1.2. A previous study (Lin et al., 2015) in other megacities reported that χ of all particles was around 2 with Da at around 100 nm, which is close to our results.
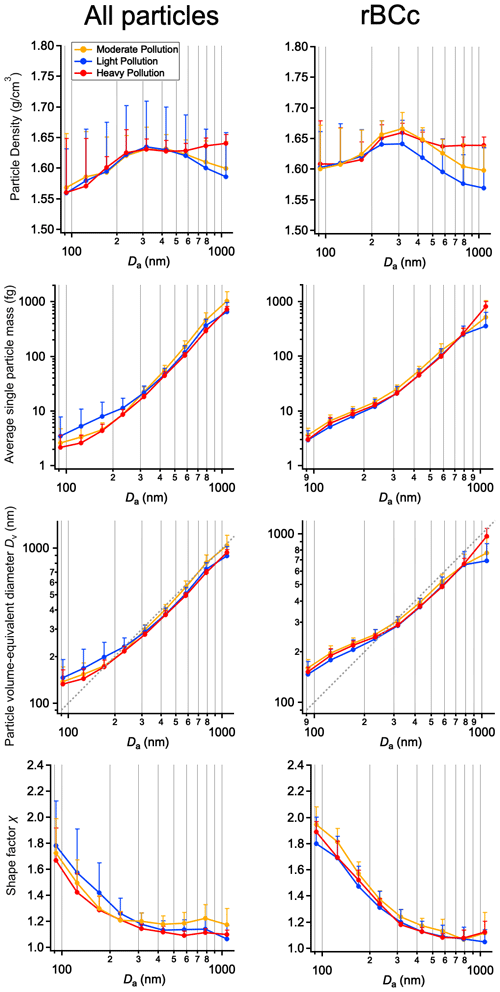
Figure 6The particle density, average single-particle mass, shape factor and volume-equivalent diameter for all particles (all particles, left) and refractory-black-carbon-containing particles (rBCc, right) under different pollution levels.
This result indicates that smaller particles have more irregular shapes, while particles with larger aerodynamic size are more spherical in the ambient atmosphere. Previous experiments have shown that the irregular rBCc from fresh emissions can transform to be more spherical-like by acquiring more secondary substances (Ahern et al., 2016). K. Hu et al. (2021) illustrate that the acquisition of coating material is more important for the overall shape of rBCc, while the shape of the rBC core is not sufficient to describe the change of the overall shape of rBCc. Our results confirm that the spherical assumption is suitable for large rBCc in aerodynamic size in a typical anthropogenic polluted environment. This also implies that larger and spherical particles tend to have a larger deposition rate, while particles with more irregularity may experience higher drag force in the air, towards decelerating the settlement.
3.4 Size-resolved CN and CCN number concentrations
Figure 7a and b present the distribution of rBCc, CN and CCN number concentrations at different polluted conditions. The peak of the number concentration of rBCc during heavy pollution periods was at around 300 nm, while the peak for moderate and light pollution was slightly smaller (at around 200 nm). This agrees with the previous studies in Beijing showing that the average total size of rBCc was associated with the pollution levels (Yu et al., 2020; Liu et al., 2019). A similar trend was also observed for the CN concentrations, and the peak of CN concentrations shifted to the larger particle size with increasing pollution levels. Higher levels of pollution enlarged the particle size through condensation and coagulation. Because of the increase of the average particle size, a larger fraction of particles can be activated as CCN under heavy pollution.
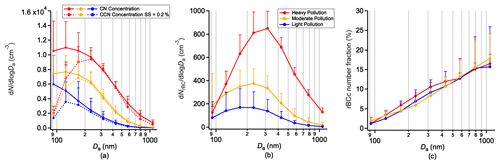
Figure 7Size-resolved (a) CN and CCN at SS=0.2 % number concentrations, (b) rBC number concentration and (c) number fraction of rBCc.
By using the aerodynamic size-resolved number concentration of rBC and CN, a remarkable increase of rBC number fraction at larger aerodynamic size was found (Fig. 7c); i.e. with Da from 100–1000 nm, the rBC number fraction increased almost linearly from 3 % to 15 %, and this applied to all pollution levels. This tends to represent a generic phenomenon for a suburban environment with continuous influence of anthropogenic emissions, and the primary emissions had been aged in a timescale of hours. Fine rBC coagulated with pre-existing larger particles during the ageing process (Riemer et al., 2009). The coagulation process dominated the formation of thickly coated rBC particles (Reddington et al., 2013), and the coagulation rate of smaller rBC may be fast due to the higher number concentration of fine-mode particles (Matsui et al., 2018). The coagulation occurs more rapidly near the source due to the higher number concentration (Jacobson, 2005). Zhang et al. (2020) reported an increase in BC number concentrations with increasing rBC core size for fresh residential firewood burning emission in Beijing surrounding regions. Due to the high concentration of both rBC and co-emitted NR particles, the coagulation of rBC is rapid, and thickly coated rBCc with relatively large rBC core formed shortly after emission. Liu et al. (2019) also reported a higher ageing degree of rBCc during the heating season in North China. However, the very fresh fossil fuel emissions such as from diesel engine emissions, which mostly contain thinly coated small rBC (Han et al., 2019), may not show the same rBC number fraction distribution. Previous studies also reported a relatively fast ageing process for BC, also in the order of hours (Peng et al., 2017); if under a polluted environment rich in precursors, the ageing could be even faster (Peng et al., 2016). The cause of this increased rBC number fraction at larger particle size is therefore the non-rBC compounds associated with it. The results presented here indicated that the higher contribution from regional pollution to the rBC number at larger aerodynamic size may apply, albeit the various features of primary sources in winter (Wang et al., 2019; Liu et al., 2019).
It is also possible that these larger rBCc may have experienced in-cloud processing. If the air parcel has passed through a cloud, the large and thickly coated rBCc are expected to have be scavenged through activation, and the size of rBC core may increase effectively within the cloud because of the cloud droplet collision (Ding et al., 2019b). Through in-cloud aqueous reactions, sulfate or organic matter may be added to the rBCc (Zhang et al., 2017). When the cloud dries out through the cloud evaporation or the air parcel descending in a downdraft, the core size and coatings may be enlarged for these released rBCc, although the results presented here cannot alone test this hypothesis.
The rBC associated with larger coatings was more spherical (with χ close to 1, as discussed above) and therefore more likely to have an absorption enhancement from the lensing effect of coatings (Liu et al., 2017). In addition, these size-resolved rBC number fraction results will improve the understanding of the lung deposition of BC in human health studies (Rissler et al., 2017). This means that particles with a higher deposition rate tend to contain a higher number fraction of rBC, which may provide some indications for constituents deposited in different parts of the human respiratory system (Carvalho et al., 2011; Manigrasso et al., 2020).
3.5 CCN ability and activation of rBCc
Presented in Fig. 8a, the D50,All varied smoothly and was slightly higher than 100 nm for most of the experiment period. The mean D50,All and D50,rBCc were 112±6 and 193±41 nm respectively. Shown in Fig. 8d, most of the D50,rBCc was around 200 nm, which illustrates that the number concentrations of rBCc with Da above 200 nm made a significant contribution to the overall AFrBCc.
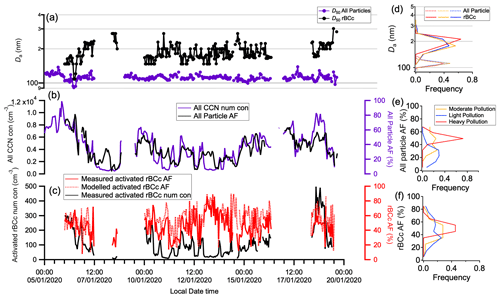
Figure 8CCN activities of all particles and rBCc. (a) Time series of D50 for all particles and rBCc. (b) Time series of all CCN number concentrations and the activation fractions of all particles. (c) Time series of measured number concentrations of activated rBCc, and activation fractions of rBCc from two methods. (d) Frequency of D50 for all particles and rBCc. (e) Frequency of the activation fraction of all particles. (f) Frequency of measured activation fraction of rBCc.
Figure 8b presents the temporal evolution of the CCN number concentration and activation fraction for all particles and rBCc. Figure 8e showed 50±4 % of the measured particles can be activated with SS=0.2 % under the heavy polluted period, while the AFall for the light pollution period was generally lower than the AFall of the other two periods, which was 24±10 % on average. The AFall for the moderate pollution period was 39±9 % on average. Shown in Fig. 8e and f, both all particles and rBCc showed a high activation fraction of around 50 % during the heavy pollution period, while for moderate and light pollution conditions, rBCc exhibited a substantially higher activation fraction than all particles, especially under light pollution periods, where the average activation fraction was 44±18 % for rBCc compared to 24±10 % for all particles. The high activation fraction of rBCc maintained at all pollution levels resulted from the relatively higher rBC number fractions at larger Da (Fig. 8c) because of the higher associated coatings. The directly measured CCN activity of rBCc showed that particles at larger sizes had contained a larger fraction of rBCc that were CCN-active, due to the larger particle size. This in turn implies that rBCc have the potential to be more efficiently incorporated into cloud droplets. The measured and modelled AFrBCc were close and agreed within 22 % (shown in Fig. S3 in the Supplement), and the modelled AFrBCc was slightly higher than the measurement results. This underestimation of modelled D50,rBCc may result from an overestimation of the κrBCc as here a consistent κ was applied between coatings of rBCc and all non-refractory materials in bulk, though the coatings on rBC may not have contained as many hygroscopic materials as the bulk non-rBC aerosols. Freshly emitted rBC particles contain substantial amounts of organic matter (Peng et al., 2017), while the more hygroscopic secondary inorganic materials require atmospheric ageing to be mixed with rBC (Hu et al., 2020b). Our results confirm that while rBCc can be CCN-active, the size of rBCc is crucial to the CCN ability of rBCc in a polluted suburban environment. This agrees with the previous study done by Motos et al. (2019b), who also found that the size of rBCc is important for the activation of rBCc at certain SS. The AFrBCc result presented in our study is generally consistent with previous field measurements in anthropogenic polluted environments in China: Wu et al. (2019) and D. Hu et al. (2021) reported 59 % and 60 % of total rBCc could be activated at SS=0.2 % respectively. Studies performed in other environments also found that coated BC can be CCN-active: Motos et al. (2019a, b) reported that ∼6 %–12 % and ∼40 %–70 % of total BC mass fraction can be activated with SS≈0.05 % in Zurich and SS≈0.2 % at Jungfraujoch respectively.
The AAC combination applied in this study introduced a new way to explore the physiochemical properties of aerosols. The comprehensive size-resolved aerosol information presented in this study can contribute to future studies focusing on the evolution and lifetime of BC and improve particle-resolved model simulations (i.e. Riemer et al., 2009) for anthropogenic polluted environments. Importantly, our results showed that thickly coated rBCc accounted for a higher number fraction at larger particle size than the smaller particle size in the Beijing suburban region. As indicated in Fig. 9, the mass absorption coefficient (MAC) of rBCc at 550 and 880 nm wavelength is calculated through the core–shell Mie model described in the Supplement. The MAC550 and MAC880 were enhanced about 2-fold for rBCc with Da>500 nm. These larger rBCc with high absorption efficiency importantly contributed to the total absorption. When transported into the top of the boundary layer, these highly coated and absorbing rBCc can be efficiently incorporated into clouds (Ding et al., 2019a). The absorption effects of these rBCc will be further magnified by mixing with the cloud water droplets (Wu et al., 2016), and the lensing effect may reduce the cloud lifetime (Ramanathan et al., 2001) or the cloud albedo (Chuang et al., 2002). In addition to the strong radiative absorption, these large rBCc may also alter the regional precipitation rate (Johnson et al., 2019).
In this study, a new aerodynamic size selection technique was applied for the direct size-resolved characterization of aerosol constituents and properties on both a mass and number basis in a suburban Beijing region in winter. Besides the size selection without relying on particle charging efficiency, this technique allows for reliable size-resolved particle properties. Organic compounds accounted for around 40 % of the total PM1 mass, and we found higher contribution of particulate nitrate at larger sizes under polluted cases in the Beijing suburban region. In particular, particles with larger aerodynamic diameter (Da) were found to contain a higher number fraction of refractory black carbon (rBC), which means rBC could be more efficiently mixed with larger particles during atmospheric processes. Mie calculation results show that these thickly coated refractory-black-carbon-containing particles (rBCc) as included in large particles may have an absorption enhanced up to 2-fold. The dynamic shape factors for both refractory and non-refractory particles have also been derived. Particles with Da larger than 300 nm tended to have a more spherical-like shape, while smaller particles had a more irregular shape in the polluted environment. By applying the method introduced by D. Hu et al. (2021), as high as a 46±15 % number fraction of rBCc was observed to be activated under SS=0.2 %. Our results suggest that the size of rBCc is key to the cloud condensation nuclei (CCN) activities of rBCc. Though rBC was small and hydrophobic initially, after being mixed with non-refractory compositions and becoming larger, the rBCc can become CCN-active. The higher number fraction of rBCc at larger particle size (Da>300 nm) emphasizes the importance of the rBCc as a considerable CCN source. In summary, the rBCc from anthropogenic emissions, after short ageing on a regional scale, may therefore alter the regional radiative forcing directly or indirectly through altering cloud properties and deposit in the human respiratory system efficiently.
Raw data are archived at Zhejiang University and are available on request.
The supplement related to this article is available online at: https://doi.org/10.5194/acp-22-4375-2022-supplement.
CY and DL designed the experiments and wrote the paper; DL and JDA provided guidance with the analysis and writing; CY, DL, KH, PT, YW, DZ, WG, MH and DD performed experiments; CY, DL, KH, HW, DH and JDA contributed to the data analysis; QL provided the AAC and guided the operations.
At least one of the (co-)authors is a member of the editorial board of Atmospheric Chemistry and Physics. The peer-review process was guided by an independent editor, and the authors also have no other competing interests to declare.
Publisher's note: Copernicus Publications remains neutral with regard to jurisdictional claims in published maps and institutional affiliations.
The authors acknowledge Cambustion Ltd for providing the AAC instrument.
This research has been supported by the National Natural Science Foundation of China (grant nos. 41875167, 42175116, and 41975177) and the National Key Research and Development Programme of China (grant no. 2019YFC0214703).
This paper was edited by Xiaohong Liu and reviewed by two anonymous referees.
Ahern, A. T., Subramanian, R., Saliba, G., Lipsky, E. M., Donahue, N. M., and Sullivan, R. C.: Effect of secondary organic aerosol coating thickness on the real-time detection and characterization of biomass-burning soot by two particle mass spectrometers, Atmos. Meas. Tech., 9, 6117–6137, https://doi.org/10.5194/amt-9-6117-2016, 2016.
Bessho, K., Date, K., Hayashi, M., Ikeda, A., Imai, T., Inoue, H., Kumagai, Y., Miyakawa, T., Murata, H., Ohno, T., Okuyama, A., Oyama, R., Sasaki, Y., Shimazu, Y., Shimoji, K., Sumida, Y., Suzuki, M., Taniguchi, H., Tsuchiyama, H., Uesawa, D., Yokota, H., and Yoshida, R.: An Introduction to Himawari-8/9 – Japan's New-Generation Geostationary Meteorological Satellites, J. Meteorol. Soc. Jpn., Ser. II, 94, 151–183, https://doi.org/10.2151/jmsj.2016-009, 2016.
Bond, T. C., Doherty, S. J., Fahey, D. W., Forster, P. M., Berntsen, T., DeAngelo, B. J., Flanner, M. G., Ghan, S., Kärcher, B., Koch, D., Kinne, S., Kondo, Y., Quinn, P. K., Sarofim, M. C., Schultz, M. G., Schulz, M., Venkataraman, C., Zhang, H., Zhang, S., Bellouin, N., Guttikunda, S. K., Hopke, P. K., Jacobson, M. Z., Kaiser, J. W., Klimont, Z., Lohmann, U., Schwarz, J. P., Shindell, D., Storelvmo, T., Warren, S. G., and Zender, C. S.: Bounding the role of black carbon in the climate system: A scientific assessment, J. Geophys. Res.-Atmos., 118, 5380–5552, https://doi.org/10.1002/jgrd.50171, 2013.
Broekhuizen, K., Chang, R. Y.-W., Leaitch, W. R., Li, S.-M., and Abbatt, J. P. D.: Closure between measured and modeled cloud condensation nuclei (CCN) using size-resolved aerosol compositions in downtown Toronto, Atmos. Chem. Phys., 6, 2513–2524, https://doi.org/10.5194/acp-6-2513-2006, 2006.
Brown, A., Milton, S., Cullen, M., Golding, B., Mitchell, J., and Shelly, A.: Unified Modeling and Prediction of Weather and Climate: A 25-Year Journey, B. Am. Meteorol. Soc., 93, 1865–1877, https://doi.org/10.1175/BAMS-D-12-00018.1, 2012.
Cappa, C. D., Onasch, T. B., Massoli, P., Worsnop, D. R., Bates, T. S., Cross, E. S., Davidovits, P., Hakala, J., Hayden, K. L., Jobson, B. T., Kolesar, K. R., Lack, D. A., Lerner, B. M., Li, S.-M., Mellon, D., Nuaaman, I., Olfert, J. S., Petäjä, T., Quinn, P. K., Song, C., Subramanian, R., Williams, E. J., and Zaveri, R. A.: Radiative Absorption Enhancements Due to the Mixing State of Atmospheric Black Carbon, Science, 337, 1078, https://doi.org/10.1126/science.1223447, 2012.
Carvalho, T. C., Peters, J. I., and Williams, R. O.: Influence of particle size on regional lung deposition – What evidence is there?, Int. J. Pharm., 406, 1–10, https://doi.org/10.1016/j.ijpharm.2010.12.040, 2011.
Chen, Y., Cai, J., Wang, Z., Peng, C., Yao, X., Tian, M., Han, Y., Shi, G., Shi, Z., Liu, Y., Yang, X., Zheng, M., Zhu, T., He, K., Zhang, Q., and Yang, F.: Simultaneous measurements of urban and rural particles in Beijing – Part 1: Chemical composition and mixing state, Atmos. Chem. Phys., 20, 9231–9247, https://doi.org/10.5194/acp-20-9231-2020, 2020a.
Chen, Y., Shi, G., Cai, J., Shi, Z., Wang, Z., Yao, X., Tian, M., Peng, C., Han, Y., Zhu, T., Liu, Y., Yang, X., Zheng, M., Yang, F., Zhang, Q., and He, K.: Simultaneous measurements of urban and rural particles in Beijing – Part 2: Case studies of haze events and regional transport, Atmos. Chem. Phys., 20, 9249–9263, https://doi.org/10.5194/acp-20-9249-2020, 2020b.
Ching, J. and Kajino, M.: Aerosol mixing state matters for particles deposition in human respiratory system, Sci. Rep., 8, 8864, https://doi.org/10.1038/s41598-018-27156-z, 2018.
Chuang, C. C., Penner, J. E., Prospero, J. M., Grant, K. E., Rau, G. H., and Kawamoto, K.: Cloud susceptibility and the first aerosol indirect forcing: Sensitivity to black carbon and aerosol concentrations, J. Geophys. Res.-Atmos., 107, AAC 10-11–AAC 10-23, https://doi.org/10.1029/2000JD000215, 2002.
DeCarlo, P. F., Slowik, J. G., Worsnop, D. R., Davidovits, P., and Jimenez, J. L.: Particle Morphology and Density Characterization by Combined Mobility and Aerodynamic Diameter Measurements. Part 1: Theory, Aerosol Sci. Tech., 38, 1185–1205, https://doi.org/10.1080/027868290903907, 2004.
DeCarlo, P. F., Kimmel, J. R., Trimborn, A., Northway, M. J., Jayne, J. T., Aiken, A. C., Gonin, M., Fuhrer, K., Horvath, T., Docherty, K. S., Worsnop, D. R., and Jimenez, J. L.: Field-Deployable, High-Resolution, Time-of-Flight Aerosol Mass Spectrometer, Anal. Chem., 78, 8281–8289, https://doi.org/10.1021/ac061249n, 2006.
Ding, S., Liu, D., Zhao, D., Hu, K., Tian, P., Zhou, W., Huang, M., Yang, Y., Wang, F., Sheng, J., Liu, Q., Kong, S., Cui, P., Huang, Y., He, H., Coe, H., and Ding, D.: Size-Related Physical Properties of Black Carbon in the Lower Atmosphere over Beijing and Europe, Environ. Sci. Technol., 53, 11112–11121, https://doi.org/10.1021/acs.est.9b03722, 2019a.
Ding, S., Zhao, D., He, C., Huang, M., He, H., Tian, P., Liu, Q., Bi, K., Yu, C., Pitt, J., Chen, Y., Ma, X., Chen, Y., Jia, X., Kong, S., Wu, J., Hu, D., Hu, K., Ding, D., and Liu, D.: Observed Interactions Between Black Carbon and Hydrometeor During Wet Scavenging in Mixed-Phase Clouds, Geophys. Res. Lett., 46, 8453–8463, https://doi.org/10.1029/2019GL083171, 2019b.
Fan, X., Liu, J., Zhang, F., Chen, L., Collins, D., Xu, W., Jin, X., Ren, J., Wang, Y., Wu, H., Li, S., Sun, Y., and Li, Z.: Contrasting size-resolved hygroscopicity of fine particles derived by HTDMA and HR-ToF-AMS measurements between summer and winter in Beijing: the impacts of aerosol aging and local emissions, Atmos. Chem. Phys., 20, 915–929, https://doi.org/10.5194/acp-20-915-2020, 2020.
Fierce, L., Onasch, T. B., Cappa, C. D., Mazzoleni, C., China, S., Bhandari, J., Davidovits, P., Fischer, D. A., Helgestad, T., Lambe, A. T., Sedlacek, A. J., Smith, G. D., and Wolff, L.: Radiative absorption enhancements by black carbon controlled by particle-to-particle heterogeneity in composition, P. Natl. Acad. Sci. USA, 117, 5196, https://doi.org/10.1073/pnas.1919723117, 2020.
Fukuda, S., Nakajima, T., Takenaka, H., Higurashi, A., Kikuchi, N., Nakajima, T. Y., and Ishida, H.: New approaches to removing cloud shadows and evaluating the 380 nm surface reflectance for improved aerosol optical thickness retrievals from the GOSAT/TANSO-Cloud and Aerosol Imager, J. Geophys. Res.-Atmos., 118, 13520–13531, https://doi.org/10.1002/2013JD020090, 2013.
Gong, X., Zhang, C., Chen, H., Nizkorodov, S. A., Chen, J., and Yang, X.: Size distribution and mixing state of black carbon particles during a heavy air pollution episode in Shanghai, Atmos. Chem. Phys., 16, 5399–5411, https://doi.org/10.5194/acp-16-5399-2016, 2016.
Gunthe, S. S., Rose, D., Su, H., Garland, R. M., Achtert, P., Nowak, A., Wiedensohler, A., Kuwata, M., Takegawa, N., Kondo, Y., Hu, M., Shao, M., Zhu, T., Andreae, M. O., and Pöschl, U.: Cloud condensation nuclei (CCN) from fresh and aged air pollution in the megacity region of Beijing, Atmos. Chem. Phys., 11, 11023–11039, https://doi.org/10.5194/acp-11-11023-2011, 2011.
Gysel, M., Crosier, J., Topping, D. O., Whitehead, J. D., Bower, K. N., Cubison, M. J., Williams, P. I., Flynn, M. J., McFiggans, G. B., and Coe, H.: Closure study between chemical composition and hygroscopic growth of aerosol particles during TORCH2, Atmos. Chem. Phys., 7, 6131–6144, https://doi.org/10.5194/acp-7-6131-2007, 2007.
Han, C., Li, S. M., Liu, P., and Lee, P.: Size Dependence of the Physical Characteristics of Particles Containing Refractory Black Carbon in Diesel Vehicle Exhaust, Environ. Sci. Technol., 53, 137–145, https://doi.org/10.1021/acs.est.8b04603, 2019.
Han, Y., Chen, W., Chatzidiakou, L., Krause, A., Yan, L., Zhang, H., Chan, Q., Barratt, B., Jones, R., Liu, J., Wu, Y., Zhao, M., Zhang, J., Kelly, F. J., Zhu, T., and the AIRLESS team: Effects of AIR pollution on cardiopuLmonary disEaSe in urban and peri-urban reSidents in Beijing: protocol for the AIRLESS study, Atmos. Chem. Phys., 20, 15775–15792, https://doi.org/10.5194/acp-20-15775-2020, 2020.
Hu, D., Wang, Y., Yu, C., Xie, Q., Yue, S., Shang, D., Fang, X., Joshi, R., Liu, D., Allan, J., Wu, Z., Hu, M., Fu, P., and McFiggans, G.: Vertical profile of particle hygroscopicity and CCN effectiveness during winter in Beijing: insight into the hygroscopicity transition threshold of black carbon, Faraday Discuss., 226, 239–254, https://doi.org/10.1039/d0fd00077a, 2020a.
Hu, D., Liu, D., Zhao, D., Yu, C., Liu, Q., Tian, P., Bi, K., Ding, S., Hu, K., Wang, F., Wu, Y., Wu, Y., Kong, S., Zhou, W., He, H., Huang, M., and Ding, D.: Closure Investigation on Cloud Condensation Nuclei Ability of Processed Anthropogenic Aerosols, J. Geophys. Res.-Atmos., 125, e2020JD032680, https://doi.org/10.1029/2020jd032680, 2020b.
Hu, D., Liu, D., Kong, S., Zhao, D., Wu, Y., Li, S., Ding, S., Zheng, S., Cheng, Y., Hu, K., Deng, Z., Wu, Y., Tian, P., Liu, Q., Huang, M., and Ding, D.: Direct Quantification of Droplet Activation of Ambient Black Carbon Under Water Supersaturation, J. Geophys. Res.-Atmos., 126, e2021JD034649, https://doi.org/10.1029/2021JD034649, 2021.
Hu, K., Liu, D., Tian, P., Wu, Y., Deng, Z., Wu, Y., Zhao, D., Li, R., Sheng, J., Huang, M., Ding, D., Li, W., Wang, Y., and Wu, Y.: Measurements of the Diversity of Shape and Mixing State for Ambient Black Carbon Particles, Geophys. Res. Lett., 48, e2021GL094522, https://doi.org/10.1029/2021GL094522, 2021.
Hu, W., Hu, M., Hu, W., Jimenez, J. L., Yuan, B., Chen, W., Wang, M., Wu, Y., Chen, C., Wang, Z., Peng, J., Zeng, L., and Shao, M.: Chemical composition, sources, and aging process of submicron aerosols in Beijing: Contrast between summer and winter, J. Geophys. Res.-Atmos., 121, 1955–1977, https://doi.org/10.1002/2015JD024020, 2016.
Jacobson, M. Z.: Fundamentals of Atmospheric Modeling, 2, Cambridge University Press, Cambridge, https://doi.org/10.1017/CBO9781139165389, 2005.
Johnson, B. T., Haywood, J. M., and Hawcroft, M. K.: Are Changes in Atmospheric Circulation Important for Black Carbon Aerosol Impacts on Clouds, Precipitation, and Radiation?, J. Geophys. Res.-Atmos., 124, 7930–7950, https://doi.org/10.1029/2019JD030568, 2019.
Johnson, T. J., Irwin, M., Symonds, J. P. R., Olfert, J. S., and Boies, A. M.: Measuring aerosol size distributions with the aerodynamic aerosol classifier, Aerosol Sci. Tech., 52, 655–665, https://doi.org/10.1080/02786826.2018.1440063, 2018.
Jones, A., Thomson, D., Hort, M., and Devenish, B.: The U. K. Met Office's Next-Generation Atmospheric Dispersion Model, NAME III, Air Pollution Modeling and Its Application XVII, Springer, Boston, MA, ISBN: 978-0-387-28255-8, 580–589, 2007.
Joshi, R., Liu, D., Nemitz, E., Langford, B., Mullinger, N., Squires, F., Lee, J., Wu, Y., Pan, X., Fu, P., Kotthaus, S., Grimmond, S., Zhang, Q., Wu, R., Wild, O., Flynn, M., Coe, H., and Allan, J.: Direct measurements of black carbon fluxes in central Beijing using the eddy covariance method, Atmos. Chem. Phys., 21, 147–162, https://doi.org/10.5194/acp-21-147-2021, 2021.
Kim, J. H., Mulholland, G. W., Kukuck, S. R., and Pui, D. Y. H.: Slip Correction Measurements of Certified PSL Nanoparticles Using a Nanometer Differential Mobility Analyzer (Nano-DMA) for Knudsen Number From 0.5 to 83, J. Res. Natl. Inst. Stan., 110, 31–54, https://doi.org/10.6028/jres.110.005, 2005.
Kwon, H.-S., Ryu, M. H., and Carlsten, C.: Ultrafine particles: unique physicochemical properties relevant to health and disease, Exp. Mol. Med., 52, 318–328, https://doi.org/10.1038/s12276-020-0405-1, 2020.
Laborde, M., Schnaiter, M., Linke, C., Saathoff, H., Naumann, K.-H., Möhler, O., Berlenz, S., Wagner, U., Taylor, J. W., Liu, D., Flynn, M., Allan, J. D., Coe, H., Heimerl, K., Dahlkötter, F., Weinzierl, B., Wollny, A. G., Zanatta, M., Cozic, J., Laj, P., Hitzenberger, R., Schwarz, J. P., and Gysel, M.: Single Particle Soot Photometer intercomparison at the AIDA chamber, Atmos. Meas. Tech., 5, 3077–3097, https://doi.org/10.5194/amt-5-3077-2012, 2012.
Lack, D. A. and Cappa, C. D.: Impact of brown and clear carbon on light absorption enhancement, single scatter albedo and absorption wavelength dependence of black carbon, Atmos. Chem. Phys., 10, 4207–4220, https://doi.org/10.5194/acp-10-4207-2010, 2010.
Levin, E. J. T., Prenni, A. J., Palm, B. B., Day, D. A., Campuzano-Jost, P., Winkler, P. M., Kreidenweis, S. M., DeMott, P. J., Jimenez, J. L., and Smith, J. N.: Size-resolved aerosol composition and its link to hygroscopicity at a forested site in Colorado, Atmos. Chem. Phys., 14, 2657–2667, https://doi.org/10.5194/acp-14-2657-2014, 2014.
Li, H., Zhang, Q., Zheng, B., Chen, C., Wu, N., Guo, H., Zhang, Y., Zheng, Y., Li, X., and He, K.: Nitrate-driven urban haze pollution during summertime over the North China Plain, Atmos. Chem. Phys., 18, 5293–5306, https://doi.org/10.5194/acp-18-5293-2018, 2018.
Li, J., Cao, L., Gao, W., He, L., Yan, Y., He, Y., Pan, Y., Ji, D., Liu, Z., and Wang, Y.: Seasonal variations in the highly time-resolved aerosol composition, sources and chemical processes of background submicron particles in the North China Plain, Atmos. Chem. Phys., 21, 4521–4539, https://doi.org/10.5194/acp-21-4521-2021, 2021.
Li, L., Li, M., Huang, Z., Gao, W., Nian, H., Fu, Z., Gao, J., Chai, F., and Zhou, Z.: Ambient particle characterization by single particle aerosol mass spectrometry in an urban area of Beijing, Atmos. Environ., 94, 323–331, https://doi.org/10.1016/j.atmosenv.2014.03.048, 2014.
Lin, G.-Y., Lee, G.-R., Lin, S.-F., Hung, Y.-H., Li, S.-W., Wu, G.-J., Ye, H., Huang, W., and Tsai, C.-J.: Ultrafine Particles and PM2.5 at Three Urban Air Monitoring Stations in Northern Taiwan from 2011 to 2013, Aerosol Air Qual. Res., 15, 2305–2317, https://doi.org/10.4209/aaqr.2015.04.0271, 2015.
Lipworth, B., Manoharan, A., and Anderson, W.: Unlocking the quiet zone: the small airway asthma phenotype, Lancet Resp. Med., 2, 497–506, https://doi.org/10.1016/S2213-2600(14)70103-1, 2014.
Liu, D., Allan, J., Whitehead, J., Young, D., Flynn, M., Coe, H., McFiggans, G., Fleming, Z. L., and Bandy, B.: Ambient black carbon particle hygroscopic properties controlled by mixing state and composition, Atmos. Chem. Phys., 13, 2015–2029, https://doi.org/10.5194/acp-13-2015-2013, 2013.
Liu, D., Quennehen, B., Darbyshire, E., Allan, J. D., Williams, P. I., Taylor, J. W., Bauguitte, S. J.-B., Flynn, M. J., Lowe, D., Gallagher, M. W., Bower, K. N., Choularton, T. W., and Coe, H.: The importance of Asia as a source of black carbon to the European Arctic during springtime 2013, Atmos. Chem. Phys., 15, 11537–11555, https://doi.org/10.5194/acp-15-11537-2015, 2015.
Liu, D., Whitehead, J., Alfarra, M. R., Reyes-Villegas, E., Spracklen, D. V., Reddington, C. L., Kong, S., Williams, P. I., Ting, Y.-C., Haslett, S., Taylor, J. W., Flynn, M. J., Morgan, W. T., McFiggans, G., Coe, H., and Allan, J. D.: Black-carbon absorption enhancement in the atmosphere determined by particle mixing state, Nat. Geosci., 10, 184–188, https://doi.org/10.1038/ngeo2901, 2017.
Liu, D., Joshi, R., Wang, J., Yu, C., Allan, J. D., Coe, H., Flynn, M. J., Xie, C., Lee, J., Squires, F., Kotthaus, S., Grimmond, S., Ge, X., Sun, Y., and Fu, P.: Contrasting physical properties of black carbon in urban Beijing between winter and summer, Atmos. Chem. Phys., 19, 6749–6769, https://doi.org/10.5194/acp-19-6749-2019, 2019.
Liu, D., He, C., Schwarz, J. P., and Wang, X.: Lifecycle of light-absorbing carbonaceous aerosols in the atmosphere, npj Climate and Atmospheric Science, 3, 40, https://doi.org/10.1038/s41612-020-00145-8, 2020.
Liu, Q., Liu, D., Wu, Y., Bi, K., Gao, W., Tian, P., Zhao, D., Li, S., Yu, C., Tang, G., Wu, Y., Hu, K., Ding, S., Gao, Q., Wang, F., Kong, S., He, H., Huang, M., and Ding, D.: Reduced volatility of aerosols from surface emissions to the top of the planetary boundary layer, Atmos. Chem. Phys., 21, 14749–14760, https://doi.org/10.5194/acp-21-14749-2021, 2021.
Liu, Z., Hu, B., Zhang, J., Yu, Y., and Wang, Y.: Characteristics of aerosol size distributions and chemical compositions during wintertime pollution episodes in Beijing, Atmos. Res., 168, 1–12, https://doi.org/10.1016/j.atmosres.2015.08.013, 2016.
Manigrasso, M., Costabile, F., Liberto, L. D., Gobbi, G. P., Gualtieri, M., Zanini, G., and Avino, P.: Size resolved aerosol respiratory doses in a Mediterranean urban area: From PM10 to ultrafine particles, Environ. Int., 141, 105714, https://doi.org/10.1016/j.envint.2020.105714, 2020.
Marple, V. A., Rubow, K. L., and Behm, S. M.: A Microorifice Uniform Deposit Impactor (MOUDI): Description, Calibration, and Use, Aerosol Sci. Tech., 14, 434–446, https://doi.org/10.1080/02786829108959504, 1991.
Matsui, H., Hamilton, D. S., and Mahowald, N. M.: Black carbon radiative effects highly sensitive to emitted particle size when resolving mixing-state diversity, Nat. Commun., 9, 3446, https://doi.org/10.1038/s41467-018-05635-1, 2018.
Middlebrook, A. M., Bahreini, R., Jimenez, J. L., and Canagaratna, M. R.: Evaluation of Composition-Dependent Collection Efficiencies for the Aerodyne Aerosol Mass Spectrometer using Field Data, Aerosol Sci. Tech., 46, 258–271, https://doi.org/10.1080/02786826.2011.620041, 2012.
Motos, G., Schmale, J., Corbin, J. C., Zanatta, M., Baltensperger, U., and Gysel-Beer, M.: Droplet activation behaviour of atmospheric black carbon particles in fog as a function of their size and mixing state, Atmos. Chem. Phys., 19, 2183–2207, https://doi.org/10.5194/acp-19-2183-2019, 2019a.
Motos, G., Schmale, J., Corbin, J. C., Modini, Rob. L., Karlen, N., Bertò, M., Baltensperger, U., and Gysel-Beer, M.: Cloud droplet activation properties and scavenged fraction of black carbon in liquid-phase clouds at the high-alpine research station Jungfraujoch (3580 m a.s.l.), Atmos. Chem. Phys., 19, 3833–3855, https://doi.org/10.5194/acp-19-3833-2019, 2019b.
Myhre, G. and Samset, B. H.: Standard climate models radiation codes underestimate black carbon radiative forcing, Atmos. Chem. Phys., 15, 2883–2888, https://doi.org/10.5194/acp-15-2883-2015, 2015.
Peng, J., Hu, M., Guo, S., Du, Z., Zheng, J., Shang, D., Levy Zamora, M., Zeng, L., Shao, M., Wu, Y.-S., Zheng, J., Wang, Y., Glen, C. R., Collins, D. R., Molina, M. J., and Zhang, R.: Markedly enhanced absorption and direct radiative forcing of black carbon under polluted urban environments, P. Natl. Acad. Sci. USA, 113, 4266, https://doi.org/10.1073/pnas.1602310113, 2016.
Peng, J., Hu, M., Guo, S., Du, Z., Shang, D., Zheng, J., Zheng, J., Zeng, L., Shao, M., Wu, Y., Collins, D., and Zhang, R.: Ageing and hygroscopicity variation of black carbon particles in Beijing measured by a quasi-atmospheric aerosol evolution study (QUALITY) chamber, Atmos. Chem. Phys., 17, 10333–10348, https://doi.org/10.5194/acp-17-10333-2017, 2017.
Petters, M. D. and Kreidenweis, S. M.: A single parameter representation of hygroscopic growth and cloud condensation nucleus activity, Atmos. Chem. Phys., 7, 1961–1971, https://doi.org/10.5194/acp-7-1961-2007, 2007.
Ramanathan, V., Crutzen, P. J., Kiehl, J. T., and Rosenfeld, D.: Aerosols, Climate, and the Hydrological Cycle, Science, 294, 2119, https://doi.org/10.1126/science.1064034, 2001.
Ravishankara, A. R., Rudich, Y., and Wuebbles, D. J.: Physical Chemistry of Climate Metrics, Chem. Rev., 115, 3682–3703, https://doi.org/10.1021/acs.chemrev.5b00010, 2015.
Reddington, C. L., McMeeking, G., Mann, G. W., Coe, H., Frontoso, M. G., Liu, D., Flynn, M., Spracklen, D. V., and Carslaw, K. S.: The mass and number size distributions of black carbon aerosol over Europe, Atmos. Chem. Phys., 13, 4917–4939, https://doi.org/10.5194/acp-13-4917-2013, 2013.
Riemer, N., West, M., Zaveri, R. A., and Easter, R. C.: Simulating the evolution of soot mixing state with a particle-resolved aerosol model, J. Geophys. Res., 114, D09202, https://doi.org/10.1029/2008jd011073, 2009.
Riemer, N., Ault, A. P., West, M., Craig, R. L., and Curtis, J. H.: Aerosol Mixing State: Measurements, Modeling, and Impacts, Rev. Geophys., 57, 187–249, https://doi.org/10.1029/2018rg000615, 2019.
Rissler, J., Gudmundsson, A., Nicklasson, H., Swietlicki, E., Wollmer, P., and Löndahl, J.: Deposition efficiency of inhaled particles (15–5000 nm) related to breathing pattern and lung function: an experimental study in healthy children and adults, Part. Fibre Toxicol., 14, 10, https://doi.org/10.1186/s12989-017-0190-8, 2017.
Schwarz, J. P., Spackman, J. R., Gao, R. S., Perring, A. E., Cross, E., Onasch, T. B., Ahern, A., Wrobel, W., Davidovits, P., Olfert, J., Dubey, M. K., Mazzoleni, C., and Fahey, D. W.: The Detection Efficiency of the Single Particle Soot Photometer, Aerosol Sci. Tech., 44, 612–628, https://doi.org/10.1080/02786826.2010.481298, 2010.
Seinfeld, J. H. and Pandis, S. N.: Atmospheric Chemistry and Physics: From Air Pollution to Climate Change, 3rd edn., Wiley-Interscience publication, Wiley, ISBN: 978-1-118-94740-1, 2016.
Shi, Z., Vu, T., Kotthaus, S., Harrison, R. M., Grimmond, S., Yue, S., Zhu, T., Lee, J., Han, Y., Demuzere, M., Dunmore, R. E., Ren, L., Liu, D., Wang, Y., Wild, O., Allan, J., Acton, W. J., Barlow, J., Barratt, B., Beddows, D., Bloss, W. J., Calzolai, G., Carruthers, D., Carslaw, D. C., Chan, Q., Chatzidiakou, L., Chen, Y., Crilley, L., Coe, H., Dai, T., Doherty, R., Duan, F., Fu, P., Ge, B., Ge, M., Guan, D., Hamilton, J. F., He, K., Heal, M., Heard, D., Hewitt, C. N., Hollaway, M., Hu, M., Ji, D., Jiang, X., Jones, R., Kalberer, M., Kelly, F. J., Kramer, L., Langford, B., Lin, C., Lewis, A. C., Li, J., Li, W., Liu, H., Liu, J., Loh, M., Lu, K., Lucarelli, F., Mann, G., McFiggans, G., Miller, M. R., Mills, G., Monk, P., Nemitz, E., O'Connor, F., Ouyang, B., Palmer, P. I., Percival, C., Popoola, O., Reeves, C., Rickard, A. R., Shao, L., Shi, G., Spracklen, D., Stevenson, D., Sun, Y., Sun, Z., Tao, S., Tong, S., Wang, Q., Wang, W., Wang, X., Wang, X., Wang, Z., Wei, L., Whalley, L., Wu, X., Wu, Z., Xie, P., Yang, F., Zhang, Q., Zhang, Y., Zhang, Y., and Zheng, M.: Introduction to the special issue “In-depth study of air pollution sources and processes within Beijing and its surrounding region (APHH-Beijing)”, Atmos. Chem. Phys., 19, 7519–7546, https://doi.org/10.5194/acp-19-7519-2019, 2019.
Squires, F. A., Nemitz, E., Langford, B., Wild, O., Drysdale, W. S., Acton, W. J. F., Fu, P., Grimmond, C. S. B., Hamilton, J. F., Hewitt, C. N., Hollaway, M., Kotthaus, S., Lee, J., Metzger, S., Pingintha-Durden, N., Shaw, M., Vaughan, A. R., Wang, X., Wu, R., Zhang, Q., and Zhang, Y.: Measurements of traffic-dominated pollutant emissions in a Chinese megacity, Atmos. Chem. Phys., 20, 8737–8761, https://doi.org/10.5194/acp-20-8737-2020, 2020.
Stokes, R. H. and Robinson, R. A.: Interactions in Aqueous Nonelectrolyte Solutions. I. Solute-Solvent Equilibria, J. Phys. Chem., 70, 2126–2131, https://doi.org/10.1021/j100879a010, 1966.
Sturm, R.: Theoretical models for dynamic shape factors and lung deposition of small particle aggregates originating from combustion processes, Z. Med. Phys., 20, 226–234, https://doi.org/10.1016/j.zemedi.2010.04.001, 2010.
Sturm, R.: Computer-aided generation and lung deposition modeling of nano-scale particle aggregates, Inhal. Toxicol., 29, 160–168, https://doi.org/10.1080/08958378.2017.1329362, 2017.
Sun, J., Liu, L., Xu, L., Wang, Y., Wu, Z., Hu, M., Shi, Z., Li, Y., Zhang, X., Chen, J., and Li, W.: Key Role of Nitrate in Phase Transitions of Urban Particles: Implications of Important Reactive Surfaces for Secondary Aerosol Formation, J. Geophys. Res.-Atmos., 123, 1234–1243, https://doi.org/10.1002/2017jd027264, 2018.
Tavakoli, F. and Olfert, J. S.: An Instrument for the Classification of Aerosols by Particle Relaxation Time: Theoretical Models of the Aerodynamic Aerosol Classifier, Aerosol Sci. Tech., 47, 916–926, https://doi.org/10.1080/02786826.2013.802761, 2013.
Tavakoli, F. and Olfert, J. S.: Determination of particle mass, effective density, mass–mobility exponent, and dynamic shape factor using an aerodynamic aerosol classifier and a differential mobility analyzer in tandem, J. Aerosol Sci., 75, 35–42, https://doi.org/10.1016/j.jaerosci.2014.04.010, 2014.
Taylor, J. W., Allan, J. D., Allen, G., Coe, H., Williams, P. I., Flynn, M. J., Le Breton, M., Muller, J. B. A., Percival, C. J., Oram, D., Forster, G., Lee, J. D., Rickard, A. R., Parrington, M., and Palmer, P. I.: Size-dependent wet removal of black carbon in Canadian biomass burning plumes, Atmos. Chem. Phys., 14, 13755–13771, https://doi.org/10.5194/acp-14-13755-2014, 2014.
Vu, T. V., Zauli-Sajani, S., Poluzzi, V., and Harrison, R. M.: Factors controlling the lung dose of road traffic-generated sub-micrometre aerosols from outdoor to indoor environments, Air Qual. Atmos. Hlth., 11, 615–625, https://doi.org/10.1007/s11869-018-0568-2, 2018.
Wang, J., Liu, D., Ge, X., Wu, Y., Shen, F., Chen, M., Zhao, J., Xie, C., Wang, Q., Xu, W., Zhang, J., Hu, J., Allan, J., Joshi, R., Fu, P., Coe, H., and Sun, Y.: Characterization of black carbon-containing fine particles in Beijing during wintertime, Atmos. Chem. Phys., 19, 447–458, https://doi.org/10.5194/acp-19-447-2019, 2019.
Wang, J., Ye, J., Liu, D., Wu, Y., Zhao, J., Xu, W., Xie, C., Shen, F., Zhang, J., Ohno, P. E., Qin, Y., Zhao, X., Martin, S. T., Lee, A. K. Y., Fu, P., Jacob, D. J., Zhang, Q., Sun, Y., Chen, M., and Ge, X.: Characterization of submicron organic particles in Beijing during summertime: comparison between SP-AMS and HR-AMS, Atmos. Chem. Phys., 20, 14091–14102, https://doi.org/10.5194/acp-20-14091-2020, 2020.
Wang, L., Zhang, F., Pilot, E., Yu, J., Nie, C., Holdaway, J., Yang, L., Li, Y., Wang, W., Vardoulakis, S., and Krafft, T.: Taking Action on Air Pollution Control in the Beijing-Tianjin-Hebei (BTH) Region: Progress, Challenges and Opportunities, Int. J. Env. Res. Pub. He., 15, 306, https://doi.org/10.3390/ijerph15020306, 2018.
West, J. J., Cohen, A., Dentener, F., Brunekreef, B., Zhu, T., Armstrong, B., Bell, M. L., Brauer, M., Carmichael, G., Costa, D. L., Dockery, D. W., Kleeman, M., Krzyzanowski, M., Künzli, N., Liousse, C., Lung, S.-C. C., Martin, R. V., Pöschl, U., Pope, C. A., Roberts, J. M., Russell, A. G., and Wiedinmyer, C.: What We Breathe Impacts Our Health: Improving Understanding of the Link between Air Pollution and Health, Environ. Sci. Technol., 50, 4895–4904, https://doi.org/10.1021/acs.est.5b03827, 2016.
Wu, Q. Z., Wang, Z. F., Gbaguidi, A., Gao, C., Li, L. N., and Wang, W.: A numerical study of contributions to air pollution in Beijing during CAREBeijing-2006, Atmos. Chem. Phys., 11, 5997–6011, https://doi.org/10.5194/acp-11-5997-2011, 2011.
Wu, Y., Zhang, R., Tian, P., Tao, J., Hsu, S. C., Yan, P., Wang, Q., Cao, J., Zhang, X., and Xia, X.: Effect of ambient humidity on the light absorption amplification of black carbon in Beijing during January 2013, Atmos. Environ., 124, 217–223, https://doi.org/10.1016/j.atmosenv.2015.04.041, 2016.
Wu, Y., Liu, D., Wang, J., Shen, F., Chen, Y., Cui, S., Ge, S., Wu, Y., Chen, M., and Ge, X.: Characterization of Size-Resolved Hygroscopicity of Black Carbon-Containing Particle in Urban Environment, Environ. Sci. Technol., 53, 14212–14221, https://doi.org/10.1021/acs.est.9b05546, 2019.
Wu, Z., Zheng, J., Wang, Y., Shang, D., Du, Z., Zhang, Y., and Hu, M.: Chemical and physical properties of biomass burning aerosols and their CCN activity: A case study in Beijing, China, Sci. Total Environ., 579, 1260–1268, https://doi.org/10.1016/j.scitotenv.2016.11.112, 2017.
Xing, Y.-F., Xu, Y.-H., Shi, M.-H., and Lian, Y.-X.: The impact of PM2.5 on the human respiratory system, J. Thorac. Dis., 8, E69–E74, https://doi.org/10.3978/j.issn.2072-1439.2016.01.19, 2016.
Xu, Q., Li, X., Wang, S., Wang, C., Huang, F., Gao, Q., Wu, L., Tao, L., Guo, J., Wang, W., and Guo, X.: Fine Particulate Air Pollution and Hospital Emergency Room Visits for Respiratory Disease in Urban Areas in Beijing, China, in 2013, PLOS ONE, 11, e0153099, https://doi.org/10.1371/journal.pone.0153099, 2016.
Xu, W., Sun, Y., Wang, Q., Du, W., Zhao, J., Ge, X., Han, T., Zhang, Y., Zhou, W., Li, J., Fu, P., Wang, Z., and Worsnop, D. R.: Seasonal Characterization of Organic Nitrogen in Atmospheric Aerosols Using High Resolution Aerosol Mass Spectrometry in Beijing, China, ACS Earth and Space Chemistry, 1, 673–682, https://doi.org/10.1021/acsearthspacechem.7b00106, 2017.
Yu, C., Liu, D., Broda, K., Joshi, R., Olfert, J., Sun, Y., Fu, P., Coe, H., and Allan, J. D.: Characterising mass-resolved mixing state of black carbon in Beijing using a morphology-independent measurement method, Atmos. Chem. Phys., 20, 3645–3661, https://doi.org/10.5194/acp-20-3645-2020, 2020.
Zhang, G., Lin, Q., Peng, L., Bi, X., Chen, D., Li, M., Li, L., Brechtel, F. J., Chen, J., Yan, W., Wang, X., Peng, P., Sheng, G., and Zhou, Z.: The single-particle mixing state and cloud scavenging of black carbon: a case study at a high-altitude mountain site in southern China, Atmos. Chem. Phys., 17, 14975–14985, https://doi.org/10.5194/acp-17-14975-2017, 2017.
Zhang, J. K., Sun, Y., Liu, Z. R., Ji, D. S., Hu, B., Liu, Q., and Wang, Y. S.: Characterization of submicron aerosols during a month of serious pollution in Beijing, 2013, Atmos. Chem. Phys., 14, 2887–2903, https://doi.org/10.5194/acp-14-2887-2014, 2014.
Zhang, Q., He, K., and Huo, H.: Cleaning China's air, Nature, 484, 161–162, https://doi.org/10.1038/484161a, 2012.
Zhang, X. Y., Wang, J. Z., Wang, Y. Q., Liu, H. L., Sun, J. Y., and Zhang, Y. M.: Changes in chemical components of aerosol particles in different haze regions in China from 2006 to 2013 and contribution of meteorological factors, Atmos. Chem. Phys., 15, 12935–12952, https://doi.org/10.5194/acp-15-12935-2015, 2015.
Zhang, Y., Lang, J., Cheng, S., Li, S., Zhou, Y., Chen, D., Zhang, H., and Wang, H.: Chemical composition and sources of PM1 and PM2.5 in Beijing in autumn, Sci. Total Environ., 630, 72–82, https://doi.org/10.1016/j.scitotenv.2018.02.151, 2018.
Zhang, Y., Zhang, Q., Yao, Z., and Li, H.: Particle Size and Mixing State of Freshly Emitted Black Carbon from Different Combustion Sources in China, Environ. Sci. Technol., 54, 7766–7774, https://doi.org/10.1021/acs.est.9b07373, 2020.