the Creative Commons Attribution 4.0 License.
the Creative Commons Attribution 4.0 License.
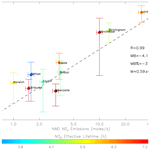
Exploiting satellite measurements to explore uncertainties in UK bottom-up NOx emission estimates
Richard J. Pope
Rebecca Kelly
Eloise A. Marais
Ailish M. Graham
Chris Wilson
Jeremy J. Harrison
Savio J. A. Moniz
Mohamed Ghalaieny
Steve R. Arnold
Martyn P. Chipperfield
Nitrogen oxides (NOx, NO + NO2) are potent air pollutants which directly impact on human health and which aid the formation of other hazardous pollutants such as ozone (O3) and particulate matter. In this study, we use satellite tropospheric column nitrogen dioxide (TCNO2) data to evaluate the spatiotemporal variability and magnitude of the United Kingdom (UK) bottom-up National Atmospheric Emissions Inventory (NAEI) NOx emissions. Although emissions and TCNO2 represent different quantities, for UK city sources we find a spatial correlation of ∼0.5 between the NAEI NOx emissions and TCNO2 from the high-spatial-resolution TROPOspheric Monitoring Instrument (TROPOMI), suggesting a good spatial distribution of emission sources in the inventory. Between 2005 and 2015, the NAEI total UK NOx emissions and long-term TCNO2 record from the Ozone Monitoring Instrument (OMI), averaged over England, show annually decreasing trends of 4.4 % and 2.2 %, respectively. Top-down NOx emissions were derived in this study by applying a simple mass balance approach to TROPOMI-observed downwind NO2 plumes from city sources. Overall, these top-down estimates were consistent with the NAEI, but for larger cities such as London and Birmingham the inventory is significantly (>25 %) less than the top-down emissions.
- Article
(4867 KB) - Full-text XML
- BibTeX
- EndNote
Poor air quality (AQ) can have a substantial impact on human health, increasing risk of ailments such as asthma, cancer, diabetes and heart disease (Royal College of Physicians, 2016). A key air pollutant is nitrogen dioxide (NO2), which was responsible for approximately 9600 premature deaths from long-term exposure in the United Kingdom (UK) in 2015 (EEA, 2018). NO2 is also a precursor to tropospheric ozone and nitrate aerosol in the UK (DEFRA, 2018a). Legislation (e.g. the EU directive 2008/50/EC Ambient AQ regulation; DEFRA, 2018a) is in place to reduce concentrations of NO2 and other pollutants. However, many regions in the UK (33 out of 43 in 2019; DEFRA, 2020) still fail to meet the annual mean NO2 limit of 40 µg m−3 (WHO, 2018). To meet the UK's statutory reporting requirements and to help inform policy, the Department for Environment, Food and Rural Affairs (DEFRA) uses the National Atmospheric Emissions Inventory (NAEI) (NAEI, 2021a). However, like all emission inventories, the NAEI is subject to uncertainties which are difficult to quantify. These uncertainties include unreported sources, diffuse sources such as agriculture, and the use of proxy data (e.g. population or housing density data) to distribute emissions and updates to the NAEI methodologies between years (NAEI, 2017). In addition, the NAEI only includes emissions from anthropogenic sources. Spatial verification of the NAEI AQ emissions, until recently (Tsagatakis et al., 2021), has been restricted to comparisons with surface sites, which have limited and disproportional spatial coverage. The NAEI is also used to drive regional models (e.g. the UK Met Office Air Quality in the Unified Model (AQUM, Savage et al., 2013), which provides the official national AQ forecasts), land use regression models (e.g. Wu et al., 2017), and pollutant climate mapping (PCM) models (e.g. Dibbens and Clemens, 2015), where uncertainties in the emissions can then feed into the simulated AQ predictions and resultant public health advisories.
Satellite measurements of tropospheric column NO2 (TCNO2) have frequently been used to derive top-down emissions of nitrogen oxides (NOx=nitric oxide (NO) + NO2), which can be used to evaluate bottom-up inventories. Some studies have used statistical fitting of observed downwind plumes of TCNO2 from anthropogenic sources (e.g. Beirle et al., 2011; Liu et al., 2016; Verstraeten et al., 2018), while others have used complex atmospheric chemistry models deploying approaches such as data assimilation (e.g. Miyazaki et al., 2016), mass balance (Martin et al., 2003), and model sensitivity experiments (e.g. Potts et al., 2021).
While model-derived estimates of NOx emissions (e.g. from data assimilation) are robust, the methodology is computationally expensive and time-intensive. Therefore, the statistical fitting to downwind plumes approach is a more achievable approach to derive top-down emissions, especially for government departments and agencies. Beirle et al. (2011) presented one of the first studies to use statistical fitting to downwind plumes for Riyadh, Saudi Arabia. The method was also applied to multiple megacities and compared with the bottom-up Environmental Database for Global Atmospheric Research (EDGAR) emission inventory (version 4.1). Verstraeten et al. (2018) used a similar, but modified, approach of simple mass balance which assumes that the observed total mass of NO2 is a product of the emission rate and the effective lifetime. The assumption is that the removal of NO2 can be described by a first-order loss (i.e. the chemical decay of NO2 follows an exponential decay function with an e-folding time and therefore distance from the source).
In this study, we use satellite TCNO2 records to evaluate the spatial distribution and temporal evolution of the NAEI. In the past, and still presently, this is a challenge given the climatological meteorological conditions (i.e. frequent frontal systems with widespread precipitation and cloud cover; Pena-Angulo et al., 2020) experienced in the UK. Frequent cloud cover means that satellite instruments are severely restricted in their ability to retrieve information on trace gases and aerosols through the atmosphere (i.e. retrievals only between the top of atmosphere and cloud top). Therefore, the lack of robust observations makes it more difficult to clearly resolve large emission sources from space. Also, previous sensors (e.g. the Ozone Monitoring Instrument, OMI) have had relatively coarse horizontal spatial resolutions (in the order of 10–100 km) which are larger than most UK emissions sources. However, this work represents the first attempt to derive UK city-scale NOx emissions from the new state-of-the-art TROPOspheric Monitoring Instrument (TROPOMI), which has unparalleled spatial resolution in comparison to previous sensors (e.g. OMI). We apply a similar approach to Verstraeten et al. (2018) but determine the background NO2 value and e-folding distance in different ways to derive top-down NOx emission estimates of UK cities and thereby directly evaluate the NAEI estimates. Therefore, we can derive NOx emissions from previously undetectable sources (e.g. Manchester and Birmingham). From here on, we refer to this methodology as the simple mass balance approach (SMBA). The satellite observations used, NAEI and SMBA, are described in Sect. 2, the results presented in Sect. 3, and our conclusions discussed in Sect. 4.
2.1 NAEI emissions
The NAEI is the official UK bottom-up inventory of primary sources of emissions, used for statutory reporting and national air quality policy and driving regional air quality models (NAEI, 2021a). The contract to deliver the NAEI is led by a consortium managed by Ricardo Energy and Environment for the UK Department for Business, Energy and Industrial Strategy (BEIS) and DEFRA. The NAEI is compiled on an annual basis according to internationally agreed methodologies (EMEP/EEA, 2019), encompassing sectors ranging from transport and industry through to agriculture and domestic sources (Ricardo Energy and Environment, 2021). Here, we use the NAEI emissions from 2019, which is the most recent version publicly available.
2.2 Satellite data
OMI and TROPOMI are both nadir-viewing instruments on board the NASA Aura and ESA Sentinel 5 – Precursor (S5P) polar-orbiting satellites, respectively, and have local overpass times of 13:30 local time (LT). TROPOMI measures in the ultraviolet-visible (UV-Vis, 270–500 nm), similarly to OMI (Boersma et al., 2007), as well as near-infrared (NIR, 675–775 nm) and short-wave infrared (SWIR, 2305–2385 nm) spectral ranges (Veefkind et al., 2012). TROPOMI and OMI have nadir pixel sizes of 3.5 km × 5.5 km (in the UV-Vis, 7.0 km × 7.0 km for other spectral ranges) and 13 km × 24 km, respectively. The OMI (DOMINO version 2 product) and TROPOMI (TM5-MP-DOMINO version 1.2/3x – OFFLINE product) data were downloaded from the Tropospheric Emissions Monitoring Internet Service (TEMIS) for January 2005 to December 2015 and February 2018 to January 2020, respectively. Given the issues with large cloud cover in the UK, we use 2 years of TROPOMI TCNO2 data to help increase the spatiotemporal sample size when deriving top-down emissions to evaluate the 2019 NAEI NOx emissions. The OMI row anomaly first occurred in 2008 (Torres et al., 2018) and over time has progressively had a detrimental impact on retrieved TCNO2. The study by Pope et al. (2018) successfully used the OMI record to look at long-term trends in UK TCNO2. However, after 2015, while still retrieving robust signals over source regions, the row anomaly appears to be substantially artificially enhancing background TCNO2. Therefore, as we consider regional trends in TCNO2 in Sect. 3.2, we did not use OMI TCNO2 after 2015. The data have been processed using the methodology of Pope et al. (2018) to map the TCNO2 data onto a high-resolution spatial grid (0.025∘ × 0.025∘, ∼2–3 km –3 km for TROPOMI, , ∼5 km km for OMI). The TROPOMI data were quality controlled for a cloud radiance fraction <0.5, a quality control flag >0.75, and where the TCNO2 value was mol m−2 (i.e. random values round 0.0 may be slightly negative or positive, so we filter for TCNO mol m−2; otherwise, a positive bias in average TCNO2 is imposed). While TROPOMI provides the greatest spatial resolution of any satellite instrument to measure air pollutants, suitable for deriving TCNO2 emission estimates over UK city-scale sources, the retrieved TCNO2 has been shown to have a low bias. Over north-western Europe, Verhoelst et al. (2021) found that TROPOMI underestimated TCNO2 by approximately 20 %–30 % when compared with surface TCNO2 measurements, which is consistent with Chan et al. (2020) and Dimitropoulou et al. (2020). OMI data were processed for a geometric cloud fraction of <0.2, quality flag =0 (which also flags pixels influenced by the row anomaly; Braak, 2010), and TCNO mol m2.
2.3 Simplified mass balance approach
To derive top-down emissions of NOx, we use the SMBA, which is based on downwind plumes of TROPOMI-observed TCNO2 from the target source, where the observed total mass of NO2 (i.e. the source-related enhancement of TCNO2 above the background level) is assumed to be a product of the emission rate and the effective lifetime. Therefore, we can derive the NOx emission rate based on Eq. (1):
where E is the emission rate (mol s−1), NO2 LD is the NO2 line density (mol m−1), BLD is the background NO2 line density value (mol m−1), Δd is the grid box length (m), i is the grid box number between the source and background value, t is time (s) and is the e-folding loss term with τ as the effective lifetime. N represents the number of satellite TCNO2 grid boxes between the source and background level B. t is calculated as the distance between the source and B divided by the wind speed (ws). To derive the full NO2 loading emitted from the source, the wind flow NO2 LD has the background NO2 LD (i.e. BLD) value subtracted from all points between the source and B and each grid box is multiplied by its grid box distance and is then summed, yielding the total NO2 mass (mol). f is the factor required to convert to NOx emissions.
The ws and wind direction at a particular source are determined from the European Centre for Medium-Range Weather Forecasts (ECMWF, 2021) ERA5 u- and v-wind component data. The wind data are sampled at 13:00 UTC (around 13:00 LT over the UK) to coincide with the TROPOMI overpass (i.e. 13:30 LT) and averaged across boundary layer pressure levels (i.e. surface to 900 hPa). In all cases, the ws had to be greater than 2 m s−1 to avoid near-stable meteorological conditions. Wind data are only used on days where there are TROPOMI NO2 data available downwind of the target source when deriving the average directional wind speed. Studies such as Beirle et al. (2011) and Verstraeten et al. (2018) averaged the wind speeds over the surface to 500 m layer. Beirle et al. (2011) suggested that the average winds across this altitude range yielded uncertainties of approximately 30 %, but neither study provided definitive reasoning why 500 m was selected. In the UK, 500 m is approximately 950 hPa, which sits comfortably within the boundary layer (approximately 1000 m or 880.0 to 910 hPa in Fig. 1a based on ERA-5 data sampled at 13:00 LT and averaged for 2019). In this study, we argue that wind speeds throughout the boundary layer are likely to be important in controlling the spatial distribution of NO2 downwind of sources. Figure 1b shows the zonally averaged latitude–pressure NO2 profile from the Copernicus Atmosphere Monitoring Service (CAMS, 2021), sampled at 13:00 LT and averaged for 2019, over the UK. The bulk of the NO2 loading is near the surface, with NO2 concentrations of 0.5 to >1.0 ppbv between the surface and 900 hPa. As shown by the white dashed lines, 60 %–70 % of the surface to 500 hPa NO2 loading exists between the surface and 900 hPa. The zonally averaged boundary layer pressure (red dashed line) also straddles the 900 hPa level. In Fig. 1c, the wind speed profile for London sampled under westerly flow increases with altitude until between 925 and 900 hPa. For each pressure level, London westerly days are defined based average u and v components between the surface and the respective pressure level. As shown by the blue text, the wind speed gradient with respect to pressure substantially decreases (i.e. from −0.0406 m s−1 hPa−1 between 950 and 925 hPa to −0.0045 m s−1 hPa−1 between 925 and 900 hPa) at 900 hPa. Therefore, this profile gradient and the information in Fig. 1a and b suggest that 900 hPa is a suitable level for deriving the boundary layer average wind speed and flow direction. The table (panel d) in Fig. 1 shows the sensitivity of the NOx emission parameters to the pressure layer used. The derivation of emissions is discussed further in this section. The surface-850 hPa average and surface-only winds show substantially different NOx emission rates of 61.6 and 30.1 mol s−1, respectively. However, the intermediate levels (900 and 950 hPa) show less dramatic step changes with emission rates of 55.2 and 49.8 mol s−1. Therefore, the surface-900 hPa layer is used to help derive NOx emission rates in this study.
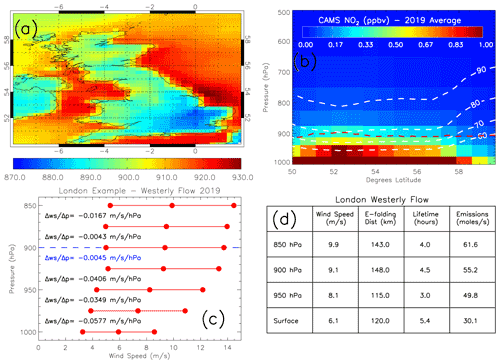
Figure 1(a) ERA-5 UK boundary layer pressure (hPa) sampled at 13:00 LT (to coincide with the TROPOMI overpass time) and averaged for 2019. (b) CAMS reanalysis zonal (8.0∘ W–2.0∘ E) average latitude–pressure NO2 (ppbv) cross section over the UK between the surface and 500 hPa. White dashed lines represent the percentage of the surface-500 hPa NO2 loading between the surface and the respective pressure levels. The red dashed line represents the zonal average boundary layer pressure (hPa). (c) Average (surface to pressure level) wind speed (m s−1), ± the standard deviation, profile over London under westerly flow (determined from the ERA-5 u-wind and v-wind components at each pressure level). is the wind speed gradient between pressure levels. The blue text indicates the first small step change in the gradient indicative of reduced flow turbulence and a suitable surface-altitude range to average the winds speeds over. (d) The table shows the impact on the NOx emission parameters when using different altitudes over which to average the wind speeds.
The NO2 LD is the product of the source width, which is perpendicular to the wind flow, and the source-width-average TCNO2 (i.e. for each downwind grid box from the source, the corresponding perpendicular rows between the source edges are averaged together) profile downwind from the source on a grid box by grid box basis as shown in Eq. (2).
where NO2 LD (mol m−1) is the NO2 line density, i is the grid box index downwind of the source starting at i=1 going to i=N at background point B, TCNO2 is the tropospheric column NO2 grid box value (mol m−2) at point i and j is the grid box index for the number of grid boxes n, perpendicular to the downwind profile, which fit across the width of the source at grid box i downwind, and w is the source width (m) (i.e. source width perpendicular to the downwind profile) of the NO2 source. Though the source width and length are subjective choices between the source edge locations, the same source width and length values are used when deriving the TROPOMI NOx emissions and summing up the NAEI NOx emissions over the source region. As the source emissions will be a function of the source width (i.e. larger at the source centre and lower at the source edge), the mean TCNO2 downwind profile (i.e. rows averaged across the source width) is representative of the source-average NO2 emission.
Figure 2a shows the difference between TROPOMI TCNO2 sampled under westerly flow and the long-term average based on London u- and v-wind components, where there are clear downwind positive anomalies mol m−2. Similarly, in Fig. 2b, the downwind plume (e.g. westerly flow over London) has typically larger NO2 LD values than the all-flow (i.e. all wind directions) NO2 LD. The full NO2 mass emitted from the source in the NO2 LD is the summation of the wind-flow NO2 LD from the source up to point B minus the background value from all downwind pixels over this profile segment. A reasonable estimate of when the wind-flow NO2 LD reaches B, for more isolated NO2 sources, is when it intersects with the all-flow NO2 LD profile (i.e. returns to normal levels). However, when there are substantial upwind NO2 sources, this can yield wind-flow NO2 LD profiles which never intersect with the all-flow NO2 LD profile within the domain (e.g. see the Birmingham example in Fig. 3a and b). Therefore, to determine when B, in the downwind direction, has been reached, a running t-test was applied to the wind-flow NO2 LD profile to determine where turning points or levelling off occurred. Such a substantial change in the NO2 LD profile gradient is indicative of the background level being reached and/or potentially another source being identified (e.g. in Fig. 2b there is evidence of other NO2 sources downwind of London several hundred kilometres away over continental Europe). As such a test can be sensitive to noise in the TCNO2 data, a 10-pixel (0.5∘) running average wind-flow NO2 LD profile was calculated. This smoothed out the noise from the downwind profile and allowed for the detection of larger-scale NO2 LD changes. The running t-test was applied to this using two windows (i.e. a moving centre point with a window each side of 0.5∘) and the t-test significance between the two window averages determined. This yielded a t-test significance/p-value distance series from the source. When a substantial change in the NO2 LD gradient occurred, the t-test p-values would increase, peak and then drop off. This change in the gradient of the t-test p-values identified the location of any NO2 LD step changes in the profile. The green line in Fig. 2b shows where the t-test p-values peaked and that there are turning points in the wind-flow NO2 LD profile. Such a reduction in the wind-flow NO2 LD profile gradient is suggestive of the plume reaching B as NO2 levels have stabilised. However, in Fig. 2b, there are multiple locations potentially meeting these criteria. In reality, the turning points further downwind of London are sources from the Benelux region. The red dot represents the first instance, after the initial near-source wind-flow NO2 LD peak, where the gradient in the running t-test p-value profile changes sign (i.e. positive to negative or vice versa).
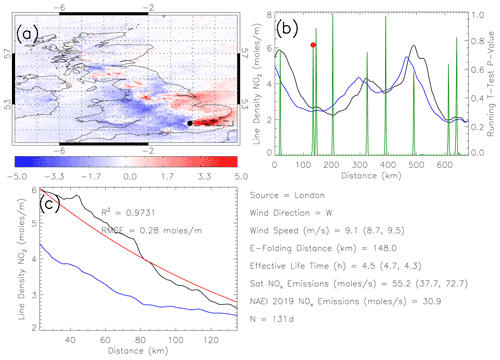
Figure 2(a) TROPOMI TCNO2 (10−5 mol m−2) sub-sampled under westerly flow (defined over London, black dot) minus the long-term average (February 2018 to January 2020). The dashed box represents the width of the source and distance between the source and background. (b) Downwind NO2 LD from London (black: westerly flow, blue: all-flow average) with the corresponding running t-test p-value (green line). The red dot represents the location of the background level determined by the turning point in the running t-test p-value time series. (c) The westerly flow and all-flow NO2 LD between the peak westerly flow NO2 LD value and the background value. The red line represents the e-folding distance fit with the corresponding R2 and root mean square error (RMSE) between the westerly flow NO2 LD and fit profile. N represents the number of days classified under westerly flow over London.
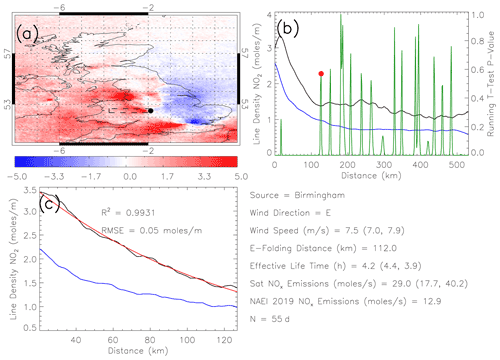
Figure 3(a) TROPOMI TCNO2 (10−5 mol m−2) sub-sampled under easterly flow (defined over Birmingham, black dot) minus the long-term average (February 2018 to January 2020). The dashed box represents the width of the source and distance between the source and background. (b) Downwind NO2 LD from Birmingham (black: easterly flow, blue: all-flow average) with the corresponding running t-test p-value (green line). The red dot represents the location of the background level determined by the turning point in the running t-test p-value time series. (c) The easterly flow and all-flow NO2 LD between the peak easterly flow NO2 LD value and the background value. The red line represents the e-folding distance fit with the corresponding R2 and RMSE between the easterly flow NO2 LD and fit profile. N represents the number of days classified under easterly flow over Birmingham.
The loss term is dependent upon τ and is determined by applying an e-folding distance fit between the near-source peak wind-flow NO2 LD value and B (i.e. we assume this function is valid only between these two points) before dividing by ws to get τ. Here, a range of e-folding distances are tested in the loss term to find the distance value which yields the lowest root mean square error (RMSE) and a large R2 (Pearson correlation coefficient squared) value between the e-folding distance fit (red line, Fig. 2c) and the wind-flow NO2 LD (black line, Fig. 2c). In the case of London, this yielded an e-folding distance of 148.0 km and τ of 4.5 h (4.7 and 4.3 h) based on the average ws=9.1 m s−1 with an uncertainty range (±0.4 m s−1; i.e. ±1-sigma standard error) of 8.7 to 9.5 m s−1 (i.e. a slower/faster wind speed yields a longer/shorter lifetime). The effective lifetime derived here for London and other UK cities is typically consistent with values from other studies (e.g. Beirle et al., 2011, and Verstraeten et al., 2018) for European cities (i.e. 1.0–10.0 h).
The top-down E is calculated from Eq. (1), and this emissions flux of NO2 (mol s−1) is converted to emissions of NOx (mol s−1) using the factor f for comparison with the bottom-up inventories. This is done by scaling the NO2 emissions by 1.32 based on the NO:NO2 concentration ratio (0.32) in urban environments at midday (Seinfeld and Pandis, 2006; Liu et al., 2016). Verstraeten et al. (2018) used modelled NO and NO2 concentrations to derive a scaling more representative of the chemistry of the source. They estimate there is a 10 % uncertainty (similar to Beirle et al., 2011), but as the modelled NO2:NOx ratio is based on the input emissions, for which the satellite data are being used to evaluate, this process is rather circular and not independent.
Here, the top-down NOx emissions are derived by sampling TCNO2 data under different wind directions in all seasons. Several studies, such as Beirle et al. (2011), have gone a step further and used TCNO2 data to derive seasonal emissions. Unfortunately, here we are restricted to looking at annually derived emissions due to (1) the TROPOMI TCNO2 record only starting in February 2018, (2) the COVID-19 pandemic resulting in a dramatic reduction in UK (and global) NOx emissions (Potts et al., 2021), meaning TCNO2 data beyond February 2020 could not be used to derive top-down emissions under normal conditions, and (3) the UK being subject to frequently cloudy conditions yielding a reduction in the number of observations from TROPOMI. The latter point predominantly influences TROPOMI retrievals in the winter-time. Therefore, even though we sample TCNO2 data in all seasons, there is likely to be a tendency towards summer-time TCNO2 values, when TCNO2 values tend to be lower (e.g. Pope et al., 2015), potentially leading to a low bias in the derived top-down NOx emissions.
To investigate the total errors in the derived NOx emissions from TROPOMI, we have included errors from all the input terms. These include the enhancement in the TNCO2 data, the e-folding distance xo, the wind speed ws, the source width w, the NO2-to-NOx conversion factor f and the distance d between the source and B. When combined, this yields the total error in Eq. (3).
In the total error expression, we have set , where is the average TCNO2 value (mol m−2) for all grid cells between the source and B (i.e. background TCNO2 value) in the downwind profile. Here, we take to be a suitable estimate of the full NO2 emission loading from the source (i.e. the numerator of Eq. 1). Regarding the errors (i.e. terms with Δ in front), based on Beirle et al. (2011), we assign errors of 10 % to f and w. As xo and d are distance metrics as well, with no clear way to quantify the errors in these terms, we have assigned them with 10 % errors also. The ws error is based on the standard error in the sample (i.e. the number of days selected for each flow regime). For the enhancement in TCNO2 from the source (i.e. ϕ), we have conservatively taken the largest precision error value from all TCNO2 values between the source and B, which forms .
3.1 NOx sources
Surface emissions and observed TCNO2 represent different quantities and are influenced by different processes. However, the short NO2 lifetime of a few hours (Schaub et al., 2007; Pope et al., 2015) means there is a sharp gradient between sources and the background levels. Therefore, we can use the satellite TCNO2 observations to provide some constraint on the spatial distribution of the NOx emissions. In Fig. 4, spatial maps over south-eastern (Fig. 4a and c) and northern England (Fig. 4b and d) show evidence of co-located TCNO2 and NOx emission hotspots, especially over many of the UK cities shown by circles. Here, both data sets have been mapped onto the spatial resolution of . In south-eastern England, TCNO2 and NOx emissions peak over London at over mol m−2 and approximately >2.0 µg m−2 s−1, respectively. A secondary peak is also observed over western London for both quantities at similar levels. There are further co-located hotspots over Southampton (TCNO2 ∼ 8.0– mol m−2, NOx > 2.0 µg m−2 s−1), Portsmouth (TCNO2 ∼ 6.0–7.0 × 10−5 mol m−2, NOx ∼ 1.0–1.5 µg m−2 s−1), Brighton (TCNO2 ∼ 5.0– mol m−2, NOx∼0.5–0.8 µg m−2 s−1), Oxford (TCNO2∼7.0– mol m−2, NOx∼0.7–1.0 µg m−2 s−1) and Chelmsford (TCNO2∼8.5– mol m−2, NOx∼0.5 µg m−2 s−1). In northern England and the Midlands, peak TCNO2 and NOx emissions are located over Manchester (TCNO2∼10.0– mol m−2, NOx∼1.0–1.5 µg m−2 s−1), Birmingham (TCNO2∼8.0– mol m−2, NOx∼1.0–1.5 µg m−2 s−1), Leeds (TCNO2∼8.0– mol m−2, NOx∼1.0–1.5 µg m−2 s−1) and Liverpool (TCNO2∼7.0– mol m−2, NOx∼0.5–1.0 µg m−2 s−1).
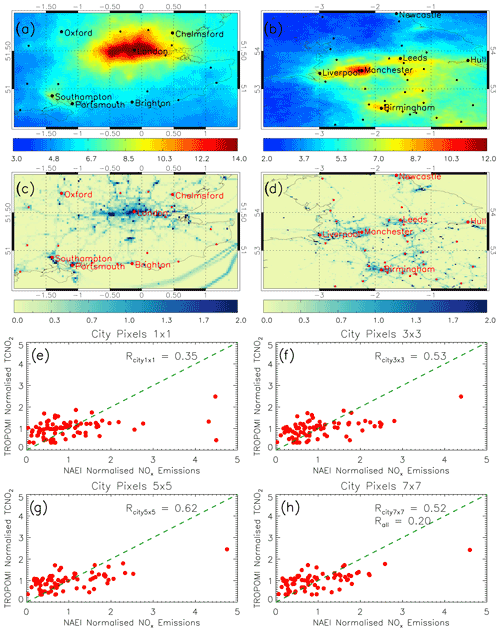
Figure 4TROPOMI TCNO2 ( mol m−2) average for February 2018 to January 2020 across (a) south-eastern and (b) northern England. Black circles represent city locations. NAEI NOx emissions (µg m−2 s−1) for 2019 across (c) south-eastern and (d) northern England. Red circles represent city locations. Panels (e)–(h) represent the correlation of normalised TCNO2 and NOx emissions for UK cities. The green dashed line is the 1:1 line. Each source is normalised by the average of all the sources. The four panels also represent city means using varying pixel ranges around the source (i.e. 1×1, 3×3, 5×5 and 7×7 grid pixels). The correlations between the city-scale normalised NOx emissions and TCNO2 are shown (R).
To quantify the spatial relationship between the TCNO2 and NOx emissions over source regions, the corresponding pixels of both data sets were sub-sampled for each UK city (79 in total), normalised by the sample mean and correlated against each other (red circles, Fig. 4e), which yielded a correlation (i.e. city1×1 represents 1 grid box ×1 grid box or around where the city centre is located). However, as atmospheric NO2 is subject to chemical reactions and meteorological processes (e.g. transport), the signal around source regions is more diluted and the peak TCNO2 not necessarily centred on the source. To allow for that, the spatial resolution of the quantities over each source was degraded, averaging over 3×3 (Fig. 4f), 5×5 (Fig. 4g) and 7×7 (Fig. 4h) grid cells, and the correlation recalculated (e.g. city3×3 represents 3 grid boxes ×3 grid boxes or around where the city centre is located). This resulted in correlations of , and . The correlation for the full domain (i.e. the UK) was Rall=0.20. As expected, the correlation for all grid pixels (e.g. including pixels over the sea) is weak, where long-range transport of NO2 can yield spatial variability in background regions with corresponding zero emission pixels. The Rcity1×1, Rcity3×3, Rcity5×5 and Rcity7×7 correlations were all larger. The largest city-scale correlation was for the Rcity5×5 values, where the spatial variability has been smoothed and is representative of the more diffuse pattern of TCNO2. However, the Rcity7×7 ( or ∼15–20 km ×15–20 km) correlation is lower than the Rcity5×5 value, suggesting that this scale is larger than most UK city sizes. Overall, for all R values, except for Rall, there are statistically significant positive correlations at the 90 % confidence level (CL) or above (>95 % CL for Rcity3×3, Rcity5×5 and Rcity7×7). Therefore, the city-scale emission-satellite correlations provide confidence in the spatial distribution of the NAEI NOx emissions based on the observed satellite TCNO2.
3.2 Satellite NO2 and emission NOx trends
To evaluate the temporal evolution of the NAEI emissions, we use the long-term satellite record of TCNO2 from OMI between 2005 and 2015. Annual total UK emissions of NOx (expressed as NO2 here) from the NAEI start in 1970 and continue to the present day (typically with a lag of approximately 2 years). Annual spatial maps of the NAEI also exist over the same time period. However, while there is a consistent methodology for the UK total estimates, the mapping methodology updates between years (NAEI, 2017). Therefore, instead of performing trends on the maps, we focus on trends in the UK NOx emission totals. For OMI, we have taken a similar broad-scale approach focussing on averaged TCNO2 across England (defined as 3∘ W–2∘ E, 50–54∘ N). We focus on England as the majority of large UK sources with reasonable spatiotemporal coverage are located here and have clearly defined trends over source regions. Pope et al. (2018) showed significantly (at the 95 % CL) decreasing trends over London, Birmingham, Manchester and the Yorkshire power stations of between 1.5 % and 2.3 % per year. OMI measurements can be subject to large uncertainties and variability, so this analysis also investigates trends in a range of OMI TCNO2 percentiles over time. To estimate the annual absolute England total NAEI NOx emissions, we summed the emissions data for England (same geographical definition as for OMI above) from the 2019 NAEI NOx emissions map and imposed the UK total NOx trend on it. Here, we use a simple linear fit which yields an annual decrease in the UK total NOx emission of 4.4 %. The relative rate of change is the same for the England total NOx emissions, but the absolute values are lower than the UK total NOx emissions (Fig. 5a).
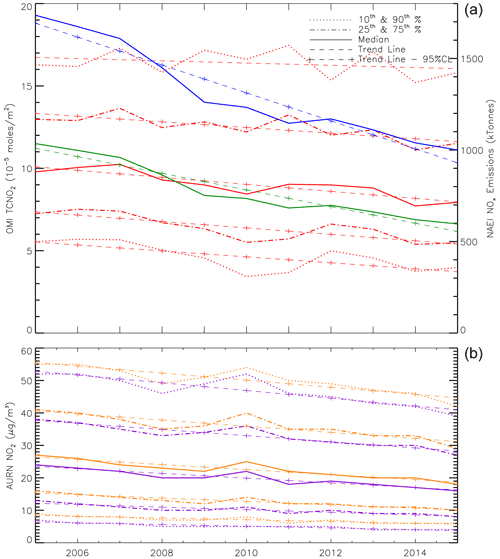
Figure 5Panel (a) shows time series (2005 to 2015) in OMI TCNO2 ( mol m−2) and NAEI NOx emission totals (kt or Gg). OMI median, 10th and 90th and 25th and 75th percentiles are represented by solid, dotted and dot-dashed lines, respectively. NAEI NOx emission totals for the UK and England are represented by the blue and green solid lines. Here, the OMI TCNO2 has been averaged over England (defined as 3∘ W–2∘ E, 50–54∘ N), and while the UK NOx emission totals are directly reported by the NAEI, the England NOx emission totals have been summed over the emissions maps for the same England definition used for OMI (see Sect. 3.2 for more information). In panel (b), AURN surface NO2 (µg m−3) times series are shown for the UK (purple) and England (orange). Trend lines are shown by dashed and dash-crossed lines for insignificant and significant trends (at the 95 % confidence level).
Over the 2005–2015 period, the England average OMI TCNO2 trends in the 10th, 25th, 50th, 75th and 90th percentiles are mol m−2 yr−1 (−3.2 % yr−1), −0.20 × 10−5 mol m−2 yr−1 (−2.7 % yr−1), −0.21 × 10−5 mol m−2 yr−1 (−2.2 % yr−1), −0.17 mol m−2 yr−1 (−1.3 % yr−1) and −0.07 × 10−5 mol m−2 yr−1 (−0.4 % yr−1), respectively (Fig. 5). All of the satellite trends are significant at the 95 % CL, except for the 90th percentile. The UK and England total NOx emission trends between 2005 and 2015 are −76.3 and −45.5 kt yr−1 (both −4.4 % yr−1). The OMI TCNO2 trends range between −3.2 % and −0.4 % depending on the data percentile used to generate the average England TCNO2 annual time series. We also calculated annual trends in UK and England (same definition as above) surface NO2 observations (Fig. 5b) from the Automated Urban and Rural Network (AURN) (AURN, 2021a). Here, we used urban background, suburban and rural sites. For the 10th, 25th, 50th, 75th and 90th percentiles, UK (England) trends are −0.26 (−0.27) µg m−3 yr−1, −0.40 (−0.52) µg m−3 yr−1, −0.73 (−0.77) µg m−3 yr−1, −0.95 (−0.95) µg m−3 yr−1 and −1.19 (−1.09) µg m−3 yr−1. This corresponds to −3.77 % yr−1 (−3.03 % yr−1), −3.07 % yr−1 (−3.24 % yr−1), −3.03 % yr−1 (−2.86 % yr−1), −2.49 % yr−1 (−2.31 % yr−1) and −2.29 % yr−1 (−1.98 % yr−1). Therefore, the NAEI NOx emissions trend is of similar magnitude and direction to that of the observations. The differences are most likely explained by the non-linear conversion of emissions to atmospheric concentrations (i.e. complex meteorology and chemistry). The likely drivers for decreases in UK NOx emissions and NO2 concentrations include a shift to cleaner energy sources (e.g. National Emissions Ceilings Regulations 2018; DEFRA, 2018b), regulations on industrial and power generation emissions (Environmental Permitting Regulations 2016; UK Government, 2016) and tighter emissions for vehicles (e.g. Euro 6 emissions standards). Overall, these results provide confidence in the use of the satellite data as a tool to evaluate bottom-up emission trends.
3.3 Top-down NOx emissions
The top-down NOx emission rate for London under westerly flow (Fig. 2) is 55.2 mol s−1 (37.7, 72.7 mol s−1, i.e. satellite total error range), while the NAEI flux is 30.9 mol s−1. Here, the NAEI has a low bias with the top-down estimate and sits outside the uncertainty range. The top-down emissions are based on 2 years, so the flux should be representative of an annual emission rate, corresponding to the NAEI reporting. In the case of Birmingham (Fig. 3a), under easterly flow, there is a visible plume (i.e. positive differences of 2.0– mol m−2) superimposed on a background enhancement (0.5– mol m−2). As a result, the wind-flow NO2 LD is always larger than the all-flow NO2 LD and never reaches the background level (i.e. zero differences in Fig. 3a) within the domain for which the TROPOMI TCNO2 data have been processed (e.g. there are positive differences in between the source, Birmingham, and the west of the domain, 8∘ W). Therefore, the running t-test methodology is used to determine when the wind-flow NO2 LD reaches a steady background state B, as shown in Fig. 3b. Overall, the NAEI (12.9 mol s−1) underestimates the top-down emissions for Birmingham under easterly flow (29.0 (17.7, 40.2) mol s−1).
Our methodology was applied to 10 city sources where sources had suitable downwind TCNO2 enhancements to derive NO2 LDs and top-down emissions (Fig. 6). A suitable downwind TCNO2 enhancement was subjectively identified when a clear TCNO2 enhancement (i.e. positive anomalies) under a specific wind flow/direction occurred and a realistic lifetime (i.e. in the range of the literature – e.g. Verstraeten et al., 2018) could be derived from the downwind TCNO2 profile of the target source. These are shown in Table 1. Where top-down emissions could be derived for sources over several wind directions, they were averaged together. The TCNO2 response to mesoscale and synoptic weather systems (i.e. large-scale flow) can be seasonally influenced (e.g. Pope et al., 2015), with some wind directions occurring more frequently in certain seasons. Therefore, top-down NOx emission estimates derived from several wind directions for a particular source, though sampled throughout all months, can vary depending on the seasonal influence on the observed TCNO2 for which the wind direction more frequently occurs. The top-down emissions derived here suggest that the NAEI bottom-up emissions for the largest sources such as London and Birmingham are underestimated. The top-down emissions for London and Birmingham are 47.9 (31.2, 64.5) mol s−1 and 22.1 (13.3, 30.9) mol s−1, with corresponding NAEI emissions of 30.9 mol and 12.9 mol s−1, respectively. The NAEI (10.0 mol s−1) also underestimates the emissions for Manchester 20.5 (3.3, 37.7) mol s−1, but the top-down emission uncertainty is large (dominated by the smaller sample size of 29 d and large precision errors in the TCNO2 data) and so sits within its uncertainty range.
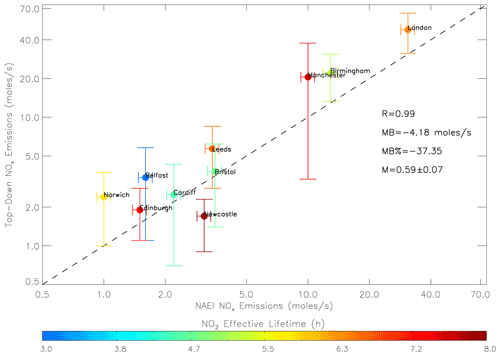
Figure 6NAEI and top-down (TROPOMI) NOx emissions (mol s−1) for 10 UK cities coloured by the NO2 effective lifetime (h). Where there is more than one top-down estimate for a city from multiple wind directions, the corresponding emission rates and lifetimes have been averaged together. The correlation (R), mean bias (MB, mol s−1, i.e. NAEI-top down), percentage mean bias (MB%) and linear fit (M, i.e. top-down vs. NAEI) are also shown. NAEI uncertainty is ±7.8 % (DEFRA, 2018b), and the top-down uncertainty range is based on the satellite errors. The black dashed line represents the 1:1 relationship, and both axes are on log scales.
For the smaller sources (e.g. Edinburgh, Bristol, Cardiff, Leeds, Norwich and Belfast), the comparisons are in better agreement with the NAEI and are located within the top-down emissions uncertainty ranges. However, for Newcastle the NAEI emissions (3.1 mol s−1) are substantially larger than the top-down estimate (1.7 (0.9, 2.3) mol s−1). For the NO2 effective lifetime, we find it ranges between 2.9 and 7.9 h, which is consistent with values in the literature (e.g. Schaub et al., 2007; Pope et al., 2015). For all cities in Fig. 6 there is a strong correlation (0.99) between the NAEI and top-down emission sources investigated here, but the NAEI has a low bias of −4.18 mol s−1 (−37.4 %) on average, dominated by the larger sources (i.e. London and Birmingham). These metrics were calculated in linear space.
We have evaluated relationships between satellite observations (TROPOspheric Monitoring Instrument, TROPOMI) of tropospheric column nitrogen dioxide (TCNO2) and the UK National Atmospheric Emissions Inventory (NAEI) for nitrogen oxides (NO). Although they are different quantities, the short NO2 lifetime means that our comparison can serve as a useful and important tool to evaluate bottom-up emissions. Here, spatial comparison of the TROPOMI TCNO2 with the NAEI highlights consistency over the source regions, with co-located peak values in the respective data sets. Correlation analysis of TCNO2 and NOx emissions over the UK cities indicates moderate spatial agreement, with R ranging between 0.4 and 0.6 (significant at the >90 % confidence level). Analysis of long-term satellite records of TCNO2 (from the Ozone Monitoring Instrument (OMI), 2005–2015) show comparable negative trends with the NAEI NOx emissions with rates of −2.2 % yr−1 and −4.4 % yr−1, respectively. Though the relative NAEI trend is larger than OMI, meteorological conditions and photochemistry will control the atmospheric response to a change in NOx emissions, as seen by OMI. It is also possible that the NAEI overestimates the decreasing NOx emissions trend.
We have also used TROPOMI data to derive top-down city-scale estimates of UK NOx emissions. While it can still be challenging to derive emissions from city-scale sources (e.g. frequent cloud cover in the UK), we estimate top-down emissions fluxes (using satellite data between February 2018 and January 2020) for several cities. Most of the city sources show reasonable agreement, but for larger sources like London and Birmingham, the top-down emission values are substantially larger than those in the NAEI for 2019. Overall, as far as we are aware, this study represents the first robust attempt to use satellite observations of TCNO2 to evaluate and constrain the official UK bottom-up NAEI. We find spatial and temporal agreement between the two quantities but find evidence that the NAEI NOx emissions for larger sources (e.g. London) may be too low (i.e. by >25 %), sitting outside the top-down emission uncertainty ranges. To fully understand the discrepancies and the drivers of these NOx emissions differences, further investigation is required.
TROPOMI and OMI tropospheric column NO2 data come from the Tropospheric Emissions Monitoring Internet Service (https://www.temis.nl/airpollution/no2.php, TEMIS, 2021). The bottom-up NOx emissions come from the National Atmospheric Emissions Inventory (https://naei.beis.gov.uk/data/data-selector?view=air-pollutants, NAEI, 2021b), and the point and area sources can be obtained from https://naei.beis.gov.uk/data/map-uk-das?pollutant_id=6&emiss_maps_submit=naei-20210325121854 (NAEI, 2021c). Meteorological wind, temperature and boundary layer height data came from ECMWF (2021) (https://cds.climate.copernicus.eu/cdsapp#!/dataset/reanalysis-era5-pressure-levels?tab=overview). CAMS NO2 data were retrieved from https://ads.atmosphere.copernicus.eu/cdsapp#!/dataset/cams-global-reanalysis-eac4?tab=form (CAMS, 2021). The AURN data were obtained from https://uk-air.defra.gov.uk/networks/network-info?view=aurn (AURN, 2021b).
RJP undertook the research looking at the spatial maps and long-term trends. SJAM and MG provided advice on the use of the NAEI emissions and data access. EAM provided advice on the TROPOMI and OMI TCNO2 data. RJP, RK, CW and AMG worked on the satellite top-down city-scale NOx emission estimates. MPC, SRA and JJH provided input and advice on the results in the manuscript. RJP prepared the manuscript with contributions from all the co-authors.
The contact author has declared that neither they nor their co-authors have any competing interests.
Publisher’s note: Copernicus Publications remains neutral with regard to jurisdictional claims in published maps and institutional affiliations.
This work was funded by the Department for Environment, Food and Rural Affairs through the “Applying Earth Observation (EO) to Reduce Uncertainties in Emission Inventories” project and by the UK Natural Environment Research Council (NERC) by providing funding for the National Centre for Earth Observation (NCEO, award reference NE/R016518/1).
This paper was edited by Andreas Richter and reviewed by Andy Delcloo and two anonymous referees.
AURN (Automated Urban and Rural Network): https://uk-air.defra.gov.uk/networks/network-info?view=aurn, last access: 30 March 2021a.
AURN (Automated Urban and Rural Network): Data Selector, AURN [data set], https://uk-air.defra.gov.uk/data/data_selector, last access: 29 November 2021b.
Beirle, S., Boersma, B. F., Platt, U., Lawrence, M. G., and Wagner, T.: Megacity emissions and lifetimes of nitrogen oxides probed from space, Science, 333, 1737, https://doi.org/10.1126/science.1207824, 2011.
Boersma, K. F., Eskes, H. J., Veefkind, J. P., Brinksma, E. J., van der A, R. J., Sneep, M., van den Oord, G. H. J., Levelt, P. F., Stammes, P., Gleason, J. F., and Bucsela, E. J.: Near-real time retrieval of tropospheric NO2 from OMI, Atmos. Chem. Phys., 7, 2103–2118, https://doi.org/10.5194/acp-7-2103-2007, 2007.
Braak, R.: Row Anomaly Flagging Rules Lookup Table, KNMI Technical Document TN-OMIE-KNMI-950, KNMI, Netherlands, 2010.
CAMS: CAMS global reanalysis (EAC4), Atmosphere Data Store [data set], https://ads.atmosphere.copernicus.eu/cdsapp#!/dataset/cams-global-reanalysis-eac4?tab=form, last access: 29 November 2021.
Chan, K. L., Wiegner, M., van Geffen, J., De Smedt, I., Alberti, C., Cheng, Z., Ye, S., and Wenig, M.: MAX-DOAS measurements of tropospheric NO2 and HCHO in Munich and the comparison to OMI and TROPOMI satellite observations, Atmos. Meas. Tech., 13, 4499–4520, https://doi.org/10.5194/amt-13-4499-2020, 2020.
DEFRA: Air Pollution in the UK 2017, https://uk-air.defra.gov.uk/assets/documents/annualreport/air_pollution_uk_2017_issue_1.pdf (last access: 30 March 2021), 2018a.
DEFRA: UK Informative Inventory Report (1990 to 2016), https://uk-air.defra.gov.uk/library/reports?report_id=956, (last access: 30 March 2021), 2018b.
DEFRA: Air Pollution in the UK 2019, https://uk-air.defra.gov.uk/library/annualreport/viewonline?year=2019_issue_1#report_pdf, (last access: 30 March 2021), 2020.
Dibbens, C. and Clemens, T.: Place of work and residential exposure to ambient air pollution and birth outcomes in Scotland, using geographically fine pollution climate mapping estimates, Environ. Res., 140, 535–541, https://doi.org/10.1016/j.envres.2015.05.010, 2015.
Dimitropoulou, E., Hendrick, F., Pinardi, G., Friedrich, M. M., Merlaud, A., Tack, F., De Longueville, H., Fayt, C., Hermans, C., Laffineur, Q., Fierens, F., and Van Roozendael, M.: Validation of TROPOMI tropospheric NO2 columns using dual-scan multi-axis differential optical absorption spectroscopy (MAX-DOAS) measurements in Uccle, Brussels, Atmos. Meas. Tech., 13, 5165–5191, https://doi.org/10.5194/amt-13-5165-2020, 2020.
ECMWF: ERA5 hourly data on pressure levels from 1979 to present, ECMWF [data set], https://cds.climate.copernicus.eu/cdsapp#!/dataset/reanalysis-era5-pressure-levels?tab=overview, last access: 29 November 2021.
EEA: Air quality in Europe – 2018 report, https://www.eea.europa.eu/publications/air-quality-in-europe-2018 (last access: 30 March 2021), 2018.
EMEP/EAA: EMEP/EEA air pollutant emission inventory guidebook 2019, EEA Report No 13/2019, ISSN 1977-8449, https://www.eea.europa.eu/publications/emep-eea-guidebook-2019 (last access: 2 July 2021), 2019.
Liu, F., Beirle, S., Zhang, Q., Dörner, S., He, K., and Wagner, T.: NOx lifetimes and emissions of cities and power plants in polluted background estimated by satellite observations, Atmos. Chem. Phys., 16, 5283–5298, https://doi.org/10.5194/acp-16-5283-2016, 2016.
Martin, R.V., Jacob, D.J., Chance, K., Kurosu, T.P., Palmer, P.I. and Evans, M.J.: Global inventory of nitrogen oxide emissions constrained by space-based observations of NO2 columns, J. Geophys. Res.-Atmos., 108, 4537, https://doi.org/10.1029/2003JD003453, 2003.
Miyazaki, K., Eskes, H., Sudo, K., Boersma, K. F., Bowman, K., and Kanaya, Y.: Decadal changes in global surface NOx emissions from multi-constituent satellite data assimilation, Atmos. Chem. Phys., 17, 807–837, https://doi.org/10.5194/acp-17-807-2017, 2017.
National Atmospheric Emissions Inventory (NAEI): UK Emission Mapping Methodology – 2015, https://uk-air.defra.gov.uk/assets/documents/reports/cat07/1710261436_Methodology_for_NAEI_2017.pdf (last access: 30 March 2021), 2017.
National Atmospheric Emissions Inventory (NAEI): UK-NAEI – National Atmospheric Emissions Inventory, https://naei.beis.gov.uk/, last access: 30 March 2021a.
National Atmospheric Emissions Inventory (NAEI): UK emissions data selector, NAEI [data set], https://naei.beis.gov.uk/data/data-selector?view=air-pollutants, last access: 29 November 2021b.
National Atmospheric Emissions Inventory (NAEI): Download emissions maps, NAEI [data set], https://naei.beis.gov.uk/data/map-uk-das?pollutant_id=6&emiss_maps_submit=naei-20210325121854, last access: 29 November 2021c.
Pena-Angulo, D., Reig-Gracia, F., Dominguez-Castro, F., Revuelto, J., Aguilar, E., van der Schrier, G. and Vicente-Serrano, S. M.: ECTACI: European Climatology and Trends Atlas of Climate Indices (1979–2017), J. Geophys. Res.-Atmos., 125, e2020JD032798, https://doi.org/10.1029/2020JD032798, 2020.
Pope, R. J., Savage, N. H., Chipperfield, M. P., Ordóñez, C., and Neal, L. S.: The influence of synoptic weather regimes on UK air quality: regional model studies of tropospheric column NO2, Atmos. Chem. Phys., 15, 11201–11215, https://doi.org/10.5194/acp-15-11201-2015, 2015.
Pope, R. J., Arnold, S. R., Chipperfield, M. P., Latter, B. G., Siddans, R., and Kerridge, B. J.: Widespread changes in UK air quality observed from space, Atmos. Sci. Lett., 19, e817, https://doi.org/10.1002/asl.817, 2018.
Potts, D. A., Marais, E. A., Boesch, H., Pope, R. J., Lee, J., Drysdale, W., Chipperfield, M. P., Kerridge, B., and Siddans, R.: Diagnosing air quality changes in the UK during the COVID-19 lockdown using TROPOMI and GEOS-Chem, Environ. Res. Lett., 16, 054031, https://doi.org/10.1088/1748-9326/abde5d, 2021.
Ricardo Energy and Environment: UK Informative Inventory Report (1990 to 2019), https://uk-air.defra.gov.uk/assets/documents/reports/cat09/2103151107_GB_IIR_2021_FINAL.pdf, last access: 2 July 2021.
Royal College of Physicians: Every breath we take: the lifelong impact of air pollution, https://www.rcplondon.ac.uk/projects/outputs/every-breath-we-take-lifelong-impact-air-pollution (last access: 30 March 2021), 2016.
Savage, N. H., Agnew, P., Davis, L. S., Ordóñez, C., Thorpe, R., Johnson, C. E., O'Connor, F. M., and Dalvi, M.: Air quality modelling using the Met Office Unified Model (AQUM OS24-26): model description and initial evaluation, Geosci. Model Dev., 6, 353–372, https://doi.org/10.5194/gmd-6-353-2013, 2013.
Schaub, D., Brunner, D., Boersma, K. F., Keller, J., Folini, D., Buchmann, B., Berresheim, H., and Staehelin, J.: SCIAMACHY tropospheric NO2 over Switzerland: estimates of NOx lifetimes and impact of the complex Alpine topography on the retrieval, Atmos. Chem. Phys., 7, 5971–5987, https://doi.org/10.5194/acp-7-5971-2007, 2007.
Seinfeld, J. and Pandis, S.: Atmospheric Chemistry and Physics: From Air Pollution to Climate Change, 3rd edn., John Wiley and Sons Inc., New Jersey, USA, ISBN 978-1-118-94740-1, 2006.
TEMIS: Tropospheric NO2 from satellites, TEMIS [data set], https://www.temis.nl/airpollution/no2.php, last access: 29 November 2021.
Torres, O., Bhartia, P. K., Jethva, H., and Ahn, C.: Impact of the ozone monitoring instrument row anomaly on the long-term record of aerosol products, Atmos. Meas. Tech., 11, 2701–2715, https://doi.org/10.5194/amt-11-2701-2018, 2018.
Tsagatakis, I., Richardson, J., Evangelides, C., Pizzolato, M., Pearson, B., Passant, N., Pommier, M., and Otto, A.: UK Spatial Emissions Methodology: A report of the National Atmospheric Emission Inventory 2019, https://uk-air.defra.gov.uk/assets/documents/reports/cat09/2107291052_UK_Spatial_Emissions_Methodology_for_NAEI_2019_v1.pdf, last access: 29 November 2021.
UK Government: The Environmental Permitting (England and Wales) Regulations 2016, https://www.legislation.gov.uk/uksi/2016/1154/contents/made (last access: 2 July 2021), 2016.
Veefkind, J. P., Aben, I., McMullan, K., Forster, H., de Vries, J., Otter, G., Claas, J., Eskes, H. J., de Haan, F., Kleipool, Q., van Weele, M., Hasekamp, O., Hoogeveen, R., Landgraf, J., Snel, R., Tol, P., Ingmann, P., Voors, R., Kruizinga, B., Vink, R., Visser, H., and Levelt, P. F.: TROPOMI on the ESA Sentinel-5 Precursor: A GMES mission for global observations of atmospheric composition for climate, air quality and ozone layer applications, Remote Sens. Environ., 120, 70–83, https://doi.org/10.1016/j.rse.2011.09.027, 2012.
Verhoelst, T., Compernolle, S., Pinardi, G., Lambert, J.-C., Eskes, H. J., Eichmann, K.-U., Fjæraa, A. M., Granville, J., Niemeijer, S., Cede, A., Tiefengraber, M., Hendrick, F., Pazmiño, A., Bais, A., Bazureau, A., Boersma, K. F., Bognar, K., Dehn, A., Donner, S., Elokhov, A., Gebetsberger, M., Goutail, F., Grutter de la Mora, M., Gruzdev, A., Gratsea, M., Hansen, G. H., Irie, H., Jepsen, N., Kanaya, Y., Karagkiozidis, D., Kivi, R., Kreher, K., Levelt, P. F., Liu, C., Müller, M., Navarro Comas, M., Piters, A. J. M., Pommereau, J.-P., Portafaix, T., Prados-Roman, C., Puentedura, O., Querel, R., Remmers, J., Richter, A., Rimmer, J., Rivera Cárdenas, C., Saavedra de Miguel, L., Sinyakov, V. P., Stremme, W., Strong, K., Van Roozendael, M., Veefkind, J. P., Wagner, T., Wittrock, F., Yela González, M., and Zehner, C.: Ground-based validation of the Copernicus Sentinel-5P TROPOMI NO2 measurements with the NDACC ZSL-DOAS, MAX-DOAS and Pandonia global networks, Atmos. Meas. Tech., 14, 481–510, https://doi.org/10.5194/amt-14-481-2021, 2021.
Verstraeten, W. W., Boersma, K. F., Douros, J., Williams, J. E., Eskes, H., Liu, F., Beirle, S., and Delcloo, A.: Top-down NOx emissions of European cities based on the downwind plume of modelled and space-borne tropospheric NO2 column, Sensors, 18, 2893, https://doi.org/10.3390/s18092893, 2018.
WHO: Ambient (outdoor) air pollution, https://www.who.int/news-room/fact-sheets/detail/ambient-(outdoor)-air-quality-and-health (last access: 30 March 2021), 2018.
Wu, H., Reis, S., Lin, C., and Heal, M. R.: Effect of monitoring network design on land use regression models for estimating residential NO2 concentration, Atmos. Environ., 149, 24–33, https://doi.org/10.1016/j.atmosenv.2016.11.014, 2017.