the Creative Commons Attribution 4.0 License.
the Creative Commons Attribution 4.0 License.
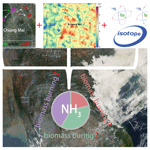
Convergent evidence for the pervasive but limited contribution of biomass burning to atmospheric ammonia in peninsular Southeast Asia
Yunhua Chang
Sawaeng Kawichai
Qian Wang
Martin Van Damme
Lieven Clarisse
Tippawan Prapamontol
Moritz F. Lehmann
Ammonia (NH3) is an important agent involved in atmospheric chemistry and nitrogen cycling. Current estimates of NH3 emissions from biomass burning (BB) differ by more than a factor of 2, impeding a reliable assessment of their environmental consequences. Combining high-resolution satellite observations of NH3 columns with network measurements of the concentration and stable nitrogen isotope composition (δ15N) of NH3, we present coherent estimates of the amount of NH3 derived from BB in the heartland of Southeast Asia, a tropical monsoon environment. Our results reveal a strong variability in atmospheric NH3 levels in time and space across different landscapes. All of the evidence on hand suggests that anthropogenic activities are the most important modulating control with respect to the observed patterns of NH3 distribution in the study area. N-isotope balance considerations revealed that during the intensive fire period, the atmospheric input from BB accounts for no more than 21±5 % (1σ) of the ambient NH3, even at the rural sites and in the proximity of burning areas. Our N-isotope-based assessment of the variation in the relative contribution of BB-derived NH3 is further validated independently through the measurements of particulate K+, a chemical tracer of BB. Our findings underscore that BB-induced NH3 emissions in tropical monsoon environments can be much lower than previously anticipated, with important implications for future modeling studies to better constrain the climate and air quality effects of wildfires.
- Article
(9251 KB) - Full-text XML
-
Supplement
(1162 KB) - BibTeX
- EndNote
Biomass burning (BB) in tropical vegetation regions due to wildfires has been recognized as a globally important source of trace gases (including CO2, CO and ozone precursors) and aerosols (mostly black and organic carbon) (Crutzen and Andreae, 1990; Andreae and Merlet, 2001; Shi et al., 2015; Andreae, 2019; Crutzen et al., 1979). Most BB hot spots occur in West Africa and South America (Crutzen and Andreae, 1990; van der Werf et al., 2006; Shi et al., 2015), but recent studies have also highlighted the importance of Southeast (SE) Asia in this regard, mainly because of the much higher population densities near intensive fire burning areas (Huang et al., 2013; Marlier et al., 2013; Lee et al., 2017; Betha et al., 2014). The climate over large parts of SE Asia is governed by a wet (typically May–July) and dry (typically February–April) season caused by seasonal shifts in the monsoon winds. During the dry season, dry plant materials (e.g., forest, peatland, banana leaf) readily ignite, resulting in large wildfires that can markedly modify the atmospheric composition in the tropics, whereas the tropical rain belt causes plentiful rainfall during summer, preventing such fires during the rainy season (Lee et al., 2017; Chu et al., 2018).
Besides carbon soot, BB also emits large amounts of reactive nitrogen compounds (Lobert et al., 1990; Bauters et al., 2018), in particular ammonia (NH3), which is believed to represent the major source of NH3 during intensive fire periods (Akagi et al., 2011; Whitburn et al., 2015). However, these emissions are subject to large uncertainties (differences of a factor of 2 or greater) (Bray et al., 2018; Whitburn et al., 2015, 2016b; Van Damme et al., 2015b) For example, BB is probably the second most important NH3 source after agriculture, contributing 11 %–23 % of the global burden (Paulot et al., 2017; Bouwman et al., 1997). Minor NH3 sources include fossil fuel burning and biogenic activity (Chang et al., 2012, 2016b, 2019b, 2020). A recent paper also highlighted the underestimated importance of industrial emissions (Van Damme et al., 2018). Once emitted in the atmosphere, NH3 is rapidly removed by dry or wet deposition (Asman et al., 1998). Excess NH3 is known to be responsible for several environmental issues: eutrophication of terrestrial and aquatic ecosystem, soil acidification, and loss of plant diversity (Sutton et al., 2008, 2011; Aneja et al., 2008). In the atmosphere, NH3 can neutralize acid gases (mostly sulfuric acid, nitric acid or hydrochloric acid), resulting in the formation of secondary aerosols that in turn negatively affect climate and human health (Wang et al., 2011, 2013; Paulot and Jacob, 2014; Souri et al., 2017).
To assess the environmental impacts of BB (e.g., air quality and climate change), atmospheric chemistry models incorporating BB-related emissions have widely been used over the past decades (Huang et al., 2013; Aouizerats et al., 2015; Wang et al., 2011, 2013; Souri et al., 2017), but these models are afflicted with a relatively large uncertainty regarding the input parameters used (Hantson et al., 2016; Whitburn et al., 2015; Paulot et al., 2017). The uncertainties, for example, for carbon emissions and for other trace gases (including NH3), can be over 200 % (Whitburn et al., 2015; Paulot et al., 2017; Zhu et al., 2013; Pan et al., 2020). In recent years, hyperspectral sounders on board satellites have demonstrated their capabilities to directly measure tropospheric column concentrations of NH3 gas (Van Damme et al., 2014, 2015b, 2018; Clarisse et al., 2009). Therefore, satellite observations offer a “top-down” alternative to the bottom-up estimates. However, the biggest challenge of satellite-based NH3 assessments is the requirement for the atmosphere to be cloud-free during observations as well as the need for a sizable temperature difference between the land or sea surface and the atmosphere (Van Damme et al., 2015a; Whitburn et al., 2015; Martin, 2008; Streets et al., 2013; Clarisse et al., 2010).
Large uncertainties remain regarding global or regional atmospheric budgets of NH3, and the attribution of emissions to specific sources, emphasizing the need for independent verification methods. An impressive body of previous work has studied the BB influence on the concentration and composition of aerosols in SE Asia (Betha et al., 2014; Aouizerats et al., 2015; Lee et al., 2017; Bikkina et al., 2019). However, to our knowledge, there are no reports on the detailed spatiotemporal patterns of the atmospheric NH3 concentration and nitrogen isotopic composition (δ15N–NH3) associated with BB in this region. Due to isotopic fractionation associated with NH3 production, pyrogenic NH3 displays a distinctly higher δ15N–NH3 (δ15N defined as , where R refers to the ratio in a sample or a standard) than temperature-dependent volatilized sources (Felix et al., 2013; Chang et al., 2016a). The N isotopic analysis of ambient NH3 has been proven to be a useful tool to constrain sources of NH3 emissions in the atmosphere, where both natural and anthropogenic activities are relevant (Chang et al., 2019a, b; Elliott et al., 2019). Here, we integrate high-resolution satellite observations with discrete NH3 concentration measurements and δ15N–NH3 data obtained from a regional passive monitoring network during and after the dry season of large-scale forest fires in the mountain areas of northern Thailand, SE Asia.
2.1 Site description
Surrounded by the mountain ranges of the northern Thailand highlands, the Chiang Mai Province covers an area of approximately 20 107 km2, with a total population of over 1.7 million. Chiang Mai is characterized by a tropical monsoon climate, tempered by the low latitude and moderate elevation, with warm to hot weather year-round. Some 70 % of the area is covered by forests, and 13.4 % of the area is used for agriculture. A continuing environmental issue in Chiang Mai is smoke pollution from wildfires that primarily occur every year towards the end of the dry season between February and April (Tsai et al., 2013) before the relatively cool and rainy season from May on. During the period from March to July 2018, ambient NH3 concentrations and δ15N–NH3 values were determined at nine monitoring stations across the Chiang Mai Province. Figure 1 illustrates the location of sampling sites (with the different land use regimes indicated), Fig. S1 in the Supplement reports meteorological data for Chiang Mai and Table S1 in the Supplement details the information of each station.
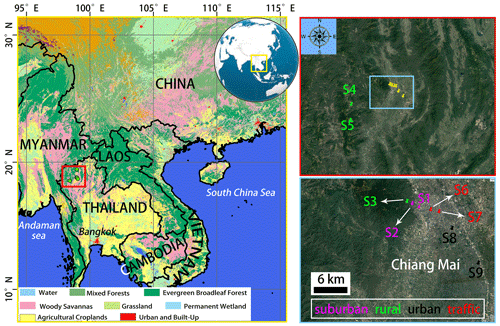
Figure 1Location of sampling sites: a land cover map (left; revised from Chang et al., 2016b) of the area, and zoomed in sections (right) showing the sampling sites. The chosen sampling sites are representative of a gradient in land use from urban to rural. The images on the right were obtained from © Google Maps.
2.2 Sampling and laboratory analysis
In order to obtain information regarding the spatial and temporal variability in NH3 concentrations over Chiang Mai, ambient gas-phase NH3 concentrations at each site were collected weekly using passive sampling devices (PSDs; ALPHA – Adapted Low-cost, Passive High Absorption; Centre for Ecology and Hydrology, Edinburgh, UK) (Chang et al., 2016a). The ALPHA PSD is a circular polyethylene vial (26 mm height, 27 mm diameter) with one open end. The vial holds a 25 mm phosphorous acid-impregnated filter and a polytetrafluoroethylene (PTFE) membrane for gaseous NH3 diffusion. These PSDs have been widely used in Europe, China and the US, and they are capable of detecting NH3 concentrations as low as 0.03 µg m−3 (Chang et al., 2016a; Puchalski et al., 2011; Liu et al., 2013; Tang et al., 2018). In the laboratory, the ALPHA filter samples were soaked in 10 mL deionized water (18 MΩ cm−1) in a 15 mL vial for 30 min with occasional shaking. Concentrations of NH3-derived in extracts were determined using a DionexTM ICS-5000+ system (Thermo Fisher Scientific, Sunnyvale, USA). The IC (ion chromatograph) system was equipped with an automated sampler (AS-DV), an IonPac CG12A guard column and a CS12A separation column. Aqueous methanesulfonic acid (MSA, 30 mM L−1) served as eluent at a flow rate of 1 mL min−1. The isotopic analysis of the extracted was based on the isotopic analysis of nitrous oxides (N2O) after chemical conversion (Liu et al., 2014). More precisely, dissolved in deionized water (DIW) extracts was oxidized to by alkaline hypobromite (BrO−) and then reduced to N2O by hydroxylamine hydrochloride (NH2OH ⋅ HCl). The produced N2O was analyzed using a purge and cryogenic trap system (Gilson GX-271, IsoPrime Ltd., Cheadle Hulme, UK) coupled to an isotope ratio mass spectrometer (PT-IRMS; IsoPrime 100, IsoPrime Ltd., Cheadle Hulme, UK) (Liu et al., 2014). In order to correct for any machine drift and procedural blank contribution, international (IAEA N1, USGS 25 and USGS 26) standards were processed in the same way as samples (Liu et al., 2014). The analytical precision for N isotope analyses was better than 0.5 ‰ (n=5).
2.3 Isotope-based source apportionment
Isotopic mixing models represent valuable tools to estimate the fractional contributions of multiple sources (emission sources of NH3 in this study) within a mixture (the ambient NH3 in this study) (Layman et al., 2012). By explicitly considering the uncertainties associated with the isotopic signatures of single sources and the N isotope fractionation during transformations, the application of Bayesian methods to stable isotope mixing models yields robust probability estimates of source apportionments, and its application to natural systems is more appropriate than the application of simple linear mixing models (Parnell et al., 2010). Here, a novel Bayesian approach using a mixing model, implemented in the SIAR (Stable Isotope Analysis in R) software package, was used to resolve multiple NH3 source categories by generating potential solutions of source apportionment as true probability distributions of the single source contribution to the total NH3 pool. The generation of such source contribution probability distributions allows for the estimation of likelihood ranges of source contributions even under under-constrained conditions (i.e., the number of potential sources exceeds the number of different isotope system parameters + 1). The SIAR package is available for download from the packages section of the Comprehensive R Archive Network site (CRAN; http://cran.r-project.org/, last access: 10 May 2021), which has been widely applied in a number of fields (Chang et al., 2019a, b). The model frame and computing methods are detailed in Sect. S1 in the Supplement.
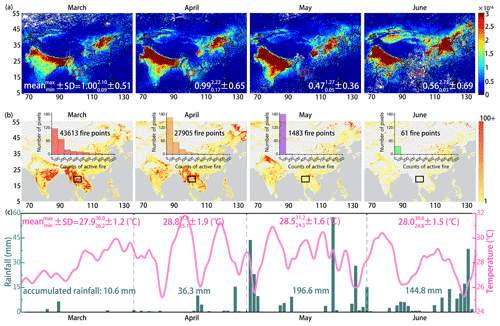
Figure 2(a) Monthly (March–June) spatial distributions of the NH3 total columns (molec. cm−2) in 2018 obtained from satellite measurements by the Infrared Atmospheric Sounding Interferometer (IASI)/MetOp-A instrument in cells. (b) Monthly distributions of gridded counts of active fire pixels ( per cell) derived from the 375 m Visible Infrared Imaging Radiometer Suite (VIIRS). The red and black squares indicate our study area in Chiang Mai. (c) Daily variations in temperature (∘C) and rainfall (mm) in Chiang Mai city.
2.4 Satellite observations of ammonia and fires
NH3 total columns (molec. cm−2) are retrieved from the Infrared Atmospheric Sounding Interferometer (IASI) observations. The IASI instruments are on board the MetOp satellite series; in this work, we use IASI/MetOp-A (launched in 2006) and IASI/MetOp-B (launched in 2012) data. Both instruments have an overpass time of around 09:30 and 21:30 LST (local solar time when crossing the Equator) and, therefore, provide in total a global coverage four times a day. The retrieval strategy, based on artificial neural networks, is fully detailed in previous work (Whitburn et al., 2016a; Van Damme et al., 2017). Here, we only consider morning observations, as they are more sensitive to the lower layer of the atmosphere. Fire radiative power (FRP) from the Moderate Resolution Imaging Spectroradiometer (MODIS) and fire counts derived from the 375 m Visible Infrared Imaging Radiometer Suite (VIIRS) are also used (Li et al., 2020).
3.1 Satellite-observed NH3 distributions
Figure 2a illustrates the monthly spatial distribution of NH3 columns obtained from IASI in 2018 at a spatial resolution of cells. Our study area is set within a large domain of (red and black rectangles in Fig. 2a and b, respectively), in which a total of 260 gridded pixels ( per cell) are used for dividing active fire points (Fig. 2b). Intriguingly, from this plot, one is tempted to conclude that fires do play a very important role in NH3 emissions, as the NH3 columns are much higher in March and April (dry season), which is coincident with a high number of monthly fire activities (indicated by the number of fire points). Further, using 11 years (2008–2018) of IASI satellite data, Fig. S2 presents a climatology of monthly NH3 columns over Chiang Mai at a much finer spatial resolution, which also support the pervasive contribution of BB during dry season (March and April). Based on the average observed temporal distribution of satellite-constrained wildfires, the sampling period in this study can be divided into two contrasting fire-regime periods: the BB season (March and April) and the non-BB season (May and June). Interestingly, however, although the number of fire points in March (43 613 points) is significantly (p<0.01) higher than that in April (27 905 points) (Fig. 2b), the average NH3 column in March is nearly the same as that in April (Fig. 2a). This implies that there is not a one-to-one relationship between BB and NH3 emissions and, in turn, that other sources or factors (e.g., soil dryness, agricultural emissions, precipitation and temperature dependence) must also play a significant role.
Given that the average monthly temperature varies only slightly in contrast to the drastic change in rainfall during our study period (Fig. 2c), it is reasonable to assume that temperature-dependent NH3 volatilization is not the main driver of changes in the NH3 columns. The amount of rainfall, in contrast, can have a multifaceted impact on NH3 emissions. Firstly, there is an obvious link between precipitation rates and the number of wildfires, and, if BB is a major NH3 emission source, we can also expect a relationship between the NH3 columns and monthly rainfall rates. Secondly, and maybe more importantly, rain will dissolve atmospheric particulate and will act to clean the air of NH3, which may partly explain the low NH3 levels during May and June. On the other hand, comparison between March and April reveals higher NH3 levels in April despite higher rain rates, suggesting that processes other than BB and rain-scavenging of BB-derived NH3 must be relevant factors. In Fig. 3a and b, we superimposed the orography at the scale of the study area (Chiang Mai and surrounding mountains) onto the images of the year-long averaged MODIS FRP (fire radiative power) and IASI-NH3 for 2018, respectively. Based on visual evaluation alone, it seems obvious that there is no strong correlation between fire intensity/number of fires and the observed IASI-NH3, suggesting only limited influence of BB on NH3. However, more strikingly, the IASI-NH3 distribution matches that of the population density quite well (Fig. 3c). More precisely, hot spots of atmospheric NH3 (Fig. 3b) appear to be concentrated in urban areas with a dense population. Hence, our satellite remote sensing observations suggest a significant influence of non-BB emissions on NH3 concentrations, seemingly related to urban anthropogenic activities.
3.2 Discrete concentration measurements confirm urban areas as hot spots of NH3 emissions
A total of more than 150 samples were collected in this study for analyzing NH3 concentrations (Fig. 4). The atmospheric NH3 concentrations over Chiang Mai ranged from 2.5 to 46.4 µg m−3, with mean (±1σ) and median values of 14.5 (±9.2) and 11.4 µg m−3, respectively. Consistent with the IASI satellite-based NH3 assessment, the weighted average NH3 concentration (mean) during the dry season (i.e., when wildfires were markedly more abundant) was significantly (p<0.01) higher (20.6 µg m−3) than during the rainy season (10.2 µg m−3). Again, it is tempting to conclude that there is a direct link between higher atmospheric NH3 levels and the higher number of BB events. However, there are several aspects that appear to speak against BB as the main, or only, driver of ambient NH3 concentrations. Firstly, from a global perspective, the ambient NH3 concentrations that we measured in northern Thailand are generally lower than in tropical regions with a dense population or intensive agricultural production (also see Fig. 2a) (Carmichael et al., 2003; Chang et al., 2016b). Secondly, within the study area, large spatial differences in NH3 concentrations were found (Fig. 4). Yet, despite their proximity to wildfires at the time, the three rural sites always displayed the lowest NH3 concentrations (8.3 µg m−3; Fig. 4; see detailed discussion in the next section).
Despite relatively large uncertainties, it is well accepted that, globally, atmospheric NH3 is primarily emitted by agricultural activities and biomass burning (Asman et al., 1998; Bouwman et al., 1997). As a consequence, one would expect the NH3 concentrations in the atmosphere over rural environments with lush vegetation and agricultural land use to be higher than those in (sub)urban areas, where agricultural activities are mostly absent. In our study, the average NH3 concentrations at the nine respective sites are 19.5 µg m−3 (S1; suburban), 11.9 µg m−3 (S2; suburban), 8.8 µg m−3 (S3; rural), 9.0 µg m−3 (S4; rural), 7.0 µg m−3 (S5; rural), 20.2 µg m−3 (S6; urban traffic), 18.1 µg m−3 (S7; urban traffic), 19.6 µg m−3 (S8; urban) and 16.6 µg m−3 (S9; urban) (see also compilation in Fig. 4). Thus, against current paradigms, the observed NH3 concentrations clearly reflect an urban (18.6, n=68) to suburban (, n=34) to rural (8.3, n=51) gradient. Such a concentration gradient can be taken as evidence that nonagricultural activities (including on-road traffic), at least in some regions, can outweigh agriculture and/or BB as the dominant NH3 source in urban areas.
Indeed, a growing body of studies confirm that the urban atmosphere can be a hot spot of NH3 release. Nonagricultural activities, such as wastewater treatment, coal combustion, solid garbage handling, vehicular exhaust and urban green space contribute strongly to urban to NH3 emissions (Chang et al., 2016a, 2015, 2019b; Teng et al., 2017; Li et al., 2016; Sun et al., 2017). For example, high vehicular NH3 emissions from three-way catalysts (TWCs) have been demonstrated in chassis dynamometer vehicle experiments, road tunnel tests and through ambient air measurements (Huang et al., 2018; Chang et al., 2016b, 2019b).
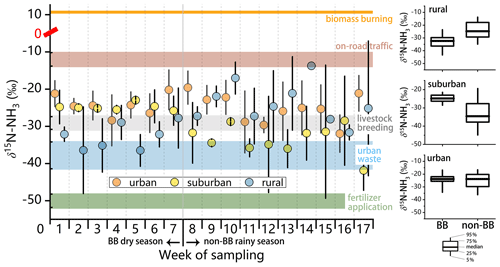
Figure 5The left panel shows weekly variations in the δ15N values (‰) of ambient NH3 measured in urban, suburban and rural environments (setting 0 as the breaking point). The error bars indicate 2 standard deviations. The right panels present box plots of the distribution of δ15N–NH3 during the BB season and non-BB season for each type of sampling site.
3.3 N isotopic constraints on the sources of natural and anthropogenic NH3
The correlative analysis of the spatiotemporal concentration patterns with the variations in land use effects provides the first qualitative constraints with respect to the relative importance of natural/BB and anthropogenic NH3 emissions, but it is insufficient when a more quantitative assessment is required. The N-isotopic composition of NH3 (i.e., δ15N–NH3) can provide help in this regard, as it is sensitive to changes in NH3 sources with distinct isotopic composition (Elliott et al., 2019; Felix et al., 2013). δ15N–NH3 values determined in this study ( ‰, n=145) show a relatively large variability in time and space (Fig. 5). NH3 emitted from the five major NH3 sources displays distinct isotopic signatures (N-fertilizer application, ‰; urban waste volatilized sources, ‰; livestock breeding, ‰; on-road traffic, ‰; biomass burning, 12 ‰) (see colored bars in Fig. 4) (Chang et al., 2016a; Kawashima and Kurahashi, 2011; Chang and Ma, 2016). Thus, the measurement of δ15N–NH3 can be used to distinguish between specific sources and to quantify their contribution to the measured total NH3 pool. (Note that the isotopic signature determined by Kawashima and Kurahashi (2011) is for particulate , not NH3.) As a first step, we examine the spatiotemporal characteristics of the measured δ15N–NH3 relative to the N isotopic source signatures to infer seasonal changes in NH3 sources.
The lowest δ15N–NH3 values were observed at the rural sites (S3–S5) during the dry season ( ‰, n=21). These δ15N values are much lower than the δ15N of BB-related NH3 and indicate the pervasive influence of agricultural NH3 emissions in rural environments, rather than BB. During the rainy season, a drastic increase in δ15N–NH3 ( ‰, n=22) at the rural sites was observed. Again, if BB was the dominating modulator of NH3 levels, an increased contribution from BB-derived NH3 during the dry versus the wet season in rural areas should have resulted in higher, not lower, δ15N–NH3 values. The increased δ15N–NH3 during the non-BB (i.e., rainy) period can probably be explained by the fact that agricultural NH3 emissions with low δ15N–NH3 can be dramatically lowered by continuous and heavy rainfall (Zheng et al., 2018; Chang et al., 2019a) so that at low levels, local sources can become more important (e.g., residential kitchens, nearby burning of biofuels for cooking).
As for the urban sites, the mean δ15N–NH3 values at S6–S9 were ‰, ‰, ‰and ‰, respectively. The overall average δ15N–NH3 value at the four urban sites ( ‰, n=68) was significantly (p<0.01; one-way analysis of variance and paired-sample t test; similarly hereinafter) higher than that at the rural ( ‰, n=43) and suburban ( ‰, n=34) sites, respectively, indicating a greater contribution of NH3 emissions from pyrogenic (e.g., on-road traffic) sources. The average value of urban δ15N–NH3 during the dry season ( ‰, n=28) was very similar to the average value observed during the rainy season ( ‰, n=40), after the pronounced decrease in NH3 concentrations due to wet removal. This rather minor difference can hardly be ascribed to the influence of BB emissions, given the large seasonal fluctuation in wildfire intensity mentioned above. Based on the absolute δ15N–NH3 values in the urban settings, and their rather invariant temporal trends, we argue that vehicle/transport is a more important and apparently steady source of pyrogenic NH3 in the studied urban areas.
The two suburban sites (S1 and S2) are located geographically within the transition zone between the urban and rural environments, and this transitional character seems also indicated by their intermediate δ15N–NH3 values ( ‰, n=34). However, interestingly, in comparison to the urban and rural sites, the overall δ15N–NH3 value for the two suburban sites was significantly (p<0.01) higher during the BB season ( ‰, n=14) than that during non-BB season ( ‰, n=20) (Fig. 5). In fact, among the three different land use regimes, the average δ15N–NH3 was highest for the suburban sites during the BB season, and it was also closer to the NH3 isotopic signatures of pyrogenic sources during this time, raising questions regarding the importance of the contribution of BB versus road traffic in the suburban areas during the BB season.
3.4 Isotope-based quantification of BB contribution to ambient NH3
There are several challenges that need to be overcome when trying to more accurately quantify the contribution of BB emissions to ambient NH3 based on N isotope data. Firstly, given the use of only one isotope parameter (δ15N; in contrast to NOx where the δ18O can also be analyzed), more than three potential NH3 sources (e.g., urban and suburban sites) will introduce large uncertainties in isotopic end-member mixing models in terms of quantifying their relative contributions to the ambient NH3 (Chang et al., 2015). Secondly, atmospheric wet scavenging could further compromise or alter the primary NH3 N isotopic signatures (Elliott et al., 2019; Zheng et al., 2018; Chang et al., 2019a). For these reasons, we focus here on the samples collected at the three rural sites during the dry BB season (lasting 7 weeks) to isotopically examine the contribution of BB emissions to ambient NH3. We separated these samples into seven groups based on the week of their sampling, and we integrated the measured δ15N–NH3 values as well as the N isotopic signatures of potential NH3 sources (i.e., biomass burning, livestock breeding, fertilizer application) into the Bayesian isotopic mixing model (see Sect. S1 for details). The results of NH3 source apportionment are reported in Fig. 6. With a certain degree of variability, the contribution of BB to the ambient NH3 in the rural areas during the 7 weeks of sampling in the dry season was only 21.0 % (±4.7 %). Hence, NH3 emission from BB is significantly less important than from livestock breeding (37.1±7.1 %) and fertilizer application (41.8±5.9 %). This comes as a surprise, given the fact that the study area belongs to one of the most important BB regions in SE Asia, or even in the world, and the samples used for isotopic source apportionment were collected during the season of intensive BB.
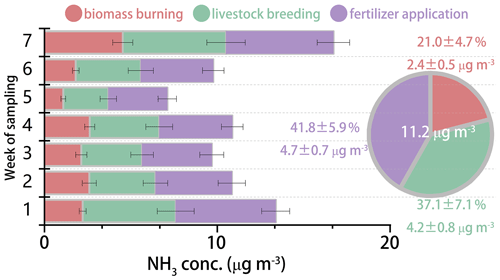
Figure 6Source apportionment results of ambient NH3 in rural areas during the dry season based on Bayesian isotopic mixing modeling and the isotopic source signatures. The error bars indicate 2 standard deviations.
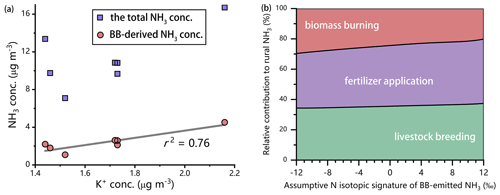
Figure 7(a) Scatterplots of the aerosol K+ concentrations versus total NH3 concentrations, as well as the NH3 concentrations from BB emissions, at the rural sites during the dry season. (b) Bayesian isotope modeling-based source apportionment results of ambient NH3 at the rural sites during the dry season, as function of the assumed N isotopic signatures of BB-emitted NH3.
During the dry season, we also analyzed particulate potassium (K+), a chemical tracer of biomass combustion, at two rural sites (S4, S5; in 39 daily fine-particle (PM2.5) samples). The particulate K+ data offer a valuable opportunity to validate our isotope-based source apportionment results. Again, we divided the dry-season data set into seven groups based on the week of NH3 passive sampling. The correlation between the particulate K+ concentration and the total NH3 concentration at the rural sites was rather poor (r2=0.43; blue symbols in Fig. 7a). Such a weak correlation supports our conclusion regarding the isotope-based source apportionment results (see above), providing additional independent evidence that BB can hardly be the dominant source of NH3 during the sampling period at the studied rural areas. In contrast, the correlation between the particulate K+ concentration and the estimated BB-derived NH3 concentration (instead of total NH3) is much better (r2=0.76; Fig. 7a) and, thus, further validates our modeling approach. While the independent particulate K+ data further increase our confidence in the N-isotope-based assessment, some uncertainty still remains with respect to the robustness of the end-member source δ15N values, potential source-altering effects, and in turn our estimates of the BB-associated NH3 contribution. In other words, the latter is probably sensitive to the considered range in the δ15N of potential NH3 emission sources, and this range may be quite large or uncertain for at least some of the sources. The δ15N–NH3 from BB, in particular, is only poorly constrained, with hardly any reports from the literature (e.g., Kawashima and Kurahashi, 2011). In recent chamber experiments, we found that the δ15N–NH3 produced by the combustion of a variety of biomass types (subtropical trees and agricultural residues) ranged between −11.8 ‰ and −4.6 ‰ (Chang and Ma, 2016, Chang et al., 2019a), which is distinctly lower than the N isotopic signature of BB-emitted (12 ‰) determined previously (Kawashima and Kurahashi, 2011) and adopted in this study. Assuming that the true N isotopic signatures of BB-emitted NH3 in the study area falls somewhere within the range of −12 ‰ to 12 ‰ (based on our published data in Chang and Ma, 2016; Chang et al., 2019a) and the value reported in Kawashima and Kurahashi, 2011), we re-calculated the source apportionment estimates as function of the different δ15N values for BB-emitted NH3 (Fig. 7b). The estimates are not sensitive to the choice of the N isotopic composition of the BB-associated NH3 source. Specifically, independent of the chosen δ15N–NH3 value, BB is always the least important of the three main NH3 sources in rural areas, contributing no more than 29.6 %. This is because although the isotopic signatures of BB-emitted NH3 have a wide range of δ15N values, their δ15N–NH3 values are still significantly (p<0.01) higher (i.e., without overlap as shown in Fig. 4) than the measured δ15N values of ambient NH3 at the rural sites.
As illustrated by the pie chart in Fig. 6, the average contribution of BB to ambient NH3 at the rural sites during the season of intensive fire events is 2.4 µg m−3; this value can be regarded as the maximum possible concentration of BB-emitted NH3 for the urban and rural sites, which are much further away from the fire areas. Based on the total NH3 concentrations measured at the other sites, we calculate that the contribution of BB to the ambient NH3 in the urban and suburban areas is of the order of 9.6 % (ranging from 5.2 % to 14.8 %) and 12.3 % (ranging from 6.1 % to 19.9 %), respectively.
In this study, we integrated satellite constraints on atmospheric NH3 levels and fire intensity, discrete NH3 concentration measurement, and N isotopic analysis of NH3 in order to assess the regional-scale contribution of BB to ambient NH3 in the heartland of Southeast Asia. The combined approach provides a cross-validation framework for source apportioning of NH3 in the lower atmosphere and will thus help to ameliorate predictions of BB emissions beyond the tropics, particularly in areas of high vegetation fire risk. Our results suggest that during the dry wildfire season, BB emissions represent a ubiquitous but comparatively small NH3 source, which accounts for 9.6 %, 12.3 % and 21.0 % of ambient NH3 in urban, suburban and rural environments, respectively. While we do not claim that our results necessarily apply at the global scale, and we do not question that BB is one of the most important global NH3 sources, we find that at least in the heartland of SE Asia, BB related NH3 emissions to the atmosphere are rather moderate and vary significantly in time and space. Both satellite observations and field and ground-based measurements capture these variations. Our findings underscore that BB-induced NH3 emissions in tropical monsoon environments can be much lower than previously anticipated. Existing atmospheric transport models may overestimate current, and likely future, NH3 emissions under changing climate conditions. While the full implications of our results remain to be explored, they promise to provide important guidance for revising NH3 emissions from BB in atmospheric transport models to assess their impacts on air quality, human health and climate change.
Time series of data used in this paper can be found online (https://doi.org/10.5281/zenodo.4025673) (Chang, 2020).
The supplement related to this article is available online at: https://doi.org/10.5194/acp-21-7187-2021-supplement.
YHC and YLZ designed the study. YHC wrote the original paper, organized the data and performed the analysis. YHC, MVD, LC and MFL revised and edited the text. All authors contributed to the final paper.
The authors declare that they have no conflict of interest.
This article is part of the special issue “Regional assessment of air pollution and climate change over East and Southeast Asia: results from MICS-Asia Phase III”. It is not associated with a conference.
This study was supported by the International (Regional) Cooperation and Exchange project (NSFC-TRF project; grant no. 41761144056), the National Natural Science Foundation of China (grant nos. 41975166, 41977305, 41761144056 and 41705100), the Natural Science Foundation of Jiangsu Province (grant nos. BK20180040 and BK20170946), the opening project of the State Environmental Protection Key Laboratory of Formation and Prevention of Urban Air Pollution Complex (Shanghai Academy of Environment Sciences; grant no. CX2020080583), the special fund of State Key Joint Laboratory of Environment Simulation and Pollution Control (grant no. 19K01ESPCT), the Young Elite Scientist Sponsorship Program by the Jiangsu Provincial Association for Science and Technology, the opening project of Shanghai Key Laboratory of Atmospheric Particle Pollution and Prevention (LAP3; grant no. FDLAP19001), the Joint Open Project of KLME and CIC-FEMD (grant no. KLME201909), and the Gao-Tingyao Scholarship for outstanding PhD students. Lieven Clarisse and Martin Van Damme (research associate and a postdoctoral researcher, respectively) are supported by the F.R.S.-FNRS.
This research has been supported by the International (Regional) Cooperation and Exchange project (NSFC-TRF project; grant no. 41761144056).
This paper was edited by Leiming Zhang and reviewed by two anonymous referees.
Akagi, S. K., Yokelson, R. J., Wiedinmyer, C., Alvarado, M. J., Reid, J. S., Karl, T., Crounse, J. D., and Wennberg, P. O.: Emission factors for open and domestic biomass burning for use in atmospheric models, Atmos. Chem. Phys., 11, 4039–4072, https://doi.org/10.5194/acp-11-4039-2011, 2011.
Andreae, M. O.: Emission of trace gases and aerosols from biomass burning – an updated assessment, Atmos. Chem. Phys., 19, 8523–8546, https://doi.org/10.5194/acp-19-8523-2019, 2019.
Andreae, M. O. and Merlet, P.: Emission of trace gases and aerosols from biomass burning, Global Biogeochem. Cy., 15, 955–966, https://doi.org/10.1029/2000gb001382, 2001.
Aneja, V. P., Schlesinger, W. H., and Erisman, J. W.: Farming pollution, Nat. Geosci., 1, 409–411, https://doi.org/10.1038/Ngeo236, 2008.
Aouizerats, B., van der Werf, G. R., Balasubramanian, R., and Betha, R.: Importance of transboundary transport of biomass burning emissions to regional air quality in Southeast Asia during a high fire event, Atmos. Chem. Phys., 15, 363–373, https://doi.org/10.5194/acp-15-363-2015, 2015.
Asman, W. A., Sutton, M. A., and Schjørring, J. K.: Ammonia: emission, atmospheric transport and deposition, New Phytol., 139, 27–48, 1998.
Bauters, M., Drake, T. W., Verbeeck, H., Bodé, S., Hervé-Fernández, P., Zito, P., Podgorski, D. C., Boyemba, F., Makelele, I., Cizungu Ntaboba, L., Spencer, R. G. M., and Boeckx, P.: High fire-derived nitrogen deposition on central African forests, P. Natl. Acad. Sci. USA, 115, 549–554, https://doi.org/10.1073/pnas.1714597115, 2018.
Betha, R., Behera, S. N., and Balasubramanian, R.: 2013 Southeast Asian Smoke Haze: Fractionation of Particulate-Bound Elements and Associated Health Risk, Environ. Sci. Technol., 48, 4327–4335, https://doi.org/10.1021/es405533d, 2014.
Bikkina, S., Haque, M. M., Sarin, M., and Kawamura, K.: Tracing the Relative Significance of Primary versus Secondary Organic Aerosols from Biomass Burning Plumes over Coastal Ocean Using Sugar Compounds and Stable Carbon Isotopes, ACS Earth Space Chem., 3, 1471–1484, https://doi.org/10.1021/acsearthspacechem.9b00140, 2019.
Bouwman, A. F., Lee, D. S., Asman, W. A. H., Dentener, F. J., VanderHoek, K. W., and Olivier, J. G. J.: A global high-resolution emission inventory for ammonia, Global Biogeochem. Cy., 11, 561–587, 1997.
Bray, C. D., Battye, W., Aneja, V. P., Tong, D. Q., Lee, P., and Tang, Y.: Ammonia emissions from biomass burning in the continental United States, Atmos. Environ., 187, 50–61, https://doi.org/10.1016/j.atmosenv.2018.05.052, 2018.
Carmichael, G. R., Ferm, M., Thongboonchoo, N., Woo, J.-H., Chan, L. Y., Murano, K., Viet, P. H., Mossberg, C., Bala, R., Boonjawat, J., Upatum, P., Mohan, M., Adhikary, S. P., Shrestha, A. B., Pienaar, J. J., Brunke, E. B., Chen, T., Jie, T., Guoan, D., Peng, L. C., Dhiharto, S., Harjanto, H., Jose, A. M., Kimani, W., Kirouane, A., Lacaux, J.-P., Richard, S., Barturen, O., Cerda, J. C., Athayde, A., Tavares, T., Cotrina, J. S., and Bilici, E.: Measurements of sulfur dioxide, ozone and ammonia concentrations in Asia, Africa, and South America using passive samplers, Atmos. Environ., 37, 1293–1308, https://doi.org/10.1016/S1352-2310(02)01009-9, 2003.
Chang, Y.: Thailand ammonia [Data set], Zenodo, https://doi.org/10.5281/zenodo.4025673, 2020.
Chang, Y. and Ma, H.: Comment on “Fossil Fuel Combustion-Related Emissions Dominate Atmospheric Ammonia Sources during Severe Haze Episodes: Evidence from 15N-Stable Isotope in Size-Resolved Aerosol Ammonium”, Environ. Sci. Technol., 50, 10765–10766, https://doi.org/10.1021/acs.est.6b03458, 2016.
Chang, Y., Deng, C., Dore, A. J., and Zhuang, G.: Human Excreta as a Stable and Important Source of Atmospheric Ammonia in the Megacity of Shanghai, Plos One, 10, e0144661, https://doi.org/10.1371/journal.pone.0144661, 2015.
Chang, Y., Liu, X., Deng, C., Dore, A. J., and Zhuang, G.: Source apportionment of atmospheric ammonia before, during, and after the 2014 APEC summit in Beijing using stable nitrogen isotope signatures, Atmos. Chem. Phys., 16, 11635–11647, https://doi.org/10.5194/acp-16-11635-2016, 2016a.
Chang, Y., Zou, Z., Deng, C., Huang, K., Collett, J. L., Lin, J., and Zhuang, G.: The importance of vehicle emissions as a source of atmospheric ammonia in the megacity of Shanghai, Atmos. Chem. Phys., 16, 3577–3594, https://doi.org/10.5194/acp-16-3577-2016, 2016b.
Chang, Y., Zhang, Y. L., Li, J., Tian, C., Song, L., Zhai, X., Zhang, W., Huang, T., Lin, Y. C., Zhu, C., Fang, Y., Lehmann, M. F., and Chen, J.: Isotopic constraints on the atmospheric sources and formation of nitrogenous species in clouds influenced by biomass burning, Atmos. Chem. Phys., 19, 12221–12234, https://doi.org/10.5194/acp-19-12221-2019, 2019a.
Chang, Y., Zou, Z., Zhang, Y., Deng, C., Hu, J., Shi, Z., Dore, A. J., and Collett, J.: Assessing contributions of agricultural and non-agricultural emissions to atmospheric ammonia in a Chinese megacity, Environ. Sci. Technol., 53, 1822–1833, https://doi.org/10.1021/acs.est.8b05984, 2019b.
Chang, Y., Clarisse, L., Van Damme, M., Tao, Y., Zou, Z., Dore, A. J., and Collett, J. L.: Ammonia Emissions from Mudflats of River, Lake, and Sea, ACS Earth Space Chem., 4, 614–619, https://doi.org/10.1021/acsearthspacechem.0c00017, 2020.
Chang, Y. H., Liu, X. J., Dore, A. J., and Li, K.: Stemming PM2.5 pollution in China: Re-evaluating the role of ammonia, aviation and non-exhaust road traffic emissions, Environ. Sci. Technol., 46, 13035–13036, https://doi.org/10.1021/es304806k, 2012.
Chu, J.-E., Kim, K.-M., Lau, W. K. M., and Ha, K.-J.: How Light-Absorbing Properties of Organic Aerosol Modify the Asian Summer Monsoon Rainfall?, J. Geophys. Res.-Atmos., 123, 2244–2255, https://doi.org/10.1002/2017jd027642, 2018.
Clarisse, L., Clerbaux, C., Dentener, F., Hurtmans, D., and Coheur, P.-F.: Global ammonia distribution derived from infrared satellite observations, Nat. Geosci., 2, 479–483, https://doi.org/10.1038/ngeo551, 2009.
Clarisse, L., Shephard, M. W., Dentener, F., Hurtmans, D., Cady-Pereira, K., Karagulian, F., Van Damme, M., Clerbaux, C., and Coheur, P.-F.: Satellite monitoring of ammonia: A case study of the San Joaquin Valley, J. Geophys. Res.-Atmos., 115, D13302, https://doi.org/10.1029/2009JD013291, 2010.
Crutzen, P. J. and Andreae, M. O.: Biomass Burning in the Tropics: Impact on Atmospheric Chemistry and Biogeochemical Cycles, Science, 250, 1669–1678, https://doi.org/10.1126/science.250.4988.1669, 1990.
Crutzen, P. J., Heidt, L. E., Krasnec, J. P., Pollock, W. H., and Seiler, W.: Biomass burning as a source of atmospheric gases CO, H2, N2O, NO, CH3Cl and COS, Nature, 282, 253–256, https://doi.org/10.1038/282253a0, 1979.
Elliott, E. M., Yu, Z., Cole, A. S., and Coughlin, J. G.: Isotopic advances in understanding reactive nitrogen deposition and atmospheric processing, Sci. Total Environ., 662, 393–403, https://doi.org/10.1016/j.scitotenv.2018.12.177, 2019.
Felix, J. D., Elliott, E. M., Gish, T. J., McConnell, L. L., and Shaw, S. L.: Characterizing the isotopic composition of atmospheric ammonia emission sources using passive samplers and a combined oxidation-bacterial denitrifier approach, Rapid Commun. Mass Spectrom., 27, 2239–2246, https://doi.org/10.1002/rcm.6679, 2013.
Hantson, S., Arneth, A., Harrison, S. P., Kelley, D. I., Prentice, I. C., Rabin, S. S., Archibald, S., Mouillot, F., Arnold, S. R., Artaxo, P., Bachelet, D., Ciais, P., Forrest, M., Friedlingstein, P., Hickler, T., Kaplan, J. O., Kloster, S., Knorr, W., Lasslop, G., Li, F., Mangeon, S., Melton, J. R., Meyn, A., Sitch, S., Spessa, A., van der Werf, G. R., Voulgarakis, A., and Yue, C.: The status and challenge of global fire modelling, Biogeosciences, 13, 3359–3375, https://doi.org/10.5194/bg-13-3359-2016, 2016.
Huang, C., Hu, Q., Lou, S., Tian, J., Wang, R., Xu, C., An, J., Ren, H., Ma, D., Quan, Y., Zhang, Y., and Li, L.: Ammonia Emission Measurements for Light-Duty Gasoline Vehicles in China and Implications for Emission Modeling, Environ. Sci. Technol., 52, 11223–11231, https://doi.org/10.1021/acs.est.8b03984, 2018.
Huang, K., Fu, J. S., Hsu, N. C., Gao, Y., Dong, X., Tsay, S.-C., and Lam, Y. F.: Impact assessment of biomass burning on air quality in Southeast and East Asia during BASE-ASIA, Atmos. Environ., 78, 291-302, https://doi.org/10.1016/j.atmosenv.2012.03.048, 2013.
Kawashima, H. and Kurahashi, T.: Inorganic ion and nitrogen isotopic compositions of atmospheric aerosols at Yurihonjo, Japan: Implications for nitrogen sources, Atmos. Environ., 45, 6309–6316, 2011.
Layman, C. A., Araujo, M. S., Boucek, R., Hammerschlag-Peyer, C. M., Harrison, E., Jud, Z. R., Matich, P., Rosenblatt, A. E., Vaudo, J. J., Yeager, L. A., Post, D. M., and Bearhop, S.: Applying stable isotopes to examine food-web structure: an overview of analytical tools, Biolog. Rev., 87, 545–562, https://doi.org/10.1111/j.1469-185X.2011.00208.x, 2012.
Lee, H. H., Bar-Or, R. Z., and Wang, C.: Biomass burning aerosols and the low-visibility events in Southeast Asia, Atmos. Chem. Phys., 17, 965–980, https://doi.org/10.5194/acp-17-965-2017, 2017.
Li, F., Zhang, X., and Kondragunta, S.: Biomass Burning in Africa: An Investigation of Fire Radiative Power Missed by MODIS Using the 375 m VIIRS Active Fire Product, Remote Sens., 12, 1561–1580, https://doi.org/10.3390/rs12101561, 2020.
Li, Q., Jiang, J., Cai, S., Zhou, W., Wang, S., Duan, L., and Hao, J.: Gaseous Ammonia Emissions from Coal and Biomass Combustion in Household Stoves with Different Combustion Efficiencies, Environ. Sci. Technol. Lett., 3, 98–103, https://doi.org/10.1021/acs.estlett.6b00013, 2016.
Liu, D., Fang, Y., Tu, Y., and Pan, Y.: Chemical method for nitrogen isotopic analysis of ammonium at natural abundance, Anal. Chem., 86, 3787–3792, https://doi.org/10.1021/ac403756u, 2014.
Liu, X., Zhang, Y., Han, W., Tang, A., Shen, J., Cui, Z., Vitousek, P., Erisman, J. W., Goulding, K., Christie, P., Fangmeier, A., and Zhang, F.: Enhanced nitrogen deposition over China, Nature, 494, 459–462, https://doi.org/10.1038/nature11917, 2013.
Lobert, J. M., Scharffe, D. H., Hao, W. M., and Crutzen, P. J.: Importance of biomass burning in the atmospheric budgets of nitrogen-containing gases, Nature, 346, 552–554, https://doi.org/10.1038/346552a0, 1990.
Marlier, M. E., DeFries, R. S., Voulgarakis, A., Kinney, P. L., Randerson, J. T., Shindell, D. T., Chen, Y., and Faluvegi, G.: El Niño and health risks from landscape fire emissions in southeast Asia, Nat. Clim. Change, 3, 131–136, https://doi.org/10.1038/nclimate1658, 2013.
Martin, R. V.: Satellite remote sensing of surface air quality, Atmos. Environ., 42, 7823-7843, https://doi.org/10.1016/j.atmosenv.2008.07.018, 2008.
Pan, X., Ichoku, C., Chin, M., Bian, H., Darmenov, A., Colarco, P., Ellison, L., Kucsera, T., da Silva, A., Wang, J., Oda, T., and Cui, G.: Six global biomass burning emission datasets: intercomparison and application in one global aerosol model, Atmos. Chem. Phys., 20, 969–994, https://doi.org/10.5194/acp-20-969-2020, 2020.
Parnell, A. C., Inger, R., Bearhop, S., and Jackson, A. L.: Source partitioning using stable isotopes: coping with too much variation, Plos One, 5, e9672, https://doi.org/10.1371/journal.pone.0009672, 2010.
Paulot, F. and Jacob, D. J.: Hidden cost of US agricultural exports: particulate matter from ammonia emissions, Environ. Sci. Technol., 48, 903–908, 2014.
Paulot, F., Paynter, D., Ginoux, P., Naik, V., Whitburn, S., Van Damme, M., Clarisse, L., Coheur, P.-F., and Horowitz, L. W.: Gas-aerosol partitioning of ammonia in biomass burning plumes: Implications for the interpretation of spaceborne observations of ammonia and the radiative forcing of ammonium nitrate, Geophys. Res. Lett., 44, 8084–8093, https://doi.org/10.1002/2017gl074215, 2017.
Puchalski, M. A., Sather, M. E., Walker, J. T., Lehmann, C. M., Gay, D. A., Mathew, J., and Robarge, W. P.: Passive ammonia monitoring in the United States: Comparing three different sampling devices, J. Environ. Monit., 13, 3156–3167, 2011.
Shi, Y., Matsunaga, T., and Yamaguchi, Y.: High-Resolution Mapping of Biomass Burning Emissions in Three Tropical Regions, Environ. Sci. Technol., 49, 10806–10814, https://doi.org/10.1021/acs.est.5b01598, 2015.
Souri, A. H., Choi, Y., Jeon, W., Kochanski, A. K., Diao, L., Mandel, J., Bhave, P. V., and Pan, S.: Quantifying the Impact of Biomass Burning Emissions on Major Inorganic Aerosols and Their Precursors in the U.S., J. Geophys. Res.-Atmos., 122, 12020–12041, https://doi.org/10.1002/2017jd026788, 2017.
Streets, D. G., Canty, T., Carmichael, G. R., de Foy, B., Dickerson, R. R., Duncan, B. N., Edwards, D. P., Haynes, J. A., Henze, D. K., Houyoux, M. R., Jacob, D. J., Krotkov, N. A., Lamsal, L. N., Liu, Y., Lu, Z., Martin, R. V., Pfister, G. G., Pinder, R. W., Salawitch, R. J., and Wecht, K. J.: Emissions estimation from satellite retrievals: A review of current capability, Atmos. Environ., 77, 1011–1042, https://doi.org/10.1016/j.atmosenv.2013.05.051, 2013.
Sun, K., Tao, L., Miller, D. J., Pan, D., Golston, L. M., Zondlo, M. A., Griffin, R. J., Wallace, H. W., Leong, Y. J., Yang, M. M., Zhang, Y., Mauzerall, D. L., and Zhu, T.: Vehicle Emissions as an Important Urban Ammonia Source in the United States and China, Environ. Sci. Technol., 51, 2472–2481, https://doi.org/10.1021/acs.est.6b02805, 2017.
Sutton, M. A., Erisman, J. W., Dentener, F., and Moller, D.: Ammonia in the environment: From ancient times to the present, Environ. Pollut., 156, 583–604, https://doi.org/10.1016/j.envpol.2008.03.013, 2008.
Sutton, M. A., Oenema, O., Erisman, J. W., Leip, A., van Grinsven, H., and Winiwarter, W.: Too much of a good thing, Nature, 472, 159–161, https://doi.org/10.1038/472159a, 2011.
Tang, Y. S., Braban, C. F., Dragosits, U., Dore, A. J., Simmons, I., van Dijk, N., Poskitt, J., Dos Santos Pereira, G., Keenan, P. O., Conolly, C., Vincent, K., Smith, R. I., Heal, M. R., and Sutton, M. A.: Drivers for spatial, temporal and long-term trends in atmospheric ammonia and ammonium in the UK, Atmos. Chem. Phys., 18, 705–733, https://doi.org/10.5194/acp-18-705-2018, 2018.
Teng, X., Hu, Q., Zhang, L., Qi, J., Shi, J., Xie, H., Gao, H., and Yao, X.: Identification of Major Sources of Atmospheric NH3 in an Urban Environment in Northern China During Wintertime, Environ. Sci. Technol., 51, 6839–6848, https://doi.org/10.1021/acs.est.7b00328, 2017.
Tsai, Y. I., Sopajaree, K., Chotruksa, A., Wu, H.-C., and Kuo, S.-C.: Source indicators of biomass burning associated with inorganic salts and carboxylates in dry season ambient aerosol in Chiang Mai Basin, Thailand, Atmos. Environ., 78, 93-104, https://doi.org/10.1016/j.atmosenv.2012.09.040, 2013.
Van Damme, M., Clarisse, L., Heald, C. L., Hurtmans, D., Ngadi, Y., Clerbaux, C., Dolman, A. J., Erisman, J. W., and Coheur, P. F.: Global distributions, time series and error characterization of atmospheric ammonia (NH3) from IASI satellite observations, Atmos. Chem. Phys., 14, 2905–2922, https://doi.org/10.5194/acp-14-2905-2014, 2014.
Van Damme, M., Clarisse, L., Dammers, E., Liu, X., Nowak, J. B., Clerbaux, C., Flechard, C. R., Galy-Lacaux, C., Xu, W., Neuman, J. A., Tang, Y. S., Sutton, M. A., Erisman, J. W., and Coheur, P. F.: Towards validation of ammonia (NH3) measurements from the IASI satellite, Atmos. Meas. Tech., 8, 1575–1591, https://doi.org/10.5194/amt-8-1575-2015, 2015a.
Van Damme, M., Erisman, J. W., Clarisse, L., Dammers, E., Whitburn, S., Clerbaux, C., Dolman, A. J., and Coheur, P.-F.: Worldwide spatiotemporal atmospheric ammonia (NH3) columns variability revealed by satellite, Geophys. Res. Lett., 42, 8660–8668, https://doi.org/10.1002/2015gl065496, 2015b.
Van Damme, M., Whitburn, S., Clarisse, L., Clerbaux, C., Hurtmans, D., and Coheur, P. F.: Version 2 of the IASI NH3 neural network retrieval algorithm: near-real-time and reanalysed datasets, Atmos. Meas. Tech., 10, 4905–4914, https://doi.org/10.5194/amt-10-4905-2017, 2017.
Van Damme, M., Clarisse, L., Whitburn, S., Hadji-Lazaro, J., Hurtmans, D., Clerbaux, C., and Coheur, P.-F.: Industrial and agricultural ammonia point sources exposed, Nature, 564, 99–103, https://doi.org/10.1038/s41586-018-0747-1, 2018.
van der Werf, G. R., Randerson, J. T., Giglio, L., Collatz, G. J., Kasibhatla, P. S., and Arellano Jr., A. F.: Interannual variability in global biomass burning emissions from 1997 to 2004, Atmos. Chem. Phys., 6, 3423–3441, https://doi.org/10.5194/acp-6-3423-2006, 2006.
Wang, S. X., Xing, J., Jang, C. R., Zhu, Y., Fu, J. S., and Hao, J. M.: Impact Assessment of Ammonia Emissions on Inorganic Aerosols in East China Using Response Surface Modeling Technique, Environ. Sci. Technol., 45, 9293–9300, 2011.
Wang, Y., Zhang, Q. Q., He, K., Zhang, Q., and Chai, L.: Sulfate-nitrate-ammonium aerosols over China: response to 2000–2015 emission changes of sulfur dioxide, nitrogen oxides, and ammonia, Atmos. Chem. Phys., 13, 2635–2652, https://doi.org/10.5194/acp-13-2635-2013, 2013.
Whitburn, S., Van Damme, M., Kaiser, J. W., van der Werf, G. R., Turquety, S., Hurtmans, D., Clarisse, L., Clerbaux, C., and Coheur, P. F.: Ammonia emissions in tropical biomass burning regions: Comparison between satellite-derived emissions and bottom-up fire inventories, Atmos. Environ., 121, 42–54, https://doi.org/10.1016/j.atmosenv.2015.03.015, 2015.
Whitburn, S., Van Damme, M., Clarisse, L., Bauduin, S., Heald, C. L., Hadji-Lazaro, J., Hurtmans, D., Zondlo, M. A., Clerbaux, C., and Coheur, P. F.: A flexible and robust neural network IASI-NH3 retrieval algorithm, J. Geophys. Res.-Atmos., 121, 6581–6599, https://doi.org/10.1002/2016JD024828, 2016a.
Whitburn, S., Van Damme, M., Clarisse, L., Turquety, S., Clerbaux, C., and Coheur, P.-F.: Doubling of annual ammonia emissions from the peat fires in Indonesia during the 2015 El Niño, Geophys. Res. Lett., 43, 11007–11014, https://doi.org/10.1002/2016gl070620, 2016b.
Zheng, X.-D., Liu, X.-Y., Song, W., Sun, X.-C., and Liu, C.-Q.: Nitrogen isotope variations of ammonium across rain events: Implications for different scavenging between ammonia and particulate ammonium, Environ. Pollut., 239, 392–398, https://doi.org/10.1016/j.envpol.2018.04.015, 2018.
Zhu, L., Henze, D. K., Cady-Pereira, K. E., Shephard, M. W., Luo, M., Pinder, R. W., Bash, J. O., and Jeong, G.-R.: Constraining U.S. ammonia emissions using TES remote sensing observations and the GEOS-Chem adjoint model, J. Geophys. Res.-Atmos., 118, 3355–3368, https://doi.org/10.1002/jgrd.50166, 2013.