the Creative Commons Attribution 4.0 License.
the Creative Commons Attribution 4.0 License.
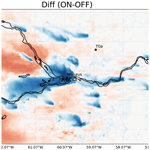
Aerosols from anthropogenic and biogenic sources and their interactions – modeling aerosol formation, optical properties, and impacts over the central Amazon basin
Janaína P. Nascimento
Megan M. Bela
Bruno B. Meller
Alessandro L. Banducci
Luciana V. Rizzo
Angel Liduvino Vara-Vela
Henrique M. J. Barbosa
Helber Gomes
Sameh A. A. Rafee
Marco A. Franco
Samara Carbone
Glauber G. Cirino
Rodrigo A. F. Souza
Stuart A. McKeen
Paulo Artaxo
The Green Ocean Amazon experiment – GoAmazon 2014–2015 – explored the interactions between natural biogenic forest emissions from central Amazonia and urban air pollution from Manaus. Previous GoAmazon 2014–2015 studies showed that nitrogen oxide (NOx = NO + NO2) and sulfur oxide (SOx) emissions from Manaus strongly interact with biogenic volatile organic compounds (BVOCs), affecting secondary organic aerosol (SOA) formation. In previous studies, ground-based and aircraft measurements provided evidence of SOA formation and strong changes in aerosol composition and properties. Aerosol optical properties also evolve, and their impacts on the Amazonian ecosystem can be significant. As particles age, some processes, such as SOA production, black carbon (BC) deposition, particle growth and the BC lensing effect change the aerosol optical properties, affecting the solar radiation flux at the surface. This study analyzes data and models SOA formation using the Weather Research and Forecasting with Chemistry (WRF-Chem) model to assess the spatial variability in aerosol optical properties as the Manaus plumes interact with the natural atmosphere. The following aerosol optical properties are investigated: single scattering albedo (SSA), asymmetry parameter (gaer), absorption Ångström exponent (AAE) and scattering Ångström exponent (SAE). These simulations were validated using ground-based measurements at three experimental sites, namely the Amazon Tall Tower Observatory – ATTO (T0a), downtown Manaus (T1), Tiwa Hotel (T2) and Manacapuru (T3), as well as the U.S. Department of Energy (DOE) Gulfstream 1 (G-1) aircraft flights. WRF-Chem simulations were performed over 7 d during March 2014. Results show a mean biogenic SOA (BSOA) mass enrichment of 512 % at the T1 site, 450 % in regions downwind of Manaus, such as the T3 site, and 850 % in areas north of the T3 site in simulations with anthropogenic emissions. The SOA formation is rather fast, with about 80 % of the SOA mass produced in 3–4 h. Comparing the plume from simulations with and without anthropogenic emissions, SSA shows a downwind reduction of approximately 10 %, 11 % and 6 % at the T1, T2 and T3 sites, respectively. Other regions, such as those further downwind of the T3 site, are also affected. The gaer values increased from 0.62 to 0.74 at the T1 site and from 0.67 to 0.72 at the T3 site when anthropogenic emissions are active. During the Manaus plume-aging process, a plume tracking analysis shows an increase in SSA from 0.91 close to Manaus to 0.98 160 km downwind of Manaus as a result of SOA production and BC deposition.
- Article
(11570 KB) - Full-text XML
-
Supplement
(7689 KB) - BibTeX
- EndNote
Aerosol particles are present in the atmosphere in highly variable types and concentrations, which contribute differently to climate forcing, cloud formation and development, as well as ecosystem impacts. Particles may have a cooling or heating effect on the atmosphere, and their climatic roles are defined by their interactions with solar and terrestrial radiation fluxes, which strongly depend on their optical properties (extinction coefficient, single scattering albedo (SSA), gaer, etc.). Radiation attenuation by atmospheric constituents is described by the radiative transfer equation, which requires information on the intensive and extensive optical properties of particulates and gases (Boucher, 2015). The aerosol's effect on radiation can be direct, semidirect or indirect. Direct effects are related to scattering and absorption of solar radiation by aerosol particles. These effects tend to dominate under clear-sky conditions. Indirect effects involve the aerosol influence on cloud formation and development through cloud droplet activation via cloud condensation nuclei (CCN; Haywood and Boucher, 2000). Semidirect effects involve the absorption (by black carbon, BC, and other absorbing aerosol compounds) of solar radiation affecting temperature, humidity, atmospheric stability and cloud formation (Forkel et al., 2012; Boucher, 2013).
Recent studies in Amazonia that integrated data from ground-based sensors (e.g., Martin et al., 2016; Rizzo et al., 2013; Andreae et al., 2015; Artaxo et al., 2013) with regional numerical simulations (e.g., Abou Rafee et al., 2017; Shrivastava et al., 2019; Medeiros et al., 2017) advanced our understanding of the interactions between background aerosol with urban anthropogenic emissions in Amazonia. However, none of these studies have quantified the impact of atmospheric aerosols on the Amazonian radiative forcing.
A previous study conducted over the Amazonian region during the GoAmazon 2014–2015 experiment found strong secondary organic aerosol (SOA) production, with an enhancement of biogenic SOA (BSOA) formation in both the Manaus plume and its outflow by a factor of 100 %–400 % on average during the afternoon of 13 March 2014 (Shrivastava et al., 2019). In southeastern Manaus, de Sá et al. (2018) showed an increase in SOA, ranging from 25 % to 200 % under polluted conditions relative to background conditions, including contributions from both primary and secondary particulate matter (PM). All of these studies are related to an idea suggested in Palm et al. (2018), which is that anthropogenic emissions play a significant role in SOA production. Cirino et al. (2018) indicate that, during the dry season, an increase of 40 % in the mass concentration of organic aerosols is attributed to SOA formation during transport from Manaus to downwind sites (T2 and T3). Conversely, the same increase was not observed during the wet season. The Manaus anthropogenic emissions are rather constant over the year. These emissions are the major source of anthropogenic organic aerosols and contribute to the organic aerosol (OA) increase downwind of Manaus (de Sá et al., 2018; Shrivastava et al., 2019; Martin et al., 2010).
A possible strategy for improving estimates of the urban plume impact on optical properties downwind of Manaus is to create regional scenario models with and without anthropogenic emissions and compare them to analyze how the emissions affect aerosol properties. Other studies have used sensitivity scenarios to understand how aerosol optical properties and secondary formation can be affected by events such as biomass burning (Vara-Vela et al., 2018) or urban pollution (Shrivastava et al., 2019). Many studies have focused on improving the understanding of an urban plume's impact on aerosol optical properties by comparing measurements during background conditions with periods affected by the pollution plume (Palacios et al., 2020; de Sá et al., 2019; Brito et al., 2014; Rizzo et al., 2013, 2011). However, little work has been done to analyze the atmospheric chemistry in the regions typically within the plume but without the plume's effects. This is particularly critical during the wet season, when aerosol levels associated with biomass burning are low and biogenic aerosols become more sensitive to external disturbance. Numerical simulations with high-resolution regional models, such as the Weather Research and Forecasting Model with Chemistry (WRF-Chem; Grell et al., 2005), are necessary for this strategy to quantify the effects of urban areas on aerosol levels and, ultimately, on the ecosystem, especially in regions that lack ground-based observations.
Different aerosol optical properties have been used to study aerosol impacts on ecosystems and the radiation balance, such as SSA (e.g., Dubovik and King, 2000; Lim et al., 2014; Russell et al., 2010; Rizzo et al., 2013), absorption Ångström exponent (AAE) and scattering Ångström exponent (SAE; e.g., Romano et al., 2019; Palacios et al., 2020) and gaer (Korras-Carraca et al., 2015). The impacts of Manaus urban emissions on the characteristics of the aerosol population (size distribution, quantity, chemical and physical composition) in regions downwind of Manaus have been described by Rizzo et al. (2013). However, there are no results considering simulated scenarios with the Manaus pollution plume component turned on and off.
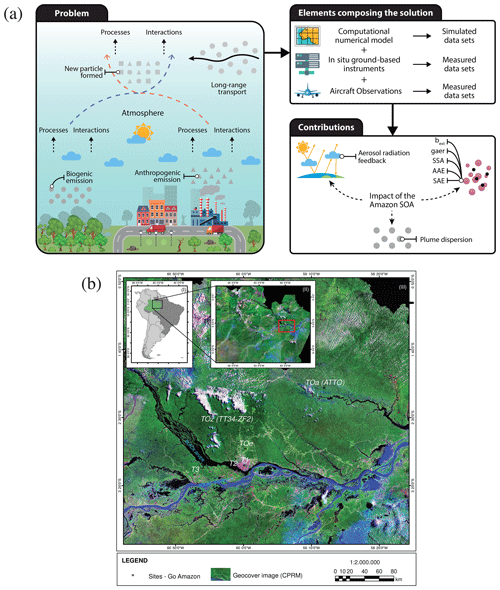
Figure 1(a) Problem: the atmosphere has different natural and anthropogenic sources and the interactions between them. The elements composing the solution include computational numerical model and observational data sets. The contributions are understanding the impact of the Manaus air pollution plume on aerosol optical property variability over the Amazon rainforest during the GoAmazon (Green Ocean Amazon) 2014–2015 experiment. (b) Sampling stations TT34-ZF2 (T0z; ca. 60 km northwest) and Amazon Tall Tower Observatory (ATTO; T0a; ca. 150 km northeast) are both upwind of Manaus; downtown Manaus (T1), Tiwa Hotel (T2; ca. 8 km southwest) and Manacapuru (T3; ca. 70 km southwest) are all downwind of Manaus. The insets show a map of (I) South America and Brazil and (II) a magnified view of the Amazon region, with the red rectangle indicating the area where the GoAmazon 2014–2015 experiment meteorology stations were located and the region used for the WRF-Chem simulations.
The objective of this work is to model secondary aerosol formation in central Amazonia, comparing modeled scenarios with and without anthropogenic emission, examining the interactions between natural biogenic emissions and urban air pollution from Manaus and investigating their impact on aerosol optical properties. We have extensively validated the model predictions with ground-based measurements and estimated how the optical properties may be affected by the plume-aging process (see Fig. 1a). This is the first study, to our knowledge, that focuses on aerosol optical properties such as SSA, gaer, absorption and scattering coefficients over a geographically extended area in central Amazonia, using numerical simulations and ground-based data.
2.1 Study region and methodology
The Amazonian region has an annual mean temperature of around 26 ∘C due to the intense solar radiation reaching the surface (Nobre et al., 2009), with an annual average precipitation of 2300 mm yr−1 (Fisch et al., 1998; de Souza and dos Santos Alvalá, 2014). In the wet season (between January and May), when the Intertropical Convergence Zone (ITCZ) extends south over Manaus, it is possible to find one of the lowest particle number concentrations over a continental area in the world (Andreae et al., 2015; Artaxo et al., 1994; Martin et al., 2016). In the wet season, the very high precipitation rate makes it virtually impossible for fires to occur, so the atmosphere is dominated by biogenic emissions, with an episodic component of Sahara desert dust and biomass burning emissions transported from Africa (Artaxo et al., 1990, 1993, 2013; Martin et al., 2016; Pöhlker et al., 2018, 2019). The central Amazon region has unique topographic characteristics, including the Amazon, Negro and Solimões rivers (Marinho et al., 2020), resulting in meteorological systems, such as local circulations and the so-called friagem events, which occur when a frontal system reaches the central Amazon basin (Marengo et al., 1997; Lu et al., 2005), and have important influences on the local and mesoscale circulations (dos Santos et al., 2014; Pereira Oliveira and Fitzjarrald, 1993; Silva Dias et al., 2004). These events may affect the wind direction, air subsidence and temperature patterns. Thus, under a combination of all these conditions, the Amazon basin is one of the cleanest continental regions on Earth, making the anthropogenic emissions plume of Manaus a significant perturbation on many environment components, such as SOA production (Artaxo et al., 2013; de Sá et al., 2018; Shrivastava et al., 2019), ozone (O3) formation (Medeiros et al., 2017; Abou Rafee et al., 2017) and the amount of precipitation (Sátyro et al., 2021).
Manaus is a city located in central Amazonia (3∘06′07′′, 60∘01′30′′). In 2014, it had a population of about 2.2 million and an urban area of 377 km2. For this study, we focus on a region centered on Manaus extending from −5.3 to S in latitude and −63.07 to −56.90∘ W in longitude (see Fig. 1b). This 600 km by 450 km, approximately rectangular, area comprises the urban area of Manaus, its satellite cities and the surrounding Amazonian forests.
Our WRF-Chem simulation was performed over 7 d between 8 and 14 March 2014. This period is part of the wet season in the region (Fisch et al., 1998; Martin et al., 2017). The first day was used as a spin-up period; as such, it was discarded from any analysis. Corrections based on the methodology used in Cosgrove et al. (2003) were applied to the simulated temperature values, aiming for better agreement between the topography height represented by the model and the one from the GoAmazon 2014–2015 experimental site.
The choice of the simulated days was made based upon ground-based data availability, which is necessary for evaluating the performance of the model, and the suggestions of Shilling et al. (2018), Shrivastava et al. (2019) and Martin et al. (2017), who highlight 13 March 2014 as a golden day for studying the evolution of the Manaus plume as it advected to the surrounding Amazon tropical forest. Our investigation focuses on a detailed analysis of 13 March 2014 because it had steady winds during the daytime, few clouds, mostly sunny skies, no precipitation and no interference from biomass burning (Shilling et al., 2018). This avoids the complex meteorology that would be expected from a river breeze circulation or convective system, which is discussed in detail in works dealing with the chemistry–meteorology connection, such as Cirino et al. (2018); de Sá et al. (2018); Thalman et al. (2017). For these reasons, the day we focused on can be regarded as a characteristic wet season sunny day, where the plume reached regions downwind of Manaus, such as the T2 and T3 sites. Furthermore, we tracked the simulated Manaus plume as it aged in order to investigate the evolution of optical properties. Different analyses of atmospheric variables, with and without anthropogenic emissions, were used to characterize changes in aerosol properties downwind of Manaus due to anthropogenic activity.
To track the plume as it ages, its approximate location and extent over time were determined using the Hybrid Single-Particle Lagrangian Integrated Trajectory (HYSPLIT) model (Draxler, 2007; Stein et al., 2007). HYSPLIT, forced by the WRF-Chem-simulated winds and other forcing meteorological fields, allow us to visualize and quantify the plume trajectory and also to determine the age of a plume it travels downwind of Manaus. WRF-Chem alone is not capable of providing this, as it does not give a time axis for each air parcel, and all pollutants are packaged together, depending on their source (e.g., CO emitted from Manaus is indistinguishable from background CO).
Forward trajectories were calculated starting from eight points defined at 200 m a.s.l. (above sea level), defining a disk with a radius of 0.03∘ (∼ 3.4 km) centered on the plume's initial location at 06:00 LT (local time). Average optical properties, gas and aerosol concentrations were calculated in an octagonal-prism-shaped volume defined, in latitude and longitude, by the eight points that were time evolved by HYSPLIT and, in altitude, by the heights 100 and 500 m above ground level. The averaging regions are shown in Fig. S15 in the Supplement, and the altitude of the plume, as given by HYSPLIT, is shown in Figs. S17 and S18. ΔCO was determined by taking the difference in carbon monoxide (CO) between simulations with anthropogenic emissions turned on and off in the simulated region selected by HYSPLIT.
We used CO as a passive tracer of the plumes. It is a common choice as a tracer because has a long residence time in the atmosphere (much longer than the transport time of the Manaus plume), and it is almost entirely anthropogenic in origin, as it is emitted during combustion and other anthropogenic processes. In addition, it is significantly enhanced in urban plumes relative to the background, and it is routinely and robustly measured (Shilling et al., 2018; Shrivastava et al., 2019). The HYSPLIT plume tracking results were verified by comparing them with the plume locations given by the CO tracer.
The HYSPLIT plume tracking approach was used on the morning plumes of 10–14 March 2014 in order to investigate the change in SOA formation due to different NOx concentrations. Other than 13 March, these were not exemplary days for observing the evolution of the Manaus plume due to meteorological factors such as precipitation. Additionally, the plume did not appear until 08:00 LT. As such, our analysis focuses on 13 March 2014.
2.2 WRF-Chem model description and setup
The study region was simulated with the WRF-Chem regional model, version 3.9.1.1 (Grell et al., 2005; Fast et al., 2006) using full coupled and online meteorology, gas-phase chemistry and aerosol feedback. The model grid covers the study region with a horizontal grid spacing of 3 km and nx=200 and ny=150 grid points. Vertically, hybrid sigma coordinates were used to split the atmosphere into 51 levels, the bottom 10 within the planetary boundary layer (PBL). Data from the Global Model Data Assimilation System (GDAS), with a horizontal grid spacing of 1∘ and 26 vertical levels was used for the initial and boundary conditions of the meteorological variables. Chemistry initial and boundary conditions were provided in 3 h increments at a horizontal resolution of about 40 km × 40 km with 60 vertical levels from the surface up to 60 km by the European Centre for Medium-Range Weather Forecasts (ECMWF) operational model.
The physics, chemistry and emission options used in this study, as well as their corresponding references, are listed in Table 1. The most significant ones for this application are as follows: the Rapid Radiative Transfer Model for General Circulation Model applications (RRTMG) scheme for longwave and shortwave radiation (Iacono et al., 2008), the revised mesoscale model version 5 Monin–Obukhov scheme for surface layer (Jiménez et al., 2012), the unified Noah land surface model for land surface (Tewari et al., 2004), land use provided by the Moderate-resolution Imaging Spectroradiometer (MODIS), with spatial resolution and 20 different classes, the Yonsei University scheme for the boundary layer (Hong et al., 2006), the Morrison 2-moment scheme for microphysics (Morrison et al., 2009), and the Grell–Freitas ensemble convective scheme (Grell and Freitas, 2014). We simulated atmospheric chemistry using the Regional Atmospheric Chemistry model (RACM) coupled with the Modal Aerosol Dynamics model for Europe/Volatility Basis Set (MADE/VBS) aerosol scheme, which treats the organic gas/particle partitioning within a spectrum of volatilities (Ahmadov et al., 2012). The RACM includes 21 stable inorganic species (four being intermediates) and 32 stable organic species (four of which are primarily of biogenic origin). In addition, RACM includes 237 chemical reactions (23 of which are photolysis). MADE/VBS has an advanced SOA module, based on VBS approach, to simulate concentrations of the main organic and inorganic gas/particle partitions within a spectrum of volatilities, using saturation vapor concentrations as surrogates for volatility. It also includes less complex aqueous reactions (sulfate – SO4 and nitrate NO3 wet deposition), following Community Multiscale Air Quality (CMAQ) methodology (Sarwar et al., 2011). MADE/VBS has a four-bin VBS, with the SOA precursor yields based on previous smog chamber studies under both high- and low-NOx conditions (Murphy and Pandis, 2009; Ahmadov et al., 2012). Yields are for four volatility bins, with saturation concentrations of 1, 10, 100 and 1000 µg m−3, and represent aerosol modes, i.e., Aitken (< 0.1 µm), accumulation (0.1–1 µm) and coarse (> 1 µm). The VBS option used in this paper has no explicit accommodation for SOA species with equivalent saturation concentrations of less than 1 µg m−3. It would be better to have bins at 0.1 µg m−3 in order to better fit the VBS model, but it is difficult to experimentally determine mass loadings for volatilities below 1 µg m−3 (Shrivastava et al., 2019). MADE/VBS is able to extrapolate volatilities beyond and between its bins (Kroll and Seinfeld, 2008), minimizing this issue.
We used the approach by Fast et al. (2006), according to Mie theory (Mie, 1908), in order to account for aerosol radiative properties, such as absorption and scattering coefficients, SSA and gaer. These properties are then transferred to the RRTMG shortwave radiation scheme in order to calculate the corresponding radiative forcing. In addition, the feedback effects of clouds on aerosol size and composition via aqueous-phase chemistry (Sarwar et al., 2011), as well as wet scavenging processes (Easter et al., 2004), are considered.
Simulations were conducted in order to analyze how Manaus emissions affect SOA production and aerosol optical properties over the Amazon. We considered the following two scenarios: (i) Manaus on, which represents anthropogenic emissions and background emissions from initial and boundary conditions, and (ii) Manaus off, which represents a background scenario, dominated by biogenic emissions, with any anthropogenic contributions coming from the boundary conditions.
(Iacono et al., 2008)(Tewari et al., 2004)(Jiménez et al., 2012)(Hong et al., 2006)(Morrison et al., 2009)(Grell and Freitas, 2014)(Papiez et al., 2009)(Ahmadov et al., 2012)(Abdul-Razzak and Ghan, 2000)(Madronich, 1987)(MEGAN; Guenther et al., 2006)Abou Rafee et al. (2017)2.2.1 Anthropogenic emissions
Anthropogenic emissions were calculated using the Abou Rafee et al. (2017) inventory, which considers emissions of all classes of mobile (light-duty and heavy-duty vehicles and motorcycles) and stationary (thermal power plants, TPPs, and refineries) sources. Both components were calculated according to emission factor estimates based on experiments conducted inside road traffic tunnels in São Paulo (Martins et al., 2006; Sánchez-Ccoyllo et al., 2009; Brito et al., 2013), providing the only vehicle emission factor measurements available in Brazil. Fine particle matter emission fractionation into size and chemical classes were based on studies developed for São Paulo (Ynoue and Andrade, 2004; Miranda and Andrade, 2005; Albuquerque et al., 2012).
2.2.2 Biogenic emissions
Biogenic emissions were calculated online using the Model of Emissions of Gases and Aerosols from Nature (MEGAN) version 2 (Guenther et al., 2006). Based on driving variables such as ambient temperature, solar radiation, leaf area index and plant functional types, this model estimates the net terrestrial biosphere emission rates for different trace gases and aerosols with a global coverage of ≈ 1 km2 spatial resolution.
2.3 Observed data
We used in situ real-time measurements at several GoAmazon 2014–2015 surface sites (see Fig. 1b). The particle scattering coefficient (σs) was measured using a three-wavelength nephelometer (450, 550 and 700 nm; TSI 3563 Integrating Nephelometer). The particle absorption coefficient (σa) was measured at the T3 site with a seven-wavelength Magee AE31 Aethalometer that operates at λ=370, 430, 470, 520, 565, 700 and 880 nm and was subjected to the correction scheme outlined by Rizzo et al. (2011). The observed (σa) values have been interpolated to the nephelometer's wavelengths to allow a proper comparison and calculation of the intensive parameters, such as SSA. The BC mass concentration at the T3 site was estimated using AE31 measurements of the absorption coefficient at 880 nm and a mass absorption cross section (MAC) of 7.77 m2 g−1 (Drinovec et al., 2015). At ATTO, the BC concentration was measured using a Thermo Fisher Scientific Environment multiangle absorption photometer (MAAP) 5012, using a σa of 637 nm and a MAC of 6.6 m2 g−1. The absorption data was corrected according to Müller et al. (2011). Organic and inorganic submicron aerosol mass loadings were measured with a time-of-flight aerosol mass spectrometer (ToF-AMS) (de Sá et al., 2018). Mixing rations of O3 and CO were obtained with a 49i O3 Analyzer (Thermo Fisher Scientific) and a N2O CO analyzer (Los Gatos Research – LGR). Meteorological observations were made with a Vaisala weather transmitter WXT520, and PBL values were measured using ceilometer and lidar (Carneiro and Fisch, 2020). Observed data were averaged at 1 h intervals for comparison with WRF. Standard temperature and pressure (STP) corrections were also applied to all measurements. We also used aircraft measurements of σa from the Department of Energy (DoE) Gulfstream 1 (G-1), as part of the GoAmazon 2014–2015 experiment (Shilling et al., 2018; Martin et al., 2016), measured using a three-wavelength (461, 522 and 648 nm) particle/soot absorption photometer (PSAP) from Radiance Research.
2.3.1 GoAmazon 2014–2015 experiment
The observations and modeling of the Green Ocean Amazon experiment, GoAmazon 2014–2015, were designed to understand how aerosol and cloud life cycles are influenced by the pollutant outflow from Manaus into the tropical rain forest (Martin et al., 2016). The experiment used a set of detailed aerosol, trace gas and cloud measurements at six different sites (see Fig. 1b) in order to better understand the atmospheric processes caused by the interaction between urban pollution emissions with volatile organic compounds (VOCs) emitted from the forest and the environmental impacts on the natural microphysical properties of clouds and aerosols, such as optical properties and particle size distributions (Gu et al., 2017; Fraund et al., 2017).
3.1 Meteorological analysis
To study the impact the Manaus pollution plume has on SOA production and aerosol optical properties in the area downwind of Manaus, meteorological conditions, especially temperature, humidity and PBL height, must be properly characterized and represented in the WRF-Chem model. Comparisons at the T3 site between observed and simulated hourly variations in accumulated total precipitation, temperature and relative humidity at 2 m, wind speed at 10 m, and PBL height (Figs. S1 and S2) show that the model performs well in terms of diurnal representation and trends. Simulated temperature and humidity tend to be underestimated (mean bias, MB, is equal to −0.5 and −1.6, respectively), with a short delay between peak observed (11:00 LT) and simulated (15:00 LT) values. The simulation has difficulty in obtaining the observed maximum temperature (Fig. S1). According to statistical indices (Table S1), the correlation coefficient (r) and root mean square error (RMSE) show consistent results for relative humidity (r=0.7, and RMSE is equal to 1.8), temperature (r=0.8, and RMSE is equal to 0.4) and wind speed (r=0.7, and RMSE is equal to 0.2). The relative humidity profile agrees well with ground-based measurements, but the simulated values exhibit the diurnal minimum with a 3 h delay. The accumulated precipitation simulated was not sufficient to represent most of the observed data at T3, with the model representing less than 50 % of the observed values.
The model is indeed underestimating the total amount of the precipitation during the simulated days (Fig. S2). However, the 4 d that were simulated which we focus on show little precipitation compared to the average during the wet season. Because of this, precipitation had quite a small impact on the chemistry during these days, and we do not expect this precipitation bias to affect our atmospheric chemistry simulations very much. Individual calculations of performance statistics for meteorological and chemical variables are presented in Table S1.
Figure S3 compares the simulated and observed vertical wind component during nighttime at the T3 site. In the early morning hours (05:00–11:00 LT), downdraft movement is not sufficient at the T3 site to inhibit pollutant dispersion. However, during the nighttime (20:00–22:00 LT), the simulation captured an organic aerosol concentration peak (see Fig. 5a) consistent with the presence of downdraft movement and a temperature inversion at low levels (Figs. S4–S7) observed at the T3 site.
3.1.1 Background conditions
Verifying model background conditions is important as it allows us to use comparisons between simulations with local anthropogenic emissions turned on and off to calculate enhancement factors. BC is an ideal aerosol for determining background anthropogenic conditions because, other than biomass burning sources, it is entirely anthropogenic. Outside of local emissions plumes, average observed BC values are influenced by biogenic aerosol absorption, the global BC background and by long-range transport of BC from Saharan dust and African biomass burning. The BC transported from Africa is episodic, depending on the ITCZ positioning, and the air mass trajectories from Africa to the central Amazon. As we have several years of BC background measurements at the ATTO tower, it is possible to separate African episodic events from the rather constant regional BC concentrations that are relevant when comparing with modeled values not under anthropogenic influences (Artaxo et al., 2020).
Generally, global and regional models contain uncertainties associated with the wet/dry deposition scheme (Wang et al., 2015). For example, the BC residence time in the atmosphere is typically larger in global models than in the real atmosphere. During the wet season, the T0a site is upwind of Manaus and, thus, has low anthropogenic influence. However, the T0a site receives sporadic air masses loaded with marine aerosol transported from the Atlantic Ocean, dust outflows from the Sahara desert and smoke derived from fires in West Africa (Ben-Ami et al., 2010; Andreae et al., 2012, 2015; Rizzolo et al., 2017; Pöhlker et al., 2018). Air mass transport from Africa during the wet season occurs when the ITCZ is shifted to the south of the central Amazonian basin, allowing air masses from the Northern Hemisphere to reach the central portion of the basin.
Figure 2 shows that, on 10 and 11 March 2014, BC values (both simulated and observed) were above the expected background level (0.035 µg m−3; Artaxo et al., 2020), consistent with long-range coherent BC transport from West Africa (Moran-Zuloaga et al., 2018; Pöhlker et al., 2019). On these days, the simulation follows the BC variability shown in the observed data somewhat, though the baseline behavior is not captured. The differences between our simulation and the ECMWF global model during these transport events is likely related to changes in the mixing and deposition mechanisms and model resolution. In addition, differences in the emission schemes between these two models can influence, through transport, the BC concentrations.
On 12 and 13 March, when no long-range transport effects are present, both simulations are consistent with observation, suggesting that our models accurately captured the background behavior. This, combined with successful modeling of regions down wind of Manaus, allows the successful calculation of aerosol and other enhancement factors in the plume region.
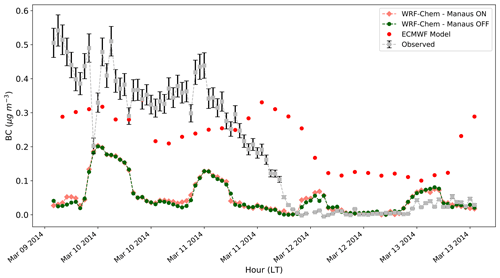
Figure 2Observed and simulated surface black carbon (BC) concentration from 10 to 13 March 2014 at the T0a site. Standard deviation bars are shown for each set of measurements. Events due to long-range transport of Saharan dust and biomass burning emissions from West Africa are visible on 10 and 11 March (Moran-Zuloaga et al., 2018). The Manaus off (green) and Manaus on (orange) simulations show BC concentrations simulated at a height of ca. 8 m above the surface. During BC transport event days, we can see that the simulation had the largest peaks as it tried to represent the BC transport coming from West Africa. The global model contribution (red dots) also represents BC transport event days, showing the largest values during 10 and 11 March.
3.2 Chemical analyses
To better understand the impact of the Manaus urban plume on SOA formation and mixing ratios at the T3 site during 13 March 2014, we must be able to separate time periods representing clean and polluted episodes and compare observed and simulated values. Previous studies have developed methods to separate these episodes in the Amazon region (Thalman et al., 2017; de Sá et al., 2018; Cirino et al., 2018).
In our analysis, with observed data from the GoAmazon 2014–2015 experiment (T3 site), adjusted cluster centroids were used to analyze clean and polluted conditions during 2 months in the wet season (February and March 2014). We define three different clusters, i.e., (i) low pollution (low pol), (ii) middle pollution (mid pol) and (iii) high pollution (high pol; see Table 2). These three clusters were chosen because the pollution conditions arriving at T3 are heterogeneous. Our cluster analysis (see Fig. 3) was made with a fuzzy c-means (FCM) clustering algorithm (Bezdek et al., 1984). On 13 March 2014, our analysis shows a day with mostly polluted conditions (at 10:00–17:00 LT). Previous work (Thalman et al., 2017; de Sá et al., 2018) reported the same polluted conditions on this day.
Because the concentration values of high pol and mid pol, episodes are substantially larger than those at low pol, and we distinguish time periods representing clean episodes as low pol and polluted episodes as high pol and mid pol. Quantitatively, we separated clean from polluted episodes with the degree of cluster membership. When membership for low pol is > 0.5, we consider this a clean episode. When the sum of mid pol and high pol membership is > 0.5, we consider this a polluted episode. Initially, we attempted clustering with only two clusters (one for clean and one for polluted episodes), but we were unable to separate polluted from background conditions. In this case, the nominal background cluster had high BC and total reactive nitrogen (NOy) concentrations.
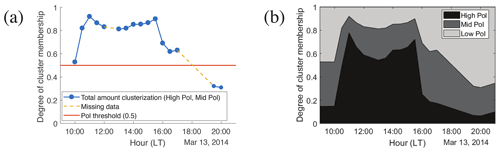
Figure 3Results of FCM clusters analysis during 13 March 2014 from 10:00 to 20:00 LT (local time) at the T3 site. (a) Total clustering, considering polluted conditions with the degree of cluster membership >0.5. (b) Degree of membership in each of the three clusters. The sum of the degrees of membership across all clusters is unity. Background conditions are abbreviated as low pol, intermediate conditions as mid pol and polluted conditions as high pol.
Table 2Cluster centroids used to analyze clean and polluted conditions. Note: ppbv – parts per billion by volume.
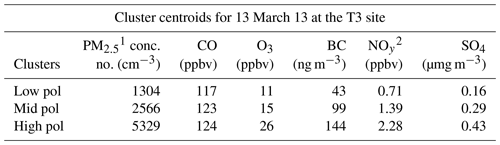
1 Particulate matter <2.5 µm. 2 Nitrogen oxides.
Given the abundance of BVOCs in the Amazon region (Alves et al., 2018; Yáñez-Serrano et al., 2020), we expect O3 to be especially sensitive to changes in NOx emissions. This can be seen in Fig. 4a and e, which show high O3 and low NOx values downwind of Manaus. According to the WRF-Chem chemical mechanism, isoprene is rapidly oxidized by hydroxyl radicals (OHs) to form peroxy radicals (HO2) in a few hours (Ahmadov et al., 2012). The T1 site, located in an urban area, has a low isoprene concentration. As the Manaus plume passes through forest regions with high isoprene production, the high plume NOx concentration oxidizes the isoprene. This can be seen in Fig. 4, where the Manaus plume consumes the isoprene around the T3 site, producing O3 and HO2. Because the enhancement of HO2 radicals occurs downwind of Manaus, such as at the T3 site, the concentration of NOx is significantly lower than the values in Manaus, leading to a significant enhancement of O3 (ca. 8–30 ppbv; Fig. 4a). Because NOx and isoprene emissions vary in different regions, our results suggest that NOx in southeastern Manaus (Abou Rafee et al., 2017) has important impacts on the O3 concentration in the Manaus urban area. This is primarily due to the rapid reactions of radicals with NOx, which deplete the radicals.
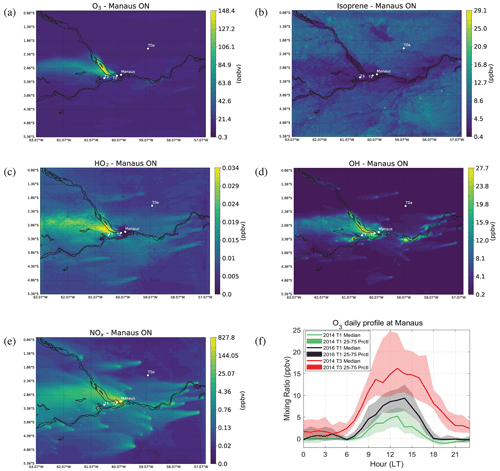
Figure 4Temporal mean (06:00 to 15:00 LT; 13 March) spatial distribution of simulated surface level concentrations of (a) ozone (O3), (b) isoprene, (c) peroxy radicals (HO2), (d) hydroxyl radicals (OH), (e) nitrogen oxides (NOx) and (f) daily median O3 profile for the month of March (wet season) at the T1 site during 2014 (green line), 2016 (black line) and at the T3 site during 2014 (red line). The red, gray and green shaded areas show the 25th to 75th percentiles (Prctl) of the respective median line.
The O3 values are highest during the day as VOC production peaks and solar radiation is available for the photochemical processes that produce O3 (Graham et al., 2003a, b; Chen et al., 2015; Schultz et al., 2017). The O3 enhancement 8 to 300 km downwind of Manaus suggests that the interaction between forest biogenic emissions and the pollution from Manaus could have an important impact on the chemical production of O3 (Fig. 4a). The interaction between anthropogenic and biogenic trace gases has strong regional characteristics, such the ones found near Manaus. They also depend on the distributions of BVOCs and anthropogenic NOx. O3 mixing ratios downwind of Manaus under the influence of anthropogenic pollution were also reported by Trebs et al. (2012) and were, on average, 31±14 ppbv, with peak values of 60 ppbv at a distance of 19 km downwind of Manaus. Our simulations showed an O3 average of 30±11 ppbv at the T3 site (70 km downwind of Manaus), with high peak values of 148 ppbv in regions northwest of Manaus (Fig. 4a). The Manaus pollution plume's influence on O3 production is clearly observed in the surrounding area, predominantly to the west and northwest of Manaus.
In regions downwind of Manaus, the simulations showed O3 concentrations extending more than 300 km. It is also interesting to note the lower O3 values around T1, which are represented in both observed (ca. 8 ppbv, on average) and simulated (ca. 12 ppbv, on average) data (Fig. 4a). O3 with ca. 8 ppbv, on average, is uncommonly low for a metropolis of nearly 2.2 million people. The agreement between observed and simulated O3 values around T1 indicates that the chemistry there is being successfully reproduced by the simulation. Our explanation for this anomaly is that VOCs are abundant all around Manaus (Kuhn et al., 2010; Alves et al., 2016), and HOx and O3 are low despite having high NOx in a typically VOC-limited regime. We hypothesize that, in areas with very high NOx emissions (averaging 129.02 ppbv), such as the power plant cluster surrounding T1 (Fig. 4e), radicals react quickly with NOx (). This depletes the O3, creating radicals and causing a decrease in O3 formation. Conversely, downwind of Manaus, the radicals last long enough to form O3, and we observe an increase in O3 formation and an increase in HO2 radicals (Fig. 4c).
Our results imply that the high NOx conditions within Manaus affect the O3 formation around Manaus, decreasing O3 production within the city and providing a great enhancement downwind of Manaus (Fig. 4a). The wind direction is predominantly from the northeast, which allows the plume be transported to the T2 and T3 sites and have a great impact on the surrounding areas (Martin et al., 2017). Interestingly, our results show that when O3 concentrations change by a factor of between 2 and 4, NOx levels may be affected, decreasing the rate of BVOC reactions and, consequently, the rate and efficiency of SOA production.
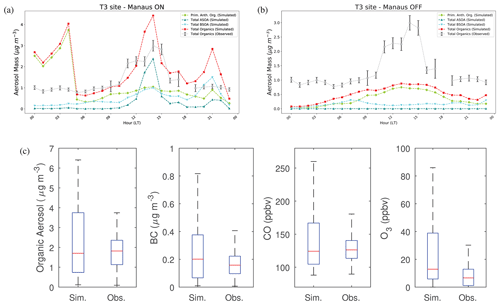
Figure 5Time series and box plot comparison of measured and WRF-Chem-simulated surface-level gases and aerosols at the T3 site. Contributions from simulated primary anthropogenic organic aerosol (POA), biogenic SOA (BSOA) and anthropogenic SOA (ASOA) to total organic aerosol (OA), as simulated by WRF-Chem, with (a) and without (b) anthropogenic emissions, on 13 March 2014 at the T3 site. (c) Comparison between observed and simulated surface-level gases and aerosols. Box plot of simulated and observed organics, black carbon (BC), carbon monoxide (CO) and ozone (O3) from 9 to 13 March 2014 at the T3 site. Median values are shown in red lines, and the blue box indicates data between the 25th and 75th percentiles.
According to Fig. 5a, the simulated organic PM2.5 at the T3 site has one of the highest values during the first hours of 13 March 2014 (02:00 to 04:00 LT), with the largest contribution coming from POA. We suggest that the large contributions of BC and CO emissions, coming from Manaus (Fig. S10) together with a prevailing northeast wind direction, are the most plausible explanations as to why simulated total organics present high values during the first hours of the day. The BC and CO contributions can end up reaching the T3 site, increasing the POA amount. In addition, the simulated BC concentration also showed simultaneous high values during the same first hours of 13 March (Fig. S8).
Between 10:00 and 16:00 LT, there is an increase in the total organic aerosol concentration, which was successfully reproduced by our simulation. This evolution of the organic aerosol concentration was expected on that day due to the Manaus plume arriving at the T3 site (Shilling et al., 2018). This increase is mostly due to a sharp increase in anthropogenic SOA (ASOA), peaking at 15:00 LT, and the BSOA and POA at the same time. The highest value (4.4 µg m−3) of simulated total organics occurred at 14:00 LT (Fig. 5a) and is mostly comprised of the SOA component, with increases in BSOA contributing 1.0 µg m−3 (22.6 %), ASOA contributing 2.4 µg m−3 (53.9 %) and POA contributing 1.04 µg m−3 (23.5 %). Conversely, when the simulation is run with anthropogenic emissions turned off, the total organic aerosol simulated at 14:00 LT is 0.9 µg m−3, with BSOA contributing 0.14 µg m−3 (16.3 %), ASOA contributing 0.02 µg m−3 (2.3 %) and POA contributing 0.7 µg m−3 (81.4 %). We attribute most of the difference in total organic aerosol between simulations with and without anthropogenic emissions to the ASOA amount as it is related to the Manaus plume. The same analysis, now considering the entire day of 13 March, shows a contribution coming mostly from POA, of 26.4 µg m−3 (57.1 %), BSOA, of 12.4 µg m−3 (26.8 %), and ASOA, of 7.4 µg m−3 (16 %). Considering the immensely complex mixture of organic aerosol particles, gas-phase VOCs, and other species in continuous evolution in the atmosphere and the large number of chemical reactions with oxidant species such as OH (daytime) and NO3 (nighttime) (Kuhn et al., 2010), we emphasize that there may be a relationship between BSOA and ASOA simulated peaks (see Fig. 5a) and the O3 peak at 15:00 LT (Fig. S9), since those chemical reactions are associated with the production of tropospheric O3 and also oxygenated VOCs (Bela et al., 2016).
A third total organic aerosol simulated peak is observed between 20:00 and 21:00 LT (see Fig. 5a). The simulated peak may be explained by the transport of air pollutants from the regions south of the T3 site (Fig. S11). We propose two possible explanations for this phenomenon. Our first explanation involves the Negro river breeze effect. Since most thermal power plants and the Isaac Sabbá Refinery (REMAN) are located near the banks of the Negro and Solimões rivers (Abou Rafee et al., 2017), the plume transport could be influenced by the river breeze circulation, which defines the trajectory of pollutants. It may be that, between 19:00 and 21:00 LT (Fig. S11), the wind direction was affected by the Negro river breeze effect due the horizontal thermal gradient caused by the different energy partitioning of the water and land surfaces. Consistent with dos Santos et al. (2014), the water surface temperature of the Negro river starts to increase in the afternoon (13:00 LT), affecting the vertical heat and mass transport. Our second explanation is that there is an air subsidence pattern at the T3 site between 19:00 and 22:00 LT (see Fig. S3). At 20:00 LT, the T3 site presents a saturation trend from 850 to 900 m and also from 520 to 600 m, with temperature and dew point temperature close to each other, creating a dry air region and, consequently, air subsidence (see Figs. S4–S7). This causes upward movement inhibition, which confines the atmospheric pollutants to low levels, impeding their spread.
An example of the differences between the measured and modeled concentration distributions is shown for organics, BC, CO and O3 in Fig. 5c. Both simulated and observed BC show a median value of 0.2 µg m−3, demonstrating that our simulation represents BC well. The same behavior is shown for OA and CO, with simulated and observed median values of 1.8 µg m−3 and 122 ppbv, respectively. However, the simulation presents a larger range of values compared with observations. The simulations present some high peaks not seen in the observed data, such as the ones in BC (see Fig. S8) and OA, with a high contributions from POA emissions (see Fig. 5a). Both have peaks in the early morning on 13 March 2014. In addition, the simulation shows a median O3 value of ca. 12 ppbv (observed at 7 ppbv). Conversely, looking at just 10:00 to 17:00 LT on 13 March 2014, with pollution contributions at the T3 site coming from Manaus (Shilling et al., 2018), both simulated and observed O3 present high median values of 38 and 30 ppbv, respectively. This agreement of the observed and simulated median values during a day with polluted conditions is remarkable, particularly when noting the uncertainties in emissions (speciation, spatial and temporal distribution), measurements, boundary conditions, meteorological components, and other input parameterization of the model. Overall, the comparisons of the median measured and predicted chemical concentrations are satisfactory, with the best match obtained in OA (observed 1.8 µg m−3; simulated 1.7 µg m−3), CO (observed 124 ppbv; simulated 126 ppbv) and BC (observed 0.201 µg m−3; simulated 0.203 µg m−3).
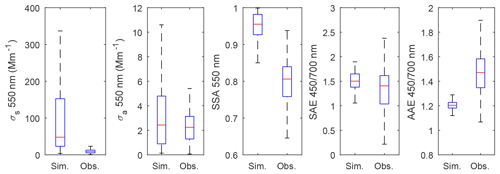
Figure 6Comparisons between observed and simulated aerosol optical properties. Box plot of simulated and observed single scattering albedo (SSA), scattering Ångström exponent (SAE), absorption Ångström exponent (AAE), scattering (σs) and absorption (σa) coefficients from 9 to 13 March 2014 at the T3 site. Median values are shown in red lines, and the blue box indicates data between the 25th and 75th percentiles.
3.3 Variability in Amazonian aerosol optical properties
Understanding how optical properties such as SSA and gaer vary downwind of Manaus is key for an understanding of the impact of the pollution plume on radiative forcing, its contributions to the local radiative budget, its impacts on the hydrological cycle and unknown indirect consequences on photosynthesis rates. These effects suggest the possibility of investigating aerosol direct radiative effects (DREs) by examining gaer, which presents, in general, higher values associated with stronger forward scattering of radiation by atmospheric aerosols (Korras-Carraca et al., 2015).
Figure 6 shows that the simulation overestimates the observed scattering coefficient by a factor of 6. The overestimate in the observed scattering coefficient is due the fact that our WRF-Chem simulations are producing more SO4 than in the real atmosphere, with 30 % of the observed PM1 attributed to SO4 in the accumulation mode (Fig. S14). Observed scattering coefficient values are significantly lower than simulated, likely due to decreases in the aerosol loading during the transect, modulated by the effects of gas and particle dilution. On the other hand, the median simulated absorption coefficient of 2.2 M m−1 is in good agreement with the observed median value of 2.4 M m−1. We observe the simulated SSA being affected by the overestimation of the simulated scattering coefficient. Comparing simulated and observed SAE values, we again have good agreement between the simulation and observations, with the simulation representing the mean size of the aerosol population 70 km downwind of Manaus quite well. These results are important for the plume-aging mechanism discussed in Sect. 3.4. Additionally, the observed AAE is considerably higher than in our simulation. This suggests that the brown carbon component, not accounted for our simulations, could have an critical effect on the AAE value, contributing to the lower median simulated AAE (1.2) compared with the median observed value (1.5).
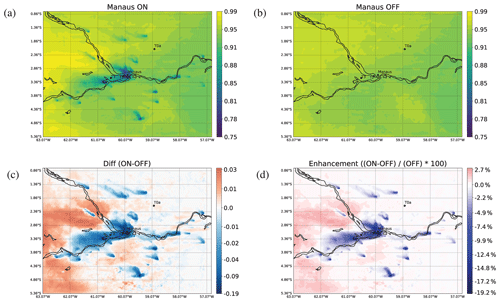
Figure 7WRF-Chem simulated values of single scattering albedo (SSA) in the presence or absence of Manaus emissions. (a) SSA, when all emissions are on. (b) SSA, when just biogenic emissions are on and anthropogenic emissions are off. (c) SSA difference between the two simulations, with anthropogenic emissions turned on and off, i.e., (on − off). (d) SSA enhancement (in percent) calculated from the two simulations, with anthropogenic emissions turned on or off, i.e., ((on − off) off) × 100. WRF-Chem predictions are at ca. 8 m altitude, averaged over 13 March 2014 (00:00–23:00 LT).
3.3.1 Calculations and measurements of SSA
According to our simulation results, the Manaus plume modifies the amount of radiation absorbed by the atmosphere and is responsible for an SSA reduction of approximately 10 % at Manaus, 12 % at the T2 site and 5.3 % at the T3 site (see Fig. 7d). This indicates a large fraction of absorbing material present in the Manaus plume, potentially warming the local atmosphere. These regions are associated with thermal power plants, vehicular emissions and other stationary sources (refineries; Medeiros et al., 2017), indicating that the regional emissions are dominated by small absorbing particles like BC, while biogenic particles are found mostly in the coarse mode and efficiently scatter radiation due to their organic-carbon-dominated composition.
During simulations with the Manaus pollution plume component turned on, average SSA values vary between 0.75 and 0.90 in regions downwind of Manaus. This represents the contribution from the interactions of urban aerosols with biogenic components of the forest. Similar results were found by Cirino et al. (2018) at the T3 site (0.80), Ramachandran and Rajesh (2007) in western India (0.88), He et al. (2009) in China (0.80) and Backman et al. (2012) in São Paulo, Brazil (0.76). These SSA values are associated with the formation of SOA aerosols which scatter radiation efficiently (Figs. S12 and S13). The decrease in the SSA is associated with a significant fraction of aerosol loading from small particles of anthropogenic origin, e.g., BC. The average simulated and observed SSA on 550 nm values during 13 March 2014 at the T3 site were 0.86 ± 0.09 and 0.78 ± 0.09, respectively.
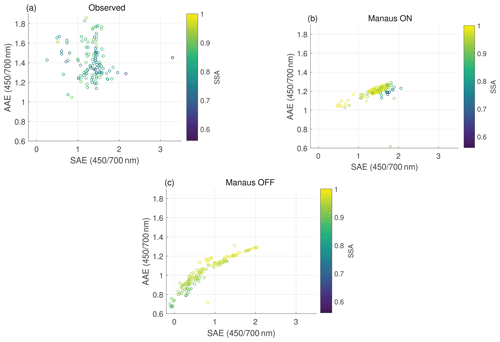
Figure 8Absorption Ångström exponents (AAEs) at the wavelength pair 470 and 660 nm, as a function of the corresponding scattering Ångström exponents (SAEs) at the wavelength pair 470 and 660 nm, which are color coded using the related SSA at the wavelength pair 470 and 660 nm. The 1 h averaged instantaneous observed data values (a) from simultaneous nephelometer and aethalometer measurements from 9 to 14 March 2014 at the T3 site. (b) The 1 h averaged simulated values when all emissions are on. (c) The 1 h average simulated values when just biogenic emissions are on and anthropogenic emissions are off.
3.3.2 Calculations of AAE and SAE
Figure 8 shows the simulated and observed SAE and AAE distributions from 9 to 14 March 2014. The simulation with anthropogenic emissions is mostly characterized by and , corresponding to a large organic carbon (OC) particle contribution, including primary and secondary components (POC and SOC, respectively; Cazorla et al., 2013). Additionally, the simulated SAE (Manaus on), when variability ranges between 1 to 1.8, indicates a contribution of fine and absorbing particles, which increases the SAE (see Fig. 8).
In general, these SAE and AAE values show that the simulated values with anthropogenic emissions are, on average, associated with the fine fraction of PM2.5 sampled particles. In contrast, some values are mostly associated with large-sized PM2.5 particles (SAE < 1), consistent with the Manaus plume not having a strong influence on the T3 site during those days. Conversely, the SAE with anthropogenic emissions (see Fig. 8b) shows a range between 0.5 and 2.1, with the values associated with the presence of fine aerosols originating from industrial activities in Manaus and the thermal power plants (TTPs) located in the surrounding area. The simulation with the Manaus plume turned off (see Fig. 8c) shows a coarse mode predominance, with SAE values varying mainly between 0.0 and 1.5. Thus, we can assume those values have a large OC contribution because of the predominance of aerosols coming from coarse mode biogenic sources.
The observed AAE values in the simulation without anthropogenic emissions express a large variability (1.1 to 1.8) compared to the ones from simulation with anthropogenic emissions (1 to 1.3). This behavior is assumed to be caused by the lack of a brown carbon component in the aerosol population in our simulation. When the anthropogenic emissions are off, the SAE variability is mostly related to the significant contribution from large aerosols, as already mentioned (Cazorla et al., 2013; Seinfeld and Pandis, 2016; Romano et al., 2019).
3.3.3 Asymmetry parameter
The gaer is an important optical property in radiative transfer, climate and general circulation models (Korras-Carraca et al., 2015). The gaer describes the angular distribution of scattered radiation and determines whether the particles preferentially scatter radiation forwards or backwards (Boucher, 2015).
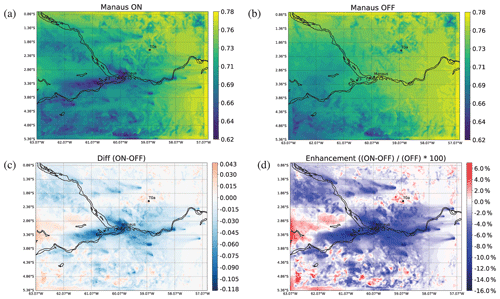
Figure 9WRF-Chem simulated values of 600 nm asymmetry parameter (gaer) in the presence or absence of Manaus emissions. (a) gaer, when all emissions are on. (b) gaer, when just biogenic emissions are on and anthropogenic emissions are off. (c) gaer difference between the two simulations, with anthropogenic emissions turned on and off, i.e., ON − OFF. (d) gaer (percent) enhancement calculated from the two simulations, when anthropogenic emissions turned on and off, i.e., ((ON − OFF) OFF) × 100. WRF-Chem predictions are at ca. 8 m altitude, averaged over 13 March 2014 (00:00–23:00 LT).
Figure 9a shows low 600 nm gaer values (0.64) that could be associated with industrial activities, such as TTPs and biomass burning, in nearby areas. A region of special interest is between Manaus and T3, since it hosts a large variety of mixing interactions between anthropogenic, biogenic and dust aerosols (e.g., Artaxo et al., 2002; Saturno et al., 2018; Martin et al., 2016; Rizzo et al., 2013). In this region, it can be seen that gaer decreases by 8 % compared with when there are no anthropogenic emissions (see Fig. 9d). This is associated with the presence of fine anthropogenic aerosols transported from adjacent urban and industrial areas in the northwest, especially from central Manaus (Medeiros et al., 2017; Abou Rafee et al., 2017; Shrivastava et al., 2019). Those smaller gaer values are seen in places where a significant fraction of the aerosol loading comes from small-sized particles of anthropogenic origin, with the smallest values appearing over the regions containing industrial activities. Previous studies (Cirino et al., 2018) have shown a period in the late afternoon around T3 in which particles with the smallest geometric diameter (ca. 50 nm) were observed, and the same period coincides with smaller gaer found in simulations for the same station (see Fig. 9a). On the other hand, when anthropogenic emissions are off, gaer predominately has large values varying between 0.75 and 0.76 at 300 nm, 0.73 and 0.75 at 400 nm, 0.71 and 0.74 at 600 nm and 0.63 and 0.71 at 1000 nm. These values indicate strong forward scattering of radiation by atmospheric aerosols and are related with the presence of coarse biogenic particles. According to the obtained results, anthropogenic emissions decrease gaer values by between 2 % and 16 %, especially in regions with large mobile and stationary anthropogenic activities. Those smaller values can induce modifications of the DREs.
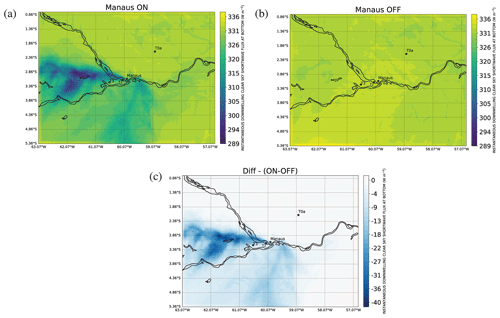
Figure 10WRF-Chem-simulated mean incoming solar radiation (instantaneous downwelling clear-sky shortwave flux at the bottom – SWDNBC), in watts per square meter, in the presence and absence of Manaus emissions. (a) SWDNBC, when all emissions are on. (b) SWDNBC, when biogenic emissions are on and anthropogenic emissions are off. (c) SWDNBC difference between the simulations, with and without anthropogenic emissions, i.e., ON − OFF.
3.3.4 Irradiance
In regions like the Amazon with sufficiently high levels of NOx, and VOCs, such as isoprenes and monoterpenes, an enhanced formation of near-surface O3 is expected. Solar radiation is another element that contributes to photochemical activity and, consequently, the formation of O3. In Figs. 10c and 4a, it is possible to notice that O3 formation near the surface is not reduced, even in regions with average surface downward shortwave flux values decreased by ca. 20 W m−2. This is due to the presence of anthropogenic emissions near T2 and T3, which more than compensate for the effects of the reduced solar radiation. The lower solar radiation over the west side of Manaus, seen in simulations with anthropogenic emissions (see Fig. 10a), is accompanied by a general increase in mean O3 values (see Fig. 4a). Studies of regional direct and indirect aerosol effects are important and still challenging due to their complexity, making an accurate determination of the direct and indirect effects difficult (Forkel et al., 2012; Wang et al., 2015; Zhang et al., 2010).
In our simulations, we considered both direct and indirect aerosol effects during the wet season in the Amazon region. Incoming shortwave radiation at the surface is predicted to drop by up to ca. 40 W m−2 due the direct aerosol effect. In regions within and up to ca. 100 km southwest of Manaus, Fig. 10c shows an aerosol cooling effect, with a maximum SWDNBC difference of ca. −40 W m−2. The same behavior can also be seen in the region northwest of the T3 site. The aerosol cooling effect is mostly related to SOA production caused by the interaction between VOCs and NOx. When the Manaus plume reaches regions downwind of the city, as seen on 13 March 2014 with few clouds, low precipitation and biomass burning, the plume has a cooling effect on the region as the plume evolves. The SWDNBC (clear sky) variable was used in this study to investigate the aerosol radiative effect on the surface due limitations in simulating the cloud coverage over the Amazonian region. The results found in this paper require further investigation to better understand the Manaus plume's effects on the diffuse and direct radiation.
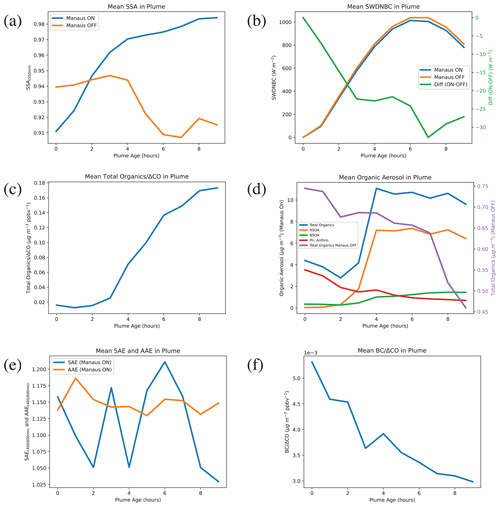
Figure 11Averaged aerosol concentrations and optical properties within simulated plume between 06:00 and 15:00 LT on 13 March 2014. (a) Single scattering albedo (SSA) at 550 nm, (b) instantaneous downwelling clear-sky shortwave flux at bottom (watts per square meter), (c) total organics (micrograms per cubic meter) normalized by ΔCO, (d) organic aerosol (micrograms per cubic meter), (e) SAE and AAE and (f) BC (micrograms per cubic meter) normalized by ΔCO. The plume age represents the time the plume tracking started. Tracking was accomplished with the HYSPLIT method described in section 2.1
3.4 Plume-aging impact on the optical properties
In this section, we examine how aging of the Manaus plume may affect its optical properties. SSA initially has low values of ca. 0.91, then increases after the plume is 1 h old (07:00 LT). Some processes which affect SSA values as the plume ages are dilution, BC deposition, SOA formation and the lensing effect (Holanda et al., 2020; Shrivastava et al., 2019; Cirino et al., 2018). The SSA values in the plume continue to increase during the plume-aging process, consistent with SOA (ASOA + BSOA) production over the plume lifetime (see Fig. 11d). Our simulations show that, on 13 March 2014, the increase in SSA as the plume ages is mostly related to a combination of an increase in SOA formation and BC dilution. Figure 11f shows that BC and CO diluted in similar proportions, suggesting that, over this timescale, dilution is more important than deposition. When the plume is 3 h old, total organics reach ca. 11 µg m−3, and at that time, the plume is north of the T3 site (see Fig. S15d). Similar results were found by de Sá et al. (2018) at the T3 site.
During plume aging, a decrease in anthropogenic primary organic aerosol and an increase in SOA was observed, similar to results reported by Shilling et al. (2018). The biggest contribution to total SOA during the plume aging comes from anthropogenic emissions, ca. 70 % of the total SOA. SOA production increases rapidly and saturates when the plume is 4 h old, indicating that it is a challenge to represent these processes in tropical regions with global models, especially without the correct treatments of subgrid effects, such as SOA production. The simulated plume used in the tracking analysis traveled 160 km from Manaus (Fig. S16). The distance between T1 and T3 is around 70 km, so when the plume reaches that distance from Manaus, it is ca. 3 h old.
Figure 11b shows instantaneous downwelling clear-sky shortwave flux at the bottom with the Manaus plume turned on and off. After 3 h of plume aging, incoming solar radiation is reduced by ca. −15 W m−2 and is further reduced by about −30 W m−2 after the plume is 7 h old. This reduction in solar flux by 3 %, and the resulting increase in diffuse radiation, results in a significant increase in net primary productivity (Cirino et al., 2014; Rap et al., 2015). As the plume ages and dilutes, its impact on the solar radiation remains constant. Between hours 7 and 9, although the plume's attenuation of incoming solar radiation decreases in absolute terms, from ca. −32 to −27 W m−2 as a percentage of total solar radiation, the plume's attenuation remains constant at ca. 3 %.
BC simulations at an altitude of ca. 500 m above the ground were evaluated using aircraft measurements from the Manaus plume on 13 March 2014. For the most part, our simulation shows good agreement with the G1 measurements (Fig. S19), particularly for background conditions. The offset in the third and fourth peaks is due to differences between the meteorological conditions of the simulation and reality. Similar offsets between simulations and observations were found by Shrivastava et al. (2019).
As the plume ages, SAE begins to increase at 08:00 LT (after 2 h of plume aging) and remains constant with values of ca. 1.17 until 13:00 LT (after 7 h of plume aging). During this period, AAE is mostly close to 1, which can be explained by increased concentrations of fine (SOA > 10 µg m−3) and absorbing (BC > 0.4 µg m−3) particles near Manaus. Similar results were found by Romano et al. (2019) in southeastern Italy, from 22 December 2015 to 30 March 2016, with 1 < AAE < 1.5 and SAE > 1, using the classification defined by Cappa et al. (2016).
Numerical simulations with the WRF-Chem model were performed in order to investigate the impact of the Manaus plume on secondary organic aerosol production and aerosol optical properties downwind of Manaus. This study also shows how the plume-aging process can affect aerosol optical properties. Modeling the interactions between anthropogenic and biogenic emissions allows us to better understand the effects of demographic changes taking place in areas surrounded by tropical forests. We used the simulations to investigate the impact of anthropogenic emissions on SOA formation over the Amazon region during the wet season and the effect of anthropogenic NOx on O3 production from VOC precursors emitted by the forest. Aerosol characteristics have important impacts on Amazonian ecosystem functioning on a regional and basin-wide scale. We focus on 13 March 2014, a golden day (Shilling et al., 2018), to analyze the Manaus plume's influence at the T3 site and regions further downwind. During this day, the plume transport event brought elevated gas and aerosol concentrations from Manaus, associated with favorable meteorological atmospheric transport conditions. The results from this study show that, downwind of Manaus at the T3 site, the total organic aerosol mass increases by ca. 75 % when anthropogenic emissions are present. This increase in organic aerosol mass suggests that the interaction between the Manaus plume and natural biogenic emissions is primarily responsible for the changes in the physical and chemical properties of the aerosol population in those regions.
From model experiments, we conclude that the influence of the Manaus plume can reach areas up to 300 km downwind of Manaus, and we also provide a quantitative assessment of the effects urban pollution can cause in Amazonian forests surrounding urban centers. Overall, the simulations show that the aerosol impact of the Manaus plume is an increase in ground irradiance values by 20 % near the T3 site. We also separated the contributions of the different aerosol components from our estimate of the total aerosol mass concentration and their impact on optical properties. Especially striking is the impact on O3 formation. Due to the high NOx concentrations present in Manaus, we show that increased O3 production (ca. 12–80 ppbv) mostly occurs in the regions southwest of Manaus, where an atmosphere favorable to O3 enrichment can be found.
According to our results, the lowest gaer values were generally found in regions with a significant fraction of the aerosol load coming from small-sized particles of anthropogenic origin, e.g., from TPPs and refineries in the Manaus region. Conversely, the largest gaer values were observed over regions with aerosol dominated by large particles of biogenic origin, such as the T0a site. Further investigations are necessary to determine if different SO4 amounts from anthropogenic emissions may change the strong direct effect for high aerosol particle concentrations. More ground-based aerosol and trace gas observations over the western Amazon region could help to further evaluate the magnitude of the aerosol effect in this area, but this is a mostly inaccessible region of pristine primary forests.
This study contributes to the investigation of the optical properties of PM2.5 over the Amazon region during the wet season, when no local biomass burning can be observed. To assess the impact of Manaus emissions on SOA production and, consequently, on aerosol optical properties, WRF-Chem model runs were conducted with and without anthropogenic emissions. With anthropogenic emissions turned off and only biogenic emissions and boundary conditions from the global model, OA production decreased by 75 % at the T3 site. This study also shows that, on 13 March 2014, the aerosol-aging process caused a gradual increase in SSA (ca. 0.91–98). Additionally, due to the deposition process, significantly decreasing concentrations of BC were found during plume evolution. This process, combined with SOA formation, contributes to the increase in SSA as the plume ages. The results of this study demonstrated that uncertainties in coating processes of organic aerosols involving BC particles also call for additional research to better account for decreasing SSA during the plume-aging process. In addition, the results here demonstrate that, in order to precisely calculate the radiative forcing impact, it is important to take into account all SOA formation mechanisms, including VOC oxidation, especially for tropical forest regions such as the Amazon.
Investigating the urban plume as it changes in time is a challenge, due to the complex meteorology, particularly in determining the effects of emissions from both Manaus and the surrounding tropical forest. The approach used in this study was able to show interesting results, quantifying OA formation in a source plume as it ages. The simulations showed an increase in the total organics normalized by ΔCO as the plume photochemically ages. The total organics concentration in the plume begins to increase rapidly when the plume is 2–4 h old, due to boundary layer growth and increased solar irradiance. This indicates active photochemistry, with VOCs being transformed into SOA. Our results also show that the majority of SOA produced as the plume ages is anthropogenic in origin. A possible direction for further study may be to compare the aging process in plumes emitted at different times of the day, over many days, and analyze how the aerosols and gases are affected by changes in irradiance and varying emissions profiles throughout the day. Future studies may be able to improve SOA model accuracy by using updated MEGAN model inputs when new data, such as emission factors and vegetation coverage for this region, become available. In addition, there is very little long-term ASOA and BSOA observational data, which could help evaluate models and improve their accuracy.
The atmospheric behaviors described in this study are applicable to other urban areas in the Amazon and may be mirrored in cities located in tropical forests around the world. Medium-sized cities, such as Belém, Santarém, Rio Branco, Porto Velho and others, can impact SOA and O3 production in ways similar to those shown in this study of Manaus. The increased SOA decreases total solar radiation flux but increases diffuse radiation. Several studies have shown that the forest enhances primary productivity when larger amounts of diffuse radiation are present. This strongly affects the carbon balance of undisturbed forests. Additionally, this study shows significant O3 production downwind of urban centers surrounded by forests with strong VOC emissions. O3 is known to affect plant productivity at levels above ca. 40 ppbv in temperate forest species. Very little is known about how O3 affects tropical species. The very large biodiversity and the very species-dependent impact of O3 makes an assessment of its effects on tropical forests a challenging task. These effects are not yet accounted for in regional and global models. Similar urbanization trends are occurring in other tropical forested regions in Africa and Southeast Asia, impacting SOA and O3 production. Studies similar to ours are important in tropical regions throughout the world.
The GoAmazon 2014–2015 experiment data are available from the ARM website (https://www.arm.gov/research/campaigns/amf2014goamazon, last access: 4 April 2020, ARM, 2013, 2014) and from the Laboratory of Atmospheric Physics (LFA) website (http://ftp.lfa.if.usp.br/ftp/public/LFA_Processed_Data/, last access: 4 April 2020).
The simulations and analysis code generated for this study are available upon request from the corresponding author, Janaína P. Nascimento.
The supplement related to this article is available online at: https://doi.org/10.5194/acp-21-6755-2021-supplement.
JPN, MMB and PA conceptualized and defined the methodology. JPN carried out the formal analysis and the investigation of the model results, with support from BM, MMB, ALB, HG, LVR, SC, HJB, MAF, MT, SAM and PA. ALVV, SAAR, HG and MMB supported the design and running of the simulations. GGC, PA, LVR, MLB, BM and RAFS collected and curated the experimental data. JPN wrote the original draft, and all authors discussed the results and commented on the paper.
The authors declare that they have no conflict of interest.
We acknowledge support from the central office of the Large-Scale Biosphere–Atmosphere Experiment in Amazonia (LBA), coordinated by the National Institute of Amazonian Research (INPA) and the Amazonas State University (UEA), Amazonas, Brazil. JPN thanks the Brazilian Federal Agency for Support and Evaluation of Graduate Education (CAPES) for a graduate fellowship, linked to the doctoral program in Climate and Environment (CLIAMB), and for supporting 7 months of a visiting graduate student program at the NOAA Earth System Research Laboratory. Janaína P. Nascimento also thanks the Institute of Physics of the University of São Paulo (IFUSP), for student mobility and logistical support, and CIRES and NOAA ESRL for financial and logistical support. We thank Michael Trainer for providing support and knowledge during the research. We thank Manish Shrivastava for providing WRF-Chem simulation output for comparison with this work. We thank Gilberto Fish for providing the planetary boundary layer observed data. We thank Steven Jefferts, Stefania Romisch and Samuel Brewer, for facilitating communication between members of this collaboration. We are grateful to Bruno Takeshi, Luiz Cândido, Renata Teixeira and Delano Campos, for instrument operation and data analysis. Finally, we thank Richard Tisinai, for IT support. Marco A. Franco acknowledges a scholarship from CNPq (grant no. 169842/2017-7), for supporting his doctoral studies at the IFUSP, São Paulo, Brazil, and CAPES (grant no. 88887.368025/2019-00), for supporting 6 months of a visiting graduate student program at the Max Planck Institute for Chemistry, Mainz, Germany. Bruno Meller acknowledges a scholarship from CNPq (grant no. 133393/2019-4), for supporting his Masters studies at the IFUSP, São Paulo, Brazil. Helber Gomes acknowledges funding from CAPES (grant no. 757/2017). Paulo Artaxo acknowledges funding from FAPESP (grant no. 2017/17047-0).
This research has been supported by the Fundação de Amparo à Pesquisa do Estado de São Paulo (grant no. 2017/17047-0) and the Coordenação de Aperfeiçoamento de Pessoal de Nível Superior (grant nos. 88882.444345/2018-01 and 88881.190103/2018-01).
This paper was edited by David Topping and reviewed by two anonymous referees.
Abdul-Razzak, H. and Ghan, S. J.: A parameterization of aerosol activation: 2. multiple aerosol types, J. Geophys. Res., 105, 6837–6844, https://doi.org/10.1029/1999JD901161, 2000. a
Abou Rafee, S. A., Martins, L. D., Kawashima, A. B., Almeida, D. S., Morais, M. V. B., Souza, R. V. A., Oliveira, M. B. L., Souza, R. A. F., Medeiros, A. S. S., Urbina, V., Freitas, E. D., Martin, S. T., and Martins, J. A.: Contributions of mobile, stationary and biogenic sources to air pollution in the Amazon rainforest: a numerical study with the WRF-Chem model, Atmos. Chem. Phys., 17, 7977–7995, https://doi.org/10.5194/acp-17-7977-2017, 2017. a, b, c, d, e, f, g
Ahmadov, R., McKeen, S., Robinson, A., Bahreini, R., Middlebrook, A., De Gouw, J., Meagher, J., Hsie, E.-Y., Edgerton, Shaw, S., and Trainer, M.: A volatility basis set model for summertime secondary organic aerosols over the eastern United States in 2006, J. Geophys. Res., 117, D06301, https://doi.org/10.1029/2011JD016831, 2012. a, b, c, d
Albuquerque, T. T. A., Andrade, M. F., and Ynoue, R. Y.: Characterization of atmospheric aerosols in the city of São Paulo, Brazil: comparisons between polluted and unpolluted periods, Environ. Monit. Assess., 184, 969–984, 2012. a
Alves, E. G., Jardine, K., Tota, J., Jardine, A., Yãnez-Serrano, A. M., Karl, T., Tavares, J., Nelson, B., Gu, D., Stavrakou, T., Martin, S., Artaxo, P., Manzi, A., and Guenther, A.: Seasonality of isoprenoid emissions from a primary rainforest in central Amazonia, Atmos. Chem. Phys., 16, 3903–3925, https://doi.org/10.5194/acp-16-3903-2016, 2016. a
Alves, E. G., Tóta, J., Turnipseed, A., Guenther, A. B., Vega Bustillos, J. O. W., Santana, R. A., Cirino, G. G., Tavares, J. V., Lopes, A. P., Nelson, B. W., de Souza, R. A., Gu, D., Stavrakou, T., Adams, D. K., Wu, J., Saleska, S., and Manzi, A. O.: Leaf phenology as one important driver of seasonal changes in isoprene emissions in central Amazonia, Biogeosciences, 15, 4019–4032, https://doi.org/10.5194/bg-15-4019-2018, 2018. a
Andreae, M. O., Artaxo, P., Beck, V., Bela, M., Freitas, S., Gerbig, C., Longo, K., Munger, J. W., Wiedemann, K. T., and Wofsy, S. C.: Carbon monoxide and related trace gases and aerosols over the Amazon Basin during the wet and dry seasons, Atmos. Chem. Phys., 12, 6041–6065, https://doi.org/10.5194/acp-12-6041-2012, 2012. a
Andreae, M. O., Acevedo, O. C., Araùjo, A., Artaxo, P., Barbosa, C. G. G., Barbosa, H. M. J., Brito, J., Carbone, S., Chi, X., Cintra, B. B. L., da Silva, N. F., Dias, N. L., Dias-Júnior, C. Q., Ditas, F., Ditz, R., Godoi, A. F. L., Godoi, R. H. M., Heimann, M., Hoffmann, T., Kesselmeier, J., Könemann, T., Krüger, M. L., Lavric, J. V., Manzi, A. O., Lopes, A. P., Martins, D. L., Mikhailov, E. F., Moran-Zuloaga, D., Nelson, B. W., Nölscher, A. C., Santos Nogueira, D., Piedade, M. T. F., Pöhlker, C., Pöschl, U., Quesada, C. A., Rizzo, L. V., Ro, C.-U., Ruckteschler, N., Sá, L. D. A., de Oliveira Sá, M., Sales, C. B., dos Santos, R. M. N., Saturno, J., Schöngart, J., Sörgel, M., de Souza, C. M., de Souza, R. A. F., Su, H., Targhetta, N., Tóta, J., Trebs, I., Trumbore, S., van Eijck, A., Walter, D., Wang, Z., Weber, B., Williams, J., Winderlich, J., Wittmann, F., Wolff, S., and Yáñez-Serrano, A. M.: The Amazon Tall Tower Observatory (ATTO): overview of pilot measurements on ecosystem ecology, meteorology, trace gases, and aerosols, Atmos. Chem. Phys., 15, 10723–10776, https://doi.org/10.5194/acp-15-10723-2015, 2015. a, b, c
ARM: Atmospheric Radiation Measurement (ARM) user facility, updated hourly. Aerosol Intensive Properties (AIP1OGREN), 2014-01-31–2014-03-31, ARM Mobile Facility (MAO) Manacapuru, Amazonas, Brazil; AMF1 (M1), compiled by Flynn, C., ARM Data Center, https://doi.org/10.5439/1027280, 2013. a
ARM: Atmospheric Radiation Measurement (ARM) user facility, updated hourly. Aethalometer (AOSAETH), 2014-01-31–2014-04-30, ARM Mobile Facility (MAO) Manacapuru, Amazonas, Brazil; MAOS (S1), compiled by: Sedlacek, A., Andrews, E., and Salwen, C., ARM Data Center, https://doi.org/10.5439/1095580, 2014. a
Artaxo, P., Maenhaut, W., Storms, H., and Van Grieken, R.: Aerosol characteristics and sources for the Amazon Basin during the wet season, J. Geophys. Res.-Atmos., 95, 16971–16985, https://doi.org/10.1029/JD095iD10p16971, 1990. a
Artaxo, P., Yamasoe, M., Martins, J., Kocinas, S., Car-Vallo, S., and Maenhaut, W.: Case study of atmospheric measurements in Brazil : aerosol emissions from Amazon Basin fires, in: Environmental Sciences Research Report, edited by: Crutzen, P. J. and Goldammer, J., Wiley, 13, 139–158, 1993. a
Artaxo, P., Gerab, F., Yamasoe, M. A., and Martins, J. V.: Fine mode aerosol composition at three long-term atmospheric monitoring sites in the Amazon Basin, J. Geophys. Res.-Atmos., 99, 22857–22868, https://doi.org/10.1029/94JD01023, 1994. a
Artaxo, P., Martins, J. V., Yamasoe, M. A., Procópio, A. S., Pauliquevis, T. M., Andreae, M. O., Guyon, P., Gatti, L. V., and Leal, A. M. C.: Physical and chemical properties of aerosols in the wet and dry seasons in Rondônia, Amazonia, J. Geophys. Res., 107, 8081, https://doi.org/10.1029/2001JD000666, 2002. a
Artaxo, P., Rizzo, L. V., Brito, J. F., Barbosa, H. M., Arana, A., Sena, E. T., Cirino, G. G., Bastos, W., Martin, S. T., and Andreae, M. O.: Atmospheric aerosols in Amazonia and land use change: from natural biogenic to biomass burning conditions, Faraday Discuss., 165, 203–235, https://doi.org/10.1039/C3FD00052D, 2013. a, b, c
Artaxo, P., Hansson, H.-C., Andreae, M. O., Bäck, J., Alves, E. G., Barbosa, H. M. J., Bender, F., Bourtsoukidis, E., Carbone, S., Chi, J., Decesari, S., Després, V. R., Ditas, F., Ezhova, E., Fuzzi, S., Hasselquist, N. J., Heintzenberg, J., Holanda, B. A., Guenther, A., Hakola, H., Heikkinen, L., Kerminen, V.-M., Kontkanen, J., Krejci, R., Kulmala, M., Lavric, J., de Leeuw, G., Lehtipalo, K., Machado, L. A. T., McFiggans, G., Franco, M. A. M., Mohr, C., Morgan, W., Nilsson, M. B., Peichl, M., Petäjä, T., Praß, M., Pöhlker, C., Pöhlker, M. L., Pöschl, U., Randow, C. V., Riipinen, I., Rinne, J., Rizzo, L. V., Rosenfeld, D., Dias, M. A. F. S., Sogacheva, L., Stier, P., Swietlicki, E., Sörgel, M., Tunved, P., Virkkula, A., Wang, J., Weber, B., Yáñez-Serrano, A. M., Zieger, P., Mikhailov, E., Smith, J., and Kesselmeier, J.: Tropical and Boreal Forest – Atmosphere Interactions: A Review, Tellus B, in review, 2020. a, b
Backman, J., Rizzo, L. V., Hakala, J., Nieminen, T., Manninen, H. E., Morais, F., Aalto, P. P., Siivola, E., Carbone, S., Hillamo, R., Artaxo, P., Virkkula, A., Petäjä, T., and Kulmala, M.: On the diurnal cycle of urban aerosols, black carbon and the occurrence of new particle formation events in springtime São Paulo, Brazil, Atmos. Chem. Phys., 12, 11733–11751, https://doi.org/10.5194/acp-12-11733-2012, 2012. a
Bela, M. M., Barth, M. C., Toon, O. B., Fried, A., Homeyer, C. R., Morrison, H., Cummings, K. A., Li, Y., Pickering, K. E., Allen, D. J., Yang, Q., Wennberg, P. O., Crounse, J. D., St. Clair, J. M., Teng, A. P., O’Sullivan, D., Huey, L. G., Chen, D., Liu, X., Blake, D. R., Blake, N. J., Apel, E. C., Hornbrook, R. S., Flocke, F., Campos, T., and Diskin, G.: Wet scavenging of soluble gases in DC3 deep convective storms using WRF-Chem simulations and aircraft observations, J. Geophys. Res.-Atmos., 121, 4233–4257, https://doi.org/10.1002/2015JD024623, 2016. a
Ben-Ami, Y., Koren, I., Rudich, Y., Artaxo, P., Martin, S. T., and Andreae, M. O.: Transport of North African dust from the Bodélé depression to the Amazon Basin: a case study, Atmos. Chem. Phys., 10, 7533–7544, https://doi.org/10.5194/acp-10-7533-2010, 2010. a
Bezdek, J. C., Ehrlich, R., and Full, W.: FCM: The fuzzy c-means clustering algorithm, Comput. Geosci., 10, 191–203, https://doi.org/10.1016/0098-3004(84)90020-7, 1984. a
Boucher, O.: Atmospheric Aerosols: Properties and Climate Impacts, Springer, 2013. a
Boucher, O.: Atmospheric Aerosols: Properties and Climate Impacts, Springer Netherlands, https://doi.org/10.1007/978-94-017-9649-1, 2015. a, b
Brito, J., Rizzo, L. V., Herckes, P., Vasconcellos, P. C., Caumo, S. E. S., Fornaro, A., Ynoue, R. Y., Artaxo, P., and Andrade, M. F.: Physical–chemical characterisation of the particulate matter inside two road tunnels in the São Paulo Metropolitan Area, Atmos. Chem. Phys., 13, 12199–12213, https://doi.org/10.5194/acp-13-12199-2013, 2013. a
Brito, J., Rizzo, L. V., Morgan, W. T., Coe, H., Johnson, B., Haywood, J., Longo, K., Freitas, S., Andreae, M. O., and Artaxo, P.: Ground-based aerosol characterization during the South American Biomass Burning Analysis (SAMBBA) field experiment, Atmos. Chem. Phys., 14, 12069–12083, https://doi.org/10.5194/acp-14-12069-2014, 2014. a
Cappa, C. D., Kolesar, K. R., Zhang, X., Atkinson, D. B., Pekour, M. S., Zaveri, R. A., Zelenyuk, A., and Zhang, Q.: Understanding the optical properties of ambient sub- and supermicron particulate matter: results from the CARES 2010 field study in northern California, Atmos. Chem. Phys., 16, 6511–6535, https://doi.org/10.5194/acp-16-6511-2016, 2016. a
Carneiro, R. G. and Fisch, G.: Observational analysis of the daily cycle of the planetary boundary layer in the central Amazon during a non-El Niño year and El Niño year (GoAmazon project 2014/5), Atmos. Chem. Phys., 20, 5547–5558, https://doi.org/10.5194/acp-20-5547-2020, 2020. a
Cazorla, A., Bahadur, R., Suski, K. J., Cahill, J. F., Chand, D., Schmid, B., Ramanathan, V., and Prather, K. A.: Relating aerosol absorption due to soot, organic carbon, and dust to emission sources determined from in-situ chemical measurements, Atmos. Chem. Phys., 13, 9337–9350, https://doi.org/10.5194/acp-13-9337-2013, 2013. a, b
Chen, Q., Farmer, D. K., Rizzo, L. V., Pauliquevis, T., Kuwata, M., Karl, T. G., Guenther, A., Allan, J. D., Coe, H., Andreae, M. O., Pöschl, U., Jimenez, J. L., Artaxo, P., and Martin, S. T.: Submicron particle mass concentrations and sources in the Amazonian wet season (AMAZE-08), Atmos. Chem. Phys., 15, 3687–3701, https://doi.org/10.5194/acp-15-3687-2015, 2015. a
Cirino, G., Brito, J., Barbosa, H. M. J., Rizzo, L. V., Tunved, P., de Sá, S. S., Jimenez, J. L., Palm, B. B., Carbone, S., Lavric, J., Souza, R. A. F., Wolff, S., Walter, D., Tota, J., Oliveira, M. B. L., Martin, S. T., and Artaxo, P.: Observations of Manaus urban plume evolution and interaction with biogenic emissions in GoAmazon 2014/5, Atmos. Environ., 191, 513–524, https://doi.org/10.1016/j.atmosenv.2018.08.031, 2018. a, b, c, d, e, f
Cirino, G. G., Souza, R. A. F., Adams, D. K., and Artaxo, P.: The effect of atmospheric aerosol particles and clouds on net ecosystem exchange in the Amazon, Atmos. Chem. Phys., 14, 6523–6543, https://doi.org/10.5194/acp-14-6523-2014, 2014. a
Cosgrove, B. A., Lohmann, D., Kenneth, M. E., Houser, P. R., Wood, E. F., Schaake, J. C., Robock, A., Marshall, C., Sheffield, J., Duan, Q., Lifeng, L., Higgins, W. R., Pinker, R. T., Tarpley, D. J., and Meng, J.: Real-time and retrospective forcing in the North American Land Data Assimilation System (NLDAS) project, J. Geophys. Res.-Atmos., 108, 8842, https://doi.org/10.1029/2002JD003118, 2003. a
de Sá, S. S., Palm, B. B., Campuzano-Jost, P., Day, D. A., Hu, W., Isaacman-VanWertz, G., Yee, L. D., Brito, J., Carbone, S., Ribeiro, I. O., Cirino, G. G., Liu, Y., Thalman, R., Sedlacek, A., Funk, A., Schumacher, C., Shilling, J. E., Schneider, J., Artaxo, P., Goldstein, A. H., Souza, R. A. F., Wang, J., McKinney, K. A., Barbosa, H., Alexander, M. L., Jimenez, J. L., and Martin, S. T.: Urban influence on the concentration and composition of submicron particulate matter in central Amazonia, Atmos. Chem. Phys., 18, 12185–12206, https://doi.org/10.5194/acp-18-12185-2018, 2018. a, b, c, d, e, f, g, h
de Sá, S. S., Rizzo, L. V., Palm, B. B., Campuzano-Jost, P., Day, D. A., Yee, L. D., Wernis, R., Isaacman-VanWertz, G., Brito, J., Carbone, S., Liu, Y. J., Sedlacek, A., Springston, S., Goldstein, A. H., Barbosa, H. M. J., Alexander, M. L., Artaxo, P., Jimenez, J. L., and Martin, S. T.: Contributions of biomass-burning, urban, and biogenic emissions to the concentrations and light-absorbing properties of particulate matter in central Amazonia during the dry season, Atmos. Chem. Phys., 19, 7973–8001, https://doi.org/10.5194/acp-19-7973-2019, 2019. a
de Souza, D. O. and dos Santos Alvalá, R. C.: Observational evidence of the urban heat island of Manaus City, Brazil, Meteorol. Appl., 21, 186–193, 2014. a
dos Santos, M. J., Silva Dias, M. A. F., and Freitas, E. D.: Influence of local circulations on wind, moisture, and precipitation close to Manaus City, Amazon Region, Brazil, J. Geophys. Res.-Atmos., 119, 13233–13249, https://doi.org/10.1002/2014JD021969, 2014. a, b
Draxler, R. R.: Demonstration of a global modeling methodology to determine the relative importance of local and long-distance sources, Atmos. Environ., 41, 776–789, https://doi.org/10.1016/j.atmosenv.2006.08.052, 2007. a
Drinovec, L., Močnik, G., Zotter, P., Prévôt, A. S. H., Ruckstuhl, C., Coz, E., Rupakheti, M., Sciare, J., Müller, T., Wiedensohler, A., and Hansen, A. D. A.: The ”dual-spot” Aethalometer: an improved measurement of aerosol black carbon with real-time loading compensation, Atmos. Meas. Tech., 8, 1965–1979, https://doi.org/10.5194/amt-8-1965-2015, 2015. a
Dubovik, O. and King, M. D.: A flexible inversion algorithm for retrieval of aerosol optical properties from Sun and sky radiance measurements, J. Geophys. Res.-Atmos., 105, 20673–20696, 2000. a
Easter, R. C., Ghan, S. J., Zhang, Y., Saylor, R. D., Chapman, E. G., Laulainen, N. S., Abdul-Razzak, H., Leung, L. R., Bian, X., and Zaveri, R. A.: MIRAGE: Model description and evaluation of aerosols and trace gases, J. Geophys. Res.-Atmos., 109, D20210, https://doi.org/10.1029/2004JD004571, 2004. a
Fast, J. D., Gustafson, W. I., Easter, R. C., Zaveri, R. A., Barnard, J. C., Chapman, E. G., Grell, G. A., and Peckham, S. E.: Evolution of ozone, particulates, and aerosol direct radiative forcing in the vicinity of Houston using a fully coupled meteorology-chemistry-aerosol model, J. Geophys. Res.-Atmos., 111, D21305, https://doi.org/10.1029/2005JD006721, 2006. a, b
Fisch, G., Marengo, J. A., and Nobre, C. A.: Uma revisão geral sobre o clima da Amazônia, Acta amazônica, 28, 101–101, 1998. a, b
Forkel, R., Werhahn, J., Hansen, A. B., McKeen, S., Peckham, S., Grell, G., and Suppan, P.: Effect of aerosol-radiation feedback on regional air quality – A case study with WRF/Chem, Atmos. Environ., 53, 202–211, https://doi.org/10.1016/j.atmosenv.2011.10.009, 2012. a, b
Fraund, M., Pham, D. Q., Bonanno, D., Harder, T., Wang, B., Brito, J., de Sá, S. S., Carbone, S., China, S., Artaxo, P., Martin, S., Pöhlker, C., Andreae, M., Laskin, A., Gilles, M., and Moffet, R.: Elemental mixing state of aerosol particles collected in Central Amazonia during GoAmazon2014/15, Atmosphere, 8, 173, https://doi.org/10.3390/atmos8090173, 2017. a
Graham, B., Guyon, P., Maenhaut, W., Taylor, P. E., Ebert, M., Matthias-Maser, S., Mayol-Bracero, O. L., Godoi, R. H. M., Artaxo, P., Meixner, F. X., Lima Moura, M. A., Eça D’Almeida Rocha, C. H., Van Grieken, R., Glovsky, M. M., Flagan, R. C., and Andreae, M. O.: Composition and diurnal variability of the natural Amazonian aerosol, J. Geophys. Res.-Atmos., 108, 4765, https://doi.org/10.1029/2003JD004049, 2003a. a
Graham, B., Guyon, P., Taylor, P. E., Artaxo, P., Maenhaut, W., Glovsky, M. M., Flagan, R. C., and Andreae, M. O.: Organic compounds present in the natural Amazonian aerosol: Characterization by gas chromatography–mass spectrometry, J. Geophys. Res.-Atmos., 108, 4766, https://doi.org/10.1029/2003JD003990, 2003b. a
Grell, G. A., Peckham, S. E., Schmitz, R., McKeen, S., Frost, G., Skamarock, W. C., and Eder, B.: Fully coupled “online” chemistry within the WRF model, Atmos. Environ., 39, 6957–6975, https://doi.org/10.1016/j.atmosenv.2005.04.027, 2005. a, b
Grell, G. A. and Freitas, S. R.: A scale and aerosol aware stochastic convective parameterization for weather and air quality modeling, Atmos. Chem. Phys., 14, 5233–5250, https://doi.org/10.5194/acp-14-5233-2014, 2014. a, b
Gu, D., Guenther, A. B., Shilling, J. E., Yu, H., Huang, M., Zhao, C., Yang, Q., Martin, S. T., Artaxo, P., Kim, S., Seco, R., Stavrakou, T., Longo, K. M., Tóta, J., de Souza, R. A. F., Vega, O., Liu, Y., Shrivastava, M., Alves, E. G., Santos, F. C., Leng, G., and Hu, Z.: Airborne observations reveal elevational gradient in tropical forest isoprene emissions, Nat. Commun., 8, 1–7, https://doi.org/10.1038/ncomms15541, 2017. a
Guenther, A., Karl, T., Harley, P., Wiedinmyer, C., Palmer, P. I., and Geron, C.: Estimates of global terrestrial isoprene emissions using MEGAN (Model of Emissions of Gases and Aerosols from Nature), Atmos. Chem. Phys., 6, 3181–3210, https://doi.org/10.5194/acp-6-3181-2006, 2006. a, b
Haywood, J. and Boucher, O.: Estimates of the direct and indirect radiative forcing due to tropospheric aerosols: A review, Rev. Geophys., 38, 513–543, https://doi.org/10.1029/1999RG000078, 2000. a
He, X., Li, C. C., Lau, A. K. H., Deng, Z. Z., Mao, J. T., Wang, M. H., and Liu, X. Y.: An intensive study of aerosol optical properties in Beijing urban area, Atmos. Chem. Phys., 9, 8903–8915, https://doi.org/10.5194/acp-9-8903-2009, 2009. a
Holanda, B. A., Pöhlker, M. L., Walter, D., Saturno, J., Sörgel, M., Ditas, J., Ditas, F., Schulz, C., Franco, M. A., Wang, Q., Donth, T., Artaxo, P., Barbosa, H. M. J., Borrmann, S., Braga, R., Brito, J., Cheng, Y., Dollner, M., Kaiser, J. W., Klimach, T., Knote, C., Krüger, O. O., Fütterer, D., Lavrič, J. V., Ma, N., Machado, L. A. T., Ming, J., Morais, F. G., Paulsen, H., Sauer, D., Schlager, H., Schneider, J., Su, H., Weinzierl, B., Walser, A., Wendisch, M., Ziereis, H., Zöger, M., Pöschl, U., Andreae, M. O., and Pöhlker, C.: Influx of African biomass burning aerosol during the Amazonian dry season through layered transatlantic transport of black carbon-rich smoke, Atmos. Chem. Phys., 20, 4757–4785, https://doi.org/10.5194/acp-20-4757-2020, 2020. a
Hong, S.-Y., Noh, Y., and Dudhia, J.: A new vertical diffusion package with an explicit treatment of entrainment processes, Mon. Weather Rev., 134, 2318–2341, https://doi.org/10.1175/MWR3199.1, 2006. a, b
Iacono, M. J., Delamere, J. S., Mlawer, E. J., Shephard, M. W., Clough, S. A., and Collins, W. D.: Radiative forcing by long-lived greenhouse gases: Calculations with the AER radiative transfer models, J. Geophys. Res.-Atmos., 113, D13103, https://doi.org/10.1029/2008JD009944, 2008. a, b
Jiménez, P. A., Dudhia, J., González-Rouco, J. F., Navarro, J., Montávez, J. P., and García-Bustamante, E.: A revised scheme for the WRF surface layer formulation, Mon. Weather Rev., 140, 898–918, https://doi.org/10.1175/MWR-D-11-00056.1, 2012. a, b
Korras-Carraca, M. B., Hatzianastassiou, N., Matsoukas, C., Gkikas, A., and Papadimas, C. D.: The regime of aerosol asymmetry parameter over Europe, the Mediterranean and the Middle East based on MODIS satellite data: evaluation against surface AERONET measurements, Atmos. Chem. Phys., 15, 13113–13132, https://doi.org/10.5194/acp-15-13113-2015, 2015. a, b, c
Kroll, J. H. and Seinfeld, J. H.: Chemistry of secondary organic aerosol: Formation and evolution of low-volatility organics in the atmosphere, , Atmos. Environ., 42, 3593–3624, 2008. a
Kuhn, U., Ganzeveld, L., Thielmann, A., Dindorf, T., Schebeske, G., Welling, M., Sciare, J., Roberts, G., Meixner, F. X., Kesselmeier, J., Lelieveld, J., Kolle, O., Ciccioli, P., Lloyd, J., Trentmann, J., Artaxo, P., and Andreae, M. O.: Impact of Manaus City on the Amazon Green Ocean atmosphere: ozone production, precursor sensitivity and aerosol load, Atmos. Chem. Phys., 10, 9251–9282, https://doi.org/10.5194/acp-10-9251-2010, 2010. a, b
Lim, S., Lee, M., Kim, S.-W., Yoon, S.-C., Lee, G., and Lee, Y. J.: Absorption and scattering properties of organic carbon versus sulfate dominant aerosols at Gosan climate observatory in Northeast Asia, Atmos. Chem. Phys., 14, 7781–7793, https://doi.org/10.5194/acp-14-7781-2014, 2014. a
Lu, L., Denning, A. S., da Silva-Dias, M. A., da Silva-Dias, P., Longo, M., Freitas, S. R., and Saatchi, S.: Mesoscale circulations and atmospheric CO2 variations in the Tapajós Region, Pará, Brazil, J. Geophys. Res.-Atmos., 110, 1–17, https://doi.org/10.1029/2004JD005757, 2005. a
Madronich, S.: Photodissociation in the atmosphere: 1. Actinic flux and the effects of ground reflections and clouds, J. Geophys. Res.-Atmos., 92, 9740–9752, https://doi.org/10.1029/JD092iD08p09740, 1987. a
Marengo, J. A., Nobre, C. A., and Culf, A. D.: Climatic impacts of “friagens” in forested and deforested areas of the Amazon basin, J. Appl. Meteorol., 36, 1553–1566, https://doi.org/10.1175/1520-0450(1997)036<1553:CIOFIF>2.0.CO;2, 1997. a
Marinho, R. R., Filizola Junior, N. P., and Cremon, É. H.: Analysis of Suspended Sediment in the Anavilhanas Archipelago, Rio Negro, Amazon Basin, Water, 12, 1073, https://doi.org/10.3390/w12041073, 2020. a
Martin, S. T., Artaxo, P., Machado, L. A. T., Manzi, A. O., Souza, R. A. F., Schumacher, C., Wang, J., Andreae, M. O., Barbosa, H. M. J., Fan, J., Fisch, G., Goldstein, A. H., Guenther, A., Jimenez, J. L., Pöschl, U., Silva Dias, M. A., Smith, J. N., and Wendisch, M.: Introduction: Observations and Modeling of the Green Ocean Amazon (GoAmazon2014/5), Atmos. Chem. Phys., 16, 4785–4797, https://doi.org/10.5194/acp-16-4785-2016, 2016. a, b, c, d, e, f
Martin, S. T., Andreae, M. O., Althausen, D., Artaxo, P., Baars, H., Borrmann, S., Chen, Q., Farmer, D. K., Guenther, A., Gunthe, S. S., Jimenez, J. L., Karl, T., Longo, K., Manzi, A., Müller, T., Pauliquevis, T., Petters, M. D., Prenni, A. J., Pöschl, U., Rizzo, L. V., Schneider, J., Smith, J. N., Swietlicki, E., Tota, J., Wang, J., Wiedensohler, A., and Zorn, S. R.: An overview of the Amazonian Aerosol Characterization Experiment 2008 (AMAZE-08), Atmos. Chem. Phys., 10, 11415–11438, https://doi.org/10.5194/acp-10-11415-2010, 2010. a
Martin, S. T., Artaxo, P., Machado, L., Manzi, A. O., Souza, R., Schumacher, C., Wang, J., Biscaro, T., Brito, J., Calheiros, A., Jardine, K., Medeiros, A., Portela, B., Sá, S. S. d., Adachi, K., Aiken, A. C., Albrecht, R., Alexander, L., Andreae, M. O., Barbosa, H. M. J., Buseck, P., Chand, D., Comstock, J. M., Day, D. A., Dubey, M., Fan, J., Fast, J., Fisch, G., Fortner, E., Giangrande, S., Gilles, M., Goldstein, A. H., Guenther, A., Hubbe, J., Jensen, M., Jimenez, J. L., Keutsch, F. N., Kim, S., Kuang, C., Laskin, A., McKinney, K., Mei, F., Miller, M., Nascimento, R., Pauliquevis, T., Pekour, M., Peres, J., Petäjä, T., Pöhlker, C., Pöschl, U., Rizzo, L., Schmid, B., Shilling, J. E., Dias, M. A. S., Smith, J. N., Tomlinson, J. M., Tóta, J., and Wendisch, M.: The Green Ocean Amazon experiment (GoAmazon2014/5) observes pollution affecting gases, aerosols, clouds, and rainfall over the rain forest, B. Am. Meteorol. Soc., 98, 981–997, https://doi.org/10.1175/BAMS-D-15-00221.1, 2017. a, b, c
Martins, L. D., Andrade, M. F., Freitas, E. D., Pretto, A., Gatti, L. V., Albuquerque, É. L., Tomaz, E., Guardani, M. L., Martins, M. H., and Junior, O. M.: Emission factors for gas-powered vehicles traveling through road tunnels in São Paulo, Brazil, Environ. Sci. Technol., 40, 6722–6729, 2006. a
Medeiros, A. S. S., Calderaro, G., Guimarães, P. C., Magalhaes, M. R., Morais, M. V. B., Rafee, S. A. A., Ribeiro, I. O., Andreoli, R. V., Martins, J. A., Martins, L. D., Martin, S. T., and Souza, R. A. F.: Power plant fuel switching and air quality in a tropical, forested environment, Atmos. Chem. Phys., 17, 8987–8998, https://doi.org/10.5194/acp-17-8987-2017, 2017. a, b, c, d
Mie, G.: Beiträge zur Optik trüber Medien, speziell kolloidaler Metallösungen, Ann. Phys., 330, 377–445, 1908. a
Miranda, R. M. and Andrade, M. F.: Physicochemical characteristics of atmospheric aerosol during winter in the São Paulo Metropolitan area in Brazil, Atmos. Environ, 39, 6188–6193, 2005. a
Moran-Zuloaga, D., Ditas, F., Walter, D., Saturno, J., Brito, J., Carbone, S., Chi, X., Hrabě de Angelis, I., Baars, H., Godoi, R. H. M., Heese, B., Holanda, B. A., Lavrič, J. V., Martin, S. T., Ming, J., Pöhlker, M. L., Ruckteschler, N., Su, H., Wang, Y., Wang, Q., Wang, Z., Weber, B., Wolff, S., Artaxo, P., Pöschl, U., Andreae, M. O., and Pöhlker, C.: Long-term study on coarse mode aerosols in the Amazon rain forest with the frequent intrusion of Saharan dust plumes, Atmos. Chem. Phys., 18, 10055–10088, https://doi.org/10.5194/acp-18-10055-2018, 2018. a, b
Morrison, H., Thompson, G., and Tatarskii, V.: Impact of cloud microphysics on the development of trailing stratiform precipitation in a simulated squall line: Comparison of one-and two-moment schemes, Mon. Weather Rev., 137, 991–1007, https://doi.org/10.1175/2008MWR2556.1, 2009. a, b
Müller, T., Henzing, J. S., de Leeuw, G., Wiedensohler, A., Alastuey, A., Angelov, H., Bizjak, M., Collaud Coen, M., Engström, J. E., Gruening, C., Hillamo, R., Hoffer, A., Imre, K., Ivanow, P., Jennings, G., Sun, J. Y., Kalivitis, N., Karlsson, H., Komppula, M., Laj, P., Li, S.-M., Lunder, C., Marinoni, A., Martins dos Santos, S., Moerman, M., Nowak, A., Ogren, J. A., Petzold, A., Pichon, J. M., Rodriquez, S., Sharma, S., Sheridan, P. J., Teinilä, K., Tuch, T., Viana, M., Virkkula, A., Weingartner, E., Wilhelm, R., and Wang, Y. Q.: Characterization and intercomparison of aerosol absorption photometers: result of two intercomparison workshops, Atmos. Meas. Tech., 4, 245–268, https://doi.org/10.5194/amt-4-245-2011, 2011. a
Murphy, B. N. and Pandis, S. N.: Simulating the formation of semivolatile primary and secondary organic aerosol in a regional chemical transport model, Environ. Sci. Technol., 43, 4722–4728, https://doi.org/10.1021/es803168a, 2009. a
Nobre, C. A., Obregón, G. O., , J. A., Fu, R., and Poveda, G.: Characteristics of Amazonian climate: Main features, Amazonia and Global Change, edited by: Keller, M., Bustamante, M., Gash, J., Silva Dias, P., Geophys. Mon. Ser, 186, 149–162, https://doi.org/10.1029/2002JD002911, 2009. a
Palacios, R. d. S., Romera, K. S., Curado, L. F., Banga, N. M., Rothmund, L. D., Sallo, F. d. S., Morais, D., Santos, A. C., Moraes, T. J., Morais, F. G., Santos, A. C., Moraes, T. J., Morais, F. G., Landufo, E., Franco, M, A. d. M., Kuhnen, I. A., Marques, J, B., Nogueira, J. d. S., Valle Junior, L, C., and Rodrigues, T.: Long term analysis of optical and radiative properties of aerosols in the Amazon Basin, Aerosol Air Qual. Res., https://doi.org/10.4209/aaqr.2019.04.0189, 2020. a, b
Palm, B. B., de Sá, S. S., Day, D. A., Campuzano-Jost, P., Hu, W., Seco, R., Sjostedt, S. J., Park, J.-H., Guenther, A. B., Kim, S., Brito, J., Wurm, F., Artaxo, P., Thalman, R., Wang, J., Yee, L. D., Wernis, R., Isaacman-VanWertz, G., Goldstein, A. H., Liu, Y., Springston, S. R., Souza, R., Newburn, M. K., Alexander, M. L., Martin, S. T., and Jimenez, J. L.: Secondary organic aerosol formation from ambient air in an oxidation flow reactor in central Amazonia, Atmos. Chem. Phys., 18, 467–493, https://doi.org/10.5194/acp-18-467-2018, 2018. a
Papiez, M. R., Potosnak, M. J., Goliff, W. S., Guenther, A. B., Matsunaga, S. N., and Stockwell, W. R.: The impacts of reactive terpene emissions from plants on air quality in Las Vegas, Nevada, Atmos. Environ, 43, 4109–4123, https://doi.org/10.1016/j.atmosenv.2009.05.048, 2009. a
Pereira Oliveira, A. and Fitzjarrald, D. R.: The Amazon river breeze and the local boundary layer: I. Observations, Bound.-Lay. Meteorol., 63, 141–162, https://doi.org/10.1007/BF00705380, 1993. a
Pöhlker, C., Walter, D., Paulsen, H., Könemann, T., Rodríguez-Caballero, E., Moran-Zuloaga, D., Brito, J., Carbone, S., Degrendele, C., Després, V. R., Ditas, F., Holanda, B. A., Kaiser, J. W., Lammel, G., Lavrič, J. V., Ming, J., Pickersgill, D., Pöhlker, M. L., Praß, M., Löbs, N., Saturno, J., Sörgel, M., Wang, Q., Weber, B., Wolff, S., Artaxo, P., Pöschl, U., and Andreae, M. O.: Land cover and its transformation in the backward trajectory footprint region of the Amazon Tall Tower Observatory, Atmos. Chem. Phys., 19, 8425–8470, https://doi.org/10.5194/acp-19-8425-2019, 2019. a, b
Pöhlker, M. L., Ditas, F., Saturno, J., Klimach, T., Hrabě de Angelis, I., Araùjo, A. C., Brito, J., Carbone, S., Cheng, Y., Chi, X., Ditz, R., Gunthe, S. S., Holanda, B. A., Kandler, K., Kesselmeier, J., Könemann, T., Krüger, O. O., Lavrič, J. V., Martin, S. T., Mikhailov, E., Moran-Zuloaga, D., Rizzo, L. V., Rose, D., Su, H., Thalman, R., Walter, D., Wang, J., Wolff, S., Barbosa, H. M. J., Artaxo, P., Andreae, M. O., Pöschl, U., and Pöhlker, C.: Long-term observations of cloud condensation nuclei over the Amazon rain forest – Part 2: Variability and characteristics of biomass burning, long-range transport, and pristine rain forest aerosols, Atmos. Chem. Phys., 18, 10289–10331, https://doi.org/10.5194/acp-18-10289-2018, 2018. a, b
Ramachandran, S. and Rajesh, T.: Black carbon aerosol mass concentrations over Ahmedabad, an urban location in western India: comparison with urban sites in Asia, Europe, Canada, and the United States, J. Geophys. Res.-Atmos., 112, D06211, https://doi.org/10.1029/2006JD007488, 2007. a
Rap, A., Spracklen, D. V., Mercado, L., Reddington, C. L., Haywood, J. M., Ellis, R. J., Phillips, O. L., Artaxo, P., Bonal, D., C., R., and Butt, N.: Fires increase Amazon forest productivity through increases in diffuse radiation, Geophys. Res. Lett., 42, 4654–4662, https://doi.org/10.1002/2015GL063719, 2015. a
Rizzo, L. V., Correia, A. L., Artaxo, P., Procópio, A. S., and Andreae, M. O.: Spectral dependence of aerosol light absorption over the Amazon Basin, Atmos. Chem. Phys., 11, 8899–8912, https://doi.org/10.5194/acp-11-8899-2011, 2011. a, b
Rizzo, L. V., Artaxo, P., Müller, T., Wiedensohler, A., Paixão, M., Cirino, G. G., Arana, A., Swietlicki, E., Roldin, P., Fors, E. O., Wiedemann, K. T., Leal, L. S. M., and Kulmala, M.: Long term measurements of aerosol optical properties at a primary forest site in Amazonia, Atmos. Chem. Phys., 13, 2391–2413, https://doi.org/10.5194/acp-13-2391-2013, 2013. a, b, c, d, e
Rizzolo, J. A., Barbosa, C. G. G., Borillo, G. C., Godoi, A. F. L., Souza, R. A. F., Andreoli, R. V., Manzi, A. O., Sá, M. O., Alves, E. G., Pöhlker, C., Angelis, I. H., Ditas, F., Saturno, J., Moran-Zuloaga, D., Rizzo, L. V., Rosário, N. E., Pauliquevis, T., Santos, R. M. N., Yamamoto, C. I., Andreae, M. O., Artaxo, P., Taylor, P. E., and Godoi, R. H. M.: Soluble iron nutrients in Saharan dust over the central Amazon rainforest, Atmos. Chem. Phys., 17, 2673–2687, https://doi.org/10.5194/acp-17-2673-2017, 2017. a
Romano, S., Perrone, M. R., Pavese, G., Esposito, F., and Calvello, M.: Optical properties of PM2.5 particles: Results from a monitoring campaign in southeastern Italy, Atmos. Environ, 203, 35–47, https://doi.org/10.1016/j.atmosenv.2019.01.037, 2019. a, b, c
Russell, P. B., Bergstrom, R. W., Shinozuka, Y., Clarke, A. D., DeCarlo, P. F., Jimenez, J. L., Livingston, J. M., Redemann, J., Dubovik, O., and Strawa, A.: Absorption Angstrom Exponent in AERONET and related data as an indicator of aerosol composition, Atmos. Chem. Phys., 10, 1155–1169, https://doi.org/10.5194/acp-10-1155-2010, 2010. a
Sánchez-Ccoyllo, O. R., Ynoue, R. Y., Martins, L. D., Astolfo, R., Miranda, R. M., Freitas, E. D., Borges, A. S., Fornaro, A., Freitas, H., Moreira, A., and Andrade, M. F.: Vehicular particulate matter emissions in road tunnels in Sao Paulo, Brazil, Environ. Monitor. Assess., 149, 241–249, https://doi.org/10.1007/s10661- 008-0198-5, 2009. a
Sarwar, G., Fahey, K., Napelenok, S., Roselle, S., and Mathur, R.: Examining the impact of CMAQ model updates on aerosol sulfate predictions, in: The 10th Annual CMAS Models-3 User's Conference, October, Chapel Hill, NC, 26–30 October 2011. a, b
Saturno, J., Holanda, B. A., Pöhlker, C., Ditas, F., Wang, Q., Moran-Zuloaga, D., Brito, J., Carbone, S., Cheng, Y., Chi, X., Ditas, J., Hoffmann, T., Hrabe de Angelis, I., Könemann, T., Lavrič, J. V., Ma, N., Ming, J., Paulsen, H., Pöhlker, M. L., Rizzo, L. V., Schlag, P., Su, H., Walter, D., Wolff, S., Zhang, Y., Artaxo, P., Pöschl, U., and Andreae, M. O.: Black and brown carbon over central Amazonia: long-term aerosol measurements at the ATTO site, Atmos. Chem. Phys., 18, 12817–12843, https://doi.org/10.5194/acp-18-12817-2018, 2018. a
Sátyro, Z. C., Farias, C., Candido, L. A., and Veiga, J. A.: The relative and joint effect of rivers and urban area on a squall line in the Central Amazonia, Sci. Total Enviro., 755, 142178, https://doi.org/10.1016/j.scitotenv.2020.142178, 2021. a
Schultz, M. G., Schroder, S., Lyapina, O., Cooper, O. R., Galbally, I., Petropavlovskikh, I., von Schneidemesser, E., Tanimoto, H., Elshorbany, Y., Naja, M., Seguel, R. J., Dauert, U., Eckhardt, P., Feigenspan, S., Fiebig, M., Hjellbrekke, A.-G., Hong, Y.-D., Kjeld, P. C., Koide, H., Lear, G., Tarasick, D., Ueno, M., Wallasch, M., Baumgardner, D., Chuang, M.-T., Gillett, R., Lee, M., Molloy, S., Moolla, R., Wang, T., Sharps, K., Adame, J. A., Ancellet, G., Apadula, F., Artaxo, P., Barlasina, M. E., Bogucka, M., Bonasoni, P., Chang, L., Colomb, A., CuevasAgullo, E., Cupeiro, M., Degorska, A., Ding, A., Fröhlich, M., Frolova, M., Gadhavi, H., Gheusi, F., Gilge, S., Gonzalez, M. Y., Gros, V., Hamad, S. H., Helmig, D., Henriques, D., Hermansen, O., Holla, R., Hueber, J., Im, U., Jaffe, D. A., Komala, N., Kubistin, D., Lam, K.-S., Laurila, T., Lee, H., Levy, I., Mazzoleni, C., Mazzoleni, L. R., McClure-Begley, A., Mohamad, M., Murovec, M., Navarro-Comas, M., Nicodim, F., Parrish, D., Read, K. A., Reid, N., Ries, L., Saxena, P., Schwab, J. J., Scorgie, Y., Senik, I., Simmonds, P., Sinha, V., Skorokhod, A. I., Spain, G., Spangl, W., Spoor, R., Springston, S. R., Steer, K., Steinbacher, M., Suharguniyawan, E., Torre, P., Trickl, T., Lin, W., Weller, R., Xu, X., Xue, L., and Ma, Z.: Tropospheric Ozone Assessment Report: Database and metrics data of global surface ozone observations, Elementa, 5, 58, https://doi.org/10.1525/elementa.244, 2017. a
Seinfeld, J. H. and Pandis, S. N.: Atmospheric Chemistry and Physics: from air pollution to climate change, 3rd edn., John Wiley & Sons, 2016. a
Shilling, J. E., Pekour, M. S., Fortner, E. C., Artaxo, P., de Sá, S., Hubbe, J. M., Longo, K. M., Machado, L. A. T., Martin, S. T., Springston, S. R., Tomlinson, J., and Wang, J.: Aircraft observations of the chemical composition and aging of aerosol in the Manaus urban plume during GoAmazon 2014/5, Atmos. Chem. Phys., 18, 10773–10797, https://doi.org/10.5194/acp-18-10773-2018, 2018. a, b, c, d, e, f, g, h
Shrivastava, M., Andreae, M. O., Artaxo, P., Barbosa, H. M. J., Berg, L. K., Brito, J., Ching, J., Easter, R. C., Fan, J. W., Fast, J. D., Feng, Z., Fuentes, J. D., Glasius, M., Goldstein, A. H., Alves, E. G., Gomes, H., Gu, D., Guenther, A., Jathar, S. H., Kim, S., Liu, Y., Lou, S. J., Martin, S. T., McNeill, V. F., Medeiros, A., de Sa, S. S., Shilling, J. E., Springston, S. R., Souza, R. A. F., Thornton, J. A., Isaacman-VanWertz, G., Yee, L. D., Ynoue, R., Zaveri, R. A., Zelenyuk, A., and Zhao, C.: Urban pollution greatly enhances formation of natural aerosols over the Amazon rainforest, Nat. Commun., 10, 1046, https://doi.org/10.1038/s41467-019-08909-4, 2019. a, b, c, d, e, f, g, h, i, j, k
Silva Dias, M. A. F., Silva Dias, P. L., Longo, M., Fitzjarrald, D. R., and Denning, A. S.: River breeze circulation in eastern Amazonia: observations and modelling results, Theor. Appl. Climatol., 78, 111–121, https://doi.org/10.1007/s00704-004-0047-6, 2004. a
Stein, A. F., Isakov, V., Godowitch, J., and Draxler, R. R.: A hybrid modeling approach to resolve pollutant concentrations in an urban area, Atmos. Environ., 41, 9410–9426, https://doi.org/10.1016/j.atmosenv.2007.09.004, 2007. a
Tewari, M., Chen, F., Wang, W., Dudhia, J., LeMone, M., Mitchell, K., Ek, M., Gayno, G., Wegiel, J., and Cuenca, R.: Implementation and verification of the unified NOAH land surface model in the WRF model, in: 20th conference on weather analysis and forecasting/16th conference on numerical weather prediction,American Meteorological Society Seattle, WA, 1115, 2165–2170, 2004. a, b
Thalman, R., de Sá, S. S., Palm, B. B., Barbosa, H. M. J., Pöhlker, M. L., Alexander, M. L., Brito, J., Carbone, S., Castillo, P., Day, D. A., Kuang, C., Manzi, A., Ng, N. L., Sedlacek III, A. J., Souza, R., Springston, S., Watson, T., Pöhlker, C., Pöschl, U., Andreae, M. O., Artaxo, P., Jimenez, J. L., Martin, S. T., and Wang, J.: CCN activity and organic hygroscopicity of aerosols downwind of an urban region in central Amazonia: seasonal and diel variations and impact of anthropogenic emissions, Atmos. Chem. Phys., 17, 11779–11801, https://doi.org/10.5194/acp-17-11779-2017, 2017. a, b, c
Trebs, I., Mayol-Bracero, O. L., Pauliquevis, T., Kuhn, U., Sander, R., Ganzeveld, L., Meixner, F. X., Kesselmeier, J., Artaxo, P., and Andreae, M. O.: Impact of the Manaus urban plume on trace gas mixing ratios near the surface in the Amazon Basin: Implications for the NO-NO2-O3 photostationary state and peroxy radical levels, J. Geophys. Res.-Atmos., 117, D05307, https://doi.org/10.1029/2011JD016386, 2012. a
Vara-Vela, A., de Fátima Andrade, M., Zhang, Y., Kumar, P., Ynoue, R. Y., Souto-Oliveira, C. E., da Silva Lopes, F. J., and Landulfo, E.: Modeling of Atmospheric Aerosol Properties in the São Paulo Metropolitan Area: Impact of Biomass Burning, J. Geophys. Res.-Atmos., 123, 9935–9956, https://doi.org/10.1029/2018JD028768, 2018. a
Wang, K., Zhang, Y., Yahya, K., Wu, S.-Y., and Grell, G.: Implementation and initial application of new chemistry-aerosol options in WRF/Chem for simulating secondary organic aerosols and aerosol indirect effects for regional air quality, Atmos. Environ, 115, 716–732, 2015. a, b
Yáñez-Serrano, A. M., Bourtsoukidis, E., Alves, E. G., Bauwens, M., Stavrakou, T., Llusià, J., Filella, I., Guenther, A., Williams, J., Artaxo, P., Sindelarova, K., Doubalova, J., Kesselmeier, J., and Peñuelas, J.: Amazonian biogenic volatile organic compounds under global change, Glob. Change Biol., 26, 4722–4751, https://doi.org/10.1111/gcb.15185, 2020. a
Ynoue, R. Y. and Andrade, M. F.: Size-resolved mass balance of aerosol particles over the São Paulo metropolitan area of Brazil, Aerosol Sci. Tech., 38, 52–62, 2004. a
Zhang, Y., Wen, X.-Y., and Jang, C.: Simulating chemistry–aerosol–cloud–radiation–climate feedbacks over the continental US using the online-coupled Weather Research Forecasting Model with chemistry (WRF/Chem), Atmos. Environ, 44, 3568–3582, 2010. a