the Creative Commons Attribution 4.0 License.
the Creative Commons Attribution 4.0 License.
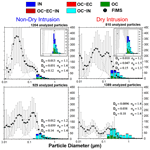
Impact of dry intrusion events on the composition and mixing state of particles during the winter Aerosol and Cloud Experiment in the Eastern North Atlantic (ACE-ENA)
Jay M. Tomlin
Kevin A. Jankowski
Daniel P. Veghte
Swarup China
Peiwen Wang
Matthew Fraund
Johannes Weis
Guangjie Zheng
Yang Wang
Felipe Rivera-Adorno
Shira Raveh-Rubin
Daniel A. Knopf
Jian Wang
Mary K. Gilles
Ryan C. Moffet
Long-range transport of continental emissions has a far-reaching influence over remote regions, resulting in substantial change in the size, morphology, and composition of the local aerosol population and cloud condensation nuclei (CCN) budget. Here, we investigate the physicochemical properties of atmospheric particles collected on board a research aircraft flown over the Azores during the winter 2018 Aerosol and Cloud Experiment in the Eastern North Atlantic (ACE-ENA) campaign. Particles were collected within the marine boundary layer (MBL) and free troposphere (FT) after long-range atmospheric transport episodes facilitated by dry intrusion (DI) events. Chemical and physical properties of individual particles were investigated using complementary capabilities of computer-controlled scanning electron microscopy and X-ray spectromicroscopy to probe particle external and internal mixing state characteristics. Furthermore, real-time measurements of aerosol size distribution, cloud condensation nuclei (CCN) concentration, and back-trajectory calculations were utilized to help bring into context the findings from offline spectromicroscopy analysis. While carbonaceous particles were found to be the dominant particle type in the region, changes in the percent contribution of organics across the particle population (i.e., external mixing) shifted from 68 % to 43 % in the MBL and from 92 % to 46 % in FT samples during DI events. This change in carbonaceous contribution is counterbalanced by the increase in inorganics from 32 % to 57 % in the MBL and 8 % to 55 % in FT. The quantification of the organic volume fraction (OVF) of individual particles derived from X-ray spectromicroscopy, which relates to the multi-component internal composition of individual particles, showed a factor of 2.06 ± 0.16 and 1.11 ± 0.04 increase in the MBL and FT, respectively, among DI samples. We show that supplying particle OVF into the κ-Köhler equation can be used as a good approximation of field-measured in situ CCN concentrations. We also report changes in the κ values in the MBL from κMBL, non-DI=0.48 to κMBL, DI=0.41, while changes in the FT result in κFT, non-DI=0.36 to κFT, DI=0.33, which is consistent with enhancements in OVF followed by the DI episodes. Our observations suggest that entrainment of particles from long-range continental sources alters the mixing state population and CCN properties of aerosol in the region. The work presented here provides field observation data that can inform atmospheric models that simulate sources and particle composition in the eastern North Atlantic.
- Article
(8964 KB) - Full-text XML
-
Supplement
(18050 KB) - BibTeX
- EndNote
Marine low clouds play a significant role in the world's climate and energy balance (Wood et al., 2015). They are the major factor in increasing the Earth's albedo, which is the fraction of solar energy reflected back into space, leading to an overall cooling effect (Wood, 2012; Wood et al., 2015). Marine low clouds represent one of the leading sources of uncertainty in atmospheric models due to limited observational data, insufficient understanding of the microphysical changes that regulate these clouds, and the lack of fine model resolution to account for such processes (Bony, 2005; Klein et al., 2013). Other relevant boundary layer processes also contribute to the challenges in assessing marine low clouds such as turbulent mixing, entrainment, and emissions of aerosols and their precursors (Pincus and Baker, 1994; Ackerman et al., 2004). In particular, the response of low-altitude clouds is sensitive to aerosol perturbations, which requires a greater understanding of the processes that govern regional aerosol budget and source attribution (Levin and Cotton, 2009; Altaratz et al., 2014; Rosenfeld et al., 2019; Zheng et al., 2018, 2021). Source-dependent particle size and composition can lead to changes in clouds' albedo and precipitation due to their varying efficiency to act as cloud condensation nuclei (CCN) and ice-nucleating particles (INPs) (Johnson et al., 2004; Hamilton et al., 2014; Zheng et al., 2020a).
Atmospheric particles exhibit complex internal heterogeneity (Murphy and Thomson, 1997; Buseck and Posfai, 1999; Prather et al., 2008; Li et al., 2016; Riemer et al., 2019; Laskin et al., 2019). These particles can come from direct emissions (i.e., primary particles) or from gas–particle conversion in atmospheric reactions (i.e., secondary particles) (Reddington et al., 2011). Primary particles with complex composition include primary organic aerosols, elemental carbon (i.e., black carbon and soot), inorganic species from combustion and biomass burning sources (Toner et al., 2006; Souri et al., 2017), and sea spray aerosol with organic components influenced by ocean biological activity (Prather et al., 2013; Pham et al., 2017). On the other hand, secondary organic aerosol (SOA) is formed from the oxidation products of volatile organic compounds (VOCs) of either biogenic or anthropogenic origin. Secondary fine particles of nitrate and sulfate are similarly formed from the oxidation of their inorganic gaseous precursors NOx and SO2, respectively (National Research Council, US, 2002). In marine areas, formation of sulfate aerosol is further influenced by gas-phase emissions of dimethyl sulfide (DMS) from biota, which upon oxidation yield low-volatility products such as sulfuric acid (H2SO4) (Kulmala et al., 2000) and methylsulfonic acid (MSA) (Andreae et al., 1985; Hodshire et al., 2019). Physical and chemical characteristics of individual particles such as morphology, chemical composition, hygroscopicity, lifetime, and chemical mixing state have a profound effect on their CCN activity (Cruz and Pandis, 1997; VanReken, 2003; King et al., 2012; Schmale et al., 2017; Riemer et al., 2019). Note that the term “chemical mixing state” refers to how various chemical species are mixed within individual particles (Riemer et al., 2019). The chemical mixing state depends on emission sources and atmospheric aging events, which include but are not limited to biomass burning influence (Levin et al., 2010), anthropogenic emissions (Jacobson, 2001), and large continental dust events (Fraund et al., 2017; Adachi et al., 2020). For example, previous studies found that within a few hours urban non-hygroscopic aerosol (i.e., mixed organic and black carbon aerosol) can accumulate a sufficient coating of hygroscopic sulfates and nitrates to increase their hygroscopicity parameter (κ) (Petters and Kreidenweis, 2007) from 0 to 0.1 (Wang et al., 2010).
The variability within individual atmospheric particles has been well documented by both model and field measurements across different regions worldwide such as urban (Wang et al., 2010; Ault et al., 2010, 2012; Wang et al., 2012; Fraund et al., 2017; Ren et al., 2018), rural (Vakkari et al., 2018; Tomlin et al., 2020), remote forests (Bondy et al., 2018), Arctic (Gunsch et al., 2017; Gonçalves et al., 2021), and marine (Ault et al., 2013; Zheng et al., 2020a, b). Long-range transport and meteorological processes such as dry intrusions (DIs) and vertical mixing of air also play a significant role in the continuous evolution of particle composition in the atmosphere (Raes, 1995; Pratt and Prather, 2010; Cubison et al., 2011; Igel et al., 2017; Zheng et al., 2020b). DIs are events of dry, slantwise descending airflow from the upper troposphere in midlatitudes down through the boundary layer at lower latitudes (Raveh-Rubin, 2017). Such intrusions of dry air, typically peaking in winter, occur with the passage of extratropical cyclones and their trailing cold fronts, i.e., in the post-cold-frontal region (Wernli, 1997; Browning, 1997; Catto and Raveh-Rubin, 2019). Events of DI are strongly coupled to the boundary layer, which cools and deepens during DI, and were shown to induce enhanced ocean heat fluxes (Raveh-Rubin and Catto, 2019; Ilotoviz et al., 2021). DI events are of particular interest as they can contain air masses with a complex distribution of aged particles having drastically different size, morphology, and composition compared to local regional aerosols, leading to changes in the local aerosol–cloud interactions and cloud lifetimes (Zheng et al., 2020b; Wang et al., 2020). For example, it has been shown that the CCN population in the remote marine boundary layer (MBL) of the eastern North Atlantic can be influenced by long-range transport of wildfire aerosols originating from North America (Zheng et al., 2020b; Y. Wang et al., 2021). The properties of these wildfire aerosols, facilitated by long-range transport processes, are altered as they undergo aging (e.g., multi-phase particle chemistry, photo-bleaching, and gas–particle partitioning of organics), resulting in changes in both the optical properties and the cloud-forming potential (Jacobson, 2001; Levin et al., 2010; Zheng et al., 2020b). In particular, aged wildfire aerosol is typically dominated by accumulation-mode particles, which readily serve as CCN in the region despite a substantially lower κ value (i.e., 0.2 to 0.4) than regional highly hygroscopic aerosol of marine origin (e.g., sea spray aerosol, κ=1.1) (Zieger et al., 2017; Zheng et al., 2020b). Lastly, long-range-transported and atmospherically aged free-tropospheric particles can contribute to the ice-nucleating particle population and potentially impact cloud formation (China et al., 2017).
This paper investigates the physicochemical properties of atmospheric particles during the Aerosol and Cloud Experiment in the Eastern North Atlantic (ACE-ENA) field campaign conducted at the Azores in January–February 2018. Aircraft measurements and onboard sampling of particles (followed by laboratory-based particle analysis) were utilized to characterize the difference in the contributions of various sources to FT and MBL aerosols under representative synoptic conditions (i.e., DI vs. non-DI periods) in this geographical area. Particle analysis included particle-type classification with statistical depth provided by computer-controlled scanning electron microscopy, and a subset of particles were sampled by X-ray spectromicroscopy to characterize particle chemical mixing state (internal heterogeneity). The particle-type composition, chemical mixing state, and morphology from analyzed periods were then combined with real-time measurement of aerosol size distribution, CCN concentration, and back-trajectory calculations to obtain the representative composition of particles present in the MBL during the DI events and entrainment of particles originating from North America. The data presented here provide observational input for atmospheric process models to simulate sources and particle composition in the broader North Atlantic region.
2.1 Field campaign and meteorological conditions
Samples of atmospheric particles were collected aboard the U.S. Department of Energy Gulfstream aircraft (G-1). Flight patterns were flown between Terceira Island (38∘45′43′′ N, 27∘5′27′′ W) and Graciosa Island (39∘3′12′′ N, 28∘7′26′′ W), Portugal, and within 20–30 km of Graciosa Island (J. Wang et al., 2021). Flight plans were based on the projected meteorological conditions from various global forecast modes including Monitoring Atmospheric Composition and Climate, Global Forecast System, and European Centre for Medium-Range Weather Forecasts (ECMWF). A subset of collected samples was selected for analysis based on synoptic conditions (identifying DI vs. non-DI periods) and altitudes (clear MBL and FT layers) for each day. Samples analyzed were collected during the second intensive operation period of ACE-ENA, on the dates of 19 January 2018, 21 January 2018, 24 January 2018, 25 January 2018, 26 January 2018, 28 January 2018, 30 January 2018, 1 February 2018, 8 February 2018, 11 February 2018, 15 February 2018, 16 February 2018, and 19 February 2018. These dates were selected due to unique transport episodes associated with the sampling periods. DI days we identified objectively using the Lagrangian analysis tool (LAGRANTO) version 2.0 (Sprenger and Wernli, 2015) and wind field data obtained from the ECMWF interim reanalysis (ERA-Interim) available 6-hourly, interpolated to 1∘ × 1∘ horizontal grid resolution, and at 60 vertical hybrid levels (Dee et al., 2011). DIs were identified by a systematic calculation of forward trajectories at altitudes higher than 600 hPa, while the DI trajectories were identified based on the vertical descent of the air masses. For a trajectory to be termed a DI, their pressure must increase (i.e., descend in altitude) by at least 400 hPa in 48 h (Raveh-Rubin, 2017). If such a DI trajectory is found within a 3∘ radius around Graciosa, the date is considered to be “DI”. In addition, backward trajectories for each sampling period were calculated for the end points at relevant flight altitudes (Figs. S1 and S2) using the Hybrid Single-Particle Lagrangian Integrated Trajectory (HYSPLIT) model (Stein et al., 2015; Rolph et al., 2017). Atmospheric data from ERA-Interim are additionally analyzed for the atmospheric column at Graciosa, namely potential temperature, equivalent potential temperature, potential vorticity, and boundary layer height. The latter is diagnosed in ERA-Interim using the critical bulk Richardson number upon its first passing of the threshold 0.2 when scanning from the surface upwards (ECMWF, 2007).
2.2 Particle collection and in situ measurements of particle and cloud properties
The G-1 aircraft is equipped with sensor modules to deliver precise real-time inertial measurement, GPS, meteorological, and turbulence data such as position, altitude, temperature, pressure relative humidity, and three-dimensional winds. For particle collection, the G-1 was equipped with an isokinetic aerosol inlet, from which ambient aerosol was transported to individual instruments. Particle samples were collected using a custom-built time-resolved aerosol collector (TRAC) that autonomously collected particles on substrates at pre-set time intervals (Laskin et al., 2006). The TRAC is a single-stage impactor with an aerodynamic cutoff size (D50 %) of 0.36 µm (Laskin et al., 2003) coupled to a rotating disk that can hold up to 160 samples. The disk was preloaded with microscopy substrates (carbon Type-B film on 400-mesh copper grids, Ted Pella, Inc.). The sampling was performed at a single spot on the center of each substrate for 7–10 min, depending upon the flight. After each flight, sample disks were taken off the TRAC, plated, and hermetically sealed prior to transport. Once samples were received in the lab, the sample grids used for the analysis were removed from the sealed plate and transferred into grid boxes stored at room temperature and dry conditions in a desiccator cabinet. Online measurements of aerosols aboard the G-1 include a passive cavity aerosol spectrometer 100X probe (PCASP, Dp=0.1–3.0 µm, 1 Hz resolution) and a fast integrated mobility spectrometer (FIMS, Dp=0.01–0.5 µm, 1 Hz resolution), which provided size distributions and concentrations of ambient particles (Kulkarni and Wang, 2006; Wang et al., 2018). During all research flights, a Nafion dryer reduced the relative humidity of the airstream in the sampling line. A CCN counter (Droplet Measurement Technologies) measured the concentration of particles that activate at a supersaturation of 0.14 %. A high-resolution time-of-flight aerosol mass spectrometer (HR-ToF-AMS) was deployed on board to characterize bulk non-refractory aerosol composition (i.e., organics, sulfate, ammonium, and chlorine) (DeCarlo et al., 2006; Zawadowicz et al., 2021). The particle size distributions and CCN concentrations were analyzed when the liquid water content was below 0.001 g m3 to avoid periods when cloud-shattering artifacts could influence the sampled particles (Korolev et al., 2011). The liquid water content was obtained by integrating the droplet size distributions measured by a fast cloud droplet probe (FCDP; Droplet Measurement Technologies).
Additional information on the sampling conditions is presented in Table S1 of the Supplement and incudes sampling time and date, average sampling altitude, boundary layer height, particle concentration, and wind speed. The boundary layer height was calculated based on potential temperature measurements collected for each flight. The boundary layer is limited by a well-defined temperature inversion, resulting in a maximum value of the temperature gradient as a function of height (Stull, 1988). A summary of each flight (altitude and aerosol particle concentration vs. time) with the collection times highlighted is shown in Figs. S3 and S4. Guided by meteorological analysis and wind field data to identify DI periods, we performed offline microscopy analysis of collected particle samples across different atmospheric layers and transport episodes during the ACE-ENA campaign.
2.3 Methods of particle analysis
Morphology and elemental analysis of individual particles were performed using computer-controlled scanning electron microscopy coupled with energy dispersive X-ray spectroscopy operated at 20 kV (CCSEM/EDX; FEI Quanta 3D, EDAX Genesis). During CCSEM/EDX analysis particle samples were systematically imaged, and particles larger than 100 nm are recognized. Of note is that the particle size reported from CCSEM/EDX analysis is defined as the area equivalent diameter (AED, µm), which is based on fitting a circle with an area equivalent to the particle's 2D projected image. This is followed by an automated acquisition of individual EDX spectra for each particle (Laskin et al., 2005). EDX spectra with sufficient X-ray counting statistics (40–1500 photons s−1) were then processed to quantify relative atomic fractions of 15 elements: C, N, O, Na, Mg, Al, Si, P, S, Cl, K, Ca, Mn, Fe, and Cu. The EDX peak of Cu is heavily influenced by a background signal from the copper TEM grid and the sample holder made of beryllium–copper alloy. Therefore, quantified atomic fractions of Cu were excluded from particle-type classification of the analyzed particles. Two independent methods were employed for the particle-type grouping and classification: (1) k-means clustering and (2) rule-based particle classification. The k-means clustering is an unsupervised machine-learning algorithm designed to group similar datasets without user intervention (Rebotier and Prather, 2007; Moffet et al., 2012). The second approach for the categorization of particles utilizes a series of user-defined rules to separate analyzed particles into groups of typical elemental contribution (Laskin et al., 2012). For this work, the k-means clustering was used as a primary method for particle-type classification, while the rule-based approach was used as a complementary method to build confidence in the identification of different particle types. Details of the classification schemes are provided in the Supplement (Figs. S5 and S6) and in previous works (Moffet et al., 2012; Tomlin et al., 2020).
Scanning transmission X-ray microscopy with near-edge X-ray absorption fine-structure (STXM/NEXAFS) spectroscopy was used to elucidate the chemical mixing state of individual particles based on the NEXAFS spectral data acquired at the carbon K-edge (278–320 eV) (Hopkins et al., 2007; Moffet et al., 2010b, c). STXM/NEXAFS was performed at the synchrotron facilities on beamlines 11.0.2.2 and 5.3.2.2 in the Advanced Light Source, Lawrence Berkeley National Laboratory, and on beamline 10ID-1 at the University of Saskatchewan Canadian Light Source. STXM instrument operation is similar in both locations as described elsewhere (Kilcoyne et al., 2003). Briefly, a set of raster scan STXM images at each of the pre-set energy levels was acquired from a synchrotron monochromated incident light focused on the sample using a Fresnel zone plate. The transmitted light is detected at each of the energy settings, and spectra of individual particles could then be reconstructed based on the Beer–Lambert law from the intensity of transmitted light over the projection area of particles compared to the particle-free regions. The recorded intensity at each energy setting (E) across individual pixels was converted into optical density (ODE) as follows:
where I(E) is the intensity of light transmitted through a particle, I0(E) is the intensity of incoming light (determined as intensity of light in the particle-free areas), μ is the mass absorption coefficient, ρ corresponds to the density, and t is the thickness of a particle. Sequences of STXM images are acquired at closely spaced energies of I0(E) to record a “stack” of images. Then, NEXAFS spectra from individual pixels of detected particles are extracted from the stack (∼96 energies over 278 to 320 eV range, 30–35 nm spatial resolution, 1 ms dwell time).
In addition, faster acquisition of STXM images at four key energies of 278 eV (pre-edge), 285.4 eV (C = C), 288.5 eV (−COOH), and 320 eV (post-edge) (15 × 15 µm, 30–35 nm spatial resolution, 1 ms dwell time) was employed to construct “maps” of individual particles using image processing methods reported in our earlier studies (Moffet et al., 2010a, 2013, 2016; Fraund et al., 2017). Briefly, a series of thresholds were used to identify the mapping components including inorganics (IN), organic carbon (OC), and soot–elemental carbon (EC). The total carbon (TC) was calculated as the difference between the carbon post-edge and pre-edge OD (TC = OD320 eV−OD278 eV). IN-rich regions were defined with pixels having an ratio greater than 0.5. OC regions are those with abundant features corresponding to the carboxylic acid functional group (−COOH), defined by the difference between the intensity of the −COOH peak and carbon pre-edge peak greater than 0 (i.e., OD288.5 eV–OD278 eV>0). Finally, EC areas are identified by comparing the value of the sp2 total carbon to that of highly oriented pyrolytic graphite (HOPG) according to (, which indicates extensive sp2 bonding of carbon corresponding to graphitic-like components (Hopkins et al., 2007).
3.1 Identification of dry intrusion periods
Research flights were conducted under different synoptic conditions to allow for the characterization of common aerosols, trace gases, clouds, and precipitation. Figure 1a illustrates the typical flight pattern of the G-1 aircraft, which includes multiple legs at different altitudes while maneuvering perpendicular and along the wind direction. These patterns allowed for the full profile of aerosol and cloud layer along the MBL and lower FT altitudes. Figure 1b shows daily time series from 1 January to 28 February 2018 in relation to DI events identified from ERA-Interim reanalysis. The marked black dots indicate DI air masses within a 3∘ radius from 39∘ N, 28∘ W (i.e., the ENA site). The high frequency of the black dots (i.e., vertical distribution) indicates an increase in trajectories that satisfy the DI criterion at different pressure altitudes. For example, on 24 January 2018 in Fig. 1b, we see a series of DI air parcels (black dots) at different pressure altitudes ranging from 611 to 2360 m a.m.s.l. found to be below or above the boundary layer as indicated by the dashed red line. Guided by the frequency of the DI air masses, we selected a subset of the time-tagged particle samples for analysis by the complementary microscopy techniques as summarized in Table S1. To evaluate the consistency of the sources and long-range transport trajectories, we calculated back trajectories using the HYSPLIT model (Stein et al., 2015; Rolph et al., 2017). Figure 1c shows results of representative HYSPLIT 72 h back-trajectory calculations for the research flight on 24 January 2018, which identifies long-range transport of an air mass originating from North America. Trajectories were calculated every 6 h from 13:00 UTC on 24 January 2018 to 12:00 UTC on 22 January 2018 at three starting altitudes: 100, 2000, and 3000 m. This process was repeated with the same HYSPLIT input meteorological parameters for all research flights utilized in this work, as shown in Figs. S1 and S2.
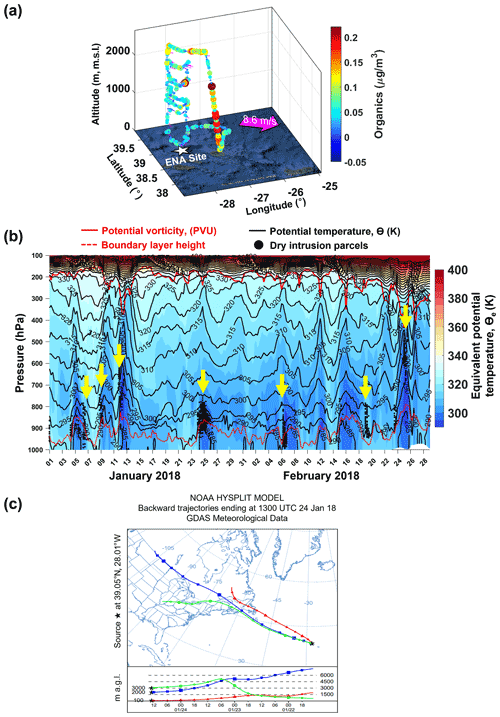
Figure 1(a) A representative flight path of the G-1 aircraft during one of the DI events (24 January 2018) during ACE-ENA campaign (Azores, Portugal). The size and color scale correspond to organic concentration provided by onboard Aerodyne HR-ToF-AMS. (b) A time–height cross section at 39∘ N, 28∘ W using ERA-Interim reanalysis from the ECMWF, showing equivalent potential temperature (K, shading), potential temperature (black contours), and boundary layer height (red dashed line). The solid red line is the 2 PVU contour of potential vorticity, marking the tropopause. The time periods of DI events (marked by black dots and indicated by yellow arrows) were identified from calculated forward trajectories based on the wind field data (ERA-Interim; see text for more details). (c) Calculated HYSPLIT 72 h back trajectory for 24 January 2018 utilizing GDAS1 archived datasets starting at three elevations: 100 m (red), 2000 m (blue), 3000 m (green).
3.2 Particle-type classification
A total of 38 particle sample grids from 13 (out of 19) research flights were analyzed. First, CCSEM/EDX analysis was carried out to characterize the particle-type composition typical of different synoptic scenarios. Figure 2 shows the results of the size-segregated particle-type population (right column) obtained from k-means clustering analysis of ∼36 400 individual particles with the backscattering-mode scanning electron microscope (SEM) imaging of a representative subset of particles (left column), separated between MBL versus FT flight altitudes and between synoptic conditions of DI and non-DI sampling periods. The onboard FIMS instrument measurement provided particle size distribution data in a range of 0.01–0.5 µm. By superimposing the CCSEM/EDX particle analysis data with the FIMS size distribution data, we can approximate the representative composition and number concentration of potentially CCN active particles (>0.1 µm) in the MBL and FT during non-DI and DI periods, respectively. Note that the error bars in the particle number concentration indicate variation in the particle size distribution values averaged across different days and synoptic conditions. Also, comparison of AED and FIMS sizes needs to be considered with caution because particle flattering on the substrate results in overestimated AED sizes compared to more realistic FIMS values. Here, the AED-based particle distributions are scaled to match the Y axis of FIMS data and therefore to provide visual illustration of the chemical makeup of CCN particles.
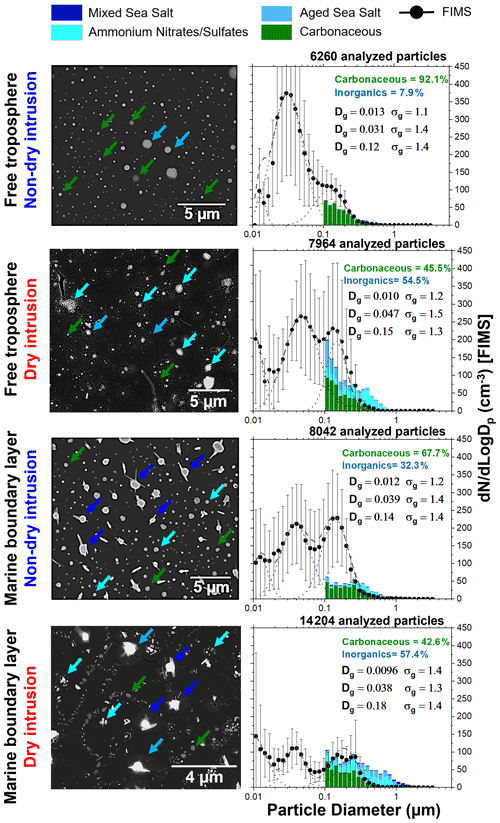
Figure 2Representative backscattering-mode SEM imaging of particles (left column) and relative particle-type populations (right column) determined by CCSEM/EDX and k-means clustering analysis, summarized as a 16-bin per decade histogram representative of MBL and FT atmospheric layers and DI versus non-DI synoptic conditions. The compositions of the size-segregated particle-type population were broken down into carbonaceous and inorganics (i.e., mixed sea salt + aged sea salt + ammonium nitrate–sulfate). The average FIMS aerosol size distribution measured on board G1 is superimposed and anchored at 0.25 µm to facilitate a visual assessment of particle types and number concentrations for CCN active particles (>100 nm). Lognormal-mode diameter (Dg) and standard deviation (σg) were fitted for the FIMS particle size distribution (gray dashed lines).
The k-means algorithm identified four key clusters termed as carbonaceous, ammonium nitrates–sulfates, mixed sea salt, and aged sea salt based on the mean elemental contribution (Fig. S5). Note that the element fraction values obtained from individual EDX spectra were filtered to remove values less than 0.5 %. Carbonaceous is the dominant type and represents the majority of analyzed particles. It is defined based on the sole contributions of C and O elements in the particle EDX spectra. The second-most abundant cluster is the ammonium nitrates–sulfates, to which the contributions of N, O, and S are greater than 1 %. The aged sea salt and mixed sea salt clusters contain similar elemental signatures, with the latter containing significant amounts of refractory elements typical for sea salt and mineral dust including Mg, Cl, K, Ca, Mn, and Fe.
First, we compared the change in particle-type population among samples in the MBL during non-DI and DI periods. The fraction of carbonaceous particles within the MBL contributed around 68 % in non-DI samples and decreased to 43 % in DI samples. Organic aerosol in the remote MBL has been suggested to originate from VOCs such as isoprenes, monoterpenes, formic acid, nitrogenized, and aliphatic organics released from biological activities near the sea surface, which undergo oxidation reactions leading to SOA formation (Facchini et al., 2008; Dall'Osto et al., 2012; Mungall et al., 2017). The lower fraction of carbonaceous particles during DI periods is counterbalanced by the increase in inorganics shifting from 32 % (non-DI periods) to 57 % (DI periods). Here, we operationally defined inorganics as the sum of mixed sea salt (4 %), aged sea salt (20 %), and ammonium nitrate–sulfate (33 %), which in fact may also contain organic carboxylic acids as components of aged sea salt. Regardless, shifting focus to the comparison of FT samples during non-DI and DI periods, we found that background carbonaceous particles contribute around 92 % (non-DI periods), decreasing to 46 % (DI periods). Similar to MBL observations, the shift in carbonaceous contribution can be attributed to an increase in inorganic influence during DI events changing from 8 % (non-DI periods) to 55 % (DI periods). We observed that most of the inorganic influence originates from ammonium nitrate and sulfate, contributing between 32 % and 33 % during DI periods regardless of sampling altitude (MBL vs. FT). Both carbonaceous particles and ammonium nitrate–sulfate can originate from ocean biological activity or anthropogenic sources. Typically, over marine areas, sulfate aerosol forms from oxidation of dimethyl sulfide (DMS), a common gas species emitted by biota. Sulfates are major components of accumulation-mode particles in the remote marine environment (Sanchez et al., 2018; Korhonen et al., 2008). Nitrate in marine particles can also come from vertical mixing in the ocean that surges nitrate-rich deep waters to the surface, followed by the aerosolization through wave motion (Zehr and Ward, 2002). However, the elevated contribution of ammonium nitrates and sulfates during the DI periods suggests likely influence from anthropogenic emissions originating from North America. Inorganic aerosols such as ammonium nitrates and sulfates are predominantly formed from the condensation of atmospheric precursors such as SO2, NH3, HOx, and NOx, which are common components of biomass burning emissions, urban areas, and agriculture activities among others (Reff et al., 2009). A study utilizing regional chemical models has found that the mass enhancements in inorganic aerosol can reach 23 % of carbonaceous enhancements as biomass burning processes accelerate secondary formation of inorganic aerosols (Souri et al., 2017). Uptake of S- and N-containing acidic species, as well as soluble organic acids, onto the preexisting sea salt particles modifies their composition through acid-displacement reactions that can be expressed in the general form of (Finlayson-Pitts, 2003; Laskin et al., 2012)
where *NaCl denotes sea salt, and HA represents atmospheric water-soluble acids (e.g., HNO3, H2SO4, CH3SO3H, and carboxylic acids). These reactions release the volatile HCl(g) product, leaving particles depleted in chloride and enriched in corresponding HA(aq) salts. Related to this acid-displacement chemistry, mixed sea salt and aged sea salt particle types were identified by the k-clustering analysis as illustrated in Fig. S5. The mixed sea salt particles contain key components of seawater (i.e., Na, Mg, and Cl; atomic fractions of Na and Cl >10 % with characteristic ratio of ∼0.6) and minor fractions (<2 %) of additional elements (e.g., Ca, Mn, Fe, Al, and Si), suggesting internal mixing of relatively fresh sea salt with other inorganic components without extensive chloride depletion. The other cluster of aged sea salt particles shows significant fractions of Na (∼10 %) but with substantially lower ratios of <0.1, which indicates chloride depletion (Fig. S5) due to atmospheric aging. Atomic fractions of C and N elements in this type of particle are much higher than those in the mixed sea salt cluster, while the fraction of S is much smaller. These observations suggest that in this geographical region acid-displacement reactions in the aged sea salt particles are mostly driven by water-soluble carboxylic acids (common components of SOA) (Laskin et al., 2012) and nitric acid (Finlayson-Pitts, 2003), while contributions by sulfonic or sulfuric acids are minor during the wintertime. Based on the k-means clustering, fractions of mixed sea salt range from 0.5 % to 3 %, while fractions of aged sea salt are overall more populous and range between 0.1 % and 20 % across all investigated samples. Additionally, both the aged sea salt and mixed sea salt cluster groups include minor contributions of Al and Si, indicative of possible mixing with mineral dust transported from the long-range continental sources.
To better discriminate particle-type groups according to their composition and the acid-displacement chemistry identified through the k-means clustering, a supplemental rule-based classification was performed using previously published definitions of particle-type classes common in marine environments (Laskin et al., 2012; Tomlin et al., 2020). Results of the particle-type characterization utilizing the rule-based assessment of their elemental composition (assigned into five major classes) are presented in Fig. S6. The applied rule-based classification scheme distinguishes among particle types common in the remote marine environment of sea salt, sea salt–sulfate, carbonaceous–sulfate, carbonaceous, and other (Fig. S6). For each sample, 600–3000 particles were analyzed, depending on particle loading on the substrates. The size-resolved particle-type classification identified using the rule-based schematic was overlaid on the acquired FIMS size distribution as shown in Fig. S7. Similar to the k-means clustering breakdown, we first compared the impact of DI events in MBL samples. Significant fractions of carbonaceous and carbonaceous–sulfate particles were identified in the background MBL samples, amounting to 86 % (non-DI periods) and decreasing to 49 % (DI period). Furthermore, the combined fraction of sea salt and mixed sea salt–sulfate is substantially smaller at around 10 % (non-DI periods) to 21 % (DI period). Fractions of uncategorized “other” particles contribute around 30 % (DI period), while only having a minimal contribution of 4 % during non-DI events. In contrast, background FT samples were dominated by carbonaceous and carbonaceous–sulfate, contributing as much as 95 % (non-DI periods) then decreasing to 55 % (DI period). Unlike the MBL samples, there was only minimal change in larger sea salt + mixed sea salt–sulfate from 2 % (non-DI period) to 4 % (DI period). However, the reduction in the carbonaceous and carbonaceous–sulfate contribution among FT samples during DI periods is associated with the large change in the “other” fraction shifting from 4 % (non-DI period) to 41 % (DI period). Based on the mean elemental composition of the “other” category, this group contains a combination of dust, sea salt, and carbonaceous components, suggesting extensive internal mixing of particles consistent with long-range transport (Froyd et al., 2019). This finding is also consistent with the k-means clustering results that indicated elevated contributions of particles with inorganic components during the DI periods. Overall, the particle-type fractions identified by both the k-means clustering and the rule-based classification schemes are consistent across all samples, suggesting that the mixing state population significantly changes from heavily organic-dominated to a mixture of inorganic–organic particle-type distribution, resulting in the observation of more complex particle compositions during DI periods.
Relative contributions of the particle-type fractions among separate DI events show substantial variability between different flights and MBL versus FT altitudes (Fig. S8). Furthermore, the dominant carbonaceous particle-type groups identified by CCSEM/EDX elemental analysis may exhibit significant differences in the spectral characteristics of carbon bonding, indicative of its long-range transport from North America during the DI periods. Furthermore, a previous study tracked the origin of air masses transported over long distances across the Atlantic Ocean to the Azores utilizing the Lagrangian Flexible Particle (FLEXPART) dispersion model to show detailed spatial resolution of air masses across different locations and altitudes (China et al., 2017). The influence of North American emissions on distant remote regions is well documented, with occurrences of continental pollutant transport events accompanied by strong influence from urban city emissions from Boston, Toronto, Detroit, and Chicago (Owen et al., 2006). On the other hand, extensive boreal wildfires in northern North America release large amounts of trace gases and aerosols into the atmosphere, which then can be transported to other remote regions including North America (Val Martín et al., 2006). In particular, boreal wildfires emit around 10 % of the annual anthropogenic aerosol black carbon in the Northern Hemisphere (Bond et al., 2004). The eastward transport of North American emissions begins as hot plumes of biomass burning emissions from wildfires rapidly rise to high altitudes (∼8 to 13 km a.g.l.) under favorable conditions (Zhu et al., 2018; Yu et al., 2019; Kloss et al., 2019). These plumes can be lofted into a warm conveyor belt preceding a cold front from an associated cyclone, which is followed by the entrainment of a cold descending airstream (from the same cyclone) that ultimately results in the air parcels containing continental emissions reaching the lower altitudes of the eastern North Atlantic (Owen et al., 2006; Zheng et al., 2020b). The transported aerosol undergoes substantial atmospheric aging through photochemical reactions (Hems et al., 2021), gas–particle partitioning (Vakkari et al., 2018), and coagulation (Ramnarine et al., 2019) processes as it travels across the Atlantic Ocean and descends into the MBL during the DI events.
3.3 Internal mixing of individual particles
Results of the elemental microanalysis of particles presented above provide statistics on broad particle classes identified and show the significant contribution of organic-dominated particles in the region well. However, CCSEM/EDX analysis is limited in providing detailed information on the carbon speciation within individual particles and other metrics of particle internal composition (chemical mixing state). To investigate chemical differences in the carbon components of particles we employed STXM/NEXAFS spectromicroscopy methods, which provide spatially resolved carbon bonding speciation and differentiate between EC and OC regions within individual particles (Moffet et al., 2010a, c). It is also worth mentioning that the definitions of carbonaceous particles identified by CCSEM/EDX and described in the previous section is somewhat different from OC particles defined by STXM/NEXAFS. The former corresponds to the distribution of organics across a population of particles (i.e., external mixing), while the latter is related to the multi-component internal heterogeneity of individual particles (i.e., internal mixing). Figure 3a shows an illustrative carbon K-edge map of individual particles from one of the DI period samples (the cumulative map of all ∼4300 particles from all samples analyzed in this study is included in the Supplement, Fig. S9). The carbon K-edge composition map distinguishes three main components based on the spectral information (Moffet et al., 2010a) as described earlier: IN (blue), OC (green), and EC (red). Each pixel within an individual particle may contain either single or multiple components (i.e., components can overlap) that are grouped to yield five typical classes based on the internal mixing between OC, EC, and IN components: (1) IN, (2) OC-EC-IN, (3) OC-EC, (4) OC-IN, and (5) OC. The size-resolved histograms of these five classes superimposed with the onboard particle size distribution data measured by FIMS are shown in Fig. 3b to highlight the organic and inorganic contributions within individual particles as a function of particle size. A mixture of organic and inorganic particles (OC-IN) appears to be the dominant class across all samples, contributing 40 %–76 % to the total particle population. Furthermore, the consideration of multiple sources of EC from wildfires (Park et al., 2007), residential wood smoke (Allen and Rector, 2020), agricultural burning (Liu et al., 2016; Holder et al., 2017), and urban emissions (Paredes-Miranda et al., 2013) in North America led us to expect a large contribution of EC within our sample. However, OC-EC and OC-EC-IN particles contributed only 0.4 %–1.3 % to the total particle population. EC–soot lifetime is primarily governed by its wet deposition rate, which is dependent on the particle's affinity to absorb water (Barrett et al., 2019). Freshly emitted soot particles are hydrophobic; however, atmospheric processes can increase the hydroscopicity properties of soot particles through the accumulation of OH-initiated oxidation of organics during long-range transport and atmospheric aging (Dzepina et al., 2015), leading to decreased atmospheric lifetime of EC regardless of initial composition (Khalizov et al., 2013; Browne et al., 2015; China et al., 2015). IN particles (i.e., inorganics such as sea salt and sulfates) appear to be consistent with the particle-type observations inferred from CCSEM/EDX data. Singe-component IN particles contribute up to 15 % in the MBL at the time of non-DI periods, while their contribution during DI decreases to ∼0.8 %. Subject to long-range transport, IN-dominant particles also accumulate substantial OC components when encountering DI, and as they entrain into the MBL and create ensembles of ambient particles with complex multi-component internal mixing states through different atmospheric processes such as condensation (Mozurkewich, 1986) and coagulation (Holmes, 2007). Consistently, fractions of single-component OC particles within the MBL during DI periods increased (from 7 % to 22 %) and slightly decreased in the FT layer (from 26 % to 20 %). These observations suggest that entrainment of aerosols with higher extents of internal mixing (from long-range transport) are present in the MBL and can contribute to the regional aerosol composition, which in turn may modify aerosol–cloud interactions typical for the area.
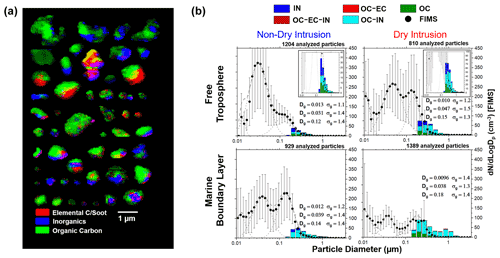
Figure 3(a) Carbon speciation map of a subset of particles acquired by STXM from DI periods. Note that components can overlap, and each pixel can contain a different combination of the individual components: EC + IN constituents as purple; OC + EC as yellow; OC + IN as cyan. (b) Size distribution of analyzed particles identified via STXM/NEXAFS shown as an 8-bin per decade histogram to compare particle multi-component internal mixing state between atmospheric transport events. FIMS particle size distribution is overlaid to facilitate a visual comparison from the same atmospheric episodes. Abbreviations are as follows: IN – inorganics, OC – organic carbon (i.e., COOH), EC – elemental carbon (i.e., sp2 C = C carbon).
NEXAFS spectra (285–294 eV) of individual particles were used to assess the carbon chemical bonding environment allowing, us to identify representative types of OC-containing particles (Moffet et al., 2010a). Figure 4 shows the representative NEXAFS spectra acquired for 103 individual carbon-containing particles. This resulted in the identification of six carbon “types”, as shown along with their illustrative secondary electron-mode SEM imaging. Each carbon type is classified based on characteristic spectral features such as peak positions and relative intensities. For all spectra shown in Fig. 4a, the individual contribution of carbon energy transitions was quantified via spectral deconvolution. Details on the deconvolution process are described in previous works (Moffet et al., 2010b, 2013; Tomlin et al., 2020). Figure 5a shows the deconvolution fit of the averaged NEXAFS spectra for each carbon type identified across different sampling conditions, with Fig. 5b illustrating the contribution of each functional group based on the individual peak area. It is worth noting that the difference in absorption between the post-edge (OD320 eV) and pre-edge (OD278 eV) energies is a measure of the amount of total carbonaceous material in the particles.
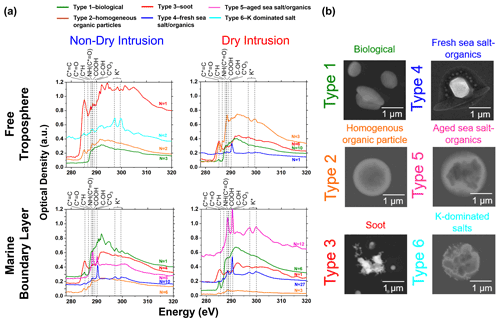
Figure 4(a) Individual NEXAFS spectra showing differences in carbon content of representative particles collected at MBL and FT altitudes under different synoptic conditions. Identified carbon types are the following: type 1 – biological (green), type 2 – homogeneous organic particles (orange), type 3 – soot (red), type 4 – fresh sea salt–organics (blue), type 5 – aged sea salt–organics (pink), and type 6 – K-dominated salt (teal). Dashed lines correspond to the transition energies: 285.4 eV (C* = C), 286.7 eV (C* = O), 287.7 eV (C*–H), 288.3 eV (R-NH(C* = O)R), 288.5 eV (R(C* = O)OH), 289.5 eV (RC*–OH), 290.0 eV (C edge step), 290.4 eV (C*O3), 297.1 eV (K), and 299.7 eV (K). (b) Representative secondary electron-mode SEM imaging of particles corresponding to the different carbon types identified with the STXM/NEXAFS analysis.
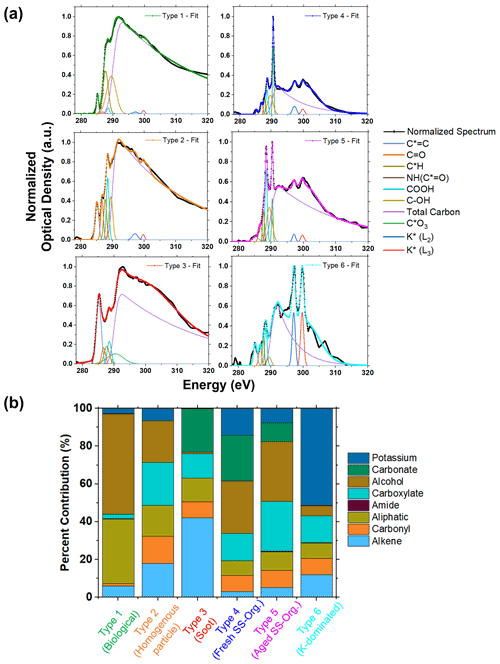
Figure 5(a) Carbon K-edge NEXAFS spectra of six carbon types identified in individual particles: type 1 – biological (green), type 2 – homogeneous organic particles (orange), type 3 – soot (red), type 4 – fresh sea salt–organics (blue), type 5 – aged sea salt–organics (pink), type 6 – K-dominated salt (teal). (b) The contributions of the different carbon functional groups are reported as a percentage of the total peak area.
The type 1 (biological) class has some contribution from alkene groups (C* = C at 285.4 eV) with significant enhancement of aliphatic hydrocarbons (C*–H at 287.7 eV) and alcohol groups (C*–OH at 289.5 eV). These spectra appear to be similar to the reported NEXAFS spectrum for phospholipids, a constituent of cell walls (Lawrence et al., 2003; Nováková et al., 2008). Lipid material is concentrated in the sea surface microlayer through the rupturing of phytoplankton cell membranes (i.e., cell lysis) (X. Wang et al., 2015). A majority of lipid compounds produced by phytoplankton in seawater include glyceroglycolipids, phospholipids, and triacylglycerols containing significant amounts of aliphatic and alcohol groups (Harwood and Guschina, 2009). The transition of aliphatic-rich organic species into the aerosol phase is governed by the bursting of bubble films (Blanchard, 1989) enriched in lipid organic species found on the surface of seawater (X. Wang et al., 2015). The type 2 (homogeneous organic particles) class has almost equivalent peak contributions from each reported functional group as shown in Fig. 5b. The NEXAFS spectrum for type 2 is quantitatively similar to those reported for organic particles from anthropogenic emissions in urban areas of Mexico City (Moffet et al., 2010b) and central California (Moffet et al., 2013). As the aerosol plume is transported away from the source of emission, organic mass increases, while the fraction of C = C decreases (Doran et al., 2007; Kleinman et al., 2008; Moffet et al., 2010b). As a result, organic functional groups build up with particle age such as carboxylic acids, carbonyl, alcohol, and other carbon–oxygen functional groups. It has been suggested that formation of these homogeneous organic particles likely results from the accumulation growth of primary emitted particles as they travel further away from their emission source (Moffet et al., 2010b). Type 3 (soot) had the largest contribution of C* = C at 285.4 eV spectral feature (42 % of peak area contribution). Based on reported literature, this spectrum is comparable with atmospheric particles collected during various field studies of biomass burning emissions (Hopkins et al., 2007). Interestingly, particles collected during aircraft measurements during the Aerosol Characterization Experiment in Asia (ACE-Asia) campaign (Maria et al., 2004) from emissions over mixed combustion sources had nearly identical percentage of sp2 values of around 41 % (Hopkins et al., 2007).
Field and laboratory studies showed that sea salt particles can react with atmospheric water-soluble organic acids, leading to chloride depletion within particles (Laskin et al., 2012; B. Wang et al., 2015). Consistent with these previous studies, fresh sea salt typically has an intact rectangular inorganic core with a carbon outer shell arising from a thin layer of carboxylic acid coating as indicated by the peak for R(C* = O)OH at 288.5 eV. Accordingly, type 4 is referred to as “fresh sea salt” in this work. In addition, the minor quantity of carbonaceous material in type 4, as inferred from the small difference between the post- and pre-edge energies (OD320 eV–OD278 eV) apparent from Fig. 5a, further supports the observation of freshly emitted sea salt particles. In contrast, type 5 (aged sea salt–organics) is made up of sea salt particles that have reacted with carboxylic acid components of organic aerosol condensate, which results in a substantial contribution of the R(C* = O)OH at 288.5 eV peak while retaining the carbonate peak C*O3 at 290.4 eV. Of note is that type 5 (aged sea salt–organics) contains significantly more carbon mass than type 4 (fresh sea salt–organics), as indicated by the NEXAFS spectrum. Finally, the type 6 (K-dominated) class is identified based on the appearance of characteristic potassium peaks at 297.1 eV (K*) and 299.7 eV (K*), with a percent contribution of ∼51 % relative to the total peak area. Potassium-salt particles are common markers of biomass burning smoke (Andreae, 1983; Li et al., 2003). Large fractions of KCl particles are commonly emitted from both flaming and smoldering fires, while atmospheric aging can transform them into K2SO4 and KNO3 through multi-phase acid-displacement reactions similar to those of NaCl (Li et al., 2003). However, these K-dominated particles can also be released as mixed secondary particles containing fractions of organic species, methylsulfonic acid, trimethylamine, SO, NH, and K from potential biogenic sources in oceans (Willis et al., 2017).
3.4 Organic volume fraction of individual mixed organic–inorganic particles
Organic volume fraction (OVF) is a practical parameter to assess reactivity (Worsnop et al., 2002; Folkers et al., 2003) and hygroscopicity (Wang et al., 2008; Schill et al., 2015; Ruehl et al., 2016) of mixed inorganic–organic particles. Based on the STXM/NEXAFS measurements of individual particles, OVF is defined as a ratio of the optical thickness of the organic components (torg) divided by the total optical thickness of the particle (torg+tinorg) (Moffet et al., 2010a; Pham et al., 2017; Fraund et al., 2019). STXM images collected at the carbon K-edge were used to calculate the OVF. The values of absorbance at the pre-edge (278 eV) and the post-edge (320 eV) energies are related to the inorganic mass and the sum of inorganic + organic mass, respectively. Assuming specific values for densities (ρ) and mass absorption coefficients (μ) for the organic and inorganic components, values of torg and tinorg can be determined, allowing OVF calculation (Fraund et al., 2019). For this study, we assumed that the inorganic component of particles corresponds to (NH4)2SO4 based on the particle elemental composition identified by CCSEM/EDX analysis, while oxalic acid (C2H2O4) is used as a proxy for the organic component. Oxalic acid was chosen to represent biomass burning (Yamasoe et al., 2000) and vehicular exhaust (Kawamura and Kaplan, 1987). Of note, based on previous reported studies, is that assumptions of chemically different organic components have a minor effect on the resulting OVF values, while choice of the inorganic components resulted in a larger variation in the OVF calculations (Pham et al., 2017; Fraund et al., 2019). Here, we estimate the systematic error in OVF when assuming different inorganic–organic components, as shown in Table S2. Assuming NaCl to be the inorganic component instead of (NH4)2SO4 yields a difference of ∼35 %. On the other hand, assuming the organic component to be oxalic acid yields a ∼5 %–30 % difference in OVF when compared to other organics such as sucrose, adipic acid, and glucose.
Figure 6 shows representative chemical mixing state maps and OVF values of particles sampled during different atmospheric transport episodes during this study. Particles appear to have a varying amount of organic coating for different sampling episodes as shown on the OVF maps. The comparison of the OVF map and the carbon speciation map illustrates overlap between the two mapping schemes. Finally, histograms show particle fractions at varying OVF values during different atmospheric transport episodes. Layers of organics are seen encapsulating inorganic cores. As expected, background particles collected in the MBL show inorganic NaCl cores (as indicated by a rectangular core morphology) with modest organic coating (OVF <30 %), consistent with a previous report (Chi et al., 2015). However, during the DI periods, the majority of particles have equal or greater fractions of organic-to-inorganic components (40 %–60 % OVF), while only a few particles exhibit core–shell morphology typical for background particles (i.e., non-DI periods). Furthermore, FT particles during non-DI periods have OVF <10 % when compared to FT samples during DI periods (10 %–20 % OVF). In general, samples collected at the FT altitudes show reduced OVF values compared to the MBL samples regardless of the occurrence of DIs. Core–shell particle morphologies were also observed in FT samples, although not frequently (see Fig. S9). FT samples were dominated by inorganic–organic particles in the size range of 0.20–0.25 µm, which are likely mixed sulfate–organic particles based on the size-resolved particle-type datasets obtained from CCSEM/EDX analysis. A recent study conducted in central Oregon found that the organic mass fraction from FT samples was between 27 % and 84 %, while sulfate mass fractions ranged from 39 %–50 % (Zhou et al., 2019). Based on these reported studies the elevated contributions of organics and sulfate in the FT may be attributed to the enrichment of organonitrates and organosulfate compounds originating from biogenic sources in the absence of wildfire influence. However, FT organic and sulfate aerosol mass is also known to be associated with urban and biomass burning emissions (Bahreini, 2003; Dunlea et al., 2009; Roberts et al., 2010; Y. Wang et al., 2021). Studies in the northeastern Pacific found that submicron aerosol mass was dominated by sulfate and organic components originating from aged Asian pollution plumes (Dunlea et al., 2009). FT organic and sulfate particles can then experience long-range transport and aging as the air parcels are carried across the Atlantic and descend into the MBL of the ENA site (China et al., 2017). To summarize, we observe enhancements in the OVF values of individual particles during the DI periods, quantified as 2.06 ± 0.16- and 1.11 ± 0.04-fold increases in OVF for the MBL and FT samples, respectively, assuming (NH4)2SO4–oxalic acid components. The larger total OVF in the MBL (relative to FT samples) regardless of DI events is most likely due to the additional contribution of marine organic sources within the boundary layer. The background organic concentration in the MBL is different than FT due to other sources of organics such as dissolved organic matter on the seawater surface (Doval et al., 2001; Miyazaki et al., 2018). The transport of organics from the ocean surface directly into the atmosphere is primarily driven by turbulent winds (O'Dowd et al., 2004; Prather et al., 2013), resulting in the enhancement of the background organic concentration in the MBL. Furthermore, the observed enhancements in OVF in the MBL during DI periods could be the result of organic-rich air parcels (originating from North America) descending from the FT into the MBL, leading to changes in the total organic concentration (Zheng et al., 2020b; Y. Wang et al., 2021).
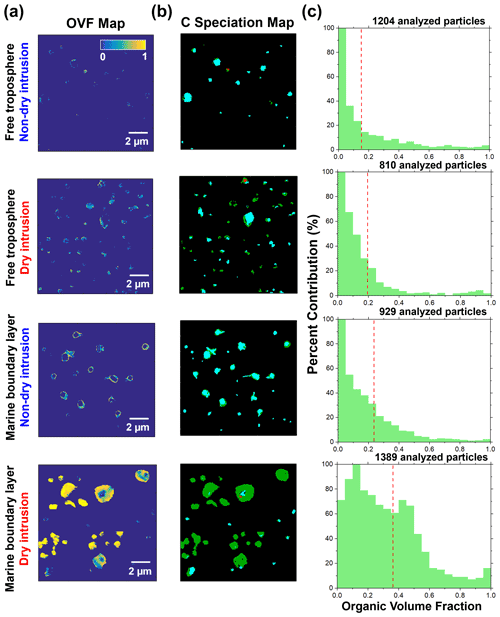
Figure 6(a) Representative organic volume fraction (OVF) maps of individual particles. (a) Carbon speciation maps of the identical particles; teal – inorganic dominant regions; green – organic dominant regions (i.e., COOH); red – elemental carbon (i.e., sp2 carbon). (c) Histogram of particle fractions as a function of their OVF values with average OVF (red dashed line). The rows correspond to the different atmospheric layers and synoptic conditions to highlight the differences in organic–inorganic composition and multi-component internal mixing state of particles identified in this study.
3.5 Evaluating CCN activity of mixed organic–inorganic particles
CCN activity of individual particles is governed by both their size and chemical composition. In particular, condensation of organic carbon onto atmospheric inorganic particles can impact the efficiency at which particles of mixed organic–inorganic composition can act as CCN and INPs due to changes in particles' hygroscopicity and viscosity (Beydoun et al., 2017; Ovadnevaite et al., 2017; Altaf et al., 2018). To account for the effects of organics on aerosol hygroscopicity, we use the κ-Köhler equation (Petters and Kreidenweis, 2007) to estimate the hygroscopicity parameter κ corresponding to mixed inorganic–organic particles:
where forg is the OVF value derived from the STXM data, κorg=0.1 is the hygroscopicity of the organic component, and κinorg=0.6 is that of (NH4)2SO4 (Petters and Kreidenweis, 2007). We derived κ values for different synoptic and atmospheric layer conditions using the size-resolved OVF ratio shown in Fig. S10, and we found that κMBL, DI=0.41 and κFT, DI=0.33 for DI periods and that κMBL, non-DI=0.48 and κFT, non-DI=0.36 for non-DI periods. The lower κ values under DI periods are consistent with enhancements in the organic contribution. Of note is that the values of κ obtained here using Eq. (2) need to be considered the low-limit values, which might be somewhat higher considering possible contributions from more hygroscopic components of particles related to original and aged sea salt (κNaCl=1.3 and ).
Using κ, we can calculate the critical size of a dry particle (Fig. S11) that can be activated under the supersaturation of 0.14 % (setting of the CCN counter deployed on G-1) (Petters and Kreidenweis, 2007). The theoretical CCN number concentrations are then estimated by integrating the FIMS-measured aerosol size distributions above the critical dry particle diameter. Figure 7 shows the results for the OVF-based calculations of theoretical CCN concentrations compared to the onboard CCN measurements at 0.14 % supersaturation. There is general agreement between calculated and measured CCN concentration, but FT cases appear to have better agreement. The uncertainty in the calculated CCN is due to the supersaturation fluctuation of the CCN counter (0.13 %–0.15 %), as shown in Fig. S11. The large error bars in the measured CCN are a result of the variability of the measured CCN during different sampling periods. We also note that the exact value of κorg may play a role in affecting the CCN calculation. So, theoretical CCN concentrations were also calculated using κorg=0, and the results are compared against the measured CCN concentrations in Fig. 7b. However, the impact of this change in κorg does not significantly change the agreement between the calculated and measured CCN concentrations. This result shows that calculating the CCN concentration using OVF values derived from the STXM data and the κ-Köhler theory can be a good estimate of the actual CCN concentrations.
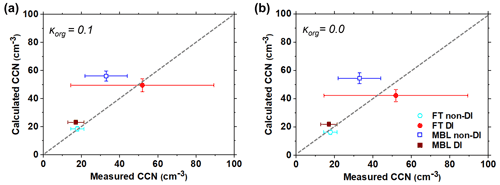
Figure 7Comparison of the CCN concentration predicted from the particle size distribution and OVF with field-measured CCN by onboard instruments across the different atmospheric layer and transport event. (a) κorg=0.1; (b) κorg=0.0. The gray dashed line corresponds to the 1:1 calculated CCN to measured CCN.
Here, we presented detailed chemical imaging of individual atmospheric particles collected over the Azores during long-range transport events. Air mass back-trajectory calculations suggest that air parcels in the ENA region can be traced from more than 4000 km away from North America within a span of 48–72 h. During these long-range transport episodes, aerosols undergo substantial changes in size, morphology, and chemical composition among others as they are carried across the Atlantic Ocean and descend from the FT into the MBL altitudes over the ENA region. Chemical compositions of elements of individual particles (∼36 400) were quantified using CCSEM/EDX, while a subset of particles (∼4300) was analyzed using STXM/NEXAFS to determine the particle internal mixing state and organic spatial distribution. Based on CCSEM/EDX analysis, we observe a substantial contribution of carbonaceous particles, which are the dominant particle type across all samples. The fraction of externally mixed carbonaceous particles decreases during the DI periods, compensated for by the increase in the ammonium nitrate–sulfate fraction. The elevated contribution of atmospheric nitrate suggests influence from anthropogenic and biomass burning emissions (Reff et al., 2009). This observation is consistent with the DI periods, suggesting that air masses originating from North America descend from FT to MBL over the ENA region. Interestingly, there is also an increase in particle-type diversity in the FT during DI periods, most likely due to significant mixing during DI episodes based on measured particle number concentrations. Among these identified carbonaceous particles, the OVF across individual particles derived from STXM measurements is enhanced in DI samples. Aged aerosols accumulate organics through condensation of secondary semi-volatile species, resulting in an increase in the organic contribution among individual particles. We utilize the STXM-derived OVF values and implement them in the calculation of particle hygroscopicity using κ-Köhler theory (Petters and Kreidenweis, 2007). Particles collected during DI periods resulted in lower κ values with respect to background marine aerosols common in the ENA region, resulting in reduced CCN propensity. We calculated κ values between ∼0.29 and ∼0.44, corresponding to mixed organic–inorganic aerosol in the FT and MBL, respectively. These values are consistent with previous studies on mixed organic particles (Petters and Kreidenweis, 2007; Schmale et al., 2018; Zheng et al., 2020b).
Current atmospheric models lack the representation of aerosol mixing states, limiting studies to only simple assumptions and leading to high uncertainty of the aerosol impact on the Earth system. It is traditionally assumed that sulfate particles dictate particle growth over remote ocean regions, while the influence of organic particles on the CCN activity over remote oceans is underestimated. We have shown that particles transported from North America can have a substantial impact on the aerosol mixing state and aerosol population over the region of study, as organic contribution and particle-type diversity are significantly enhanced during the DI periods. These observations need to be considered in current atmospheric models to have a better predictive understanding of the impact of long-range transport episodes on the source apportionment of specific aerosol particle types and the extent of particle internal heterogeneity.
The scripts used in this work to process STXM/NEXAFS datasets are available at https://github.com/MFraund/OrganicVolumeFraction_StandardAerosols (last access: 8 December 2021) (https://doi.org/10.5194/amt-12-1619-2019, Fraund et al., 2019) and at https://www.mathworks.com/matlabcentral/fileexchange/29085-stxm-spectromicroscopy-particle-analysis-routines (last access: 8 December 2021) (https://doi.org/10.1021/ac1012909, Moffet et al., 2010a).
The dataset used for this work is available for download as a .zip file from https://doi.org/10.4231/6CT5-3R55 (Tomlin et al., 2021).
The supplement related to this article is available online at: https://doi.org/10.5194/acp-21-18123-2021-supplement.
DPV, SC, DAK, RCM, JWa, and AL designed the study. DPV and JWa executed sample collection and data acquisition during field deployment. SRR performed modeling tasks of the study. JMT, KAJ, DPV, SC, PW, MF, JWe, FRA, DAK, RCM, and MKG performed chemical imaging experiments and analyzed associated data. GZ, YW, and JWa analyzed real-time data from G-1. JMT and AL wrote the paper with contributions from all coauthors.
The contact author has declared that neither they nor their co-authors have any competing interests.
Publisher's note: Copernicus Publications remains neutral with regard to jurisdictional claims in published maps and institutional affiliations.
This article is part of the special issue “Marine aerosols, trace gases, and clouds over the North Atlantic (ACP/AMT inter-journal SI)”. It is not associated with a conference.
We would like to thank the ACE-ENA campaign team for their help and support. The Purdue University, Washington University, Stonybrook University, and STI groups gratefully acknowledge support from the U.S. Department of Energy’s Atmospheric System Research (ASR) program, Office of Biological and Environmental Research (OBER). Shira Raveh-Rubin acknowledges support from the Israel Science Foundation. The research used STXM/NEXAFS instruments at beamline 5.3.2.2 and 11.0.2 at the Advanced Light Source at Lawrence Berkeley National Laboratory with guidance from David Kilcoyne, Matthew Markus, Hendrik Ohldag, and David Shapiro. In addition, the soft X-ray spectromicroscopy 10ID-1 beamline at the Canadian Light Source was also used in this study, with assistance from beamline scientist Jian Wang. We used the CCSEM/EDX instrument at the Environmental Molecular Sciences Laboratory located at the Pacific Northwest National Laboratory. We thank John Shilling for providing the data collected from the Aerodyne HR-ToF-AMS on board the G-1 aircraft during the ACE-ENA campaign. The authors gratefully acknowledge the NOAA Air Resources Laboratory (ARL) for the provision of the HYSPLIT transport and dispersion model as well as the READY website (https://www.ready.noaa.gov, last access: 31 August 2020) used in this publication.
This research has been supported by the Office of Biological and Environmental Research (grant no. DE-SC0018948, Purdue/STI group; grant no. DE-SC0020259, Washington University; and grant nos. SC0016370 and SC0021034, Stonybrook University) and the Israel Science Foundation (grant no. 1347/18, Weizmann Institute).
This paper was edited by Armin Sorooshian and reviewed by two anonymous referees.
Ackerman, A. S., Kirkpatrick, M. P., Stevens, D. E., and Toon, O. B.: The impact of humidity above stratiform clouds on indirect aerosol climate forcing, Nature, 432, 1014–1017, https://doi.org/10.1038/nature03174, 2004.
Adachi, K., Oshima, N., Gong, Z., de Sá, S., Bateman, A. P., Martin, S. T., de Brito, J. F., Artaxo, P., Cirino, G. G., Sedlacek III, A. J., and Buseck, P. R.: Mixing states of Amazon basin aerosol particles transported over long distances using transmission electron microscopy, Atmos. Chem. Phys., 20, 11923–11939, https://doi.org/10.5194/acp-20-11923-2020, 2020.
Allen, G. and Rector, L.: Characterization of Residential Woodsmoke PM2.5 in the Adirondacks of New York, Aerosol Air Qual. Res., 20, 2419–2432, https://doi.org/10.4209/aaqr.2020.01.0005, 2020.
Altaf, M. B., Dutcher, D. D., Raymond, T. M., and Freedman, M. A.: Effect of Particle Morphology on Cloud Condensation Nuclei Activity, ACS Earth Space Chem., 2, 634–639, https://doi.org/10.1021/acsearthspacechem.7b00146, 2018.
Altaratz, O., Koren, I., Remer, L. A., and Hirsch, E.: Review: Cloud invigoration by aerosols – Coupling between microphysics and dynamics, Atmos. Res., 140–141, 38–60, https://doi.org/10.1016/j.atmosres.2014.01.009, 2014.
Andreae, M. O.: Soot Carbon and Excess Fine Potassium: Long-Range Transport of Combustion-Derived Aerosols, Science, 220, 1148–1151, https://doi.org/10.1126/science.220.4602.1148, 1983.
Andreae, M. O., Ferek, R. J., Bermond, F., Byrd, K. P., Engstrom, R. T., Hardin, S., Houmere, P. D., LeMarrec, F., Raemdonck, H., and Chatfield, R. B.: Dimethyl sulfide in the marine atmosphere, J. Geophys. Res., 90, 12891, https://doi.org/10.1029/JD090iD07p12891, 1985.
Ault, A. P., Gaston, C. J., Wang, Y., Dominguez, G., Thiemens, M. H., and Prather, K. A.: Characterization of the Single Particle Mixing State of Individual Ship Plume Events Measured at the Port of Los Angeles, Environ. Sci. Technol., 44, 1954–1961, https://doi.org/10.1021/es902985h, 2010.
Ault, A. P., Peters, T. M., Sawvel, E. J., Casuccio, G. S., Willis, R. D., Norris, G. A., and Grassian, V. H.: Single-Particle SEM-EDX Analysis of Iron-Containing Coarse Particulate Matter in an Urban Environment: Sources and Distribution of Iron within Cleveland, Ohio, Environ. Sci. Technol., 46, 4331–4339, https://doi.org/10.1021/es204006k, 2012.
Ault, A. P., Moffet, R. C., Baltrusaitis, J., Collins, D. B., Ruppel, M. J., Cuadra-Rodriguez, L. A., Zhao, D., Guasco, T. L., Ebben, C. J., Geiger, F. M., Bertram, T. H., Prather, K. A., and Grassian, V. H.: Size-Dependent Changes in Sea Spray Aerosol Composition and Properties with Different Seawater Conditions, Environ. Sci. Technol., 47, 5603–5612, https://doi.org/10.1021/es400416g, 2013.
Bahreini, R.: Aircraft-based aerosol size and composition measurements during ACE-Asia using an Aerodyne aerosol mass spectrometer, J. Geophys. Res., 108, 8645–8658, https://doi.org/10.1029/2002JD003226, 2003.
Barrett, T. E., Ponette-González, A. G., Rindy, J. E., and Weathers, K. C.: Wet deposition of black carbon: A synthesis, Atmos. Environ., 213, 558–567, https://doi.org/10.1016/j.atmosenv.2019.06.033, 2019.
Beydoun, H., Polen, M., and Sullivan, R. C.: A new multicomponent heterogeneous ice nucleation model and its application to Snomax bacterial particles and a Snomax–illite mineral particle mixture, Atmos. Chem. Phys., 17, 13545–13557, https://doi.org/10.5194/acp-17-13545-2017, 2017.
Blanchard, D. C.: The Ejection of Drops from the Sea and Their Enrichment with Bacteria and Other Materials: A Review, Estuaries, 12, 127–137, https://doi.org/10.2307/1351816, 1989.
Bond, T. C., Streets, D. G., Yarber, K. F., Nelson, S. M., Woo, J.-H., and Klimont, Z.: A technology-based global inventory of black and organic carbon emissions from combustion, J. Geophys. Res., 109, D14203, https://doi.org/10.1029/2003JD003697, 2004.
Bondy, A. L., Bonanno, D., Moffet, R. C., Wang, B., Laskin, A., and Ault, A. P.: The diverse chemical mixing state of aerosol particles in the southeastern United States, Atmos. Chem. Phys., 18, 12595–12612, https://doi.org/10.5194/acp-18-12595-2018, 2018.
Bony, S.: Marine boundary layer clouds at the heart of tropical cloud feedback uncertainties in climate models, Geophys. Res. Lett., 32, L20806, https://doi.org/10.1029/2005GL023851, 2005.
Browne, E. C., Franklin, J. P., Canagaratna, M. R., Massoli, P., Kirchstetter, T. W., Worsnop, D. R., Wilson, K. R., and Kroll, J. H.: Changes to the Chemical Composition of Soot from Heterogeneous Oxidation Reactions, J. Phys. Chem. A, 119, 1154–1163, https://doi.org/10.1021/jp511507d, 2015.
Browning, K. A.: The dry intrusion perspective of extra-tropical cyclone development, Meteorol. Appl., 4, 317–324, https://doi.org/10.1017/S1350482797000613, 1997.
Buseck, P. R. and Posfai, M.: Airborne minerals and related aerosol particles: Effects on climate and the environment, P. Natl. Acad. Sci. USA, 96, 3372–3379, https://doi.org/10.1073/pnas.96.7.3372, 1999.
Catto, J. L. and Raveh-Rubin, S.: Climatology and dynamics of the link between dry intrusions and cold fronts during winter. Part I: global climatology, Clim. Dynam., 53, 1873–1892, https://doi.org/10.1007/s00382-019-04745-w, 2019.
Chi, J. W., Li, W. J., Zhang, D. Z., Zhang, J. C., Lin, Y. T., Shen, X. J., Sun, J. Y., Chen, J. M., Zhang, X. Y., Zhang, Y. M., and Wang, W. X.: Sea salt aerosols as a reactive surface for inorganic and organic acidic gases in the Arctic troposphere, Atmos. Chem. Phys., 15, 11341–11353, https://doi.org/10.5194/acp-15-11341-2015, 2015.
China, S., Scarnato, B., Owen, R. C., Zhang, B., Ampadu, M. T., Kumar, S., Dzepina, K., Dziobak, M. P., Fialho, P., Perlinger, J. A., Hueber, J., Helmig, D., Mazzoleni, L. R., and Mazzoleni, C.: Morphology and mixing state of aged soot particles at a remote marine free troposphere site: Implications for optical properties, Geophys. Res. Lett., 42, 1243–1250, https://doi.org/10.1002/2014GL062404, 2015.
China, S., Alpert, P. A., Zhang, B., Schum, S., Dzepina, K., Wright, K., Owen, R. C., Fialho, P., Mazzoleni, L. R., Mazzoleni, C., and Knopf, D. A.: Ice cloud formation potential by free tropospheric particles from long-range transport over the Northern Atlantic Ocean: Ice cloud formation by aged particles, J. Geophys. Res.-Atmos., 122, 3065–3079, https://doi.org/10.1002/2016JD025817, 2017.
Cruz, C. N. and Pandis, S. N.: A study of the ability of pure secondary organic aerosol to act as cloud condensation nuclei, Atmos. Environ., 31, 2205–2214, https://doi.org/10.1016/S1352-2310(97)00054-X, 1997.
Cubison, M. J., Ortega, A. M., Hayes, P. L., Farmer, D. K., Day, D., Lechner, M. J., Brune, W. H., Apel, E., Diskin, G. S., Fisher, J. A., Fuelberg, H. E., Hecobian, A., Knapp, D. J., Mikoviny, T., Riemer, D., Sachse, G. W., Sessions, W., Weber, R. J., Weinheimer, A. J., Wisthaler, A., and Jimenez, J. L.: Effects of aging on organic aerosol from open biomass burning smoke in aircraft and laboratory studies, Atmos. Chem. Phys., 11, 12049–12064, https://doi.org/10.5194/acp-11-12049-2011, 2011.
Dall'Osto, M., Ceburnis, D., Monahan, C., Worsnop, D. R., Bialek, J., Kulmala, M., Kurtén, T., Ehn, M., Wenger, J., Sodeau, J., Healy, R., and O'Dowd, C.: Nitrogenated and aliphatic organic vapors as possible drivers for marine secondary organic aerosol growth: Marine secondary organic aerosol growth, J. Geophys. Res.-Atmos., 117, D12311, https://doi.org/10.1029/2012JD017522, 2012.
DeCarlo, P. F., Kimmel, J. R., Trimborn, A., Northway, M. J., Jayne, J. T., Aiken, A. C., Gonin, M., Fuhrer, K., Horvath, T., Docherty, K. S., Worsnop, D. R., and Jimenez, J. L.: Field-Deployable, High-Resolution, Time-of-Flight Aerosol Mass Spectrometer, Anal. Chem., 78, 8281–8289, https://doi.org/10.1021/ac061249n, 2006.
Dee, D. P., Uppala, S. M., Simmons, A. J., Berrisford, P., Poli, P., Kobayashi, S., Andrae, U., Balmaseda, M. A., Balsamo, G., Bauer, P., Bechtold, P., Beljaars, A. C. M., van de Berg, L., Bidlot, J., Bormann, N., Delsol, C., Dragani, R., Fuentes, M., Geer, A. J., Haimberger, L., Healy, S. B., Hersbach, H., Hólm, E. V., Isaksen, L., Kållberg, P., Köhler, M., Matricardi, M., McNally, A. P., Monge-Sanz, B. M., Morcrette, J.-J., Park, B.-K., Peubey, C., de Rosnay, P., Tavolato, C., Thépaut, J.-N., and Vitart, F.: The ERA-Interim reanalysis: configuration and performance of the data assimilation system, Q. J. Roy. Meteor. Soc., 137, 553–597, https://doi.org/10.1002/qj.828, 2011.
Doran, J. C., Barnard, J. C., Arnott, W. P., Cary, R., Coulter, R., Fast, J. D., Kassianov, E. I., Kleinman, L., Laulainen, N. S., Martin, T., Paredes-Miranda, G., Pekour, M. S., Shaw, W. J., Smith, D. F., Springston, S. R., and Yu, X.-Y.: The T1-T2 study: evolution of aerosol properties downwind of Mexico City, Atmos. Chem. Phys., 7, 1585–1598, https://doi.org/10.5194/acp-7-1585-2007, 2007.
Doval, M. D., Álvarez-Salgado, X. A., and Pérez, F. F.: Organic matter distributions in the Eastern North Atlantic–Azores Front region, J. Mar. Syst., 30, 33–49, https://doi.org/10.1016/S0924-7963(01)00036-7, 2001.
Dunlea, E. J., DeCarlo, P. F., Aiken, A. C., Kimmel, J. R., Peltier, R. E., Weber, R. J., Tomlinson, J., Collins, D. R., Shinozuka, Y., McNaughton, C. S., Howell, S. G., Clarke, A. D., Emmons, L. K., Apel, E. C., Pfister, G. G., van Donkelaar, A., Martin, R. V., Millet, D. B., Heald, C. L., and Jimenez, J. L.: Evolution of Asian aerosols during transpacific transport in INTEX-B, Atmos. Chem. Phys., 9, 7257–7287, https://doi.org/10.5194/acp-9-7257-2009, 2009.
Dzepina, K., Mazzoleni, C., Fialho, P., China, S., Zhang, B., Owen, R. C., Helmig, D., Hueber, J., Kumar, S., Perlinger, J. A., Kramer, L. J., Dziobak, M. P., Ampadu, M. T., Olsen, S., Wuebbles, D. J., and Mazzoleni, L. R.: Molecular characterization of free tropospheric aerosol collected at the Pico Mountain Observatory: a case study with a long-range transported biomass burning plume, Atmos. Chem. Phys., 15, 5047–5068, https://doi.org/10.5194/acp-15-5047-2015, 2015.
ECMWF: Integrated forecasting system's documentation, Part IV: Physical processes, (IFS Documentation CY31R1), https://doi.org/10.21957/4whwo8jw0, 2007.
Facchini, M. C., Rinaldi, M., Decesari, S., Carbone, C., Finessi, E., Mircea, M., Fuzzi, S., Ceburnis, D., Flanagan, R., Nilsson, E. D., de Leeuw, G., Martino, M., Woeltjen, J., and O'Dowd, C. D.: Primary submicron marine aerosol dominated by insoluble organic colloids and aggregates, Geophys. Res. Lett., 35, L17814, https://doi.org/10.1029/2008GL034210, 2008.
Finlayson-Pitts, B. J.: The Tropospheric Chemistry of Sea Salt: A Molecular-Level View of the Chemistry of NaCl and NaBr, Chem. Rev., 103, 4801–4822, https://doi.org/10.1021/cr020653t, 2003.
Folkers, M., Mentel, Th. F., and Wahner, A.: Influence of an organic coating on the reactivity of aqueous aerosols probed by the heterogeneous hydrolysis of N2O5: Organic coatings and aerosol reactivity, Geophys. Res. Lett., 30, 1644, https://doi.org/10.1029/2003GL017168, 2003.
Fraund, M., Pham, D., Bonanno, D., Harder, T., Wang, B., Brito, J., de Sá, S., Carbone, S., China, S., Artaxo, P., Martin, S., Pöhlker, C., Andreae, M., Laskin, A., Gilles, M., and Moffet, R.: Elemental Mixing State of Aerosol Particles Collected in Central Amazonia during GoAmazon2014/15, Atmosphere, 8, 173–201, https://doi.org/10.3390/atmos8090173, 2017.
Fraund, M., Park, T., Yao, L., Bonanno, D., Pham, D. Q., and Moffet, R. C.: Quantitative capabilities of STXM to measure spatially resolved organic volume fractions of mixed organic/inorganic particles, Atmos. Meas. Tech., 12, 1619–1633, https://doi.org/10.5194/amt-12-1619-2019, 2019 (data available at: https://github.com/MFraund/OrganicVolumeFraction_StandardAerosols, last access: 8 December 2021).
Froyd, K. D., Murphy, D. M., Brock, C. A., Campuzano-Jost, P., Dibb, J. E., Jimenez, J.-L., Kupc, A., Middlebrook, A. M., Schill, G. P., Thornhill, K. L., Williamson, C. J., Wilson, J. C., and Ziemba, L. D.: A new method to quantify mineral dust and other aerosol species from aircraft platforms using single-particle mass spectrometry, Atmos. Meas. Tech., 12, 6209–6239, https://doi.org/10.5194/amt-12-6209-2019, 2019.
Gonçalves, S. J., Weis, J., China, S., Evangelista, H., Harder, T. H., Müller, S., Sampaio, M., Laskin, A., Gilles, M. K., and Godoi, R. H. M.: Photochemical reactions on aerosols at West Antarctica: A molecular case-study of nitrate formation among sea salt aerosols, Sci. Total Environ., 758, 143586, https://doi.org/10.1016/j.scitotenv.2020.143586, 2021.
Gunsch, M. J., Kirpes, R. M., Kolesar, K. R., Barrett, T. E., China, S., Sheesley, R. J., Laskin, A., Wiedensohler, A., Tuch, T., and Pratt, K. A.: Contributions of transported Prudhoe Bay oil field emissions to the aerosol population in Utqiaġvik, Alaska, Atmos. Chem. Phys., 17, 10879–10892, https://doi.org/10.5194/acp-17-10879-2017, 2017.
Hamilton, D. S., Lee, L. A., Pringle, K. J., Reddington, C. L., Spracklen, D. V., and Carslaw, K. S.: Occurrence of pristine aerosol environments on a polluted planet, P. Natl. Acad. Sci. USA, 111, 18466–18471, https://doi.org/10.1073/pnas.1415440111, 2014.
Harwood, J. L. and Guschina, I. A.: The versatility of algae and their lipid metabolism, Biochimie, 91, 679–684, https://doi.org/10.1016/j.biochi.2008.11.004, 2009.
Hems, R. F., Schnitzler, E. G., Liu-Kang, C., Cappa, C. D., and Abbatt, J. P. D.: Aging of Atmospheric Brown Carbon Aerosol, ACS Earth Space Chem., 5, 722–748, https://doi.org/10.1021/acsearthspacechem.0c00346, 2021.
Hodshire, A. L., Campuzano-Jost, P., Kodros, J. K., Croft, B., Nault, B. A., Schroder, J. C., Jimenez, J. L., and Pierce, J. R.: The potential role of methanesulfonic acid (MSA) in aerosol formation and growth and the associated radiative forcings, Atmos. Chem. Phys., 19, 3137–3160, https://doi.org/10.5194/acp-19-3137-2019, 2019.
Holder, A. L., Gullett, B. K., Urbanski, S. P., Elleman, R., O'Neill, S., Tabor, D., Mitchell, W., and Baker, K. R.: Emissions from prescribed burning of agricultural fields in the Pacific Northwest, Atmos. Environ., 166, 22–33, https://doi.org/10.1016/j.atmosenv.2017.06.043, 2017.
Holmes, N. S.: A review of particle formation events and growth in the atmosphere in the various environments and discussion of mechanistic implications, Atmos. Environ., 41, 2183–2201, https://doi.org/10.1016/j.atmosenv.2006.10.058, 2007.
Hopkins, R. J., Tivanski, A. V., Marten, B. D., and Gilles, M. K.: Chemical bonding and structure of black carbon reference materials and individual carbonaceous atmospheric aerosols, J. Aerosol Sci., 38, 573–591, https://doi.org/10.1016/j.jaerosci.2007.03.009, 2007.
Igel, A. L., Ekman, A. M. L., Leck, C., Tjernström, M., Savre, J., and Sedlar, J.: The free troposphere as a potential source of arctic boundary layer aerosol particles: Free Troposphere and Boundary Layer Arctic Aerosol, Geophys. Res. Lett., 44, 7053–7060, https://doi.org/10.1002/2017GL073808, 2017.
Ilotoviz, E., Ghate, V. P., and Raveh-Rubin, S.: The Impact of Slantwise Descending Dry Intrusions on the Marine Boundary Layer and Air-Sea Interface Over the ARM Eastern North Atlantic Site, J. Geophys. Res.-Atmos., 126, e2020JD033879, https://doi.org/10.1029/2020JD033879, 2021.
Jacobson, M. Z.: Strong radiative heating due to the mixing state of black carbon in atmospheric aerosols, Nature, 409, 695–697, https://doi.org/10.1038/35055518, 2001.
Johnson, B. T., Shine, K. P., and Forster, P. M.: The semi-direct aerosol effect: Impact of absorbing aerosols on marine stratocumulus, Q. J. Roy. Meteor. Soc., 130, 1407–1422, https://doi.org/10.1256/qj.03.61, 2004.
Kawamura, K. and Kaplan, I. R.: Motor exhaust emissions as a primary source for dicarboxylic acids in Los Angeles ambient air, Environ. Sci. Technol., 21, 105–110, https://doi.org/10.1021/es00155a014, 1987.
Khalizov, A. F., Lin, Y., Qiu, C., Guo, S., Collins, D., and Zhang, R.: Role of OH-Initiated Oxidation of Isoprene in Aging of Combustion Soot, Environ. Sci. Technol., 47, 2254–2263, https://doi.org/10.1021/es3045339, 2013.
Kilcoyne, A. L. D., Tyliszczak, T., Steele, W. F., Fakra, S., Hitchcock, P., Franck, K., Anderson, E., Harteneck, B., Rightor, E. G., Mitchell, G. E., Hitchcock, A. P., Yang, L., Warwick, T., and Ade, H.: Interferometer-controlled scanning transmission X-ray microscopes at the Advanced Light Source, J. Synchrotron Radiat., 10, 125–136, https://doi.org/10.1107/S0909049502017739, 2003.
King, S. M., Butcher, A. C., Rosenoern, T., Coz, E., Lieke, K. I., de Leeuw, G., Nilsson, E. D., and Bilde, M.: Investigating Primary Marine Aerosol Properties: CCN Activity of Sea Salt and Mixed Inorganic–Organic Particles, Environ. Sci. Technol., 46, 10405–10412, https://doi.org/10.1021/es300574u, 2012.
Klein, S. A., Zhang, Y., Zelinka, M. D., Pincus, R., Boyle, J., and Gleckler, P. J.: Are climate model simulations of clouds improving? An evaluation using the ISCCP simulator: Evaluating clouds in climate models, J. Geophys. Res.-Atmos., 118, 1329–1342, https://doi.org/10.1002/jgrd.50141, 2013.
Kleinman, L. I., Springston, S. R., Daum, P. H., Lee, Y.-N., Nunnermacker, L. J., Senum, G. I., Wang, J., Weinstein-Lloyd, J., Alexander, M. L., Hubbe, J., Ortega, J., Canagaratna, M. R., and Jayne, J.: The time evolution of aerosol composition over the Mexico City plateau, Atmos. Chem. Phys., 8, 1559–1575, https://doi.org/10.5194/acp-8-1559-2008, 2008.
Kloss, C., Berthet, G., Sellitto, P., Ploeger, F., Bucci, S., Khaykin, S., Jégou, F., Taha, G., Thomason, L. W., Barret, B., Le Flochmoen, E., von Hobe, M., Bossolasco, A., Bègue, N., and Legras, B.: Transport of the 2017 Canadian wildfire plume to the tropics via the Asian monsoon circulation, Atmos. Chem. Phys., 19, 13547–13567, https://doi.org/10.5194/acp-19-13547-2019, 2019.
Korhonen, H., Carslaw, K. S., Spracklen, D. V., Mann, G. W., and Woodhouse, M. T.: Influence of oceanic dimethyl sulfide emissions on cloud condensation nuclei concentrations and seasonality over the remote Southern Hemisphere oceans: A global model study, J. Geophys. Res., 113, D15204, https://doi.org/10.1029/2007JD009718, 2008.
Korolev, A. V., Emery, E. F., Strapp, J. W., Cober, S. G., Isaac, G. A., Wasey, M., and Marcotte, D.: Small Ice Particles in Tropospheric Clouds: Fact or Artifact? Airborne Icing Instrumentation Evaluation Experiment, B. Am. Meteorol. Soc., 92, 967–973, https://doi.org/10.1175/2010BAMS3141.1, 2011.
Kulkarni, P. and Wang, J.: New fast integrated mobility spectrometer for real-time measurement of aerosol size distribution – I: Concept and theory, J. Aerosol Sci., 37, 1303–1325, https://doi.org/10.1016/j.jaerosci.2006.01.005, 2006.
Kulmala, M., Pirjola, L., and Mäkelä, J. M.: Stable sulphate clusters as a source of new atmospheric particles, Nature, 404, 66–69, https://doi.org/10.1038/35003550, 2000.
Laskin, A., Iedema, M. J., and Cowin, J. P.: Time-Resolved Aerosol Collector for CCSEM/EDX Single-Particle Analysis, Aerosol Sci. Technol., 37, 246–260, https://doi.org/10.1080/02786820300945, 2003.
Laskin, A., Wietsma, T. W., Krueger, B. J., and Grassian, V. H.: Heterogeneous chemistry of individual mineral dust particles with nitric acid: A combined CCSEM/EDX, ESEM, and ICP-MS study, J. Geophys. Res., 110, D10208, https://doi.org/10.1029/2004JD005206, 2005.
Laskin, A., Cowin, J. P., and Iedema, M. J.: Analysis of individual environmental particles using modern methods of electron microscopy and X-ray microanalysis, J. Electron Spectrosc. Relat. Phenom., 150, 260–274, https://doi.org/10.1016/j.elspec.2005.06.008, 2006.
Laskin, A., Moffet, R. C., Gilles, M. K., Fast, J. D., Zaveri, R. A., Wang, B., Nigge, P., and Shutthanandan, J.: Tropospheric chemistry of internally mixed sea salt and organic particles: Surprising reactivity of NaCl with weak organic acids: Mixed Sea Salt/Organics Particles, J. Geophys. Res.-Atmos., 117, D15302, https://doi.org/10.1029/2012JD017743, 2012.
Laskin, A., Moffet, R. C., and Gilles, M. K.: Chemical Imaging of Atmospheric Particles, Acc. Chem. Res., 52, 3419–3431, https://doi.org/10.1021/acs.accounts.9b00396, 2019.
Lawrence, J. R., Swerhone, G. D. W., Leppard, G. G., Araki, T., Zhang, X., West, M. M., and Hitchcock, A. P.: Scanning Transmission X-Ray, Laser Scanning, and Transmission Electron Microscopy Mapping of the Exopolymeric Matrix of Microbial Biofilms, Appl. Environ. Microbiol., 69, 5543–5554, https://doi.org/10.1128/AEM.69.9.5543-5554.2003, 2003.
Levin, E. J. T., McMeeking, G. R., Carrico, C. M., Mack, L. E., Kreidenweis, S. M., Wold, C. E., Moosmüller, H., Arnott, W. P., Hao, W. M., Collett, J. L., and Malm, W. C.: Biomass burning smoke aerosol properties measured during Fire Laboratory at Missoula Experiments (FLAME), J. Geophys. Res., 115, D18210, https://doi.org/10.1029/2009JD013601, 2010.
Levin, Z. and Cotton, W. R. (Eds.): Aerosol pollution impact on precipitation: a scientific review, Springer, Dordrechet, the Netherlands, 18–19 pp., IBSN 978-1-4020-8690-8, https://doi.org/10.1007/978-1-4020-8690-8, 2009.
Li, J., Pósfai, M., Hobbs, P. V., and Buseck, P. R.: Individual aerosol particles from biomass burning in southern Africa: 2, Compositions and aging of inorganic particles: Composition and Aging of Inorganic Particles, J. Geophys. Res.-Atmos., 108, 8484–8496, https://doi.org/10.1029/2002JD002310, 2003.
Li, W., Sun, J., Xu, L., Shi, Z., Riemer, N., Sun, Y., Fu, P., Zhang, J., Lin, Y., Wang, X., Shao, L., Chen, J., Zhang, X., Wang, Z., and Wang, W.: A conceptual framework for mixing structures in individual aerosol particles: Individual Aerosol Mixing Structure, J. Geophys. Res.-Atmos., 121, 13784–13798, https://doi.org/10.1002/2016JD025252, 2016.
Liu, X., Zhang, Y., Huey, L. G., Yokelson, R. J., Wang, Y., Jimenez, J. L., Campuzano-Jost, P., Beyersdorf, A. J., Blake, D. R., Choi, Y., St. Clair, J. M., Crounse, J. D., Day, D. A., Diskin, G. S., Fried, A., Hall, S. R., Hanisco, T. F., King, L. E., Meinardi, S., Mikoviny, T., Palm, B. B., Peischl, J., Perring, A. E., Pollack, I. B., Ryerson, T. B., Sachse, G., Schwarz, J. P., Simpson, I. J., Tanner, D. J., Thornhill, K. L., Ullmann, K., Weber, R. J., Wennberg, P. O., Wisthaler, A., Wolfe, G. M., and Ziemba, L. D.: Agricultural fires in the southeastern U.S. during SEAC4RS: Emissions of trace gases and particles and evolution of ozone, reactive nitrogen, and organic aerosol: Agricultural Fires in the SE US, J. Geophys. Res.-Atmos., 121, 7383–7414, https://doi.org/10.1002/2016JD025040, 2016.
Maria, S. F., Lynn, R. M., Gilles, M. K., and Myneni, S. C. B.: Organic Aerosol Growth Mechanisms and Their Climate-Forcing Implications, Science, 306, 1921–1924, https://doi.org/10.1126/science.1103491, 2004.
Miyazaki, Y., Yamashita, Y., Kawana, K., Tachibana, E., Kagami, S., Mochida, M., Suzuki, K., and Nishioka, J.: Chemical transfer of dissolved organic matter from surface seawater to sea spray water-soluble organic aerosol in the marine atmosphere, Sci. Rep., 8, 14861, https://doi.org/10.1038/s41598-018-32864-7, 2018.
Moffet, R. C., Henn, T., Laskin, A., and Gilles, M. K.: Automated Chemical Analysis of Internally Mixed Aerosol Particles Using X-ray Spectromicroscopy at the Carbon K-Edge, Anal. Chem., 82, 7906–7914, https://doi.org/10.1021/ac1012909, 2010a (data available at: https://www.mathworks.com/matlabcentral/fileexchange/29085-stxm-spectromicroscopy-particle-analysis-routines, last access: 8 December 2021).
Moffet, R. C., Henn, T. R., Tivanski, A. V., Hopkins, R. J., Desyaterik, Y., Kilcoyne, A. L. D., Tyliszczak, T., Fast, J., Barnard, J., Shutthanandan, V., Cliff, S. S., Perry, K. D., Laskin, A., and Gilles, M. K.: Microscopic characterization of carbonaceous aerosol particle aging in the outflow from Mexico City, Atmos. Chem. Phys., 10, 961–976, https://doi.org/10.5194/acp-10-961-2010, 2010b.
Moffet, R. C., Tivanski, A. V., and Gilles, M. K. (Eds.): Scanning Transmission X-ray Microscopy: Applications in Atmospheric Aerosol Research, in: Fundamentals and Applications in Aerosol Spectroscopy, Taylor and Francis Books, New York, 438–481, https://doi.org/10.1201/b10417-21, 2010c.
Moffet, R. C., Furutani, H., Rödel, T. C., Henn, T. R., Sprau, P. O., Laskin, A., Uematsu, M., and Gilles, M. K.: Iron speciation and mixing in single aerosol particles from the Asian continental outflow: Aerosol Iron Speciation in Asian Outflow, J. Geophys. Res.-Atmos., 117, D07204, https://doi.org/10.1029/2011JD016746, 2012.
Moffet, R. C., Rödel, T. C., Kelly, S. T., Yu, X. Y., Carroll, G. T., Fast, J., Zaveri, R. A., Laskin, A., and Gilles, M. K.: Spectro-microscopic measurements of carbonaceous aerosol aging in Central California, Atmos. Chem. Phys., 13, 10445–10459, https://doi.org/10.5194/acp-13-10445-2013, 2013.
Moffet, R. C., O'Brien, R. E., Alpert, P. A., Kelly, S. T., Pham, D. Q., Gilles, M. K., Knopf, D. A., and Laskin, A.: Morphology and mixing of black carbon particles collected in central California during the CARES field study, Atmos. Chem. Phys., 16, 14515–14525, https://doi.org/10.5194/acp-16-14515-2016, 2016.
Mozurkewich, M.: Aerosol Growth and the Condensation Coefficient for Water: A Review, Aerosol Sci. Technol., 5, 223–236, https://doi.org/10.1080/02786828608959089, 1986.
Mungall, E. L., Abbatt, J. P. D., Wentzell, J. J. B., Lee, A. K. Y., Thomas, J. L., Blais, M., Gosselin, M., Miller, L. A., Papakyriakou, T., Willis, M. D., and Liggio, J.: Microlayer source of oxygenated volatile organic compounds in the summertime marine Arctic boundary layer, P. Natl. Acad. Sci. USA, 114, 6203–6208, https://doi.org/10.1073/pnas.1620571114, 2017.
Murphy, D. M. and Thomson, D. S.: Chemical composition of single aerosol particles at Idaho Hill: Negative ion measurements, J. Geophys. Res.-Atmos., 102, 6353–6368, https://doi.org/10.1029/96JD00859, 1997.
National Research Council (U.S.) (Ed.): The Congestion Mitigation and Air Quality Improvement Program: assessing 10 years of experience, Transportation Research Board, National Research Council: National Academy Press, Washington, D.C, 175–176 pp., 2002.
Nováková, E., Mitrea, G., Peth, C., Thieme, J., Mann, K., and Salditt, T.: Solid supported multicomponent lipid membranes studied by x-ray spectromicroscopy, Biointerphases, 3, FB44–FB54, https://doi.org/10.1116/1.2976445, 2008.
O'Dowd, C. D., Facchini, M. C., Cavalli, F., Ceburnis, D., Mircea, M., Decesari, S., Fuzzi, S., Yoon, Y. J., and Putaud, J.-P.: Biogenically driven organic contribution to marine aerosol, Nature, 431, 676–680, https://doi.org/10.1038/nature02959, 2004.
Ovadnevaite, J., Zuend, A., Laaksonen, A., Sanchez, K. J., Roberts, G., Ceburnis, D., Decesari, S., Rinaldi, M., Hodas, N., Facchini, M. C., Seinfeld, J. H., and O' Dowd, C.: Surface tension prevails over solute effect in organic-influenced cloud droplet activation, Nature, 546, 637–641, https://doi.org/10.1038/nature22806, 2017.
Owen, R. C., Cooper, O. R., Stohl, A., and Honrath, R. E.: An analysis of the mechanisms of North American pollutant transport to the central North Atlantic lower free troposphere, J. Geophys. Res.-Atmos., 111, D23S58, https://doi.org/10.1029/2006JD007062, 2006.
Paredes-Miranda, G., Arnott, W. P., Moosmüller, H., Green, M. C., and Gyawali, M.: Black Carbon Aerosol Concentration in Five Cities and Its Scaling with City Population, B. Am. Meteorol. Soc., 94, 41–50, https://doi.org/10.1175/BAMS-D-11-00225.1, 2013.
Park, R. J., Jacob, D. J., and Logan, J. A.: Fire and biofuel contributions to annual mean aerosol mass concentrations in the United States, Atmos. Environ., 41, 7389–7400, https://doi.org/10.1016/j.atmosenv.2007.05.061, 2007.
Petters, M. D. and Kreidenweis, S. M.: A single parameter representation of hygroscopic growth and cloud condensation nucleus activity, Atmos. Chem. Phys., 7, 1961–1971, https://doi.org/10.5194/acp-7-1961-2007, 2007.
Pham, D. Q., O'Brien, R., Fraund, M., Bonanno, D., Laskina, O., Beall, C., Moore, K. A., Forestieri, S., Wang, X., Lee, C., Sultana, C., Grassian, V., Cappa, C. D., Prather, K. A., and Moffet, R. C.: Biological Impacts on Carbon Speciation and Morphology of Sea Spray Aerosol, ACS Earth Space Chem., 1, 551–561, https://doi.org/10.1021/acsearthspacechem.7b00069, 2017.
Pincus, R. and Baker, M. B.: Effect of precipitation on the albedo susceptibility of clouds in the marine boundary layer, Nature, 372, 250–252, https://doi.org/10.1038/372250a0, 1994.
Prather, K. A., Hatch, C. D., and Grassian, V. H.: Analysis of Atmospheric Aerosols, Annu. Rev. Anal. Chem., 1, 485–514, https://doi.org/10.1146/annurev.anchem.1.031207.113030, 2008.
Prather, K. A., Bertram, T. H., Grassian, V. H., Deane, G. B., Stokes, M. D., DeMott, P. J., Aluwihare, L. I., Palenik, B. P., Azam, F., Seinfeld, J. H., Moffet, R. C., Molina, M. J., Cappa, C. D., Geiger, F. M., Roberts, G. C., Russell, L. M., Ault, A. P., Baltrusaitis, J., Collins, D. B., Corrigan, C. E., Cuadra-Rodriguez, L. A., Ebben, C. J., Forestieri, S. D., Guasco, T. L., Hersey, S. P., Kim, M. J., Lambert, W. F., Modini, R. L., Mui, W., Pedler, B. E., Ruppel, M. J., Ryder, O. S., Schoepp, N. G., Sullivan, R. C., and Zhao, D.: Bringing the ocean into the laboratory to probe the chemical complexity of sea spray aerosol, P. Natl. Acad. Sci. USA, 110, 7550–7555, https://doi.org/10.1073/pnas.1300262110, 2013.
Pratt, K. A. and Prather, K. A.: Aircraft measurements of vertical profiles of aerosol mixing states, J. Geophys. Res., 115, D11305, https://doi.org/10.1029/2009JD013150, 2010.
Raes, F.: Entrainment of free tropospheric aerosols as a regulating mechanism for cloud condensation nuclei in the remote marine boundary layer, J. Geophys. Res., 100, 2893, https://doi.org/10.1029/94JD02832, 1995.
Ramnarine, E., Kodros, J. K., Hodshire, A. L., Lonsdale, C. R., Alvarado, M. J., and Pierce, J. R.: Effects of near-source coagulation of biomass burning aerosols on global predictions of aerosol size distributions and implications for aerosol radiative effects, Atmos. Chem. Phys., 19, 6561–6577, https://doi.org/10.5194/acp-19-6561-2019, 2019.
Raveh-Rubin, S.: Dry Intrusions: Lagrangian Climatology and Dynamical Impact on the Planetary Boundary Layer, J. Climate, 30, 6661–6682, https://doi.org/10.1175/JCLI-D-16-0782.1, 2017.
Raveh-Rubin, S. and Catto, J. L.: Climatology and dynamics of the link between dry intrusions and cold fronts during winter, Part II: Front-centred perspective, Clim. Dynam., 53, 1893–1909, https://doi.org/10.1007/s00382-019-04793-2, 2019.
Rebotier, T. P. and Prather, K. A.: Aerosol time-of-flight mass spectrometry data analysis: A benchmark of clustering algorithms, Anal. Chim. Acta, 585, 38–54, https://doi.org/10.1016/j.aca.2006.12.009, 2007.
Reddington, C. L., Carslaw, K. S., Spracklen, D. V., Frontoso, M. G., Collins, L., Merikanto, J., Minikin, A., Hamburger, T., Coe, H., Kulmala, M., Aalto, P., Flentje, H., Plass-Dülmer, C., Birmili, W., Wiedensohler, A., Wehner, B., Tuch, T., Sonntag, A., O'Dowd, C. D., Jennings, S. G., Dupuy, R., Baltensperger, U., Weingartner, E., Hansson, H.-C., Tunved, P., Laj, P., Sellegri, K., Boulon, J., Putaud, J.-P., Gruening, C., Swietlicki, E., Roldin, P., Henzing, J. S., Moerman, M., Mihalopoulos, N., Kouvarakis, G., Ždímal, V., Zíková, N., Marinoni, A., Bonasoni, P., and Duchi, R.: Primary versus secondary contributions to particle number concentrations in the European boundary layer, Atmos. Chem. Phys., 11, 12007–12036, https://doi.org/10.5194/acp-11-12007-2011, 2011.
Reff, A., Bhave, P. V., Simon, H., Pace, T. G., Pouliot, G. A., Mobley, J. D., and Houyoux, M.: Emissions Inventory of PM 2.5 Trace Elements across the United States, Environ. Sci. Technol., 43, 5790–5796, https://doi.org/10.1021/es802930x, 2009.
Ren, J., Zhang, F., Wang, Y., Collins, D., Fan, X., Jin, X., Xu, W., Sun, Y., Cribb, M., and Li, Z.: Using different assumptions of aerosol mixing state and chemical composition to predict CCN concentrations based on field measurements in urban Beijing, Atmos. Chem. Phys., 18, 6907–6921, https://doi.org/10.5194/acp-18-6907-2018, 2018.
Riemer, N., Ault, A. P., West, M., Craig, R. L., and Curtis, J. H.: Aerosol Mixing State: Measurements, Modeling, and Impacts, Rev. Geophys., 57, 187–249, https://doi.org/10.1029/2018RG000615, 2019.
Roberts, G. C., Day, D. A., Russell, L. M., Dunlea, E. J., Jimenez, J. L., Tomlinson, J. M., Collins, D. R., Shinozuka, Y., and Clarke, A. D.: Characterization of particle cloud droplet activity and composition in the free troposphere and the boundary layer during INTEX-B, Atmos. Chem. Phys., 10, 6627–6644, https://doi.org/10.5194/acp-10-6627-2010, 2010.
Rolph, G., Stein, A., and Stunder, B.: Real-time Environmental Applications and Display sYstem: READY, Environ. Model. Softw., 95, 210–228, https://doi.org/10.1016/j.envsoft.2017.06.025, 2017.
Rosenfeld, D., Zhu, Y., Wang, M., Zheng, Y., Goren, T., and Yu, S.: Aerosol-driven droplet concentrations dominate coverage and water of oceanic low-level clouds, Science, 363, eaav0566, https://doi.org/10.1126/science.aav0566, 2019.
Ruehl, C. R., Davies, J. F., and Wilson, K. R.: An interfacial mechanism for cloud droplet formation on organic aerosols, Science, 351, 1447–1450, https://doi.org/10.1126/science.aad4889, 2016.
Sanchez, K. J., Chen, C.-L., Russell, L. M., Betha, R., Liu, J., Price, D. J., Massoli, P., Ziemba, L. D., Crosbie, E. C., Moore, R. H., Müller, M., Schiller, S. A., Wisthaler, A., Lee, A. K. Y., Quinn, P. K., Bates, T. S., Porter, J., Bell, T. G., Saltzman, E. S., Vaillancourt, R. D., and Behrenfeld, M. J.: Substantial Seasonal Contribution of Observed Biogenic Sulfate Particles to Cloud Condensation Nuclei, Sci. Rep., 8, 3235, https://doi.org/10.1038/s41598-018-21590-9, 2018.
Schill, S. R., Collins, D. B., Lee, C., Morris, H. S., Novak, G. A., Prather, K. A., Quinn, P. K., Sultana, C. M., Tivanski, A. V., Zimmermann, K., Cappa, C. D., and Bertram, T. H.: The Impact of Aerosol Particle Mixing State on the Hygroscopicity of Sea Spray Aerosol, ACS Cent. Sci., 1, 132–141, https://doi.org/10.1021/acscentsci.5b00174, 2015.
Schmale, J., Henning, S., Henzing, B., Keskinen, H., Sellegri, K., Ovadnevaite, J., Bougiatioti, A., Kalivitis, N., Stavroulas, I., Jefferson, A., Park, M., Schlag, P., Kristensson, A., Iwamoto, Y., Pringle, K., Reddington, C., Aalto, P., Äijälä, M., Baltensperger, U., Bialek, J., Birmili, W., Bukowiecki, N., Ehn, M., Fjæraa, A. M., Fiebig, M., Frank, G., Fröhlich, R., Frumau, A., Furuya, M., Hammer, E., Heikkinen, L., Herrmann, E., Holzinger, R., Hyono, H., Kanakidou, M., Kiendler-Scharr, A., Kinouchi, K., Kos, G., Kulmala, M., Mihalopoulos, N., Motos, G., Nenes, A., O'Dowd, C., Paramonov, M., Petäjä, T., Picard, D., Poulain, L., Prévôt, A. S. H., Slowik, J., Sonntag, A., Swietlicki, E., Svenningsson, B., Tsurumaru, H., Wiedensohler, A., Wittbom, C., Ogren, J. A., Matsuki, A., Yum, S. S., Myhre, C. L., Carslaw, K., Stratmann, F., and Gysel, M.: Collocated observations of cloud condensation nuclei, particle size distributions, and chemical composition, Sci. Data, 4, 170003, https://doi.org/10.1038/sdata.2017.3, 2017.
Schmale, J., Henning, S., Decesari, S., Henzing, B., Keskinen, H., Sellegri, K., Ovadnevaite, J., Pöhlker, M. L., Brito, J., Bougiatioti, A., Kristensson, A., Kalivitis, N., Stavroulas, I., Carbone, S., Jefferson, A., Park, M., Schlag, P., Iwamoto, Y., Aalto, P., Äijälä, M., Bukowiecki, N., Ehn, M., Frank, G., Fröhlich, R., Frumau, A., Herrmann, E., Herrmann, H., Holzinger, R., Kos, G., Kulmala, M., Mihalopoulos, N., Nenes, A., O'Dowd, C., Petäjä, T., Picard, D., Pöhlker, C., Pöschl, U., Poulain, L., Prévôt, A. S. H., Swietlicki, E., Andreae, M. O., Artaxo, P., Wiedensohler, A., Ogren, J., Matsuki, A., Yum, S. S., Stratmann, F., Baltensperger, U., and Gysel, M.: Long-term cloud condensation nuclei number concentration, particle number size distribution and chemical composition measurements at regionally representative observatories, Atmos. Chem. Phys., 18, 2853–2881, https://doi.org/10.5194/acp-18-2853-2018, 2018.
Souri, A. H., Choi, Y., Jeon, W., Kochanski, A. K., Diao, L., Mandel, J., Bhave, P. V., and Pan, S.: Quantifying the Impact of Biomass Burning Emissions on Major Inorganic Aerosols and Their Precursors in the U.S.: Burning Impact on Inorganic Aerosols, J. Geophys. Res.-Atmos., 122, 12020–12041, https://doi.org/10.1002/2017JD026788, 2017.
Sprenger, M. and Wernli, H.: The LAGRANTO Lagrangian analysis tool – version 2.0, Geosci. Model Dev., 8, 2569–2586, https://doi.org/10.5194/gmd-8-2569-2015, 2015.
Stein, A. F., Draxler, R. R., Rolph, G. D., Stunder, B. J. B., Cohen, M. D., and Ngan, F.: NOAA's HYSPLIT Atmospheric Transport and Dispersion Modeling System, B. Am. Meteorol. Soc., 96, 2059–2077, https://doi.org/10.1175/BAMS-D-14-00110.1, 2015.
Stull, R. B.: Mean Boundary Layer Characteristics, in: An Introduction to Boundary Layer Meteorology, Springer Netherlands, Dordrecht, 1–27, https://doi.org/10.1007/978-94-009-3027-8_1, 1988.
Tomlin, J. M., Jankowski, K. A., Rivera-Adorno, F. A., Fraund, M., China, S., Stirm, B. H., Kaeser, R., Eakins, G. S., Moffet, R. C., Shepson, P. B., and Laskin, A.: Chemical Imaging of Fine Mode Atmospheric Particles Collected from a Research Aircraft over Agricultural Fields, ACS Earth Space Chem., 4, 2171–2184, https://doi.org/10.1021/acsearthspacechem.0c00172, 2020.
Tomlin, J. M., Jankowski, K., Veghte, D., China, S., Wang, P., Fraund, M., Weis, J., Zheng, G., Wang, Y., Rivera-Adorno, F., Raveh-Rubin, S., Knopf, D., Wang, J., Gilles, M., Moffet, R., and Laskin, A.: Impact of dry intrusion events on the composition and mixing state of particles during the winter Aerosol and Cloud Experiment in the Eastern North Atlantic (ACE-ENA), Purdue University Research Repository [data set], https://doi.org/10.4231/6CT5-3R55 2021.
Toner, S. M., Sodeman, D. A., and Prather, K. A.: Single Particle Characterization of Ultrafine and Accumulation Mode Particles from Heavy Duty Diesel Vehicles Using Aerosol Time-of-Flight Mass Spectrometry, Environ. Sci. Technol., 40, 3912–3921, https://doi.org/10.1021/es051455x, 2006.
Vakkari, V., Beukes, J. P., Dal Maso, M., Aurela, M., Josipovic, M., and van Zyl, P. G.: Major secondary aerosol formation in southern African open biomass burning plumes, Nat. Geosci., 11, 580–583, https://doi.org/10.1038/s41561-018-0170-0, 2018.
Val Martín, M., Honrath, R. E., Owen, R. C., Pfister, G., Fialho, P., and Barata, F.: Significant enhancements of nitrogen oxides, black carbon, and ozone in the North Atlantic lower free troposphere resulting from North American boreal wildfires, J. Geophys. Res.-Atmos., 111, D23S60, https://doi.org/10.1029/2006JD007530, 2006.
VanReken, T. M.: Toward aerosol/cloud condensation nuclei (CCN) closure during CRYSTAL-FACE, J. Geophys. Res., 108, 4633, https://doi.org/10.1029/2003JD003582, 2003.
Wang, B., Lambe, A. T., Massoli, P., Onasch, T. B., Davidovits, P., Worsnop, D. R., and Knopf, D. A.: The deposition ice nucleation and immersion freezing potential of amorphous secondary organic aerosol: Pathways for ice and mixed-phase cloud formation, J. Geophys. Res.-Atmos., 117, D16209, https://doi.org/10.1029/2012JD018063, 2012.
Wang, B., Gilles, M. K., and Laskin, A.: Reactivity of Liquid and Semisolid Secondary Organic Carbon with Chloride and Nitrate in Atmospheric Aerosols, J. Phys. Chem. A, 119, 4498–4508, https://doi.org/10.1021/jp510336q, 2015.
Wang, J., Lee, Y.-N., Daum, P. H., Jayne, J., and Alexander, M. L.: Effects of aerosol organics on cloud condensation nucleus (CCN) concentration and first indirect aerosol effect, Atmos. Chem. Phys., 8, 6325–6339, https://doi.org/10.5194/acp-8-6325-2008, 2008.
Wang, J., Cubison, M. J., Aiken, A. C., Jimenez, J. L., and Collins, D. R.: The importance of aerosol mixing state and size-resolved composition on CCN concentration and the variation of the importance with atmospheric aging of aerosols, Atmos. Chem. Phys., 10, 7267–7283, https://doi.org/10.5194/acp-10-7267-2010, 2010.
Wang, J., Wood, R., Jensen, M. P., Chiu, J. C., Liu, Y., Lamer, K., Desai, N., Giangrande, S. E., Knopf, D. A., Kollias, P., Laskin, A., Liu, X., Lu, C., Mechem, D., Mei, F., Starzec, M., Tomlinson, J., Wang, Y., Yum, S. S., Zheng, G., Aiken, A. C., Azevedo, E. B., Blanchard, Y., China, S., Dong, X., Gallo, F., Gao, S., Ghate, V. P., Glienke, S., Goldberger, L., Hardin, J. C., Kuang, C., Luke, E. P., Matthews, A. A., Miller, M. A., Moffet, R., Pekour, M., Schmid, B., Sedlacek, A. J., Shaw, R. A., Shilling, J. E., Sullivan, A., Suski, K., Veghte, D. P., Weber, R., Wyant, M., Yeom, J., Zawadowicz, M., and Zhang, Z.: Aerosol and Cloud Experiments in the Eastern North Atlantic (ACE-ENA), B. Am. Meteorol. Soc., 1–51, https://doi.org/10.1175/BAMS-D-19-0220.1, in press, 2021.
Wang, X., Sultana, C. M., Trueblood, J., Hill, T. C. J., Malfatti, F., Lee, C., Laskina, O., Moore, K. A., Beall, C. M., McCluskey, C. S., Cornwell, G. C., Zhou, Y., Cox, J. L., Pendergraft, M. A., Santander, M. V., Bertram, T. H., Cappa, C. D., Azam, F., DeMott, P. J., Grassian, V. H., and Prather, K. A.: Microbial Control of Sea Spray Aerosol Composition: A Tale of Two Blooms, ACS Cent. Sci., 1, 124–131, https://doi.org/10.1021/acscentsci.5b00148, 2015.
Wang, Y., Pinterich, T., and Wang, J.: Rapid measurement of sub-micrometer aerosol size distribution using a fast integrated mobility spectrometer, J. Aerosol Sci., 121, 12–20, https://doi.org/10.1016/j.jaerosci.2018.03.006, 2018.
Wang, Y., Zheng, X., Dong, X., Xi, B., Wu, P., Logan, T., and Yung, Y. L.: Impacts of long-range transport of aerosols on marine-boundary-layer clouds in the eastern North Atlantic, Atmos. Chem. Phys., 20, 14741–14755, https://doi.org/10.5194/acp-20-14741-2020, 2020.
Wang, Y., Zheng, G., Jensen, M. P., Knopf, D. A., Laskin, A., Matthews, A. A., Mechem, D., Mei, F., Moffet, R., Sedlacek, A. J., Shilling, J. E., Springston, S., Sullivan, A., Tomlinson, J., Veghte, D., Weber, R., Wood, R., Zawadowicz, M. A., and Wang, J.: Vertical profiles of trace gas and aerosol properties over the eastern North Atlantic: variations with season and synoptic condition, Atmos. Chem. Phys., 21, 11079–11098, https://doi.org/10.5194/acp-21-11079-2021, 2021.
Wernli, H.: A Lagrangian-based analysis of extratropical cyclones. II: A detailed case-study, Q. J. Roy. Meteor. Soc., 123, 1677–1706, https://doi.org/10.1002/qj.49712354211, 1997.
Willis, M. D., Köllner, F., Burkart, J., Bozem, H., Thomas, J. L., Schneider, J., Aliabadi, A. A., Hoor, P. M., Schulz, H., Herber, A. B., Leaitch, W. R., and Abbatt, J. P. D.: Evidence for marine biogenic influence on summertime Arctic aerosol, Geophys. Res. Lett., 44, 6460–6470, https://doi.org/10.1002/2017GL073359, 2017.
Wood, R.: Stratocumulus Clouds, Mon. Weather Rev., 140, 2373–2423, https://doi.org/10.1175/MWR-D-11-00121.1, 2012.
Wood, R., Wyant, M., Bretherton, C. S., Rémillard, J., Kollias, P., Fletcher, J., Stemmler, J., de Szoeke, S., Yuter, S., Miller, M., Mechem, D., Tselioudis, G., Chiu, J. C., Mann, J. A. L., O'Connor, E. J., Hogan, R. J., Dong, X., Miller, M., Ghate, V., Jefferson, A., Min, Q., Minnis, P., Palikonda, R., Albrecht, B., Luke, E., Hannay, C., and Lin, Y.: Clouds, Aerosols, and Precipitation in the Marine Boundary Layer: An Arm Mobile Facility Deployment, B. Am. Meteorol. Soc., 96, 419–440, https://doi.org/10.1175/BAMS-D-13-00180.1, 2015.
Worsnop, D. R., Morris, J. W., Shi, Q., Davidovits, P., and Kolb, C. E.: A chemical kinetic model for reactive transformations of aerosol particles: Reactive transformation of aerosol particles, Geophys. Res. Lett., 29, 1996, https://doi.org/10.1029/2002GL015542, 2002.
Yamasoe, M. A., Artaxo, P., Miguel, A. H., and Allen, A. G.: Chemical composition of aerosol particles from direct emissions of vegetation fires in the Amazon Basin: water-soluble species and trace elements, Atmos. Environ., 34, 1641–1653, https://doi.org/10.1016/S1352-2310(99)00329-5, 2000.
Yu, P., Toon, O. B., Bardeen, C. G., Zhu, Y., Rosenlof, K. H., Portmann, R. W., Thornberry, T. D., Gao, R.-S., Davis, S. M., Wolf, E. T., de Gouw, J., Peterson, D. A., Fromm, M. D., and Robock, A.: Black carbon lofts wildfire smoke high into the stratosphere to form a persistent plume, Science, 365, 587–590, https://doi.org/10.1126/science.aax1748, 2019.
Zawadowicz, M. A., Suski, K., Liu, J., Pekour, M., Fast, J., Mei, F., Sedlacek, A. J., Springston, S., Wang, Y., Zaveri, R. A., Wood, R., Wang, J., and Shilling, J. E.: Aircraft measurements of aerosol and trace gas chemistry in the eastern North Atlantic, Atmos. Chem. Phys., 21, 7983–8002, https://doi.org/10.5194/acp-21-7983-2021, 2021.
Zehr, J. P. and Ward, B. B.: Nitrogen Cycling in the Ocean: New Perspectives on Processes and Paradigms, Appl. Environ. Microbiol., 68, 1015–1024, https://doi.org/10.1128/AEM.68.3.1015-1024.2002, 2002.
Zheng, G., Wang, Y., Aiken, A. C., Gallo, F., Jensen, M. P., Kollias, P., Kuang, C., Luke, E., Springston, S., Uin, J., Wood, R., and Wang, J.: Marine boundary layer aerosol in the eastern North Atlantic: seasonal variations and key controlling processes, Atmos. Chem. Phys., 18, 17615–17635, https://doi.org/10.5194/acp-18-17615-2018, 2018.
Zheng, G., Kuang, C., Uin, J., Watson, T., and Wang, J.: Large contribution of organics to condensational growth and formation of cloud condensation nuclei (CCN) in the remote marine boundary layer, Atmos. Chem. Phys., 20, 12515–12525, https://doi.org/10.5194/acp-20-12515-2020, 2020a.
Zheng, G., Sedlacek, A. J., Aiken, A. C., Feng, Y., Watson, T. B., Raveh-Rubin, S., Uin, J., Lewis, E. R., and Wang, J.: Long-range transported North American wildfire aerosols observed in marine boundary layer of eastern North Atlantic, Environ. Int., 139, 105680, https://doi.org/10.1016/j.envint.2020.105680, 2020b.
Zheng, G., Wang, Y., Wood, R., Jensen, M. P., Kuang, C., McCoy, I. L., Matthews, A., Mei, F., Tomlinson, J. M., Shilling, J. E., Zawadowicz, M. A., Crosbie, E., Moore, R., Ziemba, L., Andreae, M. O., and Wang, J.: New particle formation in the remote marine boundary layer, Nat. Commun., 12, 527, https://doi.org/10.1038/s41467-020-20773-1, 2021.
Zhou, S., Collier, S., Jaffe, D. A., and Zhang, Q.: Free tropospheric aerosols at the Mt. Bachelor Observatory: more oxidized and higher sulfate content compared to boundary layer aerosols, Atmos. Chem. Phys., 19, 1571–1585, https://doi.org/10.5194/acp-19-1571-2019, 2019.
Zhu, L., Val Martin, M., Gatti, L. V., Kahn, R., Hecobian, A., and Fischer, E. V.: Development and implementation of a new biomass burning emissions injection height scheme (BBEIH v1.0) for the GEOS-Chem model (v9-01-01), Geosci. Model Dev., 11, 4103–4116, https://doi.org/10.5194/gmd-11-4103-2018, 2018.
Zieger, P., Väisänen, O., Corbin, J. C., Partridge, D. G., Bastelberger, S., Mousavi-Fard, M., Rosati, B., Gysel, M., Krieger, U. K., Leck, C., Nenes, A., Riipinen, I., Virtanen, A., and Salter, M. E.: Revising the hygroscopicity of inorganic sea salt particles, Nat. Commun., 8, 15883, https://doi.org/10.1038/ncomms15883, 2017.