the Creative Commons Attribution 4.0 License.
the Creative Commons Attribution 4.0 License.
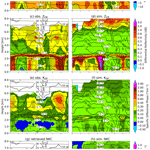
Overview: Fusion of radar polarimetry and numerical atmospheric modelling towards an improved understanding of cloud and precipitation processes
Silke Trömel
Clemens Simmer
Ulrich Blahak
Armin Blanke
Sabine Doktorowski
Florian Ewald
Michael Frech
Mathias Gergely
Martin Hagen
Tijana Janjic
Heike Kalesse-Los
Stefan Kneifel
Christoph Knote
Jana Mendrok
Manuel Moser
Gregor Köcher
Kai Mühlbauer
Alexander Myagkov
Velibor Pejcic
Patric Seifert
Prabhakar Shrestha
Audrey Teisseire
Leonie von Terzi
Eleni Tetoni
Teresa Vogl
Christiane Voigt
Yuefei Zeng
Tobias Zinner
Johannes Quaas
Cloud and precipitation processes are still a main source of uncertainties in numerical weather prediction and climate change projections. The Priority Programme “Polarimetric Radar Observations meet Atmospheric Modelling (PROM)”, funded by the German Research Foundation (Deutsche Forschungsgemeinschaft, DFG), is guided by the hypothesis that many uncertainties relate to the lack of observations suitable to challenge the representation of cloud and precipitation processes in atmospheric models. Such observations can, however, at present be provided by the recently installed dual-polarization C-band weather radar network of the German national meteorological service in synergy with cloud radars and other instruments at German supersites and similar national networks increasingly available worldwide. While polarimetric radars potentially provide valuable in-cloud information on hydrometeor type, quantity, and microphysical cloud and precipitation processes, and atmospheric models employ increasingly complex microphysical modules, considerable knowledge gaps still exist in the interpretation of the observations and in the optimal microphysics model process formulations. PROM is a coordinated interdisciplinary effort to increase the use of polarimetric radar observations in data assimilation, which requires a thorough evaluation and improvement of parameterizations of moist processes in atmospheric models. As an overview article of the inter-journal special issue “Fusion of radar polarimetry and numerical atmospheric modelling towards an improved understanding of cloud and precipitation processes”, this article outlines the knowledge achieved in PROM during the past 2 years and gives perspectives for the next 4 years.
Among the main sources of uncertainty in the models used in numerical weather prediction (NWP) and climate change projections are the parameterizations of cloud and precipitation processes (Bauer et al., 2015). A major part of these uncertainties can be attributed to missing observations suitable to challenge the representation of cloud and precipitation processes employed in atmospheric models. A wealth of new information on precipitation microphysics and generating processes can be gained from observations from polarimetric weather radars and their synergistic analysis at different frequencies. The dual-polarization upgrade of the United States National Weather Service (NWS) S-band Weather Surveillance Radar 1988 Doppler (WSR-88D) network was completed in 2013. Germany finished upgrading its C-band network to polarimetry in 2015 in parallel with other European countries. The synergistic exploitation of polarimetric precipitation radars together with measurements from cloud radars and other instrumentation available at supersites and research institutions enables a thorough evaluation and potential improvement of current microphysical parameterizations based on detailed multi-frequency remote-sensing observations for the first time. Data assimilation merges observations and models for state estimation as a prerequisite for prediction and can be seen as a smart interpolation between observations while exploiting the physical consistency of atmospheric models as mathematical constraint.
Considerable knowledge gaps still exist, however, in both radar polarimetry and atmospheric models, which still impede the full exploitation of the triangle between radar polarimetry, atmospheric models, and data assimilation and call for a coordinated interdisciplinary effort. The German Research Foundation (Deutsche Forschungsgemeinschaft, DFG) responded to this call and established the Priority Programme “Polarimetric Radar Observations meet Atmospheric Modelling (PROM)”; its first 3-year funding period began in 2019, which will be followed by a second funding period starting in 2022. PROM exploits the synergy of polarimetric radar observations and state-of-the-art atmospheric models to better understand moist processes in the atmosphere, and to improve their representation in climate and weather prediction models. The overarching goal is to extend our scientific understanding at the verges of the three disciplines, radar polarimetry – atmospheric models – data assimilation, for better predictions of precipitating cloud systems. To approach this goal the initiators of PROM at the Universities of Bonn and Leipzig in Germany identified the following five objectives (see also Trömel et al., 2018):
-
exploitation of radar polarimetry for quantitative process detection in precipitating clouds and for model evaluation including a quantitative analysis of polarimetric fingerprints and microphysical retrievals;
-
improvement of cloud and precipitation schemes in atmospheric models based on process fingerprints detectable in polarimetric observations;
-
monitoring of the energy budget evolution due to phase changes in the cloudy, precipitating atmosphere for a better understanding of its dynamics;
-
analysis of precipitation system by assimilation of polarimetric radar observations into atmospheric models for weather forecasting; and
-
radar-based detection of the initiation of convection for the improvement of thunderstorm prediction.
In the first funding period, each of the 14 projects (see https://www2.meteo.uni-bonn.de/spp2115, last access: 25 October 2021) distributed over Germany contributes to at least one of these objectives. In most projects, a radar meteorologist works together with a modeller in order to successfully combine expert knowledge from both research fields. This overview article of the ACP–AMT–GMD inter-journal special issue entitled “Fusion of radar polarimetry and numerical atmospheric modelling towards an improved understanding of cloud and precipitation processes” outlines methodologies developed and results achieved from a selection of the projects during the past 2 years and provides overall perspectives for the next 4 years. The paper is organized as follows: Sect. 2 explains prevailing challenges in the representation of clouds in atmospheric models, while Sect. 3 provides methodologies to extend our insight into the microphysics of clouds and precipitation by exploiting radar polarimetry. Section 4 addresses the fusion of numerical modelling and radar polarimetry via model evaluation in radar observation space either using observation operators or using microphysical retrievals. First conclusions for improved model parameterizations and for a better representation of model uncertainty in radar data assimilation are drawn. Section 5 provides a summary and perspectives for the following years.
The representation of cloud and precipitation processes in atmospheric models is a central challenge for NWP and climate projections (e.g. Bauer et al., 2015; Forster et al., 2021), which also impacts offline hydrological models by modulating the distribution of incoming solar radiation and precipitation and affecting the simulated hydrological processes such as evapotranspiration, runoff, and groundwater depths (e.g. Shrestha, 2021). While the primitive equations provide a solid theoretical basis for atmospheric model dynamics, the key diabatic processes that drive energetics and thus circulation are poorly resolved. Important diabatic processes are linked to cloud and precipitation microphysics acting at scales of micrometres and turbulent processes ranging from several to hundreds of metres. While significant progress has been achieved by high-resolution modelling at the coarser end of this range (e.g. Heinze et al., 2017; Stevens et al., 2020), the intricate and complex microphysical processes still require parameterizations in any dynamic atmospheric model down to and including the scale of direct numerical simulations (e.g. Mellado et al., 2009).
A key uncertainty in weather prediction and climate modelling results from the still-rudimentary representation of moist processes and from the diabatic heating–cooling the models induce due to latent heat and their interaction with radiation. The generation and interpretation of past and future climate states additionally has to consider changes in microphysical processes due to anthropogenic aerosol acting for example as cloud condensation nuclei and ice-nucleating particles. For short-term weather prediction, the location and evolution of convective events with lifetimes of hours or less are particularly challenging, while relatively slowly moving and frontal systems with lifetimes of days show reasonable predictability (Alifieri et al., 2012).
Atmospheric modelling in Germany has recently seen substantial advances in terms of both cloud-resolving simulations in NWP mode and the implementation of ice and mixed-phase precipitation formation processes. Traditionally, different model systems were used for NWP and climate modelling, which were also both heavily used in academic research. The modelling system for long-term climate integrations is the ECHAM model (Stevens et al., 2013). Since it was created by modifying global forecast models developed by ECMWF (European Centre for Medium-Range Weather Forecasts), its name is a combination of ECMWF and Hamburg, the place of development of its parameterization package. The COSMO model, however, was operated at horizontal resolutions down to 2.8 km and used for NWP and reanalysis studies. Both model families are currently being replaced by the ICOsahedral Nonhydrostatic (ICON) modelling framework (Zängl et al., 2015) jointly developed by the Max Planck Institute for Meteorology and the German national meteorological service (Deutscher Wetterdienst, DWD). Its climate version (the ICON general circulation model, ICON GCM) inherited its physics package from the ECHAM model, and the NWP version incorporated the one from the COSMO model. A third version largely based on the COSMO physics package was developed for higher resolutions (Dipankar et al., 2015) and employs a large-eddy turbulence scheme (ICON-LEM). The latter is able to operate on large domains (Heinze et al., 2017; Stevens et al., 2020) and includes aerosol–cloud interactions (Costa-Surós et al., 2020). In PROM primarily the three ICON model variants (ICON-LEM, ICON-NWP, and ICON-A/GCM) are used.
In most atmospheric models, cloud and precipitation microphysical processes are represented by bulk microphysical schemes that distinguish between different hydrometeor classes and include their specific masses as prognostic variables while their size distributions are parameterized (the ICON model considered here uses the scheme by Seifert and Beheng, 2006). Computationally much more demanding are so-called spectral-bin microphysics schemes (Khain et al., 2015), which evolve cloud and precipitation particle size distributions discretized into size-interval bins. An example is the Hebrew University Cloud Model (HUCM) created by Khain et al. (2005) that treats both liquid and much more intricate (since ice may occur in various shapes and densities) ice crystal distributions. The model is employed by some of the PROM projects in addition to the liquid-only bin-microphysics model by Simmel et al. (2015) extended to the ice phase based on the scheme by Hashino and Tripoli (2007). For the simulation of the evolution of specific air volumes, a Lagrangian particle model (McSnow; Brdar and Seifert, 2018) is used in PROM that models ice and mixed-phase microphysical processes such as depositional growth, aggregation, riming, secondary ice generation, and melting closer to the real processes than bulk formulations. Microphysical processes including radiation–particle interactions obviously depend on particle shape; thus, the evolution of shapes in particle models – and their signatures in radar observations – is instrumental for a full understanding and adequate representation of the microphysical processes in models. Advanced microphysical parameterizations such as spectral-bin or Lagrangian particle schemes are relevant for cloud-resolving models and exploited in PROM for the development and improvement of bulk parameterizations. Scientific questions about global climate require long model integrations and thus coarse spatial resolutions due to computing time constraints. At these resolutions (usually of the order of 100×100 km2 in the horizontal), fractional cloudiness needs to be considered when the grid-box mean relative humidity is below 100 %, which requires parameterizations of subgrid-scale variability in relative humidity. Here, PROM builds on assumptions employed in the global ICON model (ICON GCM) to predict fractional cloudiness (e.g. Quaas, 2012).
DWD operates 17 state-of-the-art polarimetric Doppler C-band weather radars which provide a 3-D sampling of precipitating particles above Germany every 5 min. Together with their Doppler information, radars are the backbone for precipitation and nowcasting products for all meteorological services. Although precipitation monitoring is still the most widespread application of weather radars, their upgrade to polarimetry worldwide not only improves precipitation estimates, but their observations are also increasingly exploited for the evaluation and improvement of the representation of cloud and precipitation processes in atmospheric models (e.g. Gao et al., 2011; Jung et al., 2012; You et al., 2020; Wang et al., 2020). Additional observations from cloud radars available at so-called supersites (in Germany, e.g. the Jülich Observatory for Cloud Evolution – Core Facility; JOYCE-CF; Löhnert et al., 2015; http://www.cpex-lab.de, last access: 25 October 2021), universities, and research facilities (e.g. the Leipzig Aerosol and Cloud Remote Observations System; LACROS; Bühl et al., 2013) open opportunities to inform and improve atmospheric models. The use of shorter wavelengths of cloud radars shifts the sensitivity of the observations towards smaller particles and partly increases the magnitude of the received polarimetric signals (e.g. KDP – the differential phase shift between horizontal and vertical polarization per distance called specific differential phase – scales with λ−1), which allows for more detailed studies of ice and cloud microphysics. Polarimetric and multi-frequency radar observations allow for a more granular look at microphysical processes and provide a great database for model evaluation, the improvement of microphysical parameterizations, and data assimilation and thus have the potential to significantly improve both weather forecasts and climate predictions.
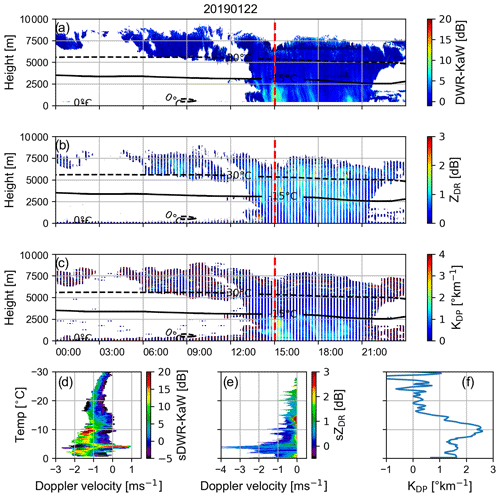
Figure 1Observations at JOYCE-CF show (a) DWR-KaW, (b) ZDR (measured at a 30∘ elevation angle), and (c) KDP (also measured at 30∘ elevation angle) on 22 January 2019. Panels (d–f) show the observed DWR spectrum, ZDR spectrum, and KDP profile at 15:00 UTC; indicated by the red line in (a–c).
3.1 Multi-frequency and spectral polarimetry for ice and cloud microphysics
The PROM project “Understanding Ice Microphysical Processes by combining multi-frequency and spectral Radar polarImetry aNd super-parTicle modelling” (IMPRINT) improves ice microphysical process understanding by using spectral multi-frequency and radar polarimetric observations in combination with Monte Carlo Lagrangian super-particle modelling (Brdar and Seifert, 2018). Mid-latitude stratiform clouds, which occur frequently during wintertime over JOYCE-CF, are the main focus. Radar polarimetric variables are well known to be particularly sensitive to the presence of asymmetric ice particles (e.g. Kumjian, 2013). Only recently have polarimetric cloud radars operating at the Ka- or W-band also become routinely available (Oue et al., 2018; Myagkov et al., 2016; Bühl et al., 2016; Matrosov et al., 2012). Some polarimetric variables are wavelength dependent (KDP is inversely proportional to the wavelength), which provides enhanced sensitivity to ice particle concentration at higher frequencies. Multi-frequency approaches are complementary to radar polarimetry as they are sensitive to larger ice particles. Most commonly, the dual wavelength ratio (DWR), defined as the logarithmic difference of the effective reflectivity Ze at two frequencies, is used. When ice particles transition from Rayleigh into non-Rayleigh scattering from one wavelength to a higher one, the DWR increases, which allows one to infer the characteristic size of the underlying size distribution. The use of three radar frequencies (e.g. X, Ka, W) extends the discernable size range; for example, the DWR of the Ka–W combination saturates for very large particles (Kneifel et al., 2015; Ori et al., 2021). The information content can be further extended when the Doppler spectral information is also explored. The different fall velocities allow for the separation of different hydrometeors; the high differential reflectivity (ZDR) signal originating from small, slowly falling ice crystals can be distinguished from the also low ZDR signal of faster falling snow aggregates, which usually dominate the total ZDR. Only a few studies so far have used spectral polarimetric observations for ice and snow microphysical studies (Luke et al., 2021; Oue et al., 2018; Pfitzenmayer et al., 2018; Spek et al., 2008). The observations collected during the first multi-month winter campaign carried out at JOYCE-CF as part of the IMPRINT project provide the opportunity to investigate both polarimetry and multi-frequency observations in the Doppler spectra space for the first time. An example is the analysis of the dendritic growth layer (DGL) illustrated in Fig. 1 for a snowfall event observed on 22 January 2019 at JOYCE-CF. Especially in the upper half of the cloud, ZDR is enhanced while KDP values are low (Fig. 1b–c). Starting at the −15 ∘C isotherm, ZDR sharply decreases and shows an anti-correlation with the enhanced DWR (Fig. 1a) and KDP values. These polarimetric signatures have been reported by previous studies (e.g. Moisseev et al., 2015, among others), and the DWR increase below the −15 ∘C level also resembles the examples shown in Oue et al. (2018). Oue et al. (2018) concluded, in agreement with findings in Moisseev et al. (2015), that an increasing concentration of asymmetric aggregates is partly responsible for enhanced KDP values because the number of small ice particles decreases due to aggregation. The spectrally resolved ZDR (sZDR, Fig. 1e), however, reveals that high-ZDR-producing, slowly falling ice particles are still present down to the −5 ∘C level. The spectrally resolved DWR (Fig. 1d) shows that the particles falling from above into the DGL are already partly aggregated. At −17 ∘C, the spectra are much wider, and a new spectral mode appears which is linked to the rapid sZDR increase (Fig. 1e). The new ice particle mode increases in Doppler velocity and sDWR until 20 dB is reached. Unlike ZDR, KDP (Fig. 1c and f) remains at values between 1 and 2 ∘ km−1 down to the −5 ∘C level. A possible explanation of the bimodal spectra – increased sZDR and KDP – might be secondary ice processes such as collisional fragmentation (Field et al., 2017). The few existing laboratory studies indicate that the number of fragments rapidly increases at −20 ∘C, reaching a maximum at −17 ∘C and decreasing again towards −10 ∘C (Takahashi et al., 1995; Takahashi, 2014). This temperature dependence fits well to the observed radar signatures in the DGL, although the laboratory studies only considered collisions of solid ice spheres. As we can exclude strongly rimed particles in the snowfall case shown in Fig. 1, fragile dendritic structures growing on the surface of aggregates might be responsible, which precipitate into the DGL and might easily break into smaller pieces during particle collisions (Fig. 1d). Monte Carlo Lagrangian super-particle model (Brdar and Seifert, 2018) simulations were recently extended in IMPRINT by a habit prediction scheme and a parameterization of ice collisional fragmentation following Phillips et al. (2017). The role of ice fragmentation and other ice microphysical processes is currently investigated with a radar observation operator for explaining the observed radar signatures of intense aggregation shown in Fig. 1.
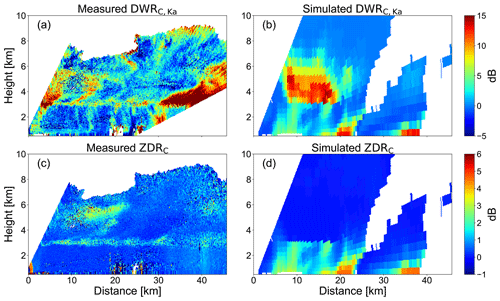
Figure 2(a) Dual-wavelength ratio between the C-band POLDIRAD and Ka-band miraMACS measurements on 7 July 2019, (b) simulated dual-wavelength ratio, (c) differential radar reflectivity ZDR measured by the C-band radar POLDIRAD, and (d) simulated ZDR of a comparable, but not identical, precipitation event using the P3 scheme (Morrison and Milbrandt, 2015).
The PROM project “Investigation of the initiation of convection and the evolution of precipitation using simulations and polarimetric radar observations at C- and Ka-band” (IcePolCKa) combines observations of the C-band Polarization Diversity Doppler Radar (POLDIRAD) at the German Aerospace Center (DLR), Oberpfaffenhofen, with those of the Ka-band, Milimeter-wave cloud RAdar of the Munich Aerosol Cloud Scanner (miraMACS) at Ludwig-Maximilians-Universität (LMU), Munich. While IMPRINT combines triple-frequency zenith-pointing observations with spectral cloud radar polarimetry, IcePolCKa explores the life cycle of convective precipitation with spatially separated weather and cloud radars in order to quantify ice crystal properties in precipitation formation. The project focuses on ice particle growth and its role in precipitation formation within convective cells. Coordinated range–height indicator (RHI, varying elevation at constant azimuth) scans along the 23 km long cross section between both radars allow observation of DWR (Fig. 2a) and ZDR (Fig. 2b) fingerprints of individual convective cells. While the deviation from Rayleigh scattering with increasing ice crystal size at the cloud radar wavelength allows one to distinguish regions dominated by aggregation from regions with depositional growth, the slanted perspective of the weather radar helps to narrow down the aspect ratio of ice crystals. Although the DWR technique to infer ice crystal size is well established (e.g. Kneifel et al., 2015), assumptions about the unknown ice crystal shape are necessary. Here, simultaneous polarimetric measurements, like ZDR, help to narrow down estimates of the average asphericity of ice crystals and reduce ambiguities in retrieving ice crystal size and ice water content. IcePolCKa develops an algorithm, which uses ZH, ZDR, and DWR measurements from the two radars to retrieve ice water content (IWC), the mean particle diameter Dm, and the aspect ratio of ice crystals using a least-squares fit between measurements and T-matrix scattering simulations. The model of horizontally aligned spheroids in combination with an effective medium approximation following Hogan et al (2012) is used to find the simplest ice particle model which explains the multi-wavelength polarimetric measurements. The approach allows the study of the covariance of DWR and ZDR while varying particle density, mean particle diameter Dm, and aspect ratio. More sophisticated models, such as discrete dipole approximation (DDA) simulations of specific ice crystals, would require the knowledge of the aspect ratio, and they make it hard to identify ice shape collections along these free variables. The multi-wavelength polarimetric measurements are also used as a benchmark for convective precipitation formation in NWP models, where cloud microphysics introduce substantial uncertainty (e.g. Morrison et al., 2020; Xue et al., 2017). In IcePolCKa, simulated microphysical processes in NWP models are compared to fingerprints in radar observations: a nested WRF setup covering the overlap area of both radars is used to simulate convective events with microphysical schemes of varying complexity while the Cloud-resolving model Radar SIMulator (CR-SIM; Oue et al., 2020) produces synthetic radar observations, such as DWR (Fig. 2c) and ZDR (Fig. 2d). Figure 2 illustrates that the Predicted Particle Properties (P3) scheme (Morrison and Milbrandt, 2015) is able to produce DWR features of similar magnitude and variability compared to the observations, while a realistic ice particle asphericity is still missing. IcePolCKa compiled over 30 convective days of polarimetric measurements and simulations with five different schemes over a 2-year period, which is currently used to analyse how well these different microphysical schemes reproduce the polarimetric observations. A cell-tracking algorithm (TINT; Fridlind et al., 2019) facilitates the comparison on a cell object basis. Comparison of macrophysical cloud characteristics, such as echo top height or maximum cell reflectivity, shows that the model simulates too few weak and small-scale convective cells, independent of the microphysics scheme. In ongoing studies, the P3 scheme seems to better represent radar signatures within the ice phase, while a spectral bin scheme tends to better simulate radar signatures within rain, where all other schemes are not able to correctly reproduce observed ZDR features.
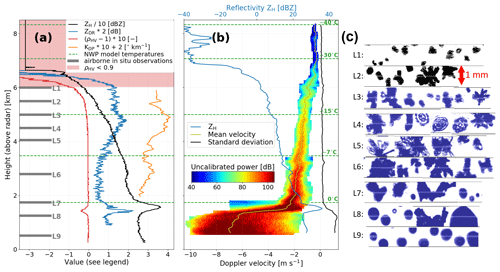
Figure 3Measurements of slant-viewing and zenith-pointing polarimetric C-band weather radar scans with NWP model-based temperature levels and airborne in situ observations: (a) quasi-vertical profiles (QVPs) of radar reflectivity ZH, differential reflectivity ZDR, copolar cross-channel correlation coefficient ρHV, and the specific differential phase KDP estimated from (noisy) measurements of the differential phase by aggressive filtering above the melting layer. (b) Average Doppler spectra from a 15 s birdbath scan and corresponding first three moments at each radar bin height: reflectivity, power-weighted mean velocity, and standard deviation. (c) In situ particle images (downward-looking projection images) collected at altitudes L1 to L9.
The PROM project “A seamless column of the precipitation process from mixed-phase clouds employing data from a polarimetric C-band radar, a micro-rain radar and disdrometers” (HydroColumn) characterizes precipitation processes inside a vertical atmospheric column by combining polarimetric Doppler weather radar observations with co-located measurements from micro-rain radars, disdrometers, and in situ measurements and by relating these observations to the large-scale atmospheric thermodynamics derived from NWP models. To date, spectral analyses are mostly performed with cloud radars operating at shorter wavelengths (see previous paragraphs or, e.g. Shupe et al., 2004; Verlinde et al., 2013; Kalesse et al., 2016; Gehring et al., 2020; Li and Moisseev, 2020), but their implementation across the national C-band radar network offers prospects for operational area-wide applications, e.g. the identification of dominant precipitation particle growth processes such as aggregation or riming. While the operational DWD birdbath scan has so far been used primarily to monitor ZDR (Frech and Hubbert, 2020), HydroColumn now also exploits the Doppler spectra measured at C-band for the analysis of microphysical process information. Figure 3 shows quasi-vertical profiles (QVPs; Trömel et al., 2014; Ryzhkov et al., 2016) of polarimetric variables and Doppler spectra from birdbath scans for a stratiform precipitation event monitored with the Hohenpeißenberg C-band research radar (47.8014∘ N, 11.0097∘ E) of DWD together with in situ particle images obtained by the Falcon research aircraft from DLR during the BLUESKY campaign (Voigt et al., 2021) within the POLICE project (Sect. 4.2.1). In situ measurements have been performed with the Cloud, Aerosol and Precipitation Probe (CAPS; Kleine et al., 2018) integrated in a wing station on the Falcon flying within a horizontal distance of about 20 km from the radar site and within about ±15 min of the radar measurements. The dendritic growth layer (DGL; Ryzhkov and Zrnic, 2019) centred around −15 ∘C is characterized by ZDR maxima of ∼ 1 dB and KDP of ∼ 0.2 ∘ km−1 and a strong ZH increase towards lower levels (Fig. 3a). Particle images collected at temperatures below about −15 ∘C indicate mostly small irregular ice particles with the number of larger particles increasing toward −15 ∘C (see levels L1 and L2 in Fig. 3c) and further down also reveal dendrites and plates (L3, L4). In general, aggregation and riming become highly effective particle growth mechanisms at temperatures around −7 ∘C (Libbrecht, 2005), and both processes result in a reduction of ZDR (Fig. 3a). The vertically pointing Doppler measurements can be used here to gain a deeper insight into the particle growth process. In this case study, the Doppler measurements illustrated in Fig. 3b indicate typical ice-particle fall speeds increasing to about 2 m s−1 just above the melting layer and thus suggest a transition from predominantly aggregates to moderately rimed particles based on the relationship between Doppler velocity and riming degree found by Kneifel and Moisseev (2020). This conclusion is supported by the corresponding in situ images showing increasing riming of polycrystals and aggregates toward the melting layer (L6). The analysis confirms the benefit of interpreting radar signatures from polarimetric weather radar observations in combination with vertically pointing Doppler radar measurements, which was previously pointed out for higher-frequency cloud research radars (Oue et al., 2018; Kumjian et al., 2020). This novel application of radar spectral analysis to vertically pointing operational weather radar scans may provide a more detailed view into intense precipitation events, such as hailstorms, where the use of cloud radars is severely limited due to the strong attenuation at high radar frequencies.
3.2 Anthropogenic modifications of precipitation microphysics
The PROM project “Polarimetry Influenced by CCN aNd INP in Cyprus and Chile” (PICNICC) seeks to improve our understanding of aerosol effects on microphysical growth processes in mixed-phase clouds. PICNICC exploits unique remote-sensing datasets from the LACROS suite (Radenz et al., 2021) extended with ground-based remote sensing instruments installed at Leipzig University, Universidad de Magallanes (Punta Arenas), and Cyprus University of Technology (Limassol). Thus, dual-frequency polarimetric radar observations from the polluted, aerosol-burdened Northern Hemisphere and from the clean, pristine Southern Hemisphere can be contrasted for microphysical process studies as already performed in the project for stratiform mixed-phase clouds to investigate inter-hemispheric contrasts in the efficiency of heterogeneous ice formation (Radenz et al., 2021). The PICNICC project challenges the hypothesis that higher ice crystal concentrations favour aggregation, which is expected to be more frequent for high aerosol loads and accordingly higher ice-nucleating particle (INP) concentrations, while riming should prevail when supercooled liquid layers are sustained due to a scarcity of INPs. Evaluating this hypothesis requires the distinction between aggregation and riming in mixed-phase cloud systems. Figure 4 demonstrates for a deep mixed-phase cloud system passing the low-aerosol site in Punta Arenas (53∘ S, 71∘ W), Chile, on 30 August 2019, the capability of the LACROS suite to distinguish between aggregates and rimed particles when combined with a 94 GHz Doppler radar. The pattern of the 94 GHz radar reflectivity factor (Ze, Fig. 4a) underlines the complex structure of the system. The height spectrogram of the vertical-pointing 94 GHz slanted linear depolarization ratio (SLDR, Fig. 4e) from 08:30 UTC exhibits regions of changing shape signatures and multi-modality in the cloud radar Doppler spectra, where multiple hydrometeor populations coexist. The polarizability ratio ξe (Myagkov et al., 2016; Fig. 4d) obtained from the RHI scans of SLDR and the co-cross-correlation coefficient of horizontally and vertically polarized channels in the slanted basis ρs at 35 GHz (Fig. 4b, c) allows the estimation of a density-weighted hydrometeor shape. SLDR is more suited for shape classification compared to LDR. By slanting the polarization basis by 45 ∘, the returned LDR signatures are much less sensitive to the canting angle distribution of the targets, especially at low elevation angles (Matrosov et al., 2001; Myagkov et al., 2016). The polarimetric RHI scans and the Doppler spectra data enable the retrieval of the vertical profile of the hydrometeors: columnar-shaped bullet rosettes are formed between 2.5 km height and cloud top as indicated in the RHI scans by an elevation-constant SLDR (Fig. 4b) and an increase in ρs with decreasing elevation (Fig. 4c). ξe values around 1.3 (Fig. 4d) are characteristic for slightly columnar crystals. The decreasing elevation dependence of ρs already at around 3 km height (−15 to −20 ∘C) suggests more random particle orientations; here the W-band SLDR spectra (Fig. 4e) show reduced values, likely due to the co-existence of dendritic ice crystals, which are preferably formed in this temperature range. The co-location of dendrites and columnar crystals can be explained by either splintering of the arms of the dendritic crystals or a mixing of locally produced dendrites with columnar crystals from higher up, or both. Below 2.5 km, ξe decreases toward unity, indicating the growth of isometric particles. In addition, the vertical-pointing W-band SLDR slowly decreases toward the cloud base, while fall velocities increase (Fig. 4e). Both features are characteristic for riming, which is corroborated by co-located lidar observations that indicate liquid water in the cloud-base region (not shown). Doppler spectra profiles such as the one presented in Fig. 4e are also used in a new neural-network-based riming detection algorithm recently tailored by Vogl et al. (2021) for vertical-pointing cloud radar observations. This new approach is insensitive to the mean Doppler velocity, which is – especially at Punta Arenas – strongly influenced by orographic mountain waves, because the radar reflectivity factor, skewness, and edge width of the Doppler spectrum are used instead.
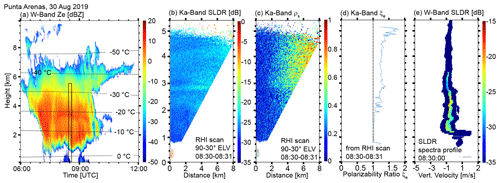
Figure 4Case study of a deep mixed-phase cloud event observed with multiwavelength polarimetric cloud radars at Punta Arenas, Chile, on 30 August 2019. (a) Vertical-pointing W-band (94 GHz) radar reflectivity factor Ze and isolines of modelled air temperature. (b) (c) Ka-band (35 GHz) RHI scans (90–30∘ elevation) of slanted linear depolarization ratio (SLDR) and co-cross-correlation coefficient in the slanted basis ρs, respectively, from 08:30–08:31 UTC. (d) Profile of the shape index polarizability ratio (ξe) obtained from the RHI scans shown in (b) and (c) and (e) height spectrogram (at 90∘ elevation) of W-band SLDR from 08:30:00 UTC. The time and height frame of (b–e) is indicated by the black rectangle in (a).
The PROM project “Investigating the impact of Land-use and land-cover change on Aerosol-Cloud-precipitation interactions using Polarimetric Radar retrievals” (ILACPR) analyses polarimetric radar observations and model simulations simultaneously in order to improve our understanding of land–aerosol–cloud–precipitation interactions. The Terrestrial Systems Modelling Platform (TSMP; Shrestha et al., 2014; Gasper et al., 2014) developed under the DFG-funded Transregional Research Center TR32 (Simmer et al., 2015) is used to simulate summertime convective storms passing the polarimetric X-band radar (BoXPol; e.g. Diederich et al., 2015a, b) located in Bonn, Germany. TSMP generally underestimates the convective area fraction, high reflectivities, and width–magnitude of differential reflectivity (ZDR) columns indicative of updrafts, all leading to an underestimation of the frequency distribution for high precipitation values (Shrestha et al., 2021a). A decadal-scale simulation over the region using the hydrological component of TSMP also shows that much of the variability in the simulated seasonal cycle of shallow groundwater could be linked to the distribution of clouds and vegetation (Shrestha, 2021), which further emphasizes the importance of evaluating the representation of clouds and precipitation in numerical models. The fusion of radar observations and models with the aid of observation operators allows for an extended interrogation of the effects of anthropogenic interventions on precipitation-generating processes and the capabilities of numerical models to reproduce them. Here, findings from one simulated hailstorm observed on 5 July 2015 passing the city of Bonn, Germany, are explained. Sensitivity simulations are conducted using large-scale aerosol perturbations and different land-cover types reflecting actual, reduced, and enhanced human disturbances. While the differences in modelled precipitation in response to the prescribed forcing are below 5 %, the micro- and macrophysical pathways differ, acting as a buffered system to the prescribed forcings (Stevens and Feingold, 2009; Seifert and Beheng, 2012). Figure 5 shows vertical cross sections reconstructed from volume scans measured with BoXPol together with simulated ZH and ZDR for the TSMP simulations with actual land cover but perturbed condensation nuclei (CN) and ice-nucleating particle (INP) concentrations. CN concentrations are 100 cm−3 for maritime and 1700 cm−3 for continental aerosol. Similarly, default INP concentrations for dust, soot, and organics are 162×103, 15×106, and 177×106 m−3, respectively. For low (high) INPs, the concentration of soot and organics are decreased (increased) by 1 order of magnitude. To generate the synthetic radar observations, the Bonn Polarimetric Radar observation Operator, B-PRO (Xie et al., 2021, 2016; Heinze et al., 2017; Shrestha et al., 2021b), is applied. B-PRO is based on the non-polarimetric version of EMVORADO (Zeng et al., 2016); its code part for computing unattenuated radar reflectivity on the original model grid (Blahak, 2016) has been expanded to unattenuated polarimetric variables based on spheroidal shape assumptions (T-matrix). Because the full polarimetric version of EMVORADO (Pol-EMVORADO; see Sect. 4.1) was only released very recently, the model data in ILACPR have been processed using B-PRO. Preliminary comparisons between B-PRO and Pol-EMVORADO (not shown here) exhibit negligible differences in their results on the model grid, but Pol-EMVORADO is much more computationally efficient and takes effects of beam broadening and attenuation along the actual radar ray paths into account. The vertical cross sections are compared at different times marked by the vertical grey bars in the time series of convective area fraction (CAF, Fig. 5a), defined as the ratio of area with ZH>40 dBZ (at 2 km a.g.l.) to total storm area. On average BoXPol observations show a bit higher CAF compared to the simulations. The evolution is always similar in terms of an initial increase and intensification in the second part of the observation period, where the experiment with maritime aerosols and low INPs (Mar-lowIn) is closest to the observations. All simulations show ZH and ZDR patterns comparable to BoXPol observations; however, the experiment with continental aerosol and default INPs (Con-defIN, Fig. 5c) shows weaker ZH while Mar-lowIN (Fig. 5d) shows somewhat higher ZH values compared to BoXPol (see Fig. 5a). The simulations with maritime CN produce low cloud droplet concentrations with larger mean diameters compared to the simulations with continental CN. Accompanied by a very strong updraft, this also leads to high concentrations of supercooled raindrops above the melting layer with broader spatial extent (due to a broader updraft region) compared to the simulations with continental CN and contributes to an enhanced growth of hail resulting in higher ZH. Also, as shown in the CAF time series, simulations with continental aerosol and default/high IN tend to exhibit similar behaviour in radar space, with the latter exhibiting higher CAF only at latter stages of the storm. The continental CN simulations with default and high IN differ in terms of simulated updraft speed and total hydrometeor content, being higher for the latter one. However, Cont-highIN produces smaller graupel and hail particles compared to Cont-defIN, resulting in similar ZH. The experiment with continental aerosol and high INP concentration (Con-highIN, not shown) generates similar polarimetric moments to Con-defIN. All experiments exhibit vertically extensive columns of (slightly) enhanced ZDR, collocated with intense simulated updrafts reaching up to 13 to 14 km. Indeed, ZDR columns emerged as proxies for updraft strength and ensuing precipitation enhancement (Weissmann et al., 2014; Simmer et al., 2014; Kumjian et al., 2014; Kuster et al., 2020), and research on their exploitation for nowcasting and data assimilation is ongoing. In Fig. 5c, d synthetic ZDR columns are vertically extensive, while ZDR values within the column stay below 0.3 dB. BoXPol observations show ZDR columns reaching up to 6 km height only but with ZDR values exceeding 1 dB. While ZDR values in the lower part of the columns are mostly generated by large raindrops, freezing drops and wet hail determine ZDR in the upper parts of the column (Kumjian et al., 2014; Snyder et al., 2015). The diverging appearance of observed and synthetic ZDR columns may point to deficiencies in the treatment of raindrops undergoing freezing and motivates further research. Too rapid freezing of drops combined with graupel generated from the frozen drops may generate enhanced but still low ZDR up to high altitudes. Following Ilotoviz et al. (2018) such attributes of ZDR columns are highly determined by the vertical velocity, hail size, and aerosol concentration; e.g. higher CN concentrations lead to higher columns with higher ZDR values inside and also higher ZH. In this case study and the specific time step shown, Mar-lowIN (i.e. with lower CN concentration) shows a wider and somewhat taller ZDR column together with a more intense ZH core (compare Fig. 5c, d). Further explanations require an improved representation of the ZDR columns in the model.
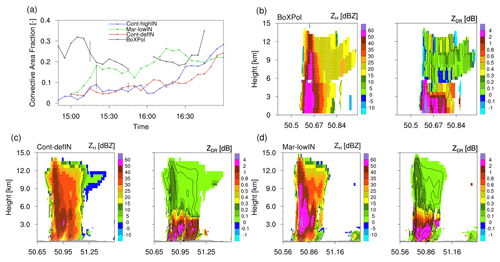
Figure 5Time series of convective area fraction (CAF) evolution (a) and reconstructed observed (b) and simulated/synthetic range–height indicators (RHIs) of horizontal reflectivity ZH and differential reflectivity ZDR (c, d). Synthetic RHIs are based on simulations for actual land cover with different perturbations of CN and IN concentrations, where Cont-defIN indicates continental aerosol with default IN concentration, and Mar-lowIN indicates maritime aerosol with low IN concentration. The gaps in the BoXPol-observed CAF time series are due to strong attenuation. The vertical grey bars (a) indicate the times at which the RHIs are compared.
Probably the most important and central tool for connecting polarimetric observations with numerical atmospheric models are observation operators, which generate virtual observations from the model state. These virtual observations can be directly compared with the real observations and signatures of microphysical processes including their temporal evolution. Thus, the accuracy of precipitation and cloud parameterizations can be indirectly evaluated, and a database can be established for model optimization. Missing polarimetric process fingerprints (e.g. Kumjian, 2012) in the virtual observations may hint at model deficiencies, and model parameterizations can be adapted in order to increase the coherence between real and virtual observations. Moreover, sufficiently accurate and fast observation operators are mandatory for the direct assimilation of observations using ensemble methods.
However, bulk cloud microphysical parameterizations required for NWP models include assumptions on several critical parameters and processes which are not explicitly prognosed or resolved by the governing numerical model. An example is the inherently assumed particle size distributions and their relations to the prognostic moments (hydrometeor mass and number densities). Another challenge is the handling of hydrometeor parameters that are not or only insufficiently constrained by the model's microphysics but are highly relevant for the calculation of virtual observations in the (radar) observation operator. For example, the melting state and shape, microstructure, and spatial orientation of the different hydrometeors are not prognostic (or not even implicitly assumed) in most operational bulk schemes. Therefore, suitable assumptions are required in observation operators in order to compute meaningful virtual observations. Moreover, bulk cloud microphysical schemes may only insufficiently approximate the natural variability, and the interactions between the few assumed hydrometeor classes and the size distribution moments are mainly tuned to get, e.g. the surface precipitation right. The current approximations in both numerical models and observation operators may hence translate into different sources of errors and biases of the simulated radar variables (e.g. Schinagl et al., 2019; Shrestha et al., 2021b). As an example, Fig. 7 shows too low polarimetric signals above the melting layer, which are partly caused by assumptions inherent in the observation operator (see Sect. 4.2.1). Such problems challenge both model evaluation and data assimilation. Accordingly, central science questions concern the realism of the sensitivities of simulated radar variables to parameters in the observation operators and the models as well as effective approaches for the evaluation and improvement of moist process parameterizations.
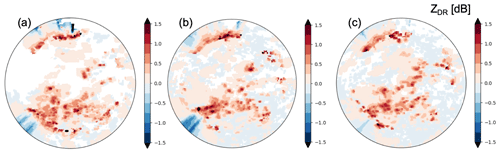
Figure 6Synthetic PPI of ZDR at 0.5 ∘ elevation for the DWD radar site Neuheilenbach based on the analysis obtained for 4 June at 16:00 UTC by assimilation of radar reflectivity and using three different ways to specify the model error: large-scale uncertainty (a), large plus unresolved scales of uncertainty (b), and in addition the use of the warm bubble approach (c).
Another challenge for large-scale applications such as long-term model evaluations or operational real-time data assimilation based on large radar networks is the high computational demand and low speed of current polarimetric radar observation operators. Often, the operators apply some kind of pre-calculated lookup tables (LUTs) of scattering properties and parallelization techniques for speed optimizations (e.g. Wolfensberger and Berne, 2018; Matsui et al., 2019; Oue et al., 2020). Despite that, radar simulations for a single time step take – depending on the computer – on the order of minutes for one single plan position indicator (PPI) scan (Wolfensberger and Berne, 2018) or for a single model scene (CR-SIM; Oue et al., 2020). Matsui et al. (2019) state the LUT generation process of their POLARRIS operator to only take a few minutes when distributed to a few thousand processors, but they do not elaborate on the required times for the actual simulation of the radar measurement. The operator B-PRO (Xie et al., 2016), which uses neither of these techniques, is much slower, as applications within SPP-PROM have demonstrated (Shresta et al., 2021b). While acceptable for research, real-time operational applications may pose much stricter time constraints. Therefore, an important technical goal is to provide an efficient, yet physically accurate and “state-of-the-art”, polarimetric radar operator to the community, which reduces the simulation time for multi-elevation PPI scans of many stations to a few seconds.
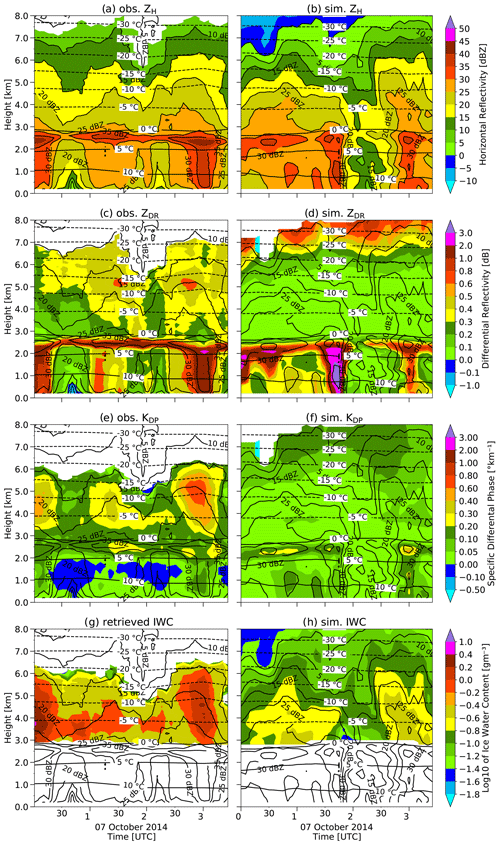
Figure 7Quasi-vertical profiles (QVPs) of observed (left column) and simulated (right column) polarimetric radar variables' horizontal reflectivity ZH (a, b), differential reflectivity ZDR (c, d), and specific differential phase KDP (e, f) together with radar-retrieved (g) and simulated ice water content (IWC, h). The QVPs show a stratiform rain event observed on 7 October 2014 between 00:00 and 03:30 UTC with the polarimetric X-band radar in Bonn, BoXPol, and simulated with COSMO version 5.1 and the two-moment cloud microphysics scheme.
4.1 Polarimetric radar observation operator development
Within the PROM project Operation Hydrometeors, the up-to-now non-polarimetric radar observation operator EMVORADO (Zeng et al., 2016; Blahak and de Lozar, 2020; Blahak, 2016) has been extended to polarimetry (Mendrok et al., 2021). (Non-polarimetric) EMVORADO has been designed to efficiently simulate PPI volume scan measurements of entire radar networks from the prognostic model state of an NWP model for direct comparisons with the radar observations. EMVORADO is part of the executable of both the COSMO and ICON NWP models, which allows us to run the operator within a NWP model run and to access the model state and radar variables in memory. The code is MPI- and OpenMP-parallelized and thus fully exploits the computational power of modern high-performance computers (HPCs) and avoids storing and re-reading extensive model state data to and from hard drives. This enables large-scale real-time applications such as operational data assimilation and extensive NWP model verifications using whole radar networks at high temporal resolution. Its modular nature allows for relatively easy interface development to other NWP models. An offline framework is also available, which accesses model states of one model time step from hard disc. EMVORADO includes detailed modular schemes to simulate beam bending, beam broadening, and melting effects and allows users to choose for each process between computationally cheap and physically accurate options. The operator has been used for the assimilation of radar reflectivity with a positive impact on precipitation forecasts (Bick et al., 2016; Zeng et al., 2018, 2019, 2020). Currently, DWD uses EMVORADO to operationally assimilate 3D volumetric reflectivity and radial wind observations of its C-band radar network. Key for this application is also the extensive use of precomputed lookup tables that relate (Mie-theory-based) bulk reflectivity directly to hydrometeor densities and temperature. The effects of neglecting radar beam pattern and broadening and of hydrometeor fall speeds on data assimilation have been investigated in a joint effort together with the PROM project “Representing model error and observation Error uncertainty for Data assimilation of POLarimetric radar measurements” (REDPOL) (Zeng et al., 2021a).
The polarimetry-extended EMVORADO, in the following referred to as Pol-EMVORADO, has inherited all features of EMVORADO, which in turn have been expanded where necessary to calculate and handle polarimetric variables. This includes, e.g. beam bending, beam broadening, and beam smoothing schemes; effective medium approximations allowing one- and two-layered hydrometeors with different water–ice–air mixing schemes and melting topologies; and a lookup table approach for efficient access to polarimetric observables such as ZDR, LDR, ρHV, and KDP. Optionally, attenuation effects can be considered, specific and differential attenuation (AH and ADP, respectively) can be provided, and further output quantities derivable from the complex scattering amplitudes can be easily added. Pol-EMVORADO applies state-of-the-art scattering properties of spheroidal particles derived by one-layered (Mishchenko, 2000) and two-layered T-matrix approaches (Ryzhkov et al., 2011). Assumptions on spheroid shape and orientation follow parameterizations introduced in Ryzhkov et al. (2011). The lookup table approach has been revised to accommodate additional parameters necessary to derive the full set of polarimetric radar output. For a given set of parameters affecting the hydrometeor scattering properties, the lookup tables are created only once, stored in files, and re-used for subsequent runs.
Using pre-existing lookup tables, the computations for virtual polarimetric volume scans of radar networks are very fast. For example, simulating the volume scan observations of all polarimetric parameters for all 17 German radars takes only a few seconds on a Linux workstation (8 cores) and adds only about 1 s per radar output time step to the model runtime when performed online during a run of ICON-D2 (DWD's operational convection-allowing ICON version with 2 km grid spacing) on DWD's NEC Aurora supercomputer. That is, simulating polarimetric radar data in intervals of 5 min as observed by DWD's weather radar network adds only a few percent of the total model runtime (Mendrok et al., 2021), enabling the exploitation of Pol-EMVORADO for the assimilation of high-temporal-resolution polarimetric radar data in an operational framework. Pol-EMVORADO has been incorporated into the official version of EMVORADO and can be run online (i.e. within a COSMO or ICON run) as well as offline (i.e. stand-alone with model fields from data files). Although designed as a PPI volume scan observation operator for a radar network, its output can also be provided on NWP model grids. An example of a ZDR volume scan simulated by Pol-EMVORADO for the REDPOL project is shown in Fig. 6 (see also Sect. 4.2.3).
In summary, (Pol-)EMVORADO comprises a wide set of state-of-the-art features. While each of these features is also provided by other observation operators (Pol-)EMVORADO is, to our knowledge, unique in combining them into one operator that allows the simulation of virtual observations, including instrumental effects and in formats directly comparable to real observational scans, from NWP model runs in a comparably accurate and very fast manner targeted at operational applications. Mendrok et al. (2021) give a comprehensive description of the features developed or updated for Pol-EMVORADO including details on their implementation and performance.
However, from the application of Pol-EMVORADO (or B-PRO; see Sect. 3.2) within PROM, a number of problems became evident. Modelling hydrometeors as homogeneous effective-medium particles (e.g. oblate spheroids) does not reproduce the polarimetric signatures of low-density hydrometeors like dendrites or aggregates typical for snow while keeping their microphysical properties well (e.g. aspect ratio, degree of orientation) within realistic – observed or model-predicted – ranges and consistent between different radar frequencies. This deficiency has been demonstrated and explained from electromagnetic theory by Schrom et al. (2018). It is obvious in one case study (Shrestha et al., 2021b) and in Fig. 7, where ZDR and KDP in the snow-dominated layer between 2.5 and 5 km height almost entirely lack the typical observed features, i.e. bands of enhanced ZDR and KDP in the dendritic growth layer that then smoothly decrease to mostly positive, non-zero values towards the melting layer. This deficiency can also be observed with other polarimetric observation operators applying a T-matrix approach (see simulation-to-observation comparisons in Wolfensberger and Berne (2018), Matsui et al. (2019), and Oue et al. (2020), where the lack of ZDR and KDP signatures is not discussed at all or exclusively explained by a lack of secondary ice, though), which nevertheless currently constitutes the state of the art in radar polarimetry. Orientation and shape of frozen and melting hydrometeors are very variable, both in nature and in the assumptions used in observation operators, which translates into large uncertainties in polarimetric radar signatures (e.g. Matsui et al., 2019; Shrestha et al., 2021b).
To tackle these challenges, it is planned to interface Pol-EMVORADO to scattering databases or other scattering models in order to enable more realistic cloud ice and aggregate snowflake scattering properties and allow for improvements or extensions of the polarimetry-related microphysical assumptions (shape/habit/microstructure, orientation, and their distribution, e.g. Wolfensberger et al., 2018), particularly for (partly) frozen hydrometeors. For PROM's second phase, we have proposed taking this up guided with Lagrangian particle model information as well as testing the application of Pol-EMVORADO in an operational data assimilation environment.
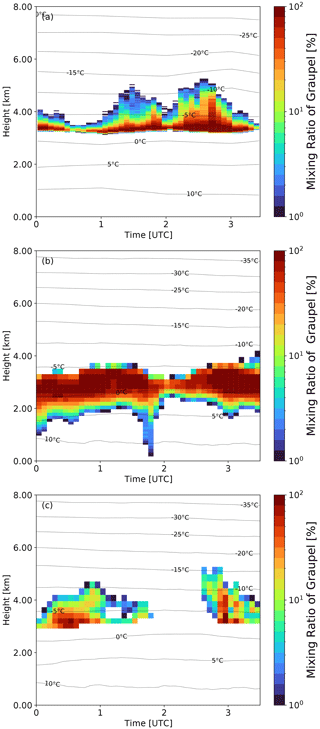
Figure 8Retrieved and simulated graupel mixing ratios, defined as the percentage of graupel in the total hydrometeor mass, for the stratiform rain event shown in Fig. 7 (7 October 2014, 00:00–03:30 UTC). An advanced hydrometeor classification and quantification algorithm has been applied to polarimetric BoXPol measurement (a) and to simulated radar variables based on COSMO simulations (c) and compared to the COSMO-simulated graupel mixing (b).
4.2 Model evaluation and improvements using forward simulations and microphysical retrievals
4.2.1 Convection-resolving simulations with COSMO
In a joint effort, the PROM projects Operation Hydrometeors and ILACPR evaluate simulated stratiform precipitation events in radar observation space and develop a sophisticated polarimetry-based hydrometeor classification and quantification for the evaluation of the representation of hydrometeors in numerical models. Based on a stratiform event monitored on 7 October 2014 with the Bonn polarimetric X-band radar BoXPol, Fig. 7 illustrates the potential of using polarimetric observations for the evaluation and improvement of microphysical parameterizations. Figure 7a–f compare QVPs of measured and virtual ZH, ZDR, and KDP with the Bonn Polarimetric Radar observation Operator B-PRO (Xie et al., 2021) to forecasts simulated with COSMO version 5.1 using its two-moment cloud microphysics scheme (itype_gscp = 2683; Seifert and Beheng, 2016). Due to a small spatial shift of the precipitation event in the simulations, the observations at 50.7305∘ N, 7.0717∘ E are compared with simulations at a close-by grid point at 51.1∘ N, 7.0717∘ E. As demonstrated in Shrestha et al. (2021b) using a similar stratiform precipitation event, COSMO tends to simulate considerable amounts of melting graupel partly reaching the surface, which results in higher synthetic ZDR than observed (compare Fig. 7c, d) within and below the melting layer (ML). Above the ML, however, synthetic ZDR already approaches 0 dB at around 6 km height, which indicates deficiencies in the ice–snow partitioning in COSMO as well as in the assumed snow morphology (soft spheroids) in the observation operator, both resulting in too low polarimetric signals. While the observed and simulated ZH is comparable in terms of structure and magnitude – except a more pronounced observed ML – larger differences exist with respect to KDP above the ML (Fig. 7e, f). While observations show bands of enhanced KDP within the dendritic growth layer (DGL) centred around −15 ∘C, the simulated KDP is very weak, indicating a lower concentration of crystals and early aggregates compared to observations (e.g. Moisseev et al., 2015). Ice water content (IWC) above the ML retrieved from measured KDP and differential reflectivity in linear-scale Zdr, i.e. IWC(KDP, Zdr) following Ryzhkov et al. (2018), agrees well with IWC modelled by COSMO in terms of structure but has lower magnitudes (compare Fig. 7g, h) in line with the lower simulated KDP. Overall, Fig. 7 supports the hypothesis of a too strong graupel production in the simulations. Operation Hydrometeors also developed a robust radar-based hydrometeor classification (HMC) and mixing ratio quantification algorithm following Grazioli et al. (2015) and Besic et al. (2016, 2018) for the evaluation of the representation of hydrometeors in NWP models (standard output is the dominant hydrometeor type only). This HMC is based on clustering and has the advantage that the radar data are separated into clusters based on their polarimetric similarity (no theoretical preliminary calculation is needed), which are then identified as hydrometeor classes. Various clustering methods can be used here (e.g. Lukach et al., 2021). The new method is relatively insensitive to uncertainties in the scattering properties of ice particles. Its application to the BoXPol observations does not indicate graupel below the ML (Fig. 8a), while COSMO simulates a pronounced thick graupel layer (Fig. 8b), including some melting graupel particles reaching the ground around 01:45 UTC. Applying the HMC to the virtual observations, however, does not reproduce a graupel layer of similar intensity (Fig. 8c), probably caused by a too strong ZH and temperature influence (compare with Fig. 7) relative to the polarimetric variables in the classification scheme which needs further investigation. A persistent challenge in according routines is that clusters are always separated by the 0 ∘C level (e.g. Ribaud et al., 2019); i.e. hail or graupel are identified as clusters only below or above the melting layer. For the case study in Shrestha et al. (2021b) the simulated graupel layer was even more pronounced and sensitivity experiments were performed to guide model improvement: increasing the minimum critical particle diameter Dcrit, which is required for self-collection of ice particles (aggregation), increased/improved the ice–snow partitioning, and a lower temperature threshold for snow and ice riming, Trime, considerably reduced the graupel production.
Comparing state-of-the-art polarimetric retrievals of liquid water content (LWC), ice water content (IWC), particle number concentration Nt, and mean particle diameter Dm (e.g. Ryzhkov et al., 2018; Ryzhkov and Zrnic, 2019; Bukovčić et al., 2020; Reimann et al., 2021; Trömel et al., 2019) with their simulated counterparts can also be used for evaluating NWP models and for data assimilation (Carlin et al., 2016). Figure 7g, h show higher IWC(KDP, Zdr) than simulated by COSMO for the case study discussed earlier. However, for more solid conclusions about possible model errors, as well as for the use of retrieved quantities for data assimilation, the retrieval uncertainties must be estimated. The analysis of data collected in the ice regions of tropical convective clouds indicate that IWC(KDP, Zdr) yields a root-mean-square error of 0.49 g m−3 with the bias within 6 % (Nguyen et al., 2019). Murphy et al. (2020) introduced the columnar vertical profile (CVP) methodology to follow the track of research aircraft and better co-locate in situ data to radar microphysical retrievals. Applying the methodology to two mesoscale convective systems, they found the best performance of polarimetric microphysical retrievals in regions of high ZDR and high KDP but recommend a much larger dataset to finally conclude on the accuracy of these retrievals.
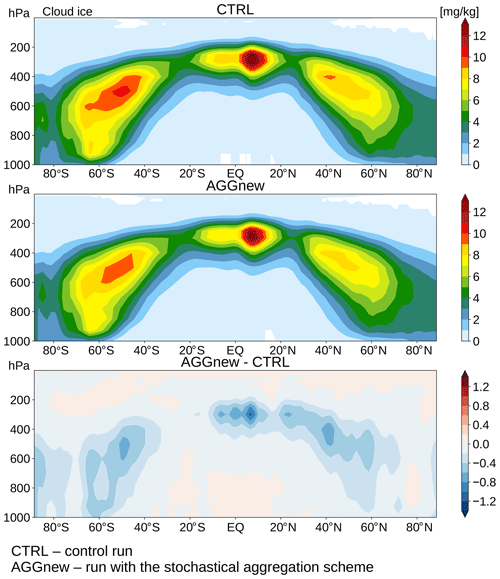
Figure 9Specific ice water, qi (g kg−1), as zonal, annual mean for (top) standard ICON GCM output, (middle) aggregation parameterization revised as stochastic parameterization drawing from the qi subgrid-variability PDF, and (bottom) difference between the two.
The PROM project “POLarimetric signatures of ICE microphysical processes and their interpretation using in situ observations and cloud modelling” (POLICE) evaluates radar retrievals and models using in particular in situ observations of microphysical cloud parameters from the research aircraft HALO (e.g. Wendisch et al., 2016; Voigt et al., 2017) and Falcon (e.g. Voigt et al., 2010, 2014; Flamant et al., 2017). Currently, ground-based polarimetric radar measurements and aircraft in situ data from the Olympic Mountain Experiment (OLYMPEX; Houze et al., 2017; Heymsfield et al., 2018) are exploited to investigate riming processes and to evaluate retrievals of ice water content (IWC), particle number concentration Nt, and mean particle diameter Dm (e.g. Ryzhkov et al., 2018; Ryzhkov and Zrnic, 2019; Bukovčić et al., 2020; Carlin et al. 2021). The OLYMPEX mission took place on the Olympic Peninsula of Washington State (USA) from November 2015 through February 2016. University of North Dakota's (UND) Cessna Citation II equipped with an in situ cloud payload overpassed the National Science Foundation (NSF) Doppler On Wheels (DOW, mobile polarimetric X-band radar with about 60 km range and 74 m radial resolution), placed in the Chehalis Valley at Lake Quinault (47.48∘ N, 123.86∘ W, 64 m altitude) performing RHI scans within an azimuthal sector of 22 ∘. Measurements and microphysical retrievals of the DOW and the Citation, respectively, are currently evaluated and will then be compared at matched space-time coordinates for several flight transects.
4.2.2 Climate simulations with ICON-GCM
A major part of the uncertainties in representing clouds and precipitation in atmospheric models can be attributed to unresolved variability that affects resolved variables via non-linear processes. Current climate model horizontal resolutions are on the order of 100 km. But even for NWP models, which have resolutions between 10 km for global and 1 km for regional simulations, most cloud processes remain unresolved. The project Climate model PArameterizations informed by RAdar (PARA) evaluates and improves the representation of cloud and precipitation processes in particular for climate models and focuses on precipitation formation in ice clouds. Since most surface precipitation over continents and extra-tropical oceans involves the ice phase (Mülmenstädt et al., 2015; Field and Heymsfield, 2015), its reliable representation is paramount and thus the focus of PARA. Microphysical parameterizations typically consider only the mean cloud liquid or ice water content to compute process rates, which causes biases in all nonlinear processes including radiation (e.g. Cahalan, 1994; Carlin et al., 2002) and precipitation formation (e.g. Pincus and Klein, 2000). Realistic results thus require the tuning of process rates (e.g. Rotstayn, 2000) or realistic estimates of subgrid-scale cloud variability and its inclusion in the process parameterizations. To tackle this issue, PARA exploits inherent model assumptions for treating fractional cloudiness. Since the early works of Sommeria and Deardorff (1977), atmospheric models assume or predict some notion of subgrid-scale variability of relative humidity. Some models do so by predicting cloud fraction (e.g. Tiedtke, 1993), and others use a diagnostic representation of the subgrid-scale probability density function (PDF) of total water specific humidity, qt (e.g. Sundqvist et al., 1989; Smith, 1990; Le Treut and Li, 1991; Rosch et al., 2015). Another option is to utilize a prognostic probability density function (PDF) of qt by assuming a functional form and predicting the shape parameters of the PDF (e.g. Tompkins, 2002; Neggers, 2009). The German climate and weather prediction model ICON in its version dedicated to climate simulations (general circulation model version, ICON-GCM) inherits the representation of physical processes from its predecessor ECHAM6 (Stevens et al., 2013) and uses the Sundqvist et al. (1989) parameterization for a diagnostic PDF of the total-water specific humidity, qt.
As a first step, PARA analyses the implied PDF of cloud ice using satellite observations from combined CloudSat-CALIPSO radar–lidar satellite observations (DARDAR; Delanoë et al., 2014). Interestingly, a first direct comparison of IWC profiles obtained from DARDAR with polarimetric retrievals based on the ground-based BoXPol radar shows an overall good agreement, except for columns with an integrated ice water path integrated water path (IWP) > 1 kg m−2. In these regions pronounced polarimetric signatures result in high IWC at higher altitudes, which are not reproduced by reflectivity-only retrievals or the DARDAR retrievals. The statistics are currently evaluated on a larger database, which is also used to investigate the impact on the parameterizations in ICON-GCM. In the second step, a stochastic parameterization approach is taken to allow for an unbiased computation of cloud microphysical process rates on average. Based on the cumulative distribution function (CDF), a random number generator draws from the CDF according to the simulated likelihood a plausible value of the specific ice mass based on which the microphysical process is computed. This specifically considers the formation of solid precipitation (snow) from ice clouds via aggregation and accretion processes (Lohmann and Roeckner, 1996; Stevens et al., 2013), and subsequently the evaporation of precipitation below the clouds. The result of the revised aggregation parameterization is shown in Fig. 9. The increased aggregation rate, which is a linear function of the specific cloud ice, qi, leads to an average decrease in qi. The aggregation rate is directly linked to the accretion rate, which lowers the effect of qi decrease. An investigation of the influence of the revised aggregation parameterization on the different microphysical process rates – which are related to the ice phase – is currently performed. A detailed evaluation of the new versus old parameterization with the ground-based polarimetric radar is on its way and will in particular focus on the timescales of evaporation of precipitation below the cloud.
4.2.3 Data assimilation
Within an idealized framework, Jung et al. (2008, 2010) and Zhu et al. (2020) demonstrated benefits of assimilating simulated polarimetric data for the estimation of microphysical state variables. Up to now, however, direct assimilation of real polarimetric data poses great challenges due to the deficiencies of cloud and precipitation schemes in NWP models in realistically representing and providing the necessary information (optimally the distribution of particle size, shape, and orientations in all model grid boxes) required by a polarimetric radar observation operator and therefore causing large representation error (Janjic et al., 2018). Both the specification of model error to examine uncertainty in microphysics (Feng et al., 2021) and the specification of the observation error for polarimetric radar observations that include estimates of the representation error (Zeng et al., 2021b) are investigated in the PROM project REDPOL. For the assimilation of radar reflectivity with an ensemble Kalman filter, several approaches for including model errors during data assimilation are explored, including (1) additive noise with samples representing large-scale uncertainty (see Zeng et al., 2018), (2) combination of large-scale and unresolved-scale uncertainty (Zeng et al., 2019), and finally (3) adding to these warm bubble triggering of convective storms in case they are missing in the 1 h forecast but present in corresponding observations (Zeng et al., 2020). Applying Pol-EMVORADO to the analysis obtained by assimilating radar reflectivity from the German C-band network, Fig. 6 illustrates the resulting differences of these three techniques in ZDR space. Obviously, synthetic ZDR values depend on the strategy used to specify the model error, putting another weight to the argument that assimilation of radar reflectivity alone is not sufficient to constrain the estimation of microphysical state variables and that polarimetric information is also required. First results in this direction were reported by Putnam et al. (2019), who assimilated ZDR below the melting layer but reported problems with the assimilation of KDP data for a supercell case due to high observation errors as a result of contamination from wet hail, dust, and debris and nonuniform beam filling.
The Priority Programme Polarimetric Radar Observations meet Atmospheric Modelling (PROM) (SPP 2115, https://www2.meteo.uni-bonn.de/spp2115/, last access: 25 October 2021) was established in April 2017 by the Senate of the Deutsche Forschungsgemeinschaft (DFG, German Research Foundation) and is designed to run for 6 years. PROM is a coordinated effort to foster partnerships between cloud modellers and radar meteorologists and thus to accelerate the exploitation of polarimetric weather radars to improve the representation of cloud and precipitation processes in numerical models. The first funding phase engaged in an as-complete-as-possible exploitation and understanding of nation-wide polarimetric measurements complemented by state-of-the-art measurement devices and techniques available at supersites. Bulk polarimetric measurements available over Germany are complemented with multi-frequency observations and spectral polarimetry for detailed studies of ice and cloud microphysics. Thus, modellers now hold an unprecedented amount of three-dimensional microphysics-related observational data in their hands to improve parameterizations. Key tools for the fusion of radar polarimetry and atmospheric modelling, e.g. the Monte Carlo Lagrangian particle model McSnow and the polarimetric observation operator Pol-EMVORADO, have been developed. PROM started with detailed investigations of the representation of cloud and precipitation processes in the COSMO and ICON atmospheric models exploiting polarimetric observation operators. First improvements of the two-moment cloud and precipitation microphysics scheme are made and more are expected in phase 2. In addition, intercomparisons of microphysics schemes in radar space have been performed. Phase 1 further developed microphysical retrievals, determined their uncertainties, and started their exploitation for model evaluation and radar-informed parameterizations. The developed prerequisites pave the way to finally exploit polarimetry for indirect and direct data assimilation in the upcoming second funding phase.
Some tools developed in phase 1, however, still require refinement in phase 2. The T-matrix calculations for electromagnetic scattering by spheroidal particles represent only a crude approximation to frozen and mixed-phase hydrometeors, especially for pristine ice particles and aggregate snowflakes at cloud radar wavelengths. It is not possible to reproduce observed polarimetric signatures of snow with the T-matrix approach (i.e. homogeneous ice–air spheroids) and realistic microphysics (shape, orientation). Refinements include interfacing to a new scattering database based on discrete dipole approximation (DDA) for realistic ice and snow particles for all relevant weather radar wavelengths and improvements of the melting scheme of graupel and hail.
Based on the progress made, the fusion of radar polarimetry and atmospheric modelling can be approached even more aggressively in phase 2. While objective 1 received most attention in phase 1, more projects will exploit the observational insights and tools developed to finally improve parameterizations and assimilate polarimetric information; i.e. more emphasis will be put on objectives 2 and 4 in phase 2. Direct assimilation of polarimetric variables remains challenging, because NWP models need to realistically represent and provide the necessary information required by a polarimetric radar observation operator; ideally the distribution of particle size, shape, and orientation would be required in all model grid boxes. Indirect assimilation of polarimetric information (e.g. microphysical retrievals and process signatures), however, is less demanding to the model and should be pursued in parallel. Modern Bayesian data assimilation techniques are sensitive to both model and observation operator biases, so that further work on these issues is of great importance for a successful data assimilation.
The data presented in this paper are available through the authors upon request. Polarimetric radar data from the operational C-band radar network are also available from the German Weather Service (DWD). Specific campaign data will be published in addition.
ST had the initial idea and mainly organized and structured the joint publication. ST, JQ, and CS formed the editorial team consolidating the text. All authors contributed to specific sections of the paper and commented on the paper.
Some authors are members of the editorial board of Atmospheric Chemistry and Physics. The peer-review process was guided by an independent editor, and the authors have also no other competing interests to declare.
Publisher’s note: Copernicus Publications remains neutral with regard to jurisdictional claims in published maps and institutional affiliations.
This article is part of the special issue “Fusion of radar polarimetry and numerical atmospheric modelling towards an improved understanding of cloud and precipitation processes (ACP/AMT/GMD inter-journal SI)”. It is not associated with a conference.
We gratefully acknowledge the funding of the German Research Foundation (DFG) to initialize the special priority programme on the Fusion of Radar Polarimetry and Atmospheric Modelling (SPP-2115, PROM). The work of contributing authors was carried out in the framework of the projects Operation Hydrometeors (grants TR 1023/16-1 and BL 945/2-1), IcePolCKa (HA 3314/9-1 and ZI 1132/5-1), ILACPR (grant SH 1326/1-1), IMPRINT (grant KN 1112/3-1), POLICE (grants TR 1023/13-1 and VO 1504/5-1), PARA (grants QU 311/21-1 and TR 1023/15-1), HydroColumn (grant FR 4119/1-1), REDPOL (grant JA 1077/5-1), and PICNICC (grants KA 4162/2-1 and SE 2464/1-1). ILACPR gratefully acknowledges the computing time (project HBN33) granted by the John von Neumann Institute for Computing (NIC) and provided on the supercomputer JUWELS at Jülich Supercomputing Centre (JSC).
This research has been supported by the Deutsche Forschungsgemeinschaft (grant nos. TR 1023/16-1, BL 945/2-1, SH 1326/1-1, TR 1023/13-1, VO 1504/5-1, QU 311/21-1, TR 1023/15-1, FR 4119/1-1, JA 1077/5-1, KA 4162/2-1, SE 2464/1-1, KN 1112/3-1, ZI 1132/5-1, and HA 3314/9-1).
This paper was edited by Timothy Garrett and reviewed by two anonymous referees.
Alfieria, L., Thielen, J., and Pappenberger, J.: Ensemble hydro-meteorological simulation for flash flood early detection in southern Switzerland, J. Hydrol., 424, 143–153, https://doi.org/10.1016/j.jhydrol.2011.12.038, 2012.
Bauer, P., Thorpe, A., and Brunet, G.: The quiet revolution of numerical weather prediction, Nature 525, 47–55, https://doi.org/10.1038/nature14956, 2015.
Besic, N., Figueras i Ventura, J., Grazioli, J., Gabella, M., Germann, U., and Berne, A.: Hydrometeor classification through statistical clustering of polarimetric radar measurements: a semi-supervised approach, Atmos. Meas. Tech., 9, 4425–4445, https://doi.org/10.5194/amt-9-4425-2016, 2016.
Besic, N., Gehring, J., Praz, C., Figueras i Ventura, J., Grazioli, J., Gabella, M., Germann, U., and Berne, A.: Unraveling hydrometeor mixtures in polarimetric radar measurements, Atmos. Meas. Tech., 11, 4847–4866, https://doi.org/10.5194/amt-11-4847-2018, 2018.
Bick, T., Simmer, C., Trömel, S., Wapler, K., Stephan, K., Blahak, U., Zeng, Y., and Potthast, R.: Assimilation of 3D-radar Reflectivities with an Ensemble Kalman Filter on the Convective Scale, Q. J. Roy. Meteor. Soc., 142, 1490–1504, 2016.
Blahak, U.: RADAR_MIE_LM and RADAR_MIELIB – Calculation of Radar Reflectivity from Model Output, COSMO Technical Report No. 28, Consortium for Small Scale Modeling (COSMO), available at: http://www.cosmo-model.org/content/model/documentation/techReports/cosmo/docs/techReport28.pdf (last access: 25 October 2021), 2016.
Blahak, U. and De Lozar, A.: EMVORADO – Efficient Modular VOlume scan RADar Operator. A User's Guide, Deutscher Wetterdienst, available at: http://www.cosmo-model.org/content/model/documentation/core/emvorado_userguide.pdf (last access: 25 October 2021), 2020.
Brdar, S. and Seifert, A.: McSnow: A Monte-Carlo Particle Model for Riming and Aggregation of Ice Particles in a Multidimensional Microphysical Phase Space, J. Adv. Model. Earth Syst., 10, 187–206, https://doi.org/10.1002/2017MS001167, 2018.
Bukovčić, P., Ryzhkov, A., and Zrnić, D.: Polarimetric Relations for Snow Estimation – Radar Verification, J. Appl. Meteorol. Clim., 59, 991–1009, https://doi.org/10.1175/JAMC-D-19-0140.1, 2020
Bühl, J., Seifert, P., Wandinger, U., Baars, H., Kanitz, T., Schmidt, J., Myagkov, A., Engelmann, R., Skupin, A., Heese, B., Klepel, A., Althausen, D., and Ansmann, A.: LACROS: The Leipzig Aerosol and Cloud Remote Observations System, in: SPIE Remote Sensing, edited by: Comeron, A., Kassianov, E. I., Schäfer, K., Stein, K., and Gonglewski, J. D., p. 889002, Dresden, Germany, https://doi.org/10.1117/12.2030911, 2013.
Bühl, J., Seifert, P., Myagkov, A., and Ansmann, A.: Measuring ice- and liquid-water properties in mixed-phase cloud layers at the Leipzig Cloudnet station, Atmos. Chem. Phys., 16, 10609–10620, https://doi.org/10.5194/acp-16-10609-2016, 2016.
Cahalan, R. F.: Bounded cascade clouds: albedo and effective thickness, Nonlinear Proc. Geoph., 1, 156–167, 1994.
Carlin, B., Fu, Q., Lohmann, U., Mace, G. G., Sassen, K., and Comstock, J. M.: High-cloud horizontal inhomogeneity and solar albedo bias, J. Climate, 15, 2321–2339, 2002.
Carlin, J. T., Ryzhkov, A. V., Snyder, J. C., and Khain, A.: Hydrometeor Mixing Ratio Retrievals for Storm-Scale Radar Data Assimilation: Utility of Current Relations and Potential Benefits of Polarimetry, Mon. Weather Rev. 144, 2981–3001, https://doi.org/10.1175/MWR-D-15-461 0423.1., 2016.
Carlin, J. T., Reeves, H. D., and Ryzhkov, A. V.: Polarimetric Observations and Simulations of Sublimating Snow: Implications for Nowcasting, J. Appl. Meteor. Climatol., 60, 1035–1054, https://doi.org/10.1175/JAMC-D-21-0038.1, 2021.
Costa-Surós, M., Sourdeval, O., Acquistapace, C., Baars, H., Carbajal Henken, C., Genz, C., Hesemann, J., Jimenez, C., König, M., Kretzschmar, J., Madenach, N., Meyer, C. I., Schrödner, R., Seifert, P., Senf, F., Brueck, M., Cioni, G., Engels, J. F., Fieg, K., Gorges, K., Heinze, R., Siligam, P. K., Burkhardt, U., Crewell, S., Hoose, C., Seifert, A., Tegen, I., and Quaas, J.: Detection and attribution of aerosol–cloud interactions in large-domain large-eddy simulations with the ICOsahedral Non-hydrostatic model, Atmos. Chem. Phys., 20, 5657–5678, https://doi.org/10.5194/acp-20-5657-2020, 2020.
Delanoë, J., Heymsfield, A. J., Protat, A., Bansemer, A., and Hogan, R. J.: Normalized particle size distribution for remote sensing application, J. Geophys. Res.-Atmos., 119, 4204–4227, https://doi.org/10.1002/2013JD020700, 2014.
Diederich, M., Ryzhkov, A., Simmer, C., Zhang, P., and Trömel, S.: Use of specific attenuation for rainfall measurement at X-band radar wavelengths – Part 1: Radar calibration and partial beam blockage estimation, J. Hydrometeor., 16, 2, 487–502, https://doi.org/10.1175/JHM-D-14-0066.1, 2015a.
Diederich, M., Ryzhkov, A., Simmer, C., Zhang, P., and Trömel, S.: Use of specific attenuation for rainfall measurement at X-band radar wavelengths – Part 2: Rainfall estimates and comparison with rain gauges, J. Hydrometeor., 16, 2, 503–516, https://doi.org/10.1175/JHM-D-14-0067.1, 2015b.
Dipankar, A., Stevens, B., Heinze, R., Moseley, C., Zängl, G., Giorgetta, M., and Brdar, S.: Large eddy simulations using the general circulation model ICON, J. Adv. Model. Earth Sy., 7, 963–986, https://doi.org/10.1002/2015MS000431, 2015.
Feng, Y., Janjić, T., Zeng, Y., Seifert, A., and Min, J.: Representing microphysical uncertainty in convective-scale data assimilation using additive noise, J. Adv. Model. Earth Sys., 13, e2021MS002606, https://doi.org/10.1029/2021MS002606, 2021.
Field, P. R. and Heymsfield, A. J.: Importance of snow to global precipitation, Geophys. Res. Lett., 42, 9512–9520, https://doi.org/10.1002/2015GL065497, 2015.
Field, P. R., Lawson, R. P., Brown, P. R. A., Lloyd, G., Westbrook, C., Moisseev, D., Miltenberger, A., Nenes, A., Blyth, A., Choularton, T., Connolly, P., Buehl, J., Crosier, J., Cui, Z., Dearden, C., DeMott, P., Flossmann, A., Heymsfield, A., Huang, Y., Kalesse, H., Kanji, Z. A., Korolev, A., Kirchgaessner, A., Lasher-Trapp, S., Leisner, T., McFarquhar, G., Phillips, V., Stith, J., and Sullivan, S.: Secondary Ice Production: Current State of the Science and Recommendations for the Future, Meteorol. Monogr., 58, 1–20, https://doi.org/10.1175/AMSMONOGRAPHS-D-16-0014.1, 2017.
Forster, P., Storelvmo, T., Armour, K., Collins, W., Dufresne, J. L., Frame, D., Lunt, D. J., Mauritsen, T., Palmer, M. D., Watanabe, M., Wild, M., and Zhang, H.: The Earth's Energy Budget, Climate Feedbacks, and Climate Sensitivity, in: Climate Change 2021: The Physical Science Basis. Contribution of Working Group I to the Sixth Assessment Report of the Intergovernmental Panel on Climate Change, Cambridge University Press, in press, 2021.
Frech, M. and Hubbert, J.: Monitoring the differential reflectivity and receiver calibration of the German polarimetric weather radar network, Atmos. Meas. Tech., 13, 1051–1069, https://doi.org/10.5194/amt-13-1051-2020, 2020.
Gao, W., Sui, C.-H., Chen Wang, T.-C., and Chang, W.-Y.: An evaluation and improvement of microphysical parameterization from a two-moment cloud microphysics scheme and the Southwest Monsoon Experiment (SoWMEX)/Terrain-influenced Monsoon Rainfall Experiment (TiMREX) observations, J. Geophys. Res.-Atmos., 116, 1–13, https://doi.org/10.1029/2011JD015718, 2011.
Gasper, F., Goergen, K., Shrestha, P., Sulis, M., Rihani, J., Geimer, M., and Kollet, S.: Implementation and scaling of the fully coupled Terrestrial Systems Modeling Platform (TerrSysMP v1.0) in a massively parallel supercomputing environment – a case study on JUQUEEN (IBM Blue Gene/Q), Geosci. Model Dev., 7, 2531–2543, https://doi.org/10.5194/gmd-7-2531-2014, 2014.
Gehring, J., Oertel, A., Vignon, É., Jullien, N., Besic, N., and Berne, A.: Microphysics and dynamics of snowfall associated with a warm conveyor belt over Korea, Atmos. Chem. Phys., 20, 7373–7392, https://doi.org/10.5194/acp-20-7373-2020, 2020.
Grazioli, J., Tuia, D., and Berne, A.: Hydrometeor classification from polarimetric radar measurements: a clustering approach, Atmos. Meas. Tech., 8, 149–170, https://doi.org/10.5194/amt-8-149-2015, 2015.
Flamant, C., Knippertz, P., Fink, A.H., Akpo, A., Brooks, B., Chiu, C.J., Coe, H., Danuor, S., Evans, M., Jegede, O., Kalthoff, N., Konaré, A., Liousse, C., Lohou, F., Mari, C., Schlager, H., Schwarzenboeck, A., Adler, B., Amekudzi, L., Aryee, J., Ayoola, M., Batenburg, A.M., Bessardon, G., Borrmann, S., Brito, J., Bower, K., Burnet, F., Catoire, V., Colomb, A., Denjean, C., Fosu-Amankwah, K., Hill, P.G., Lee, J., Lothon, M., Maranan, M., Marsham, J., Meynadier, R., Ngamini, J., Rosenberg, P., Sauer, D., Smith, V., Stratmann, G., Taylor, J.W., Voigt, C., and Yoboué, V.: The Dynamics–Aerosol–Chemistry–Cloud Interactions in West Africa Field Campaign: Overview and Research Highlights, B. Am. Meteorol. Soc., 99, 83–104, https://doi.org/10.1175/BAMS-D-16-0256.1, 2018.
Fridlind, A. M., van Lier-Walqui, M., Collis, S., Giangrande, S. E., Jackson, R. C., Li, X., Matsui, T., Orville, R., Picel, M. H., Rosenfeld, D., Ryzhkov, A., Weitz, R., and Zhang, P.: Use of polarimetric radar measurements to constrain simulated convective cell evolution: a pilot study with Lagrangian tracking, Atmos. Meas. Tech., 12, 2979–3000, https://doi.org/10.5194/amt-12-2979-2019, 2019.
Hashino, T., and Tripoli, G. J.: The Spectral Ice Habit Prediction System (SHIPS), Part I: Model Description and Simulation of the Vapor Deposition Process, J. Atmos. Sci., 64, 2210–2237, https://doi.org/10.1175/JAS3963.1, 2007.
Heinze, R., Dipankar, A., Henken, C. C., Moseley, C., Sourdeval, O., Trömel, S., Xie, X., Adamidis, P., Ament, F., Baars, H. Barthlott, C., Behrendt, A., Blahak, U. , Bley, S. , Brdar, S., Brueck, M., Crewell, S., Deneke, H., Girolamo, P. D., Evaristo, R., Fischer, J., Frank, C., Friederichs, P., Göcke, T., Gorges, K., Hande, L., Hanke, M., Hansen, A., Hege, H.-C., Hoose, C., Jahns, T., Kalthoff, N., Klocke, D., Kneifel, S., Knippertz, P., Kuhn, A., Laar, T., Macke, A., Maurer, V., Mayer, B., Meyer, C. I., Muppa, S. K., Neggers, R. A. J., Orlandi, E., Pantillon, F. , Pospichal, B., Röber, N., Scheck, L., Seifert, A., Seifert, P., Senf, F., Siligam, P., Simmer, C., Steinke, S., Stevens, B., Wapler, K., Weniger, M., Wulfmeyer, V., Zängl, G., Zhang, D., and Quaas, J.: Large-eddy simulations over Germany using ICON: A comprehensive evaluation, Q. J. Roy. Meteor. Soc., 143, 69–100, https://doi.org/10.1002/qj.2947, 2017.
Heymsfield, A., Bansemer, A., Wood, N. B., Liu, G., Tanelli, S., Sy, O. O., Poellot, M., and Liu, C.: Toward Improving Ice Water Content and Snow-Rate Retrievals from Radars, Part II: Results from Three Wavelength Radar–Collocated In Situ Measurements and CloudSat–GPM–TRMM Radar Data, J. Appl. Meteor. Climatol., 57, 365–389, 2018.
Hogan, R. J., Tian, L., Brown, P. R. A., Westbrook, C. D., Heymsfield, A. J., and Eastment, J. D.:. Radar Scattering from Ice Aggregates Using the Horizontally Aligned Oblate Spheroid Approximation, J. Appl. Meteor. Climatol., 51, 655–671, https://doi.org/10.1175/JAMC-D-11-074.1, 2012.
Ilotoviz, E., Khain, A., Ryzhkov, A. V., and Snyder, J. C.: Relation between Aerosols, Hail Microphysics, and ZDR Columns, J. Atmos. Sci., 75, 1755–1781, https://doi.org/10.1175/JAS-D-17-0127.1, 2018.
Janjic, T., Bormann, N., Bocquet, M., Carton, J. A., Cohn, S. E., Dance, S. L., Losa, S. N., Nichols, N. K., Potthast, R., Waller, J. A., and Weston, P.: On the representation error in data assimilation, Q. J. R. Meteorol. Soc., 144, 1257–1278, 2018.
Jung, Y., Xue, M., Zhang, G., and Straka, J.: Assimilation of simulated polarimetric radar data for a convective storm using ensemble Kalman filter. Part II: Impact of polarimetric data on storm analysis, Mon. Weather Rev., 136, 2246–2260, https://doi.org/10.1175/2007MWR2288.1, 2008.
Jung, Y., Xue, M., and Zhang, G.: Simultaneous Estimation of Microphysical Parameters and the Atmospheric State Using Simulated Polarimetric Radar Data and an Ensemble Kalman Filter in the Presence of an Observation Operator Error, Mon. Weather Rev., 138, 539–562, https://doi.org/10.1175/2009MWR2748.1, 2010.
Jung, Y., Xue, M., and Tong, M.: Ensemble Kalman Filter Analyses of the 29—30 May 2004 Oklahoma Tornadic Thunderstorm Using One- and Two-Moment Bulk Microphysics Schemes, with Verification against Polarimetric Radar Data, Mon. Weather Rev., 140, 1457–1475, 2012.
Kalesse, H., Szyrmer, W., Kneifel, S., Kollias, P., and Luke, E.: Fingerprints of a riming event on cloud radar Doppler spectra: observations and modeling, Atmos. Chem. Phys., 16, 2997–3012, https://doi.org/10.5194/acp-16-2997-2016, 2016.
Khain, A., Rosenfeld, D., and Pokrovsky, A.: Aerosol impact on the dynamics and microphysics of convective clouds, Q. J. R. Meteorol. Soc., 131, 2639–2663, https://doi.org/10.1256/qj.04.62, 2005.
Khain, A. P., Beheng, K. D., Heymsfield, A., Korolev, A., Krichak, S. O., Levin, Z., Pinsky, M., Phillips, V., Prabhakaran, T., Teller, A., et al.: Representation of microphysical processes in cloud-resolving models: Spectral (bin) microphysics versus bulk parameterization, Rev. Geophys., 53, 247–322, https://doi.org/10.1002/2014RG000468, 2015.
Kleine, J., Voigt, C., Sauer, D., Schlager, H., Scheibe, M., Kaufmann, S. , Jurkat-Witschas, T., Kärcher, B., and Anderson B.: In situ observations of ice particle losses in a young persistent contrail, Geophs. Res. Lett., 45, 13553–13561, https://doi.org/10.1029/2018GL079390, 2018.
Kneifel, S., von Lerber, A., Tiira, J., Moisseev, D., Kollias, P., and Leinonen, J.: Observed Relations between Snowfall Microphysics and Triple-frequency Radar Measurements, J. Geophys. Res., 120, 6034–6055, https://doi.org/10.1002/2015JD023156, 2015.
Kneifel, S. and Moisseev, D.: Long-term statistics of riming in non-convective clouds derived from ground-based Doppler cloud radar observations, J. Atmos. Sci., 77, 3495–3508, https://doi.org/10.1175/JAS-D-20-0007.1, 2020.
Kollias, P., Albrecht, B. A., and Marks Jr, F.: Why Mie Accurate observations of vertical air velocities and raindrops using a cloud radar, B. Am. Meteorol. Soc., 83, 1471–1484, https://doi.org/10.1175/BAMS-83-10-1471 2002.
Kumjian, M. R.: Principles and applications of dual-püolarization wheather radar, Part I: Description of the polarimetric radar variables, J. Operational Meteor., 1, 226–242, https://doi.org/10.15191/nwajom.2013.0119, 2013.
Kumjian, M. R.: The impact of precipitation physical processes on the polarimetric radar variables, Dissertation, University of Oklahoma, Norman Campus, available at: https://hdl.handle.net/11244/319188 (last access: 25 October 2021), 2012.
Kumjian, M. R., Khain, A. P., Benmoshe, N., Ilotoviz, E., Ryzhkov, A. V., and Phillips, V. T. J.: The anatomy and physics of ZDR columns: Investigating a polarimetric radar signature with a spectral bin microphysical model, J. Appl. Meteor. Climatol., 53, 1820–1843, 2014.
Kumjian, M. R., Tobin, D. M., Oue, M., and Kollias, P.: Microphysical insights into ice pellet formation revealed by fully polarimetric Ka-band Doppler radar, J. Appl. Meteor. Climatol., 59, 1557–1580, https://doi.org/10.1175/JAMC-D-20-0054.1, 2020.
Kuster, C. M., Schuur, T. J., Lindley, T. T., and Snyder, J. C.: Using ZDR Columns in Forecaster Conceptual Models and Warning Decision-Making, Weather Forecast., 35, 2507–2522, 2020.
Le Treut, H. and Li, Z.-X.: Sensitivity of an atmospheric general circulation model to prescribed SST changes: Feedback effects associated with the simulation of cloud optical properties, Clim. Dynam., 5, 175–187, 1991.
Li, H. and Moisseev, D.: Two layers of melting ice particles within a single radar bright band: interpretation and implications, Geophys. Res. Lett., 47, e2020GL087499, https://doi.org/10.1029/2020GL087499, 2020.
Libbrecht, K. G.: The physics of snow crystals, Rep. Prog. Phys., 68, 855–895, https://doi.org/10.1088/0034-4885/68/4/R03, 2005.
Lohmann U. und E. Roeckner, Design and performance of a new cloud microphysics scheme developed for the ECHAM general circulation model, Clim. Dynam., 12, 557–572, 1996.
Lukach, M., Dufton, D., Crosier, J., Hampton, J. M., Bennett, L., and Neely III, R. R.: Hydrometeor classification of quasi-vertical profiles of polarimetric radar measurements using a top-down iterative hierarchical clustering method, Atmos. Meas. Tech., 14, 1075–1098, https://doi.org/10.5194/amt-14-1075-2021, 2021.
Luke, E. P., Yang, F., Kollias, P., Vogelmann, A. M., and Maahn, M.: New insights into ice multiplication using remote-sensing observations of slightly supercooled mixed-phase clouds in the Arctic, P. Natl. Acad. Sci. USA, 118, e2021387118, https://doi.org/10.1073/pnas.2021387118, 2021.
Matrosov, S. Y., Reinking, R. F., Kropfli, R. A., Martner, B. E., and Bartram, B. W.: On the use of radar depolarization ratios for estimating shapes of ice hydrometeors in winter clouds, J. Appl. Meteorol., 40, 479–490, https://doi.org/10.1175/1520-0450(2001)040h0479:OTUORDi2.0.CO;2, 2001.
Matsui, T., Dolan, B., Rutledge, S. A., Tao, W.-K., Iguchi, T., Barnum, J., and Lang, S. E.: POLARRIS: A POLArimetric Radar Retrieval and Instrument Simulator, J. Geophys. Res.-Atmos., 124, 4634–4657, https://doi.org/10.1029/2018JD028317, 2019.
Mellado, J. P., Stevens, B., Schmidt, H., and Peters, N.: Buoyancy reversal in cloud-top mixing layers, Q.J.R. Meteorol. Soc., 135, 963–978, https://doi.org/10.1002/qj.417, 2009.
Mendrok, J., Blahak, U., Snyder, J. C., and Carlin, J. T.: Implementation of radar polarimetry into the efficient modular volume scan radar forward operator EMVORADO, in preparation to Geosci. Model Dev., 2021.
Mishchenko, M. I.: Calculation of the amplitude matrix for a nonspherical particle in a fixed orientation, Appl. Opt., 39, 1026–1031, 2000.
Moisseev, D. N., Lautaportti, S., Tyynela, J., and Lim, S.: Dualpolarization radar signatures in snowstorms: Role of snowflake aggregation, J. Geophys. Res.-Atmos., 120, 12644–12655, https://doi.org/10.1002/2015JD023884, 2015.
Morrison, H. and Milbrandt, J. A.: Parameterization of Cloud Microphysics Based on the Prediction of Bulk Ice Particle Properties. Part I: Scheme Description and Idealized Tests, J. Atmos. Sci., 72, 287–311, 2015.
Morrison, H., van Lier-Walqui, M., Fridlind, A. M., Grabowski, W. W., Harrington, J. Y., and Hoose, C., et al.: Confronting the challenge of modeling cloud and precipitation microphysics, J. Adv. Model. Earth Sys., 12, e2019MS001689, https://doi.org/10.1029/2019MS001689, 2020.
Mülmenstädt, J., Sourdeval, O., Delanoë, J., and Quaas, J.: Frequency of occurrence of rain from liquid-, mixed- and ice-phase clouds derived from A-Train satellite retrievals, Geophys. Res. Lett., 42, 6502–6509, https://doi.org/10.1002/2015GL064604, 2015.
Murphy, A. M., Ryzhkov, A., and Zhang, P.: Columnar vertical profile (CVP) methodology for validating polarimetric radar retrievals in ice using in situ aircraft measurements, J. Atmos. Oceanic Technol., 37, 1623–1642, https://doi.org/10.1175/JTECH-D-20-0011.1, 2020.
Myagkov, A., Seifert, P., Bauer-Pfundstein, M., and Wandinger, U.: Cloud radar with hybrid mode towards estimation of shape and orientation of ice crystals, Atmos. Meas. Tech., 9, 469–489, https://doi.org/10.5194/amt-9-469-2016, 2016.
Neggers, R. A.: A dual mass flux framework for boundary layer convection. Part II: Clouds, J. Atmos. Sci., 66, 1489–1506, https://doi.org/10.1175/2008JAS2636.1, 2009.
Dias Neto, J., Kneifel, S., Ori, D., Trömel, S., Handwerker, J., Bohn, B., Hermes, N., Mühlbauer, K., Lenefer, M., and Simmer, C.: The TRIple-frequency and Polarimetric radar Experiment for improving process observations of winter precipitation, Earth Syst. Sci. Data, 11, 845–863, https://doi.org/10.5194/essd-11-845-2019, 2019.
Nguyen, C. M., Wolde, M., and Korolev, A.: Determination of ice water content (IWC) in tropical convective clouds from X-band dual-polarization airborne radar, Atmos. Meas. Tech., 12, 5897–5911, https://doi.org/10.5194/amt-12-5897-2019, 2019.
Ori, D., Schemann, V., Karrer, M., Dias Neto, J., von Terzi, L., Seifert, A., and Kneifel, S.: Evaluation of ice particle growth in ICON using statistics of multi-frequency Doppler cloud radar observations, Q. J. Roy. Meteor. Soc., 146, 3830–3849, https://doi.org/10.1002/qj.3875, 2020.
Oue, M., Tatarevic, A., Kollias, P., Wang, D., Yu, K., and Vogelmann, A. M.: The Cloud-resolving model Radar SIMulator (CR-SIM) Version 3.3: description and applications of a virtual observatory, Geosci. Model Dev., 13, 1975–1998, https://doi.org/10.5194/gmd-13-1975-2020, 2020.
Oue, M., Kollias, P., Ryzhkov, A., and Luke, E. P.: Toward exploring the synergy between cloud radar polarimetry and Doppler spectral analysis in deep cold precipitating systems in the Arctic, J. Geophys. Res.-Atmos., 123, 2797–2815, https://doi.org/10.1002/2017JD027717, 2018.
Phillips, V. T. J., Yano, J., and Khain, A.: Ice Multiplication by Breakup in Ice–Ice Collisions, Part I: Theoretical Formulation, J. Atmos. Sci., 74, 1705–1719, 2017.
Pfitzenmaier, L., Unal, C. M. H., Dufournet, Y., and Russchenberg, H. W. J.: Observing ice particle growth along fall streaks in mixed-phase clouds using spectral polarimetric radar data, Atmos. Chem. Phys., 18, 7843–7862, https://doi.org/10.5194/acp-18-7843-2018, 2018.
Pincus, R. and Klein, S.: Unresolved spatial variability and microphysical process rates in large-scale models, J. Geophys. Res., 105, 27059–27065, 2000.
Putnam, B., Xue, M., Jung, Y., Snook, N., and Zhang, G.: Ensemble Kalman Filter Assimilation of Polarimetric Radar Observations for the 20 May 2013 Oklahoma Tornadic Supercell Case, Mon. Weather Rev., 147, 2511–2533, https://doi.org/10.1175/MWR-D-18-0251.1, 2019.
Radenz, M., Bühl, J., Seifert, P., Baars, H., Engelmann, R., Barja González, B., Mamouri, R.-E., Zamorano, F., and Ansmann, A.: Hemispheric contrasts in ice formation in stratiform mixed-phase clouds: Disentangling the role of aerosol and dynamics with ground-based remote sensing, Atmos. Chem. Phys. Discuss. [preprint], https://doi.org/10.5194/acp-2021-360, in review, 2021.
Reimann, L., Simmer, C., and Trömel, S.: Dual-polarimetric radar estimators of liquid water content over Germany, Meteorol. Z., 30, 237–249, https://doi.org/10.1127/metz/2021/1072, 2021.
Ribaud, J.-F., Machado, L. A. T., and Biscaro, T.: X-band dual-polarization radar-based hydrometeor classification for Brazilian tropical precipitation systems, Atmos. Meas. Tech., 12, 811–837, https://doi.org/10.5194/amt-12-811-2019, 2019.
Rosch, J., Heus, T., Brueck, M., Salzmann, M., Mülmenstädt, J., Schlemmer, L., Quaas, J.: Analysis of diagnostic climate model cloud parameterisations using large-eddy simulations, Q. J. R. Meteorol. Soc., 141, 2199–2205, https://doi.org/10.1002/qj.2515, 2015.
Rotstayn, L. D.: On the tuning of autoconversion parameterizations in climate models, J. Geophys. Res., 105, 15495–15507, 2000.
Ryzhkov, A. V., Zrnic, D. S., and Gordon, B. A.: Polarimetric Method for Ice Water Content Determination, J. Appl. Meteor. Clim., 37, 125–134, 1998.
Ryzhkov, A., Pinsky, M., Pokrovsky, A., and Khain, A.: Polarimetric Radar Observation Operator for a Cloud Model with Spectral Microphysics, J. Appl. Meteor. Clim., 50, 873–894, 2011.
Ryzhkov, A., Zhang, P., Reeves, H., Kumjian, M., Tschallener, T., Trömel, S., and Simmer, C.: Quasi-vertical profiles – a new way to look at polarimetric radar data, J. Atmos. Oceanic Technol., 33, 551–562, https://doi.org/10.1175/JTECH-D-15-0020.1, 2016.
Ryzhkov, A., Bukovcic, P., Murphy, A., Zhang, P., and McFarquhar, G.: Ice Microphysical Retrievals Using Polarimetric Radar Data, in: Proceedings of the 10th European Conference on Radar in Meteorology and Hydrology, Ede, The Netherlands, 1–6 July 2018.
Ryzhkov, A. and Zrnic, D.: Radar Polarimetry for Weather Observations, Springer Atmospheric Sciences, 486 pp., 2019.
Schinagl, K., Friederichs, P., Trömel, S., and Simmer, C.: Gamma Drop Size Distribution Assumptions in Bulk Model Parameterizations and Radar Polarimetry and Their Impact on Polarimetric Radar Moments, J. Appl. Meteor. Clim., 58, 467–478, https://doi.org/10.1175/JAMC-D-18-0178.1, 2019.
Schrom, R. S. and Kumjian, M. R.: Bulk-Density Representations of Branched Planar Ice Crystals: Errors in the Polarimetric Radar Variables, J. Appl. Meteor. Clim., 57, 333–346, 2018.
Seifert, A. and Beheng, K. D.: A two-moment cloud microphysics parameterization for mixed-phase clouds, Part 1: Model description, Meteorol. Atmos. Phys., 92, 45–66, https://doi.org/10.1007/s00703-005-0112-4, 2006.
Shrestha, P., Sulis, M., Masbou, M., Kollet, S., and Simmer, C: A scale-consistent Terrestrial System Modeling Platform based on COSMO, CLM and ParFlow, Mon. Weather Rev., 142, 3466–3483, https://doi.org/10.1175/MWR-D-14-00029.1, 2014.
Shrestha, P.: Clouds and vegetation modulate shallow groundwater table depth, 22, 753–763, https://doi.org/10.1175/JHM-D-20-0171.1, 2021.
Shrestha, P., Trömel, S., Evaristo, R., and Simmer, C.: Evaluation of modeled summertime convective storms using polarimetric radar observations, Atmos. Chem. Phys. Discuss. [preprint], https://doi.org/10.5194/acp-2021-404, in review, 2021a.
Shrestha, P., Mendrok, J., Pejcic, V., Trömel, S., Blahak, U., and Carlin, J. T.: Evaluation of the COSMO model (v5.1) in polarimetric radar space – Impact of uncertainties in model microphysics, retrievals, and forward operator, Geosci. Model Dev. Discuss. [preprint], https://doi.org/10.5194/gmd-2021-188, in review, 2021b.
Shupe, M. D., Kollias, P., Matrosov, S. Y., and Schneider, T. L.: Deriving mixed-phase cloud properties from Doppler radar spectra, J. Atmos. Ocean. Technol., 21, 660–670, 2004.
Simmel, M., Bühl, J., Ansmann, A., and Tegen, I.: Ice phase in altocumulus clouds over Leipzig: remote sensing observations and detailed modeling, Atmos. Chem. Phys., 15, 10453–10470, https://doi.org/10.5194/acp-15-10453-2015, 2015.
Simmer, C., Thiele-Eich, I., Masbou, M., Amelung, W., Crewell, S., Diekkrueger, B., Ewert, F., Hendricks Franssen, H.-J., Huisman, A. J., Kemna, A., Klitzsch, N., Kollet, S., Langensiepen, M., Löhnert, U., Rahman, M., Rascher, U., Schneider, K., Schween, J., Shao, Y., Shrestha, P., Stiebler, M., Sulis, M., Vanderborght, J., Vereecken, H., van der Kruk, J., Zerenner, T., and Waldhoff, G.: Monitoring and Modeling the Terrestrial System from Pores to Catchments – the Transregional Collaborative Research Center on Patterns in the Soil-Vegetation-Atmosphere System, B. Am. Meteorol. Soc., 96, 1765–1787, https://doi.org/10.1175/BAMS-D-13-00134.1, 2015.
Simmer, C., Adrian, G., Jones, S., Wirth, V., Goeber, M., Hohenegger, C., Janjic, T., Keller, J., Ohlwein, C., Seifert, A., Trömel, S., Ulbrich, T., Wapler, K., Weissmann, M., Keller, J., Masbou, M., Meilinger, S., Riss, N., Schomburg, A., Vormann, A., and Weingaertner, C.: HErZ – The German Hans-Ertel Centre for Weather Research, B. Am. Meteorol. Soc., 97, 1057–1068, doi:10.1175/BAMS-D-13-00227.1, 2014.
Smith, R. N.: A scheme for predicting layer clouds and their water content in a general circulation model, Q. J. R. Meteorol. Soc., 116, 435–460, https://doi.org/10.1002/qj.49711649210, 1990.
Snyder, J. C., Ryzhkov, A. V., Kumjian, M. R., Khain, A. P., and Picca, J. C.: A ZDR column detection algorithm to examine convective storm updrafts, Weather Forecast., 30, 1819–1844, 2015.
Sommeria, G. and Deardorff, J. W.: Subgrid-scale condensation models of non-precipitating clouds, J. Atmos. Sci., 34, 344–355, 1977.
Sourdeval, O., Gryspeerdt, E., Krämer, M., Goren, T., Delanoë, J., Afchine, A., Hemmer, F., and Quaas, J.: Ice crystal number concentration estimates from lidar–radar satellite remote sensing – Part 1: Method and evaluation, Atmos. Chem. Phys., 18, 14327–14350, https://doi.org/10.5194/acp-18-14327-2018, 2018.
Spek, A. L. J., Unal, C. M. H., Moisseev, C. N., Russchenberg, H. W. J., Chandrasekar, V., and Dufournet, Y.: A New Techniques to Categorize and Retrieve the Microphysical Properties of Ice Particles above the Melting Layer Using Radar Dual-Polarization Spectral Analysis, Jtech, https://doi.org/10.1175/2007JTECHA944.1, 2008.
Stevens, B., Acquistapace, C., Hansen, A., Heinze, R., Klinger, C., Klocke, D., Schubotz, W., Windmiller, J., Adamidis, P., Arka, I., Barlakas, V., Biercamp, J., Brueck, M., Brune, S., Buehler, S., Burkhardt, U., Cioni, G., Costa-Surós, M., Crewell, S., Crueger, T., Deneke, H., Friederichs, P., Carbajal Henken, C., Hohenegger, C., Jacob, M., Jakub, F., Kalthoff, N., Köhler, M., Van Laar, T. W., Li, P., Löhnert, U., Macke, A., Madenach, N., Mayer, B., Nam, C., Naumann, A. K., Peters, K., Poll, S. , Quaas, J., Röber, N., Rochetin, N., Rybka, H., Scheck, L., Schemann, V., Schnitt, S., Seifert, A., Senf, F., Shapkalijevski, M., Simmer, C., Singh, S., Sourdeval, O., Spickermann, D., Strandgren, J., Tessiot, O., Vercauteren, N., Vial, J., Voigt, A., and Zängl, G.: Large-eddy and storm resolving models for climate prediction – the added value for clouds and precipitation, J. Meteorol. Soc. Jpn, 98, 395–435, https://doi.org/10.2151/jmsj2020-021, 2020.
Stevens, B., Giorgetta, M., Esch, M., Mauritsen, T., Crueger, T., Rast, S., Salzmann, M., Schmidt, H., Bader, J., Block, K., Brokopf, R., Fast, I., Kinne, S., Kornblueh, L., Lohmann, U., Pincus, R., Reichler, T., Roeckner, E.: Atmospheric component of the MPI-M Earth System Model: ECHAM6, J. Adv. Model. Earth Syst., 5, 146–172, https://doi.org/10.1002/jame.20015, 2013.
Stevens, B. and Feingold, G.: Untangling Aerosol Effects on Clouds and Precipitation in a Buffered System, Nature, 461, 607–613, 2009.
Sundqvist, H., Berge, E., and Kristjánsson, J. E.: Condensation and cloud parameterization studies with a mesoscale numerical weather prediction model, Mon. Weather Rev., 117, 1641–1657, 1989.
Takahashi, T.: High ice crystal production in winter cumuli over the Japan Sea, Geophys. Res. Lett., 20, 451–454, 1993.
Takahashi, T., Yoshihiro, N., and Yuzuru, K.: Possible high ice particle production during graupel–graupel collisions, J. Atmos. Sci., 52, 4523–4527, 1995.
Takahashi, T.: Influence of liquid water content and temperature on the form and growth of branched planar snow crystals in a cloud, J. Atmos. Sci., 71, 4127–4142, 2014.
Tiedtke, M.: Representation of clouds in large scale models, Mon. Weather Rev., 121, 3040–3061, 1993.
Tompkins, A.: A prognostic parameterization for the subgrid-scale variability of water vapor and clouds in large-scale models and its use to diagnose cloud cover, J. Atmos. Sci., 59, 1917–1942, 2002.
Trömel, S., Quaas, J., Crewell, S., Bott, A., and Simmer, C.: Polarimetric Radar Observations Meet Atmospheric Modelling, 19th International Radar Symposium (IRS), Bonn, https://doi.org/10.23919/IRS.2018.8448121, 2018.
Trömel, S., Ryzhkov, A. V., Hickman, B., Mühlbauer, K., and Simmer, C.: Polarimetric Radar Variables in the Layers of Melting and Dendritic Growth at X Band – Implications for a Nowcasting Strategy in Stratiform Rain, J. Appl. Meteor. Climatol., 58, 2497–2522, https://doi.org/10.1175/JAMC-D-19-0056.1, 2019.
Trömel, S., Ryzhkov, A. V., Zhang, P., and Simmer, C.: The microphysical information of backscatter differential phase δ in the melting layer, J. Appl. Meteor. Climatol., 53, 2344–2359, 2014.
Verlinde, J., Rambukkange, M. P., Clothiaux, E. E., McFarquhar, G. M., and Eloranta, E. W.: Arctic multilayered, mixed-phase cloud processes revealed in millimeter-wave cloud radar Doppler spectra, J. Geophys. Res.-Atmos., 118, 13199–13213, https://doi.org/10.1002/2013JD020183, 2013.
Vogl, T., Maahn, M., Kneifel, S., Schimmel, W., Moisseev, D., and Kalesse-Los, H.: Using artificial neural networks to predict riming from Doppler cloud radar observations, Atmos. Meas. Tech. Discuss. [preprint], https://doi.org/10.5194/amt-2021-137, in review, 2021.
Voigt, C., Schumann, U., Jurkat, T., Schäuble, D., Schlager, H., Petzold, A., Gayet, J.-F., Krämer, M., Schneider, J., Borrmann, S., Schmale, J., Jessberger, P., Hamburger, T., Lichtenstern, M., Scheibe, M., Gourbeyre, C., Meyer, J., Kübbeler, M., Frey, W., Kalesse, H., Butler, T., Lawrence, M. G., Holzäpfel, F., Arnold, F., Wendisch, M., Döpelheuer, A., Gottschaldt, K., Baumann, R., Zöger, M., Sölch, I., Rautenhaus, M., and Dörnbrack, A.: In-situ observations of young contrails – overview and selected results from the CONCERT campaign, Atmos. Chem. Phys., 10, 9039–9056, https://doi.org/10.5194/acp-10-9039-2010, 2010.
Voigt, C., Jessberger, P., Jurkat, T., Kaufmann, S., Baumann, R., Schlager, H., Bobrowski, N., Giuffrida, G., Salerno, G.: Evolution of CO2, SO2, HCl and HNO3 in the volcanic plumes from Etna, Geophys. Res. Lett., 41, 6, 2196-2203, https://doi.org/10.1002/2013GL058974, 2014.
Voigt, C., Schumann, U., Minikin, A., Abdelmonem, A., Afchine, A., Borrmann, S., Boettcher, M., Buchholz, B., Bugliaro, L., Costa, A., Curtius, J., Dollner, M., Dörnbrack, A., Dreiling, V., Ebert, V., Ehrlich, A., Fix, A., Forster, L., Frank, F., Fütterer, D., Giez, A., Graf, K., Grooß, J.-U., Groß, S., Heimerl, K., Heinold, B., Hüneke, T., Järvinen, E., Jurkat, T., Kaufmann, S., Kenntner, M., Klingebiel, M., Klimach, T., Kohl, R., Krämer, M., Krisna, T. C., Luebke, A., Mayer, B., Mertes, S., Molleker, S., Petzold, A., Pfeilsticker, K., Port, M., Rapp, M., Reutter, P., Rolf, C., Rose, D., Sauer, D., Schäfler, A., Schlage, R., Schnaiter, M., Schneider, J., Spelten, N., Spichtinger, P., Stock, P., Walser, A., Weigel, R., Weinzierl, B., Wendisch, M., Werner, F., Wernli, H., Wirth, M., Zahn, A., Ziereis, H., and Zöger, M.: ML-CIRRUS – The airborne experiment on natural cirrus and contrail cirrus with the high-altitude long-range research aircraft HALO, B. Am. Meteorol. Soc., 271–288, https://doi.org/10.1175/BAMS-D-15-00213.1, 2017.
Voigt, C., Lelieveld, J. , Schlager, H., Schneider, J., Sauer, D., Meerkötter, R., Pöhlker, M., Bugliaro, L., Curtius, J., Erbertseder, T., Hahn, V., Jöckel, P., Li, Q., Marsing, A., Mertens, M., Pöhlker, C., Pöschl, U., Pozzer, A., Tomsche, L., and Schumann, U.: Aerosol and Cloud Changes during the Corona Lockdown in 2020 – First highlights from the BLUESKY campaign; EGU21-13134, available at: https://meetingorganizer.copernicus.org/EGU21/session/40818, 2021.
Wang, M., Zhao, K., Pan, Y., and Xue, M.: Evaluation of simulated drop size distributions and microphysical processes using polarimetric radar observations for landfalling Typhoon Matmo (2014), J. Geophys. Res.-Atmos., 125, 1–20, https://doi.org/10.1029/2019JD031527, 2020.
Weissmann, M., M. Göber, C., Hohenegger, T., Janjic, J., Keller, C., Ohlwein, A., Seifert, S., Trömel, T., Ulbrich, K., Wapler, C., Bollmeyer, H., and Denke, H.: The Hans-Ertel Centre for Weather Research – Research objectives and highlights from its first three years. Meteorol. Z., 23, 193–208, 2014.
Wendisch, M., Pöschl, U., Andreae, M. O., Machado, L. A. T., Albrecht, R., Schlager, H., Rosenfeld, D., Martin, S. T., Abdelmonem, A., Afchine, A., Araùjo, A. C., Artaxo, P., Aufmhoff, H., Barbosa, H. M. J., Borrmann, S., Braga, R., Buchholz, B., Cecchini, M. A., Costa, A., Curtius, J., Dollner, M., Dorf, M., Dreiling, V., Ebert, V., Ehrlich, A., Ewald, F., Fisch, G., Fix, A., Frank, F., Fütterer, D., Heckl, C., Heidelberg, F., Hüneke, T., Jäkel, E., Järvinen, E., Jurkat, T., Kanter, S., Kästner, U., Kenntner, M., Kesselmeier, J., Klimach, T., Knecht, M., Kohl, R., Kölling, T., Krämer, M., Krüger, M., Krisna, T. C., Lavric, J. V., Longo, K., Mahnke, C., Manzi, A. O., Mayer, B., Mertes, S., Minikin, A., Molleker, S., Münch, S., Nillius, B., Pfeilsticker, K., Pöhlker, C., Roiger, A., Rose, D., Rosenow, D., Sauer, D., Schnaiter, M., Schneider, J., Schulz, C., de Souza, R. A. F., Spanu, A., Stock, P., Vila, D., Voigt, C., Walser, A., Walter, D., Weigel, R., Weinzierl, B., Werner, F., Yamasoe, M. A., Ziereis, H., Zinner, T., and Zöger, M.: ACRIDICON–CHUVA Campaign: Studying Tropical Deep Convective Clouds and Precipitation over Amazonia Using the New German Research Aircraft HALO, B. Am. Meteorol. Soc., 97, 1885–1908, 2016.
Wolfensberger, D. and Berne, A.: From model to radar variables: a new forward polarimetric radar operator for COSMO, Atmos. Meas. Tech., 11, 3883–3916, https://doi.org/10.5194/amt-11-3883-2018, 2018.
Xie, X., Evaristo, R., Trömel, S., Saavedra, P., Simmer, C., and Ryzhkov, A.: Radar Observation of Evaporation and Implications for Quantitative Precipitation and Cooling Rate Estimation, J. Atmos. Ocean. Technol., 33, 1779–1792, https://doi.org/10.1175/JTECH-D-15-0244.1, 2016.
Xie, X., Shrestha, P., Mendrok, J., Carlin, J., Trömel, S., and Blahak, U.: Bonn Polarimetric Radar forward Operator (B-PRO), CRC/TR32 Database (TR32DB), https://doi.org/10.5880/TR32DB.41, 2021.
Xue, L., Fan, J., Lebo, Z. J., Wu, W., Morrison, H., Grabowski, W. W., Chu, X., Geresdi, I., North, K., Stenz, R., Gao, Y., Lou, X., Bansemer, A., Heymsfield, A. J., McFarquhar, G. M., and Rasmussen, R. M.: Idealized Simulations of a Squall Line from the MC3E Field Campaign Applying Three Bin Microphysics Schemes: Dynamic and Thermodynamic Structure, Mon. Weather Rev., 145, 4789–4812, https://doi.org/10.1175/MWR-D-16-0385.1, 2017.
You, C.-R., Chung, K.-S., and Tsai, C.-C.: Evaluating the performance of convection-permitting model by using dual-polarimetric radar parameters: Case study of SoWMEX IOP8, Remote Sens., 12, 1–25, https://doi.org/10.3390/rs12183004, 2020.
Zängl, G., Reinert, D., Rípodas, P., and Baldauf, M.: The ICON (icosahedral non-hydrostatic) modelling framework of DWD and MPI-M: Description of the non-hydrostatic dynamical core, Q. J. Roy. Meteor. Soc., 141, 563–579, 2015.
Zeng, Y., Janjic, T., Lozar, A. de, Welzbacher, C. A., Blahak, U., and Seifert, A.: Assimilating radar radial wind and reflectivity data in an idealized setup of the COSMO-KENDA system, Atmos. Res., 249, 105282, https://doi.org/10.1016/j.atmosres.2020.105282, 2021a.
Zeng, Y., Janjic, T., Feng, Y., Blahak, U., de Lozar, A., Bauernschubert, E., Stephan, K., and Min, J.: Interpreting estimated observation error statistics of weather radar measurements using the ICON-LAM-KENDA system, Atmos. Meas. Tech., 14, 5735–5756, https://doi.org/10.5194/amt-14-5735-2021, 2021b.
Zeng, Y., Blahak, U., and Jerger, D.: An efficient modular volume-scanning radar forward operator for NWP models: description and coupling to the COSMO model, Q. J. Roy. Meteor. Soc., 142, 3234–3256, 2016.
Zeng, Y., Janjic, T., Lozar, A. de, Blahak, U., Reich, H., Keil, C., and Seifert, A.: Representation of model error in convective-scale data assimilation: Additive noise, relaxation methods and combinations, J. Adv. Model. Earth Sy., 10, 2889–2911, 2018.
Zeng, Y., Janjic, T., Sommer, M., Lozar, A. de, Blahak, U., and Seifert, A.: Representation of model error in convective-scale data assimilation: additive noise based on model truncation error, J. Adv. Model. Earth Sy., 11, 752–770, 2019.
Zeng, Y., Janjic, T., Lozar, A. de, Rasp, S., Blahak, U., Seifert, A., and Craig, G. C.: Comparison of methods accounting for subgrid-scale model error in convective-scale data assimilation, Mon. Weather Rev., 148, 2457–2477, 2020.
Zhu, K., Xue, M., Ouyang, K., and Jung, Y.: Assimilating polarimetric radar data with an ensemble Kalman filter: OSSEs with a tornadic supercell storm simulated with a two-moment microphysics scheme, Q. J. Roy. Meteor. Soc., 146, 1880–1900, https://doi.org/10.1002/qj.3772, 2020.
- Abstract
- Introduction and objectives of the priority programme
- Representation of clouds in atmospheric models
- Observational insights from polarimetric radar observations and challenges
- Fusion of radar polarimetry and atmospheric models
- Summary and perspectives
- Data availability
- Author contributions
- Competing interests
- Disclaimer
- Special issue statement
- Acknowledgements
- Financial support
- Review statement
- References
- Abstract
- Introduction and objectives of the priority programme
- Representation of clouds in atmospheric models
- Observational insights from polarimetric radar observations and challenges
- Fusion of radar polarimetry and atmospheric models
- Summary and perspectives
- Data availability
- Author contributions
- Competing interests
- Disclaimer
- Special issue statement
- Acknowledgements
- Financial support
- Review statement
- References