the Creative Commons Attribution 4.0 License.
the Creative Commons Attribution 4.0 License.
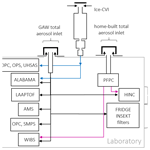
Sources and nature of ice-nucleating particles in the free troposphere at Jungfraujoch in winter 2017
Hans-Christian Clemen
Xiaoli Shen
Stephan Mertes
Martin Gysel-Beer
Alireza Moallemi
Martin Steinbacher
Stephan Henne
Harald Saathoff
Ottmar Möhler
Kristina Höhler
Thea Schiebel
Daniel Weber
Jann Schrod
Johannes Schneider
Primary ice formation in mixed-phase clouds is initiated by a minute subset of the ambient aerosol population, called ice-nucleating particles (INPs). The knowledge about their atmospheric concentration, composition, and source in cloud-relevant environments is still limited. During the 2017 joint INUIT/CLACE (Ice Nuclei research UnIT/CLoud–Aerosol Characterization Experiment) field campaign, observations of INPs as well as of aerosol physical and chemical properties were performed, complemented by source region modeling. This aimed at investigating the nature and sources of INPs. The campaign took place at the High-Altitude Research Station Jungfraujoch (JFJ), a location where mixed-phase clouds frequently occur. Due to its altitude of 3580 m a.s.l., the station is usually located in the lower free troposphere, but it can also receive air masses from terrestrial and marine sources via long-range transport. INP concentrations were quasi-continuously detected with the Horizontal Ice Nucleation Chamber (HINC) under conditions representing the formation of mixed-phase clouds at −31 ∘C. The INP measurements were performed in parallel to aerosol measurements from two single-particle mass spectrometers, the Aircraft-based Laser ABlation Aerosol MAss Spectrometer (ALABAMA) and the laser ablation aerosol particle time-of-flight mass spectrometer (LAAPTOF). The chemical identity of INPs is inferred by correlating the time series of ion signals measured by the mass spectrometers with the time series of INP measurements. Moreover, our results are complemented by the direct analysis of ice particle residuals (IPRs) by using an ice-selective inlet (Ice-CVI) coupled with the ALABAMA. Mineral dust particles and aged sea spray particles showed the highest correlations with the INP time series. Their role as INPs is further supported by source emission sensitivity analysis using atmospheric transport modeling, which confirmed that air masses were advected from the Sahara and marine environments during times of elevated INP concentrations and ice-active surface site densities. Indeed, the IPR analysis showed that, by number, mineral dust particles dominated the IPR composition (∼58 %), and biological and metallic particles are also found to a smaller extent (∼10 % each). Sea spray particles are also found as IPRs (17 %), and their fraction in the IPRs strongly varied according to the increased presence of small IPRs, which is likely due to an impact from secondary ice crystal formation. This study shows the capability of combining INP concentration measurements with chemical characterization of aerosol particles using single-particle mass spectrometry, source region modeling, and analysis of ice residuals in an environment directly relevant for mixed-phase cloud formation.
- Article
(3825 KB) - Full-text XML
-
Supplement
(4433 KB) - BibTeX
- EndNote
Ice-nucleating particles (INPs) are a rare subset of the ambient aerosol particle population (e.g., Rogers et al., 1998; DeMott et al., 2010) and are an important atmospheric constituent since they can modulate the microphysical properties of cirrus and mixed-phase clouds (MPCs) by initiating ice crystal formation. INPs have the ability to change the sensitive balance between the liquid and ice water content of MPCs, which can lead to rapid cloud glaciation and associated dissipation (e.g., Lohmann et al., 2002; Sassen et al., 2003; Fu et al., 2017; Desai et al., 2019), or to cloud brightening and related changes in the radiative properties (Solomon et al., 2018). Moreover, precipitation is formed efficiently via the ice phase (Pruppacher and Klett, 1997; Mülmenstädt et al., 2015; Field and Heymsfield, 2015; Heymsfield et al., 2020). In the absence of efficient secondary ice formation processes (Korolev and Leisner, 2020), ice formation induced by INPs is directly relevant for the Earth's radiation and water budget, and even relevant for the initiation of secondary ice processes. Despite their importance, the knowledge about the abundance and nature of INPs in the atmosphere still needs to be improved, in part because of their low ambient concentration and high spatiotemporal variability. Ambient INP concentrations were found to range between less than 10−5 and greater than 103 stdL−1 between −10 and −35 ∘C, respectively (Kanji et al., 2017). While their ambient concentration is a strong function of ice nucleation temperature, variations over several orders of magnitude are reported at any one temperature, implying the importance of the ice nucleation ability of individual aerosol particle types. Depending on temperature range as well as atmospheric abundance, several aerosol particle types might dominate the INP population. Mineral dust is recognized as a key ice nucleator in the troposphere at temperatures below −15 ∘C (DeMott et al., 2003a; Cziczo et al., 2003; Richardson et al., 2007; Chou et al., 2011; Atkinson et al., 2013; Tang et al., 2016; Boose et al., 2016a, b; Jiang et al., 2016; Kanji et al., 2017; Price et al., 2018; Welti et al., 2018). However, in the absence of highly ice-active material or at warmer temperatures, other aerosol particle types are important. Aerosol particles emitted from the ocean were identified to be ice active at temperatures below −5 ∘C (e.g., Brier and Kline, 1959; Bigg, 1973; Schnell, 1977; Wilson et al., 2015; DeMott et al., 2016; Veragra-Temprado et al., 2017; McCluskey et al., 2018; Si et al., 2018) and showed their potential to impact cloud properties in remote marine environments (Huang et al., 2018; Vergara-Temprado et al., 2018a; McCluskey et al., 2019). Also, primary biological particles might contribute significantly to the INP population, especially at temperatures warmer than −15 ∘C (Pratt et al., 2009; Schmidt et al., 2017; O'Sullivan et al., 2018), but their atmospheric contribution on a global scale is still unclear (Hoose et al., 2010; Burrows et al., 2013; Sesartic et al., 2013). Moreover, metallic particles might act as ambient INPs at MPC conditions (Cziczo et al., 2009; Kamphus et al., 2010; Ebert et al., 2011; Worringen et al., 2015) as well as aerosol particles emitted by anthropogenic sources, such as from combustion processes; however, contradictory results exist (Cozic et al., 2008; Kupiszeswski et al., 2016; Mahrt et al., 2018; Kanji et al., 2020).
Next to laboratory experiments using a specific aerosol particle type representative for ambient conditions, attempts to directly identify INPs in ambient air are made by sampling in air masses which are dominated by one aerosol particle type, such as Saharan dust particles (DeMott et al., 2015; Boose et al., 2016a), marine aerosols (e.g., Bigg, 1973; McCluskey et al., 2018; Irish et al., 2019; Ladino et al., 2019), biological aerosols (e.g., Huffman et al., 2013; Mason et al., 2015), or aerosols from anthropogenic sources in an urban environment (e.g., Chen et al., 2018). Nevertheless, this approach is limited since it is not guaranteed that just a single aerosol particle type is present in ambient air and at relevant concentrations, which is crucial in light of the very low fraction of ice-active particles. An indirect approach is the investigation of relationships between INP concentrations and parallel-measured parameters of aerosol particle and air mass properties (e.g., Mason et al., 2015; Boose et al., 2016b; Lacher et al., 2018b), which can result in useful parameterizations of INPs (e.g., DeMott et al., 2010). For example, the chemical composition of aerosol particles can be investigated in parallel to INP measurements with a single-particle mass spectrometer (SPMS; Murphy et al., 2007; Chen et al., 2021). In addition, an SPMS together with a pumped counterflow virtual impactor (PCVI; e.g., DeMott et al., 2003b; Hiranuma et al., 2016) can also be connected to a continuous-flow-diffusion chamber (CFDC) and thus directly analyze the activated INPs chemically (e.g., Cziczo et al., 2003; Richardson et al., 2007; Cornwell et al., 2019). The SPMS is a common tool in the field of ice nucleation research (Cziczo et al., 2017), and its use led to an improved understanding of ice formation in clouds (Cziczo et al., 2004, 2013; Pratt et al., 2009; Cziczo and Froyd, 2014; Lin et al., 2017; Cornwell et al., 2019). Another direct method to identify INPs is the application of ice-selective inlets (e.g., Mertes et al., 2007), which sample freshly nucleated ice crystals in clouds and sublimate them, and the resulting initial INPs are analyzed with different methods such as, for example, single-particle mass spectrometry and scanning electron microscopy (Cziczo et al., 2009; Kamphus et al., 2010; Schmidt et al., 2017, Eriksen Hammer et al., 2018).
Here, we present results from an intensive field campaign conducted at a high-altitude site during January and February 2017. A suite of instruments to detect INPs, as well as two SPMSs to characterize aerosol particle composition, were applied in combination with measurements with an ice-selective inlet, aiming at getting better insights into INP characteristics and sources and aerosol particle properties relevant for MPC formation.
2.1 Location and overview of the field campaign
The INUIT/CLACE 2017 (Ice Nuclei research UnIT/CLoud–Aerosol Characterization Experiment) field campaign took place from 22 January–22 February 2017 at the High Altitude Research Station Jungfraujoch (JFJ), as a joint activity of international research groups to study aerosol–cloud interactions (e.g., Eriksen Hammer et al., 2018). Being located in the Swiss Alps at an altitude of 3580 m a.s.l. ( N, E), JFJ is an outstanding location for such a purpose, since it is not only a natural environment for cloud formation and occurrence (e.g., Herrmann et al., 2015), but also because it receives air masses of different origins (Cui et al., 2011). Due to its elevation, the site is usually exposed to the lower free troposphere (>60 % of the time in winter; Herrmann et al., 2015), where the larger fraction of the aerosol particle population (accumulation and coarse mode particles >90 nm; Herrmann et al., 2015) is not formed in situ or from local point sources, but is only present at the site due to long-range transport. This is confirmed by analysis of trace gases such as the ratio of reactive nitrogen species (NOy) to carbon monoxide (CO), which can be used as an indicator for air mass age (Zellweger et al., 2003). Therefore, JFJ is a site representative for detecting particles from various source regions being transported within the free troposphere. However, the aerosol particle measurements can be impacted in the short term by daytime tourist activities (Bukowiecki et al., 2021). Air masses that originate from the Sahara frequently reach the site, which results in strongly elevated mineral dust loads (Collaud Coen et al., 2004; Chou et al., 2011; Boose et al., 2016a; Lacher et al., 2018a). Also, air masses originating in the marine boundary layer reach JFJ (Cui et al., 2011; Lacher et al., 2018a). Particularly in the warm season, convectively lifted boundary layer air can reach the site (e.g., Collaud Coen et al., 2011), injecting air masses from local and regional sources such as, for example, forests or anthropogenically polluted environments. Hence, at JFJ a large variety of aerosol particle types can be found, ranging from relatively fresh emissions from the polluted boundary layer (typically in the summer) to aged aerosol particles from different regions as transported in the free troposphere over large distances. This site is therefore ideal to study the impact of different aerosol populations on cloud properties.
JFJ is part of the Global Atmospheric Watch (GAW) monitoring program as well as of the Aerosol, Clouds and Trace Gases Research Infrastructure (ACTRIS), the Swiss National Air Pollution Monitoring Network (NABEL), and the SwissMetNet meteorological network. As such, important parameters of aerosol particle physical properties (Baltensperger et al., 1997; Herrmann et al., 2015; Bukowiecki et al., 2016) as well as trace gases are routinely monitored (Steinbacher et al., 2020) and accompanied by measurements of meteorological parameters (Appenzeller et al., 2008). Some of the routine observations like the NOy to CO ratio and the wind direction are used for the interpretation of the results below. One important parameter is the aerosol particle size distribution, which is measured using a scanning mobility particle sizer (SMPS; 0.02–0.6 µm), consisting of a differential mobility analyzer (DMA; TSI 3071, TSI Inc. Shoreview, USA) and a condensation particle counter (CPC; TSI 3775, TSI Inc. Shoreview, USA), and an optical particle sizer (OPS; 0.3–10 µm; TSI 3300, TSI Inc. Shoreview, USA). Aerosol particle measurements are conducted at the GAW total aerosol inlet (Weingartner et al., 1999), as well as at an ice selective inlet (Ice-CVI; Mertes et al., 2007) as depicted in Fig. 1 and described in more detail in the following sections.
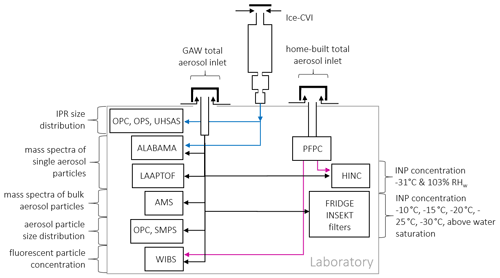
Figure 1Setup of instruments at the Sphinx observatory at JFJ. The instruments were mostly sampling via the GAW total aerosol inlet (black line). The aerosol particle chemical composition was measured with ALABAMA, the LAAPTOF, and an AMS; aerosol particle size was detected using an OPC and a SMPS; fluorescent biological particles were measured with a WIBS; online INP concentrations were measured with HINC and offline with INSEKT and FRIDGE. HINC and WIBS measurements were alternated at the GAW and a home-built total aerosol inlet (black line) connected to the portable fine particle concentrator (magenta line). Measurements of IPR were performed at the Ice-CVI inlet (blue line) for IPR size (using an OPC, OPS, and UHSAS) and for chemical composition (using ALABAMA).
2.2 INP measurements
2.2.1 Online INP measurements
The number concentration of INPs ([INP]) was determined using the Horizontal Ice Nucleation Chamber (HINC; Lacher et al., 2017) at −31 ∘C (±0.4 ∘C; [INP]−31) and at a relative humidity with respect to water (RHw) of 103 % (±2 %), representing condensation and/or immersion freezing, relevant for the formation of MPCs (e.g., DeBoer et al., 2011; Murray et al., 2012; DeMott et al., 2015, 2018). A nucleation temperature of −31 ∘C was chosen in order to avoid measurements below the limit of detection (LOD) of the instrument. This is crucial at a remote location such as JFJ where the [INP] is naturally low but is generally higher at colder nucleation temperatures. Moreover, a nucleation temperature of −31 ∘C ensures that a large spectrum of INP activation at MPC conditions is covered. The RHw of 103 % ensures that the entire aerosol layer, which experiences a varying RH between 101 %–103 %, is above water saturation such that the particles can activate into droplets in the given residence time of HINC. Cloud-like temperature and saturation conditions in HINC are established by applying a temperature gradient along ice-coated parallel plates, held at sub-zero temperatures. INPs are sampled into the chamber guided within a sheath flow and exposed to the temperature and supersaturation in the center of the chamber and can activate into water droplets and/or ice crystals. Due to particle losses in the system, mainly due to the tubing upstream of the chamber and its horizontal orientation, the measurements are representative for aerosol particles <2 µm (56 % transmission efficiency at this size), which is the dominant size range for the aerosols present at the site (Baltensperger et al., 1997; Nyeki et al., 1998). We note that in this way some of the larger (>2 µm) particles may not be detected. For more details on the transmission efficiency see Lacher et al. (2017). [INP]−31 was measured with a time resolution of 20 min, which is alternated with 10 min of background measurements via a filter to exclude the impact of counting undesired frost particles generated within HINC. The instrument's LOD is thereby calculated based on these background measurements. To achieve better measurement statistics due to the naturally low [INP] in the free troposphere, an aerosol particle concentrator (the portable fine particle concentrator, PFPC; Gute et al., 2019) was deployed upstream of HINC during the field campaign, allowing an enrichment in aerosol particles >0.1 µm. The enrichment is thereby size dependent due to the working principle of the PFPC, with an enrichment factor of ∼10 at particle sizes of 0.3 µm and a maximum enrichment of ∼20 for particles >0.75 µm (Gute et al., 2019). The INP enrichment factors were determined by consecutive measurements on and off the concentrator and showed a large variability between values of 1 and 23, reflecting the variability in the size of the present INP population (see Lacher et al., 2018a, for a more detailed description of this setup). For comparison and correlation with other measurements, only quantifiable [INP]−31 > LOD () was considered for this study. Here we present results from [INP]−31 and its related ice-active surface site density (ns), which normalizes [INP] to the available surface area per volume of air of the ambient aerosol particles (Connolly et al., 2009; Hoose and Möhler, 2012):
given in m−2, and which requires the surface area distribution concentration to be calculated from the number size distribution. Measurements from the GAW SMPS and OPS are used to calculate the available surface area of the aerosol population by assuming a uniform particle shape and that the refractive index of the ambient aerosol population is represented by the calibrated value of the OPS. We acknowledge that this can lead to higher uncertainties, which are not quantified here. The concept of ns is based on the assumption of a uniform composition of the investigated aerosol sample and assumes that the temperature dependence of ice nucleation is more important than the time dependence, which therefore can be neglected (e.g., Welti et al., 2012).
In order to investigate the relationship between [INP]−31 and ns with meteorological and aerosol parameters, we use Spearman's rank correlation coefficient (Spearman, 1904). Spearman's rank correlation determines to what extent two variables are monotonically related by applying a linear correlation analysis to the rank-ordered values of the parameters. As we would not necessarily await a linear relationship amongst parameters in atmospheric science, this test is well suited for our purposes. Examples for the correlation analysis are presented in Fig. S6 in the Supplement.
2.2.2 Offline INP measurements
Filter-based aerosol collection followed by offline quantification of [INP] was performed with the FRankfurt Ice nucleation Deposition freezinG Experiment (FRIDGE; Bundke et al., 2008; Klein et al., 2010; Schrod et al., 2016) and the Ice Nucleation Spectrometer of the Karlsruhe Institute of Technology (INSEKT), which is a re-built version of the ice spectrometer freezing method (Garcia et al., 2012; Hill et al., 2016).
For FRIDGE, the aerosol particles are sampled on silicon wafers using electrostatic precipitation with a time resolution of 1–8 h. After collection, the wafers were analyzed on site in an isostatic diffusion chamber. The sample is first cooled to the desired sub-zero temperature, which is measured with an accuracy of ±0.2 ∘C. Then, the sample is exposed to a defined amount of water vapor (i.e., here corresponding to 101 % RHw), relevant for the formation of MPCs. Subsequently, ice crystals start to grow on INPs, which causes a change in brightness that is detected and recorded by a camera.
The INSEKT samples were collected on precleaned (10 % H2O2 solution followed by ultrapure water) polycarbonate filters with pore sizes of 0.2 µm (Whatman plc, Kent, United Kingdom) with a flow of 9.6 L min−1 for 8 h (daytime) or 12 h (nighttime). The samples were shipped to the laboratory at the Karlsruhe Institute of Technology for analysis. In brief, the collected aerosol particles are washed off the filter with 8 mL of nanopure water, and the resulting suspension is then pipetted into 80 wells of polymerase chain reaction (PCR) plates, with each well having a volume of 50 µL, together with aliquots from the nanopure water used to create the suspension. The samples are then placed into aluminum holders which are cooled using a cooling bath, measuring down to temperatures of ∘C at a 0.25 cooling rate. The frozen wells are detected optically by a camera due to a brightness change in the frozen aliquots. For each experiment, a background correction is applied, taking into account the freezing of the pure water used to create the suspension.
The calculation of the cumulative [INP] is based on the sampled volume during filter collection and follows the equation from Vali (1971). Here, we present [INP] at −10, −15, and −20 ∘C for results from INSEKT and −20, −25, and −30 ∘C for FRIDGE ([INP]−10 to [INP]−30).
2.3 Aerosol composition measurements by mass spectrometry
2.3.1 Instrument description
Aerosol particle composition was measured with three different aerosol mass spectrometers: the laser ablation aerosol particle time-of-flight mass spectrometer (LAAPTOF, AeroMegt GmbH), the Aircraft-based Laser ABlation Aerosol MAss spectrometer (ALABAMA), and a compact time-of-flight aerosol mass spectrometer (C-ToF-AMS).
The LAAPTOF and ALABAMA are based on similar measurement concepts: aerosol particles are transmitted from ambient air into a vacuum chamber through an aerodynamic lens which focuses the particles between 0.07–2.5 µm onto a narrow beam. The particles are then detected by two continuous wave lasers, which allows for measuring the velocity and thereby their vacuum aerodynamic diameter. Particle detection at both detection stages triggers an ablation laser that emits a laser pulse onto the particle, thereby ablating the particle and ionizing its components. The ions are detected in bipolar time-of-flight mass spectrometers. The overall detection efficiency (combining the detection efficiency and the hit rate) of the LAAPTOF is between 0.01 % (±0.01 %) and 4.2 % (±2.4 %), in the size range of 0.2 to 2 µm based on polystyrene latex (PSL) particles. The highest overall detection efficiency is for 1, and the lowest is for 2 µm (Shen et al., 2018). Note that such efficiency is also particle type dependent (Shen et al., 2018, 2019). More details on the LAAPTOF can be found in Gemayel et al. (2016), Reitz et al. (2016), and Shen et al. (2018, 2019). Details on ALABAMA have been presented in Brands et al. (2011), Roth et al. (2016), Schmidt et al. (2017), and Clemen et al. (2020). The detection efficiency of ALABAMA during this campaign was between 40 % and 60 % in the size range of 0.3 to 1.0 µm based on PSL particles. Up to a particle size of about 1.3 µm, the detection efficiency of ALABAMA decreased to less than 30 % and is estimated to be about 5 % (±5 %) for 2 µm. At the same time, the hit rate during those tests using PSL particles was lower, such that the overall detection efficiency for ALABAMA was only between 1 % and 16 % in the size range from 0.3 to 1 µm. As those values are based on measurements using PSL particles, they can vary considerably during field applications; for example, the ALABAMA hit rates were significantly higher than those of the PSL test measurements (which is attributed to particle charge effects during the nebulization of the PSL particles). In light of our research objectives, focusing on the general trend of the aerosol particle composition, we therefore provide an overview of the size-dependent overall detection efficiency from the LAAPTOF and ALABAMA normalized to the maximum value measured, together with the normalized transmission efficiency from HINC (Fig. S1 in the Supplement). From those normalized values it is visible that both SPMSs detect aerosol particles in the same size range, with a maximum between 0.5 and 1 µm, and therefore yield comparable information on the particle composition in this size range. HINC measures particles below 2 µm with a high efficiency, which can have an impact on the comparison between the INP measurements from HINC and the results obtained from ALABAMA and the LAAPTOF.
The data product of both instruments is a bipolar ion signature on a single-particle basis. The main difference between the LAAPTOF and ALABAMA with respect to the chemical analysis is the laser wavelength that is used for ablation. The LAAPTOF uses an ArF excimer laser with a wavelength of 193 nm (pulse duration: 4 to 8 ns), whereas ALABAMA uses a quadrupled Nd:YAG with a wavelength of 266 nm (pulse duration: 6 ns). The laser energy per pulse used during this campaign ranged between 3 and 4 mJ for the LAAPTOF and between 7.2 and 9 mJ for ALABAMA. These differences in laser wavelength and energy result in different ablation and ionization efficiencies that affect the relative ion signals in the particle mass spectra. More specifically, the different lasers lead to different power densities. ALABAMA generates a power density of , with an assumed effective diameter of the laser at the ablation spot of the particles of about 400 µm (Clemen et al., 2020), whereas the LAAPTOF uses a power density of , with a laser beam diameter of 99 (±31) µm at the ablation spot (Ramisetty et al., 2018). The C-ToF-AMS has been described in detail in the literature (e.g., Drewnick et al., 2005; Canagaratna et al., 2007). Its data product is a quantitative mass concentration of non-refractory aerosol compounds, e.g., ammonium, nitrate, sulfate, and organics, in particles with an aerodynamic diameter below 1 µm (PM1), based on averaging over an ensemble of particles. The focus of the analysis presented here lies on the single-particle instruments, because they are able to provide information on both non-refractory and refractory particles indicating the presence of mineral dust, elemental carbon, sea spray, metals, or primary biological particles.
2.3.2 Data analysis
For an overview of the ambient aerosol properties of the whole measurement period, we used a clustering algorithm to infer the dominating aerosol particle type. This was done using the LAAPTOF data, which covered a longer time period. ALABAMA was not operated in optimal configuration in the beginning of the campaign because the extraction voltages for the mass spectrometer had to be optimized after the installation of a so-called delayed ion extractor (see Clemen et al., 2020, for more details). Furthermore, during most of the time when the station was in clouds, ALABAMA was connected to the Ice-CVI (see Sect. 2.6) to sample freshly formed ice particle residuals. The mass spectra recorded by the LAAPTOF were classified by fuzzy c-means clustering method as described in Reitz et al. (2016) and Shen et al. (2018). Briefly, this classification method attributes individual particles to multiple classes or clusters according to spectral similarities, typically calculated by linear correlation or Eulerian distance. The particle classes are attributed to particle types based on marker ions that have been identified in reference spectra of particle types.
To show that both the LAAPTOF and the ALABAMA yield comparable results in spite of the different laser wavelengths and energies for ablation and ionization, cation and anion mass spectra from both instruments averaged over 24 h of parallel sampling are compared (see Fig. S2 in the Supplement). Spearman's rank correlation between both mass spectra yields a correlation coefficient of 0.81 for cations and 0.59 for anions. Although many peaks can be identified in both mass spectra, the relative height of many ion signals is different, due to the different ionization laser energy. In general, this suggests a reasonable agreement in the overall detection of aerosol particles between the two instruments.
To evaluate the chemical information from the single-particle mass spectrometry against the [INP]−31 and ns, we did not use the cluster analysis, because, as explained above, cluster algorithms group mass spectra by similarity. This similarity is calculated by linear correlation or Eulerian distance and is therefore mainly influenced by large ion signals. Thus, small ion signals that might still represent a chemical component that is important for ice nucleation may be overlooked by this method. Instead, we chose to correlate the time series of individual ions with the time series of the [INP]−31 and ns as follows: the HINC INP data represented average values over 20 min. To transpose the mass spectrometer data onto these 20 min intervals, we used two methods. Method 1 counts whether a certain ion is present in a mass spectrum during the 20 min interval (i.e., above the noise threshold) and reports the fraction of mass spectra that contain this ion. For example, a fraction of 0.1 means that 10 % of all mass spectra in this 20 min interval contain this ion. Method 2 averages all mass spectra in this 20 min interval after normalizing the spectra to their total ion signal and reports the relative height of a certain ion in the average mass spectrum. Thus, a fraction of 0.1 means that this ion has a relative signal of 10 % with respect to the total ion signal of the 20 min averaged mass spectrum. Method 1 therefore still contains the single-particle information, reflecting the relative abundance of ions on a particle-by-particle basis, whereas method 2 is more representative of the average composition of all particles during this time interval. We note that the sensitivity of the instruments can vary substantially for different ions, which can have a significant impact on the apparent average composition.
The resulting time series of all ions up to 250 were then correlated to the time series of the [INP]−31 and ns using Spearman's rank correlation. This was done for the positive ions (cations) and for the negative ions (anions) separately. Non-significant rank correlations (p>0.05) were excluded. The remaining rank correlation coefficients (r) for all ions up to 250 were squared (r2) and averaged, and their standard deviation (σ) was calculated. Only ions whose r2 values were greater than 1σ above the mean value were selected as meaningful correlators (see Fig. S3 in the Supplement) to [INP]−31 or ns. To correlate the peaks from the particle spectra with [INP]−31 and ns, we used the non-squared coefficients (r) of the selected ions to allow investigation for negative correlations. We note that the instruments used here for the correlation coefficient analysis have different size-dependent particle transmission efficiencies but overlap in the region <2 µm. While HINC, the LAAPTOF, and ALABAMA have their maximum transmission and detection efficiency for particles <1 µm, HINC has a higher transmission efficiency for larger particles (56 % for 2 µm particles), compared to the LAAPTOF and ALABAMA (∼0.01 % and ∼5 %, respectively). The advantage of the ion correlation method is that it looks at the correlation of chemical substances rather than whole particle types, which means that fewer initial assumptions have to be made and a cross-particle type approach can be taken.
2.4 Fluorescent particles and black carbon
Fluorescent aerosol particles were monitored during the INUIT/CLACE 2017 field campaign using the Wideband Integrated Bioaerosol Sensor (WIBS; model 5/NEO, DMT, Longmont, Colorado, USA) to investigate the potential impact from biological particles. The WIBS detects single particles in the size range of 0.5–20 µm and categorizes them based on their fluorescence and light-scattering properties (e.g., Kaye et al., 2005). Specifically, aerosol size and shape are detected by forward-scattered light from a 635 nm diode laser, and detection of fluorescent particles is achieved by irradiating the particles with UV pulses from two xenon sources at ∼280 and ∼370 nm. Those are the optimal wavelengths in which typical bio-fluorophores are excited (e.g., tryptophan and nicotinamide adenine dinucleotide; Pöhlker et al., 2013). The fluorescence signals are detected using two photomultipliers equipped with bandpass filters to detect signals in the wavebands from 310–400 and 420–650 nm. The combination of two excitation wavelengths and two detection wavebands provides three fluorescence channels (the fourth channel cannot be used due to interference from excitation). Channels 1 and 2 are both excited by the 280 nm xenon source and detected at 310–400 and at 420–650 nm, respectively. Channel 3 is excited by the 370 nm xenon source and detected at 420–650 nm. In this study, the focus is placed on the total concentration of fluorescent particles, which fluoresce in at least one of all three channels, and on the fluorescent biological aerosol particle (FBAP) concentration. FBAP is thereby identified based on the criterion from Toprak and Schnaiter (2013) and refers to particles which simultaneously fluoresce in both channel 1 and channel 3, or which simultaneously fluoresce in channels 1, 2, and 3. It should be pointed out that fluorescence in any of the three channels can be impacted by non-biological particles such as dust; at the same time, using the criterion from Toprak and Schnaiter (2013) to determine FBAP can lead to an underestimation of biological particles (Savage et al., 2017). Moreover, a particle is considered fluorescent in a certain channel if the fluorescence signal exceeds the threshold set for this channel. Typically, the threshold for each channel is obtained using the mean + 3 times the standard deviation of force trigger background measurements. However, during the campaign, no forced-trigger background measurements were conducted; hence, the fluorescence threshold was inferred from forced-trigger data acquired after the INUIT/CLACE campaign during free tropospheric conditions.
Moreover, the concentration of equivalent black carbon (eBC) was measured with an Aethalometer (model AE31, MAGEE scientific, Berkeley, California, USA). The eBC is calculated from the attenuation coefficient measurements by applying the factory standard mass attenuation cross section of 16.6 m2 g−1 at 880 nm. At this wavelength, eBC is the primary absorber such that an impact from mineral dust and brown carbon can be neglected.
2.5 Ice particle residual (IPR) analysis during in-cloud conditions
Small and thus freshly formed ambient ice particles were sampled using the Ice-CVI (Mertes et al., 2007) to analyze the residuals of the ice particles when the sampling site was in cloud. Briefly, by the geometry of the inlet head, only particles <50 µm enter the inlet. A further reduction of sampled particle sizes (<20 µm) is achieved by a virtual impactor. Since supercooled cloud droplets are still within this size range, they are impacted on two cold plates where they freeze upon collision, while the ice particles will bounce off. Any non-activated aerosol particles are excluded by following the streamlines of a downstream counterflow virtual impactor. This allows the selection of ice particles only, which are in a size range between 5 µm (lower particle cutoff diameter of the counterflow virtual impactor) and 20 µm (upper particle cutoff diameter of the virtual impactor). The ice particles are then sublimated such that the IPR can be further analyzed. Here, the IPRs are investigated for their size using an ultra-high-sensitivity aerosol spectrometer (UHSAS; Droplet Measurement Techniques, Longmont, USA), an OPS (0.3–10 µm; TSI 3300, TSI Inc. Shoreview, USA), and an optical particle counter (OPC, Grimm Aerosol Technik, Freilassing, Germany), which are all optical-scattering, laser-based aerosol particle spectrometer systems. Moreover, their chemical composition was analyzed using ALABAMA. For different analysis methods (scanning electron microscopy), we refer the reader to Eriksen Hammer et al. (2018).
2.6 Particle transport model and source sensitivities
Source emission sensitivities are derived with the Lagrangian Dispersion model FLEXPART (FLEXible PARTicle dispersion model; Stohl et al., 2005; Sturm et al., 2013; Pandey Deolal et al., 2014), which has a high grid resolution of in the Alpine region, in combination with the numerical weather prediction model COSMO (Consortium for Small-scale Modeling). Every 3 h, a total of 50 000 particles are released from JFJ and tracked backward in time to determine contact with the boundary layer. The calculations are driven by ECMWF IFS (European Centre for Medium-Range Weather Forecasts Integrating Forecasting System) wind fields. Assumptions for the FLEXPART simulations are as follows: (1) 3000 m a.s.l. was assumed to be the ideal starting altitude for the backwards simulations of the aerosol particles sampled at JFJ, after tests with CO as a tracer for the current model configuration (Brunner et al., 2012). (2) For the determination of the source emission sensitivities, only particles that have contact with the first 100 m above ground are considered. This is also the minimum mixing layer height in the model. (3) For the simulations, particles were tracked for 10 d backward in time. (4) Furthermore, these are normalized residence times, which were divided by the air density (approx. factor of 1.2 at sea level). To compare the air mass origin calculated with FLEXPART with the measured chemical composition of the aerosol particles, we consider only clearly definable source regions, such as marine surface areas and desert areas. Marine surface areas in this study consist of the following oceans: the Atlantic Ocean north of the Equator, the Mediterranean Sea, the Black Sea, the Baltic Sea, the north polar sea, and the North Sea. For the selection of the desert areas, a country-specific selection was made. In this study, the countries making up North Africa (from the Mediterranean to the Sahel) and the countries of the Middle East are taken together. All those countries are listed in Table S2 in the Supplement and are assumed to have at least partially semiarid or arid areas that are potential sources of mineral dust particles.
Moreover, to improve the understanding of travel times from the marine boundary layer to JFJ, the FLEXPART simulations are tracked for the location, time, and intensity of the marine boundary contact for air masses sampled at JFJ. The residence time of model particles below 100 m a.s.l. is considered, and their travel time after leaving the marine boundary layer is recorded.
In principle, the FLEXPART simulations for mineral dust and sea salt remain only qualitative, as the wind speeds at the potential source region of the particles are not considered. High residence times above source regions could therefore coincide with windless conditions resulting in little aerosol emission and thus cause a mismatch. However, short residence times could also be accompanied by high wind speeds above the source regions and high particle loading of the air, which could also result in a misinterpretation of the particle source. A further limitation of the FLEXPART simulations results from the non-consideration of deposition processes. This means that, for example, if air masses experience precipitation along the transport pathway to the measurement site, low aerosol particle concentrations can coincide with high residence times above source regions. This could be more pronounced for sea surfaces, since air masses coming from the sea are more humid, and therefore there is a higher probability of precipitation than, for example, for dry Saharan air. However, the dry deposition of the particles is also decisive and depends not only on the meteorological conditions but also on the traveling time of the air mass from the source region to the measurement site. Despite such limitations, we consider the FLEXPART simulations to be a useful addition to chemical particle analysis to better identify potential source regions of INPs as has been shown in Boose et al. (2016a) and Lacher et al. (2017, 2018a).
3.1 General description of measurement period
Observations of particle concentrations and ratios indicated that JFJ was, for the vast majority of the sampling time, within the free troposphere during the field campaign (>90 %; see Fig. S4 in the Supplement). The ambient temperature ranged from −5 to −18 ∘C (Fig. S4b), which is a relevant range in which MPCs can form. The prevailing local wind directions were southeast and northwest (Fig. S4c), which reflects the dominating wind directions at JFJ due to its location between the peaks of Mönch and Jungfrau. Wind velocities were below 20 m s−1 (Fig. S4c), which is the upper threshold for effective aerosol particle sampling via the GAW total inlet (Weingartner et al., 1999). The frequency of cloud presence (Fig. S4e) is calculated using the difference of ambient temperature and sky temperature following the Stefan–Boltzmann law (Herrmann et al., 2015) and shows that the station was frequently exposed to in-cloud conditions.
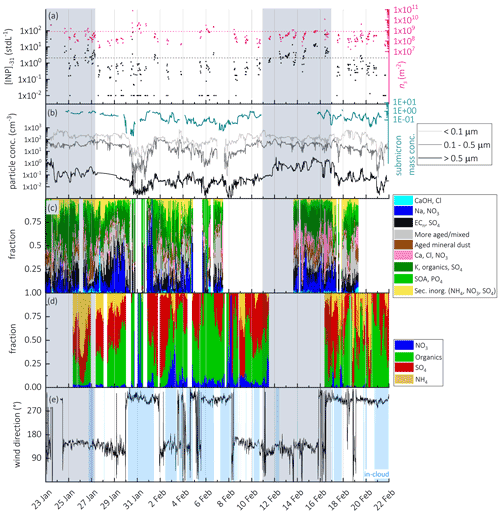
Figure 2Time series of (a) [INP]−31 (incl. INP<LOD) (black) and ns (based on INP>LOD) (pink) at , determined with HINC (dashed lines indicate median [INP]−31 and ns values from Lacher et al. (2018a); (b) particle number concentration for different size bins from the SMPS (<0.5 µm) and OPC (>0.5 µm), and submicron mass concentration derived from AMS measurements; (c) relative abundance of particle types (based on particle number) during the campaign as measured with the LAAPTOF; (d) fractional contribution of the non-refractory aerosol compounds derived from the AMS measurements; (e) wind direction and in-cloud conditions.
Online measurements of [INP]−31 with HINC show a fluctuation of several orders of magnitudes (Fig. 2a). Two periods with elevated [INP]−31 are identified, from 22–27 January and from 11–17 February. Those periods occur simultaneously with an increase in particle concentrations >0.5 µm (Figs. 2a and S5 in the Supplement). In addition to the online INP measurements, filter-based [INP] values were determined with FRIDGE and INSEKT in the temperatures between −20 and −30 ∘C (FRIDGE) and between −10 and −20 ∘C (INSEKT), providing an insight into [INP] at higher temperatures (Fig. 3). Those measurements show that during the periods 22–27 January and 11–17 February [INP] values are elevated while [INP] values do not show higher concentrations, which reveals that the elevated INP population is only ice active below −20 ∘C. A common and important INP type in this temperature range is mineral dust (Hoose and Möhler, 2012; Kanji et al., 2017; Murray et al., 2012), which might have dominated the INP population and would be supported by the increase in large particle fraction (Figs. 2b and S5), also supported by Fig. 6 and related discussion in Sect. 3.3.7. Indeed, it was found by Kammermann et al. (2010), that the concentration of supermicron particles is indicative of dust influence at JFJ. Moreover, as depicted in Fig. 3, the different INP instruments show a general good agreement at similar measurement temperatures (−20 ∘C for INSEKT and FRIDGE; −30 ∘C for FRIDGE and HINC), which supports a temperature-dependent INP variability rather than an instrument-specific variability.
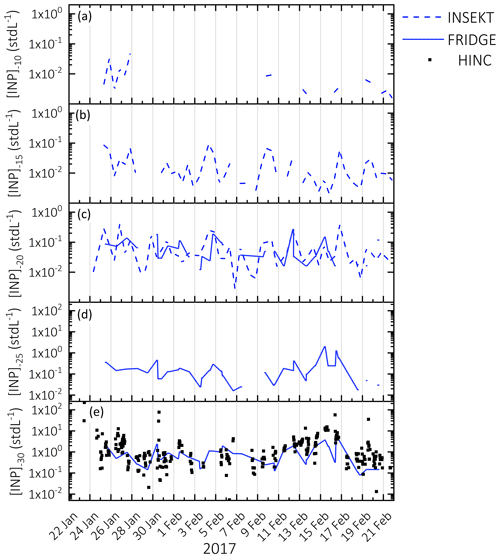
Figure 3Time series of [INP] measured at (a) (INSEKT), (b) (INSEKT), (c) (INSEKT and FRIDGE), (d) (FRIDGE), and (e) (FRIDGE/HINC respectively). Elevated [INP] values during 22–27 January and 11–17 February are only detected at temperatures of −25 and .
Figure 2c also shows the relative abundance of particle types (by number) measured by the LAAPTOF. The corresponding representative mass spectra and the size-resolved number fraction can be found in the Supplement, Fig. S10. ALABAMA data are not included in Fig. 2, because it started to measure with some delay, and it was connected to the CVI inlet during most of the cloud times. Figure 2d shows the fractional mass contribution of the non-refractory compounds measured by the C-ToF-AMS. Sulfate and organics dominate the mass fractions, with occasional episodes of high nitrate and ammonium. Some general features of the chemical composition are observed in both the LAAPTOF and the C-ToF-AMS results. For example, times with high sulfate mass fraction measured by the C-ToF-AMS coincide with high number fractions of the particle types “secondary inorganics (NH4, NO3, SO4)”, “K, organics, sulfate”, and “more mixed/aged” measured by the LAAPTOF. Times with high organic mass fraction coincide with high number fractions of the “SOA and phosphate” and “K, org, sulfate” types. Figure 2b (right axis) also shows the total non-refractory submicron mass concentration measured by the C-ToF-AMS. The measured mass concentration was low, typically below 1 µ g m−3. The time series of the non-refractory mass concentration closely follows the number concentration for particles larger than 0.1 µm (panel b, left axis), indicating that the mass concentration is dominated by accumulation mode particles larger than 0.1 µm. No general dependence of particle composition or number concentration on the wind direction (Fig. 2e) is observed.
3.2 Source region analysis using FLEXPART
In order to identify potential source regions for INPs, we performed a particle transport analysis for times during which elevated (above median values) ns and [INP]−31 were measured ( and [INP], as indicated by the dashed lines in Fig. 2a). Those median values are determined from a multi-year and multi-season analysis for free tropospheric conditions at JFJ, using the same INP instrument (Lacher et al., 2018a). This analysis reveals that potential source regions during times when elevated ns values were measured are the Atlantic Ocean and part of the Sahara (Fig. 4a). During times of high [INP]−31, the air mass origins were traced back to be the Sahara, the Mediterranean Sea, east-southeast Europe, and the Middle East (Fig. 4b). This is a first-order assessment of potential source regions for INPs, and in the following we will investigate the relationship between INPs and aerosol particle chemistry.
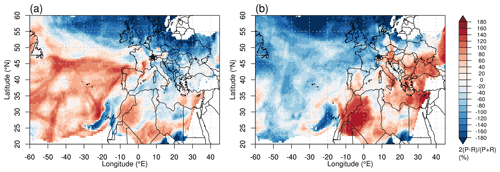
Figure 4FLEXPART source emission sensitivities for particles released at JFJ (indicated by black star) every 3 h and tracked backward in time, for time periods during this campaign with (a) elevated ns and (b) [INP], at and above water saturation. Elevated values are above median values from a multi-year and multi-season analysis for free tropospheric conditions at JFJ ( and [INP]; Lacher et al., 2018a). The color code indicates the relative surface residence time, from low (blue) to high (red).
3.3 Single-particle ions correlating with [INP] and ns
The time series of all ions up to 250 of both polarities, measured with the LAAPTOF and ALABAMA, were correlated to the time series of [INP]−31 and ns. This results in a total of 16 data sets: two single-particle instruments, two correlation methods (see Sect. 2.3.2), two polarities, two variables (ns and [INP]−31) (Table 1, and Figs. S7 and S8 in the Supplement). In the following, we present the results of those correlations for the possible particle types inferred from assigning ions to the observed values. A selection of possible ions for each meaningful correlator value listed in Table 1 and the assignment to possible particle types can be found in Table S1 in the Supplement. The interpretation of particle components and particle types was achieved by the comparison with existing reference mass spectra from both mass spectrometers (see Figs. S9 and S10a in the Supplement), as well as a -to- correlation analysis. The latter method provides information about which ions show a similar time series and thus can either represent isotopes of one element or different molecular fragments of the same original substance. Finally, single meaningful correlators were only assigned to a particle type if other meaningful correlators also indicated the same particle type and if this could be confirmed by both single-particle mass spectrometers. When assigning ions to values, it must be taken into account that different ions can be assigned to an integer value, which in turn means that a single value can be assigned to several particle types. This may result, for example, in two different ions of the same value having increased correlations with the INP variables and thus appearing for the same polarity and value for different particle types. Moreover, we also investigate ions with negative correlation coefficients. Furthermore, it should be noted that several particle types can be mixed internally due to long-range transport. Therefore, it would not be surprising to find the marker ions in almost all the particle types. At the end of this section, we also discuss the differences between the two single-particle mass spectrometers and the correlation methods.
Table 1Ranked correlation coefficients for [INP]−31 and ns (both determined > LOD) with values for the LAAPTOF (a) and ALABAMA (b), for methods 1 and 2, respectively. The assignment of potential ions to values can be found in the Supplement (Table S1).
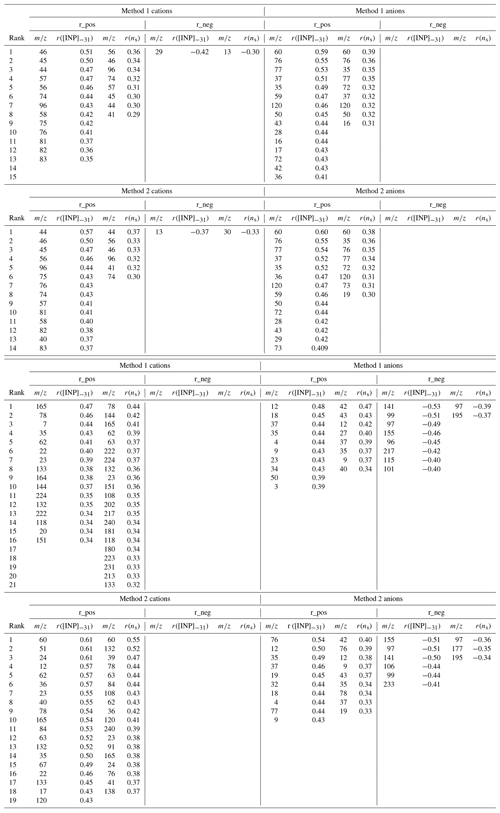
3.3.1 Sea spray
In the analysis of both instruments, we find that sea-spray-related ions have elevated correlation coefficients with both [INP]−31 and ns (Tables 1 and S1). The chlorine anions 35Cl− and 37Cl− as well as cations with 46 (), 81 (), and 83 () in the LAAPTOF (Table 1a) and 35Cl−, 37Cl−, 23 (Na+), 62 (Na2O+), 63 (Na2OH+), 78 (), 12 (Mg2+), 108 (), 181 (), and 165 () in ALABAMA show positive correlation coefficients (r) between 0.31 and 0.57 (Table 1b). Whether these correlations indicate that sea spray particles act as INPs or that sea spray particles arrive in air masses together with other particles that act as INPs remains open and cannot be verified by this correlation analysis. Particles of marine origin can be ice-active (e.g., Wilson et al., 2015), which is further discussed below by including data on air mass origin (see Sect. 3.3.7).
3.3.2 Mineral dust
Mineral dust (Tables 1 and S1) is certainly a particle type that is expected to act as an INP. In general, we find correlation coefficients between ions indicative of mineral dust with both [INP]−31 and ns in the range of 0.32 to 0.60. For example, the cations 44 (SiO+), 56 (CaO+), 57 (CaOH+), and 75 (CaCl+) as well as the anions 60 (), 76 (), and 77 () appear in the LAAPTOF correlation table with correlation coefficients between 0.3 and 0.6. In the ALABAMA data set we find cations such as 7 (Li+), 12 (Mg2+), 23 (Na+), 24 (Mg+), 39 (K+), 41 (K+), 40 (Ca+), 133 (Cs+), and 138 (Ba+) and anions with 43 (AlO+), 76, and 77 ( and ) on the list with correlation coefficients above the threshold. Differences between both instruments can be explained by the laser energy differences used for ablation and ionization and are discussed in more detail below (Sect. 3.3.5). Moreover, the instruments do not cover the same sampling time, which can also be an explanation for this finding.
3.3.3 Elemental carbon (EC)
Another interesting group of ions is the pure carbon ions or (Tables 1 and S1). The number of carbon atoms (n) reaches up to 12 for ALABAMA cations (), but for anions in ALABAMA only ( 12) is found in the best-correlating ion analysis (Table 1b), with correlation coefficients between 0.34 and 0.62. However, it should be mentioned that ( 12) in particular is not a unique feature for elemental carbon, but it is also frequently observed in mass spectra of other particle types. In the LAAPTOF data set, higher carbon atom numbers (up to ) are found for the anions (r=0.32–0.47), but no cations were detected (Table 1a). Pure carbon ion signals can also be contributed by organics; for example, in the LAAPTOF, SOA can generate very intensive C+. However, our finding shows that the other organic ions, also containing hydrogen or oxygen atoms, are almost absent in the results with positive correlation coefficients. This indicates that the pure carbon ion signals are mainly from EC. Nevertheless, a few exceptions exist, which are 59 () and 73 () as well as 42 (CNO−), 58 (C3H8N+), and 76 (C3H10NO+), the latter indicating biological material or amines, but the pure carbon signals are dominant. The increased correlation of particles showing a typical ionic pattern of elemental carbon (see Fig. S9a, presumably soot or other combustion-related particles) with [INP]−31 and ns might indicate a possible connection to heterogeneous ice nucleation. In addition, [INP]−31 also has a correlation coefficient of 0.5 with eBC, which is slightly higher compared to correlation coefficients for meteorological parameters and aerosol particle concentration <0.1 µm (Fig. S6). We note that this does not necessarily translate to elemental-carbon-containing particles being ice nucleation active, but other species transported in the same air mass could possibly be the INPs (see also Sect. 3.3.7). Laboratory studies of BC particles produced from synthetic fossil fuel are shown to be poor INPs in the immersion freezing mode for temperatures above −38 ∘C (Mahrt et al., 2018; Kanji et al., 2020, Friedman et al., 2011; Chou et al., 2013), which questions the role of pure fossil fuel BC in ice nucleation in MPCs. Recent studies indicate the regional importance of BC particles from biomass burning events (Schill et al., 2020) and are related to mineral phases of the biomass burning particles (Jahn et al., 2020). Interestingly, BC being a tracer with appreciable correlation with [INP] has also been reported for measurements conducted in the winter in the boreal forest at Hyytiäla (Paramonov, 2020). Thus, our findings do not necessarily suggest that EC-containing particles are ice nucleation active, but other internally or externally mixed particles could potentially be the INPs, revealing that there was an anthropogenic impact on ice nucleation during this observation.
3.3.4 Ions with negative correlation coefficients
Although r2 was chosen to determine which ions are meaningfully correlated with [INP]−31 and ns, we consider r to also identify negative correlations. Here, we find two organic cations in the LAAPTOF data set ( 13, CH+ and 29, ), but several sulfate-related anions in the ALABAMA data: 96 (), 97 (), 99 (), 141 ( or ), 155 ( or ), 177 (, ), 195 (), 217 (, or ), and 233 (). Some of the listed ion identifications are tentative and have been reported in the literature (e.g., Froyd et al., 2010), but all represent possible sulfate-containing fragments being of inorganic or organic sulfate compounds. Such compounds are typically water soluble and are therefore not expected to contribute to immersion freezing INPs (e.g., Pruppacher and Klett, 1997; Cantrell and Heymsfield, 2005; Kanji et al., 2017) at conditions assessed in HINC. Moreover, we do not expect that those components reduced the ice nucleation ability of the ambient aerosols by a coating effect in the immersion freezing regime (e.g., Kulkarni et al., 2014; Kanji et al., 2019).
3.3.5 Differences between the mass spectrometers
In most of the cases we find agreement between the two mass spectrometer ions that showed a relationship to [INP]−31 and ns. There are two noteworthy exceptions: (a) mineral-dust-related ions and (b) sulfate-containing ions. There are more mineral-dust-related ions clearly correlated with [INP]−31 and ns in the LAAPTOF data set than in the ALABAMA data set. A likely reason for this is the different particle size and particle-type-dependent detection efficiency of the two instruments (see Sect. 2.3.1). As mentioned before, the LAAPTOF employs a higher power density than ALABAMA, resulting in a higher degree of fragmentation in the mass spectra of the LAAPTOF. These smaller organic fragments interfere less with mineral-dust-related fragments. Thus, interferences between organic fragments and mineral-dust-related fragments, e.g., 40 (Ca+ or ), 44 (SiO2, or ), and 57 (CaOH+, , C2O2H+, C2H5CO+, C3H5O+), are more likely to occur in the ALABAMA mass spectra, as these organic ions are composed of longer chains of atoms and are thus more likely to undergo fragmentation in the ablation and ionization process. The time series of the mineral-dust-related ions detected in ALABAMA can therefore be superimposed by the organic ions. As our data show almost no correlation between organic ions and [INP]−31 or ns, this explains the finding that the apparent correlation between mineral-dust-related ion and [INP]−31 or ns is weaker in the ALABAMA than the LAAPTOF data set. Possible reasons for differences in detecting sulfate-containing ions could be the higher power density of the LAAPTOF, which results in smaller fragments; thus it is weaker in identifying organosulfates, e.g., 141 ( or ) and 155 ( or ), and large inorganic sulfate ions, e.g., 195 (), 217 (, or , and 233 () than the ALABAMA.
3.3.6 Differences between the correlation methods (method 1, method 2, [INP]−31, and ns)
In general, we find that correlations between time series of ions and [INP]−31 are higher than for ns (Table 1, Figs. S7 and S8). This does not imply that ice activation is not controlled by the aerosol particle surface, but it could imply that the particle composition plays an important role. The calculation methods can also contribute to this: ns is derived by normalizing [INP]−31 to the total available aerosol surface area (see Eq. 1). Thus, at high aerosol load it can be that ns will decrease (or stay constant) while [INP]−31 increases only marginally compared to the total aerosol surface, thus potentially leading to a better correlation between the ions and [INP]−31. On the other hand, both methods 1 and 2, which were used to calculate the time series of ions, are normalized methods: method 1 is normalized to the total number of recorded single-particle mass spectra in the time interval (20 min). Method 2 is normalized to the total signal height of the spectra. Thus, both methods are not sensitive to the total aerosol particle number, surface, or mass concentration in the way that is used in the calculation of ns. Further, we find that the highest correlation coefficients between the time series occur using method 2 and [INP]−31. The ion fraction in method 2 represents the average composition of all particles during the 20 min time interval, whereas method 1 reports the fraction of particles that contain a certain ion. The higher correlation coefficient found between [INP]−31 and averaged chemical compositions, compared to the fraction of single-particle ions present in the 20 min time interval reported by method 1, suggests that not only the presence of a substance causes ice activation, but that the relative amount of the substance in the averaged particle ensemble is also important to the ice activation properties of the particle ensemble. The higher correlation with [INP]−31 than with ns supports our initial statement that a non-negligible influence of particle composition impacts ice nucleation. Thus, not only aerosol physical properties such as size or surface area, which are typically dominant predictors of [INP] (e.g., DeMott et al., 2010), but also chemical composition are important.
3.3.7 Time series of best correlating ions
To illustrate the findings discussed above, we summed the time series of the best correlating ions of a specific particle type to create a proxy for a more general particle type. For this, we chose correlation coefficients with [INP]−31 based on method 2, as this combination yielded in general the highest correlation coefficients. For example, for the sea spray time series we added the time series of +23, −35, −37, +62, +63, +78, and +165 (ALABAMA). Similarly, we also created a time series for sea spray from LAAPTOF data, for mineral dust and for elemental carbon (ALABAMA and the LAAPTOF), and for sulfate-containing particles (ALABAMA only) using the marker ions of the respective particle types listed in Table S1 (bold marked). The signals at +12, +24, +39, and +41 were not considered for the sea spray and mineral dust time series, because these are very common signals across all particle types. The ions at +12 and +24 can be attributed to both carbon and magnesium. Although +12 clearly shows an increased intensity in the EC type compared to the other particle types listed, it is less clear for +24. Therefore, +24 was not used as a marker for any of the particle types. The signals at +39 and +41 are mainly indicative of potassium, which is a common component of mass spectra due to its ionization energy. For example, potassium occur in biomass burning particles (e.g., Silva et al., 1999) but is also present in sea water and in mineral dust. Thus, the ions +39 and +41 were not considered here in the analysis.
These time series are displayed in Figs. 6 and 7, along with the [INP]−31 time series and particle source region information. For the latter, we used emission footprint sensitivities derived from FLEXPART. We summarized the North African and Middle East countries to account for possible source regions of mineral dust and the marine surfaces to account for possible sea spray particle sources (as explained in Sect. 2.6). Figure 5a shows that the time series of the footprint emission sensitivity calculation for dust surfaces for the North African and Middle East countries are elevated during times when [INP]−31 is high and are accompanied by elevated mineral dust marker values, e.g., on 23, 27, and 29 January and 3, 12–17, and 19 February (Fig. 5b). Moreover, the mineral dust proxies from both mass spectrometers agree well (Fig. 5b). The correlation with the [INP]−31 time series has already been discussed above and indicates that mineral dust particles transported from source regions in North Africa and the Middle East to JFJ act as INPs at −31 ∘C.
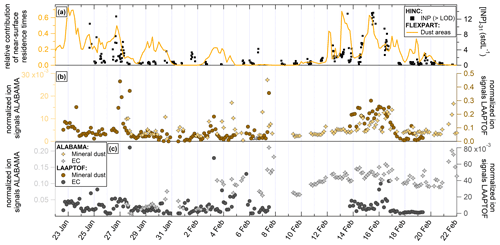
Figure 5Time series of FLEXPART emission footprint sensitivities for desert dust surfaces (northern Africa and Middle East, Sect. 2.7) along with INP concentration (a); timeline of single particle mass spectrometer marker ions for mineral dust particles (b) and EC marker ions (c) for both ALABAMA and the LAAPTOF. SPMS reference spectra of the mineral dust and EC-containing particle types are shown in Figs. S10 and S11.
The time series of the EC proxies from ALABAMA and the LAAPTOF are shown in Fig. 5c. Here, there is some discrepancy between the instruments, for example during the period between 14 and 19 February. Then ALABAMA data show a higher contribution of EC than during the first part of the campaign (29–31 January), whereas in the LAAPTOF data the EC contribution remains similar when comparing 23 January–7 February and 14–19 February, which might be related to differences in the instruments (see Sect. 3.3.5). Thus, the elevated [INP]−31 during 14–19 February occurs during times of not only elevated dust but also elevated EC concentrations. As mentioned before, many studies have reported EC particles do not contribute to heterogeneous ice nucleation above −38 ∘C (e.g., Kupiszewski et al., 2016; Vergara-Temprado et al., 2018b; Mahrt et al., 2018; Kanji et al., 2020). It is possible that the air masses transporting mineral dust from the North African and Middle East source regions also transport polluted air bringing EC-containing particles along the transport pathway. This may result in the observed correlation between INPs and EC-containing particles without the latter being active as INPs and is supported by an increase in the EC signal occurring simultaneously (ALABAMA; see Fig. 5b and c) with an increase in the dust markers on 31 January and 14–18 February.
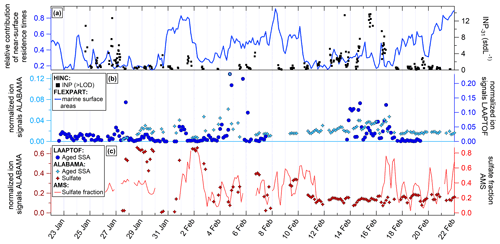
Figure 6Time series of FLEXPART emission footprint sensitivities for marine surface areas along with INP concentration (a); timeline of mass spectrometer marker ions for aged sea spray aerosol (SSA) for both ALABAMA and the LAAPTOF (b) and sulfate marker ions and sulfate fraction for ALABAMA and AMS (c). SPMS reference spectra of the aged sea spray and sulfate-containing particle types are shown in the Supplement (Figs. S9 and S10).
Figure 6 shows the time series of the relative contribution of marine surfaces from FLEXPART, together with [INP]−31 (Fig. 6a), and the proxies for aged sea spray particles from both ALABAMA and the LAAPTOF (Fig. 6b). Both measurements from the single-particle mass spectrometers show similar trends, although the ALABAMA data show much less variation in the second part of the campaign (after 11 February) than the LAAPTOF data set. The differences in the ALABAMA time series between the first and second halves of the campaign are also partly due to changes in the instrument configurations (as described in Sect. 2.3.2). However, the time series of the sea spray proxies from the mass spectra do not match the FLEXPART emission footprint sensitivities for open ocean and sea surfaces (Fig. 6a). Also, the [INP]−31 time series does not match the open water source regions from FLEXPART. Interestingly, the calculated air mass travel times from FLEXPART between the marine boundary layer and the measurement site reveal similarities with the sea spray proxies. For example, the period when the single-particle mass spectrometers recorded a stronger signal from sea spray particles (2 to 5 February) coincides with comparatively short travel times of the air masses to JFJ (12 to 24 h), mainly originating from the marine boundary layer over the western Mediterranean Sea (Fig. S11 in the Supplement). The short travel time is in line with a reduced impact of sedimentation losses, wet removal, and mixing/dilution with other air masses of different origins. The FLEXPART analysis shows that travel times from the marine boundary layer to JFJ can vary between approximately 24 and more than 96 h. However, we should point out that the FLEXPART model is not able to separate coastal regions from open ocean for this analysis, such that the open water classification used may not be the best way to represent sea spray source regions which tend to occur along shorelines during wave breaking and as a function of wind speed (e.g., Vergara-Temprado et al., 2017).
Apart from this, both the sea spray and the [INP]−31 time series show a similar temporal evolution as the dust source region emission sensitivities (Fig. 5). A possible explanation for this might be that the dry saline lake beds in the deserts have been identified as source regions for salt particles (Prospero et al., 2002). For example, Formenti et al. (2003) observed that airborne particles originating from the Sahara that were sampled off the North African coast were a mixture of aluminum-silicate-based minerals and NaCl-bearing salts. This may well explain our observed correlation between dust particles, NaCl-containing particles, and INPs.
Figure 6c shows the time series of the sulfate proxy ions detected by the ALABAMA. For sulfate ions, we observed a negative correlation with [INP]−31 (see also Table 1). Especially in the first half of the campaign, it can be seen that the sulfate signal in the ALABAMA is high at times when [INP]−31 is low (28–30 January, 2–3 February, 7 February, and 9 February). After 11 February, when [INP]−31 values are higher along with the mineral dust signals and the emission sensitivities of the dust source regions, the sulfate ion time series shows only slight fluctuation and shows no similarities to the [INP]−31 time series. Furthermore, no similarities with the dust source region emission sensitivities are observed. A comparison of the ALABAMA sulfate ion time series with the sulfate fraction measured with the AMS (Fig. 6c, right panel) shows a good agreement in the time points of the variations, although not in the strength of the fluctuations. Thus, the time series of the ALABAMA and AMS sulfate measurements are anticorrelated to the [INP]−31 time series, which is not surprising given soluble aerosol particles are not expected to contribute to heterogeneous ice nucleation above −38 ∘C.
3.4 Fluorescent particles
During the duration of the campaign, a WIBS instrument measured fluorescent particles in a size range between 0.5–20 µm. Only a minor fraction of fluorescent particles was found to be FBAP (0.013) according to the criteria of Toprak and Schnaiter (2013). However, it should be noted that the application of the chosen criterion to identify FBAP (simultaneous fluorescence in channels 1 and 3 or in channels 1, 2, and 3; see Sect. 2.4) can lead to an underestimation of biological particles (Savage et al., 2017). Thus, we also considered the total fluorescent particle concentration, with fluorescence in at least one of the three channels, for the correlation coefficient analysis. We find the ranked correlation coefficient between [INP]−31 and FBAP to be low (0.16), while it is quite high for fluorescent particles (0.63; Fig. S6). However, the correlation with the total particle concentration (sum of fluorescent and non-fluorescent particles) determined with the WIBS is even higher (0.75). As the WIBS measures only particles >0.5 µm, the observed relationship between [INP]−31 and fluorescent particle concentrations is confounded by a size effect, as the large particle size fraction is enough to explain this good correlation. As depicted in Fig. S10b, mineral dust particles mainly occur in the size ranges >0.5 µm, and they can also show fluorescence. Thus, we cannot clearly verify a contribution from biological particles to INPs active at −31 ∘C during the INUIT/CLACE field campaign, which is unsurprising, given the season, dominant snow cover, and free tropospheric conditions at the site.
Moreover, we would like to point out that the strong correlation between [INP]−31 and the total particle concentration measured with the WIBS is the highest observed during this campaign, together with the particle concentration >0.5 µm measured with the OPS (Fig. S6).
3.5 Ice particle residuals (IPRs)
3.5.1 Physical cloud characteristics related to the number and size distribution of IPRs
Cloud residuals of 16 separate cloud events were sampled by the Ice-CVI, sub-divided into 43 cloud periods by the shape of the residual particle size distribution. This was done on the basis of the observed concentration ratio R of the size range 300–600 to 80–100 nm:
For R, three types of cloud periods were defined: R<1, , and R>3. R>3 denotes cloud periods where the IPR number size distribution shows a clear minimum or at least a strong decrease at around 100 nm (Fig. S12 in the Supplement), whereas R<1 denotes cloud periods where the relative number of small IPRs is very high compared to larger IPR sizes (Fig. S13 in the Supplement). The cloud period type is in between and thus rather inconclusive and therefore not included in the further evaluation. Considering sufficient counting statistics based on the UHSAS and the ALABAMA measurements, 20 cloud periods remained for the condition R>3 and R<1 (Tables S3, S4, and S5 in the Supplement), corresponding to 12 separate cloud events.
Since it is very unlikely to find INPs at a diameter below 100 nm (e.g., Vali, 1966; Pruppacher and Klett, 1997; Richardson et al., 2007; Cornwell et al., 2019), this feature of small IPRs is attributed to the presence of secondary ice in the examined clouds. Therefore, the cloud periods presented in Fig. S12 and Table S3 as well as Fig. S13 and Table S4 are interpreted as MPCs with less and more impact of secondary ice production, respectively. This assumption is further supported by the fact that on average the cloud periods interpreted as being more strongly influenced by secondary ice contribution have significantly higher IPR number concentrations. Such an implication of small cloud residual sizes (<200 nm) on secondary ice formation is also reported for measurements conducted at the Zeppelin observatory (Ny Ålesund) in the Arctic (Karlsson et al., 2021). During these periods, the IPR concentration is also higher in the IPR diameter range larger than 300 nm. This implies that residuals from secondary ice particles can also contribute to the size range of IPRs expected from primary ice particles. Figure 7a shows the mean IPR number size distribution for both cloud categories averaged with respect to the duration of the single cloud periods.
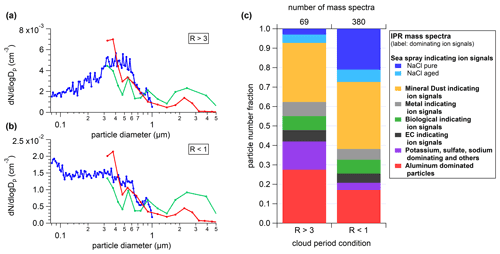
Figure 7Comparison of duration-weighted mean IPR number size distribution of cloud periods with lower (R>3, a) and higher (R<1, b) proportion of small IPRs (<300 nm), and respective IPR composition (c), which is interpreted as lower and higher contribution of secondary ice, respectively (see description in Sect. 3.5.1). Details about the individual cloud periods are presented in Tables S3 and S4. The size distributions are shown in (a) and (b) and were measured using a UHSAS (blue), an OPS (green), and a Sky-OPC (red). The IPR composition shown in (c) was determined with the ALABAMA.
3.5.2 Chemical composition of IPRs
The IPR mass spectra recorded with the ALABAMA downstream of the Ice-CVI were analyzed separately for cloud periods with lower and higher contributions of small IPRs as defined in Sect. 3.5.1 (see Tables S3 and S4). During the 20 analyzed MPC periods, a total of 640 IPR mass spectra were recorded with the ALABAMA. These spectra were clustered using a fuzzy c-means algorithm (Roth et al., 2016; Hinz et al., 1999; Bezdek et al., 1984), and the resulting clusters were assigned to particle types based on marker ions and reference particle mass spectra, resulting in eight different particle types. Figure 7a and b show the IPR size distribution for cases of lower (Table S3; R>3) and higher (listed in Table S4; R<1) contributions of small IPRs, respectively. The particle number fractions of those two cloud conditions are depicted in Fig. 7c, and the size-resolved particle number fraction of the two cloud conditions is shown in Fig. 8. The IPR particle types will be discussed in the following. Additionally, a temporal evolution of the particle composition during a selected cloud event is shown in the Supplement (Figs. S14–S16).
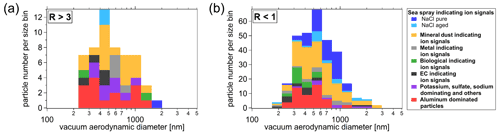
Figure 8Size-resolved particle number fraction of the determined IPR types for cloud periods with lower (R>3; a) and higher (R<1; b) proportions of small IPRs (<300 nm), which is interpreted as lower and higher contribution of secondary ice, respectively (see description in Sect. 3.5.1).
Mineral dust and biological particles. In agreement with previous IPR studies conducted at the JFJ station (Kamphus et al., 2010; Worringen et al., 2015; Schmidt et al., 2017; Eriksen Hammer et al., 2018), IPRs of mineral origin were detected in almost every cloud event and were the most frequently detected IPR type by number (58 %, also including Al type particles; see discussion below about “Aluminum and other metal containing particles”), even though ambient temperatures during the campaign were relatively high ( ∘C). Activation of ice by mineral dust at such relatively high temperatures might be caused by biological material on the surface of dust particles, because many IPRs contained ions indicating biological material (see Fig. S17 in the Supplement). Another possible explanation for this relatively high activation temperature could be that the mineral dust particles were highly ice-active dust particles, such as potassium-rich feldspars, which generally nucleate ice more efficiently and at higher temperatures than other mineral dust particles (Harrison et al., 2016). Moreover, the fraction of mineral dust containing IPRs does not appear to be related to the proportion of small IPRs, as it is found in both cloud period classifications. Moreover, mineral dust IPRs were detected in size ranges between 250 and 1500 nm (Fig. 8). Interestingly, the majority of dust IPRs are submicron in size. Particles dominated by ion signals indicating biological particles were also consistently found in both cloud period classifications, but to a general smaller proportion (<10 %), which was also reported by Schmidt et al. (2017) and Kupiszewski et al. (2015).
Aluminum and other metal-containing particles. The aluminum particle type fraction is the second largest particle fraction, contributing more than 25 % by number during cloud periods with a smaller contribution from small IPRs and more than 15 % during periods of high contributions from small IPRs. Although aluminum ions in single-particle mass spectra can indicate mineral dust, Eriksen Hammer et al. (2018) identified a subset of IPRs containing aluminum as possible contamination from the sampling setup, despite all surfaces of the Ice-CVI being coated with a nickel alloy prior to the 2017 campaign. However, in this work the aluminum particle type detected by the ALABAMA contains additional ion signals of Na+, Cl−, cyanide (CN−), and cyanate (CNO−); thus we regard them as real ambient particles and count them as mineral dust particles (see discussion above) rather than sampling artifacts. Such aluminum-dominated particles have also been observed in IPRs by Schmidt et al. (2017) using the ALABAMA and by Kamphus et al. (2010) using an aerosol time-of-flight mass spectrometer (ATOFMS, TSI model 3800) in combination with the Ice-CVI at JFJ in winter 2013 and in winter 2007, respectively. Other metallic particles were detected with a similar fraction (below 10 %) during times of low and high small IPRs, indicating that metal-containing particles act as INPs, which agrees with previous findings (Kamphus et al., 2010; Eriksen Hammer et al., 2018; Schmidt et al., 2017).
Sodium chloride particles. Another frequently observed particle type is dominated by NaCl, indicating sea spray particles (e.g., Sierau et al., 2014). It should be mentioned that the described correlation between sea spray particles and INPs (see Sect. 3.3.1) refers to total sea spray. In the following, we distinguish between aged sea spray (derived from ions such as Na2O+, Na2O(H)+, , , and ; Gard et al., 1998; Sierau et al., 2014) and pure NaCl mass spectra (containing only Na+, Cl−, and various NaCl compounds such as Na2Cl+, , and ). The main difference between the IPR composition during the two cloud period classes is the fraction of the pure NaCl particle type, which is clearly higher (about 20 %) during the cloud periods with a high concentration of small IPRs (R<1). This indicates that these pure NaCl particles do not originate from freshly formed small ice crystals as INPs, but rather result from sampling ice crystals formed by secondary ice production. The same effect may explain the previously reported observations of NaCl particles in IPRs (Kamphus et al., 2010; Eriksen Hammer et al., 2018). This interpretation is further discussed in Sect. 3.5.3.
Size-resolved chemical fraction and internal mixtures. The size distribution of the IPRs reveals a dominant submicron fraction (Fig. 8). This challenges the assumption that most of the INPs are supermicron in size (e.g., Mason et al., 2016) and might be related to the sampling location in the free troposphere, where particles typically undergo long-range transport and sedimentation processes prior to being sampled. The size-resolved examination of the particle fractions (see Fig. 8) shows that IPRs containing mineral dust were detected in all size channels between about 150 and 3000 nm (vacuum aerodynamic diameter). IPRs with biological and elemental carbon ion signals are mainly found in the size range between about 150 and 600 nm, whereas the pure NaCl particles have the highest contribution between about 400 and 1000 nm. We note that the attribution of the IPR spectra to particles types is based on the dominant ion signals of the mass spectra and is not an absolute assignment to a pure particle type. Thus, almost all IPR types also show traces of internal mixtures. For example, over 90 % of the IPRs contain signals of sodium (Na+), over 80 % contain signals of potassium (K+), and over 70 % contain signals of Cl− and CN−. Mineral dust particles in turn contain a variety of other signals that indicate sea spray and biological and biomass burning substances. Therefore, the IPR types cannot be considered completely independent of each other and represent internal mixtures.
3.5.3 Dependence of the IPR composition on the wind direction
At JFJ, two main local wind directions prevail due to the topography around the station. To study the influence of wind direction on IPR composition, we selected air masses coming from either the southeast or northwest to the JFJ station and sorted the cloud periods of Table S4 (those with a high contribution of small IPRs, R<1, indicating more impact from secondary ice formation) for these two main flow directions (Fig. 9). Figure 9a and b show the size distribution of the IPR for the two dominant wind directions, and panel c depicts the IPR composition. The fraction of the pure NaCl IPR type is significantly higher (>40 %) for the northwesterly flow than for the southeasterly flow (about 3 %), whereas the other IPR types show only slight differences between the two cases. Pure NaCl particles are generally considered poor INPs and freeze homogeneously or heterogeneously at temperatures below −38 ∘C (Kanji et al., 2017, and references therein) and thus should not be expected to occur in primary IPRs under the temperature conditions at JFJ. If the ice crystals would have originated from homogeneous freezing at higher altitude and settled to JFJ, they would have grown to sizes too large to be sampled with the Ice-CVI, which only collects freshly (small) nucleated ice crystals. We can also rule out that the observation of pure NaCl particles in the IPR population is due to collected cloud droplets based on the working principle of the Ice-CVI, which impacts supercooled cloud droplets on a cold plate where they freeze upon collision. If the Ice-CVI would also transmit liquid cloud droplets, one would also expect an enhanced abundance of sulfate and nitrate signals, which were detected in cloud droplet residuals (Roth et al., 2016; Kamphus et al., 2010). However, this was not the case in our work. Further, scavenging is unlikely, because firstly, the Ice-CVI samples only small, freshly nucleated ice crystals that have been growing only by diffusion (5–20 µm; Mertes et al., 2007), and secondly, scavenging would also apply to sulfate, nitrate, and organic particles that dominate the aerosol population at JFJ (see Fig. 2).
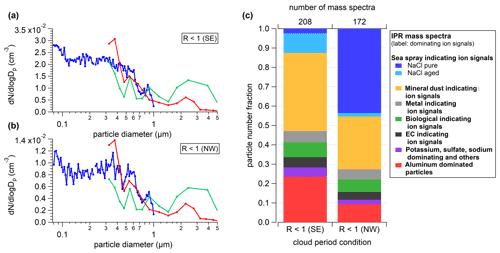
Figure 9Comparison of the IPR number size distribution of cloud periods with a higher (R<1) proportion of small IPRs (<300 nm), which is interpreted as higher contribution of secondary ice (see description in Sect. 3.5.1) as a function of air mass flow direction, in the case of a southeasterly flow (SE, a) and northwesterly flow (NW, b), and respective IPR composition (c). The size distributions shown in panels (a) and (b) were measured using a UHSAS (blue), an OPS (green), and a Sky-OPC (red). The IPR composition shown in (c) was determined with the ALABAMA.
Thus, we conclude that the pure NaCl IPR type is associated with secondary ice processes. At JFJ, the different orographic terrain profiles northwest and southeast of the station lead to stronger vertical wind speeds for the steep rise on the northern side of the research station, resulting in high peak supersaturations (Hammer et al., 2014). This is expected to favor the occurrence of persistent MPCs, compared to the gentler slope on the south of the measuring station (Lohmann et al., 2016). These stronger updrafts at the northwesterly slope can transport larger droplets to higher regions with lower temperatures, which in turn increases the formation of secondary ice crystals (Lohmann et al., 2016; Korolev and Leisner, 2020). Various secondary ice processes, such as ice fragmentation due to surface and spicule bubble bursting during thermal shock, fragmentation during ice–ice collision, or splintering during riming (Hallett–Mossop process), are favored by freezing of large droplets, although the Hallett–Mossop process has its maximum secondary ice production rate at higher temperatures than those occurring most of the time during our measurements (Hallett and Mossop, 1974; Korolev and Leisner, 2020). Furthermore, blowing snow or fluxes of surface hoar frost are also possible sources of secondary ice (Vali et al., 2012; Farrington et al., 2016; Lloyd et al., 2015; Beck et al., 2018). However, no correlation was observed between horizontal wind speeds at JFJ and IPR composition. We note that the sulfate fraction in the IPR composition during times of an increased influence from secondary ice crystal formation (during small IPR samples) is low as for NaCl, although one would expect that sulfates are also present in droplets which contribute to secondary ice crystals. This could be explained by the original cloud condensation nuclei (CCN) mass being distributed over many small ice fragments upon fragmentation of the ice crystal. Thus, the sampled IPR is smaller than the initial CCN. This shifts the IPR size distributions to smaller sizes, such that sulfate-containing IPRs are possibly too small to be detected in the ALABAMA size range, and a preferential sampling of NaCl particles is detected. Indeed, the size distribution from ambient sea spray particles shows a maximum >1 µm (Fig. S10a) while the IPR NaCl particles under northwest wind conditions have a maximum <1 µm (Fig. 10b). Figure S10 also shows that the sulfate-containing aerosol particles in the ambient air have a maximum at smaller sizes than NaCl-containing particles. Moreover, the faster updraft velocities lead to a shorter lifetime of the cloud droplets before freezing, such that aqueous-phase reactions that lead to aged sea spray particle signatures (e.g., and ; Gard et al., 1998) are less likely to occur compared to a slow updraft cloud formation situation, which supports the dominance of the small IPRs with pure NaCl signatures. Which process is ultimately responsible for the secondary ice formation with the simultaneous occurrence of pure NaCl IPR cannot be determined from our measurements.
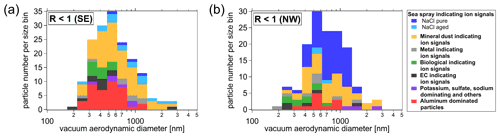
Figure 10Size-resolved particle number fraction of the determined IPR types for cloud periods with higher proportion (R<1) of small IPR (<300 nm), which is interpreted as a higher contribution of secondary ice (see description in Sect. 3.5.1), selected by southeasterly flow (a) and northwesterly flow (b).
In this study we investigate the potential chemical characteristics and sources of immersion freezing INPs at −31 ∘C at the High Altitude Research Station Jungfraujoch (JFJ) in winter 2017, by combining INP concentration measurements from the online instrument HINC with measurements from two single-particle mass spectrometers, the ALABAMA and the LAAPTOF, and with FLEXPART source emission model calculations. We correlate the time series of individual ions from the two mass spectrometers with the time series of [INP]−31 and ns to determine potential relationships. Such correlation analysis allows us to also include small ion signals which still might represent chemical substances rather than whole particle types, such that fewer initial assumptions have to be made, allowing a cross-particle-type approach. Based on our analyses, sodium-, calcium-, silicon-, and chlorine-containing ions in particular showed increased correlation with [INP]−31 and ns. We concluded that these ions originate from substances that are essentially due to mineral dust and sea salt particles. From those results we find consistent evidence for an impact of mineral dust and particles of marine origin on ice nucleation, which is also supported by particle transport simulations. Moreover, the investigation of the time series of [INP] at temperatures from −10 to −30 ∘C, as measured by the offline methods INSEKT and FRIDGE, reveals that only [INP] values below −20 ∘C follow similar trends to those of [INP]−31, which could be due to mineral dust particles dominating the INP population. The different INP methods thereby show a good agreement in INP concentrations at similar measurement temperatures.
While mineral dust is known to play a major role in ice nucleation (e.g., Hoose and Möhler, 2012; Murray et al., 2012; Kanji et al., 2017), marine INPs are mostly assumed to be present in remote marine environments (Vergara-Temprado et al., 2018a; McCluskey et al., 2019). From this work it is evident that locations far away from the sampling site, like the Sahara and the oceans, can still have a substantial contribution to the INP population. We also observe correlations between INPs and elemental-carbon-related ion markers, which coincide with those of dust, and we interpret this as a dust-related correlation. This is supported by the conclusions in Kupiszewski et al. (2016) and Eriksen Hammer et al. (2018), who do not find an enrichment of BC in IPRs, but contrasts the finding of Cozic et al. (2008). The discrepancy with the study by Cozic et al. (2008) might be caused by the different absorption-based methods, which were likely impacted by dust interference in Cozic et al. (2008).
Our findings of the INP population at JFJ in winter 2017 are supported by the measurement of ice particle residuals (IPRs). Although ice crystals in the cloud formed at markedly higher temperatures compared to the freezing temperature of the INP−31, it is still apparent that the major contributors are mineral dust (58 %±32 % of IPRs, including an aluminum-type particle) and sea spray particles (17 %±20 % of IPRs, referring to pure and aged sea spray particle types), consistent with our conclusions from the correlations of ion signals from mass spectrometry and INP−31 and ns. In addition, biological, metallic, and elemental-carbon-containing particles were also found to be present in the IPRs to a lesser extent. However, it must be pointed out that the attribution of all mass spectra to particle types is only based on the dominant ion signals of the mass spectra and is not an absolute assignment to a pure particle type. Most particles detected were internally mixed, which is not surprising given the typical residence time (several days) of particles in the free troposphere, during which they can undergo aging processes. In addition, most of the IPRs were submicron size, which indicates that smaller particles are contributors to INPs at the given ambient temperatures in the free troposphere. Moreover, a possible influence of ice crystals formed by secondary production processes on the IPR population was believed to occur during cloud periods with increased small sizes (80–100 nm) and high IPR concentrations possibly related to secondary ice crystals. This is in agreement with a recent study of small cloud residuals (<200 nm) impacting secondary ice in arctic clouds (Karlsson et al., 2021). The investigations showed that in particular pure NaCl particles were observed more frequently as IPRs during such cloud periods with a simultaneous northwesterly inflow to the measurement site, which cannot be attributed to primary INPs at the prevailing temperatures.
Our study presents the physical and chemical properties of INPs and overall aerosol particles, using measurements from single-particle mass spectrometry, aerosol mass spectrometry, an ice selective inlet, and model simulation for source emission sensitivities to identify potential source regions of INPs and IPRs. At the same time, our findings highlight the need for further investigations for the sources of INPs in a longer time period and in different seasons and years (e.g., using a similar measurement setup) and to directly investigate the identity of INPs with a different instrumental setup, such as, for example, a coupled cloud chamber with single-particle mass spectrometry and with ice-selective inlets.
The data are available at https://doi.org/10.3929/ethz-b-000484207 (Lacher et al., 2021).
The supplement related to this article is available online at: https://doi.org/10.5194/acp-21-16925-2021-supplement.
LL, HCC, SM, JoS, XS, and ZAK conceived the study. LL led the overall writing of the manuscript with specific contributions from HCC, JoS, SM, and XS. The manuscript was reviewed and edited by HS and ZAK. JoS coordinated the field activities. LL performed and analyzed the online INP measurements. HCC, XS, and JoS performed and analyzed the mass spectrometry measurements. SM operated the Ice-CVI and analyzed the IPR size distribution. SH and HCC conducted and analyzed the FLEXPART model calculations. MS performed and analyzed the trace gas measurements. MGB provided the aerosol particle size distributions, the Aethalometer measurements, and WIBS measurements. AM analyzed the WIBS data. DW and JaS conducted and analyzed the offline INP measurements from FRIDGE. LL, XS, OM, KH, and TS contributed to the filter collection and analysis of INSEKT. LL, HCC, XS, JoS, SM, HS, and ZAK interpreted the data, and all authors contributed to reviewing the manuscript. JoS and ZAK oversaw the project.
The contact author has declared that neither they nor their co-authors have any competing interests.
The opinions
expressed and arguments employed herein do not necessarily reflect the
official views of the Swiss government.
Publisher's note: Copernicus Publications remains neutral with regard to jurisdictional claims in published maps and institutional affiliations.
We thank the International Foundation High Altitude Research Station Jungfraujoch and Gornergrat (HFSJG) for the support and the opportunity to perform measurements, with a special thanks to the custodians Maria and Urs Otz and Joan and Martin Fischer. Meteorological data were provided by MeteoSwiss. We also acknowledge all the participants of the INUIT/CLACE 2017 campaign. Erik Herrmann and Nicolas Bukowiecki are acknowledged for operating various aerosol instruments during the campaign. We thank Oliver Eppers and Udo Kästner for their support during the campaign, as well as the KIT technical team.
Larissa Lacher and Zamin A. Kanji received funding from Global Atmospheric Watch, Switzerland (MeteoSwiss GAW-CH+ 2014–2017). Johannes Schneider and Hans-Christian Clemen were funded by the Deutsche Forschungsgemeinschaft (DFG, German Research Foundation) (grant no. 170852269), within the research unit INUIT (FOR 1525), and from the EU Horizon 2020 research and innovation program (ACTRIS-2 (grant no. 654109)). Stephan Mertes, Jann Schrod, and Daniel Weber were funded by the DFG (grant no. 170852269) within the research unit INUIT (FOR 1525). Ottmar Möhler, Thea Schiebel, and Kristina Höhler were partially funded by the Deutsche Forschungsgemeinschaft (DFG), project no. 170852269 (Research Unit INUIT, FOR 1525). Harald Saathoff and Xiaoli Shen received funding from the European Union's Horizon 2020 research and innovation programme as part of the ACTRIS-2 project (grant no. 654109). The aerosol measurements by PSI were performed with financial support from MeteoSwiss (GAW-CH 2014–2017) and from the ACTRIS2 project (EU H2020-INFRAIA-2014-2015 (grant no. 654109)) and the Swiss State Secretariat for Education, Research and Innovation (contract no. 15.0159-1).
This paper was edited by Daniel Knopf and reviewed by two anonymous referees.
Appenzeller, C., Begert, M., Zenklusen, E., and Scherrer, S. C.: Monitoring climate at Jungfraujoch in the high Swiss Alpine region, Sci. Total Environ., 391, 262–268, https://doi.org/10.1016/j.scitotenv.2007.10.005, 2008.
Atkinson, J. D., Murray, B. J., Woodhouse, M. T., Whale, T. F., Baustian, K. J., Carslaw, K. S., Dobbie, S., O'Sullivan, D., and Malkin, T. L.: The importance of feldspar for ice nucleation by mineral dust in mixed-phase clouds, Nature, 498, 355–358, https://doi.org/10.1038/nature12278, 2013.
Baltensperger, U., Gäggeler, H. W., Jost, D. T. A. L., M., Schwikowski, M., and Weingartner, E.: Aerosol climatology at the high-alpine site Jungfraujoch, Switzerland, J. Geophys. Res., 102, 19707–19715, https://doi.org/10.1029/97JD00928, 1997.
Beck, A., Henneberger, J., Fugal, J. P., David, R. O., Lacher, L., and Lohmann, U.: Impact of surface and near-surface processes on ice crystal concentrations measured at mountain-top research stations, Atmos. Chem. Phys., 18, 8909–8927, https://doi.org/10.5194/acp-18-8909-2018, 2018.
Bezdek, J. C., Ehrlich, R., and Full, W.: FCM: The fuzzy c-means clustering algorithm, Comput. Geosci., 10, 191–203, https://doi.org/10.1016/0098-3004(84)90020-7, 1984.
Bigg, E. K.: Ice Nucleus Concentrations in Remote Areas, J. Atmos. Sci., 30, 1153–1157, https://doi.org/10.1175/1520-0469(1973)030<1153:Incira>2.0.Co;2, 1973.
Boose, Y., Kanji, Z. A., Kohn, M., Sierau, B., Zipori, A., Crawford, I., Lloyd, G., Bukowiecki, N., Herrmann, E., Kupiszewski, P., Steinbacher, M., and Lohmann, U.: Ice Nucleating Particle Measurements at 241 K during Winter Months at 3580 m MSL in the Swiss Alps, J. Atmos. Sci., 73, 2203–2228, https://doi.org/10.1175/JAS-D-15-0236.1, 2016a.
Boose, Y., Sierau, B., García, M. I., Rodríguez, S., Alastuey, A., Linke, C., Schnaiter, M., Kupiszewski, P., Kanji, Z. A., and Lohmann, U.: Ice nucleating particles in the Saharan Air Layer, Atmos. Chem. Phys., 16, 9067–9087, https://doi.org/10.5194/acp-16-9067-2016, 2016b.
Brands, M., Kamphus, M., Böttger, T., Schneider, J., Drewnick, F., Roth, A., Curtius, J., Voigt, C., Borbon, A., Beekmann, M., Bourdon, A., Perrin, T., and Borrmann, S.: Characterization of a Newly Developed Aircraft-Based Laser Ablation Aerosol Mass Spectrometer (ALABAMA) and First Field Deployment in Urban Pollution Plumes over Paris During MEGAPOLI 2009, Aerosol Sci. Tech., 45, 46–64, https://doi.org/10.1080/02786826.2010.517813, 2011.
Brier, G. W. and Kline, D. B.: Ocean water as a source for ice nuclei, Science, 130, 717–718, https://doi.org/10.1126/science.130.3377.717, 1959.
Brunner, D., Henne, S., Keller, C. A., Vollmer, M. K., Reimann, S., and Buchmann, B.: Estimating European Halocarbon Emissions Using Lagrangian Backward Transport Modeling and in Situ Measurements at the Jungfraujoch High-Alpine Site, in: Lagrangian Modeling of the Atmosphere, American Geophysical Union, Washington D.C., 207–222, 2012.
Bukowiecki, N., Weingartner, E., Gysel, M., Collaud Coen, M., Zieger, P., Herrmann, E., Steinbacher, M., Gäggeler, H. W., and Baltensperger, U.: A Review of More than 20 Years of Aerosol Observation at the High Altitude Research Station Jungfraujoch, Switzerland (3580 m asl), Aerosol Air Qual. Res., 16, 764–788, https://doi.org/10.4209/aaqr.2015.05.0305, 2016.
Bukowiecki, N., Brem, B. T., Wehrle, G., Močnik, G., Affolter, S., Leuenberger, M., Collaud Coen, M., Hervo, M., Baltensperger, U., and Gysel-Beer, M.: Elucidating local pollution and site representativeness at the Jungfraujoch, Switzerland through parallel aerosol measurements at an adjacent mountain ridge, Environ. Res. Commun., 3, 021001, https://doi.org/10.1088/2515-7620/abe987, 2021.
Bundke, U., Nillius, B., Jaenicke, R., Wetter, T., Klein, H., and Bingemer, H.: The fast Ice Nucleus chamber FINCH, Atmos. Res., 90, 180–186, https://doi.org/10.1016/j.atmosres.2008.02.008, 2008.
Burrows, S. M., Hoose, C., Pöschl, U., and Lawrence, M. G.: Ice nuclei in marine air: biogenic particles or dust?, Atmos. Chem. Phys., 13, 245–267, https://doi.org/10.5194/acp-13-245-2013, 2013.
Canagaratna, M. R., Jayne, J. T., Jimenez, J. L., Allan, J. D., Alfarra, M. R., Zhang, Q., Onasch, T. B., Drewnick, F., Coe, H., Middlebrook, A., Delia, A., Williams, L. R., Trimborn, A. M., Northway, M. J., DeCarlo, P. F., Kolb, C. E., Davidovits, P., and Worsnop, D. R.: Chemical and microphysical characterization of ambient aerosols with the aerodyne aerosol mass spectrometer, Mass Spectrom. Rev., 26, 185–222, https://doi.org/10.1002/mas.20115, 2007.
Cantrell, W. and Heymsfield, A.: Production of Ice in Tropospheric Clouds: A Review, B. Am. Meteorol. Soc., 86, 795–808, https://doi.org/10.1175/bams-86-6-795, 2005.
Chen, J., Wu, Z., Augustin-Bauditz, S., Grawe, S., Hartmann, M., Pei, X., Liu, Z., Ji, D., and Wex, H.: Ice-nucleating particle concentrations unaffected by urban air pollution in Beijing, China, Atmos. Chem. Phys., 18, 3523–3539, https://doi.org/10.5194/acp-18-3523-2018, 2018.
Chen, K., Yin, Y., Liu, S., Liu, C., Wang, H., He, C., Jiang, H., and Chen, J.: Concentration and variability of deposition-mode ice nucleating particles from Mt. Tai of China in the early summer, Atmos. Res., 253, 105426, https://doi.org/10.1016/j.atmosres.2020.105426, 2021.
Chou, C., Stetzer, O., Weingartner, E., Jurányi, Z., Kanji, Z. A., and Lohmann, U.: Ice nuclei properties within a Saharan dust event at the Jungfraujoch in the Swiss Alps, Atmos. Chem. Phys., 11, 4725–4738, https://doi.org/10.5194/acp-11-4725-2011, 2011.
Chou, C., Kanji, Z. A., Stetzer, O., Tritscher, T., Chirico, R., Heringa, M. F., Weingartner, E., Prévôt, A. S. H., Baltensperger, U., and Lohmann, U.: Effect of photochemical ageing on the ice nucleation properties of diesel and wood burning particles, Atmos. Chem. Phys., 13, 761–772, https://doi.org/10.5194/acp-13-761-2013, 2013.
Clemen, H.-C., Schneider, J., Klimach, T., Helleis, F., Köllner, F., Hünig, A., Rubach, F., Mertes, S., Wex, H., Stratmann, F., Welti, A., Kohl, R., Frank, F., and Borrmann, S.: Optimizing the detection, ablation, and ion extraction efficiency of a single-particle laser ablation mass spectrometer for application in environments with low aerosol particle concentrations, Atmos. Meas. Tech., 13, 5923–5953, https://doi.org/10.5194/amt-13-5923-2020, 2020.
Collaud Coen, M., Weingartner, E., Schaub, D., Hueglin, C., Corrigan, C., Henning, S., Schwikowski, M., and Baltensperger, U.: Saharan dust events at the Jungfraujoch: detection by wavelength dependence of the single scattering albedo and first climatology analysis, Atmos. Chem. Phys., 4, 2465–2480, https://doi.org/10.5194/acp-4-2465-2004, 2004.
Collaud Coen, M., Weingartner, E., Furger, M., Nyeki, S., Prévôt, A. S. H., Steinbacher, M., and Baltensperger, U.: Aerosol climatology and planetary boundary influence at the Jungfraujoch analyzed by synoptic weather types, Atmos. Chem. Phys., 11, 5931–5944, https://doi.org/10.5194/acp-11-5931-2011, 2011.
Connolly, P. J., Möhler, O., Field, P. R., Saathoff, H., Burgess, R., Choularton, T., and Gallagher, M.: Studies of heterogeneous freezing by three different desert dust samples, Atmos. Chem. Phys., 9, 2805–2824, https://doi.org/10.5194/acp-9-2805-2009, 2009.
Cornwell, G. C., McCluskey, C. S., Levin, E. J. T., Suski, K. J., DeMott, P. J., Kreidenweis, S. M., and Prather, K. A.: Direct Online Mass Spectrometry Measurements of Ice Nucleating Particles at a California Coastal Site, J. Geophys. Res.-Atmos., 124, 12157–12172, https://doi.org/10.1029/2019JD030466, 2019.
Cozic, J., Mertes, S., Verheggen, B., Cziczo, D. J., Gallavardin, S. J., Walter, S., Baltensperger, U., and Weingartner, E.: Black carbon enrichment in atmospheric ice particle residuals observed in lower tropospheric mixed phase clouds, J. Geophys. Res.-Atmos., 113, D15209, https://doi.org/10.1029/2007JD009266, 2008.
Cui, J., Pandey Deolal, S., Sprenger, M., Henne, S., Staehelin, J., Steinbacher, M., and Nédélec, P.: Free tropospheric ozone changes over Europe as observed at Jungfraujoch (1990–2008): An analysis based on backward trajectories, J. Geophys. Res.-Atmos., 116, D10304, https://doi.org/10.1029/2010JD015154, 2011.
Cziczo, D. J. and Froyd, K. D.: Sampling the composition of cirrus ice residuals, Atmos. Res., 142, 15–31, https://doi.org/10.1016/j.atmosres.2013.06.012, 2014.
Cziczo, D. J., DeMott, P. J., Brock, C., Hudson, P. K., Jesse, B., Kreidenweis, S. M., Prenni, A. J., Schreiner, J., Thomson, D. S., and Murphy, D. M.: A Method for Single Particle Mass Spectrometry of Ice Nuclei, Aerosol Sci. Tech., 37, 460–470, https://doi.org/10.1080/02786820300976, 2003.
Cziczo, D. J., Murphy, D. M., Hudson, P. K., and Thomson, D. S.: Single particle measurements of the chemical composition of cirrus ice residue during CRYSTAL-FACE, J. Geophys. Res.-Atmos., 109, D04201, https://doi.org/10.1029/2003jd004032, 2004.
Cziczo, D. J., Stetzer, O., Worringen, A., Ebert, M., Weinbruch, S., Kamphus, M., Gallavardin, S. J., Curtius, J., Borrmann, S., Froyd, K. D., Mertes, S., Möhler, O., and Lohmann, U.: Inadvertent climate modification due to anthropogenic lead, Nat. Geosci., 2, 333–336, https://doi.org/10.1038/ngeo499, 2009.
Cziczo, D. J., Froyd, K. D., Hoose, C., Jensen, E. J., Diao, M. H., Zondlo, M. A., Smith, J. B., Twohy, C. H., and Murphy, D. M.: Clarifying the Dominant Sources and Mechanisms of Cirrus Cloud Formation, Science, 340, 1320–1324, https://doi.org/10.1126/science.1234145, 2013.
Cziczo, D. J., Ladino, L., Boose, Y., Kanji, Z. A., Kupiszewski, P., Lance, S., Mertes, S., and Wex, H.: Measurements of Ice Nucleating Particles and Ice Residuals, Meteor. Mon., 58, 8.1–8.13, https://doi.org/10.1175/amsmonographs-d-16-0008.1, 2017.
de Boer, G., Morrison, H., Shupe, M. D., and Hildner, R.: Evidence of liquid dependent ice nucleation in high-latitude stratiform clouds from surface remote sensors, Geophys. Res. Lett., 38, L01803, https://doi.org/10.1029/2010GL046016, 2011.
DeMott, P. J., Sassen, K., Poellot, M. R., Baumgardner, D., Rogers, D. C., Brooks, S. D., Prenni, A. J., and Kreidenweis, S. M.: African dust aerosols as atmospheric ice nuclei, Geophys. Res. Lett., 30, 1732, https://doi.org/10.1029/2003GL017410, 2003a.
DeMott, P. J., Cziczo, D. J., Prenni, A. J., Murphy, D. M., Kreidenweis, S. M., Thomson, D. S., Borys, R., and Rogers, D. C.: Measurements of the concentration and composition of nuclei for cirrus formation, P. Natl. Acad. Sci. USA, 100, 14655–14660, https://doi.org/10.1073/pnas.2532677100, 2003b.
DeMott, P. J., Prenni, A. J., Liu, X., Kreidenweis, S. M., Petters, M. D., Twohy, C. H., Richardson, M. S., Eidhammer, T., and Rogers, D. C.: Predicting global atmospheric ice nuclei distributions and their impacts on climate, P. Natl. Acad. Sci. USA, 107, 11217–11222, https://doi.org/10.1073/pnas.0910818107, 2010.
DeMott, P. J., Prenni, A. J., McMeeking, G. R., Sullivan, R. C., Petters, M. D., Tobo, Y., Niemand, M., Möhler, O., Snider, J. R., Wang, Z., and Kreidenweis, S. M.: Integrating laboratory and field data to quantify the immersion freezing ice nucleation activity of mineral dust particles, Atmos. Chem. Phys., 15, 393–409, https://doi.org/10.5194/acp-15-393-2015, 2015.
DeMott, P. J., Hill, T. C. J., McCluskey, C. S., Prather, K. A., Collins, D. B., Sullivan, R. C., Ruppel, M. J., Mason, R. H., Irish, V. E., Lee, T., Hwang, C. Y., Rhee, T. S., Snider, J. R., McMeeking, G. R., Dhaniyala, S., Lewis, E. R., Wentzell, J. J. B., Abbatt, J., Lee, C., Sultana, C. M., Ault, A. P., Axson, J. L., Diaz Martinez, M., Venero, I., Santos-Figueroa, G., Stokes, M. D., Deane, G. B., Mayol-Bracero, O. L., Grassian, V. H., Bertram, T. H., Bertram, A. K., Moffett, B. F., and Franc, G. D.: Sea spray aerosol as a unique source of ice nucleating particles, P. Natl. Acad. Sci. USA, 113, 5797–5803, https://doi.org/10.1073/pnas.1514034112, 2016.
DeMott, P. J., Möhler, O., Cziczo, D. J., Hiranuma, N., Petters, M. D., Petters, S. S., Belosi, F., Bingemer, H. G., Brooks, S. D., Budke, C., Burkert-Kohn, M., Collier, K. N., Danielczok, A., Eppers, O., Felgitsch, L., Garimella, S., Grothe, H., Herenz, P., Hill, T. C. J., Höhler, K., Kanji, Z. A., Kiselev, A., Koop, T., Kristensen, T. B., Krüger, K., Kulkarni, G., Levin, E. J. T., Murray, B. J., Nicosia, A., O'Sullivan, D., Peckhaus, A., Polen, M. J., Price, H. C., Reicher, N., Rothenberg, D. A., Rudich, Y., Santachiara, G., Schiebel, T., Schrod, J., Seifried, T. M., Stratmann, F., Sullivan, R. C., Suski, K. J., Szakáll, M., Taylor, H. P., Ullrich, R., Vergara-Temprado, J., Wagner, R., Whale, T. F., Weber, D., Welti, A., Wilson, T. W., Wolf, M. J., and Zenker, J.: The Fifth International Workshop on Ice Nucleation phase 2 (FIN-02): laboratory intercomparison of ice nucleation measurements, Atmos. Meas. Tech., 11, 6231–6257, https://doi.org/10.5194/amt-11-6231-2018, 2018.
Desai, N., Chandrakar, K. K., Kinney, G., Cantrell, W., and Shaw, R. A.: Aerosol-Mediated Glaciation of Mixed-Phase Clouds: Steady-State Laboratory Measurements, Geophys. Res. Lett., 46, 9154–9162, https://doi.org/10.1029/2019gl083503, 2019.
Drewnick, F., Hings, S. S., DeCarlo, P., Jayne, J. T., Gonin, M., Fuhrer, K., Weimer, S., Jimenez, J. L., Demerjian, K. L., Borrmann, S., and Worsnop, D. R.: A New Time-of-Flight Aerosol Mass Spectrometer (TOF-AMS) – Instrument Description and First Field Deployment, Aerosol Sci. Technol., 39, 637–658, https://doi.org/10.1080/02786820500182040, 2005.
Ebert, M., Worringen, A., Benker, N., Mertes, S., Weingartner, E., and Weinbruch, S.: Chemical composition and mixing-state of ice residuals sampled within mixed phase clouds, Atmos. Chem. Phys., 11, 2805–2816, https://doi.org/10.5194/acp-11-2805-2011, 2011.
Eriksen Hammer, S., Mertes, S., Schneider, J., Ebert, M., Kandler, K., and Weinbruch, S.: Composition of ice particle residuals in mixed-phase clouds at Jungfraujoch (Switzerland): enrichment and depletion of particle groups relative to total aerosol, Atmos. Chem. Phys., 18, 13987–14003, https://doi.org/10.5194/acp-18-13987-2018, 2018.
Farrington, R. J., Connolly, P. J., Lloyd, G., Bower, K. N., Flynn, M. J., Gallagher, M. W., Field, P. R., Dearden, C., and Choularton, T. W.: Comparing model and measured ice crystal concentrations in orographic clouds during the INUPIAQ campaign, Atmos. Chem. Phys., 16, 4945–4966, https://doi.org/10.5194/acp-16-4945-2016, 2016.
Field, P. R. and Heymsfield, A. J.: Importance of snow to global precipitation, Geophys. Res. Lett., 42, 9512–9520, https://doi.org/10.1002/2015gl065497, 2015.
Formenti, P., Elbert, W., Maenhaut, W., Haywood, J., and Andreae, M. O.: Chemical composition of mineral dust aerosol during the Saharan Dust Experiment (SHADE) airborne campaign in the Cape Verde region, September 2000, J. Geophys. Res.-Atmos., 108, 8576, https://doi.org/10.1029/2002JD002648, 2003.
Friedman, B., Kulkarni, G., Beránek, J., Zelenyuk, A., Thornton, J. A., and Cziczo, D. J.: Ice nucleation and droplet formation by bare and coated soot particles, J. Geophys. Res.-Atmos., 116, D17203, https://doi.org/10.1029/2011JD015999, 2011.
Froyd, K. D., Murphy, S. M., Murphy, D. M., de Gouw, J. A., Eddingsaas, N. C., and Wennberg, P. O.: Contribution of isoprene-derived organosulfates to free tropospheric aerosol mass, P. Natl. Acad. Sci. USA, 107, 21360–21365, https://doi.org/10.1073/pnas.1012561107, 2010.
Fu, S. and Xue, H.: The Effect of Ice Nuclei Efficiency on Arctic Mixed-Phase Clouds from Large-Eddy Simulations, J. Atmos. Sci., 74, 3901–3913, https://doi.org/10.1175/jas-d-17-0112.1, 2017.
Garcia, E., Hill, T. C. J., Prenni, A. J., DeMott, P. J., Franc, G. D., and Kreidenweis, S. M.: Biogenic ice nuclei in boundary layer air over two U. S. High Plains agricultural regions, J. Geophys. Res.-Atmos., 117, D18209, https://doi.org/10.1029/2012JD018343, 2012.
Gard, E. E., Kleeman, M. J., Gross, D. S., Hughes, L. S., Allen, J. O., Morrical, B. D., Fergenson, D. P., Dienes, T., E. Gälli, M., Johnson, R. J., Cass, G. R., and Prather, K. A.: Direct Observation of Heterogeneous Chemistry in the Atmosphere, Science, 279, 1184–1187, https://doi.org/10.1126/science.279.5354.1184, 1998.
Gemayel, R., Hellebust, S., Temime-Roussel, B., Hayeck, N., Van Elteren, J. T., Wortham, H., and Gligorovski, S.: The performance and the characterization of laser ablation aerosol particle time-of-flight mass spectrometry (LAAP-ToF-MS), Atmos. Meas. Tech., 9, 1947–1959, https://doi.org/10.5194/amt-9-1947-2016, 2016.
Gute, E., Lacher, L., Kanji, Z. A., Kohl, R., Curtius, J., Weber, D., Bingemer, H., Clemen, H.-C., Schneider, J., Gysel-Beer, M., Ferguson, S. T., and Abbatt, J. P. D.: Field evaluation of a Portable Fine Particle Concentrator (PFPC) for ice nucleating particle measurements, Aerosol Sci. Tech., 53, 1067–1078, https://doi.org/10.1080/02786826.2019.1626346, 2019.
Hallett, J. and Mossop, S. C.: Production of secondary ice particles during the riming process, Nature, 249, 26–28, https://doi.org/10.1038/249026a0, 1974.
Hammer, E., Bukowiecki, N., Gysel, M., Jurányi, Z., Hoyle, C. R., Vogt, R., Baltensperger, U., and Weingartner, E.: Investigation of the effective peak supersaturation for liquid-phase clouds at the high-alpine site Jungfraujoch, Switzerland (3580 m a.s.l.), Atmos. Chem. Phys., 14, 1123–1139, https://doi.org/10.5194/acp-14-1123-2014, 2014.
Harrison, A. D., Whale, T. F., Carpenter, M. A., Holden, M. A., Neve, L., O'Sullivan, D., Vergara Temprado, J., and Murray, B. J.: Not all feldspars are equal: a survey of ice nucleating properties across the feldspar group of minerals, Atmos. Chem. Phys., 16, 10927–10940, https://doi.org/10.5194/acp-16-10927-2016, 2016.
Herrmann, E., Weingartner, E., Henne, S., Vuilleumier, L., Bukowiecki, N., Steinbacher, M., Conen, F., Collaud Coen, M., Hammer, E., Jurányi, Z., Baltensperger, U., and Gysel, M.: Analysis of long-term aerosol size distribution data from Jungfraujoch with emphasis on free tropospheric conditions, cloud influence, and air mass transport, J. Geophys. Res.-Atmos., 120, 9459–9480, https://doi.org/10.1002/2015JD023660, 2015.
Heymsfield, A. J., Schmitt, C., Chen, C.-C.-J., Bansemer, A., Gettelman, A., Field, P. R., and Liu, C.: Contributions of the Liquid and Ice Phases to Global Surface Precipitation: Observations and Global Climate Modeling, J. Atmos. Sci., 77, 2629–2648, https://doi.org/10.1175/jas-d-19-0352.1, 2020.
Hill, T. C. J., DeMott, P. J., Tobo, Y., Fröhlich-Nowoisky, J., Moffett, B. F., Franc, G. D., and Kreidenweis, S. M.: Sources of organic ice nucleating particles in soils, Atmos. Chem. Phys., 16, 7195–7211, https://doi.org/10.5194/acp-16-7195-2016, 2016.
Hinz, K. P., Greweling, M., Drews, F., and Spengler, B.: Data processing in on-line laser mass spectrometry of inorganic, organic, or biological airborne particles, J. Am. Soc. Mass Spectr., 10, 648–660, https://doi.org/10.1016/S1044-0305(99)00028-8, 1999.
Hiranuma, N., Möhler, O., Kulkarni, G., Schnaiter, M., Vogt, S., Vochezer, P., Järvinen, E., Wagner, R., Bell, D. M., Wilson, J., Zelenyuk, A., and Cziczo, D. J.: Development and characterization of an ice-selecting pumped counterflow virtual impactor (IS-PCVI) to study ice crystal residuals, Atmos. Meas. Tech., 9, 3817–3836, https://doi.org/10.5194/amt-9-3817-2016, 2016.
Hoose, C. and Möhler, O.: Heterogeneous ice nucleation on atmospheric aerosols: a review of results from laboratory experiments, Atmos. Chem. Phys., 12, 9817–9854, https://doi.org/10.5194/acp-12-9817-2012, 2012.
Hoose, C., Kristjánsson, J. E., and Burrows, S. M.: How important is biological ice nucleation in clouds on a global scale?, Environ. Res. Lett., 5, 024009, https://doi.org/10.1088/1748-9326/5/2/024009, 2010.
Huang, W. T. K., Ickes, L., Tegen, I., Rinaldi, M., Ceburnis, D., and Lohmann, U.: Global relevance of marine organic aerosol as ice nucleating particles, Atmos. Chem. Phys., 18, 11423–11445, https://doi.org/10.5194/acp-18-11423-2018, 2018.
Huffman, J. A., Prenni, A. J., DeMott, P. J., Pöhlker, C., Mason, R. H., Robinson, N. H., Fröhlich-Nowoisky, J., Tobo, Y., Després, V. R., Garcia, E., Gochis, D. J., Harris, E., Müller-Germann, I., Ruzene, C., Schmer, B., Sinha, B., Day, D. A., Andreae, M. O., Jimenez, J. L., Gallagher, M., Kreidenweis, S. M., Bertram, A. K., and Pöschl, U.: High concentrations of biological aerosol particles and ice nuclei during and after rain, Atmos. Chem. Phys., 13, 6151–6164, https://doi.org/10.5194/acp-13-6151-2013, 2013.
Irish, V. E., Hanna, S. J., Willis, M. D., China, S., Thomas, J. L., Wentzell, J. J. B., Cirisan, A., Si, M., Leaitch, W. R., Murphy, J. G., Abbatt, J. P. D., Laskin, A., Girard, E., and Bertram, A. K.: Ice nucleating particles in the marine boundary layer in the Canadian Arctic during summer 2014, Atmos. Chem. Phys., 19, 1027–1039, https://doi.org/10.5194/acp-19-1027-2019, 2019.
Jahn, L. G., Polen, M. J., Jahl, L. G., Brubaker, T. A., Somers, J., and Sullivan, R. C.: Biomass combustion produces ice-active minerals in biomass-burning aerosol and bottom ash, P. Natl. Acad. Sci. USA, 117, 21928–21937, https://doi.org/10.1073/pnas.1922128117, 2020.
Jiang, H., Yin, Y., Wang, X., Gao, R., Yuan, L., Chen, K., and Shan, Y.: The measurement and parameterization of ice nucleating particles in different backgrounds of China, Atmos. Res., 181, 72–80, https://doi.org/10.1016/j.atmosres.2016.06.013, 2016.
Kammermann, L., Gysel, M., Weingartner, E., and Baltensperger, U.: 13 month climatology of the aerosol hygroscopicity at the free tropospheric site Jungfraujoch (3580 m a.s.l.), Atmos. Chem. Phys., 10, 10717–10732, https://doi.org/10.5194/acp-10-10717-2010, 2010.
Kamphus, M., Ettner-Mahl, M., Klimach, T., Drewnick, F., Keller, L., Cziczo, D. J., Mertes, S., Borrmann, S., and Curtius, J.: Chemical composition of ambient aerosol, ice residues and cloud droplet residues in mixed-phase clouds: single particle analysis during the Cloud and Aerosol Characterization Experiment (CLACE 6), Atmos. Chem. Phys., 10, 8077–8095, https://doi.org/10.5194/acp-10-8077-2010, 2010.
Kanji, Z. A., Ladino, L. A., Wex, H., Boose, Y., Burkert-Kohn, M., Cziczo, D. J., and Krämer, M.: Overview of Ice Nucleating Particles, Meteor. Mon., 58, 1.1–1.33, https://doi.org/10.1175/amsmonographs-d-16-0006.1, 2017.
Kanji, Z. A., Sullivan, R. C., Niemand, M., DeMott, P. J., Prenni, A. J., Chou, C., Saathoff, H., and Möhler, O.: Heterogeneous ice nucleation properties of natural desert dust particles coated with a surrogate of secondary organic aerosol, Atmos. Chem. Phys., 19, 5091–5110, https://doi.org/10.5194/acp-19-5091-2019, 2019.
Kanji, Z. A., Welti, A., Corbin, J. C., and Mensah, A. A.: Black Carbon Particles Do Not Matter for Immersion Mode Ice Nucleation, Geophys. Res. Lett., 47, e2019GL086764, https://doi.org/10.1029/2019GL086764, 2020.
Karlsson, L., Krejci, R., Koike, M., Ebell, K., and Zieger, P.: A long-term study of cloud residuals from low-level Arctic clouds, Atmos. Chem. Phys., 21, 8933–8959, https://doi.org/10.5194/acp-21-8933-2021, 2021.
Kaye, P. H., Stanley, W. R., Hirst, E., Foot, E. V., Baxter, K. L., and Barrington, S. J.: Single particle multichannel bio-aerosol fluorescence sensor, Opt. Express, 13, 3583–3593, https://doi.org/10.1364/OPEX.13.003583, 2005.
Klein, H., Nickovic, S., Haunold, W., Bundke, U., Nillius, B., Ebert, M., Weinbruch, S., Schuetz, L., Levin, Z., Barrie, L. A., and Bingemer, H.: Saharan dust and ice nuclei over Central Europe, Atmos. Chem. Phys., 10, 10211–10221, https://doi.org/10.5194/acp-10-10211-2010, 2010.
Korolev, A. and Leisner, T.: Review of experimental studies of secondary ice production, Atmos. Chem. Phys., 20, 11767–11797, https://doi.org/10.5194/acp-20-11767-2020, 2020.
Kulkarni, G., Sanders, C., Zhang, K., Liu, X., and Zhao, C.: Ice nucleation of bare and sulfuric acid-coated mineral dust particles and implication for cloud properties, J. Geophys. Res.-Atmos., 119, 9993–10011, https://doi.org/10.1002/2014JD021567, 2014.
Kupiszewski, P., Weingartner, E., Vochezer, P., Schnaiter, M., Bigi, A., Gysel, M., Rosati, B., Toprak, E., Mertes, S., and Baltensperger, U.: The Ice Selective Inlet: a novel technique for exclusive extraction of pristine ice crystals in mixed-phase clouds, Atmos. Meas. Tech., 8, 3087–3106, https://doi.org/10.5194/amt-8-3087-2015, 2015.
Kupiszewski, P., Zanatta, M., Mertes, S., Vochezer, P., Lloyd, G., Schneider, J., Schenk, L., Schnaiter, M., Baltensperger, U., Weingartner, E., and Gysel, M.: Ice residual properties in mixed-phase clouds at the high-alpine Jungfraujoch site, J. Geophys. Res.-Atmos., 121, 12343–12362, https://doi.org/10.1002/2016JD024894, 2016.
Lacher, L., Lohmann, U., Boose, Y., Zipori, A., Herrmann, E., Bukowiecki, N., Steinbacher, M., and Kanji, Z. A.: The Horizontal Ice Nucleation Chamber (HINC): INP measurements at conditions relevant for mixed-phase clouds at the High Altitude Research Station Jungfraujoch, Atmos. Chem. Phys., 17, 15199–15224, https://doi.org/10.5194/acp-17-15199-2017, 2017.
Lacher, L., DeMott, P. J., Levin, E. J. T., Suski, K. J., Boose, Y., Zipori, A., Herrmann, E., Bukowiecki, N., Steinbacher, M., Gute, E., Abbatt, J. P. D., Lohmann, U., and Kanji, Z. A.: Background Free-Tropospheric Ice Nucleating Particle Concentrations at Mixed-Phase Cloud Conditions, J. Geophys. Res.-Atmos., 123, 10506–10525, https://doi.org/10.1029/2018JD028338, 2018a.
Lacher, L., Steinbacher, M., Bukowiecki, N., Herrmann, E., Zipori, A., and Kanji, Z. A.: Impact of Air Mass Conditions and Aerosol Properties on Ice Nucleating Particle Concentrations at the High Altitude Research Station Jungfraujoch, Atmosphere, 9, 363, https://doi.org/10.3390/atmos9090363, 2018b.
Lacher, L., Clemen, H.-C., Shen, X., Mertes, S., Gysel-Beer, M., Moallemi, A., Steinbacher, M., Henne, S., Saathoff, H., Möhler, O., Höhler, K., Schiebel, T., Weber, D., Schrod, J., Schneider, J., and Kanji, Z. A.: Sources and nature of ice-nucleating particles in the free troposphere at Jungfraujoch in winter 2017, ETH Zürich [data set], https://doi.org/10.3929/ethz-b-000484207, 2021.
Ladino, L. A., Raga, G. B., Alvarez-Ospina, H., Andino-Enríquez, M. A., Rosas, I., Martínez, L., Salinas, E., Miranda, J., Ramírez-Díaz, Z., Figueroa, B., Chou, C., Bertram, A. K., Quintana, E. T., Maldonado, L. A., García-Reynoso, A., Si, M., and Irish, V. E.: Ice-nucleating particles in a coastal tropical site, Atmos. Chem. Phys., 19, 6147–6165, https://doi.org/10.5194/acp-19-6147-2019, 2019.
Lin, Q., Zhang, G., Peng, L., Bi, X., Wang, X., Brechtel, F. J., Li, M., Chen, D., Peng, P., Sheng, G., and Zhou, Z.: In situ chemical composition measurement of individual cloud residue particles at a mountain site, southern China, Atmos. Chem. Phys., 17, 8473–8488, https://doi.org/10.5194/acp-17-8473-2017, 2017.
Lloyd, G., Choularton, T. W., Bower, K. N., Gallagher, M. W., Connolly, P. J., Flynn, M., Farrington, R., Crosier, J., Schlenczek, O., Fugal, J., and Henneberger, J.: The origins of ice crystals measured in mixed-phase clouds at the high-alpine site Jungfraujoch, Atmos. Chem. Phys., 15, 12953–12969, https://doi.org/10.5194/acp-15-12953-2015, 2015.
Lohmann, U.: A glaciation indirect aerosol effect caused by soot aerosols, Geophys. Res. Lett., 29, 11-11–11-14, https://doi.org/10.1029/2001GL014357, 2002.
Lohmann, U., Henneberger, J., Henneberg, O., Fugal, J. P., Bühl, J., and Kanji, Z. A.: Persistence of orographic mixed-phase clouds, Geophys. Res. Lett., 43, 10512–10519, https://doi.org/10.1002/2016gl071036, 2016.
Mahrt, F., Marcolli, C., David, R. O., Grönquist, P., Barthazy Meier, E. J., Lohmann, U., and Kanji, Z. A.: Ice nucleation abilities of soot particles determined with the Horizontal Ice Nucleation Chamber, Atmos. Chem. Phys., 18, 13363–13392, https://doi.org/10.5194/acp-18-13363-2018, 2018.
Mason, R. H., Si, M., Li, J., Chou, C., Dickie, R., Toom-Sauntry, D., Pöhlker, C., Yakobi-Hancock, J. D., Ladino, L. A., Jones, K., Leaitch, W. R., Schiller, C. L., Abbatt, J. P. D., Huffman, J. A., and Bertram, A. K.: Ice nucleating particles at a coastal marine boundary layer site: correlations with aerosol type and meteorological conditions, Atmos. Chem. Phys., 15, 12547–12566, https://doi.org/10.5194/acp-15-12547-2015, 2015.
Mason, R. H., Si, M., Chou, C., Irish, V. E., Dickie, R., Elizondo, P., Wong, R., Brintnell, M., Elsasser, M., Lassar, W. M., Pierce, K. M., Leaitch, W. R., MacDonald, A. M., Platt, A., Toom-Sauntry, D., Sarda-Estève, R., Schiller, C. L., Suski, K. J., Hill, T. C. J., Abbatt, J. P. D., Huffman, J. A., DeMott, P. J., and Bertram, A. K.: Size-resolved measurements of ice-nucleating particles at six locations in North America and one in Europe, Atmos. Chem. Phys., 16, 1637–1651, https://doi.org/10.5194/acp-16-1637-2016, 2016.
McCluskey, C. S., Hill, T. C. J., Humphries, R. S., Rauker, A. M., Moreau, S., Strutton, P. G., Chambers, S. D., Williams, A. G., McRobert, I., Ward, J., Keywood, M. D., Harnwell, J., Ponsonby, W., Loh, Z. M., Krummel, P. B., Protat, A., Kreidenweis, S. M., and DeMott, P. J.: Observations of Ice Nucleating Particles Over Southern Ocean Waters, Geophys. Res. Lett., 45, 11989–11997, https://doi.org/10.1029/2018gl079981, 2018.
McCluskey, C. S., DeMott, P. J., Ma, P.-L., and Burrows, S. M.: Numerical Representations of Marine Ice-Nucleating Particles in Remote Marine Environments Evaluated Against Observations, Geophys. Res. Lett., 46, 7838–7847, https://doi.org/10.1029/2018GL081861, 2019.
Mertes, S., Verheggen, B., Walter, S., Connolly, P., Ebert, M., Schneider, J., Bower, K. N., Cozic, J., Weinbruch, S., Baltensperger, U., and Weingartner, E.: Counterflow Virtual Impactor Based Collection of Small Ice Particles in Mixed-Phase Clouds for the Physico-Chemical Characterization of Tropospheric Ice Nuclei: Sampler Description and First Case Study, Aerosol Sci. Tech., 41, 848–864, https://doi.org/10.1080/02786820701501881, 2007.
Mülmenstädt, J., Sourdeval, O., Delanoë, J., and Quaas, J.: Frequency of occurrence of rain from liquid-, mixed-, and ice-phase clouds derived from A-Train satellite retrievals, Geophys. Res. Lett., 42, 6502–6509, https://doi.org/10.1002/2015GL064604, 2015.
Murphy, D. M.: The design of single particle laser mass spectrometers, Mass Spectrom. Rev., 26, 150–165, https://doi.org/10.1002/mas.20113, 2007.
Murray, B. J., O'Sullivan, D., Atkinson, J. D., and Webb, M. E.: Ice nucleation by particles immersed in supercooled cloud droplets, Chem. Soc. Rev., 41, 6519–6554, https://doi.org/10.1039/C2CS35200A, 2012.
Nyeki, S., Li, F., Weingartner, E., Streit, N., Colbeck, I., Gäggeler, H. W., and Baltensperger, U.: The background aerosol size distribution in the free troposphere: An analysis of the annual cycle at a high-alpine site, J. Geophys. Res., 103, 31749–31761, https://doi.org/10.1029/1998JD200029, 1998.
O'Sullivan, D., Adams, M. P., Tarn, M. D., Harrison, A. D., Vergara-Temprado, J., Porter, G. C. E., Holden, M. A., Sanchez-Marroquin, A., Carotenuto, F., Whale, T. F., McQuaid, J. B., Walshaw, R., Hedges, D. H. P., Burke, I. T., Cui, Z., and Murray, B. J.: Contributions of biogenic material to the atmospheric ice-nucleating particle population in North Western Europe, Sci. Rep.-UK, 8, 13821, https://doi.org/10.1038/s41598-018-31981-7, 2018.
Pandey Deolal, S., Henne, S., Ries, L., Gilge, S., Weers, U., Steinbacher, M., Staehelin, J., and Peter, T.: Analysis of elevated springtime levels of Peroxyacetyl nitrate (PAN) at the high Alpine research sites Jungfraujoch and Zugspitze, Atmos. Chem. Phys., 14, 12553–12571, https://doi.org/10.5194/acp-14-12553-2014, 2014.
Paramonov, M., Drossaart van Dusseldorp, S., Gute, E., Abbatt, J. P. D., Heikkilä, P., Keskinen, J., Chen, X., Luoma, K., Heikkinen, L., Hao, L., Petäjä, T., and Kanji, Z. A.: Condensation/immersion mode ice-nucleating particles in a boreal environment, Atmos. Chem. Phys., 20, 6687–6706, https://doi.org/10.5194/acp-20-6687-2020, 2020.
Pöhlker, C., Huffman, J. A., Förster, J.-D., and Pöschl, U.: Autofluorescence of atmospheric bioaerosols: spectral fingerprints and taxonomic trends of pollen, Atmos. Meas. Tech., 6, 3369–3392, https://doi.org/10.5194/amt-6-3369-2013, 2013.
Pratt, K. A., DeMott, P. J., French, J. R., Wang, Z., Westphal, D. L., Heymsfield, A. J., Twohy, C. H., Prenni, A. J., and Prather, K. A.: In situ detection of biological particles in cloud ice-crystals, Nat. Geosci., 2, 398, https://doi.org/10.1038/ngeo521, 2009.
Price, H. C., Baustian, K. J., McQuaid, J. B., Blyth, A., Bower, K. N., Choularton, T., Cotton, R. J., Cui, Z., Field, P. R., Gallagher, M., Hawker, R., Merrington, A., Miltenberger, A., Neely III, R. R., Parker, S. T., Rosenberg, P. D., Taylor, J. W., Trembath, J., Vergara-Temprado, J., Whale, T. F., Wilson, T. W., Young, G., and Murray, B. J.: Atmospheric Ice-Nucleating Particles in the Dusty Tropical Atlantic, J. Geophys. Res.-Atmos., 123, 2175–2193, https://doi.org/10.1002/2017jd027560, 2018.
Prospero, J. M., Ginoux, P., Torres, O., Nicholson, S. E., and Gill, T. E.: Environmental characterization of global sources of atmospheric soil dust identified with the Nimbus 7 Total Ozone Mapping Spectrometer (TOMS) absorbing aerosol product, Rev. Geophys., 40, 2-1–2-31, https://doi.org/10.1029/2000RG000095, 2002.
Pruppacher, H. R. and Klett, J. D.: Microphysics of Clouds and Precipitation, Kluwer Acad., Norwell, Mass, 1997.
Ramisetty, R., Abdelmonem, A., Shen, X., Saathoff, H., Leisner, T., and Mohr, C.: Exploring femtosecond laser ablation in single-particle aerosol mass spectrometry, Atmos. Meas. Tech., 11, 4345–4360, https://doi.org/10.5194/amt-11-4345-2018, 2018.
Reitz, P., Zorn, S. R., Trimborn, S. H., and Trimborn, A. M.: A new, powerful technique to analyze single particle aerosol mass spectra using a combination of OPTICS and the fuzzy c-means algorithm, J. Aerosol Sci., 98, 1–14, https://doi.org/10.1016/j.jaerosci.2016.04.003, 2016.
Richardson, M. S., DeMott, P. J., Kreidenweis, S. M., Cziczo, D. J., Dunlea, E. J., Jimenez, J. L., Thomson, D. S., Ashbaugh, L. L., Borys, R. D., Westphal, D. L., Casuccio, G. S., and Lersch, T. L.: Measurements of heterogeneous ice nuclei in the western United States in springtime and their relation to aerosol characteristics, J. Geophys. Res.-Atmos., 112, D02209, https://doi.org/10.1029/2006jd007500, 2007.
Rogers, D. C., DeMott, P. J., Kreidenweis, S. M., and Chen, Y.: Measurements of ice nucleating aerosols during SUCCESS, Geophys. Res. Lett., 25, 1383–1386, https://doi.org/10.1029/97GL03478, 1998.
Roth, A., Schneider, J., Klimach, T., Mertes, S., van Pinxteren, D., Herrmann, H., and Borrmann, S.: Aerosol properties, source identification, and cloud processing in orographic clouds measured by single particle mass spectrometry on a central European mountain site during HCCT-2010, Atmos. Chem. Phys., 16, 505–524, https://doi.org/10.5194/acp-16-505-2016, 2016.
Sassen, K., DeMott, P. J., Prospero, J. M., and Poellot, M. R.: Saharan dust storms and indirect aerosol effects on clouds: CRYSTAL-FACE results, Geophys. Res. Lett., 30, 1633, https://doi.org/10.1029/2003gl017371, 2003.
Savage, N. J., Krentz, C. E., Könemann, T., Han, T. T., Mainelis, G., Pöhlker, C., and Huffman, J. A.: Systematic characterization and fluorescence threshold strategies for the wideband integrated bioaerosol sensor (WIBS) using size-resolved biological and interfering particles, Atmos. Meas. Tech., 10, 4279–4302, https://doi.org/10.5194/amt-10-4279-2017, 2017.
Schill, G. P., DeMott, P. J., Emerson, E. W., Rauker, A. M. C., Kodros, J. K., Suski, K. J., Hill, T. C. J., Levin, E. J. T., Pierce, J. R., Farmer, D. K., and Kreidenweis, S. M.: The contribution of black carbon to global ice nucleating particle concentrations relevant to mixed-phase clouds, P. Natl. Acad. Sci. USA, 117, 22705–22711, https://doi.org/10.1073/pnas.2001674117, 2020.
Schmidt, S., Schneider, J., Klimach, T., Mertes, S., Schenk, L. P., Kupiszewski, P., Curtius, J., and Borrmann, S.: Online single particle analysis of ice particle residuals from mountain-top mixed-phase clouds using laboratory derived particle type assignment, Atmos. Chem. Phys., 17, 575–594, https://doi.org/10.5194/acp-17-575-2017, 2017.
Schnell, R. C.: Ice Nuclei in Seawater, Fog Water and Marine Air off the Coast of Nova Scotia: Summer 1975, J. Atmos. Sci., 34, 1299–1305, https://doi.org/10.1175/1520-0469(1977)034<1299:inisfw>2.0.co;2, 1977.
Schrod, J., Danielczok, A., Weber, D., Ebert, M., Thomson, E. S., and Bingemer, H. G.: Re-evaluating the Frankfurt isothermal static diffusion chamber for ice nucleation, Atmos. Meas. Tech., 9, 1313–1324, https://doi.org/10.5194/amt-9-1313-2016, 2016.
Sesartic, A., Lohmann, U., and Storelvmo, T.: Modelling the impact of fungal spore ice nuclei on clouds and precipitation, Environ. Res. Lett., 8, 014029, https://doi.org/10.1088/1748-9326/8/1/014029, 2013.
Shen, X., Ramisetty, R., Mohr, C., Huang, W., Leisner, T., and Saathoff, H.: Laser ablation aerosol particle time-of-flight mass spectrometer (LAAPTOF): performance, reference spectra and classification of atmospheric samples, Atmos. Meas. Tech., 11, 2325–2343, https://doi.org/10.5194/amt-11-2325-2018, 2018.
Shen, X., Saathoff, H., Huang, W., Mohr, C., Ramisetty, R., and Leisner, T.: Understanding atmospheric aerosol particles with improved particle identification and quantification by single-particle mass spectrometry, Atmos. Meas. Tech., 12, 2219–2240, https://doi.org/10.5194/amt-12-2219-2019, 2019.
Si, M., Irish, V. E., Mason, R. H., Vergara-Temprado, J., Hanna, S. J., Ladino, L. A., Yakobi-Hancock, J. D., Schiller, C. L., Wentzell, J. J. B., Abbatt, J. P. D., Carslaw, K. S., Murray, B. J., and Bertram, A. K.: Ice-nucleating ability of aerosol particles and possible sources at three coastal marine sites, Atmos. Chem. Phys., 18, 15669–15685, https://doi.org/10.5194/acp-18-15669-2018, 2018.
Sierau, B., Chang, R. Y.-W., Leck, C., Paatero, J., and Lohmann, U.: Single-particle characterization of the high-Arctic summertime aerosol, Atmos. Chem. Phys., 14, 7409–7430, https://doi.org/10.5194/acp-14-7409-2014, 2014.
Silva, P. J., Liu, D.-Y., Noble, C. A., and Prather, K. A.: Size and Chemical Characterization of Individual Particles Resulting from Biomass Burning of Local Southern California Species, Environ. Sci. Technol., 33, 3068–3076, https://doi.org/10.1021/es980544p, 1999.
Solomon, A., de Boer, G., Creamean, J. M., McComiskey, A., Shupe, M. D., Maahn, M., and Cox, C.: The relative impact of cloud condensation nuclei and ice nucleating particle concentrations on phase partitioning in Arctic mixed-phase stratocumulus clouds, Atmos. Chem. Phys., 18, 17047–17059, https://doi.org/10.5194/acp-18-17047-2018, 2018.
Spearman, C.: The Proof and Measurement of Association between Two Things, Am. J. Psychol., 15, 72–101, https://doi.org/10.2307/1412159, 1904.
Steinbacher, M., Hüglin, C., and Emmenegger, L.: Surface ozone observations in the historical perspective, High Altitude Research Stations Jungfraujoch and Gornergrat Activity Report 2019, 25–29, https://www.hfsjg.ch/en/publications/activity-reports/activity-report-2019/ (last access: 14 November 2021), 2020.
Stohl, A., Forster, C., Frank, A., Seibert, P., and Wotawa, G.: Technical note: The Lagrangian particle dispersion model FLEXPART version 6.2, Atmos. Chem. Phys., 5, 2461–2474, https://doi.org/10.5194/acp-5-2461-2005, 2005.
Sturm, P., Tuzson, B., Henne, S., and Emmenegger, L.: Tracking isotopic signatures of CO2 at the high altitude site Jungfraujoch with laser spectroscopy: analytical improvements and representative results, Atmos. Meas. Tech., 6, 1659–1671, https://doi.org/10.5194/amt-6-1659-2013, 2013.
Tang, M., Cziczo, D. J., and Grassian, V. H.: Interactions of Water with Mineral Dust Aerosol: Water Adsorption, Hygroscopicity, Cloud Condensation, and Ice Nucleation, Chem. Rev., 116, 4205–4259, https://doi.org/10.1021/acs.chemrev.5b00529, 2016.
Toprak, E. and Schnaiter, M.: Fluorescent biological aerosol particles measured with the Waveband Integrated Bioaerosol Sensor WIBS-4: laboratory tests combined with a one year field study, Atmos. Chem. Phys., 13, 225–243, https://doi.org/10.5194/acp-13-225-2013, 2013.
Vali, G.: Sizes of Atmospheric Ice Nuclei, Nature, 212, 384–385, https://doi.org/10.1038/212384a0, 1966.
Vali, G.: Quantitative Evaluation of Experimental Results an the Heterogeneous Freezing Nucleation of Supercooled Liquids, J. Atmos. Sci., 28, 402–409, https://doi.org/10.1175/1520-0469(1971)028<0402:qeoera>2.0.co;2, 1971.
Vali, G., Leon, D., and Snider, J. R.: Ground-layer snow clouds, Q. J. Roy. Meteorol. Soc., 138, 1507–1525, https://doi.org/10.1002/qj.1882, 2012.
Vergara-Temprado, J., Murray, B. J., Wilson, T. W., O'Sullivan, D., Browse, J., Pringle, K. J., Ardon-Dryer, K., Bertram, A. K., Burrows, S. M., Ceburnis, D., DeMott, P. J., Mason, R. H., O'Dowd, C. D., Rinaldi, M., and Carslaw, K. S.: Contribution of feldspar and marine organic aerosols to global ice nucleating particle concentrations, Atmos. Chem. Phys., 17, 3637–3658, https://doi.org/10.5194/acp-17-3637-2017, 2017.
Vergara-Temprado, J., Miltenberger, A. K., Furtado, K., Grosvenor, D. P., Shipway, B. J., Hill, A. A., Wilkinson, J. M., Field, P. R., Murray, B. J., and Carslaw, K. S.: Strong control of Southern Ocean cloud reflectivity by ice-nucleating particles, P. Natl. Acad. Sci. USA, 115, 2687–2692, https://doi.org/10.1073/pnas.1721627115, 2018a.
Vergara-Temprado, J., Holden, M. A., Orton, T. R., O'Sullivan, D., Umo, N. S., Browse, J., Reddington, C., Baeza-Romero, M. T., Jones, J. M., Lea-Langton, A., Williams, A., Carslaw, K. S., and Murray, B. J.: Is Black Carbon an Unimportant Ice-Nucleating Particle in Mixed-Phase Clouds?, J. Geophys. Res.-Atmos., 123, 4273–4283, https://doi.org/10.1002/2017JD027831, 2018b.
Weingartner, E., Nyeki, S., and Baltensperger, U.: Seasonal and diurnal variation of aerosol size distributions ( nm) at a high-alpine site (Jungfraujoch 3580 m asl), J. Geophys. Res., 104, 26809–26820, https://doi.org/10.1029/1999JD900170, 1999.
Welti, A., Lüönd, F., Kanji, Z. A., Stetzer, O., and Lohmann, U.: Time dependence of immersion freezing: an experimental study on size selected kaolinite particles, Atmos. Chem. Phys., 12, 9893–9907, https://doi.org/10.5194/acp-12-9893-2012, 2012.
Welti, A., Müller, K., Fleming, Z. L., and Stratmann, F.: Concentration and variability of ice nuclei in the subtropical maritime boundary layer, Atmos. Chem. Phys., 18, 5307–5320, https://doi.org/10.5194/acp-18-5307-2018, 2018.
Wilson, T. W., Ladino, L. A., Alpert, P. A., Breckels, M. N., Brooks, I. M., Browse, J., Burrows, S. M., Carslaw, K. S., Huffman, J. A., Judd, C., Kilthau, W. P., Mason, R. H., McFiggans, G., Miller, L. A., Najera, J. J., Polishchuk, E., Rae, S., Schiller, C. L., Si, M., Temprado, J. V., Whale, T. F., Wong, J. P. S., Wurl, O., Yakobi-Hancock, J. D., Abbatt, J. P. D., Aller, J. Y., Bertram, A. K., Knopf, D. A., and Murray, B. J.: A marine biogenic source of atmospheric ice-nucleating particles, Nature, 525, 234–238, https://doi.org/10.1038/nature14986, 2015.
Worringen, A., Kandler, K., Benker, N., Dirsch, T., Mertes, S., Schenk, L., Kästner, U., Frank, F., Nillius, B., Bundke, U., Rose, D., Curtius, J., Kupiszewski, P., Weingartner, E., Vochezer, P., Schneider, J., Schmidt, S., Weinbruch, S., and Ebert, M.: Single-particle characterization of ice-nucleating particles and ice particle residuals sampled by three different techniques, Atmos. Chem. Phys., 15, 4161–4178, https://doi.org/10.5194/acp-15-4161-2015, 2015.
Zellweger, C., Forrer, J., Hofer, P., Nyeki, S., Schwarzenbach, B., Weingartner, E., Ammann, M., and Baltensperger, U.: Partitioning of reactive nitrogen (NOy) and dependence on meteorological conditions in the lower free troposphere, Atmos. Chem. Phys., 3, 779–796, https://doi.org/10.5194/acp-3-779-2003, 2003.