the Creative Commons Attribution 4.0 License.
the Creative Commons Attribution 4.0 License.
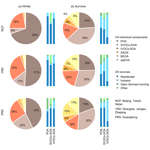
Process-based and observation-constrained SOA simulations in China: the role of semivolatile and intermediate-volatility organic compounds and OH levels
Ruqian Miao
Manish Shrivastava
Youfan Chen
Lin Zhang
Jianlin Hu
Yan Zheng
Keren Liao
Organic aerosol (OA) is a major component of tropospheric submicron aerosol that contributes to air pollution and causes adverse effects on human health. Chemical transport models have difficulties in reproducing the variability in OA concentrations in polluted areas, hindering understanding of the OA budget and sources. Herein, we apply both process-based and observation-constrained schemes to simulate OA in GEOS-Chem. Comprehensive data sets of surface OA, OA components, secondary organic aerosol (SOA) precursors, and oxidants were used for model–observation comparisons. The base models generally underestimate the SOA concentrations in China. In the revised schemes, updates were made on the emissions, volatility distributions, and SOA yields of semivolatile and intermediate-volatility organic compounds (SVOCs and IVOCs) and additional nitrous acid sources. With all the model improvements, both the process-based and observation-constrained SOA schemes can reproduce the observed mass concentrations of SOA and show spatial and seasonal consistency with each other. Our best model simulations suggest that anthropogenic SVOCs and IVOCs are the dominant source of SOA, with a contribution of over 50 % in most of China, which should be considered for pollution mitigation in the future. The residential sector may be the predominant source of SVOCs and IVOCs in winter, despite large uncertainty remaining in the emissions of IVOCs from the residential sector in northern China. The industry sector is also an important source of IVOCs, especially in summer. More SVOC and IVOC measurements are needed to constrain their emissions. Besides, the results highlight the sensitivity of SOA to hydroxyl radical (OH) levels in winter in polluted environments. The addition of nitrous acid sources can lead to over 30 % greater SOA mass concentrations in winter in northern China. It is important to have good OH simulations in air quality models.
- Article
(4726 KB) - Full-text XML
-
Supplement
(1413 KB) - BibTeX
- EndNote
Organic aerosol (OA) is a major component of tropospheric submicron aerosol, which can be directly emitted as primary organic aerosol (POA) or formed from atmospheric oxidation processes as secondary organic aerosol (SOA) (Zhang et al., 2007). Accurate OA simulation is important for understanding the aerosol budget as well as evaluating the impacts of fine particles on air quality and human health. High OA concentrations occur in populated and polluted areas, especially in China and India (Li et al., 2017; Gani et al., 2019). However, atmospheric chemical transport models (CTMs) have difficulties in reproducing the magnitude and the variability in OA mass in polluted environments, mainly resulting from the underestimation of SOA (Park et al., 2021; Miao et al., 2020; Jiang et al., 2019).
SOA is generally simulated in CTMs by the process-based scheme, for which the oxidation of each category of lumped SOA precursors is parameterized with specific SOA yields (Chung and Seinfeld, 2002; Hodzic et al., 2016). Some of the SOA sources are uncertain. For example, the estimated annual production of anthropogenic SOA varied by tens of teragrams per year in different models, which has been attributed largely to the uncertain contribution from semivolatile and intermediate-volatility organic compounds (SVOCs and IVOCs) (Spracklen et al., 2011; Hodzic et al., 2016; Pai et al., 2020). The SVOCs and IVOCs have been recognized as key SOA precursors in polluted areas for over a decade (Robinson et al., 2007; Grieshop et al., 2009). Transportation, industry, and residential use of solid fuel, for example, are all important sources of SVOCs and IVOCs. Although tremendous efforts have been made to characterize their SOA production, CTMs treat their emissions, volatility distributions, reactivities, and SOA yields differently. The emissions of SVOCs and IVOCs are estimated by applying empirical scale factors to different proxies such as POA, non-methane volatility organic compounds (NMVOCs), and speciated IVOCs (Pye and Seinfeld, 2010; Jathar et al., 2011; Shrivastava et al., 2015; Hodzic et al., 2016). The uncertainties can be over 200 % for individual emission sectors, especially at a regional scale (Wu et al., 2021; Lu et al., 2020). For IVOCs, some CTMs use one lumped precursor with specific SOA yields (Pye and Seinfeld, 2010; Hodzic et al., 2016; Ots et al., 2016). Some CTMs use a volatility basis set (VBS) approach for which continuous oxidation occurs to decrease the volatilities of oxidation products and alters gas-to-particle partitioning (Li et al., 2020; Chrit et al., 2018; Shrivastava et al., 2015). Although a recent study categorizes IVOCs into six groups based on volatility and molecular structure for which SOA yield parameters of each group are derived from laboratory experiments of mobile emissions (Lu et al., 2020), there is still a lack of a source-dependent model framework for IVOC-related SOA simulations. A new observation-constrained scheme has been developed in CTMs to improve the simulation of SOA mass in polluted areas, which estimates anthropogenic SOA formation potential based on the emission of carbon monoxide (CO) (Hodzic and Jimenez, 2011). This SOA scheme was able to reproduce the OA mass concentrations in the Mexico City metropolitan area, the United States, and China (Hodzic and Jimenez, 2011; Kim et al., 2015; Woody et al., 2016; Miao et al., 2020). However, its parameterization is too generalized to differentiate specific source contributions. The contribution of SVOCs and IVOCs to SOA in polluted environments remains unclear.
On the other hand, the model performance on atmospheric oxidation capacity may affect the simulation of SOA production. The measured concentrations of hydroxyl radicals (OHs) show high values in polluted environments in China, caused by strong production from ozone (O3) and nitrous acid (HONO) as well as fast radical recycling under high concentrations of nitrogen oxide (NO) (Lu et al., 2019). For CTMs, a large model discrepancy exists in the OH simulation in the Northern Hemisphere (Zhao et al., 2019). Miao et al. (2020) show underestimated surface OH concentrations by over a factor of 2 at noon (local time) in winter in Beijing. The biased OH concentrations affect the magnitude and the spatial distribution of SOA formation in the model, which has not yet been well investigated and quantified (Feng et al., 2019; J. Zhang et al., 2019). An accurate budget analysis and source apportionment of SOA needs to consider the oxidant bias.
Herein, we conduct the OA simulations in China with both of the process-based and observation-constrained schemes in the atmospheric chemical transport model GEOS-Chem. Model improvements are made on the emissions, volatility distributions, and SOA yields of SVOCs and IVOCs as well as the HONO sources. The model simulations are evaluated against nationwide measurements and the positive matrix factorization (PMF)-based source apportionment results of OA. The improved model simulations provide insights into the budget and sources of SOA in China and hence assist in developing control strategies for the SOA pollution.
2.1 Ambient observations
The campaign-average mass concentrations of OA were taken from 68 surface measurements at urban sites, 18 measurements at suburban sites, and 8 measurements at remote sites from 2011 to 2019 (Table S1 in the Supplement). These measurements were conducted by Aerodyne aerosol mass spectrometers (AMSs) and aerosol chemical speciation monitors (ACSMs) and covered mains regions in China, including the North China Plain (NCP), the Yangtze River Delta (YRD), the Pearl River Delta (PRD), and Northwest China (NW). The campaign-average mass concentrations of OA factors that were resolved by PMF analysis were also synthesized. These OA factors include hydrocarbon-like OA (HOA), cooking-related OA (COA), biomass-burning-related OA (BBOA), coal-combustion-related OA (CCOA), and various oxygenated OAs (OOAs). We named the summed concentrations of HOA, COA, BBOA, and CCOA as PMF-derived POA and those of OOAs as PMF-derived SOA. Unlike our previous study (Miao et al., 2020), we did not divide the measured concentrations by the empirical submicron-to-fine mass ratio because of the lack of such information for different regions and seasons (Y. Zheng et al., 2020; Sun et al., 2020a). Because the model simulations consistently underestimate the OA concentrations, taking into account the potential supermicron mass may lead to greater model–observation gaps but not affect the analysis herein. Moreover, we synthesized a data set of the campaign-average concentrations of benzene, toluene, and xylene from 49 measurements in China from 2011 to 2018 that were conducted by online gas chromatography (GC) coupled with a flame ionization detector (FID) and/or mass spectrometer (MS). Table S2 in the Supplement lists the sampling information and the results of these measurements. In addition, a recent result of primary IVOCs measured by offline sampling with thermal desorption (TD)–GC–MS in urban Shanghai (31∘17′ N, 121∘44′ E) from 5 December 2016 to 3 January 2017 and from 16 July to 8 August 2017 was used for comparisons in this study (Y. Li et al., 2019). We also included 28 measurements of HONO from 2011 to 2019 and 10 measurements of OH and hydroperoxy radical (HO2) from 2014 to 2019 in China in the analysis (Tables S3 and S4 in the Supplement).
2.2 Model configurations
Model simulations were conducted on an atmospheric chemical transport model GEOS-Chem v12.6.3 (https://doi.org/10.5281/zenodo.3552959) with a horizontal resolution of 0.5∘ × 0.625∘ over Asia and the adjacent area (11∘ S–55∘ N, 60–150∘ E). The model was set for 47 vertical levels from the surface to 0.01 hPa and was driven by the MERRA2 reanalysis assimilated meteorological data. The boundary conditions were generated by global simulations under a horizontal resolution of 2∘ × 2.5∘. The model simulated the ozone–nitrogen oxides (NOx)–hydrocarbon–aerosol chemistry with the ISORROPIA-II thermodynamic equilibrium model (Park et al., 2004; Fountoukis and Nenes, 2007). Global anthropogenic and biogenic emissions and global biomass burning emissions were provided by the Community Emissions Data System (CEDS) (Hoesly et al., 2018), the Model of Emissions of Gases and Aerosols from Nature (MEGAN v2.1) (Guenther et al., 2012), and the Global Fire Emission Database (GFED4) (Giglio et al., 2013), respectively. In China, anthropogenic emissions were taken from Zhang et al. (2018) for ammonia and the Multi-resolution Emission Inventory for China (MEIC v1.3; http://meicmodel.org, last access: 10 October 2021) for other pollutants. More details of the model settings are provided in our previous study (Miao et al., 2020). For computation efficiency, the model simulations were run for the year 2014 and sampled the time and location of each campaign except for the specific year for model–observation comparisons. Recent studies show that the long-term trend of particulate matter is mainly driven by the change in anthropogenic emissions in China (Zhai et al., 2019; Geng et al., 2021). The emissions of NOx, NMVOCs, and organic carbon (OC) changed by −17 %, +11 %, and −35 % from 2011 to 2017 (Zheng et al., 2018), suggesting a minor emission change in anthropogenic SOA precursors over years. The change in primary OC emission is significant and can reduce the surface POA concentrations (e.g., 20–30 µg m−3 as observed in NCP) (Duan et al., 2020). Its impact on SOA concentrations due to loading-dependent gas–particle partitioning is however less than 10 % given the OA mass loadings and the volatility distributions of semivolatile organic vapors (e.g., with mean saturation concentrations of 0.5–0.75 µg m−3 in Beijing) (Xu et al., 2019; Xu et al., 2021). The majority of the surface OA observations (52 out of 86 in Table S1) are from 2013 to 2015, during which the emission changes related to OA are even smaller.
Table 1Descriptions of the OA simulations in this study. Additional HONO sources include direct emissions from traffic, soil, and biomass burning as well as secondary formation from the heterogeneous reaction of NO2 on the ground and the photolysis of nitrate.
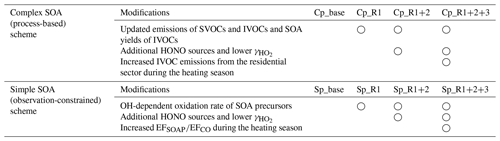
We focus here on the simulations of OA. OA is simulated by so-called complex (i.e., process-based) and simple SOA (i.e., observation-constrained) schemes (Pai et al., 2020). The Cp_base simulation represents the default complex SOA configuration, in which SOA is produced by the oxidation of lumped biogenic, aromatic, and SVOC and IVOC precursors; heterogeneous uptake of glyoxal and methylglyoxal; and isoprene multi-phase chemistry (Marais et al., 2016; Fisher et al., 2016; Pye and Seinfeld, 2010; Pye et al., 2010). The emissions of SVOCs are treated as 1.27 times the primary OC emissions, and the emissions of IVOCs are set as 66 times the naphthalene emissions (Pye and Seinfeld, 2010). Primary SVOCs are emitted as two tracers with saturation concentrations (C∗) of 1646 and 20 µg m−3 (Shrivastava et al., 2006). Once emitted, SVOCs partition to the particle phase to form POA. The remaining gas-phase SVOCs are oxidized by OH with a reaction rate constant of 2 × 10−11 , which produces two SOA surrogates that have volatilities 2 orders of magnitude lower compared to their precursors (Grieshop et al., 2009). The organic-matter-to-OC ratios for POA and SOA are 1.4 and 2.1, respectively (Turpin and Lim, 2001). SOA produced by the oxidation of monoterpenes, sesquiterpenes, aromatics, and IVOCs is parameterized by using the VBS approach with NOx-dependent SOA yields. Naphthalene is used as a surrogate of IVOCs (Chan et al., 2009). Only photooxidation is considered for aromatics and IVOCs, whereas the oxidations by OH, O3, and nitrate radicals (NO3) are all included for monoterpenes and sesquiterpenes (Pye et al., 2010). For isoprene, SOA is simulated by the heterogeneous uptake of isoprene oxidation products that are produced under low- or high-NOx conditions (Marais et al., 2016; Pai et al., 2020). By contrast, the Sp_base simulation represents the default simple SOA configuration. Primary OC emissions from the MEIC inventory are treated as non-volatile. The ratios of the emissions of anthropogenic and biomass burning surrogate precursors to CO (EFSOAP/EFCO) are fixed to 0.069 and 0.013, respectively. The SOA yields for isoprene and terpenes are set to be 0.03 and 0.10, respectively, for simplification. Good model performance has been found for biogenic-dominant regions in the US, indicating that such a simplified yield parameterization works in ambient environments, although the yields for terpenes observed in the laboratory can be quite different (Pai et al., 2020). SOA precursors are converted to SOA with a fixed lifetime of 1 d (Pai et al., 2020; Miao et al., 2020), which generally represents the e-folding timescale of the SOA formation observed in polluted environments (DeCarlo et al., 2010; Hayes et al., 2013).
Modifications to the SOA schemes are listed in Table 1. The Cp_R1 and Sp_R1 simulations have updates on precursor emissions, SOA yields, or parameters related to the production and removal processes. Specifically, the Cp_R1 simulation applies a more reasonable scale factor of 1.0 for SVOC emissions instead of 1.27 that is used in the Cp_base simulation (Lu et al., 2018). Instead of using two bins for all sources, the volatility distributions of SVOC emissions are specified for transportation, other anthropogenic sources, and biomass burning and contain five bins with C∗ of 10−2 to 102 µg m−3 (Fig. S1 in the Supplement), which have lower volatilities compared with the default distribution in the Cp_base simulation (Zhao et al., 2015; May et al., 2013b, a). The updates on the emissions and SOA yields of IVOCs are described in detail in Sect. 2.3. Additionally, the scavenging efficiency of POA in wet deposition is set to be 50 % instead of 0 % (Shah et al., 2019). In the Sp_R1 simulation, an OH-dependent oxidation rate of SOA precursors is used for the daytime simulations, which applies a rate constant of 1.25 × 10−11 instead of a fixed rate of 1.2 × 10−5 s−1 (Hodzic and Jimenez, 2011). For the nighttime simulations, a fixed oxidation rate of 2.5 × 10−6 s−1 is used instead of 1.2 × 10−5 s−1 to account for the NO3 and O3 oxidation at night, which is equivalent to the daytime oxidation rate for an OH level of 0.2 × 106 (Slater et al., 2020; Whalley et al., 2021; Yang et al., 2021, and references therein).
Table 2The annual emissions of IVOCs derived by different approaches in the literature and in our study. The values in parentheses are the IVOC emissions when the residential emission is multiplied by a factor of 7.
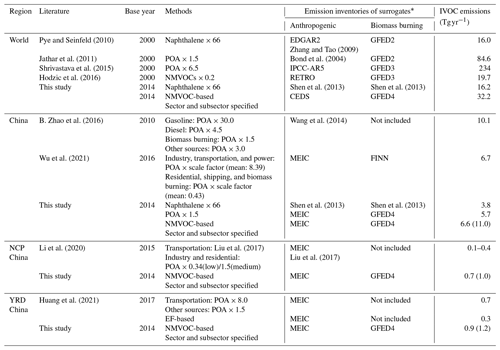
∗ Emissions Database for Global Atmospheric Research version 2 (EDGAR2), Intergovernmental Panel on Climate Change Fifth Assessment Report emission data set (IPCC-AR5), REanalysis of the TROpospheric chemical composition emission inventory (RETRO).
The Cp_R1+2 and Sp_R1+2 schemes aim at improving the OH simulation upon the Cp_R1 and Sp_R1 configurations. The GEOS-Chem model underestimates daytime surface OH concentrations in Beijing (Miao et al., 2020), which is partially driven by inadequate HONO sources. In the default model, HONO is produced by the gas-phase reaction of NO with OH as well as the heterogeneous reaction of nitrogen dioxide (NO2) on aerosols. We first revised the heterogeneous uptake coefficient of HO2 () on aerosols from 0.2 to 0.08 as suggested by Tan et al. (2020) and then added additional HONO sources in the model (Table S5 in the Supplement). Specifically, the HONO emissions from traffic sources (EHONO,traffic) are estimated as 1.7 % of the traffic NOx emissions () (Rappengluck et al., 2013), which can reproduce well the diurnal cycle of HONO concentrations in urban environments (Czader et al., 2015). The emissions from soil (EHONO,soil) are estimated from the soil NOx emissions () by applying scale factors that depend on biomes and soil water content (Hudman et al., 2012; Oswald et al., 2013; Rasool et al., 2019). The HONO emissions from biomass burning are calculated on the basis of the burned areas provided by GFED4 and combustion-type-dependent emission factors (Giglio et al., 2013; Andreae, 2019). Moreover, the heterogeneous reaction of NO2 on the ground is added to the surface layer of the model. The reaction rate (kg) depends on the mean molecular speed of NO2 (), the ground-surface-to-volume ratio (, and the uptake coefficient of NO2 on the ground (γg-NO2) (Li et al., 2010). The is set to be 0.1 m−1 for urban areas (Vogel et al., 2003) but varies by the leaf area index and the boundary layer height in non-urban areas (Sarwar et al., 2008). The γg-NO2 value is set to be 10−6 for nighttime (Kurtenbach et al., 2001) and 2 × 10−5 multiplied by a photo-enhancement scale factor associated with the photolysis rate of NO2 () for daytime (J. Zheng et al., 2020). In addition, the photolysis of nitrate is considered. The photolysis rate (Jnitrate) is set to be 100 times the photolysis rate of HNO3 () with a HONO molar yield of 0.67 (Kasibhatla et al., 2018). Finally, in the Cp_R1+2+3 and Sp_R1+2+3 simulations, we tested the impacts of potentially underrepresented heating season emissions of SOA precursors from the residential sector upon the previous modifications. The IVOC emissions from the residential sector during November to March are multiplied by 7 in the Cp_R1+2+3 simulation according to the observed IVOC concentrations. In the Sp_R1+2+3 simulation, the value of is updated from 0.069 to 0.080 for anthropogenic emissions during November to March. The factor of 0.080 has been used in other model studies for urban plumes (Shah et al., 2019).
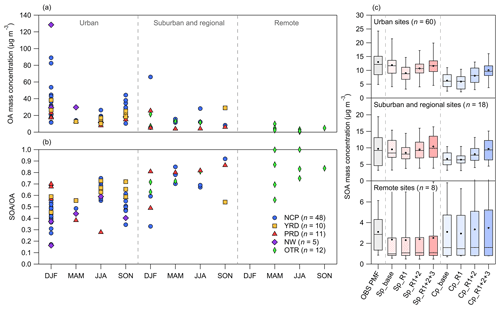
Figure 1(a, b) The observed campaign-average mass concentrations of OA and the mass fraction of SOA in different seasons. (c) The box-and-whisker plots of the observed and simulated campaign-average mass concentrations of SOA. The upper and lower edges of the boxes, the whiskers, the middle lines, and the solid dots denote the 25th and 75th percentiles, the 5th and 95th percentiles, the median values, and the mean values of the SOA concentrations.
2.3 Emissions and SOA yields of IVOCs
We estimated the IVOC emissions from the emissions of NMVOCs instead of naphthalene in the revised model schemes because laboratory experiments show a better correlation of the total IVOC emissions with NMVOCs than with individual IVOC species (e.g., naphthalene) or POA (Zhao et al., 2015; Y. Zhao et al., 2016). Global anthropogenic emissions of NMVOCs are provided by CEDS (Hoesly et al., 2018), and the emissions from biomass burning are provided by GFED4 (Giglio et al., 2013; Andreae, 2019). In China, anthropogenic emissions of NMVOCs are taken from MEIC. The NMVOC emission profiles of sectors (i.e., power, transportation, industry, and residential) and subsectors (i.e., gasoline, diesel, coal, solvent, and biofuel or biomass burning) and the IVOC/NMVOC emission ratios of the subsectors as well as the volatility distributions of IVOCs for the subsectors with C∗ of 106 (IVOC6), 105 (IVOC5), and 10≤4 (IVOC4) µg m−3 are obtained from the literature (Fig. S2 and Table S6 in the Supplement) (M. Li et al., 2019; Lu et al., 2018; Cai et al., 2019; Lim et al., 2019; Khare and Gentner, 2018). Table S7 in the Supplement lists the annual emissions of IVOC6, IVOC5, and IVOC4 in 2014. Industry and residential sectors are the major sources of IVOCs in China. The reaction rate constant with OH for these IVOC species used in the model is 2.3 × 10−11 at 298 K, which is the same as the rate constant of naphthalene photooxidation (Chan et al., 2009). Table S8 in the Supplement lists the SOA yield parameterizations of IVOCs used in this study. For high-NOx conditions, mass-weighted yields of the photooxidation of C12–C14, C15–C16, and C≥17 n-alkanes are used for IVOC6, IVOC5, and IVOC4, respectively (Presto et al., 2010; Zhao et al., 2015). For low-NOx conditions, a fixed yield of 0.73 obtained from naphthalene photooxidation is applied to all IVOCs because of the lack of low-NOx yields for n-alkanes (Chan et al., 2009). The corresponding IVOC yields for 10 µg m−3 OA range from 0.19 to 0.44, which are greater than the yields in the Cp_base simulation but within the range of the yields used in other studies (Pye and Seinfeld, 2010; Koo et al., 2014; Jathar et al., 2014; Lu et al., 2020).
Table 2 lists the total IVOC emissions estimated in various studies. Globally, the IVOC emissions range from 16.0 to 234 Tg yr−1, for which the POA-based methods have the highest estimates, and the naphthalene-based methods have the lowest (Pye and Seinfeld, 2010; Jathar et al., 2011; Shrivastava et al., 2015; Hodzic et al., 2016). Our new NMVOC-based method suggests a global emission of 32.2 Tg yr−1 and an emission of 6.6 Tg yr−1 in China that is similar to the POA-based estimate made by Wu et al. (2021). The spatial distribution of IVOC emissions shows that the greatest increase in the new NMVOC-based emission occurs in urban areas compared with the naphthalene × 66 and the POA × 1.5 estimates of other models (Fig. S3 in the Supplement). The POA × 1.5 estimate of IVOC emissions has a greater winter–summer emission difference compared with the naphthalene × 66 and the new NMVOC-based emissions (Fig. S4 in the Supplement). The additional increase in IVOC emissions in the Cp_R1+2+3 simulation (i.e., 7 times the residential IVOC emissions during the heating season) leads to a large emission enhancement in northern China (Fig. S3) and a greater winter–summer emission difference than that in the Cp_R1+2 simulation (Fig. S4), which agrees better with the PMF-derived SOA results (Sect. 3).
Table 3The statistics of model–observation comparisons of the campaign-average mass concentrations of OA, POA, and SOA in China. “OBS” and “SIM” represent the mean values of the observations and the simulations, respectively. NMB is normalized mean bias, NME is normalized mean error, RMSE is root mean square error, and R is Pearson's correlation coefficient. The units of OBS, SIM, and RMSE are µg m−3.
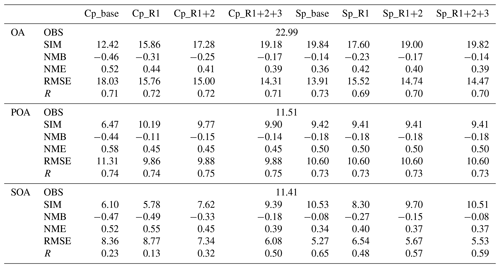
3.1 Model evaluations of OA and SOA
Figure 1a shows the observed campaign-average mass concentrations of OA in China, which range from 0.7 to 128.5 µg m−3. The trend of increased OA concentrations and decreased SOA mass fractions (Fig. 1b) from remote to urban regions is consistent with our understanding of the primary contribution of anthropogenic sources in urban areas (Zhang et al., 2007; Li et al., 2017). The highest OA concentrations occurred in winter in northern China, corresponding to high POA fractions that may go over 50 % at some urban sites. In particular, residential solid fuel consumption emits a large quantity of POA and SOA precursors, and stagnant meteorological conditions often happen in winter, leading to severe haze in northern China (Li et al., 2017; Peng et al., 2019). The OA concentrations are typically low in summer, when meteorological conditions favor particle dilution and deposition, and in southern China, where primary contributions are less than in northern China. The SOA fractions are generally high in southern China (above 65 %), which may be explained by low primary emissions and high oxidation capacity that leads to fast conversion of organic vapors to SOA (Li et al., 2015). The lowest OA concentrations were observed in remote regions (e.g., Tibetan Plateau) in summer, representing natural background conditions in China.
The statistical values such as normalized mean bias (NMB), normalized mean error (NME), root mean square error (RMSE), and Pearson's correlation coefficient (R) for the model–observation comparisons of campaign-average concentrations of OA, POA, and SOA are listed in Table 3. As is consistent with our previous results (Miao et al., 2020), the Cp_base simulation underestimates the concentrations of OA (NMB = − 0.46) as well as POA (NMB = −0.44) and SOA (NMB = − 0.47) in China. Because a fraction of aerosol particles may be present in the supermicron domain that cannot be detected efficiently by AMS or ACSM (Sun et al., 2020a), such model underestimation of OA can be greater in certain circumstances, e.g., in northern China under winter haze conditions. By contrast, the Sp_base simulation may reproduce the OA loadings (NMB = −0.14). The POA simulations are improved in Sp_base (NMB = − 0.18) and Cp_R1 (NMB = −0.11). The Sp_base simulation considers primary OC as non-volatile, for which the model results agree with the PMF-derived POA results at urban sites but significantly overestimate the POA concentrations in suburban and remote regions (Fig. S5 in the Supplement). The Cp_R1 simulation considers primary OC to be semivolatile, with lower volatility distributions compared with the Cp_base simulation, leading to more OC mass in the particle phase as emitted (i.e., “POA”). This simulation is slightly better than the Sp_base simulation for POA but still overestimates its concentrations in suburban and remote regions.
Figures 1c and S6 in the Supplement show the simulated SOA concentrations compared with the observations at urban, suburban, and remote sites. The underestimation of the Cp_base simulation of SOA mainly occurs in urban and suburban regions, which is consistent with previous understanding about the underrepresented sources of anthropogenic SOA in process-based models (B. Zhao et al., 2016; Z. Han et al., 2016). The Sp_base simulation captures well anthropogenic SOA (NMB = − 0.08) because of the use of ambient-constrained parameterization to represent anthropogenic sources (Hodzic and Jimenez, 2011; Woody et al., 2016). The R of SOA increases from 0.23 in the Cp_base simulation to 0.65 in the Sp_base simulation (Table 3). For Cp_R1, the simulated SOA mass concentrations show insignificant changes (NMB = − 0.49) because the increased SOA mass from increased emissions and updated SOA yields of IVOCs is offset by the decreased SOA mass from the updated SVOC emissions and volatility distributions. The SVOC emissions and volatility distributions in Cp_R1 are supposed to be more reasonable according to recent laboratory results (Lu et al., 2018; Zhao et al., 2015; May et al., 2013b, a). Further updates to HONO and residential IVOC emissions in Cp_R1+2 and Cp_R1+2+3 improve the SOA simulations (NMB = − 0.18). For the observation-constrained scheme, the SOA concentrations at urban sites are lower in the Sp_R1 simulation than in the Sp_base simulation after applying OH-dependent oxidation rates for SOA precursors. This update is physically sound and may represent better the diurnal and spatial patterns of SOA formation. It is however sensitive to the simulated OH concentrations in the model. Further updates to Sp_R1+2 for HONO are therefore needed to improve the OH and subsequently the SOA simulations. The Sp_R1+2+3 simulation demonstrates additional improvements from the potentially biased wintertime anthropogenic emissions. Figure S7 in the Supplement shows the NMB values of the SOA simulations for different seasons. The model underestimation is more significant in autumn and winter than in spring and summer. The implementation of additional HONO sources in the Cp_R1+2 and Sp_R1+2 simulations effectively reduces the NMB values at urban and suburban sites in all seasons except in summer. Increasing the emissions of SOA precursors in the Cp_R1+2+3 and Sp_R1+2+3 simulations further reduces the model–observation discrepancy in winter at urban sites, although it causes slight overestimation at suburban sites. By contrast, the model modifications have a minor influence on the NMB values for remote sites in all seasons.
In addition, the model performance of meteorological parameters (e.g., temperature, relative humidity, wind speed and direction, and boundary layer height), oxidants (e.g., OH, O3 and NO3), and aerosol precursors in GEOS-Chem has been evaluated elsewhere (Miao et al., 2020). The results show the model overestimations of surface wind speed, the peak O3 concentration by a factor of 2 in winter, and the peak NO3 concentration by a factor of 3 in summer. On the other hand, the model underestimates the boundary layer height and the daytime surface OH concentrations by a factor of 2–4 in NCP in China. Sensitivity analysis indicates that uncertainties in chemistry dominate the model biases in particulate matter and its components. The impact of the overestimated surface concentrations of O3 and NO3 on the SOA simulation is probably minor compared with the model bias of OH because OH is the dominant oxidant in China, and the influences of O3 and NO3 limit the formation of biogenic SOA (BSOA), which is a minor contributor to the SOA mass compared with anthropogenic sources in polluted environments (Zhu et al., 2020; Pye et al., 2010).
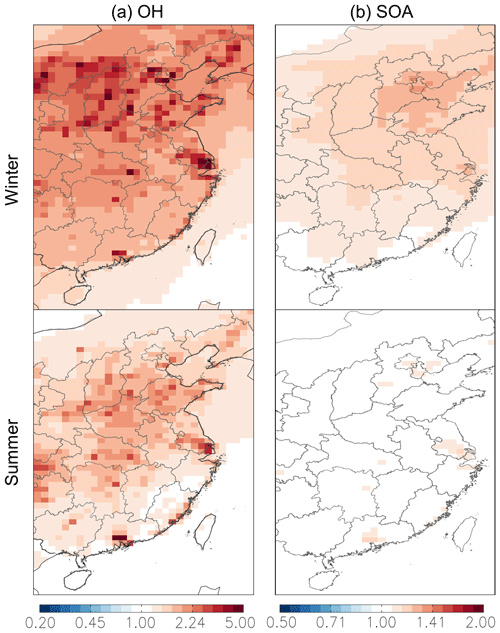
Figure 2The ratios of seasonal mean concentrations of (a) OH and (b) SOA simulated by the Sp_R1+2 simulation to those simulated by the Sp_R1 simulation.
3.2 Sensitivities of SOA simulation to the OH concentrations and IVOC emissions
Figure 2a shows the ratios of seasonal mean OH concentrations simulated by Sp_R1+2 to those simulated by Sp_R1. The modifications in Sp_R1+2 and similarly in Cp_R1+2 increase the modeled HONO concentrations (Table S3) and improve NMB from −0.58 in the base simulations to −0.14 (Fig. 3). The addition of HONO sources can increase the surface mean OH concentrations by a factor of 2 to 4, especially in winter in northern China, when the photolysis of HONO contributes predominantly to the primary production of OH (Tan et al., 2018; Slater et al., 2020). Table S4 lists the observed and modeled surface OH and HO2 concentrations in China. The modified HONO sources significantly improve the simulations of peak concentrations of OH and HO2 in winter, which improves the SOA simulations. As shown in Fig. 2b, the increased OH concentrations lead to greater SOA concentrations nationwide. In particular, the increase can be over 30 % in winter in northern China, suggesting that the SOA simulation is more sensitive to the OH simulation in northern China than in southern China. Consistently, a recent study suggests that enhanced OH levels likely promote fresh SOA formation in northern China but increase the oxidation state of OA in southern China (J. Li et al., 2019b). In summer, the enhancements of SOA mass mainly occur in the near-source regions in the Sp_R1+2 simulation. Although the OH and HO2 concentrations in summer in southern and southwestern China are overestimated in the Sp_R1+2 and Cp_R1+2 simulations (Table S4), this overestimation has little impact on the model–observation comparisons of SOA herein.
The remarkable increase in the SOA concentrations in northern China in the Sp_R1+2 and Cp_R1+2 simulations highlights the importance of HONO chemistry to SOA formation in polluted environments. Among the added HONO sources, the heterogeneous reaction of NO2 on the ground contributes predominantly to the enhancements of surface HONO and OH concentrations, which is consistent with the results from budget analysis of ambient observations (Xue et al., 2020; Liu et al., 2019; Huang et al., 2017). The greatest enhancements of OH concentrations therefore occur in urban areas where high NOx emissions and large facilitate the heterogeneous formation of HONO. The model parameters such as γg-NO2 and the HONO yield vary significantly by relative humidity, light intensity, and NO2 concentrations and are associated with large uncertainties (C. Han et al., 2016; Liu et al., 2020), which requires more future observations to constrain.
Further improved model performances in Sp_R1+2+3 and Cp_R1+2+3 in winter compared with Sp_R1+2 and Cp_R1+2 suggest that large uncertainties remain in SOA precursor emissions. For the observation-constrained scheme, the emissions of SOA precursors depend on the emissions of CO. The observed CO concentrations in China are generally greater than the modeled surface concentrations, indicating possibly underestimated CO emissions especially in winter (Kong et al., 2020). Consistently, top-down estimates suggest greater CO emissions than those in the MEIC inventory (X. Zhang et al., 2019; Feng et al., 2020; Gaubert et al., 2020). On the other hand, recent measurements of SOA formation potential show a wide range of reference values for (Table S10 in the Supplement). The fixed ratios used in the model may not fully represent ambient conditions (Liao et al., 2021). The higher value of (i.e., 0.08 instead of 0.069) for all anthropogenic sources during the heating season in the Sp_R1+2+3 simulation increases the SOA concentrations in winter at urban sites and reduces NMB from −0.26 in Sp_R1+2 to −0.15 (Fig. S7a). The spatial distributions of winter-mean SOA concentrations show greater SOA concentrations in NCP and YRD in the Sp_R1+2+3 simulation than in the Sp_R1+2 simulation (Fig. S8 in the Supplement), which agrees better with the observations. Some overestimation occurs at suburban sites (Fig. S7b), highlighting the need of using source-specified in future model development. The increased has little influence on the model performance at remote sites (Fig. S7c).
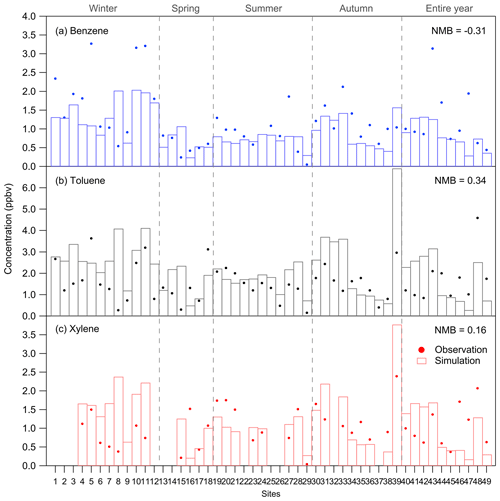
Figure 4Comparisons of the observed campaign-average mixing ratios of benzene, toluene, and xylene in China with those simulated by the Cp_base simulation. Note that the mixing ratios of these aromatic compounds in the Cp_base, Cp_R1, Cp_R1+2, and Cp_R1+2+3 simulations are similar.
For the process-based scheme, anthropogenic aromatics, IVOCs, and SVOCs are uncertain precursors in the model. Figure 4 shows the model–observation comparison of campaign-average concentrations of benzene, toluene, and xylene in China. The model simulations in general agree with the observations, with NMBs of −0.31 to 0.34. The biases show insignificant seasonality, suggesting that the uncertainty in aromatic emissions is perhaps not the driven factor of the underestimated SOA concentration in winter. Measurements of SVOCs and IVOCs are rare (Y. Li et al., 2019). Table S9 in the Supplement lists the observed and simulated campaign-average concentrations of primary IVOCs in China. The Cp_R1+2 simulation largely underestimates the IVOC concentrations, especially in winter. The underestimated IVOC emissions are likely from the residential sector, which has highly uncertain emission activity (Tao et al., 2018; Peng et al., 2019; J. Li et al., 2019a). The emission factors of IVOCs from residential combustion vary in a wide range and are sensitive to the fuel types and combustion conditions (Cai et al., 2019; Qian et al., 2021). We tested sevenfold IVOC emissions from the residential sector in the Cp_R1+2+3 simulation to eliminate the potential seasonal bias of IVOC emissions. The simulation-to-observation ratio of primary IVOC concentrations in winter became 0.44, which is similar to the ratio in summer. The Cp_R1+2+3 simulation indeed improves the winter SOA simulations at urban sites significantly and reduces NMB from −0.55 in Cp_R1+2 to −0.28 (Fig. S7a).
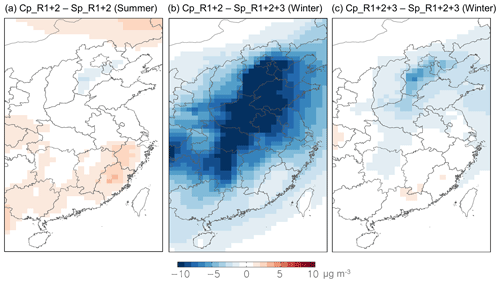
Figure 5The differences in seasonal mean mass concentrations of SOA between (a) the Cp_R1+2 and Sp_R1+2 simulations in summer, (b) the Cp_R1+2 and Sp_R1+2+3 simulations in winter, and (c) the Cp_R1+2+3 and Sp_R1+2+3 simulations in winter.
The inter-comparisons of the process-based and observation-constrained simulations of season-mean mass concentrations of SOA highlight the importance of improving the IVOC emissions in winter for modeling SOA in China (Fig. 5). The spatial distributions of the SOA concentrations in the Sp_R1+2 and Cp_R1+2 simulations are similar in summer, with differences below 2 µg m−3 (Fig. 5a), suggesting the comparable emission of SOA precursors between the two schemes in summer. Note that the SOA concentrations in the Sp_R1+2 and Sp_R1+2+3 simulations are the same in summer because the updates to Sp_R1+2+3 only affect the heating season. The SOA concentrations in the Cp_R1+2 and Cp_R1+2+3 simulations in summer are also the same. In winter, the difference in the simulated SOA concentrations between Sp_R1+2+3 and Cp_R1+2 is at most 13 µg m−3, and the main difference is evident in NCP and central China, where anthropogenic SOA sources are possibly still underestimated (Fig. 5b). Increasing residential IVOC emissions in Cp_R1+2+3 effectively reduce the difference in the simulated SOA concentrations between Sp_R1+2+3 and Cp_R1+2+3, although some underestimation still exists in Hebei Province (Fig. 5c). Overall, the revised process-based simulations have greater biases than the revised observation-constrained ones at urban sites (Fig. S7), perhaps resulting from the missing cooking source in the process-based scheme.
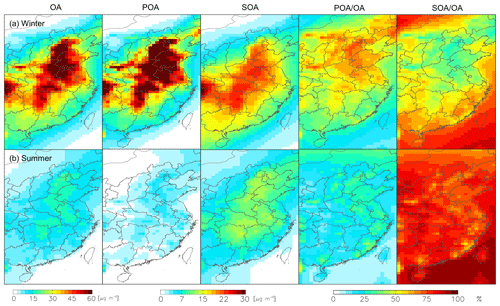
Figure 6Seasonal mean mass concentrations of OA, POA, and SOA and the mass fractions of POA and SOA simulated by the Cp_R1+2+3 simulation in (a) winter and (b) summer.
3.3 Budget and sources of OA in eastern China
The Cp_R1+2+3 simulation represents our best-estimate scenario for the process-based scheme that captures well the seasonal and spatial patterns of OA as well as the split of POA and SOA in the observations (Fig. 1a and b). Figure 6 shows the concentrations of OA, POA, and SOA as well as the mass fractions of POA and SOA in eastern China simulated in the Cp_R1+2+3 simulation. The POA concentrations are several times greater in winter than in summer because of higher emissions of SVOCs and IVOCs as well as low temperature and high OA concentrations that favor the gas-to-particle partitioning of organic vapors. The seasonal difference in SOA is smaller than that of POA. One explanation is the enhanced formation of BSOA in summer. SOA is the dominant component of OA in summer and contributes over 60 % of OA nationally, whereas POA contributes more than SOA in winter in northern China. Figure 7 shows the corresponding OA compositions in different regions as well as their sources. The SOA mass concentrations are dominated by anthropogenic sources, among which SVOCs and IVOCs contribute over 50 % in the three regions. The contribution of SVOCs to SOA depends on the season. In summer, SVOC-related SOA (SVOCs-SOA) is the largest OA component in all regions (30 %–39 %), for which residential and industry sectors are the main sources. The contributions of IVOCs to OA are 15 %–20 %, for which industry is the predominant contributor. In winter, the residential sector is the major source of SVOCs and IVOCs. SVOCs-SOA contributes less to OA (6 %–23 %) than in summer because SVOCs favorably form POA at low temperatures. The contribution of IVOCs to OA is 22 %–30 %, with great uncertainties from the residential sector.
Other model studies that considered the contributions of SVOCs and IVOCs in China also show that SVOCs and IVOCs contribute greatly to the simulated SOA (B. Zhao et al., 2016; Yang et al., 2019; Li et al., 2020). The mass fractions of each SOA component vary among studies. For example, Li et al. (2020) suggested a lower contribution of IVOC-related SOA (IVOCs-SOA) and a higher contribution of aromatic SOA (ASOA) in NCP in winter compared to our study, explained by lower emissions of IVOCs and enhanced formation of ASOA from the aging process in their study. B. Zhao et al. (2016) and Yang et al. (2019) suggested over 50 % contributions of IVOCs-SOA to SOA in all seasons, which is greater than our estimations. Their studies considered the multi-generation oxidation of IVOCs for which the mechanism and parameterization remain unclear. The formation of SOA from IVOCs is the most uncertain part, calling for more constraints from ambient measurements and experiments.
For BSOA, its contribution to total OA is negligible in winter but can increase to 15 % in PRD in summer because of the enhanced emissions of biogenic precursors. The contribution of SOA formed by aqueous-phase ways (aqSOA) is also much greater in summer (9 %–13 %) because high emissions of isoprene enhance the formation of isoprene epoxydiols (IEPOX), glyoxal, and methylglyoxal (Hu et al., 2017). Field observations suggest an important role of aqSOA in SOA formation during the winter haze periods (Kuang et al., 2020; Wang et al., 2021). The simulated mass fraction of aqSOA is only 3 %–5 % in SOA in winter herein, indicating that more precursors are perhaps involved in the SOA formation related to aerosol liquid water than the model has considered (Gkatzelis et al., 2021). The estimated contribution of aqSOA is similar to the estimation of Li et al. (2021) in NCP but is much lower than the estimations made by Qiu et al. (2020) in Beijing and Ling et al. (2020) in PRD. Further improvements of aqSOA simulation may be important for capturing the variability in SOA during the winter haze episodes.
In this study, we applied both process-based and observation-constrained schemes to simulate OA in China. Compared with the PMF results from observations, the model underestimation of SOA mainly occurs in winter in northern China in the default model. Updates to the emissions, volatility distributions, and SOA yields of SVOCs and IVOCs as well as the addition of HONO sources lead to significant model improvements. The bias of SOA simulation is sensitive to the model performance of OH levels and IVOC emissions. For the former, the addition of HONO sources can significantly improve the simulations of surface OH concentrations in winter and increase the simulated SOA concentrations by over 30 % in northern China, highlighting the importance of HONO chemistry in polluted environments. Greater sensitivity of the SOA formation to the OH levels is more present in winter than in summer. For the latter, the most uncertain part of IVOC emissions is from the residential sector, which needs future efforts to constrain.
With all improvements, the two types of SOA schemes show similar seasonal and spatial variations that reasonably agree with the observations. SVOCs and IVOCs are the main contributors to SOA in most of China, with mass contributions of over 50 %. Control measures of primary OC emissions have already been taken since 2014 and resulted in an effective reduction in the POA concentrations in NCP (Lei et al., 2021; Duan et al., 2020). High concentrations of SOA were still observed in NCP during the COVID-19 lockdown period, when the emissions from industry and transportation were largely reduced (Sun et al., 2020b; Zheng et al., 2021). Further reduction should focus on the SVOC and IVOC emissions. The seasonal variations in the OA composition and the source contribution suggest that the control strategies for SOA pollution should vary by season. The model suggests the residential sector as the major source of POA, SVOCs-SOA, and IVOCs-SOA in winter in polluted areas in China. The control of residential emissions may reduce POA and SOA simultaneously besides the reduction in other primary aerosols and secondary inorganic aerosols (Meng et al., 2020). In summer, the industry sector becomes the predominant source of SVOCs- and IVOCs-SOA, which has not yet been effectively controlled in China.
Data presented in this paper are available upon request to the corresponding author.
The supplement related to this article is available online at: https://doi.org/10.5194/acp-21-16183-2021-supplement.
QC and RM designed the study. RM performed the model simulations and conducted the data analysis. LZ and YC provided the emission inventory of ammonia. QC and RM prepared the manuscript with contributions from all co-authors.
The contact author has declared that neither they nor their co-authors have any competing interests.
Publisher's note: Copernicus Publications remains neutral with regard to jurisdictional claims in published maps and institutional affiliations.
This work was supported by the National Natural Science Foundation of China (grant nos. 41961134034, 91544107, 41875165, and 51861135102) and the 111 Project of Urban Air Pollution and Health Effects (B20009). Manish Shrivastava was supported by the US DOE, Office of Science, Office of Biological and Environmental Research, through the Early Career Research Program.
This research has been supported by the National Natural Science Foundation of China (grant nos. 41961134034, 91544107, 41875165, and 51861135102) and the Higher Education Discipline Innovation Project (grant no. B20009).
This paper was edited by Qiang Zhang and reviewed by two anonymous referees.
Andreae, M. O.: Emission of trace gases and aerosols from biomass burning – an updated assessment, Atmos. Chem. Phys., 19, 8523–8546, https://doi.org/10.5194/acp-19-8523-2019, 2019.
Bond, T. C., Streets, D. G., Yarber, K. F., Nelson, S. M., Woo, J. H., and Klimont, Z.: A technology-based global inventory of black and organic carbon emissions from combustion, J. Geophys. Res.-Atmos., 109, D14203, https://doi.org/10.1029/2003JD003697, 2004.
Cai, S., Zhu, L., Wang, S., Wisthaler, A., Li, Q., Jiang, J., and Hao, J.: Time-Resolved Intermediate-Volatility and Semivolatile Organic Compound Emissions from Household Coal Combustion in Northern China, Environ. Sci. Technol., 53, 9269–9278, https://doi.org/10.1021/acs.est.9b00734, 2019.
Chan, A. W. H., Kautzman, K. E., Chhabra, P. S., Surratt, J. D., Chan, M. N., Crounse, J. D., Kürten, A., Wennberg, P. O., Flagan, R. C., and Seinfeld, J. H.: Secondary organic aerosol formation from photooxidation of naphthalene and alkylnaphthalenes: implications for oxidation of intermediate volatility organic compounds (IVOCs), Atmos. Chem. Phys., 9, 3049–3060, https://doi.org/10.5194/acp-9-3049-2009, 2009.
Chrit, M., Sartelet, K., Sciare, J., Majdi, M., Nicolas, J., Petit, J.-E., and Dulac, F.: Modeling organic aerosol concentrations and properties during winter 2014 in the northwestern Mediterranean region, Atmos. Chem. Phys., 18, 18079–18100, https://doi.org/10.5194/acp-18-18079-2018, 2018.
Chung, S. H. and Seinfeld, J. H.: Global distribution and climate forcing of carbonaceous aerosols, J. Geophys. Res.-Atmos., 107, 4407, https://doi.org/10.1029/2001JD001397, 2002.
Czader, B. H., Choi, Y., Li, X., Alvarez, S., and Lefer, B.: Impact of updated traffic emissions on HONO mixing ratios simulated for urban site in Houston, Texas, Atmos. Chem. Phys., 15, 1253–1263, https://doi.org/10.5194/acp-15-1253-2015, 2015.
DeCarlo, P. F., Ulbrich, I. M., Crounse, J., de Foy, B., Dunlea, E. J., Aiken, A. C., Knapp, D., Weinheimer, A. J., Campos, T., Wennberg, P. O., and Jimenez, J. L.: Investigation of the sources and processing of organic aerosol over the Central Mexican Plateau from aircraft measurements during MILAGRO, Atmos. Chem. Phys., 10, 5257–5280, https://doi.org/10.5194/acp-10-5257-2010, 2010.
Duan, J., Huang, R.-J., Li, Y., Chen, Q., Zheng, Y., Chen, Y., Lin, C., Ni, H., Wang, M., Ovadnevaite, J., Ceburnis, D., Chen, C., Worsnop, D. R., Hoffmann, T., O'Dowd, C., and Cao, J.: Summertime and wintertime atmospheric processes of secondary aerosol in Beijing, Atmos. Chem. Phys., 20, 3793–3807, https://doi.org/10.5194/acp-20-3793-2020, 2020.
Feng, S., Jiang, F., Wu, Z., Wang, H., Ju, W., and Wang, H.: CO Emissions Inferred From Surface CO Observations Over China in December 2013 and 2017, J. Geophys. Res.-Atmos., 125, e2019JD031808, https://doi.org/10.1029/2019JD031808, 2020.
Feng, T., Zhao, S., Bei, N., Wu, J., Liu, S., Li, X., Liu, L., Qian, Y., Yang, Q., Wang, Y., Zhou, W., Cao, J., and Li, G.: Secondary organic aerosol enhanced by increasing atmospheric oxidizing capacity in Beijing–Tianjin–Hebei (BTH), China, Atmos. Chem. Phys., 19, 7429–7443, https://doi.org/10.5194/acp-19-7429-2019, 2019.
Fisher, J. A., Jacob, D. J., Travis, K. R., Kim, P. S., Marais, E. A., Chan Miller, C., Yu, K., Zhu, L., Yantosca, R. M., Sulprizio, M. P., Mao, J., Wennberg, P. O., Crounse, J. D., Teng, A. P., Nguyen, T. B., St. Clair, J. M., Cohen, R. C., Romer, P., Nault, B. A., Wooldridge, P. J., Jimenez, J. L., Campuzano-Jost, P., Day, D. A., Hu, W., Shepson, P. B., Xiong, F., Blake, D. R., Goldstein, A. H., Misztal, P. K., Hanisco, T. F., Wolfe, G. M., Ryerson, T. B., Wisthaler, A., and Mikoviny, T.: Organic nitrate chemistry and its implications for nitrogen budgets in an isoprene- and monoterpene-rich atmosphere: constraints from aircraft (SEAC4RS) and ground-based (SOAS) observations in the Southeast US, Atmos. Chem. Phys., 16, 5969–5991, https://doi.org/10.5194/acp-16-5969-2016, 2016.
Fountoukis, C. and Nenes, A.: ISORROPIA II: a computationally efficient thermodynamic equilibrium model for K+–Ca2+–Mg2+––Na+–––Cl−–H2O aerosols, Atmos. Chem. Phys., 7, 4639–4659, https://doi.org/10.5194/acp-7-4639-2007, 2007.
Gani, S., Bhandari, S., Seraj, S., Wang, D. S., Patel, K., Soni, P., Arub, Z., Habib, G., Hildebrandt Ruiz, L., and Apte, J. S.: Submicron aerosol composition in the world's most polluted megacity: the Delhi Aerosol Supersite study, Atmos. Chem. Phys., 19, 6843–6859, https://doi.org/10.5194/acp-19-6843-2019, 2019.
Gaubert, B., Emmons, L. K., Raeder, K., Tilmes, S., Miyazaki, K., Arellano Jr., A. F., Elguindi, N., Granier, C., Tang, W., Barré, J., Worden, H. M., Buchholz, R. R., Edwards, D. P., Franke, P., Anderson, J. L., Saunois, M., Schroeder, J., Woo, J.-H., Simpson, I. J., Blake, D. R., Meinardi, S., Wennberg, P. O., Crounse, J., Teng, A., Kim, M., Dickerson, R. R., He, H., Ren, X., Pusede, S. E., and Diskin, G. S.: Correcting model biases of CO in East Asia: impact on oxidant distributions during KORUS-AQ, Atmos. Chem. Phys., 20, 14617–14647, https://doi.org/10.5194/acp-20-14617-2020, 2020.
Geng, G., Zheng, Y., Zhang, Q., Xue, T., Zhao, H., Tong, D., Zheng, B., Li, M., Liu, F., Hong, C., He, K., and Davis, S.: Drivers of PM2.5 air pollution deaths in China 2002-2017, Nat. Geosci., 14, 645–650, https://doi.org/10.1038/s41561-021-00792-3, 2021.
Giglio, L., Randerson, J. T., and van der Werf, G. R.: Analysis of daily, monthly, and annual burned area using the fourth-generation global fire emissions database (GFED4), J. Geophys. Res.-Biogeo., 118, 317–328, https://doi.org/10.1002/jgrg.20042, 2013.
Gkatzelis, G. I., Papanastasiou, D. K., Karydis, V. A., Hohaus, T., Liu, Y., Schmitt, S. H., Schlag, P., Fuchs, H., Novelli, A., Chen, Q., Cheng, X., Broch, S., Dong, H., Holland, F., Li, X., Liu, Y., Ma, X., Reimer, D., Rohrer, F., Shao, M., Tan, Z., Taraborrelli, D., Tillmann, R., Wang, H., Wang, Y., Wu, Y., Wu, Z., Zeng, L., Zheng, J., Hu, M., Lu, K., Hofzumahaus, A., Zhang, Y., Wahner, A., and Kiendler-Scharr, A.: Uptake of Water-soluble Gas-phase Oxidation Products Drives Organic Particulate Pollution in Beijing, Geophys. Res. Lett., 48, e2020GL091351, https://doi.org/10.1029/2020GL091351, 2021.
Grieshop, A. P., Logue, J. M., Donahue, N. M., and Robinson, A. L.: Laboratory investigation of photochemical oxidation of organic aerosol from wood fires 1: measurement and simulation of organic aerosol evolution, Atmos. Chem. Phys., 9, 1263–1277, https://doi.org/10.5194/acp-9-1263-2009, 2009.
Guenther, A. B., Jiang, X., Heald, C. L., Sakulyanontvittaya, T., Duhl, T., Emmons, L. K., and Wang, X.: The Model of Emissions of Gases and Aerosols from Nature version 2.1 (MEGAN2.1): an extended and updated framework for modeling biogenic emissions, Geosci. Model Dev., 5, 1471–1492, https://doi.org/10.5194/gmd-5-1471-2012, 2012.
Han, C., Yang, W., Wu, Q., Yang, H., and Xue, X.: Heterogeneous Photochemical Conversion of NO2 to HONO on the Humic Acid Surface under Simulated Sunlight, Environ. Sci. Technol., 50, 5017–5023, https://doi.org/10.1021/acs.est.5b05101, 2016.
Han, Z., Xie, Z., Wang, G., Zhang, R., and Tao, J.: Modeling organic aerosols over east China using a volatility basis-set approach with aging mechanism in a regional air quality model, Atmos. Environ., 124, 186–198, https://doi.org/10.1016/j.atmosenv.2015.05.045, 2016.
Hayes, P. L., Ortega, A. M., Cubison, M. J., Froyd, K. D., Zhao, Y., Cliff, S. S., Hu, W. W., Toohey, D. W., Flynn, J. H., Lefer, B. L., Grossberg, N., Alvarez, S., Rappenglück, B., Taylor, J. W., Allan, J. D., Holloway, J. S., Gilman, J. B., Kuster, W. C., de Gouw, J. A., Massoli, P., Zhang, X., Liu, J., Weber, R. J., Corrigan, A. L., Russell, L. M., Isaacman, G., Worton, D. R., Kreisberg, N. M., Goldstein, A. H., Thalman, R., Waxman, E. M., Volkamer, R., Lin, Y. H., Surratt, J. D., Kleindienst, T. E., Offenberg, J. H., Dusanter, S., Griffith, S., Stevens, P. S., Brioude, J., Angevine, W. M., and Jimenez, J. L.: Organic aerosol composition and sources in Pasadena, California, during the 2010 CalNex campaign, J. Geophys. Res.-Atmos., 118, 9233–9257, https://doi.org/10.1002/jgrd.50530, 2013.
Hodzic, A. and Jimenez, J. L.: Modeling anthropogenically controlled secondary organic aerosols in a megacity: a simplified framework for global and climate models, Geosci. Model Dev., 4, 901–917, https://doi.org/10.5194/gmd-4-901-2011, 2011.
Hodzic, A., Kasibhatla, P. S., Jo, D. S., Cappa, C. D., Jimenez, J. L., Madronich, S., and Park, R. J.: Rethinking the global secondary organic aerosol (SOA) budget: stronger production, faster removal, shorter lifetime, Atmos. Chem. Phys., 16, 7917–7941, https://doi.org/10.5194/acp-16-7917-2016, 2016.
Hoesly, R. M., Smith, S. J., Feng, L., Klimont, Z., Janssens-Maenhout, G., Pitkanen, T., Seibert, J. J., Vu, L., Andres, R. J., Bolt, R. M., Bond, T. C., Dawidowski, L., Kholod, N., Kurokawa, J.-I., Li, M., Liu, L., Lu, Z., Moura, M. C. P., O'Rourke, P. R., and Zhang, Q.: Historical (1750–2014) anthropogenic emissions of reactive gases and aerosols from the Community Emissions Data System (CEDS), Geosci. Model Dev., 11, 369–408, https://doi.org/10.5194/gmd-11-369-2018, 2018.
Hu, J., Wang, P., Ying, Q., Zhang, H., Chen, J., Ge, X., Li, X., Jiang, J., Wang, S., Zhang, J., Zhao, Y., and Zhang, Y.: Modeling biogenic and anthropogenic secondary organic aerosol in China, Atmos. Chem. Phys., 17, 77–92, https://doi.org/10.5194/acp-17-77-2017, 2017.
Huang, L., Wang, Q., Wang, Y., Emery, C., Zhu, A., Zhu, Y., Yin, S., Yarwood, G., Zhang, K., and Li, L.: Simulation of secondary organic aerosol over the Yangtze River Delta region: The impacts from the emissions of intermediate volatility organic compounds and the SOA modeling framework, Atmos. Environ., 246, 118079, https://doi.org/10.1016/j.atmosenv.2020.118079, 2021.
Huang, R.-J., Yang, L., Cao, J., Wang, Q., Tie, X., Ho, K. F., Shen, Z., Zhang, R., Li, G., Zhu, C., Zhang, N., Dai, W., Zhou, J., Liu, S., Chen, Y., Chen, J., and O'Dowd, C. D.: Concentration and sources of atmospheric nitrous acid (HONO) at an urban site in Western China, Sci. Total Environ., 593–594, 165–172, https://doi.org/10.1016/j.scitotenv.2017.02.166, 2017.
Hudman, R. C., Moore, N. E., Mebust, A. K., Martin, R. V., Russell, A. R., Valin, L. C., and Cohen, R. C.: Steps towards a mechanistic model of global soil nitric oxide emissions: implementation and space based-constraints, Atmos. Chem. Phys., 12, 7779–7795, https://doi.org/10.5194/acp-12-7779-2012, 2012.
Jathar, S. H., Farina, S. C., Robinson, A. L., and Adams, P. J.: The influence of semi-volatile and reactive primary emissions on the abundance and properties of global organic aerosol, Atmos. Chem. Phys., 11, 7727–7746, https://doi.org/10.5194/acp-11-7727-2011, 2011.
Jathar, S. H., Gordon, T. D., Hennigan, C. J., Pye, H. O., Pouliot, G., Adams, P. J., Donahue, N. M., and Robinson, A. L.: Unspeciated organic emissions from combustion sources and their influence on the secondary organic aerosol budget in the United States, P. Natl. Acad. Sci. USA, 111, 10473–10478, https://doi.org/10.1073/pnas.1323740111, 2014.
Jiang, J., Aksoyoglu, S., El-Haddad, I., Ciarelli, G., Denier van der Gon, H. A. C., Canonaco, F., Gilardoni, S., Paglione, M., Minguillón, M. C., Favez, O., Zhang, Y., Marchand, N., Hao, L., Virtanen, A., Florou, K., O'Dowd, C., Ovadnevaite, J., Baltensperger, U., and Prévôt, A. S. H.: Sources of organic aerosols in Europe: a modeling study using CAMx with modified volatility basis set scheme, Atmos. Chem. Phys., 19, 15247–15270, https://doi.org/10.5194/acp-19-15247-2019, 2019.
Kasibhatla, P., Sherwen, T., Evans, M. J., Carpenter, L. J., Reed, C., Alexander, B., Chen, Q., Sulprizio, M. P., Lee, J. D., Read, K. A., Bloss, W., Crilley, L. R., Keene, W. C., Pszenny, A. A. P., and Hodzic, A.: Global impact of nitrate photolysis in sea-salt aerosol on NOx, OH, and O3 in the marine boundary layer, Atmos. Chem. Phys., 18, 11185–11203, https://doi.org/10.5194/acp-18-11185-2018, 2018.
Khare, P. and Gentner, D. R.: Considering the future of anthropogenic gas-phase organic compound emissions and the increasing influence of non-combustion sources on urban air quality, Atmos. Chem. Phys., 18, 5391–5413, https://doi.org/10.5194/acp-18-5391-2018, 2018.
Kim, P. S., Jacob, D. J., Fisher, J. A., Travis, K., Yu, K., Zhu, L., Yantosca, R. M., Sulprizio, M. P., Jimenez, J. L., Campuzano-Jost, P., Froyd, K. D., Liao, J., Hair, J. W., Fenn, M. A., Butler, C. F., Wagner, N. L., Gordon, T. D., Welti, A., Wennberg, P. O., Crounse, J. D., St. Clair, J. M., Teng, A. P., Millet, D. B., Schwarz, J. P., Markovic, M. Z., and Perring, A. E.: Sources, seasonality, and trends of southeast US aerosol: an integrated analysis of surface, aircraft, and satellite observations with the GEOS-Chem chemical transport model, Atmos. Chem. Phys., 15, 10411–10433, https://doi.org/10.5194/acp-15-10411-2015, 2015.
Kong, L., Tang, X., Zhu, J., Wang, Z., Fu, J. S., Wang, X., Itahashi, S., Yamaji, K., Nagashima, T., Lee, H.-J., Kim, C.-H., Lin, C.-Y., Chen, L., Zhang, M., Tao, Z., Li, J., Kajino, M., Liao, H., Wang, Z., Sudo, K., Wang, Y., Pan, Y., Tang, G., Li, M., Wu, Q., Ge, B., and Carmichael, G. R.: Evaluation and uncertainty investigation of the NO2, CO and NH3 modeling over China under the framework of MICS-Asia III, Atmos. Chem. Phys., 20, 181–202, https://doi.org/10.5194/acp-20-181-2020, 2020.
Koo, B., Knipping, E., and Yarwood, G.: 1.5-Dimensional volatility basis set approach for modeling organic aerosol in CAMx and CMAQ, Atmos. Environ., 95, 158–164, https://doi.org/10.1016/j.atmosenv.2014.06.031, 2014.
Kuang, Y., He, Y., Xu, W., Yuan, B., Zhang, G., Ma, Z., Wu, C., Wang, C., Wang, S., Zhang, S., Tao, J., Ma, N., Su, H., Cheng, Y., Shao, M., and Sun, Y.: Photochemical Aqueous-Phase Reactions Induce Rapid Daytime Formation of Oxygenated Organic Aerosol on the North China Plain, Environ. Sci. Technol., 54, 3849–3860, https://doi.org/10.1021/acs.est.9b06836, 2020.
Kurtenbach, R., Becker, K. H., Gomes, J. A. G., Kleffmann, J., Lörzer, J. C., Spittler, M., Wiesen, P., Ackermann, R., Geyer, A., and Platt, U.: Investigations of emissions and heterogeneous formation of HONO in a road traffic tunnel, Atmos. Environ., 35, 3385–3394, https://doi.org/10.1016/s1352-2310(01)00138-8, 2001.
Lei, L., Zhou, W., Chen, C., He, Y., Li, Z., Sun, J., Tang, X., Fu, P., Wang, Z., and Sun, Y.: Long-term characterization of aerosol chemistry in cold season from 2013 to 2020 in Beijing, China, Environ. Pollut., 268, 115952, https://doi.org/10.1016/j.envpol.2020.115952, 2021.
Li, G., Lei, W., Zavala, M., Volkamer, R., Dusanter, S., Stevens, P., and Molina, L. T.: Impacts of HONO sources on the photochemistry in Mexico City during the MCMA-2006/MILAGO Campaign, Atmos. Chem. Phys., 10, 6551–6567, https://doi.org/10.5194/acp-10-6551-2010, 2010.
Li, J., Hao, Y., Simayi, M., Shi, Y., Xi, Z., and Xie, S.: Verification of anthropogenic VOC emission inventory through ambient measurements and satellite retrievals, Atmos. Chem. Phys., 19, 5905–5921, https://doi.org/10.5194/acp-19-5905-2019, 2019a.
Li, J., Liu, Q., Li, Y., Liu, T., Huang, D., Zheng, J., Zhu, W., Hu, M., Wu, Y., Lou, S., Hallquist, A. M., Hallquist, M., Chan, C. K., Canonaco, F., Prevot, A. S. H., Fung, J. C. H., Lau, A. K. H., and Yu, J. Z.: Characterization of Aerosol Aging Potentials at Suburban Sites in Northern and Southern China Utilizing a Potential Aerosol Mass (Go:PAM) Reactor and an Aerosol Mass Spectrometer, J. Geophys. Res.-Atmos., 124, 5629–5649, https://doi.org/10.1029/2018JD029904, 2019b.
Li, J., Han, Z., Li, J., Liu, R., Wu, Y., Liang, L., and Zhang, R.: The formation and evolution of secondary organic aerosol during haze events in Beijing in wintertime, Sci. Total Environ., 703, 134937, https://doi.org/10.1016/j.scitotenv.2019.134937, 2020.
Li, J., Han, Z. W., Sun, Y. L., Li, J. W., and Liang, L.: Chemical formation pathways of secondary organic aerosols in the Beijing–Tianjin–Hebei region in wintertime, Atmos. Environ., 244, 117996, https://doi.org/10.1016/j.atmosenv.2020.117996, 2021.
Li, M., Zhang, Q., Zheng, B., Tong, D., Lei, Y., Liu, F., Hong, C., Kang, S., Yan, L., Zhang, Y., Bo, Y., Su, H., Cheng, Y., and He, K.: Persistent growth of anthropogenic non-methane volatile organic compound (NMVOC) emissions in China during 1990–2017: drivers, speciation and ozone formation potential, Atmos. Chem. Phys., 19, 8897–8913, https://doi.org/10.5194/acp-19-8897-2019, 2019.
Li, Y., Ren, B., Qiao, Z., Zhu, J., Wang, H., Zhou, M., Qiao, L., Lou, S., Jing, S., Huang, C., Tao, S., Rao, P., and Li, J.: Characteristics of atmospheric intermediate volatility organic compounds (IVOCs) in winter and summer under different air pollution levels, Atmos. Environ., 210, 58–65, https://doi.org/10.1016/j.atmosenv.2019.04.041, 2019.
Li, Y. J., Lee, B. P., Su, L., Fung, J. C. H., and Chan, C. K.: Seasonal characteristics of fine particulate matter (PM) based on high-resolution time-of-flight aerosol mass spectrometric (HR-ToF-AMS) measurements at the HKUST Supersite in Hong Kong, Atmos. Chem. Phys., 15, 37–53, https://doi.org/10.5194/acp-15-37-2015, 2015.
Li, Y. J., Sun, Y., Zhang, Q., Li, X., Li, M., Zhou, Z., and Chan, C. K.: Real-time chemical characterization of atmospheric particulate matter in China: A review, Atmos. Environ., 158, 270–304, https://doi.org/10.1016/j.atmosenv.2017.02.027, 2017.
Liao, K., Chen, Q., Liu, Y., Li, Y. J., Lambe, A. T., Zhu, T., Huang, R.-J., Zheng, Y., Cheng, X., Miao, R., Huang, G., Khuzestani, R. B., and Jia, T.: Secondary Organic Aerosol Formation of Fleet Vehicle Emissions in China: Potential Seasonality of Spatial Distributions, Environ. Sci. Technol., 55, 7276–7286, https://doi.org/10.1021/acs.est.0c08591, 2021.
Lim, C. Y., Hagan, D. H., Coggon, M. M., Koss, A. R., Sekimoto, K., de Gouw, J., Warneke, C., Cappa, C. D., and Kroll, J. H.: Secondary organic aerosol formation from the laboratory oxidation of biomass burning emissions, Atmos. Chem. Phys., 19, 12797–12809, https://doi.org/10.5194/acp-19-12797-2019, 2019.
Ling, Z., Xie, Q., Shao, M., Wang, Z., Wang, T., Guo, H., and Wang, X.: Formation and sink of glyoxal and methylglyoxal in a polluted subtropical environment: observation-based photochemical analysis and impact evaluation, Atmos. Chem. Phys., 20, 11451–11467, https://doi.org/10.5194/acp-20-11451-2020, 2020.
Liu, H., Man, H., Cui, H., Wang, Y., Deng, F., Wang, Y., Yang, X., Xiao, Q., Zhang, Q., Ding, Y., and He, K.: An updated emission inventory of vehicular VOCs and IVOCs in China, Atmos. Chem. Phys., 17, 12709–12724, https://doi.org/10.5194/acp-17-12709-2017, 2017.
Liu, J., Deng, H., Li, S., Jiang, H., Mekic, M., Zhou, W., Wang, Y., Loisel, G., Wang, X., and Gligorovski, S.: Light-Enhanced Heterogeneous Conversion of NO2 to HONO on Solid Films Consisting of Fluorene and Fluorene/Na2SO4: An Impact on Urban and Indoor Atmosphere, Environ. Sci. Technol., 54, 11079–11086, https://doi.org/10.1021/acs.est.0c02627, 2020.
Liu, Y., Nie, W., Xu, Z., Wang, T., Wang, R., Li, Y., Wang, L., Chi, X., and Ding, A.: Semi-quantitative understanding of source contribution to nitrous acid (HONO) based on 1 year of continuous observation at the SORPES station in eastern China, Atmos. Chem. Phys., 19, 13289–13308, https://doi.org/10.5194/acp-19-13289-2019, 2019.
Lu, K., Guo, S., Tan, Z., Wang, H., Shang, D., Liu, Y., Li, X., Wu, Z., Hu, M., and Zhang, Y.: Exploring atmospheric free-radical chemistry in China: the self-cleansing capacity and the formation of secondary air pollution, Natl. Sci. Rev., 6, 579–594, https://doi.org/10.1093/nsr/nwy073, 2019.
Lu, Q., Zhao, Y., and Robinson, A. L.: Comprehensive organic emission profiles for gasoline, diesel, and gas-turbine engines including intermediate and semi-volatile organic compound emissions, Atmos. Chem. Phys., 18, 17637–17654, https://doi.org/10.5194/acp-18-17637-2018, 2018.
Lu, Q., Murphy, B. N., Qin, M., Adams, P. J., Zhao, Y., Pye, H. O. T., Efstathiou, C., Allen, C., and Robinson, A. L.: Simulation of organic aerosol formation during the CalNex study: updated mobile emissions and secondary organic aerosol parameterization for intermediate-volatility organic compounds, Atmos. Chem. Phys., 20, 4313–4332, https://doi.org/10.5194/acp-20-4313-2020, 2020.
Marais, E. A., Jacob, D. J., Jimenez, J. L., Campuzano-Jost, P., Day, D. A., Hu, W., Krechmer, J., Zhu, L., Kim, P. S., Miller, C. C., Fisher, J. A., Travis, K., Yu, K., Hanisco, T. F., Wolfe, G. M., Arkinson, H. L., Pye, H. O. T., Froyd, K. D., Liao, J., and McNeill, V. F.: Aqueous-phase mechanism for secondary organic aerosol formation from isoprene: application to the southeast United States and co-benefit of SO2 emission controls, Atmos. Chem. Phys., 16, 1603–1618, https://doi.org/10.5194/acp-16-1603-2016, 2016.
May, A. A., Levin, E. J. T., Hennigan, C. J., Riipinen, I., Lee, T., Collett, J. L., Jimenez, J. L., Kreidenweis, S. M., and Robinson, A. L.: Gas-particle partitioning of primary organic aerosol emissions: 3. Biomass burning, J. Geophys. Res.-Atmos., 118, 11327–11338, https://doi.org/10.1002/jgrd.50828, 2013a.
May, A. A., Presto, A. A., Hennigan, C. J., Nguyen, N. T., Gordon, T. D., and Robinson, A. L.: Gas-Particle Partitioning of Primary Organic Aerosol Emissions: (2) Diesel Vehicles, Environ. Sci. Technol., 47, 8288–8296, https://doi.org/10.1021/es400782j, 2013b.
Meng, W., Shen, H., Yun, X., Chen, Y., Zhong, Q., Zhang, W., Yu, X., Xu, H., Ren, Y., Shen, G., Ma, J., Liu, J., Cheng, H., Wang, X., Zhu, D., and Tao, S.: Differentiated-Rate Clean Heating Strategy with Superior Environmental and Health Benefits in Northern China, Environ. Sci. Technol., 54, 13458–13466, https://doi.org/10.1021/acs.est.0c04019, 2020.
Miao, R., Chen, Q., Zheng, Y., Cheng, X., Sun, Y., Palmer, P. I., Shrivastava, M., Guo, J., Zhang, Q., Liu, Y., Tan, Z., Ma, X., Chen, S., Zeng, L., Lu, K., and Zhang, Y.: Model bias in simulating major chemical components of PM2.5 in China, Atmos. Chem. Phys., 20, 12265–12284, https://doi.org/10.5194/acp-20-12265-2020, 2020.
Oswald, R., Behrendt, T., Ermel, M., Wu, D., Su, H., Cheng, Y., Breuninger, C., Moravek, A., Mougin, E., Delon, C., Loubet, B., Pommerening-Roser, A., Sorgel, M., Poschl, U., Hoffmann, T., Andreae, M. O., Meixner, F. X., and Trebs, I.: HONO Emissions from Soil Bacteria as a Major Source of Atmospheric Reactive Nitrogen, Science, 341, 1233–1235, https://doi.org/10.1126/science.1242266, 2013.
Ots, R., Young, D. E., Vieno, M., Xu, L., Dunmore, R. E., Allan, J. D., Coe, H., Williams, L. R., Herndon, S. C., Ng, N. L., Hamilton, J. F., Bergström, R., Di Marco, C., Nemitz, E., Mackenzie, I. A., Kuenen, J. J. P., Green, D. C., Reis, S., and Heal, M. R.: Simulating secondary organic aerosol from missing diesel-related intermediate-volatility organic compound emissions during the Clean Air for London (ClearfLo) campaign, Atmos. Chem. Phys., 16, 6453–6473, https://doi.org/10.5194/acp-16-6453-2016, 2016.
Pai, S. J., Heald, C. L., Pierce, J. R., Farina, S. C., Marais, E. A., Jimenez, J. L., Campuzano-Jost, P., Nault, B. A., Middlebrook, A. M., Coe, H., Shilling, J. E., Bahreini, R., Dingle, J. H., and Vu, K.: An evaluation of global organic aerosol schemes using airborne observations, Atmos. Chem. Phys., 20, 2637–2665, https://doi.org/10.5194/acp-20-2637-2020, 2020.
Park, R. J., Jacob, D. J., Field, B. D., Yantosca, R. M., and Chin, M.: Natural and transboundary pollution influences on sulfate-nitrate-ammonium aerosols in the United States: Implications for policy, J. Geophys. Res.-Atmos., 109, D15204, https://doi.org/10.1029/2003JD004473, 2004.
Park, R. J., Oak, Y. J., Emmons, L. K., Kim, C.-H., Pfister, G. G., Carmichael, G. R., Saide, P. E., Cho, S.-Y., Kim, S., Woo, J.-H., Crawford, J. H., Gaubert, B., Lee, H. J., Park, S.-Y., Jo, Y.-J., Gao, M., Tang, B. M., Stanier, C. O., Shin, S. S., Park, H. Y., Bae, C., and Kim, E.: Multi-model intercomparisons of air quality simulations for the KORUS-AQ campaign, Elementa: Science of the Anthropocene, 9, 00139, https://doi.org/10.1525/elementa.2021.00139, 2021.
Peng, L., Zhang, Q., Yao, Z., Mauzerall, D. L., Kang, S., Du, Z., Zheng, Y., Xue, T., and He, K.: Underreported coal in statistics: A survey-based solid fuel consumption and emission inventory for the rural residential sector in China, Appl. Energ., 235, 1169–1182, https://doi.org/10.1016/j.apenergy.2018.11.043, 2019.
Presto, A. A., Miracolo, M. A., Donahue, N. M., and Robinson, A. L.: Secondary Organic Aerosol Formation from High-NOx Photo-Oxidation of Low Volatility Precursors: n-Alkanes, Environ. Sci. Technol., 44, 2029–2034, https://doi.org/10.1021/es903712r, 2010.
Pye, H. O. T. and Seinfeld, J. H.: A global perspective on aerosol from low-volatility organic compounds, Atmos. Chem. Phys., 10, 4377–4401, https://doi.org/10.5194/acp-10-4377-2010, 2010.
Pye, H. O. T., Chan, A. W. H., Barkley, M. P., and Seinfeld, J. H.: Global modeling of organic aerosol: the importance of reactive nitrogen (NOx and NO3), Atmos. Chem. Phys., 10, 11261–11276, https://doi.org/10.5194/acp-10-11261-2010, 2010.
Qian, Z., Chen, Y., Liu, Z., Han, Y., Zhang, Y., Feng, Y., Shang, Y., Guo, H., Li, Q., Shen, G., Chen, J., and Tao, S.: Intermediate Volatile Organic Compound Emissions from Residential Solid Fuel Combustion Based on Field Measurements in Rural China, Environ. Sci. Technol., 55, 5689–5700, https://doi.org/10.1021/acs.est.0c07908, 2021.
Qiu, X., Wang, S., Ying, Q., Duan, L., Xing, J., Cao, J., Wu, D., Li, X., Chengzhi, X., Yan, X., Liu, C., and Hao, J.: Importance of Wintertime Anthropogenic Glyoxal and Methylglyoxal Emissions in Beijing and Implications for Secondary Organic Aerosol Formation in Megacities, Environ. Sci. Technol., 54, 11809–11817, https://doi.org/10.1021/acs.est.0c02822, 2020.
Rappengluck, B., Lubertino, G., Alvarez, S., Golovko, J., Czader, B., and Ackermann, L.: Radical precursors and related species from traffic as observed and modeled at an urban highway junction, J. Air Waste Manage., 63, 1270–1286, https://doi.org/10.1080/10962247.2013.822438, 2013.
Rasool, Q. Z., Bash, J. O., and Cohan, D. S.: Mechanistic representation of soil nitrogen emissions in the Community Multiscale Air Quality (CMAQ) model v 5.1, Geosci. Model Dev., 12, 849–878, https://doi.org/10.5194/gmd-12-849-2019, 2019.
Robinson, A. L., Donahue, N. M., Shrivastava, M. K., Weitkamp, E. A., Sage, A. M., Grieshop, A. P., Lane, T. E., Pierce, J. R., and Pandis, S. N.: Rethinking Organic Aerosols: Semivolatile Emissions and Photochemical Aging, Science, 315, 1259–1262, https://doi.org/10.1126/science.1133061, 2007.
Sarwar, G., Roselle, S. J., Mathur, R., Appel, W., Dennis, R. L., and Vogel, B.: A comparison of CMAQ HONO predictions with observations from the northeast oxidant and particle study, Atmos. Environ., 42, 5760–5770, https://doi.org/10.1016/j.atmosenv.2007.12.065, 2008.
Shah, V., Jaegle, L., Jimenez, J. L., Schroder, J. C., Campuzano-Jost, P., Campos, T. L., Reeves, J. M., Stell, M., Brown, S. S., Lee, B. H., Lopez-Hilfiker, F. D., and Thornton, J. A.: Widespread Pollution From Secondary Sources of Organic Aerosols During Winter in the Northeastern United States, Geophys. Res. Lett., 46, 2974–2983, https://doi.org/10.1029/2018GL081530, 2019.
Shen, H., Huang, Y., Wang, R., Zhu, D., Li, W., Shen, G., Wang, B., Zhang, Y., Chen, Y., Lu, Y., Chen, H., Li, T., Sun, K., Li, B., Liu, W., Liu, J., and Tao, S.: Global Atmospheric Emissions of Polycyclic Aromatic Hydrocarbons from 1960 to 2008 and Future Predictions, Environ. Sci. Technol., 47, 6415–6424, https://doi.org/10.1021/es400857z, 2013.
Shrivastava, M., Easter, R. C., Liu, X. H., Zelenyuk, A., Singh, B., Zhang, K., Ma, P. L., Chand, D., Ghan, S., Jimenez, J. L., Zhang, Q., Fast, J., Rasch, P. J., and Tiitta, P.: Global transformation and fate of SOA: Implications of low-volatility SOA and gas-phase fragmentation reactions, J. Geophys. Res.-Atmos., 120, 4169–4195, https://doi.org/10.1002/2014JD022563, 2015.
Shrivastava, M. K., Lipsky, E. M., Stanier, C. O., and Robinson, A. L.: Modeling Semivolatile Organic Aerosol Mass Emissions from Combustion Systems, Environ. Sci. Technol., 40, 2671–2677, https://doi.org/10.1021/es0522231, 2006.
Slater, E. J., Whalley, L. K., Woodward-Massey, R., Ye, C., Lee, J. D., Squires, F., Hopkins, J. R., Dunmore, R. E., Shaw, M., Hamilton, J. F., Lewis, A. C., Crilley, L. R., Kramer, L., Bloss, W., Vu, T., Sun, Y., Xu, W., Yue, S., Ren, L., Acton, W. J. F., Hewitt, C. N., Wang, X., Fu, P., and Heard, D. E.: Elevated levels of OH observed in haze events during wintertime in central Beijing, Atmos. Chem. Phys., 20, 14847–14871, https://doi.org/10.5194/acp-20-14847-2020, 2020.
Spracklen, D. V., Jimenez, J. L., Carslaw, K. S., Worsnop, D. R., Evans, M. J., Mann, G. W., Zhang, Q., Canagaratna, M. R., Allan, J., Coe, H., McFiggans, G., Rap, A., and Forster, P.: Aerosol mass spectrometer constraint on the global secondary organic aerosol budget, Atmos. Chem. Phys., 11, 12109–12136, https://doi.org/10.5194/acp-11-12109-2011, 2011.
Sun, Y., He, Y., Kuang, Y., Xu, W., Song, S., Ma, N., Tao, J., Cheng, P., Wu, C., Su, H., Cheng, Y., Xie, C., Chen, C., Lei, L., Qiu, Y., Fu, P., Croteau, P., and Worsnop, D. R.: Chemical Differences Between PM1 and PM2.5 in Highly Polluted Environment and Implications in Air Pollution Studies, Geophys. Res. Lett., 47, e2019GL086288, https://doi.org/10.1029/2019GL086288, 2020a.
Sun, Y., Lei, L., Zhou, W., Chen, C., He, Y., Sun, J., Li, Z., Xu, W., Wang, Q., Ji, D., Fu, P., Wang, Z., and Worsnop, D. R.: A chemical cocktail during the COVID-19 outbreak in Beijing, China: Insights from six-year aerosol particle composition measurements during the Chinese New Year holiday, Sci. Total Environ., 742, 140739, https://doi.org/10.1016/j.scitotenv.2020.140739, 2020b.
Tan, Z., Rohrer, F., Lu, K., Ma, X., Bohn, B., Broch, S., Dong, H., Fuchs, H., Gkatzelis, G. I., Hofzumahaus, A., Holland, F., Li, X., Liu, Y., Liu, Y., Novelli, A., Shao, M., Wang, H., Wu, Y., Zeng, L., Hu, M., Kiendler-Scharr, A., Wahner, A., and Zhang, Y.: Wintertime photochemistry in Beijing: observations of ROx radical concentrations in the North China Plain during the BEST-ONE campaign, Atmos. Chem. Phys., 18, 12391–12411, https://doi.org/10.5194/acp-18-12391-2018, 2018.
Tan, Z., Hofzumahaus, A., Lu, K., Brown, S. S., Holland, F., Huey, L. G., Kiendler-Scharr, A., Li, X., Liu, X., Ma, N., Min, K. E., Rohrer, F., Shao, M., Wahner, A., Wang, Y., Wiedensohler, A., Wu, Y., Wu, Z., Zeng, L., Zhang, Y., and Fuchs, H.: No Evidence for a Significant Impact of Heterogeneous Chemistry on Radical Concentrations in the North China Plain in Summer 2014, Environ. Sci. Technol., 54, 5973–5979, https://doi.org/10.1021/acs.est.0c00525, 2020.
Tao, S., Ru, M. Y., Du, W., Zhu, X., Zhong, Q. R., Li, B. G., Shen, G. F., Pan, X. L., Meng, W. J., Chen, Y. L., Shen, H. Z., Lin, N., Su, S., Zhuo, S. J., Huang, T. B., Xu, Y., Yun, X., Liu, J. F., Wang, X. L., Liu, W. X., Cheng, H. F., and Zhu, D. Q.: Quantifying the rural residential energy transition in China from 1992 to 2012 through a representative national survey, Nature Energy, 3, 567–573, https://doi.org/10.1038/s41560-018-0158-4, 2018.
Turpin, B. J. and Lim, H.-J.: Species Contributions to PM2.5 Mass Concentrations: Revisiting Common Assumptions for Estimating Organic Mass, Aerosol Sci. Tech., 35, 602–610, https://doi.org/10.1080/02786820119445, 2001.
Vogel, B., Vogel, H., Kleffmann, J., and Kurtenbach, R.: Measured and simulated vertical profiles of nitrous acid – Part II. Model simulations and indications for a photolytic source, Atmos. Environ., 37, 2957–2966, https://doi.org/10.1016/S1352-2310(03)00243-7, 2003.
Wang, J., Ye, J., Zhang, Q., Zhao, J., Wu, Y., Li, J., Liu, D., Li, W., Zhang, Y., Wu, C., Xie, C., Qin, Y., Lei, Y., Huang, X., Guo, J., Liu, P., Fu, P., Li, Y., Lee, H. C., Choi, H., Zhang, J., Liao, H., Chen, M., Sun, Y., Ge, X., Martin, S. T., and Jacob, D. J.: Aqueous production of secondary organic aerosol from fossil-fuel emissions in winter Beijing haze, P. Natl. Acad. Sci. USA, 118, e2022179118, https://doi.org/10.1073/pnas.2022179118, 2021.
Wang, S. X., Zhao, B., Cai, S. Y., Klimont, Z., Nielsen, C. P., Morikawa, T., Woo, J. H., Kim, Y., Fu, X., Xu, J. Y., Hao, J. M., and He, K. B.: Emission trends and mitigation options for air pollutants in East Asia, Atmos. Chem. Phys., 14, 6571–6603, https://doi.org/10.5194/acp-14-6571-2014, 2014.
Whalley, L. K., Slater, E. J., Woodward-Massey, R., Ye, C., Lee, J. D., Squires, F., Hopkins, J. R., Dunmore, R. E., Shaw, M., Hamilton, J. F., Lewis, A. C., Mehra, A., Worrall, S. D., Bacak, A., Bannan, T. J., Coe, H., Percival, C. J., Ouyang, B., Jones, R. L., Crilley, L. R., Kramer, L. J., Bloss, W. J., Vu, T., Kotthaus, S., Grimmond, S., Sun, Y., Xu, W., Yue, S., Ren, L., Acton, W. J. F., Hewitt, C. N., Wang, X., Fu, P., and Heard, D. E.: Evaluating the sensitivity of radical chemistry and ozone formation to ambient VOCs and NOx in Beijing, Atmos. Chem. Phys., 21, 2125–2147, https://doi.org/10.5194/acp-21-2125-2021, 2021.
Woody, M. C., Baker, K. R., Hayes, P. L., Jimenez, J. L., Koo, B., and Pye, H. O. T.: Understanding sources of organic aerosol during CalNex-2010 using the CMAQ-VBS, Atmos. Chem. Phys., 16, 4081–4100, https://doi.org/10.5194/acp-16-4081-2016, 2016.
Wu, L., Ling, Z., Liu, H., Shao, M., Lu, S., Wu, L., and Wang, X.: A gridded emission inventory of semi-volatile and intermediate volatility organic compounds in China, Sci. Total Environ., 761, 143295, https://doi.org/10.1016/j.scitotenv.2020.143295, 2021.
Xu, W., Xie, C., Karnezi, E., Zhang, Q., Wang, J., Pandis, S. N., Ge, X., Zhang, J., An, J., Wang, Q., Zhao, J., Du, W., Qiu, Y., Zhou, W., He, Y., Li, Y., Li, J., Fu, P., Wang, Z., Worsnop, D. R., and Sun, Y.: Summertime aerosol volatility measurements in Beijing, China, Atmos. Chem. Phys., 19, 10205–10216, https://doi.org/10.5194/acp-19-10205-2019, 2019.
Xu, W., Chen, C., Qiu, Y., Li, Y., Zhang, Z., Karnezi, E., Pandis, S. N., Xie, C., Li, Z., Sun, J., Ma, N., Xu, W., Fu, P., Wang, Z., Zhu, J., Worsnop, D. R., Ng, N. L., and Sun, Y.: Organic aerosol volatility and viscosity in the North China Plain: contrast between summer and winter, Atmos. Chem. Phys., 21, 5463–5476, https://doi.org/10.5194/acp-21-5463-2021, 2021.
Xue, C., Zhang, C., Ye, C., Liu, P., Catoire, V., Krysztofiak, G., Chen, H., Ren, Y., Zhao, X., Wang, J., Zhang, F., Zhang, C., Zhang, J., An, J., Wang, T., Chen, J., Kleffmann, J., Mellouki, A., and Mu, Y.: HONO Budget and Its Role in Nitrate Formation in the Rural North China Plain, Environ. Sci. Technol., 54, 11048–11057, https://doi.org/10.1021/acs.est.0c01832, 2020.
Yang, W., Li, J., Wang, W., Li, J., Ge, M., Sun, Y., Chen, X., Ge, B., Tong, S., Wang, Q., and Wang, Z.: Investigating secondary organic aerosol formation pathways in China during 2014, Atmos. Environ., 213, 133–147, https://doi.org/10.1016/j.atmosenv.2019.05.057, 2019.
Yang, X., Lu, K., Ma, X., Liu, Y., Wang, H., Hu, R., Li, X., Lou, S., Chen, S., Dong, H., Wang, F., Wang, Y., Zhang, G., Li, S., Yang, S., Yang, Y., Kuang, C., Tan, Z., Chen, X., Qiu, P., Zeng, L., Xie, P., and Zhang, Y.: Observations and modeling of OH and HO2 radicals in Chengdu, China in summer 2019, Sci. Total Environ., 772, 144829, https://doi.org/10.1016/j.scitotenv.2020.144829, 2021.
Zhai, S., Jacob, D. J., Wang, X., Shen, L., Li, K., Zhang, Y., Gui, K., Zhao, T., and Liao, H.: Fine particulate matter (PM2.5) trends in China, 2013–2018: separating contributions from anthropogenic emissions and meteorology, Atmos. Chem. Phys., 19, 11031–11041, https://doi.org/10.5194/acp-19-11031-2019, 2019.
Zhang, J., An, J., Qu, Y., Liu, X., and Chen, Y.: Impacts of potential HONO sources on the concentrations of oxidants and secondary organic aerosols in the Beijing–Tianjin–Hebei region of China, Sci. Total Environ., 647, 836–852, https://doi.org/10.1016/j.scitotenv.2018.08.030, 2019.
Zhang, L., Chen, Y., Zhao, Y., Henze, D. K., Zhu, L., Song, Y., Paulot, F., Liu, X., Pan, Y., Lin, Y., and Huang, B.: Agricultural ammonia emissions in China: reconciling bottom-up and top-down estimates, Atmos. Chem. Phys., 18, 339–355, https://doi.org/10.5194/acp-18-339-2018, 2018.
Zhang, Q., Jimenez, J. L., Canagaratna, M. R., Allan, J. D., Coe, H., Ulbrich, I., Alfarra, M. R., Takami, A., Middlebrook, A. M., Sun, Y. L., Dzepina, K., Dunlea, E., Docherty, K., DeCarlo, P. F., Salcedo, D., Onasch, T., Jayne, J. T., Miyoshi, T., Shimono, A., Hatakeyama, S., Takegawa, N., Kondo, Y., Schneider, J., Drewnick, F., Borrmann, S., Weimer, S., Demerjian, K., Williams, P., Bower, K., Bahreini, R., Cottrell, L., Griffin, R. J., Rautiainen, J., Sun, J. Y., Zhang, Y. M., and Worsnop, D. R.: Ubiquity and dominance of oxygenated species in organic aerosols in anthropogenically-influenced Northern Hemisphere midlatitudes, Geophys. Res. Lett., 34, 6, https://doi.org/10.1029/2007GL029979, 2007.
Zhang, X., Jones, D. B. A., Keller, M., Walker, T. W., Jiang, Z., Henze, D. K., Worden, H. M., Bourassa, A. E., Degenstein, D. A., and Rochon, Y. J.: Quantifying Emissions of CO and NOx Using Observations From MOPITT, OMI, TES, and OSIRIS, J. Geophys. Res.-Atmos., 124, 1170–1193, https://doi.org/10.1029/2018JD028670, 2019.
Zhang, Y. and Tao, S.: Global atmospheric emission inventory of polycyclic aromatic hydrocarbons (PAHs) for 2004, Atmos. Environ., 43, 812–819, https://doi.org/10.1016/j.atmosenv.2008.10.050, 2009.
Zhao, B., Wang, S., Donahue, N. M., Jathar, S. H., Huang, X., Wu, W., Hao, J., and Robinson, A. L.: Quantifying the effect of organic aerosol aging and intermediate-volatility emissions on regional-scale aerosol pollution in China, Sci. Rep.-UK, 6, 28815, https://doi.org/10.1038/srep28815, 2016.
Zhao, Y., Nguyen, N. T., Presto, A. A., Hennigan, C. J., May, A. A., and Robinson, A. L.: Intermediate Volatility Organic Compound Emissions from On-Road Diesel Vehicles: Chemical Composition, Emission Factors, and Estimated Secondary Organic Aerosol Production, Environ. Sci. Technol., 49, 11516–11526, https://doi.org/10.1021/acs.est.5b02841, 2015.
Zhao, Y., Nguyen, N. T., Presto, A. A., Hennigan, C. J., May, A. A., and Robinson, A. L.: Intermediate Volatility Organic Compound Emissions from On-Road Gasoline Vehicles and Small Off-Road Gasoline Engines, Environ. Sci. Technol., 50, 4554–4563, https://doi.org/10.1021/acs.est.5b06247, 2016.
Zhao, Y., Saunois, M., Bousquet, P., Lin, X., Berchet, A., Hegglin, M. I., Canadell, J. G., Jackson, R. B., Hauglustaine, D. A., Szopa, S., Stavert, A. R., Abraham, N. L., Archibald, A. T., Bekki, S., Deushi, M., Jöckel, P., Josse, B., Kinnison, D., Kirner, O., Marécal, V., O'Connor, F. M., Plummer, D. A., Revell, L. E., Rozanov, E., Stenke, A., Strode, S., Tilmes, S., Dlugokencky, E. J., and Zheng, B.: Inter-model comparison of global hydroxyl radical (OH) distributions and their impact on atmospheric methane over the 2000–2016 period, Atmos. Chem. Phys., 19, 13701–13723, https://doi.org/10.5194/acp-19-13701-2019, 2019.
Zheng, B., Tong, D., Li, M., Liu, F., Hong, C., Geng, G., Li, H., Li, X., Peng, L., Qi, J., Yan, L., Zhang, Y., Zhao, H., Zheng, Y., He, K., and Zhang, Q.: Trends in China's anthropogenic emissions since 2010 as the consequence of clean air actions, Atmos. Chem. Phys., 18, 14095–14111, https://doi.org/10.5194/acp-18-14095-2018, 2018.
Zheng, B., Zhang, Q., Geng, G., Chen, C., Shi, Q., Cui, M., Lei, Y., and He, K.: Changes in China's anthropogenic emissions and air quality during the COVID-19 pandemic in 2020, Earth Syst. Sci. Data, 13, 2895–2907, https://doi.org/10.5194/essd-13-2895-2021, 2021.
Zheng, J., Shi, X., Ma, Y., Ren, X., Jabbour, H., Diao, Y., Wang, W., Ge, Y., Zhang, Y., and Zhu, W.: Contribution of nitrous acid to the atmospheric oxidation capacity in an industrial zone in the Yangtze River Delta region of China, Atmos. Chem. Phys., 20, 5457–5475, https://doi.org/10.5194/acp-20-5457-2020, 2020.
Zheng, Y., Cheng, X., Liao, K., Li, Y., Li, Y. J., Huang, R.-J., Hu, W., Liu, Y., Zhu, T., Chen, S., Zeng, L., Worsnop, D. R., and Chen, Q.: Characterization of anthropogenic organic aerosols by TOF-ACSM with the new capture vaporizer, Atmos. Meas. Tech., 13, 2457–2472, https://doi.org/10.5194/amt-13-2457-2020, 2020.
Zhu, J., Wang, S., Wang, H., Jing, S., Lou, S., Saiz-Lopez, A., and Zhou, B.: Observationally constrained modeling of atmospheric oxidation capacity and photochemical reactivity in Shanghai, China, Atmos. Chem. Phys., 20, 1217–1232, https://doi.org/10.5194/acp-20-1217-2020, 2020.