the Creative Commons Attribution 4.0 License.
the Creative Commons Attribution 4.0 License.
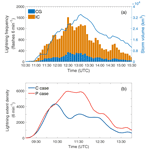
Aerosol effects on electrification and lightning discharges in a multicell thunderstorm simulated by the WRF-ELEC model
Mengyu Sun
Dongxia Liu
Xiushu Qie
Edward R. Mansell
Yoav Yair
Alexandre O. Fierro
Shanfeng Yuan
Zhixiong Chen
Dongfang Wang
To investigate the effects of aerosols on lightning activity, the Weather Research and Forecasting (WRF) Model with a two-moment bulk microphysical scheme and bulk lightning model was employed to simulate a multicell thunderstorm that occurred in the metropolitan Beijing area. The results suggest that under polluted conditions lightning activity is significantly enhanced during the developing and mature stages. Electrification and lightning discharges within the thunderstorm show characteristics distinguished by different aerosol conditions through microphysical processes. Elevated aerosol loading increases the cloud droplets numbers, the latent heat release, updraft and ice-phase particle number concentrations. More charges in the upper level are carried by ice particles and enhance the electrification process. A larger mean-mass radius of graupel particles further increases non-inductive charging due to more effective collisions. In the continental case where aerosol concentrations are low, less latent heat is released in the upper parts and, as a consequence, the updraft speed is weaker, leading to smaller concentrations of ice particles, lower charging rates and fewer lightning discharges.
- Article
(10574 KB) - Full-text XML
- BibTeX
- EndNote
Lightning activity is related to two important factors: dynamic–thermodynamic and microphysical characteristics (e.g., Williams et al., 2005; Rosenfeld et al., 2008; Guo et al., 2016; Wang et al., 2018; Zhao et al., 2020). Since the dynamic–thermodynamic processes affect the development of thunderstorm significantly, lightning activity is influenced by various dynamic–thermodynamic variables: temperature (Price, 1993), relative humidity in the lower and middle troposphere (Xiong et al., 2006; Fan et al., 2007), convective available potential energy (Qie et al., 2004; Stolz et al., 2015), and many others.
The impacts of aerosols on the development of thunderstorms especially in metropolitan areas have been researched extensively. Observational studies have indicated that the enhancement of lightning activity is related to increased cloud condensation nuclei (CCN) concentration (e.g., Westcott, 1995; Orville et al., 2001; Kar et al., 2009; Wang et al., 2011; Chaudhuri and Middey, 2013; Thornton et al., 2017; Yair, 2018; Qie et al., 2021). Kar et al. (2009) found a positive correlation between PM10 and SO2 concentration and lightning flash densities around major cities in South Korea. A positive relationship between levels of particle pollution and lightning flash counts was also indicated by Chaudhuri and Middey (2013).
Furthermore, a variety of numerical simulations (e.g., Mitzeva et al., 2006) have demonstrated the effects of aerosol on enhancing lightning activity. Using the Weather Research and Forecasting (WRF) Model with explicit spectral bin microphysics, Khain et al. (2010) found elevated aerosols increased the number of cloud droplets and the release of latent heat by acting as CCN. Therefore, more liquid water was lifted to the mixed-phase region by strong updrafts, with more ice-phase particles produced which can affect charge separation and lightning formation (Takahashi, 1978; Saunders and Peck, 1998; Takahashi, 1983; Mansell et al., 2005; Yair, 2008; Yair et al., 2010, 2021). Mansell and Ziegler (2013) suggested that greater CCN concentration led to greater lightning activity up to a point by testing a wide range of CCN concentrations in a 3D model with two-moment bulk microphysics and stochastically branched discharge parameterization (Mansell et al., 2002). They also noted that average graupel density stayed high at lower CCN but dropped at higher CCN because smaller droplets caused lower rime density. Zhao et al. (2015) showed that enhancing aerosol concentration resulted in an enhancement of electrification processes due to the increasing growth rate of snow and graupel particles. However, Tan et al. (2017) simulated a thunderstorm in the city of Changchun with a 3D cumulus model coupled with an aerosol module, electrification and lightning discharge, showing that the ice crystal and graupel number increased while the graupel mixing ratio decreased as the aerosol concentration increased.
The microphysical processes under different CCN concentrations, especially the initiation and growth of ice-phase particles, varied in different simulation studies. There are few studies that have discussed the aerosol effects on thunderstorm with explicit electrification and discharge parameterization in the model simultaneously (e.g., Mitzeva et al., 2006; Mansell and Ziegler, 2013; Zhao et al., 2015). The detailed effects of aerosols on the discharging need further study.
By analyzing lightning data from the Beijing Lightning Network (BLNET) and PM2.5 (particulate matter with aerodynamic diameter less than or equal to 2.5 µm) data, Sun et al. (2020) found a positive relationship between flash counts and PM2.5 concentration prior to the occurrence of a thunderstorm. As a megacity, Beijing has higher aerosol concentration resulting from anthropogenic air pollution. Still, the effects of aerosols on both electrification and discharges have been rarely discussed in this area using numerical simulation. Therefore, in this paper we present sensitivity studies on how the different CCN concentrations influence the characteristics of thunderclouds over the metropolitan Beijing area using WRF-ELEC (Fierro et al., 2013). We conducted sensitivity studies to evaluate the response of the microphysical properties, as well as electrification and lightning processes, to aerosol characteristics. This paper is organized as follows: Sect. 2 describes the data and methodology used in the study, Sect. 3 introduces the design of simulations, Sect. 4 presents the results, and Sect. 5 discusses and summarizes the study.
2.1 Observational dataset
Total flash numbers were obtained from the Beijing Lightning Network (BLNET), which consists of 16 stations which have covered areas extending 110 km east–west and 120 km north–south since 2015 (refer to Fig. 1). The BLNET provides 3D location results of flashes, including both intra-cloud (IC) and cloud-to-ground (CG) lightning (Wang et al., 2016). The average detection efficiency of the BLNET is 93.2 % for the total flashes (Srivastava et al., 2017). In this study, the 3D location lightning radiation pulses were grouped into flashes based on the criteria of 400 ms and 15 km. These grouping criteria were modified from the algorithm in Srivastava et al. (2017). In Sect. 3, the lightning frequency from BLNET was calculated in 6 min intervals, corresponding to the time span of Doppler radar scanning. In addition, the radar reflectivity data were obtained from an S-band Doppler radar (Chinese CINRAD-SA) near the Beijing urban area (39.81∘ N, 116.47∘ E) and were updated every 6 min. The vertical levels vary from 500 m to 20 km and were processed into composite radar reflectivity with a horizontal resolution (0.01∘ × 0.01∘). The precipitation data were taken from 295 gauge stations in a weather monitoring network of automatic weather stations in the Beijing region (refer to Fig. 1), with spacing of approximately 3 km in the urban area. The real-time hourly average ground levels of PM2.5 are from the China National Environmental Monitoring Center (http://106.37.208.233:20035/, last access: 16 August 2021).
2.2 Synoptic background
A mesoscale convective system over the Beijing area influenced by a strong Mongolia cold vortex on 11 August 2017 was simulated in this study. Based on the weather map at 00:00 UTC (figure not shown), there was a prevailing westward airflow in the south of the cold vortex, which brought dry cold air in the middle layer. At a low level of 850 hPa, the southwesterly jet transported a warm and humid air mass, forming an unstable condition together with the cold air mass above. The sounding profile over Beijing (39.9∘ N, 116.2∘ E) exhibited an unstable thermodynamic condition for thunderstorm initialization, as shown in Fig. 2, with surface-based convective available potential energy (CAPE) of 3937 J kg−1 at 00:00 UTC. The special terrain condition with mountains in the northwest and ocean in the southeast (Qie et al., 2020), as well as the heat island effect and elevated aerosol loading in the urban region (Zhang et al., 2013; Liu et al., 2018), likely enhanced the convection and was responsible for the occurrence of heavy rainfall and large hail as well as intensive lightning activity in the Beijing area. According to the surface-based automatic weather observation network in Beijing, the average rainfall in the urban area and the eastern region was 10–30 mm, locally exceeding 100 mm. The total lightning flashes of this case accounted for one-third of the total number of lightning flashes during the 2017 warm season (Chen et al., 2020).
2.3 Model overview
The WRF Model (version 3.9.1) coupled with a bulk lightning model (BLM; Fierro et al., 2013) and a two-moment bulk microphysics scheme (Mansell et al., 2010; Mansell and Ziegler, 2013) was used to simulate the multicell thunderstorm that occurred on 11 August 2017 in the Beijing metropolitan area.
The simulations employ the two-moment bulk microphysics scheme of Mansell et al. (2010), which predicts both the mass mixing ratio and number concentration for a range of hydrometeor species (droplets, rain, ice crystals, snow, graupel and hail). Microphysical processes include cloud droplet nucleation, condensation, collection–coalescence, riming, ice multiplication, freezing and melting, and conversion between different hydrometeors. It is noted that the predicted graupel density is variable (300–900 kg m−3), which makes it possible for the single graupel category to represent a range of particles from high-density frozen drops (or small hail) to low-density graupel (Mansell et al., 2010). The graupel growth processes include the collection of ice crystals by graupel, collection of snow particles by graupel, deposition of vapor to graupel, collection of supercooled water (cloud droplets and/or raindrops) by graupel and conversions between hydrometeors. Further details of the interactions among particles can be found in Mansell and Ziegler (2013), Mansell et al. (2010), and Ziegler (1985). The CCN concentration is predicted as a bulk activation spectrum and initially mixed well vertically, following Eq. (1) of Mansell et al. (2010):
where CCN is the assumed CCN concentration, S is the supersaturation with respect to liquid water and k=0.6. The initiation of cloud droplets (for both cloud base and in-cloud) is based on Twomey (1959) and adjusted by Mansell et al. (2010).
Explicit charging physics includes both non-inductive charging (Saunders and Peck, 1998) and inductive or polarization charging (Ziegler et al., 1991). We employed the non-inductive electrification scheme described by Saunders and Peck (1998) and adjusted by Mansell et al. (2005) in this study. The magnitude of charge separated within a grid cell (δq) is calculated from the non-inductive critical charging curve as a function of temperature and the riming accretion rate (RAR), following Eq. (2) of Mansell et al. (2005):
where B,a and b are a function of crystal size; is the mean volume diameter of the ice crystal or snow category; and are the mass-weighted mean terminal fall speeds for graupel and ice crystal; and q±(RAR) is the charge separation as a function of the RAR from Brooks et al. (1997) adjusted by Mansell et al. (2005). Non-inductive (i.e., independent of external electric fields) charge separation resulting from rebounding collisions between various ice-phase particles (ice, graupel, snow, hail) is parameterized based on results obtained from laboratory experiments (Takahashi, 1978; Saunders et al., 2001; Mansell et al., 2005). Inductive charging requires a pre-existing electric field to induce charge on the surfaces of the colliding particles (Mansell et al., 2005). Numerical experiments (Mansell et al., 2010) have found that total inductive charging is about an order of magnitude weaker than non-inductive charging but can be important for lower-charge regions. Only collisions between cloud droplets and ice-phase particles (graupel, ice, hail) are considered for inductive electrification. The electric field is simulated by solving the Poisson equation for the electric potential Φ:
where ρtot is the net space charge and ε is the electric permittivity of air ( F m−1). A message-passing-interface (MPI) black box multigrid iterative solver or BoxMG algorithm (Dendy, 1987) is extended to solved Eq. (3). And then the three components of the electric field and its magnitude are computed from Eq. (4):
The discharge model parameterization from Ziegler and MacGorman (1994) assumes a cylindrical region (Fierro et al., 2013). A flash is initiated when the electric field exceeds a breakdown threshold, which is a variant of the vertical electric profile of Dwyer (2003) at a model grid point (from here on, we shall use the term “grid points” for short). A discharge is centered at the initiation grid points within a cylinder that extends vertically through the depth of the domain. If the space charge magnitude at a grid point exceeds a specific space charge threshold (0.1 nC m−3 herein), this grid point will be involved in discharge within the cylinder during this time step. After each discharge, the charge magnitude is set to 70 % (Rawlins, 1982; Ziegler and MacGorman, 1994) of the summed magnitude for all grid points. Then the charges will be redistributed throughout all discharge volumes and the electric field is recalculated. The discharge in each time step will be terminated until the maximum electric field no longer exceeds the breakdown threshold. An estimate of the flash origin density (FOD) rate (over a time period ) is computed following Eq. (5):
where G is the horizontal grid cell area and C the cylinder cross-sectional area (set in the following simulations to radius R=12 km; Fierro et al., 2013). In this study, the integral represents the sum of flashes B(t) that extend into the grid column for all the time steps within the time period T. Further, flash extent density (FED) is given by Eq. (6). Thus, the predicted flash extent density over the Beijing area in Sect. 3 is the FED calculated in 6 min intervals:
2.4 Design of the simulations
The nested model configuration for the simulations is shown in Table 1. The WRF-ELEC model is configured by a two-way interactive nested domain. The outer domain (D01) has horizontal grid spacing of 6 km (442 × 391 grid points), and the inner domain (D02) is 2 km (496 × 496 grid points), both centering at 40∘ N, 116.05∘ E. The number of vertical levels is 40, and the top is set to 50 hPa for the two domains. The model physics configuration is the Unified Noah Land Surface Model (LSM; Chen and Dudhia, 2001). The longwave and shortwave radiation are parameterized following the Rapid Radiation Transfer Model (RRTM; Mlawer et al., 1997) and the Dudhia scheme (Dudhia, 1989), respectively. The Bougeault–Lacarrere planetary boundary layer (BouLac PBL) scheme is used to parameterize the boundary layer processes (Bougeault and Lacarrere, 1989). Simulations began at 00:00 UTC on 11 August 2017 and were integrated for 24 h. The period of interest was from 09:00 UTC until 17:00 UTC (time in the simulations). The 3-hourly National Centers for Environmental Prediction (NCEP) Global Forecast System (GFS) data with a 0.5∘ × 0.5∘ resolution are used to establish the initial and boundary conditions.
To survey the aerosol effects on the structure of thunderstorm and lightning activity, two sensitivity experiments are performed with different CCN concentrations: a polluted case (P case) and a continental case (C case). Figure 3 shows hourly average mass concentration of PM2.5 on 11 August 2017. The hourly average value of the observed PM2.5 concentration before the thunderstorm initiation (more than 110 µg m−3) is much higher than the 3-year mean PM2.5 concentration (69.4±54.8 µg m−3) in the Beijing area (Liu et al., 2018). Therefore, the CCN concentration is selected as the P case which is consistent with observation. The initial value for the P case is set as a number mixing ratio relative to sea level air density ρ0: kg−1, where ρ0=1.225 kg m−3, and the local number concentration is ) cm−3. And the initial number concentration for the C case is set at ) cm−3, consistent with typical continental conditions (e.g., Hobbs and Rangno, 1985; Mansell et al., 2005). The relatively high prescribed CCN concentration guaranteed small droplet diameters and should effectively delay the warm-rain process in the model (Mansell and Ziegler, 2013).
3.1 Radar reflectivity, precipitation and lightning flashes of multicell
Figure 4 shows the observed and simulated radar reflectivity in different periods for both cases, with the formation of thunderstorms in the simulation earlier than the observation by about 1.5 h. Data assimilation was not applied in the current study, although assimilation of observational data can effectively improve high-impact weather forecasting (Sun et al., 2014; Lynn et al., 2015; Gustafsson et al., 2018). And the spin-up of the background aerosols is relatively short (Lynn et al., 2020). These reasons probably lead to the earlier occurrence of the simulated thunderstorm. So we display the simulation and observation with a ∼ 90 min time difference. It is clear that both simulated times in the P case exhibit an overall good agreement with the evolution and morphology of the radar echo, especially evidenced by the northeast–southwest orientation of the radar echo at 11:54 UTC in the simulated polluted case (13:24 UTC in the observation). We also present the comparison of radar reflectivity as a function of height from the observation and simulations in the corresponding periods (Fig. 5). According to the intensity and top height of the radar echo, the observed radar reflectivity is in better agreement with simulated radar reflectivity only in the polluted case. Note that the modeled reflectivity differs from the observation in the northwestern area (115.4–116.0∘ E; Fig. 4a, c and e); the impacts of aerosol on lightning activity will only be evaluated in the southeastern Beijing area (39.4–40.6∘ N, 116.0–117.5∘ E, shown in Fig. 4d; hereafter “domain” for short).
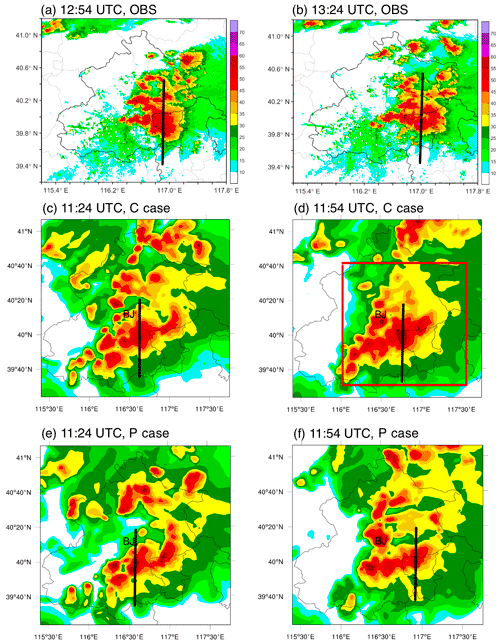
Figure 4Radar reflectivity (unit dBZ) between observation and simulation for the C and P cases; the simulation was earlier than the observation by about 1.5 h. (a–b) Observation at 12:54 and 13:24 UTC. (c–d) Simulation for the C case at 11:24 and 11:54 UTC. (e–f) Simulation for the P case at 11:24 and 11:54 UTC. The red rectangle in (d) denotes the region where the simulated results are analyzed in this study. BJ is Beijing.
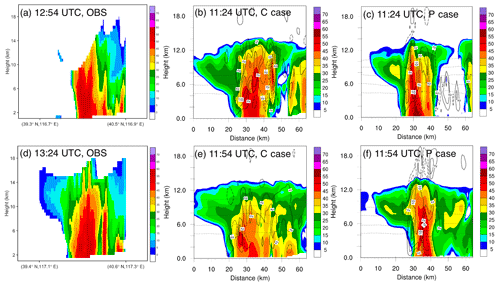
Figure 5Comparison between observation and simulations of vertical cross section of radar reflectivity (unit dBZ) along the black line shown in Fig. 4a–f. (a) Observation (black line shown in Fig. 4a). (b) C case (black line in Fig. 4c). (c) P case (black line in Fig. 4e). (d) Observation (black line shown in Fig. 4b). (e) C case (black line in Fig. 4d). (f) P case (black line in Fig. 4f).
Precipitation measurements from around 300 gauge stations in the Beijing area are compared with the WRF simulations. Figure 6 shows the hourly peak rainfall rate from the rain gauges and from simulations for the P case and C case. As noted, the formation of the thunderstorm in the simulations occurred about 1.5 h earlier than in the observation. So we display the simulations and observation with a 1 h time shift. It can be seen that the peak rainfall rate reaches the maximum at the same stage of development in both simulations (at 12:00 UTC) and the measurement (at 13:00 UTC). The rainfall in the P case continues for around 9 h, which is consistent with the gauge measurement, while the rainfall in the C case lasts 1 h less than the observation. The maximum peak rainfall rate in the P case is 97.3 mm h−1, which is larger than the measurement (and the C case) with a value of 80 mm h−1 (77.3 mm h−1). The difference in the rainfall rate is further analyzed through a comparison of the spatial distribution of precipitation. Figure 7 displays the 6-hourly accumulated precipitation from the observation (11:00–17:00 UTC) and from the simulations for the P and C cases (10:00–16:00 UTC). Both the simulations reproduce the precipitation in the southeastern region, where the gauge measurements show the accumulated rainfall exceed 100 mm. The coverage of the simulated precipitation in the P case extends to the northeast area compared to the C case (Fig. 7c), which is more consistent with the observation. This area is included in our analyzed region shown in Fig. 4d.
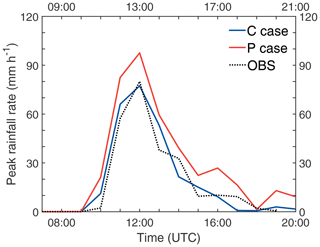
Figure 6Temporal evolution of the peak rainfall rate for observation and simulations. The dashed black line represents the observation; the red line corresponds to the P case, and the blue line corresponds to the C case. The x axis above is for the observation; the x axis below is for the simulations.
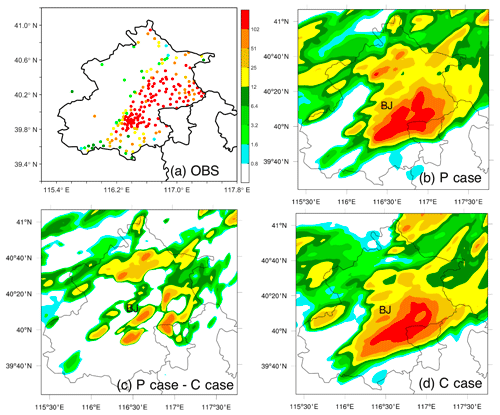
Figure 7Comparison of accumulated precipitation (units: mm) between observation (11:00–17:00 UTC) and simulations (10:00–16:00 UTC). (a) Observation. (b) P case and (d) C case. And (c) difference in accumulated precipitation (units: mm) between the P and C cases. BJ is Beijing.
The temporal variation in total flashes from BLNET is shown in Fig. 8a, including both intra-cloud (IC) and cloud-to-ground (CG) lightning. The lightning frequency gradually increased during 11:00–12:00 UTC and rose significantly after 12:00 UTC, reached its peak value at 12:30 UTC, and then decreased gradually. According to the evolution of radar reflectivity and lightning activity (Van Den Broeke et al., 2008; Kumjian et al., 2010; Liu et al., 2021), the real and simulated developments of the thunderstorm are shown in Table 2. The temporal evolution of predicted FED over the Beijing area under the polluted and continental cases are shown in Fig. 8b; both of them start earlier than the observation by about 1.5 h. Compared to the continental case, the variation in predicted flashes under polluted conditions is more consistent with the observation. The predicted FED for the P case and measured flashes increase significantly after 10:00 UTC (11:30 UTC in the observation) and reach a peak at around 11:00 UTC (12:30 UTC in the observation). In contrast, the predicted flashes for the C case reach a peak at around 10:30 UTC, earlier than the P case and measured lightning flashes, and then decrease dramatically. Within the duration of the thunderstorm, the overall FED in the polluted case is noticeably about 50 % higher than in the C case. The enhanced lightning activity simulated in the P case is in good agreement with the observation. Simulations under the polluted case do not outperform the C case in comparison to the observations in some aspects. For example, the maximum peak rainfall rate is larger than the measurement (and the C case, Fig. 6). The intensity of radar reflectivity and precipitation are strengthened under polluted conditions. Previous numerical simulations also suggested that greater aerosol concentrations lead to enhanced convection up to a point (e.g., Wang et al., 2011; Mansell et al., 2013; Lynn et al., 2020). Given that the developments of the thunderstorm were well simulated, here we try to analyze the differences in the lightning activity for both cases.
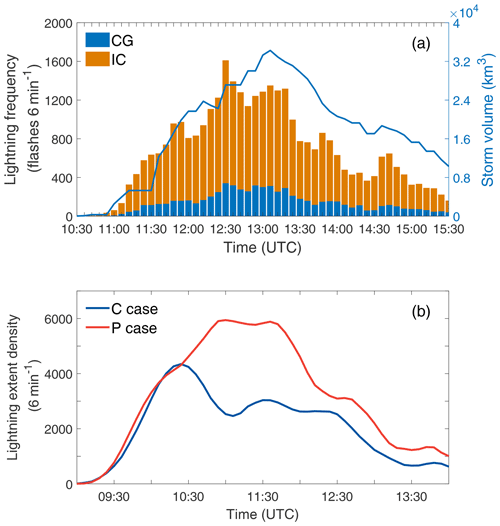
Figure 8Temporal variation in (a) observed total lightning frequency and (b) simulated flash extent density (FED). In (a), orange represents IC lightning and blue represents CG lightning. The solid line represents the storm volume associated with radar reflectivity exceeding 30 dBZ. In (b), the red line represents the P case and the blue line represents the C case.
Figure 9 displays the number of initiations over the Beijing area for the C case and P case during different periods. To examine the details of the lightning response to aerosols, the intensity of lightning activity can be categorized into four levels by the lightning grid points in each time step: light (50–100 grid points), moderate (100–200 grid points), heavy (200–300 grid points) and extreme (> 300 grid points). Then the number of points (grid columns) in each category is counted hourly as the “number of initiations”. A comparison of the different lightning intensity categories reveals that the simulated lightning activities increase during 10:30–12:30 UTC (Fig. 9b and c) under high aerosol loading, corresponding to the developing and mature stages of the thunderstorm. During 09:30–10:30 UTC, while different categories of lightning intensity are enhanced for both the P case and the C case (Fig. 9a), it is noted that the maximum lightning initiation occurs at the extreme level for the P case. In the dissipating stage, lightning activities decrease dramatically in the P and C case (Fig. 9d), but the lightning intensity under polluted conditions is still stronger compared to the C case. Hence, the results indicate that elevated aerosol loading enhances lightning activities especially in the developing and mature stages of thunderstorms. In the following we will offer a possible explanation for this effect.
3.2 Microphysical properties of multicell
To investigate the effects of aerosols on lightning activities, we first analyze the simulated microphysical properties in both the continental and polluted sensitivity studies. Figure 10a–h show the temporal variations in the vertical profiles for different hydrometeors. For each quantity, the mass mixing ratio and number concentration of hydrometeors are averaged horizontally over the analyzed region at a given altitude. The domain-averaged microphysical properties for the various hydrometeors are summarized in Table 3. The domain-averaged mean-mass radiush of hydrometeors in Table 3 is calculated following Eq. (7):
where ρair is the air density and ch, qh and nh are the density, mass concentration, and number concentration of hydrometeor species h (Mansell et al., 2010), respectively. Figure 10i–j display the time–height plots of maximum radar reflectivity and vertical velocities. The related convective properties are shown in Table 4.
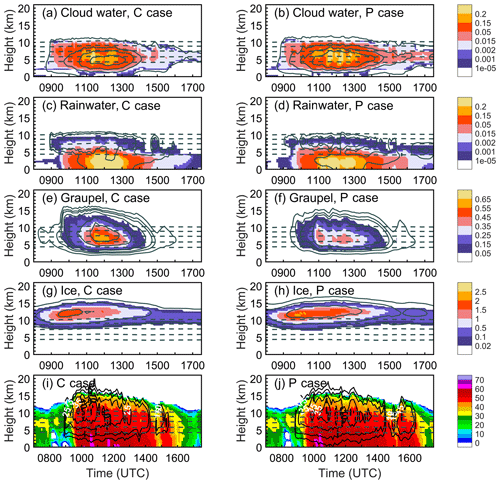
Figure 10(a–h) Temporal variation in the vertical profiles of the domain-averaged mass mixing ratio (g kg−1, shaded) and number concentration (kg−1, solid lines) of (a) cloud water in the C case, (b) cloud water in the P case, (c) rainwater in the C case, (d) rainwater in the P case, (e) graupel in the C case, (f) graupel in the P case, (g) ice in the C case and (h) ice in the P case. Contour levels in (a–h) are 106, 2×107, 5×107 and 108 kg−1 for the cloud water number concentration; 100 and 300 kg−1 for rainwater; 10, 30, 50, 100, 300, 500, 700 and 1000 kg−1 for graupel; and 0.1×107, 1×107 and 5×107 kg−1 for ice. (i–j) Time–height maximum simulated radar reflectivity (color shading, unit dBZ) and maximum vertical velocities (solid line and white label: 10, 15, 25, 35, 45 m s−1; dashed line and black label: −10, −15 m s−1) for (i) the C case and (j) the P case. The 0, −10, −20, −30 and −40 ∘C isotherms are shown by the dashed gray lines in (a)–(j).
It can be seen that elevated aerosol loading results in increasing cloud droplet concentrations (Fig. 10b and Table 3). Under polluted conditions, more aerosols could be activated into cloud droplets and more water vapor condenses onto these droplets, leading to large cloud water content and a small droplet size (Lynn et al., 2007; Wang et al., 2011; Zhao et al., 2015; Jiang et al., 2017). Thereby, relatively more latent heat of condensation is released in the P case where large cloud water content exists, which can be seen in the vertical distribution of peak latent heat (after 10:00 UTC, Fig. 12). The temporal variation in the domain-averaged mean-mass radius for cloud droplets is shown in Fig. 11. Under polluted conditions, cloud droplets with smaller mean-mass radii are too small to be converted into raindrops. As a consequence, the rainwater mass mixing ratio is less in the polluted case compared to in the continental one (Fig. 10d). Instead, these cloud droplets could be transported to higher levels (< −40 ∘C) by the strong updrafts resulting from increased latent heat. Previous studies have shown that larger vertical velocities are driven by increased microphysical latent heating (Wang et al., 2011; Mansell and Ziegler, 2013; Altaratz et al., 2017; Fan et al., 2018; Li et al., 2019). As shown in Table 4, the maximum updraft in the P case (53.5 m s−1) occurs above 12 km, while the height of maximum velocity for the C case (50.4 m s−1) is 10.5 km. As a result, the mixed-phase processes are enhanced and there are more ice crystals in the P case above 10 km (Fig. 10h), probably due to the homogeneous freezing of more but smaller cloud droplets (Straka and Mansell, 2005; Mansell et al., 2010). Observations and simulations also found that the content of ice crystals could be greater under polluted conditions, resulting from more condensation latent heat and strengthened updrafts (Khain et al., 2008; Koren et al., 2014; Wang et al., 2011; Zhao et al., 2015; Tan et al., 2017; Lynn et al., 2020). The number concentration of ice crystals is much larger under polluted conditions (Table 3), with a domain average of 3850 × 103 kg−1 for the polluted case and 2280 × 103 kg−1 for the continental case. The size of raindrops in the P case is larger, which is also found in Wang et al. (2011), probably due to the melting of ice-phase particles. These differences between cloud, rain droplets and ice crystals are directly influenced by the increasing aerosol loading. It is worth noting that the maximum of peak latent heat in the P case occurs above 10 km at 09:30 UTC (Fig. 12). As noted, the latent heat shown in Fig. 12 results from both condensation and freezing. At the beginning stage of the thunderstorm, the cloud and rainwater contents in both simulations are close (Fig. 10), which could be seen from the similar vertical distribution of peak latent heat for the temperatures warmer than −30 ∘C (before 10:00 UTC, Fig. 12). The high value of latent heat that existed in the higher levels (above 10 km) reveals a large release of frozen latent heat, indicating that more cloud droplets are lifted to the upper levels (< −40 ∘C) and converted into ice crystals (e.g., homogeneous freezing). Previous studies have also found that elevated aerosol loading contributes to the increase in frozen latent heat (e.g., Khain et al., 2005; Lynn et al., 2007; Storer et al., 2010; Li et al., 2017). The increased frozen latent heat during this period, together with relatively enhanced condensation latent heat, further ensures vigorous vertical growth and leads to the maximum updraft occurring at 10:48 UTC in the P case.
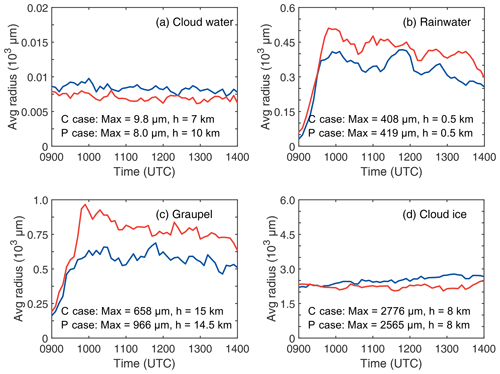
Figure 11Temporal variation in the domain-averaged mean-mass radius for the different hydrometeors. (a) Cloud water, (b) rainwater, (c) graupel, (d) ice. The red lines represent the P case, and the blue lines represent the C case.
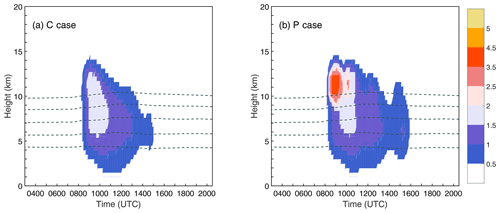
Figure 12Temporal variation in the vertical profiles of peak latent heating (K h−1, shaded) of (a) C case, and (b) P case. The 0, −10, −20, −30 and −40 ∘C isotherms are shown by the dashed gray lines in (a)–(b).
In contrast, the domain-averaged mass mixing ratio of graupel is less in the P case (Fig. 10e and f). Less graupel content under polluted conditions is rather surprising, since previous simulation studies (Wang et al., 2011; Zhao et al., 2015) have found that there could be more graupel at the mature stage of thunderstorms, by virtue of enhanced convection and more cloud droplets lifted to the mixed-phase region. This could happen if starting from a much lower CCN concentration (< 400 cm−3); in this study, with higher CCN concentration (> 1000 cm−3), the reduced raindrop freezing (Fig. 10d) probably explains the lower density of graupel. As mentioned before, the predicted graupel density is variable (Mansell et al., 2010). When graupel collects supercooled water in wet growth mode, the supercooled water is assumed to increase the graupel density if it is less than 900 kg m−3. And the collected ice crystals are only allowed to add graupel volume at the minimum density of graupel (300 kg m−3) in the simulations. This probably means that the reduced rainwater content results in significant reduction in the graupel mass mixing ratio under polluted conditions. Other simulations also found a decrease in the graupel mixing ratio under polluted conditions and partly attributed the decrease to the melting of graupel particles (Tan et al., 2017). In this study, the graupel content is higher in the C case, probably owing to higher rainwater content and corresponding raindrop freezing. It is worth noting that the number concentration of graupel in the polluted case is rather less compared to the continental one (Table 3), with 12 kg−1 for the P case and 28 kg−1 for the C case, respectively. Such a phenomenon could offer a partial explanation for the graupel of larger mean-mass radii appearing in the P case (Fig. 11c and Table 3). The domain-averaged mean-mass radius of graupel reaches 479.5 µm for the P case, compared to 322.4 µm for the C case. In contrast to the small difference in the mean-mass radius of ice crystals between the polluted and continental cases (Fig. 11d), the radius of graupel is much larger in the P case. This likely results in a larger collision efficiency between graupel and other ice-phase particles, enhancing non-inductive charging. Snow and hail are also involved in the electrification. By collecting droplets and ice-phase particles, the aggregation of snow is partially similar to the accretion of graupel (Zrnic et al., 1993; Ziegler, 1985) and the snow content is also less in the P case (figure not shown). Small hail could be represented by frozen drops in the graupel category (Mansell et al., 2010). And the differences in the hail between the two simulations (figure not shown) are not as obvious as those of graupel or ice crystal.
Increasing aerosol loading affects the key microphysical processes, especially in the ice-phase processes, yielding larger ice crystal content (or mass) and larger graupel size. Both larger ice crystal content and graupel size would inevitably affect lightning activity by affecting the rate and magnitude of charge separated during ice–graupel collisions.
3.3 The relationship between electrification, microphysics and dynamics
The time series of the peak positive (negative) charge density in the two cases are shown in Fig. 13. The domain-averaged peak charge structure in the P case is similar to that of the C case before 12:00 UTC, with the positive charge region distributed above the negative charge region. In both cases, the maximum peak positive charge density occurs above 8.5 km (< −30 ∘C), while the peak charge density for the polluted case is significantly greater, especially at the developing and mature stages (10:00–12:00 UTC). The peak positive charge density for the P case is more than +4 nC m−3 during this period, but the peak charge density is less than +2 nC m−3 in the C case. With the development of the thunderstorm, the charge density decreases gradually for both cases. At the upper levels, the peak charge density is still greater and lasts longer under polluted conditions.
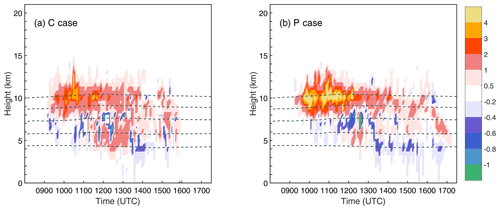
Figure 13Temporal variation in the vertical profiles of peak positive (negative) charge density (nC m−3, shaded) of (a) C case, and (b) P case. The 0, −10, −20, −30 and −40 ∘C isotherms are shown by the dashed gray lines in (a)–(b).
To analyze the relationship between hydrometeors and electrification, vertical cross sections are shown in Figs. 14a and 15a, which display the total charge distribution at the mature stage of the thunderstorm in the polluted (11:54 UTC) and continental cases (11:24 UTC), respectively. The cross sections were taken near the urban region, and the location varied depending on the location of the maximum value of radar reflectivity in both simulations. It is noted that the vertical profiles of the charge distribution are more detailed than the domain-averaged charge structure shown in Fig. 13. The charge structure with positive charge in the upper levels and negative charge in the lower levels was simulated in the C case. There were positive charge appeared in the lower-negative-charge center (Fig. 15a), which means that this charge structure is a little different from the normal dipole (upper charge positive, lower charge negative; e.g., Thomas et al., 2001). While the positive charge magnitude in the lower levels for the C case is relatively small to form normal tripole, in which a dominant region of negative charge with positive charge above and a positive charge below with approximately the same order of magnitude of charge (Simpson and Scrase, 1937; Williams, 1989). In the polluted case, with a negative charge region in the upper level (above 13 km), the updraft region exhibited a charge structure with a positive charge center located at the middle and a negative charge center at the lower level (e.g., Mansell et al., 2005; Zhang et al., 2021). For the total net space charge density, the maximum of positive charge density at the mature stage in the P case is up to +1 nC m−3, which is much higher than that in the C case (less than +0.5 nC m−3).
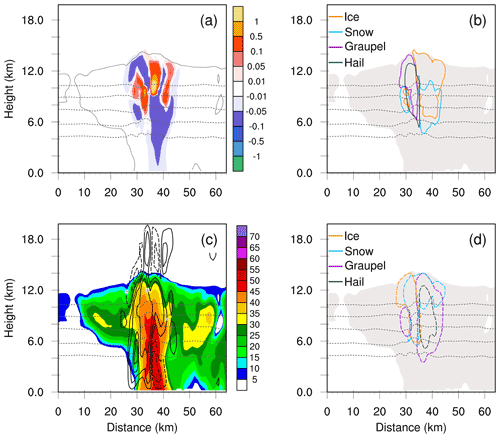
Figure 14Vertical cross sections (south to north) at the location shown in Fig. 4f of simulated variables at the mature stage of the thunderstorm (11:54 UTC) in the P case. (a) Total net space charge (nC m−3, shaded). The 0, −10, −20, −30 and −40 ∘C isotherms are shown by dashed gray lines in (a)–(d). (b) The +0.1 nC m−3 space charge density contours for cloud ice (orange), snow (blue), graupel (purple) and hail (black). The cloud outline (reflectivity echoes ≥5 dBZ) is denoted by the gray-shaded contour. (c) Radar reflectivity (unit dBZ), with black lines for vertical velocities (solid line: 2, 5, 10 m s−1; dashed line: −2 m s−1). (d) As in (b) but for a −0.1 nC m−3 charge density.
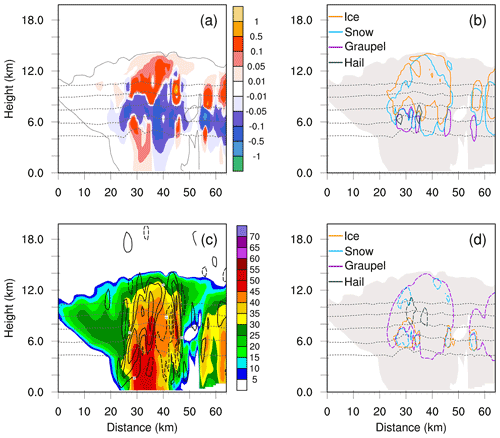
Figure 15As in Fig. 14 but for the vertical cross sections at the location shown in Fig. 4c of simulated variables at the mature stage of the thunderstorm (11:24 UTC) in the C case.
We attempt to explain the origins of the charge distribution by examining the polarity and amount of charge carried by different hydrometeor species (namely by ice, graupel, snow and hail particles). The negative charge region in the upper levels (12–15 km) for the P case resulted from collisions of graupel particles with smaller ice crystals and snow particles (Fig. 14d), with the 30 dBZ echo tops reaching 13 km. The simulated vertical distribution of net charge in the C case was caused by ice and snow particles charged positively at 8–12 km and graupel particles charged negatively at 4–7 km, respectively (Fig. 15b and d). The collisions between graupel and hail particles could partially explain the intense positive charge center located at 8–12 km in the P case. Less ice-phase particles appear in upper level in the continental case compared to the polluted one, corresponding to a relatively weaker charge center. Figures 14c and 15c show the cross sections of the simulated radar reflectivity and vertical velocity at 11:54 UTC (11:24 UTC) under different aerosol conditions. It is evident that both updraft and downdraft for the polluted case are greater than those for the continental one at higher levels, resulting from more frozen latent heat, and as a consequence, the total charge density is significantly greater above 12 km.
According to the non-inductive charging curve of Saunders and Peck (1998), graupel charged negatively within regions of relatively weak updrafts (< 5 m s−1) and lower liquid water content (LWC), forming a negative charge region at 4–8 km in the P case (Fig. 14a and d). With higher LWC in the polluted case, graupel, ice and hail were charged positively, forming a strong positive charge center at 9 km (< −20 ∘C), as shown in Fig. 14a. The simulations show that the non-inductive charging mechanism plays a main role at the mature stage, the rate of which is 1 order of magnitude larger than inductive charging (Fig. 16). As described in Sect. 4.2, more ice particles and graupel with larger radii appeared at this stage in the P case, evidenced by the larger simulated radar reflectivity (Fig. 14c), and the ensuing collision rates led to significantly stronger non-inductive charging at 6–10 km (Fig. 16b). In consequence, it is obvious in Figs. 14a and 15a that the charge density for the P case is much higher than for the C case, indicating that aerosols play an important role in affecting the accumulated charge density through microphysical and further electrical processes.
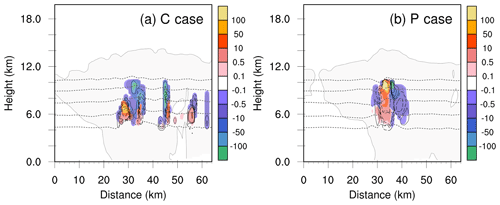
Figure 16Vertical cross sections (south to north) at the locations shown in Fig. 4c and f of non-inductive (pC m−3 s−1, shaded) and inductive (solid lines: 0.1, 0.5, 1 pC m−3 s−1; dashed lines: −0.1, −0.5, −1, −5, −10 pC m−3 s−1) charging rates at the mature stage of the (a) C case (11:24 UTC, Fig. 4c) and (b) P case (11:54 UTC, Fig. 4f). The 0, −10, −20, −30 and −40 ∘C isotherms are shown by dashed gray lines.
The appearance of more ice-phase particles in the upper level, increasing the ice crystal number and mean-mass radius of graupel particles, led to greater charge densities and as a consequence to stronger electric field intensities. Lightning discharge in WRF-ELEC occurs if the electric field magnitude exceeds a prescribed, fixed threshold, which further supports the important role of aerosols in enhancing storm electrification. Mansell et al. (2013) found that greater CCN concentration led to increased lightning activity up to a point, by affecting microphysical and electrical characteristics, with a large sensitivity to ice multiplication. In agreement with Mansell et al. (2013), this study shows that higher CCN concentration in the polluted case results in a relatively strong upper charge region, together with increased charge density and electric field intensity, finally enhancing lightning activity, as shown in Fig. 8b.
To elucidate the effects of aerosols on lightning activity, a two-moment bulk microphysics scheme (Mansell et al., 2010; Mansell and Ziegler, 2013) and bulk lightning model (BLM, Fierro et al., 2013) were coupled in the WRF Model to simulate a multicell thunderstorm that occurred on 11 August 2017 in the metropolitan Beijing area. The simulated distribution and spatio-temporal development of radar reflectivity under polluted conditions are in overall agreement with observations.
Sensitivity experiments show that the intensity and duration of lightning activity are evidently different between moderate (continental) and high (polluted) aerosol concentrations, resulting from microphysical processes. Elevated aerosol concentrations lead to increasing cloud droplet contents and a smaller droplet size. Smaller droplets suppress collection and coalescence processes and lead to less rainwater under polluted conditions. These cloud droplets which could not accrete by raindrops will be transported to higher levels and be converted into ice crystals. Increased latent heat release leads to strong updrafts, and in turn more cloud droplets could be lifted up. As a result, the ice crystal contents are much greater in the P case. Although the graupel contents are lower under polluted conditions resulting from less raindrop freezing, the radius of graupel is much larger in the P case due to a much lower number concentration. Consequently, elevated aerosol loading enhances the development of ice-phase microphysical processes, evidenced by more ice crystals and the larger radius of graupel participating in charge-separation and electrification processes. Non-inductive charging increases due to more frequent and effective collisions between graupel and other ice-phase particles. These bring about higher charge density, together with a larger upper charge region caused by more ice-phase particles lifted to higher levels, leading to electric field magnitudes which exceed the breakdown threshold value, eventually culminating in enhanced lightning activity. During the early stages of the thunderstorm, the latent heat release at a higher altitude is noticeably greater in the P case, mainly due to the release of frozen latent heat from cloud droplet freezing.
Observation and simulation studies have found that elevated aerosol loading enhances the electrical activity (e.g., Koren et al., 2010; Wang et al., 2011). Some previous studies have suggested that the mass mixing ratio of ice and graupel increases with enhanced CCN concentration, eventually resulting in stronger lightning activity (e.g., Wang et al., 2011; Zhao et al., 2015), while a decrease in the graupel mixing ratio has been found by Tan et al. (2017). It should also be noted that when aerosol concentrations are too large, this leads to the inhibition of convection, resulting in less lightning, as discovered by Altaratz et al. (2010) in the Amazon basin, as well as by Hu et al. (2019) in the Houston region, and simulated by Mansell and Ziegler (2013). In this study, we found the lightning activity was enhanced under polluted conditions, resulting from an increasing ice crystal number and radius of graupel particles. More ice-phase particles existed at upper levels under polluted conditions, forming a relatively strong charge region, which is also indicated by Zhao et al. (2015).
The impacts of aerosols on lightning were investigated acting as CCN; however, aerosols also tend to affect electrification and lightning discharge by acting as ice nuclei (IN) through microphysical processes (Tao et al., 2012; Fan et al., 2017). More sensitive experiments are still needed to discuss the influences of aerosols, acting as IN, on lightning due to microphysical and thermodynamic processes.
To request the data given in this study, please contact Dongxia Liu at the Institute of Atmospheric Physics, Chinese Academy of Sciences, via email (liudx@mail.iap.ac.cn).
MS and XQ designed the research ideas for this study. MS and DL carried the study out and prepared the paper. EM provided analysis ideas for the microphysics and electrification. YY and AF edited the paper. Other co-authors participated in science discussions and article modification.
The authors declare that they have no conflict of interest.
Publisher's note: Copernicus Publications remains neutral with regard to jurisdictional claims in published maps and institutional affiliations.
This research was jointly supported by the National Natural Science Foundation of China (grant nos. 41630425, 41875007) and the National Natural Science Foundation of China in collaboration with the Israel Science Foundation (grant no. 41761144074 NSFC-ISF and 2640/17 ISF-NSFC). The authors are thankful for the effort of all the people who participated in coordinated observations of dynamic–microphysical–electrical processes in severe thunderstorms and lightning hazards. Thanks go to the data support from the Ministry of Ecology and Environment of the People’s Republic of China. Thanks go to Jinyuan Xin (State Key Laboratory of Atmospheric Boundary Layer Physics and Atmospheric Chemistry (LAPC), Institute of Atmospheric Physics, Chinese Academy of Sciences) for the aerosol data analysis. Finally, we wish to thank the editor and four anonymous reviewers for their most helpful comments and suggestions.
This research has been supported by the National Natural Science Foundation of China (grant nos. 41630425, 41875007, and 41761144074 (NSFC-ISF) and 2640/17 (ISF-NSFC).
This paper was edited by Franziska Glassmeier and reviewed by four anonymous referees.
Altaratz, O., Koren, I., Yair, Y., and Price, C.: Lightning response to smoke from Amazonian fires, Geophys. Res. Lett., 37, L07801, https://doi.org/10.1029/2010GL042679, 2010.
Altaratz, O., Kucienska, B., Kostinski, A., Raga, G. B., and Koren, I.: Global association of aerosol with flash density of intense lightning, Environ. Res. Lett., 12, 114037, https://doi.org/10.1088/1748-9326/aa922b, 2017.
Bougeault, P. and Lacarrere, P.: Parameterization of orography-induced turbulence in a mesobeta–scale model, Mon. Weather Rev., 117, 1872–1890, https://doi.org/10.1175/1520-0493(1989)117<1872:POOITI>2.0.CO;2, 1989.
Brooks, I. M., Saunders, C. P. R., Mitzeva, R. P., and Peck, S. L.: The effect on thunderstorm charging of the rate of rime accretion by graupel, Atmos. Res., 43, 277–295, https://doi.org/10.1016/S0169-8095(96)00043-9, 1997.
Chaudhuri, S. and Middey, A.: Effect of meteorological parameters and environmental pollution on thunderstorm and lightning activity over an urban metropolis of India, Urban Clim., 3, 67–75, https://doi.org/10.1016/j.uclim.2013.03.003, 2013.
Chen, F. and Dudhia, J.: Coupling an advanced land surface–hydrology model with the Penn State–NCAR MM5 modeling system. Part I: Model implementation and sensitivity, Mon. Weather Rev., 129, 569–585, https://doi.org/10.1175/1520-0493(2001)129<0569:CAALSH>2.0.CO;2, 2001.
Chen, Z., Qie, X., Yair, Y., Liu, D., Xiao, X., Wang, D., and Yuan, S.: Electrical evolution of a rapidly developing MCS during its vigorous vertical growth phase, Atmos. Res., 246, 105201, https://doi.org/10.1016/j.atmosres.2020.105201, 2020.
Dendy Jr., J. E.: Two multigrid methods for three-dimensional problems with discontinuous and anisotropic coefficients, SIAM J. Sci. Stat. Comp., 8, 673–685, https://doi.org/10.1137/0908059, 1987.
Dudhia, J.: Numerical study of convection observed during the winter monsoon experiment using a mesoscale two-dimensional model, J. Atmos. Sci., 46, 3077–3107, https://doi.org/10.1175/1520-0469(1989)046<3077:NSOCOD>2.0.CO;2, 1989.
Dwyer, J. R.: A fundamental limit on electric fields in air, Geophys. Res. Lett., 30, 2055, https://doi.org/10.1029/2003GL017781, 2003.
Fan, J., Zhang, R., Li, G., and Tao, W., K.: Effects of aerosols and relative humidity on cumulus clouds, J. Geophys. Res., 112, D14204, https://doi.org/10.1029/2006jd008136, 2007.
Fan, J., Leung, L. R., Rosenfeld, D., and DeMott, P. J.: Effects of cloud condensation nuclei and ice nucleating particles on precipitation processes and supercooled liquid in mixed-phase orographic clouds, Atmos. Chem. Phys., 17, 1017–1035, https://doi.org/10.5194/acp-17-1017-2017, 2017.
Fan, J., Rosenfeld, D., Zhang, Y., Giangrande, S., Li, Z., Machado, L., Martin, S., Yang, Y., Wang, J., Artaxo, P., Barbosa, H., Braga, R., Comstock, J., Feng, Z., Gao, W., Gomes, H., Mei, F., Pöhlker, C., Pöhlker, M., Pöschl, U., and Souza, R.: Substantial convection and precipitation enhancements by ultrafine aerosol particles, Science, 359, 411–418, https://doi.org/10.1126/science.aan8461, 2018.
Fierro, A. O., Mansell, E. R., MacGorman, D. R., and Ziegler, C. L.: The implementation of an explicit charging and discharge lightning scheme within the WRF-ARW model: Benchmark simulations of a continental squall line, a tropical cyclone, and a winter storm, Mon. Weather Rev., 141, 2390–2415, https://doi.org/10.1175/MWR-D-12-00278.1, 2013.
Guo, J., Deng, M., Lee, S.S., Wang, F., Li, Z., Zhai, P., Liu, H., Lv, W., Yao, W., and Li, X.: Delaying precipitation and lightning by air pollution over the Pearl River Delta. Part I: Observational analyses, J. Geophys. Res.-Atmos., 121, 6472–6488, https://doi.org/10.1002/2015JD023257, 2016.
Gustafsson, N., Janjić, T., Schraff, C., Leuenberger, D., Weissmann, M., Reich, H., et al.: Survey of data assimilation methods for convective-scale numerical weather prediction at operational centres, Q. J. Roy. Meteor. Soc., 144, 1218–1256, https://doi.org/10.1002/qj.3179, 2018.
Hobbs, P. V. and Rangno, A. L.: Ice particle concentrations in clouds, J. Atmos. Sci., 42, 2523–2549, https://doi.org/10.1175/1520-0469(1985)042<2523:IPCIC>2.0.CO;2, 1985.
Hu, J. X., Rosenfeld, D., Ryzhkov, A., Zrnic, D., Williams, E., Zhang, P. F., Snyder, J. C., Zhang, R. Y., and Weitz, R.: Polarimetric radar convective cell tracking reveals large sensitivity of cloud precipitation and electrification properties to CCN, J. Geophys. Res.-Atmos., 124, 12194–12205, https://doi.org/10.1029/2019jd030857, 2019.
Jiang, M., Feng, J., Li, Z., Sun, R., Hou, Y.-T., Zhu, Y., Wan, B., Guo, J., and Cribb, M.: Potential influences of neglecting aerosol effects on the NCEP GFS precipitation forecast, Atmos. Chem. Phys., 17, 13967–13982, https://doi.org/10.5194/acp-17-13967-2017, 2017.
Kar, S. K., Liou, Y. A., and Ha, K. J.: Aerosol effects on the enhancement of cloud-to-ground lightning over major urban areas of South Korea, Atmos. Res., 92, 80–87, https://doi.org/10.1016/j.atmosres.2008.09.004, 2009.
Khain, A., Rosenfeld, D., and Pokrovsky, A.: Aerosol impact on the dynamics and microphysics of deep convective clouds, Q. J. Roy. Meteor. Soc., 131, 2639–2663, https://doi.org/10.1256/qj.04.62, 2005.
Khain, A., Cohen, N., Lynn, B., and Pokrovsky, A.: Possible aerosol effects on lightning activity and structure of hurricanes, J. Atmos. Sci., 65, 3652–3667, https://doi.org/10.1175/2008JAS2678.1, 2008.
Khain, A., Lynn, B., and Dudhia, J.: Aerosol Effects on Intensity of Landfalling Hurricanes as Seen from Simulations with the WRF Model with Spectral Bin Microphysics, J. Atmos. Sci., 67, 365–384, https://doi.org/10.1175/2009JAS3210.1, 2010.
Koren, I., Dagan, G., and Altaratz, O.: From aerosol-limited to invigoration of warm convective clouds, Science, 344, 1143–1146, https://doi.org/10.1126/science.1252595, 2014.
Kumjian, M. R., Ryzhkov, A. V., Melnikov, V. M., and Schuur, T. J.: Rapid-scan super-resolution observations of a cyclic supercell with a dual-polarization WSR-88D, Mon. Weather Rev., 138, 3762–3786, https://doi.org/10.1175/2010MWR3322.1, 2010.
Li, X., Zhang, Q., and Xue, H.: The role of initial cloud condensation nuclei concentration in hail using the WRF NSSL 2-moment microphysics scheme, Adv. Atmos. Sci., 34, 1106–1120, https://doi.org/10.1007/s00376-017-6237-9, 2017.
Li, Z. Q., Wang, Y., Guo, J. P., Zhao, C. F., Cribb, M. C., Dong, X. Q., Fan, J. W., Gong, D. Y., Huang, J. P., Jiang, M. J., Jiang, Y. Q., Lee, S. S., Li, H., Li, J. M., Liu, J. J., Qian, Y., Rosenfeld, D., Shan, S. Y., Sun, Y. L., Wang, H. J., Xin, J. Y., Yan, X., Yang, X., Yang, X. Q., Zhang, F., and Zheng, Y. T.: East Asian study of tropospheric aerosols and their impact on regional clouds, precipitation, and climate (EAST-AIR(CPC)), J. Geophys. Res.-Atmos., 124, 13026–13054, https://doi.org/10.1029/2019jd030758, 2019.
Liu, D., Sun, M., Su, D., Xu, W., Yu, H., and Chen, Y.: A five-year climatological lightning characteristics of linear mesoscale convective systems over North China, Atmos. Res., 256, 105580, https://doi.org/10.1016/j.atmosres.2021.105580, 2021.
Liu, Z., Gao, W., Yu, Y., Hu, B., Xin, J., Sun, Y., Wang, L., Wang, G., Bi, X., Zhang, G., Xu, H., Cong, Z., He, J., Xu, J., and Wang, Y.: Characteristics of PM2.5 mass concentrations and chemical species in urban and background areas of China: emerging results from the CARE-China network, Atmos. Chem. Phys., 18, 8849–8871, https://doi.org/10.5194/acp-18-8849-2018, 2018.
Lynn, B. H., Khain, A., Rosenfeld, D., and Woodley, W. L.: Effects of aerosols on precipitation from orographic clouds, J. Geophys. Res., 112, D10225, https://doi.org/10.1029/2006JD007537, 2007.
Lynn, B. H., Kelman, G., and Ellrod, G.: An evaluation of the efficacy of using observed lightning to improve convective lightning forecasts, Weather Forecast., 30, 405–423, https://doi.org/10.1175/WAF-D-13-00028.1, 2015.
Lynn, B. H., Yair, Y., Shpund, J., Levi, Y., Qie, X., and Khain, A.: Using factor separation to elucidate the respective contributions of desert dust and urban pollution to the 4 January 2020 Tel Aviv lightning and flash flood disaster, J. Geophys. Res.-Atmos., 125, e2020JD033520, https://doi.org/10.1029/2020JD033520, 2020.
Mansell, E. R. and Ziegler, C. L.: Aerosol effects on simulated storm electrification and precipitation in a two-moment bulk microphysics model, J. Atmos. Sci. 70, 2032–2050, https://doi.org/10.1175/JAS-D-12-0264.1, 2013.
Mansell, E. R., MacGorman, D. R., Ziegler, C. L., and Straka, J. M.: Simulated three-dimensional branched lightning in a numerical thunderstorm model, J. Geophys. Res., 107, https://doi.org/10.1029/2000JD000244, 2002.
Mansell, E. R., MacGorman, D. R., Ziegler, C. L., and Straka, J. M.: Charge structure and lightning sensitivity in a simulated multicell thunderstorm, J. Geophys. Res.-Atmos., 110, D12101, https://doi.org/10.1029/2004JD005287, 2005.
Mansell, E. R., Ziegler, C. L., and Bruning, E. C.: Simulated electrification of a small thunderstorm with two-moment bulk microphysics, J. Atmos. Sci., 67, 171–194, https://doi.org/10.1175/2009jas2965.1, 2010.
Mitzeva, R., Latham, J., and Petrova, S.: A comparative modeling study of the early electrical development of maritime and continental thunderstorms, Atmos. Res., 82, 26–36, https://doi.org/10.1016/j.atmosres.2005.01.006, 2006.
Mlawer, E. J., Taubman, S. J., Brown, P. D., Iacono, M., and Clough, S.: Radiative transfer for inhomogeneous atmospheres: RRTM, a validated correlated-k model for the longwave, J. Geophys. Res.-Atmos., 102, 16663–16682, https://doi.org/10.1029/97JD00237, 1997.
Orville, R. E., Huffines, G., Nielsen-Gammon, J., Zhang, R., Ely, B., Steiger, S., Phillips, S., Allen, S., and Read, W.: Enhancement of cloud-to-ground lightning over Houston, Texas, Geophys. Res. Lett., 28, 2597–2600, https://doi.org/10.1029/2001GL012990, 2001.
Price, C.: Global surface temperatures and the atmospheric electrical circuit, Geophys. Res. Lett., 20, 1363–1366, https://doi.org/10.1029/93GL01774, 1993.
Qie, X., Yuan, T., Xie, Y., and Ma, Y.: Spatial and temporal distribution of lightning activities on the Tibetan Plateau, Chinese J. Geophys., 47, 1122–1127, https://doi.org/10.1002/cjg2.596, 2004 (in Chinese).
Qie, K., Qie, X., and Tian, W.: Increasing trend of lightning activity in the South Asia region, Sci. Bull., 66, 78–84, https://doi.org/10.1016/j.scib.2020.08.033, 2021.
Qie, X., Yuan, T., Xie, Y., et al.: Spatial and temporal distribution of lightning activities on the Tibetan Plateau, Chinese J. Geophys., 47, 1122–1127, https://doi.org/10.1002/cjg2.596, 2004 (in Chinese).
Qie, X., Yuan, S., Chen, Z., Wang, D., Liu, D., Sun, M., Sun, Z., Srivastava, A., Zhang, H., Lu, J., Xiao, H., Bi, Y., Feng, L., Tian, Y., Xu, Y., Jiang, R., Liu, M., Xiao, X., Duan, S., Su, D., Sun, C., Xu, W., Zhang, Y., Lu, G., Zhang, D., Yin, Y., and Yu, Y.: Understanding the dynamical-microphysical-electrical processes associated with severe thunderstorms over the Beijing metropolitan region, Sci. China Earth Sci., 64, 10–26, https://doi.org/10.1007/s11430-020-9656-8, 2020.
Rawlins, F.: A numerical study of thunderstorm electrification using a three dimensional model incorporating the ice phase, Quart. J. Meteor. Soc., 108, 779–800, https://doi.org/10.1002/qj.49710845804, 1982.
Rosenfeld, D., Lohmann, U., Raga, G. B., O'Dowd, C. D., Kulmala, M., Fuzzi, S., Reissell, A., and Andreae, M. O.: Flood or Drought: How Do Aerosols Affect Precipitation, Science, 321, 1309–1313, https://doi.org/10.1126/science.1160606, 2008.
Saunders, C. P. R. and Peck, S. L.: Laboratory studies of the influence of the rime accretion rate on charge transfer during crystal/graupel collisions, J. Geophys. Res.-Atmos., 103, 13949–13956, https://doi.org/10.1029/97JD02644, 1998.
Saunders, C. P. R., Peck, S. L., Varela, G. G. A., Avila, E. E., and Castellano, N. E.: A laboratory study of the influence of water vapour and mixing on the charge transfer process during collisions between ice crystals and graupel, Atmos. Res., 58, 187–203, https://doi.org/10.1016/S0169-8095(01)00090-4, 2001.
Simpson, G. C. and Scrase, F. J.: The distribution of electricity in thunderclouds, P. Roy. Soc. Lond. A, 161, 309–352, https://doi.org/10.1098/rspa.1937.0148, 1937.
Srivastava, A., Tian, Y., Qie, X., Wang, D., Sun, Z., Yuan, S., Wang, Y., Chen, Z., Xu, W., Zhang, H., Jiang, R., and Su, D.: Performance assessment of Beijing Lightning Network (BLNET) and comparison with other lightning location networks across Beijing, Atmos. Res., 197, 76–83, https://doi.org/10.1016/j.atmosres.2017.06.026, 2017.
Stolz, D. C., Rutledge, S. A., and Pierce, J. R.: Simultaneous influences of thermodynamics and aerosols on deep convection and lightning in the tropics, J. Geophys. Res.-Atmos., 120, 6207–6231, https://doi.org/10.1002/2014JD023033, 2015.
Storer, R. L., van den Heever, S. C., and Stephens, G. L.: Modeling aerosol impacts on convective storms in different environments, J. Atmos. Sci., 67, 3904–3915, https://doi.org/10.1175/2010JAS3363.1, 2010.
Straka, J. M. and Mansell, E. R.: A bulk microphysics parameterization with multiple ice precipitation categories, J. Appl. Meteorol. Clim., 44, 445–466, https://doi.org/10.1175/JAM2211.1, 2005.
Sun, J., Xue, M., Wilson, J. W., Zawadzki, I., Ballard, S. P., Onvlee-Hooimeyer, J., Joe, P., Barker, D. M., Li, P., Golding, B., Xu, M., and Pinto, J.: Use of NWP for nowcasting convective precipitation: Recent progress and challenges, Bull. Am. Meteorol. Soc., 95, 409–426, https://doi.org/10.1175/BAMS-D-11-00263.1, 2014.
Sun, M., Qie, X., Liu, D., Yair, Y., Xia, X., Yuan, S., Wang, D., Lu, J., Srivastava, A., and Ntwali, D.: Analysis of potential effects of aerosol on lightning activity in Beijing metropolitan region, Chinese J. of Geophys., 63, 1766–1774, https://doi.org/10.6038/cjg2020N0095, 2020 (in Chinese).
Takahashi, T.: Riming electrification as a charge generation mechanism in thunderstorms, J. Atmos. Sci., 35, 1536–1548, https://doi.org/10.1175/1520-0469(1978)035<1536:REAACG>2.0.CO;2, 1978.
Takahashi, T.: A numerical simulation of winter cumulus electrification. Part I: Shallow cloud, J. Atmos. Sci., 40, 1257–1280, https://doi.org/10.1175/1520-0469(1983)040<1257:ANSOWC>2.0.CO;2, 1983.
Tan, Y., Ma, X., Xiang, C., Xia, Y., and Zhang, X.: A numerical study of the effects of aerosol on electrification and lightning discharges during thunderstorms, Chinese J. Geophys., 60, 3041–3050, https://doi.org/10.6038/cjg20170812, 2017 (in Chinese).
Tao, W. K., Chen, J. P., Li, Z. Q., Wang, C., and Zhang, C. D.: Impact of aerosols on convective clouds and precipitation, Rev. Geophys., 50, RG2001, https://doi.org/10.1029/2011rg000369, 2012.
Thomas, R. J., Krehbiel, P. R., Rison, W., Hamlin, T., Harlin, J., and Shown, D.: Observations of VHF source powers radiated by lightning, Geophys. Res. Lett., 28, 143–146, https://doi.org/10.1029/2000GL011464, 2001.
Thornton, J. A., Virts, K. S., Holzworth, R. H., and Mitchell, T. P.: Lightning enhancement over major oceanic shipping lanes, Geophys. Res. Lett., 44, 9102–9111, https://doi.org/10.1002/2017GL074982, 2017.
Twomey, S.: The nuclei of natural cloud formation, Part II: The supersaturation in natural clouds and the variation of cloud droplet concentration, Geofis. Pura Appl., 43, 243–249, https://doi.org/10.1007/BF01993560, 1959.
Van Den Broeke, M. S., Straka, J. M., and Rasmussen, E. N.: Polarimetric radar observations at low levels during tornado life cycles in a small sample of classic southern plains supercells, J. Appl. Meteorol. Clim., 47, 1232–1247, https://doi.org/10.1175/2007JAMC1714.1, 2008.
Wang, Q., Li, Z., Guo, J., Zhao, C., and Cribb, M.: The climate impact of aerosols on the lightning flash rate: is it detectable from long-term measurements?, Atmos. Chem. Phys., 18, 12797–12816, https://doi.org/10.5194/acp-18-12797-2018, 2018.
Wang, Y., Wan, Q., Meng, W., Liao, F., Tan, H., and Zhang, R.: Long-term impacts of aerosols on precipitation and lightning over the Pearl River Delta megacity area in China, Atmos. Chem. Phys., 11, 12421–12436, https://doi.org/10.5194/acp-11-12421-2011, 2011.
Wang, Y., Qie, X., Wang, D., Liu, M., Su, D., Wang, Z., Liu, D., Wu, Z., Sun, Z., and Tian, Y.: Beijing Lightning Network (BLNET) and the observation on preliminary breakdown processes, Atmos. Res., 171, 121–132, https://doi.org/10.1016/j.atmosres.2015.12.012, 2016.
Westcott, N. E.: Summertime cloud-to-ground lightning activity around major Midwestern urban areas, J. Appl. Meteorol., 34, 1633–1642, https://doi.org/10.1175/1520-0450-34.7.1633, 1995.
Williams, E. R.: The tripole structure of thunderstorms, J. Geophys. Res.-Atmos., 94, 13151–13167, https://doi.org/10.1029/JD094iD11p13151, 1989.
Williams, E. R., Mushtak, V., Rosenfeld, D., Goodman, S., and Boccippio, D.: Thermodynamic conditions favorable to superlative thunderstorm updraft, mixed phase microphysics and lightning flash rate, Atmos. Res., 76, 288–306, https://doi.org/10.1016/j.atmosres.2004.11.009, 2005.
Xiong, Y., Qie, X., Zhou, Y., Yuan, T., and Zhang, T.: Regional responses of lightning activities to relative humidity of the surface, Chinese J. Geophys., 49, 311–318, https://doi.org/10.1002/cjg2.840, 2006 (in Chinese).
Yair, Y.: Charge generation and separation processes, Space Sci. Rev., 137, 119–131, https://doi.org/10.1007/s11214-008-9348-x, 2008.
Yair, Y.: Lightning hazards to human societies in a changing climate, Environ. Res. Lett., 13, 123002, https://doi.org/10.1088/1748-9326/aaea86, 2018.
Yair, Y., Lynn, B., Price, C., Kotroni, V., Lagouvardos, K., Morin, E., Mugnai, A., and Llasat, M. D. C.: Predicting the potential for lightning activity in Mediterranean storms based on the Weather Research and Forecasting (WRF) model dynamic and microphysical fields, J. Geophys. Res.-Atmos., 115, D04205, https://doi.org/10.1029/2008JD010868, 2010.
Yair, Y., Lynn, B., Yaffe, M., Ziv, B., and Shpund, J.: Observations and numerical simulations of the October 25th, 2015 super-cell thunderstorm over Central Israel, Atmos. Res., 247, 105165, https://doi.org/10.1016/j.atmosres.2020.105165, 2021.
Zhang, R., Jing, J., Tao, J., Hsu, S.-C., Wang, G., Cao, J., Lee, C. S. L., Zhu, L., Chen, Z., Zhao, Y., and Shen, Z.: Chemical characterization and source apportionment of PM2.5 in Beijing: seasonal perspective, Atmos. Chem. Phys., 13, 7053–7074, https://doi.org/10.5194/acp-13-7053-2013, 2013.
Zhang, H., Qie, X., Liu, M., Jiang, R., Lu, G., Liu, R., Liu, D., Chen, Z., Sun, Z., Li, Z., Li, J., and Ma, Z.: The charge structure in a thunderstorm based on three-dimensional electric field sonde, Chinese J. Geophys., 64, 1155–1166, https://doi.org/10.6038/cjg2021O0187, 2021 (in Chinese).
Zhao, P., Yin, Y., and Xiao, H.: The effects of aerosol on development of thunderstorm electrification: A numerical study, Atmos. Res., 153, 376–391, https://doi.org/10.1016/j.atmosres.2014.09.011, 2015.
Zhao, P., Li, Z., Xiao, H., Wu, F., Zheng, Y., Cribb, M. C., Jin, X., and Zhou, Y.: Distinct aerosol effects on cloud-to-ground lightning in the plateau and basin regions of Sichuan, Southwest China, Atmos. Chem. Phys., 20, 13379–13397, https://doi.org/10.5194/acp-20-13379-2020, 2020.
Ziegler, C. L.: Retrieval of thermal and microphysical variables in observed convective storms. Part 1: Model development and preliminary testing, J. Atmos. Sci., 42, 1487–1509, https://doi.org/10.1175/1520-0469(1985)042<1487:ROTAMV>2.0.CO;2, 1985.
Ziegler, C. L. and MacGorman, D. R.: Observed lightning morphology relative to modeled space charge and electric field distributions in a tornadic storm, J. Atmos. Sci., 51, 833–851, https://doi.org/10.1175/1520-0469(1994)051<0833:OLMRTM>2.0.CO;2, 1994.
Ziegler, C. L., MacGorman, D. R., Dye, J. E., and Ray, P. S.: A model evaluation of noninductive graupel‐ice charging in the early electrification of a mountain thunderstorm, J. Geophys. Res.-Atmos., 96, 12833–12855, https://doi.org/10.1029/91JD01246, 1991.
Zrnic, D. S., Balakrishnan, N., Ziegler, C. L., Bringi, V. N., Aydin, K., and Matejka, T.: Polarimetric signatures in the stratiform region of a mesoscale convective system, J. Appl. Meteorol. Climatol., 32, 678–693, https://doi.org/10.1175/1520-0450(1993)032<0678:PSITSR>2.0.CO;2, 1993.