the Creative Commons Attribution 4.0 License.
the Creative Commons Attribution 4.0 License.
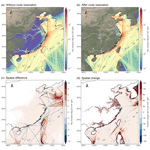
Ship emissions around China under gradually promoted control policies from 2016 to 2019
Xiaotong Wang
Wen Yi
Zhaofeng Lv
Fanyuan Deng
Songxin Zheng
Hailian Xu
Junchao Zhao
Kebin He
Ship emissions and coastal air pollution around China are expected to be alleviated with the gradual implementation of ship domestic emission control area (DECA) policies. However, a comprehensive post-assessment on the policy's effectiveness is still lacking. This study developed a series of high-spatiotemporal ship emission inventories around China from 2016 to 2019 based on an updated Ship Emission Inventory Model (SEIM v2.0) and analyzed the interannual changes in emissions under the influence of both ship activity increases and gradually promoted policies. In this model, NOx, SO2, PM and HC emissions from ships in China's inland rivers and the 200 Nm (nautical miles) coastal zone were estimated every day with a spatial resolution of based on a combination of automatic identification system (AIS) data and the Ship Technical Specifications Database (STSD). The route restoration technology and classification of ocean-going vessels (OGVs), coastal vessels (CVs) and river vessels (RVs) has greatly improved our model in the spatial distribution of ship emissions. From 2016 to 2019, SO2 and PM emissions from ships decreased by 29.6 % and 26.4 %, respectively, while ship NOx emissions increased by 13.0 %. Although the DECA 1.0 policy was implemented in 2017, it was not until 2019 when DECA 2.0 came into effect that a significant emission reduction was achieved, e.g., a year-on-year decrease of 33.3 %, regarding SO2. Considering the potential emissions brought by the continuous growth of maritime trade, however, an even larger SO2 emission reduction effect of 39.8 % was achieved in these 4 years compared with the scenario without switching to cleaner fuel. Containers and bulk carriers are still the dominant contributors to ship emissions, and newly built, large ships and ships using clean fuel oil account for an increasingly large proportion of emission structures. A total of 4 years of consecutive daily ship emissions were presented for major ports, which reflects the influence of the step-by-step DECA policy on emissions in a timely manner and may provide useful references for port observation experiments and local policy making. In addition, the spatial distribution shows that a number of ships detoured outside the scope of DECA 2.0 in 2019, perhaps to save costs on more expensive low-sulfur oil, which would increase emissions in farther maritime areas. The multiyear ship emission inventory provides high-quality datasets for air-quality and dispersion modeling, as well as verifications for in situ observation experiments, which may also guide further ship emission control directions in China.
- Article
(8391 KB) -
Supplement
(2754 KB) - BibTeX
- EndNote
Shipping is a significant anthropogenic source of air pollutants and greenhouse gases and has come into the view of scientists and the public since the end of the last century (Corbett and Fischbeck, 1997; Capaldo et al., 1999; Lawrence and Crutzen, 1999). Air pollutants emitted from ships can be further transported to inland areas by the onshore flow, along with atmospheric chemical transformations, aggravating air pollution and endangering human health (Endresen et al., 2003; Eyring et al., 2007, 2010; Corbett et al., 2007). In recent decades, despite the improvement of global fuel quality and engine posttreatment technology, shipping emissions have continued to increase due to ever-growing maritime trade (IMO, 2020; UNCTAD, 2019). Recent studies showed that global shipping emissions constituted 3 % of anthropogenic CO2 emissions in 2017 (IMO, 2020) and much more proportions of reactive gases, e.g., 20 % of NOx and 12 % of SO2 emissions (McDuffie et al., 2020). China, as the world's largest maritime trading country and sitting on seven of the world's top 10 ports with even more densely distributed coastal ports, is meeting an even tougher challenge due to its lagging emission control measurements compared to European and American countries (Mao and Rutherford, 2018b).
In recent years, numerous researchers have attempted to quantify ship emissions in China and evaluate their air-quality impacts. These studies suggest that ship emissions of SO2 in China are nearly 5 times those from road transportation (Chen et al., 2017a), and emissions within 12 nautical miles (Nm) account for ∼40 % of the total emissions from all ship emissions in coastal areas (Zhang et al., 2017; Li et al., 2018). The influence of coastal ships on the annual average PM2.5 concentration (>0.1 µg m−3) can reach as far as 960 km inland in China (Lv et al., 2018). Exhaust emissions from ships have contributed significantly to air pollution in major port clusters, e.g., the Bohai Rim Area (BRA), the Yangtze River Delta (YRD) and the Pearl River Delta (PRD) regions, and the maximum increase in annual PM2.5 concentrations has reached ∼ 2–5 µg m−3, with the greatest impact on the YRD region (Chen et al., 2018; Liu et al., 2018; Lv et al., 2018; Feng et al., 2019). During ship plume-influenced periods, ships can even contribute to over 20 % of the total PM2.5 concentrations in port centers, e.g., Shanghai Port and Qingdao Port (Fan et al., 2016; Chen et al., 2017b). The adverse impact of ship emissions also places an enormous burden on human health, causing ∼ 14 500–37 500 premature deaths in East Asia and hundreds of those in the PRD of China (Liu et al., 2016; Chen et al., 2019).
These previous evaluations have made great efforts to support the formulation of China's domestic emission control area (DECA) initially designed for BRA, YRD and PRD and later expanded to the entire water area of 12 Nm from the baseline of mainland China. Ships entering the DECA are required to switch to clean fuel oil with a lower sulfur content. However, these assessments are mostly so-called “prior assessments”, namely, evaluations of the cost and benefits of environmental and health improvement by assuming control scenarios based on earlier ship activities before implementing the policy. With the increased shipping demand and the step-by-step implementation of control measures, “post evaluation” is of equal importance to assess whether the policies are effective and to provide powerful foundations for in situ observation experiments (Wu et al., 2021). Although a number of studies have demonstrated air-quality benefits due to ships switching to low-sulfur oil in local port areas (Y. Zhang et al., 2019a; X. Zhang et al., 2019; Zou et al., 2020; Zhang et al., 2018), there is so far a lack of a comprehensive national-scale evaluation that reflects the benefits of gradually promoted DECA policy, which is vital to guide further ship emission control direction in China.
With the advent of the big data era, the characterization of ship emissions has evolved from the earlier “top-down” estimation based on global fuel consumption (Corbett et al., 1999; Endresen et al., 2003) to the “bottom-up” model based on big data from a ship's automatic identification system (AIS) (Jalkanen et al., 2009; Winther et al., 2014; Liu et al., 2016; Johansson et al., 2017; Nunes et al., 2017). AIS-based ship emission inventories have great advantages in improving the spatiotemporal resolution for numerical simulations, as well as providing possibilities for near-real-time emission estimations to meet regulatory needs (Miola and Ciuffo, 2011; Nunes et al., 2017; Huang et al., 2020). However, emission calculation methods based on big data greatly depend on the data quality, thus demanding complicated steps for data cleaning. As the loss of AIS signal occurs in many cases, dealing with long-term missing AIS signals has been one of the key technical problems for both scientific research and supervision (Y. Zhang et al., 2019b; Peng et al., 2020; Zhang et al., 2020). Without targeted measures, the estimated ship emissions would be spatially and temporally misallocated, thus further raising uncertainties in environmental impact assessments.
In this study, we developed a series of ship emission inventories (, daily) for the inland rivers and the 200 Nm coastal zone of China from 2016 to 2019 based on global AIS data and the updated version of the Ship Emission Inventory Model (SEIM v2.0). The global AIS database with ∼30 billion signals annually was combined with the Ship Technical Specifications Database (STSD) covering over 350 000 individual vessels, creating the fundamental data for emission calculation. The technical details of upgrading the previous SEIM v1.0 to SEIM v2.0 are introduced in the Methods section. Based on the multiyear ship inventory data, the 4-year consecutive daily ship emissions and emission structure were analyzed from the national to port level to track variations at a fine timescale. The interannual spatial changes in emissions from ocean-going vessels (OGVs), coastal vessels (CVs) and river vessels (RVs) were presented and compared. In addition, a scenario without the DECA policy was performed in order to evaluate the effect of China's gradually implemented DECA policy, considering the actual change in interannual ship activities. The results of this study provide high-quality emission inventory data for the further numerical simulation of air quality and health benefits of ship emission reduction.
2.1 Ship Emission Inventory Model (SEIM v2.0)
The SEIM v1.0 model was established in our previous work to develop a multiscale ship emission inventory with a high spatial and temporal resolution, which is driven by high-frequency ship AIS data (Liu et al., 2016, 2018; Fu et al., 2017). In this model, emissions were calculated based on the instantaneous operating status and power changes for each individual ship between two successive AIS signals, usually ranging from a few seconds to a few minutes. Each active ship in the AIS data was dynamically matched with its technical profiles for identification and emission calculation. With a high-frequency AIS signal transmission time and geographic locations, the emissions could ultimately be aggregated by taking those from all ships at all time intervals in the entire year, resulting in an inventory with a high temporal and spatial resolution. Technical details including the data collection and cleaning, calculation formula, emission factor (EF) adoption, and default parameter setting of the SEIM model were introduced in our previous studies (Liu et al., 2016; Fu et al., 2017). The general calculation formula of the SEIM model is summarized in Sect. S1. Currently, the SEIM considers ship emissions for both air pollutants (e.g., SO2, PM, NOx, CO and HC) and greenhouse gases (e.g., CO2, CH4 and N2O) from the main engines, auxiliary engines and boilers.
To reduce the uncertainties in emission calculations, we have previously introduced several techniques in SEIM v1.0 (Liu et al., 2016): (1) a double-nested research domain was applied to reduce the boundary effects (i.e., sharp increase/decrease on the boundary when calculating the emission inventory in a defined region); (2) the gradient boosting regression tree (GBRT) method was adopted to predict missing values of the ship properties; (3) the propeller law was used to calculate the instantaneous engine loads; and (4) the 10 min linear interval interpolation method was used to fill long-distance AIS signal gaps. These factors all contributed to improving the reliability of the ship emission inventories. Here, we introduce an upgraded version of SEIM (SEIM v2.0). The major improvements include (1) developing a route restoration module to restore the most likely trajectory for missing AIS signals, (2) distinguishing river vessels from AIS data based on spatial frequency distribution of ship trajectories, and (3) incorporating a step-by-step Chinese emission control policy with a daily scale to reflect the actual emission level in a timely manner. These improvements contributed to the consistency of the model in the real world and, to some extent, alleviated the uncertainties in our model. However, several uncertainties inevitably still exist in this model, including AIS data gaps and anomalies (influenced by methodological conditions, equipment maintenance, etc.), accuracy and coverage of STSD information, accuracy of RV, CV and OGV classification, route restoration algorithm, obedience of ships to DECA policy, etc.
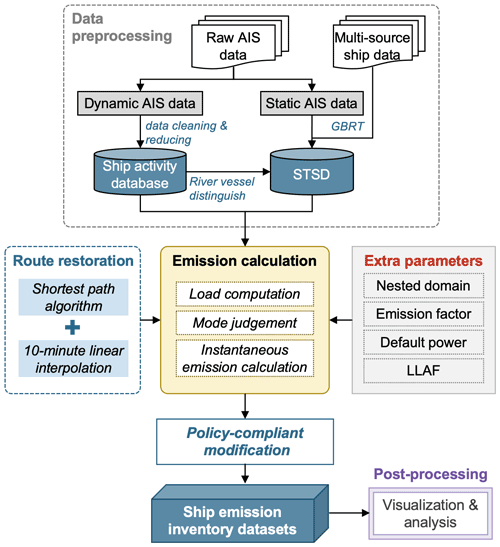
Figure 1Structure and flow chart of the SEIM v2.0. The STSD stands for the ship technical specification database. The GBRT stands for the gradient boosting regression tree. The LLAF stands for the low load adjustment factor.
Figure 1 shows the flow chart of SEIM v2.0, composed of several key modules: data pre-processing, route restoration, emission calculation, policy-compliant modification and post-processing. First, the originally collected raw AIS data and ship profile data from multiple sources are combined to form a ship activity database and STSD, and the RVs are identified based on the ship trajectories. Second, a route restoration module is applied for cross-land trajectory with a long distance in the AIS data, in which the 10 min linear interpolation will be applied on the shorted paths instead. Third, the instantaneous emission along with the movement of the ship's trajectory will be calculated based on the ship's static technical parameters, dynamic load changes, and extra parameters and factors. Then, the policy-compliant modification will be applied for vessels entering the DECAs to switch to low-sulfur fuels (LSFs). Finally, ship emission inventory datasets will be established and used for visualization and analyses from multiple perspectives. As most of the technical methods have been described in our previous work, such as GBRT methods, emission calculation algorithms and extra parameter preparations, we focus on the study area definition, the latest data evaluations and the improvements in SEIM v2.0 to introduce the technical details for developing ship emission inventories around China.
2.2 Study area
Ships have a strong spatial mobility, unlike on-road mobile sources, which mostly have a fixed geographical range of activities. Due to the complexity brought by the inconsistency of a ship's flag state, operating country and activity location, it is difficult to determine the attribution country of ship emissions. In this study, the target area for developing a ship emission inventory is navigable inland rivers and the coastal waters approximately within 200 Nm away from the Chinese mainland's territorial sea baseline (hereinafter referred to as the 200 Nm zone), as shown in Fig. 2. We defined the target area for the following reasons. First, the 200 Nm zone is the water region with the most intensive ship traffic and complex routes. Ship emissions occurring in this region have been proven to have a significant impact on the air pollution and human health in China (Lv et al., 2018). Second, as the current DECA is limited to 12 Nm to the baseline of the territorial sea, which is far less than the proposed area of the international emission control area (ECA; 200 Nm), it is possible to provide a scientific reference by investigating the emission variation in the 200 Nm zone for China's future policy design. In addition, the study area is also generally consistent with the research scope of other AIS-based ship emission inventories of China for comparison with other studies' results.
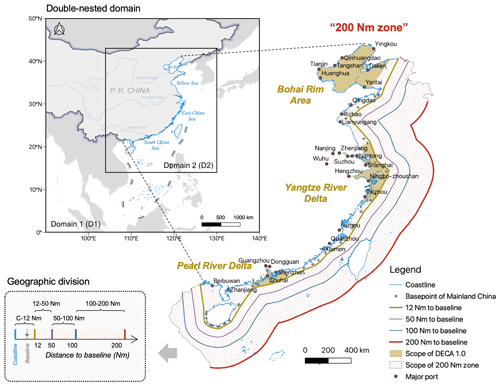
Figure 2Definition of the study area for ship emission estimation around China. The double-nested domain is used to filter global AIS data and reduce the boundary effect. The distances on the map all refer to the distance from the baseline of the Chinese mainland's territorial waters. The 200 Nm zone is the coastal area approximately within 200 Nm away from the baseline, which is further divided into different geographic regions according to the distance lines.
A double-nested domain is set to calculate the ship emissions and reduce the boundary effect. As illustrated in Fig. 2, the outer domain (D1) is 0–90∘ N and 90–140∘ E, and the inner domain (D2) is 14–43∘ N and 104–130∘ E. The spatial distribution of emissions will be retained and presented with D2 as the boundary, and the statistical results for China will finally be made for the inland rivers and the 200 Nm zone. Figure 2 also shows the scope of DECA 1.0, which includes three areas, namely, BRA, YRD and PRD, and the scope of DECA 2.0, which is approximately equal to the area from the coastline to 12 Nm from the Chinese mainland's territorial sea baseline (hereinafter referred to as baseline). Meanwhile, ship emissions within different coastal areas, i.e., from the coastline to 12, 12–50, 50–100 and 100–200 Nm from the baseline, illustrated in Fig. 2, are also decomposed and compared.
2.3 Data pre-processing and evaluations
The global dynamic AIS data for the entire years of 2016–2019 (from 1 January to 31 December) with on average 30 billion signals per year, integrating both satellite-based signals and terrestrial-based signals, were collected to build a ship activity database. This database provides high-frequency information including signal time, coordinate location, navigational speed, operating status, etc. As the AIS data are composed of satellite AIS signals and terrestrial-based AIS signals, the same messages received from multiple base stations may lead to large quantities of duplicates, especially when ships are berthing. To deal with the redundant information, only one record was kept every 10 min if the continuous AIS signals met the condition that their instantaneous speeds equalled 0 with displacements less than 0.01∘. In this way, on the premise of keeping the total operation time unchanged, the volume of the raw AIS data was reduced. After reduction, the AIS homogeneity in our study area was examined in terms of time and space (see Sect. S2 and Fig. S1 in the Supplement). Short period drops were probably the result of missing or abnormal AIS signals for many reasons, such as disruption to satellites, equipment maintenance, data transmission faults, ships sailing beyond the terrestrial station receiving range, etc. AIS signals missing or being anomalous is a common phenomenon that has been noted by previous studies (Goldworthy et al., 2019; Johansson et al., 2017; IMO, 2020). To ensure the reliability of total emissions, it is important to have data from the entire year instead of using several weeks and then scaling them to the annual total.
The STSD describes ship properties such as the vessel type, dead weight tonnage (DWT), engine power, designed speed, flag state, etc., which has also been updated to 2019. The extended STSD currently contains over 350 000 vessels, of which 101 638 are OGVs, which is consistent with the statistics of the United Nations (UNCTAD, 2019). In addition to the ship data collected from Lloyd's Register and the classification societies of various countries, we have also incorporated fishing ships and smaller ships that do not have International Maritime Organization (IMO) numbers from Global Fishing Watch (GFW) (Kroodsma et al., 2018). These ships were observed to be quite active along China's coast. A further introduction to the updated STSD is provided in the Sect. S3.
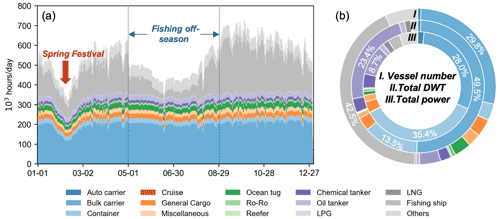
Figure 3Statistics of vessels' dynamic and static information for 2016–2019. (a) Daily average operating hours. (b) Vessel fleet compositions from different aspects.
During the emission calculation method, vessels in AIS data need to match their technical profiles in STSD. Detailed processing methods of data collection, cleaning and matching are described in our previous work (Liu et al., 2016). Table 1 shows the statistical results of the AIS messages and active ships for different years in this study. From 2016 to 2019, an annual average of approximately 90 000 vessels were observed in inland rivers and the 200 Nm zone of China, where the number of vessels showed a downward trend year by year. Figure 3 presents the statistics for dynamic activities and static technical specifications for different ships in the target region of China after matching AIS and STSD. As shown in Fig. 3a, the average daily operating time of all vessels within the study area is approximately 5.3×105 h d−1. Among all the vessel types, bulk carriers operate for an especially long time, followed by fishing ships and containers. Most vessels show constant daily operating hours but a slight decrease in the Spring Festival. However, fishing ships drop significantly in summer due to the fishing off-season. Figure 3b shows the cargo fleet structure from the perspectives of the vessel number, total DWT and total installed power of the main engines. In terms of the vessel numbers, the fishing ship accounts for the largest proportion of 42.5 %, while general cargo also accounts for 29.8 %. For the total DWT, the proportion of bulk carriers reaches 49.5 %, and the oil tanker also occupies a considerable proportion (23.4 %). For the total power of the main engines, the proportion of containers (35.4 %) exceeds that of the bulk carrier (28.0 %), indicating a higher engine power demand per unit volume for containers. Owning to the distinct technical specifications of different ship types, the total DWT, power or navigation time, as well as emissions, would not be linear with the number of vessels of each type.
2.4 Model improvements
2.4.1 Route restoration
Even if the AIS data have a high frequency of reporting ship activities, there are sometimes long periods of signal loss due to equipment failure or a manual shutdown. This type of signal only accounts for a minority of AIS data but may lead to a large deviation in terms of the amount and distribution of ship emissions, especially in the case of long operating hours. To solve this problem, a route restoration module was developed in SEIM v2.0 to predict the most likely navigation trajectories of the lost signals and spatially reallocate ship emissions. Similar methods but with featured details have been previously experimented with by Aulinger et al. (2016) on a regional scale and Johansson et al. (2017) on a global scale. Here, we refer to their method and apply it to China with a more refined resolution.
The ship route restoration method is based on the Dijkstra algorithm (Cherkassky et al., 1996) which interpolates the lost signals evenly on the shortest shipping route connecting two endpoints, namely, the experiential routes. Thus, a comprehensive ship route network needs to be established before applying the route restoration algorithm. As the global AIS data provide massive signals of ship locations, the historical navigation trajectories for all in-service vessels are clearly visible on the map. Based on the aggregated ship traffic distribution and the geographic domain of D1 in this study, the shipping route map was drawn and split into 870 arcs connected by 656 nodes, as depicted in Fig. S2. Regarding the shipping route map as an undirected graph, by applying the Dijkstra shortest-path algorithm, the shortest route path between each node pair can be calculated, as well as the geodesic distance aggregated by all arcs. In this way, the ship route network connected with nodes and arcs was established ahead of time, and the shortest geodesic paths for all the node pairs were pre-stored as a database to improve the operation efficiency.
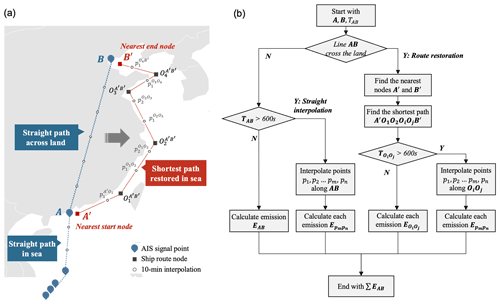
Figure 4Diagrammatic sketch of the ship route restoration algorithm. (a) Sketch map of the route restoration algorithm with an example of route AB. (b) Algorithm flow chart of the example of route AB.
Figure 4 illustrates the ship route restoration algorithm, taking a segment of the AIS positions as an example. The method can be summarized using the following steps: (1) for each two consecutive AIS points A and B, judge the geographical relationship between line AB and the continent; (2) if line AB intersects the continent and is not contained in the continent, apply the route restoration algorithm by first finding the nearest start node A′ and end node B′ by traversing the pre-stored node library; (3) look up the shortest path connecting nodes A′ and B′ (e.g., ) from the pre-stored ship route network database and calculate the average speed resulting from the geodesic distance of and time internal TAB; (4) for each segment OiOj in route , interpolate points with a time span of 600 s along the OiOj if ; (5) for each arc pmpn, calculate the ship emissions based on the average speed, instantaneous power and emission factors; and (6) calculate emissions ∑E summed from each time span along the restored route. However, as it was rather time consuming to judge the geographical relationship between the trajectory line and the continent polygon, an additional distance threshold of 50 km was finally added in the model; i.e., the restoration method would only be applied for “cross-land trajectory with a long distance”. This setting would skip some cases in which ships were sailing in the estuaries, their trajectories crossing the coastlines.
2.4.2 Classification of OGV, CV and RV
In SEIM v2.0, vessels are classified as OGVs, CVs and RVs for emission estimation. In China, the number of inland vessels with the AIS equipment installed has been increasing in recent years. As the fuel standard for RVs is more stringent than that for OGVs, it is necessary to distinguish them from the AIS data in order to calculate emissions accurately. In the methodology, since OGVs are mostly engaged in international trade following the management of the IMO, they are identified by both valid IMO numbers and the Maritime Mobile Service Identity (MMSI) numbers. CVs and RVs are both domestic vessels designed to operate in coastal and river areas, respectively. However, in some cases, they do cross one another's navigational waters when the inland waterway system borders the coastline (Mao and Rutherford, 2018a). Thus, we identified RVs with a frequency distribution method based on the navigation trajectories for each vessel. By defining the geographic domain of D2 in Fig. 2, vessels with more than 50 % of the AIS signals throughout the entire year occurring on inland rivers are considered as RVs (Fig. 5a). This method allows the possibilities for CVs and OGVs to sometimes travel into estuaries. Finally, vessels that are not identified as OGVs or RVs are regarded as CVs.
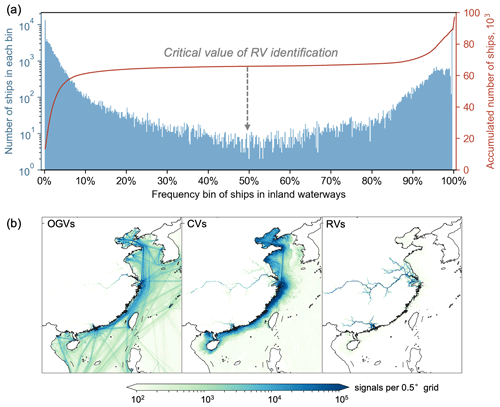
Figure 5Identification test and results of OGVs, CVs and RVs. (a) Frequency test of ships in inland waterways. (b) Spatial distribution results of AIS signals of OGVs, CVs and RVs. The sample year is 2016.
Figure 5b shows the identification results of OGVs, CVs and RVs, taking 2016 as an example. It is clear that the OGVs navigate between the major coastal ports of China and other countries, with a few entering the Yangtze River. CVs operate around the coastal seas of China, seldom contacting other countries. RVs mostly sail on the Yangtze River and Pearl River systems, with a small proportion wandering in coastal seas. The spatial distribution of the AIS signals of OGVs, CVs and RVs was essentially consistent with experience, with OGVs mainly at seas, CVs near the coast and RVs in inland waters.
2.4.3 Ship emission control policy
In recent years, a series of policy documents have been issued to control air pollution from ships, among which the most effective measure is the establishment and implementation of DECA (MOT, 2015, 2018). China's DECA policy was put into effect step by step from 2016 to 2019. Figure 6 summarizes the evolution of DECA, including the control area and fuel standards, as well as their comparison with the international ECA. Before the global sulfur cap taking effect in 2020, heavy fuel oil (HFO) with a sulfur content as high as 3.5 % had long been used in ships worldwide. In 2017, China initially established three DECAs along the coastline (DECA 1.0), covering the most busy port clusters in the world, with gradual mandates for ships to use LSF with sulfur content <0.5 % m/m (mass by mass). Later, DECA 1.0 evolved from regulating ships in core ports to the whole port clusters and ships berthing to all operating modes. In 2019, an upgraded DECA 2.0 was proposed to expand the region to cover the entire coastline (within 12 Nm from the Chinese mainland's territorial sea baseline; Fig. 2) in which ships are required to use LSF regardless of the operating status. In addition to fuel requirements, the DECA 2.0 policy also defined the control requirement of NOx emissions from ships, in which diesel engines above 130 kW built or modified on or after 1 March 2015 must meet the Tier II NOx emission limits of revised MARPOL Annex VI rules, which is in line with international ships under the control requirement of the IMO.
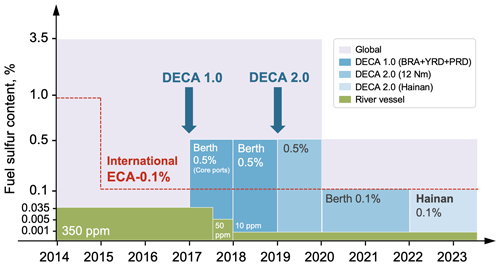
Figure 6Evolution of sulfur content requirements for fuels in DECAs and inland rivers in China. The percentages refer to the sulfur content of the fuel. The italics refer to the operating mode constrained by DECA policy. The y axis is unevenly distributed to show the standard of fuel sulfur content.
Despite the mandatory implementation time of DECA, some developed regions were encouraged to experiment in advance. To provide a timely feedback on the effect of policies, a broad investigation of the actual performance of DECA was conducted, including both coastal seas and inland rivers in 2016–2019 (Table S1 in the Supplement). Before the mandatory date of 1 January 2017, core ports in the YRD and Shenzhen port pioneered the DECA 1.0 policy 9 months and 3 months earlier, respectively. Core ports in YRD were supposed to implement the DECA 2.0 policy 3 months before it fully came into effect on 1 January 2019. Meanwhile, RVs are required to use the general diesel fuel (GDO) with a much lower sulfur content, gradually iterating from 350 to 10 ppm and finally keeping pace with the China V standard of on-road diesel fuel in 2018.
To be consistent with the DECA policy, a policy-compliant modification module was developed in SEIM 2.0. Firstly, each AIS signal point will be dynamically judged as to whether the vessel is located inside the scope of DECAs. Combined with the signal transmission time and the vessel's operating mode, the module will then determine whether the vessel needs to switch fuels or not. Finally, for vessels demanding fuel switching, a fuel correction factor, which is the quotient of the emission factors of the switched fuel and original fuel, will be further applied to correct the emissions. Details about the emission factors regarding different fuel types are introduced in Sect. S4. It is worth noting that, as far as we know, there has not been sufficient evidence showing that all vessels stick to DECAs or the violation rate each year. However, there have been studies indicating the effectiveness of DECAs in recent years (Liu et al., 2018; X. Zhang et al., 2019; Y. Zhang et al., 2019a; Zou et al., 2020). Not only have fuels been found to be cleaner (X. Zhang et al., 2019; Y. Zhang et al., 2019a), but air pollution caused by shipping activities has also been less significant in busy ports alongside the Chinese coast (Zou et al., 2020). Guaranteed by the authority of the Chinese government, we assume that the DECA policy should mostly be effective, but there is a lack of evidence about violations of DECAs, which would add to uncertainties in this model.
2.5 Simulation scenario setting
To comprehensively investigate the effects of gradually implemented DECA policies under the condition of a growing waterway transport demand, we designed a scenario (No-DECA scenario) in SEIM v2.0, with its details listed in Table 2. Compared to the base condition embedded with the actual DECA policy described in Sect. 2.4.3, the No-DECA scenario was designed to simulate the ship emissions around China by assuming vessels do not implement the DECA policy, namely, keep using fuels with sulfur contents at pre-DECA levels. By comparing the emission results from the base condition and the No-DECA scenario, the absolute emission reduction effect of gradually implemented DECA policies could be vividly illustrated.
3.1 Overall
With the development of China's waterway transport, seaborne trade increased through 2016–2019. As illustrated in Fig. 7a, Chinese ports' total passenger turnover, cargo turnover and cargo throughput increased by 10.9 %, 6.8 % and 17.4 % in 2019 compared to 2016, respectively. A growing demand for water transport has stimulated ship activities and fleet loading capacity improvements, coinciding with gradually implemented DECA policies and upgraded vessel engine standards, resulting in different interannual trends in ship emissions for different pollutants. Figure 7b and c show the annual ship emissions of SO2 and NOx in China's inland waters and the 200 Nm zone from 2013 to 2019. Before the enforcement of DECA policy, ship emissions of SO2, NOx, PM and HC in 2016 were estimated to be 1.8×106, 2.5×106, 2.3×105 and 1.1×105 Mg yr−1, respectively. The emission results are generally higher than other AIS-based ship emission inventories of China in recent years (Table S2 in the Supplement) (Chen et al., 2017a; Li et al., 2018; Fu et al., 2017; Huang et al., 2019). The primary reason might be that our study established a larger ship activity database based on global AIS data (∼30 billion signals per year) and that the incorporation of the GFW database also improved the recognition of ships, especially CVs and RVs in China. In addition, the annual increase in ship activity driven by maritime trade could also contribute to ship emission growth.
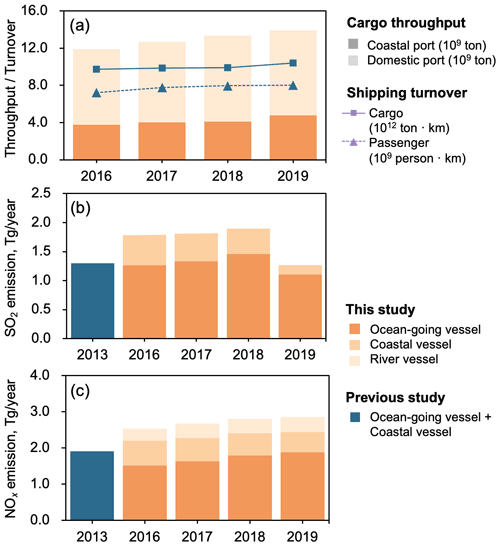
Figure 7Annual changes in (a) seaborne trade and ship emissions of (b) SO2 and (c) NOx from 2016 to 2019. Data in (a) are collected from the Chinese Statistical Yearbook (NBS, 2020). Emissions in 2013 are derived from our previous work for comparison (Fu et al., 2017).
Among all vessels, OGVs composed the largest part of ship emissions, with a proportion of 70.4 % regarding SO2 and 59.7 % regarding NOx in 2016. Compared to a recent estimation of global ship emissions (IMO, 2020), it is striking that OGVs in the 200 Nm zone of China contributed to ∼ 9.7–14.3 % of global OGV emissions (Table S3 in the Supplement) despite only being <1 % of the world's sea area. Such a result suggests a substantially high emission intensity around China generated from the activities of the global fleet. CVs are ranked after OGVs with a 29.4 % contribution to SO2 emissions and 27.1 % to NOx emissions, while the RV composition was relatively small, accounting for 13.2 % for NOx and <1 % for SO2. The emission shares of RVs may differ from those by Li et al. (2018), considering two major reasons. On one hand, we identified RVs based on the spatial frequency distribution of ship trajectories, which allows vessels to sometimes operate in coastal waters. Given that CVs and even OGVs sometimes sail in inland waters, it is possible that some CVs and OGVs are mistakenly identified as RVs. Thus, the identified vessels of RVs might be higher than that in Li et al. (2018). On the other hand, since we applied GDOs with sulfur content up to the national standard to RVs, for which the emission factors of SO2 would also be much lower, the emission share of SO2 appeared to be lower than that in Li et al. (2018), but it was opposite for NOx and other pollutants.
From 2016 to 2019, ship emissions of SO2 and PM have decreased by 29.6 % and 26.4 %, respectively (Table S2). During the DECA 1.0 period, the annual ship emissions of SO2 around China increased by 1.6 % and 3.8 % year-on-year in 2017 and 2018, respectively. After the implementation of DECA 2.0, however, ship SO2 emissions in 2019 dropped significantly by 33.3 % in 2019 compared to 2018, even 2.8 % lower than those in 2013 (Fu et al., 2017), showing great benefits with the extended control area and more stringent requirements. In terms of NOx, however, emissions continuously increased year by year, with a total increase of 13.0 % from 2016 to 2019, while emissions of other pollutants also showed a gradually increasing trend (Table S2). Therefore, the ship DECA policy has a significant impact on reducing SO2 and PM emissions, but the current vessel engine emission standard only has a limited influence on controlling NOx emissions.
3.2 The 4-year consecutive daily emissions
3.2.1 Emission composition variation
On a more refined timescale, we investigated the 5 d moving average ship SO2 and NOx emissions on a daily basis for inland rivers and the 200 Nm zone of China from 2016 to 2019, as shown in Fig. 8. It is evident that ship emissions of SO2 seasonally grew in 2016–2018 until a sharp drop on 1 January 2019 due to the implementation of the stringent DECA 2.0 control policy. The maximum daily ship emission of SO2 reached 6.4×103 Mg d−1 on 22 September 2018, which was 2.9 times that of the lowest point, 2.2×103 Mg d−1 on 1 January 2019, while the daily discrepancy of ship NOx emission intensity also reached 3.0 times throughout the 4 years. The monthly variation in ship emissions for most vessel types was generally constant, except for a temporary decrease during the Spring Festival in February (Fig. 8a). However, fishing ships showed significant seasonal variations, which declined annually in the summer and returned in autumn due to the fishing ban in China. This has also been demonstrated by other studies (Chen et al., 2017a; Fu et al., 2017).
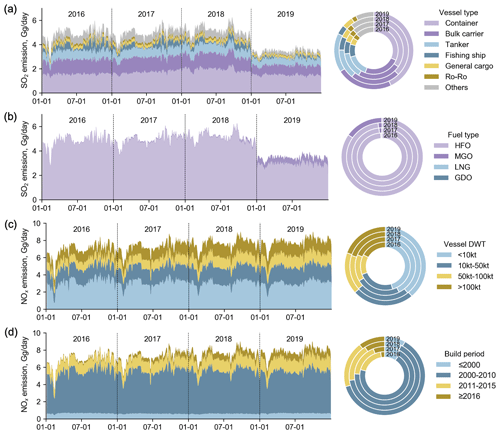
Figure 8The 5 d moving average of SO2 and NOx emissions from ships around China from 2016 to 2019. Ship SO2 emission composition by (a) vessel type and (b) fuel type and ship NOx emission composition by (c) vessel build period and (d) dead weight tonnage (DWT).
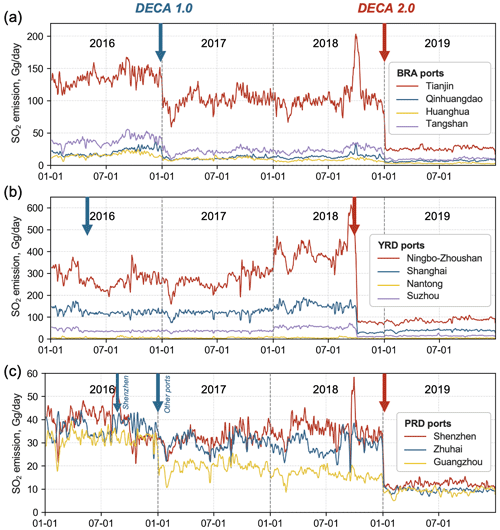
Figure 9The 5 d moving average of SO2 emissions from ships in major ports of China from 2016 to 2019: (a) Bohai Sea area (BRA), (b) Yangtze River Delta (YRD) and (c) Pearl River Delta (PRD). The blue and red arrows mark the actual implementation dates of DECA 1.0 and DECA 2.0 policies, respectively.
Figure 8 also exhibits the emission structure of SO2 according to vessel type and fuel type and NOx according to building year and DWT. The full composition of the emission contribution for all pollutants from different aspects is summarized in Table S4 in the Supplement. Containers accounted for the largest part, and the contribution increased over the 4 years, e.g., from 31.7 % in 2016 to 42.9 % in 2019 for SO2 (Fig. 8a). Although containers accounted for only about 3.5 % of the vessel numbers and 4.6 % of the operating hours in Chinese waters (Fig. 3), their relatively higher engine power contributed to significant emission intensities compared to other ships of the same size, such as bulk carriers. The HFO contributed to the majority of ship SO2 emissions due to its high content of sulfur, part of which, however, was gradually being substituted by marine gas oil (MGO) with the implementation of the DECA policy (Fig. 8b). In 2019, the MGO had accounted for 15.4 % of the ship SO2 emissions and 38.9 % of the NOx emissions (Table S4). In terms of vessel build year, ships built after 2016 made an increasing contribution to annual NOx emissions, reaching 10.6 % in 2019 (Fig. 8c). Even though the Tier II engine standard had been applied to domestic ships built after 2016, ship NOx emissions were not found to decrease as the emission standard of Tier II only has minor improvements compared to Tier I. In addition, we also found that ships with larger DWTs make up a growing proportion of vessel fleets, as well as emission contributions (Fig. 8d), indicating the developing trend of ship upsizing in the past few years. However, even though the newly built, large-scale ships and ships using clean fuel oil all play an increasingly large part in emission structure, the rising trend of NOx emissions has not yet reversed.
3.2.2 Emission variation in major ports
As the DECA policy was implemented step by step in different ports in China, we extracted the 5 d moving average ship SO2 emissions of the major ports in the BRA, YRD and PRD to track the consecutive emission changes throughout the 4 years, as shown in Fig. 9. In the initial stage, restriction on fuels with no more than a 0.5 % sulfur content was only imposed on ships at berth for core ports in these three crucial port clusters (Fig. 2 and Table S1). Before the mandatory date of DECA 1.0, core ports in the YRD and Shenzhen port pioneered the implementation 9 months and 3 months earlier, respectively, which showed a significant decrease in ship SO2 emissions beginning on 1 April and 1 October 2016, respectively. For other core ports in BRA and PRD, a noticeable decline could be observed on schedule on 1 January 2017. However, the emission of ships at berth accounted for a relatively smaller percentage (∼ 7.5 %–13.7 %) in the 200 Nm zone according to our results (Table S4); thus, the emission reduction was rather conservative inside the DECA 1.0 region in 2018 even though the requirement was extended to all ports. In contrast, due to intensified ship activities, ship SO2 emissions for some ports even largely increased, such as Ningbo-Zhoushan Port and Shenzhen Port, which increased by 19.4 % and 11.4 % in 2018 compared to 2017. Fortunately, in 2019 when the more rigorous DECA 2.0 policy was implemented, it is clearly illustrated in Fig. 9 that all ports' SO2 emissions were sharply reduced. Core ports in the YRD were supposed to implement the DECA 2.0 policy 3 months before fully coming into effect. Notably, those pilots witnessed an earlier decline in SO2 emissions, which also proved the timely and flexible response of the SEIM 2.0 model to the changeable DECA policy.
In addition to policy-driven emission changes, different ports showed distinct monthly emission variations that were highly related to their geographical location and ocean resources. For example, ship emissions in the YRD region had a low point in July as their activities were influenced by typhoons, particularly in the YRD (Weng et al., 2020), while ship emissions in Ningbo-Zhoushan Port, Tianjin Port and Shenzhen appeared to be larger in spring and autumn, probably owing to large-scale fishing ship operations (Chen et al., 2016; Yin et al., 2017). In addition, steep short-term increases in SO2 emissions were observed for the Tianjin, Ningbo Zhoushan and Shenzhen ports in September 2019. These peaks were speculated to be due to the inaccurate vessel dynamic information in AIS signals caused by the interference of adverse weather, i.e., Super Typhoon Mangkhut. However, more evidence is needed to verify the influence of extreme meteorological conditions on AIS signals. The above port-based emissions fully presented the daily ship emission variations for a long period from 2016 to 2019, which may also provide useful data references for port observation experiments.
3.3 Spatial distribution change
3.3.1 Evaluation of the route restoration
Since the shipping route restoration module was developed in SEIM v2.0 to solve the problem of AIS discontinuity, the spatial distribution of ship emissions after route restoration was evaluated, as shown in Fig. 10. Direct interpolations for AIS signals along the loxodrome would lead to part of the emissions being distributed on unrealistic routes, e.g., crossing the land areas, which could even be as long as connecting the South China Sea and the Bohai Sea (Fig. 10a). By using the route restoration method, the ship's navigation trajectory and emissions can be restored to more realistic shipping routes, thus reducing the deviation in the spatial distribution of emissions (Fig. 10b). Statistically, 15.3 % of NOx emissions and 7.5 % of SO2 emissions were spatially corrected in the study area. More improvements were obtained around Taiwan Island, the Korean Peninsula and the Philippine islands, probably due to the worse accessibility of high-quality shore-based AIS signals. The misallocation of emissions in China's land areas resulted in NOx underestimates of up to ∼ 2–4 Mg per grid downstream of the Yangtze River and Pearl River, and the misallocation of emissions in water regions is more notable on shipping routes farther from the coast. This spatial improvement of ship emissions with the route restoration method is expected to improve the results of any air-quality model applications.
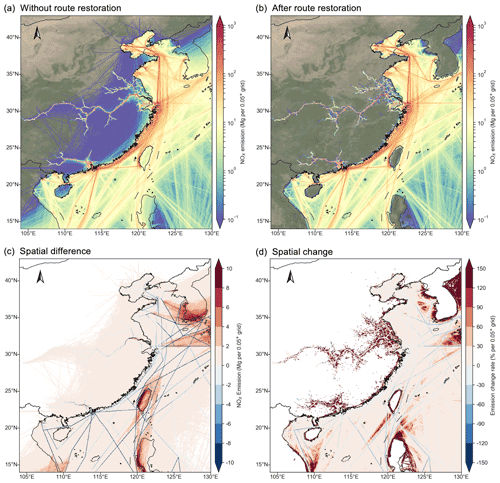
Figure 10Evaluation of estimating ship NOx emissions in China after route restoration. (a) Emissions without route restoration. (b) Emissions with route restoration. (c) Spatial difference of emissions (before−after). (d) Spatial change rate of emissions (before − after before × 100). The selected year is 2016.
3.3.2 Spatial change in ship emissions
Figure 11 presents the spatial changes in SO2 and NOx emissions from ships in different coastal regions defined in Fig. 2 from 2016 to 2019. Remarkably, within 12 Nm, which approximately equates to the scope of DECA 2.0 in 2019, SO2 emissions decreased by 78.8 % (7.2×105 Mg yr−1) compared to 2016. Despite the year-by-year growth of seaborne trade, the DECA policy effectively reduced ship-emitted SO2 overall and was especially beneficial to coastal cities. However, we discovered that SO2 emissions increased by 41.5 % (1.3×105 Mg yr−1) from 2016 to 2019 in areas between 12 and 50 Nm from the baseline, especially along the 12 Nm boundary. The proportion of ship SO2 emissions from 12 to 50 Nm rose from 17.5 % in 2016 to 35.3 % in 2019, becoming the major spatial contributor in 2019. The emission of PM exhibited a similar pattern (Fig. S3a in the Supplement). This peculiar phenomenon implies the fact that some ships possibly made a detour to evade switching to clean fuel oil, which could also be demonstrated by the larger growth rate in the cargo turnover than throughput (Fig. 6a).
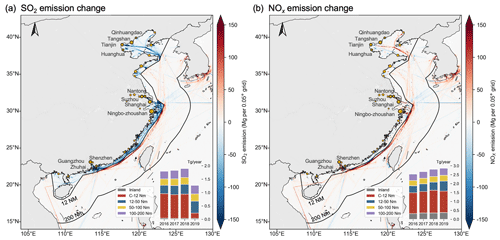
Figure 11Spatial distribution changes in SO2 and NOx emissions from ships in China in 2019 compared to 2016. The stacked bar plots indicate the annual emissions occurred at different distances off the coastline from 2016 to 2019. The “C-12 Nm” in the legend refers to the area from the coastline to 12 Nm from the baseline of the territorial sea (the same below), which is approximately equal to the scope of DECA 2.0.
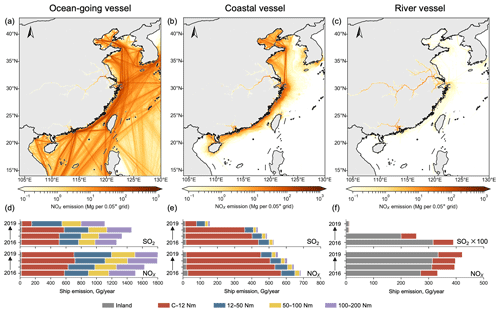
Figure 12Interannual spatial changes in NOx and SO2 emissions from ships over China from 2016 to 2019. Annual average spatial distribution comparison of NOx emission for (a) OGVs, (b) CVs and (c) RVs. Interannual variations in NOx and SO2 emissions in different geographic regions for (d) OGVs, (e) CVs and (f) RVs.
Figure 12b shows that NOx emissions from ships that occurred within 12 Nm of the baseline continuously increased from 2016 to 2018, until it declined by 5.0 % (6.4×104 Mg yr−1) in 2019 compared to the last year. Meanwhile, NOx emissions occurring in areas between 12 and 50 Nm also showed a higher annual increase rate in 2019 (21.4 %) than in the previous 2 years (∼ 7.4 %–8.2 %). Such a phenomenon once again proves the possibility of ship detours. Other species generally showed emission patterns similar to those of NOx (e.g., HC in Fig. S3b in the Supplement). In summary, the DECA 2.0 policy has a positive effect on ships' SO2 and PM emission control as a whole, especially for coastal areas. However, several ships detoured outside the scope of DECA 2.0, perhaps to save on the cost of more expensive clean fuel oil, which further elongated the sailing distance and thus increased emissions in farther maritime areas.
3.3.3 Spatial changes in OGV, CV and RV emissions
Interannual spatial changes in OGVs, CVs and RVs were further compared for the ship emissions of NOx and SO2, as shown in Fig. 12. The emission intensity of identified OGVs was apparently higher than that of CVs and RVs, demonstrating certain routes. The most intensive near-sea routes included China–Korean Peninsula, mainland-China–Taiwan, the North Pacific Route, routes from Chinese ports to the Malacca Strait and routes between busy ports of China, such as the main ports in the BRA, YRD and PRD (Fig. 12a). Since the main shipping routes are rather close to the land, OGVs within 12 Nm of the baseline make up approximately 38 % and 32 % of the total OGV emissions for NOx and SO2, respectively. From 2016 to 2019, OGV emissions generally increased in all regions, except SO2 emissions at 0–12 Nm, which showed a significant drop due to the DECA 2.0 policy.
For CVs, approximately 80 % of NOx emissions and 70 % of SO2 emissions were annually distributed mainly within 12 Nm of the baseline, and the proportions that occurred outside 12 Nm were greatly reduced compared to the OGVs. Despite intensive emission routes between coastal ports, notable emissions from CVs were more evenly distributed off the major routes (Fig. 12b), which was attributed to large quantities of fishing ships operating (Kroodsma et al., 2018). In the region of 0–12 Nm to the baseline, the annual SO2 emission reduction ratio of CVs (81.0 %) in 2019 was even higher than that of OGVs (76.9 %), indicating that CVs were more affected by the DECA 2.0 policy.
Compared to OGVs and CVs, RVs have specific routes that were constrained by inland waterways, with the most intensive emissions located on the Yangtze River and the Pearl River (Fig. 12c). Meanwhile, RVs also operate along the Chinese coast and produce a considerable proportion of emissions within 12 Nm of the baseline. With the increasingly stringent national fuel oil standards for RVs (MEE, 2018), i.e., a sulfur content from 350 ppm before 30 June 2017 to the current 10 ppm beginning on 1 January 2018, SO2 emissions from RVs had been reduced to a rather low level, both for inland rivers and coastal areas. However, other pollutants, such as NOx emissions, were still increasing. In addition, although China has required certain categories of ships to install AIS equipment since 2010, a large part of small RVs in China have not been equipped with AIS (Zhang et al., 2017). The lack of ship activity data and highly reliable local emission factors all bring uncertainties to the emission estimation of RVs. However, the air quality and human health of inland cities near waterways could be severely impacted by RV emissions (Wang et al., 2018). Therefore, RV emissions need to be stressed and are worth further investigation.
3.4 Emission reduction effect of the DECA policy
3.4.1 Monthly effect evaluation
Since the shipping activity increase and emission control policy collectively influenced ship emissions, we designed a No-DECA scenario to evaluate the real emission reduction effect of DECA policy. Figure 13 illustrates the monthly ship emissions of SO2 for the base (real) condition and the No-DECA scenario, which are aggregated from inland rivers and the 200 Nm zone of China. Without the DECA policy, ship emissions of SO2 were estimated to increase from 1.8×106 Mg yr−1 in 2016 to 2.1×106 Mg yr−1 in 2019, with an annual increase rate of 4.5 %. Beginning in April 2016, the prior implementation of DECA 1.0 led by core ports of the YRD began to see the emission reduction benefit. Since DECA 1.0, ship SO2 emissions were reduced by 4.6×104, 1.1×105 and 1.4×105 Mg yr−1 in 2016, 2017 and 2018, respectively, compared with the No-DECA scenario. Emissions were reduced even more remarkably in 2019 owning to the expansion of DECA 2.0, with an 8.4×105 Mg SO2 reduction compared to the No-DECA scenario. In retrospect, although ship SO2 emissions were reduced by 29.6 % in 2019 compared to 2016 under base condition, the DECA policy actually achieved a larger benefit with a reduction of 39.8 % compared to the same year considering the actual seaborne trade growth and ship activity increase.
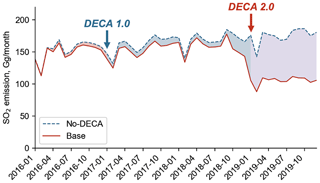
Figure 13Monthly variation in ship SO2 emissions in inland rivers and the 200 Nm zone of China under the base condition and the No-DECA scenario in 2016–2019. The base condition refer to the real condition. The No-DECA scenario reflects the emissions based on the real ship activities without DECA policies.
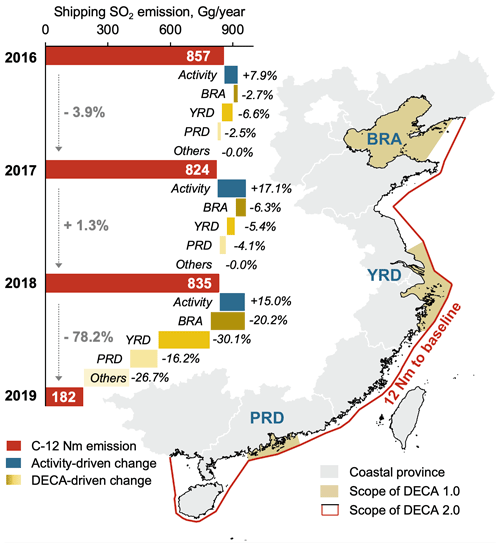
Figure 14Regional contributions to annual reduction in SO2 emissions from ships within 12 Nm of the baseline of China's territorial sea. The figures inside the blue bars refer to the annual emissions. The percentages refer to the relative change in emissions due to total ship activity change in the C-12 Nm region or the DECA policies in each region.
3.4.2 Annual regional contribution
To date, the implementation of the DECA policy and the effect of ship emission reduction have been focused within 12 Nm of the baseline of China's territorial sea. To further investigate the regional contribution of emission changes in different regions, we finally summarized the ship activity and emissions in the BRA, YRD and PRD from 2016 to 2019. As shown in Fig. 14, although the annual change in SO2 emissions in 2017 and 2018 was not significant, i.e., it decreased by 3.9 % and increased by 1.3 %, respectively, during the implementation of DECA 1.0, it is undeniable that the policy indeed effectively reduced emissions as the growth of ship activities would lead to 7.9 % and 17.1 % increases in emissions without the DECA 1.0 policy. Moreover, the YRD and BRA played a leading role in reducing ship SO2 emissions in 2017 and 2018, respectively. However, the further tightened DECA 2.0 policy implemented in 2019 more effectively reduced SO2 emissions by 78.2 %, in which the YRD, BRA and PRD contributed 30.1 %, 20.2 % and 16.2 %, respectively, while other waters contributed the remaining 26.7 %. Therefore, even though the controlling area of DECA 2.0 was enlarged to 2.5 times that of DECA 1.0, the dominant regions of emission reduction were still the three major port clusters. The primary factor driving DECA 2.0 to achieve a larger emission reduction is the fuel switching regulation for all operating statuses of ships sailing in the region rather than only limiting the berthing status in DECA 1.0.
4.1 Conclusions
The DECA policy effectively reduced SO2 and PM emissions from ships in sea areas around China from 2016 to 2019. Although the preliminary DECA 1.0 policy targeting berthing ships only had limited effects on ship-emitted SO2 and PM, the DECA 2.0 policy, tightening its limitation by putting ships in all operating statuses under its control and expanding the control areas from major ports to 12 Nm from the Chinese mainland's territorial sea baseline, resulted in a significant emission reduction. As a result, SO2 and PM emissions from ships decreased by 29.6 % and 26.4 %, respectively, in the 200 Nm zone of China in 2019 compared to 2016. Considering the potential emissions brought about by the continuous growth of maritime trade, a more substantial benefit was even achieved, e.g., an SO2 emission reduction of 39.8 % in 2019 compared with the scenario without any emission control policy. However, NOx emissions from ships increased by 13.0 % throughout the 4 years, indicating the limited effect of the current control standard.
Based on a 4-year consecutive daily emission analysis, it is noticeable that the ship emission structure had been gradually changing, i.e., newly built, large ships and ships using clean fuel oil were making up an increasingly large proportion in the emission structure. Containers and bulk carriers were still the dominant vessel type in ship emission composition. On a local scale, ship emissions in various ports exhibited different patterns in terms of daily variation. For example, ports in the YRD were likely to encounter typhoons in July, and fishing ships were particularly abundant in the BRA. Relevant findings may help provide useful data references for port observation experiments and local policy making.
The interannual spatial change in ship emissions also showed particular characteristics. By contrasting ship emissions within different distances from the Chinese coastal baseline, we discovered that in 2019, a number of ships detoured outside the scope of DECA 2.0. However, this elongated the sailing distance and resulted in more air pollutant emissions. This reminds us to pay attention to additional environmental effects brought by detoring ships during the continuous implementation of the DECA 2.0 policy. In addition, the route restoration method developed in SEIM v2.0 effectively restored the ship's navigation trajectory and emissions to more realistic shipping routes, thus reducing the deviation of the spatial distribution of emissions which could be expected to reduce uncertainties in the air-quality model.
4.2 Policy implications
Compared to the increasingly strict emission control policies of land-based sources and improving the air quality in China, policies and regulations for the prevention and control of ship emissions could be more urgent to facilitate China's air quality to achieve the annual PM2.5 concentration standard of the World Health Organization (WHO) Air Quality Guidelines (Wang et al., 2020; Q. Zhang et al., 2019). Although the current emission policy has achieved a significant control effect on SO2 and PM emissions, under the global low-sulfur oil demand, China still needs to further apply for the international ECA to enlarge the control area and strengthen the requirements for fuel quality. To make a comprehensive evaluation of and in-depth improvement to the policy, attention is also needed during the design process of the ECA scheme, such as the corresponding impact of ship detours and further expansion of DECA 2.0 so as to enlarge the reduction effects within the 200 Nm zone. Meanwhile, international cooperation is also urgently needed to jointly control ship emissions due to ships' strong spatial mobility and the intricate relations between the state of registration, the ship owner and the actual operator. With the gradual cleaning of marine fuel and the obsolescence of HFO, ship emissions of SO2 and PM will be effectively mitigated in the near future. However, ship NOx emissions are still expected to increase until the gradual elimination of old ships and the iteration of the more stringent Tier III standard for newly built ships. Other related factors, such as the engine type, NOx post-treatment technology, etc., should be taken into consideration in the future. For local decision makers, it is also important to clarify the local ship emission structure and meteorological conditions to conduct effective measures.
Python codes used during the current study are available from the corresponding author on reasonable request.
The AIS data and STSD are not available to third parties and are used under license for the current study.
The supplement related to this article is available online at: https://doi.org/10.5194/acp-21-13835-2021-supplement.
XW and WY contributed equally. XW extended the SEIM model and did the model runs. WY did the data analysis. XW and WY are responsible for writing the manuscript and preparing the figures and tables presented in this paper. ZL and FD provided valuable ideas on data analysis for this research. SZ and HX helped collect and clean the ship data. JZ assisted in the model development work. HL and KH provided guidance on the research and revised the paper. All authors contributed to the discussion and revision.
The authors declare that they have no conflict of interest.
Publisher's note: Copernicus Publications remains neutral with regard to jurisdictional claims in published maps and institutional affiliations.
This work is supported by the National Natural Science Foundation of China (grant nos. 42061130213 and 41822505). Huan Liu is supported by the Royal Society of the UK through the Newton Advanced Fellowship (NAF\R1\201166).
This research has been supported by the National Natural Science Foundation of China (grant nos. 42061130213 and 41822505). Huan Liu is supported by the Royal Society of the UK through the Newton Advanced Fellowship (grant no. NAF\R1\201166).
This paper was edited by James Allan and reviewed by two anonymous referees.
Aulinger, A., Matthias, V., Zeretzke, M., Bieser, J., Quante, M., and Backes, A.: The impact of shipping emissions on air pollution in the greater North Sea region – Part 1: Current emissions and concentrations, Atmos. Chem. Phys., 16, 739–758, https://doi.org/10.5194/acp-16-739-2016, 2016.
Capaldo, K., Corbett, J. J., Kasibhatla, P., Fischbeck, P., and Pandis, S. N.: Effects of ship emissions on sulphur cycling and radiative climate forcing over the ocean, Nature, 400, 743–746, https://doi.org/10.1038/23438, 1999.
Chen, C., Saikawa, E., Comer, B., Mao, X., and Rutherford, D.: Ship Emission Impacts on Air Quality and Human Health in the Pearl River Delta (PRD) Region, China, in 2015, With Projections to 2030, GeoHealth, 3, 284–306, https://doi.org/10.1029/2019GH000183, 2019.
Chen, D., Zhao, Y., Nelson, P., Li, Y., Wang, X., Zhou, Y., Lang, J., and Guo, X.: Estimating ship emissions based on AIS data for port of Tianjin, China, Atmos. Environ., 145, 10–18, https://doi.org/10.1016/j.atmosenv.2016.08.086, 2016.
Chen, D., Wang, X., Li, Y., Lang, J., and Zhao, Y.: High-spatiotemporal-resolution ship emission inventory of China based on AIS data in 2014, Sci. Total Environ., 609, 776–787, 2017a.
Chen, D., Wang, X., Nelson, P., Li, Y., Zhao, N., Zhao, Y., Lang, J., Zhou, Y., and Guo, X.: Ship emission inventory and its impact on the PM2.5 air pollution in Qingdao Port, North China, Atmos. Environ., 166, 351–361, https://doi.org/10.1016/j.atmosenv.2017.07.021, 2017b.
Chen, D., Zhao, N., Lang, J., Zhou, Y., Wang, X., Li, Y., Zhao, Y., and Guo, X.: Contribution of ship emissions to the concentration of PM2.5: A comprehensive study using AIS data and WRFChem model in Bohai Rim Region, China, Sci. Total Environ., 610–611, 1476–1486, https://doi.org/10.1016/j.scitotenv.2017.07.255, 2018.
Cherkassky, B. V., Goldberg, A. V., and Radzik, T.: Shortest paths algorithms: Theory and experimental evaluation, Math. Program., 73, 129–174, https://doi.org/10.1007/BF02592101, 1996.
Corbett, J. J. and Fischbeck, P.: Emissions from Ships, Science, 278, 823–824, 1997.
Corbett, J. J., Fischbeck, P. S., and Pandis, S. N.: Global nitrogen and sulfur inventories for oceangoing ships, J. Geophys. Res.-Atmos., 104, 3457–3470, https://doi.org/10.1029/1998JD100040, 1999.
Corbett, J. J., Winebrake, J. J., Green, E. H., Kasibhatla, P., Eyring, V., and Lauer, A.: Mortality from ship emissions: a global assessment, Environ. Sci. Technol., 41, 8512–8518, 2007.
Endresen, Ø., Sørgård, E., Sundet, J. K., Dalsøren, S. B., Isaksen, I. S. A., Berglen, T. F., and Gravir, G.: Emission from international sea transportation and environmental impact, J. Geophys. Res.-Atmos., 108, 4560, https://doi.org/10.1029/2002JD002898, 2003.
Eyring, V., Stevenson, D. S., Lauer, A., Dentener, F. J., Butler, T., Collins, W. J., Ellingsen, K., Gauss, M., Hauglustaine, D. A., Isaksen, I. S. A., Lawrence, M. G., Richter, A., Rodriguez, J. M., Sanderson, M., Strahan, S. E., Sudo, K., Szopa, S., van Noije, T. P. C., and Wild, O.: Multi-model simulations of the impact of international shipping on Atmospheric Chemistry and Climate in 2000 and 2030, Atmos. Chem. Phys., 7, 757–780, https://doi.org/10.5194/acp-7-757-2007, 2007.
Eyring, V., Isaksen, I. S. A., Berntsen, T., Collins, W. J., Corbett, J. J., Endresen, O., Grainger, R. G., Moldanova, J., Schlager, H., and Stevenson, D. S.: Transport impacts on atmosphere and climate: Shipping, Atmos. Environ., 44, 4735–4771, 2010.
Fan, Q., Zhang, Y., Ma, W., Ma, H., Feng, J., Yu, Q., Yang, X., Ng, S. K., Fu, Q., and Chen, L.: Spatial and Seasonal Dynamics of Ship Emissions over the Yangtze River Delta and East China Sea and Their Potential Environmental Influence, Environ. Sci. Technol., 50, 1322–1329, https://doi.org/10.1021/acs.est.5b03965, 2016.
Feng, J., Zhang, Y., Li, S., Mao, J., Patton, A. P., Zhou, Y., Ma, W., Liu, C., Kan, H., Huang, C., An, J., Li, L., Shen, Y., Fu, Q., Wang, X., Liu, J., Wang, S., Ding, D., Cheng, J., Ge, W., Zhu, H., and Walker, K.: The influence of spatiality on shipping emissions, air quality and potential human exposure in the Yangtze River Delta/Shanghai, China, Atmos. Chem. Phys., 19, 6167–6183, https://doi.org/10.5194/acp-19-6167-2019, 2019.
Fu, M., Liu, H., Jin, X., and He, K.: National- to port-level inventories of shipping emissions in China, Environ. Res. Lett., 12, 114024, https://doi.org/10.1088/1748-9326/aa897a, 2017.
Goldsworthy, B., Enshaei, H., and Jayasinghe, S.: Comparison of large-scale ship exhaust emissions across multiple resolutions: From annual to hourly data, Atmos. Environ., 214, 116829, https://doi.org/10.1016/j.atmosenv.2019.116829, 2019.
Huang, L., Wen, Y., Zhang, Y., Zhou, C., Zhang, F., and Yang, T.: Dynamic calculation of ship exhaust emissions based on real-time AIS data, Transport. Res. D-Tr. E., 80, 102277, https://doi.org/10.1016/j.trd.2020.102277, 2020.
Huang, Z., Wang, H., Tang, Y., Peng, D., and Ma, D.: Emission Prediction of Marine for 2030 in China, Vehicle Emission Control Center (VECC), Beijing, 60 pp., 2019.
IMO: Fourth IMO GHG Study – Final Report, CE Delft, 2020.
Jalkanen, J.-P., Brink, A., Kalli, J., Pettersson, H., Kukkonen, J., and Stipa, T.: A modelling system for the exhaust emissions of marine traffic and its application in the Baltic Sea area, Atmos. Chem. Phys., 9, 9209–9223, https://doi.org/10.5194/acp-9-9209-2009, 2009.
Johansson, L., Jalkanen, J.-P., and Kukkonen, J.: Global assessment of shipping emissions in 2015 on a high spatial and temporal resolution, Atmos. Environ., 167, 403–415, https://doi.org/10.1016/j.atmosenv.2017.08.042, 2017.
Kroodsma, D. A., Mayorga, J., Hochberg, T., Miller, N. A., Boerder, K., Ferretti, F., Wilson, A., Bergman, B., White, T. D., Block, B. A., Woods, P., Sullivan, B., Costello, C., and Worm, B.: Tracking the global footprint of fisheries, Science, 359, 904, https://doi.org/10.1126/science.aao5646, 2018.
Lawrence, M. G., and Crutzen, P. J.: Influence of NOx emissions from ships on tropospheric photochemistry and climate, Nature, 402, 167–170, https://doi.org/10.1038/46013, 1999.
Li, C., Borken-Kleefeld, J., Zheng, J., Yuan, Z., Ou, J., Li, Y., Wang, Y., and Xu, Y.: Decadal evolution of ship emissions in China from 2004 to 2013 by using an integrated AIS-based approach and projection to 2040, Atmos. Chem. Phys., 18, 6075–6093, https://doi.org/10.5194/acp-18-6075-2018, 2018.
Liu, H., Fu, M., Jin, X., Shang, Y., Shindell, D., Faluvegi, G., Shindell, C., and He, K.: Health and climate impacts of ocean-going vessels in East Asia, Nat. Clim. Change, 6, 1037–1041, https://doi.org/10.1038/nclimate3083, 2016.
Liu, H., Jin, X., Wu, L., Wang, X., Fu, M., Lv, Z., Morawska, L., Huang, F., and He, K.: The impact of marine shipping and its DECA control on air quality in the Pearl River Delta, China, Sci. Total Environ., 625, 1476–1485, 2018.
Lv, Z., Liu, H., Ying, Q., Fu, M., Meng, Z., Wang, Y., Wei, W., Gong, H., and He, K.: Impacts of shipping emissions on PM2.5 pollution in China, Atmos. Chem. Phys., 18, 15811–15824, https://doi.org/10.5194/acp-18-15811-2018, 2018.
Mao, X. and Rutherford, D.: NOX emissions from merchant vessels in coastal China: 2015 and 2030, International Council on Clean Transportation, Washington, USA, 12 pp., 2018a.
Mao, X. and Rutherford, D.: Delimitation of China's emission control zone: Potential impact of vessel diversions on emissions, International Council on Clean Transportation, Washington, USA, 33 pp., 2018b.
McDuffie, E. E., Smith, S. J., O'Rourke, P., Tibrewal, K., Venkataraman, C., Marais, E. A., Zheng, B., Crippa, M., Brauer, M., and Martin, R. V.: A global anthropogenic emission inventory of atmospheric pollutants from sector- and fuel-specific sources (1970–2017): an application of the Community Emissions Data System (CEDS), Earth Syst. Sci. Data, 12, 3413–3442, https://doi.org/10.5194/essd-12-3413-2020, 2020.
Ministry of ecological environment of the people's Republic of China (MEE): Law of the People's Republic of China on the Prevention and Control of Atmospheric Pollution, available at: http://www.mee.gov.cn/ywgz/fgbz/fl/201811/t20181113_673567.shtml (last access: 12 September 2021), 2018.
Ministry of Transport (MOT): Notice of the Ministry of Transport on printing and distributing the implementation plan of ship emission control zone in the waters of Pearl River Delta, Yangtze River Delta and Bohai Rim (Beijing Tianjin Hebei), available at: http://xxgk.mot.gov.cn/2020/jigou/haishi/202006/t20200630_3319179.html (last access: 12 September 2021), 2015.
Ministry of Transport (MOT): Notice of the Ministry of Transport on printing and distributing the implementation plan for the control area of air pollutant emission from ships, available at: http://www.mot.gov.cn/xiazaizhongxin/ziliaoxiazai/201901/P020200709632649953259.pdf (last access: 12 September 2021), 2018.
Miola, A. and Ciuffo, B.: Estimating air emissions from ships: Meta-analysis of modelling approaches and available data sources, Atmos. Environ., 45, 2242–2251, 2011.
National Bureau of Statistics of China (NBS): China Statistical Yearbook, China Statistics Press, Beijing, 2020.
Nunes, R. A. O., Alvim-Ferraz, M. C. M., Martins, F. G., and Sousa, S. I. V.: The activity-based methodology to assess ship emissions – A review, Environ. Pollut., 231, 87–103, https://doi.org/10.1016/j.envpol.2017.07.099, 2017.
Peng, X., Wen, Y., Wu, L., Xiao, C., Zhou, C., and Han, D.: A sampling method for calculating regional ship emission inventories, Transport. Res. D-Tr. E., 89, 102617, https://doi.org/10.1016/j.trd.2020.102617, 2020.
UNCTAD: Review of Maritime Transport 2019, United Nations, New York, 2019.
Wang, H., He, X., Liang, X., Choma, E. F., Liu, Y., Shan, L., Zheng, H., Zhang, S., Nielsen, C. P., Wang, S., Wu, Y., and Evans, J. S.: Health benefits of on-road transportation pollution control programs in China, P. Natl. Acad. Sci. USA, 117, 25370, https://doi.org/10.1073/pnas.1921271117, 2020.
Wang, Y., Li, C., Huang, Z., Yin, X., Ye, X., Wang, X., and Zheng, J.: Impact of ship emissions on air quality over Chinese waters in 2013, Acta Scientiae Circumstantiae, 38, 2157–2166, https://doi.org/10.13671/j.hjkxxb.2018.0139, 2018.
Weng, J., Shi, K., Gan, X., Li, G., and Huang, Z.: Ship emission estimation with high spatial-temporal resolution in the Yangtze River estuary using AIS data, J. Clean. Prod., 248, 119297, https://doi.org/10.1016/j.jclepro.2019.119297, 2020.
Winther, M., Christensen, J. H., Plejdrup, M. S., Ravn, E. S., Eriksson, Ó. F., and Kristensen, H. O.: Emission inventories for ships in the arctic based on satellite sampled AIS data, Atmos. Environ., 91, 1–14, https://doi.org/10.1016/j.atmosenv.2014.03.006, 2014.
Wu, Y., Liu, D., Wang, X., Li, S., Zhang, J., Qiu, H., Ding, S., Hu, K., Li, W., Tian, P., Liu, Q., Zhao, D., Ma, E., Chen, M., Xu, H., Ouyang, B., Chen, Y., Kong, S., Ge, X., and Liu, H.: Ambient marine shipping emissions determined by vessel operation mode along the East China Sea, Sci. Total Environ., 769, 144713, https://doi.org/10.1016/j.scitotenv.2020.144713, 2021.
Yin, P., Huang, Z., Zheng, D., Wang, X., Tian, X., Zheng, J., and Zhang, Y.: Marine vessel emission and its temporal and spatial distribution characteristics in Ningbo-Zhoushan Port, China Environmental Science, 37, 27–37, 2017.
Zhang, Q., Zheng, Y., Tong, D., Shao, M., Wang, S., Zhang, Y., Xu, X., Wang, J., He, H., Liu, W., Ding, Y., Lei, Y., Li, J., Wang, Z., Zhang, X., Wang, Y., Cheng, J., Liu, Y., Shi, Q., Yan, L., Geng, G., Hong, C., Li, M., Liu, F., Zheng, B., Cao, J., Ding, A., Gao, J., Fu, Q., Huo, J., Liu, B., Liu, Z., Yang, F., He, K., and Hao, J.: Drivers of improved PM2.5 air quality in China from 2013 to 2017, P. Natl. Acad. Sci. USA, 116, 24463, https://doi.org/10.1073/pnas.1907956116, 2019.
Zhang, X., Zhang, Y., Liu, Y., Zhao, J., Zhou, Y., Wang, X., Yang, X., Zou, Z., Zhang, C., Fu, Q., Xu, J., Gao, W., Li, N., and Chen, J.: Changes in the SO2 Level and PM2.5 Components in Shanghai Driven by Implementing the Ship Emission Control Policy, Environ. Sci. Technol., 53, 11580–11587, https://doi.org/10.1021/acs.est.9b03315, 2019.
Zhang, Y., Yang, X., Brown, R., Yang, L., Morawska, L., Ristovski, Z., Fu, Q., and Huang, C.: Shipping emissions and their impacts on air quality in China, Sci. Total Environ., 581–582, 186–198, https://doi.org/10.1016/j.scitotenv.2016.12.098, 2017.
Zhang, Y., Loh, C., Louie, P. K. K., Liu, H., and Lau, A. K. H.: The roles of scientific research and stakeholder engagement for evidence-based policy formulation on shipping emissions control in Hong Kong, J. Environ. Manage., 223, 49–56, https://doi.org/10.1016/j.jenvman.2018.06.008, 2018.
Zhang, Y., Deng, F., Man, H., Fu, M., Lv, Z., Xiao, Q., Jin, X., Liu, S., He, K., and Liu, H.: Compliance and port air quality features with respect to ship fuel switching regulation: a field observation campaign, SEISO-Bohai, Atmos. Chem. Phys., 19, 4899–4916, https://doi.org/10.5194/acp-19-4899-2019, 2019a.
Zhang, Y., Fung, J. C. H., Chan, J. W. M., and Lau, A. K. H.: The significance of incorporating unidentified vessels into AIS-based ship emission inventory, Atmos. Environ., 203, 102–113, https://doi.org/10.1016/j.atmosenv.2018.12.055, 2019b.
Zhang, Z., Huang, Z., Xu, Y., Chen, W., Huang, L., Bai, L., Huang, J., Zheng, J., and Yan, M.: Ship emissions spatial characterization improved method and application based on AIS trajectory restoration, Acta Scientiae Circumstantiae, 40, 1931–1942, 2020.
Zou, Z., Zhao, J., Zhang, C., Zhang, Y., Yang, X., Chen, J., Xu, J., Xue, R., and Zhou, B.: Effects of cleaner ship fuels on air quality and implications for future policy: A case study of Chongming Ecological Island in China, J. Clean. Prod., 267, 122088, https://doi.org/10.1016/j.jclepro.2020.122088, 2020.