the Creative Commons Attribution 4.0 License.
the Creative Commons Attribution 4.0 License.
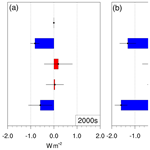
Using CESM-RESFire to understand climate–fire–ecosystem interactions and the implications for decadal climate variability
Yun Qian
Hanqin Tian
Jia Yang
Ernesto Alvarado
Large wildfires exert strong disturbance on regional and global climate systems and ecosystems by perturbing radiative forcing as well as the carbon and water balance between the atmosphere and land surface, while short- and long-term variations in fire weather, terrestrial ecosystems, and human activity modulate fire intensity and reshape fire regimes. The complex climate–fire–ecosystem interactions were not fully integrated in previous climate model studies, and the resulting effects on the projections of future climate change are not well understood. Here we use the fully interactive REgion-Specific ecosystem feedback Fire model (RESFire) that was developed in the Community Earth System Model (CESM) to investigate these interactions and their impacts on climate systems and fire activity. We designed two sets of decadal simulations using CESM-RESFire for present-day (2001–2010) and future (2051–2060) scenarios, respectively, and conducted a series of sensitivity experiments to assess the effects of individual feedback pathways among climate, fire, and ecosystems. Our implementation of RESFire, which includes online land–atmosphere coupling of fire emissions and fire-induced land cover change (LCC), reproduces the observed aerosol optical depth (AOD) from space-based Moderate Resolution Imaging Spectroradiometer (MODIS) satellite products and ground-based AErosol RObotic NETwork (AERONET) data; it agrees well with carbon budget benchmarks from previous studies. We estimate the global averaged net radiative effect of both fire aerosols and fire-induced LCC at W m−2, which is dominated by fire aerosol–cloud interactions ( W m−2), in the present-day scenario under climatological conditions of the 2000s. The fire-related net cooling effect increases by ∼170 % to W m−2 in the 2050s under the conditions of the Representative Concentration Pathway 4.5 (RCP4.5) scenario. Such considerably enhanced radiative effect is attributed to the largely increased global burned area (+19 %) and fire carbon emissions (+100 %) from the 2000s to the 2050s driven by climate change. The net ecosystem exchange (NEE) of carbon between the land and atmosphere components in the simulations increases by 33 % accordingly, implying that biomass burning is an increasing carbon source at short-term timescales in the future. High-latitude regions with prevalent peatlands would be more vulnerable to increased fire threats due to climate change, and the increase in fire aerosols could counter the projected decrease in anthropogenic aerosols due to air pollution control policies in many regions. We also evaluate two distinct feedback mechanisms that are associated with fire aerosols and fire-induced LCC, respectively. On a global scale, the first mechanism imposes positive feedbacks to fire activity through enhanced droughts with suppressed precipitation by fire aerosol–cloud interactions, while the second one manifests as negative feedbacks due to reduced fuel loads by fire consumption and post-fire tree mortality and recovery processes. These two feedback pathways with opposite effects compete at regional to global scales and increase the complexity of climate–fire–ecosystem interactions and their climatic impacts.
- Article
(19855 KB) -
Supplement
(7336 KB) - BibTeX
- EndNote
Large wildfires show profound impacts on human society and the environment, with increasing trends in many regions around the world during recent decades (Abatzoglou and Williams, 2016; Barbero et al., 2015; Clarke et al., 2013; Dennison et al., 2014; Jolly et al., 2015; Westerling et al., 2006; Yang et al., 2011, 2015). They pose a great threat to the safety of communities in the vicinity of fire-prone regions and distant downstream areas by both destructive burning and increased health risks from fire smoke exposure. The global annual average number of premature deaths due to fire smoke exposure was estimated at about 339 000 (interquartile range: 260 000–600 000) during 1997 to 2006 (Johnston et al., 2012), while the total cost of the fire-related socioeconomic burden would surge much higher if other societal and environmental outcomes, such as respiratory morbidity and cardiovascular diseases, expenditures for defensive actions and disutility, and ecosystem service damage, were taken into account (Fann et al., 2018; Hall, 2014; Richardson et al., 2012; Thomas et al., 2017). In addition to hazardous impacts on human society, fire also exerts strong disturbance on regional and global climate systems and ecosystems by perturbing the radiation budget and carbon balance between the atmosphere and land surface. In return, these short-term and long-term changes in fire weather, terrestrial ecosystems, and human activity modulate fire intensity and reshape fire regimes in many climate-change-sensitive regions. These processes were not fully included in previous climate model studies, increasing uncertainties in the projections of future climate variability and fire activity (Flannigan et al., 2009; Hantson et al., 2016; Harris et al., 2016; Liu et al., 2018). Most fire-related climate studies used a one-way perturbation approach by examining a unidirectional forcing and response between climate change and fire activity without feedback. For instance, many historical and future-projected fire responses to climate drivers were mainly based on offline statistical regression or one-way coupled prognostic fire models in earth system models, while fire feedback to weather, climate, and vegetation was neglected (e.g., Abatzoglou et al., 2019; Flannigan et al., 2013; Hurteau et al., 2014; Liu et al., 2010; Moritz et al., 2012; Parks et al., 2016; Wotton et al., 2017; Young et al., 2017; Yue et al., 2013). The neglected feedback could affect regional to global radiative forcing, biogeochemical and hydrological cycles, and ecological functioning that may in turn modulate fire activity in local and remote regions (Harris et al., 2016; Liu, 2018; Pellegrini et al., 2018; Seidl et al., 2017; Shuman et al., 2017). Similarly, climate studies (e.g., Jiang et al., 2016; Tosca et al., 2013; Ward et al., 2012) that focused on climate responses to fire forcing used the same unidirectional approach but from an opposite perspective, in which multiple fire impacts on climate systems were evaluated through fire aerosols, greenhouse gases, and land albedo effects using climate sensitivity experiments with and without prescribed fire emissions as model inputs. However, possible fire activity and emission changes in response to these fire weather and climate variations were missing in such one-way perturbation modeling approaches.
To tackle these problems, we developed the two-way coupled RESFire model (Zou et al., 2019) with online land–atmosphere coupling of fire-related mass and energy fluxes as well as fire-induced land cover change in CESM (hereafter CESM-RESFire). CESM-RESFire performs well using either offline observation- and reanalysis-based atmosphere data or an online simulated atmosphere, which is applied in this study to investigate complex climate–fire–ecosystem interactions as well as to project future climate change with fully interactive fire disturbance. In this work, we use the state-of-the-science CESM-RESFire model to evaluate major climate–fire–ecosystem interactions through biogeochemical, biogeophysical, and hydrological pathways and to assess future changes in decadal climate variability and fire activity with consideration of these interactive feedback processes. We provide a brief model description and sensitivity experiment settings in Sect. 2 and present modeling results and analyses on radiative effects, carbon balance, and feedback evaluation in Sect. 3. Final conclusions and implications follow in Sect. 4.
2.1 Fire model and sensitivity simulation experiments
RESFire (Zou et al., 2019) is a process-based fire model developed in the CESM version 1.2 modeling framework that incorporates ecoregion-specific natural and anthropogenic constraints on fire occurrence, fire spread, and fire impacts in both the CESM land component – the Community Land Model version 4.5 (CLM4.5) (Oleson et al., 2013) – and the atmosphere component – the Community Atmosphere Model version 5.3 (CAM5) (Neale et al., 2012). It is compatible with either an observation- and reanalysis-based data atmosphere or the CAM5 atmosphere model with online land–atmosphere coupling through aerosol–climate effects and fire–vegetation interactions. It includes two major fire feedback pathways: atmosphere-centric fire feedback through fire-related mass and energy fluxes; and vegetation-centric fire feedback through fire-induced land cover change. These feedback pathways correspond to two key climate variables, radiative forcing and carbon balance, through which fires exert their major climatic and ecological impacts. Other features in CLM4.5 and CAM5, such as the photosynthesis scheme (Sun et al., 2012), the three-mode modal aerosol module (MAM3; Liu et al., 2012), and the cloud microphysics (Morrison and Gettelman, 2008; Gettelman et al., 2008) and macrophysics (Park et al., 2014) schemes, allow for more comprehensive assessments of the climate effects of fires through interactions with vegetation and clouds. A simple treatment of secondary organic aerosol (SOA) is used in CAM5 to derive SOA formation from anthropogenic and biogenic volatile organic compounds (VOCs) with fixed mass fields (Table S1 in the Supplement). The total SOA mass is emitted as the SOA (gas) species from the surface, and then the condensation and evaporation of gas-phase SOA to and from different aerosol modes are calculated in the MAM3 module (Neale et al., 2012). The gas-phase photochemistry is not included in the CAM5 simulations, which precludes the possibility of evaluating chemistry–climate interactions. We also implement distribution-mapping-based online bias corrections for key fire weather variables (i.e., surface temperature, precipitation, and relative humidity) to reduce the negative influences of climate model biases in atmosphere simulation and projection. Fire plume rise is globally universal parameterized based on atmospheric boundary layer height (PBLH), fire radiative power (FRP), and Brunt–Väisälä frequency in the free troposphere (Sofiev et al., 2012). Please refer to Zou et al. (2019) for more detailed fire model descriptions and to Sofiev et al. (2012) for the fire plume rise parameterization. To quantify the impacts of fire–climate interactions under different climatic conditions, we designed two groups of sensitivity simulations for present-day and future scenarios (Table 1). In each simulation group, we conducted one control run (CTRLx, where x =1 or 2 indicates the present-day or future scenario, respectively) and two sensitivity runs (SENSxA–B, where x is the same as that in CTRL runs; the notations of A and B are explained below). The CTRL runs were designed with fully interactive fire disturbance, such as fire emissions with plume rise and fire-induced LCC with different boundary conditions for a present-day scenario (CTRL1; 2001–2010), and a moderate future emission scenario (CTRL2) of the Representative Concentration Pathway 4.5 (RCP4.5; 2051–2060), respectively. In each scenario, we turned off the atmosphere-centric feedback mechanisms (e.g., fire aerosol climate effects) in SENSxA simulations (where x =1 or 2) and then turned off both atmospheric-centric and vegetation-centric fire feedback (e.g., fire-induced LCC) in SENSxB simulations. Consequently, we estimated the atmosphere-centric impacts of fire emissions on radiative forcing in the present-day scenario (RCP4.5 future scenario) by comparing SENS1A (SENS2A) with CTRL1 (CTRL2). We also estimated the vegetation-centric impacts of fire-induced LCC on the terrestrial carbon balance in the present-day scenario (RCP4.5 future scenario) by comparing SENS1B (SENS2B) with SENS1A (SENS2A). The net fire-related effects were evaluated by comparing CTRL runs with SENSxB runs as both fire feedback mechanisms were turned off in the SENSxB runs. Using these sensitivity experiments, we are able to evaluate two-way climate–fire–ecosystem interactions under the same integrated modeling framework, which is not possible in one-way perturbation studies considering either climate impacts on fires (Kloster et al., 2010, 2012; Thonicke et al., 2010) or fire feedback to climate (Jiang et al., 2016; Li et al., 2014; Ward et al., 2012; Yue et al., 2015, 2016).
2.2 Model input data
We used the spun-up files from previous long-term runs (Zou et al., 2019) as initial conditions for the present-day experiments (CTRL1 and SENS1A–B). The boundary conditions, including the prescribed climatological (1981–2010 average) sea surface temperature and sea ice data for the present-day scenario, were obtained from the Met Office Hadley Centre (HadISST) (Rayner et al., 2003). Similarly, the nitrogen and aerosol deposition rates were also prescribed from a time-invariant spatially varying annual mean file for 2000 and a time-varying (monthly cycle) globally gridded deposition file, respectively, as the standard datasets necessary for the present-day CAM5 simulations (Hurrell et al., 2013). The climatological 3-hourly cloud-to-ground lightning data via bilinear interpolation from the NASA LIS–OTD grid product v2.2 (https://ghrc.nsstc.nasa.gov/uso/ds_docs/lis_climatology/lolrdc_dataset.html, last access: 18 January 2019), hourly lightning frequency data, and the world population density data were fixed at the 2000 levels for all the present-day simulations. The non-fire emissions from anthropogenic sources (e.g., industrial, domestic, and agriculture activity sectors) in the present-day scenario were from the emission dataset (Lamarque et al., 2010) representing the year 2000 for the Fifth Assessment Report of the Intergovernmental Panel on Climate Change (IPCC AR5). Emissions of natural aerosols such as dust and sea salt were calculated online (Neale et al., 2012), while vertically resolved volcanic sulfur and dimethyl sulfide (DMS) emissions were prescribed from the AEROCOM emission dataset (Dentener et al., 2006). Emission fluxes for the five VOC species (isoprene, monoterpenes, toluene, big alkenes, and big alkanes) to derive SOA mass yields were prescribed from the MOZART-2 dataset (Horowitz et al., 2003). For fire emissions, we replaced the prescribed GFED2 fire emissions (van der Werf et al., 2006) from the default offline emission data with online coupled fire emissions generated by the RESFire model in the CTRL runs. We then decoupled online simulated fire emissions in the SENS1A runs, in which fire emissions were not transported to the CAM5 atmosphere model, to isolate the atmosphere-centric impacts of fire–climate interactions. In both the CTRL1 and SENS1A experiments, we allowed the semi-static historical LCC data for the year 2000 from version 1 of the Land-Use History A product (LUHa.v1) (Hurtt et al., 2006) to be affected by post-fire vegetation changes (Zou et al., 2019). We then used the fixed LCC data for the year 2000 in the SENS1B run and compared two SENS1 runs (SENS1A–SENS1B) to evaluate the vegetation-centric fire impacts on terrestrial ecosystems and the carbon balance in the 2000s.
For the future scenario experiments, we replaced all the present-day datasets with the RCP4.5 projection datasets including the initial conditions and prescribed boundary conditions of global sea surface temperature and sea ice data in 2050, the cyclical non-fire emissions and deposition rates fixed in 2050 under the RCP4.5 scenario, and the annual LCC data for the RCP4.5 transient period in 2050 based on the Future Land-Use Harmonization A products (LUHa.v1_future) (Hurtt et al., 2006). All these datasets were described in the technical note of CAM5 (Neale et al., 2012) and stored on the Cheyenne computing system (CISL, 2017) at the National Center for Atmospheric Research (NCAR) Wyoming Supercomputing Center (NWSC). It is worth noting that we used the present-day demographic data and observation-based climatological lightning data in the future scenario given the pathway dependence and great uncertainties in future projections of these inputs (Clark et al., 2017; Riahi et al., 2017; Tost et al., 2007;). In other words, we did not consider the influence of fire ignition changes associated with human activity or lightning flash density in our future projection simulations but focused on the broad impacts of future climate change on fuel loads and combustibility as well as fire weather conditions.
The global mean greenhouse gas (GHG) mixing ratios in the CAM5 atmosphere model were fixed at the year 2000 levels (CO2: 367.0 ppmv; CH4: 1760.0 ppbv; N2O: 316.0 ppbv) in all present-day experiments, and they were replaced by the prescribed RCP4.5 projection datasets with the well-mixed assumption and monthly variations in the future scenarios. These GHG mixing ratios were then passed to the CLM4.5 land model in all sensitivity experiments. In return, the land model provided the diagnostics of the balance of all carbon fluxes between net ecosystem production (NEP; g C m−2 s−1, positive for carbon sink) and depletion from fire emissions, land cover change fluxes, and carbon loss from wood products pools; then the computed net CO2 flux was passed to the atmosphere model in the form of net ecosystem exchange (NEE; g C m−2 s−1). Though fire emissions could perturb the value of NEE at short-term scales, it is often assumed that fire is neither a source nor a sink for CO2 since fire carbon emissions are offset by the carbon absorption of vegetation regrowth over long-term scales (Bowman et al., 2009). Therefore, we did not consider the radiative effect of fire-related GHGs in our sensitivity experiments. These kinds of “concentration-driven” simulations with prescribed atmospheric CO2 concentrations for a given scenario have been used extensively in previous fire–climate interaction assessments (e.g., Kloster et al., 2010; Li et al., 2014; Thonicke et al., 2010) and most of the RCP simulations (Ciais et al., 2013).
2.3 Model evaluation benchmarks and datasets
Multiple observational and assimilated datasets were applied to evaluate the modeling performance regarding radiative forcing. We collected space-based column aerosol optical depth (AOD) from the level 3 MODIS Aqua monthly global product (MYD08_M3; Platnick et al., 2015) and ground-based version 3 aerosol optical thickness (AOT) level 2.0 data from the Aerosol Robotic Network (AERONET, Holben et al., 1998; https://aeronet.gsfc.nasa.gov, last access: 18 January 2019) for comparison with the model-simulated AOD data at 550 nm. The AERONET AOTs at 550 nm were interpolated by estimating Ångström exponents based on the measurements taken at the two closest wavelengths at 500 and 675 nm (see the Supplement for details). We then followed the Ghan method (Ghan, 2013) to estimate fire aerosol radiative effects (REaer) on the planetary energy balance in terms of aerosol–radiation interactions (REari), aerosol–cloud interactions (REaci), and fire-aerosol-related surface albedo change (REsac) in Eq. (1). The radiative effect related to fire-induced land cover change (RElcc) was estimated by comparing shortwave radiative fluxes at the top of the atmosphere (TOA) between the SENSxA (with fire-induced LCC) and SENSxB (without fire-induced LCC) experiments. By summing up all these terms, we estimated the fire-related net radiative effect (REfire) as the shortwave radiative flux difference between the CTRLx (with fire aerosols and fire-induced LCC) and SENSxB (without fire aerosols and fire-induced LCC) experiments.
RE of interaction of radiation with fire aerosol:
RE of interaction of clouds with fire aerosol:
RE of surface albedo change induced by fire aerosol:
Net RE of fire aerosol:
RE of fire-induced land cover change:
Net RE of fire:
Here, Δ is the difference between control and sensitivity simulations, F is the shortwave radiative flux at the TOA, Fclean is the radiative flux calculated as an additional diagnostic from the same simulations but neglecting the scattering and absorption of solar radiation by all aerosols, and Fclear,clean is the flux calculated as an additional diagnostic but neglecting scattering and absorption by both clouds and aerosols. The surface albedo effect is largely the contribution of changes in surface albedo induced by fire aerosol deposition and land cover change, which is small but non-negligible in some regions (Ghan, 2013). We used similar modeling settings, including the three-mode modal aerosol scheme (MAM3) (Liu et al., 2012) and the Snow, Ice, and Aerosol Radiative (SNICAR) module (Flanner and Zender, 2005), and compare our online coupled fire modeling results against previous offline prescribed fire modeling studies (Jiang et al., 2016; Ward et al., 2012) in the next section.
We also examined the modeling performance on burned area and the terrestrial carbon balance such as fire carbon emissions, gross primary production (GPP, g C m−2 s−1; positive for vegetation carbon uptake), net primary production (NPP, g C m−2 s−1; positive for vegetation carbon uptake), net ecosystem productivity (NEP, g C m−2 s−1; positive for net ecosystem carbon uptake), and net ecosystem exchange (NEE, g C m−2 s−1; positive for net ecosystem carbon emission). The model-simulated burned area and fire carbon emissions were evaluated against the satellite-based GFED4.1s datasets (https://www.globalfiredata.org/, last access: 18 January 2019; Giglio et al., 2013; Randerson et al., 2012; van der Werf et al., 2017), and these carbon-budget-related variables were calculated in Eqs. (2) and (3) and compared with the MODIS primary production products (Zhao et al., 2005; Zhao and Running, 2010), previous modeling results used for terrestrial model comparison projects (Piao et al., 2013) and the IPCC AR5 report (Ciais et al., 2013), and the global carbon budget assessment (Le Quéré et al., 2013) by the broad carbon cycle science community.
Ra is the total ecosystem autotrophic respiration (g C m−2 s−1), Rh is the total heterotrophic respiration (g C m−2 s−1), Cfe is the fire carbon emissions (g C m−2 s−1), and Clh is the carbon loss (g C m−2 s−1) due to land cover change, wood products, and harvest.
3.1 Evaluation of fire-related radiative effects
Figure 1 shows the comparison of the model-simulated 10-year annual average column AOD at 550 nm from CTRL1 and space-based AOD from MODIS aboard the Aqua satellite. It is noted that both sets of AOD data result from all sources including fire and non-fire emissions, and significant differences exist in specific regions due to large biases in model emission inputs and aerosol parameterization. In the MODIS AOD data, the most noticeable hotspot regions include eastern China, South Asia including India, and Africa. The first two regions are dominated mostly by anthropogenic emissions, while the last one is dominated by fire emissions. Since the non-fire emissions used in CAM5 simulations are based on the year 2000 (Lamarque et al., 2010) and low biased compared to the rapid emission increases in many Asian developing countries (Kurokawa et al., 2013), the simulated hotspot regions in East and South Asia are not as appreciable as those observed in the remote sensing data. The model results also show underestimation in rainforests over South America and central Africa, where large fractions of aerosols are contributed by primary and secondary organic aerosols from biogenic sources and precursors (Gilardoni et al., 2011) that are missing in the simulation. Another possible cause for the underestimation problem is underrepresented burning activity due to deforestation and forest degradation, with consequently underestimated fire aerosol emissions in these regions. The AOD simulations over tropical savanna regions with pervasive biomass burning activities are also lower than the satellite observations, which might be attributable to both underestimated online fire emissions and wet scavenging of primary carbonaceous aerosols that is too strong in the CAM5–MAM3 model (Liu et al., 2012). The CAM5 model overestimates dust emissions significantly, with some spuriously high AOD hotspots emerging over the Saharan, Arabian, South African, and central Australian desert regions. This dust AOD overestimation problem was also found in a previous dust modeling study using the release version of the CAM5–MAM3 model (Albani et al., 2014).
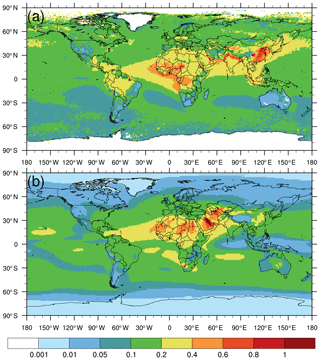
Figure 1Comparison of annual average column AOD at 550 nm from (a) MODIS aboard the Aqua satellite (2003–2010); (b) CAM5 simulation averaged from 2001 to 2010.
To further evaluate the fire-related AOD modeling performance, we compare the difference between CTRL1 and SENS1A to isolate aerosol contributions from fire sources in Fig. 2. The spatial distribution of fire-related AOD clearly highlighted African savanna as a major biomass burning region. We also compare monthly AOD at six fire-prone regions with AERONET observations to get a better understanding of temporal variations in fire aerosols. Most sites show strong seasonal variations in monthly AOD, as observed by AERONET, and the CESM-RESFire model captures fire seasonality well in these regions. Generally, the model AOD results are at the lower ends of the uncertainty ranges of ground-based observations in most regions due to the limited spatial representativeness of coarse model grid resolution and fire emissions, especially over African savannas like Ilorin (Fig. 2e) and Southeast Asian rainforests like Jambi (Fig. 2g) where agricultural and deforestation-related burning activity prevails.
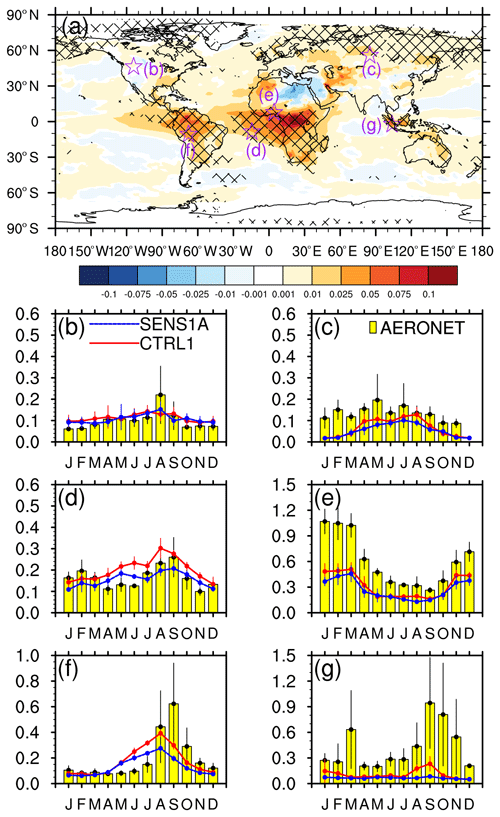
Figure 2CESM-RESFire simulation of (a) annual average fire-contributed AOD at 550 nm (shading) in the present-day scenario (CTRL1–SENS1A). The stars denote the AERONET site location, and the hatching denotes the 0.05 significance level of the two-tailed Student's t test; (b) comparison with AERONET monthly AOT observations at 550 nm in Missoula (114.1∘ W, 46.9∘ N) during the 2000s. The error bars denote ±1 standard deviation of interannual variations in the simulations and observations, respectively; (c) same as panel (b) but in Tomsk (85.1∘ E, 56.5∘ N); (d) same as panel (b) but on Ascension Island (14.4∘ W, 8.0∘ S); (e) same as panel (b) but in Ilorin (4.3∘ E, 8.3∘ N); (f) same as panel (b) but in Rio Branco (67.9∘ W, 10.0∘ S); (g) same as panel (b) but in Jambi (103.6∘ E, 1.6∘ S).
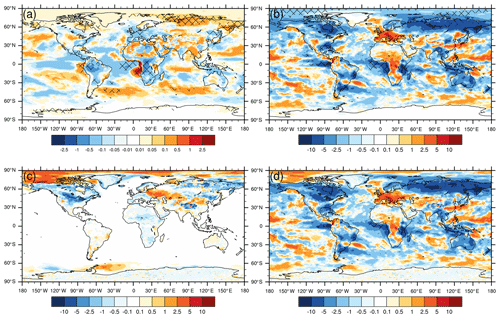
Figure 3Present-day simulation of fire-contributed annual average radiative effects through (a) aerosol–radiation interactions (REari, W m−2), (b) aerosol–cloud interactions (REaci, W m−2), (c) fire-aerosol-induced surface albedo change (REsac, W m−2), and (d) fire-aerosol-related net radiative effects (REaer, W m−2). All these radiative effects are estimated as changes in the shortwave radiative flux at the TOA between the CTRL1 and SENS1A experiments. The hatching denotes the 0.05 significance level.
Table 2Comparison of fire-related radiative effects in the present-day (CTRL1–SENS1A) and RCP4.5 future (CTRL2–SENS2A) scenarios based on this work and previous studies.
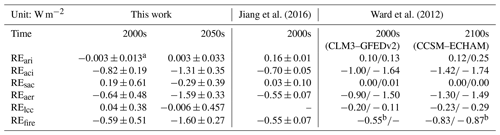
a The numbers after ± denote standard deviations of interannual variations; b the net radiative forcing includes other effects such as GHGs and climate–biogeochemistry (BGC) feedback.
Lastly, we estimate the present-day radiative effects of fire aerosols and fire-induced land cover change and compare the results with previous studies in Fig. 3 and Table 2. The radiative effect of fire aerosol–radiation interactions (REari) is most prominent in tropical Africa and downwind Atlantic Ocean areas as well as South America and the eastern Pacific. High-latitude regions like eastern Siberia also show significant positive radiative effects due to fire-emitted light-absorbing aerosols such as black carbon (BC). The land–sea contrast of radiative warming and cooling effects over Africa and South America is attributed to differences in cloud cover fractions over land and ocean areas (Jiang et al., 2016). In these regions, cloud fractions and liquid water path are much larger over downwind ocean areas than land areas during the fire season. The cloud reflection of solar radiation strongly enhances light absorption by fire aerosols residing above low-level marine clouds (Abel et al., 2005; Zhang et al., 2016).
The radiative effect of fire aerosol–cloud interactions (REaci) generally shows cooling effects in most regions due to scattering and reflections by enhanced cloudiness, and these cooling effects are more pervasive over high-latitude regions such as boreal forests in North America and eastern Siberia. The land–sea contrast of radiative effects emerges again in the vicinity of Africa and South America, but the signs of the contrasting effect related to aerosol–cloud interactions are opposite to those from aerosol–radiation interactions. The large amounts of fire aerosols suppress low-level clouds over the African land region by stabilizing the lower atmosphere through a reduction in the radiative heating of the surface. However, fire aerosols increase cloud cover and brightness in the downwind Atlantic Ocean areas because they increase the number of cloud condensation nuclei, and the larger cloud droplet number density reduces cloud droplet sizes (Lu et al., 2018; Rosenfeld et al., 2019; Fig. S1 in the Supplement). The radiative effect of fire-aerosol-related surface albedo change (REsac) shows contrasting radiation effects, with strong warming effects over most Arctic regions caused by the deposition of light-absorbing aerosol over ice and snow as well as a reduction of surface albedo, but moderate cooling effects in boreal land regions such as Canada and eastern Siberia, which are related to fire-aerosol-induced snowfall and snow cover change as well as associated surface albedo change (Ghan, 2013; Fig. S2 in the Supplement). Besides spatial heterogeneity in fire-induced radiative effects, these radiative effects also show significant temporal variations that are related to fire seasonality. Figure 4 shows zonally averaged time–latitude cross sections of fire aerosol emissions and fire-induced changes in clouds and radiative effects. Massive fire carbonaceous emissions shift from the Northern Hemisphere tropical regions in boreal winter to the Southern Hemisphere tropical regions in boreal summer, when similar amounts of fire emissions are also observed in boreal midlatitude and high-latitude regions (Fig. 4a, b). Fire aerosols greatly increase the number of cloud condensation nuclei (CCN; Fig. 4c) and cloud droplet number concentrations (CDNUMC; Fig. 4d) in these regions, while the increases in cloud water path (CWP; Fig. 4e) and low cloud fraction (CLDLOW; Fig. 4f) are more significant in boreal high-latitude regions than in the tropics. The low solar zenith angle in high-latitude regions enhances solar radiation absorption by light-absorbing aerosols and results in stronger changes in radiative effects by aerosol–radiation interactions during boreal summer (Fig. 4g). In the meantime, increased CWP and CLDLOW in high-latitude regions also lead to much stronger cooling effects by aerosol–cloud interactions (REaci) (Fig. 4h), which overwhelm the increase in REari. These modeling results based on the online coupled RESFire model show similar spatiotemporal patterns as these in Jiang et al. (2016), which used the same version of the CAM5 atmosphere model with a four-mode modal aerosol module (MAM4) that was driven by offline prescribed fire emissions.
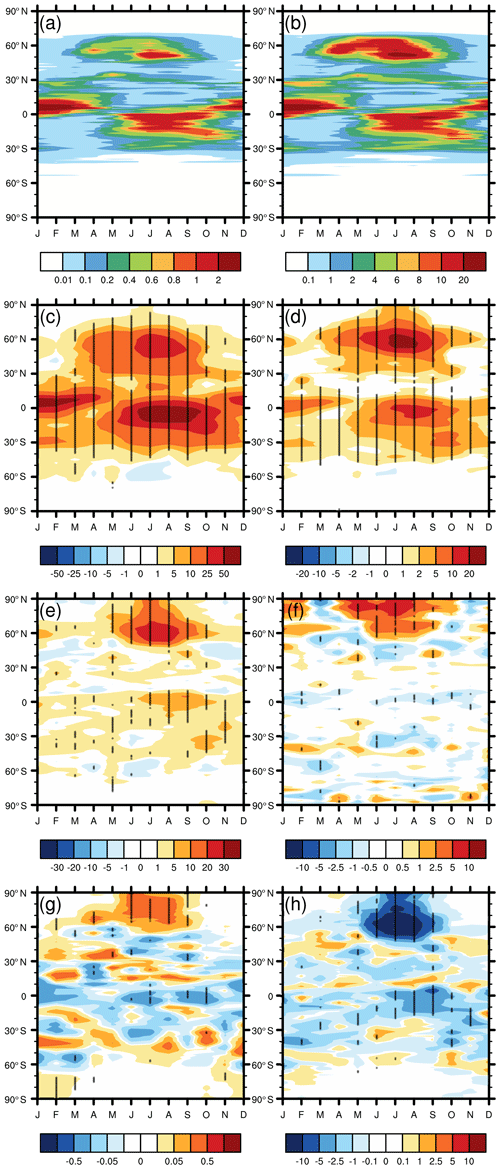
Figure 4Present-day simulation of zonally averaged time–latitude cross sections of the following: (a) monthly BC fire emission fluxes (mg m−2) in CTRL1; (b) monthly POM fire emission fluxes (mg m−2) in CTRL1; (c) fire-induced low-level (averaged below 800 hPa) cloud condensation nuclei (CCN, no. m−3) concentration changes (CTRL1–SENS1A); (d) vertically integrated cloud droplet number concentration (CDNUMC, 109 no. m−2) changes (CTRL1–SENS1A); (e) cloud water path (CWP, g m−2) changes (CTRL1–SENS1A); (f) low cloud cover fraction (100 %) changes (CTRL1–SENS1A); (g) radiative effect changes (CTRL1–SENS1A) by fire aerosol–radiation interactions (REari, W m−2); and (h) radiative effect changes (CTRL1–SENS1A) by fire aerosol–cloud interactions (REaci, W m−2). The dots in panels (c)–(h) denote the 0.05 significance level.
Table 3Comparison of fire and carbon budget variables between CESM-RESFire simulations and previous studies and benchmarks.
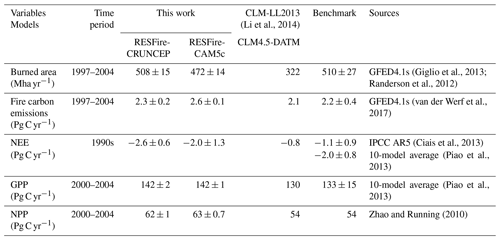
In general, the 10-year average global mean values and standard deviations of interannual variations for fire aerosol-related REari, REaci, and REsac in the 2000s are −0.003 ± 0.013, , and 0.19±0.61 W m−2, respectively, and fire-induced RElcc is 0.04±0.38 W m−2. After combining all these forcing terms, we estimate a net REfire of W m−2 for the present-day scenario that is larger than the estimate of −0.55 W m−2 in previous fire radiative effect studies (Jiang et al., 2016; Ward et al., 2012). It is noted that both Ward et al. (2012) and Jiang et al. (2016) used prescribed fire emissions from CLM3 model simulations (Kloster et al., 2010, 2012) and GFED datasets (Giglio et al., 2013; Randerson et al., 2012), respectively, for their uncoupled fire sensitivity simulations. The annual fire carbon emissions used by Ward et al. (2012) ranged from 1.3 Pg C yr−1 for the present-day simulation to 2.4 Pg C yr−1 for the future projection with ECHAM atmospheric forcing, while the fire BC, particulate organic matter (POM), and SO2 emissions used by Jiang et al. (2016) were based on the GFEDv3.1 dataset with an annual average fire carbon emission of 1.98 Pg C yr−1 (Randerson et al., 2012). Their fire emissions are lower than the RESFire model simulation of 2.6 Pg C yr−1 (Table 3) in this study, which contributes to the differences in the estimates of fire aerosol radiative effects. It is also worth noting that all fire emissions were released into the lowest CAM level as surface sources by Ward et al. (2012), and a default vertical profile of fire emissions based on the AEROCOM protocol (Dentener et al., 2006) was used by Jiang et al. (2016) in their CAM5 simulations. In our simulations, we used a simplified plume rise parameterization (Sofiev et al., 2012) based on online calculated fire burning intensity (FRP) and atmospheric stability conditions (PBLH and Brunt–Väisälä frequency) in CESM-RESFire and applied vertical profiles with diurnal cycles to the vertical distribution of fire emissions. The simulations of the annual median heights of fire plumes for the present-day and RCP4.5 future scenarios are shown in Fig. 5. Previous observation-based injection height studies suggested that only 4 %–12 % of fire plumes could penetrate planetary boundary layers, with most fire plumes staying within the near-surface atmosphere layers (Val Martin et al., 2010). Our plume rise simulation results agree with these estimates, though a quantitative comparison is beyond the scope of this study because of the inconsistency between simulated and actual meteorological conditions. It is also noted that there is no systematic change in plume rise height distributions between the RCP4.5 future scenario and present-day scenarios, both of which show most fire plumes (∼80 %) rising less than 1000 m. Compared to surface-released fire emissions in previous studies (Ward et al., 2012), our higher elevated fire plumes affect the vertical distribution and lifetime of fire aerosols and further influence regional radiative effects after the long-range transport of fire aerosols.
3.2 Fire-related disturbance to carbon balance
In addition to the atmosphere-centric fire-induced radiative effects, we also quantify the vegetation-centric terrestrial carbon budget changes to evaluate fire disturbance to terrestrial ecosystems. We use the previous model inter-comparison studies and the latest GFEDv4.1s datasets as evaluation benchmarks and examine fire-related metrics including global burned area and fire carbon emissions (Fig. 6 and Table 3). We also collect global-scale GPP, NPP, and NEE from the previous literature (Ciais et al., 2013; Piao et al., 2013; Zhao and Running, 2010) to compare with our simulation results (Table 3). The RESFire model performs well in global burned area and fire carbon emissions driven by either offline observation- and reanalysis-based CRUNCEP atmosphere data (RESFire_CRUNCEP) or online CAM5-simulated atmosphere data after bias corrections (RESFire_CAM5c). The annual averaged burned area results of both RESFire_CRUNCEP (508±15 Mha yr−1) and RESFire_CAM5c (472±14 Mha yr−1) are very close to the GFEDv4.1s benchmark value of 510±27 Mha yr−1, while the default fire model in CLM (322 Mha yr−1) is significantly low biased. For fire carbon emissions, the offline RESFire_CRUNCEP result (2.3±0.2 Pg C yr−1) agrees well with the GFEDv4.1s benchmark of around 2.2±0.4 Pg C yr−1, and the online RESFire_CAM5c result shows an 18 % higher value (2.6±0.1 Pg C yr−1) than the benchmark. Since the GFED emission datasets are low biased due to low satellite detection rates for small fires under canopy and clouds, previous fire studies (Johnston et al., 2012; Ward et al., 2012) rescaled fire emissions in their practice for climate and health impact assessment. Here, a moderate increase in online estimated fire carbon emissions would reduce the need for fire emission rescaling. Such a difference is also consistent with the changes in different versions of the GFED datasets, which show an 11 % increase in global fire carbon emissions in the latest GFED4s compared with the previous GFED3 for the overlapping 1997–2011 time period (van der Werf et al., 2017). This increased global fire carbon emissions in the GFED4s dataset result from a substantial increase in global burned area (+37 %) due to the inclusion of small fires and a modest decrease in mean fuel consumption (−19 %) according to van der Werf et al. (2017). Since carbon emissions from deforestation fires and other land use change processes are a key component to estimate the global carbon budget (Le Quéré et al., 2013), improved fire emission estimations would benefit carbon budget simulations in the land model.
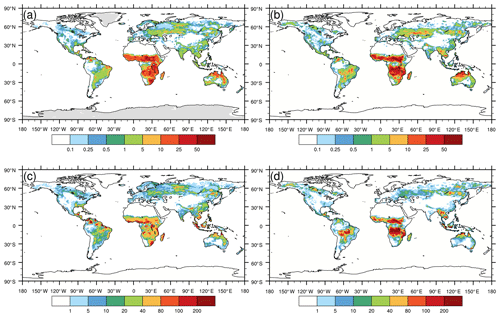
Figure 6Comparison of CESM-RESFire simulations and GFED4.1s data. (a) Ensemble-averaged annual fractional burned area (% yr−1) simulation; (b) 10-year averaged (2001–2010) annual fractional burned area (% yr−1) based on the GFED4.1s data; (c) ensemble-averaged annual fire carbon emission (g C m−2 yr−1) simulation; (d) 10-year averaged (2001–2010) annual fire carbon emission (g C m−2 yr−1) based on the GFED4.1s data.
We then compare the CLM-simulated carbon budget variables such as GPP and NEE against 10 process-based terrestrial biosphere models that were used for the IPCC Fifth Assessment Report (Piao et al., 2013). Both the offline and online CLM GPP results are around 142 Pg C yr−1, which is higher than the MODIS primary production products (MOD17) of 109.29 Pg C yr−1 (Zhao et al., 2005) and near the upper bound of ensemble modeling results (133±15 Pg C yr−1) (Piao et al., 2013). Such high GPP estimation leads to ∼11 % higher NPP in the CLM simulations than the MODIS global average annual NPP product of 53.5 Pg C yr−1 from 2001 to 2009 (Zhao and Running, 2010) as well as the previous modeling result (54 Pg C yr−1) based on the default fire model in CLM developed by Li et al. (2013, 2014) (hereafter CLM-LL2013). These differences may result from the different atmosphere forcing data used to drive the CLM land model. However, the NEE results based on the CESM-RESFire model are consistent with the benchmarks from the IPCC AR5 (Ciais et al., 2013) and ensemble modeling results (Piao et al., 2013), indicating a good land modeling performance with online fire disturbance in CESM.
Table 4Comparison of carbon budget variables between fire simulations driven by the CRUNCEP data atmosphere based on CESM-RESFire and CLM-LL2013.
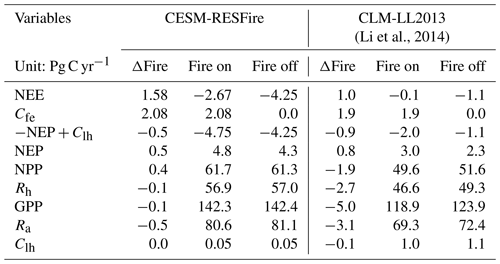
After the evaluation of the carbon budget in the CLM land model, we further decompose the components in NEE and compare the new CESM-RESFire simulation results with previous fire model simulations by Li et al. (2014). Following the experiment setting in Li et al. (2014), we isolate fire contributions to each carbon budget variable by differencing the fire-on and fire-off experiments driven by the CRUNCEP data atmosphere in Table 4. We find a 58 % increase in fire-induced NEE variations simulated by CESM-RESFire than CLM-LL2013. This increase is attributed to enhanced fire emissions and suppressed NEP in CESM-RESFire. As discussed in the previous section, CESM-RESFire simulates higher annual average fire carbon emissions (2.08 Pg C yr−1) than CLM-LL2013 (1.9 Pg C yr−1), which contributes 31 % of the difference in their NEE changes. Furthermore, CESM-RESFire simulates smaller NEP changes due to fire disturbance, which is attributable to fire-induced land cover change in RESFire. Fire-induced whole-plant mortality and post-fire vegetation recovery are implemented in the new CESM-RESFire model (Zou et al., 2019), both of which are not included in the default CLM-LL2013 model. The newly incorporated fire-induced land cover change would influence ecosystem productivity and respiration, as shown by the carbon budget variables in Table 4. Specifically, fire-induced whole-plant mortality and recovery would moderate the variations in ecosystem productivity and respiration and further suppress fire-induced NEP changes. The suppressed NEP change explains 52 % of the total difference between CESM-RESFire and CLM-LL2013 in simulated NEE changes.
Similar suppression effects of fires on NEP were also found in Seo and Kim (2019), in which they used the CLM-LL2013 fire model but enabled the dynamic vegetation (DV) mode to simulate post-fire vegetation changes. Though the DV mode of the CLM model is capable of simulating vegetation dynamics, considerable biases exist in the online simulation of land cover change by the coupled CLM–DV model (Quillet et al., 2010) and may undermine the interpretation of fire-related ecological effects. For instance, the global fractions of bare ground and needleleaf trees in the CLM–DV simulations are much larger than those in the non-DV (BGC only) simulation in Seo and Kim (2019), while the fractions of shrub and broadleaf trees with active DV are smaller than those without DV regardless of whether or not fire disturbance is included in the simulations. These biases could distort ecosystem properties such as primary production and carbon exchange as well as fire-related ecological effects.
Table 5Comparison of carbon budget variables between CESM-RESFire sensitivity experiments and previous studies.
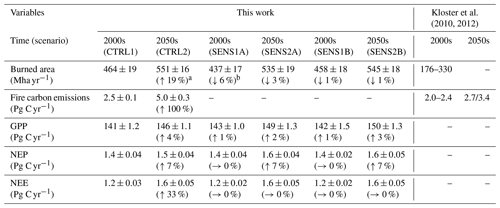
a Percentage numbers in parentheses under CTRL2 denote relative changes compared with the CTRL1 scenario. b Percentage numbers in parentheses under SENSx (x =1 or 2) denote relative changes compared with the corresponding CTRLx (x =1 or 2) scenarios.
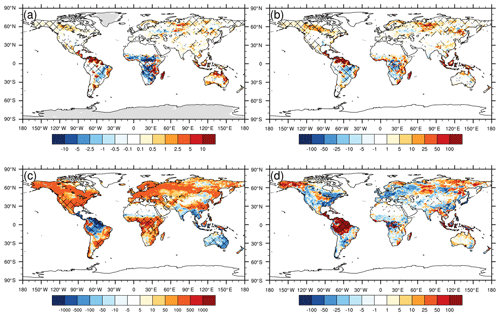
Figure 7CESM-RESFire-simulated changes between the RCP4.5 future scenario and the present-day scenario (CTRL2–CTRL1) in (a) annual fractional burned area (% yr−1), (b) annual averaged fire carbon emissions (g C m−2 yr−1), (c) annual averaged GPP (g C m−2 yr−1), and (d) annual averaged NEE (g C m−2 yr−1). The hatching denotes the 0.05 significance level.
Similar to fire-related radiative effects, we examine changes in carbon budget variables in the RCP4.5 future scenario in Table 5 and Fig. 7. The global burned area increases by 19 % from the present-day scenario in CTRL1 (464±19 Mha yr−1) to the RCP4.5 future scenario in CTRL2 (551±16 Mha yr−1) (Fig. 7a). Accordingly, the annual average fire carbon emissions increase by 100 % from 2.5 ± 0.1 Pg C yr−1 at present to 5.0±0.3 Pg C yr−1 in the future (Fig. 7b). This increase is larger than a previous CLM-simulated result of 25 %–52 % by Kloster et al. (2010, 2012), which might result from different climate sensitivity between CESM-RESFire and the previous fire model in CLM. It is noted that recent satellite-based studies found decreasing trends in burned area over specific regions such as Northern Hemisphere Africa driven by human activity and agricultural expansion (Andela and van der Werf, 2014; Andela et al., 2017). Though we mainly focus on fire–climate interactions without consideration of human impacts in this study, the RESFire model is capable of capturing anthropogenic interference in fire activity and reproducing observation-based long-term trends of regional burning activity driven by climate change and human factors (Zou et al., 2019). The carbon budget variables including GPP, NEP, and NEE increase by 4 %, 7 %, and 33 %, respectively (Fig. 7c–d). These carbon variables affect terrestrial ecosystem productivity as well as fuel load supply for biomass burning, which further modulate fire emissions that lead to discrepancies between burned area and emission changes. For instance, most decreasing changes in burned area occur in tropical and subtropical savannas and grasslands, while significant increasing changes are evident in boreal forest and tropical rainforests of Southeast Asia (Fig. 7a). This spatial shift of burning activity from low fuel loading areas (e.g., grassland) to high fuel loading areas (e.g., forest) greatly amplifies the changes in fire emissions due to boosted fuel consumption. The complex climate–fire–ecosystem interactions will be discussed in the next section.
3.3 Simulations of climate–fire–ecosystem interactions using CESM-RESFire
In the last section, we find a 19 % increase in global burned area in the RCP4.5 future scenario compared with the present-day scenario. We examine spatial distributions and driving factors of this change in Fig. 8. The fire ignition distribution shows heterogeneous changes, with significant increases in boreal forest regions over Eurasia and rainforest regions in South America but decreases in South American savanna as well as African rainforests and savanna. These changes in fire ignition are mainly driven by changes in fuel combustibility as shown by fire combustion factors (Fig. 8b), which are computed using fire weather conditions including 10 d running means of surface air temperature, precipitation, and soil moisture (Zou et al., 2019). The spatial distribution changes in fire spread (Fig. 8c) show similar but more apparent patterns of increased fire spread rates over most regions except savanna and rainforests in Africa and South America, which are attributed to the changes in fire spread factors (Fig. 8d). These fire spread factors depend on surface temperature, relative humidity, soil wetness, and wet canopy fractions that modulate fuel moisture and fire spread rates in the model (Zou et al., 2019). The burned area changes are driven by changes in fire weather conditions affecting both fire ignition and fire spread, with a global spatial correlation coefficient of 0.4 between differences in fractional burned area (Fig. 7a) and fire counts (Fig. 8a) and of 0.38 between burned area (Fig. 7a) and fire spread rates (Fig. 8c). These burning activity changes found in this study also agree quite well with previous long-term projections based on an empirical statistical framework and a multi-model ensemble of 16 general circulation models (GCMs), in which good model agreement was found on increasing fire probabilities (∼62 %) at middle to high latitudes as well as decreasing fire probabilities (∼20 %) in the tropics (Moritz et al., 2012).
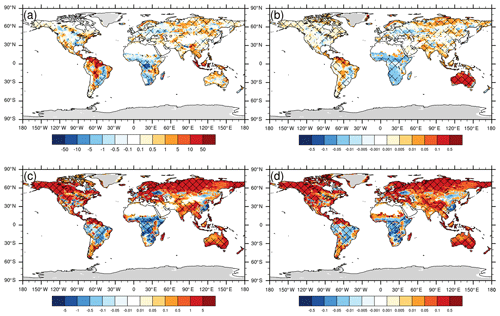
Figure 8CESM-RESFire-simulated changes in fire-related variables between the RCP4.5 future scenario and the present-day scenario (CTRL2–CTRL1). (a) Changes in annual total fire ignition (NFIRE, count km−2 yr−1); (b) changes in annual average fire combustion factors (FCF, unitless); (c) changes in annual average fire spread rates (FSR_DW, cm s−1); (d) changes in annual average fire spread factors (FSF, unitless). The hatching denotes the 0.05 significance level.
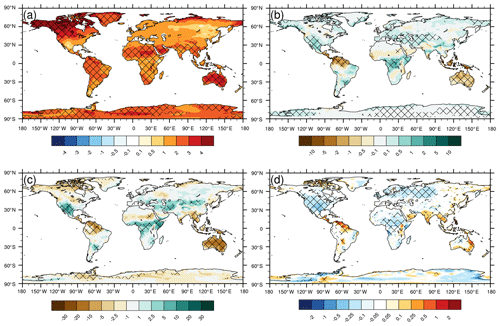
Figure 9CESM-RESFire-simulated changes in fire weather variables between the RCP4.5 future scenario and the present-day scenario (CTRL2–CTRL1). (a) Changes in surface temperature (K); (b) changes in total precipitation rate (mm d−1); (c) changes in surface relative humidity (%); (d) changes in surface wind speed (m s−1). The hatching denotes the 0.05 significance level. For clear comparison with fire changes in Figs. 7 and 8, only fire weather changes over land are shown.
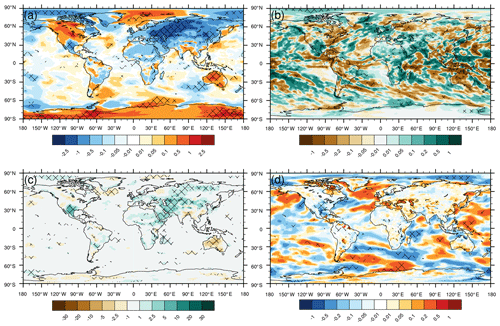
Figure 10Fire-induced changes in fire weather variables between the RCP4.5 future scenario and the present-day scenario ((CTRL2–CTRL1)–(SENS2B–SENS1B)). (a) Fire-induced changes in surface temperature (K); (b) fire-induced changes in total precipitation rate (mm d−1); (c) fire-induced changes in surface relative humidity (%); (d) fire-induced changes in surface wind speed (m s−1). The hatching denotes the 0.05 significance level.
To understand the changes in specific fire weather variables, we compare the differences of surface air temperature, total precipitation rates, relative humidity, and surface wind speed between the future (CTRL2) and present-day (CTRL1) scenarios in Fig. 9. As expected in a modest warming scenario, the global annual mean temperature is projected to increase by 1.7 ∘C on average with pervasive warming over land areas (Fig. 9a). The temperature increases are stronger in high-latitude regions like Alaska, northern Canada, and Antarctica as well as Australia. Meanwhile, hydrological conditions also undergo significant but nonhomogeneous changes in many regions in the projection, with hot and dry weather conditions favorable for fire in Australia, Southeast Asia, Central America, and the northern coast of South America (Fig. 9b and c). Most of these regions also show increased surface wind speed that is conducive to faster fire spread (Fig. 9d). Since these variations in fully coupled CTRL experiments can be induced by either global-warming-driven weather changes or fire feedback, we further decompose the total changes into two components: one without fire feedback (i.e., SENS2B–SENS1B) and the other purely by fire feedback (i.e., (CTRL2–CTRL1)–(SENS2B–SENS1B)). We show the fire-induced weather changes in Fig. 10 and those without fire feedbacks in Fig. S3 in the Supplement. It is clear that the majority of the changes in fire weather conditions is driven by atmospheric conditions associated with global warming since the spatial patterns in Figs. 9 and S3 almost resemble each other over most land regions. However, fire feedbacks also exert non-negligible effects on local and remote weather conditions that manifest as positive or negative feedback mechanisms to regional fire activities. For instance, Australia shows increased temperature (Fig. 10a) and surface wind speed (Fig. 10d), as well as decreased precipitation (Fig. 10b) and relative humidity (Fig. 10c) induced by fire, which are consistent with the changes without fire feedbacks (Fig. S3 in the Supplement) and the total changes (Fig. 9). In contrast, most Eurasian regions show decreased temperature (Fig. 10a) and increased relative humidity (Fig. 10c), with nonhomogeneous changes in precipitation (Fig. 10b) in response to fire perturbations. These regionally varying results suggest complex interactions between fire and climate systems that merit further investigation.
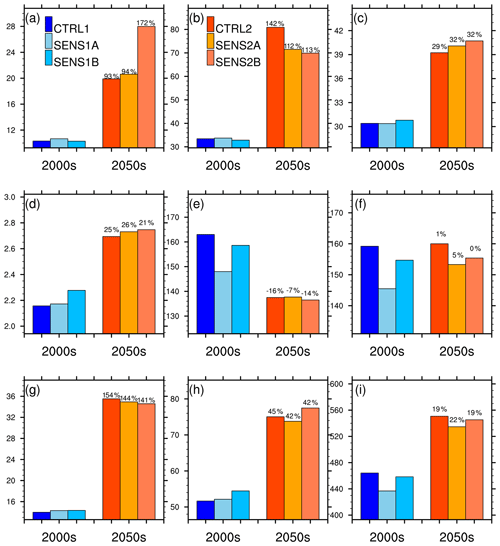
Figure 11Comparison of annual burned area (Mha yr−1) in each region among different time periods and sensitivity experiments. (a) North America; (b) South America; (c) Eurasia excluding the Middle East and South Asia; (d) the Middle East and North Africa; (e) Northern Hemisphere Africa; (f) Southern Hemisphere Africa; (g) South and Southeast Asia; (h) Oceania; (i) global total BA. The percentage numbers above the projection columns are changes in burned area in the 2050s relative to their counterpart experiments in the 2000s. The spatial distributions of these regions are shown in Fig. S4 of the Supplement.
Therefore, we aggregate regional burned areas in each experiment and compare their changes between the two scenarios to quantify the regional effects of different feedback mechanisms (Fig. 11). An atmosphere-centric feedback pathway is identified by comparing relative changes in regional burned area with (i.e., CTRL2–CTRL1) and without (i.e., SENS2A–SENS1A) fire aerosol effects, while a vegetation-centric feedback pathway is identified by comparing relative changes in regional burned area with (i.e., SENS2A–SENS1A) and without (i.e., SENS2B–SENS1B) fire-induced LCC. The comparison of relative changes in regional burned area with different feedback pathways reveals distinct regional responses to these fire-related atmospheric and vegetation processes. The most significant fire feedback effects occur in North America (Fig. 11a) and South America (Fig. 11b), with the former dominated by negative vegetation-centric fire feedback and the latter dominated by positive atmosphere-centric fire feedback. By including fire-induced LCC, the projected burned area increases over North America in the 2050s are greatly suppressed and reduced from +172 % in SENS2B to +94 % in SENS2A and +93 % in CTRL2. In contrast, the burned area increases over South America considerably enlarge after incorporating fire aerosol effects in the projection, from +112 % in SENS2A and +113 % in SENS2B to +142 % in CTRL2. The fire feedback effects are also evident in many other regions, such as similar positive atmosphere-centric feedbacks in Southeast Asia (Fig. 11g) and Oceania (Fig. 11h) but negative atmosphere-centric feedbacks in Africa (Fig. 11e and f). The signs of these feedback effects are determined by fire perturbation on regional fuel and fire weather conditions such as precipitation through fire aerosol–cloud–precipitation interactions or changed vegetation evapotranspiration due to fire-induced LCC (Fig. S5 in the Supplement). It is worth noting that these feedback effects could enhance (e.g., North America and Southeast Asia) or compensate for (e.g., Northern Hemisphere and Southern Hemisphere Africa) each other in different regions, which further increases the complexity of climate–fire–ecosystem interactions at regional and global scales. On global average, the net effect of fire feedbacks is almost neutral (Fig. 11i and Table 5) due to the offsetting between positive vegetation-centric and negative atmosphere-centric feedbacks, which are largely dominated by burning activity in African regions.
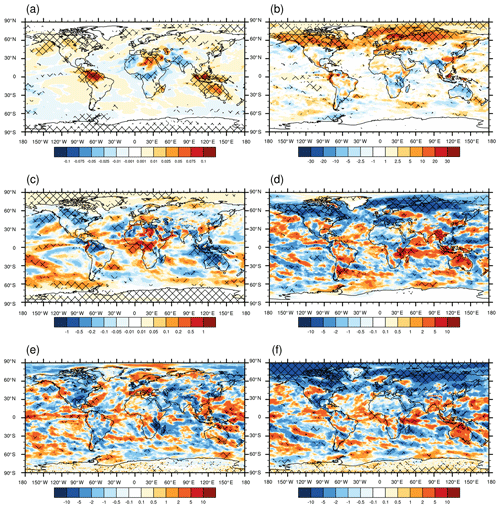
Figure 12Changes in fire-induced weather conditions and climate radiative forcing between the RCP4.5 future scenario and the present-day scenario. (a) Changes in annual average column AOD at 550 nm (unitless, (CTRL2–SENS2A)–(CTRL1–SENS1A)); (b) changes in cloud liquid water path (g m−2, (CTRL2–SENS2A)–(CTRL1–SENS1A)); (c) changes in REari, (W m−2, (CTRL2–SENS2A)–(CTRL1–SENS1A)); (d) changes in REaci (W m−2, (CTRL2–SENS2A)–(CTRL1–SENS1A)); (e) changes in RElcc (W m−2, (SENS2A–SENS2B)–(SENS1A–SENS1B)); (f) changes in REfire (W m−2, (CTRL2–SENS2B)–(CTRL1–SENS1B)). The hatching denotes the 0.05 significance level.
Lastly, we compare the difference of climate radiative forcing associated with these burning activity changes between the future and present-day scenarios in Table 2 and Fig. 12. Due to broadly increased burning activities in the future projection, fire aerosols are strongly enhanced over most fire-prone regions except Northern Hemisphere Africa and South Asia (Fig. 12a), where the projected burning activity is suppressed as discussed in previous sections. Increased fire aerosols lead to diverse responses in cloud liquid water path, with large increases in high-latitude regions but general decreases in the tropics and subtropics (Fig. 12b). These fire and weather changes result in pronounced responses in radiative forcing through multiple pathways including aerosol–radiation interaction (Fig. 12c), aerosol–cloud interaction (Fig. 12d), and fire-induced LCC (Fig. 12e). The fire-aerosol-related RE changes show more consistent and statistically significant changes over fire-prone regions than those induced by LCC. Previous studies have suggested a net cooling effect of deforestation that could compensate for GHG warming effects on a global scale (Bala et al., 2007; Jin et al., 2012; Randerson et al., 2006). Though our model captures the reduction of forest coverage and increased springtime albedo in high-latitude regions (Fig. S6 in the Supplement), the radiative effect of fire-induced LCC is almost neutral on a global basis in both present-day and future scenarios (Table 2). In general, most burning regions with increased fire aerosols show cooling effects due to enhanced aerosol scattering of solar radiation, while those with decreased fire aerosols show warming effects (Fig. 12c). Fire aerosol direct radiative forcing is overwhelmed by much stronger indirect effects through aerosol–cloud interactions (Fig. 12d), with pervasive cooling effects in high-latitude regions with increased cloudiness (Fig. 12b). Such indirect effects also dominate the net fire radiative effects at both regional and global scales, contributing to a 171 % increase in the global net fire radiative effect in the RCP4.5 future scenario (Table 2). This projection result is larger than the change in net fire radiative forcing based on the CCSM future projection in Ward et al. (2012), which suggested a 51 % increase from −0.55 W m−2 in the 2000s to −0.83 W m−2 in the 2100s (Table 2). It is noted that their net estimate of fire radiative forcing changes includes other offline-based fire climate effects such as fire-related GHG impacts and climate–biogeochemical cycle feedbacks, which could dampen the cooling effect of fire aerosols.
3.4 Discussion of modeling uncertainties
As discussed in previous sections, the complex climate–fire–ecosystem interactions in fire-related atmospheric and vegetation processes can introduce large uncertainties in the fire projections and associated climate effects. Here we list major uncertainty sources that deserve further investigation in the future.
The future projection of fire triggers such as lightning and human activity is highly uncertain and difficult to explicitly parameterize in global climate models at present. Previous studies suggested different and even contradictory changes in projected lightning in the future (Clark et al., 2017; Finney et al., 2018), likely due to differences in the lightning parameterization schemes used. Pathway-dependent long-term projections of demographic data and socioeconomic conditions are also highly uncertain (Riahi et al., 2017). For these reasons, we did not consider these factors in our projection experiments by using fixed demographic and lightning data. Assessing the impacts of these factors will require implementations of different lightning parameterizations and socioeconomic scenarios in climate simulations.
Similar uncertainties arise from future projections of land use and land cover changes as well as dynamic global vegetation modeling (DGVM). These anthropogenic and ecological processes could directly or indirectly modulate fire activities by changing fire risks and fuel availability. In this study, we used semi-static land use and land cover data with the sole consideration of fire perturbations in both historical and projection scenarios. The inclusion of DGVM will enable the projection of vegetation distributions but introduce additional uncertainties (Zou et al., 2019).
The uncertainties of fire emission estimates arise from those in surface fuel loads, combustion completeness, emission factors, and vertical distributions with rising fire plumes. More measurements of these parameters over extended temporal–spatial scales are needed to fully evaluate these terms in the fire models. A newly developed fire plume rise scheme (Ke et al., 2020) has been recently implemented in the fire model used in this study and will be used for future fire modeling and evaluation studies.
Last but not least, fire aerosol radiative effects and aerosol–cloud interactions play an important role in simulating the climate effects of fire aerosols. Though the atmosphere model used in this study incorporates aerosol–cloud interactions, these atmospheric processes across multiple spatial and temporal scales are major contributors to the uncertainties of climate change assessments (Ciais et al., 2013; Seinfeld et al., 2016). Community-wide efforts are ongoing to quantify and reduce the uncertainties of climate modeling discussed above.
In this study, we conducted a series of fire–climate modeling experiments for present-day and future scenarios with an explicit implementation of multiple climate–fire–ecosystem feedback mechanisms. We evaluated the CESM-RESFire modeling performance in the context of fire-related radiative effects and the terrestrial carbon balance. Various fire radiative effects for the present-day and the RCP4.5 future scenarios are summarized in Fig. 13. We focus on radiative forcing changes related to fire aerosols and fire-induced land cover change. We find an enhanced net fire radiative effect, which is caused by increased global burning activity and subsequent aerosol–cloud interactions, increasing from W m−2 in the 2000s to W m−2 in the 2050s. Annual global burned area and fire carbon emissions increase by 19 % and 100 %, respectively, with large amplifications in boreal regions due to suppressed precipitation and enhanced fire ignition and spread rates. These changes imply increasing fire danger over high-latitude regions with prevalent peatlands, which will be more vulnerable to increased fire threats due to climate change. Potentially increasing burning activity in these regions may greatly increase fire carbon, tracer gas, and aerosol emissions, which could have enormous impacts on the terrestrial carbon balance and radiative budget. Our modeling results imply that the increase in fire aerosols could compensate for the projected decrease in anthropogenic aerosols due to air pollution control policies in many regions (e.g., the eastern US and China) (US EPA, 2019; McClure and Jaffe, 2018; Wang et al., 2017; Zhao et al., 2014), where significant aerosol cooling effects dampen GHG warming effects (Goldstein et al., 2009; Rosenfeld et al., 2019). Such counteractive effects to anthropogenic emission reduction would also slow down air quality improvement and reduce the associated health benefits revealed by previous studies (Markandya et al., 2018; Zhang et al., 2018).
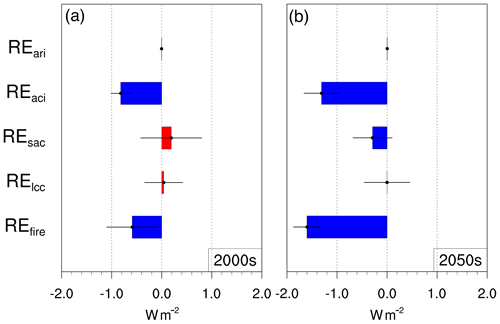
Figure 13Comparison of CESM-RESFire-simulated fire radiative effects (W m−2) in (a) the present-day scenario and (b) the RCP4.5 future scenario. The error bars denote the standard deviations of interannual variations during each 10-year simulation period. REfire denotes the net radiative effect of the four fire-related radiative effects investigated in this study ().
Fire aerosol emissions and fire-induced land cover change manifest two major feedback mechanisms in climate–fire–ecosystem interactions, showing synergistic or antagonistic effects at regional to global scales. These two distinct feedback mechanisms compete with each other and increase the complexity of interactions among each interactive component. It is noted that we only included the atmosphere and land modeling components of CESM to investigate the climate effects of global fires with other major components of the earth system including the ocean and sea–land ice in the prescribed data mode. Enhanced climate sensitivity as well as feedback and uncertainties on a multi-decadal scale might be expected in a fully coupled climate modeling system as previous studies revealed (Dunne et al., 2012, 2013; Hazeleger et al., 2010; Andrews et al., 2012). We suggest more comprehensive evaluations at regional scales to investigate these complex interactions for major fire-prone regions. More advanced fire modeling capabilities are also needed by integrating additional fire-related processes and climate effects such as fire-emitted brown carbon (Brown et al., 2018; Feng et al., 2013; Forrister et al., 2015; Liu et al., 2015; Wang et al., 2018; Zhang et al., 2017, 2019) and fire–vegetation–climate interactions and teleconnections (Garcia et al., 2016; Stark et al., 2016). More evaluation metrics such as large wildfire extreme events should be considered in future studies to improve our understanding of global and regional fire activities, their variations and trends, and their relationship to decadal climate change.
The level 3 MODIS monthly AOD data from the Aqua platform (MYD08_M3; https://doi.org/10.5067/MODIS/MYD08_M3.006; Platnick et al., 2015) used for model evaluation are available via the NASA level 1/Atmosphere Archive and Distribution System (LAADS) Distributed Active Archive Center (DAAC) at https://ladsweb.modaps.eosdis.nasa.gov/missions-and-measurements/products/MYD08_M3/ (Platnick et al., 2015). The AERONET version 3 level 2.0 AOT data are available at https://aeronet.gsfc.nasa.gov/ (Holben et al., 1998). The GFED burned area and fire emission datasets are available at https://www.geo.vu.nl/~gwerf/GFED/GFED4/ (Giglio et al., 2013; Randerson et al., 2012; van der Werf et al., 2017). The CESM-RESFire simulation results of the six numerical experiments in the main text are deposited at the Figshare website (https://doi.org/10.6084/m9.figshare.9765356; Zou, 2020). The modeling source code and input data materials are available upon request, which should be addressed to Yufei Zou (yufei.zou@pnnl.gov).
The supplement related to this article is available online at: https://doi.org/10.5194/acp-20-995-2020-supplement.
YZ and YW designed the experiments, and YZ carried them out. YZ developed the model code and performed the simulations. YZ and YW wrote the paper, and all coauthors reviewed and edited the paper.
The authors declare that they have no conflict of interest.
This work has not been subjected to any NSF review and therefore does not necessarily reflect the views of the foundation, and no official endorsement should be inferred.
We would like to acknowledge high-performance computing support from Cheyenne (https://doi.org/10.5065/D6RX99HX; CISL, 2017) provided by NCAR's CISL, sponsored by the National Science Foundation. The v2.2 gridded satellite lightning data were produced by the NASA LIS/OTD Science Team (Principal Investigator, Dr. Hugh J. Christian, NASA/Marshall Space Flight Center) and are available from the Global Hydrology Resource Center (http://ghrc.nsstc.nasa.gov, last access: 18 January 2019). We are thankful to Steve Platnick for processing the MODIS AOD data. We thank all the GFED team members for providing the GFED data at http://www.globalfiredata.org/. We thank Wei Min Hao, Brent Holben, Paulo Artaxo, Mikhail Panchenko, Sergey Sakerin, Rachel T. Pinker, and their staff for establishing and maintaining the six AERONET sites used in this study. We thank Chandan Sarangi and two anonymous reviewers for the helpful discussion to improve the presentation of this work.
This research has been supported by the National Science Foundation (grant nos. 1243220 and 1243232) and the U.S. Department of Energy (DOE) Office of Science as part of the Regional and Global Climate Modeling Program (NSF-DOE-USDA EaSM2). The Pacific Northwest National Laboratory (PNNL) is operated for the DOE by the Battelle Memorial Institute under contract DE-AC05-76RL01830.
This paper was edited by Kostas Tsigaridis and reviewed by two anonymous referees.
Abatzoglou, J. T. and Williams, A. P.: Impact of anthropogenic climate change on wildfire across western US forests, P. Natl. Acad. Sci. USA, 113, 11770–11775, https://doi.org/10.1073/pnas.1607171113, 2016.
Abatzoglou, J. T., Williams, A. P., and Barbero, R.: Global Emergence of Anthropogenic Climate Change in Fire Weather Indices, Geophys. Res. Lett., 46, 326–336, https://doi.org/10.1029/2018gl080959, 2019.
Abel, S. J., Highwood, E. J., Haywood, J. M., and Stringer, M. A.: The direct radiative effect of biomass burning aerosols over southern Africa, Atmos. Chem. Phys., 5, 1999–2018, https://doi.org/10.5194/acp-5-1999-2005, 2005.
Albani, S., Mahowald, N. M., Perry, A. T., Scanza, R. A., Zender, C. S., Heavens, N. G., Maggi, V., Kok, J. F., and Otto-Bliesner, B. L.: Improved dust representation in the Community Atmosphere Model, J. Adv. Model. Earth Syst., 6, 541–570, https://doi.org/10.1002/2013MS000279, 2014.
Andela, N. and van der Werf, G. R.: Recent trends in African fires driven by cropland expansion and El Nino to La Nina transition, Nat. Clim. Change, 4, 791–795, https://doi.org/10.1038/nclimate2313, 2014.
Andela, N., Morton, D. C., Giglio, L., Chen, Y., van der Werf, G. R., Kasibhatla, P. S., DeFries, R. S., Collatz, G. J., Hantson, S., Kloster, S., Bachelet, D., Forrest, M., Lasslop, G., Li, F., Mangeon, S., Melton, J. R., Yue, C., and Randerson, J. T.: A human-driven decline in global burned area, Science, 356, 1356–1362, https://doi.org/10.1126/science.aal4108, 2017.
Andrews, T., Gregory, J. M., Webb, M. J., and Taylor, K. E.: Forcing, feedbacks and climate sensitivity in CMIP5 coupled atmosphere-ocean climate models, Geophys. Res. Lett., 39, L09712, https://doi.org/10.1029/2012gl051607, 2012.
Bala, G., Caldeira, K., Wickett, M., Phillips, T. J., Lobell, D. B., Delire, C., and Mirin, A.: Combined climate and carbon-cycle effects of large-scale deforestation, P. Natl. Acad. Sci. USA, 104, 6550–6555, https://doi.org/10.1073/pnas.0608998104, 2007.
Barbero, R., Abatzoglou, J. T., Larkin, N. K., Kolden, C. A., and Stocks, B.: Climate change presents increased potential for very large fires in the contiguous United States, Int. J. Wildland Fire, 24, 892–899, https://doi.org/10.1071/Wf15083, 2015.
Bowman, D. M. J. S., Balch, J. K., Artaxo, P., Bond, W. J., Carlson, J. M., Cochrane, M. A., D'Antonio, C. M., DeFries, R. S., Doyle, J. C., Harrison, S. P., Johnston, F. H., Keeley, J. E., Krawchuk, M. A., Kull, C. A., Marston, J. B., Moritz, M. A., Prentice, I. C., Roos, C. I., Scott, A. C., Swetnam, T. W., van der Werf, G. R., and Pyne, S. J.: Fire in the Earth System, Science, 324, 481–484, https://doi.org/10.1126/science.1163886, 2009.
Brown, H., Liu, X., Feng, Y., Jiang, Y., Wu, M., Lu, Z., Wu, C., Murphy, S., and Pokhrel, R.: Radiative effect and climate impacts of brown carbon with the Community Atmosphere Model (CAM5), Atmos. Chem. Phys., 18, 17745–17768, https://doi.org/10.5194/acp-18-17745-2018, 2018.
Ciais, P., Sabine, C., Bala, G., Bopp, L., Brovkin, V., Canadell, J., Chhabra, A., DeFries, R., Galloway, J., Heimann, M., Jones, C., Le Quéré, C., Myneni, R. B., and Thornton, P.: Carbon and Other Biogeochemical Cycles, in: Climate Change 2013: The Physical Science Basis. Contribution of Working Group I to the Fifth Assessment Report of the Intergovernmental Panel on Climate Change, edited by: Stocker, T. F., Qin, D., Plattner, G.-K., Tignor, M., Allen, S. K., Boschung, J., Nauels, A., Xia, Y., Bex, V., and Midgley, P. M., Cambridge University Press, Cambridge, UK and New York, NY, USA, 2013.
Clark, S. K., Ward, D. S., and Mahowald, N. M.: Parameterization-based uncertainty in future lightning flash density, Geophys. Res. Lett., 44, 2893–2901, https://doi.org/10.1002/2017GL073017, 2017.
Clarke, H., Lucas, C., and Smith, P.: Changes in Australian fire weather between 1973 and 2010, Int. J. Climatol., 33, 931–944, https://doi.org/10.1002/joc.3480, 2013.
Computational and Information Systems Laboratory (CISL): Cheyenne: HPE/SGI ICE XA System (University Community Computing), National Center for Atmospheric Research, Boulder, CO, USA, https://doi.org/10.5065/D6RX99HX, 2017.
Dennison, P. E., Brewer, S. C., Arnold, J. D., and Moritz, M. A.: Large wildfire trends in the western United States, 1984–2011, Geophys. Res. Lett., 41, 2928–2933, https://doi.org/10.1002/2014gl059576, 2014.
Dentener, F., Kinne, S., Bond, T., Boucher, O., Cofala, J., Generoso, S., Ginoux, P., Gong, S., Hoelzemann, J. J., Ito, A., Marelli, L., Penner, J. E., Putaud, J.-P., Textor, C., Schulz, M., van der Werf, G. R., and Wilson, J.: Emissions of primary aerosol and precursor gases in the years 2000 and 1750 prescribed data-sets for AeroCom, Atmos. Chem. Phys., 6, 4321–4344, https://doi.org/10.5194/acp-6-4321-2006, 2006.
Dunne, J. P., John, J. G., Adcroft, A. J., Griffies, S. M., Hallberg, R. W., Shevliakova, E., Stouffer, R. J., Cooke, W., Dunne, K. A., Harrison, M. J., Krasting, J. P., Malyshev, S. L., Milly, P. C. D., Phillipps, P. J., Sentman, L. T., Samuels, B. L., Spelman, M. J., Winton, M., Wittenberg, A. T., and Zadeh, N.: GFDL's ESM2 Global Coupled Climate-Carbon Earth System Models. Part I: Physical Formulation and Baseline Simulation Characteristics, J. Climate, 25, 6646–6665, https://doi.org/10.1175/Jcli-D-11-00560.1, 2012.
Dunne, J. P., John, J. G., Shevliakova, E., Stouffer, R. J., Krasting, J. P., Malyshev, S. L., Milly, P. C. D., Sentman, L. T., Adcroft, A. J., Cooke, W., Dunne, K. A., Griffies, S. M., Hallberg, R. W., Harrison, M. J., Levy, H., Wittenberg, A. T., Phillips, P. J., and Zadeh, N.: GFDL's ESM2 Global Coupled Climate-Carbon Earth System Models. Part II: Carbon System Formulation and Baseline Simulation Characteristics, J. Climate, 26, 2247–2267, https://doi.org/10.1175/Jcli-D-12-00150.1, 2013.
Fann, N., Alman, B., Broome, R. A., Morgan, G. G., Johnston, F. H., Pouliot, G., and Rappold, A. G.: The health impacts and economic value of wildland fire episodes in the US: 2008–2012, Sci. Total. Environ., 610, 802–809, https://doi.org/10.1016/j.scitotenv.2017.08.024, 2018.
Feng, Y., Ramanathan, V., and Kotamarthi, V. R.: Brown carbon: a significant atmospheric absorber of solar radiation?, Atmos. Chem. Phys., 13, 8607–8621, https://doi.org/10.5194/acp-13-8607-2013, 2013.
Finney, D. L., Doherty, R. M., Wild, O., Stevenson, D. S., MacKenzie, I. A., and Blyth, A. M.: A projected decrease in lightning under climate change, Nat. Clim. Change, 8, 210–213, https://doi.org/10.1038/s41558-018-0072-6, 2018.
Flanner, M. G. and Zender, C. S.: Snowpack radiative heating: Influence on Tibetan Plateau climate, Geophys. Res. Lett., 32, L06501, https://doi.org/10.1029/2004gl022076, 2005.
Flannigan, M., Cantin, A. S., de Groot, W. J., Wotton, M., Newbery, A., and Gowman, L. M.: Global wildland fire season severity in the 21st century, Forest Ecol. Manag., 294, 54–61, https://doi.org/10.1016/j.foreco.2012.10.022, 2013.
Flannigan, M. D., Krawchuk, M. A., de Groot, W. J., Wotton, B. M., and Gowman, L. M.: Implications of changing climate for global wildland fire, Int. J. Wildland Fire, 18, 483–507, https://doi.org/10.1071/Wf08187, 2009.
Forrister, H., Liu, J., Scheuer, E., Dibb, J., Ziemba, L., Thornhill, K. L., Anderson, B., Diskin, G., Perring, A. E., Schwarz, J. P., Campuzano-Jost, P., Day, D. A., Palm, B. B., Jimenez, J. L., Nenes, A., and Weber, R. J.: Evolution of brown carbon in wildfire plumes, Geophys. Res. Lett., 42, 4623–4630, https://doi.org/10.1002/2015gl063897, 2015.
Garcia, E. S., Swann, A. L. S., Villegas, J. C., Breshears, D. D., Law, D. J., Saleska, S. R., and Stark, S. C.: Synergistic Ecoclimate Teleconnections from Forest Loss in Different Regions Structure Global Ecological Responses, Plos One, 11, e0165042, https://doi.org/10.1371/journal.pone.0165042, 2016.
Gettelman, A., Morrison, H., and Ghan, S. J.: A new two-moment bulk stratiform cloud microphysics scheme in the community atmosphere model, version 3 (CAM3). Part II: Single-column and global results, J. Climate, 21, 3660–3679, https://doi.org/10.1175/2008jcli2116.1, 2008.
Ghan, S. J.: Technical Note: Estimating aerosol effects on cloud radiative forcing, Atmos. Chem. Phys., 13, 9971–9974, https://doi.org/10.5194/acp-13-9971-2013, 2013.
Giglio, L., Randerson, J. T., and van der Werf, G. R.: Analysis of daily, monthly, and annual burned area using the fourth-generation global fire emissions database (GFED4), J. Geophys. Res.-Biogeo., 118, 317–328, https://doi.org/10.1002/jgrg.20042, 2013 (data available at: https://www.geo.vu.nl/~gwerf/GFED/GFED4/, last access: 18 January 2019).
Gilardoni, S., Vignati, E., Marmer, E., Cavalli, F., Belis, C., Gianelle, V., Loureiro, A., and Artaxo, P.: Sources of carbonaceous aerosol in the Amazon basin, Atmos. Chem. Phys., 11, 2747–2764, https://doi.org/10.5194/acp-11-2747-2011, 2011.
Goldstein, A. H., Koven, C. D., Heald, C. L., and Fung, I. Y.: Biogenic carbon and anthropogenic pollutants combine to form a cooling haze over the southeastern United States, P. Natl. Acad. Sci. USA, 106, 8835–8840, https://doi.org/10.1073/pnas.0904128106, 2009.
Hall, J. R.: The total cost of fire in the United States, National Fire Protection Association, Quincy, MA, USA, 38 pp., 2014.
Hantson, S., Arneth, A., Harrison, S. P., Kelley, D. I., Prentice, I. C., Rabin, S. S., Archibald, S., Mouillot, F., Arnold, S. R., Artaxo, P., Bachelet, D., Ciais, P., Forrest, M., Friedlingstein, P., Hickler, T., Kaplan, J. O., Kloster, S., Knorr, W., Lasslop, G., Li, F., Mangeon, S., Melton, J. R., Meyn, A., Sitch, S., Spessa, A., van der Werf, G. R., Voulgarakis, A., and Yue, C.: The status and challenge of global fire modelling, Biogeosciences, 13, 3359–3375, https://doi.org/10.5194/bg-13-3359-2016, 2016.
Harris, R. M. B., Remenyi, T. A., Williamson, G. J., Bindoff, N. L., and Bowman, D. M. J. S.: Climate-vegetation-fire interactions and feedbacks: trivial detail or major barrier to projecting the future of the Earth system?, Wires Clim. Change, 7, 910–931, https://doi.org/10.1002/wcc.428, 2016.
Hazeleger, W., Severijns, C., Semmler, T., Stefanescu, S., Yang, S. T., Wang, X. L., Wyser, K., Dutra, E., Baldasano, J. M., Bintanja, R., Bougeault, P., Caballero, R., Ekman, A. M. L., Christensen, J. H., van den Hurk, B., Jimenez, P., Jones, C., Kallberg, P., Koenigk, T., McGrath, R., Miranda, P., Van Noije, T., Palmer, T., Parodi, J. A., Schmith, T., Selten, F., Storelvmo, T., Sterl, A., Tapamo, H., Vancoppenolle, M., Viterbo, P., and Willen, U.: EC-Earth A Seamless Earth-System Prediction Approach in Action, B. Am. Meteorol. Soc., 91, 1357–1363, https://doi.org/10.1175/2010bams2877.1, 2010.
Holben, B. N., Eck, T. F., Slutsker, I., Tanre, D., Buis, J. P., Setzer, A., Vermote, E., Reagan, J. A., Kaufman, Y. J., Nakajima, T., Lavenu, F., Jankowiak, I., and Smirnov, A.: AERONET – A federated instrument network and data archive for aerosol characterization, Remote Sens. Environ., 66, 1–16, https://doi.org/10.1016/S0034-4257(98)00031-5, 1998 (data available at: https://aeronet.gsfc.nasa.gov/, last access: 18 January 2019).
Horowitz, L. W., Walters, S., Mauzerall, D. L., Emmons, L. K., Rasch, P. J., Granier, C., Tie, X., Lamarque, J. F., Schultz, M. G., Tyndall, G. S., and Orlando, J. J.: A global simulation of tropospheric ozone and related tracers: Description and evaluation of MOZART, version 2. J. Geophys. Res.-Atmos., 108, 4784, https://doi.org/10.1029/2002jd002853, 2003.
Hurrell, J. W., Holland, M. M., Gent, P. R., Ghan, S., Kay, J. E., Kushner, P. J., Lamarque, J. F., Large, W. G., Lawrence, D., Lindsay, K., Lipscomb, W. H., Long, M. C., Mahowald, N., Marsh, D. R., Neale, R. B., Rasch, P., Vavrus, S., Vertenstein, M., Bader, D., Collins, W. D., Hack, J. J., Kiehl, J., and Marshall, S.: The Community Earth System Model A Framework for Collaborative Research, B. Am. Meteorol. Soc., 94, 1339–1360, https://doi.org/10.1175/Bams-D-12-00121.1, 2013.
Hurteau, M. D., Westerling, A. L., Wiedinmyer, C., and Bryant, B. P.: Projected Effects of Climate and Development on California Wildfire Emissions through 2100, Environ. Sci. Technol., 48, 2298–2304, https://doi.org/10.1021/es4050133, 2014.
Hurtt, G. C., Frolking, S., Fearon, M. G., Moore, B., Shevliakova, E., Malyshev, S., Pacala, S. W., and Houghton, R. A.: The underpinnings of land-use history: three centuries of global gridded land-use transitions, wood-harvest activity, and resulting secondary lands, Glob. Change Biol., 12, 1208–1229, https://doi.org/10.1111/j.1365-2486.2006.01150.x, 2006.
Jiang, Y., Lu, Z., Liu, X., Qian, Y., Zhang, K., Wang, Y., and Yang, X.-Q.: Impacts of global open-fire aerosols on direct radiative, cloud and surface-albedo effects simulated with CAM5, Atmos. Chem. Phys., 16, 14805–14824, https://doi.org/10.5194/acp-16-14805-2016, 2016.
Jin, Y. F., Randerson, J. T., Goetz, S. J., Beck, P. S. A., Loranty, M. M., and Goulden, M. L.: The influence of burn severity on postfire vegetation recovery and albedo change during early succession in North American boreal forests, J. Geophys. Res.-Biogeo., 117, G01036, https://doi.org/10.1029/2011jg001886, 2012.
Johnston, F. H., Henderson, S. B., Chen, Y., Randerson, J. T., Marlier, M., DeFries, R. S., Kinney, P., Bowman, D. M. J. S., and Brauer, M.: Estimated Global Mortality Attributable to Smoke from Landscape Fires, Environ. Health Persp., 120, 695–701, https://doi.org/10.1289/ehp.1104422, 2012.
Jolly, W. M., Cochrane, M. A., Freeborn, P. H., Holden, Z. A., Brown, T. J., Williamson, G. J., and Bowman, D. M. J. S.: Climate-induced variations in global wildfire danger from 1979 to 2013, Nat. Commun., 6, 7537, https://doi.org/10.1038/ncomms8537, 2015.
Ke, Z., Wang, Y., Zou, Y., Song, Y., and Liu, Y.: The global plume-rise dataset and its climate model implement, in preparation, 2020.
Kloster, S., Mahowald, N. M., Randerson, J. T., Thornton, P. E., Hoffman, F. M., Levis, S., Lawrence, P. J., Feddema, J. J., Oleson, K. W., and Lawrence, D. M.: Fire dynamics during the 20th century simulated by the Community Land Model, Biogeosciences, 7, 1877–1902, https://doi.org/10.5194/bg-7-1877-2010, 2010.
Kloster, S., Mahowald, N. M., Randerson, J. T., and Lawrence, P. J.: The impacts of climate, land use, and demography on fires during the 21st century simulated by CLM-CN, Biogeosciences, 9, 509–525, https://doi.org/10.5194/bg-9-509-2012, 2012.
Kurokawa, J., Ohara, T., Morikawa, T., Hanayama, S., Janssens-Maenhout, G., Fukui, T., Kawashima, K., and Akimoto, H.: Emissions of air pollutants and greenhouse gases over Asian regions during 2000–2008: Regional Emission inventory in ASia (REAS) version 2, Atmos. Chem. Phys., 13, 11019–11058, https://doi.org/10.5194/acp-13-11019-2013, 2013.
Lamarque, J.-F., Bond, T. C., Eyring, V., Granier, C., Heil, A., Klimont, Z., Lee, D., Liousse, C., Mieville, A., Owen, B., Schultz, M. G., Shindell, D., Smith, S. J., Stehfest, E., Van Aardenne, J., Cooper, O. R., Kainuma, M., Mahowald, N., McConnell, J. R., Naik, V., Riahi, K., and van Vuuren, D. P.: Historical (1850–2000) gridded anthropogenic and biomass burning emissions of reactive gases and aerosols: methodology and application, Atmos. Chem. Phys., 10, 7017–7039, https://doi.org/10.5194/acp-10-7017-2010, 2010.
Le Quéré, C., Andres, R. J., Boden, T., Conway, T., Houghton, R. A., House, J. I., Marland, G., Peters, G. P., van der Werf, G. R., Ahlström, A., Andrew, R. M., Bopp, L., Canadell, J. G., Ciais, P., Doney, S. C., Enright, C., Friedlingstein, P., Huntingford, C., Jain, A. K., Jourdain, C., Kato, E., Keeling, R. F., Klein Goldewijk, K., Levis, S., Levy, P., Lomas, M., Poulter, B., Raupach, M. R., Schwinger, J., Sitch, S., Stocker, B. D., Viovy, N., Zaehle, S., and Zeng, N.: The global carbon budget 1959–2011, Earth Syst. Sci. Data, 5, 165–185, https://doi.org/10.5194/essd-5-165-2013, 2013.
Li, F., Levis, S., and Ward, D. S.: Quantifying the role of fire in the Earth system – Part 1: Improved global fire modeling in the Community Earth System Model (CESM1), Biogeosciences, 10, 2293–2314, https://doi.org/10.5194/bg-10-2293-2013, 2013.
Li, F., Bond-Lamberty, B., and Levis, S.: Quantifying the role of fire in the Earth system – Part 2: Impact on the net carbon balance of global terrestrial ecosystems for the 20th century, Biogeosciences, 11, 1345–1360, https://doi.org/10.5194/bg-11-1345-2014, 2014.
Liu, J., Scheuer, E., Dibb, J., Diskin, G. S., Ziemba, L. D., Thornhill, K. L., Anderson, B. E., Wisthaler, A., Mikoviny, T., Devi, J. J., Bergin, M., Perring, A. E., Markovic, M. Z., Schwarz, J. P., Campuzano-Jost, P., Day, D. A., Jimenez, J. L., and Weber, R. J.: Brown carbon aerosol in the North American continental troposphere: sources, abundance, and radiative forcing, Atmos. Chem. Phys., 15, 7841–7858, https://doi.org/10.5194/acp-15-7841-2015, 2015.
Liu, X., Easter, R. C., Ghan, S. J., Zaveri, R., Rasch, P., Shi, X., Lamarque, J.-F., Gettelman, A., Morrison, H., Vitt, F., Conley, A., Park, S., Neale, R., Hannay, C., Ekman, A. M. L., Hess, P., Mahowald, N., Collins, W., Iacono, M. J., Bretherton, C. S., Flanner, M. G., and Mitchell, D.: Toward a minimal representation of aerosols in climate models: description and evaluation in the Community Atmosphere Model CAM5, Geosci. Model Dev., 5, 709–739, https://doi.org/10.5194/gmd-5-709-2012, 2012.
Liu, Y., Zhang, K., Qian, Y., Wang, Y., Zou, Y., Song, Y., Wan, H., Liu, X., and Yang, X.-Q.: Investigation of short-term effective radiative forcing of fire aerosols over North America using nudged hindcast ensembles, Atmos. Chem. Phys., 18, 31–47, https://doi.org/10.5194/acp-18-31-2018, 2018.
Liu, Y. Q.: New development and application needs for Earth system modeling of fire-climate-ecosystem interactions, Environ. Res. Lett., 13, 011001, https://doi.org/10.1088/1748-9326/aaa347, 2018.
Liu, Y. Q., Stanturf, J., and Goodrick, S.: Trends in global wildfire potential in a changing climate, Forest Ecol. Manag., 259, 685–697, https://doi.org/10.1016/j.foreco.2009.09.002, 2010.
Lu, Z., Liu, X., Zhang, Z., Zhao, C., Meyer, K., Rajapakshe, C., Wu, C., Yang, Z., and Penner, J. E.: Biomass smoke from southern Africa can significantly enhance the brightness of stratocumulus over the southeastern Atlantic Ocean, P. Natl. Acad. Sci. USA, 115, 2924–2929, https://doi.org/10.1073/pnas.1713703115, 2018.
Markandya, A., Sampedro, J., Smith, S. J., Dingenen, R. V., Pizarro-Irizar, C., Arto, I., and González-Eguino, M.: Health co-benefits from air pollution and mitigation costs of the Paris Agreement: a modelling study, The Lancet Planetary Health, 2, e126–e133, https://doi.org/10.1016/S2542-5196(18)30029-9, 2018.
McClure, C. D. and Jaffe, D. A.: US particulate matter air quality improves except in wildfire-prone areas, P. Natl. Acad. Sci. USA, 115, 7901–7906, https://doi.org/10.1073/pnas.1804353115, 2018.
Moritz, M. A., Parisien, M. A., Batllori, E., Krawchuk, M. A., Van Dorn, J., Ganz, D. J., and Hayhoe, K.: Climate change and disruptions to global fire activity, Ecosphere, 3, Unsp49, https://doi.org/10.1890/Es11-00345.1, 2012.
Morrison, H. and Gettelman, A.: A new two-moment bulk stratiform cloud microphysics scheme in the community atmosphere model, version 3 (CAM3). Part I: Description and numerical tests, J. Climate, 21, 3642–3659, https://doi.org/10.1175/2008jcli2105.1, 2008.
Neale, R. B., Chen, C. C., Gettelman, A., Lauritzen, P. H., Park, S., Williamson, D. L., Conley, A. J., Garcia, R., Kinnison, D., Lamarque, J. F., Marsh, D., Mills, M., Smith, A. K., Tilmes, S., Vitt, F., Morrison, H., Cameron-Smith, P., Collins, W. D., Iacono, M. J., Easter, R. C., Ghan, S. J., Liu, X. H., Rasch, P. J., and Taylor, M. A.: Description of the NCAR Community Atmosphere Model (CAM 5.0), NCAR Tech. Note NCAR/TN-486+STR, Natl. Cent. for Atmos. Res., Boulder, CO, USA, 289 pp., 2012.
Oleson, K. W., Lawrence, D. M., Bonan, G. B., Drewniak, B., Huang, M., Koven, C. D., Levis, S., Li, F., Riley, W. J., Subin, Z. M., Swenson, S. C., Thornton, P. E., Bozbiyik, A., Fisher, R., Heald, C. L., Kluzek, E., Lamarque, J.-F., Lawrence, P. J., Leung, L. R., Lipscomb, W., Muszala, S., Ricciuto, D. M., Sacks, W., Sun, Y., Tang, J., and Yang, Z.-L.: Technical description of version 4.5 of the Community Land Model (CLM), NCAR Tech. Note NCAR/TN-503+STR, Natl. Cent. for Atmos. Res., Boulder, CO, USA, 434 pp., 2013.
Park, S., Bretherton, C. S., and Rasch, P. J.: Integrating Cloud Processes in the Community Atmosphere Model, Version 5, J. Climate, 27, 6821–6856, https://doi.org/10.1175/Jcli-D-14-00087.1, 2014.
Parks, S. A., Miller, C., Abatzoglou, J. T., Holsinger, L. M., Parisien, M. A., and Dobrowski, S. Z.: How will climate change affect wildland fire severity in the western US?, Environ. Res. Lett., 11, 035002, https://doi.org/10.1088/1748-9326/11/3/035002, 2016.
Pellegrini, A. F. A., Ahlstrom, A., Hobbie, S. E., Reich, P. B., Nieradzik, L. P., Staver, A. C., Scharenbroch, B. C., Jumpponen, A., Anderegg, W. R. L., Randerson, J. T., and Jackson, R. B.: Fire frequency drives decadal changes in soil carbon and nitrogen and ecosystem productivity, Nature, 553, 194–198, https://doi.org/10.1038/nature24668, 2018.
Piao, S. L., Sitch, S., Ciais, P., Friedlingstein, P., Peylin, P., Wang, X. H., Ahlstrom, A., Anav, A., Canadell, J. G., Cong, N., Huntingford, C., Jung, M., Levis, S., Levy, P. E., Li, J. S., Lin, X., Lomas, M. R., Lu, M., Luo, Y. Q., Ma, Y. C., Myneni, R. B., Poulter, B., Sun, Z. Z., Wang, T., Viovy, N., Zaehle, S., and Zeng, N.: Evaluation of terrestrial carbon cycle models for their response to climate variability and to CO2 trends, Glob. Change Biol., 19, 2117–2132, https://doi.org/10.1111/gcb.12187, 2013.
Platnick, S., Hubanks, P., Meyer, K., and King, M. D.: MODIS Atmosphere L3 Monthly Product. NASA MODIS Adaptive Processing System, Goddard Space Flight Center, USA, https://doi.org/10.5067/MODIS/MYD08_M3.061, 2015.
Quillet, A., Peng, C., and Garneau, M.: Toward dynamic global vegetation models for simulating vegetation–climate interactions and feedbacks: recent developments, limitations, and future challenges, Environ. Rev., 18, 333–353, https://doi.org/10.1139/A10-016, 2010.
Randerson, J. T., Liu, H., Flanner, M. G., Chambers, S. D., Jin, Y., Hess, P. G., Pfister, G., Mack, M. C., Treseder, K. K., Welp, L. R., Chapin, F. S., Harden, J. W., Goulden, M. L., Lyons, E., Neff, J. C., Schuur, E. A. G., and Zender, C. S.: The impact of boreal forest fire on climate warming, Science, 314, 1130–1132, https://doi.org/10.1126/science.1132075, 2006.
Randerson, J. T., Chen, Y., van der Werf, G. R., Rogers, B. M., and Morton, D. C.: Global burned area and biomass burning emissions from small fires, J. Geophys. Res.-Biogeo., 117, G04012, https://doi.org/10.1029/2012jg002128, 2012.
Rayner, N. A., Parker, D. E., Horton, E. B., Folland, C. K., Alexander, L. V., Rowell, D. P., Kent, E. C., and Kaplan, A.: Global analyses of sea surface temperature, sea ice, and night marine air temperature since the late nineteenth century, J. Geophys. Res.-Atmos., 108, 4407, https://doi.org/10.1029/2002jd002670, 2003.
Riahi, K., Van Vuuren, D. P., Kriegler, E., Edmonds, J., O'neill, B. C., Fujimori, S., Bauer, N., Calvin, K., Dellink, R., Fricko, O., and Lutz, W.: The shared socioeconomic pathways and their energy, land use, and greenhouse gas emissions implications: an overview, Global Environ. Chang., 42, 153–168, https://doi.org/10.1016/j.gloenvcha.2016.05.009, 2017.
Richardson, L. A., Champ, P. A., and Loomis, J. B.: The hidden cost of wildfires: Economic valuation of health effects of wildfire smoke exposure in Southern California, J. Forest. Econ., 18, 14–35, https://doi.org/10.1016/j.jfe.2011.05.002, 2012.
Rosenfeld, D., Zhu, Y. N., Wang, M. H., Zheng, Y. T., Goren, T., and Yu, S. C.: Aerosol-driven droplet concentrations dominate coverage and water of oceanic low-level clouds, Science, 363, eaav0566, https://doi.org/10.1126/science.aav0566, 2019.
Seidl, R., Thom, D., Kautz, M., Martin-Benito, D., Peltoniemi, M., Vacchiano, G., Wild, J., Ascoli, D., Petr, M., Honkaniemi, J., Lexer, M. J., Trotsiuk, V., Mairota, P., Svoboda, M., Fabrika, M., Nagel, T. A., and Reyer, C. P. O.: Forest disturbances under climate change, Nat. Clim. Change, 7, 395–402, https://doi.org/10.1038/Nclimate3303, 2017.
Seinfeld, J. H., Bretherton, C., Carslaw, K. S., Coe, H., DeMott, P. J., Dunlea, E. J., Feingold, G., Ghan, S., Guenther, A. B., Kahn, R., and Kraucunas, I.: Improving our fundamental understanding of the role of aerosol–cloud interactions in the climate system, P. Natl. Acad. Sci. USA, 113, 5781–5790, 2016.
Seo, H. and Kim, Y.: Interactive impacts of fire and vegetation dynamics on global carbon and water budget using Community Land Model version 4.5, Geosci. Model Dev., 12, 457–472, https://doi.org/10.5194/gmd-12-457-2019, 2019.
Shuman, J. K., Foster, A. C., Shugart, H. H., Hoffman-Hall, A., Krylov, A., Loboda, T., Ershov, D., and Sochilova, E.: Fire disturbance and climate change: implications for Russian forests, Environ. Res. Lett., 12, 035003, https://doi.org/10.1088/1748-9326/aa5eed, 2017.
Sofiev, M., Ermakova, T., and Vankevich, R.: Evaluation of the smoke-injection height from wild-land fires using remote-sensing data, Atmos. Chem. Phys., 12, 1995–2006, https://doi.org/10.5194/acp-12-1995-2012, 2012.
Stark, S. C., Breshears, D. D., Garcia, E. S., Law, D. J., Minor, D. M., Saleska, S. R., Swann, A. L. S., Villegas, J. C., Aragao, L. E. O. C., Bella, E. M., Borma, L. S., Cobb, N. S., Litvak, M. E., Magnusson, W. E., Morton, J. M., and Redmond, M. D.: Toward accounting for ecoclimate teleconnections: intra- and inter-continental consequences of altered energy balance after vegetation change, Landscape Ecol., 31, 181–194, https://doi.org/10.1007/s10980-015-0282-5, 2016.
Sun, Y., Gu, L. H., and Dickinson, R. E.: A numerical issue in calculating the coupled carbon and water fluxes in a climate model, J. Geophys. Res.-Atmos., 117, D22103, https://doi.org/10.1029/2012jd018059, 2012.
Thomas, D., Butry, D., Gilbert, S., Webb, D., and Fung, J.: The costs and losses of wildfires: A literature review, National Institute of Standards and Technology Special Publication 1215, 72 pp., https://doi.org/10.6028/NIST.SP.1215, 2017.
Thonicke, K., Spessa, A., Prentice, I. C., Harrison, S. P., Dong, L., and Carmona-Moreno, C.: The influence of vegetation, fire spread and fire behaviour on biomass burning and trace gas emissions: results from a process-based model, Biogeosciences, 7, 1991–2011, https://doi.org/10.5194/bg-7-1991-2010, 2010.
Tosca, M. G., Randerson, J. T., and Zender, C. S.: Global impact of smoke aerosols from landscape fires on climate and the Hadley circulation, Atmos. Chem. Phys., 13, 5227–5241, https://doi.org/10.5194/acp-13-5227-2013, 2013.
Tost, H., Jöckel, P., and Lelieveld, J.: Lightning and convection parameterisations – uncertainties in global modelling, Atmos. Chem. Phys., 7, 4553–4568, https://doi.org/10.5194/acp-7-4553-2007, 2007.
United States Environmental Protection Agency (US EPA): Particulate Matter (PM2.5) trends, available at: https://www.epa.gov/air-trends/particulate-matter-pm25-trends, last access: 19 February 2019.
Val Martin, M., Logan, J. A., Kahn, R. A., Leung, F.-Y., Nelson, D. L., and Diner, D. J.: Smoke injection heights from fires in North America: analysis of 5 years of satellite observations, Atmos. Chem. Phys., 10, 1491–1510, https://doi.org/10.5194/acp-10-1491-2010, 2010.
van der Werf, G. R., Randerson, J. T., Giglio, L., Collatz, G. J., Kasibhatla, P. S., and Arellano Jr., A. F.: Interannual variability in global biomass burning emissions from 1997 to 2004, Atmos. Chem. Phys., 6, 3423–3441, https://doi.org/10.5194/acp-6-3423-2006, 2006.
van der Werf, G. R., Randerson, J. T., Giglio, L., van Leeuwen, T. T., Chen, Y., Rogers, B. M., Mu, M., van Marle, M. J. E., Morton, D. C., Collatz, G. J., Yokelson, R. J., and Kasibhatla, P. S.: Global fire emissions estimates during 1997–2016, Earth Syst. Sci. Data, 9, 697–720, https://doi.org/10.5194/essd-9-697-2017, 2017.
Wang, J. D., Zhao, B., Wang, S. X., Yang, F. M., Xing, J., Morawska, L., Ding, A. J., Kulmala, M., Kerminen, V. M., Kujansuu, J., Wang, Z. F., Ding, D. A., Zhang, X. Y., Wang, H. B., Tian, M., Petaja, T., Jiang, J. K., and Hao, J. M.: Particulate matter pollution over China and the effects of control policies, Sci. Total Environ., 584, 426–447, https://doi.org/10.1016/j.scitotenv.2017.01.027, 2017.
Wang, X., Heald, C. L., Liu, J., Weber, R. J., Campuzano-Jost, P., Jimenez, J. L., Schwarz, J. P., and Perring, A. E.: Exploring the observational constraints on the simulation of brown carbon, Atmos. Chem. Phys., 18, 635–653, https://doi.org/10.5194/acp-18-635-2018, 2018.
Ward, D. S., Kloster, S., Mahowald, N. M., Rogers, B. M., Randerson, J. T., and Hess, P. G.: The changing radiative forcing of fires: global model estimates for past, present and future, Atmos. Chem. Phys., 12, 10857–10886, https://doi.org/10.5194/acp-12-10857-2012, 2012.
Westerling, A. L., Hidalgo, H. G., Cayan, D. R., and Swetnam, T. W.: Warming and earlier spring increase western US forest wildfire activity, Science, 313, 940–943, https://doi.org/10.1126/science.1128834, 2006.
Wotton, B. M., Flannigan, M. D., and Marshall, G. A.: Potential climate change impacts on fire intensity and key wildfire suppression thresholds in Canada, Environ. Res. Lett., 12, 095003, https://doi.org/10.1088/1748-9326/aa7e6e, 2017.
Yang, G., Di, X. Y., Guo, Q. X., Shu, Z., Zeng, T., Yu, H. Z., and Wang, C.: The impact of climate change on forest fire danger rating in China's boreal forest, J. Forest Res., 22, 249–257, https://doi.org/10.1007/s11676-011-0158-8, 2011.
Yang, J., Tian, H. Q., Tao, B., Ren, W., Pan, S. F., Liu, Y. Q., and Wang, Y. H.: A growing importance of large fires in conterminous United States during 1984-2012, J. Geophys. Res.-Biogeo., 120, 2625–2640, https://doi.org/10.1002/2015jg002965, 2015.
Young, A. M., Higuera, P. E., Duffy, P. A., and Hu, F. S.: Climatic thresholds shape northern high-latitude fire regimes and imply vulnerability to future climate change, Ecography, 40, 606–617, https://doi.org/10.1111/ecog.02205, 2017.
Yue, C., Ciais, P., Cadule, P., Thonicke, K., and van Leeuwen, T. T.: Modelling the role of fires in the terrestrial carbon balance by incorporating SPITFIRE into the global vegetation model ORCHIDEE – Part 2: Carbon emissions and the role of fires in the global carbon balance, Geosci. Model Dev., 8, 1321–1338, https://doi.org/10.5194/gmd-8-1321-2015, 2015.
Yue, C., Ciais, P., Zhu, D., Wang, T., Peng, S. S., and Piao, S. L.: How have past fire disturbances contributed to the current carbon balance of boreal ecosystems?, Biogeosciences, 13, 675–690, https://doi.org/10.5194/bg-13-675-2016, 2016.
Yue, X., Mickley, L. J., Logan, J. A., and Kaplan, J. O.: Ensemble projections of wildfire activity and carbonaceous aerosol concentrations over the western United States in the mid-21st century, Atmos. Environ., 77, 767–780, https://doi.org/10.1016/j.atmosenv.2013.06.003, 2013.
Zhang, A., Wang, Y., Zhang, Y., Weber, R. J., Song, Y., Ke, Z., and Zou, Y.: Modeling global radiative effect of brown carbon: A larger heating source in the tropical free troposphere than black carbon, Atmos. Chem. Phys. Discuss., https://doi.org/10.5194/acp-2019-594, in review, 2019.
Zhang, Y., West, J. J., Mathur, R., Xing, J., Hogrefe, C., Roselle, S. J., Bash, J. O., Pleim, J. E., Gan, C.-M., and Wong, D. C.: Long-term trends in the ambient PM2.5- and O3-related mortality burdens in the United States under emission reductions from 1990 to 2010, Atmos. Chem. Phys., 18, 15003–15016, https://doi.org/10.5194/acp-18-15003-2018, 2018.
Zhang, Y. Z., Forrister, H., Liu, J. M., Dibb, J., Anderson, B., Schwarz, J. P., Perring, A. E., Jimenez, J. L., Campuzano-Jost, P., Wang, Y. H., Nenes, A., and Weber, R. J.: Top-of-atmosphere radiative forcing affected by brown carbon in the upper troposphere, Nat. Geosci., 10, 486–489, https://doi.org/10.1038/Ngeo2960, 2017.
Zhang, Z., Meyer, K., Yu, H., Platnick, S., Colarco, P., Liu, Z., and Oreopoulos, L.: Shortwave direct radiative effects of above-cloud aerosols over global oceans derived from 8 years of CALIOP and MODIS observations, Atmos. Chem. Phys., 16, 2877–2900, https://doi.org/10.5194/acp-16-2877-2016, 2016.
Zhao, M. S. and Running, S. W.: Drought-Induced Reduction in Global Terrestrial Net Primary Production from 2000 Through 2009, Science, 329, 940–943, https://doi.org/10.1126/science.1192666, 2010.
Zhao, M. S., Heinsch, F. A., Nemani, R. R., and Running, S. W.: Improvements of the MODIS terrestrial gross and net primary production global data set, Remote Sens. Environ., 95, 164–176, https://doi.org/10.1016/j.rse.2004.12.011, 2005.
Zhao, Y., Zhang, J., and Nielsen, C. P.: The effects of energy paths and emission controls and standards on future trends in China's emissions of primary air pollutants, Atmos. Chem. Phys., 14, 8849–8868, https://doi.org/10.5194/acp-14-8849-2014, 2014.
Zou, Y.: CESM-RESFire fire simulation results, figshare, https://doi.org/10.6084/m9.figshare.9765356, 2020.
Zou, Y., Wang, Y., Ke, Z., Tian, H., Yang, J., and Liu, Y.: Development of a REgion-Specific ecosystem feedback Fire (RESFire) model in the Community Earth System Model, J. Adv. Model. Earth Syst., 11, 417–445, https://doi.org/10.1029/2018MS001368, 2019.