the Creative Commons Attribution 4.0 License.
the Creative Commons Attribution 4.0 License.
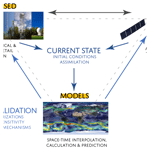
Overview: Integrative and Comprehensive Understanding on Polar Environments (iCUPE) – concept and initial results
Ella-Maria Duplissy
Ksenia Tabakova
Julia Schmale
Barbara Altstädter
Gerard Ancellet
Mikhail Arshinov
Yurii Balin
Urs Baltensperger
Jens Bange
Alison Beamish
Boris Belan
Antoine Berchet
Rossana Bossi
Warren R. L. Cairns
Ralf Ebinghaus
Imad El Haddad
Beatriz Ferreira-Araujo
Anna Franck
Lin Huang
Antti Hyvärinen
Angelika Humbert
Athina-Cerise Kalogridis
Pavel Konstantinov
Astrid Lampert
Matthew MacLeod
Olivier Magand
Alexander Mahura
Louis Marelle
Vladimir Masloboev
Dmitri Moisseev
Vaios Moschos
Niklas Neckel
Tatsuo Onishi
Stefan Osterwalder
Aino Ovaska
Pauli Paasonen
Mikhail Panchenko
Fidel Pankratov
Jakob B. Pernov
Andreas Platis
Olga Popovicheva
Jean-Christophe Raut
Aurélie Riandet
Torsten Sachs
Rosamaria Salvatori
Roberto Salzano
Ludwig Schröder
Martin Schön
Vladimir Shevchenko
Henrik Skov
Jeroen E. Sonke
Andrea Spolaor
Vasileios K. Stathopoulos
Mikko Strahlendorff
Jennie L. Thomas
Vito Vitale
Sterios Vratolis
Carlo Barbante
Sabine Chabrillat
Aurélien Dommergue
Konstantinos Eleftheriadis
Jyri Heilimo
Kathy S. Law
Andreas Massling
Steffen M. Noe
Jean-Daniel Paris
André S. H. Prévôt
Ilona Riipinen
Birgit Wehner
Zhiyong Xie
Hanna K. Lappalainen
The role of polar regions is increasing in terms of megatrends such as globalization, new transport routes, demography, and the use of natural resources with consequent effects on regional and transported pollutant concentrations. We set up the ERA-PLANET Strand 4 project “iCUPE – integrative and Comprehensive Understanding on Polar Environments” to provide novel insights and observational data on global grand challenges with an Arctic focus. We utilize an integrated approach combining in situ observations, satellite remote sensing Earth observations (EOs), and multi-scale modeling to synthesize data from comprehensive long-term measurements, intensive campaigns, and satellites to deliver data products, metrics, and indicators to stakeholders concerning the environmental status, availability, and extraction of natural resources in the polar areas. The iCUPE work consists of thematic state-of-the-art research and the provision of novel data in atmospheric pollution, local sources and transboundary transport, the characterization of arctic surfaces and their changes, an assessment of the concentrations and impacts of heavy metals and persistent organic pollutants and their cycling, the quantification of emissions from natural resource extraction, and the validation and optimization of satellite Earth observation (EO) data streams. In this paper we introduce the iCUPE project and summarize initial results arising out of the integration of comprehensive in situ observations, satellite remote sensing, and multi-scale modeling in the Arctic context.
- Article
(23943 KB) - Full-text XML
- BibTeX
- EndNote
The project “iCUPE – integrative and Comprehensive Understanding on Polar Environments” is motivated by the increasing role of Arctic regions in terms of megatrends such as globalization, new transport routes, demography, and the use of natural resources. These megatrends will rapidly and drastically affect the environment. In particular, the Arctic will face such grand challenges as soil and water pollution, climate change, land use change, higher demand for resource extraction, increased anthropogenic emissions due to year-round shipping in the Arctic Ocean and other local sources, and long-range-transported pollution from Europe, Asia, and North America (e.g., Buixadé Farré et al., 2014). Overall, land and ocean areas located in the polar latitudes are currently undergoing and will undergo substantial changes due to increased anthropogenic activities and shipping during the next decades. These activities will put the fragile Arctic environment and the population living in this area in a vulnerable position. The changes will pose unpredictable consequences for food chains, biodiversity, and the primary production of different plant ecosystems and ecosystem capacity to recover from pollution exposure and environmental changes (e.g., Arnold et al., 2016).
The future warming of the Arctic will affect demographic trends by increasing urbanization and migration to northern regions and by accelerating changes in societal issues and air quality (Schmale et al., 2018). One major consequence of warming in the northern latitudes is related to changes in the cryosphere, including ice sheet mass loss (Helm et al., 2014), the thawing of permafrost, and the Arctic Ocean becoming sea-ice-free part of the year (Kokelj et al., 2017; Meier et al., 2014; Kulmala et al., 2015; Lappalainen et al., 2016; Boy et al., 2019). Even with limiting global warming to 1.5 or 2 ∘C, temperatures over the high Arctic, in particular north Greenland, will rise by 3–4 ∘C by 2100 due to polar amplification, enhancing impacts like mass loss of the ice sheet (Rückamp et al., 2018). This will accelerate global trade activities in the Arctic region; then the northern sea route will likely be seasonally opened for shipping between the Atlantic and Asia's Far East. Northern ecosystems and Arctic regions are a source of major natural resources such as oil, natural gas, and minerals. The availability and exploitation of natural resources also depends on how significantly the permafrost thaw will damage existing infrastructure.
Human activities have had a profound impact on the composition of the atmosphere and pollution in the environment through the introduction of increasing quantities of heavy metals and other trace elements (Barbante et al., 2001), radioactive nuclides (Ežerinkis et al., 2014), synthesized organic compounds (Hermanson et al., 2010), aerosols such as black carbon (McConnell and Edwards, 2008), trace gases, and greenhouse gases. Anthropogenic contaminants can be transported over long distances and accumulate in polar areas. Persistent organic pollutants (POPs), such as polybrominated diphenyl ethers (PBDEs), polycyclic aromatic hydrocarbons (PAHs), polychlorinated biphenyl (PCB), and persistent contaminants of emerging concern (CECs) (Sauvé and Desrosiers, 2014), are rarely produced in the Arctic but have been found in Arctic wildlife, lake sediments, annual snow, and ice (Herbert et al., 2005; Ma et al., 2011; Seki et al., 2015). Mercury and other heavy metals, such as As, Cd, and Pb, are considered toxic at any level. Their presence is generally determined by local geochemistry, but they can be emitted by human activities, resulting in their increased abundance in polar areas (Barbante et al., 2001; Zheng et al., 2015; Angot et al., 2016). Black carbon (BC), a fine component of almost pure carbon from incomplete combustion, is able to modify the snow albedo by absorbing incoming solar radiation (Bond et al., 2013; Jiao et al., 2014). Human activities are impacting the net abundance of these pollutants in the atmosphere, but there is a lack of data exploring the deposition patterns and the abundance of anthropogenic contaminants in polar areas. We also need an improved understanding of their redistribution into different environmental spheres, including the biota of the Arctic and Antarctic, and the full life cycle of these pollutants (Wöhrnschimmel et al., 2013).
Local emissions currently make only a small contribution to the atmospheric loadings of various pollutants in polar areas, but this might change in the near future as Arctic ice-free areas will extend and more extensive Arctic shipping will become possible (Corbett et al., 2010; Yumashev et al., 2018). Nevertheless, air pollutants from other areas in the world do reach high-Arctic regions and have been estimated to have significant impacts on the regional ecosystem and climate (Di Pierro et al., 2011; Breider et al., 2014). Knowledge of the source contribution of atmospheric pollution is very limited, and further efforts in terms of detailed source identification are urgently needed to formulate and settle mitigation strategies (e.g., Law et al., 2014). Measurements of short-lived climate forcers (SLCFs) and their precursors are necessary for evaluating the impacts of increased regional and international activities, e.g., in relation to natural resource extraction, especially in fragile Arctic environments. Correspondingly, similar activities need to be carried out in Antarctica, which has a minimal amount of anthropogenic influence and can provide clean reference observations.
The existing observational networks with comprehensive in situ observational capacity for measurements of the atmospheric concentrations of air pollutants extend to Arctic and Antarctic environments (e.g., Uttal et al., 2016). However, there are still large gaps in the current measurement networks (Lappalainen et al., 2016), and the interaction between the networks, made up of different national activities, needs to be improved. Polar activities are often based on national activities and missing synergistic benefits of cooperation in challenging environments. In summary, the methodology of data acquisition, data quality control, and future strategies on data flows and data streams are not harmonized on either the European or global scale. Furthermore, particularly in the polar areas, measurements are not always continuous but often carried out campaign-wise due to economic, environmental, or logistical challenges.
Satellite remote sensing in the Arctic is based on active and passive missions of varying spatial resolution, repeat visit times, and coverage of high latitudes. Monitoring the surface properties and their variations in the Arctic region is a powerful tool to assess the impacts of changes induced in this vulnerable environment. The distribution of different types of land cover (snow, ice, vegetation, soil) can be efficiently analyzed using optical data obtained from the new satellite missions merged with data collected during field campaigns and data acquired from cooperative observer networks. In particular, different patterns of snow cover (as well as soil and vegetation cover) exercise considerable influence on the surface energy balance, since variations in land cover change the surface albedo. While there are established methods for retrieving basic variables, changing snow and ice surfaces and ice-free areas are still challenging, and the large variability of the system itself is limiting the accuracy of such retrievals (Bokhorst et al., 2016). New Sentinel series of Copernicus sensors in orbit make it possible to retrieve improved land surface variables due to increased capacities in terms of spatial, temporal, spectral, and angular observations. As a consequence, new Earth observation (EO) techniques will lead to the multi-mission time series needed for data assimilation into models of Earth system compartments in the Arctic.
In summary, in order to address the current state of the environment in polar areas and to provide fact-based decision-making tools for society in the future, comprehensive high-quality observations of atmospheric concentrations of aerosols, trace gases, and related environmental variables from in situ observations are required in concert with EO from space (Petäjä et al., 2014; Hari et al., 2016). The EO data can be used to study the interactions between different types of surfaces and the atmosphere. The results obtained allow us to evaluate the impact of pollutants on the equilibrium of the Arctic system and provide an important input for evolutionary scenarios of the Arctic environment. The picture needs to be harmonized and supported with complementary multi-scale modeling (e.g., Kulmala et al., 2011a; Kulmala, 2018). The need to establish and maintain long-term, coherent, and coordinated observations and research activities on environmental quality and natural resources in polar areas drives iCUPE activities. The core idea of iCUPE is the development of novel, integrated, quality-controlled, and harmonized in situ observations and satellite data in polar areas, as well as the supply of data products to end users. The impact of this integrated Arctic observing system needs to be demonstrated for changing campaign practices to continuous monitoring activities. The Sustaining Arctic Observing Networks (SAON) initiative under the auspices of the Arctic Council has been developing an assessment framework for this since 2017 (IDA Science and Technology Policy Institute and Sustaining Arctic Observing Networks, 2017), and iCUPE has been helping to make the first full value tree analysis for this. It connects observing activities in situ and from satellites through modeling and services to key objectives of societal benefit areas. This will greatly help in forming impact assessments in the future.
The iCUPE project aims to synthesize data from comprehensive long-term measurements, intensive campaigns, and satellites collected during the project or provided by ongoing international initiatives. The aim of this paper is to introduce an ongoing project, iCUPE, and summarize its initial results. We put a specific emphasis on black carbon and persistent pollutants in the Arctic context. We explore snow and ice core samples to put the current concentrations in a longer perspective. We underline the capacity of continuous observations to monitor the impact of policies to reduce emissions. We showcase the potential to address pollution in the Arctic environment by integrating satellite remote sensing, airborne observations, in situ data, and modeling. Modern comprehensive source apportionment can resolve the different sources of atmospheric aerosols and differentiate between sources within and outside the Arctic environment. We also discuss the iCUPE impact and relevance for Arctic research and for stakeholder communities.
The motivation behind iCUPE stems from the need to address global environmental challenges in a polar context (Fig. 1). The underpinning concept of iCUPE is that transdisciplinary research utilizing the full capacity of comprehensive in situ observations together with state-of-the-art satellite observations is required to make advances in the understanding of atmospheric and cryospheric processes in the Arctic environment (Fig. 2). Therefore, the work in iCUPE utilizes both expertise with in situ observations and satellite remote sensing in a close connection to modeling frameworks to address this need (Fig. 3). The work is closely connected to ongoing activities such as the Integrated Arctic Observation System (INTAROS; Sandven et al., 2018) and the Multidisciplinary drifting Observatory for the Study of Arctic Climate (MOSAiC; Shupe et al., 2018) expedition conducted in polar areas through collaboration. These connections enable the iCUPE consortium partners to facilitate interactions and strengthen coordination between national and international activities in polar areas. A wide spectrum of observational quantities, data products, and modeled variables is required to enable the delivery of the integrated data required for decisions related to Arctic pollution.
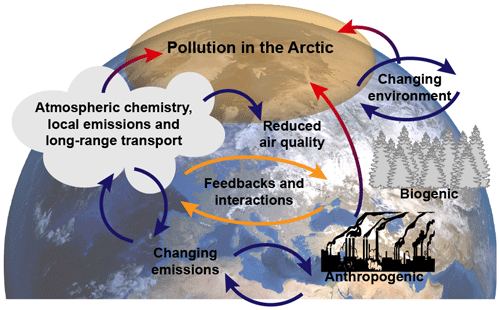
Figure 1Atmospheric concentrations of pollutants and their life cycles in high latitudes are affected by local and regional anthropogenic activities and long-range transport from lower latitudes. Pollutant distributions and life cycles are modulated by transport patterns, changes in the biosphere, increased natural resource extraction, and increased shipping in the Arctic Sea. Various feedbacks and interactions can either speed up or hinder the changes.
In this section we summarize the results and findings of the iCUPE project regarding the in situ observations (Sect. 3.1–3.6) and the satellite component (Sect. 3.7). We selected integrating examples in Sect. 3.8, which also include method development and conceptualization. This is consistent with the iCUPE concept as a whole (multi-platform observations, modeling, synthesis; Fig. 2).
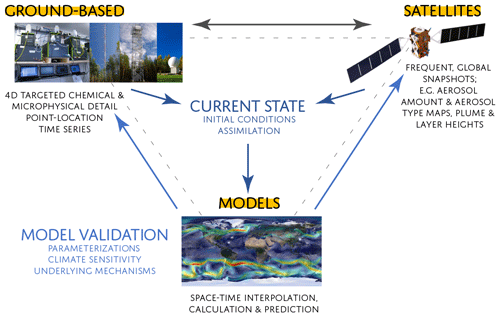
Figure 2The integrative concept of iCUPE incorporates data and knowledge from ground-based observations, satellite remote sensing, and modeling results, providing a comprehensive view on the state of the environment in polar areas.
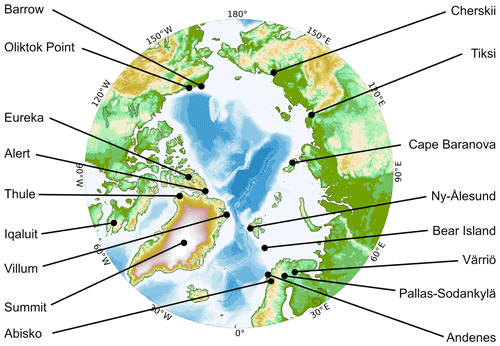
Figure 3A map of stations with year-round observations in the Arctic with atmospheric aerosol measurements (note that Barrow is now Utqiaġvik).
3.1 Atmospheric observation capacity in the Arctic
Figure 3 depicts year-round monitoring aerosol measurements in the Arctic. Within the International Arctic Systems for Observing the Atmosphere (IASOA; Uttal et al., 2016), there are 14 stations around the Arctic with a clear prevalence of the west longitudes. In addition to the IASOA sites, the Stations for Measuring Ecosystem–Atmosphere Relations (SMEAR; Hari et al., 2016) observation network extends to the Arctic; the SMEAR I in Värriö, Finnish Lapland, has provided aerosol and trace gas observations since 1992 (Hari et al., 1994). Many of these observation sites contribute to the World Meteorological Organization's Global Atmospheric Watch (WMO-GAW) as well as to many thematic European research infrastructures, such as Integrated Carbon Observation System (ICOS) and the Aerosols, Clouds and Trace Gases Research Infrastructure (ACTRIS). Regionally, the Svalbard Integrated Arctic Observing System (SIOS) provides a platform for comprehensive measurement activities within the Svalbard area. Overall, the length and breadth of monitoring programs vary greatly from site to site. Some of these sites have been operational for almost 30 years, measuring a large number of parameters, while others, such as Cape Baranova, have only been open for the past 2–3 years. A historical perspective of aerosol measurements in the Arctic (and Antarctica) is presented by several review papers via the POLAR-AOD network (Tomasi et al., 2007, 2012, 2015) and Pan-Eurasian Experiment (PEEX; Lappalainen et al., 2018; Vihma et al., 2019).
A geographically representative distribution of measurements is of particular importance. The atmospheric in situ observation network in the Arctic has been developed during the last 3 decades by individual scientists and groups utilizing the resources available. National interests and logistical possibilities have played a role in the initial establishment of the stations and their maintenance. Considering all this, it is somewhat surprising to see that the distribution of measurement sites covers the entire Arctic Circle with relatively high homogeneity. A few gaps can be identified on the Canadian coast and in the Russian Arctic along the eastern edge of Siberia and the Russian Far East (Petäjä et al., 2020).
The coastline of the Russian Arctic is over 24 000 km long, thus being a significant region where more comprehensive atmospheric and aerosol observations are needed. Within the PEEX program, we have performed a gap analysis in terms of atmospheric and environmental observations within the Russian Arctic (Alekseychik et al., 2016). The work is ongoing, and the PEEX catalog consists of metadata from 59 stations altogether at the moment. The stations are operated by universities, the Russian Academy of Sciences, and Roshydromet. The most comprehensive stations in the Russian Arctic providing atmospheric observations and aerosol data are the Tiksi Hydrometeorological Observatory in the Russian Far East (71.6∘ N, 128.9∘ E) and the Northeast Science Station (Cherskii; 68.73∘ N, 161.38∘ E).
3.2 Long-term atmospheric in situ observations
As an example, we present results from long-term observations of atmospheric aerosol particle measurements at the high-Arctic site Villum Research Station (VRS) in north Greenland and long-term data for black carbon data from Mt. Zeppelin at Svalbard. Furthermore, we summarize recent mass spectrometric analyses of organic matter collected on filters within the Arctic during iCUPE and present results from vertical measurements of aerosol particle number concentrations.
3.2.1 Aerosol particle number concentrations at the high-Arctic site Villum Research Station (VRS) in North Greenland
Particle number size distributions (PNSDs) have been continuously measured at VRS from July 2010 onwards. The aerosol number size distributions were determined with a scanning mobility particle sizer (SMPS) in the size range from 9 to 915 nm following the standardization presented in Wiedensohler et al. (2012).
The long-term data show that the seasonality of the atmospheric aerosol number concentration at VRS is largely governed by synoptic weather patterns. Particularly important is the location of the Arctic Front, which describes the boundary between the cold Arctic air masses and the midlatitude atmosphere. The Arctic Front effectively blocks atmospheric transport from the midlatitudes to the high Arctic (e.g., Law and Stohl, 2007). There is considerable variability in the location of the Arctic Front geographically and seasonally (e.g., Klonecki et al., 2003). This leads to a variable contribution between local emissions and the long-range-transported aerosols consequently affecting the observed aerosol number size distribution at VRS (Nguyen et al., 2016). The described seasonality is evident from Fig. 4, which displays the monthly averaged particle number concentration in the accumulation mode (Fig. 4a) and ultrafine modes (Fig. 4b).
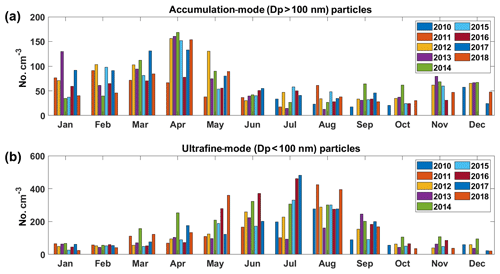
Figure 4Monthly mean accumulation-mode (a) and ultrafine-mode (b) number concentration (no. cm−3) measured at VRS from 2010 to 2018. Note the difference in the scale of the y axis for each panel.
Our results from VRS show that in the wintertime (October–April), efficient meridional transport coupled with inefficient wet removal processes permits emissions from anthropogenic sources in the midlatitudes to reach VRS (Freud et al., 2017). This Arctic haze is characterized by high concentrations of sulfate, black carbon, and accumulation-mode (particle diameter >100 nm) aerosol particles (Fig. 4a). The expansion of the Polar Front and inefficient wet removal in the high Arctic allows for the transport and buildup of relatively high concentrations of accumulation-mode particles at VRS. This mode increases in concentration from November until it reaches a maximum in April. When the Polar Front retreats, the transport of anthropogenic emissions is limited to the high Arctic as only emissions north of the Polar Front can find a direct way to high-Arctic sites, and the front is located much further north. Also, wet removal processes reduce the buildup of high concentrations. Other transport processes would only be pathways in high altitudes penetrating the Polar Dome by descending air masses through entrainment reaching high-Arctic sites, which is rarely observed.
The decrease in Arctic haze, coupled with increases in sunlight and the melting of the sea ice–snowpack, allows natural sources of aerosols and their corresponding precursor gases to become an important source to the atmospheric burden of Arctic aerosols. During the summer months, local and regional emissions from natural sources govern the aerosol burden (Barrie, 1986). There is a minimum of ultrafine-mode aerosol concentrations in the winter months, followed by an increase in April–May, reaching a maximum in July, before decreasing during August–September until reaching a minimum again in October. The aerosol and precursor sources for the ultrafine aerosols (aerosol particle diameter <100 nm), particularly during the summer, can arise from primary marine aerosols and secondary sources such as the gas-to-particle conversion of precursor gases (e.g., dimethyl sulfide, oxygenated volatile organic compounds, and halogenated compounds) (Dall'Osto et al., 2018a; Giamarelou et al., 2016; Mungall et al., 2017; Sipilä et al., 2016).
Due to anthropogenically induced climate change, Arctic sea ice extent is rapidly decreasing, as is thickness with the proportion shifting from multiyear ice to first-year ice (IPCC, 2014). There are also indications for a high contribution of Atlantic warm water contributions to the sea ice loss in the Arctic (Polyakov et al., 2017). This has consequences for the atmospheric burden of ultrafine-mode aerosols or their precursors originating during the summer from open waters, ice edges, or open leads. In general, these changes are expected to impact precursor gas emission rates and therefore new particle formation events (Dall'Osto et al., 2018), which implies that the clean Arctic lower troposphere may have a large impact on the radiative balance if the newly formed particles reach accumulation-mode sizes and therefore act as cloud condensation nuclei (CCN). Elucidating the impact of sea ice loss on the aerosol population is needed to understand the radiative forcing of Arctic aerosol in the future. Furthermore, climate change may impact the Arctic atmospheric circulation systems and consequently change the atmospheric transport patterns or intensity of those with respect to the occurrence of accumulation-mode aerosol in late winter and early spring in the high Arctic (Arnold et al., 2016). A long-term observation record of the atmospheric aerosol number size distribution in the Arctic will enable us to resolve these interactions and feedbacks in the future.
3.2.2 Black carbon concentrations at Mt. Zeppelin, Svalbard
Black carbon (BC) is one of the key short-lived climate forcers contributing to the warming of the Arctic by both absorbing solar radiation and by enhancing snowmelt and ice melt by surface deposition (e.g., Bond et al., 2013). As part of the atmospheric observations, the ACTRIS and IASOA networks (Fig. 3) operate a network of aethalometers to determine the atmospheric concentration of BC in the air (Uttal et al., 2016). Although BC is the common term we use for light-absorbing carbon, it is now more appropriate to report mass concentrations in terms of equivalent black carbon (eBC), especially when filter-based optical techniques are employed. The equivalent black carbon mass concentration is considered to be the mass of an equivalent amount of light-absorbing carbon with a given mass absorption efficiency causing the attenuation of light observed by the instrument at a given wavelength (Petzold, 2013). The quality assurance of data for eBC and the corresponding aerosol absorption coefficient has greatly improved over the last years. Compensation schemes for measurement artifacts and harmonization of data obtained by different instruments have been established (Backman et al., 2017) and are continuously updated (Zanatta et al., 2018).
The results from long-term observations at Zeppelin have been discussed and assessed in several works elaborating on the climatology of BC in the Arctic (Eleftheriadis et al., 2009.; Sharma et al., 2013; Breider et al., 2017; Schmeisser et al., 2018). The results presented here represent the longest continuous eBC reported record by a single instrument in the European high Arctic (Torseth at al., 2019) and globally the second-longest after those obtained between 1989 and 2009 in Alert (Sharma et al., 2019). As an example of long-term observations of BC, we show the latest eBC concentration time series from Zeppelin Station at Svalbard (Fig. 5). The results show a continued gradual reduction in the annual mean value of observed eBC, while the time series is strongly modulated by a seasonal cycle well known in the Arctic with minima in the summer and maximum in late winter spring. One can observe this long-term decline with a linear trend line applied only as a crude estimate for these data. The long-term data series we present here makes it possible to derive some descriptive statistics. The eBC annual mean value has been reduced from an annual mean value of 31 ng m−3 at the beginning of the previous decade to 12 ng m−3 during the last years, with an average reduction of 7 ng m−3 per decade, which amounts to a reduction 4 % annually or approximately 44 % per decade. However, trend analysis for aerosol climatology records needs to be practiced with caution in order to remove the effects of the seasonal cycle. When the extracted absorption coefficient from our data was thoroughly examined for a shorter period (2005–2018) the trend was not found to be statistically significant (Collaud Coen et al., 2020). Minimum values over the summer often drop below the detection limits of the instrument, while maximum values vary greatly with their occurrence, usually related to large-scale biomass burning events across Siberia and Alaska. The continuous reduction in fossil fuel usage is a reason for this reduction, but it is well known that emissions are not uniformly changing on a global scale, at least in the Northern Hemisphere (Evangeliou et al., 2018).
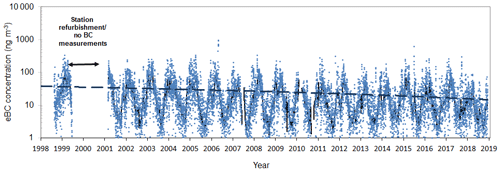
Figure 5Equivalent black carbon concentration time series obtained by a seven-wavelength aethalometer at Zeppelin Station. (Data before 2000 are obtained by an AE-9 aethalometer.)
The long-term observations can provide important insight into how abatement strategies for emission reductions or enhanced anthropogenic activities in certain areas can be responsible for the observed changes. Reverse air mass transport modeling using the FLEXPART model (Stohl et al., 2005) can provide the means to combine eBC observations at a remote station like Zeppelin and source areas globally. We simulate the backward transport of a black carbon (BC) and an air tracer at the Arctic station of Zeppelin during a period representative of the high measured concentrations (spring 2012). The analysis is done using reanalysis meteorological inputs from the European Centre for Medium-Range Weather Forecasts (ECMWF) at a resolution of 1 ∘. The potential source contribution function (PSCF) is applied to both tracers. Western Siberia appears to be the main source region in the PSCF analysis (Fig. 6).
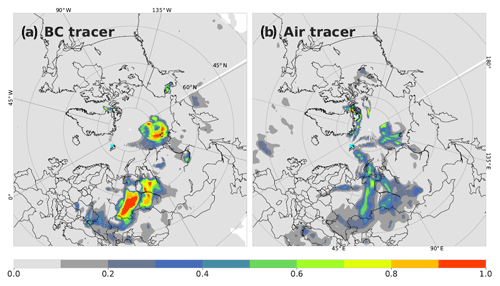
Figure 6Potential source contribution areas for BC observations at Zeppelin Station, Svalbard. The color scale represents statistical potential (1:100 %) for the 80th percentile of observed values to originate from certain areas of the Northern Hemisphere. (a, b) The air mass transport calculations using the FLEXPART model with the BC tracer and air tracer, respectively.
Differences in emission sensitivities between the two tracers stress the importance of deposition mechanisms in aerosol transport. Wet scavenging occurs in the presence of clouds and precipitation. Wet deposition is determined from a scavenging coefficient, which is also dependent on the precipitation rate. The sub-grid variability of the precipitation rate is calculated as an area fraction in each grid that experiences precipitation. Both large-scale and convective precipitation rates are accounted for. We observed that the difference in the areal distribution of source areas, the potential significance of the key emission areas to contribute to eBC in the high Arctic, and their estimated climate impact is very much dependent on the microphysical parameterization of the model since the air tracer result is considered a simplified representation of the transport, and the metrics above greatly affect the result we observe when the BC tracer parameterization is applied.
3.3 Results from targeted atmospheric field studies in the Arctic
3.3.1 Mass spectrometric measurements for offline source apportionment based on Arctic organic aerosols
Organic compounds are of high importance because they contribute between one- and two-thirds of the submicron aerosol mass in the Arctic (Willis et al., 2018; Schmale et al., 2018; Popovicheva et al., 2019) and may be co-emitted or interact with other aerosol species, such as black carbon (AMAP, 2015), sulfate (Kirpes et al., 2018), and metals (Shaw et al., 2010). If imported from lower latitudes, they also act as a vehicle of transport for persistent organic pollutants (POPs) to the Arctic (Westgate et al., 2013). Organic aerosol (OA) also absorbs (Moschos et al., 2018) and scatters light, thereby changing the radiative balance (Myhre et al., 2013), and may act as cloud condensation nuclei. OA might become increasingly important in a warming Arctic due to anthropogenic activities (Schmale et al., 2018) and natural emissions, e.g., as a result of expanded vegetation (Bhatt et al., 2010), intensified wildfires (Warneke et al., 2010), and decreasing sea ice extent and thickness, leading to higher release of marine volatile organic compounds (Mungall et al., 2017) and thawing tundra soils (permafrost) along shores and rivers (Peñuelas et al., 2014; Kramshøj et al., 2018). The continuous monitoring of organic carbon (OC) along with a detailed chemical analysis to determine its natural and anthropogenic sources, seasonal variability, and interannual evolution in the Arctic is of prime importance for improved climate simulations and a realistic assessment of the effectiveness of potential mitigation or adaptation actions.
Offline mass spectrometric aerosol analysis
OA chemical composition and the corresponding sources remain largely unknown, partly due to the challenging measurement conditions (Uttal et al., 2016; Kulmala, 2018). For example, tremendous effort is required for the deployment of online aerosol mass spectrometry in various environments for long time periods. To overcome this challenge an offline Aerodyne aerosol mass spectrometer (AMS) technique has been introduced based on re-aerosolized liquid filter extracts (Daellenbach et al., 2016). The method is capable of covering broad spatial and seasonal observations as well as determining the sources of OA (e.g., primary versus secondary, biogenic versus anthropogenic) (Moschos et al., 2018; Bozzetti et al., 2017). This is achieved with positive matrix factorization, a bilinear unmixing receptor model used to describe the input mass spectra time series as a linear combination of static OA source (factor) profiles and their time-dependent contributions to the total OA loading (Canonaco et al., 2013). Within iCUPE, we aim to extend the coverage of this technique to the most climate-change-sensitive region worldwide. The offline AMS analysis will be combined for the first time with ultrahigh-resolution mass spectrometry coupled with liquid chromatography for a two-dimensional molecular identification of primary aerosol tracers and secondary organic aerosol precursors.
Sampling sites
We have collected quartz-fiber filter samples around the Arctic. This unparalleled effort is expected to produce data for the chemical and source characterization of OA at nine sites within six countries (Pallas, Finland; Zeppelin and Gruvebadet, Norway; Villum, Greenland; Alert, Canada; Barrow (now Utqiaġvik), USA; Tiksi and Cape Baranova, Russia) from 68 to 83∘ N (Fig. 3) covering the period 2014–2019. These include both coastal and archipelago high-Arctic stations as well as boreal forest Eurasian sites near the Arctic Circle with different emission exposure characteristics. Here we show offline AMS data for samples collected at two stations: Ice Base Cape Baranova (Russia) and Alert (Canada).
The research station Cape Baranova was built in 2013. It is located near Cape Baranova on the coast of the Shokalsky Strait, which divides the Bolshevik and October Revolution islands of the archipelago Severnaya Zemlya (79.16∘ N, 101.45∘ E). The area adjacent to the station is characterized by the presence of sea ice, dome-shaped glaciers, and icebergs. Polar night and day at the station Cape Baranova last from 22 October to 22 February and from 22 April to 22 August, respectively. The air temperature in summer (June–August) is from 0 to 4 ∘C. In winter (October–April) temperatures range from −25 to −45 ∘C. The area of the station is characterized by stable winds mainly from the southern direction with an average speed of 10–15 m s−1. During the transition periods of the year, the wind speed can reach 50 m s−1.
Alert was opened in 1986 as Canada's first research station for the continuous monitoring of background concentrations of trace gases and aerosols. It is located at 82.5∘ N, 62.37∘ W (210 m a.s.l.) with prevailing winds from the southwest, which usually bring clear skies and warmer temperatures. North winds off the ocean are typically accompanied by fog and sudden drops in temperature.
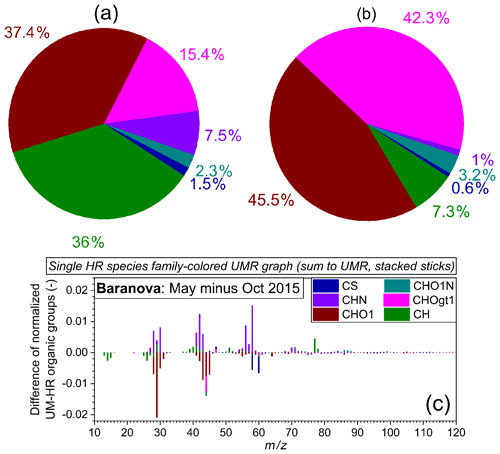
Figure 7Offline AMS-based fractional contribution of the different organic aerosol families for (a) Cape Baranova (H:C = 1.53, O:C = 0.53) and (b) Alert (H:C = 0.81, O:C = 1.64) during October 2015. (c) Single organic species family-colored (stacked sticks) difference of the May minus October 2015 normalized mass spectrum. The former sample is expected to be influenced by pollution transport from mainland Russia. Data were taken and analyzed at high resolution but are summed to unit mass resolution for display.
Preliminary results from the filter sampling
In Fig. 7, we show offline AMS-based relative fragment contributions of the different organic families in October 2015 (transition to polar night) for the station Cape Baranova (a) and for Alert (b). Even though only limited conclusions can be drawn from the analyses of single filter samples, there is considerable variability in the composition of organic fragments between the two samples. During the same autumn period, the 2 d Cape Baranova filter sample contains more than one-third hydrocarbon-like fragments (family CH) and about 50 % oxygenated species (families CHO, CHOgt1). The 2-week Alert filter sample contains more, i.e., roughly 90 %, and more strongly oxygenated fragments (especially the mass-to-charge ratio m∕z 44, family CHOgt1). This can be an indication for the extreme remoteness of the site because OA has to be advected over long distances before it reaches Alert and can hence be oxidized during transport.
The difference spectrum for Cape Baranova (Fig. 7c) emphasizes potential seasonal differences in fragments that have a relatively high overall contribution to the organic mass. Specifically, the relative abundance of N-containing fragments is indicative for May (2 d filter), whereas the October sample contains more oxygenated fragments. The former might potentially be linked to transported anthropogenic (e.g., fossil fuel) emissions. No marked significant differences are observed for the CH fragments. Ongoing analyses including the other Arctic stations suggest significant variability among the different sites and seasons in the relative fraction of fragments that are markers of certain sources, indicating largely regionally specific sources of OA across the Arctic land surface.
The data analysis is in its early stages, but we anticipate a number of outcomes that are valuable for the upcoming assessment by the Expert Group on Short-Lived Climate Forcers of the Arctic Monitoring and Assessment Programme (AMAP), future ultrahigh-resolution mass spectrometric measurements of OA in the Arctic, and comparisons of atmospheric OA with OA in ice cores for historical trends of sources and composition.
3.3.2 Vertical measurements of aerosol particle number concentrations at Svalbard
Ground-based measurements are performed continuously at specific sites around the Arctic, but vertical measurements are still rare. To investigate the spatial distribution of aerosol particles in an Arctic environment a 4-week measurement campaign was conducted with two different types of unmanned aerial system (UAS) between April and May in 2018 in Ny-Ålesund (Spitsbergen, Norway). The UAS ALADINA (see Fig. 8a) is equipped with miniaturized aerosol instrumentation (two condensation particle counters with different lower detection limits, optical particle spectrometer, aethalometer) and meteorological sensors (Altstädter et al., 2015; Lampert et al., 2020). A second fixed-wing UAS of type MASC3 (Fig. 8b) was operated in parallel focusing on meteorological measurements. MASC3 probed the lower atmosphere from around 20 to 600 m a.g.l. with flight times of up to 1.5 h. It carried a sensor payload for measuring turbulent quantities of pressure, temperature, humidity, and the 3D wind vector, providing 100 Hz data (Rautenberg et al., 2019).
ALADINA flights were focusing on aerosol profiles, while MASC3 flew at several fixed altitudes and over a large horizontal extent with high spatial resolution (less than 1 m for the wind vector and the temperature). The 3D data series show the spatial horizontal and vertical variability of the different layers, which enable characterizing the complex flow properties in the fjord around Ny-Ålesund.
The resulting data also provide a spatial variability of turbulent properties (sensible heat, turbulent kinetic energy, momentum flux). The UAS data improve the understanding of the sources and transport processes of aerosol particles in the Arctic, link the observations close to the fjord and at the Zeppelin mountain station (Ström et al., 2009; Tunved et al., 2013), and help to detect regions where new particle formation takes place. Around 200 vertical profiles were captured with the UAS ALADINA between the ground and 850 m a.g.l., thus connecting the ground-based measurements at Gruvebadet laboratory, located near the village of Ny-Ålesund, and the data sampled at the Zeppelin Observatory at a height of 474 m a.s.l. Further measurement flights were operated horizontally above snow cover and above open water in order to capture the possible impact of biogenic activity on new particle formation (NPF), as shown along the flight path in Fig. 9.
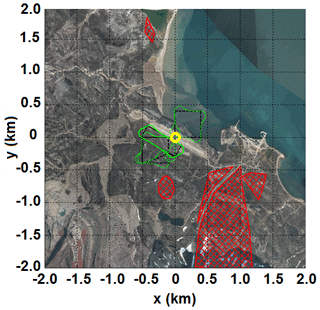
Figure 9Flight path of the UAS ALADINA (start point in the center, marked in yellow) in order to study the vertical and horizontal distribution of aerosol particles above ice surfaces near glaciers and over open water. Research flights were spatially limited by restricted areas (hatched in red), like a bird sanctuary near the coast and instrument areas south of Ny-Ålesund, and no activity was performed over inhabited buildings of the village. The TopoSvalbard map© is obtained from the Norwegian Polar Institute and retrieved from http://toposvalbard.npolar.no, last access: 29 June 2020.
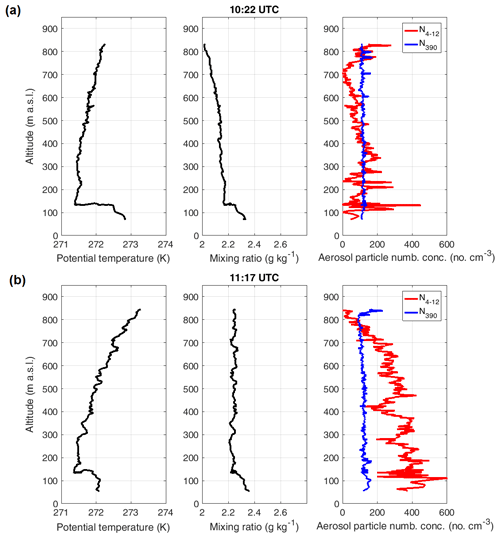
Figure 10Selected profiles measured on 23 May 2018 in Ny-Ålesund for (a) 10:22 and (b) 11:17 UTC. The panels show vertical profiles of the potential temperature, water vapor mixing ratio, and aerosol particle number concentrations between 4 and 12 nm and above 390 nm.
Figure 10 shows selected profiles of preliminary data for one selected measurement day. The potential temperature and water vapor mixing ratio profiles show that the boundary layer at Svalbard consists of a shallow unstable layer with elevated humidity at the surface. This layer grows higher during the day. However, even at 11:17 UTC the mixing reached only 150 m; higher than that, the boundary layer remained stably stratified. The number concentration of accumulation-mode particles remained rather constant as a function of height. However, nanoparticles between the sizes of 4 and 12 nm varied, and several distinct layers were identified. The nanoparticle concentrations were the highest at the elevation closest to the surface, but high number concentrations were observed at altitudes up to 500 m. High nanoparticle concentrations in the residual layer have been observed in different environments as well (e.g., Wehner et al., 2010; Altstädter et al., 2018; Leino et al., 2019; Carnerero et al., 2019). Further analysis needs to be done to conclude if this is caused by anthropogenic pollution or locally restricted NPF events. If NPF events occur on such small scales, vertical measurements of aerosol particles are needed to estimate the contribution of such small particles to the regional aerosol balance because many of these layers are not captured by ground-based measurement sites.
3.4 Mercury in the Arctic
High concentrations of mercury (Hg) in Arctic biota pose a threat to local populations and wildlife (Douglas et al., 2012; AMAP, 2011). The scarce anthropogenic Hg emission sources in the Arctic have left scientists to wonder how midlatitude emissions reach the Arctic Ocean marine ecosystem (e.g., Durnford et al., 2010). The discovery of massive Arctic atmospheric Hg depletion events in 1998 (Schroeder et al., 1998) that are associated with sea-ice-derived reactive halogen oxidants (Skov et al., 2004) has fueled a paradigm in which midlatitude urban and industrial Hg emissions reach the Arctic exclusively via the atmosphere. However, subsequent research has shown that 70 %–80 % of the deposited Hg is photochemically reemitted back to the atmosphere only hours after deposition (Obrist et al., 2017; Brooks et al., 2006). In iCUPE, we performed observations on the Hg concentrations in the Arctic cryosphere, explored the role of river systems to the Arctic Ocean mercury load, and explored the interactions between halogen compounds and Hg.
3.4.1 Arctic mercury cycle
In 2012, a coupled 3D ocean–atmosphere model of the Arctic Hg cycle suggested that a source of Hg to the Arctic Ocean was missing (Fisher et al., 2012). The missing source was suggested to be Arctic rivers, in particular Russian rivers that account for 80 % of river runoff to the Arctic Ocean. We monitored year-round Hg levels in the Yenisei and Severnaya Dvina rivers from 2012 to 2016 and confirm that Russian rivers transport large amounts of Hg to the Arctic Ocean (Sonke et al., 2018; Fig. 11b). As part of the iCUPE project these results were integrated into a 3D Arctic mercury model, developed by coworkers from Harvard University (Sonke et al., 2018). We showed that anthropogenic Hg emissions from midlatitude industrial sources do not directly reach the Arctic Ocean ecosystem. Instead, atmospheric elemental Hg0 is taken up year-round by Arctic tundra vegetation and soils (Obrist et al., 2017; Jiskra et al., 2018). Springtime snowmelt mobilizes the tundra soil mercury, which is bound to plant-derived carbon, via rivers to the Arctic Ocean, where it becomes partly available to the marine food web.
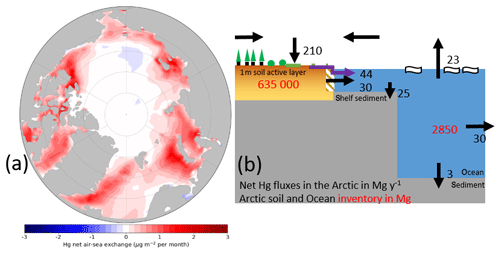
Figure 11(a) Modeled net air–sea exchange of Hg (µg m−2 per month) across the Arctic Ocean basin for the months June–July from the coupled GEOS-Chem–MITgcm Hg chemistry and transport model. Positive numbers (red) indicate a flux to the atmosphere. (b) The modern Arctic Hg cycle, showing net fluxes (metric tons per year, in black) between the different terrestrial, marine, and atmospheric reservoirs (metric tons, in red). The hatched area represents the coastal erosion Hg flux. The large river Hg flux (purple arrow) confirms a new paradigm in which tundra vegetation and soil uptake midlatitude Hg atmospheric emissions and transfer them to rivers and the Arctic Ocean. Reproduced from Sonke et al. (2018).
As a summary, the 3D model suggests that a large portion of riverine Hg is photochemically reduced in the surface Arctic Ocean and emitted into the atmosphere. The river Hg budget, together with recent observations on tundra Hg uptake and Arctic Ocean Hg dynamics, provides a consistent view of the Arctic Hg cycle in which continental ecosystems traffic anthropogenic Hg emissions to the Arctic Ocean via rivers and in which the Arctic Ocean exports Hg to the atmosphere, to the Atlantic Ocean, and to marine sediments (Sonke et al., 2018, Fig. 11). Recent iCUPE research suggests that Arctic warming and permafrost thaw risk doubling tundra soil Hg runoff to the Arctic Ocean via rivers, thereby potentially increasing health risks to humans (Lim et al., 2019).
3.4.2 Mercury deposition to the Svalbard snowpack
To expand knowledge of the role of snowpack in the Hg life cycle, we performed targeted field studies (Spolaor et al., 2018, 2019) to determine the seasonality of Hg deposition and the total Hg deposition from the atmosphere to snow preserved in the Arctic environment on the Svalbard archipelago, specifically in the Spitsbergen region (Fig. 12). The annual snowpack is defined as the snow that accumulates over a glacier surface during the winter. The snow season in Svalbard changes year by year, but typically the accumulation of snow starts at the end of September and ends at the end of May in concomitance with the temperature rise and the start of snow melting (Spolaor et al., 2016). The snow season is dependent on altitude, and sites at a higher elevation can preserve part of the annual snowpack throughout the year. The equilibrium line, which is the altitude above which snow accumulated during the snow season, is partially preserved and located at approximately 600 m a.s.l in the Svalbard region; below this height the snow is completely removed.
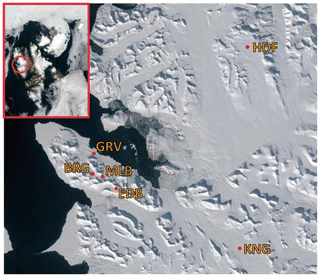
Figure 12Snow sampling locations on the Svalbard archipelago. BRG – Austre Brøggerbreen glacier; MLB – Midtre Lovénbreen glacier; EDB – Edithbreen glacier; KNG – Kongsvegen; HDF – Holthedalfonna ice field. The TopoSvalbard map© is obtained from the Norwegian Polar Institute and retrieved from http://toposvalbard.npolar.no, last access: 29 June 2020.
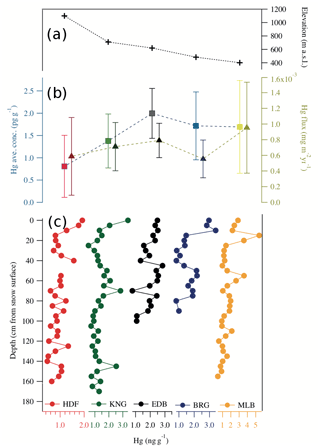
Figure 13The (a) elevation of the snow pit sampling sites. Panel (c) depicts Hg concentrations in the annual snowpack (pg g−1). The depth scale is expressed in centimeters, and the snow surface is set at 0 cm. Samples have a constant resolution of 5 cm to the bottom. (b) Mean Hg concentration (color square) and total flux (color triangles); vertical bars indicate 1 standard deviation.
In this study we selected five locations to study the Hg concentration in the snowpack (Fig. 13). The lower snow pit was dug in the Midtre Lovénbreen glacier (MLB) at an altitude of 401 m a.s.l., the Austre Brøggerbreen (BRG) snow pit at 484 m a.s.l., the snow pit on the Edithbreen (EDB) at 620 m a.s.l., the Kongsvegen (KNG) snow pit at 710 m a.s.l., and the Holthedalfonna (HDF) snow pit at 1100 m a.s.l. The sampling was done following a constant sampling step of 5 cm starting from the top to the bottom of the snowpack (identified as the glacier icy surface or by the snow preserved from the previous year; the latter case only for the KNG and HDF sites). The results show that the Hg concentrations (Fig. 13c) ranged from 0.5 pg g−1 up to 5 pg g−1 with an average concentration of 1.5 pg g−1. The higher concentrations were detected at the EDB location (2.00±0.56 pg g−1). The MLB and BRG had a concentration of 1.69±1.04 and 1.72±0.76 pg g−1, respectively. The lower mean concentration was found in the HDF and KNG snowpack with a concentration of 0.80±0.69 and 1.38±0.61 pg g−1, respectively. The snowpack sampled approximately at or below 600 m a.s.l. had rather constant Hg concentrations ranging from 1.7 to 2.0 pg g−1, while at higher altitude (from 700 to 1100 m a.s.l.) the concentration decreased in the range between 1.4 and 0.8 pg g−1. We found a linear relationship between altitude and mean Hg concentration with an R2 of 0.78.
However, the concentration of Hg is not the correct parameter to evaluate the deposition flux from different sites since higher snow accumulation might induce a dilution effect. Therefore, the average Hg concentration was converted into Hg flux considering the annual snowpack depth and its density. The estimated flux represents the total Hg load preserved in the annual snowpack. The results suggest that the Hg deposition flux is similar at all the sites investigated (BRG 0.54, EDB 0.78, MLB 0.94, KNG 0.70, and HDF 0.58 µg m−2 yr−1), and the elevation gradient is not statistically significant (R2 =0.28). In the snow pit, the snow at the bottom is representative of the fall season, the middle of the winter deposition, and the upper part of the snow accumulated during the spring. The results show that the Hg concentration in all snow pits tends to increase in the upper, most recent snow layer, in general from 0 to 40 cm depth. The upper part of the snowpack (the sampling was done in April 2018) is more representative of the spring deposition, potentially influenced by atmospheric mercury depletion events (AMDEs). More data analysis is required to verify this hypothesis.
3.4.3 Mercury in air of the Russian Arctic (Amderma)
Since June 2001, long-term monitoring of gaseous elemental Hg0 in the troposphere has been carried out near the Amderma settlement (69.45∘ N, 61.39∘ E; 49 m a.s.l.; Nenets Autonomous District, Russia), which is located on the Yugor Peninsula on the shore of the Kara Sea close to the Arctic border between Europe and Asia. A Tekran 2537A Hg0 vapor analyzer, which is a cold vapor atomic fluorescence spectrometer, was used. We carried out an analysis of the monthly, seasonal, and interannual variability in concentration patterns as well as the occurrence of atmospheric Hg depletion events (AMDEs) and elevated events (AMEEs). Subsequently, we determined Hg fluxes to the atmosphere, evaluated concentration trends, and identified possible long-range transport cases (with the Hybrid Single-Particle Lagrangian Integrated Trajectory model, HYSPLIT; Stein et al. 2015) originating from Icelandic volcanic eruptions (Pankratov et al., 2015).
During the entire period of operation at this station, the location of the analyzer was changed three times at different distances to the Kara Sea coastline. The results show that the frequency of AMDE occurrence depends on the distance to the coastline. From 2001 to 2004 the analyzer was located at a distance of about 9 km from the coast. The number of AMDEs for this period was 10 % of the total number (6765) of measurements. During the 2005–2010 period, the analyzer was placed at 2.5 km from the coast. As a result, the depletion events were registered more frequently, especially during the spring–summer period. In 2006 and 2007 such depletion events were recorded in winter with a frequency of 20 % of the total number (1898) of measurements. Starting from 2010 the analyzer was located at a distance of 200 m from the coast. As a result of this relocation, more events were recorded (30 % of 67 986 measurements) (Fig. 14a). For the first time in the Russian Arctic, we identified the intensification of the AMDE frequency as a function of distance to the sea. The results are consistent with observations in other polar stations such as Ny-Ålesund and Andøya (Norway), Alert (Canada), and Pallas (Finland) (Pankratov, 2015; Nguyen et al., 2009).
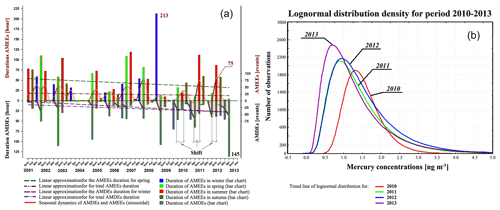
Figure 14(a) Seasonal dynamics of AMDEs and AMEEs during the monitoring period from 2001 to 2013 (sinusoidal area). Duration of AMDEs and AMEEs (bar chart – red, summer; green, spring; dark yellow, autumn; blue, winter). Linear approximation for the AMEE duration for spring (green dashed line), AMDE duration for winter (purple dashed line), total AMEE duration (brown dash with two dots), and total AMDE duration (purple dash with two dots). (b) The lognormal distribution of the atmospheric Hg0 concentration during the monitoring period from June 2010 to October 2013.
Histograms of the Hg0 concentration at Amderma during the different years are presented in Fig. 14b. The probability density distribution of the Hg0 concentration was lognormal for the monitoring period from June 2010 to October 2013. There is a significant asymmetry in the left-hand region of the Hg0 concentration probability distribution relative to the arithmetic average, pointing to the fact that low Hg0 concentrations are measured more frequently. In 2013 this asymmetry was especially evident. The shift of the concentration to lower values was due to the increased number of Hg0 depletion events recorded during the winter seasons of 2010–2013. To assess the dynamics of Hg0, a linear approximation of the average annual Hg0 concentrations for the lognormal distribution was calculated with the reliability coefficient R2=0.7 (Fig. 14b)
3.5 Halogens in the Arctic
Polar halogen activation occurs when halide ions originating from seawater are released via chemistry on snow–ice–aerosols to the atmospheric boundary layer (e.g., Simpson et al., 2007; Abbatt et al., 2012). Bromide is a source of atmospheric bromine (Br2), which is known to be an important species that controls the atmospheric ozone and Hg cycles in the Arctic (see the reviews of Abbatt et al., 2012, and Simpson et al., 2015). Nearly complete ozone depletion events (ODEs) are regularly observed during Arctic spring when molecular bromine is released to the atmosphere through a complex multiphase process, which may require the presence of sunlight and/or acids. Elevated concentrations of reactive halogens in the atmosphere are often co-observed with atmospheric Hg0 depletion events (Saiz-Lopez and von Glasow, 2012).
While observations are numerous, the ability of models to reproduce halogen activation and its impacts has remained limited. For example, WRF-Chem (Weather Research and Forecasting model coupled to Chemistry; Grell et al., 2005; Fast et al., 2006; Peckham et al., 2011) is frequently used to study Arctic aerosol and trace gas cycling. Recently, WRF-Chem was improved specifically for modeling ozone in the Arctic region (Marelle et al., 2017), although at present it does not include any description of the impact of reactive halogens on the Arctic ozone cycle. Figure 15 shows the current ozone-predicting capability of the model during spring and summer compared to surface ozone observations at two Arctic sites. Within iCUPE, we aim to improve model predictions of Arctic halogen activation and related ozone depletion in the atmosphere using the WRF-Chem model. The ultimate goal is to improve the ability to predict the role of halogens in controlling the Arctic atmospheric mercury cycle.
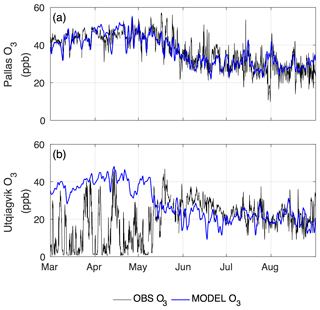
Figure 15Observed and model-predicted ozone concentrations at two Arctic surface stations for March–August 2012; WRF-Chem in blue and observations in black. The model does not capture ozone depletion events observed at Utqiaġvik during Arctic spring. At Pallas, an inland site where no bromine activation is expected, the model in the present state captures the full spring–summer seasonal cycle of ozone.
3.6 Persistent organic pollutants (POPs) and emerging contaminants (EOCs) in the Arctic
Persistent organic pollutants (POPs) and newly emerging organic contaminants (EOCs) were analyzed from five Arctic research stations, Alert (Canada), Pallas (Finland), Storhofdi (Iceland), and Zeppelin (Svalbard, Norway) since 1993 (Hung et al., 2010), as well as at VRS (Greenland) since 2008 (Hung et al., 2016). The air concentration of polychlorinated biphenyls (PCBs), which are organochlorine pesticides, showed a more or less consistent decline in the 1990s. This reduction is, however, less apparent in recent years. In contrast, concentrations of polybrominated diphenyl ethers (PBDEs), hexachlorobenzene (HCB), and some PCB congeners were still found to be increased. The results indicate that the temporal and spatial pattern of POPs in Arctic air is affected by anthropogenic emissions and may be affected by various processes driven by climate change, such as reduced ice cover, increasing seawater temperature, and increasing biomass burning in boreal regions.
EOCs such as poly- and perfluorinated alkyl substances (PFASs), novel brominated flame retardants (BFRs), and organophosphate flame retardants (OPFRs) and plasticizers were investigated in the Arctic environment (Xie et al., 2015; Li et al., 2017; Bossi et al., 2016). Long-range atmospheric processes may have moved particle-bound BFRs to the site, probably during the Arctic haze season. Several modeling studies have been conducted in an attempt to resolve the dominant transport pathway of PFASs to the Arctic, namely the atmospheric transport of precursors versus direct transport via ocean currents (Wania, 2007; Armitage et al., 2009; Stemmler et al., 2010). Atmospheric measurements have shown the widespread occurrence of PFAS precursors, e.g., fluorotelomer alcohol (FTOH) and perfluorinated sulfonamide alcohols (FOSE and FOSA) (Shoeib et al., 2006; Cai et al., 2012). The detection of perfluorocarboxylic acids (PFCAs), perfluorinated sulfonic acids (PFSAs), and neutral PFASs in snow deposition is consistent with the volatile precursor transport hypothesis (Young et al., 2007; Xie et al., 2015). The measurements of PFCAs and PFSAs in seawater from the Greenland Sea indicated melting snow and ice tend to be an input source (Zhao et al., 2012). The inconsistent temporal and spatial trends between regions may be representative of differences in emissions from source regions (Wöhrnschimmel et al., 2013).
The decline of legacy POPs in the environment is expected in response to global efforts to reduce emissions. Whereas, with their persistence in environmental matrices such as water, sediment, soil, vegetation, and ice–snow, a warming Arctic may drive them again into environmental circulation. Consequently, future research should be focused on quantifying remobilization fluxes and sinks for both legacy POPs and emerging contaminants in the Arctic, as well as on developing a quantitative understanding of global exposure pathways for POPs to support risk assessments (McLachlan et al. 2018).
3.7 Satellite remote sensing
Satellite remote sensing is a very useful tool for Earth observation in the Arctic, given the vast size of the area and constraints in accessibility. The sensors that are typically applied range from radar sensors of different frequency, typically X and C band (TerraSAR-X, TanDEM-X, Sentinel-1), to optical sensors (Landsat, MODIS, Sentinel-2 and Sentinel-3) and altimeters (CryoSat-2, ICESat-2, Sentinel-3). In the future a hyperspectral mission (EnMAP) will survey the Arctic. In the framework of iCUPE, we utilize satellite remote sensing data on topics such as Arctic vegetation, snow cover monitoring, and the supraglacial melt of glaciers. Beyond the development of products and the assessment of existing approaches, new techniques like polarimetry for glaciers were also assessed, which will result in recommendations for future satellite missions. Furthermore, we present an example for the integration of satellite remote sensing, airborne observation, and modeling applied to a case of anthropogenic emissions from oil–gas extraction in northern Russia. An analysis of the current observing systems and future needs are summarized, including value chains of satellite missions.
3.7.1 Arctic vegetation
As part of iCUPE, we are working beyond the recent retrievals of satellite remote sensing data to include advanced current and upcoming optical remote sensing missions with improved spatial, temporal, and spectral resolutions and their potential for the characterization of Arctic regions. In particular, hyperspectral remote sensing (or imaging spectroscopy) has been shown to provide superior derivation of key biophysical surface variables in snow-free permafrost areas during the summer months based on field and airborne remote sensing data (Buchhorn et al., 2013; Bratsch et al., 2016; Liu et al., 2017; Beamish et al., 2020). The datasets compiled as part of the iCUPE project (see Sect. 3.8) consist of 4 years of field data, including canopy-level spectral reflectance data and aerial hyperspectral images (Alaska and Canada), and a dense Sentinel-2 time series (Siberia). Additional ground-based data include leaf-level photosynthetic pigment data from multiple phenological phases, as well as aboveground biomass and detailed species composition data. The study sites include Toolik Lake (Alaska), Herschel Island–Qikiqtaruk (QHI; Canada), and the Lena Delta (Siberia). All three sites represent key low-Arctic research stations, with the Toolik and QHI sites having preestablished long-term vegetation monitoring plots. This dataset is unique and provides the potential for further work given the freely available nature of much of the ancillary data and the intensive international research being conducted at all locations. The main goal of this work was to provide an initial characterization and exploration of the application of hyperspectral remote sensing data in Arctic terrestrial ecosystems where existing research is limited.
As a first step to improve the estimation and monitoring of Arctic tundra vegetation, we conducted a detailed spectral characterization of dominant vegetation communities at Toolik Lake, Alaska, with the aim of better informing current and upcoming hyperspectral remote sensing platforms such as the PRISMA (Italy) mission (Loizzo et al., 2018) that started in 2019 and the EnMAP (Germany) satellite planned to be launched in mid-2021 (Guanter et al., 2015). To be noted: as part of the high-priority candidate missions for the Copernicus Sentinel expansion, the European Space Agency (ESA) is considering a hyperspectral mission named CHIME for “Copernicus Hyperspectral Imaging Mission for the Environment” (Rast et al., 2019). To accomplish the ground spectral characterization, we collected canopy-based spectral reflectance in five dominant low-Arctic vegetation communities in three major phenological phases of leaf-out, maximum canopy, and senescence (Fig. 16), and we simulated upcoming EnMAP and Sentinel-2 spectral reflectance. We then examined within and between community variability to determine the most discriminative wavelengths and phenological phase for the ground-based, EnMAP, and Sentinel-2 reflectance.
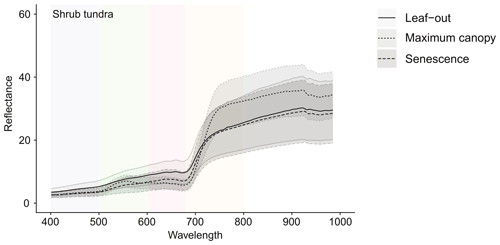
Figure 16Canopy-level spectral reflectance in percent as a function of wavelength in nanometers from a dwarf shrub community in three major phenological phases of leaf-out, maximum canopy, and senescence (Beamish et al., 2017).
The results suggest that for imaging spectroscopy (ground-based and simulated EnMAP) the senescent phase imagery is superior to leaf-out and maximum canopy for differentiating vegetation types, while for Sentinel-2 maximum canopy was superior. The difference between the narrow and broadband data is likely due to the extreme color differences observed during senescence that are well captured by imaging spectroscopy but not by broadband data. These results provide important information for better interpreting current broadband and future narrowband spectral reflectance data for a more accurate estimation of vegetation composition, vigor, and biomass (Beamish et al., 2017).
As a second step, we explored the relationship of spectral information from imaging spectroscopy and digital camera data with biochemical variables at Toolik Lake, Alaska, to assess the utility of low-tech cameras as a ground-based validation tool. To do this we looked at the relationships between narrowband and red–green–blue (RGB) indices and in turn the relationship of these indices to changes in the photosynthetic pigments of chlorophyll and carotenoids. We were again interested in how these relationships vary by phenological phase and vegetation type. The results showed that vegetation color contributes strongly to changes in both narrowband and RGB indices and that these changes are related to changes in photosynthetic pigment concentration (Fig. 17). We conclude that digital cameras can be used to estimate and track pigment development and degradation in low-Arctic vegetation, serving as crucial in situ validation for hyperspectral remote sensing.
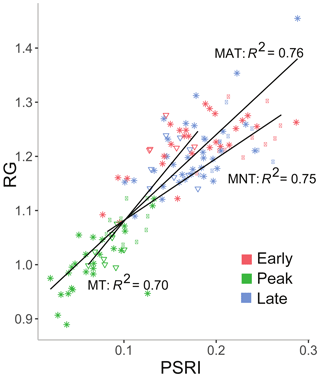
Figure 17The relationships between pigment-driven spectral indices of the plant senescence reflectance index (PSRI) calculated from canopy-level spectral reflectance and the simple normalized red–green index calculated from digital camera data in three dominant vegetation communities. MNT: moist nonacidic tundra; MAT: moist acidic tundra; MT: moss tundra. PSRI is used to track plant senescence related to the degradation of chlorophyll pigments (Beamish et al., 2018).
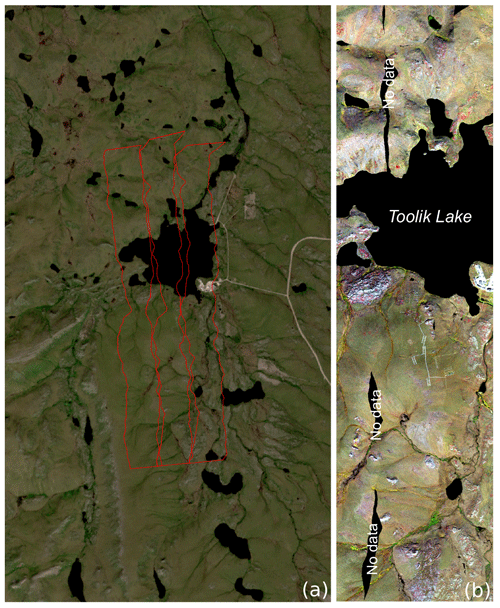
Figure 18Toolik Lake, Alaska. (a) Sentinel-2 image (21 July 2016) with airborne hyperspectral flight lines in red; (b) AISA Eagle hyperspectral image (1.3 m) (Beamish et al., 2020).
In a final effort to explore the applications of hyperspectral remote sensing to the characterization of Arctic vegetation, we explored the spectral sensitivity of airborne hyperspectral imagery to the fractional cover of plant functional types at Toolik Lake, Alaska (Fig. 18; Beamish et al., 2020).
Using detailed plant compositional data, we explored the sensitivity using simple linear regression of individual wavelengths and two-band spectral indices to varying fractional vegetation cover. We found that wavelengths and spectral indices showed overlapping areas of sensitivity to vascular and nonvascular functional types, which could confound the extraction of vegetation properties derived from vegetation indices. We also found that two-band vegetation indices do not provide consistent information across vegetation community types, and the contribution of nonvascular and nonphotosynthetic components influences index values (Fig. 19). The findings of this research point to the importance of a priori knowledge of species composition and phenological phase to accurately interpret vegetation properties derived from imaging spectroscopy.
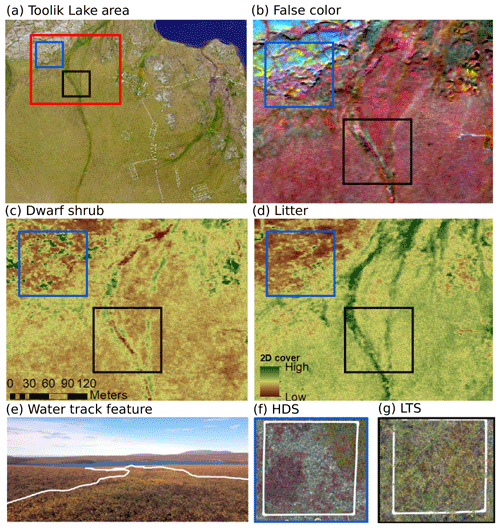
Figure 19(a) A 50 cm aerial photograph of the Toolik vegetation grid (Toolik Lake Environmental Data Centre, https://toolik. alaska.edu/edc/, 2017); the inset represents the extent of hyperspectral imagery below. (b) A false color composite of the northwest corner of the study area – R:812, G:686, B:546 nm. (c) Dwarf shrub 2D cover estimated by NRI λ1:550 and λ2:650 nm. (d) Litter 2D cover estimated by NRI λ1:525 and λ2:600 nm. (e) Field photo of a water track feature from the study area. (f) Hemiprostrate and prostrate dwarf shrub, forb, moss, and fruticose–lichen tundra. (g) Low and tall shrublands (Beamish et al., 2020). The airborne image is provided by the Toolik Lake Environmental Data Centre© (https://toolik. alaska.edu/edc/, last access: 11 January 2016).
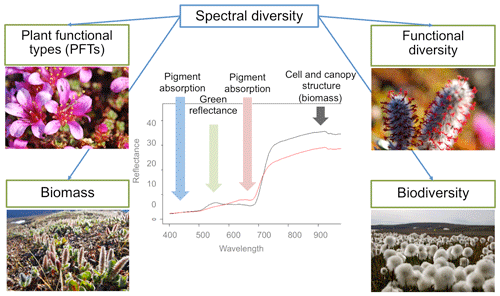
Figure 20Spectral characterization of key biophysical and biochemical variables can inform the interpretation of airborne and satellite hyperspectral remote sensing.
Using data from the Lena River Delta and Herschel Island–Qikiqtaruk, within iCUPE we plan to expand the characterization of Arctic vegetation using imaging spectroscopy to include biodiversity and biomass estimates. Using detailed species composition data and destructive biomass sampling in combination with spectral variables we will explore relationships at the ground, airborne, and satellite scale to best estimate these key biophysical variables (Fig. 20).
In the next step, we aim to provide best practices and proxies for mapping vegetation status and aboveground biomass in the low Arctic at landscape scales with aerial imagery, Sentinel-2, and upcoming hyperspectral satellite imagery. The biomass data will also be compared to volatile organic compound and atmospheric chemistry datasets.
3.7.2 Snow cover in the Arctic
Snow cover is an important component of the cryosphere that plays a key role for climate dynamics and resource availability: the seasonality of the snow cover influences weather patterns, hydropower generation, agriculture, forestry, tourism, and aquatic ecosystems (Beniston et al., 2018). Snow cover characterization and its annual spatial evolution represent important factors to be considered in the framework of climate modeling at a global scale. Furthermore, snow cover has been officially declared an essential climate variable (ECV) by the Global Climate Observing System (GCOS), and high priority is assigned to enhancing and maintaining snow cover observations (WMO, 2011, 2012). From this perspective, the continuous monitoring of snow cover is a major challenge of these last years, and the advances in remote sensing explain why optical data are so diffusely used to monitor snow cover.
The description of snow cover implies two variables: the extent and the albedo. These variables, in the framework of the iCUPE project, are critical inputs for pollutant transport and climate change models. Remote sensing is the most common tool for the routine estimation of the snow cover extent, but two different aspects must be considered for the selection of the most appropriate input: time and spatial resolutions. Both components, using remotely sensed data, are connected to each other because the higher the spatial resolution (below hundreds of meters), the lower the revisit time interval (more than 1 week) (Dietz et al., 2012). Concerning the albedo, the spectral behavior of snow reflectance in the visible and shortwave infrared ranges supports the discrimination of snowed surfaces from other matrices and, moreover, the characterization of the snow surface (Painter et al., 2003; Tedesco and Kokhanovsky, 2007). In detail, the reflectance of pure snow in the visible range of the electromagnetic spectrum (400–700 nm) is approximately 100 %, and it decreases as a function of the amount of impurity in the snow, or, conversely, it slightly increases in relation to the grain size. In the shortwave infrared part of the solar spectrum (700–2500 nm), snow reflectance decreases rapidly and is mostly controlled, in this case, by the snow grain size (Warren and Wiscombe, 1980; Wiscombe and Warren, 1980; Warren, 1982).
State-of-the-art snow products concerning snow extent are derived using remotely sensed data, and they are based mainly on multispectral optical sensors. They can investigate the snow cover and give information about the size and shape of snow grains (Dozier et al., 2009), the presence of impurity soot, the age of the snow, and the presence of depth hoar. Furthermore, the shortwave infrared signal can support the discrimination between snow and clouds (Rodell et al., 2004). Considering that snow-covered surfaces are highly reflective in the visible range and low reflective in the shortwave infrared (SWIR) (Salomonson and Appel, 2006), it is possible to define an index that enhances the discrimination between snow and non-snow in a single pixel. This index, defined as the normalized difference snow index (NDSI), is calculated as follows:
The green and SWIR parameters are the bands available for each satellite sensor, and their selection generally includes wavelength ranges centered at 500–600 nm (RG) and 1500–1600 nm (Rswir). The estimation of the snow extent from remotely sensed multispectral images is based on the relation between the radiative behavior of the surfaces and the fractional snow cover (FSC). This parameter describes the percentage of surface covered by snow (Painter et al., 2009) in a pixel element of a remotely sensed image. The relation between the FSC and the NDSI represents the most common inference required by remote sensing studies. There are two options for estimating the NDSI–FSC relation: the first one consists of combining satellite products with different spatial resolution (Yin et al., 2013), and the second one can be approached by having ground truth information. The first solution is based on Salomonson and Appel (2006), combining Landsat and MODIS data with an NDSI to FSC relation, and is defined as
This knowledge was implemented in the SNOWMAP algorithm (Hall et al., 2016), which is the core of the MODIS data chain for the definition of remotely sensed snow products. This approach is also replicated on Landsat data (Vermote et al., 2016) and in the NASA VIIRS ATBD (Riggs et al., 2015), derived by the Suomi NPP satellite platform. Similarly, it is included in the SC algorithm, which is part of the Sentinel-2A data chain (Main-Korn et al., 2017). The second solution can be approached by defining an empirical reflectance-to-snow-cover model that requires a calibration having a number of reference sites in the satellite image (Solberg et al., 2006), which is the core of the GlobSnow Snow Extent (SE) data chain (Metsämäki et al., 2015). The occurrence of different interferences (cloud cover or snow metamorphism, for example) requires the availability of additional proxies, like webcam networks (Salzano et al., 2019), that can improve the calibration and validation processes of remotely sensed products.
Several satellite products are available for the remote sensing of the cryosphere, and for this study we considered products obtained by optical sensors characterized by different spatial resolutions: high (<100 m); intermediate (100 m–1 km); and low (>1 km). The integration between those products and ground-based imagery will be tested in order to improve the dataset concerning snow cover over a decade.
As part of iCUPE, we aim to develop a semiautomatic procedure focused on preparing a snow product useful for monitoring spatial and spectral variations of the snow cover. The first year was concerned with data collection and the analysis of terrestrial images that will be complementary to Sentinel multispectral images (Fig. 21). We selected a study area located close to Ny-Ålesund (Svalbard, Norway) where the Italian Arctic station represents an important scientific facility and experimental activities are appropriately supported. The site is characterized by intense international research collaborations, and different data sources can be included as input for the integration between remotely sensed data and terrestrial images collected by webcams. We created an overview of the algorithms focused on processing multispectral images and snow index calculations as well as the automatic geo-rectification of ground-based photography and the routine estimation of fractional snow cover. From this perspective, we carried out a first field campaign aiming to survey the available webcams at the study site and deployed a new webcam on the CNR Climate Change Tower. This site has proved to be the most suitable for the collection of ancillary data necessary for a correct interpretation of both satellite and terrestrial images. This CNR infrastructure is equipped with different meteorological sensors as well as instruments focused on detecting the radiative properties of the atmosphere and the snow-covered surface.
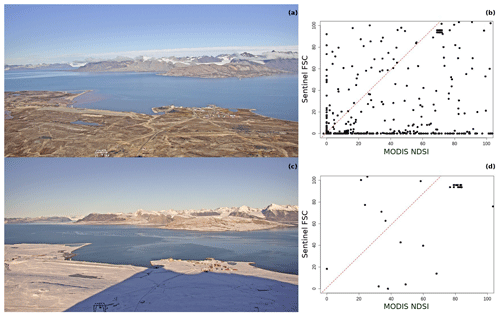
Figure 22The snow cover at Ny-Ålesund during two different seasons: (a) 20 August 2018 and (c) 17 September 2018. (b, d) Relationship between the spectral index of snow (NDSI) obtained by MODIS data and the fractional snow cover estimated by Sentinel-2 and detected by terrestrial photography (Pedersen, 2013).
The core of this activity was the development of a procedure suitable to collect remotely sensed data and match these images to FSC estimations obtained using terrestrial photography. The resampling of snow–non-snow pixels, provided by webcams (Fig. 22a and c) using grids associated with satellite imagery, provided a continuous dataset of FSC to NDSI relations (Fig. 22b and d) that can enhance the recognition of different snow cover types at the considered site. From this point of view, terrestrial photography is available daily or hourly for all the imagery that can be obtained by different satellites, with different revisit times (from 1 to 10 d) and under intense cloud cover (up to 80 % cloudy conditions per year in the Svalbard islands; Salzano et al., 2016). The final output will support the definition of the snow cover extent, including shadowed areas and variations associated with snow metamorphism. Furthermore, we will be able to describe the evolution of the snow cover year by year, with complete timelines that could assess the melt cycle variability over the last decades on a local scale with terrestrial photography or over the last few years on a regional scale using remotely sensed data.
3.7.3 Supraglacial lakes in Greenland
The surface of ice sheets and glaciers in the Arctic, e.g., in Greenland, is prone to melt during summer. As part of iCUPE we focused on the Greenland Ice Sheet given its current contribution to sea level rise and polar amplification, making this area a hot spot of future warming. Whereas the entire ice sheet surface is experiencing melt only rarely, like in the extreme melt year 2012, the margins of the Greenland Ice Sheet are melting between May and August. Meltwater may either percolate into the porous firn matrix or run off along the surface and accumulate in topographic sinks, forming supraglacial lakes. These supraglacial lakes may freeze over during winter, being covered with a lid of lake ice, or drain and deliver vast amounts of water acting as a lubricant to the glacier base. Whatever route the meltwater takes, it also transports previously deposited pollutants during snow accumulation. There are three major questions that we addressed within iCUPE: (i) how much water is drained? (ii) How often does the lake drainage occur? (iii) What are the opportunities and limitations of satellite radar remote sensing to answer these questions?
Within iCUPE we studied an area in northeast Greenland, which contains lakes with the size of 21 km2 and up to 40 m of depth containing volumes of up to 108 m3 of water. The availability of water at the glacier base leads to an immediate speed of 9 %–22 % that is declining over time as the water is discharged (Neckel et al., 2020). Estimating the volume of lakes has been done with differencing digital elevation models (DEMs) obtained from TanDEM-X at different acquisition times. With a revisit time of 11 d, we can limit the drainage duration with this no better than 11 d; however, the combination between optical (Sentinel-2) and TerraSAR-X data allowed us to constrain the duration of one particular drainage event to be shorter than 1 d (Neckel et al., 2020).
With the Copernicus Sentinel program, continuation of satellite missions is assured, which makes the development of methods to derive time series of lake drainage events based on these sensors a desirable approach for obtaining long time series in the future, as the formation, filling, and drainage of supraglacial lakes will increase in the future under warming. Radar remote sensing is most attractive for observing polar areas, as it is independent of clouds and daylight. While the Copernicus program does not contain a bistatic radar mission required for lake volume estimates, Sentinel-1 is a C-band dual polarimetric mission, for which we explore the potential to retrieve quantities for the supraglacial hydrological cycle. Polarimetry allows for the separation of surface reflections from volume reflections using the transmission and retrieval of horizontal (H) and vertical (V) polarized radar waves (Fig. 23b, c).
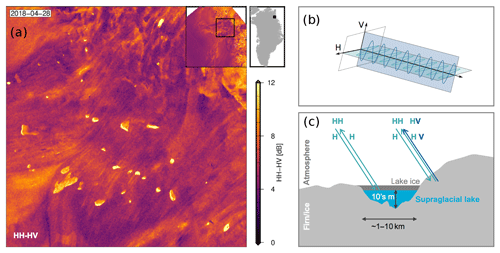
Figure 23(a) Detection of supraglacial lakes using polarimetry with Sentinel-1. Bright yellow denotes surface scattering, which arises from the flat transition between lake ice and water, as shown schematically on the right. (b, c) A schematic representation of the principle of the polarimetric method.
The hypothesis we based our analysis on is that supraglacial lakes act as surface reflectors, whereas the surrounding glacier surface acts as a volume-scattering material (Fig. 23a). With Sentinel-1 being only a dual polarimetric mission (HH, HV), the full decomposition cannot be conducted. However, the difference in amplitude between HH and HV has been used to derive a time series from May 2017 to September 2019 over the 79 N Glacier and Zachariæ Isstrøm, two outlet glaciers of the Northeast Greenland Ice Stream. Figure 23 displays the difference HH–HV in April, thus before the onset of melt. Bright yellow represents areas where surface scattering is dominant, whereas darker purple represents volume scattering. The time series (not shown in Fig. 23) of the difference in amplitude is prone to saturation during the melt period, and consequently the difference HH–HV also cannot be assessed. The areas with large differences HH–HV are coincident with lake locations detected with the DEM differencing, as well as optical satellite imagery, serving as a validation of this method. The vertical polarization arises from the reflection at the transition between lake ice and water. This is another indication for the lakes only being covered with a lid of lake ice and not freezing through entirely, which we also found in airborne radar surveys using AWI's ultrawide-band radar (UWB) on 11 April 2018.
We find polarimetry to be a promising approach for detecting multi-annual lakes of large volume. Polarimetric synthetic aperture radar (SAR) is likely to improve lake detection during the melt season and is currently being investigated. From our assessment, it would be most beneficial to have a full-polarimetric bistatic L-band mission with short repeat cycles covering the polar areas, allowing for SAR tomography, in order to observe englacial aquifers, refrozen melt layers, and their evolution in the next decades.
3.8 Integrating examples
This section presents a suite of integrative examples developed during the iCUPE project, which utilized the comprehensive observation capacities and combined technologies in order to address satellite data validation tasks based on in situ observations, the assessment of aerosol emissions from flaring in the Arctic in a combination of aircraft observations, satellite retrievals, and modeling tools. We also present work towards the development of proxy variables from the comprehensive observations and performed a value tree analysis for the Arctic observation network as a whole.
3.8.1 Satellite data validation
SMEAR II (Hari and Kulmala, 2005) in Hyytiälä is the ground-validation site for the current NASA Global Precipitation Mission (GPM; Skofronick-Jackson et al., 2018) and upcoming ESA EarthCARE satellites (Illingworth et al., 2015). The satellite calibration and validation activities can be divided into two main approaches, i.e., validation and verification of the assumptions and parameterizations used in the retrieval algorithms and direct validation of satellite observations.
Within iCUPE, the main focus of our satellite validation activities was the microphysical assumptions and parameterizations used in ice cloud and snowfall retrievals. Given the climate and comprehensive surface-based observations of precipitating ice particles, our observations allowed us to constrain retrievals and refine these parameterizations. Following the work of von Lerber et al. (2017), the snowflake masses and size distribution were retrieved and used to derive event-specific radar reflectivity–snowfall rate relations. These relations were applied in the validation of ice cloud and precipitation retrieval algorithms. For example, the performance of the “unified” algorithm, which combines the radar and lidar observations of EarthCARE in a variety of snowfall conditions (Moisseev et al., 2017; Li et al., 2018), was tested using snowfall data as shown in Mason et al. (2018, 2019). Using these studies showed that the measurements collected at Hyytiälä, namely combined observations of surface snowfall observations and multifrequency radar observations, show the potential for verification of satellite cloud and precipitation retrieval algorithms in the future.
Direct validation of satellite precipitation products was carried out by applying tuned relations between radar reflectivity and the snowfall rate, as derived from surface precipitation measurements, to the Finnish Meteorological Institute Ikaalinen radar observations. This method was developed by von Lerber et al. (2018) and applied to validate GPM snowfall rate estimates at Hyytiälä, SMEAR II. Using these observations, we showed that the current NASA GPM algorithms underestimate snowfall intensity but have good skill in detecting falling snow.
To prepare for the upcoming ESA EarthCARE validation activities, preparatory studies were performed on how ground-based radar observations can be used to validate space-based radar observations of the vertical profiles of clouds and precipitation. A comparison between such observations (in this case using CloudSat) is shown in Fig. 24. The figure shows vertical profiles of radar reflectivity as observed using ground-based and satellite-based cloud radars. As can be noticed there are detectable differences in the observed values. The CloudSat observed values are higher at cloud tops and lower in precipitation if compared to the ground-based radar. These differences are caused, on the one hand, by attenuation of ground-based radar measurements due to rain and the melting layer and, on the other hand, by attenuation of CloudSat observations due to clouds and the melting layer. Therefore, a direct comparison of observed vertical profiles requires a method that can take into account the attenuation. As part of iCUPE, Li et al. (2019) studied the impact of the melting layer of precipitation on cloud radar observations. The results of this study will be used for EarthCARE validation in the future.
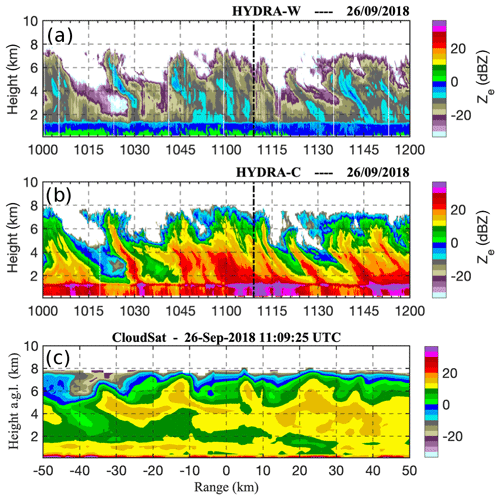
Figure 24Observations of clouds and precipitation using Hyytiälä (a) cloud, (b) precipitation radars, and (c) CloudSat cloud profiling radar. The vertical dash-dotted line in the panels indicates the CloudSat overpass time. The x axis in the CloudSat panel shows the distance between the Hyytiälä site and the satellite ground track. Simulated concentrations of BC tracer with a 5 d lifetime (µg m−3) (a) along the YAK flight on 15 October 2014 (flight altitude also given, white line) and (b) at the surface at 05:00 UTC on 15 October 2014; flight tracks are also shown. BC tracer concentrations are normalized to the total BC emissions from Huang et al. (2015) over the model domain and emitted based on annual mean flaring locations using VIIRS data.
3.8.2 Anthropogenic emissions from oil–gas extraction over northern Russia: a combined approach using aircraft, satellite data, and modeling
Aircraft data collected for the first time over northern Russian oil- and gas-producing regions were used to examine the validity of available emission inventories. The data were collected in October 2014 and July 2017 as part of the French–Russian YAK-AEROSIB program (Paris et al., 2010; Antokhina et al., 2018; Fig. 25). A combination of different approaches was applied, involving joint analysis of aircraft and satellite data together with source–receptor modeling using FLEXPART/FLEXPART-WRF and regional WRF-Chem modeling (Marelle et al., 2017, 2018) to investigate discrepancies in both black carbon (BC) and methane (CH4) anthropogenic emissions over Russia, where very few data are available to date.
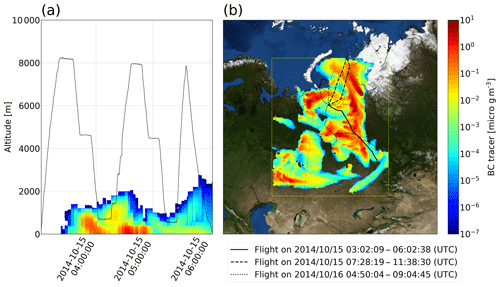
Figure 25Simulated concentrations of BC tracer with a 5 d lifetime (µg m−3) (a) along the YAK flight on 15 October 2014 (flight altitude also given, white line) and (b) at the surface at 05:00 UTC on 15 October 2014; flight tracks are also shown. BC tracer concentrations are normalized to the total BC emissions from Huang et al. (2015) over the model domain and emitted based on annual mean flaring locations using VIIRS data.
Analysis of equivalent BC data collected over northern Siberia in October 2014 showed clear signatures of enhanced concentrations in the lower troposphere, especially near the surface (Antokhina et al., 2018). The origins of polluted air masses sampled during flights over the Ob Valley, Yamal, and Kara Sea regions during October 2014 were analyzed using WRF-Chem BC tracer runs with emissions from different sectors using the ECLIPSEv5 (Klimont et al., 2017) and Arctic black carbon (ABC) (Huang et al., 2015) inventories, with several plumes clearly identified as coming from the gas flaring regions. Backward FLEXPART-WRF simulations from the plumes were used to compare emission footprints for the Huang ABC and ECLIPSE BC flaring emissions. Discrepancies were identified in both inventories, and in some cases there were completely missing emissions, particularly over the Nenets region (Onishi et al., 2020). The data are being further analyzed using daily VIIRS (Visible Infrared Imaging Radiometer Suite) satellite night-light data (provided courtesy of Earth Observation Group, Payne Institute for Public Policy, https://eogdata.mines.edu/download_viirs_fire.html, last access: 2 July 2020) to examine the sensitivity of modeled BC to daily variability in flaring emissions. Preliminary results suggest that the simulated BC is highly sensitive to significant observed variability.
Methane emissions from oil and gas activities are similarly poorly known, and their spatial and temporal distribution is related to that of BC (e.g., Gvakharia et al., 2017). Inventories are affected by (1) highly variable emission factors, (2) highly variable scheduled or unscheduled operations leading to the release of methane, and (3) the uncertain spatial distribution of regional totals (Fig. 26a). The inventory EDGAR v4.3.2 (Janssens-Maenhout et al., 2017) predicts a total emission for energy in western Siberia of 2.8 Mt CH4 yr−1, whereas ECLIPSE v5a (Höglund-Isaksson et al., 2012) yields 14.1 Mt CH4 yr−1 (Fig. 26b). Similar to BC, the YAK-AEROSIB campaigns were used to integrate in situ observations into methane inventory validation. A comparison between observations and modeled methane enhancement using the FLEXPART model revealed overall that the most descriptive signals are contained in the occurrences of boundary layer measurements. Typically, the methane enhancements in these measurements are estimated by a tagged tracer analysis to originate approximately 60 % from regional wetland emissions. We used the ORCHIDEE model (Poulter et al., 2017) to subtract the contribution of these widespread ecosystems to the methane enhancements detected in the boundary layer and estimate the compatibility of emission inventories with the airborne measurements. However, this introduces significant uncertainty in the constraint that can be applied to methane emissions from oil and gas that needs to be investigated though comparison with a different wetland model.
3.8.3 Aerosol vertical profiling in Russia and Finland
Within iCUPE, data collected at ground-based lidar sites in Siberia and in southern Finland provided new insights into aerosol sources affecting atmospheric composition in the Arctic and sub-Arctic regions.
Russia
Aerosol backscatter vertical profile data collected in Tomsk, Siberia, and corresponding aerosol optical depth (AOD) have been derived using a micropulse lidar at 808 nm and a novel lidar data processing approach based on a careful system calibration and an aerosol source apportionment based on FLEXPART backward transport simulations of an aerosol tracer including dry and wet removal processes (Ancellet et al., 2019). The potential emission sensitivity maps from the FLEXPART simulations were coupled with the spatial distribution of five aerosol sources to obtain the aerosol source apportionment: urban pollution maps (cities of more than 500 000 inhabitants), biomass burning daily maps (NASA Fire Information for Resource Management System – FIRMS using MODIS, Giglio et al., 2003; the Visible Infrared Imaging Radiometer Suite – VIIRS, Schroeder et al., 2014), forest and desert maps (Advanced Very High Resolution Radiometer – AVHRR database, Sertel et al., 2010), and gas flaring emission maps (ECLIPSEv54 database – Evaluating the CLimate and air quality ImPacts of Short-livEd pollutants; described in Klimont et al., 2017).
An example of a lidar aerosol vertical profile is shown in Fig. 27a for a mixture of gas flaring emissions from the Ob Valley below 3 km (Fig. 27e) and a dust layer transported from Kazakhstan above 3 km (Fig. 27c) during a time period with no forest fires in Siberia. Aerosol-scattering ratios at 808 nm increased by a factor of 3 due to this event, while the corresponding AOD reached 0.3, in good agreement with the Tomsk AERONET sun photometer observations and the MODIS hot spot near TOMSK (Fig. 27b). Elevated CO columns seen by the Infrared Atmospheric Sounding Interferometer (IASI) above the Ob Valley and Tomsk also support gas flaring emissions during this period. A CALIOP overpass (black line in Fig. 27b and d) also provides a latitudinal distribution of the aerosol layers, showing the limited vertical extent (2 km) of the aerosol layer at 55∘ N transported from the gas flaring region, while dust layers reached 4 km at latitudes below 50∘ N over Kazakhstan.
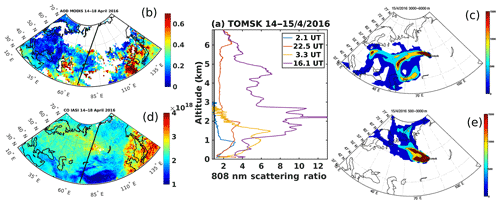
Figure 27Vertical profiles of the scattering ratio on 14 and 15 April 2016 from Tomsk lidar (a) and the corresponding 5 d average map of AOD at 532 nm from MODIS (b) and of the CO total column (in molecules per square centimeter) from IASI observations (d). The red circle is Tomsk, and the thick black lines are the CALIOP overpass over Tomsk on 14 April at 21:00 UTC. Potential emission sensitivity (PES) distributions of an aerosol tracer averaged for 9 d before the Tomsk lidar aerosol layer observations below 3 km (e) and above 3 km (c) are calculated with backward simulations of the FLEXPART model using the dry and wet deposition of the tracer during backward transport.
The fraction of all the aerosol layers detected by the lidar from April 2015 to October 2016 was derived for the five aerosol types observed in Ancellet et al. (2019). The results show that the occurrence of layers was linked to natural emissions (vegetation, forest fires, and dust) and their contribution was high (56 %). However, anthropogenic emissions still contributed up to 44 % of the detected layers (1∕3 from flaring and 2∕3 from urban emissions). In Tomsk, the frequency of dust events is very low (5 %). The contribution to the largest AOD (>0.1) showed that the frequency of forest fires (25 %) and urban pollution events (25 %) was dominant, while the frequency of flaring (10 %) and dust emissions (13 %) is equivalent and 2 times lower in frequency. The flaring emissions are indeed frequent but do not contribute very much to the large-optical-depth cases. Our results show that the aerosol particles originating from urban and flaring emissions remain confined below 2.5 km, while the aerosol particles related to dust events were mainly observed above 2.5 km. The aerosols from forest fire emissions are, in contrast, observed both within and above the planetary boundary layer.
Finland
Based on a combination of ground-based high-spectral-resolution lidar (HSRL; Shipley et al., 1983) observations and airborne in situ measurements, we have analyzed the boundary layer and aerosol layers aloft over a relatively clean SMEAR II. An HSRL was one of the deployed instruments during the BAECC (Biogenic Aerosols – Effects on Cloud and Climate) campaign (Petäjä et al., 2016) that was organized at the station from February to September 2014. A scanning mobility particle sizer (SMPS) and optical particle sizer (OPS) were installed onboard Cessna FR172F aircraft (Schobesberger et al., 2013; Leino et al. 2019) to measure aerosol size distribution from 0.1 to 0.23 µm and 0.3 to 5 µm, respectively. The flights were conducted in the vicinity of the station not higher than 4 km in altitude with a relatively low speed of 200 km h−1, thus providing observations with good spatial resolution.
We analyzed a set of clear-sky and cloudy cases (Nikandrova et al., 2018) at Hyytiälä during the BAECC campaign. HYSPLIT 96 h backward trajectories were calculated every 50 m and combined into layers based on the similarities in the origin and traveling path, then compared with the layers recognized with HSRL. Most of the layers were recognized in both approaches, yet the arrival heights of the back trajectories were not always similar to the heights of the layers from HSRL. These discrepancies are due to small-scale vertical motions that are not resolved in the HYSPLIT model (Stein et al., 2015).
In both clear-sky and cloudy cases, elevated layers with high aerosol concentrations were detected with both the HSRL and with airborne measurements. One of the clear-sky case studies of 9 April 2014 is shown in Fig. 28. The boundary layer (BL) can be visually recognized from the backscatter coefficient to be around 1.4 km in the afternoon when the flight took place (Fig. 28a). Figure 28b shows HYSPLIT backward trajectories divided into three layers and their arrival heights. The aircraft data showed a similar shape of the size distribution for the elevated layers and in the boundary layer during the flight with a higher aerosol concentration in the boundary layer (Fig. 28c). Small variability in the size distribution in the BL suggests a well-mixed BL, whereas in the elevated layers the internal variability was larger and the layers were not mixed as thoroughly. Back-trajectory analyses showed that the air mass origin was similar regardless of the arrival height, which indicated that both the BL and the elevated layers were affected by similar aerosol sources (Fig. 28d). Therefore, the differences in the number concentration and size distribution were mostly due to differences in their dilution during transport to Hyytiälä. Nucleation-mode particles are also seen in the middle layer, and, assuming that this layer did not experience strong mixing for several days, we suppose that these new particles were formed in this elevated layer.
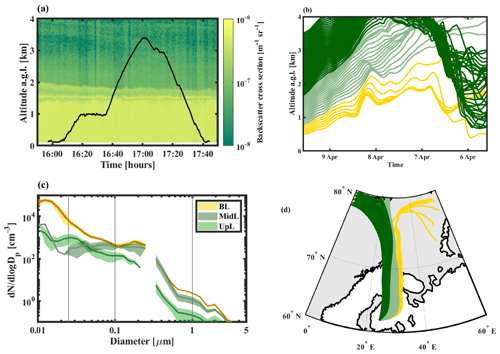
Figure 28Clear-sky case study during 9 April 2014 at SMEAR II, Finland: (a) HSRL backscatter coefficient with Cessna flight altitudes in black; (b) 96 h backward trajectories calculated every 50 m and combined into layers according to similarities in the traveling path; (c) aerosol size distribution measured onboard during the flight and combined into layers, shown with 1 standard deviation; (d) spatial coverage of backward trajectories
Certainly, higher aerosol concentrations within the elevated layers are periodically present in Finland. These layers can influence, for example, the columnar optical closure in the boreal environment (Zieger et al., 2015). More detailed analysis is needed in order to address the relative contribution of different aerosol sources to these layers and to compare the results with the data available in Tomsk, Russia.
3.8.4 Deriving proxy variables from in situ and satellite data
In iCUPE, we developed proxies for atmospheric variables from the in situ data gathered at Finnish measurement sites. In practice, this means that we derive formulas that describe the proxy variables as functions of more commonly measured parameters. Previously, proxies have been derived mainly for concentrations of gas-phase molecules, such as sulfuric acid (Petäjä et al., 2009; Mikkonen et al., 2011) and monoterpenes (Kontkanen et al., 2016). In iCUPE, we are developing proxies for condensation sink (CS), boundary layer height (BLH), and ecosystem-level gross primary production (GPP). The aim is to produce proxies that rely on variables that can also be retrieved from satellite data in order to produce proxies with good spatial coverage (e.g., Kulmala et al., 2011b). Here, we describe the CS proxy development, while the proxies for BLH and GPP remain under development.
Condensation sink (CS) describes the loss rate of vapor molecules due to their condensation on aerosol particle surfaces (Kulmala et al., 2001). Therefore, it depends strongly on the particle surface area, which is determined mainly by the concentration of particles with diameters close to or over 100 nm. Particles in this size range are introduced into the atmosphere directly from various combustion sources, especially from the residential combustion of wood and other biofuel (Paasonen et al., 2016), and grown from smaller sizes by condensation of mainly biogenic vapors (Riipinen et al., 2011; Paasonen et al., 2013). In our proxy we use carbon monoxide (CO) as a tracer for aerosols produced in combustion processes and air temperature for estimating the formation of biogenic secondary organic aerosol. Air temperature has been shown to correlate with the concentrations of particles in the relevant size range in various continental environments (Paasonen et al., 2013).
We determined the proxy for daily averages of CS for measurement sites at SMEAR II in Hyytiälä (southern Finland), at SMEAR I in Värriö, and in Pallas (northern Finland). When determining the dependence between CO concentration and CS, we observed a clear pattern in the seasonal minima of CO concentration at the sites (Fig. 29a). Since this pattern is not reflected in CS, but presumably originates from the longer atmospheric lifetime of CO during dark months, we proceeded with inspecting the relation between CS and ΔCO, which describes the difference between the observation and the 5th percentile of concentrations at the respective time of year. In Fig. 29b we show the dependence of CS on ΔCO in different temperature bins in Värriö. We calculated average slopes (bave) for the fittings in Fig. 29b for each measurement site and evaluated the intercepts of the new fittings, to which this average slope was applied. These intercepts are depicted for the sites in Fig. 29c. These intercepts reflect the expected temperature dependence, whereby CS is not impacted by temperature at T<5 ∘C when ecosystem emissions are generally low, and increasing positive temperatures cause an increase in CS. Finally, we formulated the temperature-dependent part of the proxy (a1(T)) by calculating the average intercepts at T<5 ∘C and making a fit to the intercepts at positive temperatures (Fig. 29c). This produces the final proxies for CS (Fig. 29d):
As a next step for the CS proxies, we will derive and test a proxy from a dataset combined from all different sites, study how the proxies work for data from other polar stations, and investigate whether CO and temperature retrieved from the satellite data can be applied for the proxies.
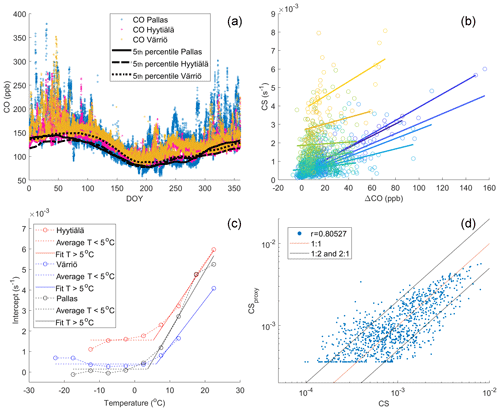
Figure 29Derivation of the proxy for condensation sink (CS) based on the carbon monoxide (CO) concentration and air temperature. (a) Annual pattern of CO concentration and its 5th percentiles at the observation sites, (b) observed CS in Värriö as a function of the difference between observed CO and the 5th percentiles from panel (a) in 5 ∘C temperature bins (blue colors for T<5 ∘C, green and yellow for T>5 ∘C), (c) intercepts ai(T) in fittings to the data in panel (b) when slopes are forced to their average bave as functions of temperature, and (d) the resulting proxy as shown in Eq. (3).
3.8.5 iCUPE data flow
One of the main outcomes of iCUPE involves new data products developed based on the comprehensive use of in situ and satellite remote sensing. The new iCUPE data products, which will fill, e.g., the observational gap for the key variables persisting organic pollutants (POPs), chemicals of emerging concern (CECs), short-lived climate forcers (SLCFs), and atmospheric trace gases in the polar context, are being codesigned together with different end users. The list of data products is presented in Table 1. End users for the iCUPE datasets include the European Environment Agency, geoengineering bodies, decision makers, intergovernmental organizations, local government and environmental administration (ministries), citizens, and national and European weather services. The data can be used to improve models, advance our scientific understanding of the Arctic regions, support policy making, and improve weather predictions and mechanisms of risk control for natural hazards, sea traffic control, tourism, and chemical weather prediction.
Table 1The iCUPE products as datasets resulting from research activities of the project and collaboration with PEEX Russian partners. The first dataset was delivered in December 2018.
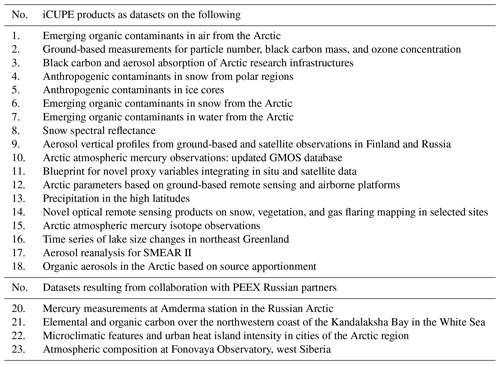
In total, iCUPE will provide more than 20 datasets as products usable for researchers, decision and policy makers, stakeholders, and end-user communities. These will be a valuable and important contribution from the iCUPE project activities in piloting open access to Arctic data. All these datasets will be publicly available for different applications and services. Focusing on the Arctic region territories, the planned datasets will include, e.g., novel data on anthropogenic contaminants in snow and ice cores, organic contaminants in the air–snow–water, and concentrations of different chemical species and aerosols, as well as their characteristics including vertical profiles. Within iCUPE we will also develop specific datasets focusing on selected geographical areas in northern latitudes. The data provision is open, and Russian iCUPE collaborators have also provided data from the Russian Arctic, such as atmospheric mercury measurements at Amderma station, elemental and organic carbon over the northwestern coast of the Kandalaksha Bay in the White Sea, and microclimatic features and urban heat island intensity in cities of the Arctic region.
Each of the datasets is promoted through “teasers” (https://www.atm.helsinki.fi/icupe/index.php/datasets/submitted-datasets, last access: 29 June 2020), which provide the metadata information pertinent to the datasets. The majority of archived datasets (as products) are directly linked (and downloadable), and corresponding metadata are included. The University of Helsinki will take responsibility for long-term storage, accessibility, and maintenance. The raw data will be hosted and maintained by the dataset providers.
As soon as more iCUPE data products are available, we will explore different platforms to disseminate the iCUPE datasets. To facilitate and standardize access to data, cloud-based online platforms, known as Data and Information Access Services (DIAS), provide storage, centralized access, and handling for data and processing tools. DIAS platforms (CREODIAS – creodias.eu; SOBLOO – sobloo.eu; MUNDI – https://mundiwebservices.com, last access: 29 June 2020; ONDA – https://www.onda-dias.eu/cms, last access: 29 June 2020; WEkEO – https://www.wekeo.eu, last access: 29 June 2020) allow users to explore, process, and download Copernicus data and information as well as to have the ability to process and combine data from other sources. It is also possible to develop and host new applications on such platforms. Other tested platforms for the preprocessing, post-processing, and analysis of iCUPE data include the Virtual Laboratory (https://vlab.geodab.org, last access: 29 June 2020), Google Earth Engine (https://earthengine.google.com, last access: 29 June 2020), Polar Thematic Exploitation Platform (https://portal.polartep.io, last access: 29 June 2020), and Global Earth Observation System (https://www.geoportal.org, last access: 29 June 2020). We will continue to explore these options for iCUPE data provision in the future.
All final data products and proxies developed will follow interoperability and data sharing principles endorsed by GEOSS. We will also explore possibilities to maximize the use of new data products and advertise these to larger communities in several community portals such as Sustaining Arctic Observation Networks (SAON), the Arctic Observation System and Arctic Portal, and the Pan-Eurasian Experiment (PEEX) program related to the Arctic–Boreal Hub Portal. The SAON portal expands the visibility of iCUPE data to the circumpolar context and PEEX to Russian and Chinese researchers and other end-user communities. For selected topical datasets, some will be included in the University of Helsinki smart-SMEAR platform (Junninen et al., 2009) and disseminated via metadata catalogs to a wider audience. As a whole we need to utilize the full capacity of the multi-platform approach (in situ, satellites, and models) to address the fate of pollutants in the Arctic. This will provide tools to perform targeted reductions but can also provide new insights into the life cycle of pollutants in the Arctic environment.
3.8.6 Observation system analysis
In addition to investigating new observation capabilities, iCUPE was tasked with evaluating the connectivity and scale of satellite observations for Arctic needs. This task was expanded and elaborated by the SAON initiative “Arctic observing system assessment framework” (STPI/SAON, 2017). The framework links societal benefits to the observing system. Within the framework we identified 170 common objectives classified under 12 societal benefit areas from international Arctic strategy documents. These objectives identify a need for Earth observation information to enable informed decision-making in the Arctic context. In this work, a team of domain experts from SAON, AMAP, and iCUPE evaluated the assessment framework value tree for atmosphere and ocean variables.
The basis of the analysis includes observation capacity. Earth observation (EO) inputs like SYNOP station measurements of the physical atmosphere and, from other stations, ocean variables, were linked to key products, outcomes, and/or services such as numerical weather prediction. Groups, in this case the weather service, were also connected to the key objectives of the assessment framework (Fig. 30), such as weather or environmental information services.
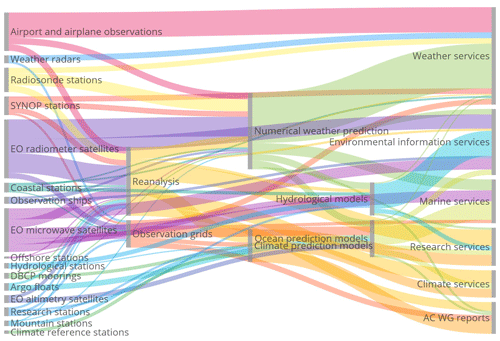
Figure 30The observations, modeling, and service parts of the value tree – the source tree; iCUPE actions are represented in research stations, EO satellites, and observation grids supporting research services. The framework foresees three levels, but modeling feeds its own components, which adds more depth to the tree. The observations (left side) originate from different platforms, such as airplanes, ships, surface synoptic observations (SYNOP), Data Buoy Cooperation Panel (DBCP) moorings, Argo floats, or Earth observations (EOs) from satellites. Some observations are utilized in services directly, and some are integrated into observation grids, or they provide data for reanalysis. The combination of observations and various models provides the required data for the different services (right side), such as weather, marine, and climate services as well as, e.g., data for Arctic Council Working Group (AC WG) reports.
We weighted the analysis by annual costs for operation. Representative yearly unit costs of EO inputs and modeling components were estimated by station experts or estimated based on European Union projects or Copernicus program tenders. For observational capacity and its monetary value, we utilized the WMO OSCAR database for satellite and surface observation systems (https://www.wmo-sat.info/oscar/, last access: 29 June 2020) north of 60∘ N. This provided us with the volume of stations in different station and mission categories in the Arctic (Table 2). Based on our analysis, the total yearly value of this observation system, including EO inputs and modeling, is over EUR 204 million. Compared to the observing system estimated costs in the area 30 to 60∘ N, this is only about one-fifth.
Table 2WMO OSCAR statistics on operational stations in 30∘ latitude slices; iCUPE stations are mostly nonaffiliated.
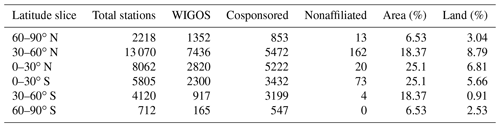
Another way to scale Arctic needs is to look at the distribution of stations globally for operational networks: the WMO has organized the integrated ground-observing system WIGOS stations on land; cosponsoring is mainly with the International Maritime Organization (IMO) for marine platforms, and nonaffiliated institutions include research organizations distributing information in near-real time. The Arctic slice compared to northern midlatitudes has about one-sixth of the stations with about one-third of the aerial coverage. Doubling current observing efforts would be needed to achieve similar coverage in the Arctic.
The value chain from the observations through modeling frameworks into services and decision-making is presented in Fig. 30. It is an estimation of the data flows from different stations and satellites to modeling systems and onwards to services carrying the weight of the total investment forward divided in terms of the importance that the next step places on the information. The costs of modeling have been estimated and added to the value tree. Services are an important element that is included by giving each connection in the tree a value of EUR 1 million annually. This cost represents the global effort and with the included connections amounts to EUR 81 million per year. To compare this value, it corresponds to the annual costs of the Finnish Meteorological Institute at EUR 74 million, which operates 429 meteorological stations, all included as part of the Arctic observation capacity in Table 2. In addition to FMI, there are at least six other similar institutes serving the Arctic, so the estimate is reasonable.
The decision-making step in the value tree is channeled through six services, which include research activities and efforts to consolidate research results in Arctic Council working group reports, the International Panel on Climate Change, or the Intergovernmental Science-Policy Platform on Biodiversity and Ecosystem Services (IPBES) for biodiversity as one-off actions. Continuous services are established for weather, climate, marine, and environmental information. The full value tree that connects all services to 170 key objectives from the societal benefit areas is complex but could help to attribute the costs of an observing system fairly to all the areas that use it. Browsing the full tree can be done on the web at http://arctic-obs.fmi.fi/ (last access: 29 June 2020) and in Strahlendorff et al. (2019). The iCUPE activities are grouped in the value tree under research stations, observation grids, and research services. The costs could not be determined in detail, but the activities fit into the research station estimate although the in situ monitoring activities are very diverse. Travel costs can be substantial, but this component could not be averaged usefully over the range of different research stations.
In conclusion, a doubling of Arctic observing efforts from satellites and in situ is necessary and reasonable. This amounts to a yearly increase of EUR 200 million to observing infrastructure and the production value chain. The European Union space program is planning additions to the satellite component with Sentinel expansion missions, but additions to the in situ component are missing a funding action.
The polar regions are facing changes in the future, both in terms of climate change influenced by Arctic amplification and due to ongoing megatrends such as globalization that drive the development, e.g., of new transport routes through the fragile Arctic environment and extensive utilization of natural resources within the Arctic. The environment in the Arctic is at risk. To address aspects related to Arctic change, we set up the ERA-PLANET Strand 4 project “iCUPE – integrative and Comprehensive Understanding on Polar Environments” to provide novel insights and observational data on global grand challenges with a polar focus.
In iCUPE, our concept is to utilize an integrated approach combining in situ observations, satellite remote sensing Earth observations (EOs), and multi-scale modeling to synthesize data from comprehensive long-term measurements, intensive campaigns, and satellites to deliver data products, metrics, and indicators to stakeholders concerning the environmental status, availability, and extraction of natural resources in the polar areas.
The circumpolar coverage of in situ atmospheric observations has developed during the last decades. In selected locations, such as Svalbard, observations are available for assessing the decadal variability of key observables, such as black carbon. The geographical coverage should be particularly expanded in the Russian Arctic (Petäjä et al., 2020), although a lot of observation sites are operated already (Alekseychik et al., 2016). However, the connection of various continuous national activities and intensive campaigns within the Arctic should be coordinated in order to reduce operation costs. The observations should take advantage of the data harmonization procedures set up, e.g., by WMO-GAW and European research infrastructures (ICOS, ACTRIS, eLTER). Work towards open data sharing should be continued.
Harmonized and open data are crucial for the development of services based on observations within the Arctic. We need to maintain and improve a comprehensive and continuous observation network of in situ observations in the Arctic that is sustained for extended periods of time to monitor the concentration of atmospheric pollutants. This gives us verification data on political decisions to reduce the emissions of harmful compounds but also enables us to respond to arising new threats to the environment. The in situ observations analyzed and performed within iCUPE underlined that we need to utilize the full capacity of the multi-platform approach (in situ, satellites, and models) to address the fate of pollutants in the Arctic. New observational needs should be analyzed, e.g., in the framework of essential variables (EVs) that connect to the sustainable development goals of the United Nations.
The long-term observations at Svalbard revealed that aerosol concentrations are strongly modulated with seasons. This is apparent in Svalbard equivalent black carbon concentrations and in aerosol number concentrations detected at Villum Station in northern Greenland. The comprehensive source apportionment of the organic aerosol fraction at different observation sites in the Arctic indicated that the concentrations are affected by a combination of regionally specific sources and long-range transport of anthropogenic aerosol particles. Vertical profiling can provide novel insights into pollution transport and dispersion in the Arctic environment. The combined use of aircraft observations, modeling tools, and emission inventory analysis can provide novel insights into the variability of atmospheric pollutants within the Arctic.
Similar to the decrease in black carbon, the decline of legacy POPs in the environment is expected under global efforts. Whereas, with their persistence in environmental matrices such as water, sediment, soil, vegetation, and ice–snow, a warming Arctic may drive them to again be available for environmental circulation. Consequently, future research should be focused on quantifying these remobilization fluxes and sinks for both legacy POPs and emerging contaminants in the Arctic.
Within iCUPE, we determined concentrations of mercury in different compartments and fluxes between these compartments in the land–atmosphere continuum. The mercury flux to the Arctic environment through riverine discharge was found to be important. The concentration of mercury in the snow varied as a function of snow depth, indicating a crucial role of atmospheric deposition. Long-term observations of atmospheric mercury in the Russian Arctic were found to be connected with the distance to the sea. The modeling results underline the interconnected life cycle between mercury halogen compounds and atmospheric oxidants.
Furthermore, the remote sensing activities in the iCUPE project highlighted the need for a ground truth concerning the fractional snow cover. This kind of information can be obtained by terrestrial photography, and it represents a tool in common between remotely sensed products useful for integrating satellite data with different spatial and time resolutions. This approach can be very effective in high-latitude areas where illuminating conditions, topography, and cloud cover limit the use of optical remote sensing.
The satellite remote sensing work within iCUPE revealed that imaging spectroscopy, also known as hyperspectral remote sensing, for Arctic vegetation provides valuable information on vegetation status and biomass that can improve our understanding of long-term vegetation trends derived from broadband data. Spectral information stored in the narrow wavelengths of the visible spectrum is the most promising for differentiating spectrally similar vegetation communities and for delivering data on photosynthetic activity and biomass. The lack of ground-validated datasets and an overall scarcity of dense, high-quality image time series remain a challenge. The increasing availability of hyperspectral data will face similar challenges, and given the limited number of hyperspectral remote sensing studies in the Arctic, high-quality, ground-validated data are required to accurately interpret these dense and complex datasets. The rise of more advanced classification methods such as machine-learning techniques is highly promising for Arctic vegetation mapping using advanced remote sensing platforms. An increased effort to develop Arctic-specific algorithms is needed.
Within iCUPE, the benefits of combining in situ and satellite remote sensing with multi-scale modeling in cryospheric, terrestrial, and marine regimes as well as the atmospheric domain are clear. Such integrative activities need to be expanded and continued in order to provide verified environmental data and services in the changing Arctic.
The data presented in this paper are available through the authors upon request. The iCUPE data products are available through the iCUPE web page: https://www.atm.helsinki.fi/icupe/index.php/datasets (iCUPE, 2020).
TP had the initial idea. All authors contributed to specific sections of the paper and commented on the paper. TP, EMD, KT, JS, and HKL formed the editorial team consolidating the text.
The authors declare that they have no conflict of interest.
This article is part of the special issue “Pan-Eurasian Experiment (PEEX)”. It is not associated with a conference.
We thank Jacques Pelon for assistance with research conducted at LATMOS and IGE. The Royal Danish Air Force is acknowledged for providing free transport of equipment to Station Nord, and the staff at Station Nord is especially acknowledged for excellent support. For UAV measurements in Ny-Ålesund we thank the whole AWIPEV team and Kingsbay crew for support during preparation and for hosting us. Janne Levula and the staff of the Hyytiälä Station for Measuring Ecosystem–Atmosphere Relations II (SMEAR II), as well as Nicki Hickmon and Michael Ritche, are acknowledged for their work during the BAECC project.
This project has received funding from the European Union's Horizon 2020 research and innovation program under grant agreement no. 689443 via project iCUPE (Integrative and Comprehensive Understanding on Polar Environments) and through ACTRIS2 (654109). Additional financial support was received through the Academy of Finland (Center of Excellence in Atmospheric Sciences) under the projects PROFI-3 (decision number 311932), NANOBIOMASS (decision number 307537), and SA-MOSAiC (decision number 333397), as well as the Belmont Forum project “Community Resilience to Boreal Environmental change: Assessing Risks from fire and disease” (ACRoBEAR; decision number 334792). Olga Popovicheva received partial support from the Russian Foundation for Basic Research (RFBR) under project no. 18-05-60084. Ground-based lidar observations in Siberia were supported in part by the Ministry of Science and Education of the Russian Federation (agreement no. 14.616.21.0104; unique identifier RFMEFI61618X0104). The French groups received support from the French Arctic Initiative project “Pollution in the Arctic System” (PARCS). Part of this study was supported by the Danish Environmental Protection Agency and the Danish Energy Agency by means of DANCEA funds for Environmental Support to the Arctic Region. Part of this study was funded by the German Science Foundation (Deutsche Forschungsgemeinschaft, DFG) as project numbers LA 2907/5-3, BA 1988/14-3, and WI 1449/22-3. Julia Schmale holds the Ingvar Kamprad Chair, sponsored by Ferring Pharmaceuticals.
Open-access funding provided by Helsinki University Library.
This paper was edited by Imre SALMA and reviewed by two anonymous referees.
Abbatt, J. P. D., Thomas, J. L., Abrahamsson, K., Boxe, C., Granfors, A., Jones, A. E., King, M. D., Saiz-Lopez, A., Shepson, P. B., Sodeau, J., Toohey, D. W., Toubin, C., von Glasow, R., Wren, S. N., and Yang, X.: Halogen activation via interactions with environmental ice and snow in the polar lower troposphere and other regions, Atmos. Chem. Phys., 12, 6237–6271, https://doi.org/10.5194/acp-12-6237-2012, 2012.
Alekseychik, P., Lappalainen, H. K., Petäjä, T., Zaitseva, N., Heimann, M., Laurila, T., Lihavainen, H., Asmi, E., Arshinov, M., Shevchenko, V., Makshtas, A., Dubtsov, S., Mikhailov, E., Lapshina, E., Kirpotin, S., Kurbatova, Y., Ding, A., Guo, H., Park, S., Lavric, J. V., Reum, F., Panov, A., Prokushkin, A., and Kulmala, M.: Ground-based station network in Arctic and Subarctic Eurasia, Geogr. Environ. Sustain., 9, 75–88, https://doi.org/10.15356/2071-9388_02v09_06, 2016.
Altstädter, B., Platis, A., Wehner, B., Scholtz, A., Wildmann, N., Hermann, M., Käthner, R., Baars, H., Bange, J., and Lampert, A.: ALADINA – an unmanned research aircraft for observing vertical and horizontal distributions of ultrafine particles within the atmospheric boundary layer, Atmos. Meas. Tech., 8, 1627–1639, https://doi.org/10.5194/amt-8-1627-2015, 2015.
Altstädter, B., Platis, A., Jähn, M., Baars, H., Lückerath, J., Held, A., Lampert, A., Bange, J., Hermann, M., and Wehner, B.: Airborne observations of newly formed boundary layer aerosol particles under cloudy conditions, Atmos. Chem. Phys., 18, 8249–8264, https://doi.org/10.5194/acp-18-8249-2018, 2018.
AMAP: AMAP Assessment 2011: Mercury in the Arctic. Arctic Monitoring and Assessment Programme (AMAP), Oslo, Norway, xiv + 193 pp., 2011.
AMAP: AMAP Assessment 2015: Black carbon and ozone as Arctic climate forcers. Arctic Monitoring and Assessment Programme (AMAP), Oslo, Norway, vii + 116 pp., 2015.
Ancellet, G., Penner, I. E., Pelon, J., Mariage, V., Zabukovec, A., Raut, J. C., Kokhanenko, G., and Balin, Y. S.: Aerosol monitoring in Siberia using an 808 nm automatic compact lidar, Atmos. Meas. Tech., 12, 147–168, https://doi.org/10.5194/amt-12-147-2019, 2019.
Angot, H., Magand, O., Helmig, D., Ricaud, P., Quennehen, B., Gallée, H., Del Guasta, M., Sprovieri, F., Pirrone, N., Savarino, J., and Dommergue, A.: New insights into the atmospheric mercury cycling in central Antarctica and implications on a continental scale, Atmos. Chem. Phys., 16, 8249–8264, https://doi.org/10.5194/acp-16-8249-2016, 2016.
Antokhina, O. Y., Antokhin, P. N., Arshinova, V. G., Arshinov, M. Y., Belan, B. D., Belan, S. B., Davydov, D. K., Ivlev, G. A., Kozlov, A. V., Nedelec, P., Paris, J. D., Rasskazchikova, T. M., Savkin, D. E., Simonenkov, D. V., Sklyadneva, T. K., Tolmachev, G. N., and Fofonov, A. V.: Vertical Distributions of Gaseous and Aerosol Admixtures in Air over the Russian Arctic, Atmos. Ocean. Opt., 31, 300–310, https://doi.org/10.1134/S102485601803003x, 2018.
Armitage, J. M., MacLeod, M., and Cousins, I. T.: Comparative Assessment of the Global Fate and Transport Pathways of Long-Chain Perfluorocarboxylic Acids (PFCAs) and Perfluorocarboxylates (PFCs) Emitted from Direct Sources, Environ. Sci. Technol., 43, 5830–5836, https://doi.org/10.1021/es900753y, 2009.
Arnold, S. R., Law, K. S., Brock, C. A., Thomas, J. L., Starkweather, S. M., von Salzen, K., Stohl, A., Sharma, S., Lund, M. T., Flanner, M. G., Petäjä, T., Tanimoto, H., Gamble, J., Dibb, J. E., Melamed, M., Johnson, N., Fidel, M., Tynkkynen, V. P., Baklanov, A., Eckhardt, S., Monks, S. A., Browse, J., and Bozem, H.: Arctic air pollution: Challenges and opportunities for the next decade, Elementa-Sci. Anthrop., 4, 1–17, https://doi.org/10.12952/journal.elementa.000104, 2016.
Backman, J., Schmeisser, L., Virkkula, A., Ogren, J. A., Asmi, E., Starkweather, S., Sharma, S., Eleftheriadis, K., Uttal, T., Jefferson, A., Bergin, M., Makshtas, A., Tunved, P., and Fiebig, M.: On Aethalometer measurement uncertainties and an instrument correction factor for the Arctic, Atmos. Meas. Tech., 10, 5039–5062, https://doi.org/10.5194/amt-10-5039-2017, 2017.
Barbante, C., Veysseyre, A., Ferrari, C., Van de Velde, K., Morel, C., Capodaglio, G., Cescon, P., Scarponi, G., and Boutron, C.: Greenland snow evidence of large scale atmospheric contamination for platinum, palladium, and rhodium, Environ. Sci. Technol., 35, 835–839, https://doi.org/10.1021/es000146y, 2001.
Barrie, L. A.: Arctic Air-Pollution - an Overview of Current Knowledge, Atmos. Environ., 20, 643–663, https://doi.org/10.1016/0004-6981(86)90180-0, 1986.
Beamish, A. L., Coops, N., Chabrillat, S., and Heim, B.: A Phenological Approach to Spectral Differentiation of Low-Arctic Tundra Vegetation Communities, North Slope, Alaska, Remote Sens., 9, 1200, https://doi.org/10.3390/rs9111200, 2017.
Beamish, A. L., Coops, N. C., Hermosilla, T., Chabrillat, S., and Heim, B.: Monitoring pigment-driven vegetation changes in a low-Arctic tundra ecosystem using digital cameras, Ecosphere, 9, e02123, https://doi.org/10.1002/ecs2.2123, 2018.
Beamish, A., Raynolds, M. K., Epstein, H., Frost, G. V., Macander, M. J., Bergstedt, H., Bartsch, A., Kruse, S., Miles, V., Tanis, C. M., Heim, B., Fuchs, M., Chabrillat, S., Shevtsova, I., Verdonen, M., and Wagner, J.: Recent trends and remaining challenges for optical remote sensing of Arctic tundra vegetation: A review and outlook, Remote Sens. Environ., 246, 111872, https://doi.org/10.1016/j.rse.2020.111872, 2020.
Beniston, M., Farinotti, D., Stoffel, M., Andreassen, L. M., Coppola, E., Eckert, N., Fantini, A., Giacona, F., Hauck, C., Huss, M., Huwald, H., Lehning, M., López-Moreno, J. I., Magnusson, J., Marty, C., Morán-Tejéda, E., Morin, S., Naaim, M., Provenzale, A., Rabatel, A., Six, D., Stötter, J., Strasser, U., Terzago, S., and Vincent, C.: The European mountain cryosphere: a review of its current state, trends, and future challenges, The Cryosphere, 12, 759–794, https://doi.org/10.5194/tc-12-759-2018, 2018.
Bhatt, U. S., Walker, D. A., Raynolds, M. K., Comiso, J. C., Epstein, H. E., Jia, G. S., Gens, R., Pinzon, J. E., Tucker, C. J., Tweedie, C. E., and Webber, P. J.: Circumpolar Arctic Tundra Vegetation Change Is Linked to Sea Ice Decline, Earth Interact., 14, 1–20, https://doi.org/10.1175/2010ei315.1, 2010.
Bokhorst, S., Pedersen, S. H., Brucker, L., Anisimov, O., Bjerke, J. W., Brown, R. D., Ehrich, D., Essery, R. L. H., Heilig, A., Ingvander, S., Johansson, C., Johansson, M., Jónsdóttir, I. S., Inga, N., Luojus, K., Macelloni, G., Mariash, H., McLennan, D., Rosqvist, G. N., Sato, A., Savela, H., Schneebeli, M., Sokolov, A., Sokratov, S. A., Terzago, S., Vikhamar-Schuler, D., Williamson, S., Qiu, Y. B., and Callaghan, T. V.: Changing Arctic snow cover: A review of recent developments and assessment of future needs for observations, modelling, and impacts, Ambio, 45, 516–537, https://doi.org/10.1007/s13280-016-0770-0, 2016.
Bond, T., Doherty, S. J., Fahey, D., Forster, P., Berntsen, T., DeAngelo, B., Flanner, M., Ghan, S., Kärcher, B., Koch, D., Kinne, S., Kondo, Y., Quinn, P., Sarofim, M., Schultz, M., Schulz, M., Venkataraman, C., Zhang, H., Zhang, S., Bellouin, N., Guttikunda, S., Hopke, P., Jacobson, M., Kaiser, J., Klimont, Z., Lohmann, U., Schwarz, J., Shindell, D., Storelvmo, T., Warren, S., and Zender, C.: Bounding the role of black carbon in the climate system: A scientific assessment, J. Geophys. Res.-Atmos., 118, 5380–5552, https://doi.org/10.1002/jgrd.50171, 2013.
Bossi, R., Vorkamp, K., and Skov, H.: Concentrations of organochlorine pesticides, polybrominated diphenyl ethers and perfluorinated compounds in the atmosphere of North Greenland, Environ. Pollut., 217, 4–10, https://doi.org/10.1016/j.envpol.2015.12.026, 2016.
Boy, M., Thomson, E. S., Navarro, J. C. A., Arnalds, O., Batchvarova, E., Bäck, J., Berninger, F., Bilde, M., Brasseur, Z., Dagsson-Waldhauserova, P., Castarède, D., Dalirian, M., de Leeuw, G., Dragosics, M., Duplissy, E. M., Duplissy, J., Ekman, A. M. L., Fang, K. Y., Gallet, J. C., Glasius, M., Gryning, S. E., Grythe, H., Hansson, H. C., Hansson, M., Isaksson, E., Iversen, T., Jonsdottir, I., Kasurinen, V., Kirkevåg, A., Korhola, A., Krejci, R., Kristjansson, J. E., Lappalainen, H. K., Lauri, A., Leppäranta, M., Lihavainen, H., Makkonen, R., Massling, A., Meinander, O., Nilsson, E. D., Olafsson, H., Pettersson, J. B. C., Prisle, N. L., Riipinen, I., Roldin, P., Ruppel, M., Salter, M., Sand, M., Seland, O., Seppä, H., Skov, H., Soares, J., Stohl, A., Ström, J., Svensson, J., Swietlicki, E., Tabakova, K., Thorsteinsson, T., Virkkula, A., Weyhenmeyer, G. A., Wu, Y. S., Zieger, P., and Kulmala, M.: Interactions between the atmosphere, cryosphere, and ecosystems at northern high latitudes, Atmos. Chem. Phys., 19, 2015–2061, https://doi.org/10.5194/acp-19-2015-2019, 2019.
Bozzetti, C., Sosedova, Y., Xiao, M., Daellenbach, K. R., Ulevicius, V., Dudoitis, V., Mordas, G., Byčenkienė, S., Plauškaitė, K., Vlachou, A., Golly, B., Chazeau, B., Besombes, J. L., Baltensperger, U., Jaffrezo, J. L., Slowik, J. G., El Haddad, I., and Prévôt, A. S. H.: Argon offline-AMS source apportionment of organic aerosol over yearly cycles for an urban, rural, and marine site in northern Europe, Atmos. Chem. Phys., 17, 117–141, https://doi.org/10.5194/acp-17-117-2017, 2017.
Bratsch, S. N., Epstein, H. E., Buchhorn, M., and Walker, D. A.: Differentiating among Four Arctic Tundra Plant Communities at Ivotuk, Alaska Using Field Spectroscopy, Remote Sens., 8, 51, https://doi.org/10.3390/rs8010051, 2016.
Breider, T. J., Mickley, L. J., Jacob, D. J., Wang, Q. Q., Fisher, J. A., Chang, R. Y. W., and Alexander, B.: Annual distributions and sources of Arctic aerosol components, aerosol optical depth, and aerosol absorption, J. Geophys. Res.-Atmos., 119, 4107–4124, https://doi.org/10.1002/2013jd020996, 2014.
Breider, T. J., Mickley, L. J., Jacob, D. J., Ge, C., Wang, J., Sulprizio, M. P., Croft, B., Ridley, D. A., McConnell, J. R., Sharma, S., Husain, L., Dutkiewicz, V. A., Eleftheriadis, K., Skov, H., and Hopke, P. K.: Multidecadal trends in aerosol radiative forcing over the Arctic: Contribution of changes in anthropogenic aerosol to Arctic warming since 1980, J. Geophys. Res.-Atmos., 122, 3573–3594, https://doi.org/10.1002/2016jd025321, 2017.
Brooks, S. B., Saiz-Lopez, A., Skov, H., Lindberg, S. E., Plane, J. M. C., and Goodsite, M. E.: The mass balance of mercury in the springtime arctic environment, Geophys. Res. Lett., 33, L13812, https://doi.org/10.1029/2005gl025525, 2006.
Buchhorn, M., Walker, D. A., Heim, B., Raynolds, M. K., Epstein, H. E., and Schwieder, M.: Ground-Based Hyperspectral Characterization of Alaska Tundra Vegetation along Environmental Gradients, Remote Sens., 5, 3971–4005, https://doi.org/10.3390/rs5083971, 2013.
Buixadé Farré, A., Stephenson, S. R., Chen, L., Czub, M., Dai, Y., Demchev, D., Efimov, Y., Graczyk, P., Grythe, H., Keil, K., Kivekäs, N., Kumar, N., Liu, N., Matelenok, I., Myksvoll, M., O'Leary, D., Olsen, J., Pavithran, A. P. S., Petersen, E., Raspotnik, A., Ryzhov, I., Solski, J., Suo, L., Troein, C., Valeeva, V., van Rijckevorsel, J., and Wighting, J.: Commercial Arctic shipping through the Northeast Passage: routes, resources, governance, technology, and infrastructure, Polar Geogr., 37, 298–324, https://doi.org/10.1080/1088937X.2014.965769, 2014.
Cai, M. H., Xie, Z. Y., Möller, A., Yin, Z. G., Huang, P., Cai, M. G., Yang, H. Z., Sturm, R., He, J. F., and Ebinghaus, R.: Polyfluorinated compounds in the atmosphere along a cruise pathway from the Japan Sea to the Arctic Ocean, Chemosphere, 87, 989–997, https://doi.org/10.1016/j.chemosphere.2011.11.010, 2012.
Canonaco, F., Crippa, M., Slowik, J. G., Baltensperger, U., and Prévôt, A. S. H.: SoFi, an IGOR-based interface for the efficient use of the generalized multilinear engine (ME-2) for the source apportionment: ME-2 application to aerosol mass spectrometer data, Atmos. Meas. Tech., 6, 3649–3661, https://doi.org/10.5194/amt-6-3649-2013, 2013.
Carnerero, C., Pérez, N., Petäjä, T., Laurila, T. M., Ahonen, L. R., Kontkanen, J., Ahn, K.-H., Alastuey, A., and Querol, X.: Relating high ozone, ultrafine particles, and new particle formation episodes using cluster analysis, Atmos. Environ.: X, 4, 100051, https://doi.org/10.1016/j.aeaoa.2019.100051, 2019.
Collaud Coen, M., Andrews, E., Alastuey, A., Arsov, T. P., Backman, J., Brem, B. T., Bukowiecki, N., Couret, C., Eleftheriadis, K., Flentje, H., Fiebig, M., Gysel-Beer, M., Hand, J. L., Hoffer, A., Hooda, R., Hueglin, C., Joubert, W., Keywood, M., Kim, J. E., Kim, S.-W., Labuschagne, C., Lin, N.-H., Lin, Y., Lund Myhre, C., Luoma, K., Lyamani, H., Marinoni, A., Mayol-Bracero, O. L., Mihalopoulos, N., Pandolfi, M., Prats, N., Prenni, A. J., Putaud, J.-P., Ries, L., Reisen, F., Sellegri, K., Sharma, S., Sheridan, P., Sherman, J. P., Sun, J., Titos, G., Torres, E., Tuch, T., Weller, R., Wiedensohler, A., Zieger, P., and Laj, P.: Multidecadal trend analysis of aerosol radiative properties at a global scale, Atmos. Chem. Phys. Discuss., https://doi.org/10.5194/acp-2019-1174, in review, 2020.
Corbett, J., Lack, D., Winebrake, J., Harder, S., Silberman, J., and Gold, M.: Arctic shipping emissions inventories and future scenarios, Atmos. Chem. Phys., 10, 9689–9704, https://doi.org/10.5194/acp-10-9689-2010, 2010.
Daellenbach, K. R., Bozzetti, C., Křepelova, A. K., Canonaco, F., Wolf, R., Zotter, P., Fermo, P., Crippa, M., Slowik, J. G., Sosedova, Y., Zhang, Y., Huang, R. J., Poulain, L., Szidat, S., Baltensperger, U., El Haddad, I., and Prévôt, A. S. H.: Characterization and source apportionment of organic aerosol using offline aerosol mass spectrometry, Atmos. Meas. Tech., 9, 23–39, https://doi.org/10.5194/amt-9-23-2016, 2016.
Dall'Osto, M., Geels, C., Beddows, D. C. S., Boertmann, D., Lange, R., Nøjgaard, J. K., Harrison, R. M., Simo, R., Skov, H., and Massling, A.: Regions of open water and melting sea ice drive new particle formation in North East Greenland, Sci. Rep.-UK, 8, 6109, https://doi.org/10.1038/s41598-018-24426-8, 2018a.
Dall'Osto, M., Simo, R., Harrison, R. M., Beddows, D. C. S., Saiz-Lopez, A., Lange, R., Skov, H., Nøjgaard, J. K., Nielsen, I. E., and Massling, A.: Abiotic and biotic sources influencing spring new particle formation in North East Greenland, Atmos. Environ., 190, 126–134, https://doi.org/10.1016/j.atmosenv.2018.07.019, 2018b.
Di Pierro, M., Jaeglé, L., and Anderson, T. L.: Satellite observations of aerosol transport from East Asia to the Arctic: three case studies, Atmos. Chem. Phys., 11, 2225–2243, https://doi.org/10.5194/acp-11-2225-2011, 2011.
Dietz, A. J., Kuenzer, C., Gessner, U., and Dech, S.: Remote sensing of snow – a review of available methods, Int. J. Remote Sens., 33, 4094–4134, https://doi.org/10.1080/01431161.2011.640964, 2012.
Douglas, T. A., Loseto, L. L., Macdonald, R. W., Outridge, P., Dommergue, A., Poulain, A., Amyot, M., Barkay, T., Berg, T., Chételat, J., Constant, P., Evans, M., Ferrari, C., Gantner, N., Johnson, M. S., Kirk, J., Kroer, N., Larose, C., Lean, D., Nielsen, T. G., Poissant, L., Rognerud, S., Skov, H., Sørensen, S., Wang, F. Y., Wilson, S., and Zdanowicz, C. M.: The fate of mercury in Arctic terrestrial and aquatic ecosystems, a review, Environ. Chem., 9, 321–355, https://doi.org/10.1071/En11140, 2012.
Dozier, J., Green, R. O., Nolin, A. W., and Painter, T. H.: Interpretation of snow properties from imaging spectrometry, Remote Sens. Environ., 113, S25–S37, https://doi.org/10.1016/j.rse.2007.07.029, 2009.
Durnford, D., Dastoor, A., Figueras-Nieto, D., and Ryjkov, A.: Long range transport of mercury to the Arctic and across Canada, Atmos. Chem. Phys., 10, 6063–6086, https://doi.org/10.5194/acp-10-6063-2010, 2010.
Eleftheriadis, K., Vratolis, S., and Nyeki, S.: Aerosol black carbon in the European Arctic: Measurements at Zeppelin station, Ny-Alesund, Svalbard from 1998–2007, Geophys. Res. Lett., 36, L02809, https://doi.org/10.1029/2008gl035741, 2009.
Evangeliou, N., Thompson, R. L., Eckhardt, S., and Stohl, A.: Top-down estimates of black carbon emissions at high latitudes using an atmospheric transport model and a Bayesian inversion framework, Atmos. Chem. Phys., 18, 15307–15327, https://doi.org/10.5194/acp-18-15307-2018, 2018.
Ežerinskis, Ž., Spolaor, A., Kirchgeorg, T., Cozzi, G., Vallelonga, P., Kjær, H. A., Šapolaitė, J., Barbante, C., and Druteikienė, R.: Determination of I-129 in Arctic snow by a novel analytical approach using IC-ICP-SFMS, J. Anal. Atom. Spectrom., 29, 1827–1834, https://doi.org/10.1039/c4ja00179f, 2014.
Fast, J. D., Gustafson, W. I., Easter, R. C., Zaveri, R. A., Barnard, J. C., Chapman, E. G., Grell, G. A., and Peckham, S. E.: Evolution of ozone, particulates, and aerosol direct radiative forcing in the vicinity of Houston using a fully coupled meteorology-chemistry-aerosol model, J. Geophys. Res.-Atmos., 111, D21305, https://doi.org/10.1029/2005jd006721, 2006.
Fisher, J. A., Jacob, D. J., Soerensen, A. L., Amos, H. M., Steffen, A., and Sunderland, E. M.: Riverine source of Arctic Ocean mercury inferred from atmospheric observations, Nat. Geosci., 5, 499–504, https://doi.org/10.1038/ngeo1478, 2012.
Freud, E., Krejci, R., Tunved, P., Leaitch, R., Nguyen, Q. T., Massling, A., Skov, H., and Barrie, L.: Pan-Arctic aerosol number size distributions: seasonality and transport patterns, Atmos. Chem. Phys., 17, 8101–8128, https://doi.org/10.5194/acp-17-8101-2017, 2017.
Giamarelou, M., Eleftheriadis, K., Nyeki, S., Tunved, P., Torseth, K., and Biskos, G.: Indirect evidence of the composition of nucleation mode atmospheric particles in the high Arctic, J. Geophys. Res.-Atmos., 121, 965–975, https://doi.org/10.1002/2015jd023646, 2016.
Giglio, L., Descloitres, J., Justice, C. O., and Kaufman, Y. J.: An enhanced contextual fire detection algorithm for MODIS, Remote Sens. Environ., 87, 273–282, https://doi.org/10.1016/S0034-4257(03)00184-6, 2003.
Grell, G. A., Peckham, S. E., Schmitz, R., McKeen, S. A., Frost, G., Skamarock, W. C., and Eder, B.: Fully coupled ”online” chemistry within the WRF model, Atmos. Environ., 39, 6957–6975, https://doi.org/10.1016/j.atmosenv.2005.04.027, 2005.
Guanter, L., Kaufmann, H., Segl, K., Foerster, S., Rogass, C., Chabrillat, S., Kuester, T., Hollstein, A., Rossner, G., Chlebek, C., Straif, C., Fischer, S., Schrader, S., Storch, T., Heiden, U., Mueller, A., Bachmann, M., Mühle, H., Müller, R., Habermeyer, M., Ohndorf, A., Hill, J., Buddenbaum, H., Hostert, P., van der Linden, S., Leitão, P. J., Rabe, A., Doerffer, R., Krasemann, H., Xi, H. Y., Mauser, W., Hank, T., Locherer, M., Rast, M., Staenz, K., and Sang, B.: The EnMAP Spaceborne Imaging Spectroscopy Mission for Earth Observation, Remote Sens., 7, 8830–8857, https://doi.org/10.3390/rs70708830, 2015.
Gvakharia, A., Kort, E. A., Brandt, A., Peischl, J., Ryerson, T. B., Schwarz, J. P., Smith, M. L., and Sweeney, C.: Methane, Black Carbon, and Ethane Emissions from Natural Gas Flares in the Bakken Shale, North Dakota, Environ. Sci. Technol., 51, 5317–5325, https://doi.org/10.1021/acs.est.6b05183, 2017.
Hall, D. K. and Riggs, G. A.: MODIS/Terra Snow Cover Daily L3 Global 500m SIN Grid, Version 6, Boulder, Colorado USA, NASA National Snow and Ice Data Center Distributed Active Archive Center, https://doi.org/10.5067/MODIS/MOD10A1.006 (last access: 19 December 2019), 2016.
Hari, P., Aalto, P., Hämeri, K., Kulmala, M., Lahti, T., Luoma, S., Palva, L., Pohja, T., Pulliainen, E., Siivola, E., and Vesala, T.: Air pollution in eastern Lapland: challenge for an environmental measurement station, Silva Fenn., 28, 29–39, https://doi.org/10.14214/sf.a9160, 1994.
Hari, P., Petäjä, T., Back, J., Kerminen, V. M., Lappalainen, H. K., Vihma, T., Laurila, T., Viisanen, Y., Vesala, T., and Kulmala, M.: Conceptual design of a measurement network of the global change, Atmos. Chem. Phys., 16, 1017–1028, https://doi.org/10.5194/acp-16-1017-2016, 2016.
Helm, V., Humbert, A., and Miller, H.: Elevation and elevation change of Greenland and Antarctica derived from CryoSat-2, The Cryosphere, 8, 1539–1559, https://doi.org/10.5194/tc-8-1539-2014, 2014.
Herbert, B. M. J., Halsall, C. J., Villa, S., Jones, K. C., and Kallenborn, R.: Rapid changes in PCB and OC pesticide concentrations in Arctic snow, Environ. Sci. Technol., 39, 2998–3005, https://doi.org/10.1021/es040076l, 2005.
Hermanson, M. H., Isaksson, E., Forsström, S., Texeira, C., Muir, D. C. G., Pohjola, V. A., and van de Wal, R. S. V.: Deposition history of brominated flame retardant compounds in an ice core from Holtedahlfonna, Svalbard, Norway, Environ. Sci. Technol., 44, 7405–7410, https://doi.org/10.1021/es1016608, 2010.
Höglund-Isaksson, L.: Global anthropogenic methane emissions 2005–2030: technical mitigation potentials and costs, Atmos. Chem. Phys., 12, 9079–9096, https://doi.org/10.5194/acp-12-9079-2012, 2012.
Huang, K., Fu, J. S., Prikhodko, V. Y., Storey, J. M., Romanov, A., Hodson, E. L., Cresko, J., Morozova, I., Ignatieva, Y., and Cabaniss, J.: Russian anthropogenic black carbon: Emission reconstruction and Arctic black carbon simulation, J. Geophys. Res.-Atmos., 120, 11306–11333, https://doi.org/10.1002/2015jd023358, 2015.
Hung, H., Kallenborn, R., Breivik, K., Su, Y. S., Brorström-Lundén, E., Olafsdottir, K., Thorlacius, J. M., Leppänen, S., Bossi, R., Skov, H., Manø, S., Patton, G. W., Stern, G., Sverko, E., and Fellin, P.: Atmospheric monitoring of organic pollutants in the Arctic under the Arctic Monitoring and Assessment Programme (AMAP): 1993–2006, Sci.Total Environ., 408, 2854–2873, https://doi.org/10.1016/j.scitotenv.2009.10.044, 2010.
Hung, H., Katsoyiannis, A. A., Brorström-Lundén, E., Olafsdottir, K., Aas, W., Breivik, K., Bohlin-Nizzetto, P., Sigurdsson, A., Hakola, H., Bossi, R., Skov, H., Sverko, E., Barresi, E., Fellin, P., and Wilson, S.: Temporal trends of Persistent Organic Pollutants (POPs) in Arctic air: 20 years of monitoring under the Arctic Monitoring and Assessment Programme (AMAP), Environ. Pollut., 217, 52–61, https://doi.org/10.1016/j.envpol.2016.01.079, 2016.
iCUPE: Integrative and Comprehensive Understanding on Polar Environments, available at: https://www.atm.helsinki.fi/icupe/index.php/datasets, last access: 29 June 2020.
IDA Science and Technology Policy Institute and Sustaining Arctic Observing Networks: International Arctic Observations Assessment Framework, IDA Science and Technology Policy Institute, Washington, DC, USA, and Sustaining Arctic Observing Networks, Oslo, Norway, 73 pp., 2017.
Illingworth, A. J., Barker, H. W., Beljaars, A., Ceccaldi, M., Chepfer, H., Clerbaux, N., Cole, J., Delanoë, J., Domenech, C., Donovan, D. P., Fukuda, S., Hirakata, M., Hogan, R. J., Huenerbein, A., Kollias, P., Kubota, T., Nakajima, T., Nakajima, T. Y., Nishizawa, T., Ohno, Y., Okamoto, H., Oki, R., Sato, K., Satoh, M., Shephard, M. W., Velázquez-Blázquez, A., Wandinger, U., Wehr, T., and van Zadelhoff, G.-J.: The EarthCARE Satellite the next step forward in global measurements of clouds, aerosols, precipitation, and radiation, B. Am. Meteorol. Soc., 96, 1311–1332, https://doi.org/10.1175/Bams-D-12-00227.1, 2015.
IPCC: Climate Change 2014: Synthesis Report, Contribution of Working Groups I, II and III to the Fifth Assessment Report of the Intergovernmental Panel on Climate Change, Core Writing Team, edited by: Pachauri, R. K. and Meyer, L. A., 151 pp., 2014.
Janssens-Maenhout, G., Crippa, M., Guizzardi, D., Muntean, M., Schaaf, E., Dentener, F., Bergamaschi, P., Pagliari, V., Olivier, J. G. J., Peters, J. A. H. W., van Aardenne, J. A., Monni, S., Doering, U., Petrescu, A. M. R., Solazzo, E., and Oreggioni, G. D.: EDGAR v4.3.2 Global Atlas of the three major greenhouse gas emissions for the period 1970–2012, Earth Syst. Sci. Data, 11, 959–1002, https://doi.org/10.5194/essd-11-959-2019, 2019.
Jiao, C., Flanner, M. G., Balkanski, Y., Bauer, S. E., Bellouin, N., Berntsen, T. K., Bian, H., Carslaw, K. S., Chin, M., De Luca, N., Diehl, T., Ghan, S. J., Iversen, T., Kirkevåg, A., Koch, D., Liu, X., Mann, G. W., Penner, J. E., Pitari, G., Schulz, M., Seland, Ø., Skeie, R. B., Steenrod, S. D., Stier, P., Takemura, T., Tsigaridis, K., van Noije, T., Yun, Y., and Zhang, K.: An AeroCom assessment of black carbon in Arctic snow and sea ice, Atmos. Chem. Phys., 14, 2399–2417, https://doi.org/10.5194/acp-14-2399-2014, 2014.
Jiskra, M., Sonke, J. E., Obrist, D., Bieser, J., Ebinghaus, R., Myhre, C. L., Pfaffhuber, K. A., Wängberg, I., Kyllönen, K., Worthy, D., Martin, L. G., Labuschagne, C., Mkololo, T., Ramonet, M., Magand, O., and Dommergue, A.: A vegetation control on seasonal variations in global atmospheric mercury concentrations, Nat. Geosci., 11, 244–250, https://doi.org/10.1038/s41561-018-0078-8, 2018.
Junninen, H., Lauri, A., Keronen, P., Aalto, P., Hiltunen, V., Hari, P., and Kulmala, M.: Smart-SMEAR: on-line data exploration and visualization tool for SMEAR stations, Boreal Environ. Res., 14, 447–457, 2009.
Kirpes, R. M., Bondy, A. L., Bonanno, D., Moffet, R. C., Wang, B. B., Laskin, A., Ault, A. P., and Pratt, K. A.: Secondary sulfate is internally mixed with sea spray aerosol and organic aerosol in the winter Arctic, Atmos. Chem. Phys., 18, 3937–3949, https://doi.org/10.5194/acp-18-3937-2018, 2018.
Klimont, Z., Kupiainen, K., Heyes, C., Purohit, P., Cofala, J., Rafaj, P., Borken-Kleefeld, J., and Schöpp, W.: Global anthropogenic emissions of particulate matter including black carbon, Atmos. Chem. Phys., 17, 8681–8723, https://doi.org/10.5194/acp-17-8681-2017, 2017.
Klonecki, A., Hess, P., Emmons, L., Smith, L., Orlando, J., and Blake, D.: Seasonal changes in the transport of pollutants into the Arctic troposphere-model study, J. Geophys. Res.-Atmos., 108, 8367, https://doi.org/10.1029/2002jd002199, 2003.
Kokelj, S. V., Lantz, T. C., Tunnicliffe, J., Segal, R., and Lacelle, D.: Climate-driven thaw of permafrost preserved glacial landscapes, northwestern Canada, Geology, 45, 371–374, https://doi.org/10.1130/G38626.1, 2017.
Kontkanen, J., Paasonen, P., Aalto, J., Bäck, J., Rantala, P., Petäjä, T., and Kulmala, M.: Simple proxies for estimating the concentrations of monoterpenes and their oxidation products at a boreal forest site, Atmos. Chem. Phys., 16, 13291–13307, https://doi.org/10.5194/acp-16-13291-2016, 2016.
Kramshøj, M., Albers, C. N., Holst, T., Holzinger, R., Elberling, B., and Rinnan, R.: Biogenic volatile release from permafrost thaw is determined by the soil microbial sink, Nat. Commun., 9, 3412, https://doi.org/10.1038/s41467-018-05824-y, 2018.
Kulmala, M.: Build a global Earth observatory, Nature, 553, 21–22, https://doi.org/10.1038/d41586-017-08967-y, 2018.
Kulmala, M., Dal Maso, M., Mäkelä, J. M., Pirjola, L., Väkevä, M., Aalto, P., Miikkulainen, P., Hämeri, K., and O'Dowd, C. D.: On the formation, growth and composition of nucleation mode particles, Tellus B, 53, 479–490, https://doi.org/10.1034/j.1600-0889.2001.530411.x, 2001.
Kulmala, M., Asmi, A., Lappalainen, H. K., Baltensperger, U., Brenguier, J. L., Facchini, M. C., Hansson, H. C., Hov, Ø., O'Dowd, C. D., Pöschl, U., Wiedensohler, A., Boers, R., Boucher, O., de Leeuw, G., van der Gon, H. A. C. D., Feichter, J., Krejci, R., Laj, P., Lihavainen, H., Lohmann, U., McFiggans, G., Mentel, T., Pilinis, C., Riipinen, I., Schulz, M., Stohl, A., Swietlicki, E., Vignati, E., Alves, C., Amann, M., Ammann, M., Arabas, S., Artaxo, P., Baars, H., Beddows, D. C. S., Bergström, R., Beukes, J. P., Bilde, M., Burkhart, J. F., Canonaco, F., Clegg, S. L., Coe, H., Crumeyrolle, S., D'Anna, B., Decesari, S., Gilardoni, S., Fischer, M., Fjaeraa, A. M., Fountoukis, C., George, C., Gomes, L., Halloran, P., Hamburger, T., Harrison, R. M., Herrmann, H., Hoffmann, T., Hoose, C., Hu, M., Hyvärinen, A., Hõrrak, U., Iinuma, Y., Iversen, T., Josipovic, M., Kanakidou, M., Kiendler-Scharr, A., Kirkevåg, A., Kiss, G., Klimont, Z., Kolmonen, P., Komppula, M., Kristjánsson, J. E., Laakso, L., Laaksonen, A., Labonnote, L., Lanz, V. A., Lehtinen, K. E. J., Rizzo, L. V., Makkonen, R., Manninen, H. E., McMeeking, G., Merikanto, J., Minikin, A., Mirme, S., Morgan, W. T., Nemitz, E., O'Donnell, D., Panwar, T. S., Pawlowska, H., Petzold, A., Pienaar, J. J., Pio, C., Plass-Duelmer, C., Prévôt, A. S. H., Pryor, S., Reddington, C. L., Roberts, G., Rosenfeld, D., Schwarz, J., Seland, Ø., Sellegri, K., Shen, X. J., Shiraiwa, M., Siebert, H., Sierau, B., Simpson, D., Sun, J. Y., Topping, D., Tunved, P., Vaattovaara, P., Vakkari, V., Veefkind, J. P., Visschedijk, A., Vuollekoski, H., Vuolo, R., Wehner, B., Wildt, J., Woodward, S., Worsnop, D. R., van Zadelhoff, G. J., Zardini, A. A., Zhang, K., van Zyl, P. G., Kerminen, V. M., Carslaw, K. S., and Pandis, S. N.: General overview: European Integrated project on Aerosol Cloud Climate and Air Quality interactions (EUCAARI) - integrating aerosol research from nano to global scales, Atmos. Chem. Phys., 11, 13061–13143, https://doi.org/10.5194/acp-11-13061-2011, 2011a.
Kulmala, M., Arola, A., Nieminen, T., Riuttanen, L., Sogacheva, L., de Leeuw, G., Kerminen, V.-M., and Lehtinen, K. E. J.: The first estimates of global nucleation mode aerosol concentrations based on satellite measurements, Atmos. Chem. Phys., 11, 10791–10801, https://doi.org/10.5194/acp-11-10791-2011, 2011b.
Kulmala, M., Lappalainen, H. K., Petäjä, T., Kurten, T., Kerminen, V. M., Viisanen, Y., Hari, P., Sorvari, S., Bäck, J., Bondur, V., Kasimov, N., Kotlyakov, V., Matvienko, G., Baklanov, A., Guo, H. D., Ding, A., Hansson, H. C., and Zilitinkevich, S.: Introduction: The Pan-Eurasian Experiment (PEEX) – multidisciplinary, multiscale and multicomponent research and capacity-building initiative, Atmos. Chem. Phys., 15, 13085–13096, https://doi.org/10.5194/acp-15-13085-2015, 2015.
Lampert, A., Altstädter, B., Bärfuss, K., Bretschneider, L., Sandgaard, J., Michaelis, J., Lobitz, L., Asmussen, M., Damm, E., Käthner, R., Krüger, T., Lüpkes, C., Nowak, S., Peuker, A., Rausch, T., Reiser, F., Scholtz, A., Sotomayor Zakharov, D,; Gaus, D., Bansmer, S., Wehner, B., and Pätzold, F.: Unmanned aerial systems for investigating the Polar atmospheric boundary layer – technical challenges and examples of applications, Atmosphere, 11, 1–25, https://doi.org/10.3390/atmos11040416, 2020.
Lappalainen, H. K., Kerminen, V. M., Petäjä, T., Kurten, T., Baklanov, A., Shvidenko, A., Bäck, J., Vihma, T., Alekseychik, P., Andreae, M. O., Arnold, S. R., Arshinov, M., Asmi, E., Belan, B., Bobylev, L., Chalov, S., Cheng, Y. F., Chubarova, N., de Leeuw, G., Ding, A. J., Dobrolyubov, S., Dubtsov, S., Dyukarev, E., Elansky, N., Eleftheriadis, K., Esau, I., Filatov, N., Flint, M., Fu, C. B., Glezer, O., Gliko, A., Heimann, M., Holtslag, A. A. M., Hõrrak, U., Janhunen, J., Juhola, S., Järvi, L., Järvinen, H., Kanukhina, A., Konstantinov, P., Kotlyakov, V., Kieloaho, A. J., Komarov, A. S., Kujansuu, J., Kukkonen, I., Duplissy, E. M., Laaksonen, A., Laurila, T., Lihavainen, H., Lisitzin, A., Mahura, A., Makshtas, A., Mareev, E., Mazon, S., Matishov, D., Melnikov, V., Mikhailov, E., Moisseev, D., Nigmatulin, R., Noe, S. M., Ojala, A., Pihlatie, M., Popovicheva, O., Pumpanen, J., Regerand, T., Repina, I., Shcherbinin, A., Shevchenko, V., Sipilä, M., Skorokhod, A., Spracklen, D. V., Su, H., Subetto, D. A., Sun, J. Y., Terzhevik, A. Y., Timofeyev, Y., Troitskaya, Y., Tynkkynen, V. P., Kharuk, V. I., Zaytseva, N., Zhang, J. H., Viisanen, Y., Vesala, T., Hari, P., Hansson, H. C., Matvienko, G. G., Kasimov, N. S., Guo, H. D., Bondur, V., Zilitinkevich, S., and Kulmala, M.: Pan-Eurasian Experiment (PEEX): towards a holistic understanding of the feedbacks and interactions in the land-atmosphere-ocean-society continuum in the northern Eurasian region, Atmos. Chem. Phys., 16, 14421–14461, https://doi.org/10.5194/acp-16-14421-2016, 2016.
Lappalainen, H. K., Altimir, N., Kerminen, V., Petäjä, T., Makkonen, R., Alekseychik, P., Zaitseva, N., Bashmakova, I., Kujansuu, J., Lauri, A., Haapanala, P., Mazon, S. B., Borisova, A., Konstantinov, P., Chalov, S., Laurila, T., Asmi, E., Lihavainen, H., Bäck, J., Arshinov, M., Mahura, A., Arnold, S., Vihma, T., Uotila, P., de Leeuw, G., Kukkonen, I., Malkhazova, S., Tynkkynen, V., Fedorova, I., Hansson, H. C., Dobrolyubov, S., Melnikov, V., Matvienko, G., Baklanov, A., Viisanen, Y., Kasimov, N., Guo, H., Bondur, V., Zilitinkevich, S., and Kulmala, M.: Pan-eurasian experiment (peex) program: An overview of the first 5 years in operation and future prospects, Geogr. Environ. Sustain., 11, 6–19, https://doi.org/10.24057/2071-9388-2018-11-1-6-19, 2018.
Law, K. S. and Stohl, A.: Arctic air pollution: Origins and impacts, Science, 315, 1537–1540, https://doi.org/10.1126/science.1137695, 2007.
Law, K. S., Stohl, A., Quinn, P. K., Brock, C. A., Burkhart, J. F., Paris, J. D., Ancellet, G., Singh, H. B., Roiger, A., Schlager, H., Dibb, J., Jacob, D. J., Arnold, S. R., Pelon, J., and Thomas, J. L.: Arctic Air Pollution: New Insights from POLARCAT-IPY, B. Am. Meteorol. Soc., 95, 1873-+, https://doi.org/10.1175/Bams-D-13-00017.1, 2014.
Leino, K., Lampilahti, J., Poutanen, P., Väänänen, R., Manninen, A., Mazon, S. B., Dada, L., Franck, A., Wimmer, D., Aalto, P. P., Ahonen, L. R., Enroth, J., Kangasluoma, J., Keronen, P., Korhonen, F., Laakso, H., Matilainen, T., Siivola, E., Manninen, H. E., Lehtipalo, K., Kerminen, V. M., Petäjä, T., and Kulmala, M.: Vertical profiles of sub-3nm particles over the boreal forest, Atmos. Chem. Phys., 19, 4127–4138, https://doi.org/10.5194/acp-19-4127-2019, 2019.
Li, H. R. and Moisseev, D.: Melting layer attenuation at Ka- and W-Bands as derived from multifrequency radar doppler spectra observations, J. Geophys. Res.-Atmos., 124, 9520–9533, https://doi.org/10.1029/2019jd030316, 2019.
Li, H. R., Moisseev, D., and von Lerber, A.: How does riming affect dual-polarization radar observations and snowflake shape?, J. Geophys. Res.-Atmos., 123, 6070–6081, https://doi.org/10.1029/2017jd028186, 2018.
Li, J., Xie, Z. Y., Mi, W. Y., Lai, S. C., Tian, C. G., Emeis, K. C., and Ebinghaus, R.: Organophosphate Esters in Air, Snow, and Seawater in the North Atlantic and the Arctic, Environ. Sci. Technol., 51, 6887–6896, https://doi.org/10.1021/acs.est.7b01289, 2017.
Lim, A. G., Sonke, J. E., Krickov, I. V., Manasypov, R. M., Loiko, S. V., and Pokrovsky, O. S.: Enhanced particulate Hg export at the permafrost boundary, western Siberia, Environ. Pollut., 254, 113083, https://doi.org/10.1016/j.envpol.2019.113083, 2019.
Liu, N. F., Budkewitsch, P., and Treitz, P.: Examining spectral reflectance features related to Arctic percent vegetation cover: Implications for hyperspectral remote sensing of Arctic tundra, Remote Sens. Environ., 192, 58–72, https://doi.org/10.1016/j.rse.2017.02.002, 2017.
Loizzo, R., Guarini, R., Longo, F., Scopa, T., Formaro, R., Facchinetti, C., and Varacalli, G.: Prisma: The Italian Hyperspectral Mission, Igarss 2018 – 2018 Ieee International Geoscience and Remote Sensing Symposium, 175–178, https://doi.org/10.1109/IGARSS.2018.8518512, 2018.
Ma, J. M., Hung, H. L., Tian, C., and Kallenborn, R.: Revolatilization of persistent organic pollutants in the Arctic induced by climate change, Nat. Clim. Change, 1, 255–260, https://doi.org/10.1038/Nclimate1167, 2011.
Main-Knorn, M., Pflug, B., Louis, J., Debaecker, V., Muller-Wilm, U., and Gascon, F.: Sen2Cor for Sentinel-2, Proc. SPIE 10427, Image and Signal Processing for Remote Sensing XXIII, 1042704 (4 October 2017), https://doi.org/10.1117/12.2278218, 2017.
Marelle, L., Raut, J. C., Law, K. S., Berg, L. K., Fast, J. D., Easter, R. C., Shrivastava, M., and Thomas, J. L.: Improvements to the WRF-Chem 3.5.1 model for quasi-hemispheric simulations of aerosols and ozone in the Arctic, Geosci. Model Dev., 10, 3661–3677, https://doi.org/10.5194/gmd-10-3661-2017, 2017.
Marelle, L., Raut, J. C., Law, K. S., and Duclaux, O.: Current and future Arctic aerosols and ozone from remote emissions and emerging local sources-modeled source contributions and radiative effects, J. Geophys. Res.-Atmos., 123, 12942–12963, https://doi.org/10.1029/2018jd028863, 2018.
Mason, S. L., Chiu, C. J., Hogan, R. J., Moisseev, D., and Kneifel, S.: Retrievals of riming and snow density from vertically pointing doppler radars, J. Geophys. Res.-Atmos., 123, 13807–13834, https://doi.org/10.1029/2018jd028603, 2018.
Mason, S. L., Hogan, R. J., Westbrook, C. D., Kneifel, S., Moisseev, D., and von Terzi, L.: The importance of particle size distribution and internal structure for triple-frequency radar retrievals of the morphology of snow, Atmos. Meas. Tech., 12, 4993–5018, https://doi.org/10.5194/amt-12-4993-2019, 2019.
McConnell, J. R. and Edwards, R.: Coal burning leaves toxic heavy metal legacy in the Arctic, P. Natl. Acad. Sci. USA, 105, 12140–12144, https://doi.org/10.1073/pnas.0803564105, 2008.
Meier, W. N., Hovelsrud, G. K., van Oort, B. E. H., Key, J. R., Kovacs, K. M., Michel, C., Haas, C., Granskog, M. A., Gerland, S., Perovich, D. K., Makshtas, A., and Reist, J. D.: Arctic sea ice in transformation: A review of recent observed changes and impacts on biology and human activity, Rev. Geophys., 52, 185–217, https://doi.org/10.1002/2013rg000431, 2014.
Metsämäki, S., Pulliainen, J., Salminen, M., Luojus, K., Wiesmann, A., Solberg, R., Böttcher, K., Hiltunen, M., and Ripper, E.: Introduction to GlobSnow Snow Extent products with considerations for accuracy assessment, Remote Sens. Environ., 156, 96–108, https://doi.org/10.1016/j.rse.2014.09.018, 2015.
Mikkonen, S., Romakkaniemi, S., Smith, J. N., Korhonen, H., Petäjä, T., Plass-Duelmer, C., Boy, M., McMurry, P. H., Lehtinen, K. E. J., Joutsensaari, J., Hamed, A., Mauldin, R. L., Birmili, W., Spindler, G., Arnold, F., Kulmala, M., and Laaksonen, A.: A statistical proxy for sulphuric acid concentration, Atmos. Chem. Phys., 11, 11319–11334, https://doi.org/10.5194/acp-11-11319-2011, 2011.
Moisseev, D., von Lerber, A., and Tiira, J.: Quantifying the effect of riming on snowfall using ground-based observations, J. Geophys. Res.-Atmos., 122, 4019–4037, https://doi.org/10.1002/2016jd026272, 2017.
Moschos, V., Kumar, N. K., Daellenbach, K. R., Baltensperger, U., Prévôt, A. S. H., and El Haddad, I.: Source apportionment of brown carbon absorption by coupling ultraviolet-visible spectroscopy with aerosol mass spectrometry, Environ. Sci. Tech. Lett., 5, 302–308, https://doi.org/10.1021/acs.estlett.8b00118, 2018.
Mungall, E. L., Abbatt, J. P. D., Wentzell, J. J. B., Lee, A. K. Y., Thomas, J. L., Blais, M., Gosselin, M., Miller, L. A., Papakyriakou, T., Willis, M. D., and Liggio, J.: Microlayer source of oxygenated volatile organic compounds in the summertime marine Arctic boundary layer, P. Natl. Acad. Sci. USA, 114, 6203–6208, https://doi.org/10.1073/pnas.1620571114, 2017.
Myhre, G., Shindell, D., Bréon, F.-M., Collins, W., Fuglestvedt, J., Huang, J., Koch, D., Lamarque, J.-F., Lee, D., Mendoza, B., Nakajima, T., Robock, A., Stephens, G., Takemura, T., and Zhang, H.: Anthropogenic and Natural Radiative Forcing, in: Climate Change 2013: The Physical Science Basis. Contribution of Working Group I to the Fifth Assessment Report of the Intergovernmental Panel on Climate Change, edited by: Stocker, T. F., Qin, D., Plattner, G.-K., Tignor, M., Allen, S. K., Boschung, J., Nauels, A., Xia, Y., Bex, V., and Midgley, P. M., Cambridge University Press, Cambridge, United Kingdom and New York, NY, USA, 2013.
Neckel, N., Zeising, O., Steinhage, D., Helm, V., and Humbert, A.: Seasonal Observations at 79∘ N Glacier (Greenland) From Remote Sensing and in situ Measurements, Front. Earth Sci., 8, 142, https://doi.org/10.3389/feart.2020.00142, 2020.
Nguyen, H. T., Kim, K. H., Shon, Z. H., and Hong, S.: A Review of Atmospheric Mercury in the Polar Environment, Crit. Rev. Env. Sci. Tec., 39, 552–584, https://doi.org/10.1080/10643380701764308, 2009.
Nguyen, Q. T., Glasius, M., Sørensen, L. L., Jensen, B., Skov, H., Birmili, W., Wiedensohler, A., Kristensson, A., Nøjgaard, J. K., and Massling, A.: Seasonal variation of atmospheric particle number concentrations, new particle formation and atmospheric oxidation capacity at the high Arctic site Villum Research Station, Station Nord, Atmos. Chem. Phys., 16, 11319–11336, https://doi.org/10.5194/acp-16-11319-2016, 2016.
Nikandrova, A., Tabakova, K., Manninen, A., Väänänen, R., Petäjä, T., Kulmala, M., Kerminen, V. M., and O'Connor, E.: Combining airborne in situ and ground-based lidar measurements for attribution of aerosol layers, Atmos. Chem. Phys., 18, 10575–10591, https://doi.org/10.5194/acp-18-10575-2018, 2018.
Obrist, D., Agnan, Y., Jiskra, M., Olson, C. L., Colegrove, D. P., Hueber, J., Moore, C. W., Sonke, J. E., and Helmig, D.: Tundra uptake of atmospheric elemental mercury drives Arctic mercury pollution, Nature, 547, 201–, https://doi.org/10.1038/nature22997, 2017.
Onishi, T., Law, K. S., Paris, J.-D., Ancellet, G., Raut, J.-C., Nédèlec, P., Panchenko, M., Chernov, D., Arshinov M., and Belan, B.: Towards improved quantification of black carbon emissions from oil and gas extraction over Russia, in preparation, 2020.
Paasonen, P., Asmi, A., Petäjä, T., Kajos, M. K., Äijälä, M., Junninen, H., Holst, T., Abbatt, J. P. D., Arneth, A., Birmili, W., van der Gon, H. D., Hamed, A., Hoffer, A., Laakso, L., Laaksonen, A., Leaitch, W. R., Plass-Dülmer, C., Pryor, S. C., Räisänen, P., Swietlicki, E., Wiedensohler, A., Worsnop, D. R., Kerminen, V. M., and Kulmala, M.: Warming-induced increase in aerosol number concentration likely to moderate climate change, Nat. Geosci., 6, 438–442, https://doi.org/10.1038/Ngeo1800, 2013.
Paasonen, P., Kupiainen, K., Klimont, Z., Visschedijk, A., van der Gon, H. A. C. D., and Amann, M.: Continental anthropogenic primary particle number emissions, Atmos. Chem. Phys., 16, 6823–6840, https://doi.org/10.5194/acp-16-6823-2016, 2016.
Painter, T. H., Dozier, J., Roberts, D. A., Davis, R. E., and Green, R. O.: Retrieval of subpixel snow-covered area and grain size from imaging spectrometer data, Remote Sens. Environ., 85, 64–77, https://doi.org/10.1016/S0034-4257(02)00187-6, 2003.
Painter, T. H., Rittger, K., McKenzie, C., Slaughter, P., Davis, R. E., and Dozier, J.: Retrieval of subpixel snow covered area, grain size, and albedo from MODIS, Remote Sens. Environ., 113, 868–879, https://doi.org/10.1016/j.rse.2009.01.001, 2009.
Pankratov, F.: Dynamics of Atmospheric Mercury in the Russian Arctic, Ph. D. thesis, PhD thesis, Moscow State University, Moscow, https://doi.org/10.13140/RG.2.1.4255.1767, 2015.
Paris, J. D., Ciais, P., Nédélec, P., Stohl, A., Belan, B. D., Arshinov, M. Y., Carouge, C., Golitsyn, G. S., and Granberg, I. G.: New insights on the chemical composition of the Siberian air shed from the Yak-Aerosib aircraft campaigns, B. Am. Meteorol. Soc., 91, 625–642, https://doi.org/10.1175/2009bams2663.1, 2010.
Peckham, S. E., Grell, G. A., McKeen, S. A., Barth, M., Pfister, G., Wiedinmyer, C., Fast, J. D., Gustafson, W. I., Zaver, R., Easter, R. C., Barnard, J., Chapman, E., Hewson, M., Schmitz, R., Salzmann, M., and Freitas, S.: WRF/Chem version 3.3 user's guide, NOAA Technical Memo, 98 pp., 2012.
Pedersen, C.: Zeppelin Webcamera Time Series, Data set, Norwegian Polar Institute, https://doi.org/10.21334/npolar.2013.9fd6dae0, 2013.
Penuelas, J., Asensio, D., Tholl, D., Wenke, K., Rosenkranz, M., Piechulla, B., and Schnitzler, J. P.: Biogenic volatile emissions from the soil, Plant Cell Environ., 37, 1866–1891, https://doi.org/10.1111/pce.12340, 2014.
Petäjä, T., Mauldin, R. L., Kosciuch, E., McGrath, J., Nieminen, T., Paasonen, P., Boy, M., Adamov, A., Kotiaho, T., and Kulmala, M.: Sulfuric acid and OH concentrations in a boreal forest site, Atmos. Chem. Phys., 9, 7435–7448, https://doi.org/10.5194/acp-9-7435-2009, 2009.
Petäjä, T., de Leeuw, G., Lappalainen, H. K., Moisseev, D., O'Connor, E., Bondur, V., Kasimov, N., Kotlyakov, V., Guo, H. D., Zhang, J. H., Matvienko, G., Kerminen, V. M., Baklanov, A., Zilitinkevich, S., and Kulmala, M.: Connecting ground based in situ observations, ground-based remote sensing and satellite data within the Pan Eurasian Experiment (PEEX) program, Proc. Spie., 9242, 924206, https://doi.org/10.1117/12.2068111, 2014.
Petäjä, T., O'Connor, E. J., Moisseev, D., Sinclair, V. A., Manninen, A. J., Väänänen, R., von Lerber, A., Thorntoton, J. A., Nicocoll, K., Petersen, W., Chandrasekar, V., Smith, J. N., Winkler, P. M., Krüger, O., Hakola, H., Timonen, H., Brus, D., Laurila, T., Asmi, E., Riekkola, M. L., Mona, L., Massoli, P., Engelmann, R., Komppula, M., Wang, J., Kuang, C. G., Back, J., Virtanen, A., Levula, J., Ritsche, M., and Hickmon, N.: BAECC: a field campaign to elucidate the impact of biogenic aerosols on clouds and climate, B. Am. Meteorol. Soc., 97, 1909–1928, https://doi.org/10.1175/Bams-D-14-00199.1, 2016.
Petäjä, T., Ganzei, K. S., Lappalainen, H. K., Tabakova, K., Makkonen, R., Räisänen, J., Chalov, S., Kulmala, M., Zilitinkevich, S. S., Baklanov, P., Shakirov, R. B., Mishina, N. V., Egidarev, E. G., and Kondrat'ev, I. I.: Research agenda for the Russian Far East and utilization of multi-platform comprehensive environmental observations, J. Digital Earth, submitted, 2020.
Petzold, A., Ogren, J. A., Fiebig, M., Laj, P., Li, S.-M., Baltensperger, U., Holzer-Popp, T., Kinne, S., Pappalardo, G., Sugimoto, N., Wehrli, C., Wiedensohler, A., and Zhang, X.-Y.: Recommendations for reporting ”black carbon” measurements, Atmos. Chem. Phys., 13, 8365–8379, https://doi.org/10.5194/acp-13-8365-2013, 2013
Polyakov, I. V., Pnyushkov, A. V., Alkire, M. B., Ashik, I. M., Baumann, T. M., Carmack, E. C., Goszczko, I., Guthrie, J., Ivanov, V. V., Kanzow, T., Krishfield, R., Kwok, R., Sundfjord, A., Morison, J., Rember, R., and Yulin, A.: Greater role for Atlantic inflows on sea-ice loss in the Eurasian Basin of the Arctic Ocean, Science, 356, 285–291, https://doi.org/10.1126/science.aai8204, 2017.
Popovicheva, O., Diapouli, E., Makshtas, A., Shonija, N., Manousakas, M., Saraga, D., Uttal, T., and Eleftheriadis, K.: East Siberian Arctic background and black carbon polluted aerosols at HMO Tiksi, Sci. Total Environ., 655, 924–938, https://doi.org/10.1016/j.scitotenv.2018.11.165, 2019.
Poulter, B., Bousquet, P., Canadell, J. G., Ciais, P., Peregon, A., Saunois, M., Arora, V. K., Beerling, D. J., Brovkin, V., Jones, C. D., Joos, F., Gedney, N., Ito, A., Kleinen, T., Koven, C. D., McDonald, K., Melton, J. R., Peng, C. H., Peng, S. S., Prigent, C., Schroeder, R., Riley, W. J., Saito, M., Spahni, R., Tian, H. Q., Taylor, L., Viovy, N., Wilton, D., Wiltshire, A., Xu, X. Y., Zhang, B. W., Zhang, Z., and Zhu, Q. A.: Global wetland contribution to 2000–2012 atmospheric methane growth rate dynamics, Environ. Res. Lett., 12, 094013, https://doi.org/10.1088/1748-9326/aa8391, 2017.
Rast, M., Nieke, J., Ananasso, C., Bach, H., Ben Dor, E., Chabrillat, S., Colombo, R., Feret, J., Giardino, C., Green, R., Guanter, L., Marsh, S., Ong, C., Rum, G., Schaepman, M., Schlerf, M., Skidmore, A., Strobl, P., Gascon, F., Adams, J., Isola, C., and Del Bello, U.: The Copernicus hyperspectral imaging mission for the environment (CHIME), in: Abstract 2019 ESA living planet symposium, Milan, Italy, 13–17 May 2019.
Rautenberg, A., Schön, M., zum Berge, K., Mauz, M., Manz, P., Platis, A., van Kesteren, B., Suomi, I., Kral, S. T., and Bange, J.: The Multi-Purpose Airborne Sensor Carrier MASC-3 for Wind and Turbulence Measurements in the Atmospheric Boundary Layer, Sensors-Basel, 19, 2292, https://doi.org/10.3390/s19102292, 2019.
Riggs, G., Hall, D. K., and Román, M. O.: VIIRS Snow Cover Algorithm Theoretical Basis Document (ATBD), Version 1.0, available at: https://viirsland.gsfc.nasa.gov/PDF/VIIRS_snow_cover_ATBD_2015.pdf (last access: 30 June 2020), 2015.
Riipinen, I., Pierce, J. R., Yli-Juuti, T., Nieminen, T., Häkkinen, S., Ehn, M., Junninen, H., Lehtipalo, K., Petäjä, T., Slowik, J., Chang, R., Shantz, N. C., Abbatt, J., Leaitch, W. R., Kerminen, V. M., Worsnop, D. R., Pandis, S. N., Donahue, N. M., and Kulmala, M.: Organic condensation: a vital link connecting aerosol formation to cloud condensation nuclei (CCN) concentrations, Atmos. Chem. Phys., 11, 3865–3878, https://doi.org/10.5194/acp-11-3865-2011, 2011.
Rodell, M. and Houser, P. R.: Updating a land surface model with MODIS-derived snow cover, J. Hydrometeorol., 5, 1064–1075, https://doi.org/10.1175/Jhm-395.1, 2004.
Rückamp, M., Falk, U., Frieler, K., Lange, S., and Humbert, A.: The effect of overshooting 1.5 ∘C global warming on the mass loss of the Greenland ice sheet, Earth Syst. Dynam., 9, 1169–1189, https://doi.org/10.5194/esd-9-1169-2018, 2018.
Saiz-Lopez, A. and von Glasow, R.: Reactive halogen chemistry in the troposphere, Chem. Soc. Rev., 41, 6448–6472, https://doi.org/10.1039/c2cs35208g, 2012.
Salomonson, V. V. and Appel, I.: Development of the Aqua MODIS NDSI fractional snow cover algorithm and validation results, IEEE T. Geosci. Remote, 44, 1747–1756, https://doi.org/10.1109/Tgrs.2006.876029, 2006.
Salzano, R., Lanconelli, C., Salvatori, R., Esposito, G., and Vitale, V.: Continuous monitoring of spectral albedo of snowed surfaces in Ny-lesund, Rend. Lincei.-Sci. Fis., 27, 137–146, https://doi.org/10.1007/s12210-016-0513-y, 2016.
Salzano, R., Salvatori, R., Valt, M., Giuliani, G., Chatenoux, B., and Ioppi, L.: Automated Classification of Terrestrial Images: The Contribution to the Remote Sensing of Snow Cover, Geosciences, 9, 97, https://doi.org/10.3390/geosciences9020097, 2019.
Sandven, S., Ludwigsen, C. A., Sagen, H., Hamre, T., and Pirazzini, R.: Assessment of Arctic sea ice and ocean observing systems based on INTAROS survey, American Geophysical Union, Fall Meeting, 2018,
Sauvé, S. and Desrosiers, M.: A review of what is an emerging contaminant, Chem. Cent. J., 8, 15, https://doi.org/10.1186/1752-153x-8-15, 2014.
Schmale, J., Arnold, S. R., Law, K. S., Thorp, T., Anenberg, S., Simpson, W. R., Mao, J., and Pratt, K. A.: Local Arctic air pollution: a neglected but serious problem, Earths Future, 6, 1385–1412, https://doi.org/10.1029/2018ef000952, 2018.
Schmeisser, L., Backman, J., Ogren, J. A., Andrews, E., Asmi, E., Starkweather, S., Uttal, T., Fiebig, M., Sharma, S., Eleftheriadis, K., Vratolis, S., Bergin, M., Tunved, P., and Jefferson, A.: Seasonality of aerosol optical properties in the Arctic, Atmos. Chem. Phys., 18, 11599–11622, https://doi.org/10.5194/acp-18-11599-2018, 2018.
Schobesberger, S., Väänänen, R., Leino, K., Virkkula, A., Backman, J., Pohja, T., Siivola, E., Franchin, A., Mikkilä, J., Paramonov, M., Aalto, P. P., Krejci, R., Petäjä, T., and Kulmala, M.: Airborne measurements over the boreal forest of southern Finland during new particle formation events in 2009 and 2010, Boreal Environ. Res., 18, 145–163, 2013.
Schroeder, W., Oliva, P., Giglio, L., and Csiszar, I. A.: The New VIIRS 375 m active fire detection data product: Algorithm description and initial assessment, Remote Sens. Environ., 143, 85–96, https://doi.org/10.1016/j.rse.2013.12.008, 2014.
Schroeder, W. H., Anlauf, K. G., Barrie, L. A., Lu, J. Y., Steffen, A., Schneeberger, D. R., and Berg, T.: Arctic springtime depletion of mercury, Nature, 394, 331–332, https://doi.org/10.1038/28530, 1998.
Seki, O., Kawamura, K., Bendle, J. A. P., Izawa, Y., Suzuki, I., Shiraiwa, T., and Fujii, Y.: Carbonaceous aerosol tracers in ice-cores record multi-decadal climate oscillations, Sci. Rep.-UK, 5, 14450, https://doi.org/10.1038/srep14450, 2015.
Sertel, E., Robock, A., and Ormeci, C.: Impacts of land cover data quality on regional climate simulations, Int. J. Climatol., 30, 1942–1953, https://doi.org/10.1002/joc.2036, 2010.
Sharma, S., Ishizawa, M., Chan, D., Lavoué, D., Andrews, E., Eleftheriadis, K., and Maksyutov, S.: 16-year simulation of Arctic black carbon: Transport, source contribution, and sensitivity analysis on deposition, J. Geophys. Res.-Atmos., 118, 943–964, https://doi.org/10.1029/2012jd017774, 2013.
Sharma, S., Barrie, L. A., Magnusson, E., Brattstrom, G., Leaitch, W. R., Steffen, A., and Landsberger, S.: A Factor and Trends Analysis of Multidecadal Lower Tropospheric Observations of Arctic Aerosol Composition, Black Carbon, Ozone, and Mercury at Alert, Canada, J. Geophys. Res.-Atmos., 124, 14133–14161, https://doi.org/10.1029/2019jd030844, 2019.
Shaw, P. M., Russell, L. M., Jefferson, A., and Quinn, P. K.: Arctic organic aerosol measurements show particles from mixed combustion in spring haze and from frost flowers in winter, Geophys. Res. Lett., 37, L10803, https://doi.org/10.1029/2010gl042831, 2010.
Shipley, S. T., Tracy, D. H., Eloranta, E. W., Trauger, J. T., Sroga, J. T., Roesler, F. L., and Weinman, J. A.: high spectral resolution lidar to measure optical-scattering properties of atmospheric aerosols, 1. Theory and instrumentation, Appl. Optics, 22, 3716–3724, https://doi.org/10.1364/Ao.22.003716, 1983.
Shoeib, M., Harner, T., and Vlahos, P.: Perfluorinated chemicals in the Arctic atmosphere, Environ. Sci. Technol., 40, 7577–7583, https://doi.org/10.1021/es0618999, 2006.
Shupe, M., de Boer, G., Dethloff, K., Hunke, E., Maslowski, W., McComiskey, A., Perrson, O., Randall, D., Tjernstrom, M., Turner, D., and Verlinde, J.: The Multidisciplinary Drifting Observatory for the Study of Arctic Climate (MOSAIC) Atmosphere Science Plan, ARM Climate Research Facility, Pacific Northwest National Laboratory, Richland, WA; DOE Office of Science Atmospheric Radiation Measurement (ARM) Program (United States), 2018.
Simpson, W. R., von Glasow, R., Riedel, K., Anderson, P., Ariya, P., Bottenheim, J., Burrows, J., Carpenter, L. J., Friess, U., Goodsite, M. E., Heard, D., Hutterli, M., Jacobi, H. W., Kaleschke, L., Neff, B., Plane, J., Platt, U., Richter, A., Roscoe, H., Sander, R., Shepson, P., Sodeau, J., Steffen, A., Wagner, T., and Wolff, E.: Halogens and their role in polar boundary-layer ozone depletion, Atmos. Chem. Phys., 7, 4375–4418, https://doi.org/10.5194/acp-7-4375-2007, 2007.
Simpson, W. R., Brown, S. S., Saiz-Lopez, A., Thornton, J. A., and von Glasow, R.: Tropospheric Halogen Chemistry: Sources, Cycling, and Impacts, Chem. Rev., 115, 4035–4062, https://doi.org/10.1021/cr5006638, 2015.
Sipilä, M., Sarnela, N., Jokinen, T., Henschel, H., Junninen, H., Kontkanen, J., Richters, S., Kangasluoma, J., Franchin, A., Peräkylä, O., Rissanen, M. P., Ehn, M., Vehkämäki, H., Kurten, T., Berndt, T., Petäjä, T., Worsnop, D., Ceburnis, D., Kerminen, V. M., Kulmala, M., and O'Dowd, C.: Molecular-scale evidence of aerosol particle formation via sequential addition of HIO3, Nature, 537, 532–534, https://doi.org/10.1038/nature19314, 2016.
Skofronick-Jackson, G., Kirschbaum, D., Petersen, W., Huffman, G., Kidd, C., Stocker, E., and Kakar, R.: The Global Precipitation Measurement (GPM) mission's scientific achievements and societal contributions: reviewing four years of advanced rain and snow observations, Q. J. Roy. Meteor. Soc., 144, 27–48, https://doi.org/10.1002/qj.3313, 2018.
Skov, H., Christensen, J. H., Goodsite, M. E., Heidam, N. Z., Jensen, B., Wåhlin, P., and Geernaert, G.: Fate of elemental mercury in the arctic during atmospheric mercury depletion episodes and the load of atmospheric mercury to the arctic, Environ. Sci. Technol., 38, 2373–2382, https://doi.org/10.1021/es030080h, 2004.
Solberg, R., Koren, H., and Amlien, J.: A review of optical snow cover algorithms, Norwegian Computing Center Note, available at: https://www.nr.no/directdownload/4400/Solberg_-_A_review_of_optical_snow_algorithms.pdf (last access: 19 December 2019), 2006.
Sonke, J. E., Teisserenc, R., Heimbürger-Boavida, L. E., Petrova, M. V., Marusczak, N., Le Dantec, T., Chupakov, A. V., Li, C. X., Thackray, C. P., Sunderland, E. M., Tananaev, N., and Pokrovsky, O. S.: Eurasian river spring flood observations support net Arctic Ocean mercury export to the atmosphere and Atlantic Ocean, P. Natl. Acad. Sci. USA, 115, E11586–E11594, https://doi.org/10.1073/pnas.1811957115, 2018.
Spolaor, A., Barbaro, E., Christille, J. M., Kirchgeorg, T., Giardi, F., Cappelletti, D., Turetta, C., Bernagozzi, A., Björkman, M. P., Bertolini, E., and Barbante, C.: Evolution of the Svalbard annual snow layer during the melting phase, Rend. Lincei-Sci. Fis., 27, 147–154, https://doi.org/10.1007/s12210-015-0500-8, 2016.
Spolaor, A., Angot, H., Roman, M., Dommergue, A., Scarchilli, C., Vardè, M., Del Guasta, M., Pedeli, X., Varin, C., Sprovieri, F., Magand, O., Legrand, M., Barbante, C., and Cairns, W. R. L.: Feedback mechanisms between snow and atmospheric mercury: Results and observations from field campaigns on the Antarctic plateau, Chemosphere, 197, 306–317, https://doi.org/10.1016/j.chemosphere.2017.12.180, 2018.
Spolaor, A., Barbaro, E., Cappelletti, D., Turetta, C., Mazzola, M., Giardi, F., Björkman, M. P., Lucchetta, F., Dallo, F., Pfaffhuber, K. A., Angot, H., Dommergue, A., Maturilli, M., Saiz-Lopez, A., Barbante, C., and Cairns, W. R. L.: Diurnal cycle of iodine, bromine, and mercury concentrations in Svalbard surface snow, Atmos. Chem. Phys., 19, 13325–13339, https://doi.org/10.5194/acp-19-13325-2019, 2019.
Stein, A. F., Draxler, R. R., Rolph, G. D., Stunder, B. J. B., Cohen, M. D., and Ngan, F.: Noaa's Hysplit Atmospheric Transport and Dispersion Modeling System, B. Am. Meteorol. Soc., 96, 2059–2077, https://doi.org/10.1175/Bams-D-14-00110.1, 2015.
Stemmler, I. and Lammel, G.: Pathways of PFOA to the Arctic: variabilities and contributions of oceanic currents and atmospheric transport and chemistry sources, Atmos. Chem. Phys., 10, 9965–9980, https://doi.org/10.5194/acp-10-9965-2010, 2010.
Stohl, A., Forster, C., Frank, A., Seibert, P., and Wotawa, G.: Technical note: The Lagrangian particle dispersion model FLEXPART version 6.2, Atmos. Chem. Phys., 5, 2461–2474, https://doi.org/10.5194/acp-5-2461-2005, 2005.
Strahlendorff, M., Veijola, K., Gallo, J., Vitale, V., Savela, H., Smirnov, A., Tanaka, H., Sueyoshi, T., Nitu, R., and Larsen, J. R.: Value tree for physical atmosphere and ocean observations in the Arctic, Finnish Meteorological Institute, Reports 2019, 28 pp., https://helda.helsinki.fi/handle/10138/300768 (last access: 7 July 2020), 2019.
Ström, J., Engvall, A. C., Delbart, F., Krejci, R., and Treffeisen, R.: On small particles in the Arctic summer boundary layer: observations at two different heights near Ny-Alesund, Svalbard, Tellus B, 61, 473–482, https://doi.org/10.1111/j.1600-0889.2008.00412.x, 2009.
Tedesco, M. and Kokhanovsky, A. A.: The semi-analytical snow retrieval algorithm and its application to MODIS data, Remote Sens. Environ., 111, 228–241, https://doi.org/10.1016/j.rse.2007.02.036, 2007.
Tomasi, C., Vitale, V., Lupi, A., Di Carmine, C., Campanelli, M., Herber, A., Treffeisen, R., Stone, R. S., Andrews, E., Sharma, S., Radionov, V., von Hoyningen-Huene, W., Stebel, K., Hansen, G. H., Myhre, C. L., Wehrli, C., Aaltonen, V., Lihavainen, H., Virkkula, A., Hillamo, R., Strom, J., Toledano, C., Cachorro, V. E., Ortiz, P., de Frutos, A. M., Blindheim, S., Frioud, M., Gausa, M., Zielinski, T., Petelski, T., and Yamanouchi, T.: Aerosols in polar regions: A historical overview based on optical depth and in situ observations, J. Geophys. Res.-Atmos., 112, D16205, https://doi.org/10.1029/2007jd008432, 2007.
Tomasi, C., Lupi, A., Mazzola, M., Stone, R. S., Dutton, E. G., Herber, A., Radionov, V. F., Holben, B. N., Sorokin, M. G., Sakerin, S. M., Terpugova, S. A., Sobolewski, P. S., Lanconelli, C., Petkov, B. H., Busetto, M., and Vitale, V.: An update on polar aerosol optical properties using POLAR-AOD and other measurements performed during the International Polar Year, Atmos. Environ., 52, 29–47, https://doi.org/10.1016/j.atmosenv.2012.02.055, 2012.
Tomasi, C., Kokhanovsky, A. A., Lupi, A., Ritter, C., Smirnov, A., O'Neill, N. T., Stone, R. S., Holben, B. N., Nyeki, S., Wehrli, C., Stohl, A., Mazzola, M., Lanconelli, C., Vitale, V., Stebel, K., Aaltonen, V., de Leeuw, G., Rodriguez, E., Herber, A. B., Radionov, V. F., Zielinski, T., Petelski, T., Sakerin, S. M., Kabanov, D. M., Xue, Y., Mei, L. L., Istomina, L., Wagener, R., McArthur, B., Sobolewski, P. S., Kivi, R., Courcoux, Y., Larouche, P., Broccardo, S., and Piketh, S. J.: Aerosol remote sensing in polar regions, Earth-Sci. Rev., 140, 108–157, https://doi.org/10.1016/j.earscirev.2014.11.001, 2015.
Torseth, K., Andrews, A., Asmi, E., Eleftheriadis, K., Fiebig, M., Herber, A., Huang, L., Kylling, A., Lupi, A., Massling, A., Mazzola, M., Nøjgaard, J. K., Popovicheva, O., Schichtel, B., Schmale, J., and Sharm, S.: EU Action on Black Carbon in the Arctic, Review of observation capacities and data availability for Black Carbon in the Arctic region: EU Action on Black Carbon in the Arctic – Technical Report 1, December 2019, 35 pp., 2019.
Tunved, P., Ström, J., and Krejci, R.: Arctic aerosol life cycle: linking aerosol size distributions observed between 2000 and 2010 with air mass transport and precipitation at Zeppelin station, Ny-Ålesund, Svalbard, Atmos. Chem. Phys., 13, 3643–3660, https://doi.org/10.5194/acp-13-3643-2013, 2013.
Uttal, T., Starkweather, S., Drummond, J. R., Vihma, T., Makshtas, A. P., Darby, L. S., Burkhart, J. F., Cox, C. J., Schmeisser, L. N., Haiden, T., Maturilli, M., Shupe, M. D., De Boer, G., Saha, A., Grachev, A. A., Crepinsek, S. M., Bruhwiler, L., Goodison, B., McArthur, B., Walden, V. P., Dlugokencky, E. J., Persson, P. O. G., Lesins, G., Laurila, T., Ogren, J. A., Stone, R., Long, C. N., Sharma, S., Massling, A., Turner, D. D., Stanitski, D. M., Asmi, E., Aurela, M., Skov, H., Eleftheriadis, K., Virkkula, A., Platt, A., Førland, E. J., Iijima, Y., Nielsen, I. E., Bergin, M. H., Candlish, L., Zimov, N. S., Zimov, S. A., O'Neill, N. T., Fogal, P. F., Kivi, R., Konopleva-Akish, E. A., Verlinde, J., Kustov, V. Y., Vasel, B., Ivakhov, V. M., Viisanen, Y., and Intrieri, J. M.: International Arctic Systems for Observing the Atmosphere: An International Polar Year Legacy Consortium, B. Am. Meteorol. Soc., 97, 1033–1056, https://doi.org/10.1175/Bams-D-14-00145.1, 2016.
Vermote, E., Justice, C., Claverie, M., and Franch, B.: Preliminary analysis of the performance of the Landsat 8/OLI land surface reflectance product, Remote Sens. Environ., 185, 46–56, https://doi.org/10.1016/j.rse.2016.04.008, 2016.
Vihma, T., Uotila, P., Sandven, S., Pozdnyakov, D., Makshtas, A., Pelyasov, A., Pirazzini, R., Danielsen, F., Chalov, S., Lappalainen, H. K., Ivanov, V., Frolov, I., Albin, A., Cheng, B., Dobrolyubov, S., Arkhipkin, V., Myslenkov, S., Petäjä, T., and Kulmala, M.: Towards an advanced observation system for the marine Arctic in the framework of the Pan-Eurasian Experiment (PEEX), Atmos. Chem. Phys., 19, 1941–1970, https://doi.org/10.5194/acp-19-1941-2019, 2019.
von Lerber, A., Moisseev, D., Bliven, L. F., Petersen, W., Harri, A. M., and Chandrasekar, V.: Microphysical Properties of Snow and Their Link to Z(e)-S Relations during BAECC 2014, J. Appl. Meteorol. Clim., 56, 1561–1582, https://doi.org/10.1175/Jamc-D-16-0379.1, 2017.
von Lerber, A., Moisseev, D., Marks, D. A., Petersen, W., Harri, A. M., and Chandrasekar, V.: Validation of GMI Snowfall Observations by Using a Combination of Weather Radar and Surface Measurements, J. Appl. Meteorol. Clim., 57, 797–820, https://doi.org/10.1175/Jamc-D-17-0176.1, 2018.
Wania, F.: A global mass balance analysis of the source of perfluorocarboxylic acids in the Arctic ocean, Environ. Sci. Technol., 41, 4529–4535, https://doi.org/10.1021/es070124c, 2007.
Warneke, C., Froyd, K. D., Brioude, J., Bahreini, R., Brock, C. A., Cozic, J., de Gouw, J. A., Fahey, D. W., Ferrare, R., Holloway, J. S., Middlebrook, A. M., Miller, L., Montzka, S., Schwarz, J. P., Sodemann, H., Spackman, J. R., and Stohl, A.: An important contribution to springtime Arctic aerosol from biomass burning in Russia, Geophys. Res. Lett., 37, L01801, https://doi.org/10.1029/2009gl041816, 2010.
Warren, S. G.: Optical-Properties of Snow, Rev. Geophys., 20, 67–89, https://doi.org/10.1029/RG020i001p00067, 1982.
Warren, S. G. and Wiscombe, W. J.: A Model for the Spectral Albedo of Snow, 2. Snow Containing Atmospheric Aerosols, J. Atmos. Sci., 37, 2734–2745, https://doi.org/10.1175/1520-0469(1980)037<2734:Amftsa>2.0.Co;2, 1980.
Wehner, B., Siebert, H., Ansmann, A., Ditas, F., Seifert, P., Stratmann, F., Wiedensohler, A., Apituley, A., Shaw, R. A., Manninen, H. E., and Kulmala, M.: Observations of turbulence-induced new particle formation in the residual layer, Atmos. Chem. Phys., 10, 4319–4330, https://doi.org/10.5194/acp-10-4319-2010, 2010.
Westgate, J. N., Sofowote, U. M., Roach, P., Fellin, P., D'Sa, I., Sverko, E., Su, Y. S., Hung, H., and Wania, F.: In search of potential source regions of semi-volatile organic contaminants in air in the Yukon Territory, Canada from 2007 to 2009 using hybrid receptor models, Environ. Chem., 10, 22–33, https://doi.org/10.1071/En12164, 2013.
Wiedensohler, A., Birmili, W., Nowak, A., Sonntag, A., Weinhold, K., Merkel, M., Wehner, B., Tuch, T., Pfeifer, S., Fiebig, M., Fjäraa, A. M., Asmi, E., Sellegri, K., Depuy, R., Venzac, H., Villani, P., Laj, P., Aalto, P., Ogren, J. A., Swietlicki, E., Williams, P., Roldin, P., Quincey, P., Hüglin, C., Fierz-Schmidhauser, R., Gysel, M., Weingartner, E., Riccobono, F., Santos, S., Grüning, C., Faloon, K., Beddows, D., Harrison, R. M., Monahan, C., Jennings, S. G., O'Dowd, C. D., Marinoni, A., Horn, H. G., Keck, L., Jiang, J., Scheckman, J., McMurry, P. H., Deng, Z., Zhao, C. S., Moerman, M., Henzing, B., de Leeuw, G., Löschau, G., and Bastian, S.: Mobility particle size spectrometers: harmonization of technical standards and data structure to facilitate high quality long-term observations of atmospheric particle number size distributions, Atmos. Meas. Tech., 5, 657–685, https://doi.org/10.5194/amt-5-657-2012, 2012.
Willis, M. D., Leaitch, W. R., and Abbatt, J. P. D.: Processes Controlling the Composition and Abundance of Arctic Aerosol, Rev. Geophys., 56, 621–671, https://doi.org/10.1029/2018rg000602, 2018.
Wiscombe, W. J. and Warren, S. G.: A Model for the Spectral Albedo of Snow. I: Pure Snow, J. Atmos. Sci., 37, 2712–2733, https://doi.org/10.1175/1520-0469(1980)037<2712:Amftsa>2.0.Co;2, 1980.
WMO: WMO Statement on the status of the global climate in 2010, available at: https://library.wmo.int/doc_num.php?explnum_id=7739 (last access: 30 June 2020), 2011.
WMO: WMO Statement on the status of the global climate in 2011, available at: https://library.wmo.int/doc_num.php?explnum_id=3463 (last access: 30 June 2020), 2012.
Wöhrnschimmel, H., MacLeod, M., and Hungerbuhler, K.: Emissions, Fate and Transport of Persistent Organic Pollutants to the Arctic in a Changing Global Climate, Environ. Sci. Technol., 47, 2323–2330, https://doi.org/10.1021/es304646n, 2013.
Xie, Z. Y., Wang, Z., Mi, W. Y., Möller, A., Wolschke, H., and Ebinghaus, R.: Neutral Poly-/perfluoroalkyl Substances in Air and Snow from the Arctic, Sci. Rep., 5, 8912, https://doi.org/10.1038/srep08912, 2015.
Yin, D. M., Cao, X., Chen, X. H., Shao, Y. J., and Chen, J.: Comparison of automatic thresholding methods for snow-cover mapping using Landsat TM imagery, Int. J. Remote Sens., 34, 6529–6538, https://doi.org/10.1080/01431161.2013.803631, 2013.
Young, C. J., Furdui, V. I., Franklin, J., Koerner, R. M., Muir, D. C. G., and Mabury, S. A.: Perfluorinated acids in arctic snow: New evidence for atmospheric formation, Environ. Sci. Technol., 41, 3455–3461, https://doi.org/10.1021/es0626234, 2007.
Yumashev, D., van Hussen, K., Gille, J., and Whiteman, G.: Towards a balanced view of Arctic shipping: estimating economic impacts of emissions from increased traffic on the Northern Sea Route, Climatic Change, 143, 143–155, https://doi.org/10.1007/s10584-017-1980-6, 2017.
Zanatta, M., Laj, P., Gysel, M., Baltensperger, U., Vratolis, S., Eleftheriadis, K., Kondo, Y., Dubuisson, P., Winiarek, V., Kazadzis, S., Tunved, P., and Jacobi, H.-W.: Effects of mixing state on optical and radiative properties of black carbon in the European Arctic, Atmos. Chem. Phys., 18, 14037–14057, https://doi.org/10.5194/acp-18-14037-2018, 2018.
Zhao, Z., Xie, Z. Y., Möller, A., Sturm, R., Tang, J. H., Zhang, G., and Ebinghaus, R.: Distribution and long-range transport of polyfluoroalkyl substances in the Arctic, Atlantic Ocean and Antarctic coast, Environ. Pollut., 170, 71–77, https://doi.org/10.1016/j.envpol.2012.06.004, 2012.
Zheng, W., Xie, Z. Q., and Bergquist, B. A.: Mercury stable isotopes in ornithogenic deposits As tracers of historical cycling of Mercury in Ross Sea, Antarctica, Environ. Sci. Technol., 49, 7623–7632, https://doi.org/10.1021/acs.est.5b00523, 2015.
Zieger, P., Aalto, P. P., Aaltonen, V., Äijälä, M., Backman, J., Hong, J., Komppula, M., Krejci, R., Laborde, M., Lampilahti, J., de Leeuw, G., Pfüller, A., Rosati, B., Tesche, M., Tunved, P., Väänänen, R., and Petäjä, T.: Low hygroscopic scattering enhancement of boreal aerosol and the implications for a columnar optical closure study, Atmos. Chem. Phys., 15, 7247–7267, https://doi.org/10.5194/acp-15-7247-2015, 2015.