the Creative Commons Attribution 4.0 License.
the Creative Commons Attribution 4.0 License.
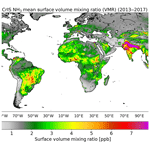
Ammonia measurements from space with the Cross-track Infrared Sounder: characteristics and applications
Enrico Dammers
Karen E. Cady-Pereira
Shailesh K. Kharol
Jesse Thompson
Yonatan Gainariu-Matz
Junhua Zhang
Chris A. McLinden
Andrew Kovachik
Michael Moran
Shabtai Bittman
Christopher E. Sioris
Debora Griffin
Matthew J. Alvarado
Chantelle Lonsdale
Verica Savic-Jovcic
Qiong Zheng
Despite its clear importance, the monitoring of atmospheric ammonia, including its sources, sinks, and links to the greater nitrogen cycle, remains limited. Satellite data are helping to fill the gap in monitoring from sporadic conventional ground- and aircraft-based observations to better inform policymakers and assess the impact of any ammonia-related policies. Presented is a description and survey that demonstrate the capabilities of the Cross-track Infrared Sounder (CrIS) ammonia product for monitoring, air quality forecast model evaluation, dry deposition estimates, and emission estimates from an agricultural hotspot. For model evaluation, while there is a general agreement in the spatial allocation of known major agricultural ammonia hotspots across North America, the satellite observations show some high-latitude regions during peak forest fire activity often have ammonia concentrations approaching those in agricultural hotspots. The CrIS annual ammonia dry depositions in Canada (excluding the territories) and the US have average and annual variability values of and Tg N yr−1, respectively. These satellite-derived dry depositions of reactive nitrogen from NH3 with NO2 show an annual ratio of NH3 compared to their sum (NH3+NO2) of ∼82 % and ∼55 % in Canada and the US, respectively. Furthermore, we show the use of CrIS satellite observations to estimate annual and seasonal emissions near Lethbridge, Alberta, Canada, a region dominated by high-emission concentrated animal feeding operations (CAFOs); the satellite annual emission estimate of 37.1±6.3 kt yr−1 is at least double the value reported in current bottom-up emission inventories for this region.
- Article
(21250 KB) - Full-text XML
- BibTeX
- EndNote
Ammonia (NH3) is the most abundant alkaline gas in the atmosphere and has major impacts on air, soil, and water quality. Ammonia generally reacts quickly with available acids (e.g. nitric and sulfuric acid from NOx and SOx) to produce a significant fraction (∼50 %) of the secondary fine particulate matter (diameter < 2.5 µm; e.g. Seinfeld and Pandis, 1988), which has significant human health impacts (e.g. cardiovascular and respiratory diseases; Schwartz et al., 2002; Reiss et al., 2007; Pope et al., 2000, 2002, 2009; Crouse et al., 2012). In addition to air quality impacts, NH3 and the aerosols formed from it (e.g. ammonium, ; ammonium nitrate, NH4NO3; and ammonium sulfate, (NH4)2SO4) deposit from the atmosphere to the surface. Deposition of reactive nitrogen (Nr) into ecosystems provides important nutrients (e.g. increases crop production) and helps feed the world population, but even in small amounts it can have negative impacts on sensitive ecosystems, such as soil acidification (Galloway et al., 2003), eutrophication (Bergstrom et al., 2006), changes to vegetation type, and biodiversity loss (Fenn et al., 2010; Bowman et al., 2012; Sheppard et al., 2011; Bauer et al., 2016). Ammonia gas is phytotoxic to certain plant species, mainly bryophytes and lichens typical of nutrient-poor natural ecosystems such as alpine areas and bogs.
Despite the importance of ammonia, historically anthropogenic emissions of NH3 have largely been unregulated, especially outside of Western Europe, which has contributed to the lack of observations and the associated large uncertainties in our knowledge of ammonia fluxes. Furthermore, traditional in situ surface-based monitoring of atmospheric ammonia is challenging due to the sticky nature of the ammonia molecule and the labour-intensive nature and high cost of performing the measurements, which result in making ammonia observations sparse over most regions, especially in remote locations. Combining this relative scarcity of observation networks with the typically high spatial and temporal variations of atmospheric ammonia concentrations, owing to its short lifetime (hours to a day) and numerous diffuse agricultural sources, leads to an overall lack of knowledge of ammonia compared to other common air quality contaminants, with the consequence that ammonia and ammonium are among the least known parts of the ecosystem's nitrogen cycle (Erisman et al., 2007).
Recent satellite observations of lower tropospheric ammonia are helping to fill in observational and knowledge gaps. Satellite observations of lower tropospheric ammonia have only been possible in the past decade through concurrent improvements in both the radiometric capabilities of infrared instruments on satellites and the radiative transfer forward modelling and inversion algorithms. This was first demonstrated by Beer et al. (2008) with NASA's Tropospheric Emission Spectrometer (TES) observations, which have since been followed by ammonia observations from the European Space Agency (ESA) Infrared Atmospheric Sounder Interferometer (IASI; Clarisse et al., 2009), the NASA/NOAA Cross-track Infrared Sounder (CrIS; Shephard and Cady-Pereira, 2015),the NASA Atmospheric Infrared Sounder (AIRS; Warner et al., 2016), and the JAXA Greenhouse Gases Observing Satellite (GOSAT; Someya et al., 2020). Out of this suite of instruments, AIRS, CrIS, and IASI all have large swaths providing daily global coverage. Furthermore, both IASI and CrIS are part of operational meteorological platforms with re-flights of instruments on several satellites in succession enabling multi-decadal time series (e.g. planned coverage spanning 2006–2021 for IASI, 2011–2038 for CrIS, and 2021–2042 for the next-generation IASI-NG). CrIS is the newest of these operational satellites and has the lowest radiometric noise in the portion of the spectrum commonly used for ammonia retrievals (Zavyalov et al., 2013). This provides CrIS with the potential for increased vertical sensitivity of ammonia near the surface along with global coverage. Presented here is a current survey of the CrIS NH3 fast physical retrieval (CFPR) product characteristics with some example applications.
The CrIS instrument is a Fourier transform spectrometer (FTS) launched by the US NOAA and NASA on the Suomi National Polar-orbiting Partnership (S-NPP) satellite on 28 October 2011 and on the NOAA-20 satellite on 29 November 2017. Here we focus only on the longer time series of data provided by the CrIS instrument flown on S-NPP. The S-NPP satellite is in a sun-synchronous low Earth orbit with overpass times of ∼01:30 and 13:30 local time. CrIS is a hyperspectral infrared instrument with a spectral resolution of 0.625 cm−1. The main advantage of CrIS is the combination of dense global coverage and the improved sensitivity in the boundary layer due to the low spectral noise of ∼0.04 K at 280 K in the NH3 spectral region (Zavyalov et al., 2013) and the early afternoon overpass that coincides with high thermal contrast (difference between the surface and air temperature) when the infrared instrument is more sensitive. A detailed description of the CrIS fast physical retrieval algorithm for deriving ammonia applied to both simulated spectra and initial sample observations was provided by Shephard and Cady-Pereira (2015). Since then the CFPR algorithm has been applied globally to CrIS spectra from May 2012 onwards. The input atmospheric state required for the radiative transfer forward model calculations is obtained from the Level 2 Cross-track Infrared and Microwave Sounding Suite (CrIMSS) Atmospheric Vertical Profile Environmental Data Record (product ID: REDRO; Divakarla et al., 2014) product from 1 May 2012 to 7 April 2014; after that the retrieved Level 2 NOAA Unique Combined Atmospheric Processing System (NUCAPS; Liu et al., 2014) is used. The CFPR retrieves the surface temperature and emissivity for each observation (field of view) prior to the ammonia retrieval. Ammonia profiles are retrieved at 14 profile levels to capture the vertical sensitivity of ammonia that varies from profile to profile depending on the atmospheric conditions. The CrIS satellite ammonia observations do not have equal sensitivity in the vertical and have coarse vertical resolution (e.g. ∼1 to 3 km). Hence, surface level values and total column values are both highly correlated with profile levels in the boundary layer where the satellite typically has peak vertical sensitivity. Note that atmospheric ammonia is typically short lived so that higher concentrations are generally close to the sources, which are generally near the surface. This is demonstrated later in Sect. 3.2 with model emissions and corresponding simulated surface concentrations showing similar patterns.
An update from the initial Shephard and Cady-Pereira (2015) analysis is that under favourable conditions CrIS detects NH3 near surface concentrations down to ∼0.3–0.5 ppbv (e.g. Kharol et al., 2018), which is less than half of the more conservative estimate of ∼1 ppbv previously reported using an Observation System Simulation Experiment (OSSE). This is mainly due to the better-than-specified noise capabilities in the observed CrIS NH3 spectra and the limited number of sampling conditions used in the original OSSE experiment.
Since the CFPR uses a mathematically robust physics-based optimal estimation framework (Rodgers, 2000) it provides the vertical sensitivity and the measurement information content (obtained from the averaging kernels), as well as an estimate of the retrieval errors (error covariance matrices), for each observation. The output sensitivity and error parameter characterization are key for utilizing CrIS observations in air quality model applications such as data assimilation, data fusion, and model-based emission inversions (e.g. Li et al., 2019). It is also important that, as done first in the TES NH3 retrieval (Shephard et al., 2011), the CFPR algorithm uses only three a priori ammonia profiles. These a priori profiles represent unpolluted, moderate, and polluted conditions with no prescribed latitudinal or seasonal dependence. For each retrieval, one of these three a priori profiles is selected based on the estimated ammonia spectral signal, as there is little known about ammonia globally (i.e. there is no spatial climatology field used for the a priori profile as is commonly done for retrievals of better-known species). The retrieval quality flags are described in Appendix A.
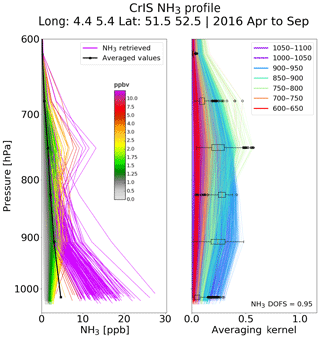
Figure 1CrIS-retrieved NH3 profiles from a 50 km radius around Cabauw, the Netherlands, from April to September 2016. The retrieved profile level values below 600 hPa are shown in (a) and coloured according to the surface value, and the corresponding rows of the averaging kernels are shown in (b). The box and whiskers showing the statistics (e.g. median, percentiles, and outliers (circles)) of the rows of the averaging kernel values at each retrieval level are also provided on the averaging kernel plot.
As shown in Fig. 1 the peak sensitivity is generally in the boundary layer below ∼700 hPa (3 km). The lower instrument noise with similar spectral resolution offered by CrIS allows for greater sensitivity near the surface, with less dependency on the thermal contrast from an operational meteorological sensor. This also follows from simulation studies performed by Clarisse et al. (2010) that show that even with twice the CrIS noise level there would be a significant reduction in the dependence on thermal contrast for sensitivity in the daytime boundary layer. As there is generally only ∼1 degree of freedom for signal (DOFS; e.g. 0.95 average in Fig. 1) with a coarse vertical resolution (half width at half maximum of the rows of the averaging kernels) of ∼1 to 3 km, the retrieved surface level concentrations are highly correlated with the retrieved levels at higher elevations in the boundary layer. The retrieved profiles in Fig. 1 still tend to have distributions that are grouped around the three a priori profiles, and therefore future updates to the retrieval will investigate various refinements to the a priori profiles and constraints. Figure 2 shows a sample single-day scene of NH3 retrievals on 15 May 2016 during the Fort McMurray fires (Adams et al., 2019) ranging from low background values of < 1 ppbv to elevated values up to 30 ppbv. Corresponding Cloud-Aerosol Lidar and Infrared Pathfinder Satellite Observation (CALIPSO; Winker et al., 2003) lidar measurements on this day show the smoke plume reaching altitudes above the ground of ∼2.0 to 3.0 km (∼800 to 700 hPa); for reference see Appendix Fig. B1 obtained from: https://www-calipso.larc.nasa.gov/data/BROWSE/production/V4-10/2016-05-15/2016-05-15_19-42-56_V4.10_3_6.png, (last access: 19 February 2020).
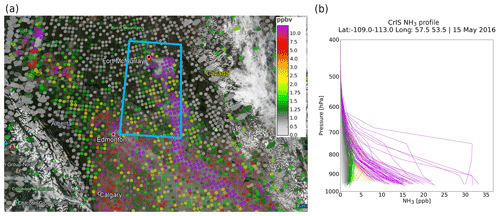
Figure 2Overlay of the CrIS-retrieved surface NH3 pixels on the VIIRS true-colour visible imagery for 15 May 2016 showing the plume from the Fort McMurray fires (a). Retrieved NH3 profiles colour coded using the surface level values in the blue box on the map (b). (Underlying VIIRS images obtained from NASA Worldview – https://worldview.earthdata.nasa.gov/, last access: 19 February 2020.)
The estimated random errors for both the observation (consisting of only measurement errors here as no cross-state errors are estimated) and the total error (includes the measurement and representative, or smoothing, error) are computed for both the individual retrieval profile levels and the integrated total column (see Shephard and Cady-Pereira, 2015). Observation errors can be used if the vertical resolution of the satellite observations has already been taken into consideration (e.g. satellite observation operator is applied to the comparison dataset), whereas the total error should be used if the satellite-retrieved value is to represent the discrete observation resolution (e.g. individual profile level value, or vertical column value, where the vertical sensitivity is not considered). Errors from a single day of global retrievals as a function of concentration amounts are provided in Fig. 3 for total column and in Fig. 4 for values from each profile level in the boundary layer below 700 hPa (∼3 km). For the total column amounts, the measurement errors are typically in the 10 % to 15 % range, whereas the total errors are ∼30 %. The individual profile retrieval levels have measurement errors of ∼10 %, except for low concentrations with amounts < 1 ppbv where the error rises to ∼30 %. When the smoothing error component is included for the individual profile levels, the profile level total random error increases to the range of 60 % to 100 %. This is expected given that current ammonia nadir infrared retrievals have limited vertical information (resolution), leading to significant smoothing of the retrieved profile.
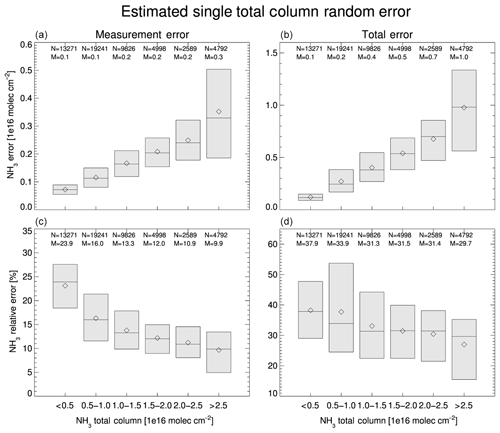
Figure 3Estimated CrIS-retrieved NH3 total column measurement and total error statistics from global values for 28 May 2017. Both absolute error estimates (a, b) and corresponding relative (fractional) errors (c, d) are shown. The diamonds are the mean values for each box range. Only retrievals over land with a quality flag of 5 (therefore DOFS ≥0.1) were included. The N and M are the number of points and the median value for each box, respectively.
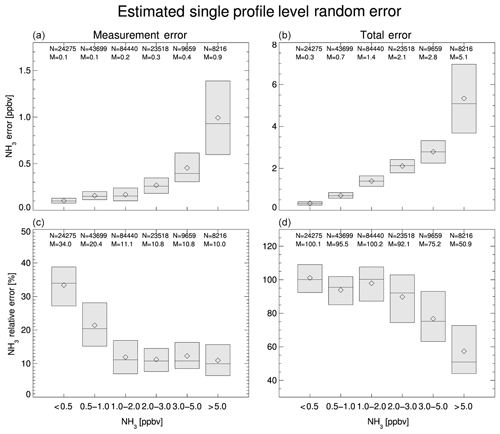
Figure 4Errors for the individual for all profile levels below 700 hPa (∼3 km) using the same plotting criteria as in Fig. 3.
The CFPR (version 1.3) was also validated by Dammers et al. (2017) against ground-based Fourier transform infrared (FTIR) spectroscopy observations to determine the actual errors (as opposed to the estimated retrieval errors). These initial results show good overall comparison results with a correlation of r∼0.8 and a slope of 1.02. For retrievals with total column values > 1.0×1016 molec. cm−2 (ranging from moderate to high levels) the relative bias difference is < 5 %, with a standard deviation ranging from 25 % to 50 %. For total column comparisons for smaller values < 1.0×1016 molec. cm−2, there are larger differences with a CrIS higher than the FTIR by ∼30 % with a standard deviation of ∼40 %. Initial CrIS comparisons of the surface level retrievals with in situ surface observations from the Ammonia Monitoring Network (AMoN) over North America show a correlation of 0.76 and an overall mean CrIS–AMoN difference of % (Kharol et al., 2018). An extension of this analysis over more surface locations and longer time periods is currently being performed. These CrIS-retrieved ammonia profiles (Level 2 products) are used to generate gridded averaged (Level 3) products over various spatial grids and time periods (e.g. monthly average on latitude and longitude grid). To reduce discontinuities between adjacent grid points and increase the effective resolution of observations that are averaged over extended time periods (e.g. monthly), oversampling with weighting (e.g. Gaussian weighting based on distance from the centre of the grid; e.g. Fioletov et al., 2011; Pommier et al., 2013) is used in the generation of the Level 3 gridded averaged products. The output Level 3 product grid size and level of oversampling are flexible and based on the purpose and number of observations (e.g monthly, annual, multi-year). Figure 5 is a sample global plot of the CrIS gridded surface level NH3 for the 2013–2017 time period showing global hotspots over land. Figure 5 shows the typical elevated large area NH3 hotspots with annual values averaging over ∼7 ppbv. These regions with high NH3 concentrations include the Indo-Gangetic Plain in India and Pakistan, the Nile Delta in Egypt, California's Central Valley and the central US and Canada, the Comarca Lagunera and Los Altos de Jalisco regions in Mexico, northern central Colombia and the west coast of Peru in South America, the Po Valley in Italy and Ebro Valley in Spain, the Fergana Valley in Central Asia, the Mekong Delta region in southern Vietnam, south central Thailand, Indonesia, and regions in eastern China. CrIS sensitivity is also demonstrated through its capability to observe ammonia over agricultural regions in the southern part of the Australian continent and forest-fire-prone regions in the north tips of the Northern Territory and Queensland. These elevated regions are also seen in the corresponding column-integrated total column values in Fig. C1. Elevated ammonia amounts seen over some desert regions such as the Taklimakan Desert in China and the Sahara with no obvious local ammonia sources need further investigation.
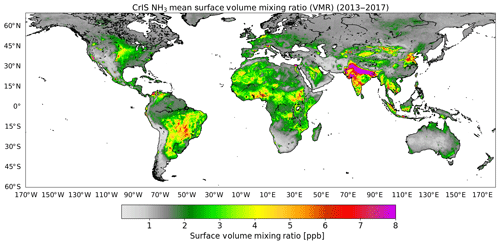
Figure 5CrIS 5-year mean (2013–2017) of surface ammonia globally over land. The CrIS mean gridded Level 3 values are generated on a uniform () grid with a quality flag of 5.
The distribution of the CrIS degrees of freedom for signal for a 5-year mean (2013–2017) corresponding to Fig. 5 is provided in Fig. 6. As noted previously, for satellite nadir NH3 observations there are often limited independent pieces of information with DOFS values of ∼1 or less. This information distribution depends on the atmospheric state (i.e. abundance of NH3, temperature, vertical thermal contrast in temperature, etc.). Thus, warmer climates with more persistent NH3 sources will tend to have more DOFSs. At higher latitudes where there are both few agricultural sources of NH3 and cooler temperatures, there are often fewer DOFSs on average; the exception is often under conditions where there are large episodic biomass burning events. What is averaged out of a 5-year mean plot is that single-pixel retrievals can reach maximum DOFSs of ∼2 in large forest fire plumes.
The CrIS NH3 product can be used for many applications such as monitoring, air quality model evaluation (e.g. Whaley et al., 2018; Pleim et al., 2019), dry deposition estimates (Kharol et al., 2018), and emission estimates for larger agriculture sources, industrial point sources (Dammers et al., 2018), and wildfires (Adams et al., 2019). Here we provide examples that demonstrate and expand upon these applications.
3.1 Monitoring
As previously noted, the operational polar-orbiting satellites (e.g. IASI and CrIS) have the benefit of providing daily global spatial coverage on local to regional (e.g. tens of kilometres) scales over many decades that can help fill in gaps in current monitoring networks. Provided here are examples of daily, seasonal, and annual observations of ammonia by the CrIS satellite. While not currently performed, it is possible to derive global daily ammonia products in near real time.
3.1.1 Daily
Ammonia in general is relatively short lived in the boundary layer, so its day-to-day atmospheric concentration levels over a region can vary greatly depending mainly on the meteorology (e.g. wind speed, temperature), episodic events (e.g. biomass burning, spreading of fertilizer), and concentration of reactant acid gases. However, ammonia in the free troposphere above the boundary layer, for example during forest fires, is not quickly scavenged or deposited and hence has a longer lifetime and travels over large distances (Lutsch et al., 2019). In addition, ammonia deposited on certain surfaces (e.g. vegetation) can be re-released into the atmosphere later (bidirectional flow) depending on the ammonia balance between the air and leaf apoplastic concentrations (compensation point; e.g. Massad et al., 2010; Bash et al., 2013; Pleim et al., 2019). Figure 7 shows CrIS surface NH3 over North America for four consecutive days from 5 to 8 July 2014 and the corresponding Aqua Moderate Resolution Imaging Spectroradiometer (MODIS) true-colour visible imagery with thermal hotspots (e.g. forest fires) overlaid in red. The elevated ammonia concentrations released from forest fires identified from the thermal hotspots and smoke plumes in the MODIS imagery are in the CrIS maps for northern central Canada on all 4 d. The impact of wind on the location of the forest fire plumes is also visible. The daily maps also show the impact of cloud cover on the CrIS NH3 retrievals, since optically thick clouds block the weaker ammonia signal from below the clouds.
In version 1.5 of the CFPR NH3 retrievals the observations with insufficient ammonia signal in the measured spectrum, mainly due to ammonia concentrations below the sensor detection limit (< 0.3–1.0 ppbv) or clouds blocking the ammonia signal, are not currently being processed. Thus, cloud filtering is presently achieved implicitly through the threshold of the ammonia signal in the spectra (i.e. no ammonia spectral signal through clouds) and the use of a surface brightness temperature threshold derived from global seasonal climatological cloud-top temperatures (based on International Satellite Cloud Climatology Project, ISCCP, maps; https://isccp.giss.nasa.gov/products/browsed2.html, last access: 19 February 2020). This cloud prescreening also improves the data processing rate as it reduces the number of potential number of retrievals globally by an average of 35 %, with this rate varying depending on location and season. Comparing the MODIS true-colour imagery with the CrIS NH3 observations in Fig. 7 demonstrates that this technique is very effective for cloud screening. Note that thin clouds (cloud optical depth < 1.0) that are near the surface with cloud-top temperatures close to the surface temperature still impact the current ammonia retrievals but in general have a non-significant impact on the overall results as seen in the examples in Fig. 7. Algorithm refinements such as directly incorporating a coincident VIIRS cloud product to distinguish the cloudy pixels from those that have concentrations levels below the detection limits are presently being tested. For regions with low concentrations, this has the potential to increase the density of observations included while tending to decrease mean background values.
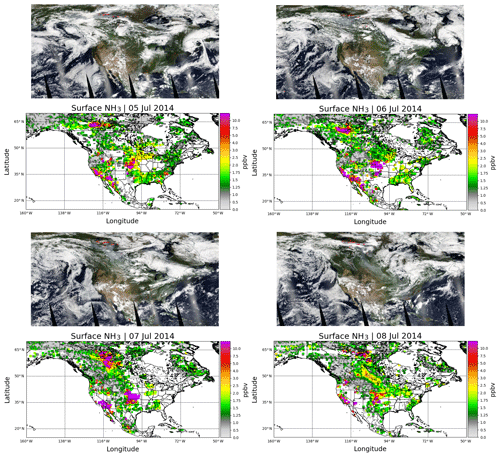
Figure 7Single-day maps of MODIS true-colour visible imagery and corresponding CrIS surface NH3 observation for four consecutive days from 1 to 4 June 2016. Overlaid on the MODIS visible images are the red thermal hotspots indicating forest fire locations. (MODIS images obtained from NASA Worldview – https://worldview.earthdata.nasa.gov/, last access: 19 February 2020).
3.1.2 Seasonality
Ammonia concentrations in the atmosphere are influenced by agricultural practices and meteorological conditions. Ammonia emissions differ over the course of the growing season due to changing farming practices and ambient temperature, leading to a month-to-month variation in concentrations. As an example, the relative spatial seasonal variability in surface concentrations over North America is shown in Fig. 8. For most of North America, there is often an increase in concentrations during the springtime associated with fertilizer and manure applications, as well as warming surfaces, at the start of the growing season, which shifts from April in the southern to central parts of the US to May in the northern states and most of Canada. There can also be an increase over some source regions (i.e. US Midwest, Idaho, Washington state) in the summertime associated with increased temperatures and certain farm practices like cleaning corals and manure storages and spreading, which promote greater volatilization. Also apparent in the plots is the increase in concentrations in the non-agricultural northern-latitude regions during the drier summer season associated with wildfires, which can inject ammonia with minimal acid reactants into the free troposphere, allowing the transport of ammonia over larger regions (Lutsch et al., 2016, 2019). The retrieved elevated concentration values at high elevation over the Rocky Mountains in the wintertime needs to be further investigated as a potential retrieval issue. The corresponding plot of total column values provided in Fig. C2 shows similar spatial seasonal patterns to those seen in the retrieved surface values. This is generally expected as ammonia is typically short lived in the boundary layer, so higher agricultural hotspots are close to source locations, plus both surface level retrievals and the corresponding integrated total column values are correlated with the profile retrievals in the boundary layer where the satellite typically has maximum sensitivity (as shown in Fig. 1).
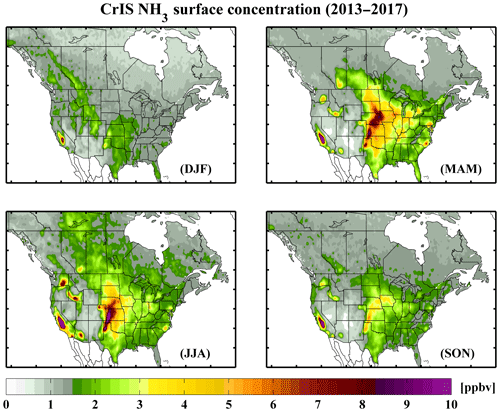
Figure 8The 5-year mean maps of surface NH3 over North America during the meteorological winter (December–January–February, DJF), spring (March–April–May, MAM), summer (June–July–August, JJA), and fall (September–October–November, SON).
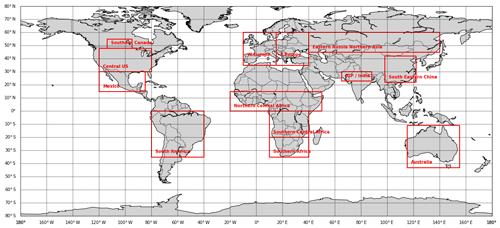
Figure 9Delimitation of large regions of interest in which CrIS NH3 are grouped together for regional time series analysis.
The time series using 5 years of CrIS data can give additional insight into the change in ammonia over time for various seasons. Time series in CrIS-retrieved surface ammonia for the 12 extended regions depicted in Fig. 9 are shown in Fig. 10. The most salient features in the regional time series reflect the seasonal cycle seen for most regions (e.g. South Eastern China, South America), where the ammonia concentrations peak in the warm growing season and are at a minimum during the colder season. Some regions also show a double peak in concentration amounts during the growing season (e.g. central US) that can be associated with the large springtime fertilizer or manure application, with a second peak a couple of months later due to increasing temperatures, which can be associated with increased agricultural ammonia volatilization and/or biomass burning. Some regional time series show an increase in peak ammonia concentration amounts with time (e.g. South America), while others show more constant seasonal patterns over the years (e.g. central US). In addition to agricultural practices, there can also be contributions to the atmospheric ammonia amounts due to biomass burning for some of the regions. When wintertime temperatures are near or below freezing there is a decrease in satellite sensitivity to ammonia, which reduces observation density and can create a small positive bias (e.g. southern Canada in 2016 and 2017).
3.1.3 Interannual variability
Even though the lifetime of ammonia in the lower boundary layer is influenced by the concentration of acid gases, especially SOx, one would still expect the mean surface concentrations to be spatially representative of the nearby surface emissions (as shown later in Sect. 3.2). Figure 11 shows the 5-year (2013–2017) mean plot of CrIS-retrieved surface concentrations over North America and the anomalies with respect to this multi-year mean. Many of the regions with elevated concentrations correspond to known agricultural emission zones across North America, such as the Central Valley in California, Washington state, Idaho, the Midwest (e.g. Nebraska), and North Carolina in the US. The agricultural hotspots in Canada are near Lethbridge in Alberta, southern Manitoba, southwestern Ontario, and the St. Lawrence River valley of Quebec. Going from southern to northern latitudes in North America, the crop-growing season and associated fertilizer application and lower animal densities, especially lower numbers of housed animals with higher emissions than pastured animals, continually decrease, which is reflected in the general decrease in CrIS-observed concentrations. The exception is the contribution of ammonia during wildfires due to both the burning vegetation and volatilized soil nitrogen (e.g. Urbanski, 2004). For example, there was a large number (∼385) of forest fires covering ∼3.4 million hectares in the Northwest Territories (NWT) during 2014 (NWT_FSRR, 2015), compared to 2013 and 2016 with less fire activity (Munoz-Alpizar et al., 2017). The year 2017 had relatively larger fire-driven emissions in the western and northern parts of the continent, especially in British Columbia, Canada (Chen et al., 2019). Most regions over the US show a maximum in surface concentrations during this 5-year period occurring in 2017, which is consistent with corresponding surface AMoN stations across the US (Kharol et al., 2020).
3.2 Model evaluation
Chemical transport models (CTMs) are used for many ammonia-related air quality applications such as estimating acid deposition and secondary particulate matter formation, as well as scenario runs to inform policy development (e.g. Engardt et. al., 2017; Liu et. al., 2019; Makar et al., 2009; Pinder et. al., 2007). Evaluation of the model performance against observations is a key part of air quality modelling validation that ultimately leads to an improved model performance (e.g. Shephard et al., 2011, Van Damme et al., 2014). An example of using CrIS NH3 observations for CTM evaluation is provided by Whaley et al. (2018). In that study a newly implemented ammonia bidirectional flux scheme and the inclusion of biomass burning into Environment and Climate Change Canada's air quality forecast model, the Global Environmental Multi-scale – Modelling Air Quality and Chemistry (GEM-MACH) model (Gong et al., 2015; Makar et al., 2015a, b; Pendlebury et al., 2018), were evaluated over northern Canada using CrIS NH3 observations. CrIS NH3 observations have also recently been used to evaluate improvements to the Community Multiscale Air Quality Model (CMAQ; Pleim et al., 2019). They demonstrated that CMAQ underestimated NH3 concentrations in the spring but also that CMAQ and CrIS present the same pattern of high NH3 in the California Central Valley, the Snake River Valley, and the western High Plains, all regions with high soil pH resulting in high NH3 fluxes, suggesting that CMAQ modelling of soil pH and the fluxes dependent on this parameter are reasonably well modelled.
Figure 12 compares CrIS-observed NH3 surface concentrations with mean values predicted by GEM-MACH and the corresponding NH3 emissions used by the model. The GEM-MACH-ready hourly gridded NH3 emissions at 10 km resolution over North America (NA) were generated using the SMOKE (Sparse Matrix Operator Kernel Emissions) emissions processing system (Baek and Seppanen, 2018; Zhang et al., 2018). These are based on the 2013 Canada's Air Pollutant Emission Inventory (APEI, accessed 2019), projected 2017 US National Emissions Inventory (NEI) obtained from the US Environmental Protection Agency's (EPA) 2011 Version 6 Air Emissions Modeling Platforms (AEMP, 2019), and the 2008 Mexican inventory obtained from the EPA's 2007/2008 Version 5 Air Emissions Modeling Platforms (https://www.epa.gov/air-emissions-modeling/20072008-version-5-air-emissions-modeling-platforms, last access: 19 February 2020). Figure 12a shows the average monthly total NH3 emissions during a 2-month summer period (July and August) over the GEM-MACH North American grid. The corresponding average NH3 surface concentration fields predicted by GEM-MACH during July and August 2016 are shown in Fig. 12b. The spatial distribution of model-predicted anthropogenic NH3 surface concentration agrees well with the spatial distribution of bottom-up model emissions in Fig. 12a. This is expected since NH3 is a short-lived reactive species and high concentrations of NH3 occur mainly in the areas with high NH3 emissions.
In general, the locations of the elevated ammonia hotspot regions in the simulated model surface concentration map in Fig. 12b spatially co-located with those observed by CrIS (Fig. 12c). This is seen in the hotspot regions such as Lethbridge, Alberta, and southwestern Ontario in Canada, as well as the Central Valley in California, Washington state, Idaho, the Midwest (e.g. Nebraska), and North Carolina in the US. The peak values in the hotspots in the upper Midwest and eastern US for these 2 months in 2016 are generally higher in the model than the satellite observations. The satellite tends to show higher values than the model in the central US (e.g. Nebraska). Comparing the model emission and concentration fields in Fig. 12 would indicate that these differences are mainly due to the input emission fields. Similar results (not shown here) are also seen with other chemical transport models (e.g. GEOS-Chem) using the same US EPA emissions inventory. In addition, in the western part of the US the satellite observations tend to be slightly higher over regions with elevated NH3, most notably over the Central Valley and High Plains. There are also several elevated regions in the satellite observations in Mexico that appear to be underreported in the emission inventories. One other potential difference between the model simulations and the satellite observations is the contribution of forest fires to the NH3 concentration amounts, especially at higher latitudes where there are limited agricultural sources. The reason for this potential difference is that the fire emissions were not considered in this GEM-MACH simulation. Comparing the model results in these northern latitudes with the satellite observations demonstrates that these large episodic wildfires in July and August in northern latitudes can produce mean bimonthly concentrations over large regions approaching the elevated anthropogenic agriculture values at lower latitudes, even for the summer of 2016 that was a relatively quiet wildfire season in Canada (Munoz-Alpizar et al., 2017). Thus, fire emissions should be included in any model simulations, especially over regions with limited agricultural sources.
3.3 Dry deposition of reactive nitrogen
Deposition of basic ammonia and ammonium-containing aerosols on land surfaces leads to acidification of the soil, when ammonium is oxidized (nitrified) to nitrate (; Goulding, 2016). The protons generated from this reaction cause the acidification. Excessive atmospheric deposition of NH3 adds reactive nitrogen into water that can contribute to eutrophication. Kharol et al. (2018) first demonstrated the utility of using CrIS NH3 observations with modelled dry deposition velocities to compute estimates of dry deposition of reactive nitrogen (Nr) from ammonia for the 2013 warm (growing, April to September) season over North America. Van der Graaf et al. (2018) followed a similar approach over Europe using the LOTOS-EUROS model to convert IASI total column NH3 observations into surface estimates. Here we expanded upon the CrIS dry deposition seasonal analysis to compute annual estimates of the relative Nr dry deposition flux from NH3 and nitrogen dioxide (NO2) for the years 2013–2017. As described in Kharol et al. (2018), the Ozone Monitoring Instrument (OMI) was used for the NO2 deposition estimates. The spatial patterns of the annual 2013 NH3 dry deposition flux shown in Fig. 13 closely resemble the warm-season results shown by Kharol et al. (2018), as ammonia has a short lifetime in the atmosphere, so the majority of the ammonia deposition occurs on leafy vegetation during the growing season close to agricultural sources. In contrast, the dry deposition of NO2 is generally associated with emissions from urbanized areas and industrial sources year-round, changing only slightly during the warm season. This results in total dry deposition in Canada (excluding the Northwest Territories) and the US in 2013 from NH3 (NO2) of ∼0.75 (0.17) and ∼1.09 (0.91) Tg N yr−1, respectively, of which ∼0.5 (0.1) Tg N per warm season and ∼0.9 (0.4) Tg N per warm season were deposited in the growing seasons as in Kharol et al. (2018). The 2013 annual ratio maps show NH3 having a larger proportion of the NH3+NO2 (∼82 % and ∼55 % over Canada and the US). As shown in Fig. 13, 31 out of the 50 US states (mostly located in central and western US) show a greater dry deposition rate from NH3 compared to NO2. In contrast, the industrial northeastern states indicate a higher dry deposition rate from NO2 than NH3. NH3 is expected to continue to be the dominant source of the reactive-nitrogen dry deposition flux over most regions in North America as NH3 emissions are projected to increase in the future (e.g. Bauer et al., 2016; Ellis et al., 2013; Paulot et al., 2013) and because of declining trends in NO2 emissions (e.g. Kharol et al., 2015; Krotkov et al., 2016; Lamsal et al., 2015).
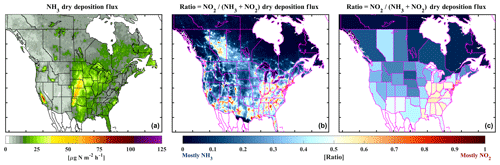
Figure 13The 2013 plots of the CrIS satellite-derived dry deposition flux of reactive nitrogen. From (a) to (c) this figure contains plots of dry deposition from NH3 and the relative ratio of NO2 to (NH3+NO2), with this ratio averaged over geopolitical boundaries.
The 5-year annual mean NH3 dry deposition flux for the period of 2013–2017 over North America is shown in Fig. 14a. The year-to-year variability in the NH3 dry deposition flux over North America is shown in Fig. 14b–f. The hotspots in the northern latitudes during 2014 and 2015 are mainly associated with large forest fires that may lead to a 2–3 fold local increase relative to the background value (Kharol et al., 2018). The NH3 dry deposition flux hotspots evident in the agricultural states of the central US and the Canadian provinces of Alberta, British Columbia, and Saskatchewan during 2017 (Fig. 14f) are mainly due to the combined effect of forest fires and warmer-than-average summers as shown in the CrIS NH3 concentration maps in Fig. 11. The annual average and variability in ammonia dry deposition of reactive nitrogen over Canada and the US is and Tg N yr−1, respectively. Note that there will be a significant contribution and variability from large forest fires in northern latitudes as seen in Fig. 14.
3.4 Emission estimate for a concentrated agricultural region
Another application of CrIS ammonia observations is emission estimates. Emission inventories are traditionally built from the bottom up, using emission factors and source locations to construct a complete inventory (e.g. see Appendix D). This process is very labour intensive, which means that the inventories are often released somewhat infrequently, with gaps of a few years between releases. Furthermore, the inventories can be incomplete or inaccurate due to a lack of knowledge on source locations, magnitudes, and temporal variations. This is particularly true for farm-based emissions that require complex data about farm activities, which can only be obtained with complex farm surveys typically conducted sporadically. Top-down satellite observations can be used to provide another source of emissions information and to supplement the inventories with more detailed information on hotspot locations and temporal variations (e.g. seasonal and inter-annual variations; e.g. Van Damme et al., 2018; Dammers et al., 2019). The use of CrIS NH3 concentration observations with corresponding wind information to derive emissions has been demonstrated for wildfire sources (e.g. Fort McMurray forest fires that occurred in 2016 in Alberta, Canada, Adams et al., 2019) and globally from localized industrial sources (e.g. fertilizer factories; Dammers et al., 2019). Here we demonstrate the potential to use CrIS NH3 observations over an extended agricultural area by adapting a similar emission estimate technique as used by Dammers et al. (2019) to estimate NH3 emissions over an agricultural region in Lethbridge, Canada, with many concentrated animal feeding operations (CAFOs). A detailed explanation on the procedure for the satellite-derived ammonia lifetime and emission estimates is provided in Dammers et al. (2019). The initial CrIS dataset is first filtered for observations with a quality factor flag of 5 and DOF filter ≥0.8. Next, we removed the influence of nearby forest fires following Dammers et al. (2019). The remaining CrIS satellite observations were matched in space and time with the ERA-Interim wind fields, and using that information the individual measurement locations are rotated according to their wind direction about a reference point in order to align their values (Pommier et al., 2013).
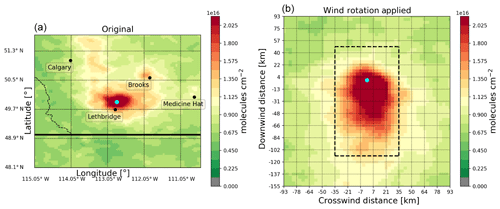
Figure 15Plot of the CrIS NH3 total column observations from the 5-year (2013–2017) period over Lethbridge, Alberta, Canada. The (a) plot shows the distribution of NH3 for the surrounding region. The black dots indicate nearby population centres for easy reference. The (b) plot shows the same set of observations for a smaller region centred on Lethbridge but now redistributed by wind direction showing the upwind and downwind total columns. The blue circle shows the location of the reference point used in the wind rotation and corresponds to the geographic centre of emissions.
Figure 15 shows the 5-year (2013–2017) non-rotated mean total column concentrations in southern Alberta (a) and the rotated total column (b) concentrations around Lethbridge, Alberta, for the same period. The concentrations peak near the Lethbridge location, with the peak transported downwind due to advection. The lifetime, plume-spread, background, and emission enhancement parameters are then estimated using a 2-D exponentially modified Gaussian (EMG) plume model. Over this region we only use warm-season observations to optimize for favourable measurement conditions (6 months from April to September) and obtained an emission of 37.35±6.3 kt per warm season, with a plume spread of 18.9±0.2 km and a lifetime of 2.66±0.04 h. Assuming that emissions are constant in time, the annual emissions would be 74.7±12.6 kt yr−1. However, emissions in this region are expected to vary, especially on a monthly/seasonal basis. The 2013 Canadian ammonia emissions from agriculture indicator (AEAI) monthly emission inventory (Sheppard and Bittman, 2016) states 30 % of all emissions are assumed to take place in the cold season (October–March) and 70 % in the warm season (April–September). Therefore, the annual emission total has to be adjusted by a factor of , which makes the adjusted emission total 53.4±9.0 kt yr−1. Similarly, the diurnal emission profile can be approximated by using the diurnal emission profile for livestock that is used to prepare emissions for the GEM-MACH model. Most of the emissions around Lethbridge are from livestock, which has a peak in the morning to middle of the day following cattle activity including feeding and excretion and the increasing surface temperatures (Denmead et al., 2014; Van Haarlem et al., 2008), and are thus emitted before the satellite overpass time (∼01:30 LT). Thus, the inventory value from an hour before the overpass (12:30 LT) is used to adjust the emissions to daily averages by a factor of 1∕1.44 leading to a final annual emission total of 37.1±6.3 kt yr−1. Figure 16 show the CrIS estimated emissions compared to the AEAI, Canada's Air Pollutant Emission Inventory (APEI, 2017; see Appendix D), and the global Hemispheric Transport of Air Pollution (HTAPv2) gridded emissions inventory (https://edgar.jrc.ec.europa.eu/htap_v2/, last access: 19 February 2020). The Lethbridge regional emissions are summed over a box with a bottom-left corner of (49.35∘ N, 113.31∘ W) and a top-right corner of (50.28∘ N, 111.10∘ W), which corresponds to an elevated emission region in the inventories.
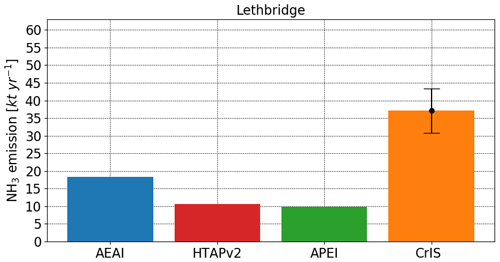
Figure 16The bars show the Lethbridge regional emissions as in the AEAI (blue), HTAPv2 (red), and APEI (green) inventories summed over a box with a bottom-left corner of (49.35∘, −113.31∘) and a top-right corner of (50.28∘, −111.10∘). The orange bar shows the CrIS estimated emissions using the free fitting algorithm and adjusted for diurnal and seasonal variability. The vertical error bar shows the uncertainty of the CrIS estimate that includes the uncertainty in the fit, the total columns, and uncertainties due to the meteorology.
In addition to the annual total, emissions are estimated using 5-year monthly observations and a moving window of 31 d in 1 d increments. This type of analysis can be used to help better constrain the seasonal and daily timing of emissions over this region. Figure 17 shows the results of the emission estimates, compared to the AEAI 2013 monthly emission inventory (blue). The orange lines and red dots show the results when applying the plume fitting algorithm to the CrIS monthly dataset and daily sets (31 d moving window), respectively. While some months might have enough information available in the mean total column fields, this is not true for each of the months as indicated by the relatively noisy results outside of the warm season. To improve the stability of the fitted results a lifetime of 2.65 h and a plume spread of 19 km as obtained from the 5-year analysis above were used here to estimate the CrIS-derived monthly and daily emissions in Fig. 17. This lifetime and plume spread distance were derived based on 5 years of CrIS data. This type of analysis can be used to help better capture the warm-season timing of emissions over this region. The general overall seasonal changes in the spring and summer are similar between the CrIS-derived emissions and the AEAI inventory with peak emissions over Lethbridge in May springtime, but CrIS is showing nearly double the amount compared with AEAI. In this example, CrIS is not showing the smaller secondary fall peak (September–October) that is seen in the AEAI inventory.
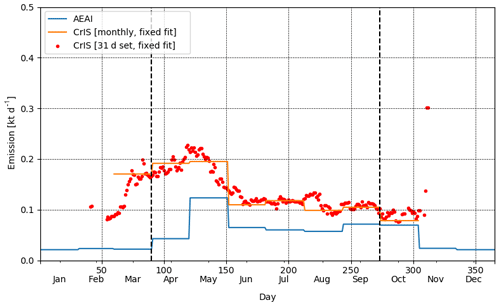
Figure 17Time series of monthly NH3 emissions over Lethbridge (Alberta, Canada). The monthly emissions following the Canadian AEAI 2013 inventory are shown in blue, which are summed over the Lethbridge region (a box with a bottom-left corner of (49.35∘, −113.31∘) and a top-right corner of (50.28∘, −111.10∘)). The orange line shows the CrIS monthly estimated emissions using a lifetime of 2.65 h and a plume spread of 19 km, both of which are derived from the 5-year data record. The red dots are the corresponding CrIS-derived emissions in daily increments that are derived using a 31 d interval (with 5 years, namely 2013–2017). The vertical dashed black lines indicate the beginning and end of the warm season over this region.
Satellite observations of lower tropospheric ammonia are a relatively new development with the initial proof of concept in the past decade (Beer et al., 2008); thus, there is a great potential for advancements in the retrievals and exploration of new applications. Presented here is an overview of CrIS' ammonia data product highlighting its current capabilities to observe lower tropospheric ammonia with sample applications for monitoring, model evaluation, dry deposition, and emission estimates. The CrIS daily observations demonstrate the influence of meteorology on the spatio-temporal variability of ammonia. These examples show the transport of ammonia concentrations from nearby agriculture sources as well as from fire emissions. Averaging these daily observations over longer time periods (e.g. monthly, seasonal, and annual) and gridding and oversampling (to yield Level 3 products) illustrate the spatio-temporal variability of ammonia at various timescales. These results demonstrate CrIS' ability to observe regional changes in ammonia concentrations due to agricultural practices, such as spring maximum values over agricultural regions when ammonia is released into the air from the fertilizing of crops. Also shown is the importance of episodic wildfire emissions in the more-wildfire-active months, especially in regions where there are few or no agriculture sources such as the northern latitudes in North America during July and August.
Initial comparisons of CrIS NH3 satellite observations with GEM-MACH air quality model simulation in summer 2016 show that in some regions there is general agreement on the spatial distribution of the anthropogenic hotspots, while other areas are markedly different and will need further investigation. For this summer period, the model tends to have higher peak values in the eastern US, whereas the satellite tends to have larger peak values in the western half of the US. As the CTM runs only have anthropogenic emission sources included, we can see that the impact of large summertime wildfires at higher latitudes on the 2-monthly mean concentration levels over large regions can be significant and can approach the values of agriculture hotspots at lower latitudes.
Expanding on the initial 2013 growing season results from Kharol et al. (2018), we show annual dry deposition rates of nitrogen from ammonia for 5 years from 2013 to 2017 over North America. CrIS satellite-derived values show the annual average and variability in the dry deposition of reactive nitrogen from ammonia over Canada and the US of and Tg N yr−1, respectively. When combining with OMI-derived NO2, the 2013 annual ratio shows NH3 accounting for ∼82 % and ∼55 % of the combined reactive-nitrogen dry deposition from these two species over Canada and the US, respectively. CrIS satellite observations are also used to derive agricultural emissions over the CAFO-dominated region of Lethbridge, Alberta, Canada, for a 5-year period (2013–2017). The satellite-derived annual emissions in the Lethbridge region are 37.1±6.3 kt yr−1, which is at least twice the value reported for emissions in current Canadian and global bottom-up emission inventories over this region. Furthermore, we demonstrated the potential of using a time series of the satellite-derived emissions to evaluate the seasonal temporal emissions profile used in bottom-up inventories over an agriculture hotspot.
Additional applications such as using the CrIS CFPR products for model inversions and data assimilation (e.g. Li et al., 2019) are currently being explored, which take advantage of the averaging kernels and error covariance matrix provided in the CrIS-retrieved product (e.g. observation operator) to provide top-down constraints on the ammonia emissions. Additionally, we will continue to refine and validate the CFPR algorithm and product. Some of these potential efforts include (i) accounting for cloud-free pixels that have no information (no ammonia signal in the spectra) in the CrIS composite (Level 3) products globally for the entire dataset, (ii) investigating retrievals over ocean and elevated concentration values over some deserts and high-elevation wintertime conditions (e.g. North American Rockies), (iii) investigating the potential enhancements to the a priori profiles and constraints used in the retrievals, and (iv) validating CrIS NH3 night-time observations against available ground-based observations.
Here are the quality flags specified for version 1.5. The quality flags become more conservative with increasing values; thus, they are applied as greater than or equal to the level you want to use.
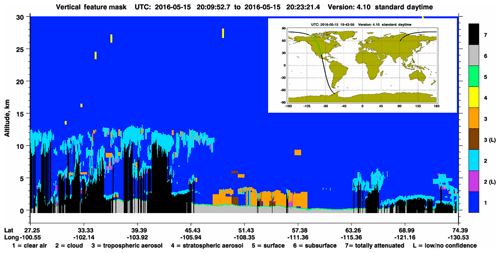
Figure B1CALIPSO plots obtained from: https://www-calipso.larc.nasa.gov/data/BROWSE/production/V4-10/2016-05-15/2016-05-15_19-42-56_V4.10_3_6.png (last access: 19 February 2020).
As a reference, this section contains total column maps that correspond to the mean surface ammonia for multi-year global, seasonal, and annual values over North America shown in the main part of the paper.
The AEAI inventory calculation (from agricultural sources) is a multi-stage process involving three sets of information. The inventory is built on very detailed census data on animals for each census district collected annually by Statistics Canada. The data on fertilizer use, including forms of nitrogen, are provided by the fertilizer industry on a provincial basis. Ammonia emissions are strongly influenced by farming practices such as manure handing systems or fertilizer application methods. These data were acquired by farm surveys, targeted to ammonia with an emphasis on timing of practices, across the main livestock sectors for 12 key Canadian ecoregions. There was a multisector survey in 2005 that targeted ammonia-related practices from feed quality, housing and storage facilities, land application practices, and grazing management. The important beef sector survey was updated in 2011 to capture large changes in practices. A new pig survey will be conducted this year. A separate survey for fertilizer practices relating to ammonia was conducted in 2006 by the polling company Ipsos.
Emission factors for the particular farm practices were obtained from scientific studies conducted in Canada and elsewhere. Some published models for emissions were used and where possible tested with Canadian data. The emission factors were adjusted for ambient temperatures relating to the practices, the regions, and the time of the practices. The manure application emissions were also adjusted for the probability of rainfall. The emission data were granulated to a 50 km×50 km grid by Environment and Climate Change Canada (ECCC), and a finer grid is being contemplated. Note that in some cases, notably where there are few operations, the data are averaged over larger areas with more operations to ensure confidentiality for the farms.
The CrIS NH3 is developed jointly at Environment and Climate Change Canada (ECCC) and Atmospheric and Environmental Research (AER) using the CFPR algorithm (Shephard and Cady-Pereira, 2015). The CrIS CFPR version 1.5 ammonia data created by Environment and Climate Change Canada are currently available upon request (mark.shephard@canada.ca) at: https://hpfx.collab.science.gc.ca/~mas001/satellite_ext/cris/snpp/nh3/v1_5/ (last access: 19 February 2020). The Python/Matlab code used to create any of the figures is available on request. We use the NASA-created OMI operational NO2 standard product (SP) version 3 available at: https://disc.gsfc.nasa.gov/datasets/OMNO2_V003/summary (last access: 19 February 2020).
MWS and KECP developed the CrIS CFPR Level 2 ammonia product. MWS, ED, JT, YGM, AK, and SKK processed the Level 2 product and developed the CrIS Level 3 gridded ammonia product. ED performed the emission calculations, and SK provided the dry deposition results. JZ, MM, VSJ, and QZ produced the GEM-MACH model results. SB provided the Canadian AEAI emissions and contributed to the analysis of the satellite-derived emission estimates. MWS, ED, KECP, SKK, CAM, CS, MA, CL, and DG contributed to the analysis of the CrIS satellite retrievals and applications.
The authors declare that they have no conflict of interest.
We would like to acknowledge the NOAA Comprehensive Large Array-data Stewardship System (CLASS; Liu et al., 2014), with special thanks to Axel Graumann (NOAA), for providing the CrIS Level 1 and Level 2 CrIS REDRO and NUCAPS input atmospheric state data. We thank Denis Tremblay (Science Data Processing, Inc.) for providing valuable insights on the performance and characteristics of the CrIS instrument, as well as Cristen Adams (EMSD, Government of Alberta) for detailed discussions on the fire observations. We are grateful to Leiming Zhang (ECCC) for his helpful discussions on dry deposition. We would like to thank Nick Krotkov (NASA) for his support with the OMI NO2 product.
Karen Cady-Pereira (AER) contribution to the research was supported by NASA (grant nos. NNH15CM65C, 80NSSC18K1652, and 80NSSC18K0689). Matthew Alvarado and Chantelle Lonsdale (AER) contributions to the research were supported by NOAA Climate Program Office (grant nos. NA13OAR4310060 and NA14OAR4310129) as well as NASA Applied Science (grant no. 80NSSC19K0190).
This paper was edited by Jianzhong Ma and reviewed by four anonymous referees.
Adams, C., McLinden, C. A., Shephard, M. W., Dickson, N., Dammers, E., Chen, J., Makar, P., Cady-Pereira, K. E., Tam, N., Kharol, S. K., Lamsal, L. N., and Krotkov, N. A.: Satellite-derived emissions of carbon monoxide, ammonia, and nitrogen dioxide from the 2016 Horse River wildfire in the Fort McMurray area, Atmos. Chem. Phys., 19, 2577–2599, https://doi.org/10.5194/acp-19-2577-2019, 2019.
AEMP: Air Emissions Modeling Platforms, available at: https://www.epa.gov/air-emissions-modeling/2011-version-6-air-emissions-modeling-platforms (last access: 19 February 2020), 2019.
APEI: Canada's Air Pollutant Emissions Inventory Report: 1990-2017, Environment and Climate Change Canada, Cat. No. En81-30-2017-eng.pdf, 2562–4903, available at: http://publications.gc.ca/collections/collection_2019/eccc/En81-30-2017-eng.pdf (last access: 19 February 2020), 2019.
Baek, B. and Seppanen, C.: Sparse Matrix Operator Kernel Emissions (SMOKE) Modeling System (Version SMOKE User's Documentation), https://doi.org/10.5281/zenodo.1421403, 2018.
Bash, J. O., Cooter, E. J., Dennis, R. L., Walker, J. T., and Pleim, J. E.: Evaluation of a regional air-quality model with bidirectional NH3 exchange coupled to an agroecosystem model, Biogeosciences, 10, 1635–1645, https://doi.org/10.5194/bg-10-1635-2013, 2013.
Bauer, S. E., Tsigaridis, K., and Miller, R.: Significant atmospheric aerosol pollution caused by world food cultivation, Geophys. Res. Lett., 43, 5394–5400, https://doi.org/10.1002/2016GL068354, 2016.
Beer, R., Shephard M. W., Kulawik, S. S., Clough, S. A., Eldering, A., Bowman, K. W., Sander, S. P., Fisher, B. M., Payne, V. H., Luo, M., Osterman, G. B., and Worden, J. R.: First satellite observations of lower tropospheric ammonia and methanol, Geophys. Res. Lett., 35, L09801, https://doi.org/10.1029/2008GL033642, 2008.
Bergstrom, A.-K. and Jansson, M.: Atmospheric nitrogen deposition has caused nitrogen enrichment and eutrophication of lakes in the northern hemisphere, Glob. Change Biol., 12, 635–643, https://doi.org/10.1111/j.1365-2486.2006.01129.x, 2006.
Bowman, W. D., Murgel, J., Blett, T., and Porter E.: Nitrogen critical loads for alpine vegetation and soils in Rocky Mountain National Park, J. Environ. Manage., 103, 165–171, https://doi.org/10.1016/j.jenvman.2012.03.002, 2012.
Chen, J., Anderson, K., Pavlovic, R., Moran, M. D., Englefield, P., Thompson, D. K., Munoz-Alpizar, R., and Landry, H.: The FireWork v2.0 air quality forecast system with biomass burning emissions from the Canadian Forest Fire Emissions Prediction System v2.03, Geosci. Model Dev., 12, 3283–3310, https://doi.org/10.5194/gmd-12-3283-2019, 2019.
Clarisse, L., Clerbaux, C., Dentener, F., Hurtmans, D., and Coheur, P.-F.: First global ammonia distributions from infrared satellite observations, Nat. Geosci., 2, 479–483, https://doi.org/10.1038/ngeo551, 2009.
Clarisse, L., Shephard, M. W., Dentener, F., Hurtmans, D., Cady-Pereira, K., Karagulian, F., Van Damme, M., Clerbaux, C., and Coheur, P.-F.: Satellite monitoring of ammonia: A case study of the San Joaquin Valley, J. Geophys. Res., 115, D13302, https://doi.org/10.1029/2009JD013291, 2010.
Crouse, D. L., Peters, P. A., van Donkelaar, A., Goldberg, M. S., Villeneuve, P. J., Brion, O., Khan, S., Atari, D. O., Jerrett, M., Pope, C. A., Brauer, M., Brook, J. R., Martin, R. V., Stieb, D., and Burnett, R. T.: Risk of Non accidental and Cardiovascular Mortality in Relation to Long-term Exposure to Low Concentrations of Fine Particulate Matter: A Canadian National-Level Cohort Study, Environ. Health Perspect., 120, 708–714, https://doi.org/10.1289/ehp.1104049, 2012.
Dammers, E., McLinden, C. A., Griffin, D., Shephard, M. W., Van Der Graaf, S., Lutsch, E., Schaap, M., Gainairu-Matz, Y., Fioletov, V., Van Damme, M., Whitburn, S., Clarisse, L., Cady-Pereira, K., Clerbaux, C., Coheur, P. F., and Erisman, J. W.: NH3 emissions from large point sources derived from CrIS and IASI satellite observations, Atmos. Chem. Phys., 19, 12261–12293, https://doi.org/10.5194/acp-19-12261-2019, 2019.
Denmead, O. T., Chen, D., Rowell, D., Loh, Z., Hill, J., Muir, S., Griffith, D. W., Naylor, T., Bai, M., Phillips, F., and McGinn, S.: Gaseous nitrogen emissions from Australian cattle feedlots, in: Nitrogen deposition, critical loads and biodiversity, Springer, Dordrecht, 23–29, 2014.
Divakarla, M., Barnet, C., Liu, X., Gu, D., Wilson, M., Kizer, S.,Xiong, X., Maddy, E., Ferraro, R., Knuteson, R., Hagan, D.,Ma, X.-L., Tan, C., Nalli, N., Reale, A., Mollner, A. K., Yang,W., Gambacorta, A., Feltz, M., Iturbide-Sanchez, F., Sun, B., and Goldberg, M.: The CrIMSS EDR algorithm: Characteriza-tion, optimization, and validation, J. Geophys. Res.-Atmos., 119, 4953–4977, https://doi.org/10.1002/2013JD020438, 2014.
Ellis, R. A., Jacob, D. J., Sulprizio, M. P., Zhang, L., Holmes, C. D., Schichtel, B. A., Blett, T., Porter, E., Pardo, L. H., and Lynch, J. A.: Present and future nitrogen deposition to national parks in the United States: Critical load exceedances, Atmos. Chem. Phys., 13, 9083–9095, https://doi.org/10.5194/acp-13-9083-2013, 2013.
Engardt, M., Simpson, D., Schwikowski, M., and Granat, L.: Deposition of sulphur and nitrogen in Europe 1900–2050, Model calculations and comparison to historical observations, Tellus B, 69, 1–20, 1328945, https://doi.org/10.1080/16000889.2017.1328945, 2017.
Erisman, J. W, Bleeker, A., Galloway, J., and Sutton, M. S.: Reduced nitrogen in ecology and the environment, Environ Pollut., 150, 140–149, 2007.
Fenn, M. E., Allen, E. B., Weiss, S. B., Jovan, S., Geiser, L. H., Tonnesen, G. S., Johnson, R. F., Rao, L. E., Gimeno, B. S., Yuan, F., Meixner, T., and Bytnerowicz, A.: Nitrogen critical loads and management alternatives for N-impacted ecosystems in California, J. Environ. Manage., 91, 2404–2423, https://doi.org/10.1016/j.jenvman.2010.07.034, 2010.
Fioletov, V. E., McLinden, C. A., Krotkov, N., Moran, M. D., and Yang, K.: Estimation of SO2 emissions using OMI retrievals, Geophys. Res. Lett., 38, L21811, https://doi.org/10.1029/2011GL049402, 2011.
Galloway, J. N., Aber, J. D., Erisman, J. W., Seitzinger, S. P., Howarth, R. W., Cowling, E. B., and Cosby, B. J.: The nitrogen cascade, Bioscience, 53, 341–356, 2003.
Gong, W., Makar, P. A., Zhang, J., Milbrandt, J., Gravel, S., Hayden, K.L., Macdonald, A. M., and Leaitch, W. R.: Modelling aerosol-cloud-meteorology interaction: A case study with a fully coupled air quality model (GEM-MACH), Atmos. Environ., 115, 695–715, https://doi.org/10.1016/j.atmosenv.2015.05.062, 2015.
Goulding, K. W. T.: Soil acidification and the importance of liming agricultural soils with particular reference to the United Kingdom, Soil Use Management, 32, 390–399, 2016.
Kharol, S. K., Martin, R. V., Philip, S., Boys, B., Lamsal, L. N., Jerrett, M., Brauer, M., Crouse, D. L., McLinden, C., and Burnett, R. T.: Assessment of the magnitude and recent trends in satellite-derived ground-level nitrogen dioxide over North America, Atmos. Environ., 118, 236–245, 2015.
Kharol, S. K., Shephard, M. W., McLinden, C. A., Zhang, L., Sioris, C. E., O'Brien, J. M., Vet, R., Cady-Pereira, K. E., Hare, E., Siemons, J., and Krotkov, N. A.: Dry deposition of reactive nitrogen from satellite observations of ammonia and nitrogen dioxide over North America, Geophys. Res. Lett., 45, 1157–1166, https://doi.org/10.1002/2017GL075832, 2018.
Kharol, S. K., Shephard, M.W., Dammers, E., O'Brien, J., Hare, E., Cady-Pereira, K. E., Sioris, C. E., Puchalski, M., Dabek, E., Wichink Kruit, R., Volten, H., and Swart, D.: Cross-track Infrared Sounder (CrIS) Satellite Comparisons with Surface Ammonia Observations, in preparation, Atmos. Meas. Tech., 2020.
Krotkov, N. A., McLinden, C. A., Li, C., Lamsal, L. N., Celarier, E. A., Marchenko, S. V., Swartz, W. H., Bucsela, E. J., Joiner, J., Duncan, B. N., Boersma, K. F., Veefkind, J. P., Levelt, P. F., Fioletov, V. E., Dickerson, R. R., He, H., Lu, Z., and Streets, D. G.: Aura OMI observations of regional SO2 and NO2 pollution changes from 2005 to 2015, Atmos. Chem. Phys., 16, 4605–4629, https://doi.org/10.5194/acp-16-4605-2016, 2016.
Lamsal, L. N., Duncan, B. N., Yoshida, Y., Krotkov, N. A., Pickering, K. E., Streets, D. G., and Lu, Z.: U.S. NO2 trends (2005–2013): EPA air quality system (AQS) data versus improved observations from the Ozone Monitoring Instrument (OMI), Atmos. Environ., 110, 130–143, 2015.
Li, C., Martin, R. V., Shephard, M. W., Cady-Pereira, K., Cooper, M. J., Kaiser, J., Lee, C. J., Zhang, L., and Henze, D. K., Assessing the iterative finite difference mass balance and 4D-Var methods to derive ammonia emissions over North America using synthetic observations, J. Geophys. Res.-Atmos., 124, 4222–4236, https://doi.org/10.1029/2018JD030183, 2019.
Liu, M., Huang, X., Song, Y., Tang, J., Cao, J., Zhang, X., Zhang, Q., Wang, S., Xu, T., Kang, L., Cai, X., Zhang, H., Yang, F., Wang, H., Yu, Lau, A. K. H., He, L., Huang, X., Duan, L., and Zhu, T.: Ammonia emission control in China would mitigate haze pollution and nitrogen deposition, but worsen acid rain, P. Natl. Acad. Sci. USA, 116, 7760–7765, https://doi.org/10.1073/pnas.1814880116, 2019.
Liu, Q., Wolf, W., Reale, T., Sharma, A., and, NOAA JPSS Program Office.: NOAA Unique Combined Atmospheric Processing System (NUCAPS) environmental data record (EDR) products, NOAA National Centers for environmental information, https://doi.org/10.7289/V52F7KG5, 2014.
Lutsch, E., Dammers, E., Conway, S., and Strong, K.: Long-range transport of NH3, CO, HCN, and C2H6 from the 2014 Canadian Wildfires, Geophys. Res. Lett., 43, 8286–8297, https://doi.org/10.1002/2016GL070114, 2016.
Lutsch, E., Strong, K., Jones, D. B. A., Ortega, I., Hannigan, J. W., Dammers, E., Shephard, M. W., Morris, E., Murphy, K., Evans, M., Parrington, M., Whitburn, S., Van Damme, M., Clarisse, L., Coheur, P.-F., Clerbaux, C., Croft, B., Martin, R. V., Wentworth, G. R., and Fisher, J. A.: Unprecedented atmospheric ammonia concentrations detected in the high Arctic from the 2017 Canadian wildfires, J. Geophys. Res.-Atmos., 124, 8178–8202, https://doi.org/10.1029/2019JD030419, 2019.
Makar, P. A., Moran, M. D., Zheng, Q., Cousineau, S., Sassi, M., Duhamel, A., Besner, M., Davignon, D., Crevier, L.-P., and Bouchet, V. S.: Modelling the impacts of ammonia emissions reductions on North American air quality, Atmos. Chem. Phys., 9, 7183–7212, https://doi.org/10.5194/acp-9-7183-2009, 2009.
Makar, P. A., Gong,W., Milbrandt, J., Hogrefe, C., Zhang, Y., Curci, G., Zabkar, R., Im, U., Balzarini, A., Baro, R., Bianconi, R., Cheung, P., Forkel, R., Gravel, S., Hirtl, H., Honzak, L., Hou, A., Jimenz-Guerrero, P., Langer, M., Moran, M. D., Pabla, B., Perez, J. L., Pirovano, G., San Jose, R., Tuccella, P., Werhahn, J., Zhang, J., and Galmarini, S.: Feedbacks between air pollution and weather, Part 1: Effects on chemistry, Atmos. Environ., 115, 442–469, https://doi.org/10.1016/j.atmosenv.2014.12.003, 2015a.
Makar, P. A., Gong, W., Hogrefe, C., Zhang, Y., Curci, G., Zabkar, R., Milbrandt, J., Im, U., Balzarini, A., Baro, R., Bianconi, R., Cheung, P., Forkel, R., Gravel, S., Hirtl, H., Honzak, L., Hou, A., Jimenz-Guerrero, P., Langer, M., Moran, M. D., Pabla, B., Perez, J. L., Pirovano, G., San Jose, R., Tuccella, P.,Werhahn, J., Zhang, J., and Galmarini, S.: Feedbacks between air pollution and weather, Part 2: Effects on chemistry, Atmos. Environ., 115, 499–526, https://doi.org/10.1016/j.atmosenv.2014.10.021, 2015b.
Massad, R.-S., Nemitz, E., and Sutton, M. A.: Review and parameterisation of bi-directional ammonia exchange between vegetation and the atmosphere, Atmos. Chem. Phys., 10, 10359–10386, https://doi.org/10.5194/acp-10-10359-2010, 2010.
Munoz-Alpizar, R., Pavlovic, R., Moran, M. D., Chen, J., Gravel, S., Henderson, S. B., Ménard, S., Racine, J., Duhamel, A., Gilbert, S., Beaulieu, P.-A., Landry, H., Davignon, D., Cousineau, S., and Bouchet, V.: Multi-year (2013–2016) PM2.5 wildfire pollution exposure over North America as determined from operational air quality forecasts, Atmosphere, 8, 179, 1–24, https://doi.org/10.3390/atmos8090179, 2017.
NASA: OMNO2: OMI/Aura Nitrogen Dioxide (NO2) Total and Tropospheric Column 1-orbit L2 Swath 13×24 km V003, available at: https://disc.gsfc.nasa.gov/datasets/OMNO2_V003/summary, last access: 19 February 2020.
NWT_FSRR: 2014 NWT Fire Season Review Report, tabled document 249-17(5) tabled on 1 June 2015, Produced by Canada's Environment and Natural Resources, available at: https://www.assembly.gov.nt.ca/sites/default/files/td_249-175.pdf (last access: 19 February 2020), 2015.
Paulot, F., Jacob, D. J., and Henze, D. K.: Sources and processes contributing to nitrogen deposition: An adjoint model analysis applied to biodiversity hotspots worldwide, Environ. Sci. Technol., 47, 3226–3233, 2013.
Pendlebury, D., Gravel, S., Moran, M. D., and Lupu, A..: The impact of chemical lateral boundary conditions in a regional air quality forecast model on surface ozone predictions during stratospheric intrusions, Atmos. Environ., 174, 148–170, https://doi.org/10.1016/j.atmosenv.2017.10.052, 2018.
Pinder, R. W., Adams, P. J., and Pandis, S. N.: Ammonia Emission Controls as a Cost-Effective Strategy for Reducing Atmospheric Particulate Matter in the Eastern United States, Environ. Sci. Technol., 41, 380–386, https://doi.org/10.1021/es060379a, 2007.
Pleim, J. E., Ran, L., Appel, W., Shephard, M. W., and Cady-Pereira, K. E.: New bidirectional ammonia flux model from managed agricultural soils in an air quality model coupled with an agricultural model, J. Adv. Model. Earth Sy., 11, 2934–2957, https://doi.org/10.1029/2019MS001728, 2019.
Pommier, M., McLinden, C. A., and Deeter, M.: Relative changes in CO emissions over megacities based on observations from space, Geophys. Res. Lett., 40, 3766–3771, https://doi.org/10.1002/grl.50704, 2013.
Pope, C. A.: Epidemiology of fine particulate air pollution and human health: Biologic mechanisms and who's at risk?, Environ. Health Perspect., 108, 713–723, 2000.
Pope, C. A., Burnett, R. T., Thun, M. J., Calle, E. E., Krewski, D., Ito, K., and Thurston, G. D.: Lung cancer, cardiopulmonary mortality, and long-term exposure to fine particulate air pollution, J. Am. Med. Assoc., 287, 1132–1141, 2002.
Pope, C. A., Ezzati, M., and Dockery, D. W.: Fine-particulate air pollution and life expectancy in the United States, N. Engl. J. Med., 360, 376–386, 2009.
Reiss, R., Anderson, E. L., Cross, C. E., Hidy, G., Hoel, D., McClellan, R., and Moolgavkar, S.: Evidence of health impacts of sulfate- and nitrate-containing particles in ambient air, Inhal. Toxicol., 19, 419–449, https://doi.org/10.1080/08958370601174941, 2007.
Rodgers, C. D.: Inverse methods for atmospheric Sounding: Theory and Practice, World Sci., Hackensack, NJ, 238 pp., 2000.
Schwartz, J., Laden, F., and Zanobetti, A.: The concentration-response relation between PM2.5 and daily deaths, Environ. Health Perspect., 110, 1025–1029, 2002.
Seinfeld, J. H. and Pandis, S. N.: Atmospheric Chemistry and Physics, John Wiley, Hoboken, NJ, 1152 pp., 1988.
Shephard, M. W. and Cady-Pereira, K. E.: Cross-track Infrared Sounder (CrIS) Satellite Observations of Tropospheric Ammonia, Atmos. Meas. Tech., 8, 1323–1336, https://doi.org/10.5194/amt-8-1323-2015, 2015.
Shephard, M. W., Cady-Pereira, K. E., Luo, M., Henze, D. K., Pinder, R. W., Walker, J. T., Rinsland, C. P., Bash, J. O., Zhu, L., Payne, V. H., and Clarisse, L.: TES ammonia retrieval strategy and global observations of the spatial and seasonal variability of ammonia, Atmos. Chem. Phys., 11, 10743–10763, https://doi.org/10.5194/acp-11-10743-2011, 2011.
Sheppard, L. J., Leith, I. D., Mizunuma, T., Cape, J. N., Crossley, A., Leeson, S., Sutton, M. A., van Dijk, N., and Fowler D.: Dry deposition of ammonia gas drives species change faster than wet deposition of ammonium ions: evidence from a long-term field manipulation, Glob. Change Biol., 17, 3589–3607, https://doi.org/10.1111/j.1365-2486.2011.02478.x, 2011.
Sheppard, S. C. and Bittman, S.: Ammonia, in: Environmental sustainability of Canadian agriculture: Agri-environmental indicator report series, edited by: Clearwater, R. L., Martin, T., and Hoppe, T., Report #4, Agriculture and Agri-Food Canada, chap. 16, 180–194, 2016.
Someya, Y., Imasu, R., Shiomi, K., and Saitoh, N.: Atmospheric ammonia retrieval from the TANSO-FTS/GOSAT thermal infrared sounder, Atmos. Meas. Tech., 13, 309–321, https://doi.org/10.5194/amt-13-309-2020, 2020.
Urbanski, S.: Wildland fire emissions, carbon, and climate: Emission factors, Forest Ecol. Manag., 317, 51–60, https://doi.org/10.1016/J.FORECO.2013.05.045, 2014.
Van Damme, M., Wichink Kruit, R. J., Schaap, M., Clarisse, L., Clerbaux, C., Coheur, P.-F., Dammers, E., Dolman, A. J., and Erisman J. W.: Evaluating 4 years of atmospheric ammonia (NH3) over Europe using IASI satellite observations and LOTOS-EUROS model results, J. Geophys. Res.-Atmos., 119, 9549–9566, https://doi.org/10.1002/2014JD021911, 2014.
Van Damme, M., Clarisse, L., Whitburn, S., Hadji-Lazaro, J., Hurtmans, D., Clerbaux, C., and Coheur, P.-F.: Industrial and agricultural ammonia point sources exposed, Nature, 564, 99–103, 2018.
van der Graaf, S. C., Dammers, E., Schaap, M., and Erisman, J. W.: Technical note: How are NH3 dry deposition estimates affected by combining the LOTOS-EUROS model with IASI-NH3 satellite observations?, Atmos. Chem. Phys., 18, 13173–13196, https://doi.org/10.5194/acp-18-13173-2018, 2018.
Van Haarlem, R. P., Desjardins, R. L., Gao, Z., Flesch, T. K., and Li, X.: Methane and ammonia emissions from a beef feedlot in western Canada for a twelve-day period in the fall, Can. J. Anim. Sci., 88, 641–649, 2008.
Warner, J. X., Dickerson, R. R., Wei, Z., Strow, L. L., Wang, Y., and Liang, Q.: Increased atmospheric ammonia over the world's major agricultural areas detected from space, Geophys. Res. Lett., 44, 2875–2884, https://doi.org/10.1002/2016GL072305, 2017.
Whaley, C. H., Makar, P. A. , Shephard, M. W., Zhang, L., Zheng, J., Zheng, Q., Akingunola, A., Wentworth, G. R., Murphy, J. G., Kharol, S. K., and Cady-Pereira, K. E.: Contributions of natural and anthropogenic sources to ambient ammonia in the Athabasca Oil Sands and north-western Canada, Atmos. Chem. Phys., 18, 2011–2034, https://doi.org/10.5194/acp-18-2011-2018, 2018.
Winker, D. M., Pelon, J., and McCormick, M. P.: The CALIPSO mission: Spaceborne lidar for observation of aerosols and clouds, Proc. SPIE, 4893, 1–11, https://doi.org/10.1117/12.466539, 2003.
Zavyalov, V., Esplin, M., Scott, D., Esplin, B., Bingham, G., Hoffman, E., Lietzke C., Predina J., Frain R., Suwinski L., Han Y., Major C., Graham B., and Phillips, L.: Noise performance of the CrIS instrument, J. Geophys. Res.-Atmos., 118, 13108–13120, https://doi.org/10.1002/2013JD020457, 2013.
Zhang, J., Moran, M. D., Zheng, Q., Makar, P. A., Baratzadeh, P., Marson, G., Liu, P., and Li, S.-M.: Emissions preparation and analysis for multiscale air quality modeling over the Athabasca Oil Sands Region of Alberta, Canada, Atmos. Chem. Phys., 18, 10459–10481, https://doi.org/10.5194/acp-18-10459-2018, 2018.
- Abstract
- Introduction
- CrIS satellite retrievals
- Application examples
- Conclusions
- Appendix A: Quality flags of version 1.5
- Appendix B: CALIPSO
- Appendix C: Total column figures
- Appendix D: Brief description of Canada's Air Pollutant Emission Inventory (APEI)
- Code and data availability
- Author contributions
- Competing interests
- Acknowledgements
- Financial support
- Review statement
- References
- Abstract
- Introduction
- CrIS satellite retrievals
- Application examples
- Conclusions
- Appendix A: Quality flags of version 1.5
- Appendix B: CALIPSO
- Appendix C: Total column figures
- Appendix D: Brief description of Canada's Air Pollutant Emission Inventory (APEI)
- Code and data availability
- Author contributions
- Competing interests
- Acknowledgements
- Financial support
- Review statement
- References