the Creative Commons Attribution 4.0 License.
the Creative Commons Attribution 4.0 License.
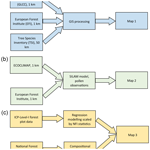
Incorporation of pollen data in source maps is vital for pollen dispersion models
Alexander Kurganskiy
Carsten Ambelas Skjøth
Alexander Baklanov
Mikhail Sofiev
Annika Saarto
Elena Severova
Sergei Smyshlyaev
Eigil Kaas
Information about distribution of pollen sources, i.e. their presence and abundance in a specific region, is important, especially when atmospheric transport models are applied to forecast pollen concentrations. The goal of this study is to evaluate three pollen source maps using an atmospheric transport model and study the effect on the model results by combining these source maps with pollen data. Here we evaluate three maps for the birch taxon: (1) a map derived by combining a land cover data and forest inventory, (2) a map obtained from land cover data and calibrated using model simulations and pollen observations, and (3) a statistical map resulting from analysis of forest inventory and forest plot data. The maps were introduced to the Enviro-HIRLAM (Environment – High Resolution Limited Area Model) as input data to simulate birch pollen concentrations over Europe for the birch pollen season 2006. A total of 18 model runs were performed using each of the selected maps in turn with and without calibration with observed pollen data from 2006. The model results were compared with the pollen observation data at 12 measurement sites located in Finland, Denmark, and Russia. We show that calibration of the maps using pollen observations significantly improved the model performance for all three maps. The findings also indicate the large sensitivity of the model results to the source maps and agree well with other studies on birch showing that pollen or hybrid-based source maps provide the best model performance. This study highlights the importance of including pollen data in the production of source maps for pollen dispersion modelling and for exposure studies.
- Article
(9256 KB) - Full-text XML
- BibTeX
- EndNote
Aeroallergens are a specific type of atmospheric aerosols causing allergic reactions among people suffering from allergic rhinitis, which is often connected with asthma (Bachert et al., 2004). The number of allergic patients sensitive to pollen is assessed to be 20 % of the European population (WHO, 2003). There are substantial variations in sensitization levels towards specific aeroallergens in Europe (Heinzerling et al., 2009). In northern Europe, pollen from the Poaceae (grasses) and Betulaceae (e.g. hazel, alder, and birch) families show the highest sensitization among patients (Heinzerling et al., 2009). Birch pollen is generally the most abundant of those four pollen types (Skjøth et al., 2013b), often with large interannual fluctuations in the overall pollen integral (e.g. Piotrowska and Kaszewski, 2011). In particular, for northern Europe the pollen season of 2006 was characterized by abundant birch pollen concentrations that resulted in an increase in patient calls for medical assistance during the spring period (Sofiev et al., 2011). Such episodes can potentially be described and forecast using atmospheric dispersion models (Sofiev et al., 2006), helping sensitized individuals to minimize their symptoms. Several European countries, such as Denmark, have seen an increase in sensitized individuals over the past few decades (e.g. Rasmussen, 2002; Linneberg et al., 2007). Generally, skin prick testing (SPT) is performed to identify sensitization of patients to common pollen allergens (e.g. Heinzerling et al., 2009). Haahtela et al. (2015) showed that the percentage of the population with positive SPT was about 35 % and 20 % in Finnish and Russian Karelia, respectively. These facts demonstrate the importance of pollen studies using atmospheric dispersion models applied for research and operational purposes (Klein et al., 2012).
A variety of pollen types are considered to be biological components by several atmospheric dispersion models such as SILAM, COSMO-ART, WRF/CMAQ, Enviro-HIRLAM, and others (see e.g. Zink et al., 2012, 2013; Zhang et al., 2014; Sofiev et al., 2015a; Baklanov et al., 2017; Sofiev, 2017; Zink et al., 2017). Information about distribution of pollen sources, i.e. their presence and abundance in a region of study, plays a crucial role in aerobiological modelling and forecasting using atmospheric dispersion models (e.g. Zink et al., 2017). In other words, it is one of the key input data used to simulate or forecast pollen emission, its atmospheric transport, and depositions in these models. For this reason, recent studies (e.g. Pauling et al., 2012; Bonini et al., 2018) are devoted to developing pollen source inventories and maps. Two main approaches aiming to build/construct the pollen source inventories are suggested in the literature: bottom-up and top-down (Skjøth et al., 2013b). The bottom-up approach is based on statistical data of pollen source distribution combined with land cover data obtained from remote sensing (Skjøth et al., 2008a). The limitation of this approach is that statistical data are either unavailable or cover too large of a territory (e.g. the whole country) in some regions. The top-down approach uses pollen observations as a starting point to estimate the abundance of different pollen taxa and it can be combined with land use data and/or models for more detailed assessment of pollen sources on a regional scale. Poor geographical and temporal coverage of the pollen observation data can limit this approach in some regions.
Skjøth et al. (2008a) used the bottom-up approach to create the Tree Species Inventory (TSI) for 39 species (including birch, oak, alder, etc.) redistributed to a 50 km grid to be used in atmospheric dispersion models. The top-down approach has been used to produce ragweed pollen inventories for the Pannonian Basin (Skjøth et al., 2010), France (Thibaudon et al., 2014), Austria (Karrer et al., 2015), and Italy (Bonini et al., 2018). The inventories are based on a combination of pollen observation data, knowledge on ragweed ecology, and detailed land cover information. The last (i.e. Bonini et al., 2018) also includes influence of the Ophraella communa beetle on the plants. Prank et al. (2013) as well as Hamaoui-Laguel et al. (2015) have both used combined occupancy and climatic habitat quality maps for ragweed from an ecological model. The map covering Europe has been introduced to the atmospheric models as input data, run for several years and calibrated using pollen observation data. A birch pollen source map has been derived by Pauling et al. (2012). The methodology includes using a forest inventory combined with local and global land use datasets. The obtained map has been calibrated by observational pollen data and it covers Europe. An urban-scale grass pollen source inventory has been obtained by Skjøth et al. (2013a) using GIS and remote sensing data. A high-resolution (1 km) pollen source inventory based on 12 taxa of trees, grass, and weeds has been produced by McInnes et al. (2017) for the UK to be mainly used in exposure studies as well as other possible applications. Many of these different pollen source maps show substantial variation, exemplified by the birch maps over the UK produced by McInnes et al. (2017) and Pauling et al. (2012) or the European scale by comparing the maps by Pauling et al. (2012) with those by Siljamo et al. (2013). This suggests substantial disagreement or uncertainty in the production of the pollen source maps – the key input data for pollen dispersion models. An additional problem is the large variability of the pollen production per plant in each specific year. Several approaches have been proposed to address this issue (e.g. Masaka and Maguchi, 2001; Ranta et al., 2005; Ritenberga et al., 2018).
Zink et al. (2017) applied an atmospheric dispersion model to compare different ragweed pollen source maps using the COSMO-ART model. The results show that the pollen source map resulting from combining land cover and pollen observation data provides the best model performance. The advantage of including a dispersion model is that, in principle, such models take into account atmospheric transport and its effect on pollen concentrations during a specific year (e.g. Prank et al., 2013). A recent study showed high potential of extended four-dimensional variational data assimilation as a rigorous procedure for obtaining the source maps' calibrations (Sofiev, 2019).
The literature review presented in this section shows there are multiple pollen source maps produced for different pollen types and regions and using different approaches and data. Despite this, they are rarely applied to and intercompared using atmospheric dispersion models. This study aims to evaluate three pollen source maps for the birch taxon using an atmospheric dispersion model and study the effect on the model results by combining these source maps with pollen data. The maps and model are described in the Methods section below.
2.1 Birch pollen source maps
Three birch pollen source maps have been chosen in this study: (1) a map derived by combining land cover data and forest inventory, (2) a map obtained from land cover data and calibrated using model simulations and pollen observations, and (3) a statistical map resulting from analysis of forest inventory and forest plot data (see Fig. A1 in the Appendix section). The maps have been preprocessed for two modelling domains: P15 and T15. P15 covers part of Spain in the south to part of Scandinavia in the north, with Denmark near the centre of the model domain (Fig. 1a). T15 covers Finland, parts of Sweden, northwest Russia, the Baltic states, and Belarus (Fig. 1b).
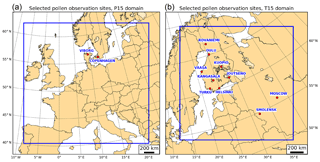
Figure 1Location of the selected birch pollen observation sites with boundaries of the modelling domains: P15 (a) and T15 (b).
Map 1 (see Fig. 2a, b) has been obtained by combining three datasets: Global Land Cover Characteristics (GLCC, https://doi.org/10.5066/F7GB230D) version 2 (Belward et al., 1999), European Forest Institute tree cover (EFI; Päivinen et al., 2001), and Tree Species Inventory (TSI; Skjøth et al. (2008a)). GLCC has global coverage and includes geographical distribution of land cover, soil type, and other various properties with 1 km horizontal resolution. EFI covers Europe, has 1 km horizontal resolution, and contains spatial distribution of forest with subclasses, i.e. broadleaved forest and coniferous forest. TSI is presented by 39 types of tree species (birch, oak, alder, etc.) and covers Europe with 50 km horizontal resolution and it is based on national forest inventories and national statistics. The following layers have been extracted from these databases and combined to derive the birch pollen source: land mask, forest, vegetation, urban territories (from GLCC), broadleaved forest (from EFI), and birch forest fraction in broadleaved forest (from TSI). For details of the combining procedure see Kurganskiy et al. (2015) and Kurganskiy (2017).
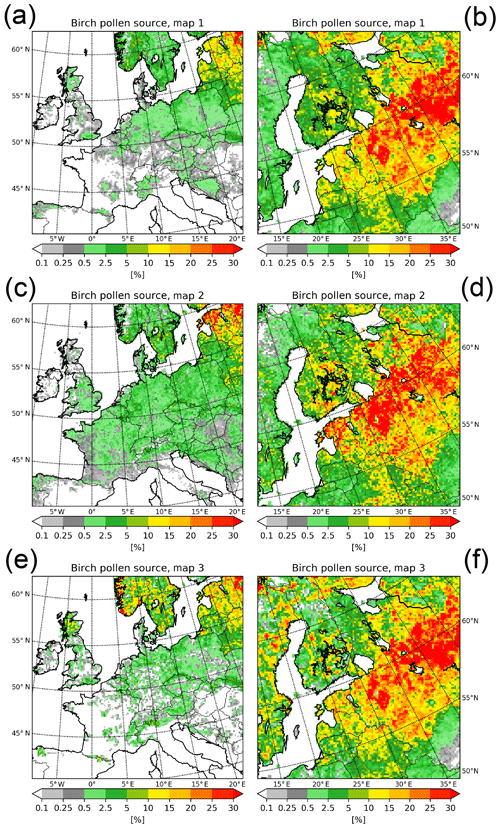
Figure 2Birch pollen source maps processed for two different modelling domains P15 (a, c, e) and T15 (b, d, f) with 15 km grid resolution. Panels (a, b) correspond to Map 1, (c, d) to Map 2, and (e, f) to Map 3. The maps are shown as a percentage – the percentage cover of birch in each 15 km×15 km grid square.
Map 2 (see Fig. 2c, d) is a product of two datasets and a calibration using model simulations. The two datasets are EFI tree cover and ECOCLIMAP (Masson et al., 2003). ECOCLIMAP is a global dataset with 1 km resolution and it includes 215 ecosystems. The model simulations have been performed with the System for Integrated modeLing of Atmospheric coMposition (SILAM, http://silam.fmi.fi, last access: 23 January 2020, Sofiev et al., 2015b) and pollen observation data for several years to ensure average unbiased representation of the Seasonal Pollen Integral (SPIn) with the SILAM model, where SPIn is the integral of pollen concentrations over time (Galán et al., 2017). The calibrated map is calculated for a 1 km grid covering the whole of Europe with nearby regions. Details of the map calibration procedure are described by Prank et al. (2013) using ragweed, and its application to birch in the SILAM model can be found in Sofiev (2017).
Map 3 (see Fig. 2e, f) is the result of combining ICP-Level-I forest plot data (http://www.icp-forests.org, last access: 23 January 2020) and National Forest Inventory (NFI) plot data as well as the NFI statistics (Brus et al., 2012). Two techniques were used to derive the map: compositional kriging in areas with NFI plot data and regression modelling outside of the areas. The National Forest Inventory statistics were used to scale the regression model results. The map has 1 km horizontal resolution and covers most of Europe. However, information about birch pollen sources in Russia is missing in Map 3. Therefore, the Russian sources were extracted from Map 1 and combined with Map 3 to fill the gap.
The maps were introduced to Enviro-HIRLAM (Environment – High Resolution Limited Area Model) as input data to simulate birch pollen concentrations for the modelling domains.
2.2 Model description
Enviro-HIRLAM is an online coupled meteorology–chemistry model allowing us to take into account spatial–temporal evolution of atmospheric chemical (Korsholm, 2009; Baklanov et al., 2017) and biological (Kurganskiy, 2017) aerosols simulated inside the meteorological HIRLAM model (Undén et al., 2002). The current version of the Enviro-HIRLAM model is based on HIRLAM v7.2 (https://hirlam.org). The detailed model description of all components can be found in Korsholm (2009), Kurganskiy (2017), and Baklanov et al. (2017) while this article is focused on description of the Enviro-HIRLAM features relevant to pollen. In order to simulate birch pollen concentrations, Enviro-HIRLAM requires external information/data on the spatial pollen source distribution and phenology, i.e. start of birch flowering, pollen production, characteristics of pollen release (emissions) within the given study region, and where the spatial pollen source distribution is represented by the maps described above in Sect. 2.1. Atmospheric transport and dispersion as well as dry and wet depositions are calculated within Enviro-HIRLAM at each time step. In numerical models, the start of birch pollen flowering is often determined by applying simple crop growth models (e.g. McMaster and Wilhelm, 1997); here the growing degree day (GDD) method is implemented in Enviro-HIRLAM. GDD is based on accumulation of 2 m daily mean air temperatures above a given threshold (cut-off temperature) as this is the WMO definition of GDD. It is assumed here that birch flowering starts as soon as the accumulated temperature (GDD) reaches a certain value depending on geographical location. These temperature sum threshold maps have been produced by Sofiev et al. (2013) and have been implemented and used as input data for the GDD parameterization in Enviro-HIRLAM. The data were obtained by the fitting procedure applied using the observed leaf unfolding dates (Siljamo et al., 2008) and modelled ones by the GDD parameterization and ECMWF’s ERA-40 reanalysis air temperature data (Uppala et al., 2005) utilized as input for GDD. It was shown in Kurganskiy (2017) that the temperature sum threshold maps are applicable for use to simulate the birch flowering start in Enviro-HIRLAM, even though another model (ERA-40) had been originally used to obtain the maps. GDD is an integral part of Enviro-HIRLAM, and GDD calculations are performed for the same dates as the model is run over. Birch pollen emission is meteorology-dependent and uses parameterizations proposed by Sofiev et al. (2013). It is based on dimensionless functions correcting the seasonal pollen productivity, here chosen to be 3.7×108 as a default value obtained for SILAM (Sofiev et al., 2013). The main meteorological parameters affecting the emissions are air temperature, relative humidity, wind speed, and accumulated precipitation. The emitted birch pollen particles are subject to atmospheric transport, which is parameterized in the same way as for aerosols using the locally mass-conserving semi-Lagrangian (LMCSL) scheme (Kaas, 2008; Sørensen et al., 2013). The scheme provides proper mass conservation, shape preservation, and multi-tracer efficiency required from a numerical point of view. Dry deposition of birch pollen particles is determined by gravitational settling (Seinfeld and Pandis, 2006). The gravitational settling parameterization is based on calculation of settling velocity according to Stokes law and taking into account density of birch pollen particles (800 kg m−3) and an estimated size of 22 µm (e.g. Mäkelä, 1996) with near spherical shape (Sofiev et al., 2006). Wet deposition distinguishes between in-cloud (Stier et al., 2005) and below-cloud (Baklanov and Sørensen, 2001) scavenging, i.e. removal of pollen particles from the atmosphere by precipitation. Both scavenging types are parameterized through the scavenging coefficients used for aerosol particles with r≥10 µm.
2.3 Model configuration
The Enviro-HIRLAM model is used to calculate pollen concentrations in two different modelling domains shown in Fig. 1. Both domains have about 15 km horizontal resolution and 40 hybrid vertical levels. Input meteorological initial and boundary conditions were taken from the ECMWF IFS model (Persson, 2011) with 15 km resolution and 6 h intervals. The birch pollen emission module has the same settings as described in Sofiev et al. (2013) and Siljamo (2013). The Enviro-HIRLAM model was run from 1 March until 15 June 2006 with the ECMWF input data covering the same period. The two model domains have been simulated with three types of calculations: (1) a standard calculation, (2) a calculation that includes a correction for 2 m air temperature (T2 m) bias between assimilated and simulated T2 m (termed COR), and (3) a calculation that includes both the correction for temperature and a grid-based scaling factor for pollen emissions (termed SCF). These calculations were performed using each of the selected pollen source maps, hence nine model calculations for each of the two domains. The model results from model simulations 2 and 3 are presented in the Results section. The correction for T2 m bias is done in two steps. Firstly, the model output from the simulations of type 1 is used to calculate the biases (differences between simulated and assimilated T2 m) for each assimilation window (i.e. 6 h). Secondly, the calculated biases are introduced in the model simulations of type 2 (COR) where the simulated T2 m is corrected at each model time step using the average bias between the two nearest/closest assimilation windows. Further details of this procedure can be found in Kurganskiy (2017). The scaling factor for pollen emissions is based on the ratio between simulated and observed seasonal pollen integral (SPIn) (see next section) from the simulations of type 2 (COR). This provides 15 point values that have been interpolated to the entire model domain, thereby providing a grid-based scaling factor for each of the tree source maps. The interpolation procedure takes into account the weighted distance between each observation point and grid cell with a constant radius of influence (1 km in this study). The procedure also ensures the scaling factors are equal to around 1 in the areas located far away from the observation points. This is especially visible in P15 domain, e.g. in France (Fig. 3a, c, e). Further details of the interpolation procedure can be found in Kurganskiy (2017). The grid-based scaling factor has then been extracted for each model domain, thus providing six grid-based scaling factors (Fig. 3) applied for the SCF simulations for all maps. The principal difference between the runs is that the COR runs include a constant value for birch pollen productivity (3.7×108 ) in each grid cell, whereas the productivity is calibrated using the grid-based scaling factor (obtained from the COR runs and shown in Fig. 3) in the SCF runs. The model results were compared with the pollen observation data at 12 measurement sites located in Finland, Denmark, and Russia (see Fig. 1) for all model runs.
2.4 Used observations and statistical evaluation of model results
Daily concentrations of birch pollen were used from 12 sites (Fig. 1a, b), and furthermore annual SPIn values were obtained from three additional sites in Lithuania, published by Veriankaite (2010), in order to strengthen the calculation of the grid-based scaling factor (Sect. 2.3). The observational data were obtained using 7 d volumetric samplers (Hirst, 1952) and analysed according to standard methods in aerobiology (Galán et al., 2014). Station names and coordinates are presented in the Appendix section along with statistical summaries for each station (see Tables A1–A4), while overall results are presented in the Results section. The statistical evaluation includes standard and threshold-based statistical approaches, e.g. previously used by Siljamo et al. (2013) and Sofiev et al. (2015a) and calculated in this paper for each map (1, 2, 3) and run (COR, SCF) type. The start and end of the birch pollen season are calculated using the retrospective method according to Nilsson and Persson (1981), here defined as the dates when the total sums of birch pollen concentrations (SPIn) reach 5 % and 95 %, correspondingly. The criteria are chosen to provide filtration of LRT (long-range-transport) episodes which have a significant impact in northern Europe, especially in the beginning of the birch pollen season (Ranta et al., 2006; Skjøth et al., 2007; Mahura et al., 2007; Veriankaite, 2010). The standard statistical approach includes calculations of correlation coefficient (R), coefficient of determination (R2), mean bias (MB), and root-mean-square error (RMSE), and the evaluation primarily concerns correlation and RMSE. Two additional metrics, normalized mean bias factor (NMBF) and normalized mean absolute error factor (NMAEF), are also calculated according to Yu et al. (2006) since RMSE is highly sensitive to outliers. The R2 values are shown on the time series plots for each station, map, and run type as well as on the global scatter plots. The standard statistical metrics have been calculated for the “main” pollen season (30 April–15 June 2006) due to non-ergodicity (i.e. non-similarity in space and time) and non-stationarity of the birch pollen time series (Sofiev et al., 2015a). The threshold-based statistical approach (Siljamo et al., 2013) comprises calculations of the model accuracy (MA), probability of detection (POD), false alarm ratio (FAR), probability of false detection (POFD), and odds ratio (OR) using the threshold value for birch pollen concentrations Cth=50 pollen m−3. The threshold value Cth is chosen since most of the population with pollen allergies might start suffering from allergic reactions when daily mean birch pollen concentration C≥Cth in the air (Jantunen et al., 2012). Additionally, the modelled and observed birch pollen concentrations have been sorted into classes in order to estimate the number of cases for low (1–10 pollen m−3), moderate (10–100 pollen m−3), high (100–1000 pollen m−3), and very high (>1000 pollen m−3) birch pollen concentrations. The sorted data plotted with corresponding standard errors are shown in the Results section. Hit rates (HRs) are calculated for the classes, and their results are shown in tables in the Results section. The results of the statistical significance test (chi-squared test) performed for the HR values are also discussed. For summary of the thresholds, classes, and metrics used to calculate the threshold-based statistics, the reader is referred to Tables A5–A6 in the Appendix section.
The simulated time series of birch pollen concentrations have been compared to the selected pollen observation sites by determining the start and end of the birch pollen season and applying the standard statistics as well as threshold-based statistics described in the Methods section. The modelled and observed time series are also shown in Figs. 4–5 for COR and SCF runs, respectively. The start of the birch pollen season is simulated quite well for both runs and all maps. Station-wise the bias does not exceed 2–3 d at all stations and indicates 2 d too early flowering on average for all maps. Simulation of the last flowering day turns out to be the most challenging for Enviro-HIRLAM. The bias shows too late flowering at almost all stations. On average, it varies from 15 d (Map 3) to 9 d (Map 2) according to the COR run. Rescaling of the pollen productivity (SCF run) reduced the bias by 2–3 d for each map. The largest biases for the last flowering day are found at five Finnish sites (Helsinki, Joutseno, Kangasala, Vaasa, and Kuopio) and two Russian sites (Moscow and Smolensk), and they are related to overestimation of the observed birch pollen concentrations at the end of the season for both COR and SCF runs (see Figs. 4 and 5). It can be addressed to large variations in the year-to-year pollen production in that region which, in turn, introduce a large bias in the model simulations.
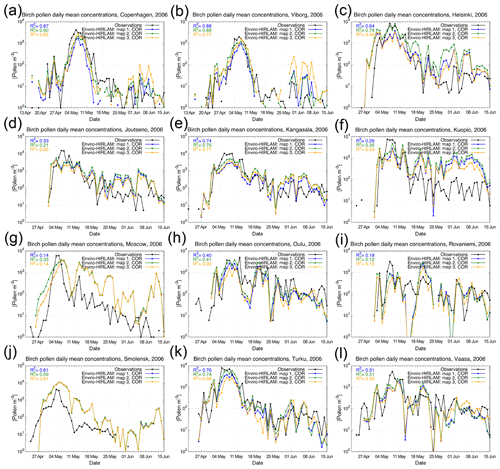
Figure 4Time series of the modelled and observed birch pollen daily mean concentrations for the selected pollen observation sites. The modelled concentrations correspond to each simulated map according to the COR run. A base-10 log scale is used for the y axis.
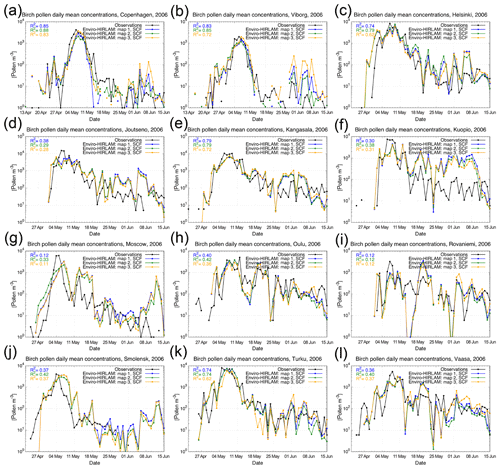
Figure 5Time series of the modelled and observed birch pollen daily mean concentrations for the selected pollen observation sites. The modelled concentrations correspond to each simulated map according to the SCF run. A base-10 log scale is used for the y axis.
Generally, the time series of the modelled birch pollen concentrations (Figs. 4 and 5) show good agreement with observations for all maps at most of the selected stations. The COR run indicates that the model behaves similarly with respect to describing variance in observations (see R2 values in Fig. 4), except for some stations (e.g. Moscow, Helsinki) where Map 2 provides higher R2 values in comparison with Map 1 and Map 3. The SCF run did not affect the R2 values significantly, but it reduced the mean bias (MB, NMBF) at the stations (see Tables A1–A4). The highest R2 values are found at two Danish sites (Copenhagen and Viborg) where the model explains more than 70 % of variance found in observations for all considered maps and model runs. It should be noted here that the R2 values decrease slightly at the Danish sites according to the SCF runs. The reason for this is that the simulated concentrations in the SCF runs are increased almost every single day, which has the effect of reducing the underestimation found in the central season (e.g. Fig. 5a) and increasing LRT, most likely originating from sources found in Germany and Poland at the beginning of the season and from Scandinavia at the end of the season. For more detailed statistical summaries at the stations, the reader is referred to tables in the Appendix section.
The global standard statistical metrics for all stations are presented in Table 1. According to the COR run the model performs slightly better with Map 2. It is supported by a higher correlation coefficient (R = 0.59) and lower mean bias (MB =69.08 pollen m−3, NMBF =0.09) in comparison with two other maps. One can assume that this result should be expected since pollen observations are already included in the methodology used to obtain Map 2. Rescaling of the pollen productivity (SCF run) provides improvement of the Enviro-HIRLAM model performance by increasing the R values (R=0.72 for Maps 1 and 2 and R=0.68 for Map 3) and decreasing the MB, NMBF, and NMAEF values. However, RMSE is large (i.e. >1000 pollen m−3) for all maps and both model runs. It can be explained by sensitivity of RMSE to large differences between modelled and observed values. The large bias for the last flowering day simulation contributes significantly to the RMSE values.
Table 1Global “standard” statistical metrics obtained by comparing the modelled () and observed () daily mean birch pollen concentrations. The metrics were calculated using all available station data for both simulated domains, over the 30 April–15 June 2006 period. , , MB, and RMSE are given (pollen m−3). The results were statistically significant for all maps (p value <0.01 according to a Student's t test).
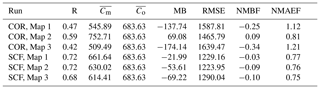
The global scatter plots (Fig. 6) also indicate improvements in the modelled–observed concentration agreement expressed as an increase in the R2 for the SCF model setup. As seen, the coefficients of determination do not exceed 0.34 (Map 2) for all maps in the COR run. The SCF run provides R2 values around 0.5 for all maps. This means that the model can explain about 50 % of variance found in observations after the rescaling using any of the maps.
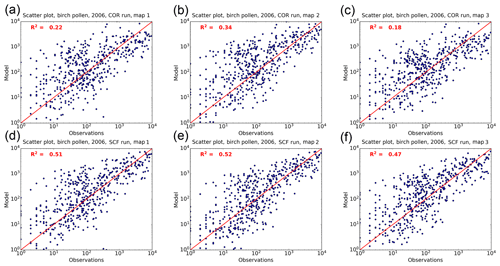
Figure 6Scatter plots for birch pollen concentrations when comparing COR (a–c) and SCF (d–f) model runs with observations for Map 1 (a, d), Map 2 (b, e), and Map 3 (c, f).
The threshold-based statistical metrics calculated for each map and model run are shown in Table 2. The results indicate similar behaviour of the model when comparing performance of the maps. The values of the statistical metrics are slightly different between the maps, but the difference does not exceed 7 %. The model accuracy (MA) – the fraction of correct simulations – is higher than 70 % for all model simulations. The POD values show that the Enviro-HIRLAM model simulates the high concentrations (>50 pollen m−3) correctly in more than 70 % of cases. The fraction of incorrect simulations for the high concentrations (FAR values) is less than 30 % for the model setups. The POFD values show that the fraction of the cases when concentrations are simulated as high but observed as low (i.e. <50 pollen m−3) is more than 30 % for the COR runs, and it decreases below 30 % after rescaling of the pollen productivity (SCF run). As expected the odds ratio (OD) values increase in the SCF run for all maps. The modelled and observed birch pollen concentrations have been grouped in order to estimate the number of cases for low, moderate, high, and very high birch pollen concentrations (see Fig. 7). As can be seen, the Enviro-HIRLAM model simulates the low and very high pollen concentrations quite well with a difference less than ±5 % between the modelled and observed number of cases for both COR (Fig. 7a) and SCF (Fig. 7b) runs and all maps. The largest differences between the modelled and observed number of cases are found for the moderate and high pollen concentrations, but the differences are within the error bars (see Fig. 7). Map 2 underestimates the number of cases for the moderate concentrations by 10 %, whereas Map 3 overestimates the high ones by more than 10 % (COR run). The SCF run slightly improves the results for the moderate and high number of cases.
Table 2The threshold-based statistical metrics obtained by comparing model simulations with observed daily mean concentrations. MA, POD, FAR, and POFD are given as a percentage.
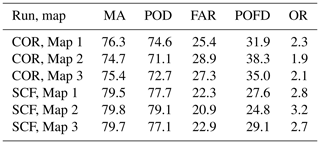
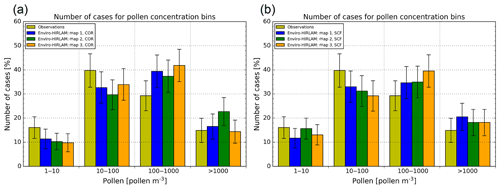
Figure 7Histogram of the observed and modelled birch pollen concentrations sorted into low (1–10 pollen m−3), moderate (10–100) pollen m−3, high (100–1000 pollen m−3), and very high (>1000 pollen m−3) classes. Panel (a) corresponds to COR and (b) to the SCF model simulations.
The HR calculations (see Tables 3–4) show that simulations of the moderate and high birch pollen concentrations are the most challenging for all model setups. The SCF run (Table 4) indicates improvements of the model score for the moderate and high birch pollen concentrations. However, the hit rates still do not exceed 50 % for all maps. This feature is also visible on the scatter plots in Fig. 6. The HR values for the low pollen concentrations are quite high and exceed 90 % for COR and 80 % for SCF runs. The very high pollen concentrations are simulated slightly better by using Map 3 with HR = 70 %. However, according to the chi-square test the differences between the HR values for different maps are not statistically significant for both COR and SCF runs.
Table 3Hit rates of the Enviro-HIRLAM birch pollen simulations (COR run) for the classes
zero or low, moderate, high, and very high. The classes are based on daily mean pollen concentrations (pollen m−3).
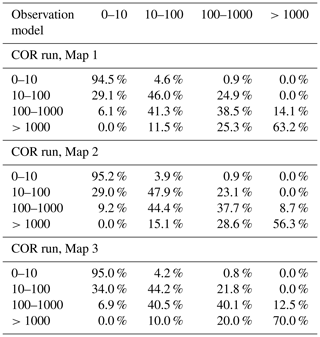
Analysis of the start of birch pollen season showed good model performance for all maps with the bias not exceeding 2 d on average. Such error is acceptable by state-of-the-art atmospheric dispersion models (e.g. Sofiev et al., 2015a). The analysis did not reveal significant dependency of the start of the birch pollen season on the underlying pollen source map and/or pollen productivity. This could be explained by the fact that the start of the birch pollen season is calculated using meteorological parameters (i.e. temperate sum thresholds) employed in GDD parameterization in the model. However, long-distance transport of birch pollen has often been observed in that region (e.g. Hjelmroos, 1992; Mahura et al., 2007), which can systematically affect the start of the pollen season (Skjøth et al., 2007). It could therefore be assumed that changing the source term in remote regions such as Poland could affect the start of the calculated pollen season in Denmark and Sweden. This phenomenon was not observed, which may be explained by the fact that the scaling factor for Poland was near unity for all three maps, while the scaling factor for Copenhagen and the close surroundings was substantially higher. This observation therefore complements the suggestion from other studies that long-distance transport of pollen is an episodic phenomenon (e.g. Smith et al., 2008; Fernández-Rodríguez et al., 2014) and that the overall contribution to locally observed birch pollen concentration is due to sources found within the region (Sofiev, 2017). Add to this that urban areas have previously been identified as areas that may enhance pollen production of flowering plants (Ziska et al., 2003). This may be particularly relevant for Copenhagen as a substantial fraction of the birch pollen observed is expected to originate from a source found in the city itself (Skjøth et al., 2008b). The last flowering day simulation was the most challenging for Enviro-HIRLAM. Map 2 showed the lowest bias estimated as 6 d (delay of end of flowering) on average for the stations according to the SCF runs. However, such bias is a known feature for state-of-the-art atmospheric models (e.g. Sofiev et al., 2015a). Introducing more pollen observation stations and performing more iterations for rescaling of pollen emissions (i.e. repeating SCF runs several times) would strengthen the current approach, and it could potentially improve the modelling of the last flowering day. Station-wise the Finnish and Russian sites had bias ≥ 2 weeks in all model runs. One of the possible reasons for this is that the large pollen emission area found in Russia, north of Moscow and Smolensk, is overestimated for this particular year. The source is found in all three source maps and the overestimation is further supported by the fact that the model simulates large amounts of birch pollen concentration very late in the season at Moscow and Smolensk (Fig. 4g and j), most likely originating from more northerly regions. This area will occasionally also affect the Finnish stations as it is well known that this region receives LRT from Russia (e.g. Sofiev et al., 2006). The study therefore complements a multi-model ensemble that demonstrated generally good results for Europe and also highlighted that large variations in the year-to-year pollen production in that region can introduce a large bias in the model simulations (Sofiev et al., 2015a). Large annual variations in the birch pollen integral are well known for a number of European countries (Latałowa et al., 2002; Dahl et al., 2013; Piotrowska and Kaszewski, 2011), as these annual fluctuations are generally not accounted for in the atmospheric models (e.g. Ritenberga et al., 2018).
The standard statistical metrics indicated a slightly better performance of the Enviro-HIRLAM with Map 2 on average for the stations according to the COR run. However, even for this map, the model explained less than 35 % of variance in observations. It is therefore plausible to attribute the remaining part of the disagreement to small-scale patterns of the birch habitation, which are neither captured by existing calibration methods nor satisfactorily reproduced by the land cover datasets. In this study, a scaling of the pollen productivity improved the model results significantly for all source terms and provided near-identical results for Map 1 and Map 2. The small-scale pattern in birch habitation will be difficult to capture by the land cover datasets, which will have the effect that a small but diffuse emission source found in many locations is not present, causing the model to underestimate medium concentration levels. Conversely, the large emission source found in parts of Russia will cause the model to overestimate periods when long-distance transport is present. This effect of over- and underestimation will vary from year to year, depending on pollen productivity, which is known to vary from year to year and between regions (Ranta et al., 2005). This suggests that accurate exposure calculations that use dispersion models should preferably use data fusion that combines a detailed inventory-based source term (e.g. Skjøth et al., 2008a; Pekkarinen et al., 2009; Sofiev, 2017) along with observed pollen concentrations covering the period of interest. Other possible sources of error (e.g. timing of pollen release, quality of meteorological parameters, simulation of atmospheric transport and removal processes) could also be taken into account. However, their effect is less important in comparison with the quality of emission (source) maps – the main uncertainty in air pollution and pollen dispersion modelling.
The threshold-based statistics calculated relative to one threshold value (Cth=50 pollen m−3) showed a good model agreement with observations independently of the map used. The threshold-based analysis was also carried out for low (1–10 pollen m−3), moderate (10–100 pollen m−3), high (100–1000 pollen m−3), and very high (>1000 pollen m−3) birch pollen concentrations. According to the analysis, simulations of the moderate and high birch pollen concentrations appeared to be the most challenging for Enviro-HIRLAM for all maps. The hit rates were less than 50 % for all model setups. This reveals a need for improvement of the rescaling of birch pollen productivity by introducing more observational points, in particular in areas sparse with data or regions with high emissions such as parts of Russia, and/or performing more iterations. The differences of the HR values appeared to be not significant during inter-comparison of the maps. This study concerning birch pollen complements other studies on ragweed (Prank et al., 2013; Hamaoui-Laguel et al., 2015), which also demonstrated the need for recalibration of the source term. However, it has since been shown by Zink et al. (2017) that source terms combining pollen data from several years with detailed land cover data can outperform other approaches minimizing the need for local calibrations. This approach is also limited by the fact that only a few regions have a sufficiently high number of pollen observations (Zink et al., 2017). Furthermore, abrupt annual changes in the underlying pollen integral can be caused by changes in the pollen emission (Bonini et al., 2015, 2018).
The insufficient density of the observational network also became the methodological limitation of the current study: we have used the same data from the same stations and for the same years for both calibration and evaluation. However, we separated them frequency-wise: calibration uses the annual or multi-annual average whereas the evaluation primarily concerned correlation and RMSE. It should be noted that an independent evaluation that does not use pollen data is shown by analysis of the COR runs in the study. Another approach could be to carry out a cross-validation procedure using the so-called leave-one-out procedure as this tests the sensitivity of individual data points. However, such a sensitivity study requires 15 additional model simulations for each map and each modelling domain, hence 90 simulations. The increased computational cost makes this exercise unfeasible, suggesting that other approaches need to be developed.
The aim of the study was to evaluate three birch pollen source maps using the atmospheric dispersion model Enviro-HIRLAM. The evaluation has been performed for 12 pollen observation sites located in Denmark, Finland, and Russia. The modelled and observed time series of birch pollen concentrations have been analysed for the start and end of flowering as well as by calculating the standard and threshold-based statistical metrics for two sets of the Enviro-HIRLAM model runs: without and with calibration using pollen observations.
The analysis did not reveal significant dependency of the start–end of the birch pollen season on the underlying pollen source map. The statistical analysis showed a good model agreement with the observed birch pollen concentrations in the region studied. The model can explain up to 50 % of variance found in observations after the calibration with all maps. It was shown that the remaining part of the disagreement should be attributed to small-scale patterns of the birch habitation, which are neither captured by existing calibration methods nor satisfactorily reproduced by the land cover datasets. The analysis also revealed that the insufficient density of the observational network was the methodological limitation of the study.
Generally, it was shown that calibration of the maps using pollen observations covering the investigation year significantly improved the model performance for all three maps. The findings also indicate the large sensitivity of the model results to the source maps and agree well with other studies on birch showing that pollen or hybrid-based source maps provide the best model performance. This study highlights the importance of including pollen data in the production of source maps for pollen dispersion modelling and for exposure studies.
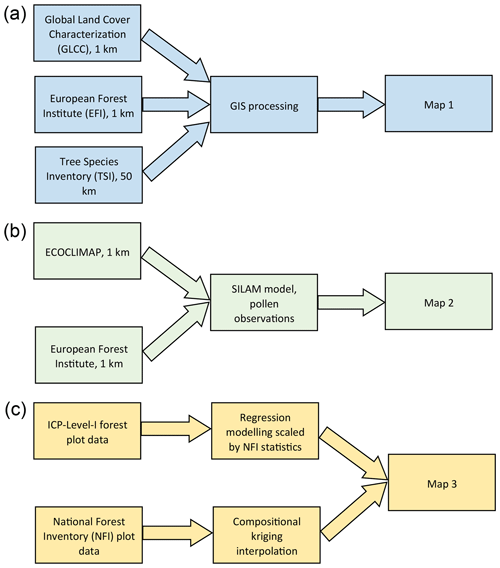
Figure A1Flowcharts outlining the data and methods used to obtain Map 1 (a), Map 2 (b), and Map 3 (c).
Table A1Birch pollen statistical metrics for the selected observation sites: COR run. , , MB, and RMSE are in pollen per cubic metre; p value <0.05.
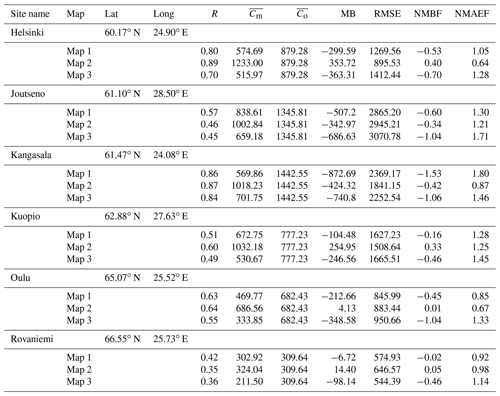
Table A2Birch pollen statistical metrics for the selected observation sites: COR run. , , MB, and RMSE are in pollen per cubic metre; p value <0.05.
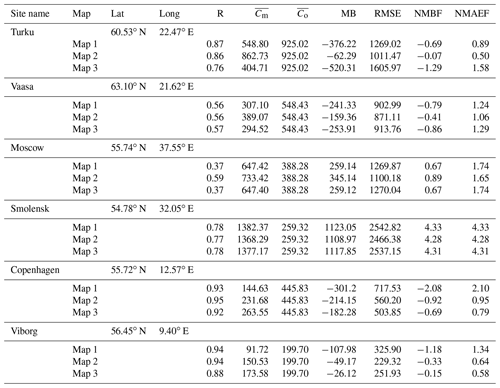
Table A3Birch pollen statistical metrics for the selected observation sites: SCF run. , , MB, and RMSE are in pollen per cubic metre; p value <0.05.
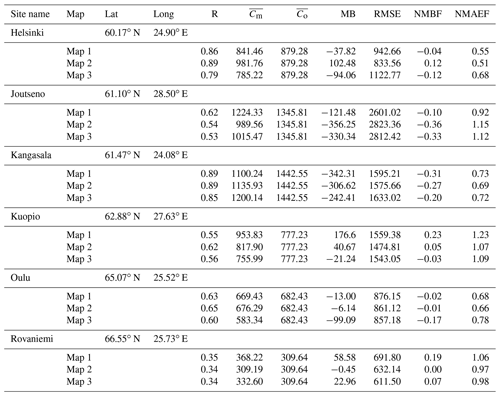
Table A4Birch pollen statistical metrics for the selected observation sites: SCF run. , , MB, and RMSE are in pollen per cubic metre; p value <0.05.
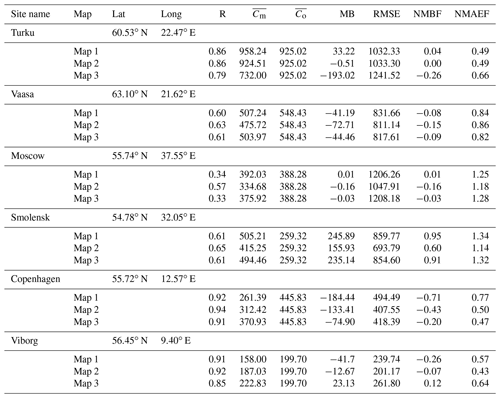
Table A5Thresholds and classes used to calculate the threshold-based statistical metrics for 12 selected pollen observational sites.
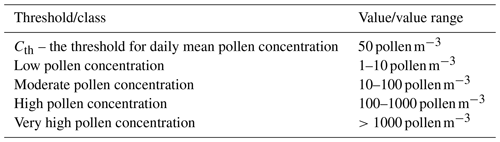
Table A6Definitions of the threshold-based statistical metrics used to compare the modelled and observed daily mean birch pollen concentrations. See Siljamo et al. (2013) for more details.
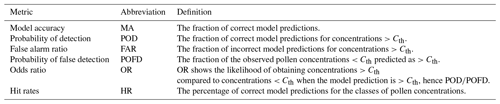
The model code and data are available from the authors upon request. The pollen observational data are available by contacting the data owners, i.e. AS, ES, and the Danish Asthma Allergy.
AK contributed to the overall developments of Enviro-HIRLAM for birch pollen, set up the model, performed the model simulations and analysis, and created graphical outputs and the initial paper draft; CAS participated in the analysis and preparation of the initial paper draft and contributed to writing the paper; EK, AB, and SS supervised AK, provided research advice and infrastructure, and contributed to the paper writing. MS participated in the experiment planning and execution and contributed to the paper writing; AS and ES provided the pollen observation data and contributed to the paper writing.
The authors declare that they have no conflict of interest.
The authors are thankful to Suleiman Mostamandy (RSHU/KAUST), Brain Højen-Sørensen (NBI UC/FCOO), and Roman Nuterman (NBI UC) for useful advice on Enviro-HIRLAM code development; Alexander Mahura (DMI/UH) and Alix Rasmussen (DMI) for fruitful discussions of the birch pollen modelling issues; the European Forest Institute (EFI) for providing broadleaved forest data; Adomas Mazeikis for useful advices on GIS analysis; the Danish Asthma Allergy Association for birch pollen observation data; and RSHU for providing HPC facilities. The SILAM pollen module was developed with Finnish Academy projects APTA (no. 266314) and PS4A (no. 318194). Support of the Copernicus CAMS-50 service is kindly acknowledged.
The research has been supported by the Scholarship of the President of the Russian Federation for Students and PhD Students Training Abroad, Eu-MetChem – COST Action (grant no. ES1004). The work was partly supported by the Russian Science Foundation (grant no. 19-17-00198). Enviro-HIRLAM model adaptation for the Russian conditions was supported by the Russian Ministry of High Education and Science state task. It has also been supported by the grant “GNSS Zenith Tropospheric Delay: supervision” (grant no. 95-7843).
This paper was edited by Yafang Cheng and reviewed by Rachel McInnes and two anonymous referees.
Bachert, C., Vignola, A. M., Gevaert, P., Leynaert, B., Van Cauwenberge, P., and Bousquet, J.: Allergic rhinitis, rhinosinusitis, and asthma: one airway disease, Immunol. Allergy Clin., 24, 19–43, 2004. a
Baklanov, A. and Sørensen, J.: Parameterisation of radionuclide deposition in atmospheric long-range transport modelling, Phys. Chem. Earth Pt. B, 26, 787–799, https://doi.org/10.1016/S1464-1909(01)00087-9, 2001. a
Baklanov, A., Smith Korsholm, U., Nuterman, R., Mahura, A., Nielsen, K. P., Sass, B. H., Rasmussen, A., Zakey, A., Kaas, E., Kurganskiy, A., Sørensen, B., and González-Aparicio, I.: Enviro-HIRLAM online integrated meteorology–chemistry modelling system: strategy, methodology, developments and applications (v7.2), Geosci. Model Dev., 10, 2971–2999, https://doi.org/10.5194/gmd-10-2971-2017, 2017. a, b, c
Belward, A., Estes, J., and Kline, K.: The IGBP-DIS 1-Km Land-Cover Data Set DISCover: A Project Overview, Photogramm. Eng. Rem. S., 65, 1013–1020, 1999. a
Bonini, M., Šikoparija, B., Prentović, M., Cislaghi, G., Colombo, P., Testoni, C., Grewling, L., Lommen, S. T. E., Müller-Schärer, H., and Smith, M.: Is the recent decrease in airborne Ambrosia pollen in the Milan area due to the accidental introduction of the ragweed leaf beetle Ophraella communa?, Aerobiologia, 31, 499–513, 2015. a
Bonini, M., Šikoparija, B., Skjøth, C. A., Cislaghi, G., Colombo, P., Testoni, C., A.I.A.-R.I.M.A.®, POLLnet, and Smith, M.: Ambrosia pollen source inventory for Italy: a multi-purpose tool to assess the impact of the ragweed leaf beetle (Ophraella communa LeSage) on populations of its host plant, Int. J. Biometeorol., 62, 597–608, https://doi.org/10.1007/s00484-017-1469-z, 2018. a, b, c, d
Brus, D. J., Hengeveld, G. M., Walvoort, D. J. J., Goedhart, P. W., Heidema, A. H., Nabuurs, G. J., and Gunia, K.: Statistical mapping of tree species over Europe, Eur. J. For. Res., 131, 145–157, https://doi.org/10.1007/s10342-011-0513-5, 2012. a
Dahl, Å., Galán, C., Hajkova, L., Pauling, A., Sikoparija, B., Smith, M., and Vokou, D.: The Onset, Course and Intensity of the Pollen Season, 29–70, Springer Netherlands, Dordrecht, 2013. a
Fernández-Rodríguez, S., Skjøth, C. A., Tormo-Molina, R., Brandao, R., Caeiro, E., Silva-Palacios, I., Gonzalo-Garijo, Á., and Smith, M.: Identification of potential sources of airborne Olea pollen in the Southwest Iberian Peninsula, Int. J. Biometeorol., 58, 337–348, 2014. a
Galán, C., Smith, M., Thibaudon, M., Frenguelli, G., Oteros, J., Gehrig, R., Berger, U., Clot, B., Brandao, R., and EAS QC Working Group: Pollen monitoring: minimum requirements and reproducibility of analysis, Aerobiologia, 30, 385–395, https://doi.org/10.1007/s10453-014-9335-5, 2014. a
Galán, C., Ariatti, A., Bonini, M., Clot, B., Crouzy, B., Dahl, A., Fernandez-González, D., Frenguelli, G., Gehrig, R., Isard, S., Levetin, E., Li, D. W., Mandrioli, P., Rogers, C. A., Thibaudon, M., Sauliene, I., Skjoth, C., Smith, M., and Sofiev, M.: Recommended terminology for aerobiological studies, Aerobiologia, 33, 293–295, https://doi.org/10.1007/s10453-017-9496-0, 2017. a
Haahtela, T., Laatikainen, T., Alenius, H., Auvinen, P., Fyhrquist, N., Hanski, I., Hertzen, L., Jousilahti, P., Kosunen, T. U., Markelova, O., Mäkelä, M. J., Pantelejev, V., Uhanov, M., Zilber, E., and Vartiainen, E.: Hunt for the origin of allergy – comparing the Finnish and Russian Karelia, Clinical & Experimental Allergy, 45, 891–901, https://doi.org/10.1111/cea.12527, 2015. a
Hamaoui-Laguel, L., Vautard, R., Liu, L., Solmon, F., Viovy, N., Khvorostyanov, D., Essl, F., Chuine, I., Colette, A., Semenov, M. A., Schaffhauser, A., Storkey, J., Thibaudon, M., and Epstein, M. M.: Effects of climate change and seed dispersal on airborne ragweed pollen loads in Europe, Nat. Clim. Change, 5, 766–771, 2015. a, b
Heinzerling, L. M., Burbach, G. J., Edenharter, G., Bachert, C., Bindslev-Jensen, C., Bonini, S., Bousquet, J., Bousquet-Rouanet, L., Bousquet, P. J., Bresciani, M., Bruno, A., Burney, P., Canonica, G. W., Darsow, U., Demoly, P., Durham, S., Fokkens, W. J., Giavi, S., Gjomarkaj, M., Gramiccioni, C., Haahtela, T., Kowalski, M. L., Magyar, P., Muraközi, G., Orosz, M., Papadopoulos, N. G., Röhnelt, C., Stingl, G., Todo-Bom, A., von Mutius, E., Wiesner, A., Wöhrl, S., and Zuberbier, T.: GA2LEN skin test study I: GA2LEN harmonization of skin prick testing: novel sensitization patterns for inhalant allergens in Europe, Allergy, 64, 1498–1506, 2009. a, b, c
Hirst, J. M.: An automatic volumetric spore trap, Ann. Appl. Biol., 39, 257–265, https://doi.org/10.1111/j.1744-7348.1952.tb00904.x, 1952. a
Hjelmroos, M.: Long-distance transport ofBetula pollen grains and allergic symptoms, Aerobiologia, 8, 231–236, 1992. a
Jantunen, J., Saarinen, K., and Rantio-Lehtimäki, A.: Allergy symptoms in relation to alder and birch pollen concentrations in Finland, Aerobiologia, 28, 169–176, https://doi.org/10.1007/s10453-011-9221-3, 2012. a
Kaas, E.: A simple and efficient locally mass conserving semi-Lagrangian transport scheme., Tellus, 60, 305–320, 2008. a
Karrer, G., Skjøth, C., Šikoparija, B., Smith, M., Berger, U., and Essl, F.: Ragweed (Ambrosia) pollen source inventory for Austria, Sci. Total Environ., 523, 120–128, https://doi.org/10.1016/j.scitotenv.2015.03.108, 2015. a
Klein, T., Kukkonen, J., Dahl, Å., Bossioli, E., Baklanov, A., Vik, A. F., Agnew, P., Karatzas, K. D., and Sofiev, M.: Interactions of physical, chemical, and biological weather calling for an integrated approach to assessment, forecasting, and communication of air quality, Ambio, 41, 851–864, https://doi.org/10.1007/s13280-012-0288-z, 2012. a
Korsholm, U. S.: Integrated modeling of aerosol indirect effects, DMI scientific report 1, DMI, available at: https://www.dmi.dk/fileadmin/Rapporter/SR/sr09-01.pdf (last access: 23 January 2020), 2009. a, b
Kurganskiy, A.: Integrated modelling of physical, chemical and biological weather, PhD thesis, Niels Bohr Institute, University of Copenhagen, Juliane Maries Vej 30, 2100, Copenhagen, Denmark, 2017. a, b, c, d, e
Kurganskiy, A., Mahura, A., Nuterman, R., Saarto, A., Rasmussen, A., Baklanov, A., Smyshlyaev, S., and Kaas, E.: Enviro-HIRLAM birch pollen modeling for Northern Europe, in: Report series in Aerosol Science, edited by: Kulmala, M., Zilitinkevich, S., Lappalainen, H., Kyrö, E.-M., and Kontkanen, J., vol. 163, 229–234, 2015. a
Latałowa, M., Miętus, M., and Uruska, A.: Seasonal variations in the atmospheric Betula pollen count in Gdańsk (southern Baltic coast) in relation to meteorological parameters, Aerobiologia, 18, 33–43, 2002. a
Linneberg, A., Gislum, M., Johansen, N., Husemoen, L., and Jørgensen, T.: Temporal trends of aeroallergen sensitization over twenty-five years, Clin. Exp. Allergy, 37, 1137–1142, 2007. a
Mahura, A. G., Korsholm, U. S., Baklanov, A. A., and Rasmussen, A.: Elevated birch pollen episodes in Denmark: contributions from remote sources, Aerobiologia, 23, 171–179, https://doi.org/10.1007/s10453-007-9061-3, 2007. a, b
Mäkelä, E. M.: Size distinctions between Betula pollen types – A review, Grana, 35, 248–256, https://doi.org/10.1080/00173139609430011, 1996. a
Masaka, K. and Maguchi, S.: Modelling the Masting Behaviour of Betula platyphylla var. japonica using the Resource Budget Model, Ann. Bot., 88, 1049–1055, https://doi.org/10.1006/anbo.2001.1547, 2001. a
Masson, V., Champeaux, J.-L., Chauvin, F., Meriguet, C., and Lacaze, R.: A Global Database of Land Surface Parameters at 1-km Resolution in Meteorological and Climate Models, J. Climate, 16, 1261–1282, https://doi.org/10.1175/1520-0442(2003)16<1261:AGDOLS>2.0.CO;2, 2003. a
McInnes, R. N., Hemming, D., Burgess, P., Lyndsay, D., Osborne, N. J., Skjøth, C. A., Thomas, S., and Vardoulakis, S.: Mapping allergenic pollen vegetation in UK to study environmental exposure and human health, Sci. Total Environ., 599–600, 483–499, https://doi.org/10.1016/j.scitotenv.2017.04.136, 2017. a, b
McMaster, G. S. and Wilhelm, W.: Growing degree-days: one equation, two interpretations, Agr. Forest Meteorol., 87, 291–300, https://doi.org/10.1016/S0168-1923(97)00027-0, 1997. a
Nilsson, S. and Persson, S.: Tree pollen spectra in the stockholm region (sweden), 1973–1980, Grana, 20, 179–182, https://doi.org/10.1080/00173138109427661, 1981. a
Päivinen, R., Lehikoinen, M., Schuck, A., Häme, T., Väätäinen, S., Kennedy, P., and Folving, S.: Combining Earth Observation Data and Forest Statistics, Tech. Rep. 14, EFI, Joensuu and Joint Research Centre/European Commission, 2001. a
Pauling, A., Rotach, M. W., Gehrig, R., Clot, B., and Contributors to the European Aeroallergen Network (EAN): A method to derive vegetation distribution maps for pollen dispersion models using birch as an example, Int. J. Biometeorol., 56, 949–958, https://doi.org/10.1007/s00484-011-0505-7, 2012. a, b, c, d
Pekkarinen, A., Reithmaier, L., and Strobl, P.: Pan-European forest/non-forest mapping with Landsat ETM+ and CORINE Land Cover 2000 data, ISPRS Journal of Photogrammetry and Remote Sensing, 64, 171–183, https://doi.org/10.1016/j.isprsjprs.2008.09.004, 2009. a
Persson, A.: User Guide to ECMWF forecast products, Tech. rep., ECMWF, 2011. a
Piotrowska, K. and Kaszewski, B. M.: Variations in birch (Betula spp.) pollen seasons in Lublin and correlations with meteorological factors in the period 2001–2010. A preliminary study, Acta Agrobot., 64, 39–50, 2011. a, b
Prank, M., Chapman, D. S., Bullock, J. M., Belmonte, J., Berger, U., Dahl, A., Jäger, S., Kovtunenko, I., Magyar, D., Niemelä, S., Rantio-Lehtimäki, A., Rodinkova, V., Sauliene, I., Severova, E., Sikoparija, B., and Sofiev, M.: An operational model for forecasting ragweed pollen release and dispersion in Europe, Agr. Forest Meteorol., 182–183, 43–53, https://doi.org/10.1016/j.agrformet.2013.08.003, 2013. a, b, c, d
Ranta, H., Oksanen, A., Hokkanen, T., Bondestam, K., and Heino, S.: Masting by Betula-species; applying the resource budget model to north European data sets, Int. J. Biometeorol., 49, 146–151, https://doi.org/10.1007/s00484-004-0228-0, 2005. a, b
Ranta, H., Kubin, E., Siljamo, P., Sofiev, M., Linkosalo, T., Oksanen, A., and Bondestam, K.: Long distance pollen transport cause problems for determining the timing of birch pollen season in Fennoscandia by using phenological observations, Grana, 45, 297–304, https://doi.org/10.1080/00173130600984740, 2006. a
Rasmussen, A.: The effects of climate change on the birch pollen season in Denmark, Aerobiologia, 18, 253–265, https://doi.org/10.1023/A:1021321615254, 2002. a
Ritenberga, O., Sofiev, M., Siljamo, P., Saarto, A., Dahl, A., Ekebom, A., Sauliene, I., Shalaboda, V., Severova, E., Hoebeke, L., and Ramfjord, H.: A statistical model for predicting the inter-annual variability of birch pollen abundance in Northern and North-Eastern Europe, Sci. Total Environ., 615, 228–239, https://doi.org/10.1016/j.scitotenv.2017.09.061, 2018. a, b
Seinfeld, J. and Pandis, S.: Atmospheric Chemistry and Physics: From Air Pollution to Climate Change, A Wiley-Interscience publication, Wiley, 2006. a
Siljamo, P.: Numerical modelling of birch pollen emissions and dispersion on regional and continental scales, PhD thesis, Finnish Meteorological Institute, Erik Palménin Aukio 1 (P.O. Box 503) 00101 Helsinki, 2013. a
Siljamo, P., Sofiev, M., Ranta, H., Linkosalo, T., Kubin, E., Ahas, R., Genikhovich, E., Jatczak, K., Jato, V., Nekovár, J., Minin, A., Severova, E., and Shalaboda, V.: Representativeness of point-wise phenological Betula data collected in different parts of Europe, Global Ecol. Biogeogr., 17, 489–502, https://doi.org/10.1111/j.1466-8238.2008.00383.x, 2008. a
Siljamo, P., Sofiev, M., Filatova, E., Grewling, Ł., Jäger, S., Khoreva, E., Linkosalo, T., Ortega Jimenez, S., Ranta, H., Rantio-Lehtimäki, A., Svetlov, A., Veriankaite, L., Yakovleva, E., and Kukkonen, J.: A numerical model of birch pollen emission and dispersion in the atmosphere. Model evaluation and sensitivity analysis, Int. J. Biometeorol., 57, 125–136, https://doi.org/10.1007/s00484-012-0539-5, 2013. a, b, c, d
Skjøth, C. A., Sommer, J., Stach, A., Smith, M., and Brandt, J.: The long-range transport of birch (Betula) pollen from Poland and Germany causes significant pre-season concentrations in Denmark, Clin. Exp. Allergy, 37, 1204–1212, https://doi.org/10.1111/j.1365-2222.2007.02771.x, 2007. a, b
Skjøth, C. A., Geels, C., Hvidberg, M., Hertel, O., Brandt, J., Frohn, L. M., Hansen, K. M., Hedegaard, G. B., Christensen, J. H., and Moseholm, L.: An inventory of tree species in Europe-An essential data input for air pollution modelling, Ecol. Model., 217, 292–304, https://doi.org/10.1016/j.ecolmodel.2008.06.023, 2008a. a, b, c, d
Skjøth, C. A., Sommer, J., Brandt, J., Hvidberg, M., Geels, C., Hansen, K. M., Hertel, O., Frohn, L. M., and Christensen, J. H.: Copenhagen – a significant source of birch (Betula) pollen?, Int. J. Biometeorol., 52, 453–462, https://doi.org/10.1007/s00484-007-0139-y, 2008b. a
Skjøth, C. A., Ørby, P. V., Becker, T., Geels, C., Schlünssen, V., Sigsgaard, T., Bønløkke, J. H., Sommer, J., Søgaard, P., and Hertel, O.: Identifying urban sources as cause of elevated grass pollen concentrations using GIS and remote sensing, Biogeosciences, 10, 541–554, https://doi.org/10.5194/bg-10-541-2013, 2013a. a
Skjøth, C. A., Šikoparija, B., Jäger, S., and EAN-Network: Pollen sources, in: Allergenic pollen, Springer, 9–27, https://doi.org/10.1007/978-94-007-4881-1_2, 2013b. a, b
Skjøth, C. A., Smith, M., Šikoparija, B., Stach, A., Myszkowska, D., Kasprzyk, I., Radišić, P., Stjepanović, B., Hrga, I., Apatini, D., Magyar, D., Páldy, A., and Ianovici, N.: A method for producing airborne pollen source inventories: An example of Ambrosia (ragweed) on the Pannonian Plain, Agr. Forest Meteorol., 150, 1203–1210, https://doi.org/10.1016/j.agrformet.2010.05.002, 2010. a
Smith, M., Skjøth, C., Myszkowska, D., Uruska, A., Puc, M., Stach, A., Balwierz, Z., Chlopek, K., Piotrowska, K., Kasprzyk, I., and Brandt, J.: Long-range transport of Ambrosia pollen to Poland, Agr. Forest Meteorol., 148, 1402–1411, https://doi.org/10.1016/j.agrformet.2008.04.005, 2008. a
Sofiev, M.: On impact of transport conditions on variability of the seasonal pollen index, Aerobiologia, 33, 1–13, https://doi.org/10.1007/s10453-016-9459-x, 2017. a, b, c, d
Sofiev, M.: On possibilities of assimilation of near-real-time pollen data by atmospheric composition models, Aerobiologia, 35, 523–531, https://doi.org/10.1007/s10453-019-09583-1, 2019. a
Sofiev, M., Siljamo, P., Ranta, H., and Rantio-Lehtimäki, A.: Towards numerical forecasting of long-range air transport of birch pollen: theoretical considerations and a feasibility study, Int. J. Biometeorol., 50, 392–402, https://doi.org/10.1007/s00484-006-0027-x, 2006. a, b, c
Sofiev, M., Siljamo, P., Ranta, H., Linkosalo, T., Jaeger, S., Jaeger, C., Rassmussen, A., Severova, E., Oksanen, A., Karppinen, A., and Kukkonen, J.: From Russia to Iceland: an evaluation of a large-scale pollen and chemical air pollution episode during April and May, 2006, in: Aerobiological Monographs, Towards a comprehensive vision, edited by: Clot, B., Comtois, P., and Escamilla Garcia, B., MeteoSwiss and University of Montreal, vol. 1, 95–114, 2011. a
Sofiev, M., Siljamo, P., Ranta, H., Linkosalo, T., Jaeger, S., Rasmussen, A., Rantio-Lehtimaki, A., Severova, E., and Kukkonen, J.: A numerical model of birch pollen emission and dispersion in the atmosphere. Description of the emission module, Int. J. Biometeorol., 57, 45–58, https://doi.org/10.1007/s00484-012-0532-z, 2013. a, b, c, d
Sofiev, M., Berger, U., Prank, M., Vira, J., Arteta, J., Belmonte, J., Bergmann, K.-C., Chéroux, F., Elbern, H., Friese, E., Galan, C., Gehrig, R., Khvorostyanov, D., Kranenburg, R., Kumar, U., Marécal, V., Meleux, F., Menut, L., Pessi, A.-M., Robertson, L., Ritenberga, O., Rodinkova, V., Saarto, A., Segers, A., Severova, E., Sauliene, I., Siljamo, P., Steensen, B. M., Teinemaa, E., Thibaudon, M., and Peuch, V.-H.: MACC regional multi-model ensemble simulations of birch pollen dispersion in Europe, Atmos. Chem. Phys., 15, 8115–8130, https://doi.org/10.5194/acp-15-8115-2015, 2015. a. a, b, c, d, e, f
Sofiev, M., Vira, J., Kouznetsov, R., Prank, M., Soares, J., and Genikhovich, E.: Construction of the SILAM Eulerian atmospheric dispersion model based on the advection algorithm of Michael Galperin, Geosci. Model Dev., 8, 3497–3522, https://doi.org/10.5194/gmd-8-3497-2015, 2015b. a
Sørensen, B., Kaas, E., and Korsholm, U. S.: A mass-conserving and multi-tracer efficient transport scheme in the online integrated Enviro-HIRLAM model, Geosci. Model Dev., 6, 1029–1042, https://doi.org/10.5194/gmd-6-1029-2013, 2013. a
Stier, P., Feichter, J., Kinne, S., Kloster, S., Vignati, E., Wilson, J., Ganzeveld, L., Tegen, I., Werner, M., Balkanski, Y., Schulz, M., Boucher, O., Minikin, A., and Petzold, A.: The aerosol-climate model ECHAM5-HAM, Atmos. Chem. Phys., 5, 1125–1156, https://doi.org/10.5194/acp-5-1125-2005, 2005. a
Thibaudon, M., Šikoparija, B., Oliver, G., Smith, M., and Skjøth, C. A.: Ragweed pollen source inventory for France – The second largest centre of Ambrosia in Europe, Atmos. Environ., 83, 62–71, https://doi.org/10.1016/j.atmosenv.2013.10.057, 2014. a
Undén, P., Rontu, L., Järvinen, H., Lynch, P., Calvo, J., Cats, G., Cuxart, J., Eerola, K., Fortelius, C., Garcia-Moya, J. A., Jones, C., Lenderlink, G., McDonald, A., Mc-Grath, R., Navascues, B., Nielsen, N. W., Øidegaard, V., Rodriguez, E., Rummukainen, M., Rõõm, R., Sattler, K., Sass, B. H., Savijärvi, H., Schreur, B. W., Sigg, R., The, H., and Tijm, A.: HIRLAM-5 Scientific Documentation, The HIRLAM project, 2002. a
Uppala, S. M., KÅllberg, P. W., Simmons, A. J., Andrae, U., Bechtold, V. D. C., Fiorino, M., Gibson, J. K., Haseler, J., Hernandez, A., Kelly, G. A., Li, X., Onogi, K., Saarinen, S., Sokka, N., Allan, R. P., Andersson, E., Arpe, K., Balmaseda, M. A., Beljaars, A. C. M., Berg, L. V. D., Bidlot, J., Bormann, N., Caires, S., Chevallier, F., Dethof, A., Dragosavac, M., Fisher, M., Fuentes, M., Hagemann, S., Hólm, E., Hoskins, B. J., Isaksen, L., Janssen, P. A. E. M., Jenne, R., Mcnally, A. P., Mahfouf, J.-F., Morcrette, J.-J., Rayner, N. A., Saunders, R. W., Simon, P., Sterl, A., Trenberth, K. E., Untch, A., Vasiljevic, D., Viterbo, P., and Woollen, J.: The ERA-40 re-analysis, Q. J. Roy. Meteor. Soc., 131, 2961–3012, https://doi.org/10.1256/qj.04.176, 2005. a
Veriankaite, L.: Pollen concentration in the air: circulating and phenological aspects, PhD thesis, Vilnius University, 3 Universiteto St, 01513 Vilnius, Lithuania, 2010. a, b
WHO: Phenology and human health: allergic disorders, Tech. rep., 2003. a
Yu, S., Eder, B., Dennis, R., Chu, S.-H., and Schwartz, S. E.: New unbiased symmetric metrics for evaluation of air quality models, Atmos. Sci. Lett., 7, 26–34, https://doi.org/10.1002/asl.125, 2006. a
Zhang, R., Duhl, T., Salam, M. T., House, J. M., Flagan, R. C., Avol, E. L., Gilliland, F. D., Guenther, A., Chung, S. H., Lamb, B. K., and VanReken, T. M.: Development of a regional-scale pollen emission and transport modeling framework for investigating the impact of climate change on allergic airway disease, Biogeosciences, 11, 1461–1478, https://doi.org/10.5194/bg-11-1461-2014, 2014. a
Zink, K., Vogel, H., Vogel, B., Magyar, D., and Kottmeier, C.: Modeling the dispersion of Ambrosia artemisiifolia L. pollen with the model system COSMO-ART, Int. J. Biometeorol., 56, 669–680, 2012. a
Zink, K., Pauling, A., Rotach, M. W., Vogel, H., Kaufmann, P., and Clot, B.: EMPOL 1.0: a new parameterization of pollen emission in numerical weather prediction models, Geosci. Model Dev., 6, 1961–1975, https://doi.org/10.5194/gmd-6-1961-2013, 2013. a
Zink, K., Kaufmann, P., Petitpierre, B., Broennimann, O., Guisan, A., Gentilini, E., and Rotach, M. W.: Numerical ragweed pollen forecasts using different source maps: a comparison for France, Int. J. Biometeorol., 61, 23–33, 2017. a, b, c, d, e
Ziska, L. H., Gebhard, D. E., Frenz, D. A., Faulkner, S., Singer, B. D., and Straka, J. G.: Cities as harbingers of climate change: Common ragweed, urbanization, and public health, J. Allergy Clin. Immun., 111, 290–295, https://doi.org/10.1067/mai.2003.53, 2003. a