the Creative Commons Attribution 4.0 License.
the Creative Commons Attribution 4.0 License.
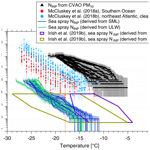
Characterization of aerosol particles at Cabo Verde close to sea level and at the cloud level – Part 2: Ice-nucleating particles in air, cloud and seawater
Heike Wex
Manuela van Pinxteren
Nadja Triesch
Khanneh Wadinga Fomba
Jasmin Lubitz
Christian Stolle
Tiera-Brandy Robinson
Thomas Müller
Hartmut Herrmann
Frank Stratmann
Ice-nucleating particles (INPs) in the troposphere can form ice in clouds via heterogeneous ice nucleation. Yet, atmospheric number concentrations of INPs (NINP) are not well characterized, and, although there is some understanding of their sources, it is still unclear to what extend different sources contribute or if all sources are known. In this work, we examined properties of INPs at Cabo Verde (a.k.a. Cape Verde) from different environmental compartments: the oceanic sea surface microlayer (SML), underlying water (ULW), cloud water and the atmosphere close to both sea level and cloud level.
Both enrichment and depletion of NINP in SML compared to ULW were observed. The enrichment factor (EF) varied from roughly 0.4 to 11, and there was no clear trend in EF with ice-nucleation temperature.
NINP values in PM10 sampled at Cape Verde Atmospheric Observatory (CVAO) at any particular ice-nucleation temperature spanned around 1 order of magnitude below −15 ∘C, and about 2 orders of magnitude at warmer temperatures ( ∘C). Among the 17 PM10 samples at CVAO, three PM10 filters showed elevated NINP at warm temperatures, e.g., above 0.01 L−1 at −10 ∘C. After heating samples at 95 ∘C for 1 h, the elevated NINP at the warm temperatures disappeared, indicating that these highly ice active INPs were most likely biological particles.
INP number concentrations in PM1 were generally lower than those in PM10 at CVAO. About 83±22 %, 67±18 % and 77±14 % (median±standard deviation) of INPs had a diameter >1 µm at ice-nucleation temperatures of −12, −15 and −18 ∘C, respectively. PM1 at CVAO did not show such elevated NINP at warm temperatures. Consequently, the difference in NINP between PM1 and PM10 at CVAO suggests that biological ice-active particles were present in the supermicron size range.
NINP in PM10 at CVAO was found to be similar to that on Monte Verde (MV, at 744 m a.s.l.) during noncloud events. During cloud events, most INPs on MV were activated to cloud droplets. When highly ice active particles were present in PM10 filters at CVAO, they were not observed in PM10 filters on MV but in cloud water samples instead. This is direct evidence that these INPs, which are likely biological, are activated to cloud droplets during cloud events.
For the observed air masses, atmospheric NINP values in air fit well to the concentrations observed in cloud water. When comparing concentrations of both sea salt and INPs in both seawater and PM10 filters, it can be concluded that sea spray aerosol (SSA) only contributed a minor fraction to the atmospheric NINP. This latter conclusion still holds when accounting for an enrichment of organic carbon in supermicron particles during sea spray generation as reported in literature.
- Article
(7050 KB) - Full-text XML
- Companion paper
-
Supplement
(1223 KB) - BibTeX
- EndNote
Ice particle formation in tropospheric clouds can affect cloud properties such as cloud lifetime, their radiative effects on the atmosphere and the formation of precipitation (Hoose and Möhler, 2012; Murray et al., 2012). Ice crystals in the atmosphere can be formed either via homogeneous nucleation below −38 ∘C or via heterogeneous nucleation aided by aerosol particles known as ice-nucleating particles (INPs) at any temperature below 0 ∘C. Immersion freezing refers to the process when an INP becomes immersed in an aqueous solution, e.g., through the process of cloud droplet activation (Vali et al., 2015). Immersion freezing is suggested to be the most important freezing process for mixed-phase clouds (Ansmann et al., 2008; Westbrook and Illingworth, 2013), and is the process we will focus on in this study.
Submicron dust particles are recognized as effective INPs below −20 ∘C (Augustin-Bauditz et al., 2014), and supermicron dust particles were reported to be ice active even up to −10 ∘C (Hoose and Möhler, 2012; Murray et al., 2012). Laboratory studies on natural mineral dusts from different regions have been conducted to quantify the ability of particles to nucleate ice (Niemand et al., 2012; DeMott et al., 2015). Mineral dust particles from deserts are composed of a variety of minerals, and K-feldspar is supposed to be more active for ice nucleation than other minerals in the mixed-phase cloud temperature regime (Atkinson et al., 2013; Augustin-Bauditz et al., 2014; Niedermeier et al., 2015). Boose et al. (2016) found that ice activity of desert dust particles at temperatures between −35 and −28 ∘C can be attributed to the sum of the feldspar and quartz content. A high clay content, in contrast, was associated with lower ice-nucleating activity. In contrast to field measurements, in laboratory studies often separate types of mineral dusts are examined. Different parameterizations have been employed to summarize the mineral dust particle's ice-nucleating ability (Niemand et al., 2012; Ullrich et al., 2017).
A few field measurements have been carried out to quantify the ice-nucleation properties of desert dust. Based on airborne measurements, DeMott et al. (2003) found that ice-nucleating aerosol particles in air masses over Florida had sources from the north African desert. Chou et al. (2011) observed a good correlation between the number concentration of larger particles and INP number concentration (NINP) during a Saharan dust event at Jungfraujoch in the Swiss Alps. Collecting airborne dust over the Sahara, Price et al. (2018) observed 2 orders of magnitude variability in NINP at any particular temperature from to ∘C, which was related to the variability in atmospheric dust loading. This desert dust's ice-nucleating activity was only weakly dependent on differences in desert sources, i.e., on the differences in mineral composition that particles emitted from different locations in the desert may have. Schrod et al. (2017) found that mineral dust or a constituent related to dust was a major contributor to NINP for the aerosol on Cyprus, and NINP in elevated dust plumes was on average a factor of 10 higher than NINP at ground level, where the dust loading was lower.
Ocean water can be a potential source of INPs (Brier and Kline, 1959). The source of INPs in ocean water might be associated with phytoplankton blooms (Schnell and Vali, 1976). Recently, Wilson et al. (2015) and Irish et al. (2017) found that organic material, with a diameter <0.2 µm, is the major ice nucleator in the sea surface microlayer (SML). Based on a long-term measurement of INPs in the marine boundary layer to the south of and around Australia, Bigg (1973) suggested that INPs in ambient air were from a distant land source, from a stratospheric source, or brought to sea level by convective mixing and possible ocean sources. Schnell and Vali (1976) also suggested a marine source could explain the observations of Bigg (1973). DeMott et al. (2016) found that the ice-nucleating activity from laboratory-generated sea spray aerosol (SSA) aligned well with measurements from diverse regions over the oceans. Furthermore, a connection between marine biological activity and NINP was uncovered in their laboratory study (DeMott et al., 2016). In pristine marine conditions, such as the Southern Ocean, SSA was the main source of the INP population, but NINP was relatively low in the Southern Ocean as well as in the clean marine northeast Atlantic (McCluskey et al., 2018a, b). These field measurements are consistent with the model work by Burrows et al. (2013), which emphasizes the importance of SSA contribution to INPs in remote marine regions.
It is currently still uncertain whether the coarse mode particles or smaller particles are the major source of atmospheric INPs. Vali (1966) found that the diameters of INPs were mostly between 0.1 and 1 µm. On the high alpine research station Jungfraujoch, Mertes et al. (2007) found that ice residuals were as small as 300 nm, and they were mostly present in the submicron particle size range. Simultaneous measurements of NINP and particle number size distributions were used to develop parameterizations in which NINP depends on a temperature-dependent fraction of all particles with sizes above 500 nm (DeMott et al., 2010, 2015). Conen et al. (2017) found that INPs at −8 ∘C were equally distributed amongst the particles with sizes up to 2.5 µm and with sizes between 2.5 and 10 µm. Other field measurements reported that coarse mode particles were more efficient INPs, e.g., INPs (mainly bacterial aggregates and fungal spores) occurred in the size range of 2–6 µm (Huffman et al., 2013). Mason et al. (2016) found for Arctic aerosol that 91±9 %, 79±17 % and 63±21 % (mean ± 1 standard deviation) of INPs had an aerodynamic diameter of >1 µm at ice activation temperatures of −15, −20 and −25 ∘C, respectively. Creamean et al. (2018) also found that supermicron or coarse mode particles are the most proficient INPs at warmer temperatures in the Arctic boundary layer, and they might be biological INPs. Concerning biological INPs, it should be mentioned that it is well understood by now that these contain macromolecules of only some 10 nm in size at the most (Pummer et al., 2015). Some of them are easily separated from their carrier (e.g., from pollen and fungal spores; see, for example, Augustin et al., 2013; O'Sullivan et al., 2016, respectively), while others are embedded in the cell membrane (e.g., for bacteria; Hartmann et al., 2013), but based on the fact that most atmospheric INPs seem to be supermicron in size, as observed in the above-cited literature, it seems that most of the biological ice-active macromolecules still occur together with their original carrier in the atmosphere.
Direct measurement of NINP in the cloud water can be used to estimate concentrations of INPs in the air assuming that most INPs activate as CCN. Joly et al. (2014) measured total and biological (i.e., heat-sensitive) INPs between −5 and −14 ∘C in cloud samples from the summit of Puy de Dôme (1465 m a.s.l., France). Petters and Wright (2015) summarized many INP spectra obtained from rain water, melted sleet, snow and hail samples at different sampling locations and reported a range of NINP for these precipitation samples. Based on a shipborne measurement of the east coast of Nova Scotia, Canada, Schnell (1977) directly compared NINP in the seawater to that in the fog water and found that NINP in fog water and seawater appeared to vary quite independently of each other. As one part of the study presented here, these field measurement values will be compared with values obtained from our measurement campaign in the framework of the MarParCloud (Marine biological production, organic aerosol particles and marine clouds: a Process Chain) project.
During the MarParCloud project, samples collected for INP analysis include: SML and underlying water (ULW) from the ocean upwind of the island; quartz fiber filter samples of atmospheric aerosol, collected on a tower installed at the island shore (inlet height: 42 m a.s.l.) and on a mountaintop (inlet height: 746 m a.s.l.); and cloud water collected during cloud events on the mountaintop. In this study, we will first discuss NINP in the SML and ULW. We will then discuss NINP in the air, including a comparison of NINP in PM10 and PM1 and a comparison of NINP close to both sea level and cloud level. Lastly, NINP in the cloud water will be discussed. In addition, we will provide a feasible way to link NINP in ambient air, ocean water and cloud water. This connection could be drawn only during times when there were cloud events on the mountaintop, together with data on number concentrations of cloud condensation nuclei (NCCN). Respective information was derived and discussed in an accompanying paper (Gong et al., 2020). For more information about the campaign itself, we refer to an upcoming overview paper by van Pinxteren et al. (2019).
2.1 Sampling sites and sample types
2.1.1 Sampling site
The measurement campaign was carried out on São Vicente island at Cabo Verde from 13 September to 13 October 2017. We set up three measurement stations at Cabo Verde: at the Cape Verde Atmospheric Observatory (CVAO), on Monte Verde (MV) and an ocean station (OS). CVAO (16∘51′49′′ N, 24∘52′02′′ W) is located in the northeastern shore of the island of São Vicente, 70 m from the coastline about 10 m a.s.l. Filter samplers were installed on top of a 32 m tower. MV (16∘52′11′′ N, 24∘56′02′′ W) is located on a mountaintop (744 m a.s.l), ∼7 km away to the west of CVAO. Filter samplers were situated on the ground with the inlet 2 m above the bottom, upwind of any installation on the mountaintop. The OS covered an area at ∼16∘53′30′′ N, ∼24∘54′00′′ W, with a distance of at least 5 km from the island. Details on the measurement sites and the meteorological conditions can be found in the accompanying paper (Gong et al., 2020). In short, the conditions at Cabo Verde were quite stable, with temperature of on average 26.6 ∘C at CVAO and 21.2 ∘C at MV and wind speeds between 0.6 and 9.7 m s−1 with directions from the northeast.
In the following, the different samples collected during the campaign are described in detail. All of these samples were stored at −20 ∘C right after sampling. After the campaign, the long-term storage and transport of the collected samples from Cabo Verde to the Leibniz Institute for Tropospheric Research (TROPOS), Germany, was carried out in a cooled container at −20 ∘C. At TROPOS, all samples were again stored frozen at −20 ∘C until analysis was done. Measurement sites, locations, sample types and additional information are summarized in Table 1.
Following the description of the sampling, we will briefly introduce the measurement methods related to INPs, including freezing devices, NINP calculation and measurement uncertainties. Note that all the times presented here are in UTC (corresponding to LT+1). For better comparison, all ambient particle number concentrations in this study are given for standard temperature and pressure (STP, 0 ∘C and 1013.25 hPa).
2.1.2 Seawater sampling
Seawater samples were taken at the OS by using a fishing boat at a distance of at least 5 km from the coast (offshore samples). The SML samples were collected using a glass plate sampler (Harvey and Burzell, 1972; Irish et al., 2017; van Pinxteren et al., 2017). The glass plate had a surface area of 2000 cm−2 and was immersed vertically into the ocean; it was then withdrawn at a slow rate (between 5 and 10 cm s−1) and allowed to drain for less than 5 s. The surface film adhering to the surface of the glass was scraped off from both sides of the glass plate with a framed Teflon wiper into a 1 L glass bottle. For each SML sample, several liters were collected and 1 L required ∼55 dips. Based on the amount of material collected, the number of dips and the area of the plate, the average thickness of the layer collected was calculated as ∼91.0 µm. ULW samples were collected at the same time and location as the SML samples. ULW was collected from a depth of 1 m by a glass bottle mounted on a telescopic rod in order to monitor sampling depth. The bottle was opened underwater at the intended sampling depth with a specifically designed seal opener. After collection, the glass bottles containing both the SML and ULW samples were kept in a freezer at −20 ∘C until analysis. During the campaign, nine SML and nine ULW samples were collected for INP analysis. Details of SML and ULW samples, including the sampling time, location, salinity and additional information, are provided in the Supplement (Table S1).
2.1.3 Aerosol particle sampling
Particle sampling was done using high-volume samplers with either a PM10 inlet and or a PM1 inlet (Digitel filter sampler DHA-80, Walter Riemer Messtechnik, Germany) operating with an average flow rate of ∼500 L min−1 for 24 h sampling periods. The high-volume samples were collected on 150 mm in diameter quartz fiber filters (Munktell, MK 360) with an effective sampling area of 140 mm in diameter. The filters were preheated in our laboratory at 110 ∘C for 24 h to remove the organic carbon background. After sampling, the filters were transported to a freezer where they were kept at −20 ∘C. For INP analysis, a circular piece of these filters of 2 cm in diameter was used from which then smaller pieces were punched out for the analysis (see Sect. 2.2). From CVAO, there were 17 and 19 filters from PM10 and PM1 collection (CVAO PM10 and CVAO PM1), respectively, and at MV 17 filters were collected for PM10 (MV PM10). Field blind filters were obtained by inserting clean filters into the Digitel sampler for a period of 24 h without loading them. Three blind filters were collected during this campaign. Details of filter samples, including sampling time, duration, total volume and additional information can be found in the Supplement (Table S2 (CVAO PM10), Table S3 (CVAO PM1) and Table S4 (MV PM10)).
2.1.4 Cloud water sampling
During the campaign, MV was in clouds roughly 58 % of the time (a detailed analysis on this can be found in Gong et al., 2020). Cloud water was collected with CASCC2 (Caltech Active Strand Cloud Collector Version 2) at MV. All cloud drop sizes were collected in one bulk sample. Drops were collected by inertial impaction on Teflon strands with a diameter of 508 µm. The 50 % lower size cut for the CASCC2 was approximately 3.5 µm diameter. The flow rate through the CASCC2 was approximately 5.8 m3 min−1. The CASCC2 is described in more detail in Demoz et al. (1996). Between cloud events, the cloud water sampler was cleaned with a large amount (∼5 L) of ultrapure water. Once the collector was cleaned, a blank was taken by spraying about 200 mL of ultrapure water into the collection strands in the collector and subsequent sampling of this water. After collection, the cloud water samples were kept in a freezer at −20 ∘C. During the campaign, 13 cloud samples were collected for INP analysis. The details of cloud samples, including sampling time, duration, volume and additional information are provided in the Supplement (Table S5).
2.2 Freezing devices
Two droplet freezing devices called LINA (Leipzig Ice Nucleation Array) and INDA (Ice Nucleation Droplet Array) have been set up at TROPOS in Germany. The design of LINA was inspired by Budke and Koop (2015). Briefly, 90 droplets with a volume of 1 µL were pipetted from the samples onto a thin hydrophobic glass slide, with each droplet being placed separately into its own compartment. After pipetting, the compartments were sealed at the top with another glass slide to prevent the droplets from evaporation and to prevent ice seeding from neighboring droplets. The droplets were cooled on a Peltier element with a cooling rate of 1 K min−1 down to −35 ∘C, while the setup was illuminated by a circular light source from above. Once the cooling started, pictures were taken every 6 s by a camera. The number of frozen versus unfrozen droplets was derived automatically by an image identification program in Python. LINA was employed to measure SML, ULW and cloud water samples in this study. More detailed parameters and the temperature calibration of LINA and its application can be found in previous studies (Chen et al., 2018; Gong et al., 2019a).
The design of INDA was inspired by Conen et al. (2012) but uses PCR (polymerase chain reaction) trays instead of separate tubes. For quartz fiber filters, circular pieces with a diameter of 1 mm were punched out. Each of the 96 wells of a PCR tray were filled with the filter piece together with 50 µL of ultrapure water. For SML, ULW and cloud water samples, 50 µL of the water samples was filled into each PCR tray. After sealing by a transparent foil, the PCR tray was placed on a sample holder and immersed into a bath thermostat, where it was illuminated from below with a LED light source. The bath thermostat then decreased the temperature with a cooling rate of approximately 1 K min−1. Real-time images of the PCR tray were recorded every 6 s by a CCD (charge-coupled device) camera. Frozen droplets can be identified based on the brightness change during the freezing process. A program recorded the actual temperature of the cooling bath and related it to the real-time images from the CCD camera. The temperature in the PCR trays had been calibrated. More detailed parameters and information of temperature calibration of INDA and its application can be found in previous studies (Chen et al., 2018; Hartmann et al., 2019).
2.3 Deriving NINP
2.3.1 Basic calculation
Based on Vali (1971), the cumulative concentration of INPs (NINP) as a function of temperature per air or water volume can be calculated by
with
where Ntotal is the number of droplets and N(θ) is the number of frozen droplets at temperature θ. Equation (1) accounts for the possibility of the presence of multiple INPs in one vial by assuming that INPs are Poisson distributed. This way, the cumulative number of INPs active at any temperature will be obtained, although only the most ice-active INPs (nucleating ice at the highest temperature) present in each droplet or well will be observed. As for the quartz fiber filters, V is the volume of air collected onto one circular 1 mm filter piece placed in each well, resulting in airborne NINP. Information on the air volume can be found in the Supplement (Tables S2, S3 and S4). As for the SML, ULW and cloud water, V is the volume of droplet or well (VLINA=1 µL, VINDA=50 µL), resulting in NINP per volume of water. Compared to the droplets examined in a LINA measurement, INDA measurements have a larger volume of water in each well. The larger volume of water corresponds to a higher probability of the presence of INPs in each well; therefore, INDA can detect INPs at warmer temperatures, where INPs are more scarce. In this study, the derived NINP values from LINA and INDA measurements were combined when both instruments were deployed.
2.3.2 Uncertainty and background
Because the number of INPs present in the water is usually small (some single up to a few tens of INPs per examined droplet or well), and the number of droplets or wells considered in our measurements is limited, statistical errors need to be considered in the data evaluation. Therefore, confidence intervals for fice were determined using the method suggested by Agresti and Coull (1998). These confidence intervals were estimated according to the improved Wald interval, which implicitly assumes a normal approximation for binomially distributed measurement errors. Previous studies (McCluskey et al., 2018a; Suski et al., 2018; Gong et al., 2019a) used the same method to calculate the measurement uncertainties of the freezing devices.
For the quartz fiber filters, a background freezing signal resulting from the field blind filters was determined by doing a regular INDA measurement with these filters. Measured NINP from the sampled filters was corrected by subtracting the averaged background concentrations determined for the blind filters, as explained in Wex et al. (2019). All values for airborne NINP presented in the following are background corrected. A detailed description of the background subtraction method and background values is provided in the Supplement. For those samples that were already collected in a liquid state (ULW, SML and cloud water), a background correction was not done.
2.3.3 Salinity correction of SML and ULW
SML and ULW samples were adjusted to account for the freezing depression caused by dissolved salts in sea water. Based on Kreidenweis et al. (2005), the water activity can be calculated by
where the nsolute and nwater are the number of moles of solute and water in solution, respectively. i is the van 't Hoff factor (Pruppacher and Klett, 2010). We assumed sea salt to be mainly sodium chloride, for which the van 't Hoff factor is 2. The freezing depression temperature as a function of aw was taken from Koop and Zobrist (2009). In our study, this was roughly a correction by 2.2 ∘C.
2.4 Active surface site density
A thorough analysis of particle number size distributions (PNSDs) has been presented in Gong et al. (2020), and based on these PNSDs we derived the particle surface area size distributions (PASDs) for use in this study (to be seen in the Supplement, Fig. S14). These PASDs were used to determine the temperature-dependent cumulative active surface site density (ns) for aerosol particles. The ns is a measure of how well an aerosol acts as a seed surface for ice nucleation. The ns can be calculated as
where Atotal is the concentration of the total particle surface area.
For cases where a single type of aerosol, such as one type of mineral dust, is examined in laboratory studies, Atotal can be the total particle surface area. However, when field experiments are done, using the total particle surface area of the atmospheric aerosol assumes that all particles contribute to INPs and have the same ns, but the vast majority of these particles will not even be an INP. On the other hand, singling out the contribution of separate INP types in the atmospheric aerosol and relating ns only to them by using their contribution to the total surface area is at least demanding if not often impossible. This has to be kept in mind when interpreting heterogeneous ice nucleation in terms of ns. An example of separating the ns for dust and marine ambient air can be found in Cornwell et al. (2019).
3.1 INPs in SML and ULW
Based on Eq. (1), the derived NINP in seawater as a function of temperature is shown in Fig. 1, for both SML and ULW. Note that for each sample a separate INP spectrum is shown. Error bars show the 95 % confidence interval. For completeness, fice of all seawater samples is shown in the Supplement (Fig. S1 (measured by LINA) and Fig. S2 (measured by INDA)). The variation in NINP at any particular temperature is within 1 order of magnitude. Included in Fig. 1 are previous studies of NINP measured east of Greenland in the Arctic (shown as a red box) and east of North America in the North Atlantic Ocean (shown as a black box) from Wilson et al. (2015).
The concentration range detected for ULW in Wilson et al. (2015) (both in the Arctic and the North Atlantic Ocean) roughly agrees with our data. In Wilson et al. (2015), NINP in the SML in the North Atlantic Ocean is at the lower end of that found in the Arctic. A possible reason for this difference could be the biological activity of the ocean water. Wilson et al. (2015) found that organic material was correlated to NINP in SML, and that NINP values per gram of total organic carbon in the Arctic and the North Atlantic Ocean were comparable. A recent study found that the SML at Cabo Verde was oligotrophic, which is supported by the low Chlorophyll-a and transparent exopolymer particle concentrations found during the MarParCloud campaign (Robinson et al., 2019). The low biological activity in the SML around Cabo Verde could be the reason why NINP in SML in this study is lower than those reported in Wilson et al. (2015).
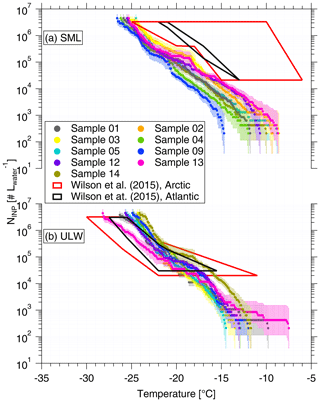
Figure 1NINP as a function of temperature in SML (a) and ULW (b). Error bars show the 95 % confidence interval. Previous field measurements of NINP in seawater by Wilson et al. (2015) are compared, as shown by red and black boxes.
To better quantify the enrichment or depletion of NINP in SML to ULW, we derived an enrichment factor (EF). An enrichment might be expected as organic material is known to attach to air bubbles rising to the ocean surface. The EF in SML was calculated by dividing NINP in SML (NINP, SML) by the respective NINP measured in ULW (NINP, ULW), as the equation below shows:
Enrichment of NINP in the SML is indicated when EF > 1, while depletion is indicated when EF < 1. Figure 2 shows the EF as a function of the temperature at which NINP was determined in the freezing devices. Both enrichment and depletion were observed, but there is no clear trend in the EF with temperature. Most of the variation seen here is likely caused by measurement uncertainties, which are indicated in Fig. S3 in the Supplement. EF varied from 0.36 to 11.40 at −15 ∘C and from 0.36 to 7.11 at −20 ∘C. By comparing T10 (the temperature at which 10 % of droplets had frozen) for the SML and ULW, Wilson et al. (2015) observed higher enrichment of INPs in SML in both the Arctic and the North Atlantic Ocean. However, Irish et al. (2017) observed both enrichment and depletion of INPs in SML in the Arctic, similar to the observation made in the present study.
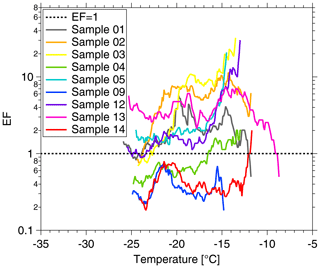
Figure 2Enrichment factor (EF) as a function of ice-nucleation temperature. The EF = 1 result is shown by the dashed line.
These differences in EF between studies might partially be due to differences in the techniques deployed and different SML thicknesses in our and the other studies. SML samples were estimated to be about ∼91.0 µm thick in this study, while for Wilson et al. (2015) they were between 6 and 83 µm. It is interesting to note that we used glass dipping for the samples analyzed herein, while both glass dipping and a rotating drum sampler were used in Wilson et al. (2015). Previous studies pointed out that the rotating drum sampler and the glass dipping method probe different thicknesses of the SML, thus making a direct comparison of both SML thickness as well as enrichment factors generally difficult (Agogué et al., 2004; Aller et al., 2017).
3.2 NINP in air
Three different sets of filter samples were collected at CVAO and MV, i.e., CVAO PM10, CVAO PM1 and MV PM10. In this section, we will discuss NINP at CVAO for the two different size classes and compare NINP from close to the sea level (CVAO) to that at cloud level (MV).
3.2.1 NINP close to sea level
CVAO PM10
NINP values as a function of temperature from CVAO PM10 filters and CVAO PM1 filters are shown in Fig. 3a and b. Error bars show the 95 % confidence interval. The respective values of fice are shown in the Supplement (Fig. S4 (CVAO PM10) and Fig. S8 (CVAO PM1)), together with the results from the blind filters. The CVAO PM10 filter samples were all active at −11.3 ∘C and the highest freezing temperature was found to be −5.0 ∘C. Filter samples collected in Cabo Verde over the period 2009–2013 for INP measurement were reported by Welti et al. (2018), and they are shown as a gray background in Fig. 3a. The measured NINP in this study is within the NINP range presented by Welti et al. (2018).
NINP values at any particular temperature span around 1 order of magnitude below −15 ∘C and about 2 orders of magnitude at warmer temperatures. This is consistent with the previous studies from O'Sullivan et al. (2018) and Gong et al. (2019a), who carried out field measurement in northwestern Europe and the eastern Mediterranean, respectively. A few samples (CVAO 1596, CVAO 1641 and CVAO 1643) showed elevated concentrations above 0.01 L−1 at −10 ∘C. Biological particles usually contribute to INPs at this moderate supercooling temperature (Kanji et al., 2017; O'Sullivan et al., 2018).
Biological INPs contain specific ice-nucleating proteins. These proteins are disrupted and denatured by heating, which causes them to lose their ice-nucleating ability. However, the inorganic ice-nucleating material, such as dust particles, is insensitive to heat (Wilson et al., 2015; O'Sullivan et al., 2018). Therefore, a commonly used heat treatment was deployed to assess the contribution of biological INPs to the total INPs in this study. Samples CVAO 1596, CVAO 1641 and CVAO 1643 were heated to 95 ∘C for 1 h, and the resulting NINP data are shown in Fig. S6. A clear comparison of before and after heating fice is shown in Fig. S7. A large reduction of more than 1 order of magnitude in NINP at ∘C was observed in the samples after heating. The reductions in NINP became smaller at colder temperature and were, for example, less than 1 order of magnitude at ∘C. This shows that biological aerosol contributed a large fraction of total INPs in PM10 at ∘C.
The correlation of NINP at different temperatures within one sample was calculated by comparing each NINP at each temperature to that at each other temperature at which a measurement had been made. That was done separately for each of the samples. For temperature steps of 0.1 ∘C, NINP at every temperature was correlated to that at every other temperature in the measurement range. With increasing difference in temperatures, the variation in NINP at two temperatures become less correlated. As long as the examined temperature difference was less than 2 ∘C, NINP were correlated. But when looking at this in a broader picture, in the temperature region down to ∘C, NINP at all temperatures correlated well with that at all other temperatures, with coefficient of determination (R2) > 0.8 and p<0.01. The same was true for NINP in the temperatures region ∘C. In between these two temperature regimes (between ∘C and ∘C), the correlation of NINP was clearly lower. Therefore, it might be expected that INPs that are active in these two temperature regimes originated from different sources.
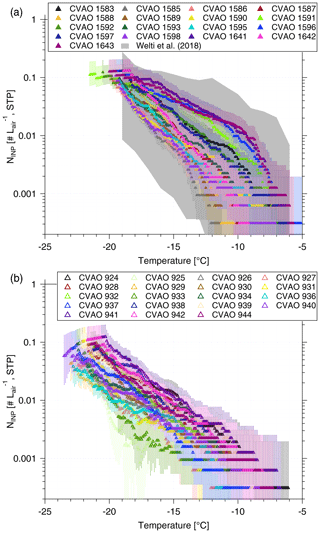
Figure 3NINP as a function of temperature from CVAO PM10 filters (a) and CVAO PM1 filters (b). The field measurement of NINP in PM10 by Welti et al. (2018) is shown by gray shading in panel (a). Error bars show the 95 % confidence interval.
CVAO PM1 in comparison to CVAO PM10
NINP values in PM1 filters are also determined in this study (as shown in Fig. 3b). An initial inspection of the data shows that the bulk of the data of NINP for CVAO PM1 is below that for CVAO PM10. Comparing NINP for PM1 and PM10, two key features are evident:
-
Larger particles, i.e., supermicron ones, were more efficient INPs, which is independent of temperature in the examined range.
-
Smaller particles, i.e., submicron ones, exhibited an equal spread of about 1 order of magnitude in NINP for the whole temperature range (see Fig. 3b). The elevated NINP values at warm temperatures, which are seen for CVAO PM10, are not observed for CVAO PM1.
As for the first feature, we calculated the ratio of NINP in supermicron size range to NINP in PM10 during the same time period and found that 83±22 %, 67±18 % and 77±14 % (median ± standard deviation) of INPs had a diameter of >1 µm at ice activation temperatures of −12, −15 and −18 ∘C, respectively. On average, over all temperatures, this INP number fraction for supermicron particles is roughly 70 % (shown for a higher temperature resolution in Fig. 4), and it is almost independent of temperature. Mason et al. (2016) and Creamean et al. (2018) also found that the majority of INPs is in the supermicron size range. However, they see even increasing fractions towards higher temperatures. For the present study, as said above, only 3 of the examined 17 filters showed clearly elevated NINP at high temperatures, so overall such an increase was not observed.
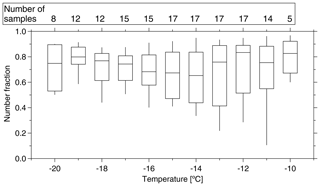
Figure 4Boxplot of number fraction of INPs in the size range of >1 µm as a function of temperature. The boxes represent the interquartile range. Whiskers represent 10th and 90th percentiles. The number of samples indicated at the top of the figure shows how many different samples contributed at the different temperatures.
As for the second feature, looking at Fig. 3b, we found that NINP spread about 1 order of magnitude at any temperature from −12 to −20 ∘C. As outlined above, a few PM10 samples showed elevated concentrations at warm temperatures, showing up as a “bump” in the freezing curves at higher temperatures. This bump at warm temperatures was not observed for the CVAO PM1 filters. NINP values of CVAO 932, CVAO 942 and CVAO 944 (sampled at the same time as CVAO 1596, CVAO 1641 and CVAO 1643) are all below 0.001 L−1 at −10 ∘C. As mentioned above, INPs active at comparably high temperatures were found to be biological in origin in this study, and the comparison between PM10 and PM1 samples shows that there are biological INPs in the CVAO PM10 samples that are absent in the CVAO PM1 samples, i.e., that the detected biological INPs are supermicron in size. This suggests that these biological INPs might originate from long-range transport, as marine biological INPs were usually reported to be submicron in size (Wilson et al., 2015; Irish et al., 2017). The contribution of SSA to INPs will be discussed further in Sect. 3.4.
3.2.2 NINP at cloud level
In the companion paper (Gong et al., 2020), we discussed PNSD and CCN number concentration (NCCN) at CVAO and MV. We found that particles are mainly well mixed in the marine boundary layer, and we derived the periods with cloud events, with a time resolution of ∼30 min, at MV. In the present study, NINP values in PM10 at CVAO and MV are compared. The fraction of time during which there was a cloud event to the total sampling time (cloud time fraction) for each filter is summarized in the Supplement (Table S4). All of the filters were affected by cloud events with a cloud time fraction from 4.17 % to 100 %, with two filters being affected only a little (cloud time fraction <10 %), i.e., MV 1602 and MV 1603. When comparing results from these two filters to those from filters sampled at the same time at CVAO (see Fig. 5a), we found that NINP values are quite similar close to sea level (CVAO) and cloud level (MV). This is in line with what was discussed in the companion paper (Gong et al., 2020), i.e., the marine boundary is often well mixed at Cabo Verde.
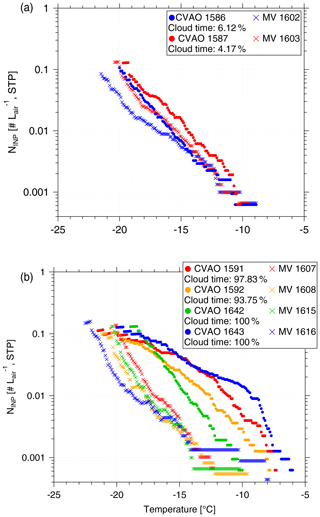
Figure 5NINP as a function of temperature from CVAO PM10 filters and MV PM10 filters during (a) less (cloud time fraction < 10 %) cloud effected periods and (b) highly (cloud time fraction > 90 %) cloud effected periods.
Figure 5b compares NINP values at CVAO and MV when MV filters were mostly collected during cloud events with cloud time fractions >90 %. During the cloud events, the filters did not collect droplets larger than 10 µm because of the inlet cutoff. It is obvious from Fig. 5 that for these cases, NINP at MV is much lower than that at CVAO, implying that particularly INPs that were ice active above ∘C were activated to cloud droplets to a large degree. But note that even when filters have a cloud time fraction of 100 % (MV 1615 and MV 1616), the respective filters still had clearly more INPs on them than the field blind filters (see Supplement, Fig. S9). This might indicate that either not all INPs are activated to cloud droplets, or, on the other hand, that some INPs were only recently activated to a cloud droplet, and the droplet size was smaller than 10 µm. These observations are consistent with results by Siebert and Shaw (2017), who observed broad cloud droplet size distributions in a size range from ∼5 to 25 µm in shallow cumulus clouds, with the maximum of the distribution still being below 10 µm.
Concerning the supermicron particles of likely biological origin that activated ice already at −10 ∘C and above, it is observed that the related corresponding bump is not seen in the corresponding data from MV (MV 1610, MV 1614 and MV 1616 – to be seen in the Supplement, Fig. S10). This indicates that these INPs were all activated to cloud droplets during the cloud events, and we will come back to this below.
3.3 INPs in cloud water
3.3.1 Main characteristics and NINP in cloud water
Thirteen cloud water samples were collected during cloud events in this study. Sampling durations varied from 2.5 to 13 h and volumes varied from 78 to 544 mL. The most abundant inorganic species were Na+ and Cl−, followed by , and Mg2+. For example, the mass concentration of Na+ and Cl− varied from 5.00 to 46.11 mg L−1 and 9.27 to 70.30 mg L−1, with a mean value of 17.31 and 28.86 mg L−1, respectively. Somewhat different values, which are still roughly in the same range, were reported by Gioda et al. (2009), who found in Puerto Rico the Na+ and Cl− concentration in the cloud water varied from 3.79 to 15.53 mg L−1 and 5.90 to 23.20 mg L−1, with a mean of 10.74 and 15.67 mg L−1, respectively. All of the abovementioned parameters are summarized in the Supplement (Table S5).
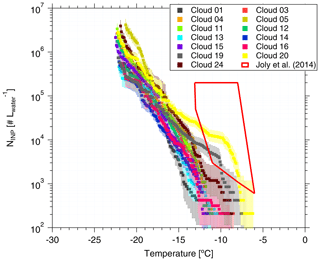
Figure 6NINP in cloud water as a function of temperature. Error bars show the 95 % confidence interval. Previous field measurements of NINP in cloud water by Joly et al. (2014) are shown as a red box for comparison.
Based on Eq. (1), the derived NINP as a function of temperature is shown in Fig. 6. Error bars represent the 95 % confidence interval. For completeness, fice for cloud water is shown in the Supplement (Fig. S12 (measured by LINA) and Fig. S13 (measured by INDA)). NINP values at any particular temperature span less than 1 order of magnitude below −15 ∘C, while they span 2 orders of magnitude at warmer temperatures. We observed elevated NINP in the cloud water at warm temperatures (above 1000 L−1 at −10 ∘C), particularly for the Cloud 19, Cloud 20 and Cloud 24 samples. Joly et al. (2014) measured the total and biological (i.e., heat-sensitive) INPs between −5 and −14 ∘C from the summit of Puy de Dôme (1465 m a.s.l., France), as shown in the red box in Fig. 6. Joly et al. (2014) observed very high concentrations of both biological particles and NINP. Agreement of NINP in cloud water all over the world was not expected, since the sources of INPs are different in different locations.
When highly ice active particles were present for CVAO PM10 filters (CVAO 1596, CVAO 1641 and CVAO 1643), they were not observed for MV PM10 (MV 1610, MV1614 and MV 1616, which had cloud time fractions of 52, 87 and 100 %, respectively), but instead they were found in cloud water samples (Cloud 19, Cloud 20 and Cloud 24). This is in line with what was outlined in Sect. 3.2.2: these highly ice active particles were activated to cloud droplets during cloud events. Periods during which clouds were present at MV, together with the sampling periods of all cloud water samples and selected CVAO PM10 filters (those that had higher NINP at warm temperatures; CVAO 1596, CVAO 1641 and CVAO 1643) can be checked in the Supplement (Fig. S11).
3.3.2 Connecting INPs in the cloud water with these in the air
In the following, NINP in the cloud water will be compared to that in the air. To be able to do this, we used measured values of NCCN to calculate cloud droplet number concentrations. These, together with an assumption on cloud droplet size (ddrop), yield the volume of cloud water per volume of air, given as Fcloud_air in Eq. (6):
For the calculation, we used NCCN measured at CVAO at a supersaturation of 0.30 % (Gong et al., 2020). NCCN was averaged for the different periods when each cloud water sample was collected. The chosen supersaturation corresponds to a critical diameter of roughly 80 nm, which is at the Hoppel minimum of the respective particle number size distributions (Gong et al., 2020), indicating that this is indeed the relevant supersaturation occurring in the prevailing clouds. Based on previous studies (Miles et al., 2000; Bréon et al., 2002; Igel and Heever, 2017; Siebert and Shaw, 2017), we assumed that ddrop varies between 7 and 20 µm, and we did separate estimates for these two values and additionally for 15 µm. The calculation based on this size range of cloud droplets should cover all that can be expected to occur.
Following this approach, Fcloud_air varied from to , with a median of m m. To see how reliable these values are, we also examined the following: assuming all sodium chloride particles were activated to cloud droplets, Fcloud_air can be also estimated from the ratio of sodium chloride mass concentration in air to that in cloud water. This ratio varied from to m m, which is at the lower end but still comparable to Fcloud_air as we derived it above. Previous studies used the liquid water content (LWC), which is a measure of the mass of the water in a cloud in a specified amount of dry air. Typical ranges for LWC in thicker clouds are between 0.2 and 0.8 g m−3 (Rangno and Hobbs, 2005; Petters and Wright, 2015), corresponding to Fcloud_air between and m m, which again agreed well with the above given values derived for this study.
With this Fcloud_air, NINP in the respective volume of air can be compared to NINP in this volume of cloud water when assuming that all INPs are CCN, which, based on the supermicron size of most of the INPs alone, is likely. To do so, NINP obtained for cloud water was multiplied by Fcloud_air (for the three different assumptions on ddrop) to yield NINP in the air (NINP,air), given in Eq. (7):
Figure 7 shows the measured NINP in the air as a function of temperature with square symbols. Derived NINP,air from cloud water (calculated with a ddrop of 15 µm) are shown with triangle symbols. The samples with comparatively high numbers of INPs active at warm temperatures are shown in different colors. CVAO 1596, CVAO 1641 and CVAO 1643 are shown by green squares (the rest are shown with blue squares) and derived NINP,air values from samples collected for Cloud 19, Cloud 20 and Cloud 24 are shown by brown triangles (the rest shown by red triangles). The range of values indicated for NINP,air was obtained by using 7 and 20 µm cloud droplet size, with 7 µm droplets yielding the lower boundary and 20 µm the upper one.
There is general agreement between measured and derived NINP in air but with some variation where the values derived from cloud water samples are somewhat lower. This might be connected to a less-than-optimal sampling efficiency of the cloud water sampler, which has a 50 % collection efficiency at 3.5 µm. Also the spread in the derived values, originating from the different assumed ddrop, is rather large. Nevertheless, it is striking that at least within an order of magnitude, based on our comparably simple assumptions, an agreement between concentrations of INPs in the air and in cloud water is found.
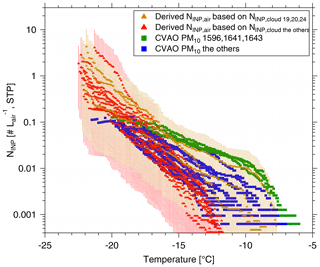
Figure 7The measured atmospheric NINP values as a function of ice-nucleation temperature are shown as squares. The derived NINP in the air (NINP,air) based on INP concentrations measured for cloud water are shown as triangles. The samples with highly ice active INPs at warm temperatures are shown in a different color than the others: CVAO 1596, CVAO 1641 and CVAO 1643 are shown as green squares and derived NINP,air based on Cloud 19, Cloud 20 and Cloud 24 are shown as brown triangles. The uncertainty range indicated for the derived NINP,air originates from calculations with 7 and 20 µm cloud droplet size.
3.4 INPs originating from sea spray
In the following section, it will briefly be discussed whether SSA contributed noticeably to INPs in the air. Assuming sea salt and INPs to be similarly distributed in both seawater and air (i.e., assuming that INPs would not be enriched during the production of sea spray), NINP in the air originating from sea spray () can be calculated based on Eq. (8):
where NaClmass,air and NaClmass,seawater are sodium chloride mass concentrations in air and seawater, respectively. is the INP number concentration in the seawater (this calculation can be done similarly for both SML and ULW).
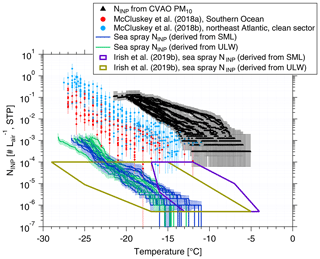
Figure 8Atmospheric NINP values are shown as a function of temperature from PM10 filters (black triangles), together with error bars showing the 95 % confidence interval. NINP values as a function of temperature from McCluskey et al. (2018a, b) are shown by red and light blue dots, respectively. Error bars show the 95 % confidence interval. NINP values in the air originating from sea spray () from this study are shown by blue (derived from SML) and green lines (derived from ULW). values from Irish et al. (2019b) are shown by purple (derived from SML) and brown (derived from ULW) boxes.
NaClmass,air and NaClmass,seawater data can be found in the Supplement (Tables S1 and S2). NaClmass,seawater was very stable, with a median value of ∼31 g L−1. NaClmass,air showed large variability from 3.40 to 17.76 µg m−3, with a median of 13.08 µg m−3. Based on Eq. (8), the resulting values are shown in blue (derived from SML) and green (derived from ULW) in Fig. 8. Irish et al. (2019b) used the same method to get in the Arctic (without considering enrichment of INPs in sea salt particles during sea spray generation), as shown by purple (derived from SML) and brown (derived from ULW) boxes in Fig. 8. As discussed in Sect. 3.1, NINP values from ULW at Cabo Verde are comparable to the Arctic, and the NaCl ratios were close to 10−10 in both studies; therefore, values (derived from ULW) are also comparable. A high enrichment of NINP in SML to ULW was observed in the Arctic (Irish et al., 2019b). Therefore, (derived from SML) in the Arctic was also higher than in this study.
Figure 8 includes NINP from PM10 in this study (shown by black triangles). These values are roughly 4 orders of magnitude above our . But Fig. 8 also shows airborne NINP as derived for the Southern Ocean (McCluskey et al., 2018a) and the northeast Atlantic (only clean sector; McCluskey et al., 2018b), which are all above our . As mentioned above, we did not consider a possible enrichment of INPs in SSA compared to the SML or ULW samples. Previous studies found an enrichment of organic carbon in submicron sea spray particles of about 104 to 105 (Keene et al., 2007; van Pinxteren et al., 2017), and this value decreased to 102 for supermicron particles (Keene et al., 2007; Quinn et al., 2015). It is not clear if INPs are included in the organic carbon for which the enrichment was observed. Also, the INPs we detected in this study were mostly in the supermicron size range. If we increased by about 2 orders of magnitude in agreement with the enrichment observed for supermicron organic carbon, the resulting becomes comparable to sea spray INPs measured in the Southern Ocean (McCluskey et al., 2018a) and the northeast Atlantic (McCluskey et al., 2018b). But even when considering such an enrichment of INPs, INPs originating from sea spray would only explain a small fraction of all INPs contributing to the measured airborne NINP in the air at Cabo Verde.
NINP values close to sea level and cloud level were compared. One major point of interest is to know whether ground-based measurements can be used to infer aerosol properties at the cloud level. In this study, we found that NINP values are quite similar close to sea level (CVAO) and cloud level (MV) during noncloud events. But it should still be noted that we only have a small number of filter samples representing noncloud events in this study. During the observed cloud events, most INPs at MV are activated to cloud droplets. The above findings are in line with what was discussed in the companion paper (Gong et al., 2020): (1) the marine boundary layer is often well mixed at Cabo Verde and PNSDs and NCCN are similar both near sea level and at the cloud level; (2) during cloud events, larger particles are activated to cloud droplets.
Most INPs are in the supermicron size range at Cabo Verde. We found that about 70 % of INPs had a diameter of >1 µm at ice activation temperatures between −10 and −20 ∘C. Mason et al. (2016) and Creamean et al. (2018) also found that the majority of INPs is in the supermicron size range in the Arctic, in agreement with the results we obtained here.
Above we derived that NINP contributed from SSA only accounted for a minor fraction of total NINP in the air, as well as in the cloud water at Cabo Verde. This still holds even when considering a possible enrichment of INPs in SSA up to 102, which is an enrichment as given in literature for supermicron organic particles (Keene et al., 2007; Quinn et al., 2015). On the other hand, mineral dust is associated with a factor of 1000 higher ice surface site density (a measure to describe the ice activity per particle surface area), compared to SSA (Niemand et al., 2012; DeMott et al., 2016; McCluskey et al., 2018a). In our study, the supermicron particles that make up a large fraction of the INPs we observed were mainly mineral dust, as described in the accompanying study (Gong et al., 2020). The comparably high ice activity of supermicron mineral dust and the presence of mainly dust particles in the supermicron size range in our study again support that indeed most INPs observed in this study were not from sea spray. This is in line with results from Si et al. (2018) and Irish et al. (2019a), both done in the Arctic, where it was also concluded that SSA only contributed a little to the INP population. The commonality of these two studies from the Arctic and the present study is that land was still close enough, so that terrestrial sources can have contributed to the observed INPs.
While the above arguments suggest that INPs in our study were mostly mineral dust particles, there were also some measurements with comparably high INP concentrations at temperatures of −10 ∘C and above. Although it can not be ruled out that desert dust particles might be ice active at such high temperatures, by examining the reaction of some highly ice active samples to heating, described in Sect. 3.2.1, we found that the most highly ice active INPs on these samples were biological particles. It is an open question as to where these biological INPs originated. The times during which these highly ice active INPs were observed were times when air masses came from southern Europe, traveling along the African coast and meanwhile crossing over the region of the Canary Islands. Therefore, for these specific samples, a contribution of INPs from these land sources might be assumed.
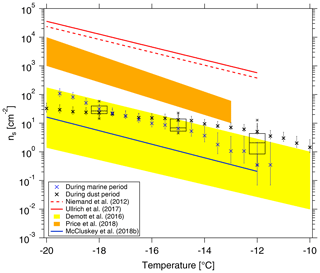
Figure 9Cumulative ns as a function of temperature in this study is shown by black boxes. The boxes represent the interquartile range. Whiskers represent 10th and 90th percentiles. Data not included between the whiskers are plotted as an outlier with a star. Two ns parameterizations (Niemand et al., 2012; Ullrich et al., 2017) for pure desert dust are shown in dashed and solid red lines, respectively. ns parameterizations from McCluskey et al. (2018b) for pristine SSA over the northeast Atlantic are shown as a solid blue line. We also make comparisons to recent data from airborne measurements in a dust layer by Price et al. (2018) in brown shading and from nascent laboratory-generated and ambient SSA by DeMott et al. (2016) in yellow shading, respectively. ns during the cleanest marine (CVAO 1585) and dustiest (CVAO 1591) periods are shown as blue and black crosses, respectively.
In the following, we will compare ns derived from our data with that from literature. In Fig. 9, we show the surface site density derived for NINP from CVAO PM10 filters (as shown by black boxes) following Niemand et al. (2012) (details on the surface area are given in the Supplement, Fig. S14), together with parameterizations for ns given by Niemand et al. (2012), Ullrich et al. (2017) and McCluskey et al. (2018b), and the measured ns given by DeMott et al. (2016) and Price et al. (2018). Niemand et al. (2012) derived ns from a laboratory study, based on aerosol consisting purely of desert dust particles. It is therefore reasonable that these mineral dust-related ns values are the largest values shown in Fig. 9, as they are purely related to the mineral dust surface area of an aerosol. All other values shown in Fig. 9 were derived for atmospheric measurements, and the surface area used to derive ns was always based on measured particle number concentrations. Price et al. (2018) carried out airborne measurements in dust-laden air over the tropical Atlantic. Parameterizations from McCluskey et al. (2018b) were done for pristine SSA over the northeast Atlantic, and both laboratory and atmospheric measurements of SSA were the base for the ns parameterization given in DeMott et al. (2016). These available ns parameterizations from previous literature may not be representative of Cabo Verde, but we will still compare them here. ns values derived for our study coincide with the upper range of parameterizations that are otherwise reported for SSA but are clearly lower than values reported for atmospheric desert dust aerosol. This is striking since, as discussed above, INPs observed in this study most likely do not originate from sea spray but are dominated by supermicron dust and/or biological particles.
CVAO is a place where marine and dust particles strongly intersect, and both particle types contribute to the surface area. In the companion paper, we have classified the aerosol at CVAO into four different types. Here, in addition to looking at average values as presented above, we selected the cleanest marine (CVAO 1585) and dustiest (CVAO 1591) samples for a separate calculation of ns, and we added the results to Fig. 9. The ns is clearly higher for the sample collected during the dusty period than during the marine period at higher temperatures (roughly ∘C). However, at temperatures below −18 ∘C it is the other way around. In general, results for these vastly different cases are both still close to the upper limit of the parameterizations reported for SSA.
These comparisons to literature raise the questions of if and how ns should be used to parameterize atmospheric INP measurements, which, however, is a question far too prominent to be answered in this study. In general, it is still an open issue as to what extent NINP can be parameterized, based on one or a few parameters, to reliably describe NINP for different locations around the globe. It might prove necessary to develop separate parameterizations for different locations or air masses, as it was already started for parameterizations based on particle number concentrations (see DeMott et al., 2010, 2015; Tobo et al., 2013).
The MarParCloud campaign took place in September and October 2018 on the islands of Cabo Verde to investigate aerosols prevailing in the Atlantic Ocean. In addition to a thorough analysis of the atmospheric aerosol particles and CCN in a companion paper (Gong et al., 2020), samples collected for INP analysis in this study include the following: sea surface microlayer (SML) and underlying water (ULW) from the ocean upwind of the island; quartz fiber filter samples of atmospheric aerosol, collected on a tower installed at the island shore and on a 744 m high mountaintop; and cloud water collected during cloud events on the mountaintop. NINP values were measured offline with two types of freezing devices, yielding results in the temperature range from roughly −5 to −25 ∘C.
Both enrichment and depletion of NINP in SML to ULW were observed. The enrichment factors (EF) varied from 0.36 to 11.40 and from 0.36 to 7.11 at −15 and −20 ∘C, respectively, and they were generally independent of the freezing temperature at which NINP was determined in the freezing devices.
A few CVAO PM10 filter samples (CVAO 1596, CVAO 1641 and CVAO 1643) showed elevated NINP at high temperatures, e.g., above 0.01 L−1 at −10 ∘C. These elevated values disappeared after heating the samples at 95 ∘C for 1 h. Therefore, biological particles appear to contribute to INPs at these moderate supercooling temperatures. About 83±22 %, 67±18 % and 77±14 % (median ± standard deviation) of INPs had a diameter >1 µm at ice activation temperatures of −12, −15 and −18 ∘C, respectively; over the whole examined temperature range, on average roughly 70 % of all INPs were supermicron, independent of the temperature. The highly ice active INPs were not found on the CVAO PM1 filters, which suggests that most of these likely biological INPs are in the supermicron size range.
As MV was in clouds most of the time, only two filters could be collected on MV that were affected by cloud for less than 10 % of the sampling time. For these, NINP values were similar at CVAO and MV. During cloud events, most INPs at MV were activated into cloud droplets. These findings aligned very well with the companion paper, i.e., during noncloud events, PNSDs and NCCN are similar at CVAO and MV, while during cloud events larger particles at MV are activated to clouds (see Fig. 8 in the companion paper, Gong et al., 2020). When highly ice active particles were present on CVAO PM10 filters, they were not observed on MV PM10 filters, but they were instead observed in the respective cloud water samples. This shows that these INPs are activated into cloud droplets during cloud events.
By comparing NINP values derived for the different examined samples, it was found that values in air and in cloud water agreed well. We also compared atmospheric NINP to those in SML and ULW, based on the ratio of sodium chloride concentrations measured for the atmosphere and for SML and ULW. From that we concluded that marine INPs from sea spray can only explain a small fraction of all atmospheric INPs at Cabo Verde, unless there would be an enrichment of INPs from SML to the atmosphere by at least a factor of 104. Such an enrichment, however, is higher than anything observed for organic compounds in supermicron particles so far. Summarizing, it can be assumed that most atmospheric INPs detected in the present study were mainly contributed by the dust particles at cold temperatures and possibly with a few contributions from biological particles at warmer temperatures.
The data are available through the World Data Center PANGAEA (https://doi.org/10.1594/PANGAEA.906946; Gong et al., 2019b).
The supplement related to this article is available online at: https://doi.org/10.5194/acp-20-1451-2020-supplement.
XG wrote the article with contributions from HW and MvP. CS, NT and BR collected ocean water samples. XG, MvP and NT collected filter samples. KWF collected cloud water samples. XG and JL performed INP measurements. XG preformed data evaluation. XG, HW and FS discussed the results and further analysis after the campaign. All co-authors proofread and commented on the article.
The authors declare that they have no conflict of interest.
This article is part of the special issue “Marine organic matter: from biological production in the ocean to organic aerosol particles and marine clouds (ACP/OS inter-journal SI)”. It is not associated with a conference.
The works were carried out in the framework of the MarParCloud project. The authors acknowledge the Leibniz Association SAW funding for the project “Marine biological production, organic aerosol particles and marine clouds: a Process Chain (MarParCloud)”, SAW-2016-TROPOS-2.
This research has been supported by the Leibniz Association SAW (MarParCloud (grant no. SAW-2016-TROPOS-2)).
The publication of this article was funded by the
Open Access Fund of the Leibniz Association.
This paper was edited by Paul Zieger and reviewed by two anonymous referees.
Agogué, H., Casamayor, E. O., Joux, F., Obernosterer, I., Dupuy, C., Lantoine, F., Catala, P., Weinbauer, M. G., Reinthaler, T., Herndl, G. J., and Lebaron, P.: Comparison of samplers for the biological characterization of the sea surface microlayer, Limnol. Oceanogr.-Meth., 2, 213–225, https://doi.org/10.4319/lom.2004.2.213, 2004. a
Agresti, A. and Coull, B. A.: Approximate is Better than “Exact” for Interval Estimation of Binomial Proportions, Am. Stat., 52, 119–126, https://doi.org/10.1080/00031305.1998.10480550, 1998. a
Aller, J. Y., Radway, J. C., Kilthau, W. P., Bothe, D. W., Wilson, T. W., Vaillancourt, R. D., Quinn, P. K., Coffman, D. J., Murray, B. J., and Knopf, D. A.: Size-resolved characterization of the polysaccharidic and proteinaceous components of sea spray aerosol, Atmos. Environ., 154, 331–347, https://doi.org/10.1016/j.atmosenv.2017.01.053, 2017. a
Ansmann, A., Tesche, M., Althausen, D., Müller, D., Seifert, P., Freudenthaler, V., Heese, B., Wiegner, M., Pisani, G., Knippertz, P., and Dubovik, O.: Influence of Saharan dust on cloud glaciation in southern Morocco during the Saharan Mineral Dust Experiment, J. Geophys. Res.-Atmos., 113, D04210, https://doi.org/10.1029/2007jd008785, 2008. a
Atkinson, J. D., Murray, B. J., Woodhouse, M. T., Whale, T. F., Baustian, K. J., Carslaw, K. S., Dobbie, S., O'Sullivan, D., and Malkin, T. L.: The importance of feldspar for ice nucleation by mineral dust in mixed-phase clouds, Nature, 498, 355, https://doi.org/10.1038/nature12278, 2013. a
Augustin, S., Wex, H., Niedermeier, D., Pummer, B., Grothe, H., Hartmann, S., Tomsche, L., Clauss, T., Voigtländer, J., Ignatius, K., and Stratmann, F.: Immersion freezing of birch pollen washing water, Atmos. Chem. Phys., 13, 10989–11003, https://doi.org/10.5194/acp-13-10989-2013, 2013. a
Augustin-Bauditz, S., Wex, H., Kanter, S., Ebert, M., Niedermeier, D., Stolz, F., Prager, A., and Stratmann, F.: The immersion mode ice nucleation behavior of mineral dusts: A comparison of different pure and surface modified dusts, Geophys. Res. Lett., 41, 7375–7382, https://doi.org/10.1002/2014gl061317, 2014. a, b
Bigg, E. K.: Ice Nucleus Concentrations in Remote Areas, J. Atmos. Sci., 30, 1153–1157, https://doi.org/10.1175/1520-0469(1973)030<1153:INCIRA>2.0.CO;2, 1973. a, b
Boose, Y., Welti, A., Atkinson, J., Ramelli, F., Danielczok, A., Bingemer, H. G., Plötze, M., Sierau, B., Kanji, Z. A., and Lohmann, U.: Heterogeneous ice nucleation on dust particles sourced from nine deserts worldwide – Part 1: Immersion freezing, Atmos. Chem. Phys., 16, 15075–15095, https://doi.org/10.5194/acp-16-15075-2016, 2016. a
Bréon, F.-M., Tanré, D., and Generoso, S.: Aerosol Effect on Cloud Droplet Size Monitored from Satellite, Science, 295, 834–838, https://doi.org/10.1126/science.1066434, 2002. a
Brier, G. W. and Kline, D. B.: Ocean Water as a Source of Ice Nuclei, Science, 130, 717–718, https://doi.org/10.1126/science.130.3377.717, 1959. a
Budke, C. and Koop, T.: BINARY: an optical freezing array for assessing temperature and time dependence of heterogeneous ice nucleation, Atmos. Meas. Tech., 8, 689–703, https://doi.org/10.5194/amt-8-689-2015, 2015. a
Burrows, S. M., Hoose, C., Pöschl, U., and Lawrence, M. G.: Ice nuclei in marine air: biogenic particles or dust?, Atmos. Chem. Phys., 13, 245–267, https://doi.org/10.5194/acp-13-245-2013, 2013. a
Chen, J., Wu, Z., Augustin-Bauditz, S., Grawe, S., Hartmann, M., Pei, X., Liu, Z., Ji, D., and Wex, H.: Ice-nucleating particle concentrations unaffected by urban air pollution in Beijing, China, Atmos. Chem. Phys., 18, 3523–3539, https://doi.org/10.5194/acp-18-3523-2018, 2018. a, b
Chou, C., Stetzer, O., Weingartner, E., Jurányi, Z., Kanji, Z. A., and Lohmann, U.: Ice nuclei properties within a Saharan dust event at the Jungfraujoch in the Swiss Alps, Atmos. Chem. Phys., 11, 4725–4738, https://doi.org/10.5194/acp-11-4725-2011, 2011. a
Conen, F., Henne, S., Morris, C. E., and Alewell, C.: Atmospheric ice nucleators active ∘C can be quantified on PM10 filters, Atmos. Meas. Tech., 5, 321–327, https://doi.org/10.5194/amt-5-321-2012, 2012. a
Conen, F., Eckhardt, S., Gundersen, H., Stohl, A., and Yttri, K. E.: Rainfall drives atmospheric ice-nucleating particles in the coastal climate of southern Norway, Atmos. Chem. Phys., 17, 11065–11073, https://doi.org/10.5194/acp-17-11065-2017, 2017. a
Cornwell, G. C., McCluskey, C. S., Levin, E. J. T., Suski, K. J., DeMott, P. J., Kreidenweis, S. M., and Prather, K. A.: Direct Online Mass Spectrometry Measurements of Ice Nucleating Particles at a California Coastal Site, J. Geophys. Res.-Atmos., 124, 12157–12172, https://doi.org/10.1029/2019jd030466, 2019. a
Creamean, J. M., Kirpes, R. M., Pratt, K. A., Spada, N. J., Maahn, M., de Boer, G., Schnell, R. C., and China, S.: Marine and terrestrial influences on ice nucleating particles during continuous springtime measurements in an Arctic oilfield location, Atmos. Chem. Phys., 18, 18023–18042, https://doi.org/10.5194/acp-18-18023-2018, 2018. a, b, c
DeMott, P. J., Sassen, K., Poellot, M. R., Baumgardner, D., Rogers, D. C., Brooks, S. D., Prenni, A. J., and Kreidenweis, S. M.: African dust aerosols as atmospheric ice nuclei, Geophys. Res. Lett., 30, 1732, https://doi.org/10.1029/2003GL017410, 2003. a
DeMott, P. J., Prenni, A. J., Liu, X., Kreidenweis, S. M., Petters, M. D., Twohy, C. H., Richardson, M. S., Eidhammer, T., and Rogers, D. C.: Predicting global atmospheric ice nuclei distributions and their impacts on climate, P. Natl. Acad. Sci. USA, 107, 11217–11222, https://doi.org/10.1073/pnas.0910818107, 2010. a, b
DeMott, P. J., Prenni, A. J., McMeeking, G. R., Sullivan, R. C., Petters, M. D., Tobo, Y., Niemand, M., Möhler, O., Snider, J. R., Wang, Z., and Kreidenweis, S. M.: Integrating laboratory and field data to quantify the immersion freezing ice nucleation activity of mineral dust particles, Atmos. Chem. Phys., 15, 393–409, https://doi.org/10.5194/acp-15-393-2015, 2015 a, b, c
DeMott, P. J., Hill, T. C. J., McCluskey, C. S., Prather, K. A., Collins, D. B., Sullivan, R. C., Ruppel, M. J., Mason, R. H., Irish, V. E., Lee, T., Hwang, C. Y., Rhee, T. S., Snider, J. R., McMeeking, G. R., Dhaniyala, S., Lewis, E. R., Wentzell, J. J. B., Abbatt, J., Lee, C., Sultana, C. M., Ault, A. P., Axson, J. L., Diaz Martinez, M., Venero, I., Santos-Figueroa, G., Stokes, M. D., Deane, G. B., Mayol-Bracero, O. L., Grassian, V. H., Bertram, T. H., Bertram, A. K., Moffett, B. F., and Franc, G. D.: Sea spray aerosol as a unique source of ice nucleating particles, P. Natl. Acad. Sci. USA, 113, 5797–5803, https://doi.org/10.1073/pnas.1514034112, 2016. a, b, c, d, e, f
Demoz, B. B., Collett, J. L., and Daube, B. C.: On the Caltech Active Strand Cloudwater Collectors, Atmos. Res., 41, 47–62, https://doi.org/10.1016/0169-8095(95)00044-5, 1996. a
Gioda, A., Mayol-Bracero, O. L., Morales-García, F., Collett, J., Decesari, S., Emblico, L., Facchini, M. C., Morales-De Jesús, R. J., Mertes, S., Borrmann, S., Walter, S., and Schneider, J.: Chemical Composition of Cloud Water in the Puerto Rican Tropical Trade Wind Cumuli, Water Air Soil Poll., 200, 3–14, https://doi.org/10.1007/s11270-008-9888-4, 2009. a
Gong, X., Wex, H., Müller, T., Wiedensohler, A., Höhler, K., Kandler, K., Ma, N., Dietel, B., Schiebel, T., Möhler, O., and Stratmann, F.: Characterization of aerosol properties at Cyprus, focusing on cloud condensation nuclei and ice-nucleating particles, Atmos. Chem. Phys., 19, 10883–10900, https://doi.org/10.5194/acp-19-10883-2019, 2019a. a, b, c
Gong, X., Wex, H., van Pinxteren, M., Triesch, N., Fomba, K. W., Lubitz, J., Stolle, C., Robinson, T.-B., Müller, T., Herrmann, H., and Stratmann, F.: Ice nucleating particles measured in air, cloud and seawater at the Cape Verde Atmospheric Observatory (CVAO), PANGAEA, https://doi.org/10.1594/PANGAEA.906946, 2019b. a
Gong, X., Wex, H., Voigtländer, J., Fomba, K. W., Weinhold, K., van Pinxteren, M., Henning, S., Müller, T., Herrmann, H., and Stratmann, F.: Characterization of aerosol particles at Cabo Verde close to sea level and at the cloud level – Part 1: Particle number size distribution, cloud condensation nuclei and their origins, Atmos. Chem. Phys., 20, 1431–1449, https://doi.org/10.5194/acp-20-1431-2020, 2020. a, b, c, d, e, f, g, h, i, j, k, l
Hartmann, M., Blunier, T., Brügger, S., Schmale, J., Schwikowski, M., Vogel, A., Wex, H., and Stratmann, F.: Variation of Ice Nucleating Particles in the European Arctic Over the Last Centuries, Geophys. Res. Lett., 46, 4007–4016, https://doi.org/10.1029/2019gl082311, 2019. a
Hartmann, S., Augustin, S., Clauss, T., Wex, H., Šantl-Temkiv, T., Voigtländer, J., Niedermeier, D., and Stratmann, F.: Immersion freezing of ice nucleation active protein complexes, Atmos. Chem. Phys., 13, 5751–5766, https://doi.org/10.5194/acp-13-5751-2013, 2013. a
Harvey, G. W. and Burzell, L. A.: A simple microlayer method for small samples, Limnol. Oceanogr., 17, 156–157, https://doi.org/10.4319/lo.1972.17.1.0156, 1972. a
Hoose, C. and Möhler, O.: Heterogeneous ice nucleation on atmospheric aerosols: a review of results from laboratory experiments, Atmos. Chem. Phys., 12, 9817–9854, https://doi.org/10.5194/acp-12-9817-2012, 2012. a, b
Huffman, J. A., Prenni, A. J., DeMott, P. J., Pöhlker, C., Mason, R. H., Robinson, N. H., Fröhlich-Nowoisky, J., Tobo, Y., Després, V. R., Garcia, E., Gochis, D. J., Harris, E., Müller-Germann, I., Ruzene, C., Schmer, B., Sinha, B., Day, D. A., Andreae, M. O., Jimenez, J. L., Gallagher, M., Kreidenweis, S. M., Bertram, A. K., and Pöschl, U.: High concentrations of biological aerosol particles and ice nuclei during and after rain, Atmos. Chem. Phys., 13, 6151–6164, https://doi.org/10.5194/acp-13-6151-2013, 2013. a
Igel, A. L. and Heever, S. C. v. d.: The Importance of the Shape of Cloud Droplet Size Distributions in Shallow Cumulus Clouds. Part II: Bulk Microphysics Simulations, J. Atmos. Sci., 74, 259–273, https://doi.org/10.1175/jas-d-15-0383.1, 2017. a
Irish, V. E., Elizondo, P., Chen, J., Chou, C., Charette, J., Lizotte, M., Ladino, L. A., Wilson, T. W., Gosselin, M., Murray, B. J., Polishchuk, E., Abbatt, J. P. D., Miller, L. A., and Bertram, A. K.: Ice-nucleating particles in Canadian Arctic sea-surface microlayer and bulk seawater, Atmos. Chem. Phys., 17, 10583–10595, https://doi.org/10.5194/acp-17-10583-2017, 2017. a, b, c, d
Irish, V. E., Hanna, S. J., Willis, M. D., China, S., Thomas, J. L., Wentzell, J. J. B., Cirisan, A., Si, M., Leaitch, W. R., Murphy, J. G., Abbatt, J. P. D., Laskin, A., Girard, E., and Bertram, A. K.: Ice nucleating particles in the marine boundary layer in the Canadian Arctic during summer 2014, Atmos. Chem. Phys., 19, 1027–1039, https://doi.org/10.5194/acp-19-1027-2019, 2019a. a
Irish, V. E., Hanna, S. J., Xi, Y., Boyer, M., Polishchuk, E., Ahmed, M., Chen, J., Abbatt, J. P. D., Gosselin, M., Chang, R., Miller, L. A., and Bertram, A. K.: Revisiting properties and concentrations of ice-nucleating particles in the sea surface microlayer and bulk seawater in the Canadian Arctic during summer, Atmos. Chem. Phys., 19, 7775–7787, https://doi.org/10.5194/acp-19-7775-2019, 2019b. a, b, c
Joly, M., Amato, P., Deguillaume, L., Monier, M., Hoose, C., and Delort, A.-M.: Quantification of ice nuclei active at near 0 ∘C temperatures in low-altitude clouds at the Puy de Dôme atmospheric station, Atmos. Chem. Phys., 14, 8185–8195, https://doi.org/10.5194/acp-14-8185-2014, 2014. a, b, c, d
Kanji, Z. A., Ladino, L. A., Wex, H., Boose, Y., Burkert-Kohn, M., Cziczo, D. J., and Krämer, M.: Overview of Ice Nucleating Particles, Meteorol. Mon., 58, 1.1–1.33, https://doi.org/10.1175/amsmonographs-d-16-0006.1, 2017. a
Keene, W. C., Maring, H., Maben, J. R., Kieber, D. J., Pszenny, A. A. P., Dahl, E. E., Izaguirre, M. A., Davis, A. J., Long, M. S., Zhou, X., Smoydzin, L., and Sander, R.: Chemical and physical characteristics of nascent aerosols produced by bursting bubbles at a model air-sea interface, J. Geophys. Res.-Atmos., 112, D21202, https://doi.org/10.1029/2007jd008464, 2007. a, b, c
Koop, T. and Zobrist, B.: Parameterizations for ice nucleation in biological and atmospheric systems, Phys. Chem. Chem. Phys., 11, 10839–10850, https://doi.org/10.1039/B914289D, 2009. a
Kreidenweis, S. M., Koehler, K., DeMott, P. J., Prenni, A. J., Carrico, C., and Ervens, B.: Water activity and activation diameters from hygroscopicity data – Part I: Theory and application to inorganic salts, Atmos. Chem. Phys., 5, 1357–1370, https://doi.org/10.5194/acp-5-1357-2005, 2005. a
Mason, R. H., Si, M., Chou, C., Irish, V. E., Dickie, R., Elizondo, P., Wong, R., Brintnell, M., Elsasser, M., Lassar, W. M., Pierce, K. M., Leaitch, W. R., MacDonald, A. M., Platt, A., Toom-Sauntry, D., Sarda-Estève, R., Schiller, C. L., Suski, K. J., Hill, T. C. J., Abbatt, J. P. D., Huffman, J. A., DeMott, P. J., and Bertram, A. K.: Size-resolved measurements of ice-nucleating particles at six locations in North America and one in Europe, Atmos. Chem. Phys., 16, 1637–1651, https://doi.org/10.5194/acp-16-1637-2016, 2016. a, b, c
McCluskey, C. S., Hill, T. C. J., Humphries, R. S., Rauker, A. M., Moreau, S., Strutton, P. G., Chambers, S. D., Williams, A. G., McRobert, I., Ward, J., Keywood, M. D., Harnwell, J., Ponsonby, W., Loh, Z. M., Krummel, P. B., Protat, A., Kreidenweis, S. M., and DeMott, P. J.: Observations of Ice Nucleating Particles Over Southern Ocean Waters, Geophys. Res. Lett., 45, 11989–11997, https://doi.org/10.1029/2018GL079981, 2018a. a, b, c, d, e, f
McCluskey, C. S., Ovadnevaite, J., Rinaldi, M., Atkinson, J., Belosi, F., Ceburnis, D., Marullo, S., Hill, T. C. J., Lohmann, U., Kanji, Z. A., O'Dowd, C., Kreidenweis, S. M., and DeMott, P. J.: Marine and Terrestrial Organic Ice-Nucleating Particles in Pristine Marine to Continentally Influenced Northeast Atlantic Air Masses, J. Geophys. Res.-Atmos., 123, 6196–6212, https://doi.org/10.1029/2017JD028033, 2018b. a, b, c, d, e, f, g
Mertes, S., Verheggen, B., Walter, S., Connolly, P., Ebert, M., Schneider, J., Bower, K. N., Cozic, J., Weinbruch, S., Baltensperger, U., and Weingartner, E.: Counterflow Virtual Impactor Based Collection of Small Ice Particles in Mixed-Phase Clouds for the Physico-Chemical Characterization of Tropospheric Ice Nuclei: Sampler Description and First Case Study, Aerosol Sci. Tech., 41, 848–864, https://doi.org/10.1080/02786820701501881, 2007. a
Miles, N. L., Verlinde, J., and Clothiaux, E. E.: Cloud Droplet Size Distributions in Low-Level Stratiform Clouds, J. Atmos. Sci., 57, 295–311, https://doi.org/10.1175/1520-0469(2000)057<0295:cdsdil>2.0.co;2, 2000. a
Murray, B. J., O'Sullivan, D., Atkinson, J. D., and Webb, M. E.: Ice nucleation by particles immersed in supercooled cloud droplets, Chem. Soc. Rev., 41, 6519–6554, https://doi.org/10.1039/C2CS35200A, 2012. a, b
Niedermeier, D., Augustin-Bauditz, S., Hartmann, S., Wex, H., Ignatius, K., and Stratmann, F.: Can we define an asymptotic value for the ice active surface site density for heterogeneous ice nucleation?, J. Geophys. Res.-Atmos., 120, 5036–5046, https://doi.org/10.1002/2014JD022814, 2015. a
Niemand, M., Möhler, O., Vogel, B., Vogel, H., Hoose, C., Connolly, P., Klein, H., Bingemer, H., DeMott, P., Skrotzki, J., and Leisner, T.: A Particle-Surface-Area-Based Parameterization of Immersion Freezing on Desert Dust Particles, J. Atmos. Sci., 69, 3077–3092, https://doi.org/10.1175/jas-d-11-0249.1, 2012. a, b, c, d, e, f, g
O'Sullivan, D., Murray, B. J., Ross, J. F., and Webb, M. E.: The adsorption of fungal ice-nucleating proteins on mineral dusts: a terrestrial reservoir of atmospheric ice-nucleating particles, Atmos. Chem. Phys., 16, 7879–7887, https://doi.org/10.5194/acp-16-7879-2016, 2016. a
O'Sullivan, D., Adams, M. P., Tarn, M. D., Harrison, A. D., Vergara-Temprado, J., Porter, G. C. E., Holden, M. A., Sanchez-Marroquin, A., Carotenuto, F., Whale, T. F., McQuaid, J. B., Walshaw, R., Hedges, D. H. P., Burke, I. T., Cui, Z., and Murray, B. J.: Contributions of biogenic material to the atmospheric ice-nucleating particle population in North Western Europe, Sci. Rep.-UK, 8, 13821, https://doi.org/10.1038/s41598-018-31981-7, 2018. a, b, c
Petters, M. and Wright, T.: Revisiting ice nucleation from precipitation samples, Geophys. Res. Lett., 42, 8758–8766, https://doi.org/10.1002/2015GL065733, 2015. a, b
Price, H. C., Baustian, K. J., McQuaid, J. B., Blyth, A., Bower, K. N., Choularton, T., Cotton, R. J., Cui, Z., Field, P. R., Gallagher, M., Hawker, R., Merrington, A., Miltenberger, A., Neely III, R. R., Parker, S. T., Rosenberg, P. D., Taylor, J. W., Trembath, J., Vergara-Temprado, J., Whale, T. F., Wilson, T. W., Young, G., and Murray, B. J.: Atmospheric Ice-Nucleating Particles in the Dusty Tropical Atlantic, J. Geophys. Res.-Atmos., 123, 2175–2193, https://doi.org/10.1002/2017JD027560, 2018. a, b, c, d
Pruppacher, H. and Klett, J.: Microphysics of Clouds and Precipitation, Vol. 18, Springer Science & Business Media, D. Reidel, Dordrecht, 2010. a
Pummer, B. G., Budke, C., Augustin-Bauditz, S., Niedermeier, D., Felgitsch, L., Kampf, C. J., Huber, R. G., Liedl, K. R., Loerting, T., Moschen, T., Schauperl, M., Tollinger, M., Morris, C. E., Wex, H., Grothe, H., Pöschl, U., Koop, T., and Fröhlich-Nowoisky, J.: Ice nucleation by water-soluble macromolecules, Atmos. Chem. Phys., 15, 4077–4091, https://doi.org/10.5194/acp-15-4077-2015, 2015. a
Quinn, P. K., Collins, D. B., Grassian, V. H., Prather, K. A., and Bates, T. S.: Chemistry and Related Properties of Freshly Emitted Sea Spray Aerosol, Chem. Rev., 115, 4383–4399, https://doi.org/10.1021/cr500713g, 2015. a, b
Rangno, A. L. and Hobbs, P. V.: Microstructures and precipitation development in cumulus and small cumulonimbus clouds over the warm pool of the tropical Pacific Ocean, Q. J. Roy. Meteor. Soc., 131, 639–673, https://doi.org/10.1256/qj.04.13, 2005. a
Robinson, T.-B., Stolle, C., and Wurl, O.: Depth is relative: the importance of depth for transparent exopolymer particles in the near-surface environment, Ocean Sci., 15, 1653–1666, https://doi.org/10.5194/os-15-1653-2019, 2019. a
Schnell, R. C.: Ice Nuclei in Seawater, Fog Water and Marine Air off the Coast of Nova Scotia: Summer 1975, J. Atmos. Sci., 34, 1299–1305, https://doi.org/10.1175/1520-0469(1977)034<1299:INISFW>2.0.CO;2, 1977. a
Schnell, R. C. and Vali, G.: Biogenic Ice Nuclei: Part I. Terrestrial and Marine Sources, J. Atmos. Sci., 33, 1554–1564, https://doi.org/10.1175/1520-0469(1976)033<1554:BINPIT>2.0.CO;2, 1976. a, b
Schrod, J., Weber, D., Drücke, J., Keleshis, C., Pikridas, M., Ebert, M., Cvetković, B., Nickovic, S., Marinou, E., Baars, H., Ansmann, A., Vrekoussis, M., Mihalopoulos, N., Sciare, J., Curtius, J., and Bingemer, H. G.: Ice nucleating particles over the Eastern Mediterranean measured by unmanned aircraft systems, Atmos. Chem. Phys., 17, 4817–4835, https://doi.org/10.5194/acp-17-4817-2017, 2017. a
Si, M., Irish, V. E., Mason, R. H., Vergara-Temprado, J., Hanna, S. J., Ladino, L. A., Yakobi-Hancock, J. D., Schiller, C. L., Wentzell, J. J. B., Abbatt, J. P. D., Carslaw, K. S., Murray, B. J., and Bertram, A. K.: Ice-nucleating ability of aerosol particles and possible sources at three coastal marine sites, Atmos. Chem. Phys., 18, 15669–15685, https://doi.org/10.5194/acp-18-15669-2018, 2018. a
Siebert, H. and Shaw, R. A.: Supersaturation Fluctuations during the Early Stage of Cumulus Formation, J. Atmos. Sci., 74, 975–988, https://doi.org/10.1175/jas-d-16-0115.1, 2017. a, b
Suski, K. J., Hill, T. C. J., Levin, E. J. T., Miller, A., DeMott, P. J., and Kreidenweis, S. M.: Agricultural harvesting emissions of ice-nucleating particles, Atmos. Chem. Phys., 18, 13755–13771, https://doi.org/10.5194/acp-18-13755-2018, 2018. a
Tobo, Y., Prenni, A. J., DeMott, P. J., Huffman, J. A., McCluskey, C. S., Tian, G., Pöhlker, C., Pöschl, U., and Kreidenweis, S. M.: Biological aerosol particles as a key determinant of ice nuclei populations in a forest ecosystem, J. Geophys. Res.-Atmos., 118, 10100–10110, https://doi.org/10.1002/jgrd.50801, 2013. a
Ullrich, R., Hoose, C., Möhler, O., Niemand, M., Wagner, R., Höhler, K., Hiranuma, N., Saathoff, H., and Leisner, T.: A New Ice Nucleation Active Site Parameterization for Desert Dust and Soot, J. Atmos. Sci., 74, 699–717, https://doi.org/10.1175/JAS-D-16-0074.1, 2017. a, b, c
Vali, G.: Sizes of Atmospheric Ice Nuclei, Nature, 212, 384–385, https://doi.org/10.1038/212384a0, 1966. a
Vali, G.: Quantitative Evaluation of Experimental Results an the Heterogeneous Freezing Nucleation of Supercooled Liquids, J. Atmos. Sci., 28, 402–409, https://doi.org/10.1175/1520-0469(1971)028<0402:qeoera>2.0.co;2, 1971. a
Vali, G., DeMott, P. J., Möhler, O., and Whale, T. F.: Technical Note: A proposal for ice nucleation terminology, Atmos. Chem. Phys., 15, 10263–10270, https://doi.org/10.5194/acp-15-10263-2015, 2015. a
van Pinxteren, M., Barthel, S., Fomba, K. W., Müller, K., Von Tümpling, W., and Herrmann, H.: The influence of environmental drivers on the enrichment of organic carbon in the sea surface microlayer and in submicron aerosol particles–measurements from the Atlantic Ocean, Elementa, 5, 35, https://doi.org/10.1525/elementa.225, 2017. a, b
van Pinxteren, M., Fomba, K. W., Triesch, N., Stolle, C., Wurl, O., Bahlmann, E., Gong, X., Voigtländer, J., Wex, H., Robinson, T.-B., Barthel, S., Zeppenfeld, S., Hoffmann, E. H., Roveretto, M., Li, C., Grosselin, B., Daële, V., Senf, F., van Pinxteren, D., Manzi, M., Zabalegui, N., Frka, S., Gašparović, B., Pereira, R., Li, T., Wen, L., Li, J., Zhu, C., Chen, H., Chen, J., Fiedler, B., von Tümpling, W., Read, K. A., Punjabi, S., C. Lewis, A. C., Hopkins, J. R., Carpenter, L. J., Peeken, I., Rixen, T., Schulz-Bull, D., Monge, M. E., Mellouki, A., George, C., Stratmann, F., and Herrmann, H.: Marine organic matter in the remote environment of the Cape Verde Islands – An introduction and overview to the MarParCloud campaign, Atmos. Chem. Phys. Discuss., https://doi.org/10.5194/acp-2019-997, in review, 2019. a
Welti, A., Müller, K., Fleming, Z. L., and Stratmann, F.: Concentration and variability of ice nuclei in the subtropical maritime boundary layer, Atmos. Chem. Phys., 18, 5307–5320, https://doi.org/10.5194/acp-18-5307-2018, 2018. a, b, c
Westbrook, C. D. and Illingworth, A. J.: The formation of ice in a long-lived supercooled layer cloud, Q. J. Roy. Meteor. Soc., 139, 2209–2221, https://doi.org/10.1002/qj.2096, 2013. a
Wex, H., Huang, L., Zhang, W., Hung, H., Traversi, R., Becagli, S., Sheesley, R. J., Moffett, C. E., Barrett, T. E., Bossi, R., Skov, H., Hünerbein, A., Lubitz, J., Löffler, M., Linke, O., Hartmann, M., Herenz, P., and Stratmann, F.: Annual variability of ice-nucleating particle concentrations at different Arctic locations, Atmos. Chem. Phys., 19, 5293–5311, https://doi.org/10.5194/acp-19-5293-2019, 2019. a
Wilson, T. W., Ladino, L. A., Alpert, P. A., Breckels, M. N., Brooks, I. M., Browse, J., Burrows, S. M., Carslaw, K. S., Huffman, J. A., Judd, C., Kilthau, W. P., Mason, R. H., McFiggans, G., Miller, L. A., Najera, J. J., Polishchuk, E., Rae, S., Schiller, C. L., Si, M., Temprado, J. V., Whale, T. F., Wong, J. P. S., Wurl, O., Yakobi-Hancock, J. D., Abbatt, J. P. D., Aller, J. Y., Bertram, A. K., Knopf, D. A., and Murray, B. J.: A marine biogenic source of atmospheric ice-nucleating particles, Nature, 525, 234–238, https://doi.org/10.1038/nature14986, 2015. a, b, c, d, e, f, g, h, i, j, k, l