the Creative Commons Attribution 4.0 License.
the Creative Commons Attribution 4.0 License.
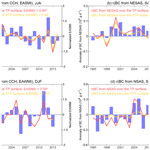
Impacts of atmospheric transport and biomass burning on the inter-annual variation in black carbon aerosols over the Tibetan Plateau
Yue Wu
Tianliang Zhao
Bingliang Zhuang
Honglei Wang
Yichen Li
Huimin Chen
Ye Zhu
Hongnian Liu
Qin'geng Wang
Shu Li
Tijian Wang
Mengmeng Li
Atmospheric black carbon (BC) in the Tibetan Plateau (TP) can largely impact regional and global climate. Still, studies on the inter-annual variation in atmospheric BC over the TP and associated variation in BC sources and controlling factors are rather limited. In this study, we characterize the variations in atmospheric BC over the TP surface layer through analysis of 20-year (1995–2014) simulations from a global chemical transport model, GEOS-Chem. The results show that surface BC concentrations over the TP vary largely in space and by season, reflecting complicated interplays of BC sources from different origins. Of all areas in the TP, surface BC concentrations are highest over the eastern and southern TP, where surface BC is susceptible to BC transport from East Asia and South Asia, respectively. Applying a backward-trajectory method that combines BC concentrations from GEOS-Chem and trajectories from the Hybrid Single-Particle Lagrangian Integrated Trajectory (HYSPLIT) model, we assess the contributions of worldwide source regions to surface BC in the TP. We estimate that on the 20-year average, 77 % of surface BC in the TP comes from South Asia (43 %) and East Asia (35 %). Regarding seasonal variation in non-local influences, South Asia and East Asia are dominant source regions in winter and summer, respectively, in terms of the amount of BC imported. However, in terms of affected areas in the TP, South Asia is the dominant contributor throughout the year. Inter-annually, surface BC over the TP is largely modulated by atmospheric transport of BC from non-local regions year-round and by biomass burning in South Asia, mostly in spring. We find that the extremely strong biomass burning in South Asia in the spring of 1999 greatly enhanced surface BC concentrations in the TP (31 % relative to the climatology). We find that the strength of the Asian monsoon correlates significantly with the inter-annual variation in the amount of BC transported to the TP from non-local regions. In summer, a stronger East Asian summer monsoon and a stronger South Asian summer monsoon tend to, respectively, lead to more BC transport from central China and north-eastern South Asia to the TP. In winter, BC transport from central China is enhanced in years with a strong East Asian winter monsoon or a strong Siberian High. A stronger Siberian High can also bring more BC from northern South Asia to the TP. This study underscores the impacts of atmospheric transport and biomass burning on the inter-annual variation in surface BC over the TP. It reveals a close connection between the Asian monsoon and atmospheric transport of BC from non-local regions to the TP.
- Article
(10519 KB) - Full-text XML
-
Supplement
(844 KB) - BibTeX
- EndNote
Black carbon (BC) is a carbonaceous aerosol formed from combustion of carbon-based fuels and materials. BC in the atmosphere is a major air pollutant and a strong absorber of solar radiation (Bond et al., 2013). Atmospheric BC can greatly influence regional (Ramanathan and Carmichael, 2008; Zhuang et al., 2018) and global (Allen et al., 2012; Chung et al., 2012) climate through multiple mechanisms. It can cause atmospheric heating (Cappa et al., 2012) and surface dimming (Flanner et al., 2009) and influence cloud formation and development processes (Jacobson et al., 2012). Furthermore, after its deposition on snow or ice, BC reduces the surface albedo and accelerates the melting of glaciers and snow cover (Hansen and Nazarenko, 2004; Flanner et al., 2007).
The Tibetan Plateau (TP) has an average altitude of over 4 km and an area of 2.6 × 106 km2, known as the Third Pole. Because of its special geography, the TP can greatly impact regional and global climate through dynamic and thermal processes (Wu et al., 2015; Li et al., 2018). The TP has a large number of glaciers (over 0.1 × 106 km2) and a wide coverage of snow (59 % of the TP in winter; Qin et al., 2006; Yao et al., 2012). Although atmospheric BC in the TP is among the lowest in the world, BC there can alter the climate (Lau et al., 2010; Jiang et al., 2017), ecosystem (Kang et al., 2019), and hydrology (Barnett et al., 2005) in the TP, consequently influencing the living environment of billions of people in the world. Atmospheric BC is an important factor driving the surface warming in the TP due to its strong absorption of solar radiation (He et al., 2014a). After its deposition to the TP ground, BC in the snow reduces the surface albedo (Ming et al., 2009; Qian et al., 2011; Qu et al., 2014). He et al. (2014a) suggested that the annual mean direct radiative forcing and snow albedo forcing of BC in the TP are respectively ∼ 2.3 and ∼ 2.9 W m−2 over the snow-covered regions. Because of the effects on radiation, BC deposited on snow can further reduce the duration of snow cover in the TP (Ménégoz et al., 2014). Zhang et al. (2018) estimated that such reduction is around 3.1 ± 0.1 d. Furthermore, BC in both the atmosphere and cryosphere over the TP is responsible for retreats of the snow cover (Menon et al., 2010; Xu et al., 2016) and glaciers (Xu et al., 2009; Ming et al., 2012; Niu et al., 2020) in the past decades.
Atmospheric BC concentrations in the TP vary with location and season, which was revealed by limited observations over different regions in the southern (Marinoni et al., 2010; Putero et al., 2014; Chen et al., 2018), northern (Zhao et al., 2012), and south-eastern (Cao et al., 2011; Wang et al., 2018, 2019) TP. Over these regions, seasonal variations in atmospheric BC show different patterns. Chen et al. (2018) observed that in the Himalayas over the southern TP, surface BC concentrations reached the highest in spring (over 0.9 µg m−3 in April) and the lowest in summer (under 0.1 µg m−3 in July) from May 2015 to May 2017. Zhao et al. (2012) reported that in the Qilian Shan over the northern TP, surface BC concentrations were less than 0.2 µg m−3 from May 2009 to March 2011, with the highest in summer and lowest in autumn. Wang et al. (2016) suggested that surface BC concentrations show a seasonality of winter high and spring low at a site in the south-eastern TP, while a pattern of winter low and spring high was at a site in the central TP from November 2012 to June 2013. Because BC observations in the TP are limited due to the harsh environment and sparse sites, we can take advantages of numerical simulations to investigate atmospheric BC and its complicated variations over the entire TP in space and by season. Furthermore, how these variations fluctuate from year to year over decades is an issue worthy of exploration.
Due to weak anthropogenic activities, the contribution of local emissions to atmospheric BC in the TP is low (Zhang et al., 2015). Concentrations of atmospheric BC in the TP are greatly influenced by the long-range transport of BC from non-local regions (Kopacz et al., 2011; Lu et al., 2012; Kang et al., 2019). South Asia and East Asia are suggested to be two main source regions of atmospheric BC in the TP (Lu et al., 2012). Zhang et al. (2015) estimated that, in 2001, the local contribution to BC column burden in the TP was only around 10 %, while the contributions from South Asia and East Asia are respectively about 50 % and 20 %. Some studies also investigated the pathways of BC transport to the TP and derived their characteristics (Cao et al., 2011). The Asian summer monsoon system was identified as an important influencing factor for transport of atmospheric species from South Asia to the TP (Chen et al., 2013; Han et al., 2014; Xu et al., 2014; Zhang et al., 2015). In summer, BC from northern India can be transported to the middle and upper troposphere and then cross the Himalayas to the TP via south-westerly winds (Yang et al., 2018). BC emitted in East Asia can be uplifted to upper layers by the summer monsoon circulation and then transported to the north-eastern TP (Zhang et al., 2015). The mid-latitude westerlies are favourable to BC transport from central Asia and northern India to the western TP (Chen et al., 2018) but unfavourable to BC transport from eastern China to the TP (Cao et al., 2011). Although previous studies explored the mechanisms of BC transport to the TP, large uncertainties remain in the quantified fractional contributions of BC transport from different source regions to the TP (Yang et al., 2018). More importantly, how BC transport to the TP varies inter-annually and what the underlying mechanisms for the variation are remain unclear. Therefore, it is necessary to examine how BC transport to the TP varies from year to year and how the Asian monsoon affects the variation.
Previous observations and simulations showed that biomass burning is a major source of atmospheric BC in the TP (Lu et al., 2012; Zhang et al., 2015). Zhang et al. (2015) estimated that biomass burning together with biofuel emissions can contribute to around half of the annual mean BC column burden over the TP. Engling et al. (2011) reported that BC emissions from fire events in South East Asia in spring could probably increase the BC concentrations over a mountain site in the south-eastern part of the TP. Putero et al. (2014) suggested that over half of the high-BC episodes in the southern Himalayas were likely affected by the fire events in South Asia. These studies demonstrated the influences of biomass burning over a relatively short time span or during some fire events, but few investigated the influences in the long term over decades (Mao and Liao, 2016). The influence of biomass burning on the inter-annual variation in atmospheric BC over the TP warrants an in-depth study.
In this study, we aim to assess the impacts of atmospheric transport and biomass burning on surface BC concentrations over the TP, especially on the inter-annual variation in BC during 1995–2014. To estimate BC transport from different source regions to the TP, we adopt an approach based on a global chemical transport model, GEOS-Chem (Bey et al., 2001), and a trajectory model, the Hybrid Single-Particle Lagrangian Integrated Trajectory model (HYSPLIT; Draxler and Hess, 1998; Stein et al., 2015). In the following, the method and models are described in Sect. 2. Section 3 discusses the seasonal variations in surface BC over the TP and in BC transport from source regions to the TP based on the mean status of the 20-year simulations. The inter-annual variation in surface BC over the TP and the impacts of biomass burning and transport on this variation are analysed in Sect. 4. Discussion and conclusions are provided in Sects. 5 and 6, respectively. In this paper, BC refers to BC aerosols in the atmosphere. Surface BC refers to atmospheric BC aerosols in the surface layer.
2.1 GEOS-Chem simulations
A global chemical transport model, GEOS-Chem (version v9-02, http://geos-chem.org, last access: 10 November 2020; Bey et al., 2001), is used to simulate global BC concentrations. GEOS-Chem is driven by the NASA Modern-Era Retrospective Analysis for Research and Applications (MERRA) meteorological data (Rienecker et al., 2011). In this study, we focused on how surface BC in the TP responds to inter-annual variations in natural influences, including biomass burning and meteorology. Therefore, anthropogenic emissions in our simulations were allowed to vary seasonally but not inter-annually; i.e. anthropogenic emissions in 2000, including their seasonality, were used for each year of the study period. We conducted three GEOS-Chem simulations: CTRL, FixBB, and FixMet. The three simulations covered the study period from 1995 to 2014 (using 1994 for spin-up) at 2∘ latitude × 2.5∘ longitude horizontal resolution with 47 vertical layers. In CTRL, both biomass burning emissions and meteorological fields varied inter-annually. In FixBB, inter-annual meteorology was allowed, and fire emissions were fixed in 2005 to remove the impact of the inter-annual variation in biomass burning on BC concentrations in the TP. In FixMet, emissions from biomass burning were allowed to vary inter-annually, and meteorology was fixed in 2005 to remove the impact of inter-annual meteorology.
In the simulations, global anthropogenic BC emissions were based on Bond et al. (2007), with an annual emission of 4.4 Tg C in 2000 (Leibensperger et al., 2012). Global biomass burning emissions of BC were from the Global Fire Emissions Database version 3 (GFED3) inventory (van der Werf et al., 2010), which covers the period of 1997–2011. BC in GEOS-Chem is represented by two tracers: hydrophobic and hydrophilic (Park et al., 2003). Freshly emitted BC is mostly (80 %) hydrophobic (Cooke et al., 1999). Hydrophobic BC becomes hydrophilic typically in a few days (McMeeking et al., 2011), which is simply assumed as 1.15 d in the model, called an e-folding time (Cooke et al., 1999; Park et al., 2005; He et al., 2014b). Simulations of aerosol dry and wet depositions follow Liu et al. (2001). Dry deposition of aerosols is simulated using a resistance-in-series model (Walcek et al., 1986) dependent on local surface type and meteorological conditions, while wet deposition scheme includes scavenging in convective flows as well as in-cloud and below-cloud scavenging from convective and large-scale precipitation. Dry deposition is generally smaller than wet deposition (He et al., 2014b; K. Li et al., 2016). Tracer advection is computed every 15 min with a flux-form semi-Lagrangian method (Lin and Rood, 1996). The tracer moist convection scheme follows Allen et al. (1996a, b), using GEOS convection, entrainment, and detrainment mass fluxes. The deep convection is parameterized using the relaxed Arakawa–Schubert scheme (Arakawa and Schubert, 1974; Moorthi and Suarez, 1992), and for the shallow convection, the scheme in Hack (1994) is used.
GEOS-Chem has been widely used for BC simulations around the world (Park et al., 2003, 2005; K. Li et al., 2016) and over the TP and its surroundings (Kopacz et al., 2011; Lu et al., 2012; He et al., 2014a, b; Mao and Liao, 2016). The performance of GEOS-Chem in simulating BC over the TP and surroundings was systematically evaluated by He et al. (2014b) using in situ measurements of BC in surface air, BC in snow, and BC absorption aerosol optical depth. He et al. (2014b) found that the simulated BC in surface air compares statistically well with observations at sites away from urban areas, and the model can generally capture the seasonality of the observations, whereas the BC concentrations in the TP are likely to be underestimated by the model. In the Supplement, we evaluated the spatial and seasonal variations in GEOS-Chem BC simulations using observational data from the literature. We found a significant correlation (r=0.99, p<0.05) between the observations and simulations at the rural and remote sites (Fig. S1 in the Supplement). We further compared surface BC concentrations from the GEOS-Chem simulations with those from MERRA2 reanalysis (the Modern-Era Retrospective analysis for Research and Applications version 2, M2TMNXAER, https://cmr.earthdata.nasa.gov/search/concepts/C1276812866-GES_DISC.html, last access: 10 November 2020). The magnitude and spatial distribution of surface BC concentrations in the TP from GEOS-Chem and MERRA2 are highly consistent, with correlation coefficients over 0.98 in the four seasons (Fig. S2). Furthermore, surface BC from GEOS-Chem and MERRA2 shows similar seasonality over the TP as well as over East Asia, South Asia, and South East Asia (Table S2). Regarding the inter-annual variations in surface BC over these regions, the similarity between the simulations and reanalysis is high in spring, summer, and autumn but low in winter (Table S2).
2.2 Fire and meteorological data
The nighttime fire count product retrieved from ATSR (Along Track Scanning Radiometer) using Algorithm 2, available from the European Space Agency (http://due.esrin.esa.int/page_wfa.php, last access: 10 November 2020), was used to verify the biomass burning emissions in GEOS-Chem. ATSR is on board the Second European Remote-Sensing Satellite (ERS-2). The spatial resolution of the data is 1 km, and the sensor achieves a global coverage every 3 d. The ATSR satellite data with the period of 1997–2011 were gridded to the GFED3 grids with a resolution of 0.5∘ × 0.5∘ in longitude and latitude.
The meteorological data used in this study for analysis and for driving the backward trajectories are the NCEP/NCAR (National Centers for Environmental Prediction/National Center for Atmospheric Research) reanalysis, provided by the National Oceanic and Atmospheric Administration (NOAA) Physical Sciences Laboratory (https://psl.noaa.gov/data/gridded/data.ncep.reanalysis.html, last access: 10 November 2020). The data include geopotential height and wind. The horizontal resolution is 2∘ latitude × 2.5∘ longitude.
2.3 Transport estimation
Combining GEOS-Chem simulations and HYSPLIT (version 4, http://www.arl.noaa.gov/HYSPLIT_info.php, last access: 10 November 2020; Draxler and Hess, 1998; Stein et al., 2015) trajectories, we estimated the contributions of different source regions in the world to surface BC in the TP during 1995–2014. HYSPLIT is an atmospheric transport and dispersion model (Fleming et al., 2012) developed by the Air Resources Laboratory of NOAA. Meteorological inputs to HYSPLIT are the NCEP/NCAR reanalysis at a resolution of 2.5∘ latitude × 2.5∘ longitude. We evenly divided the TP into 70 GEOS-Chem grids. Considering that the average lifetime of atmospheric BC is about a week, we simulated 7 d backward trajectories originating from each of the 70 grids. The trajectories were initialized 4 times a day (00:00, 06:00, 12:00, and 18:00 UTC) during 1995–2014. The starting altitude for the trajectories is 100 m above ground, which is within the typical planetary boundary layer in the TP (Ram et al., 2010). We divided the world into seven regions (Fig. 1b), including the TP; central Asia; East Asia; South Asia; South East Asia; and the region of the rest of Asia, Europe, and Africa. BC concentrations from the CTRL simulation were used in the estimation of BC from different source regions.
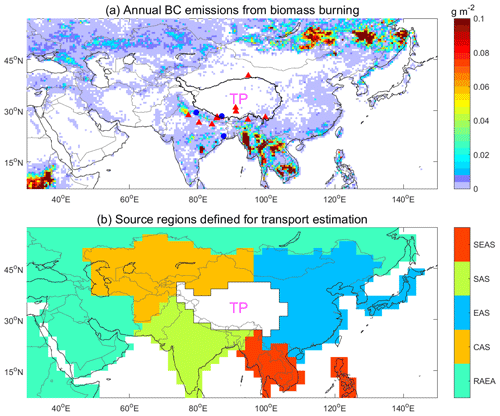
Figure 1(a) Annual BC emissions from biomass burning averaged over 1997–2011 from GFED3. Red triangles (for Table S1) and blue dots (for Table S1 and Fig. S1) indicate the locations of the observation sites used for model evaluation in the Supplement. (b) Source regions defined for the estimation of BC transport to the TP. The abbreviations are for central Asia (CAS); East Asia (EAS); South Asia (SAS); South East Asia (SEAS); and the region of the rest of Asia, Europe, and Africa (RAEA). The dark black line in (a) encloses the domain of the TP, corresponding the white areas in (b).
Lu et al. (2012) proposed a novel approach that combined BC emissions with the backward trajectories from the TP to quantify the origins of BC in the TP. Modifying the approach of Lu et al. (2012), we combine BC concentrations instead of BC emissions in Lu et al. (2012) with the backward trajectories from the TP for the same purpose. We assume that BC aerosols have a lifetime of D days, and the back trajectories using HYSPLIT are simulated for D days (D=7 in this study). To make the estimation stable, the amount of BC transported to a TP surface grid on a day is assumed to be a mean of the BC transport along the backward trajectories originating from that grid in the past D days, i.e.
where BCd is the amount of BC that is transported to that TP surface grid along the backward trajectory on a previous day d ().
Equation (1) provides a way to estimate the amount of BC that is transported to the TP from any model grid outside the TP during a period of interest. For a grid outside the TP (i, j, k are indices for the model grid in longitude, latitude, and altitude coordinates, respectively), the total amount of BC transported from to the TP during a period of interest ( can be estimated by
where n is an index for the number of trajectories. N is the total number of trajectories that have passed through the grid during the period of interest, for example, in a month; c is the daily BC concentrations at when trajectory n passes ; and v is the volume of . M is the number of trajectories in a day (M=4 in this study). Therefore, the total amount of BC transported to the TP (Ti,j) from the entire tropospheric column above a surface grid in a source region outside the TP during the period of interest can be assessed by
where K is the number of model layers in the troposphere.
Finally, the amount of BC transported from a non-local source region to the TP surface can be summed up, and the fractional contributions of different source regions to surface BC in the TP can be quantified. This developed method is inspired by Lu et al. (2012) and is robust and stable because it is not sensitive to the number of trajectories taken in a day (M) and the number of days taken for the trajectories (D).
Using this method, the amount of BC transported from a non-local grid to the TP surface is determined by both BC concentrations in that grid and the number of trajectories passing through that grid within the tropospheric column. An example of the estimation of BC transport to the TP surface in April 2005 is shown in Fig. 2. In this example, both BC total column and BC concentrations at the surface are high in central China around 110∘ E (Figs. 2a and S3). A large number of trajectories pass through central China and finally arrive at the TP surface (Fig. 2b). Therefore, the amount of BC transported from central China to the TP is high (Fig. 2c). Trajectories from Xinjiang province, north-western China (to the north of the TP), are also at low altitudes (Fig. 2b). However, BC total column and BC concentrations at the surface are both low there (Figs. 2a and S3). Therefore, the amount of BC transported from Xinjiang province to the TP surface is lower than that from central China. Air masses from the Middle East and central Asian countries are from high altitudes and transported downward to the surface over different locations in the TP (Fig. 2b). Although these trajectories are in large numbers, the amount of BC transported from the Middle East and central Asian countries is relatively small (Fig. 2c) because the backward trajectories appear at high altitudes (Fig. 2b), and BC concentrations over there are low (Fig. 2a).
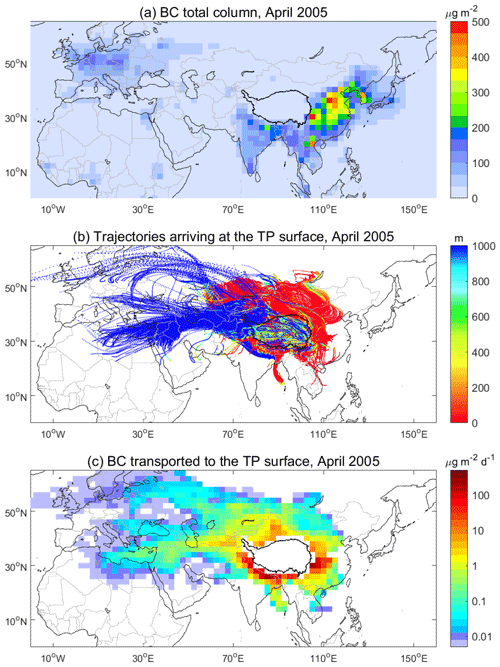
Figure 2An example of estimated BC transport to the TP surface from non-local regions in April 2005. (a) BC total column (µg m−2); (b) 7 d backward trajectories (in metres above the ground) arriving at the TP surface; (c) the amount of BC transported to the TP surface from non-local regions (). The dark black line encloses the domain of the TP.
In this section, the analysis is based on the mean status of the 20-year simulations. Figure 3 shows the amount of BC transported from each of the GEOS-Chem grids through the tropospheric column to the TP surface in the four seasons, which varies greatly in space and among seasons. Obviously, surface BC in the TP mainly originates from South Asia and East Asia, especially from the regions near the southern and eastern borders of the TP, including central China, north-eastern South Asia, and northern South Asia. These spatial distributions of non-local contributions to the BC in the TP in four seasons are similar to those in Lu et al. (2012). We also found a good agreement (r=0.72, p<0.05) in the estimation of imported BC between this study and Lu et al. (2012) at several sites in the TP, although our estimates are higher than those from Lu et al. (2012). The backward-trajectory approach modified in this study shows strong performance in identifying the source regions for BC over the TP.
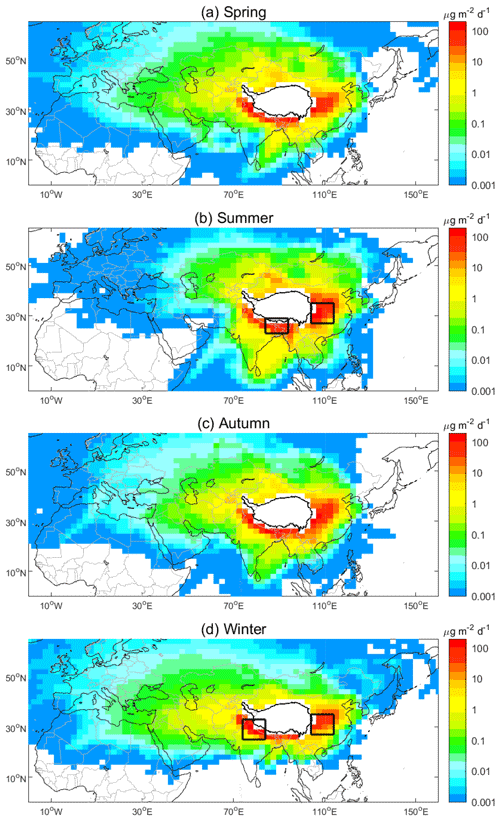
Figure 3The amount of BC transported to the TP surface () from non-local regions in (a) spring, (b) summer, (c) autumn, and (d) winter. The values are based on 20-year (1995–2014) means from the CTRL simulation. The boxed areas in (b) indicate central China (CCH; 27–35∘ N, 104–114∘ E) and north-eastern South Asia (NESAS; 23–29∘ N, 84–94∘ E). The boxed areas in (d) indicate CCH and northern South Asia (NSAS; 25–33∘ N, 74–84∘ E). The dark black line encloses the domain of the TP.
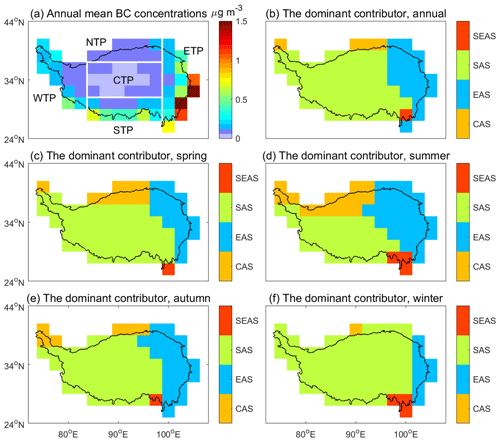
Figure 4Surface BC over the TP and the dominant BC non-local source regions for the TP. (a) Annual mean BC concentrations over the TP. (b–f) The dominant BC non-local source regions (Fig. 1b) for each model grid in the TP for (b) the annual mean, (c) spring, (d) summer, (e) autumn, and (f) winter. The values are 20-year means (1995–2014) from the CTRL simulation. Non-local source regions in (b)–(f) include central Asia (CAS), East Asia (EAS), South Asia (SAS), and South East Asia (SEAS). The solid white lines in (a) separate the subregions of the TP defined in this study, i.e. western TP (WTP), northern TP (NTP), central TP (CTP), southern TP (STP), and eastern TP (ETP). The dark black line encloses the domain of the TP.
The simulated annual mean surface BC concentrations over the entire TP are shown in Fig. 4a. The BC concentrations are high along the eastern and southern borders and low in the centre of the TP. This spatial variation in surface BC concentrations dominates in the four seasons (not shown). BC concentrations over the TP show a strong spatial gradient, which is likely due to the blocking of BC transport by the mountains with high elevations (Cao et al., 2011; Zhao et al., 2017). Figure 4b–f show the dominant non-local influences to surface BC over the TP. In terms of affected areas, South Asia and East Asia are the most important source regions, which respectively impact 67 % and 25 % of the area of the TP in the annual mean BC (Fig. 4b). In terms of the amount of BC imported, 77 % of surface BC in the TP comes from South Asia (43 %) and East Asia (35 %). Because of the leeward location of East Asia under prevailing westerlies, the influence of East Asia is constrained mainly in northern and eastern TP. Using a backward-trajectory method, Lu et al. (2012) estimated that the contributions of South Asia and East Asia are respectively 67 % and 17 %. Using a tracer-tagging approach, Zhang et al. (2015) suggested that the contributions of the two regions are respectively 50 % and 19 %. Our estimate of factional contribution of both South Asia and East Asia is comparable with the two previous studies, while the estimated fractional contribution of East Asia is higher than them.
Seasonally, the contribution of South Asia to surface BC in the TP is strongest in winter (55 %) and weakest in summer (33 %). South Asia is identified as the dominant contributor for 83 % and 41 % of the area of the TP in winter and summer, respectively (Fig. 4). In contrast, the contribution of East Asia is highest in summer (45 %) and lower in winter (23 %). East Asia is identified as the dominant contributor for 34 % and 11 % of the area of the TP, respectively, in summer and winter (Fig. 4). These seasonal variations in the contributions of South Asia and East Asia are generally in agreement with earlier studies (Lu et al., 2012; Zhang et al., 2015; Yang et al., 2018) with various numerical methods. For instance, using the emission perturbation method, Yang et al. (2018) suggested that the contribution of South Asia is 61 % in non-monsoon season (October–April) and 19 % in monsoon season (May–September).
We further divided the TP into five subregions, namely eastern TP, southern TP, western TP, northern TP, and central TP (Fig. 4a). The 20-year means in the subregions show different BC levels, seasonalities, and dominant BC source regions (Figs. 4–6). Over the eastern TP, the mean surface BC concentrations are the highest among the five subregions (Fig. 5b). Above 70 % of surface BC in the eastern TP is transported from East Asia (Fig. 6b).
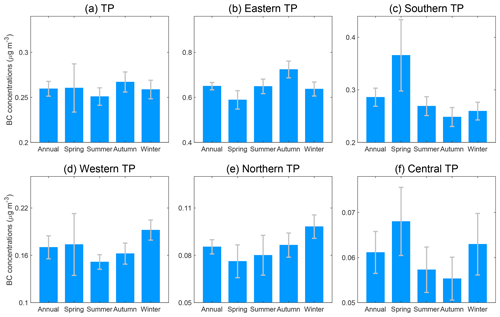
Figure 5Seasonal variations in surface BC concentrations averaged over the TP and its subregions. The values are 20-year (1995–2014) means from the CTRL simulation. The error bar indicates the standard deviation. Note that the y scales are different for different subregions, ranging from the largest in the eastern TP to the smallest in the central TP.
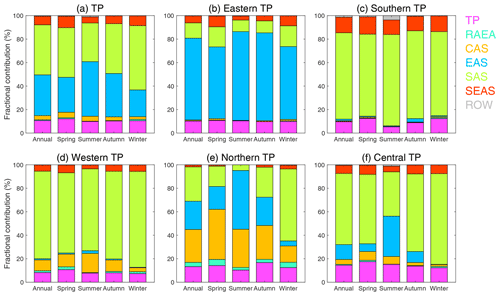
Figure 6Fractional contributions of different source regions to surface BC over the TP and its subregions. The values are 20-year means (1995–2014) from the CTRL simulation. The abbreviations are for central Asia (CAS); East Asia (EAS); South Asia (SAS); South East Asia (SEAS); the region of the rest of Asia, Europe, and Africa (RAEA); and the rest of the world (ROW).
In the southern TP, the mean surface BC concentrations are the second-highest among the five subregions, which are high in spring and low in the other seasons (Fig. 5c). Such seasonality likely results from the high fire emissions over South Asia in spring, the favourable atmospheric circulation for BC transport to the southern TP in spring, and the strong wet deposition of BC by the monsoon precipitation in summer (Chen et al., 2018). This seasonality is in consistency with observations in the literature (Marinoni et al., 2010; Cong et al., 2015). South Asia is the dominant source region for surface BC in the southern TP year-round, with fractional contributions of over 73 % (Fig. 6c). The dominant contribution of South Asia to the southern TP was also suggested in previous studies (He et al., 2014a; Zhang et al., 2015; Yang et al., 2018). The second dominant source region for the southern TP is South East Asia, which contributes 13 % of the surface BC in the region in the annual mean.
Over the western TP, the mean BC concentrations are the third-lowest among the five subregions, with a seasonality of high BC in winter and spring and low BC in summer and autumn (Fig. 5d). The higher values in spring and winter agree with the BC measurements at sites in the western Himalayas (Nair et al., 2013). BC transport from South Asia contributes 82 % of the surface BC in winter and 70 % in summer (Fig. 6d). Such seasonality with winter high and summer low in the fractional contribution of South Asia to surface BC over the western TP was also suggested by Zhang et al. (2015).
In the northern TP, the mean BC concentrations are the second-lowest among the five subregions, which are at maximum in winter and minimum in spring (Fig. 5e). This seasonality is different from an observational study, which reported that, over the Qilian Shan in the northern TP, surface BC concentrations are highest in summer and lowest in autumn (Zhao et al., 2012). The dominant source region for surface BC over the northern TP is central Asia in spring and autumn, East Asia in summer, and South Asia in winter (Fig. 6e). Influenced by the prevailing westerlies, central Asia contributes to surface BC in the western (Fig. 6d) and northern TP (Fig. 6e) more than to other TP subregions. In the annual mean, central Asia can contribute 9 % and 27 % of the surface BC in the western (Fig. 6d) and northern (Fig. 6e) TP, respectively.
Among the five subregions, the central TP has the lowest BC concentrations (Fig. 5f). Seasonally, BC concentrations over the central TP is higher in spring and winter than in summer and autumn. This simulated seasonality is different from the observed one at a site in the central TP reported in Wang et al. (2016), who showed that BC concentrations are higher in spring and lower in winter from November 2012 to June 2013. South Asia is the dominant source region for surface BC in the central TP, and its influence is strongest in winter, contributing 77 % of the surface BC in this subregion. In contrast, the influence of East Asia is strongest in summer, contributing 34 % of the surface BC there.
The local contribution of the TP and the contribution of South East Asia are much lower than those of South Asia and East Asia (Fig. 6). In the annual mean, local contribution to surface BC in the TP is approximately 11 % (Fig. 6a). Among all the TP subregions, the fraction in the local contribution is highest in the central TP, being ∼ 14 % in the annual mean (Fig. 6f). Using a tracer-tagging method, Zhang et al. (2015) also suggested that local emissions contribute about 10 % of BC in the TP. Influenced by the prevailing westerlies, BC from South East Asia cannot be efficiently transported to the TP by atmospheric circulation. The annual mean contribution of South East Asia to surface BC in the TP is ∼ 7 % (Fig. 6a). Seasonally, the contribution of South East Asia is highest in spring (10 %; Fig. 6a), when fire BC emissions are highest there. Geographically, the contribution of South East Asia is highest in the southern TP (13 % in the annual mean; Fig. 6c) because of its proximity to South East Asia.
4.1 Influences of biomass burning on the inter-annual variation in surface black carbon over the Tibetan Plateau
Figure 7 shows the anomalies of surface BC concentrations averaged over the TP from the three GEOS-Chem simulations. Note that in these simulations, only natural influences of meteorological and biomass burning in the inter-annual variation in surface BC in the TP are considered. The simulations from CTRL and FixBB are significantly correlated with each other in all the seasons (r = 0.45, p < 0.05 in spring; r > 0.8, p < 0.05 in the other three seasons), indicating the important role of meteorology in the inter-annual variation in surface BC concentrations in the TP. Remarkably, in spring (Fig. 7a), the correlation coefficient of BC anomalies between CTRL and FixMet simulations reaches 0.86 (p < 0.05), indicating the importance of biomass burning to the inter-annual variation in BC in spring. The largest anomaly of BC concentrations from the CTRL simulation is in 1999. The comparison between CTRL and FixMet simulations suggests that this strong anomaly is largely explained by biomass burning. Even if we exclude the extreme year 1999, the correlation (r = 0.77, p < 0.05) between CTRL and FixMet simulations remains significant, indicating the strong influence of biomass burning on the variation in springtime surface BC from year to year. In the Supplement (Fig. S4), we applied the mean absolute deviation and absolute per cent departure from the mean to quantitatively represent the strength of the inter-annual variation in surface BC over the TP, similar to Mao and Liao (2016). Figure S4 further confirms that biomass burning is an important driver of the inter-annual variation in surface BC over the TP in spring, while meteorology is more important than biomass burning in the other seasons.
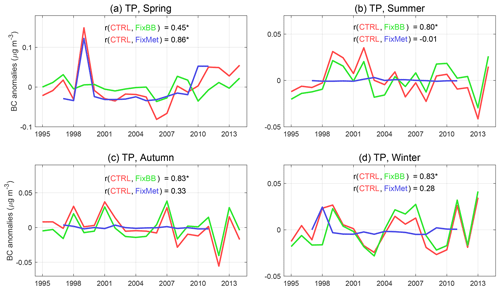
Figure 7Inter-annual variations in the anomalies of surface BC concentrations averaged over the TP from different simulations during 1995–2014 in the four seasons. Red, green, and blue lines indicate CTRL, FixBB, and FixMet simulations, respectively. Values from the FixBB simulation are shown from 1997 to 2011, corresponding to the covering period of the GFED3 inventory. The anomalies are the BC concentrations in a given year minus those in the 20-year mean. A correlation coefficient (r) with “*” indicates that the r is statistically significant (p < 0.05). Note that the anthropogenic emissions in 2000 were used in the simulations (see Sect. 2.1).
To further examine the influence of biomass burning in spring, we integrally analysed data from ATSR satellite fire counts, GFED3 fire emissions, and the GEOS-Chem simulations. Both ATSR and GFED3 data show that fires occur frequently over the Indo-Gangetic Plain, central India, and South East Asia (Figs. 1 and 8). Fire activities in Asia are well described in the GFED3 inventory that is used in the GEOS-Chem simulations (Fig. 8b and c). We found that the inter-annual variation in BC anomalies in the TP from the CTRL simulation is significantly correlated to the total number of fire counts in the Indo-Gangetic Plain (r = 0.76, p < 0.05) and central India (r = 0.67, p < 0.05). The correlation is insignificant to the total number of fire counts in South East Asia (r = 0.19, p > 0.05). In spring of 1999, extreme fire activities occurred in the Indo-Gangetic Plain and central India (Fig. 8b). Driven by the favourable atmospheric circulation, the strong BC emissions from the extreme fire activities greatly enhanced surface BC concentrations in the TP (Fig. 8d). In CTRL simulation, positive BC anomalies appear over the entire TP, with a regional mean of 0.08 µg m−3, or 31 % relative to the 1995–2014 climatology (Fig. 7a). Even when spring 1999 is removed from the time series, biomass burning still shows a large impact on inter-annual surface BC over the TP in spring (Fig. S4). Additionally, in winter, biomass burning was extremely strong in 1998 (Fig. 7d), which enhanced the regional mean surface BC concentrations in the TP by 0.01 µg m−3, or 5 % relative to the climatology.
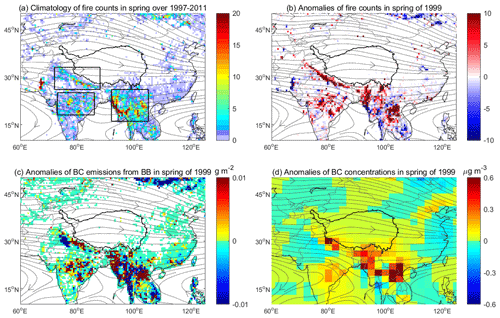
Figure 8Extremely strong biomass burning in spring of 1999 and the corresponding surface BC anomalies and wind field over the TP and surrounding regions. (a) Climatology of fire counts from ATSR in spring overlaid with streamlines at 500 hPa over 1997–2011. (b) Anomalies of fire counts from ATSR overlaid with anomalous streamlines at 500 hPa in spring of 1999. (c) The same as (b) but for the anomalies of BC emissions from biomass burning (BB) from GFED3. (d) The same as (b) but for the anomalies of BC concentrations from the CTRL simulation. The anomaly in (b)–(d) is the corresponding value in 1999 minus that over 1997–2011. The boxed areas in (a) indicate the fire regions in the Indo-Gangetic Plain, central India, and South East Asia. The dark black line encloses the domain of the TP.
In the following, the important role of meteorology in modulating the inter-annual variation in surface BC over the TP is to be explored in Sect. 4.2 and 4.3 from the perspective of the influences of the Asian monsoon on BC transport to the TP.
4.2 Influences of the Asian summer monsoon on the inter-annual variation in transport of black carbon to the Tibetan Plateau from non-local regions in summer
The TP can largely impact the Asian monsoon system through thermal and dynamic processes (Wu et al., 2015). In the meantime, the Asian monsoon can significantly influence the transport of atmospheric species to the TP (Xu et al., 2014). In this section, we show the influences of two Asian monsoon subsystems on the inter-annual variation in BC transport to the TP in summer. We employed a unified dynamical monsoon index to represent the strength of the East Asian summer monsoon (EASM) and South Asian summer monsoon (SASM). The index was proposed by Li and Zeng (2002), and it has been widely applied to quantify the impact of the Asian monsoon on air pollution in Asia (Mao et al., 2017; Lu et al., 2019). Using this index, Han et al. (2019) found a close correlation between the EASM and ozone transport from non-local regions to East Asia. The calculation of the index was introduced in Li and Zeng (2002) and Han et al. (2019). The index is respectively termed as the EASM index (EASMI) and SASM index (SASMI) when it is applied to represent the strength of EASM and SASM. A higher EASMI indicates a stronger EASM, and a higher SASMI indicates a stronger SASM.
Figure 9a shows the spatial distribution of the correlation between BC transport to the TP and wind at 850 hPa at each of the grids in summer. As known from Fig. 3b, central China is a dominant source region for the TP in summer, accounting for 63 % of the imported BC from East Asia to the TP surface. In Fig. 9a, BC from central China correlates significantly with the zonal wind at 850 hPa in central China (Fig. 9a), with a regional mean correlation coefficient of −0.55 (p < 0.05). Westward winds (negative in the zonal component of the wind vector) over central China favour BC transport from East Asia to the TP. Furthermore, the EASMI also correlates negatively with the zonal wind at 850 hPa over central China (Fig. 9b). When the EASM is stronger, the zonal wind in the monsoon circulation weakens over this region (Yang et al., 2014; Han et al., 2019), suggesting that westward winds may occur more frequently or with higher speed. Therefore, BC transport to the TP from central China is enhanced (Fig. 9c) as a significantly positive correlation is found between the strength of the EASM and BC transport from central China to the TP surface (r = 0.49, p < 0.05) and between the strength of the EASM and BC transport from central China to the eastern TP surface (r = 0.48, p < 0.05). This is further confirmed by the differences in BC transport to the TP surface in summers between strong and weak EASM years (Fig. 9d).
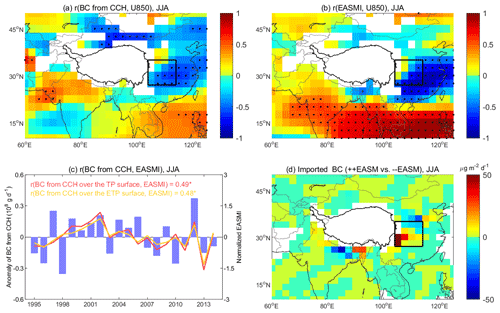
Figure 9Connection between BC transport from East Asia to the TP surface and the EASM in summer. (a) Correlation coefficients (r) between the zonal wind at 850 hPa (U850) and imported BC from central China (CCH) over the TP surface; (b) r between the EASMI and U850; (c) inter-annual variations in the intensity of the EASM and imported BC from CCH to the surface of the TP and eastern TP (ETP); (d) differences in BC transport to the TP surface between the years with strong and weak EASM. Dots in (a) and (b) indicate that the r in the corresponding grid is statistically significant (p<0.05). The unfilled grids in (a) and (b) are due to the topography. An r with “*” in (c) indicates that the r is statistically significant (p < 0.05). Boxed areas in (a), (b), and (d) indicate CCH (see Fig. 4b). The dark black line in (a), (b), and (d) encloses the domain of the TP.
Figure 10 shows how the SASM impacts the BC transport from South Asia over the TP surface in summer. Serving as a heat source in the Asian summer monsoon system, the TP promotes strong convection and modulates the meridional circulation (Xu et al., 2014). Driven by the meridional circulation, BC in South Asia can be transported northward and upward to the TP (Fig. 3b). BC transport from north-eastern South Asia to the TP accounts for 30 % of the total BC transport from South Asia. Inter-annually, BC transport from north-eastern South Asia is significantly correlated with the meridional wind at 500 hPa (r = 0.65, p < 0.05; Fig. 10a), which is also closely correlated to the strength of the SASM (Fig. 10b). In strong SASM years, an anomalous cyclone is located over the northern South Asia at 500 hPa, and correspondingly the meridional wind over the north-eastern South Asia is increased (Fig. 10d). This well explains why the inter-annual variation in BC transport from north-eastern South Asia correlates positively with the strength of the SASM (r = 0.55, p < 0.05 for the TP; r = 0.56, p < 0.05 for the STP; Fig. 10c). Among all source regions, the differences in BC transport from north-eastern South Asia to the TP are largest in summers between strong and weak EASM years (Fig. 10d).
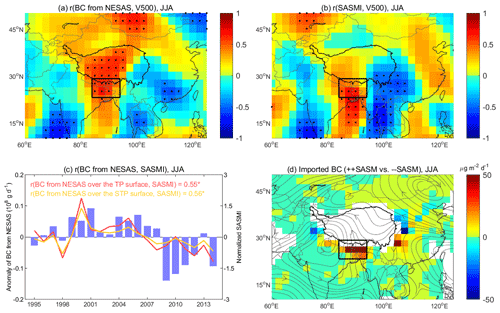
Figure 10Connection between BC transport from South Asia to the TP surface and the SASM in summer. (a) Correlation coefficients (r) between meridional wind at 500 hPa (V500) and imported BC from north-eastern South Asia (NESAS) over the TP surface; (b) r between the SASMI and V500; (c) inter-annual variations in the intensity of the SASM and imported BC from NESAS to the surface of the TP and southern TP (STP); (d) differences in BC transport to the TP surface between the years with strong and weak SASM. Dots in (a) and (b) indicate that the r in the corresponding grid is statistically significant (p<0.05). An r with “*” in (c) indicates that the r is statistically significant (p < 0.05). Streamlines in (d) are the differences between the years with strong and weak SASM at 500 hPa. Boxed areas in (a), (b), and (d) indicate NESAS (see Fig. 4b). The dark black line in (a), (b), and (d) encloses the domain of the TP.
4.3 Influences of the Asian winter monsoon on the inter-annual variation in the transport of black carbon to the Tibetan Plateau from non-local regions in winter
The Asian winter monsoon is a predominant climate feature in Asia and an important modulator of the distribution and transport of air pollutants (Mao et al., 2017; Zhu et al., 2017). However, the impact of the Asian winter monsoon on the inter-annual variation in BC transport to the TP is scantly studied. Here, we assess such impact with two climate indices. We measure the intensity of the East Asian winter monsoon (EAWM) by an index defined by Jhun and Lee (2004). The EAWM index (EAWMI) represents the EAWM intensity by the meridional wind shear associated with the jet stream in the upper troposphere. It can be calculated by the difference in the regional averaged zonal wind speed at 300 hPa between the areas 27.5–37.5∘ N, 110–170∘ E and 50–60∘ N, 80–140∘ E. Using the EAWMI, it is found that the EAWM is closely correlated with the inter-annual variation in pollution transport over East Asia (Q. Li et al., 2016; Han et al., 2019). Furthermore, the Siberian High is a key component of the EAWM system (Wu and Wang, 2002), and its strength can be described using an index defined by Wu and Wang (2002). This Siberian High index (SHI) can be calculated from the regional mean sea level pressure over the area of the Siberian High (40–60∘ N, 80–120∘ E). The EAWMI and SHI are highly correlated (r = 0.72, p < 0.05).
Figure 11 illustrates a connection between the EAWM and BC transport from East Asia to the TP surface. We mainly focused on BC transport from central China as this area contributes 54 % of the total BC transport from East Asia to the TP (Fig. 3d). BC transport from central China to the TP surface layer correlates significantly with the zonal wind at 850 hPa over central China (r = −0.73, p < 0.05; Fig. 11a). The zonal wind over this region is also correlated with the strength of the EAWM (, p < 0.05) and the strength of the Siberian High (r = −0.65, p < 0.05; Fig. 11b). A significant correlation (r = 0.59, p < 0.05) is found between the strength of the EAWM and BC transport from central China to the TP surface (Fig. 11c). When the EAWM is stronger, the more frequent or stronger westward winds can enhance BC transport from central China to the TP (Fig. 11d).
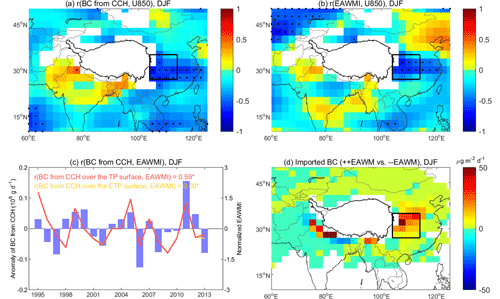
Figure 11Connection between BC transport from East Asia to the TP surface and the EAWM in winter. (a) Correlation coefficients (r) between imported BC from central China (CCH) to surface BC in the TP and zonal wind at 850 hPa (U850); (b) r between the EAWMI and U850; (c) inter-annual variations in the intensity of the EAWM and imported BC from CCH to the surface of the TP and eastern TP (ETP); (d) differences in BC transport to the TP surface between the years with strong and weak EAWM. Dots in (a) and (b) indicate that the r in the corresponding grid is statistically significant (p<0.05). The unfilled grids in (a) and (b) are due to the topography. An r with “*” in (c) indicates that the r is statistically significant (p < 0.05). Boxed areas in (a), (b), and (d) indicate CCH (see Fig. 4d). The dark black line in (a), (b), and (d) encloses the domain of the TP.
A connection between BC transport from South Asia to the TP surface and the Siberian High in winter is shown in Fig. 12. BC over northern South Asia can be transported efficiently to the TP by the prevailing subtropical westerlies. Northern South Asia contributes 70 % of the BC transported from South Asia to the TP surface (Fig. 3d). The contribution of northern South Asia to surface BC in the TP is significantly related (r = 0.72, p < 0.05) to the zonal wind at 500 hPa over the TP (Fig. 12a). The westerlies over the TP are also correlated with the strength of Siberian High (Fig. 12b). The elevated zonal wind in the middle troposphere over the TP in winters with strong Siberian High can enhance the BC transport from northern South Asia to the TP (Fig. 12d). Significant correlations are found between the strength of the Siberian High and BC transport from northern South Asia to the TP surface (r = 0.66, p < 0.05) and between the strength of the Siberian High and BC transport from northern South Asia to the southern TP surface (r = 0.65, p < 0.05; Fig. 12c). In addition, the contribution of northern South Asia to surface BC in the western TP increases significantly with the meridional wind at 500 hPa over the western TP (r = 0.64, p < 0.05). The differences in BC transport from northern South Asia to the TP are largest in winter between strong and weak SHI years (Fig. 12d).
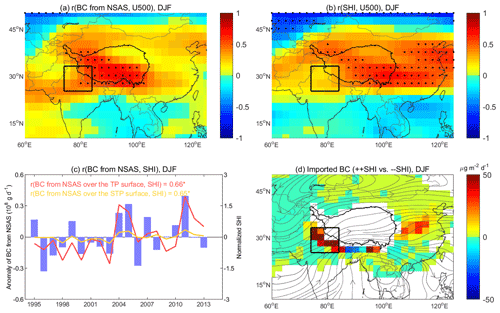
Figure 12Connection between BC transport from South Asia to the TP surface and the Siberian High in winter. (a) Correlation coefficients (r) between zonal wind at 500 hPa (U500) and imported BC from northern South Asia (NSAS) over the TP surface; (b) r between the SHI and U500; (c) inter-annual variations in the SHI and imported BC from NSAS to the surface of the TP and southern TP (STP); (d) differences in BC transport to the TP surface between the years with strong and weak Siberian High. Dots in (a) and (b) indicate that the r in the corresponding grid is statistically significant (p<0.05). An r with “*” in (c) indicates that the r is statistically significant (p < 0.05). Streamlines in (d) are the differences between the years with strong and weak Siberian High at 500 hPa. Boxed areas in (a), (b), and (d) indicate NSAS (see Fig. 4d). The dark black line in (a), (b), and (d) encloses the domain of the TP.
The findings in this study provide an enhanced understanding of the long-range transport of BC to the TP. We comprehensively assessed the BC transport from worldwide source regions to the TP. Our results reveal the source regions of surface BC over the entire TP in the four seasons, which was investigated by limited studies (Lu et al., 2012; Zhang et al., 2015). The influences of South Asia and East Asia on the TP were noticed by previous studies. Most of them were focused on limited locations (Cao et al., 2011; Engling et al., 2011; Chen et al., 2018) or in one or few seasons (Zhao et al., 2017; Wang et al., 2018). Here, we further quantified the influence of South Asia and East Asia over the entire TP in the four seasons in terms of both fractional contribution (Fig. 6) and affected areas in the TP (Fig. 4). Moreover, we identified three key areas within South Asia and East Asia and found that the contribution of BC from there to surface BC in the TP is highest among South Asia and East Asia (Fig. 3).
The estimate of the fractional contributions from various source regions to surface BC in the TP from this study is generally comparable with that from the literature (Lu et al., 2012; Zhang et al., 2015; Yang et al., 2018). (1) The total contribution of South Asia and East Asia to surface BC in the TP is estimated to be 77 %, between 69 % by Zhang et al. (2015) and 84 % by Lu et al. (2012). (2) The contribution of BC from South Asia is largest in winter and smallest in summer, which was also suggested by Lu et al. (2012), Zhang et al. (2015), and Yang et al. (2018). Modelling by Yang et al. (2018) shows that the contribution of South Asia is 61 % in non-monsoon season (October–April) and 19 % in monsoon season (May–September). (3) The local contribution is estimated to be ∼ 10 %, comparable with that in Zhang et al. (2015), indicating the predominant role of BC transport from non-local regions. Nevertheless, there are some disagreements between this and previous studies to various extents. A noticeable disagreement is that the annual mean contribution of East Asia is estimated to be approximately 35 % (Fig. 6; also see Fig. S6 for the estimated contribution at a slightly higher level according to an emission perturbation simulation), while the estimates by Lu et al. (2012) and Zhang et al. (2015) are respectively 17 % and 19 %. The discrepancies may be associated with the differences in region definitions and the estimation models.
Biomass burning is an important source for BC aerosols over the TP (Zhang et al., 2015). It was observed that BC emissions from biomass burning in South Asia could be transported to the TP by the atmospheric circulation (Cong et al., 2015), thus resulting in high-BC episodes in the southern TP (Engling et al., 2011; Putero et al., 2014). Limited numerical studies assessed the impact of biomass burning on surface BC in the TP over a long-term period (Mao and Liao, 2016). Here, we demonstrated that biomass burning is an important driver of the inter-annual variation in surface BC over the TP in spring (Fig. 7). In particular, we found that there were extremely strong fire activities over the Indo-Gangetic Plain from winter 1998 to spring 1999 that largely enhanced surface BC concentrations over the entire TP (Figs. 7 and 8). This extreme anomaly in fire activities and associated influence on BC over the TP may not have been documented in such detail.
The Asian monsoon can influence the atmospheric circulation over the TP and its surroundings (Xu et al., 2014; Han et al., 2019). We found that the Asian monsoon system can significantly modulate the inter-annual variation in BC transport from South Asia and East Asia to the TP (Figs. 9–12). We revealed that the EASM can modulate the westward transport of BC from central China to the TP in summer (Fig. 9), while previous studies mostly focused on the transport pathway build by the SASM (Zhao et al., 2017; Kang et al., 2019). In winter, this study shows significant influences of the EAWM on the BC transport from northern South Asia and central China to the TP (Figs. 11 and 12). These results can also shed some light on the transport mechanisms of other atmospheric species to the TP, such as water vapour.
Regarding methodology, numerical simulations including adjoint (Kopacz et al., 2011), backward-trajectory (Lu et al., 2012), tracer-tagging (Zhang et al., 2015), and emission perturbation (Yang et al., 2018) simulations have been used to identify BC transport from sources to the TP. Each of the methods has its advantages and limitations (Lu et al., 2012; Zhang et al., 2015). Modifying an approach proposed by Lu et al. (2012), we developed an efficient and stable method which shows strong performance in revealing the factional contribution of BC transport from different source regions to the TP by season and year. We also run a set of emission perturbation simulations using GEOS-Chem to compare with the outcomes of the trajectory method being developed in this study. The emission perturbation method can estimate the contribution of a source region to BC concentrations in a receptor region (i.e. the TP in this study) by turning off BC emissions in that source region. The detailed description of our simulation and its outcomes are provided in the Supplement (Fig. S6).
Comparing the results between the backward-trajectory and the emission perturbation methods (Fig. 6 vs. Fig. S6), we can observe the following. (1) Both methods show that South Asia and East Asia are two dominant source regions for surface BC in the TP. The total contributions of South Asia and East Asia estimated by the two methods are comparable, 77 % (Fig. 6a) and 82 % (Fig. S6a), respectively. (2) The two methods suggest similar seasonality for the contributions of South Asia and East Asia. The contribution of South Asia to surface BC in the TP is stronger in winter and spring, while the contribution of East Asia is stronger in summer and autumn. (3) The influences of BC transport on different TP subregions estimated by the two methods are generally consistent. For example, both methods show that over 70 % of surface BC in the eastern TP comes from East Asia, and over 70 % of surface BC in the southern TP comes from South Asia. Therefore, both methods show comparable results, although there are some discrepancies in various details.
Overall, the backward-trajectory method developed in this study can reasonably quantify the relative contributions of different source regions to surface BC in the TP. This method has the following advantages. (1) The transport pathway of BC can be visibly expressed (Figs. 2 and 3). (2) The spatial variation in the contribution of source regions can be shown explicitly (Figs. 3 and 4). (3) It is feasible for users of a chemical transport model to investigate the source–receptor relationships if adjoint and tagged modes are unavailable to them. Note that this method assumes a fixed lifetime of atmospheric BC (i.e. 7 d in this study), which might lead to some uncertainties. The emission perturbation method does not require this assumption. The emission perturbation method is reliable and straightforward. However, it cannot provide the spatial information shown in Figs. 2–4. It can provide an overall assessment only for the total contribution from each of the source regions defined. The number of these regions is often limited because of constraints of computation expenses.
This study is subject to some limitations. Numerical simulations have advantages of covering large areas over the entire TP and long periods, such as 20 years in this study. However, according to this and earlier studies, there are discrepancies between observations and simulations from GEOS-Chem. He et al. (2014b) suggested that BC aerosols over the TP may be underestimated by GEOS-Chem. Simulations in this study have a weaker seasonal variation in BC concentrations than observations from previous studies (Fig. S1). All of these imply uncertainty in simulating the absolute BC concentrations over the TP by GEOS-Chem. Furthermore, the simulations are with a resolution of 2∘ latitude × 2∘ longitude. Such a resolution may not fully capture processes at the sub-grid scale, such as the mountain–valley wind (Cong et al., 2015). Using a regional model, Zhang et al. (2020) demonstrated that, compared with simulations with lower resolution, simulations with higher resolution can better resolve the effects of topography and consequently yield stronger transport flux of BC from South Asia to the TP. Therefore, our results may somewhat underestimate the contribution of South Asia because of the model resolution. Using regional models at higher resolutions in the future can better describe the terrain effect in the TP.
In addition, anthropogenic BC emission inventories used in GEOS-Chem simulations may add uncertainties in our estimates. Figure S7 compares the anthropogenic emissions in 2000 used in this study and those in 2010 from the Task Force on Hemispheric Transport of Air Pollution Phase 2 (TF HTAP2). It shows that anthropogenic BC emissions in most areas of South Asia have substantially increased from 2000 to 2010 (Fig. S7). The regional mean of anthropogenic BC emissions in East Asia has also increased from 2000 to 2010 (Fig. S7). Inside East Asia, anthropogenic BC emissions in most regions of eastern China have increased, while they have decreased in Korea and Japan from 2000 to 2010 (Fig. S7). Therefore, our results may underestimate the BC transport from South Asia and East Asia to the TP in 2010. After 2010, anthropogenic BC emissions have changed as well (Zheng et al., 2018). Zheng et al. (2018) reported that anthropogenic BC emissions in China have reduced 27 % from 2010 to 2017. BC emissions from biomass burning used in this study cover 1997–2011, and fire emissions in 2011 are used for simulation years after 2011. However, fire activities in South Asia have strong inter-annual variations and have been significantly increased from 2001 to 2016 (Earl and Simmonds, 2018). Therefore, fire emissions used here may lead to biases in the BC simulations after 2011. This study is focused on natural drivers (biomass burning and meteorology) that are connective to the inter-annual variation in BC over the TP. The impact of inter-annual anthropogenic emissions warrants further studies.
Using a global chemical transport model, GEOS-Chem, we characterized the variation in surface BC over the TP in 20 years, from 1995 to 2014. Applying an approach that combines the BC concentrations from GEOS-Chem and backward trajectories from HYSPLIT, we identified the source regions for surface BC in the TP and demonstrated the influences of atmospheric transport and biomass burning on the inter-annual variation in surface BC over the TP. The major conclusions are drawn as follows.
Based on the 20-year mean, surface BC concentrations are higher in the southern and eastern TP than in the other TP subregions. Surface BC in the TP is mainly influenced by two source regions: East Asia and South Asia. The two regions totally contribute 77 % of the surface BC in the TP. The influence of East Asia is dominant in summer, while the influence of South Asia is dominant in winter. By subregion, surface BC in the southern and eastern TP comes mainly from South Asia and East Asia, respectively. Over the western and central TP, surface BC comes mainly from South Asia. Over the northern TP, the dominant source region is central Asia in spring and autumn, East Asia in summer, and South Asia in winter.
Inter-annually, from 1995 to 2014, biomass burning can explain over 74 % of the variation in springtime surface BC concentrations over the TP if biomass burning and meteorology are both considered in GEOS-Chem simulations. Springtime surface BC in the TP is significantly correlated to the total number of fire counts from ATSR satellite data at 1 km resolution over the Indo-Gangetic Plain in South Asia (r = 0.76, p < 0.05). In the spring of 1999, the extremely strong biomass burning in South Asia largely elevated surface BC concentrations (0.08 µg m−3, or 31 % relative to the climatology) over the TP. The strong biomass burning in South Asia in the winter of 1998 also enhanced BC concentrations over the TP. The implication of such large disturbances to climate in the TP and the relevant regions is yet to be explored.
The inter-annual variation in surface BC over the TP is greatly influenced by meteorology. Specifically, the Asian monsoon system alters the long-range transport of BC to the TP by modulating the atmospheric circulation. In summer, when the EASM is stronger than normal, the more frequent or stronger westward wind in the lower troposphere can enhance BC transport from central China to the TP. When the SASM is stronger, the increased meridional wind over north-eastern South Asia in the middle troposphere can enhance BC transport from north-eastern South Asia to the TP. In winter, when the EAWM is stronger than normal, the reduced zonal wind in the lower troposphere tends to increase BC transport from central China to the TP. In winters with a stronger Siberian High, the enhanced zonal wind in the middle troposphere over the TP tends to carry more BC from northern South Asia to the TP.
The GEOE-Chem model is publicly available at http://geos-chem.org (last access: 10 November 2020; Bey et al., 2001). The HYSPLIT model can be acquired from http://www.arl.noaa.gov/HYSPLIT_info.php (NOAA Air Resources Laboratory, 2020). The meteorological and fire data were downloaded from https://psl.noaa.gov/data/gridded/data.ncep.reanalysis.html (NOAA Earth System Research Laboratory, 2020) and https://earth.esa.int/web/guest/home (European Space Agency, 2020), respectively.
The supplement related to this article is available online at: https://doi.org/10.5194/acp-20-13591-2020-supplement.
JL, YW, HH, and TZ designed the research. HH and YW performed the study under the supervision of JL. HH, YW, YL, HC, and YZ analysed the data. HH, YW, and JL wrote the paper with valuable input from TZ, BZ, HW, HL, QW, SL, TW, MX, and ML.
The authors declare that they have no conflict of interest.
We are grateful to the following model and data providers. The Atmospheric Chemistry Modeling Group at Harvard University developed and manages the GEOE-Chem model. The HYSPLIT model was developed by the NOAA Air Resources Laboratory. The meteorological and fire data were respectively acquired from the NOAA Earth System Research Laboratory and the European Space Agency. We thank the anonymous reviewers for their constructive comments and suggestions.
This research has been supported by the Chinese Ministry of Science and Technology National Key R&D Program of China (grant nos. 2019YFA0606803 and 2016YFA0600204) and the Natural Science Foundation of China (grant nos. 91744209, 91544230, and 41375140).
This paper was edited by Jianzhong Ma and reviewed by two anonymous referees.
Allen, D. J., Kasibhatla, P., Thompson, A. M., Rood, R. B., Doddridge, B. G., Pickering, K. E., Hudson, R. D., and Lin, S.-J.: Transport-induced interannual variability of carbon monoxide determined using a chemistry and transport model, J. Geophys. Res.-Atmos., 101, 28655–28669, https://doi.org/10.1029/96JD02984, 1996a.
Allen, D. J., Rood, R. B., Thompson, A. M., and Hudson, R. D.: Three-dimensional radon 222 calculations using assimilated meteorological data and a convective mixing algorithm, J. Geophys. Res.-Atmos., 101, 6871–6881, https://doi.org/10.1029/95JD03408, 1996b.
Allen, R. J., Sherwood, S. C., Norris, J. R., and Zender, C. S.: Recent Northern Hemisphere tropical expansion primarily driven by black carbon and tropospheric ozone, Nature, 485, 350–354, https://doi.org/10.1038/nature11097, 2012.
Arakawa, A. and Schubert, W. H.: Interaction of a cumulus cloud ensemble with the large-scale environment, Part I, J. Atmos. Sci., 31, 674–701, https://doi.org/10.1175/1520-0469(1974)031<0674:IOACCE>2.0.CO;2, 1974.
Barnett, T. P., Adam, J. C., and Lettenmaier, D. P.: Potential impacts of a warming climate on water availability in snow-dominated regions, Nature, 438, 303–309, https://doi.org/10.1038/nature04141, 2005.
Bey, I., Jacob, D. J., Yantosca, R. M., Logan, J. A., Field, B. D., Fiore, A. M., Li, Q., Liu, H. Y., Mickley, L. J., and Schultz, M. G.: Global modeling of tropospheric chemistry with assimilated meteorology: Model description and evaluation, J. Geophys. Res.-Atmos., 106, 23073–23095, https://doi.org/10.1029/2001JD000807, 2001.
Bond, T. C., Bhardwaj, E., Dong, R., Jogani, R., Jung, S., Roden, C., Streets, D. G., and Trautmann, N. M.: Historical emissions of black and organic carbon aerosol from energy-related combustion, 1850–2000, Global Biogeochem. Cy., 21, GB2018, https://doi.org/10.1029/2006GB002840, 2007.
Bond, T. C., Doherty, S. J., Fahey, D. W., Forster, P. M., Berntsen, T., DeAngelo, B. J., Flanner, M. G., Ghan, S., Kärcher, B., Koch, D., Kinne, S., Kondo, Y., Quinn, P. K., Sarofim, M. C., Schultz, M. G., Schulz, M., Venkataraman, C., Zhang, H., Zhang, S., Bellouin, N., Guttikunda, S. K., Hopke, P. K., Jacobson, M. Z., Kaiser, J. W., Klimont, Z., Lohmann, U., Schwarz, J. P., Shindell, D., Storelvmo, T., Warren, S. G., and Zender, C. S.: Bounding the role of black carbon in the climate system: A scientific assessment, J. Geophys. Res.-Atmos., 118, 5380–5552, https://doi.org/10.1002/jgrd.50171, 2013.
Cao, J., Tie, X., Xu, B., Zhao, Z., Zhu, C., Li, G., and Liu, S.: Measuring and modeling black carbon (BC) contamination in the SE Tibetan Plateau, J. Atmos. Chem., 67, 45–60, https://doi.org/10.1007/s10874-011-9202-5, 2011.
Cappa, C. D., Onasch, T. B., Massoli, P., Worsnop, D. R., Bates, T. S., Cross, E. S., Davidovits, P., Hakala, J., Hayden, K. L., Jobson, B. T., Kolesar, K. R., Lack, D. A., Lerner, B. M., Li, S.-M., Mellon, D., Nuaaman, I., Olfert, J. S., Petäjä, T., Quinn, P. K., Song, C., Subramanian, R., Williams, E. J., and Zaveri, R. A.: Radiative absorption enhancements due to the mixing state of atmospheric black carbon, Science, 337, 1078–1081, https://doi.org/10.1126/science.1223447, 2012.
Chen, S., Huang, J., Zhao, C., Qian, Y., Leung, L. R., and Yang, B.: Modeling the transport and radiative forcing of Taklimakan dust over the Tibetan Plateau: A case study in the summer of 2006, J. Geophys. Res.-Atmos., 118, 797–812, https://doi.org/10.1002/jgrd.50122, 2013.
Chen, X., Kang, S., Cong, Z., Yang, J., and Ma, Y.: Concentration, temporal variation, and sources of black carbon in the Mt. Everest region retrieved by real-time observation and simulation, Atmos. Chem. Phys., 18, 12859–12875, https://doi.org/10.5194/acp-18-12859-2018, 2018.
Chung, C. E., Ramanathan, V., and Decremer, D.: Observationally constrained estimates of carbonaceous aerosol radiative forcing, P. Natl. Acad. Sci. USA, 109, 11624–11629, https://doi.org/10.1073/pnas.1203707109, 2012.
Cong, Z., Kang, S., Kawamura, K., Liu, B., Wan, X., Wang, Z., Gao, S., and Fu, P.: Carbonaceous aerosols on the south edge of the Tibetan Plateau: concentrations, seasonality and sources, Atmos. Chem. Phys., 15, 1573–1584, https://doi.org/10.5194/acp-15-1573-2015, 2015.
Cooke, W. F., Liousse, C., Cachier, H., and Feichter, J.: Construction of a 1∘ × 1∘ fossil fuel emission data set for carbonaceous aerosol and implementation and radiative impact in the ECHAM4 model, J. Geophys. Res.-Atmos., 104, 22137–22162, https://doi.org/10.1029/1999JD900187, 1999.
Draxler, R. R. and Hess, G.: An overview of the HYSPLIT_4 modelling system for trajectories, Aust. Meteorol. Mag., 47, 295–308, 1998.
Earl, N. and Simmonds, I.: Spatial and temporal variability and trends in 2001–2016 global fire activity, J. Geophys. Res.-Atmos., 123, 2524–2536, https://doi.org/10.1002/2017JD027749, 2018.
Engling, G., Zhang, Y.-N., Chan, C.-Y., Sang, X.-F., Lin, M., Ho, K.-F., Li, Y.-S., Lin, C.-Y., and Lee, J. J.: Characterization and sources of aerosol particles over the southeastern Tibetan Plateau during the Southeast Asia biomass-burning season, Tellus B, 63, 117–128, https://doi.org/10.1111/j.1600-0889.2010.00512.x, 2011.
European Space Agency: ATSR, available at: https://earth.esa.int/web/guest/home, last access: 10 November 2020.
Flanner, M. G., Zender, C. S., Randerson, J. T., and Rasch, P. J.: Present-day climate forcing and response from black carbon in snow, J. Geophys. Res.-Atmos., 112, D11202, https://doi.org/10.1029/2006JD008003, 2007.
Flanner, M. G., Zender, C. S., Hess, P. G., Mahowald, N. M., Painter, T. H., Ramanathan, V., and Rasch, P. J.: Springtime warming and reduced snow cover from carbonaceous particles, Atmos. Chem. Phys., 9, 2481–2497, https://doi.org/10.5194/acp-9-2481-2009, 2009.
Fleming, Z. L., Monks, P. S., and Manning, A. J.: Review: Untangling the influence of air-mass history in interpreting observed atmospheric composition, Atmos. Res., 104–105, 1–39, https://doi.org/10.1016/j.atmosres.2011.09.009, 2012.
Hack, J. J.: Parameterization of moist convection in the National Center for Atmos. Res. community climate model (CCM2), J. Geophys. Res.-Atmos., 99, 5551–5568, https://doi.org/10.1029/93JD03478, 1994.
Han, H., Liu, J., Yuan, H., Wang, T., Zhuang, B., and Zhang, X.: Foreign influences on tropospheric ozone over East Asia through global atmospheric transport, Atmos. Chem. Phys., 19, 12495–12514, https://doi.org/10.5194/acp-19-12495-2019, 2019.
Han, Y., Sun, H., Liu, J., Zhao, T., and Gong, S.: Study on simulated seasonal variations of black carbon aerosol transport and depositions over the Tibetan Plateau, Journal of Arid Meteorology, 32, 319–325, 2014 (in Chinese).
Hansen, J. and Nazarenko, L.: Soot climate forcing via snow and ice albedos, P. Natl. Acad. Sci. USA, 101, 423–428, https://doi.org/10.1073/pnas.2237157100, 2004.
He, C., Li, Q., Liou, K. N., Takano, Y., Gu, Y., Qi, L., Mao, Y., and Leung, L. R.: Black carbon radiative forcing over the Tibetan Plateau, Geophys. Res. Lett., 41, 7806–7813, https://doi.org/10.1002/2014GL062191, 2014a.
He, C., Li, Q. B., Liou, K. N., Zhang, J., Qi, L., Mao, Y., Gao, M., Lu, Z., Streets, D. G., Zhang, Q., Sarin, M. M., and Ram, K.: A global 3-D CTM evaluation of black carbon in the Tibetan Plateau, Atmos. Chem. Phys., 14, 7091–7112, https://doi.org/10.5194/acp-14-7091-2014, 2014b.
Jacobson, M. Z.: Investigating cloud absorption effects: Global absorption properties of black carbon, tar balls, and soil dust in clouds and aerosols, J. Geophys. Res.-Atmos., 117, D06205, https://doi.org/10.1029/2011JD017218, 2012.
Jhun, J.-G. and Lee, E.-J.: A new East Asian winter monsoon index and associated characteristics of the winter monsoon, J. Climate, 17, 711–726, https://doi.org/10.1175/1520-0442(2004)017<0711:ANEAWM>2.0.CO;2, 2004.
Jiang, Y., Yang, X.-Q., Liu, X., Yang, D., Sun, X., Wang, M., Ding, A., Wang, T., and Fu, C.: Anthropogenic aerosol effects on East Asian winter monsoon: The role of black carbon-induced Tibetan Plateau warming, J. Geophys. Res.-Atmos., 122, 5883–5902, https://doi.org/10.1002/2016JD026237, 2017.
Kang, S., Zhang, Q., Qian, Y., Ji, Z., Li, C., Cong, Z., Zhang, Y., Guo, J., Du, W., Huang, J., You, Q., Panday, A. K., Rupakheti, M., Chen, D., Gustafsson, Ö., Thiemens, M. H., and Qin, D.: Linking atmospheric pollution to cryospheric change in the Third Pole region: current progress and future prospects, Natl. Sci. Rev., 6, 796–809, https://doi.org/10.1093/nsr/nwz031, 2019.
Kopacz, M., Mauzerall, D. L., Wang, J., Leibensperger, E. M., Henze, D. K., and Singh, K.: Origin and radiative forcing of black carbon transported to the Himalayas and Tibetan Plateau, Atmos. Chem. Phys., 11, 2837–2852, https://doi.org/10.5194/acp-11-2837-2011, 2011.
Lau, W. K. M., Kim, M.-K., Kim, K.-M., and Lee, W.-S.: Enhanced surface warming and accelerated snow melt in the Himalayas and Tibetan Plateau induced by absorbing aerosols, Environ. Res. Lett., 5, 025204, https://doi.org/10.1088/1748-9326/5/2/025204, 2010.
Leibensperger, E. M., Mickley, L. J., Jacob, D. J., Chen, W.-T., Seinfeld, J. H., Nenes, A., Adams, P. J., Streets, D. G., Kumar, N., and Rind, D.: Climatic effects of 1950–2050 changes in US anthropogenic aerosols – Part 1: Aerosol trends and radiative forcing, Atmos. Chem. Phys., 12, 3333–3348, https://doi.org/10.5194/acp-12-3333-2012, 2012.
Li, J. and Zeng, Q.: A unified monsoon index, Geophys. Res. Lett., 29, https://doi.org/10.1029/2001GL013874, 2002.
Li, K., Liao, H., Mao, Y., and Ridley, A. D.: Source sector and region contributions to concentration and direct radiative forcing of black carbon in China, Atmos. Environ., 124, 351–366, https://doi.org/10.1016/j.atmosenv.2015.06.014, 2016.
Li, Q., Zhang, R., and Wang, Y.: Interannual variation of the wintertime fog-haze days across central and eastern China and its relation with East Asian winter monsoon, Int. J. Climatol., 36, 346–354, https://doi.org/10.1002/joc.4350, 2016.
Li, W., Guo, W., Qiu, B., Xue, Y., Hsu, P.-C., and Wei, J.: Influence of Tibetan Plateau snow cover on East Asian atmospheric circulation at medium-range time scales, Nat. Commun., 9, 4243, https://doi.org/10.1038/s41467-018-06762-5, 2018.
Lin, S. J. and Rood, R. B.: Multidimensional flux-form semi-Lagrangian transport schemes, Mon. Weather Rev., 124, 2046–2070, https://doi.org/10.1175/1520-0493(1996)124<2046:MFFSLT>2.0.CO;2, 1996.
Liu, H., Jacob, D. J., Bey, I., and Yantosca, R. M.: Constraints from 210Pb and 7Be on wet deposition and transport in a global three-dimensional chemical tracer model driven by assimilated meteorological fields, J. Geophys. Res.-Atmos., 106, 12109–12128, https://doi.org/10.1029/2000JD900839, 2001.
Lu, X., Zhang, L., Liu, X., Gao, M., Zhao, Y., and Shao, J.: Lower tropospheric ozone over India and its linkage to the South Asian monsoon, Atmos. Chem. Phys., 18, 3101–3118, https://doi.org/10.5194/acp-18-3101-2018, 2018.
Lu, Z., Streets, D. G., Zhang, Q., and Wang, S.: A novel back-trajectory analysis of the origin of black carbon transported to the Himalayas and Tibetan Plateau during 1996–2010, Geophys. Res. Lett., 39, L01809, https://doi.org/10.1029/2011GL049903, 2012.
Lu, X., Zhang, L., Zhao, Y., Jacob, D. J., Hu, Y., Hu, L., Gao, M., Liu, X., Petropavlovskikh, I., McClure-Begley, A., and Querel, R.: Surface and tropospheric ozone trends in the Southern Hemisphere since 1990: possible linkages to poleward expansion of the Hadley circulation, Sci. Bull., 64, 400–409, https://doi.org/10.1016/j.scib.2018.12.021, 2019.
Mao, Y.-H. and Liao, H.: Impacts of meteorological parameters and emissions on decadal, interannual, and seasonal variations of atmospheric black carbon in the Tibetan Plateau, Adv. Climate Change Res., 7, 123–131, https://doi.org/10.1016/j.accre.2016.09.006, 2016.
Mao, Y.-H., Liao, H., and Chen, H.-S.: Impacts of East Asian summer and winter monsoons on interannual variations of mass concentrations and direct radiative forcing of black carbon over eastern China, Atmos. Chem. Phys., 17, 4799–4816, https://doi.org/10.5194/acp-17-4799-2017, 2017.
Marinoni, A., Cristofanelli, P., Laj, P., Duchi, R., Calzolari, F., Decesari, S., Sellegri, K., Vuillermoz, E., Verza, G. P., Villani, P., and Bonasoni, P.: Aerosol mass and black carbon concentrations, a two year record at NCO-P (5079 m, Southern Himalayas), Atmos. Chem. Phys., 10, 8551–8562, https://doi.org/10.5194/acp-10-8551-2010, 2010.
McMeeking, G. R., Good, N., Petters, M. D., McFiggans, G., and Coe, H.: Influences on the fraction of hydrophobic and hydrophilic black carbon in the atmosphere, Atmos. Chem. Phys., 11, 5099–5112, https://doi.org/10.5194/acp-11-5099-2011, 2011.
Ménégoz, M., Krinner, G., Balkanski, Y., Boucher, O., Cozic, A., Lim, S., Ginot, P., Laj, P., Gallée, H., Wagnon, P., Marinoni, A., and Jacobi, H. W.: Snow cover sensitivity to black carbon deposition in the Himalayas: from atmospheric and ice core measurements to regional climate simulations, Atmos. Chem. Phys., 14, 4237–4249, https://doi.org/10.5194/acp-14-4237-2014, 2014.
Menon, S., Koch, D., Beig, G., Sahu, S., Fasullo, J., and Orlikowski, D.: Black carbon aerosols and the third polar ice cap, Atmos. Chem. Phys., 10, 4559–4571, https://doi.org/10.5194/acp-10-4559-2010, 2010.
Ming, J., Xiao, C., Cachier, H., Qin, D., Qin, X., Li, Z., and Pu, J.: Black Carbon (BC) in the snow of glaciers in west China and its potential effects on albedos, Atmos. Res., 92, 114–123, https://doi.org/10.1016/j.atmosres.2008.09.007, 2009.
Ming, J., Du, Z., Xiao, C., Xu, X., and Zhang, D.: Darkening of the mid-Himalaya glaciers since 2000 and the potential causes, Environ. Res. Lett., 7, 014021, https://doi.org/10.1088/1748-9326/7/1/014021, 2012.
Moorthi, S. and Suarez, M. J.: Relaxed Arakawa-Schubert. A parameterization of moist convection for general circulation models, Mon. Weather Rev., 120, 97–102, https://doi.org/10.1175/1520-0493(1992)120<0978:RASAPO>2.0.CO;2, 1992.
Nair, V. S., Babu, S. S., Moorthy, K. K., Sharma, A. K., Marinoni, A., and Ajai: Black carbon aerosols over the Himalayas: direct and surface albedo forcing, Tellus B, 65, 19738, https://doi.org/10.3402/tellusb.v65i0.19738, 2013.
Niu, H., Kang, S., Wang, H., Du, J., Pu, T., Zhang, G., Lu, X., Yan, X., Wang, S., and Shi, X.: Light-absorbing impurities accelerating glacial melting in southeastern Tibetan Plateau, Environ. Pollut., 257, 113541, https://doi.org/10.1016/j.envpol.2019.113541, 2020.
NOAA Air Resources Laboratory: Hysplit, available at: http://www.arl.noaa.gov/HYSPLIT_info.php, last access: 10 November 2020.
NOAA Earth System Research Laboratory: NCEP/NCAR reanalysis, available at: https://psl.noaa.gov/data/gridded/data.ncep.reanalysis.html, last access: 10 November 2020.
Park, R. J., Jacob, D. J., Chin, M., and Martin, R. V.: Sources of carbonaceous aerosols over the United States and implications for natural visibility, J. Geophys. Res.-Atmos., 108, 4355, https://doi.org/10.1029/2002JD003190, 2003.
Park, R. J., Jacob, D. J., Palmer, P. I., Clarke, A. D., Weber, R. J., Zondlo, M. A., Eisele, F. L., Bandy, A. R., Thornton, D. C., Sachse, G. W., and Bond, T. C.: Export efficiency of black carbon aerosol in continental outflow: Global implications, J. Geophys. Res.-Atmos., 110, D11205, https://doi.org/10.1029/2004JD005432, 2005.
Putero, D., Landi, T. C., Cristofanelli, P., Marinoni, A., Laj, P., Duchi, R., Calzolari, F., Verza, G. P., and Bonasoni, P.: Influence of open vegetation fires on black carbon and ozone variability in the southern Himalayas (NCO-P, 5079 m a.s.l.), Environ. Pollut., 184, 597–604, https://doi.org/10.1016/j.envpol.2013.09.035, 2014.
Qian, Y., Flanner, M. G., Leung, L. R., and Wang, W.: Sensitivity studies on the impacts of Tibetan Plateau snowpack pollution on the Asian hydrological cycle and monsoon climate, Atmos. Chem. Phys., 11, 1929–1948, https://doi.org/10.5194/acp-11-1929-2011, 2011.
Qin, D., Liu, L., and Li, P.: Snow cover distribution, variability, and response to climate change in western China, J. Climate, 19, 1820–1833, https://doi.org/10.1175/JCLI3694.1, 2006.
Qu, B., Ming, J., Kang, S.-C., Zhang, G.-S., Li, Y.-W., Li, C.-D., Zhao, S.-Y., Ji, Z.-M., and Cao, J.-J.: The decreasing albedo of the Zhadang glacier on western Nyainqentanglha and the role of light-absorbing impurities, Atmos. Chem. Phys., 14, 11117–11128, https://doi.org/10.5194/acp-14-11117-2014, 2014.
Ram, K., Sarin, M. M., and Tripathi, S. N.: A 1 year record of carbonaceous aerosols from an urban site in the Indo-Gangetic Plain: Characterization, sources, and temporal variability, J. Geophys. Res.-Atmos., 115, D24313, https://doi.org/10.1029/2010JD014188, 2010.
Ramanathan, V. and Carmichael, G.: Global and regional climate changes due to black carbon, Nat. Geosci., 1, 221–227, https://doi.org/10.1038/ngeo156, 2008.
Rienecker, M. M., Suarez, M. J., Gelaro, R., et al.: MERRA: NASA's Modern-Era Retrospective Analysis for Research and Applications, J. Climate, 24, 3624–3648, https://doi.org/10.1175/JCLI-D-11-00015.1, 2011.
Stein, A. F., Draxler, R. R., Rolph, G. D., Stunder, B. J. B., Cohen, M. D., and Ngan, F.: NOAA's HYSPLIT atmospheric transport and dispersion modeling system, B. Am. Meteorol. Soc., 96, 2059–2077, https://doi.org/10.1175/BAMS-D-14-00110.1, 2015.
van der Werf, G. R., Randerson, J. T., Giglio, L., Collatz, G. J., Mu, M., Kasibhatla, P. S., Morton, D. C., DeFries, R. S., Jin, Y., and van Leeuwen, T. T.: Global fire emissions and the contribution of deforestation, savanna, forest, agricultural, and peat fires (1997–2009), Atmos. Chem. Phys., 10, 11707–11735, https://doi.org/10.5194/acp-10-11707-2010, 2010.
Walcek, C. J., Brost, R. A., Chang, J. S., and Wesely, M. L.: SO2, sulfate and HNO3 deposition velocities computed using regional landuse and meteorological data, Atmos. Environ., 20, 949–964, https://doi.org/10.1016/0004-6981(86)90279-9, 1986.
Wang, M., Xu, B., Wang, N., Cao, J., Tie, X., Wang, H., Zhu, C., and Yang, W.: Two distinct patterns of seasonal variation of airborne black carbon over Tibetan Plateau, Sci. Total Environ., 573, 1041–1052, https://doi.org/10.1016/j.scitotenv.2016.08.184, 2016.
Wang, M., Xu, B., Yang, S., Gao, J., Zhang, T., He, Z., Kobal, M., and Hansen, A. D. A.: Black carbon profiles from tethered balloon flights over the southeastern Tibetan Plateau, Chin. Sci. Bull., 64, 2949–2958, https://doi.org/10.1360/TB-2019-0101, 2019 (in Chinese).
Wang, Q., Cao, J., Han, Y., Tian, J., Zhu, C., Zhang, Y., Zhang, N., Shen, Z., Ni, H., Zhao, S., and Wu, J.: Sources and physicochemical characteristics of black carbon aerosol from the southeastern Tibetan Plateau: internal mixing enhances light absorption, Atmos. Chem. Phys., 18, 4639–4656, https://doi.org/10.5194/acp-18-4639-2018, 2018.
Wu, B. and Wang, J.: Winter Arctic Oscillation, Siberian High and East Asian winter monsoon, Geophys. Res. Lett., 29, 3-1-3-4, https://doi.org/10.1029/2002GL015373, 2002.
Wu, G., Duan, A., Liu, Y., Mao, J., Ren, R., Bao, Q., He, B., Liu, B., and Hu, W.: Tibetan Plateau climate dynamics: recent research progress and outlook, Natl. Sci. Rev., 2, 100–116, https://doi.org/10.1093/nsr/nwu045, 2015.
Xu, B., Cao, J., Hansen, J., Yao, T., Joswia, D. R., Wang, N., Wu, G., Wang, M., Zhao, H., Yang, W., Liu, X., and He, J.: Black soot and the survival of Tibetan glaciers, P. Natl. Acad. Sci. USA, 106, 22114–22118, https://doi.org/10.1073/pnas.0910444106, 2009.
Xu, X., Zhao, T., Lu, C., Guo, Y., Chen, B., Liu, R., Li, Y., and Shi, X.: An important mechanism sustaining the atmospheric “water tower” over the Tibetan Plateau, Atmos. Chem. Phys., 14, 11287–11295, https://doi.org/10.5194/acp-14-11287-2014, 2014.
Xu, Y., Ramanathan, V., and Washington, W. M.: Observed high-altitude warming and snow cover retreat over Tibet and the Himalayas enhanced by black carbon aerosols, Atmos. Chem. Phys., 16, 1303–1315, https://doi.org/10.5194/acp-16-1303-2016, 2016.
Yang, J., Kang, S., Ji, Z., and Chen, D.: Modeling the origin of anthropogenic black carbon and its climatic effect over the Tibetan Plateau and surrounding regions, J. Geophys. Res.-Atmos., 123, 671–692, https://doi.org/10.1002/2017JD027282, 2018.
Yang, Y., Liao, H., and Li, J.: Impacts of the East Asian summer monsoon on interannual variations of summertime surface-layer ozone concentrations over China, Atmos. Chem. Phys., 14, 6867–6879, https://doi.org/10.5194/acp-14-6867-2014, 2014.
Yao, T., Thompson, G. L., Mosbrugger, V., Zhang, F., Ma, Y., Luo, T., Xu, B., Yang, X., Joswiak, R. D., Wang, W., Joswiak, E. M., Devkota, P. L., Tayal, S., Jilani, R., and Fayziev, R.: Third Pole Environment (TPE), Environ. Dev., 3, 52–64, https://doi.org/10.1016/j.envdev.2012.04.002, 2012.
Zhang, M., Zhao, C., Cong, Z., Du, Q., Xu, M., Chen, Y., Chen, M., Li, R., Fu, Y., Zhong, L., Kang, S., Zhao, D., and Yang, Y.: Impact of topography on black carbon transport to the southern Tibetan Plateau during the pre-monsoon season and its climatic implication, Atmos. Chem. Phys., 20, 5923–5943, https://doi.org/10.5194/acp-20-5923-2020, 2020.
Zhang, R., Wang, H., Qian, Y., Rasch, P. J., Easter, R. C., Ma, P.-L., Singh, B., Huang, J., and Fu, Q.: Quantifying sources, transport, deposition, and radiative forcing of black carbon over the Himalayas and Tibetan Plateau, Atmos. Chem. Phys., 15, 6205–6223, https://doi.org/10.5194/acp-15-6205-2015, 2015.
Zhang, Y., Kang, S., Sprenger, M., Cong, Z., Gao, T., Li, C., Tao, S., Li, X., Zhong, X., Xu, M., Meng, W., Neupane, B., Qin, X., and Sillanpää, M.: Black carbon and mineral dust in snow cover on the Tibetan Plateau, The Cryosphere, 12, 413–431, https://doi.org/10.5194/tc-12-413-2018, 2018.
Zhao, S., Ming, J., Xiao, C., Sun, W., and Qin, X.: A preliminary study on measurements of black carbon in the atmosphere of northwest Qilian Shan, J. Environ. Sci., 24, 152–159, https://doi.org/10.1016/S1001-0742(11)60739-0, 2012.
Zhao, S., Tie, X., Long, X., and Cao, J.: Impacts of Himalayas on black carbon over the Tibetan Plateau during summer monsoon, Sci. Total Environ., 598, 307–318, https://doi.org/10.1016/j.scitotenv.2017.04.101, 2017.
Zheng, B., Tong, D., Li, M., Liu, F., Hong, C., Geng, G., Li, H., Li, X., Peng, L., Qi, J., Yan, L., Zhang, Y., Zhao, H., Zheng, Y., He, K., and Zhang, Q.: Trends in China's anthropogenic emissions since 2010 as the consequence of clean air actions, Atmos. Chem. Phys., 18, 14095–14111, https://doi.org/10.5194/acp-18-14095-2018, 2018.
Zhu, Y., Liu, J., Wang, T., Zhuang, B., Han, H., Wang, H., Chang, Y., and Ding, K.: The impacts of meteorology on the seasonal and interannual variabilities of ozone transport from North America to East Asia, J. Geophys. Res., 122, 10612–10636, https://doi.org/10.1002/2017JD026761, 2017.
Zhuang, B. L., Li, S., Wang, T. J., Liu, J., Chen, H. M., Chen, P. L., Li, M. M., and Xie, M.: Interaction between the Black Carbon Aerosol Warming Effect and East Asian Monsoon Using RegCM4, J. Climate, 31, 9367–9388, https://doi.org/10.1175/JCLI-D-17-0767.1, 2018.
- Abstract
- Introduction
- Data and methods
- Seasonal variations in surface black carbon over the Tibetan Plateau and in black carbon transport to the Tibetan Plateau
- Inter-annual variation in surface black carbon over the Tibetan Plateau
- Discussion
- Conclusions
- Data availability
- Author contributions
- Competing interests
- Acknowledgements
- Financial support
- Review statement
- References
- Supplement
- Abstract
- Introduction
- Data and methods
- Seasonal variations in surface black carbon over the Tibetan Plateau and in black carbon transport to the Tibetan Plateau
- Inter-annual variation in surface black carbon over the Tibetan Plateau
- Discussion
- Conclusions
- Data availability
- Author contributions
- Competing interests
- Acknowledgements
- Financial support
- Review statement
- References
- Supplement