the Creative Commons Attribution 4.0 License.
the Creative Commons Attribution 4.0 License.
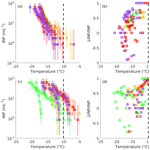
Contrasting local and long-range-transported warm ice-nucleating particles during an atmospheric river in coastal California, USA
Andrew C. Martin
Gavin Cornwell
Charlotte M. Beall
Forest Cannon
Sean Reilly
Bas Schaap
Dolan Lucero
Jessie Creamean
F. Martin Ralph
Hari T. Mix
Ice-nucleating particles (INPs) have been found to influence the amount, phase and efficiency of precipitation from winter storms, including atmospheric rivers. Warm INPs, those that initiate freezing at temperatures warmer than −10 ∘C, are thought to be particularly impactful because they can create primary ice in mixed-phase clouds, enhancing precipitation efficiency. The dominant sources of warm INPs during atmospheric rivers, the role of meteorology in modulating transport and injection of warm INPs into atmospheric river clouds, and the impact of warm INPs on mixed-phase cloud properties are not well-understood. In this case study, time-resolved precipitation samples were collected during an atmospheric river in northern California, USA, during winter 2016. Precipitation samples were collected at two sites, one coastal and one inland, which are separated by about 35 km. The sites are sufficiently close that air mass sources during this storm were almost identical, but the inland site was exposed to terrestrial sources of warm INPs while the coastal site was not. Warm INPs were more numerous in precipitation at the inland site by an order of magnitude. Using FLEXPART (FLEXible PARTicle dispersion model) dispersion modeling and radar-derived cloud vertical structure, we detected influence from terrestrial INP sources at the inland site but did not find clear evidence of marine warm INPs at either site. We episodically detected warm INPs from long-range-transported sources at both sites. By extending the FLEXPART modeling using a meteorological reanalysis, we demonstrate that long-range-transported warm INPs were observed only when the upper tropospheric jet provided transport to cloud tops. Using radar-derived hydrometeor classifications, we demonstrate that hydrometeors over the terrestrially influenced inland site were more likely to be in the ice phase for cloud temperatures between 0 and −10 ∘C. We thus conclude that terrestrial and long-range-transported aerosol were important sources of warm INPs during this atmospheric river. Meteorological details such as transport mechanism and cloud structure were important in determining (i) warm INP source and injection temperature and (ii) ultimately the impact of warm INPs on mixed-phase cloud properties.
- Article
(3758 KB) -
Supplement
(372 KB) - BibTeX
- EndNote
Atmospheric rivers (ARs) are responsible for significant precipitation in many extratropical regions (Ralph et al., 2006; Neiman et al., 2011; Ralph and Dettinger, 2012; Dettinger, 2013; Lavers and Villarini, 2013). On the windward side of some continents, including the US state of California, ARs are responsible for up to 50 % of the annual rainfall (Dettinger et al., 2011; Lavers and Villarini, 2015). It has long been known that naturally occurring tropospheric aerosols can influence precipitation by serving as heterogeneous ice-nucleating particles (INPs) (Vali, 1971; Pitter and Pruppacher, 1973; Maki et al., 1974; DeMott et al., 2011). INPs may also influence precipitation from ARs. Ault et al. (2011) compared two dynamically similar ARs that impacted California and found that precipitation residues classified as dust or biological were more plentiful in the AR that produced more precipitation and more mountain snow. By extending similar analyses, Creamean et al. (2013) showed a relationship between the amount of dust and biological precipitation residues and the precipitation amount and phase. Creamean et al. (2013, 2015) also found that precipitation occurring after the storm's cold front (CF) passed was more enriched in these residue types. Numerical weather prediction experiments (Fan et al., 2014) have demonstrated that dust aerosols can invigorate precipitation in the California AR by enhancing snow formation in mixed-phase orographic clouds.
Several studies have suggested that long-range-transported (LRT) dust aerosols are often mixed with biological remnant material (Conen et al., 2011; Murray et al., 2015; O'Sullivan et al., 2016). The source of the remnant material may allow dust and biological mixtures to serve as warm INPs. Herein, we define warm INPs as particles that cause freezing of supercooled liquid cloud droplets through immersion nucleation at temperatures warmer than −10 ∘C (Stopelli et al., 2015). Several other types of biological aerosol particles of terrestrial or marine origin may also serve as warm INPs. These particle types may include pollen, viruses, bacteria or microscopic plant material (Schnell and Vali, 1973, 1976; Pruppacher et al., 2010; Hoose et al., 2010; Murray et al., 2012). Terrestrial warm INPs can be found in high concentrations near agricultural regions (Tobo et al., 2014), forests (Tobo et al., 2013) and in biomass burning (Petters et al., 2009). Recent studies suggest terrestrial INPs can induce “bioprecipitation feedback” (Huffman et al., 2013; Prenni et al., 2013; Morris et al., 2014; Bigg et al., 2015), whereby rainfall stimulates emission of INPs from some types of terrestrial biota and rainfall efficiency and INP concentration are thereafter increased. Marine INPs are thought to be an important source for the global INP budget (Burrows et al., 2013). Indeed, it has been shown that biological material ejected to the atmosphere in sea spray may contribute to immersion mode freezing at temperatures as warm as −5 ∘C (DeMott et al., 2016; Wilson et al., 2015; McCluskey et al., 2018).
ARs often exist near upper tropospheric jet (UTJ) streams and can generate deep clouds whose tops may access air masses containing long-range-transported dust or dust and bio-INPs. Both Ault et al. (2011) and Creamean et al. (2013) hypothesized that INPs arrived at their storms near cloud top and showed through back-trajectory analysis that the likely sources of these INPs were Asiatic, Arabian and African desert regions. The degree to which terrestrial or marine warm INPs enter AR clouds is less well-established, though good evidence that marine aerosols and terrestrially emitted pollutant aerosols enter the clouds in ARs over California has been provided (Rosenfeld et al., 2008, 2014).
The impact warm INPs have on AR clouds is likewise not established. ARs support a wide variety of clouds, cloud structures and kinematic features that could allow warm INPs to encounter supercooled liquid droplets. Past authors have noted that ARs regularly generate stratiform orographic clouds containing a large amount of supercooled liquid water (Heggli et al., 1983; Heggli and Rauber, 1988) and that AR orographic clouds regularly form seeder–feeder structures (Robichaud and Austin, 1988) wherein falling ice hydrometeors grow rapidly by riming in the warmest supercooled layers (Neiman et al., 2002; White et al., 2003; Creamean et al., 2013). In the seeder–feeder model, the altitude or temperature of warm INP injection to the cloud may lead to differing hydrometeor growth outcomes by changing the relative importance of processes such as riming, ice multiplication, and/or the Wegener–Bergeron–Findeisen process (Pruppacher et al., 2010). Further complicating matters, the type of cloud, depth of cloud and amount of supercooled liquid water may vary considerably during a given AR and could depend upon local topography and short-lived kinematic regimes such as barrier jets, low-level jets and cold fronts (Kingsmill et al., 2006; Ralph et al., 2005; Kingsmill et al., 2013).
While the authors mentioned above and others have collected INPs in AR clouds and precipitation and found important links between INP source and ARs, additional contrast between (i) local marine and terrestrial and (ii) LRT warm INP sources is needed. In addition, coincident analyses of warm INPs with cloud injection temperature and hydrometeor properties are necessary to establish that warm INPs impact AR clouds rather than simply becoming removed by below-cloud precipitation. Hereafter, we will refer to local marine and terrestrial warm INPs as simply “marine” and “terrestrial”. LRT will refer to all other warm INPs.
White et al. (2013)White et al. (2013)White et al. (2013)White et al. (2013)Table 1Atmospheric river observatory (ARO) measurements by site (Bodega Bay, CA: BBY; Cazadero, CA: CZC).

For the current study, we examined hourly precipitation samples collected at two northern CA, USA, locations during an AR during 5–6 March 2016. During an extended period of this event, the coastal site – Bodega Marine Laboratory, Bodega Bay, CA – was directly upwind of the inland site – Cazadero, CA – by approximately 35 km. We will demonstrate that the geometry of the flow during this AR and the geography of the two sites create a natural contrast whereby both sites were exposed to marine and long-range-transported aerosol sources, but only the inland site was exposed to terrestrial aerosol sources. We will additionally demonstrate that the temperature lapse rates of this storm and partial beam blocking by the coastal mountain range near the measurement sites constrained weather service radar such that the retrieved signal was from hydrometeors with temperatures −9.2 ∘C ∘C. The remotely sensed hydrometeors thus approximately overlap with the temperatures of warm INP activation. We used these unique properties to inform analyses of the amount and activation spectra of ice nuclei in precipitation, cloud hydrometeor phase, kinematic regime and cloud-terminating air mass source. These analyses allowed us to address the following questions:
-
What roles do terrestrial, marine and LRT sources have in determining the warm INPs during this AR?
-
What are the transport and cloud injection mechanisms for each of these sources?
-
When warm INPs are present in precipitation, are cloud microphysics impacted?
The rest of this study will be organized as follows. We present data sources and the study location in Sect. 2. Methodology, including the detection of the kinematic forcing regime; Lagrangian dispersion modeling; and radar analyses are presented in Sect. 3. We will review the atmospheric river event and present our findings in Sect. 4. Finally, we review how our findings address the above questions in Sect. 5.
2.1 Atmospheric river observatory
The coastal atmospheric river observatory (ARO) was developed by the National Oceanic and Atmospheric Administration Earth System Research Laboratory (NOAA-ESRL) to better observe kinematic forcing, cloud and precipitation processes during landfalling ARs. The ARO is comprised of two sites in northern CA commonly exposed to AR conditions during the winter months. A coastal site at Bodega Marine Laboratory in Bodega Bay, CA (BBY; 15 m a.m.s.l., meters above mean sea level; 38.32∘ N, 123.07∘ W), and a mountain site in Cazadero, CA (CZC; 478 m a.m.s.l.; 38.61∘ N, 123.22∘ W), together measure nearly coincident weather conditions during landfalling ARs (White et al., 2013). During the event described herein, both sites had a tipping bucket rain gauge, near-surface (10 m) anemometer and GPS receiver capable of estimating integrated water vapor by means of radio occultation and a vertically oriented radar for vertical sensing of atmospheric properties. BBY had a 449 MHz wind profiling radar and CZC had a S-band precipitation radar (see Table 1 for a list of all ARO measurements and their technical references). The CZC S-band radar was used to determine the echo top height (ETH – see Sect. 3.4) during the AR. Neiman et al. (2002) contains a description of the coastal ARO and the application of the measurements to AR kinematics, cloud properties and precipitation.
2.2 Precipitation samples
Precipitation samples were collected hourly from 00:00 UTC on 5 March to 00:00 UTC on 7 March, 2016. Precipitation was captured by the Teledyne ISCO model 6712 commercial water samplers (Teledyne ISCO, Inc., US) connected by Tygon tubing to a 300 mL funnel. Precipitation was dispensed into 1 of 24 350 mL glass jars with an hourly collection time interval. Sampling began by manually initiating the program on the sampler at BBY and by triggering from the Teledyne ISCO 674 rain gauge, set to 0.5 mm threshold, at CZC. Two ISCO samplers, programmed to sample sequentially, were placed at each site, enabling a 48 h continuous collection period. Prior to collection, glass jars were cleaned with acetone, methanol and ultrapure Milli-Q water (18 MΩ cm−1), and peripheral hardware (funnel, tubing, distributor arm, etc.) was rinsed with Milli-Q water. Precipitation samples analyzed in the automated ice spectrometer (AIS, Sect. 2.5) were separated into 40 mL glass scintillation vials, frozen and stored at −20 ∘C for approximately 4 months before they were thawed for analysis.
2.3 Balloon-borne soundings
Helium balloon-borne GPS rawinsondes (Vaisala model RS-41) were launched from BBY at irregular intervals varying from 60 to 180 min during the AR. Each rawinsonde carried a package of meteorological instruments to measure ambient temperature, humidity, latitude, longitude and altitude. These data were broadcast to a ground-based antenna at BBY during balloon flight. Two-dimensional horizontal wind was derived automatically from the time derivative of rawinsonde position. Vaisala model MW41 DigiCORA sounding system software was used to postprocess and archive data from each rawinsonde. The relevant soundings used in the analysis of the AR event are listed in Table 2.
2.4 Climate Forecast System
NOAA Climate Forecast System (CFS) global short-duration (t<6 h) forecasts (Saha et al., 2014) were used as three-dimensional atmospheric forcing datasets for FLEXPART (FLEXible PARTicle dispersion model, Sect. 3.2). CFS was also used to identify large-scale meteorological features such as ARs and the Pacific upper tropospheric jet stream.
2.5 Automated ice spectrometer
INP concentrations and freezing activation spectra were determined via the droplet freezing method (Hill et al., 2014) using the automated ice spectrometer (AIS – Hill et al., 2016). Precipitation samples were distributed directly in microliter aliquots into a 96-well polypropylene assay plate. The assay plates were loaded into the AIS, which was slowly cooled until the samples were frozen. Cooling of each hourly precipitation sample was repeated in triplicate, along with a Milli-Q water sample as control for contamination from the loading process. Though the homogeneous freezing point of water is −38 ∘C, freezing of Milli-Q samples typically started at −25 to −27 ∘C, effectively setting the cold limit at which freezing due to INPs in precipitation can be determined. Cumulative droplet freezing activity spectra, INP(T) (mL−1 rainwater), were calculated using the fraction of unfrozen wells f per given temperature interval: , where V is the volume of the sample in each well (Vali, 1971). The fraction of unfrozen wells f was adjusted for contamination by subtracting the number of frozen Milli-Q water wells per temperature interval from both the total number of unfrozen wells and the total number of wells of the sample. The limit of detection for the AIS under these laboratory conditions was 0.70 mL−1. Warm INP concentration, INP−10, is herein defined as the cumulative concentration at ∘C.
A companion set of precipitation samples were heated prior to introduction to the AIS to detect ice-nucleating biological material that is sensitive to heat (Hill et al., 2014). Heated precipitation samples were subjected to heat via immersion in a hot water bath (90 ∘C) for 20 min prior to analysis with the AIS. In the analysis presented later, if heated INP(T) decreased compared to unheated INP(T) drawn from the same precipitation sample for ∘C, we consider a portion of warm INPs from that sample as bio-INP.
3.1 INP source and impact hypothesis testing
There is much concerning INPs we cannot directly observe during this event. Specifically, we cannot analyze INP chemical composition separately from the ambient aerosol or condensation nuclei population. The primary inferences about terrestrial INPs therefore come from the contrast between the AIS-measured freezing temperature and number of INPs in the coastal and inland site precipitation. We also cannot observe ice-nucleation events in cloud above our collection sites. To address the goals of this study related to INP sources upwind of both sites (Marine and LRT) and impacts on cloud microphysics, we performed backward Lagrangian air mass modeling using the FLEXPART model and analysis of weather-service-radar-derived hydrometeor type in clouds above our precipitation collection sites
Each of these analysis methods has its own shortcomings. Accordingly, results emerging from the FLEXPART and radar analyses will be supported by constructing and rejecting alternate hypotheses. These hypotheses and their accompanying experimental design will be briefly described in tandem with the FLEXPART and weather service radar technical methods.
3.2 FLEXPART
We used the FLEXPART Lagrangian dispersion model (Stohl et al., 2005) to simulate backward dispersion from discrete cloud layers (see Sect. 3.3 for definition of layers) over the ARO. FLEXPART version 9.0.2 was run in serial processor mode on a Unix workstation. Backward simulation of FLEXPART iteratively solves for element position (latitude, longitude and altitude) as a function of time prior to release. It should be noted that the distance separating BBY and CZC is approximately 35 km and is less than the horizontal resolution of the CFS grid (0.5∘ latitude by 0.5∘ longitude). However, FLEXPART performs several operations designed to resolve motions at less than grid scale (Stohl et al., 2005). We ran FLEXPART simulations for each site separately but, with a small exception, did not find significant difference in element position or transport patterns. Therefore, unless noted we present only the result of FLEXPART backward simulations ending at CZC.
3.3 FLEXPART experiments
The FLEXPART model was employed to simulate the sources of air arriving in subfreezing cloud layers over the ARO. The simulations were motivated by the following hypothesis.
-
H1: warm INP content in precipitation is limited by the cloud's access to air masses containing the specific source.
For example, LRT warm INPs will be present only if collected precipitation is falling from clouds with temperature suitable for ice nucleation that can entrain air transported from terrestrial regions across the Pacific Ocean. Note that we cannot run a full global source–receptor model to address this hypothesis, because emission and removal of warm INPs are poorly simulated processes. Therefore, in performing the FLEXPART modeling we are assuming that the INP sources contained in a given air mass are closely associated with the history of the air mass as it traveled through the atmosphere before arriving in cloud. To construct an air mass history related to LRT sources, we examined simulated air mass residence in the upper tropospheric jet and the atmospheric river. Each is a persistent and horizontally extensive feature located near the ARO during this event and contains fast horizontal winds directed from remote regions to the measurement sites. Each is therefore capable of efficiently transporting remote air masses to the cloud layers over the ARO. To construct an air mass history related to marine sources, we examined simulated residence in the northeast Pacific Ocean marine boundary layer (MBL). To construct an air mass history related to local terrestrial sources, we examined simulated residence in the terrestrial boundary layer (TBL) over California.
Warm INPs can initiate freezing in any cloud with subfreezing temperatures, but they are expected to have the greatest impact where temperatures are warmer than −10 ∘C. We separately examined injection to mixed-phase clouds (0 ∘C ∘C) and cloud tops ( ∘C for the majority of this event) by simulating the position of FLEXPART elements released from each layer. Because the atmosphere is highly stratified, we expect air mass sources entering mixed-phase and cloud top layers to differ. We also expect that the AR kinematic regime (e.g., barrier jet, low-level jet, post-cold front) will both modulate the atmospheric stratification and limit the pathways available to transport air to each cloud layer by serving as the final airflow link between clouds and the large-scale weather pattern. We used rawinsondes to define the upper and lower geopotential height boundaries of the mixed-phase and cloud top layer and to identify contiguous periods of dominant kinematic regime (hereafter “kinematic periods”) within our storm (see Sect. 3.4). Cloud top height boundaries were assigned by perturbing the S-band radar echo top height by ±500 m. Separate FLEXPART simulations were performed by releasing 2000 FLEXPART elements from each kinematic period and cloud layer and allowing the model to simulate the element position backward in time for 120 h. We examined H1 by calculating the probability of instantaneous element residence, Pres, in each feature (UTJ, AR, MBL, TBL). Element position was considered an instantaneous sample from a set of elements that would end in the mixed-phase (cloud top) layer over the ARO. The quantity Pres was calculated as the fraction of the set of positions that could be assigned to the feature, , where nres is the number of element positions residing in the desired feature, and nrel is the total number released from the given layer above the ARO.
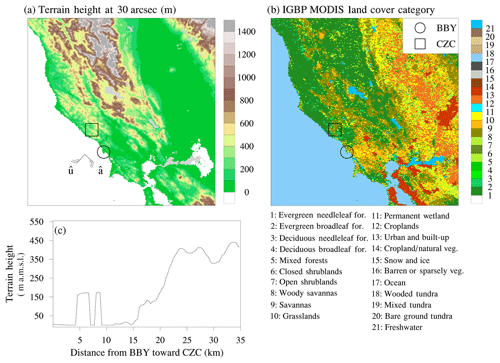
Figure 1(a) Plan view of regional terrain height (m – color fill) from the USGS 30 arcsec digital elevation map. Annotations are centered on BBY (circle) and CZC (square) and depict theoretical wind barbs aligned with the upslope () and along-slope directions (). (b) As in (a), except the dominant category from the IGBP MODIS land use database is depicted (color fill – see legend for category name). (c) Transect of terrain height (m a.m.s.l.) along a great circle path from BBY to CZC.
3.4 Identifying kinematic periods and air mass history features in atmospheric data
Definitions of cloud layer, air mass history and kinematic forcing features are described herein with a short summary of identification methodology using study datasets.
-
Atmospheric river (AR): FLEXPART elements resided in the AR when CFS integrated vapor transport (IVT) exceeded 250 kg m s−1 (Rutz et al., 2014) and relative humidity exceeded 85 % (Ralph et al., 2005).
-
Coastal barrier jet (CBJ): the CBJ forms as onshore-directed wind is deflected by the coastal mountain topography. It was identified as a time–height maximum in along-slope water vapor flux occurring below the local terrain height (450 m, Neiman et al., 2004). Along-slope water vapor flux was calculated similarly to terrain-normal water vapor flux, except the formula is expressed as , where is the magnitude of the horizontal wind projected to the along-slope direction (). See a hypothetical along-slope wind barb depicted in Fig. 1. Rawinsonde observations of two-dimensional wind speed and qv were temporally interpolated to a constant 60 min time series using a cubic spline before water vapor flux calculations were performed.
-
Echo top height (ETH): the echo top approximates the upper boundary of the cloud layer sensed by the S-band radar at CZC. See White et al. (2013) for details.
-
AR low-level jet (LLJ): the LLJ was defined as a time–height maximum in terrain-normal water vapor flux occurring below 3 (Neiman et al., 2002; Ralph et al., 2005). Terrain-normal water vapor flux was calculated from rawinsondes following the formula , where qv is the water vapor mixing ratio (g kg−1) and is the magnitude of the horizontal wind (m s−1) projected along the terrain-normal (upslope) direction for the ARO local terrain (Neiman et al., 2002). A hypothetical wind barb directed along the upslope direction () is depicted in Fig. 1.
-
Marine boundary layer (MBL): the MBL was defined where CFS geopotential height () was less than the FLEXPART planetary boundary layer depth and the FLEXPART latitude and longitude are over the northeast Pacific Ocean.
-
Terrestrial boundary layer (TBL): the TBL was defined similarly to the MBL, expect latitude and longitude must have been over the US state of California.
-
Pacific upper tropospheric jet stream (UTJ): the UTJ was identified using CFS data when horizontal wind speed exceeded 50 m s−1 between an altitude of 6.5 and 11 (hereafter referred to as the UTJ layer). The UTJ layer was defined by visual identification of the UTJ in latitude–vertical cross sections along the longitudes 135, 150 and 165∘ W extending from 25 to 60∘ N during 5 and 6 March 2016. It was confirmed that the UTJ remained quasi-stationary during 5–6 March 2016.
-
Polar cold front: the polar cold front was identified using rawinsonde data by the directional wind shear in the lowest 5 km of the troposphere. The discontinuity between horizontal wind veering/backing with height (Neiman et al., 1991) is considered to mark the transit of the cold front across the ARO.
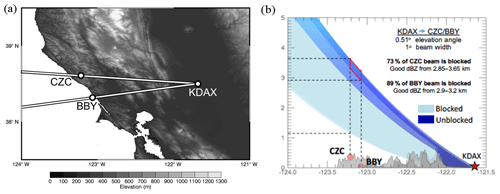
Figure 2(a) Plan view of region surrounding the study area with KDAX, BBY and CZC labeled. Beams show path of radar from KDAX to each site (BBY, CZC). White shading indicates relative terrain height (). (b) Height vs. longitude cross section with KDAX 0.51∘ elevation scan beam blocked (light blue), unblocked over CZC (medium blue) and unblocked over BBY (dark blue) layers. Red trapezoid indicates the volume from BBY azimuths that are unblocked and share the altitudes of the CZC unblocked layer. Location of BBY (CZC) indicated by red dot at respective longitude and height. Terrain profiles along BBY (CZC) azimuths also indicated in gray shading.
3.5 KDAX weather service radar
The KDAX weather service radar (Heiss et al., 1990) located in Sacramento, CA, was used to evaluate hydrometeor phase and precipitation intensity in a shallow mixed-phase cloud layer over BBY and CZC. The location of KDAX relative to BBY and CZC is shown in Fig. 2a. During each azimuthal scan, the lowest beam elevation (0.51∘) from KDAX is partially blocked by the coastal mountain range. The result of the beam blockage is that a signal is only returned from a narrow vertical slice of the scan above BBY (CZC). Figure 2b depicts the blocked and unblocked portions of the beam above both sites. The highest and lowest altitudes of the KDAX unblocked layers are 2850 and 3650 m, respectively. During this storm, rawinsondes measured the temperature range corresponding to these altitude limits (Table 2). Hydrometeors sensed by KDAX in the unblocked layer over BBY and CZC were in the temperature range 0.8 to −9.2 ∘C. Therefore, information retrieved from the KDAX unblocked layer such as hydrometeor type and radar reflectivity was indicative of mixed-phase clouds during the storm. WSR-88D polarimetric weather service radars (including KDAX) retrieve hydrometeor type (Park et al., 2009) containing 11 classifications: biological (animals, not particles), clutter, ice, dry snow, wet snow, light rain, heavy rain, big drops, graupel, hail and unknown. We designed our KDAX experiment (Sect. 3.6) to operate on binary information: frozen and not frozen. We grouped the classifications ice, dry snow, wet snow and graupel into the frozen hydrometeor category. All other classifications beside unknown were categorized as “not frozen”.
3.6 Radar experiments
After grouping and discarding the unknown classification, we were left with binary categorical information (frozen or not frozen) from the KDAX radar retrievals. We applied a chi-square independence test to the frozen or not-frozen category time series to test the hypothesis:
-
H2: the likelihood of detecting frozen hydrometeors in the mixed-phase cloud layer differed above the coastal and inland sites during the storm.
To confirm that the KDAX retrieval category time series were sufficient to test this hypothesis, we verified that the data passed the chi-square rule of thumb for minimum expected populations and that the result of the test did not change under Yates' correction (Haviland, 1990). The result of this test is only of interest if the CZC and BBY categorical data are drawn from remotely sensed hydrometeors at the same range of temperatures, namely the range of temperatures in the unblocked layer (Table 2). To preserve the same upper and lower unblocked layer altitudes over both sites, we retained only one range gate, nearest the CZC site, from the CZC azimuth. We retained the 45 range gates from the BBY azimuth that complete the red trapezoid in Fig. 2b. The KDAX radial resolution is 250 m; thus the BBY azimuth retrievals correspond to the unblocked layer over BBY along a great circle toward KDAX extending 11.5 km.
We do not possess independent observations of temperature in the KDAX unblocked layer over each site. Instead, we assume that the temperatures () are equivalently representative of both sites. Each rawinsonde's ground location was tracked to an altitude of 3650 m. The mean ground location in the height range of the KDAX unblocked beam layer varied by sounding but was nearly equidistant from both sites at a distance approximately 19.44 (26.48) km to BBY (CZC). We note that local effects related to airflow over a mountain barrier (Minder et al., 2011) could preferentially cool the lower troposphere above CZC more than above BBY. If this effect is strong, the unblocked beam layer above CZC could contain cooler air than it does over BBY. Following the methodology of Minder et al. (2011), we performed semi-idealized simulations of flow over a two-dimensional hill of approximate height (500 m) and half width (10 km) of the mountain ridge at CZC using rawinsondes from this study as the upstream boundary condition. Simulated temperatures above the CZC proxy mountain were not cooler than those above the BBY proxy coast by more than 0.25 ∘C.
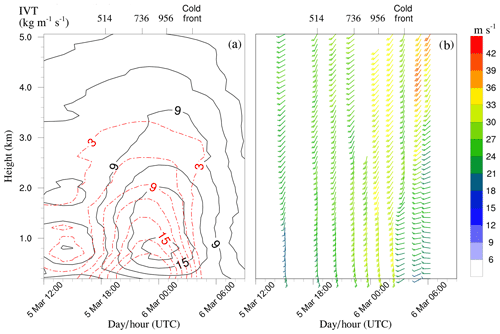
Figure 3(a) Upslope (black solid) and along-barrier (red dashed) water vapor flux () derived from rawinsondes during the storm period. (b) Rawinsonde horizontal wind profiles (m s−1, wind barbs colored by speed) during the event. In each (a) and (b), the time of significant sondes is marked along the top axis by their IVT () or by the arrival of the cold front.
4.1 Overview of the atmospheric river event
An atmospheric river caused heavy rain in northern California during 5–6 March 2016. AR conditions, measured by a combination of the 449 MHz wind profiling radar and the GPS receiver (Ralph et al., 2013), were present at the ARO from 15:00 UTC on 5 March 2016 to 06:00 UTC on 6 March 2016. Rawinsonde measurements show that IVT reached a peak value of 956 near 02:00 UTC on 6 March (see Table 2). This value is well above the range of expected peak IVT estimates for ARs impacting this region (Ralph et al., 2018). Total precipitation at CZC during AR conditions was 72 mm, placing this event in the top 20 % of all events published in Ralph et al. (2013).
4.2 Kinematic periods
Time–vertical meteograms of along-slope and upslope vapor flux () over the ARO are shown in Fig. 3a. Along-slope (upslope) vapor flux is here used to indicate transport of water vapor consistent with a coastal barrier jet (AR low-level jet) – see Sect. 3.4. CBJ vapor transport reached its maximum between 21:00 and 23:00 UTC on 5 March. Maximum values in along-slope vapor transport were located between the surface and 400 The LLJ vapor transport maxima occurred later, between 23:00 UTC on 5 March and 01:00 UTC on 6 March. the LLJ vertical maxima was located above the height of the coastal mountains, near 750 This spatiotemporal evolution of the CBJ and LLJ is consistent with previous studies. In particular, Neiman et al. (2004) found that the barrier jet typically forms before the arrival of maximum vapor transport, in response to blocking of the flow by local topography. Kingsmill et al. (2013) described the AR low-level jet as forced upward over the top of an antecedent barrier jet, with typical location near 1
Table 3Kinematic periods, their beginning and end time, maximum sounding-derived IVT, height of cloud layers (see Sect. 3b) used for FLEXPART analysis, mean INP−10 and accumulated precipitation at each site.
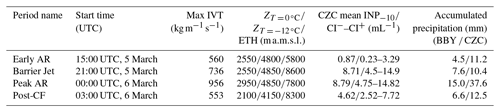
Figure 3b shows horizontal wind (m s−1) vectors from balloon-borne radiosondes. The top axis indicates the time of soundings measuring IVT values of 514, 736 and 956 , respectively. Also indicated on the top axis is the time of sounding indicating the transit of the cold front. Wind barbs back with height in the lowest 5 km of the troposphere for this and following sondes, indicating that subsequent soundings sampled the post-cold frontal air mass. The strength of each the coastal barrier jet, the low-level jet and the cold front, along with their interchange in a short period of time, suggests that the kinematic forcing for orographic clouds during this AR may have changed rapidly several times. We will hereafter use the dominance in vapor flux by the CBJ (LLJ) and the transit of the cold front to segment the AR into four kinematic periods (see Table 3).
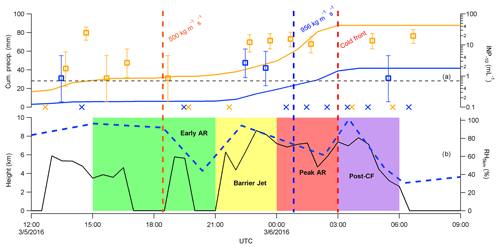
Figure 4(a) Time series of INP−10 (mL−1) at BBY (box-and-whisker plot – blue), at CZC (box-and-whisker plot – orange), accumulated precipitation (mm) at BBY (blue line) and accumulated precipitation at CZC (orange line). Blue (orange) “X” on temporal axis indicates precipitation sample with INP−10 below AIS detection limit at BBY (CZC). Timing of IVT surpassing 500 and cold-front transit are annotated in red dashed lines. Horizontal black dashed line displays the AIS detection limit 0.7 mL−1. (b) S-band radar-derived echo top (ET – black solid) and bright band (BB – black dashed) height () at CZC. Also shown is RH5 km (%) from soundings (blue dashed). Shading depicts Early AR, Barrier Jet, Peak AR, and Post-CF periods (Sect. 4d).
4.3 Warm INPs, rainfall and cloud top height
Figure 4a shows the time series of INP−10 (box-and-whisker plots) and accumulated precipitation (solid lines) during the event at BBY (CZC). Note that INP−10 at CZC is consistently between 1 and 4 mL−1 before 21:00 UTC on 5 March and between 10 and 15 mL−1 thereafter (Barrier Jet period). INP−10 content was only occasionally above detection limit at BBY. INP−10 at CZC was at least an order of magnitude higher than that at BBY with rare exception. The only samples for which the AIS registered INP−10 above detection limit at BBY occurred between 22:00 and 23:00 UTC on 5 March and near 05:00 UTC on 6 March. The sample collected at BBY at 22:00 UTC contained INP mL−1, the highest at BBY. The heaviest rainfall occurred between 21:00 UTC 5 March and 03:00 UTC 6 March at both sites (Barrier Jet and Peak AR periods).
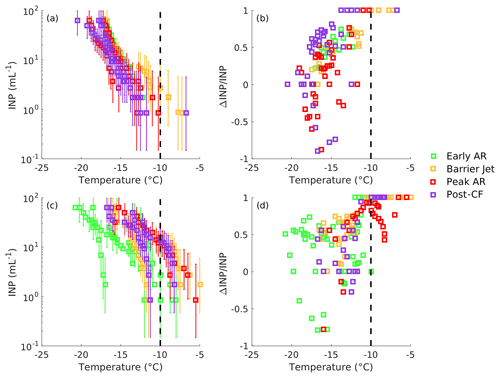
Figure 5(a) Unheated INP(T) (mL−1) from BBY precipitation during Early AR (green), Barrier Jet (yellow), Peak AR (red), and Post-CF (purple) periods. Whiskers denote technique standard error (mL−1). (b) As in a, except for ΔINP(T)∕INP(T). (c) As in (a), except for unheated precipitation samples from CZC. (d) As in (c), except for ΔINP(T)∕INP(T).
The S-band radar derivation of ETH and the relative humidity at 5 (RH5 km, %) are displayed in Fig. 4b. ETH was variable during the storm. S-band retrievals are intermittently missing between 15:00 UTC and 21:00 UTC on 5 March. Where not missing, the median value of ETH was near 5 ETH rose sharply after 21:00 UTC on 5 March, reaching an event maximum value just over 8 After 23:00 UTC on 5 March, ETH fell to a minimum value of approximately 4 km a.m.s.l. at 02:00 UTC on 6 March. This time corresponds to the maximum measured IVT (Table 2). ETH rose again near cold-front passage, passing 7 After 05:00 UTC on 6 March, ETH fell precipitously. After 06:00 UTC, S-band retrievals of ETH ceased.
After 18:00 UTC on 5 March, ETH and RH5 km are qualitatively well correlated. Echo top heights rose (fell) in the range 4 to 8 as RH5 km rose (fell). This suggests that the availability of moisture was a factor controlling the presence of upper cloud layers during the Barrier Jet and Peak AR periods. It is noteworthy that the strongest IVT and heaviest rainfall occurred when mid-levels were dry, cloud tops were lower and INP−10 was absent at BBY. We will explore whether warm INPs in BBY precipitation are related to cloud top altitude in Sect. 4.6 and 4.7.
While both sites experienced very similar weather conditions during the AR, warm INPs were much more prevalent in precipitation at CZC than at BBY. The enhancement in INP−10 (Fig. 4a) was more than a factor of 10 during most of the storm. While INP−10 remained elevated throughout the latter three periods in CZC precipitation, INP−10 presence in BBY precipitation was ephemeral. These two findings suggest that the two sites were exposed to different warm INP sources, experienced different cloud injection mechanisms or both.
4.4 Droplet freezing spectra at BBY and CZC and their response to heat treatment
Figure 5a and c show the droplet freezing activation spectra, INP(T), as measured by the AIS from precipitation samples at BBY and CZC, respectively. Vertical lines at −10 ∘C are provided so that the number of warm INPs is visually enhanced. In CZC samples, significant freezing events occurred for ∘C in all periods. Concentrations from CZC in the temperature range −15 ∘C ∘C are consistent with precipitation samples containing terrestrial bio-INPs as reported in Petters and Wright (2015). In the Barrier Jet and Peak AR periods, freezing events were detected at temperatures as warm as −5 ∘C. In agreement with Fig. 4a, few BBY samples from the Barrier Jet period and one sample from the Post-CF period similarly contained material that froze at ∘C. As time passed, the maximum INP(T) and INP−10 both increased in precipitation collected at CZC. Concentrations were greater during the Peak AR period than during Barrier Jet; and Barrier Jet concentrations were greater, in turn, than during Early AR. Rainfall also accumulated over time, with the sharpest increase in rain rate between the Early AR and Barrier Jet periods. Though the increases in rainfall rate and in INP−10 are concurrent, we do not have sufficient analysis to confidently ascribe the increasing trend in INP−10 to bioprecipitation feedback.
Further difference in INPs between BBY and CZC is found in the shapes of the freezing spectra. The freezing spectra for ∘C at BBY (Fig. 5a) are log-linear and negatively sloped with temperature. This agrees with models predicting immersion mode freezing of dust published by DeMott et al. (2010) and Niemand et al. (2012). Figure 5c, by contrast, shows freezing spectra from CZC that cannot be modeled by a simple log-linear temperature relationship. This is consistent with immersion freezing of bio-INPs (Murray et al., 2012; Tobo et al., 2013, 2014; Petters and Wright, 2015).
Figure 5b and d show the fractional change in INP(T) after precipitation samples from BBY and CZC, respectively, were heated (see Sect. 2.5). This is expressed as ΔINP(T)∕INP(T), where ΔINP(T) is the concentration from the unheated sample minus the concentration at matching temperature from the heated sample. Heating the precipitation samples prior to measuring their freezing activation denatures biological material that would otherwise have supported ice nucleation (Hill et al., 2014, 2016). It may also cause insoluble inorganic material to break apart. In some cases, this fracturing of insoluble material can lead to increases in INP(T) (McCluskey et al., 2018). For ∘C, the combination of these effects may lead to a mixture of positive and negative ΔINP(T). Additionally, heat treatment may completely nullify the ability of some bio-INPs to support freezing but may not render other types (e.g., cellular fragments) freezing inactive. At both sites, heating nullified most freezing for ∘C. The exception is for samples during the Peak AR period at CZC. Some CZC Peak AR samples partially, but not completely, lost their freezing activity for ∘C after heating. The issue of mixed trend in ΔINP(T) for ∘C is apparent in samples from both sites. INP(T) increased after heating in 23 % (11 %) of samples collected at BBY (CZC), respectively. Heat treatment and INP(T) functional form support the conclusion that biological material contributed to warm INP concentrations at CZC for most samples. However, biological material contributed to warm INP concentration at BBY only for a few samples.
Results from Sect. 4.3 and 4.4 show a large difference in INP−10 between sites, with many more collected in precipitation at CZC. Later analysis will address hypotheses related to source and impact on clouds of these INPs, but here the authors feel it is prudent to address the possibility that sample or instrument contamination led to the failure to detect warm INPs at BBY. While we cannot test the chemical composition of individual INPs, we were able to classify the chemical type of the collected insoluble precipitation residues using the aerosol time-of-flight mass spectrometer (ATOFMS). We have included ATOFMS methods, concepts of operation and particle-type classification in the Supplement. ATOFMS was used to classify single insoluble residue particles into four separate types, including a bioparticle type. The particle classification method and bioparticle type have been published in previous studies, with references provided in the Supplement. Figure S1 in the Supplement shows that the bioparticle type was the most numerous at both coastal and inland sites during all kinematic periods. While we cannot separate these bioparticles according to their marine or terrestrial sources, their ubiquity and similar concentration at both coastal and inland sites during strong onshore flow suggest that a significant number are from marine sources. These bioparticles are related to warm INPs in that all freezing events triggered for ∘C in the AIS should be caused by insoluble residue bioparticles, but not all insoluble residue bioparticles are capable of triggering freezing in the AIS (e.g., INP inactive bioparticles). Thus, Fig. S1 demonstrates that marine bioparticles were collected and preserved for laboratory analysis from both sites, while the low number warm INPs collected at the coastal site reflects the inability of the marine bioparticles collected there to trigger freezing events at ∘C.
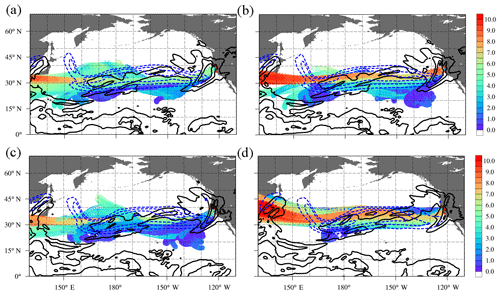
Figure 6(a) FLEXPART backward-simulated element position for releases from cloud top (“X” markers) and mixed-phase (“O” markers) layers over CZC during the Early AR period. Marker color denotes element altitude (). Period average IVT from CFS is shown by black contours from 250 to 750 every 250 . Period average horizontal wind speed in the jet layer (see Sect. 3a for layer definition) is shown by blue dashed contours from 50 to 70 m s−1 every 10 m s−1. (b) As in (a), except for the Barrier Jet period. (c) As in (a), except for the Peak AR period. (d) As in (a), except for the Post-CF period.
4.5 Qualitative transport patterns and their association with warm INPs in precipitation
The location and altitude of FLEXPART elements released in the mixed-phase and cloud top layers for each of the four periods are displayed in Fig. 6. Of note for understanding LRT warm INPs during the AR, Fig. 6b and d display the element position for releases made during the Barrier Jet and Post-CF periods. During these periods, many elements ending in the cloud top layer traveled along the upper tropospheric jet stream. Recall from Sect. 3.4 that the jet stream is located between altitudes of 6.5 and 11 ; therefore yellows, oranges and reds in Fig. 6 indicate appropriate jet altitudes. By contrast, element positions for cloud top releases during Early AR (Fig. 6a) and Peak AR (Fig. 6c) periods do not visually show transport influence from the jet stream. The difference in degree of jet stream influence between the three pre-cold-front periods likely comes from cloud top layer altitude (Table 2). Elements ending in the cloud top layer during the Post-CF period likewise appear to have traveled along the Pacific upper tropospheric jet even though cloud tops were lower during much of this period. Subsidence in the post-cold-front air mass may have linked the high-altitude UTJ and relatively lower cloud tops during the Post-CF period. The Barrier Jet and Post-CF periods were the only periods during which warm INPs were detected in precipitation collected at BBY (Fig. 5a). Figures 5a and 6 together suggest some long-range-transported warm INPs may have arrived at the AR cloud tops by traveling across the Pacific Ocean on the upper tropospheric jet stream. This result is in broad agreement with findings in Ault et al. (2011) and Creamean et al. (2013). From Fig. 6 it is also apparent that elements nearing the ARO primarily traveled along the AR during the final hours of their flight. The AR played a smaller role in transport to the cloud layers during the Post-CF period, when lower tropospheric air masses arrived at the ARO from the cold sector, or from the west of the cold front and AR, becoming incorporated into the AR just prior to arrival.
4.6 Quantitative relationships between air mass source, transport mechanism and cloud injection temperature
Table 4 presents the probability of element residence (Sect. 3.3) in the UTJ, AR, MBL and TBL. From Table 4, one can verify many of the broad qualitative findings from Fig. 6. Namely, elements were much more likely to arrive in the cloud top layer after traveling in the UTJ during the Barrier Jet and Post-CF periods, air masses arriving in the mixed-phase layer had the largest marine boundary layer influence during the Barrier Jet and Peak AR periods, and the probability that an element passed through the AR before arriving in the clouds above CZC is smallest for the Post-CF period.
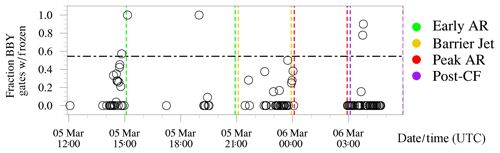
Figure 7(a) Time series of (black circles) in the unblocked layer from all KDAX scans detecting precipitation at the BBY azimuth. The all-storm mean of is shown by the horizontal dot–dash black line. Vertical dashed lines show the boundaries of kinematic periods, as coded by color in the legend.
Table 4 also offers insight to which periods were most likely to have terrestrial boundary layer air drawn into the mixed-phase cloud layer. The probability that an element both traveled through the terrestrial boundary layer and ended in either cloud layer during the Early AR period is zero. Likewise, there is zero probability that elements traveled through the terrestrial boundary layer and entered the cloud top layer during any period. The probability that an element traveled through the terrestrial boundary layer and ended in the mixed-phase cloud layer above CZC during the Barrier Jet, Peak AR and Post-CF periods is 0.062, 0.083 and 0.044, respectively. Note that all elements arrived at CZC in both layers from the west or southwest (offshore) during all periods and thus had a very short trip over or through terrestrial boundary layers. These directions of travel were the same for FLEXPART simulations over BBY (not shown). The location of BBY directly on the coastline thus yields PTBL=0 for all layers and all periods. BBY clouds were never downwind of a nearby landmass during the AR. We interpret the PTBL results to mean that approximately 4 % to 8 % of the air arriving in the mixed-phase clouds over CZC also spent time in the nearby terrestrial boundary layer. If terrestrial biomes were a source of warm INPs, mixed-phase clouds were able to entrain warm INPs into layers that could support heterogeneous freezing. Note that the concentration of INP−10 at CZC increased markedly from the Early AR period to the other periods considered (Fig. 4a), following the trend in increasing PTBL.
We can now address questions related to the warm INP source and injection mechanism. Both sites were downwind of marine particle sources for the entire storm, and the cloud layers above each site received significant contributions from the marine boundary layer during all storm periods. Only CZC precipitation contained warm INPs during all periods. The only persistent difference in air mass influence between the cloud layers over the two sites was that inflowing air to CZC passed through the terrestrial boundary layer before arriving. Thus, we conclude that the warm INPs present in CZC precipitation are predominantly terrestrial in origin and that terrestrial warm INPs are not found in BBY precipitation. There is no mechanistic explanation for the simultaneous presence (lack) of warm INPs at CZC (BBY) if the warm INP source is marine. LRT warm INPs were ephemerally present, likely at both sites. LRT warm INPs were injected at cloud top and their transport and injection were highly modulated by large-scale meteorology (e.g., the UTJ), the kinematic forcing mechanism and the availability of mid-tropospheric moisture.
Table 4 demonstrates that a transport pathway existed for terrestrial boundary layer air, potentially containing terrestrial INPs, to become injected to mixed-phase clouds. The activity of this pathway (PTBL) was modulated by the kinematic forcing regime. For example, it was inactive during the Early AR period but became active through the rest of the storm. The reader may wonder whether it is reasonable for the warm INP content of precipitation to so strongly respond (order magnitude increase, see Fig. 4a) to the onset of air parcels from the terrestrial boundary layer, given the fractional contribution of these parcels to the cloud-inflowing air mass is at most 8 %. It is prudent to note that the ambient concentration of warm INPs in the terrestrial boundary layer upstream of CZC is unknown, but work on bioprecipitation feedback (Huffman et al., 2013; Prenni et al., 2013; Morris et al., 2014; Bigg et al., 2015) demonstrates that warm INP emission often rises dramatically in response to precipitation; thus the FLEXPART analysis alone cannot estimate the increase in number concentration of cloud-inflowing terrestrial warm INPs. Additionally, Stopelli et al. (2015) argue that INPs are removed much more efficiently by precipitation than are other condensation nuclei. We can thus expect that the precipitation INP content will respond in a highly nonlinear fashion to changes in the ambient warm INP concentration of cloud-inflowing air. Indeed, because the ice-phase microphysical processes governing removal of INPs by precipitation may vary independently from air mass source, we need not expect the precipitation INP content to strongly covary with changes in terrestrial boundary layer residence.
4.7 Impact of warm INPs on mixed-phase cloud microphysics
Figure 7 displays the time series of the fraction of returns with frozen hydrometeors in the BBY azimuth () for each scan. The all-storm value of is displayed as a horizontal reference line. For the majority of the AR, was much less than the storm mean . The likelihood that KDAX observed frozen hydrometeors in the unblocked layer above CZC during the storm is . The same likelihood over BBY is . A two-category, two-site chi-square independence test was performed using all available hydrometeor class retrievals from each site. The null hypothesis, that the likelihood of observing frozen hydrometeors is independent of site, is rejected with . This result is insensitive to Yates' correction. By visual inspection of Fig. 7 and by the result of the chi-square independence test, we adopt H2 and note that frozen hydrometeors were more likely at equivalent temperatures over CZC than over BBY. As we have seen, warm INPs were also consistently more numerous, by as much as a factor of 10, in CZC precipitation. Also of note in Fig. 7 is that did not increase during the Barrier Jet period, though Barrier Jet period precipitation samples from BBY contained higher INP−10. It is possible that warm INPs over BBY were only injected through cloud top at colder temperatures, supporting the activation of other INP sources. If so, LRT warm INPs may have minimally impacted the presence of frozen hydrometeors in the mixed-phase layer. This explanation is consistent with the LRT source and injection mechanisms found for BBY in prior analyses.
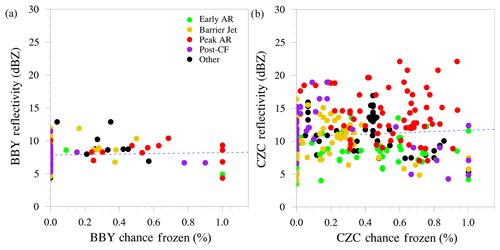
Figure 8(a) Relationship between (abscissa) and BBY mean reflectivity (dBZ – ordinate) from all KDAX scans detecting precipitation at the BBY azimuth. Marker color depicts the kinematic period each scan belonged to, as coded by color in the legend. (b) As in (a), except for and CZC mean reflectivity (dBZ).
Because we cannot directly measure the impact of warm INPs on (), we must attempt to exclude the possibility that alternate processes explain the difference in (). After noting that we ensured that the KDAX hydrometeor type sample corresponds to the same temperature range over both sites, we address the possibility that any difference in () was caused by a difference in the rate of frozen hydrometeors falling from above the KDAX unblocked layer. To address this possibility, we conducted analysis of the reflectivity in the KDAX unblocked layer over each site. For this analysis only, we relaxed the constraint on temperatures above each site in favor of also retaining 45 gates from the CZC azimuth. Radar reflectivity is closely related to the precipitation rate; thus a strong association between the KDAX unblocked reflectivity and () is considered to indicate that frozen hydrometeors are primarily falling from higher and colder layers. Radar power is also returned more strongly for liquid hydrometeors than for ice hydrometeors. Therefore, in the absence of any relationship between strength of precipitation rate and likelihood of frozen hydrometeors, we should expect a weak negative relationship between reflectivity and the likelihood of frozen hydrometeors in the unblocked layer. We also note that inter-site comparisons of reflectivity are not appropriate, since the degree of beam blockage is different over each site and we do not perform any correction to retrieved beam power based on the blockage geometry (e.g., Qi et al., 2014). The relationship between () and mean unblocked layer reflectivity for all scans is shown in Fig. 8. Note there is little to no correlation between mean reflectivity and Pfrz for either site. R2 is 0.004 (0.006) for BBY (CZC). We thus conclude that the precipitation rate had very little effect on the chance of observing frozen hydrometeors in the unblocked layer over both sites.
In this study, we examined the freezing spectra of time-resolved rainfall samples from two northern CA sites, one coastal (BBY) and one inland (CZC), during an AR with significant regional impact. We compared these spectra and their warm INP concentration (INP−10) across sites and across kinematic regimes with varying cloud depth, air mass source and transport mechanisms. These analyses were performed to address the following questions. What roles do terrestrial, marine and LRT aerosols play in determining the warm INPs during this AR? What are the transport and cloud injection mechanisms for each of these sources? When warm INPs are present in precipitation, are cloud microphysics impacted?
We summarize our findings as follows.
-
Using the AIS, we found that terrestrial warm INPs are abundant in precipitation at the inland site. It is possible that bioprecipitation feedback contributes to the terrestrial warm INP source for the inland site.
-
Through quantitative analysis of FLEXPART element residence times, we do not see evidence of marine warm INPs at either site during this storm.
-
Through similar analysis, we found that long-range-transported warm INPs may additionally be present in precipitation at both sites, but only when air mass transport patterns and kinematic regime enable cloud tops to access high-altitude-transported air masses.
-
Using the analysis of FLEXPART residence times and radar hydrometeor classifications, we found evidence that terrestrial warm INPs impacted precipitating hydrometeors in mixed-phase clouds during this storm.
The unique flow geometry and geography of the precipitation collection sites during this AR formed a critical element supporting these findings. Both sites are downwind of marine particle sources for the entire storm, and the cloud layers above each site receive significant air mass contribution from the marine boundary layer during all storm periods (Table 4). However, only the inland site shows warm INPs in precipitation during all periods (Fig. 4a and Fig. 5a, c). The only difference in air mass influence between the cloud layers over the two sites is that inflowing air to mixed-phase clouds over the inland site (CZC) passes through the terrestrial boundary layer before arriving (Table 4). When warm INPs are present in coastal site precipitation, their presence can be explained mechanistically by transport patterns and cloud top altitude favorable for LRT aerosols to become injected at cloud top. Conversely, we cannot provide an alternate hypothesis for ephemeral injection of marine warm INPs into coastal site clouds. Here we must note that it is possible that suppressed emission of marine warm INPs in nearby source regions or offshore removal led to the absence of detectable marine warm INPs during this storm but that marine INPs may be important for other ARs.
The KDAX radar hydrometeor retrievals (Fig. 7) were likewise a critical element supporting these findings. KDAX analyses show that the precipitating hydrometeor phase in clouds with −10 ∘C ∘C is significantly different at CZC than at BBY, with a higher probability of frozen hydrometeors over CZC. As we have seen (Fig. 4a), warm INPs were also consistently more numerous, by as much as a factor of 10, in CZC precipitation. We can thus hypothesize that terrestrial warm INPs became injected into mixed-phase clouds over CZC and impacted cloud hydrometeor populations through in situ ice-phase microphysics.
As we have seen in multiple analyses presented herein, the role of meteorology in modulating the warm INP source, transport and cloud injection mechanism is complex. It depends upon large-scale weather features, kinematic forcing mechanisms such as barrier and low-level jets, and the availability of moisture near cloud top. These are just the processes that determine the warm INPs in the single AR studied herein. ARs as important mechanisms for the removal of trace atmospheric constituents of remote origin and the impact of terrestrial and marine warm INPs on mixed-phase clouds and precipitation are topics deserving further study. Finally, this study demonstrated that polarimetric precipitation radar can be a useful tool to study cloud microphysics given well-constrained conditions. Future studies into the impact of aerosols on cloud microphysics may benefit from targeted polarimetric radar observations conducted in tandem with tropospheric soundings and laboratory analysis of cloud and precipitation material. It is certainly possible to enhance the analysis methods herein and deploy similar methods for multiple storms so that these or future findings may be generalized to other regions or other weather scenarios.
Datasets and code used to create analyses supporting this study are hosted within the University of California San Diego Library Digital Collections. https://doi.org/10.6075/J05X274R (Martin et al., 2019).
The supplement related to this article is available online at: https://doi.org/10.5194/acp-19-4193-2019-supplement.
GC contributed significantly to writing, preparation of figures, laboratory analysis, FLEXPART modeling and responses to review. CB oversaw analysis of precipitation samples using AIS. FC contributed significantly to the KDAX radar experiment design and to preprocessing of raw radar data. SR, BS and DL contributed to precipitation sample collection, storage, and analysis in the field and laboratory. DL additionally maintained field collection equipment and in situ documentation of collection schedules. JC is a co-principal investigator on NSF grant AGS-1632913 and advised regarding analysis and interpretation of INP spectra. FMR, HTM and KP are principal investigators on awards W912HZ-15-2-0019, AGS-1632913 and AGS-145147, respectively, and advised on manuscript writing and major findings. HTM additionally contributed to the design of precipitation sampling methods and protocols.
The authors declare that they have no conflict of interest.
The authors would like to acknowledge the University of California Davis Bodega Marine Laboratory
in Bodega Bay, CA, for providing space for sample collection, laboratory work
and housing while the field phase of this study was completed. Many thanks to
Paul DeMott and Thomas Hill of Colorado State University for helpful
guidance regarding AIS analyses. National Science Foundation grants
AGS-145147 and AGS-1632913 as well as US Army Corps of Engineers grant
W912HZ-15-2-0019 provided funding for this work. Jessie Creamean was supported
through funding from NOAA Physical Sciences Division.
Edited by: Anne Perring
Reviewed by: two anonymous referees
Ault, A. P., Williams, C. R., White, A. B., Neiman, P. J., Creamean, J. M., Gaston, C. J., Ralph, F. M., and Prather, K. A.: Detection of Asian dust in California orographic precipitation, J. Geophys. Res.-Atmos., 116, https://doi.org/10.1029/2010JD015351, 2011. a, b, c
Bigg, E. K., Soubeyrand, S., and Morris, C. E.: Persistent after-effects of heavy rain on concentrations of ice nuclei and rainfall suggest a biological cause, Atmos. Chem. Phys., 15, 2313–2326, https://doi.org/10.5194/acp-15-2313-2015, 2015. a, b
Burrows, S. M., Hoose, C., Pöschl, U., and Lawrence, M. G.: Ice nuclei in marine air: biogenic particles or dust?, Atmos. Chem. Phys., 13, 245–267, https://doi.org/10.5194/acp-13-245-2013, 2013. a
Conen, F., Morris, C. E., Leifeld, J., Yakutin, M. V., and Alewell, C.: Biological residues define the ice nucleation properties of soil dust, Atmos. Chem. Phys., 11, 9643–9648, https://doi.org/10.5194/acp-11-9643-2011, 2011. a
Creamean, J. M., Ault, A. P., White, A. B., Neiman, P. J., Ralph, F. M., Minnis, P., and Prather, K. A.: Impact of interannual variations in sources of insoluble aerosol species on orographic precipitation over California's central Sierra Nevada, Atmos. Chem. Phys., 15, 6535–6548, https://doi.org/10.5194/acp-15-6535-2015, 2015. a
Creamean, J. M., Suski, K. J., Rosenfeld, D., Cazorla, A., DeMott, P. J., Sullivan, R. C., White, A. B., Ralph, F. M., Minnis, P., Comstock, J. M., and Tomlinson, J. M.: Dust and biological aerosols from the Sahara and Asia influence precipitation in the western US, Science, 339, 1572–1578, 2013. a, b, c, d, e
DeMott, P. J., Prenni, A. J., Liu, X., Kreidenweis, S. M., Petters, M. D., Twohy, C. H., Richardson, M., Eidhammer, T., and Rogers, D.: Predicting global atmospheric ice nuclei distributions and their impacts on climate, P. Natl. Acad. Sci. USA, 107, 11217–11222, 2010. a
DeMott, P. J., Möhler, O., Stetzer, O., Vali, G., Levin, Z., Petters, M. D., Murakami, M., Leisner, T., Bundke, U., Klein, H., and Kanji, Z. A.: Resurgence in ice nuclei measurement research, B. Am. Meteorol. Soc., 92, 1623–1635, 2011. a
DeMott, P. J., Hill, T. C., McCluskey, C. S., Prather, K. A., Collins, D. B., Sullivan, R. C., Ruppel, M. J., Mason, R. H., Irish, V. E., Lee, T., and Hwang, C. Y.: Sea spray aerosol as a unique source of ice nucleating particles, P. Natl. Acad. Sci. USA, 113, 5797–5803, 2016. a
Dettinger, M. D.: Atmospheric rivers as drought busters on the US West Coast, J. Hydrometeorol., 14, 1721–1732, 2013. a
Dettinger, M. D., Ralph, F. M., Das, T., Neiman, P. J., and Cayan, D. R.: Atmospheric rivers, floods and the water resources of California, Water, 3, 445–478, 2011. a
Fan, J., Leung, L. R., DeMott, P. J., Comstock, J. M., Singh, B., Rosenfeld, D., Tomlinson, J. M., White, A., Prather, K. A., Minnis, P., Ayers, J. K., and Min, Q.: Aerosol impacts on California winter clouds and precipitation during CalWater 2011: local pollution versus long-range transported dust, Atmos. Chem. Phys., 14, 81–101, https://doi.org/10.5194/acp-14-81-2014, 2014. a
Haviland, M. G.: Yates's correction for continuity and the analysis of 2×2 contingency tables, Stat. Med., 9, 363–367, 1990. a
Heggli, M. F. and Rauber, R. M.: The characteristics and evolution of supercooled water in wintertime storms over the Sierra Nevada: A summary of microwave radiometric measurements taken during the Sierra Cooperative Pilot Project, J. Appl. Meteorol., 27, 989–1015, 1988. a
Heggli, M. F., Vardiman, L., Stewart, R. E., and Huggins, A.: Supercooled liquid water and ice crystal distributions within Sierra Nevada winter storms, J. Clim. Appl. Meteorol., 22, 1875–1886, 1983. a
Heiss, W. H., McGrew, D. L., and Sirmans, D.: NEXRAD: next generation weather radar (WSR-88D), Microwave J., 33, 79–89, 1990. a
Hill, T. C., Moffett, B. F., DeMott, P. J., Georgakopoulos, D. G., Stump, W. L., and Franc, G. D.: Measurement of ice nucleation-active bacteria on plants and in precipitation by quantitative PCR, Appl. Environ. Microb., 80, 1256–1267, 2014. a, b, c
Hill, T. C. J., DeMott, P. J., Tobo, Y., Fröhlich-Nowoisky, J., Moffett, B. F., Franc, G. D., and Kreidenweis, S. M.: Sources of organic ice nucleating particles in soils, Atmos. Chem. Phys., 16, 7195–7211, https://doi.org/10.5194/acp-16-7195-2016, 2016. a, b
Hoose, C., Kristjánsson, J., and Burrows, S.: How important is biological ice nucleation in clouds on a global scale?, Environ. Res. Lett., 5, 024009, https://doi.org/10.1088/1748-9326/5/2/024009, 2010. a
Huffman, J. A., Prenni, A. J., DeMott, P. J., Pöhlker, C., Mason, R. H., Robinson, N. H., Fröhlich-Nowoisky, J., Tobo, Y., Després, V. R., Garcia, E., Gochis, D. J., Harris, E., Müller-Germann, I., Ruzene, C., Schmer, B., Sinha, B., Day, D. A., Andreae, M. O., Jimenez, J. L., Gallagher, M., Kreidenweis, S. M., Bertram, A. K., and Pöschl, U.: High concentrations of biological aerosol particles and ice nuclei during and after rain, Atmos. Chem. Phys., 13, 6151–6164, https://doi.org/10.5194/acp-13-6151-2013, 2013. a, b
Kingsmill, D. E., Neiman, P. J., Ralph, F. M., and White, A. B.: Synoptic and topographic variability of northern California precipitation characteristics in landfalling winter storms observed during CALJET, Mon. Weather Rev., 134, 2072–2094, 2006. a
Kingsmill, D. E., Neiman, P. J., Moore, B. J., Hughes, M., Yuter, S. E., and Ralph, F. M.: Kinematic and thermodynamic structures of Sierra barrier jets and overrunning atmospheric rivers during a landfalling winter storm in northern California, Mon. Weather Rev., 141, 2015–2036, 2013. a, b
Lavers, D. A. and Villarini, G.: The nexus between atmospheric rivers and extreme precipitation across Europe, Geophys. Res. Lett., 40, 3259–3264, 2013. a
Lavers, D. A. and Villarini, G.: The contribution of atmospheric rivers to precipitation in Europe and the United States, J. Hydrol., 522, 382–390, 2015. a
Maki, L. R., Galyan, E. L., Chang-Chien, M.-M., and Caldwell, D. R.: Ice nucleation induced by Pseudomonas syringae, Appl. Microbiol., 28, 456–459, 1974. a
Martin, A. C., Cornwell, G., Beall, C., Cannon, F., Reilly, S., Schaap, B., Lucero, D., Creamean, J., Ralph, F. M., Mix, H. T., and Prather, K.: Contrasting local and long-range-transported warm ice-nucleating particles during an atmospheric river in coastal California, USA, University of California San Diego Library Digital Collections, https://doi.org/10.6075/J05X274R, last access: 1 February 2019.
McCluskey, C. S., Ovadnevaite, J., Rinaldi, M., Atkinson, J., Belosi, F., Ceburnis, D., Marullo, S., Hill, T. C., Lohmann, U., Kanji, Z. A., and O'Dowd, C.: Marine and Terrestrial Organic Ice Nucleating Particles in Pristine Marine to Continentally-Influenced Northeast Atlantic Air Masses, J. Geophys. Res.-Atmos., 123, https://doi.org/10.1029/2017JD028033, 2018. a, b
Minder, J. R., Durran, D. R., and Roe, G. H.: Mesoscale controls on the mountainside snow line, J. Atmos. Sci., 68, 2107–2127, 2011. a, b
Morris, C. E., Conen, F., Alex Huffman, J., Phillips, V., Pöschl, U., and Sands, D. C.: Bioprecipitation: a feedback cycle linking Earth history, ecosystem dynamics and land use through biological ice nucleators in the atmosphere, Glob. Change Biol., 20, 341–351, 2014. a, b
Murray, B., O'sullivan, D., Atkinson, J., and Webb, M.: Ice nucleation by particles immersed in supercooled cloud droplets, Chem. Soc. Rev., 41, 6519–6554, 2012. a, b
Murray, B. J., Ross, J. F., Whale, T. F., Price, H. C., Atkinson, J. D., Umo, N. S., and Webb, M. E.: The relevance of nanoscale biological fragments for ice nucleation in clouds, Sci. Rep.-UK, 5, 8082, https://doi.org/10.1038/srep08082, 2015. a
Neiman, P. J., May, P., Stankov, B., and Shapiro, M.: Radio acoustic sounding system observations of an arctic front, J. Appl. Meteorol., 30, 881–892, 1991. a
Neiman, P. J., Ralph, F. M., White, A., Kingsmill, D., and Persson, P.: The statistical relationship between upslope flow and rainfall in California's coastal mountains: Observations during CALJET, Mon. Weather Rev., 130, 1468–1492, 2002. a, b, c
Neiman, P. J., Martin Ralph, F., Persson, P. O. G., White, A. B., Jorgensen, D. P., and Kingsmill, D. E.: Modification of fronts and precipitation by coastal blocking during an intense landfalling winter storm in southern California: Observations during CALJET, Mon. Weather Rev., 132, 242–273, 2004. a, b
Neiman, P. J., Schick, L. J., Ralph, F. M., Hughes, M., and Wick, G. A.: Flooding in western Washington: The connection to atmospheric rivers, J. Hydrometeorol., 12, 1337–1358, 2011. a
Niemand, M., Möhler, O., Vogel, B., Vogel, H., Hoose, C., Connolly, P., Klein, H., Bingemer, H., DeMott, P., Skrotzki, J., and Leisner, T.: A particle-surface-area-based parameterization of immersion freezing on desert dust particles, J. Atmos. Sci., 69, 3077–3092, 2012. a
O'Sullivan, D., Murray, B. J., Ross, J. F., and Webb, M. E.: The adsorption of fungal ice-nucleating proteins on mineral dusts: a terrestrial reservoir of atmospheric ice-nucleating particles, Atmos. Chem. Phys., 16, 7879–7887, https://doi.org/10.5194/acp-16-7879-2016, 2016. a
Park, H. S., Ryzhkov, A., Zrnić, D., and Kim, K.-E.: The hydrometeor classification algorithm for the polarimetric WSR-88D: Description and application to an MCS, Weather Forecast., 24, 730–748, 2009. a
Petters, M. and Wright, T.: Revisiting ice nucleation from precipitation samples, Geophys. Res. Lett., 42, 8758–8766, 2015. a, b
Petters, M. D., Parsons, M. T., Prenni, A. J., DeMott, P. J., Kreidenweis, S. M., Carrico, C. M., Sullivan, A. P., McMeeking, G. R., Levin, E., Wold, C. E., and Collett Jr., J. L.: Ice nuclei emissions from biomass burning, J. Geophys. Res.-Atmos., 114, https://doi.org/10.1029/2008JD011532, 2009. a
Pitter, R. and Pruppacher, H.: A wind tunnel investigation of freezing of small water drops falling at terminal velocity in air, Q. J. Roy. Meteor. Soc., 99, 540–550, 1973. a
Prenni, A., Tobo, Y., Garcia, E., DeMott, P., Huffman, J., McCluskey, C., Kreidenweis, S., Prenni, J., Pöhlker, C., and Pöschl, U.: The impact of rain on ice nuclei populations at a forested site in Colorado, Geophys. Res. Lett., 40, 227–231, 2013. a, b
Pruppacher, H. R., Klett, J. D., and Wang, P. K.: Microphysics of clouds and precipitation, Springer, Netherlands, 2010. a, b
Ralph, F. and Dettinger, M.: Historical and national perspectives on extreme West Coast precipitation associated with atmospheric rivers during December 2010, B. Am. Meteorol. Soc., 93, 783–790, 2012. a
Ralph, F., Coleman, T., Neiman, P., Zamora, R., and Dettinger, M.: Observed impacts of duration and seasonality of atmospheric-river landfalls on soil moisture and runoff in coastal northern California, J. Hydrometeorol., 14, 443–459, 2013. a, b
Ralph, F. M., Neiman, P. J., and Rotunno, R.: Dropsonde observations in low-level jets over the northeastern Pacific Ocean from CALJET-1998 and PACJET-2001: Mean vertical-profile and atmospheric-river characteristics, Mon. Weather Rev., 133, 889–910, 2005. a, b, c
Ralph, F. M., Neiman, P. J., Wick, G. A., Gutman, S. I., Dettinger, M. D., Cayan, D. R., and White, A. B.: Flooding on California's Russian River: Role of atmospheric rivers, Geophys. Res. Lett., 33, https://doi.org/10.1029/2006GL026689, 2006. a
Ralph, F. M., Wilson, A. M., Shulgina, T., Kawzenuk, B., Sellars, S., Rutz, J. J., Lamjiri, M. A., Barnes, E. A., Gershunov, A., Guan, B., and Nardi, K. M.: ARTMIP-early start comparison of atmospheric river detection tools: how many atmospheric rivers hit northern California's Russian River watershed?, Clim. Dynam., 1–22, https://doi.org/10.1007/s00382-018-4427-5, 2018. a
Robichaud, A. J. and Austin, G. L.: On the modelling of warm orographic rain by the seeder-feeder mechanism, Q. J. Roy. Meteor. Soc., 114, 967–988, 1988. a
Rosenfeld, D., Woodley, W. L., Axisa, D., Freud, E., Hudson, J. G., and Givati, A.: Aircraft measurements of the impacts of pollution aerosols on clouds and precipitation over the Sierra Nevada, J. Geophys. Res.-Atmos., 113, D15203, https://doi.org/10.1029/2007JD009544, 2008. a
Rosenfeld, D., Chemke, R., Prather, K., Suski, K., Comstock, J. M., Schmid, B., Tomlinson, J., and Jonsson, H.: Polluting of winter convective clouds upon transition from ocean inland over central California: Contrasting case studies, Atmos. Res., 135, 112–127, 2014. a
Rutz, J. J., Steenburgh, W. J., and Ralph, F. M.: Climatological characteristics of atmospheric rivers and their inland penetration over the western United States, Mon. Weather Rev., 142, 905–921, 2014. a
Schnell, R. and Vali, G.: World-wide source of leaf-derived freezing nuclei, Nature, 246, 212–213, 1973. a
Schnell, R. and Vali, G.: Biogenic ice nuclei: Part I. Terrestrial and marine sources, J. Atmos. Sci., 33, 1554–1564, 1976. a
Stohl, A., Forster, C., Frank, A., Seibert, P., and Wotawa, G.: Technical note: The Lagrangian particle dispersion model FLEXPART version 6.2, Atmos. Chem. Phys., 5, 2461–2474, https://doi.org/10.5194/acp-5-2461-2005, 2005. a, b
Stopelli, E., Conen, F., Morris, C. E., Herrmann, E., Bukowiecki, N., and Alewell, C.: Ice nucleation active particles are efficiently removed by precipitating clouds, Sci. Rep.-UK, 5, 16433, https://doi.org/10.1038/srep16433, 2015. a, b
Tobo, Y., Prenni, A. J., DeMott, P. J., Huffman, J. A., McCluskey, C. S., Tian, G., Pöhlker, C., Pöschl, U., and Kreidenweis, S. M.: Biological aerosol particles as a key determinant of ice nuclei populations in a forest ecosystem, J. Geophys. Res.-Atmos., 118, 10100–10110, https://doi.org/10.1002/jgrd.50801, 2013. a, b
Tobo, Y., DeMott, P. J., Hill, T. C. J., Prenni, A. J., Swoboda-Colberg, N. G., Franc, G. D., and Kreidenweis, S. M.: Organic matter matters for ice nuclei of agricultural soil origin, Atmos. Chem. Phys., 14, 8521–8531, https://doi.org/10.5194/acp-14-8521-2014, 2014. a, b
Vali, G.: Quantitative evaluation of experimental results an the heterogeneous freezing nucleation of supercooled liquids, J. Atmos. Sci., 28, 402–409, 1971. a, b
White, A. B., Anderson, M. L., Dettinger, M. D., Ralph, F. M., Hinojosa, A., Cayan, D. R., Hartman, R. K., Reynolds, D. W., Johnson, L. E., Schneider, T. L., and Cifelli, R.: A twenty-first-century California observing network for monitoring extreme weather events, J. Atmos. Ocean. Tech., 30, 1585–1603, 2013. a, b, c, d, e, f
White, A. B., Neiman, P. J., Ralph, F. M., Kingsmill, D. E., and Persson, P. O. G.: Coastal orographic rainfall processes observed by radar during the California Land-Falling Jets Experiment, J. Hydrometeorol., 4, 264–282, 2003. a
Wilson, T. W., Ladino, L. A., Alpert, P. A., Breckels, M. N., Brooks, I. M., Burrows, S. M., Carslaw, K. S., Huffman, J. A., Judd, C., and Kilthau, W. P.: A marine biogenic source of atmospheric ice-nucleating particles, Nature, 525, 234, https://doi.org/10.1038/nature14986, 2015. a