the Creative Commons Attribution 4.0 License.
the Creative Commons Attribution 4.0 License.
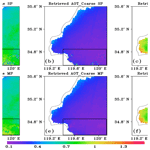
Remote sensing of aerosol properties from multi-wavelength and multi-pixel information over the ocean
Chong Shi
Makiko Hashimoto
Teruyuki Nakajima
In this study, we investigate the feasibility of a multi-pixel scheme in the inversion of aerosol optical properties for multispectral satellite instruments over the ocean. Different from the traditional satellite aerosol retrievals conducted pixel by pixel, we derive the aerosol optical thickness (AOT) of multiple pixels simultaneously by adding a smoothness constraint on the spatial variation of aerosols and oceanic substances, which helps the satellite retrieval, with higher consistency from pixel to pixel. Simulations are performed for two representative oceanic circumstances, open and coastal waters, as well as the land–ocean interface region. We retrieve the AOT for fine, sea spray, and dust aerosols simultaneously using synthetic spectral measurements, which are from the Greenhouse Gases Observing Satellite and Thermal and Near Infrared Sensor for Carbon Observation – Cloud and Aerosol Imager (GOSAT∕TANSO-CAI), with four wavelengths ranging from the ultraviolet to shortwave infrared bands. The forward radiation calculation is performed by a coupled atmosphere–ocean radiative transfer model combined with a three-component bio-optical oceanic module, where the chlorophyll a concentration, sediment, and colored dissolved organic matter are considered. Results show that accuracies of the derived AOT and spectral remote-sensing reflectance are both improved by applying smoothness constraints on the spatial variation of aerosol and oceanic substances in homogeneous or inhomogeneous surface conditions. The multi-pixel scheme can be effective in compensating for the retrieval biases induced by measurement errors and improving the retrieval sensitivity, particularly for the fine aerosols over the coastal water. We then apply the algorithm to derive AOTs using real satellite measurements. Results indicate that the multi-pixel method helps to polish the irregular retrieved results of the satellite imagery and is potentially promising for the aerosol retrieval over highly turbid waters by benefiting from the coincident retrieval of neighboring pixels. A comparison of retrieved AOTs from satellite measurements with those from the Aerosol Robotic Network (AERONET) also indicates that retrievals conducted by the multi-pixel scheme are more consistent with the AERONET observations.
- Article
(6137 KB) - Full-text XML
- BibTeX
- EndNote
Aerosols are one of the largest uncertainty factors in estimations and interpretations of the Earth's changing energy budget (Boucher et al., 2013). They exert significant and complex impacts on the radiation process through both direct and indirect effects and have detrimental influences on the air quality and public health. Since the ocean covers more than 70 % of the Earth's surface, it is indispensable to estimate the aerosol loading over the ocean. Due to the limitation of spatial and temporal coverage from ground-based measurements, satellite remote sensing has been the most efficient approach for observing the variation of aerosols over wide areas and with fine spatiotemporal resolution.
In the atmosphere–ocean system, the total radiance measured by a satellite-borne sensor at the top of atmosphere mostly comes from the atmospheric scattering, and the oceanic contribution generally accounts for ∼ 10 % of the satellite signal over open and non-glint ocean regions. In the 1990s, there were useful retrievals of aerosol optical thickness (AOT) over the global ocean, derived from the Advanced Very High Resolution Radiometer (AVHRR) generated by NOAA (Stowe et al., 1992). Moreover, Nakajima and Higurashi (1997) and Mishchenko et al. (1999) propose improved two-channel methods for deriving more information on aerosols using red and near-infrared band (NIR) measurements from the AVHRR. With the advances in the satellite instrument, several improved algorithms have also been developed using more channels that cover band ranges from ultraviolet (UV) to NIR or shortwave infrared (SWIR) to retrieve both AOT and aerosol type (Tanré et al., 1997; Torres et al., 1998; Higurashi and Nakajima, 2002; Remer et al., 2005; Kim et al., 2007; Lee et al., 2012; Wang et al., 2017; Choi et al., 2018) or layer height (Xu et al., 2017). These algorithms have been successfully adopted in the operational processing of aerosol retrieval for polar or geostationary satellite instruments with good accuracies. In addition, multiple angular or polarization measurements are conducive for deriving aerosol properties by providing more information content over the ocean (Martonchik et al., 1998; Goloub et al., 1999). As for the ocean color (OC) retrieval, such as that used in the Sea-Viewing Wide Field-of-View Sensor, an atmospheric correction scheme is always adopted to derive the AOT from sets of candidate aerosol modes based on the satellite measurements at red or NIR channels (Gordon and Wang, 1994). Specifically, these approaches compare observed and pre-calculated radiances or polarized radiances from lookup tables to estimate aerosol optical properties, assuming that the ocean surface reflectance can be empirically estimated or neglected. For example, the Moderate Resolution Imaging Spectroradiometer (MODIS) Collection 5 operational over-ocean algorithm specifies zero water-leaving radiance for all channels (550, 650, 860, 1240, 1600, and 2120 nm), except at 550 nm, where a value of reflectance 0.005 is assumed (Remer et al., 2005). These assumptions are generally reasonable due to the high absorption effect of seawater in or beyond the NIR bands in which the ocean surface can be assumed to be black. However, there is still about 50 % discrepancy in the mean AOT from several prominent aerosol products over the ocean, with the differences appearing both in terms of magnitude and temporal tendency (Li et al., 2009). Apart from the different calibration or cloud screening schemes used in different algorithms, correction of the surface effect is one of the main factors causing such discrepancy (Li et al., 2009). As for the aerosol retrieval at shorter bands or in turbid waters, the backscattering of oceanic particulates could be higher as a result of contributions from the underwater field to the satellite-observed reflectance should be accounted for. To further consider the effects of oceanic substances in the retrieval of aerosols, other studies have been conducted to simultaneously derive AOT and water-leaving radiance using coupled atmosphere–ocean radiative transfer models (Doerffer and Fischer, 1994; Stamnes et al., 2003; Fan et al., 2017; Shi and Nakajima, 2018), or a sea surface reflectance model (Sayer et al., 2010), as well as the combined polarization information (Hasekamp et al., 2011; Knobelspiesse et al., 2012; Gao et al., 2018).
Like most satellite retrievals, the aerosol inversions are performed over single pixel, one at a time, before independently moving to another pixel, independently. To overcome the deficiency of the possibly limited information contained in a single pixel regarding all the retrieved parameters, Dubovik et al. (2011) develop a generalized aerosol retrieval system known as the Generalized Retrieval of Aerosol and Surface Properties (GRASP) to derive aerosol properties, which uses polarization and multi-angle, multi-wavelength, and multi-pixel information integrated into a sophisticated statistically optimized scheme, based on the assumption that the variations of retrieved parameters are horizontally and temporally smooth from pixel to pixel and/or from day to day. A similar horizontal constraint scheme based on the adjacent pixel information has also been adopted in the retrieval of aerosol and water-leaving radiance over the open ocean (Xu et al., 2016), as well as a new correlated multi-pixel inversion approach based on the principal component analysis (Xu et al., 2019). Since these algorithms adopt polarization and multi-angle measurements that many imagers cannot provide, Hashimoto and Nakajima (2017) develop a satellite remote-sensing algorithm to retrieve aerosol properties using multi-wavelength and multi-pixel information (MWMP). Adhering to the implementation of Hashimoto and Nakajima (2017), who implement aerosol retrieval over land, in this study, we investigated the potential value of a multi-pixel scheme combined with multiple wavelength information in the remote sensing of aerosols over several oceanic conditions, i.e., both open and coastal waters, as well as over the land–ocean interface region.
In this study, we firstly use a well-established coupled atmosphere–ocean radiative transfer model to simulate the spectral measurements, namely those of the Greenhouse Gases Observing Satellite and Thermal and Near Infrared Sensor for Carbon Observation – Cloud and Aerosol Imager (GOSAT∕TANSO-CAI, hereafter referred as CAI), in four bands for two cases of open and coastal waters. Statistical samples of the MODIS ocean color data selected from two representative regions in the Lanai and Yellow seas are adopted to model the underwater optical properties in the simulation retrieval experiment. Then, we use the optimal estimation theory to investigate the effects of the multi-pixel scheme on the retrieval of aerosols by studying various numerical results in different ocean conditions. Finally, we conduct the retrievals based on the real CAI measurements and make comparisons with those from the MODIS standard aerosol products and in situ observations from the Aerosol Robotic Network (AERONET; Holben et al., 1998; Dubovik and King, 2000).
In the atmosphere and ocean system, the satellite-received multispectral radiance or reflectance vector at a subdomain of an imagery with multiple pixels can be expressed as y, with the dimensions of , where Nλ is the number of measured wavelengths, and Nu and Nv are the numbers of pixels in two horizontal orthogonal directions of the subdomain, respectively. The measurement vector can be related to the state vector x and error ε as follows:
where x denotes the set of unknown parameters in the subdomain with the dimensions of . Here, Np is the number of parameters being retrieved in each single pixel, and F(x) is the forward radiative transfer model, which describes the knowledge of the measurement process and physics of the problem. ε is the error vector that consists of the measurement and model errors. The inversion problem is deriving x from observation y by inverting the forward model F at a subdomain, i.e., simultaneous determination of the retrieved parameters with number of Np in each pixel of the subdomain with dimensions of Nu×Nv. Since the inversion is often an ill-posed problem, a prior constraint for the state vector is usually considered. Moreover, assuming that the aerosol loading is a slowly variable function of the horizontal direction (Dubovik et al., 2011; Hashimoto and Nakajima, 2017), as is the chlorophyll a concentration (Chl; Xu et al., 2016), and is extended to the sediment and colored dissolved organic matter (CDOM), which are more conspicuous in turbid waters, a spatial smoothness constraint on the variation of aerosol and oceanic substances can be added during the retrieval. If we treat the forward model as linear in the vicinity of the true state, the inversion tends to solve the equation set as follows:
where K is the Jacobian matrix expressing the sensitivity of the model to an infinitesimal change in each retrieved parameter as , xa is the a priori estimate of the state vector before retrieval, and εa is the a priori error. Bu and Bv are the boundary conditions in which values are determined from the neighboring subdomains; Du and Dv comprise the second differential coefficient matrix given by the Phillips–Twomey method (Phillips, 1962; Twomey, 1963), and these are adopted as smoothness constraints in each horizontal direction of the subdomain; εu and εv indicate the uncertainties of these derivatives in the u and v directions, respectively. It should be noted that this type of smoothing constraint has also been used in the retrieval of aerosol size distribution derived from the ground-based measurements of AERONET and SKYNET (King et al., 1978; Nakajima et al., 1996; Dubovik and King, 2000). Provided that the measurement and a priori error are characterized by a Gaussian probability distribution function, the inversion can be changed to minimize the cost function as follows:
where γu and γv denote Lagrange multipliers, which represent the strength of the spatial smoothness constraint on the norm of the second derivatives in two horizontal directions. In principle, these two parameters are interpreted by the reciprocal of the covariance of the horizontal distribution variation of the state vector in two directions, whereby the larger the Lagrange multiplier, the stronger the smoothing constraint. Sε is the measurement error covariance matrix, and Sa is the variance–covariance matrix estimated by a priori state values in which the off-diagonal elements are assumed to be 0. The optimal solution of Eq. (3) can be solved by the Gauss–Newton iteration method, calculated as follows:
with
where xi is the state vector to be retrieved at the ith iteration of the subdomain, and I is the unit matrix with a size of Np. After several iterations, the retrieved parameters over multiple pixels at the subdomain can be converged and derived simultaneously. It should be noted that when the Lagrange multipliers, i.e., γu and γv, are zero, which means that no spatial smoothness constraints are implemented in the retrieval, Eq. (4) is changed to the typical solution of the maximum a posteriori method used in the traditional single-pixel retrieval (Rodgers, 2000).
In this study, we use a coupled atmosphere–ocean vector radiative transfer model, i.e., Pstar, for the forward radiation calculation (Ota et al., 2010). Pstar was originally developed for the simulation of radiative transfer in the coupled atmosphere–ocean system by accounting for the polarization effects. It is developed based on the scalar version of Rstar (Nakajima and Tanaka, 1986, 1988) and was improved by Shi et al. (2016) to simulate the radiation process in turbid waters by combining a three-component bio-optical ocean module and water-leaving radiance calculation scheme. The accuracy of the radiative transfer scheme in the model has been proven by a serious intercomparison from IPRT (International Radiation Polarized Radiative Transfer; Emda et al., 2015) in the atmosphere and the standard underwater radiative transfer problem in the ocean system provided by Mobley et al. (1993) (Shi et al. 2015).
For the aerosol modeling, we adopted a sophisticated scattering approach that combines external and internal mixture schemes. It is assumed that three kinds of aerosol modes, i.e., fine, sea salt and dust, exist in the atmosphere, of which each mode grows and changes its refractive index with increasing humidity independently (external mixing). Moreover, an internal mixture of water-soluble, dust-like, and soot exists within the fine aerosol (internal mixing) of which the refractive index is calculated by the sum of each internal component contribution based on its volume fraction. It should be noted that the dust aerosols are considered to be non-spherical, and the scattering phase matrix is calculated using Dubovik et al.'s (2002) method. Since the CAI has only four spectral bands without multi-angle or polarization information, the size distribution for each mode is fixed in this study with log-normal assumption (Shettle and Fenn, 1979), even though they differ from pixel to pixel in reality and a Gamma distribution for sea salt aerosol might be more appropriate (Yu et al., 2019). However, a comprehensive retrieval experiment covering different ocean regions has demonstrated the reasonability of this assumption in the retrieval of the AOT and water-leaving radiance based on the systematic comparison with those from AERONET OC measurements (Shi and Nakajima, 2018).
For the ocean, we assume a four-layer system of infinite depth coupled with a wind-generated rough surface model in which the reflectance and transmission matrices are calculated based on the scheme of Nakajima and Tanaka (1983). Moreover, a three-component bio-optical ocean module is implemented to model the inherent optical properties (IOPs) of oceanic substances, i.e., Chl, sediment, and CDOM (Shi et al., 2016). To model the IOPs of seawater, particularly in the UV bands, we used newly compiled data from Lee et al. (2015), which have provided better closure for the remote-sensing reflectance (Rrs), i.e., the ratio between the water-leaving radiance and the downward irradiance just above the ocean surface, in the UV-visible domains. It should be noted that we use the Rrs instead of the water-leaving contribution to the satellite-received radiance due to its important effect on the ocean color retrieval in this study.
4.1 Retrieval using synthetic measurements
We focus on the retrieval from the GOSAT∕TANSO-CAI. GOSAT is mainly designed to measure the carbon dioxide loading using the TANSO Fourier Transform Spectrometer. In addition, the satellite carries the Cloud and Aerosol Imager (CAI) with four channels (380, 674, 870, and 1600 nm), ranging from UV to SWIR bands for cloud screening and aerosol detection. We firstly simulate the synthetic measurements from the CAI in four spectral bands (Nλ=4) based on the improved Pstar model. Moreover, we define a 5×5 pixel region as one subdomain, i.e., , though there is no limitation in these definition if the computer resource is allowed. The geometric information is determined by the mean values of the CAI observations at the solar zenith angle of 27∘ ±1, satellite zenith angle of 30∘ ±1, and relative azimuth angle of 150∘ ±1 at the subdomain (Hashimoto and Nakajima, 2017).
The simulated true AOT values at 500 nm for each mode are given as 0.02, 0.1, 0.2, and 0.3, respectively. The total AOT is the sum of each AOT in a random mixture. We defined the soot fraction in fine aerosol as randomly ranging from 0.5 % to 1.5 %. In the ocean surface, a moderate wind speed of 5 m s−1 is assumed. From the widely used suite compiled by the NASA Ocean Biology Processing Group, and based on the large statistical ocean color sample of MODIS, we selected two representative classes of oceanic scenarios: the Linai region, in which the water is typically clear, with climatological values of Chl, a_443, and bbp_443 being about 0.056 mg m−3, 0.017, and 0.0014 m−1, respectively, and the Yellow Sea, in which the water is typically coastal, with climatological values of Chl, a_443, and bbp_443 being about 3.00 mg m−3, 0.35, and 0.039 m−1, respectively, where a_443 denotes the total absorption coefficient of ocean at 443 nm, and bbp_443 is the total backscattering coefficient of oceanic particulates at 443 nm. It should be noted that the used Chl products are derived by the OCI algorithm of Hu et al. (2012), and a_443 and bbp_443 products are derived by the quasi-analytical algorithm (QAA) method of Lee et al. (2002), respectively. We assume the aerosol and oceanic-substance spatial distributions to be homogeneous in the 5×5 pixel regions. In total, we have 64 atmospheric cases of simulated observation data with a Gaussian random noise of 2 % standard deviation as measurement errors for each oceanic condition. Then eight parameters of each pixel, i.e., AOTs of fine, sea spray, and dust; the volume soot fraction in fine aerosol; wind speed; and concentrations of Chl, sediment, and CDOM for the whole subdomain, are determined simultaneously using Eq. (4). The soot fraction in fine aerosol is defined as the retrieval parameter owing to its high absorption effects. The a priori conditions are randomly defined in a ±50 % range of the true values, except for the soot fraction, which has a fixed value of 0.01. To investigate the feasibility of the multi-pixel method in the retrieval, we analyzed the simulation data, adopting different γ values of 0.0, 0.1, 0.5, 1.0, 1.5, 2.0, and 3.0. The general simulation and retrieval setup are summarized in Tables 1–2.
Table 1Aerosol and oceanic mode used in this study.
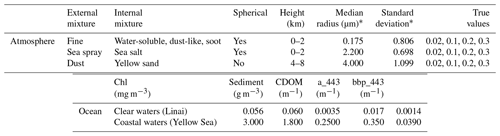
* The volume size distribution of aerosol particles is assumed to follow a log-normal function, , where V is the aerosol volume density, rmv is the median radius, and σv is the standard deviation.
Table 2Retrieval experiment sets for state vector and measurement for the CAI.
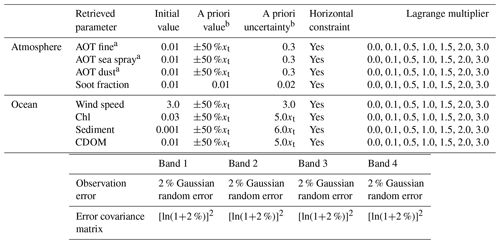
a AOT refers to the aerosol optical thickness at 500 nm. b xt is the true value defined in this simulation.
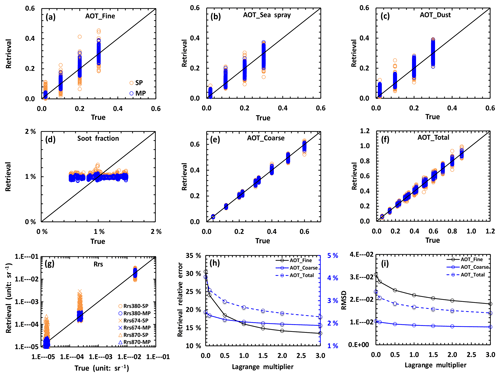
Figure 1Retrieved and true AOT values over homogeneous open-ocean areas for fine (a), sea spray (b), dust (c), coarse (i.e., sum of sea spray and dust; e), and total (i.e., sum of fine, sea spray, and dust; f) aerosols at 500 nm, as well as the soot fraction (d) and spectral remote-sensing reflectance (Rrs; g) for Lagrange multipliers of 0.0 (i.e., single-pixel method, denoted as SP) and 3.0 (i.e., multi-pixel method, denoted as MP). Retrieved relative error (h) and root-mean-square deviation (RMSD; i) are shown for each Lagrange multiplier value.
Figure 1 shows the retrieved AOT and spectral Rrs values with true conditions as well as the statistical results of the retrieved relative error and root-mean-square deviation (RMSD) at different values of γ for the open ocean. Note that Fig. 1a–g show only the retrievals of 0.0 and 3.0 at γ, which denote the use of no spatial smoothness constraint, i.e., the traditional single pixel method, and allowed a variation of about 1.35 times the retrieved parameters constrained from neighboring pixels, respectively. The results indicate that the accuracy of the retrieved AOT of each mode is generally improved by using the spatial smoothness constraint, i.e., the multi-pixel method, to correct the retrieval bias induced by measurement errors. Specifically, the retrieved relative error and RMSD of the fine AOT decrease from 30.51 % and 0.031 to 13.45 % and 0.018, respectively, when the γ values change from 0.0 to 3.0 (Fig. 1h–i), which indicates the effectiveness of the multi-pixel method in aerosol retrieval. Coarse aerosols (sum of sea spray and dust) can also be well derived, which is partly due to the adoption of the SWIR channel observation (Fig. 1e), even though larger errors are shown for the inversion of each coarse aerosol, i.e., AOT of sea spray and dust (Fig. 1b–c), compared with the true values. Additionally, due to the more significant improvement in the retrieval of the fine AOT, the multi-pixel scheme contributes to a better estimation of the total AOT (Fig. 1f). In contrast, the soot fraction is difficult to retrieve due to its low sensitivity to measurements, even though an UV channel observation, i.e., 380 nm, is implemented by the CAI, for which the retrieval results are highly dependent on the a priori value (1 % in this case; Fig. 1d). In regard to the spectral Rrs, we find that the multi-pixel method helps to facilitate the consistency of the retrieval with the true values (Fig. 1g) in comparison to those derived by the single pixel method, and this finding is similar to that of Xu et al. (2016) over open oceans. The low values of Rrs at 860 nm and at 674 nm also support the reasonability of our previous black ocean assumption in those bands in the two-channel aerosol inversion (Nakajima and Higurashi, 1997). However, the underwater influence in the retrieval of aerosols at UV channels over the open ocean is suggested to be considered due to the higher backscattering effect of ocean body.
Over coastal waters, the sediment and CDOM, which exist with higher concentrations in the ocean and show generally similar inherent optical properties to the fine aerosol and soot, exert non-negligible effects on the aerosol retrieval. Compared with the inversion of fine AOT over the open ocean (Fig. 1a), the retrieval in the low-aerosol loading over coastal regions shows larger biases when using the traditional single-pixel method, mainly due to the contamination of oceanic sediment (Fig. 2a). Nevertheless, retrieval errors can be effectively reduced using the multi-pixel scheme, with the relative error and RMSD decreasing about 27.4 % and 0.02234 (Fig. 2k–l), respectively. This improvement is also due in part to the better estimation of the spectral Rrs (Fig. 2j). It is demonstrated that the retrieved accuracy of the coarse AOT is generally similar to that in clear waters (Fig. 2e), which is partly attributable to the utilization of SWIR measurements that are not sensitive to sediment and can be used in the atmospheric correction over turbid waters (Wang and Shi, 2007). Moreover, it remains difficult to retrieve the absorptive soot over coastal waters, even when using the multi-pixel constraint (Fig. 2d). With regard to the underwater retrieval, significant improvements are evident in the inversion of oceanic substances, particularly for the sediment and CDOM, after implementing the spatial smoothness constraint (Fig. 2g–i). Such improvements also contribute to the better retrieval of the spectral Rrs, with the relative errors in the first three CAI bands decreasing from 41.87 %, 17.76 %, and 15.94 % to 22.87 %, 8.09 %, and 8.23 %, respectively. Generally, there are higher averaged kernel matrix values for fine and coarse aerosols, sediment, and CDOM than those for the soot fraction, Chl, and the wind speed in the non-glint cases during the retrieval. It should be noted that we used relatively accurate a priori values for the AOT estimation in a range of ±50 % of the true conditions, however, the retrieved sea spray and dust will have larger biases when xa exceeds true values more largely, owing to the limited spectral information of CAI and similar optical properties of these two modes. However, their sum, i.e., the total coarse AOT, can still be determined well and exhibits no obvious dependence on the a priori conditions.
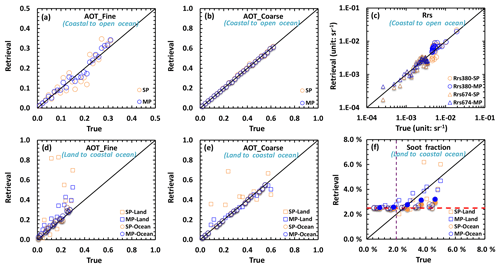
Figure 3Simulation experiment for AOT retrieval of fine and coarse aerosols, spectral Rrs with (blue points denoted as MP with γ values of 1.0) and without (orange points denoted as SP) multi-pixel constraints over inhomogeneous areas from coastal to open-ocean regions (a–c), and retrievals from land (ground covered by reddish-brown fine sandy loam) to coastal ocean regions (d–f). The purple line of (f) is the boundary line with AOT_Fine at 500 nm of 0.1 (right part of boundary line denotes retrieval under the condition of AOT_Fine greater than 0.1, and vice versa). The red line of (f) shows the a priori values used in this simulation. The retrieved soot fractions over the ocean nearest to the coastal line are denoted by solid circle in (f). Land surface reflectances at 380, 674, 870, and 1600 nm are 0.1098, 0.2775, 0.3630, and 0.4790, respectively.
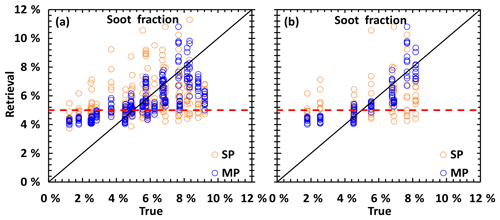
Figure 4Retrieved volume soot fraction in the fine aerosol over the coastal ocean with the condition of homogeneous aerosol distribution for the land–ocean interface region; (a) is the retrieval with AOT of fine > 0.1, and (b) is the retrieval with AOT of fine > 0.1 and ratio of fine AOT > 0.5. The red line shows the a priori values used in this simulation.
The above results demonstrate the effectiveness of the multi-pixel scheme in the retrieval of aerosols over homogeneous atmosphere–ocean areas. To consider the retrieval under inhomogeneous conditions, we conducted further inversion experiments in two situations. First, we assumed that the aerosol loading and oceanic substances change continuously from coastal to open ocean, with a large spatial variation in the 5×5 pixel subdomain. Results indicate that the multi-pixel strategy still performs better retrieval in this case, as shown in Fig. 3a–c, particularly for the estimation of fine aerosols. Similar to the retrievals over the homogeneous region, the spectral Rrs can be better derived using the multi-pixel scheme, especially over coastal regions (low values of the Rrs at 380 nm and high values of the Rrs at 674 nm), but the traditional single-pixel method tends to yield larger bias estimations for the retrieval (Fig. 3c). Another inversion experiment is performed for the retrieval over land (with a higher aerosol loading and soot fraction) and coastal ocean interface regions in the subdomain. In this simulation, the land surface is assumed to be reddish-brown fine sandy loam with spectral reflectance values of 0.1098, 0.2775, 0.3630, and 0.4790 for the four CAI bands, and values are selected from the ECOSTRESS spectral library (https://speclib.jpl.nasa.gov/library, last access: 15 February 2019). For the aerosol retrieval over land, we make simultaneous determinations of the AOT and spectral surface reflectance (Ag) with randomly defined a priori values of Ag in a range of ±10 % of the true conditions and assumed spectral uncertainties of 0.02, 0.02, 0.02, and 0.001 for the CAI channels, respectively. The results indicate that the single-pixel method generally overestimates the fine and coarse AOT values over sand due to the high ground reflectance. However, the retrieval accuracy over the sand surface improves significantly by constraining the spatial aerosol variation in the subdomain, which also benefits from the better AOT estimation over the coastal ocean region (Fig. 3d–e). With regard to the retrieval of the soot fraction (SF; Fig. 3f), it remains difficult to derive over-ocean areas with a high dependence on the a priori value (red line of Fig. 3f), whereas absorptive soot can be better estimated over land regions due to the high reflectance of the ground surface by providing more significant information to the retrieval. Moreover, the multi-pixel scheme promotes the inversion of the SF, particularly in high aerosol conditions, which is similar to the research of Hashimoto and Nakajima (2017) conducted over the whole land regions. The performance of retrieval for absorptive soot with dependence on the aerosol loading over the land, where the better retrievals are identified in the condition of fine AOT at 500 nm over 0.1 (Fig. 3f) in this study, also supports the finding that errors of retrieved single scattering albedo decrease with increasing AOT for the AERONET (Dubovik et al., 2000). It is interesting that the retrieved accuracy of the SF nearest to the coastal line over the ocean (solid circles of Fig. 3f) tends to be improved using the multi-pixel method, though it is slightly improved. This improvement is more significant in the homogeneous aerosol distribution and dominated fine-mode conditions over the land–ocean interface region, as shown in Fig. 4. The availability of retrieval for the absorptive soot over the dark surface, i.e., the ocean region, benefits from the spatial smoothness constraint from the better-derived SF over the bright surface, i.e., sand ground, where the surface albedo is near or over the neutral reflectance defined by Kaufman (1987). We then derived the relationship between the neutral reflectance (An) in which the apparent reflectance does not change with AOT and the single scattering albedo (ω), asymmetry factor (g), and phase function (P(cos Θ)) of aerosols based on the single scattering and two-stream approximation over land, calculated as follows:
where μ and μ0 are the cosines of the satellite and solar zenith angle, respecitvely. It is demonstrated that the neutral reflectance of band 2 of the CAI is ranged from 0.232 to 0.275 when the asymmetry factor and phase function are 0.7 and 0.0142, respectively, corresponding to the single scattering albedo of 0.935 and 0.950 with the soot fraction of 2.05 % and 5.10 %, which is generally similar to the threshold values of the retrieved SF in Figs. 3f and 4b. However, these threshold values are just the specific case used in this study and are also dependent on the ratio of fine AOT in real conditions.
4.2 Retrieval using real CAI measurements
Following the simulation retrieval experiment performed using synthetic spectral measurements, we then apply the proposed algorithm to the real CAI data for deriving aerosols over the ocean. Radiometric correction was conducted as prescribed in Shiomi et al. (2010). With regard to the ancillary data, we use the surface pressure and wind speed data from the National Centers for Environmental Prediction (NCEP) to correct the Rayleigh scattering and sea surface reflectance, respectively, as well as the relative humidity data to account for the effect of aerosol hygroscopic growth. The gas absorption is processed by a correlated k-distribution approach (Sekiguchi and Nakajima, 2008) where several main absorptive gases are considered, and the column ozone data are adopted from the ozone monitoring instrument (OMI). In particular, we used a relatively high spatial smoothness constraint with a γ value of 1.0 for each horizontal orthogonal direction in the multi-pixel scheme. Moreover, to keep the consistency between each subdomain, the boundary conditions of Bu∕v used in Eq. (4) are determined by the retrieved results derived from the neighbor subdomains.
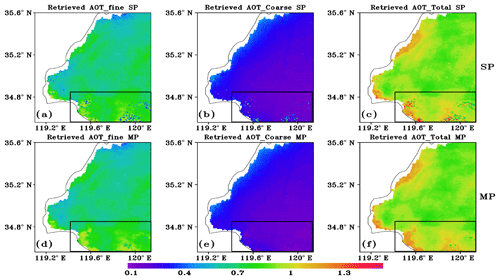
Figure 5Spatial distributions of retrieved fine, coarse, and total AOTs from CAI measurement on 13 March 2012 using traditional single-pixel method (denoted as SP) and multi-pixel scheme (denoted as MP).
Figure 5 compares the spatial distributions of retrieved fine, coarse (sum of sea spray and dust), and total AOTs using single- and multi-pixel methods. Results show that the derived AOTs by the single-pixel approach have a generally similar spatial distribution to those retrieved by the multi-pixel method, which the fine aerosol dominates. However, irregular dotted variations with abnormal retrieval results are shown in some pixels (black box in Fig. 5) when conducting the single-pixel retrieval. Although it is difficult to support these irregular dotted distributions being real, the investigation of the posterior error in those pixels demonstrates that the retrieved uncertainties are generally higher than those of other pixels (not shown). Such kinds of occasionally irregular dotted variations derived by the single-pixel method have also been identified in the aerosol retrieval over land (Hashimoto and Nakajima, 2017), which we rather consider to be caused by errors in the single-pixel inversion that tends to be affected by various observation noises. On the contrary, the multi-pixel scheme is more robust to these factors, so the irregular dotted variation of retrieved AOT can be effectively improved when considering the spatial smoothness constraint during the retrieval, as shown in Fig. 5d–f, which allows reduction of the retrieval errors constrained by adjacent pixels.
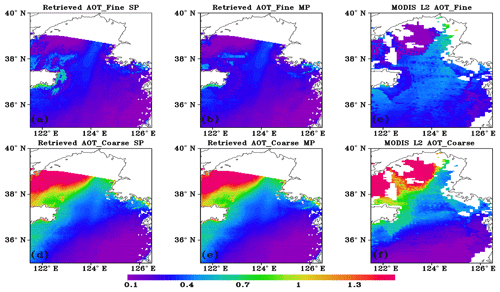
Figure 6Monitoring of the Asian dust event from CAI and MODIS. Spatial distribution of CAI-derived AOT for fine and coarse aerosols from the single-pixel method (a–b), multi-pixel method (d–e), and the MODIS Terra aerosol Level 2 products (c and f) on 27 April 2012 over the Yellow Sea.
Another retrieval experiment is performed on the monitoring of the Asian dust event from the CAI. Studies have demonstrated that dust aerosols carried by the dust storm in East Asia exert significant influence on the local ecosystem and environmental pollution (Huang et al., 2014). Figure 6 shows the spatial distribution of retrieved fine and coarse AOTs from the CAI on 27 April 2012 over the Yellow Sea. In order to have a better validation for the current algorithm, the MODIS standard Level 2 aerosol products derived using more channels, with the satellite overpass time about 3 h later than that of the CAI, are also adopted as comparison. Results show that a relatively obvious transport belt for fine aerosols between the south of the Shandong Peninsula and the middle of the Yellow Sea is derived by the CAI (Fig. 6a); meanwhile, significant dust storms are determined in the north of Yellow Sea (Fig. 6d), where the retrieved coarse AOTs at 500 nm are over 1.5 in the high-density areas. It is demonstrated that the derived AOTs for fine and coarse aerosols are generally consistent with the MODIS standard aerosol products with similar spatial distributions (Fig. 6c and f). In addition, the derived AOTs from the CAI with the multi-pixel method (Fig. 6b and e) around the Shandong Peninsula are in more agreement with MODIS aerosol products than those retrieved by the single-pixel approach, which implies the effectiveness of the multi-pixel scheme in the inversion of aerosols, particularly for fine aerosol. It should be noted that the derived fine AOT values from the CAI seem to be generally lower than those obtained using MODIS products over the Yellow Sea. Although our algorithm divides the coarse aerosols into sea spray and yellow sand, it is more appropriate to use their sum, i.e., coarse AOTs, than the yellow sand as the indicator of the Asian dust transport, since the retrieval errors of coarse AOTs determined by the CAI are much lower than those of individual yellow sand particles, as shown in Fig. 2c and e. Such a deficiency in distinguishing the sea spray and dust is expected to be better improved using the upcoming Cloud and Aerosol Imager 2 (CAI2) with seven channels (340, 380, 443, 550, 674, 869, and 1630 nm) by providing more measurement information.
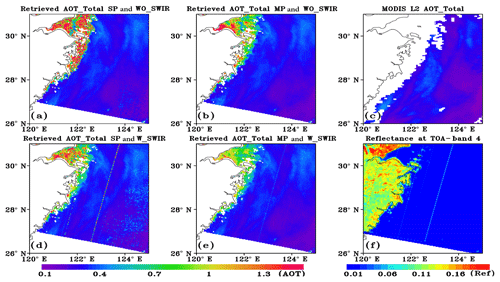
Figure 7Comparison of CAI-retrieved total AOT by the single-pixel method without or with the SWIR band, i.e., 1600 nm (a and d, respectively), with those from the multi-pixel method without or with the SWIR band (b and e, respectively), and the spatial distributions of MODIS Aqua Level 2 AOT products (c) and satellite-received reflectance at band 4 of CAI (f) on 3 December 2013.
Retrieval of aerosols over extremely highly turbid waters is still a challenging problem due to the significant contamination from the backscattering of oceanic particulates, currently. Taking the Hangzhou Bay (HZB) as a sample region, the total suspended particulate matter can be over 1000 mg L−1 sometimes (He et al., 2013), which contributes a substantial proportion into the satellite signals as a result of the aerosol being generally difficult to accurately derive. In order to investigate the feasibility of the multi-pixel scheme in the retrieval of the AOT over highly turbid water, we try to apply the current algorithm in such a circumstance. Figure 7 shows the comparison of the retrieved total AOT by using the single- and multi-pixel method with or without using the SWIR band of the CAI, i.e., 1600 nm, with those from the MODIS aerosol products in December–March 2013 over the East China Sea. It should be noted that there are several high-error observation belts for band 4 in this image (Fig. 7f) due to some instrument problems, but the data are available for most regions including the HZB. Results demonstrated that the retrieved AOTs by the CAI with or without using the SWIR measurement are all consistent with those of the MODIS aerosol product (Fig. 7c) beyond the coastlines, however, the estimated AOTs from the CAI using different strategies around highly turbid regions show large differences. Generally, the derived AOTs without using SWIR measurements (Fig. 7a) demonstrate obviously higher values than those retrieved by adding SWIR information (Fig. 7d) near coastal region. This is because the satellite reflectance at SWIR channels is much less sensitive to the suspended sediment than that at visible bands in turbid waters, so the aerosols can be estimated without significant contamination of sediment (Wang and Shi, 2007) based on the SWIR observation. Although we simultaneously conduct the oceanic sediment retrieval in the algorithm, it is still difficult to use four spectral measurements to estimate at least five free variables (AOT of fine, sea spray and dust, sediment, and CDOM) in the high backscattering surface condition, where the retrieval could be degenerated. Nevertheless, such a deficiency can be improved using the multi-pixel scheme even though the SWIR measurements are not used (Fig. 7b), which indicates the potentiality of the multi-pixel strategy in the aerosol retrieval over highly turbid waters, particularly for those multispectral instruments without the SWIR observation.
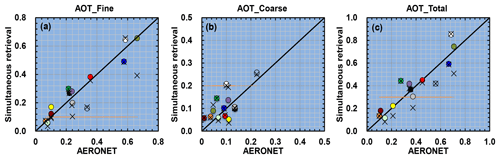
Figure 8Comparison of retrieved AOT values for fine, coarse, and total aerosols at 500 nm from CAI with those from AERONET observations. Circles and crosses indicate the retrieved values at pixels closest to AERONET sites with or without multi-pixel method implementation, respectively. Orange dotted lines denote a priori values.
To further investigate the feasibility of this algorithm, two in situ data from the AERONET, i.e., the Ieodo Station and Gageocho Station, are used for the validation. To examine the dependence of this retrieval on the a priori information, we set fixed values of xa for all cases. We selected the retrieved AOTs from the pixel closest to the AERONET site in the subdomain. The results indicate that the retrieved AOT values for fine and coarse aerosols, as well as the total aerosols, are all consistent with those of the AERONET observations without significant dependence on the a priori information (Fig. 8). It should be noted that the simultaneous retrieved AOTs for fine and coarse aerosols are denoted in same color. Generally, increased accuracy in the determination of AOTs is demonstrated by using the multi-pixel method (shown by circles), with the retrieved relative errors of the AOT for fine, coarse, and total aerosols decreasing from 26.19 %, 96.70 %, and 27.64 % to 23.52 %, 86.83 %, and 22.40 %, as well as the RMSD, which varied from 0.1062, 0.05660, and 0.1129 to 0.06838, 0.04960, and 0.08738, respectively, in comparison to those derived by the single-pixel scheme (shown by crosses). As in the simulation inversion experimental results shown in Figs. 1–3, the multi-pixel scheme tends to be more effective in the retrieval of fine aerosols than of coarse aerosols from the CAI measurements. However, we still identify a few cases in which the retrieved errors have increased using the multi-pixel scheme, which inspires us to make further studies on the better definition of γ values for each retrieved parameter or the pixel resolution of the subdomain. It should be noted that we neglect the multiple scattering influence between neighbor pixels caused by the adjacent effect in the retrieval, since the effect is generally small for the CAI instrument with moderate spatial resolution. Nevertheless, we have to consider the adjacent effect for the extremely high spatial resolution imagers using a 3-D radiative transfer model.
In this work, we focused on the aerosol retrieval from multi-pixel and multispectral satellite observations from the CAI over the ocean. Unlike most algorithms that conduct the aerosol retrieval pixel by pixel, we derive aerosol properties of multiple pixels simultaneously by considering the smoothness constraint on the spatial variation of aerosols and oceanic substances between the pixels, i.e., the multi-pixel method. We firstly investigated the availability of the multi-pixel scheme in the conditions of open and coarse ocean as well as the land–ocean interface region based on the synthetic measurements of the CAI. Results indicate that the multi-pixel scheme improves the aerosol inversion by increasing the retrieval sensitivity and correcting the retrieval bias induced by measurement errors over multiple pixels, particularly for the fine aerosol over coastal regions. In addition, the spectral remote-sensing reflectance can be also derived with a higher accuracy by constraining the spatial variation of components in the ocean. We then apply the current algorithm using real CAI measurements. The image analysis demonstrates that the irregular retrieved results can be effectively improved using the spatial smoothness constraint. In addition, the multi-pixel scheme shows promising potentiality to retrieve the aerosols over highly turbid waters, especially for those instruments without SWIR measurements. In comparisons with the AERONET observation, retrievals using the multi-pixel scheme tend to be more consistent with the measurements than those derived by the single-pixel method.
Although we compared retrievals using different Lagrange multipliers values in this study, these parameters have similar roles of covariance of the state vector and should be decided by observed variables or information from high-resolution instruments for the spatial smoothness constraint, which needs more analysis. It is demonstrated that the multi-pixel scheme shows a promising technique in the aerosol and hydrosol retrieval based on the multiple source constraints from satellite observation, a priori, and neighbor pixel information in an iteration manner. To apply the scheme used in the global ocean, we have constructed a neural network solver to accelerate the algorithm, and a related study will be explicated in another work. Moreover, investigation regarding the multi-pixel scheme's retrieval performance over the sun-glint area is also a part of our future work. Actually, the retrieved results shown in Fig. 6 are contaminated by the sun glint to some extent, with the glint angles mostly ranging from 25 to 40∘ of the imagery. As in our previous study in which the simultaneous adjustment of the wind speed value helps the aerosol retrieval over the sun-glint region by correcting the surface reflectance (Shi and Nakajima, 2018), the retrieved AOTs of fine and coarse aerosols from the CAI show general consistency with those of MODIS products without sun-glint contamination (Fig. 6). Nevertheless, it seems that the retrieved fine AOTs are still lower than those of the MODIS products overall, which also inspires us to make a further study on the application of multi-pixel scheme in this issue.
The retrieved results from the CAI can be obtained from the corresponding author upon request.
CS wrote the paper and algorithm codes and analyzed the data. TN designed all the research. MH helped with the algorithm development and CAI data usage. The scientific contributions were provided by all coauthors.
The authors declare that they have no conflict of interest.
This work was
supported by funds from MOEJ–JAXA/GOSAT–GOSAT2, JST/CREST/ JPMJCR15K4,
JAXA/EarthCARE–GCOM-C, and MOEJ/ERTDF/S-12. One of author
was supported by the National Natural Science Foundation of China (NSFC;
41590875, 41571130024) and the Key Laboratory of Meteorological Disaster of
the Ministry of Education at the Nanjing University of Information Science
and Technology (KLME1509). The authors express their sincere thanks to the relevant
PIs (Young-Je Park, Hak-Yeol You, Jae-Seol Shim, Joo-Hyung Ryu) for establishing and
maintaining the AERONET sites used in this investigation. We also appreciate
the GOSAT, NCEP, OMI, MODIS, ECOSTRESS science teams for releasing data used
in this analysis. GOSAT/TANSO-CAI data are provided by JAXA/NIES/MOE. NCEP
Reanalysis data are provided by the NOAA. OMI data are provided by NASA.
MODIS ocean color products are provided by the NASA Ocean Biology Processing
Group. Land surface spectral reflectance data are provided from the ECOSTRESS
spectral library of JPL/NASA. We also thank Kei Shiomi for providing
GOSAT/TANSO-CAI calibration material, as well as insightful suggestions, to
improve our work.
Edited by: Jianping
Huang
Reviewed by: two anonymous referees
Boucher, O., Randall, D., Artaxo, P., Bretherton, C., Feingold, G., Forster, P., Kerminen, V.-M., Kondo, Y., Liao, H., Lohmann, U., Rasch, P., S.K, S., Sherwood, S., B., S., and Zhang, X. Y.: Clouds and aerosols, in: Climate Change 2013: The Physical Science Basis. Contribution of Working Group I to the Fifth Assessment Report of the Intergovernmental Panel on Climate Change, Cambridge University Press, Cambridge, UK and New York, NY, USA, 571–657, 2013.
Choi, M., Kim, J., Lee, J., Kim, M., Park, Y.-J., Holben, B., Eck, T. F., Li, Z., and Song, C. H.: GOCI Yonsei aerosol retrieval version 2 products: an improved algorithm and error analysis with uncertainty estimation from 5-year validation over East Asia, Atmos. Meas. Tech., 11, 385–408, https://doi.org/10.5194/amt-11-385-2018, 2018.
Doerffer, R. and Fischer, J.: Concentrations of chlorophyll, suspended matter, and gelbstoff in case II waters derived from satellite coastal zone color scanner data with inverse modeling methods, J. Geophys. Res.-Oceans, 99, 7457–7466, 1994.
Dubovik, O. and King, M. D.: A flexible inversion algorithm for retrieval of aerosol optical properties from Sun and sky radiance measurements, J. Geophys. Res.-Atmos., 105, 20673–20696, 2000.
Dubovik, O., Smirnov, A., Holben, B., King, M., Kaufman, Y., Eck, T., and Slutsker, I.: Accuracy assessments of aerosol optical properties retrieved from Aerosol Robotic Network (AERONET) Sun and sky radiance measurements, J. Geophys. Res.-Atmos., 105, 9791–9806, 2000.
Dubovik, O., Holben, B., Lapyonok, T., Sinyuk, A., Mishchenko, M., Yang, P., and Slutsker, I.: Non-spherical aerosol retrieval method employing light scattering by spheroids, Geophys. Res. Lett., 29, 54-1–54-4, 2002.
Dubovik, O., Herman, M., Holdak, A., Lapyonok, T., Tanré, D., Deuzé, J. L., Ducos, F., Sinyuk, A., and Lopatin, A.: Statistically optimized inversion algorithm for enhanced retrieval of aerosol properties from spectral multi-angle polarimetric satellite observations, Atmos. Meas. Tech., 4, 975–1018, https://doi.org/10.5194/amt-4-975-2011, 2011.
Emde, C., Barlakas, V., Cornet, C., Evans, F., Korkin, S., Ota, Y., Labonnote, L. C., Lyapustin, A., Macke, A., and Mayer, B.: IPRT polarized radiative transfer model intercomparison project–Phase A, J. Quant. Spectrosc. Ra., 164, 8–36, 2015.
Fan, Y., Li, W., Gatebe, C. K., Jamet, C., Zibordi, G., Schroeder, T., and Stamnes, K.: Atmospheric correction over coastal waters using multilayer neural networks, Remote Sens. Environ., 199, 218–240, 2017.
Gao, M., Zhai, P.-W., Franz, B., Hu, Y., Knobelspiesse, K., Werdell, P. J., Ibrahim, A., Xu, F., and Cairns, B.: Retrieval of aerosol properties and water-leaving reflectance from multi-angular polarimetric measurements over coastal waters, Opt. Express, 26, 8968–8989, 2018.
Goloub, P., Tanre, D., Deuze, J.-L., Herman, M., Marchand, A., and Bréon, F.-M.: Validation of the first algorithm applied for deriving the aerosol properties over the ocean using the POLDER/ADEOS measurements, IEEE T. Geosci. Remote, 37, 1586–1596, 1999.
Gordon, H. R. and Wang, M.: Retrieval of water-leaving radiance and aerosol optical thickness over the oceans with SeaWiFS: a preliminary algorithm, Appl. Optics, 33, 443–452, 1994.
Hasekamp, O. P., Litvinov, P., and Butz, A.: Aerosol properties over the ocean from PARASOL multiangle photopolarimetric measurements, J. Geophys. Res., 116, D14204, https://doi.org/10.1029/2010JD015469, 2011.
Hashimoto, M. and Nakajima, T.: Development of a remote sensing algorithm to retrieve atmospheric aerosol properties using multi-wavelength and multi-pixel information, J. Geophys. Res.-Atmos., 122, 6347–6378, 2017.
He, X. Q., Bai, Y., Pan, D. L., Huang, N. L., Dong, X., Chen, J. S., Chen, C. T. A., and Cui, Q. F.: Using geostationary satellite ocean color data to map the diurnal dynamics of suspended particulate matter in coastal waters, Remote Sens. Environ., 133, 225–239, 2013.
Higurashi, A. and Nakajima, T.: Detection of aerosol types over the East China Sea near Japan from four-channel satellite data, Geophys. Res. Lett., 29, 1836, https://doi.org/10.1029/2002GL015357, 2002.
Holben, B., Eck, T., Slutsker, I., Tanre, D., Buis, J., Setzer, A., Vermote, E., Reagan, J., Kaufman, Y., and Nakajima, T.: AERONET – a federated instrument network and data archive for aerosol characterization, Remote Sens. Environ., 66, 1–16, 1998.
Hu, C., Lee, Z., and Franz, B.: Chlorophyll a algorithms for oligotrophic oceans: A novel approach based on three-band reflectance difference, J. Geophys. Res.-Oceans, 117, C01011, https://doi.org/10.1029/2011JC007395, 2012.
Huang, J., Wang, T., Wang, W., Li, Z., and Yan, H.: Climate effects of dust aerosols over East Asian arid and semiarid regions, J. Geophys. Res.-Atmos., 119, 11398–11416, 2014.
Kaufman, Y. J.: Satellite sensing of aerosol absorption, J. Geophys. Res.-Atmos., 92, 4307–4317, 1987.
Kim, J., Lee, J., Lee, H. C., Higurashi, A., Takemura, T., and Song, C. H.: Consistency of the aerosol type classification from satellite remote sensing during the Atmospheric Brown Cloud–East Asia Regional Experiment campaign, J. Geophys. Res.-Atmos., 112, D22S33, https://doi.org/10.1029/2006JD008201, 2007.
King, M. D., Byrne, D. M., Herman, B. M., and Reagan, J. A.: Aerosol size distributions obtained by inversions of spectral optical depth measurements, J. Atmos. Sci., 35, 2153–2167, 1978.
Knobelspiesse, K., Cairns, B., Mishchenko, M., Chowdhary, J., Tsigaridis, K., van Diedenhoven, B., Martin, W., Ottaviani, M., and Alexandrov, M.: Analysis of fine-mode aerosol retrieval capabilities by different passive remote sensing instrument designs, Opt. Express, 20, 21457–21484, 2012.
Lee, J., Kim, J., Yang, P., and Hsu, N. C.: Improvement of aerosol optical depth retrieval from MODIS spectral reflectance over the global ocean using new aerosol models archived from AERONET inversion data and tri-axial ellipsoidal dust database, Atmos. Chem. Phys., 12, 7087–7102, https://doi.org/10.5194/acp-12-7087-2012, 2012.
Lee, Z., Carder, K. L., and Arnone, R. A.: Deriving inherent optical properties from water color: a multiband quasi-analytical algorithm for optically deep waters, Appl. Optics, 41, 5755–5772, 2002.
Lee, Z., Wei, J., Voss, K., Lewis, M., Bricaud, A., and Huot, Y.: Hyperspectral absorption coefficient of “pure” seawater in the range of 350–550 nm inverted from remote sensing reflectance, Appl. Optics, 54, 546–558, 2015.
Li, Z., Zhao, X., Kahn, R., Mishchenko, M., Remer, L., Lee, K.-H., Wang, M., Laszlo, I., Nakajima, T., and Maring, H.: Uncertainties in satellite remote sensing of aerosols and impact on monitoring its long-term trend: a review and perspective, Ann. Geophys., 27, 2755–2770, https://doi.org/10.5194/angeo-27-2755-2009, 2009.
Martonchik, J. V., Diner, D. J., Kahn, R. A., Ackerman, T. P., Verstraete, M. M., Pinty, B., and Gordon, H. R.: Techniques for the retrieval of aerosol properties over land and ocean using multiangle imaging, IEEE T. Geosci. Remote, 36, 1212–1227, 1998.
Mishchenko, M. I., Geogdzhayev, I. V., Cairns, B., Rossow, W. B., and Lacis, A. A.: Aerosol retrievals over the ocean by use of channels 1 and 2 AVHRR data: sensitivity analysis and preliminary results, Appl. Optics, 38, 7325–7341, 1999.
Mobley, C. D., Gentili, B., Gordon, H. R., Jin, Z., Kattawar, G. W., Morel, A., Reinersman, P., Stamnes, K., and Stavn, R. H.: Comparison of numerical models for computing underwater light fields, Appl. Optics, 32, 7484–7504, 1993.
Nakajima, T. and Higurashi, A.: AVHRR remote sensing of aerosol optical properties in the Persian Gulf region, summer 1991, J. Geophys. Res.-Atmos., 102, 16935–16946, 1997.
Nakajima, T. and Tanaka, M.: Effect of wind-generated waves on the transfer of solar radiation in the atmosphere–ocean system, J. Quant. Spectrosc. Ra., 29, 521–537, 1983.
Nakajima, T. and Tanaka, M.: Matrix formulations for the transfer of solar radiation in a plane-parallel scattering atmosphere, J. Quant. Spectrosc. Ra., 35, 13–21, 1986.
Nakajima, T. and Tanaka, M.: Algorithms for radiative intensity calculations in moderately thick atmospheres using a truncation approximation, J. Quant. Spectrosc. Ra., 40, 51–69, 1988.
Nakajima, T., Tonna, G., Rao, R., Boi, P., Kaufman, Y., and Holben, B.: Use of sky brightness measurements from ground for remote sensing of particulate polydispersions, Appl. Optics, 35, 2672–2686, 1996.
Ota, Y., Higurashi, A., Nakajima, T., and Yokota, T.: Matrix formulations of radiative transfer including the polarization effect in a coupled atmosphere–ocean system, J. Quant. Spectrosc. Ra., 111, 878–894, 2010.
Phillips, D. L.: A technique for the numerical solution of certain integral equations of the first kind, J. ACM, 9, 84–97, 1962.
Remer, L. A., Kaufman, Y., Tanré, D., Mattoo, S., Chu, D., Mar- tins, J. V., Li, R. R., Ichoku, C., Levy, R., and Kleidman, R.: The MODIS aerosol algorithm, products, and validation, J. Atmos. Sci., 62, 947–973, 2005.
Rodgers, C. D.: Inverse Methods for Atmospheric Sounding: Theory and Practice, World Scientific, Singapore, 2000.
Sayer, A. M., Thomas, G. E., and Grainger, R. G.: A sea surface reflectance model for (A)ATSR, and application to aerosol retrievals, Atmos. Meas. Tech., 3, 813–838, https://doi.org/10.5194/amt-3-813-2010, 2010.
Sekiguchi, M. and Nakajima, T.: A k-distribution-based radiation code and its computational optimization for an atmospheric general circulation model, J. Quant. Spectrosc. Ra., 109, 2779–2793, 2008.
Shettle, E. P. and Fenn, R. W.: Models for the Aerosols of the Lower Atmosphere and the Effects of Humidity Variations on Their Optical Properties, Air Force Geophysics Laboratory, Hanscom Air Force Base, MA, USA, 1979.
Shi, C. and Nakajima, T.: Simultaneous determination of aerosol optical thickness and water-leaving radiance from multispectral measurements in coastal waters, Atmos. Chem. Phys., 18, 3865–3884, https://doi.org/10.5194/acp-18-3865-2018, 2018.
Shi, C., Wang, P., Nakajima, T., Ota, Y., Tan, S., and Shi, G.: Effects of ocean particles on the upwelling radiance and polarized radiance in the atmospheric–ocean system, Adv. Atmos. Sci., 32, 1–11, 2015.
Shi, C., Nakajima, T., and Hashimoto, M.: Simultaneous retrieval of aerosol optical thickness and chlorophyll concentration from multi-wavelength measurement over East China Sea, J. Geophys. Res.-Atmos., 121, 14084–14101, 2016.
Shiomi, K., Kawakami S., and Kina T., Operation results of initial calibration and validation of “IUBKI”, Aeronaut. Space Sci. Jpn., 58, 158–163, 2010 (in Japanese).
Stamnes, K., Li, W., Yan, B., Eide, H., Barnard, A., Pegau, W. S., and Stamnes, J. J.: Accurate and self-consistent ocean color algorithm: simultaneous retrieval of aerosol optical properties and chlorophyll concentrations, Appl. Optics, 42, 939–951, 2003.
Stowe, L., Carey, R., and Pellegrino, P.: Monitoring the Mt. Pinatubo aerosol layer with NOAA/11 AVHRR data, Geophys. Res. Lett., 19, 159–162, 1992.
Tanré, D., Kaufman, Y., Herman, M., and Mattoo, S.: Remote sensing of aerosol properties over oceans using the MODIS/EOS spectral radiances, J. Geophys. Res.-Atmos., 102, 16971–16988, 1997.
Torres, O., Bhartia, P., Herman, J., Ahmad, Z., and Gleason, J.: Derivation of aerosol properties from satellite measurements of backscattered ultraviolet radiation: Theoretical basis, J. Geophys. Res.-Atmos., 103, 17099–17110, 1998.
Twomey, S.: On Numerical Solution Of Fredholm Integral Equations Of First Kind By Inversion Of Linear System Produced By Quadrature, J. ACM, 10, 97–101, 1963.
Wang, M. and Shi, W.: The NIR-SWIR combined atmospheric correction approach for MODIS ocean color data processing, Opt. Express, 15, 15722–15733, 2007.
Wang, Y., Wang, J., Levy, R. C., Xu, X., and Reid, J. S.: MODIS Retrieval of Aerosol Optical Depth over Turbid Coastal Water, Remote Sens., 9, 595, https://doi.org/10.3390/rs9060595, 2017.
Xu, F., Dubovik, O., Zhai, P.-W., Diner, D. J., Kalashnikova, O. V., Seidel, F. C., Litvinov, P., Bovchaliuk, A., Garay, M. J., van Harten, G., and Davis, A. B.: Joint retrieval of aerosol and water-leaving radiance from multispectral, multiangular and polarimetric measurements over ocean, Atmos. Meas. Tech., 9, 2877–2907, https://doi.org/10.5194/amt-9-2877-2016, 2016.
Xu, F., Diner, D. J., Dubovik, O., and Yoav, S.: A Correlated Multi-Pixel Inversion Approach for Aerosol Remote Sensing, Remote Sens., in review, 2019.
Xu, X., Wang, J., Wang, Y., Zeng, J., Torres, O., Yang, Y., Marshak, A., Reid, J., and Miller, S.: Passive remote sensing of altitude and optical depth of dust plumes using the oxygen A and B bands: First results from EPIC/DSCOVR at Lagrange-1 point, Geophys. Res. Lett., 44, 7544–7554, 2017.
Yu, Q.-R., Zhang, F., Li, J., and Zhang, J.: Analysis of sea-salt aerosol size distributions in radiative transfer, J. Aerosol Sci., 129, 71–86, https://doi.org/10.1016/j.jaerosci.2018.11.014, 2019.