the Creative Commons Attribution 4.0 License.
the Creative Commons Attribution 4.0 License.
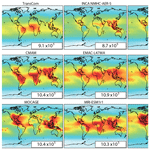
Inter-model comparison of global hydroxyl radical (OH) distributions and their impact on atmospheric methane over the 2000–2016 period
Yuanhong Zhao
Marielle Saunois
Philippe Bousquet
Antoine Berchet
Michaela I. Hegglin
Josep G. Canadell
Robert B. Jackson
Didier A. Hauglustaine
Sophie Szopa
Ann R. Stavert
Nathan Luke Abraham
Alex T. Archibald
Slimane Bekki
Makoto Deushi
Patrick Jöckel
Béatrice Josse
Douglas Kinnison
Ole Kirner
Virginie Marécal
Fiona M. O'Connor
David A. Plummer
Laura E. Revell
Eugene Rozanov
Andrea Stenke
Sarah Strode
Simone Tilmes
Edward J. Dlugokencky
The modeling study presented here aims to estimate how uncertainties in global hydroxyl radical (OH) distributions, variability, and trends may contribute to resolving discrepancies between simulated and observed methane (CH4) changes since 2000. A multi-model ensemble of 14 OH fields was analyzed and aggregated into 64 scenarios to force the offline atmospheric chemistry transport model LMDz (Laboratoire de Meteorologie Dynamique) with a standard CH4 emission scenario over the period 2000–2016. The multi-model simulated global volume-weighted tropospheric mean OH concentration ([OH]) averaged over 2000–2010 ranges between 8.7×105 and 12.8×105 molec cm−3. The inter-model differences in tropospheric OH burden and vertical distributions are mainly determined by the differences in the nitrogen oxide (NO) distributions, while the spatial discrepancies between OH fields are mostly due to differences in natural emissions and volatile organic compound (VOC) chemistry. From 2000 to 2010, most simulated OH fields show an increase of 0.1–0.3×105 molec cm−3 in the tropospheric mean [OH], with year-to-year variations much smaller than during the historical period 1960–2000. Once ingested into the LMDz model, these OH changes translated into a 5 to 15 ppbv reduction in the CH4 mixing ratio in 2010, which represents 7 %–20 % of the model-simulated CH4 increase due to surface emissions. Between 2010 and 2016, the ensemble of simulations showed that OH changes could lead to a CH4 mixing ratio uncertainty of ppbv. Over the full 2000–2016 time period, using a common state-of-the-art but nonoptimized emission scenario, the impact of [OH] changes tested here can explain up to 54 % of the gap between model simulations and observations. This result emphasizes the importance of better representing OH abundance and variations in CH4 forward simulations and emission optimizations performed by atmospheric inversions.
- Article
(9798 KB) - Full-text XML
- Companion paper 2
- Companion paper 3
-
Supplement
(2431 KB) - BibTeX
- EndNote
The hydroxyl radical (OH) is the main oxidizing agent in the troposphere (Levy, 1971). OH is produced by the reaction of water vapor with excited oxygen atoms (O(1D)), which are produced by ozone (O3) photolysis (λ<340 nm). In the troposphere, OH is rapidly removed by reactions with carbon monoxide (CO), methane (CH4), and non-methane volatile organic compounds (NMVOCs) to generate the hydroperoxyl radical (HO2) or organic peroxy radicals (RO2), resulting in a short lifetime of a few seconds (Logan et al., 1981; Lelieveld et al., 2004). HO2 and RO2 can further react with nitrogen oxide (NO) to regenerate OH (Crutzen, 1973; Zimmerman et al., 1978). At high latitudes, such a secondary production plays an important role because OH primary production is limited by the supply of O(1D) and water vapor (Spivakovsky et al., 2000). The abundance of OH reflects the combined effects of atmospheric composition (tropospheric O3, and NO, CO, CH4, and NMVOCs) and meteorological factors such as humidity, UV radiation, and temperature.
Due to its short lifetime, global [OH] is difficult to estimate from direct measurements. Current understanding of global [OH] has been obtained either from inversion of 1-1-1trichloroethane (methyl chloroform, MCF) (Prinn et al., 2005; Bousquet et al., 2005; Montzka et al., 2011; Rigby et al., 2017; Turner et al., 2017) or using atmospheric chemistry models (Naik et al., 2013; Voulgarakis et al., 2013, Lelieveld et al., 2016). The former approach relies on the fact that OH is the main sink of MCF and on the hypotheses that emissions and concentrations of MCF are well known and well measured, respectively. The latter approach relies on chemistry transport modeling with chemistry schemes of varying complexity. The global mass-weighted tropospheric mean [OH] in the 2000s calculated by atmospheric chemistry models was found to be about 11.5×105 molec cm−3, with an inter-model dispersion of ±15 % (Naik et al., 2013; Voulgarakis et al., 2013). Atmospheric chemistry models usually calculate higher [OH] over the Northern Hemisphere than the Southern Hemisphere (N ∕ S ratio > 1) (Naik et al., 2013), whereas MCF and 14CO observations indicate an N ∕ S ratio slightly smaller than 1 (Brenninkmeijer et al., 1992; Bousquet et al., 2005; Patra et al., 2014).
OH determines the lifetime of most pollutants and non-CO2 greenhouse gases including CH4, the second most important anthropogenic greenhouse gas after carbon dioxide (CO2) (Ciais et al., 2013). About 90 % of tropospheric CH4 is removed by reacting with OH (Ehhalt et al., 1974; Kirschke et al., 2013; Saunois et al., 2016). The tropospheric CH4 chemical lifetime against OH oxidation (global annual mean atmospheric CH4 burden divided by annual CH4 tropospheric loss by OH) calculated by the models that participated in the Atmospheric Chemistry and Climate Model Intercomparison Project (ACCMIP) is 9.3±1.6 years, and the CH4 total lifetime including all sink processes is 8.3±0.8 years (Naik et al., 2013; Voulgarakis et al., 2013), smaller than the of 9.1±0.9-year lifetime constrained by observations (Prather et al., 2012).
The tropospheric CH4 burden has more than doubled compared to the preindustrial era due to anthropogenic activities and climate change, resulting in about 0.62 W m−2 of additional radiative forcing (Etminan et al., 2016). The global mean CH4 growth rate decreased to near zero in the early 2000s but resumed increasing at ∼5 ppbv yr−1 since 2006 and reached more than 10 ppbv yr−1 in 2014 and in 2018 (Dlugokencky, 2019). The growth rate of CH4 is determined by the imbalance of its sources, primarily from anthropogenic activities (agriculture, waste, fossil fuel production and usage, and biomass burning) but also from natural emissions (mainly wetland and other inland waters) and sinks (OH oxidation, other chemical reactions with chlorine and oxygen radicals, and soil uptake). The precise reasons for the stagnation and renewed CH4 growth still remain unclear (e.g., Rigby et al., 2017; Saunois et al., 2017; Nisbet et al., 2019; Turner et al., 2019).
Several studies have linked such CH4 variations to interannual variations and trends of OH. Based on MCF inversions, McNorton et al. (2016) concluded that an increase in [OH] significantly contributed to the stable atmospheric CH4 before 2007; Rigby et al. (2008) found that a decrease of 4±14 % in [OH] could partly explain the CH4 growth between 2006 and 2007. Bousquet et al. (2011) found a smaller decrease in [OH] (<1 % over the 2 years) and attributed the increase in CH4 mostly to enhanced emissions over tropical regions; Montzka et al. (2011) also calculated a small interannual variation of 2.3±1.5 % in [OH] from 1998 to 2007. More recently, based on multi-species box model inversions, Rigby et al. (2017) and Turner et al. (2017) inferred a decrease of 8±11 % and 7 % in [OH] during 2004–2014 and 2003–2016, respectively. Both of these studies suggested that such a decrease in [OH] is equivalent to an increase of more than 20 Tg yr−1 in CH4 emissions and could therefore significantly contribute to explaining the post-2007 CH4 atmospheric growth, although a solution with constant OH cannot be discarded. Meanwhile, not only can the OH trend calculated by atmospheric chemistry models not reach consensus, but it can also be different from the OH trend inferred by top-down approaches from observations. Indeed, Dalsøren et al. (2016) simulated an ∼8 % increase in OH from 1970 to 2012, while other models mostly calculated only a small increase in [OH] (decrease in CH4 lifetime) or no trend in [OH] from the 1980s to 2000s (e.g., Voulgarakis et al., 2013; Nicely et al., 2018). Top-down observation-constrained approaches (e.g., Rigby et al., 2017) tend to find flat to decreasing OH trends over this period but with larger year-to-year variations than models. The discrepancy between individual process-based models and MCF proxy approaches, as well as the uncertainties, limit our ability to be conclusive on the role of [OH] changes in explaining the CH4 changes over the past decades.
To better understand OH distributions, trends, and influences on CH4 since 2000, we have performed an inter-model comparison of 14 OH fields, including 11 derived from chemistry transport and chemistry–climate models that took part in phase 1 of the Chemistry–Climate Model Initiative (CCMI) (Hegglin and Lamarque, 2015; Morgenstern et al., 2017), 2 from different configurations of the LSCE atmospheric chemistry transport model LMDz–INCA (Hauglustaine et al., 2004; Szopa et al., 2013), and 1 from the TransCom 2011 intercomparison exercise (Patra et al., 2011). We then conducted an ensemble of CH4 simulations with different OH fields using the LMDz chemistry transport model to estimate a range for the contribution of changes in [OH] to the atmospheric CH4 mixing ratio changes since 2000 and to relate this contribution to the spatiotemporal characteristics of the different OH fields. Year-to-year integrations of CCMI and INCA models driven by time-varying emissions and meteorology facilitate the investigation of interannual variability in OH, which was not possible using the ACCMIP time-slice simulations. In the following, our analyses first provides a brief description of the OH fields used in this study and the LMDz offline model (Sect. 2). Section 3 compares the OH fields, analyses the factors contributing to inter-model differences, and presents their interannual variability. Section 4 presents and discusses the impact of the different OH fields on the global CH4 burden and growth rates simulated by LMDz. Section 5 summarizes the results and provides a conclusion.
2.1 OH fields
The CCMI project aims to conduct a detailed evaluation of atmospheric chemistry models in order to assess uncertainties in the model projections of various climate-related topics such as tropospheric composition (Hegglin and Lamarque, 2015; Morgenstern et al., 2017). The CCMI OH fields used in our study are obtained from 10 different models and three CCMI reference experiments: REF-C1 (covering the time period 1960–2010), REF-C2 (covering 1960–2100), and REF-C1SD (1980–2010). The REF-C1 experiment is driven by state-of-the-art historical forcings as well as sea surface temperatures (SSTs) and sea ice concentrations (SICs) based on observations, while the REF-C2 experiment uses either coupled ocean and sea ice modules or prescribes SSTs and SICs obtained from another climate model. Since the REF-C1 experiment is supposed to be more realistic regarding sea surface conditions, our analysis focused on OH fields from the REF-C1 experiment before 2010 and only tested the influences of OH on CH4 simulations after 2010 by applying the interannual variability from the REF-C2 experiment. The models of the REF-C1SD experiment are nudged towards reanalysis datasets. The REF-C1SD experiment is not analyzed in the main text since it has been conducted by only some of the models and covers a shorter time period. A comparison of the spatial and vertical distributions of OH fields from the REF-C1 experiment with those from REF-C1SD reveals only small latitudinal differences (<10 %; see Sect. S1 in the Supplement). Detailed descriptions of CCMI simulations can be found in Morgenstern et al. (2017).
Table 1List of CCMI models included in this study with model versions and references∗.
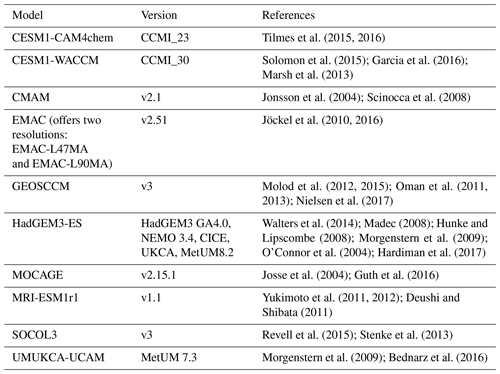
∗ The table refers to Table 2 in Morgenstern et al. (2017).
In this study, we used only the CCMI models that include detailed tropospheric ozone chemistry as listed in Table 1. Note that EMAC offers fields at two different model resolutions. The level of detail in chemical mechanisms, in particular with respect to the included NMVOCs, varies among the models. For example, CMAM does not include any NMVOC species but added 250 Tg of CO emissions to account for CO production from isoprene oxidation. UMUKCA-UCAM only includes HCHO (formaldehyde) and SOCOL3 only includes HCHO and C5H8 (isoprene). Other models include multiple primary NMVOC species and more complex VOC chemistry.
The anthropogenic emissions recommended for the two CCMI reference simulations are from the MACCity inventory (Granier et al., 2011) for 1960–2000. After 2000, the REF-C1 experiment continued to use the MACCity inventory (which follow the RCP8.5 inventory after 2000), while the REF-C2 used the RCP6.0 inventory (Masui et al., 2011). The CMAM did not follow this procedure and used the ACCMIP historical database of emissions (Lamarque et al., 2010) until 2000, followed by RCP8.5 emissions (Riahi et al., 2011). Biomass burning emissions used in REF-C1 are from the RETRO inventory (Schultz et al., 2008) before 1996 and the GFEDv3 inventory (van der Werf et al., 2010) for 1997–2010 with interannual variability. CCMI model simulations also include natural emissions from lightning, soil, and biogenic sources. Lightning NOx emissions are calculated based on meteorological data such as cloud-top height (Price and Rind, 1994; Grewe et al., 2001) and updraft mass flux (Allen and Pickering, 2002). Soil NOx emissions are calculated interactively in EMAC and GEOSCCM using the scheme described by Yienger and Levy (1995) but are prescribed in other models. Biogenic NMVOC emissions in CESM and GEOSCCM are calculated based on the distribution of plant functional types and meteorology conditions with MEGAN, whereas the other models apply prescribed biogenic NMVOC emissions.
The CCMI models do not represent CH4 emissions explicitly but prescribe CH4 surface mixing ratios to vary in time according to the RCP6.0 scenario (global mean of ∼1750 ppbv averaged over 2000–2010) with different spatial distributions: the GEOSCCM, CESM, and EMAC models consider the full latitudinal gradient and prescribe CH4 surface mixing ratios about 50 ppbv higher over the Northern Hemisphere than over the Southern Hemisphere, while CMAM, MRI-ESM1r1, and SOCOL3 use globally uniform values. Photolysis rates are calculated either following online schemes such as FAST-JX (Neu et al., 2007; Telford et al., 2013) by GEOSCCM, HadGEM3-EA, UMUKCA-UCAM, JVAL (Sander et al., 2014) by EMAC or are based on lookup tables with online cloud corrections by the rest of the models used in this study. Kinetics and photolysis data are mainly from Sander et al. (2011) with a few exceptions. More information on model characteristics can be found in Morgenstern et al. (2017) and the references listed in Table 1.
Additionally to CCMI OH fields, we also included two OH fields simulated by the Interaction with Chemistry and Aerosols (INCA) coupled to the general circulation model (GCM) of the Laboratoire de Meteorologie Dynamique (LMD), LMDz (Sadourny and Laval, 1984; Hourdin and Armengaud, 1999; Hourdin et al., 2006; Hauglustaine et al., 2004). The two INCA simulations are driven by different versions of the LMDz GCM (INCA NMHC-AER-S covering time period 2000–2010 (Terrenoire et al., 2019) and INCA NMHC covering time period 2000–2009; Szopa et al., 2013), which provide different water vapor fields and include different chemistry and emissions. The INCA NMHC-AER-S used the latest version of the INCA model including both gas-phase (NMHC) and aerosol (AER) chemistry in the troposphere and the stratosphere (S) (Terrenoire et al., 2019), while INCA NMHC used a former version that only includes tropospheric gas-phase chemistry (Szopa et al., 2013). Anthropogenic emissions from the Short-Lived Pollutants (ECLIPSE) inventory (Stohl et al., 2015) for 2005 and the RCP8.5 emission inventory (Riahi et al., 2011) for 2010 are applied to every year of INCA NMHC-AER-S and INCA NMHC simulations, respectively.
Finally, we included in this study the OH field used in TransCom simulations, which results from a combination of the semi-empirical tropospheric three-dimensional OH field from Spivakovsky et al. (2000) and a two-dimensional simulated stratospheric OH for year 2000. The tropospheric OH was calculated using prescribed chemical species (O3, nitrogen oxides, and CO) and meteorological fields (temperature, humidity, and cloud optical depth) to fit the observations. The original tropospheric [OH] has been reduced by 8 % to match CH3CCl3 observations (Patra et al., 2011). The TransCom OH field is only climatological (1 year of monthly fields).
In total, we compared 14 OH fields: 11 from CCMI, 2 from the online LMDz–INCA model, and 1 from TransCom. We analyzed spatial distributions and annual variations of OH fields by calculating volume-weighted tropospheric mean [OH] with tropopause pressure using the WMO tropopause definition on 3-D temperature for each model (World Meteorological Organization, 1957). Since employing different weightings can result in large differences in mean [OH] (Lawrence et al., 2001), we also calculated dry air mass-weighted tropospheric mean [OH] to better compare with previous studies.
2.1.1 LMDz model simulations
2.1.2 Model description and setup
We have run the offline version LMDz5B of the LMDz model (Locatelli et al., 2015) at a horizontal resolution of with 39 vertical layers up to 3 hPa to assess the impact of OH on tropospheric CH4. All monthly mean OH fields have been interpolated to the LMDz model grid. The transport of atmospheric tracers is driven by prescribed air mass fluxes provided by the general circulation model LMDz with horizontal wind fields nudged to ERA-Interim reanalysis meteorology data produced by the European Centre for Medium-Range Weather Forecasts (Dee et al., 2011). The vertical transport is parameterized according to updates of the Emanuel (1991) scheme for convection and of the Louis (1979) scheme for boundary layer mixing (Hourdin et al., 2016; Locatelli et al., 2015). The chemistry module applied here is the simplified chemistry module SACS (Pison et al., 2009). Chemical sinks of CH4 are calculated using prescribed three-dimensional OH and O(1D) fields, and variation in CH4 cannot feed back on OH. No chlorine-related sink is simulated in this version of the model. To assess the influences of OH only, all LMDz simulations used the same O(1D) fields generated by INCA model simulations. The reaction rate coefficient (k) for CH4 destruction by OH in the model is computed depending on temperature following Sander et al. (2011):
The LMDz model has been applied in various studies focusing on long-lived gases such as CH4, CO2, and MCF (Bousquet et al., 2005; Pison et al., 2009; Lin et al., 2018). It has also been used in model intercomparison projects such as the TransCom experiment (Patra et al., 2011) with the simplified chemistry module SACS (Pison et al., 2009) and CCMI (Morgenstern et al., 2017) but only with the stratospheric chemistry model REPROBUS (Jourdain et al., 2008).
The CH4 emissions input to LMDz simulations are provided by Global Carbon Project (GCP) methane and include anthropogenic and biofuel emissions from EDGARv4.3.2 (Janssens-Maenhout et al., 2017), the mean wetland emissions from Poulter et al. (2017), fire emissions from the Global Fire Emissions Database version 4.1 (GFED4) (Randerson et al., 2018), termite emissions as described by Saunois et al. (2016), geological emissions based on the spatial distribution of Etiope (2015), ocean emissions from Lambert and Schmidt (1993), and soil uptake from Ridgwell et al. (1999). EDGARv4.3.2 data, available until 2012, were extrapolated from 2013 to 2016 using economical statistics according to the methodology described by Saunois et al. (2016). Anthropogenic and fire emissions vary from 2000 to 2016, while natural emissions are applied as a climatology.
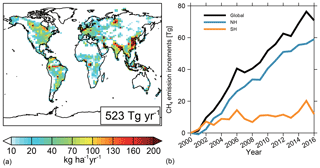
Figure 1Spatial distribution of global CH4 emissions averaged between 2000 and 2016 (a) and a time series of CH4 emissions relative to year 2000 emissions (482 Tg CH4 a−1) (b) for the globe (black line), Northern Hemisphere (NH, blue line), and Southern Hemisphere (SH, orange line).
The spatial distributions and annual variations of the CH4 emissions during the study period are shown in Fig. 1. CH4 emissions range from 10 to 40 kg ha−1 yr1 over most natural ecosystems and can exceed 100 kg ha−1 yr−1 over wetlands in Canada, South America, and Central Africa, as well as over densely populated regions such as South and East Asia. Global net CH4 emissions (soil uptake included) increased by 15 % from 482 Tg yr−1 in 2000 to 552 Tg yr−1 in 2016. Of this 70 Tg yr−1 increase, 60 Tg yr−1 (85 %) is emissions from the Northern Hemisphere, mainly contributed by livestock (18 Tg yr−1, 25 %), oil and gas (16 Tg yr−1, 23 %), coal burning (17 Tg yr−1, 24 %), and waste (13 Tg yr−1, 18 %). The three emission peaks in 2002, 2006, and 2015 are driven by biomass burning. This CH4 emission scenario is state of the art but has not been optimized for the simulated CH4 mixing ratios to fit the observations.
2.1.3 Model simulations
Two sets of experiments (steady-state and transient simulations) have been performed to examine the impacts of the input OH fields on the global CH4 burden as well as the CH4 spatial distribution and annual variation. These tests excluded the OH fields from CESM1-CAM4chem and EMAC-L47MA, since they are similar to those of CESM1-WACCM and EMAC-L90MA, respectively. We also discarded the OH fields from HadGEM3-ES and UMUKCA-UCAM because output from these two models has been supplied on vertical pressure levels that are too coarse. Finally, 10 different OH fields (seven from CCMI, two from LMDz–INCA, and one from TransCom) were used in the two sets of simulations.
Initially, for each OH field described in Sect. 3, we ran 30 consecutive years of LMDz simulations (with the same recycled emissions, sinks, and meteorology of the year 2000) to allow the simulation to reach a steady state (CH4 has an approximate lifetime of 9 years in the atmosphere). This step aims to examine the impact of the magnitude and distribution of OH on the global CH4 burden.
Secondly, we performed transient simulations starting from the year 2000, which are forced by time-varying OH fields as well as time-varying emissions and meteorology fields. In order to compare the impacts of the different OH fields on realistic CH4 mixing ratios, for each simulation (except the one using the OH fields from INCA NMHC) the OH field has been scaled to get the same LMDz simulated CH4 loss as the one calculated by INCA NMHC in 2000, as INCA is the OH field consistently obtained using the LMDz transport. Then a series of LMDz model simulations is conducted to investigate the impact of the various OH fields on CH4 growth rates between 2000 and 2016 as summarized in Table 2.
The standard simulations (Run_standard in Table 2) using the 10 different OH fields (7 are from CCMI REF-C1) included annual variations and were performed from 2000 to 2010. Since REF-C1 experiments are only available up to 2010, the influence of OH on CH4 mixing ratios after 2010 have been tested based on alternative scenarios. First, for CCMI simulations, we tested a scenario that takes into account the annual variability from the REF-C2 experiments (Run_REF-C2 in Table 2). Previous ACCMIP model experiments showed slightly decreasing or increasing [OH] from 2000 to 2030 according to the largest or lowest radiative forcing pathways (RCP8.5 or RCP2.6), respectively (Voulgarakis et al., 2013). Top-down approaches suggested that global OH decreased by 0.5 %–1 % annually from 2003 to 2016 (Rigby et al., 2017; Turner et al., 2017). In order to assess the recent change in [OH], we tested two additional scenarios between 2010 and 2016: one with an [OH] increase of +0.1 % yr−1 (Run_OH_inc) according to the slightly changing OH calculated by ACCMIP models and one with an [OH] decrease of −1 % yr−1 (Run_OH_dec) according to obviously decreasing OH calculated by top-down approaches constrained by observations. To assess influences from OH alone, we also conducted additional simulations of the period 2000 to 2016 with annually repeated prescribed [OH] equal to the year 2000 (Run_fix_OH) for each OH field. The differences between these constant OH simulations and the corresponding time-varying OH simulations indicate the impact of OH interannual variations and trends on atmospheric CH4 changes. In addition, we conducted two simulations during 2000–2010 driven by emission inventories fixed to the year 2000 to test the influences of the emission bias on our results. The two simulations use OH fields simulated by CESM-WACCM, one with interannual variations of OH (Run_fix_emis) and the other one with the OH field fixed to 2000 (Run_fix_emis_OH).
3.1 Spatial distributions of tropospheric OH
Figure 2 shows the spatial distributions of volume-weighted tropospheric mean [OH] averaged from 2000 to 2010. Based on the 14 OH fields we have assembled, the global mean volume-weighted tropospheric [OH] varies from 8.7×105 to 12.8×105 molec cm−3. SOCOL, for which an overestimation of [OH] has been reported by Staehelin et al. (2017), simulated the highest [OH]. To better compare with previous studies, we also calculated dry air mass-weighted tropospheric mean [OH] in Table 4, which varies from 9.4×105 to 14.4×105 molec cm−3 with a multi-model mean value of molec cm−3. The tropospheric chemical CH4 lifetime of the models that provided CH4 chemical loss data is 8.7±1.1 years. The multi-model mean, the (large) range of [OH], and the tropospheric CH4 chemical lifetime are consistent with previous multi-model results given by the ACCMIP project (Naik et al., 2013; Voulgarakis et al., 2013), as well as with inversions based on MCF observations (Bousquet et al., 2005; Rigby et al., 2017). The model spread remains as large as ∼50 % of the minimum value, as noted in previous studies (e.g., Naik et al., 2013).
Table 3Inter-hemispheric ratios (N ∕ S) of hemispheric mean OH and volume-weighted tropospheric mean [OH] for four latitude bands (105 molec cm−3) averaged over the years 2000 to 2010. Multi-model means and standard deviations (mean ± SD) are also shown.
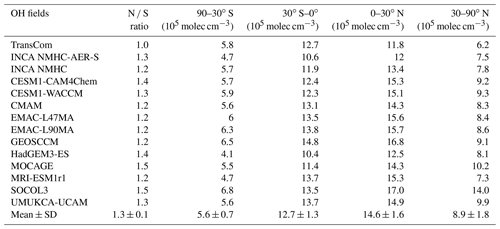
Table 3 summarizes the inter-hemispheric ratios of tropospheric OH and mean values over four latitudinal bands. The inter-hemispheric ratios (N ∕ S ratios) of CCMI and INCA OH fields are within the range of 1.2–1.5, similar to those from the ACCMIP project (Naik et al., 2013). In contrast, the TransCom OH field has a ratio of 1.0, which is more consistent with that of MCF and 14C-constrained OH (Brenninkmeijer et al., 1992; Krol and Lelieveld, 2003; Bousquet et al., 2005). However, as discussed by Spivakovsky et al. (2000), the TransCom OH field may overestimate the southern extratropics OH by ∼25 %. The lower N ∕ S ratios inferred from MCF observations are mainly due to high [OH] over the southern tropics (35 % higher than the northern tropics) (Bousquet et al., 2005). In contrast, process-based simulated OH is 10 %–26 % more abundant over the northern tropics than over the southern tropics and 35 % to >90 % higher over 30–90∘ N than 30–90∘ S in the CCMI models. Previous studies have attributed the inconsistency between the simulated and the observed OH N ∕ S ratios to a model overestimation of O3 and underestimation of CO over the Northern Hemisphere (Naik et al., 2013; Young et al., 2013; Strode et al., 2015), which have also been reported for CCMI models (Strode et al., 2016; Revell et al., 2018), as well as to a lack of OH recycling due to the presence of VOCs over rainforest (mainly located in the southern tropics) (Lelieveld et al., 2008; Archibald et al., 2011).
We further assessed the simulated OH spread by comparing the detailed spatial distributions of OH fields in Figs. 2 and S2. Nearly all CCMI models and two versions of the INCA model simulated high [OH] over eastern North America and South and East Asia, which is related to higher tropospheric O3 concentrations (Cooper et al., 2014; Lu et al., 2018) and NOx emissions from human activities (Lamarque et al., 2010; Miyazaki et al., 2012). High [OH] over these emission hot spots dominates the aforementioned simulated large N ∕ S ratio. Some models also simulated high OH values over the African savanna plains (MOCAGE and INCA excluded) in regions with intense biomass burning (van der Werf et al., 2006) and soil NOx emissions (Yienger and Levy 1995; Vinken et al., 2014). The O3 concentrations used to generate the TransCom OH field were larger in the southern tropics than in the northern latitudes (Spivakovsky et al., 2000), in contrast to recent observations (Cooper et al., 2014). Therefore, TransCom shows the highest [OH] over the southern tropics during biomass burning seasons (Spivakovsky et al., 2000) and thus a lower N ∕ S ratio.
Despite consistency on high OH values over regions influenced by human activities and biomass burning, models show the largest discrepancies over some natural ecosystem such as tropical rainforests (Fig. S2). For example, INCA, CESM, HadGEM3-Es, MRI-ESM1r1, MOCAGE, and GEOSCCM simulated overall low [OH] (4×105–14×105 molec cm−3) over tropical rainforests despite differences in details, while EMAC, CMAM, SOCOL3, and UMUKCA-UCAM simulated overall high [OH] (16×105 – more than 25×105 molec cm−3). Tropospheric mean [OH] over the Amazon forest shows the largest variations of molec cm−3, accounting for more than 50 % of the multi-model mean (Fig. S2). In a more diffuse way, high latitudes of the Northern Hemisphere also contribute to model spread (25 %–35 % of the model mean; Fig. S2). Besides these, inter-model differences also exist over the open ocean (up to 25 % of the model mean; Fig. S2). Most simulated OH fields show higher concentrations over continents or coastal areas due to higher precursor emissions, while MRI-ESM1r1, EMAC, and GEOSCCM also simulated high values ( molec cm−3) over the open ocean. Factors contributing to these inter-model differences are further discussed in Sect. 3.3
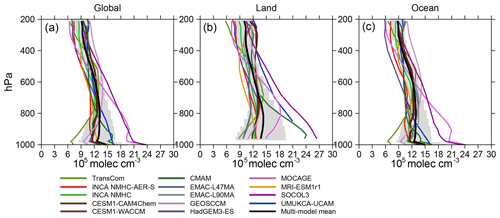
Figure 3Vertical distributions of [OH] averaged over the globe (a), land (b), and ocean (c) for 2000–2010. Color lines represent [OH] from individual model simulations, black lines represent multi-model mean values, and gray shades represent the standard deviations.
Table 4Global mean [OH] averaged over the troposphere and three vertical pressure levels (105 molec cm−3) over the years 2000 to 2010. Multi-model means and standard deviations (mean ± SD) are also shown.
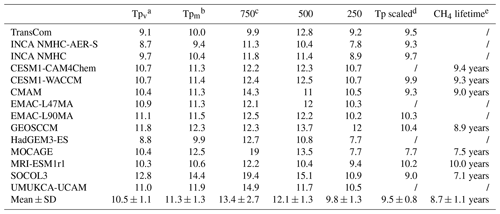
a Tpv refers to the volume-weighted tropospheric mean [OH]. b Tpm refers to the mass-weighted tropospheric mean [OH]. c 750 refers to the volume-weighted average from the surface to 750 hPa, 500 refers to the volume-weighted average from 750 to 500 hPa, and 250 refers to the volume-weighted average from 500 to 250 hPa. d “Tp scaled” refers to the volume-weighted global tropospheric mean [OH] after scaling to the same CH4 loss as with INCA NMHC in 2000. e CH4 lifetime is the calculated global atmospheric CH4 burden divided by the annual total CH4 tropospheric chemical loss.
3.2 Vertical distributions
Figure 3 shows the vertical distribution of OH fields and Table 4 provides the volume-weighted mean [OH] averaged over the troposphere and over three pressure altitudinal intervals representing the planetary boundary layer, the mid-troposphere, and the upper troposphere (surface–750, 750–500, and 500–250 hPa, respectively). At the global scale, the mean tropospheric concentration of TransCom OH increases by a factor of nearly 2 from the surface (7×105 molec cm−3) to 600 hPa (13×105 molec cm−3) and then decreases rapidly with altitude (7×105 molec cm−3 at 250 hPa). UMUKCA-UCAM, HadGEM3-ES, CMAM, MOCAGE, and SOCOL3, on the other hand, all show a continuous decrease in [OH] with altitude from the surface to the upper troposphere (e.g., the global mean concentrations of MOCAGE OH decrease from 23.6×105 molec cm−3 at the surface to 6.4×105 molec cm−3 at 250 hPa). Other OH profiles show much smaller vertical variations in the troposphere (standard deviations of the mean value below 200 hPa molec cm−3).
Model-simulated OH vertical distributions can also be different over land versus ocean (Fig. 3) and between the different latitudinal bands (Fig. S3). For example, SOCOL3 [OH] continuously decreases with altitude over both land and ocean; MOCAGE OH increases from the surface (14.9×105 molec cm−3) to 800 hPa (18.2×105 molec cm−3) and then decreases over land but almost continuously decreases over the ocean; CMAM and UMUKCA-UCAM only show significant vertical variations in [OH] over land. Vertical variations of most OH fields can be attributed to middle- and low-latitude regions, except for those of SOCOL3 and MOCAGE, which also decrease with altitude over middle and high northern latitudes (45–90∘ N, see Fig. S3).
3.3 Factors contributing to inter-model differences
Tropospheric OH is produced primarily through the reaction of O(1D) with H2O and secondarily through the reaction of NO with HO2 and RO2; it is removed primarily by reacting with CO and CH4 (Logan et al., 1981). Hence, factors controlling inter-model OH discrepancies can be complex as differences in model emissions, chemistry, physics, and dynamics can together impact [OH] (Nicely et al., 2017). Here we propose a qualitative analysis focusing on both emissions and chemical mechanisms. A more quantitative analysis would require a detailed model output of production and loss pathways that is beyond the scope of this work.
Table 5Global volume-weighted mean CO, NO, and O3 mixing ratios averaged over the whole troposphere and three pressure altitude levels for CCMI models from 2000 to 2010. Multi-model means and standard deviations (mean ± SD) are also shown.
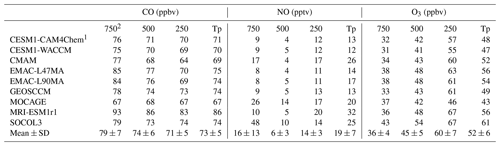
1 HadGEM3-ES and UMUKCA-UCAM are not analyzed since model output has been regridded to vertical pressure levels that are too coarse. 2 Tp refers to the total tropospheric average, 750 refers to the average from the surface to 750 hPa, 500 refers to the average from 750 hPa to 500 hPa, and 250 refers to the average from 500 to 250 hPa.
To analyze inter-model differences in OH vertical distributions, we compared CO, NO, and O3 mixing ratios in Table 5 as well as O(1D) photolysis rates and specific humidity in Table S4. The inter-model variations (calculated as the standard deviation divided by the multi-model mean) in tropospheric O(1D) photolysis rates, specific humidity, and CO mixing ratios are usually <10 %–20 %, while NO mixing ratios show a larger variation of 38 % (12–32 pptv). MRI-ESM1r1 simulated the highest NO tropospheric mixing ratio, mainly attributable to high values above 250 hPa, where OH formation is limited by H2O. In addition, MRI-ESM1r1 has ∼20 % more CO emissions than MOCAGE and GEOSCCM (Fig. S5), leading to about 10 ppbv higher CO mixing ratios and offsetting (for [OH]) its higher NOx emissions and NO mixing ratios. The high NO mixing ratios near the surface and mid-troposphere simulated by SOCOL3 (48 pptv below 750 hPa and 10 pptv from 750 to 500 hPa), MOCAGE (26 pptv below 750 hPa and 14 pptv from 750 to 500 hPa), and CMAM (17 ppbv below 750 hPa) are consistent with their high tropospheric and near-surface [OH]. Tropospheric O3 can also influence the primary production of OH, and the tropospheric O3 burden reflects the combined effects of NOx, CO, and VOCs. The high O3 over the lower troposphere simulated by SOCOL3 and the low O3 over the upper troposphere simulated by MOCAGE can contribute to explaining the high and low [OH] simulated by the two models over the corresponding altitudes, respectively.
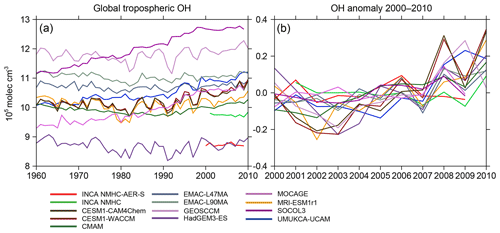
Figure 4(a) Interannual variations of global volume-weighted tropospheric mean [OH] from CCMI and INCA model simulations from 1960 to 2010. (b) OH anomaly during 2000–2010 in reference to the mean concentration over the period 2000–2010 for each model.
Lightning NOx, which is mainly emitted in the middle and upper troposphere, can contribute to inter-model differences in NO and OH distributions (Murray et al., 2013, 2014). We compare lightning NOx emissions calculated by CCMI models in Table S3. High lightning NOx emissions simulated by MRI-ESM1r1 above 250 hPa can explain high NO mixing ratios and increasing OH with altitude over the upper troposphere for this model (Fig. 3). However, high NO mixing ratios in the lower troposphere simulated by MOCAGE and SOCOL3 do not correspond to high lightning NOx emissions for these models. Besides emissions, previous studies have reported additional factors leading to high surface NO and NO2. The overestimation of NO by MOCAGE could be due to the lack of N2O5 heterogeneous hydrolysis on tropospheric aerosol, which is an efficient sink for NOx (Teyssèdre et al., 2007). SOCOL3 does not include N2O5 heterogeneous hydrolysis and also overestimates tropospheric NO production by NO2 photolysis compared to other models due to issues with the lookup tables used in the calculation of photolysis rates (Revell et al., 2018). We conclude here that physical and chemical processes related to NO production and loss can have a large impact on OH burden and its vertical distribution. In this context, an improved representation of the partitioning between NO and other nitrogen species in the models seems of great importance to correctly simulate tropospheric [OH].
Concerning the spatial distributions, as mentioned in Sect. 3.1, the largest model discrepancies are found over tropical rainforests. The [OH] over tropical rainforest regions are mostly sensitive to natural emissions including NOx and NMVOCs, which vary among the models. Previous studies showed that [OH] is more sensitive to soil and lightning emissions than to wildfires because the former sources only emit NOx (OH source), whereas the latter emit NOx, CO, and VOCs together (OH sources and OH sinks; see Murray et al., 2014). Soil NOx emissions in CCMI models range from around 4 Tg N yr−1 in MOCAGE to more than 7 Tg N yr−1 in GEOSCCM and 9 Tg N yr−1 in CMAM (Naik et al., 2013; Yienger and Levy, 1995). Lightning NOx emissions range from 3.7 to 10.2 Tg yr−1 (Table S3). In particular, lower NOx emissions over South America and Africa in MOCAGE might be linked to lower [OH] over this region (Fig. S5). Isoprene and other NMVOCs remove about 3 % and 7 % of tropospheric OH on a global scale, respectively (Spivakovsky et al., 2000; Murray et al., 2014), and can be more important over tropical regions with higher emission rates (Sindelarova et al., 2014). The higher [OH] over tropical rainforests simulated by CMAM and UMUKCA-UCAM may be due to lacking or less OH destruction by VOCs in these models. Therefore, the inter-model differences in OH spatial distributions over tropical rainforests may result from differences in natural emissions of VOC species and different related chemical reactions. Stratospheric ozone can contribute to inter-model OH discrepancies by influencing O(1D) photolysis rates. However, we find that models that simulated lower stratosphere and total ozone column do not correspond to higher O(1D) photolysis rates and [OH] (Table S5 and Fig. S4), since differences in photolysis schemes coupled to CCMI models can also influence the calculation of O(1D) photolysis rates (Sukhodolov et al., 2016).
3.4 Interannual variations of OH
Figure 4 shows the time series of volume-weighted tropospheric mean [OH] from 1960 to 2010 (from REF-C1 CCMI comparison). During this period, all OH fields show small year-to-year variations of 1.9±1.2 %, remaining within molec cm−3. CCMI models simulated significantly different OH long-term evolutions from 1960 to 1980. For example, [OH] continuously decreases in the CMAM and HadGEM3-ES simulations ( molec cm−3; −3.4 %) and increases in SOCOL3 ( molec cm−3; +4.5 %), UMUKCA-UCAM ( molec cm−3; +4.8 %), and MOCAGE ( molec cm−3; +4.8 %) during 1960–1980, while other models show no obvious long-term trend. After 1980 (1990 for CMAM), all models show stabilized or slightly increasing [OH]. For our period of interest (after 2000) and focusing on the anomaly in [OH] compared to the 2000–2010 mean (Fig. 4b), OH year-to-year variations are found to be smaller than in previous decades and [OH] only increases by about 0.1–0.3×105 molec cm−3 from 2000 to 2010.
Previous atmospheric chemistry model studies have concluded that anthropogenic activities lead to only a small perturbation of the OH burden, as increased OH production tends to be compensated for by an increased loss through reactions with CO and CH4 (Lelieveld et al., 2000; Naik et al., 2013). Recent studies highlighted the fact that the El Niño–Southern Oscillation can significantly contribute to [OH] interannual variations (Turner et al., 2018; Rowlinson et al., 2019). By combining factors that influence OH, Nicely et al. (2018) modeled a small interannual variability of 1.6 % during 1980–2015. The year-to-year variations of most CCMI and INCA OH fields are consistent with Nicely et al. (2018) but much smaller than the OH interannual variability based on MCF observations (e.g., Bousquet et al., 2005; Montzka et al., 2011), which can reach 8.5±1.0 % from 1980 to 2000 and 2.3±1.5 % from 1998 to 2007 compared to 2.1±0.8 % and 1.0±0.5 % here for these two periods. As for OH trend, the ensemble of ACCMIP models simulated large divergent OH changes (even in their signs) from 1850 to 2000 but revealed a consistent and significant increase of 3.5±2.2 % from 1980 to 2000 (Naik et al., 2013). Here, for the same period the increase in CCMI [OH] is 4.6±2.4 %, consistent with the ACCMIP project (Naik et al., 2013) and with other atmospheric chemistry model studies (Dentener et al., 2003; John et al., 2012; Holmes et al., 2013; Dalsøren et al., 2016). The slightly increasing [OH] after 2000 inferred here as well as in previous model simulations (e.g., Nicely et al., 2018) cannot help to explain stalled and renewed CH4 growth during the 2000s, as opposed to the decreasing [OH] from the mid-2000s calculated by Rigby et al. (2017) and Turner et al. (2107) based on MCF observations.
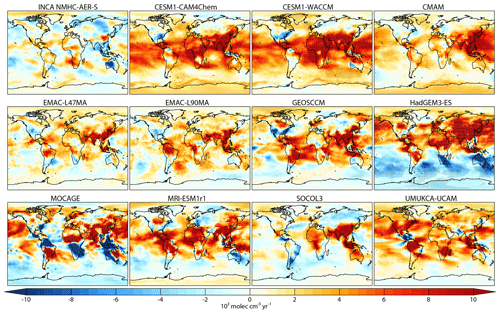
Figure 5Spatial distribution of tropospheric OH trends from 2000 to 2010 (103 molec cm−3 yr−1). Black dots denote model grid cells with statistically significant trends (p value <0.05).
We further analyzed regional [OH] trends from 2000 to 2010 in Fig. 5. Instead of dividing subdomains as Naik et al. (2013) did, we calculated the trend for each model grid cell to identify and distinguish regions with different trends. Most models show significant positive [OH] trends over tropical regions (0.05–0.1×105 molec cm−3 yr−1) and over East and Southeast Asia ( molec cm−3 yr−1). By comparing the spatial distribution of OH trends with specific humidity (Fig. S6a), NOx and CO emissions (Fig. S6b), and the stratosphere O3 column trend, we find that positive OH trends over tropical regions mainly correspond to increases in water vapor (Fig. S6a), while faster NOx emission increases (>5 % yr−1) than CO (<2 % yr−1) are consistent with positive OH trends over East and Southeast Asia (Fig. S6b). From 2000 to 2010, NOx emissions in the MACCity (RCP8.5) inventory increased by 83 % over East Asia, which is much larger than the CO increase (8 %) (Riahi et al., 2011). Over the rest of the extratropical regions such as North America and western Europe, the models disagree on the sign of OH change. In the Southern Hemisphere, where biogenic and fire emissions dominate, most OH fields do not show clear trends and the inter-model differences are even larger. For example, MOCAGE simulated an OH decrease of molec cm−3 yr−1 over the Amazon, South Africa, and Indonesia, whereas MRI-ESM and EMAC-L90MA simulated positive OH trends over these regions. CMAM and HadGEM3-ES show significant increasing and decreasing OH trends over the Antarctic region, respectively, consistent with the significant changes found for stratospheric O3 (Fig. S6c).
In the following, we investigate how the differences in mean [OH] and variations presented in this section affect the CH4 burden and its variations for the period 2000–2016.
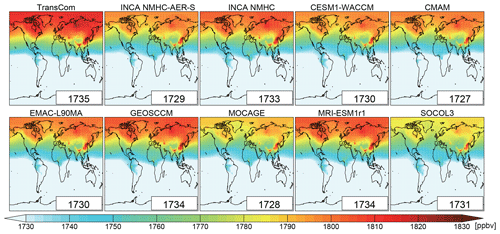
Figure 6Spatial distribution of volume-weighted tropospheric mean CH4 mixing ratios averaged from 2000 to 2010 as simulated by LMDz with different OH fields in the LMDz model. The global mean values (ppbv) are shown as insets.
4.1 Global total CH4 burden
We now present the results based on the first set of LMDz experiments, in which the LMDz model was run for 30 years, recycling the year 2000 until the steady state is reached. The simulations using the OH fields as given by the CCMI and INCA models provide a wide range of values for the tropospheric global mean CH4 mixing ratios (Table 6), from 1204 ppbv (SOCOL3; with a global volume-weighted tropospheric mean [OH] of 12.8×105 molec cm−3) to 1822 ppbv (INCA NMHC-AER-S; with a global volume-weighted tropospheric mean [OH] of 8.7×105 molec cm−3). It appears that the global CH4 burden is not only sensitive to the global mean [OH], but also to its vertical distribution. Indeed, the OH radicals in the lower troposphere are more efficient to oxidize CH4 molecules because the CH4 + OH reaction rate increases with temperature (Eq. 1). When considering the standard atmosphere, the reaction rate corresponding to the surface temperature of 288 K ( s−1) is more than twice that for the 500 hPa temperature of 253 K ( s−1). Despite similar volume-weighted tropospheric mean [OH] of molec cm−3, MOCAGE simulated much lower CH4 mixing ratios (1275 ppbv) than CMAM (1540 ppbv) and MRI-ESM1r1 (1639 ppbv) because of its higher near-surface [OH] (19×105 molec m−3) (Table 4). Previous studies have demonstrated the sensitivity of CH4 oxidation to lower tropical temperature (Spivakovsky et al., 2000; John et al., 2012), and our simulations show that 36 %–46 % of CH4 is oxidized over lower tropical regions (surface–750 hPa; 30∘ S–30∘ N) (Table S6). The spatial distribution of the OH radicals also slightly influences CH4 oxidation. Indeed, the [OH] of EMAC-L90MA is higher than that of CESM-WACCM for both tropospheric (11.1×105 versus 10.7×105 molec cm−3) and near-surface (12.5×105 versus 12.4×105 molec cm−3) means, but a slightly higher CH4 burden is found for the former (1579 versus 1575 ppbv; Table 6). This is because EMAC-L90MA simulated higher [OH] over the ocean, while CESM-WACCM OH is more concentrated over land closer to CH4 source regions. The model experiments also emphasize that volume-weighted tropospheric concentrations cannot fully indicate the atmospheric oxidizing efficiency for CH4, as has been discussed by Lawrence et al. (2001). Tropospheric mean [OH] weighted by reaction rates with CH4, which consider both temperature and CH4 distributions, can be a better indicator for CH4 oxidation (Lawrence et al., 2001).
4.2 Impacts on CH4 spatial distribution and growth rate
In order to address the question of the interannual variability of atmospheric CH4, we scaled each OH field globally to get the same CH4 loss (for the year 2000) as the one obtained with the INCA NMHC OH field (see Sect. 2.2.2). The single global scaling factor (per OH field) for the year 2000 is applied to every year between 2000 and 2010. As listed in Table 4, after scaling most OH fields have volume-weighted tropospheric mean concentrations closer to INCA NMHC (9.7×105 molec cm−3), within the range of 9.0–10.4×105 molec cm−3. One exception is MOCAGE, with tropospheric mean [OH] scaled to 7.7×105 molec cm−3, due to its distinct vertical distribution (Sect. 3.2). This scaling of OH makes it possible to start model experiments at the same initial CH4 burden. Although slightly modifying the magnitude of the global mean [OH], this scaling maintained the spatial and temporal differences and trend over the 2000–2010 period.
Table 7LMDz-simulated CH4 mixing ratios (ppbv) averaged over each latitudinal band and the years 2000 to 2010 from the standard experiment (Run_standard) using different OH fields. Multi-model means and standard deviations (mean ± SD) are also shown.
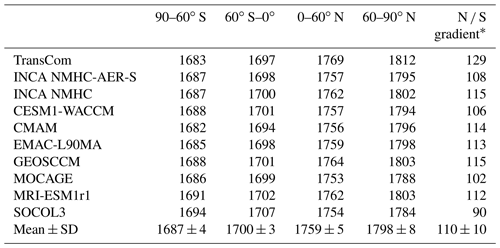
The ∗ N ∕ S gradient is defined as the difference between 60 to 90∘ N and 60 to 90∘ S.
4.2.1 Spatial distributions of tropospheric CH4 mixing ratios
We used the scaled OH fields to perform simulations between 2000 and 2010. Figure 6 shows the spatial distribution of tropospheric CH4 mixing ratios for the simulation Run_standard (Table 2; driven by OH with interannual variations) averaged over 2000–2010. Although all simulations started from the same initial conditions and OH fields were scaled to give the same global CH4 loss as INCA NMHC in 2000, LMDz simulations using the different scaled OH fields still generated a spread of tropospheric mean (8 ppbv) and a spatial distribution of CH4 mixing ratios averaged during 2000–2010. Differences between the global tropospheric mean [OH] cannot explain these differences (see Table 4). Clearly the different spatial (horizontal and vertical) and temporal variations of the OH fields (as described in Sect. 3), which were kept in this experiment by only scaling [OH] globally, significantly modify the simulated CH4 mixing ratios (Table 7 and Fig. 6). OH fields with an increasing trend will lead to lower LMDz-simulated CH4 mixing ratios. The LMDz simulations using the TransCom OH fields (without interannual variability) show the highest CH4 mixing ratios (1735 ppbv), while the one using the CMAM OH (with a slightly increasing OH trend during the decade) shows the lowest mixing ratios (1727 ppbv).
The differences in the spatial distribution of OH fields can influence LMDz-simulated CH4 spatial distributions. Looking at latitudinal CH4 mixing ratios, the inter-model differences appear larger than in the global mean (Fig. 6 and Table 7). The model spreads of the mean CH4 mixing ratios over 60–90∘ S and 60–90∘ N range from 1771 to 1794 ppbv and 1784 to 1812 ppbv, respectively. Here, we define the N ∕ S gradient of CH4 as the difference in the mean CH4 mixing ratio between the latitudinal bands 60–90∘ N and 60–90∘ S. With the TransCom OH field (N ∕ S ratio = 1.0), the model simulated 12 %–43 % larger N ∕ S gradients of CH4 (129 ppbv) than other simulations (90–115 ppbv) driven by OH fields with higher N ∕ S OH ratios of 1.2–1.5. Previous model studies have attributed the overestimation of the CH4 N ∕ S ratio to an underestimation of model inter-hemispheric exchange time (e.g., Zimmermann et al., 2018). Our results show that uncertainties in OH distributions can also contribute to such model biases.
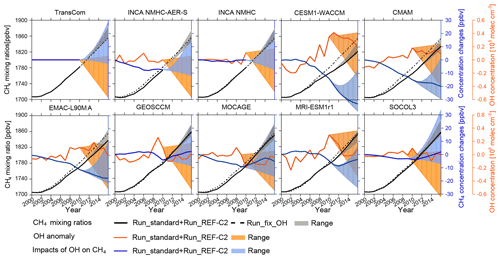
Figure 7Time series of global tropospheric CH4 mixing ratios and [OH] associated with the model experiments listed in Table 2. The black lines represent the evolution of CH4 mixing ratios with varying (solid lines) or with constant (dashed lines) OH. The varying OH case is obtained using OH inputs from Run_standard from 2000 to 2010 followed by Run_REF-C2 from 2011 to 2016 (see Table 2). The blue solid lines represent the corresponding differences between the simulations with varying OH and with constant OH. The orange solid line represents the corresponding anomalies in tropospheric [OH] (with the average over 2000–2010 as a reference). The shaded areas correspond to the range obtained from all simulations over 2010–2016 (Table 2) for tropospheric CH4 mixing ratios (gray), for changes in tropospheric CH4 mixing ratios (blue), and for changes in tropospheric [OH] (orange).
4.2.2 Changes in CH4 mixing ratios
To assess the influence of OH interannual variations on CH4 mixing ratios, we calculated the difference in the simulated CH4 between the standard run (Run_standard) and the simulations with fixed [OH] (Run_fix_OH, Table 2). The Run_fix_OH simulations show that global tropospheric mean CH4 mixing ratios increased by 75 ppbv from 2000 to 2010 (Fig. 7, black dashed lines) due to the enhanced emissions (Fig. 1). The increase in [OH] can obviously reduce CH4 growth. An increase in [OH] by 0.1–0.3×105 molec cm−3 (1 %–3 %) (Fig. 7, orange lines) during this period leads to a reduction of the CH4 mixing ratios by 5–15 ppbv by 2010 (Fig. 7, blue lines). The largest reductions are found when using CESM1-WACCM and CMAM OH fields, given the continuous OH growth in these models. Compared to Run_fix_OH, we estimated that such reductions in CH4 mixing ratios offset 7 %–20 % of the CH4 increase driven by the rising CH4 emissions of our scenario over the period 2000 to 2010.
To test whether the impacts of [OH] year-to-year variations on CH4 mixing ratios depend on the chosen emission scenarios, we compare the above results with those calculated by an extreme scenario in which model simulations are driven by fixed emissions (year 2000, Run_fix_emis and Run_ fix_emis_OH, Table 2). With emissions fixed to 2000, the CH4 mixing ratio increased by 2 ppbv from 2000 to 2010, and increasing OH (CESM-WACCM OH fields) can reduce the CH4 mixing ratio by 13.5 ppb in 2010, comparable to 13.9 ppb calculated by Run_std and Run_fix_OH with CESM-WACCM OH fields. The results indicate only a small effect of emission scenario choices on the absolute changes in CH4 mixing ratios due to OH variations. However, our choices have a large effect on relative change to the total modeled CH4 increase. Indeed, if we use the emission scenarios that match observations ( ppbv of CH4 mixing ratio increase from 2000–2010; Dlugokencky, 2019) instead of ∼70 ppb here , the CH4 mixing ratio changes due to OH can contribute to more than half (13.5–13.9 ppbv versus 25 ppbv) of the changes driven by emissions.
After 2010, CCMI REF-C2 experiments simulated increasing, relatively stable, or decreasing OH variations, thus having a variable influence on CH4 variations. Over the period 2011–2016, [OH] simulated by EMAC-L90MA, CESM-WACCM, and CMAM stabilizes at a level 0.2–0.4×105 molec cm−3 higher than the concentrations in 2000, further reducing CH4 mixing ratios by up to 20–30 ppbv in 2016 (Fig. 7, blue lines). Other OH fields have similar concentrations over 2010–2016 as in the early 2000s (Fig. 7, orange lines), thus simulating CH4 mixing ratios that remain close to Run_fix_OH with differences less than a few parts per billion by volume.
As large uncertainties remain regarding the interannual variations and trend of OH after 2010, we have tested two additional OH scenarios to assess the uncertainty range of the impact of OH changes (the orange areas in Fig. 7) on CH4 mixing ratios (the blue areas in Fig. 7): Run_OH_inc (with an annual increase of 0.1 % yr−1) and Run_OH_dec (with an annual decrease of 1 % yr−1). In these two scenarios, the mean [OH] of run_OH_dec is molec cm−3 (7 %) lower than run_OH_inc in 2016 relative to the Run_fix_OH . If OH decreases at 1 % yr−1 after 2010, by 2016 the differences in CH4 mixing ratios between Run_OH_dec and Run_fix_OH range −7–30 ppbv, with the lower end (−7 ppbv) simulated by OH from CESM1-WACCM given its highest [OH] in 2010. On the contrary, Run_OH_inc simulated 3–39 ppbv lower CH4 mixing ratios compared to Run_fix_OH (the blue areas in Fig. 7). As such, uncertainties in the OH trend can clearly lead to ppbv changes in CH4 mixing ratios (the gray areas in Fig. 7) after only 6 years of simulations compared to the fixed OH case.
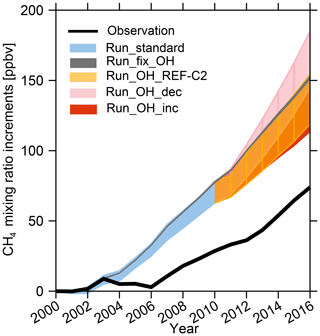
Figure 8Time series of surface CH4 mixing ratio increments compared to 2000 for NOAA observations (black line) and model ranges from all the LMDz experiments collected at observation sites (shades) and described in the text and in Table 2.
It is now interesting to compare the range of simulated [CH4] changes induced by OH scenarios to changes in surface CH4 observations in order to quantify how much of the model–observation mismatch could potentially be attributed to uncertainties in [OH] and its variability (Fig. 8). To do so, we used surface CH4 observations from the National Oceanic and Atmospheric Administration (NOAA) networks and selected stations with 17 years of continuous records over 2000–2016. The modeled surface CH4 mixing ratios are sampled according to station locations. Since the simulated absolute CH4 mixing ratios largely depend on the initial conditions and OH fields, we compared changes in the simulated and observed global CH4 mixing ratios starting at the same point in 2000. The observed CH4 shows zero growth between 2000 and 2006 and then increases by 5.6 ppbv yr−1 between 2006 and 2012 (6.4 ppb yr−1 for 2006–2010) and by 9.4 ppbv yr−1 after 2012 (Fig. 8). In this study, we do not expect to fit these CH4 trends as this intercomparison was not conducted with a set of optimized emissions. It has already been noted that standard CH4 emission inventories lead to overestimated CH4 mixing ratios (e.g., Saunois et al., 2016). Indeed, neither Run_standard nor Run_fix_OH simulations capture the stagnation during 2003–2006 and overestimate surface CH4 increments by 2.5–5.2 ppbv yr−1 during the period 2006–2010. We define the highest CH4 mixing ratios simulated by different OH as CH4−H, the lowest CH4 mixing ratios as CH4−L, and CH4 simulated by Run_fix_OH as CH4−fix_OH. Based on Run_fix_OH, on average over 2000–2016 and depending on the OH scenario, we found that [OH] changes can emphasize the model–observation mismatch by up to 19 % (mean values of (CH4-H–CH4-fix_OH) ∕ (CH4-fix_OH – observed CH4)) during 2000–2016) or limit the model–observation mismatch by up to 54 % (mean values of (CH4-fix_OH–CH4−L) ∕ (CH4-fix_OH – observed CH4) during 2000–2016) (Fig. 8). Such comparisons strongly suggest that a better understanding of OH interannual variations and trends is required in order to simulate more reliable CH4 trends in atmospheric chemistry models. Atmospheric chemistry transport model (Dalsøren et al., 2016) and box model studies (Rigby et al., 2017; Turner et al., 2017) have pointed out that variations in OH can partly explain the recent CH4 trends. However, current top-down estimates of CH4 emissions usually assume constant [OH] (Saunois et al., 2017) and attribute the model–observation discrepancies only to surface emissions rather than changes in [OH]. Our results confirm the potentially significant role played by the still uncertain OH changes in the actual changes in methane emissions since 2000.
We have analyzed 14 OH fields (11 from CCMI experiments, 2 from INCA model simulations, 1 from TransCom) to investigate inter-model differences in the spatial distributions and trends of tropospheric OH, and we estimated the influences of OH spatiotemporal distributions on tropospheric CH4 by feeding them in different simulations with the LMDz offline chemistry transport model.
Simulated global volume-weighted tropospheric mean [OH] is within the range of 8.7×105–12.8×105 molec cm−3, which is consistent with the (large) multi-model range of previous estimates. The CCMI and INCA models simulated larger [OH] in the Northern Hemisphere than in the Southern Hemisphere (N ∕ S ratio of 1.2–1.5), with consistently high OH values over anthropogenic emission hot spots in North America and East and Southeast Asia, while TransCom OH shows an N ∕ S ratio close to 1.0. In the vertical, TransCom OH reaches its maximum value at about 600 hPa, while CCMI and INCA OH fields either continuously decrease with altitude or show very small vertical variations in the troposphere. The factors most likely responsible for these inter-model differences include (i) large NO mixing ratios leading to high surface and mid-tropospheric [OH] (Teyssèdre et al., 2007; Revell et al., 2018) and (ii) different natural emissions as well as VOC species and chemical mechanisms driving spatial model discrepancies over natural ecosystems.
Simulated OH fields show small year-to-year variations, within molec cm−3 during 1960–2010. From 2000 to 2010, year-to-year variations in OH are smaller than in previous decades and all OH fields increase by about 0.01–0.03×105 molec cm−3 yr−1. Such an increase in OH is mainly attributed to the significant positive OH trend over East and Southeast Asia ( molec cm−3 yr−1) in response to more OH production by NOx than OH destruction by CO, as well as over tropical regions in response to increasing water vapor.
The inter-model differences in the tropospheric OH burden generate a wide range of CH4 burdens (1204–1882 ppbv) when used to simulate steady-state CH4 mixing ratios in the atmospheric chemistry model LMDz. Our findings suggest that not only different global mean [OH], but also differences in the horizontal and vertical distributions between OH fields are responsible for this range (CH4 destruction rates by OH increase with temperature).
The CH4 simulations for 2000–2016 using OH with interannual variation show that inter-model differences of the OH N ∕ S ratio lead to 12 %–43 % differences in the CH4 N ∕ S gradient. For the time period 2000–2010, we found that a 1 %–3 % increase in [OH] leads to a 5–15 ppb reduction of the CH4 mixing ratio until 2010, accounting for 7 %–20 % of the simulated emission-driven CH4 increase over this period. After 2010, the ensemble of OH scenarios tested here leads to differences in the CH4 mixing ratio of up to 30 ppb by 2016. Comparing with surface observations, we found that [OH] changes can emphasize the model–observation mismatch by up to 19 % or fill the gap between model simulations and observations by up to 54 % (Fig. 8). Therefore, addressing the OH variability in CH4 source inversions seems critical to avoid a wrong attribution of CH4 changes to emission changes only. Future work is needed to quantify the impact of this ensemble of OH fields on CH4 emissions obtained by inversion and to generate improved OH fields to be used in CH4 inversion studies.
Most of the CCMI datasets are available at the Centre for Environmental Data Analysis (CEDA; http://data.ceda.ac.uk/badc/wcrp-ccmi/data/CCMI-1/output; Hegglin and Lamarque, 2015), the Natural Environment Research Council's Data Repository for Atmospheric Science and Earth Observation. The output of CESM1-CAM4Chem and CESM1-WACCM for CCMI is available at http://www.earthsystemgrid.org (Climate Data Gateway at NCAR, 2019). Other datasets, including output of INCA model simulations and LMDz experiments, can be accessed by contacting the corresponding author.
The supplement related to this article is available online at: https://doi.org/10.5194/acp-19-13701-2019-supplement.
YZ, MS, and PB designed the study, analyzed data, and wrote the paper. BZ and XL helped with data analysis and model simulations. JC, RJ, and AS discussed the results. AB developed the LMDz code for running CH4 simulations. ED provided the atmospheric in situ data. Other coauthors provided numerical model outputs. All coauthors commented on the paper.
The authors declare that they have no conflict of interest.
This work benefited from the expertise of the Global Carbon Project methane initiative.
We acknowledge the modeling groups for making their simulations available for this analysis, the joint WCRP SPARC/IGAC Chemistry–Climate Model Initiative (CCMI) for organizing and coordinating the model data analysis activity, and the British Atmospheric Data Centre (BADC) for collecting and archiving the CCMI model output.
The EMAC simulations have been performed at the German Climate Computing Centre (DKRZ) through support from the Bundesministerium für Bildung und Forschung (BMBF). DKRZ and its scientific steering committee are gratefully acknowledged for providing the HPC and data archiving resources for this consortial project ESCiMo (Earth System Chemistry integrated Modelling).
The CESM project is supported primarily by the National Science Foundation.
Eugene Rozanov's work is partially supported by the Swiss National Science Foundation under grant 200020_182239 (POLE), and the information obtained will be used to improve the next versions of the CCM SOCOL.
Andrea Stenke was supported by the Swiss National Science Foundation under grant 200021_138037 (FuMES).
UMUKCA-UCAM model integrations were performed using the ARCHER UK National Supercomputing Service and MONSooN system, a collaborative facility supplied under the Joint Weather and Climate Research Programme, which is a strategic partnership between the UK Met Office and the Natural Environment Research Council.
This research has been supported by the Gordon and Betty Moore Foundation (grant no. GBMF5439), “Advancing Understanding of the Global Methane Cycle”.
This paper was edited by Astrid Kiendler-Scharr and reviewed by Vaishali Naik and one anonymous referee.
Allen, D. J. and Pickering, K. E.: Evaluation of lightning flash rate parameterizations for use in a global chemical transport model, J. Geophys. Res.-Atmos., 107, ACH 15-11-ACH 15-21, https://doi.org/10.1029/2002JD002066, 2002.
Bednarz, E. M., Maycock, A. C., Abraham, N. L., Braesicke, P., Dessens, O., and Pyle, J. A.: Future Arctic ozone recovery: the importance of chemistry and dynamics, Atmos. Chem. Phys., 16, 12159–12176, https://doi.org/10.5194/acp-16-12159-2016, 2016.
Bousquet, P., Hauglustaine, D. A., Peylin, P., Carouge, C., and Ciais, P.: Two decades of OH variability as inferred by an inversion of atmospheric transport and chemistry of methyl chloroform, Atmos. Chem. Phys., 5, 2635–2656, https://doi.org/10.5194/acp-5-2635-2005, 2005.
Bousquet, P., Ringeval, B., Pison, I., Dlugokencky, E. J., Brunke, E.-G., Carouge, C., Chevallier, F., Fortems-Cheiney, A., Frankenberg, C., Hauglustaine, D. A., Krummel, P. B., Langenfelds, R. L., Ramonet, M., Schmidt, M., Steele, L. P., Szopa, S., Yver, C., Viovy, N., and Ciais, P.: Source attribution of the changes in atmospheric methane for 2006–2008, Atmos. Chem. Phys., 11, 3689–3700, https://doi.org/10.5194/acp-11-3689-2011, 2011.
Brenninkmeijer, C. A. M., Manning, M. R., Lowe, D. C., Wallace, G., Sparks, R. J., and Volz-Thomas, A.: Interhemispheric asymmetry in OH abundance inferred from measurements of atmospheric 14CO, Nature, 356, 50–52, https://doi.org/10.1038/356050a0, 1992.
Ciais, P., Sabine, C., Bala, G., Bopp, L., Brovkin, V., and House, J. I.: Carbon and Other Biogeochemical Cycles, in: Climate Change 2013: The Physical Science Basis, Contribution of Working Group I to the Fifth Assessment Report of the Intergovernmental Panel on Climate Change Change, Cambridge University Press, Cambridge, United Kingdom and New York, NY, USA, 465–570, 2013.
Climate Data Gateway at NCAR: available at: https://www.earthsystemgrid.org/, last access: May 2019.
Cooper, O. R., Parrish, D. D., Ziemke, J., Balashov, N. V., Cupeiro, M., Galbally, I. E., Gilge, S., Horowitz, L., Jensen, N. R., Lamarque, J. F., Naik, V., Oltmans, S. J., Schwab, J., Shindell, D. T., Thompson, A. M., Thouret, V., Wang, Y., and Zbinden, R. M.: Global distribution and trends of tropospheric ozone: An observation-based review, Elementa, 2, 000029, https://doi.org/10.12952/journal.elementa.000029, 2014.
Crutzen, P.: A discussion of the chemistry of some minor constituents in the stratosphere and troposphere, Pure Appl. Geophys., 106, 1385–1399, https://doi.org/10.1007/bf00881092, 1973.
Dalsøren, S. B., Myhre, C. L., Myhre, G., Gomez-Pelaez, A. J., Søvde, O. A., Isaksen, I. S. A., Weiss, R. F., and Harth, C. M.: Atmospheric methane evolution the last 40 years, Atmos. Chem. Phys., 16, 3099–3126, https://doi.org/10.5194/acp-16-3099-2016, 2016.
Dee, D. P., Uppala, S. M., Simmons, A. J., Berrisford, P., Poli, P., Kobayashi, S., Andrae, U., Balmaseda, M. A., Balsamo, G., Bauer, P., Bechtold, P., Beljaars, A. C. M., van de Berg, L., Bidlot, J., Bormann, N., Delsol, C., Dragani, R., Fuentes, M., Geer, A. J., Haimberger, L., Healy, S. B., Hersbach, H., Hólm, E. V., Isaksen, L., Kållberg, P., Köhler, M., Matricardi, M., McNally, A. P., Monge-Sanz, B. M., Morcrette, J.-J., Park, B.-K., Peubey, C., de Rosnay, P., Tavolato, C., Thépaut, J.-N., and Vitart, F.: The ERA-Interim reanalysis: configuration and performance of the data assimilation system, Q. J. Roy. Meteor. Soc., 137, 553–597, https://doi.org/10.1002/qj.828, 2011.
Dentener, F., Peters, W., Krol, M., van Weele, M., Bergamaschi, P., and Lelieveld, J.: Interannual variability and trend of CH4 lifetime as a measure for OH changes in the 1979–1993 time period, J. Geophys. Res.-Atmos., 108, 4442, https://doi.org/10.1029/2002jd002916, 2003.
Deushi, M. and Shibata, K.: Development of a Meteorological Research Institute chemistry-climate model version 2 for the study of tropospheric and stratospheric chemistry, Pap. Meteorol. Geophys., 62, 1–46, 2011.
Dlugokencky, E. J.: NOAA/ESRL, available at: https://www.esrl.noaa.gov/gmd/ccgg/trends_ch4/ (last access: May 2019), 2018.
Ehhalt, D. H.: The atmospheric cycle of methane, Tellus, 26, 58–70, https://doi.org/10.1111/j.2153-3490.1974.tb01952.x, 1974.
Emanuel, K. A.: A Scheme for Representing Cumulus Convection in Large-Scale Models, J. Atmos. Sci., 48, 2313–2329, https://doi.org/10.1175/1520-0469(1991)048<2313:ASFRCC>2.0.CO;2, 1991.
Etiope, G.: Natural Gas Seepage: The Earth's Hydrocarbon Degassing, in: Springer International Publishing, Cham, 195 pp., 2015.
Etminan, M., Myhre, G., Highwood, E. J., and Shine, K. P.: Radiative forcing of carbon dioxide, methane, and nitrous oxide: A significant revision of the methane radiative forcing, Geophys. Res. Lett., 43, 12614–12623, https://doi.org/10.1002/2016GL071930, 2016.
Garcia, R. R. and Boville, B. A.: Downward control of the meanmeridional circulation and temperature distribution of the polar winter stratosphere, J. Atmos. Sci., 51, 2238–2245, 1994.
Granier, C., Bessagnet, B., Bond, T., D'Angiola, A., Denier van der Gon, H., Frost, G. J., Heil, A., Kaiser, J. W., Kinne, S., Klimont, Z., Kloster, S., Lamarque, J.-F., Liousse, C., Masui, T., Meleux, F., Mieville, A., Ohara, T., Raut, J.-C., Riahi, K., Schultz, M. G., Smith, S. J., Thompson, A., van Aardenne, J., van der Werf, G. R., and van Vuuren, D. P.: Evolution of anthropogenic and biomass burning emissions of air pollutants at global and regional scales during the 1980–2010 period, Clim. Change, 109, 163, https://doi.org/10.1007/s10584-011-0154-1, 2011.
Grewe, V., Brunner, D., Dameris, M., Grenfell, J. L., Hein, R., Shindell, D., and Staehelin, J.: Origin and variability of upper tropospheric nitrogen oxides and ozone at northern mid-latitudes, Atmos. Environ., 35, 3421–3433, https://doi.org/10.1016/S1352-2310(01)00134-0, 2001.
Guth, J., Josse, B., Marécal, V., Joly, M., and Hamer, P.: First implementation of secondary inorganic aerosols in the MOCAGE version R2.15.0 chemistry transport model, Geosci. Model Dev., 9, 137–160, https://doi.org/10.5194/gmd-9-137-2016, 2016.
Hardiman, S. C., Butchart, N., O'Connor, F. M., and Rumbold, S. T.: The Met Office HadGEM3-ES chemistry-climate model: evaluation of stratospheric dynamics and its impact on ozone, Geosci. Model Dev., 10, 1209–1232, https://doi.org/10.5194/gmd-10-1209-2017, 2017.
Hauglustaine, D. A., Hourdin, F., Jourdain, L., Filiberti, M.-A., Walters, S., Lamarque, J.-F., and Holland, E. A.: Interactive chemistry in the Laboratoire de Météorologie Dynamique general circulation model: Description and background tropospheric chemistry evaluation, J. Geophys. Res.-Atmos., 109, D04314, https://doi.org/10.1029/2003JD003957, 2004.
Hegglin, M. I. and Lamarque, J.-F.: The IGAC/SPARC Chemistry-Climate Model Initiative Phase-1 (CCMI-1) model data output, NCAS British Atmospheric Data Centre, ADD ACCESS DATE, available at: http://catalogue.ceda.ac.uk/uuid/9cc6b94df0f4469d8066d69b5df879d5 (last access: May 2019), 2015 (data available at: http://data.ceda.ac.uk/badc/wcrp-ccmi/data/CCMI-1/output, last access: May 2019).
Holmes, C. D., Prather, M. J., Søvde, O. A., and Myhre, G.: Future methane, hydroxyl, and their uncertainties: key climate and emission parameters for future predictions, Atmos. Chem. Phys., 13, 285–302, https://doi.org/10.5194/acp-13-285-2013, 2013.
Hourdin, F. and Armengaud, A.: The Use of Finite-Volume Methods for Atmospheric Advection of Trace Species. Part I: Test of Various Formulations in a General Circulation Model, Mon. Weather Rev., 127, 822–837, https://doi.org/10.1175/1520-0493(1999)127<0822:tuofvm>2.0.co;2, 1999.
Hourdin, F., Mauritsen, T., Gettelman, A., Golaz, J.-C., Balaji, V., Duan, Q., Folini, D., Ji, D., Klocke, D., Qian, Y., Rauser, F., Rio, C., Tomassini, L., Watanabe, M., and Williamson, D.: The Art and Science of Climate Model Tuning, B. Am. Meteorol. Soc., 98, 589–602, https://doi.org/10.1175/bams-d-15-00135.1, 2017.
Hunke, E. C. and Lipscombe, W. H.: CICE: the Los Alamos sea ice model documentation and software user's manual, Version 4.0, LA-CC-06-012, Los Alamos National Laboratory, New Mexico, 2008.
Janssens-Maenhout, G., Crippa, M., Guizzardi, D., Muntean, M., Schaaf, E., Dentener, F., Bergamaschi, P., Pagliari, V., Olivier, J. G. J., Peters, J. A. H. W., van Aardenne, J. A., Monni, S., Doering, U., and Petrescu, A. M. R.: EDGAR v4.3.2 Global Atlas of the three major Greenhouse Gas Emissions for the period 1970–2012, Earth Syst. Sci. Data Discuss., https://doi.org/10.5194/essd-2017-79, 2017.
Jourdain, L., Bekki, S., Lott, F., and Lefèvre, F.: The coupled chemistry-climate model LMDz-REPROBUS: description and evaluation of a transient simulation of the period 1980–1999, Ann. Geophys., 26, 1391–1413, https://doi.org/10.5194/angeo-26-1391-2008, 2008.
John, J. G., Fiore, A. M., Naik, V., Horowitz, L. W., and Dunne, J. P.: Climate versus emission drivers of methane lifetime against loss by tropospheric OH from 1860–2100, Atmos. Chem. Phys., 12, 12021–12036, https://doi.org/10.5194/acp-12-12021-2012, 2012.
Jöckel, P., Kerkweg, A., Pozzer, A., Sander, R., Tost, H., Riede, H., Baumgaertner, A., Gromov, S., and Kern, B.: Development cycle 2 of the Modular Earth Submodel System (MESSy2), Geosci. Model Dev., 3, 717–752, https://doi.org/10.5194/gmd-3-717-2010, 2010.
Jöckel, P., Tost, H., Pozzer, A., Kunze, M., Kirner, O., Brenninkmeijer, C. A. M., Brinkop, S., Cai, D. S., Dyroff, C., Eckstein, J., Frank, F., Garny, H., Gottschaldt, K.-D., Graf, P., Grewe, V., Kerkweg, A., Kern, B., Matthes, S., Mertens, M., Meul, S., Neumaier, M., Nützel, M., Oberländer-Hayn, S., Ruhnke, R., Runde, T., Sander, R., Scharffe, D., and Zahn, A.: Earth System Chemistry integrated Modelling (ESCiMo) with the Modular Earth Submodel System (MESSy) version 2.51, Geosci. Model Dev., 9, 1153–1200, https://doi.org/10.5194/gmd-9-1153-2016, 2016.
Josse, B., Simon, P., and Peuch, V.-H.: Radon global simulations with the multiscale chemistry and transport model MOCAGE, Tellus B, 56, 339–356, 2004.
Jonsson, A. I., de Grandpré, J., Fomichev, V. I., McConnell, J. C., and Beagley, S. R.: Doubled CO2-induced coolingin the middle atmosphere: Photochemical analysis of the ozone radiative feedback, J. Geophys. Res, 109, D24103, https://doi.org/10.1029/2004JD005093, 2004.
Kirschke, S., Bousquet, P., Ciais, P., Saunois, M., Canadell, J. G., Dlugokencky, E. J., Bergamaschi, P., Bergmann, D., Blake, D. R., Bruhwiler, L., Cameron-Smith, P., Castaldi, S., Chevallier, F., Feng, L., Fraser, A., Heimann, M., Hodson, E. L., Houweling, S., Josse, B., Fraser, P. J., Krummel, P. B., Lamarque, J.-F., Langenfelds, R. L., Le Quéré, C., Naik, V., O'Doherty, S., Palmer, P. I., Pison, I., Plummer, D., Poulter, B., Prinn, R. G., Rigby, M., Ringeval, B., Santini, M., Schmidt, M., Shindell, D. T., Simpson, I. J., Spahni, R., Steele, L. P., Strode, S. A., Sudo, K., Szopa, S., van der Werf, G. R., Voulgarakis, A., van Weele, M., Weiss, R. F., Williams, J. E., and Zeng, G.: Three decades of global methane sources and sinks, Nat. Geosci., 6, 813–823, https://doi.org/10.1038/ngeo1955, 2013.
Krol, M. and Lelieveld, J.: Can the variability in tropospheric OH be deduced from measurements of 1,1,1-trichloroethane (methyl chloroform)?, J. Geophys. Res.-Atmos., 108, 4125, https://doi.org/10.1029/2002JD002423, 2003.
Lamarque, J.-F., Bond, T. C., Eyring, V., Granier, C., Heil, A., Klimont, Z., Lee, D., Liousse, C., Mieville, A., Owen, B., Schultz, M. G., Shindell, D., Smith, S. J., Stehfest, E., Van Aardenne, J., Cooper, O. R., Kainuma, M., Mahowald, N., McConnell, J. R., Naik, V., Riahi, K., and van Vuuren, D. P.: Historical (1850–2000) gridded anthropogenic and biomass burning emissions of reactive gases and aerosols: methodology and application, Atmos. Chem. Phys., 10, 7017–7039, https://doi.org/10.5194/acp-10-7017-2010, 2010.
Lambert, G. and Schmidt, S.: Reevaluation of the oceanic flux of methane: Uncertainties and long term variations, Chemosphere, 26, 579–589, https://doi.org/10.1016/0045-6535(93)90443-9, 1993.
Lawrence, M. G., Jöckel, P., and von Kuhlmann, R.: What does the global mean OH concentration tell us?, Atmos. Chem. Phys., 1, 37–49, https://doi.org/10.5194/acp-1-37-2001, 2001.
Lelieveld, J. and Dentener, F. J.: What controls tropospheric ozone?, J. Geophys. Res.-Atmos., 105, 3531–3551, https://doi.org/10.1029/1999JD901011, 2000.
Lelieveld, J., Dentener, F. J., Peters, W., and Krol, M. C.: On the role of hydroxyl radicals in the self-cleansing capacity of the troposphere, Atmos. Chem. Phys., 4, 2337–2344, https://doi.org/10.5194/acp-4-2337-2004, 2004.
Lelieveld, J., Butler, T. M., Crowley, J. N., Dillon, T. J., Fischer, H., Ganzeveld, L., Harder, H., Lawrence, M. G., Martinez, M., Taraborrelli, D., and Williams, J.: Atmospheric oxidation capacity sustained by a tropical forest, Nature, 452, 737–740, https://doi.org/10.1038/nature06870, 2008.
Lelieveld, J., Gromov, S., Pozzer, A., and Taraborrelli, D.: Global tropospheric hydroxyl distribution, budget and reactivity, Atmos. Chem. Phys., 16, 12477–12493, https://doi.org/10.5194/acp-16-12477-2016, 2016.
Levy, H.: Normal Atmosphere: Large Radical and Formaldehyde Concentrations Predicted, Science, 173, 141–143, https://doi.org/10.1126/science.173.3992.141, 1971.
Lin, X., Ciais, P., Bousquet, P., Ramonet, M., Yin, Y., Balkanski, Y., Cozic, A., Delmotte, M., Evangeliou, N., Indira, N. K., Locatelli, R., Peng, S., Piao, S., Saunois, M., Swathi, P. S., Wang, R., Yver-Kwok, C., Tiwari, Y. K., and Zhou, L.: Simulating CH4 and CO2 over South and East Asia using the zoomed chemistry transport model LMDz-INCA, Atmos. Chem. Phys., 18, 9475–9497, https://doi.org/10.5194/acp-18-9475-2018, 2018.
Locatelli, R., Bousquet, P., Hourdin, F., Saunois, M., Cozic, A., Couvreux, F., Grandpeix, J.-Y., Lefebvre, M.-P., Rio, C., Bergamaschi, P., Chambers, S. D., Karstens, U., Kazan, V., van der Laan, S., Meijer, H. A. J., Moncrieff, J., Ramonet, M., Scheeren, H. A., Schlosser, C., Schmidt, M., Vermeulen, A., and Williams, A. G.: Atmospheric transport and chemistry of trace gases in LMDz5B: evaluation and implications for inverse modelling, Geosci. Model Dev., 8, 129–150, https://doi.org/10.5194/gmd-8-129-2015, 2015.
Logan, J. A., Prather, M. J., Wofsy, S. C., and McElroy, M. B.: Tropospheric chemistry: A global perspective, J. Geophys. Res., 86, 7210, https://doi.org/10.1029/JC086iC08p07210, 1981.
Lu, X., Hong, J., Zhang, L., Cooper, O. R., Schultz, M. G., Xu, X., Wang, T., Gao, M., Zhao, Y., and Zhang, Y.: Severe Surface Ozone Pollution in China: A Global Perspective, Environ. Sci. Technol., 5, 487–494, https://doi.org/10.1021/acs.estlett.8b00366, 2018.
Madec, G.: NEMO ocean engine, Institut Piere-Simon Laplace (IPSL), France, 27, ISSN 1288-1619, 2008.
Marsh, D., Mills, M. J., Kinnison, D. E., Garcia, R. R., Lamarque, J.-F., and Calvo, N.: Climate change from 1850–2005 simulated in CESM1 (WACCM), J. Climate, 26, 7372–7391, https://doi.org/10.1175/JCLI-D-12-00558.1, 2013.
Masui, T., Matsumoto, K., Hijioka, Y., Kinoshita, T., Nozawa, T., Ishiwatari, S., Kato, E., Shukla, P., Yamagata, Y., and Kainuma, M.: An emission pathway for stabilization at 6 W m−2 radiative forcing, Clim. Change, 109, 59, https://doi.org/10.1007/s10584-011-0150-5, 2011.
McNorton, J., Chipperfield, M. P., Gloor, M., Wilson, C., Feng, W., Hayman, G. D., Rigby, M., Krummel, P. B., O'Doherty, S., Prinn, R. G., Weiss, R. F., Young, D., Dlugokencky, E., and Montzka, S. A.: Role of OH variability in the stalling of the global atmospheric CH4 growth rate from 1999 to 2006, Atmos. Chem. Phys., 16, 7943–7956, https://doi.org/10.5194/acp-16-7943-2016, 2016.
Miyazaki, K., Eskes, H. J., and Sudo, K.: Global NOx emission estimates derived from an assimilation of OMI tropospheric NO2 columns, Atmos. Chem. Phys., 12, 2263–2288, https://doi.org/10.5194/acp-12-2263-2012, 2012.
Montzka, S. A., Krol, M., Dlugokencky, E., Hall, B., Jöckel, P., and Lelieveld, J.: Small Interannual Variability of Global Atmospheric Hydroxyl, Science, 331, 67–69, https://doi.org/10.1126/science.1197640, 2011.
Molod, A., Takacs, L., Suarez, M., Bacmeister, J., Song, I.-S., and Eichmann, A.: The GEOS-5 Atmospheric General Circulation Model: Mean Climate and Development from MERRA to Fortuna, NASA Technical Report Series on Global Modeling and Data Assimilation, NASA TM-2012-104606, 28, 117 pp., 2012.
Molod, A., Takacs, L., Suarez, M., and Bacmeister, J.: Development of the GEOS-5 atmospheric general circulation model: evolution from MERRA to MERRA2, Geosci. Model Dev., 8, 1339–1356, https://doi.org/10.5194/gmd-8-1339-2015, 2015.
Morgenstern, O., Braesicke, P., O'Connor, F. M., Bushell, A. C., Johnson, C. E., Osprey, S. M., and Pyle, J. A.: Evaluation of the new UKCA climate-composition model – Part 1: The stratosphere, Geosci. Model Dev., 2, 43–57, https://doi.org/10.5194/gmd-2-43-2009, 2009.
Morgenstern, O., Hegglin, M. I., Rozanov, E., O'Connor, F. M., Abraham, N. L., Akiyoshi, H., Archibald, A. T., Bekki, S., Butchart, N., Chipperfield, M. P., Deushi, M., Dhomse, S. S., Garcia, R. R., Hardiman, S. C., Horowitz, L. W., Jöckel, P., Josse, B., Kinnison, D., Lin, M., Mancini, E., Manyin, M. E., Marchand, M., Marécal, V., Michou, M., Oman, L. D., Pitari, G., Plummer, D. A., Revell, L. E., Saint-Martin, D., Schofield, R., Stenke, A., Stone, K., Sudo, K., Tanaka, T. Y., Tilmes, S., Yamashita, Y., Yoshida, K., and Zeng, G.: Review of the global models used within phase 1 of the Chemistry-Climate Model Initiative (CCMI), Geosci. Model Dev., 10, 639–671, https://doi.org/10.5194/gmd-10-639-2017, 2017.
Murray, L. T., Logan, J. A., and Jacob, D. J.: Interannual variability in tropical tropospheric ozone and OH: The role of lightning, J. Geophys. Res.- Atmos., 118, 11468–411480, https://doi.org/10.1002/jgrd.50857, 2013.
Murray, L. T., Mickley, L. J., Kaplan, J. O., Sofen, E. D., Pfeiffer, M., and Alexander, B.: Factors controlling variability in the oxidative capacity of the troposphere since the Last Glacial Maximum, Atmos. Chem. Phys., 14, 3589–3622, https://doi.org/10.5194/acp-14-3589-2014, 2014.
Naik, V., Voulgarakis, A., Fiore, A. M., Horowitz, L. W., Lamarque, J.-F., Lin, M., Prather, M. J., Young, P. J., Bergmann, D., Cameron-Smith, P. J., Cionni, I., Collins, W. J., Dalsøren, S. B., Doherty, R., Eyring, V., Faluvegi, G., Folberth, G. A., Josse, B., Lee, Y. H., MacKenzie, I. A., Nagashima, T., van Noije, T. P. C., Plummer, D. A., Righi, M., Rumbold, S. T., Skeie, R., Shindell, D. T., Stevenson, D. S., Strode, S., Sudo, K., Szopa, S., and Zeng, G.: Preindustrial to present-day changes in tropospheric hydroxyl radical and methane lifetime from the Atmospheric Chemistry and Climate Model Intercomparison Project (ACCMIP), Atmos. Chem. Phys., 13, 5277–5298, https://doi.org/10.5194/acp-13-5277-2013, 2013.
Neu, J. L., Prather, M. J., and Penner, J. E.: Global atmospheric chemistry: Integrating over fractional cloud cover, J. Geophys. Res.-Atmos., 112, D11306, https://doi.org/10.1029/2006JD008007, 2007.
Nicely, J. M., Salawitch, R. J., Canty, T., Anderson, D. C., Arnold, S. R., Chipperfield, M. P., Emmons, L. K., Flemming, J., Huijnen, V., Kinnison, D. E., Lamarque, J.-F., Mao, J., Monks, S. A., Steenrod, S. D., Tilmes, S., and Turquety, S.: Quantifying the causes of differences in tropospheric OH within global models, J. Geophys. Res.-Atmos., 122, 1983–2007, https://doi.org/10.1002/2016jd026239, 2017.
Nicely, J. M., Canty, T. P., Manyin, M., Oman, L. D., Salawitch, R. J., Steenrod, S. D., Strahan, S. E., and Strode, S. A.: Changes in Global Tropospheric OH Expected as a Result of Climate Change Over the Last Several Decades, J. Geophys. Res.-Atmos., 123, 10774–10795, https://doi.org/10.1029/2018JD028388, 2018.
Nielsen, J. E., Pawson, S., Molod, A., Auer, B., da Silva, A. M., Douglass, A. R., Duncan, B., Liang, Q., Manyin, M., and Oman, L. D.: Chemical mechanisms and their applications in the Goddard Earth Observing System (GEOS) earth system model, J. Adv. Model. Earth Sy., 9, 3019–3044, 2017.
Nisbet, E. G., Manning, M. R., Dlugokencky, E. J., Fisher, R. E., Lowry, D., Michel, S. E., Myhre, C. L., Platt, S. M., Allen, G., Bousquet, P., Brownlow, R., Cain, M., France, J. L., Hermansen, O., Hossaini, R., Jones, A. E., Levin, I., Manning, A. C., Myhre, G., Pyle, J. A., Vaughn, B., Warwick, N. J., and White, J. W. C.: Very strong atmospheric methane growth in the four years 2014-2017: Implications for the Paris Agreement, Global Biogeochem. Cy., 33, 318–342, https://doi.org/10.1029/2018GB006009, 2019.
O'Connor, F. M., Johnson, C. E., Morgenstern, O., Abraham, N. L., Braesicke, P., Dalvi, M., Folberth, G. A., Sanderson, M. G., Telford, P. J., Voulgarakis, A., Young, P. J., Zeng, G., Collins, W. J., and Pyle, J. A.: Evaluation of the new UKCA climate-composition model – Part 2: The Troposphere, Geosci. Model Dev., 7, 41–91, https://doi.org/10.5194/gmd-7-41-2014, 2014.
Oman, L. D., Ziemke, J. R., Douglass, A. R., Waugh, D. W., Lang, C., Rodriguez, J. M., and Nielsen, J. E.: The response of tropical tropospheric ozone to ENSO, Geophys. Res. Lett., 38, L13706, https://doi.org/10.1029/2011GL047865, 2011.
Oman, L. D., Douglass, A. R., Ziemke, J. R., Rodriguez, J. M., Waugh, D. W., and Nielsen, J. E.: The ozone response to ENSO in Aura satellite measurements and a chemistry-climate simulation, J. Geophys. Res., 118, 965–976, https://doi.org/10.1029/2012JD018546, 2013.
Patra, P. K., Houweling, S., Krol, M., Bousquet, P., Belikov, D., Bergmann, D., Bian, H., Cameron-Smith, P., Chipperfield, M. P., Corbin, K., Fortems-Cheiney, A., Fraser, A., Gloor, E., Hess, P., Ito, A., Kawa, S. R., Law, R. M., Loh, Z., Maksyutov, S., Meng, L., Palmer, P. I., Prinn, R. G., Rigby, M., Saito, R., and Wilson, C.: TransCom model simulations of CH4 and related species: linking transport, surface flux and chemical loss with CH4 variability in the troposphere and lower stratosphere, Atmos. Chem. Phys., 11, 12813–12837, https://doi.org/10.5194/acp-11-12813-2011, 2011.
Patra, P. K., Krol, M. C., Montzka, S. A., Arnold, T., Atlas, E. L., Lintner, B. R., Stephens, B. B., Xiang, B., Elkins, J. W., Fraser, P. J., Ghosh, A., Hintsa, E. J., Hurst, D. F., Ishijima, K., Krummel, P. B., Miller, B. R., Miyazaki, K., Moore, F. L., Mühle, J., O'Doherty, S., Prinn, R. G., Steele, L. P., Takigawa, M., Wang, H. J., Weiss, R. F., Wofsy, S. C., and Young, D.: Observational evidence for interhemispheric hydroxyl-radical parity, Nature, 513, 219, https://doi.org/10.1038/nature13721, 2014.
Pison, I., Bousquet, P., Chevallier, F., Szopa, S., and Hauglustaine, D.: Multi-species inversion of CH4, CO and H2 emissions from surface measurements, Atmos. Chem. Phys., 9, 5281–5297, https://doi.org/10.5194/acp-9-5281-2009, 2009.
Poulter, B., Bousquet, P., Canadell, J. G., Ciais, P., Peregon, A., Saunois, M., Arora, V. K., Beerling, D. J., Brovkin, V., and Jones, C. D.: Global wetland contribution to 2000–2012 atmospheric methane growth rate dynamics, Environ. Res. Lett., 12, 094013, https://doi.org/10.1088/1748-9326/aa8391, 2017.
Prather, M. J., Holmes, C. D., and Hsu, J.: Reactive greenhouse gas scenarios: Systematic exploration of uncertainties and the role of atmospheric chemistry, Geophys. Res. Lett., 39, L09803, https://doi.org/10.1029/2012GL051440, 2012.
Price, C. and Rind, D.: Modeling Global Lightning Distributions in a General Circulation Model, Mon. Weather Rev., 122, 1930–1939, https://doi.org/10.1175/1520-0493(1994)122<1930:mgldia>2.0.co;2, 1994.
Prinn, R. G., Huang, J., Weiss, R. F., Cunnold, D. M., Fraser, P. J., Simmonds, P. G., McCulloch, A., Harth, C., Reimann, S., Salameh, P., O'Doherty, S., Wang, R. H. J., Porter, L. W., Miller, B. R., and Krummel, P. B.: Evidence for variability of atmospheric hydroxyl radicals over the past quarter century, Geophys. Res. Lett., 32, L07809, https://doi.org/10.1029/2004GL022228, 2005.
Randerson, J. T., van der Werf, G. R., Giglio, L., Collatz, G. J., and Kasibhatla, P. S.: Global Fire Emissions Database, Version 4.1 (GFEDv4), ORNL DAAC, Oak Ridge, Tennessee, USA, https://doi.org/10.3334/ORNLDAAC/1293, 2018.
Revell, L. E., Tummon, F., Stenke, A., Sukhodolov, T., Coulon, A., Rozanov, E., Garny, H., Grewe, V., and Peter, T.: Drivers of the tropospheric ozone budget throughout the 21st century under the medium-high climate scenario RCP 6.0, Atmos. Chem. Phys., 15, 5887–5902, https://doi.org/10.5194/acp-15-5887-2015, 2015.
Revell, L. E., Stenke, A., Tummon, F., Feinberg, A., Rozanov, E., Peter, T., Abraham, N. L., Akiyoshi, H., Archibald, A. T., Butchart, N., Deushi, M., Jöckel, P., Kinnison, D., Michou, M., Morgenstern, O., O'Connor, F. M., Oman, L. D., Pitari, G., Plummer, D. A., Schofield, R., Stone, K., Tilmes, S., Visioni, D., Yamashita, Y., and Zeng, G.: Tropospheric ozone in CCMI models and Gaussian process emulation to understand biases in the SOCOLv3 chemistry-climate model, Atmos. Chem. Phys., 18, 16155–16172, https://doi.org/10.5194/acp-18-16155-2018, 2018.
Riahi, K., Rao, S., Krey, V., Cho, C., Chirkov, V., Fischer, G., Kindermann, G., Nakicenovic, N., and Rafaj, P.: RCP 8.5 – A scenario of comparatively high greenhouse gas emissions, Clim. Change, 109, 33–57, https://doi.org/10.1007/s10584-011-0149-y, 2011.
Ridgwell, A. J., Marshall, S. J., and Gregson, K.: Consumption of atmospheric methane by soils: A process-based model, Global Biogeochem. Cy., 13, 59–70, https://doi.org/10.1029/1998GB900004, 1999.
Rigby, M., Prinn, R. G., Fraser, P. J., Simmonds, P. G., Langenfelds, R. L., Huang, J., Cunnold, D. M., Steele, L. P., Krummel, P. B., Weiss, R. F., O'Doherty, S., Salameh, P. K., Wang, H. J., Harth, C. M., Mühle, J., and Porter, L. W.: Renewed growth of atmospheric methane, Geophys. Res. Lett., 35, L22805, https://doi.org/10.1029/2008gl036037, 2008.
Rigby, M., Montzka, S. A., Prinn, R. G., White, J. W. C., Young, D., O'Doherty, S., Lunt, M. F., Ganesan, A. L., Manning, A. J., Simmonds, P. G., Salameh, P. K., Harth, C. M., Muhle, J., Weiss, R. F., Fraser, P. J., Steele, L. P., Krummel, P. B., McCulloch, A., and Park, S.: Role of atmospheric oxidation in recent methane growth, P. Natl. Acad. Sci. USA, 114, 5373–5377, https://doi.org/10.1073/pnas.1616426114, 2017.
Rowlinson, M. J., Rap, A., Arnold, S. R., Pope, R. J., Chipperfield, M. P., McNorton, J., Forster, P., Gordon, H., Pringle, K. J., Feng, W., Kerridge, B. J., Latter, B. L., and Siddans, R.: Impact of El Niño–Southern Oscillation on the interannual variability of methane and tropospheric ozone, Atmos. Chem. Phys., 19, 8669–8686, https://doi.org/10.5194/acp-19-8669-2019, 2019.
Sadourny, R. and Laval, K.: January and July performances of the LMD general circulation model, New Perspectives in Climate Modeling, in: New Perspectives in Climate Modeling, edited by: Berger, A. L. and Nicolis, C., Elsevier Press, Amsterdam, 173–197, 1984.
Sander, R., Jöckel, P., Kirner, O., Kunert, A. T., Landgraf, J., and Pozzer, A.: The photolysis module JVAL-14, compatible with the MESSy standard, and the JVal PreProcessor (JVPP), Geosci. Model Dev., 7, 2653–2662, https://doi.org/10.5194/gmd-7-2653-2014, 2014.
Sander, S. P., Abbatt, J., Barker, J. R., Burkholder, J. B., Friedl, R. R., Golden, D. M., Huie, R., Kurylo, M. J., Moortgat, G. K., Orkin, V. L., and Wine, P. H.: Chemical kinetics and photochemical data for use in atmospheric studies evaluation number 17, Pasadena, CA: Jet Propulsion Laboratory, National Aeronautics and Space Administration, 2011.
Saunois, M., Bousquet, P., Poulter, B., Peregon, A., Ciais, P., Canadell, J. G., Dlugokencky, E. J., Etiope, G., Bastviken, D., Houweling, S., Janssens-Maenhout, G., Tubiello, F. N., Castaldi, S., Jackson, R. B., Alexe, M., Arora, V. K., Beerling, D. J., Bergamaschi, P., Blake, D. R., Brailsford, G., Brovkin, V., Bruhwiler, L., Crevoisier, C., Crill, P., Covey, K., Curry, C., Frankenberg, C., Gedney, N., Höglund-Isaksson, L., Ishizawa, M., Ito, A., Joos, F., Kim, H.-S., Kleinen, T., Krummel, P., Lamarque, J.-F., Langenfelds, R., Locatelli, R., Machida, T., Maksyutov, S., McDonald, K. C., Marshall, J., Melton, J. R., Morino, I., Naik, V., O'Doherty, S., Parmentier, F.-J. W., Patra, P. K., Peng, C., Peng, S., Peters, G. P., Pison, I., Prigent, C., Prinn, R., Ramonet, M., Riley, W. J., Saito, M., Santini, M., Schroeder, R., Simpson, I. J., Spahni, R., Steele, P., Takizawa, A., Thornton, B. F., Tian, H., Tohjima, Y., Viovy, N., Voulgarakis, A., van Weele, M., van der Werf, G. R., Weiss, R., Wiedinmyer, C., Wilton, D. J., Wiltshire, A., Worthy, D., Wunch, D., Xu, X., Yoshida, Y., Zhang, B., Zhang, Z., and Zhu, Q.: The global methane budget 2000–2012, Earth Syst. Sci. Data, 8, 697–751, https://doi.org/10.5194/essd-8-697-2016, 2016.
Schultz, M. G., Heil, A., Hoelzemann, J. J., Spessa, A., Thonicke, K., Goldammer, J. G., Held, A. C., Pereira, J. M. C., and van het Bolscher, M.: Global wildland fire emissions from 1960 to 2000, Global Biogeochem. Cy., 22, GB2002, https://doi.org/10.1029/2007gb003031, 2008.
Scinocca, J. F., McFarlane, N. A., Lazare, M., Li, J., and Plummer, D.: Technical Note: The CCCma third generation AGCM and its extension into the middle atmosphere, Atmos. Chem. Phys., 8, 7055–7074, https://doi.org/10.5194/acp-8-7055-2008, 2008.
Sindelarova, K., Granier, C., Bouarar, I., Guenther, A., Tilmes, S., Stavrakou, T., Müller, J.-F., Kuhn, U., Stefani, P., and Knorr, W.: Global data set of biogenic VOC emissions calculated by the MEGAN model over the last 30 years, Atmos. Chem. Phys., 14, 9317–9341, https://doi.org/10.5194/acp-14-9317-2014, 2014.
Solomon, S., Kinnison, D. E., Bandoro, J., and Garcia, R.: Simulations of polar ozone depletion: an update, J. Geophys. Res., 120, 7958–7974, https://doi.org/10.1002/2015JD023365, 2015.
Spivakovsky, C. M., Logan, J. A., Montzka, S. A., Balkanski, Y. J., Foreman-Fowler, M., Jones, D. B. A., Horowitz, L. W., Fusco, A. C., Brenninkmeijer, C. A. M., Prather, M. J., Wofsy, S. C., and McElroy, M. B.: Three-dimensional climatological distribution of tropospheric OH: Update and evaluation, J. Geophys. Res.-Atmos., 105, 8931–8980, https://doi.org/10.1029/1999jd901006, 2000.
Stenke, A., Schraner, M., Rozanov, E., Egorova, T., Luo, B., and Peter, T.: The SOCOL version 3.0 chemistry-climate model: description, evaluation, and implications from an advanced transport algorithm, Geosci. Model Dev., 6, 1407–1427, https://doi.org/10.5194/gmd-6-1407-2013, 2013.
Staehelin, J., Tummon, F., Revell, L., Stenke, A., and Peter, T.: Tropospheric Ozone at Northern Mid-Latitudes: Modeled and Measured Long-Term Changes, Atmosphere, 8, 163, https://doi.org/10.3390/atmos8090163, 2017.
Stohl, A., Aamaas, B., Amann, M., Baker, L. H., Bellouin, N., Berntsen, T. K., Boucher, O., Cherian, R., Collins, W., Daskalakis, N., Dusinska, M., Eckhardt, S., Fuglestvedt, J. S., Harju, M., Heyes, C., Hodnebrog, Ø., Hao, J., Im, U., Kanakidou, M., Klimont, Z., Kupiainen, K., Law, K. S., Lund, M. T., Maas, R., MacIntosh, C. R., Myhre, G., Myriokefalitakis, S., Olivié, D., Quaas, J., Quennehen, B., Raut, J.-C., Rumbold, S. T., Samset, B. H., Schulz, M., Seland, Ø., Shine, K. P., Skeie, R. B., Wang, S., Yttri, K. E., and Zhu, T.: Evaluating the climate and air quality impacts of short-lived pollutants, Atmos. Chem. Phys., 15, 10529–10566, https://doi.org/10.5194/acp-15-10529-2015, 2015.
Strode, S. A., Duncan, B. N., Yegorova, E. A., Kouatchou, J., Ziemke, J. R., and Douglass, A. R.: Implications of carbon monoxide bias for methane lifetime and atmospheric composition in chemistry climate models, Atmos. Chem. Phys., 15, 11789–11805, https://doi.org/10.5194/acp-15-11789-2015, 2015.
Strode, S. A., Worden, H. M., Damon, M., Douglass, A. R., Duncan, B. N., Emmons, L. K., Lamarque, J.-F., Manyin, M., Oman, L. D., Rodriguez, J. M., Strahan, S. E., and Tilmes, S.: Interpreting space-based trends in carbon monoxide with multiple models, Atmos. Chem. Phys., 16, 7285–7294, https://doi.org/10.5194/acp-16-7285-2016, 2016.
Sukhodolov, T., Rozanov, E., Ball, W. T., Bais, A., Tourpali, K., Shapiro, A. I., Telford, P., Smyshlyaev, S., Fomin, B., Sander, R., Bossay, S., Bekki, S., Marchand, M., Chipperfield, M. P., Dhomse, S., Haigh, J. D., Peter, T., and Schmutz, W.: Evaluation of simulated photolysis rates and their response to solar irradiance variability, J. Geophys. Res.-Atmos. 121, 6066–6084, 2016.
Szopa, S., Balkanski, Y., Schulz, M., Bekki, S., Cugnet, D., Fortems-Cheiney, A., Turquety, S., Cozic, A., Déandreis, C., Hauglustaine, D., Idelkadi, A., Lathière, J., Lefevre, F., Marchand, M., Vuolo, R., Yan, N., and Dufresne, J.-L.: Aerosol and ozone changes as forcing for climate evolution between 1850 and 2100, Clim. Dynam., 40, 2223–2250, https://doi.org/10.1007/s00382-012-1408-y, 2013.
Telford, P. J., Abraham, N. L., Archibald, A. T., Braesicke, P., Dalvi, M., Morgenstern, O., O'Connor, F. M., Richards, N. A. D., and Pyle, J. A.: Implementation of the Fast-JX Photolysis scheme (v6.4) into the UKCA component of the MetUM chemistry-climate model (v7.3), Geosci. Model Dev., 6, 161–177, https://doi.org/10.5194/gmd-6-161-2013, 2013.
Terrenoire, E., Hauglustaine, D., Valorso, A. R., and Cozic, A.: Impact of present and future aircraft NOx and aerosol emissions on atmospheric composition and radiative forcing of climate, Atmos. Chem. Phys., in preparation, 2019.
Teyssèdre, H., Michou, M., Clark, H. L., Josse, B., Karcher, F., Olivié, D., Peuch, V.-H., Saint-Martin, D., Cariolle, D., Attié, J.-L., Nédélec, P., Ricaud, P., Thouret, V., van der A, R. J., Volz-Thomas, A., and Chéroux, F.: A new tropospheric and stratospheric Chemistry and Transport Model MOCAGE-Climat for multi-year studies: evaluation of the present-day climatology and sensitivity to surface processes, Atmos. Chem. Phys., 7, 5815–5860, https://doi.org/10.5194/acp-7-5815-2007, 2007.
Tilmes, S., Lamarque, J.-F., Emmons, L. K., Kinnison, D. E., Ma, P.-L., Liu, X., Ghan, S., Bardeen, C., Arnold, S., Deeter, M., Vitt, F., Ryerson, T., Elkins, J. W., Moore, F., Spackman, J. R., and Val Martin, M.: Description and evaluation of tropospheric chemistry and aerosols in the Community Earth System Model (CESM1.2), Geosci. Model Dev., 8, 1395–1426, https://doi.org/10.5194/gmd-8-1395-2015, 2015.
Tilmes, S., Lamarque, J.-F., Emmons, L. K., Kinnison, D. E., Marsh, D., Garcia, R. R., Smith, A. K., Neely, R. R., Conley, A., Vitt, F., Val Martin, M., Tanimoto, H., Simpson, I., Blake, D. R., and Blake, N.: Representation of the Community Earth System Model (CESM1) CAM4-chem within the Chemistry-Climate Model Initiative (CCMI), Geosci. Model Dev., 9, 1853–1890, https://doi.org/10.5194/gmd-9-1853-2016, 2016.
Turner, A. J., Frankenberg, C., Wennberg, P. O., and Jacob, D. J.: Ambiguity in the causes for decadal trends in atmospheric methane and hydroxyl, P. Natl. Acad. Sci. USA, 114, 5367–5372, https://doi.org/10.1073/pnas.1616020114, 2017.
Turner, A. J., Fung, I., Naik, V., Horowitz, L. W., and Cohen, R. C.: Modulation of hydroxyl variability by ENSO in the absence of external forcing, P. Natl. Acad. Sci. USA, 115, 8931–8936, https://doi.org/10.1073/pnas.1807532115, 2018.
Turner, A. J., Frankenberg, C., and Kort, E. A.: Interpreting contemporary trends in atmospheric methane, P. Natl. Acad. Sci. USA, 116, 2805–2813, 10.1073/pnas.1814297116, 2019.
van der Werf, G. R., Randerson, J. T., Giglio, L., Collatz, G. J., Kasibhatla, P. S., and Arellano Jr., A. F.: Interannual variability in global biomass burning emissions from 1997 to 2004, Atmos. Chem. Phys., 6, 3423–3441, https://doi.org/10.5194/acp-6-3423-2006, 2006.
van der Werf, G. R., Randerson, J. T., Giglio, L., Collatz, G. J., Mu, M., Kasibhatla, P. S., Morton, D. C., DeFries, R. S., Jin, Y., and van Leeuwen, T. T.: Global fire emissions and the contribution of deforestation, savanna, forest, agricultural, and peat fires (1997–2009), Atmos. Chem. Phys., 10, 11707–11735, https://doi.org/10.5194/acp-10-11707-2010, 2010.
Vinken, G. C. M., Boersma, K. F., Maasakkers, J. D., Adon, M., and Martin, R. V.: Worldwide biogenic soil NOx emissions inferred from OMI NO2 observations, Atmos. Chem. Phys., 14, 10363–10381, https://doi.org/10.5194/acp-14-10363-2014, 2014.
Voulgarakis, A., Naik, V., Lamarque, J.-F., Shindell, D. T., Young, P. J., Prather, M. J., Wild, O., Field, R. D., Bergmann, D., Cameron-Smith, P., Cionni, I., Collins, W. J., Dalsøren, S. B., Doherty, R. M., Eyring, V., Faluvegi, G., Folberth, G. A., Horowitz, L. W., Josse, B., MacKenzie, I. A., Nagashima, T., Plummer, D. A., Righi, M., Rumbold, S. T., Stevenson, D. S., Strode, S. A., Sudo, K., Szopa, S., and Zeng, G.: Analysis of present day and future OH and methane lifetime in the ACCMIP simulations, Atmos. Chem. Phys., 13, 2563–2587, https://doi.org/10.5194/acp-13-2563-2013, 2013.
Walters, D. N., Williams, K. D., Boutle, I. A., Bushell, A. C., Edwards, J. M., Field, P. R., Lock, A. P., Morcrette, C. J., Stratton, R. A., Wilkinson, J. M., Willett, M. R., Bellouin, N., Bodas-Salcedo, A., Brooks, M. E., Copsey, D., Earnshaw, P. D., Hardiman, S. C., Harris, C. M., Levine, R. C., MacLachlan, C., Manners, J. C., Martin, G. M., Milton, S. F., Palmer, M. D., Roberts, M. J., Rodríguez, J. M., Tennant, W. J., and Vidale, P. L.: The Met Office Unified Model Global Atmosphere 4.0 and JULES Global Land 4.0 configurations, Geosci. Model Dev., 7, 361–386, https://doi.org/10.5194/gmd-7-361-2014, 2014.
Wang, Y. and Jacob, D. J.: Anthropogenic forcing on tropospheric ozone and OH since preindustrial times, J. Geophys. Res.-Atmos., 103, 31123–31135, https://doi.org/10.1029/1998JD100004, 1998.
World Meteorological Organization: Definition of the tropopause, Bulletin of the World Meteorological Organization, 6, 136–137, 1957.
Yienger, J. J. and Levy, H.: Empirical model of global soil-biogenic NOχ emissions, J. Geophys. Res.-Atmos., 100, 11447–11464, https://doi.org/10.1029/95JD00370, 1995.
Yukimoto, S., Yoshimura, H., Hosaka, M., Sakami, T., Tsujino, H., Hirabara, M., Tanaka, T. Y., Deushi, M., Obata, A., Nakano, H., Adachi, Y., Shindo, E., Yabu, S., Ose, T., and Kitoh, A.: Meteorological Research Institute Earth System Model Version 1 (MRIESM1), 2011.
Yukimoto, S., Adachi, Y., Hosaka, M., Sakami, T., Yoshimura, H., Hirabara, M., Tanaka, T. Y., Shindo, E., Tsujino, H., Deushi, M., Mizuta, R., Yabu, S., Obata, A., Nakano, H., Koshiro, T., Ose, T., and Kitoh, A.: A new global climate model of the Meteorological Research Institute: MRI-CGCM3 – Model description and basic performance, J. Meteorol. Soc. Jpn., 90, 23–64, 2012.
Zimmerman, P. R., Chatfield, R. B., Fishman, J., Crutzen, P. J., and Hanst, P. L.: Estimates on the production of CO and H2 from the oxidation of hydrocarbon emissions from vegetation, Geophys. Res. Lett., 5, 679–682, https://doi.org/10.1029/GL005i008p00679, 1978.
Zimmermann, P. H., Brenninkmeijer, C. A. M., Pozzer, A., Jöckel, P., Zahn, A., Houweling, S., and Lelieveld, J.: Model simulations of atmospheric methane and their evaluation using AGAGE/NOAA surface- and IAGOS-CARIBIC aircraft observations, 1997–2014, Atmos. Chem. Phys. Discuss., https://doi.org/10.5194/acp-2017-1212, in review, 2018.
- Article
(9798 KB) - Full-text XML