the Creative Commons Attribution 4.0 License.
the Creative Commons Attribution 4.0 License.
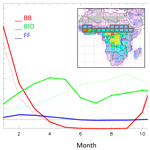
New constraints on biogenic emissions using satellite-based estimates of carbon monoxide fluxes
A. Anthony Bloom
John R. Worden
Zhe Jiang
Eloise A. Marais
Trissevgeni Stavrakou
Benjamin Gaubert
Forrest Lacey
Biogenic non-methane volatile organic compounds (NMVOCs) emitted from vegetation are a primary source for the chemical production of carbon monoxide (CO) in the atmosphere, and these biogenic emissions account for about 18 % of the global CO burden. Partitioning CO fluxes to different source types in top-down inversion methods is challenging; typically a simple scaling of the posterior flux to prior flux values for fossil fuel, biogenic and biomass burning sources is used. Here we show top-down estimates of biogenic CO fluxes using a Bayesian inference approach, which explicitly accounts for both posterior and a priori CO flux uncertainties. This approach re-partitions CO fluxes following inversion of Measurements Of Pollution In The Troposphere (MOPITT) CO observations with the GEOS-Chem model, a global chemical transport model driven by assimilated meteorology from the NASA Goddard Earth Observing System (GEOS). We compare these results to the prior information for CO used to represent biogenic NMVOCs from GEOS-Chem, which uses the Model of Emissions of Gases and Aerosols from Nature (MEGAN) for biogenic emissions. We evaluate the a posteriori biogenic CO fluxes against top-down estimates of isoprene fluxes using Ozone Monitoring Instrument (OMI) formaldehyde observations. We find similar seasonality and spatial consistency in the posterior CO and top-down isoprene estimates globally. For the African savanna region, both top-down CO and isoprene seasonality vary significantly from the MEGAN a priori inventory. This method for estimating biogenic sources of CO will provide an independent constraint on modeled biogenic emissions and has the potential for diagnosing decadal-scale changes in emissions due to land-use change and climate variability.
- Article
(3364 KB) - Full-text XML
-
Supplement
(4711 KB) - BibTeX
- EndNote
Carbon monoxide (CO) plays a critical role in tropospheric chemistry and climate as a precursor to the greenhouse gases ozone (O3) and carbon dioxide (CO2) and through its influence on methane (CH4) lifetime via its destruction by the hydroxyl radical (OH) (e.g., IPCC AR5: IPCC, 2014; Gaubert et al., 2017). CO is formed in the atmosphere from direct emission during incomplete combustion of biomass and fossil fuels and from the oxidation of hydrocarbons. Biogenic non-methane volatile organic compounds (NMVOCs) emitted from vegetation represent a significant source of precursors that oxidize and produce CO, accounting for around 18 % of the global CO budget (e.g., Folberth et al., 2006, Table 8, not including anthropogenic VOCs). Duncan et al. (2007) calculated a contribution of photochemically produced CO from biogenic NMVOC sources contributes about 15 % of total CO sources. Pfister et al. (2008) showed that oxidation from isoprene (C5H8) alone contributes to 9 % to 16 % of the global CO burden, with a global yield of CO from isoprene of 0.30, calculated on a per carbon basis, where CO production is more efficient in polluted environments (i.e., high NOx). Most biogenic NMVOC emissions have relatively short atmospheric lifetimes, typically < 1 h so that transport away from sources is negligible (e.g., Palmer et al., 2003). This allows the estimation of primary NMVOC emissions (e.g., isoprene) using secondary products such as formaldehyde (HCHO), which can be more easily observed with remote sensing (e.g., Palmer et al., 2003; Stavrakou et al., 2009a, b; Marais et al., 2012; Bauwens et al., 2016). Biogenic CO is then produced from HCHO and other NMVOCs through photolysis and reactions with OH, where HCHO lifetime is on the order of hours in tropical daytime (e.g., Miller et al., 2008; Anderson et al., 2017). The chemical production and transport of CO away from sources must be modeled using chemical transport models (CTMs) within an inversion framework. Previous efforts to estimate the amount of atmospheric CO that is produced chemically from biogenic NMVOC emissions have used MOPITT (Measurements of Pollution in The Troposphere) satellite observations as a “top-down” constraint while estimating CO fluxes from different sectors such as fossil fuels, biomass burning and biogenic NMVOCs (Fortems-Cheiney et al., 2011; Hooghiemstra et al., 2011, 2012; Yin et al., 2015; Jiang et al., 2017). These estimates have updated the prior fluxes in these sectors. However, if the prior fluxes relied on inventories with inaccurate assumptions about relative partitioning and seasonal variability, these errors are propagated into the posterior emission estimates.
The Model of Emissions of Gases and Aerosols from Nature (MEGAN; Guenther et al., 2006) and other models of biogenic emissions such as Organizing Carbon and Hydrology in Dynamic EcosystEms (ORCHIDEE; Krinner et al., 2005) have made significant strides in allowing a more accurate representation of these emissions in chemical transport models (CTMs). However, evaluation and testing of these models is challenging due to limited availability of correlative measurements, especially in tropical regions where biogenic emissions are largest. Comparisons of CTMs using MEGAN have been performed with surface and airborne in situ observations of isoprene and other biogenic NMVOCs with reasonable agreement such as in the Southeastern US (e.g., Warneke et al., 2010), but these are only over limited regional scales. Large-scale evaluation of biogenic emission models has relied on satellite observations of HCHO to constrain top-down isoprene emission estimates globally (e.g., Shim et al., 2005; Stavrakou et al., 2009b; Bauwens et al., 2016) and regionally for North America (e.g., Palmer et al., 2003, 2006; Millet et al., 2008), Southeast Asia (Fu et al., 2007), South America (Barkley et al., 2008), Europe (Dufour et al., 2009; Curci et al., 2010) and Africa (Marais et al., 2012, 2014).
The ability to accurately model and predict biogenic emissions has become increasingly important as trade-offs in land use are studied for potential climate change mitigation (e.g., Griscom et al., 2017; Luyssaert et al., 2018). These trade-offs include carbon uptake, albedo changes and the emissions of biogenic VOCs. Since biogenic emissions are precursors to both positive (ozone and methane) and negative (secondary organic aerosols) climate forcers, there is significant uncertainty in their role (e.g., Unger et al., 2014a, b; Scott et al., 2018; Harper et al., 2018; Luyssaert et al., 2018). The results presented here for CO from biogenic NMVOC sources give additional, independent information from global satellite observations that can be used to constrain biogenic emissions in areas that are not well monitored with other measurements.
The basis for estimates of CO flux from biogenic sources is a 15-year inversion analysis (Jiang et al, 2017) that used the adjoint of the GEOS-Chem model (Henze et al., 2007) and MOPITT Version 6J multispectral CO observations (Deeter et al., 2014). This approach used latitude bias-corrected MOPITT data (total CO columns and CO vertical profiles) averaged on the GEOS-Chem 5∘ longitude ×4∘latitude grid to constrain model estimates of monthly CO fluxes in each grid cell from three primary source sectors: anthropogenic fossil fuel and biofuel, biomass burning, and oxidation from biogenic NMVOCs. CO from methane oxidation, ∼28 % of the global CO budget (Folberth et al., 2006), was estimated to be 877 Tg(CO)yr−1 as an aggregated global source. The Model of Emissions of Gases and Aerosols from Nature (MEGAN) version 2.0 (Guenther et al., 2006) was used to formulate the prior CO emissions from biogenic NMVOCs. Biomass burning prior fluxes are from the Global Fire Emission Database (GFED3; van der Werf et al., 2010), and global prior fluxes for fossil fuel are from the Emission Database for Global Atmospheric Research (EDGAR 3.2FT2000; Olivier and Berdowski, 2001) with updated inventories for the Northern Hemisphere described in Jiang et al. (2017).
Model errors in atmospheric transport and chemistry typically propagate into the largest sources of uncertainty when quantifying CO fluxes with satellite observations (Jones et al., 2003; Stavrakou et al., 2006; Kopacz et al., 2010; Jiang et al., 2013; Müller et al., 2018). The impact of these errors is reduced in Jiang et al. (2017) by applying an initial assimilation of MOPITT CO over ocean regions to establish boundary conditions that are consistent with the satellite observations before the adjoint emission estimation over land source regions. This approach accounts for CO chemistry and transport over the ocean and allows continental source regions to be treated more independently (Jiang et al., 2015). To characterize remaining errors due to transport in the CO emission estimates, three different inversions are obtained using MOPITT CO total column, full profile and lower troposphere profile retrievals, and their corresponding averaging kernels (Jiang et al., 2013; Worden et al., 2013). Since CO total column observations have no vertical information, they are less sensitive to convection and local emission sources, but they provide information on advection and chemistry with better measurement precision than profile data. Vertical profiles of CO, especially when restricted to the lower troposphere, contain more information about local sources. However, since these vertical distributions have worse precision, the flux estimates are still impacted by model errors in convection, advection and chemistry. An ensemble covariance of these three inversion results provides an empirical evaluation of the sensitivity in CO fluxes to altitude-dependent constraints and their corresponding corrections in the presence of model transport and chemistry errors (Worden et al., 2017). We find the largest variation in the three emission estimates for CO in India and Indonesia where large sources, strong convection and advection from other regions all contribute significantly. As in the Worden et al. (2017) analysis, we constrain the monthly total CO flux in each 5∘ longitude ×4∘ latitude grid box using the mean and variance from the three inversion estimates described above.
One particular limitation of using the inversion results of Jiang et al. (2017) for biogenic CO fluxes is the use of different meteorological data fields over the 2001–2015 period. Due to availability at the time the inversion analysis was conducted, different versions of the NASA Goddard Earth Observing System (GEOS) assimilated meteorological fields were applied: GEOS-4 (2000–2003), GEOS-5 (2004–2012) and GEOS-FP (2013–2015). Since MEGAN uses the meteorological fields as inputs, the different GEOS versions produce non-negligible discontinuities in the a priori for biogenic CO for these time periods. For this reason, and to overlap with the availability of OMI (Ozone Monitoring Instrument) formaldehyde data for inferring isoprene fluxes, we consider the period from 2005 to 2012 for the analysis presented here.
The re-partitioned CO flux data (Bloom et al., 2019) used for this analysis were originally computed for the Worden et al. (2017) study to provide improved estimates of biomass burning emissions. Monthly, gridded estimates of biogenic (BIO), biomass burning (BB) and fossil fuel (FF) CO fluxes – and their associated uncertainties – were calculated via Bayesian inference, where
p(BIO,BB,FF) and p(BIO, BB, FF|A) are the joint prior and posterior distributions of BIO, BB and FF; A represents the atmospheric CO measurements; p(F) (Eq. 4) and p(F|A) (Eq. 3) are the prior and posterior probability distributions of total CO flux F within each monthly grid box. p(F|A) was empirically approximated using the mean and standard deviation of three different CO inversion estimates of total flux () from Jiang et al. (2017). The re-partitioned distribution (Eq. 1) was sampled using an adaptive Metropolis–Hastings Markov chain Monte Carlo (MCMC) algorithm (Bloom and Williams, 2015; Bloom et al., 2015, 2016), with probability distributions:
while effectively minimizing the cost function:
Prior uncertainties for BB () were estimated using emission factor uncertainties for fire types reported for GFED4 and prior uncertainties of ±50 % assumed for BIO and FF. This choice of uncertainty for the BIO and FF sectors is based on previous experience with error constraints and allows sufficient variability in the sector emissions for testing new probability distributions within each grid cell. While Jiang et al. (2017) also estimated sector contributions by scaling the a priori flux ratios, these estimates account for the full characterization of sectoral uncertainties given both prior and posterior uncertainty estimates. We note that chemical production of CO from methane oxidation (877 Tg(CO) yr−1 from Jiang et al., 2017) is considered a fixed term in the Bayesian attribution due to the longer chemical lifetime of methane and consequent global influence.
Uncertainties are available by grid cell, month and source sector (BB, FF or BIO) and represent the 1σ width of the posterior distributions; these distributions are critically dependent on the a priori uncertainties and therefore subject to change when different a priori distributions and covariances are assumed in the Bayesian attribution approach. Table 1 lists the sources of a priori data and uncertainties and gives average monthly values representative of the individual grid cells used in this study. For the remote tropical regions considered here, FF contributions to total CO fluxes are small and we find the most improvement over prior errors in BIO CO posterior flux uncertainties, especially in months with small or no BB emissions. This can be seen in Fig. 2, where monthly grid box posterior errors were averaged spatially for the region of interest and over years 2005–2012. One of the assumptions in this study is the prior uncertainty in BB, which only considers emission factor uncertainties (Akagi et al., 2011) and does not explicitly account for other factors in BB CO fluxes such as combustion completeness and biomass (fuel) amount (e.g., Bloom et al., 2015). Future work will examine the effects of using a wider range of prior uncertainties that reflect multiple inventories.
Table 1Uncertainties applied in the Bayesian source attribution (Eq. 1). Values are monthly averages for single grid boxes ( longitude × latitude) in the tropical study regions.
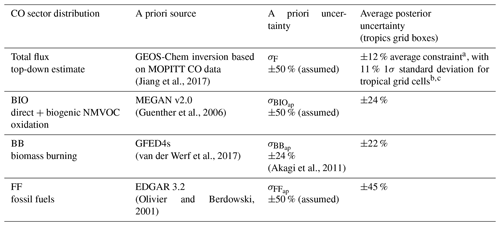
a The total flux posterior error is estimated from three flux inversion types (see text for description) to approximately account for model transport errors. b Average and standard deviation are computed for the tropics (20∘ S to 20∘ N) using grid boxes with emissions > 0.1 gCO m−2 month−1. c The variance in tropical grid cell flux errors includes both spatial and temporal variability; however, these errors have not been weighted to account for sampling effects, such as inflated errors due to fewer MOPITT observations during rainy seasons.
We also note that there is an implicit assumption in the re-partitioning for CO fluxes from biogenic emissions that monthly timescales and relatively large grid box sizes will account for the chemical production of CO from the primary biogenic emissions within the grid box. This assumption relies on the short (< 1 d) chemical lifetime of most biogenic emissions, especially isoprene and formaldehyde, the accuracy of CO chemistry in GEOS-Chem, and the relatively smaller uncertainties for BB and FF fluxes. However, the large grid boxes could also be a source of error in GEOS-Chem chemistry for the inversion results. Kaiser et al. (2018) showed that finer grid scales () and accurate representations of NOx emissions in GEOS-Chem produced top-down isoprene estimates from HCHO observations that compared better to aircraft in situ observations. Furthermore, the GEOS-Chem inversions did not consider chemical non-linearities due to changes in OH caused by changing CO emissions (Gaubert et al., 2016). This has led to an overall increase in OH over the decade 2003–2013 and thus is responsible for an overall increase in secondary CO chemical production (Gaubert et al., 2017). Model intercomparisons and scale sensitivity tests would help quantify the uncertainties from these assumptions.
Since isoprene represents the dominant biogenic NMVOC emission (e.g., Guenther et al., 2006, 2012) and accounts for 66 % of biogenic NMVOC emissions that react to produce CO (Folberth et al., 2006), we compare our estimated CO fluxes from biogenic sources with global estimates of isoprene as a way to check their spatial and temporal variability. Here we use the biogenic isoprene emission estimates provided by the GlobEmission project at http://emissions.aeronomie.be/index.php/omi-based/biogenic. Using OMI satellite observations of tropospheric formaldehyde as a constraint (De Smedt et al., 2015), the GlobEmission estimates of biogenic isoprene emission are produced on a global grid using the adjoint of the IMAGESv2 global chemistry-transport model (Stavrakou et al., 2015; Bauwens et al., 2016) with a priori isoprene emissions from MEGAN–MOHYCAN described in Stavrakou et al. (2014).
Model results for biogenic emissions depend on both static and dynamic input from the CTM and the corresponding meteorology data or reanalysis driving the CTM. Isoprene emissions using MEGAN (Guenther et al., 2006, 2012) are computed as
where Eo is the emission flux under standard conditions, and the γ parameters are dimensionless scaling factors that account for sensitivities to photosynthetically active radiation (PAR), temperature (T), leaf age distribution (AGE), soil moisture (SM) and the canopy radiative environment (CE). The last term includes the effects of leaf area index (LAI) and the plant sensitivity to the above canopy radiance. Values of Eo are specified in MEGAN using a global database of plant functional types (PFT) assuming five PFTs (broadleaf trees, needleleaf trees, grasses, crops and shrubs). The other parameters require dynamic input such as hourly temperature, wind speed, humidity, solar radiation, soil moisture from the meteorological fields used in the CTM, and monthly LAI from the Moderate Resolution Imaging Spectroradiometer (MODIS) on the EOS Terra and EOS Aqua satellites. Figure 1 shows 2005–2012 average biogenic CO and isoprene fluxes for 40∘ S to 40∘ N as estimated with MEGAN and meteorological data (GEOS-5 for CO and ECMWF for isoprene) as compared to estimated fluxes using top-down constraints from satellite observations (MOPITT for CO and OMI HCHO for isoprene).
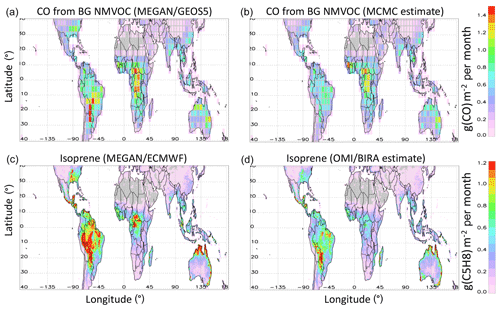
Figure 12005–2012 average biogenic flux for CO (a, b) and isoprene (c, d) with model estimates using MEGAN on the left and top-down estimates using MOPITT observations for CO (b) and isoprene inferred from OMI HCHO observations (d).
We find that the distributions for biogenic CO follow similar spatial patterns as the isoprene fluxes (albeit coarser spatial resolution) and that the top-down estimates are in general lower than the emissions predicted using MEGAN, as found in previous studies (e.g., Millet et al., 2008; Stavrakou et al., 2009a; Marais et al., 2014).
Table 2 shows the annual average fluxes of CO and C5H8 for the 2005–2012 period for 80∘ S to 80∘ N, northern midlatitudes (20 to 40∘ N), the tropics (20∘ S to 20∘ N), and the separated tropical regions of South America, Africa, and the Maritime Continent. Our global estimate for BIO CO from non-methane sources (566±49 Tg(CO) yr−1) is in agreement with a previous estimate (546 Tg(CO) yr−1, Folberth et al., 2006) which was obtained by adding the contributions to CO from isoprene (359 Tg(CO) yr−1), methanol (110 Tg(CO) yr−1), terpenes (49 Tg(CO) yr−1) and acetone (28 Tg(CO) yr−1). This contribution from BIO CO represents a larger percentage (∼41 %) of the sum of BB, FF and BIO CO sources than expected (∼27 %) based on Folberth et al. (2006), which has 811 Tg(CO) yr−1 for BB and 672 Tg(CO) yr−1 for FF. However, there is a wide range in reported biomass burning emission estimates, with large interannual variability. Stavrakou et al. (2006) used 467 Tg(CO) for the year 2000 as the BB CO a priori from GFEDv1; van der Werf et al. (2017) reported 357 Tg yr−1 mean emissions for BB CO over 1997–2016 while Granier et al. (2011) reported a range of 414 to 509 Tg yr−1 for six emission inventories in the 1997–2000 period. Because our 2005–2012 study period did not include the significant El Niño–Southern Oscillation (ENSO) episodes in 1997 and 2015, we would expect lower average values for BB CO emissions than these other annual averages. Furthermore, in recent decades, there is a decreasing contribution of BB CO associated with a decline in tropical fires (e.g., Andela et al., 2017), as well as declining FF CO emissions (Yin et al., 2015; Strode et al., 2016; Jiang et al., 2017; Zheng et al., 2018).
Figure 2 shows the seasonal behavior of posterior sectoral CO flux estimates in the North African savannas (see outlined grid cells of Fig. 2 inset map) derived by the Bayesian attribution approach described in Sect. 3. While biomass burning (BB) dominates in the northern hemispheric winter, and fossil fuel fluxes (FF) have little variability, biogenic fluxes show two broad maxima: one in April and the other in October. We note that these maxima are not likely mis-identified BB fluxes as the BB months are relatively well defined in the region for November to February.
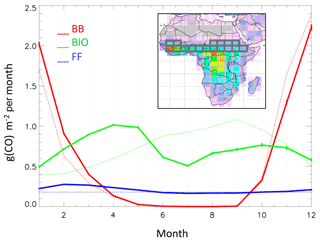
Figure 2A posteriori (solid lines) and a priori (dotted lines) CO fluxes averaged for each month over 2005–2012 for the North African savannas region for biomass burning (BB, red), biogenic (BIO, green) and fossil fuel (FF, blue) sectors. The inset map shows average BIO CO fluxes over Africa, with the same color scale as shown in the top panels of Fig. 1. The North African savannas grid boxes considered for the monthly averages are outlined in gray. Errors on the 8-year average fluxes for this region are indicated for each sector and month, with values around 2.3 % for BIO, 1.6 % for BB and 3.4 % for FF.
Figure 3 shows the time series of a priori (MEGAN with GEOS-5) vs. posterior for the North African savannas region, with surface temperature from the Modern-Era Retrospective analysis for Research and Applications (MERRA; Rienecker et al., 2011) overplotted to show the correspondence of the posterior results with temperature variability. We note that using GEOS-FP for the 2013–2015 meteorology (not shown) results in a ∼27 % increase for the peak a priori (MEGAN with GEOS-FP) biogenic CO fluxes compared to the years using MEGAN with GEOS-5.
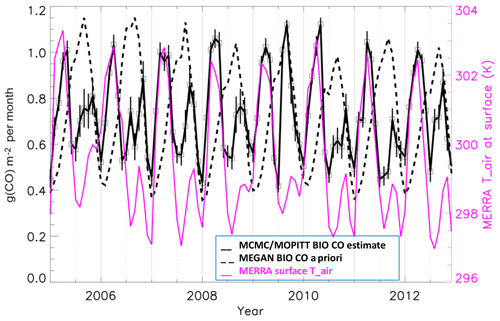
Figure 3Time series of a priori (dashed black) and posterior (solid black) CO fluxes with monthly mean 1σ errors and MERRA surface air temperature (magenta) for the North African savannas region (see inset map in Fig. 2).
As shown by Marais et al. (2014), the seasonality of isoprene fluxes in the African savannas north of the Equator also has a maximum in April followed by a minimum during the rainy season, June to September (Janicot et al., 2008). Fluxes for December to March were not estimated in Marais et al. (2014) due to interference with biomass burning emissions and secondary formation HCHO. The top-down isoprene estimates from Bauwens et al. (2016) and the CO flux estimates from the Bayesian attribution approach described here both show two minima in biogenic emissions for this region – one in the rainy season (June–August) and the other in winter (December–January) – similar to the surface temperature (Fig. 4). Marais et al. (2014) attribute the higher emissions from MEGAN to the model dependence on LAI, which has a broad maximum in August.
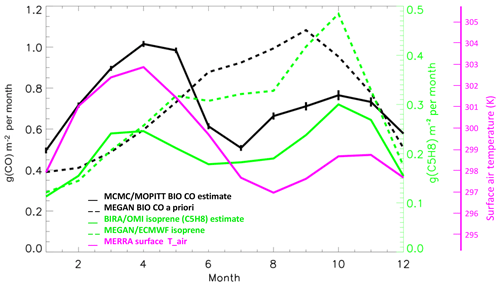
Figure 4Average monthly CO (black) and C5H8 (green) fluxes and surface air temperatures (magenta) for 2005–2012 for the North African savannas region (see inset map in Fig. 2). Solid black and green lines show the posterior “top-down” fluxes while dashed black and green lines show the emissions predicted by MEGAN with associated meteorological fields.
Other regions in South America, southern Africa and Australia that were tested for seasonality of BIO CO fluxes (see Supplement) did not show the same large inconsistency with MEGAN, suggesting that the North African savannas require special treatment and a revised parametrization within MEGAN to account for the enhanced sensitivity to surface temperature vs. LAI during the rainy season. The tabulated emissions under standard conditions, Eo, could also require revision to account for human-driven changes in plant types due to cropland expansion in the North African savannas region in recent decades (e.g., Andela et al., 2014).
This paper has presented the first results for estimates of CO from biogenic NMVOCs using a Bayesian re-partitioning of top-down flux estimates. We find that the CO flux estimates based on MOPITT CO observations are spatially consistent with biogenic isoprene flux estimates based on OMI HCHO observations. Both top-down estimates for carbon monoxide and isoprene suggest that biogenic emissions based on MEGAN are too high in the tropics by 28 % for isoprene and 10 % for carbon monoxide with the largest discrepancies in South America. As a case study in tropical North Africa, we found that the top-down estimates suggest a significant seasonality change compared to MEGAN for both CO and C5H8. The top-down estimates have seasonal cycles that match well with MERRA surface temperature and that have secondary minima during the rainy season that are not predicted well by MEGAN. These discrepancies suggest the potential for regional updates to the MEGAN model, a focus of future work. Sensitivity to model grid scales that affect transport and chemistry uncertainties will also be investigated.
In order to examine climate variability and possible trends in biogenic emissions, the methods described here will also be applied to a flux inversion estimate using a consistent meteorological reanalysis. Since MOPITT will soon have a 20-year data record, it will span several ENSO cycles and will have the potential for detecting the effects of inter-annual and long-term changes in surface temperatures on biogenic CO flux variability.
NASA/MOPITT data sets used for the CO inverse modeling component (Jiang et al., 2017) are publicly available at https://eosweb.larc.nasa.gov/datapool (Deeter et al., 2014). The isoprene emission estimates obtained from inverse modeling of OMI HCHO observations are available from the GlobEmission project at http://emissions.aeronomie.be/index.php/omi-based/biogenic (Bauwens et al., 2016). The partitioned sector CO flux estimates are available at https://dashrepo.ucar.edu/dataset/CO_Flux_Inversion_Attribution.html, which can be cited with https://doi.org/10.26024/r1r2-6620 (Bloom et al., 2019).
The supplement related to this article is available online at: https://doi.org/10.5194/acp-19-13569-2019-supplement.
HMW performed the analysis of flux estimates. AAB performed the Bayesian flux attribution. JRW and HMW contributed to the design and use of MOPITT data for the CO inverse modeling and flux attribution. ZJ performed the CO inverse modeling. EM assisted with interpretation of African results. TS provided guidance on the use of the isoprene estimates. FL and BG assisted with interpretation of global results. HMW, AAB and JRW prepared the original manuscript, and all authors contributed to the review and editing of the manuscript.
The authors declare that they have no conflict of interest.
The authors thank Rebecca Schwantes and Ivan Ortega Martinez at NCAR for their helpful review and comments. Eloise A. Marais acknowledges funding from NERC/EPSRC (grant no. EP/R513465/1). The MOPITT team acknowledges the contributions of COMDEV and ABB BOMEM with support from the Canadian Space Agency (CSA), the Natural Sciences and Engineering Research Council (NSERC), and Environment Canada. The NCAR MOPITT project is supported by the National Aeronautics and Space Administration (NASA) Earth Observing System (EOS) program. The National Center for Atmospheric Research (NCAR) is sponsored by the National Science Foundation. Part of this research was carried out at the Jet Propulsion Laboratory, California Institute of Technology, under a contract with the National Aeronautics and Space Administration.
This research has been supported by the NASA (grant no. 80GSFC19C0032) and the NERC/EPSRC (grant no. EP/R513465/1).
This paper was edited by Gabriele Stiller and reviewed by two anonymous referees.
Akagi, S. K., Yokelson, R. J., Wiedinmyer, C., Alvarado, M. J., Reid, J. S., Karl, T., Crounse, J. D., and Wennberg, P. O.: Emission factors for open and domestic biomass burning for use in atmospheric models, Atmos. Chem. Phys., 11, 4039–4072, https://doi.org/10.5194/acp-11-4039-2011, 2011.
Andela, N. and van der Werf, G.: Recent trends in African fires driven by cropland expansion and El Niño to La Niña transition, Nat. Clim. Change 4, 791–795, https://doi.org/10.1038/nclimate2313, 2014.
Andela, N., Morton, D. C., Giglio, L., Chen, Y., van der Werf, G. R., Kasibhatla, P. S., DeFries, R. S., Collatz, G. J., Hantson, S., Kloster, S., Bachelet, D., Forrest, M., Lasslop, G., Li, F., Mangeon, S., Melton, J. R., Yue, C., and Randerson, J. T.: A human-driven decline in global burned area, Science, 356, 1356–1362, 2017.
Anderson, D. C., Nicely, J. M., Wolfe, G. M., Hanisco, T. F., Salawitch, R. J., Canty, T. P., Dickerson, R. R., Apel, E. C., Baidar, S., Bannan, T. J., Blake, N. J., Chen D., Dix, B., Fernandez, R. P., Hall, S. R., Hornbrook, R. S., Huey, L. G., Josse, B., Jöckel, P., Kinnison, D. E., Koenig, T. K., Le Breton, M. Marécal, V., Morgenstern, O., Oman, L. D., Pan, L. L., Percival, C., Plummer, D., Revell, L. E., Rozanov, E., Saiz-Lopez, A., Stenke, A., Sudo, K., Tilmes., S., Ullmann, K., Volkamer, R., Weinheimer, A. J., and Zeng, G.: Formaldehyde in the tropical western Pacific: Chemical sources and sinks, convective transport, and representation in CAM-Chem and the CCMI models, J. Geophys. Res.-Atmos., 122, 11201–11226, https://doi.org/10.1002/2016JD026121, 2017.
Barkley, M. P., Palmer, P. I., Kuhn, U., Kesselmeier, J., Chance, K., Kurosu, T. P., Martin, R. V., Helmig, D., and Guenther, A.: Net ecosystem fluxes of isoprene over tropical South America inferred from Global Ozone Monitoring Experiment (GOME) observations of HCHO columns, J. Geophys. Res.-Atmos., 113, D20304, https://doi.org/10.1029/2008JD009863, 2008.
Bauwens, M., Stavrakou, T., Müller, J.-F., De Smedt, I., Van Roozendael, M., van der Werf, G. R., Wiedinmyer, C., Kaiser, J. W., Sindelarova, K., and Guenther, A.: Nine years of global hydrocarbon emissions based on source inversion of OMI formaldehyde observations, Atmos. Chem. Phys., 16, 10133–10158, https://doi.org/10.5194/acp-16-10133-2016, 2016 (data available at: http://emissions.aeronomie.be/index.php/omi-based/biogenic, last access: 4 November 2019).
Bloom, A. A. and Williams, M.: Constraining ecosystem carbon dynamics in a data-limited world: integrating ecological “common sense” in a model–data fusion framework, Biogeosciences, 12, 1299–1315, https://doi.org/10.5194/bg-12-1299-2015, 2015.
Bloom, A. A., Worden, J., Jiang, Z., Worden, H., Kurosu, T., Frankenberg, C., and Schimel, D.: Remote sensing constraints on South America fire traits by Bayesian fusion of atmospheric and surface data, Geophys. Res. Lett., 42, 1268–1274, https://doi.org/10.1002/2014GL062584, 2015.
Bloom, A. A., Exbrayat, J.-F., van der Velde, I. R., Feng, L., and Williams, M.: The decadal state of the terrestrial carbon cycle: global retrievals of terrestrial carbon allocation, pools, and residence times, P. Natl Acad. Sci. USA, 113, 1285–1290, https://doi.org/10.1073/pnas.1515160113, 2016.
Bloom, A. A., Jiang, Z., and Worden, H.: Global Carbon Monoxide (CO) Flux Estimates for 2001–2015, UCAR/NCAR – DASH Repository, https://doi.org/10.26024/r1r2-6620, 2019.
Curci, G., Palmer, P. I., Kurosu, T. P., Chance, K., and Visconti, G.: Estimating European volatile organic compound emissions using satellite observations of formaldehyde from the Ozone Monitoring Instrument, Atmos. Chem. Phys., 10, 11501–11517, https://doi.org/10.5194/acp-10-11501-2010, 2010.
Deeter, M. N., Martínez-Alonso, S., Edwards, D. P., Emmons, L. K., Gille, J. C., Worden, H. M., Sweeney, C., Pittman, J. V., Daube, B. C., and Wofsy, S. C.: The MOPITT Version 6 product: algorithm enhancements and validation, Atmos. Meas. Tech., 7, 3623–3632, https://doi.org/10.5194/amt-7-3623-2014, 2014 (data available at: https://eosweb.larc.nasa.gov/datapool, last access: 22 October 2019).
De Smedt, I., Stavrakou, T., Hendrick, F., Danckaert, T., Vlemmix, T., Pinardi, G., Theys, N., Lerot, C., Gielen, C., Vigouroux, C., Hermans, C., Fayt, C., Veefkind, P., Müller, J.-F., and Van Roozendael, M.: Diurnal, seasonal and long-term variations of global formaldehyde columns inferred from combined OMI and GOME-2 observations, Atmos. Chem. Phys., 15, 12519–12545, https://doi.org/10.5194/acp-15-12519-2015, 2015.
Dufour, G., Wittrock, F., Camredon, M., Beekmann, M., Richter, A., Aumont, B., and Burrows, J. P.: SCIAMACHY formaldehyde observations: constraint for isoprene emission estimates over Europe?, Atmos. Chem. Phys., 9, 1647–1664, https://doi.org/10.5194/acp-9-1647-2009, 2009.
Duncan, B. N., Logan, J. A., Bey, I., Megretskaia, I. A., Yantosca, R. M., Novelli, P. C., Jones, N. B., and Rinsland, C. P.: Global budget of CO, 1988–1997: Source estimates and validation with a global model, J. Geophys. Res., 112, D22301, https://doi.org/10.1029/2007JD008459, 2007.
Folberth, G. A., Hauglustaine, D. A., Lathière, J., and Brocheton, F.: Interactive chemistry in the Laboratoire de Météorologie Dynamique general circulation model: model description and impact analysis of biogenic hydrocarbons on tropospheric chemistry, Atmos. Chem. Phys., 6, 2273–2319, https://doi.org/10.5194/acp-6-2273-2006, 2006.
Fortems-Cheiney, A., Chevallier, F., Pison, I., Bousquet, P., Szopa, S., Deeter, M. N., and Clerbaux, C.: Ten years of CO emissions as seen from Measurements of Pollution in the Troposphere (MOPITT), J. Geophys. Res.-Atmos., 116, 5304, https://doi.org/10.1029/2010JD014416, 2011.
Fu, T.-M., Jacob, D. J., Palmer, P. I., Chance, K., Wang, Y. X., Barletta, B., Blake, D. R., Stanton, J. C., and Pilling, M. J.: Space-based formaldehyde measurements as constraints on volatile organic compound emissions in east and south Asia and implications for ozone, J. Geophys. Res., 112, D06312, https://doi.org/10.1029/2006JD007853, 2007.
Gaubert, B., Arellano, A. F., Barré, J., Worden, H. M., Emmons, L. K., Tilmes, S., Buchholz, R. R., Vitt, F., Raeder, K., Collins, N., and Anderson, J. L.: Toward a chemical reanalysis in a coupled chemistry-climate model: An evaluation of MOPITT CO assimilation and its impact on tropospheric composition, J. Geophys. Res.-Atmos., 121, 7310–7343, 2016.
Gaubert, B., Worden, H. M., Arellano, A. F. J., Emmons, L. K., Tilmes, S., Barre, J., Alonso, S. M., Vitt, F., Anderson, J. L., Alkemade, F., Houweling, S., and Edwards, D. P.: Chemical Feedback From Decreasing Carbon Monoxide Emissions, Geophys. Res. Lett., 44, 9985–9995, https://doi.org/10.1002/2017GL074987, 2017.
Granier, C., Bessagnet, B., Bond, T., D'Angiola, A., van der Gon, H. D., Frost, G. J., Heil, A., Kaiser, J. W., and Kinne, S., Klimont, Z., Lamarque, J.-F., Liousse, C, Masui, T., Meleux, F., Mieville, A., Ohara, T., Raut, J.-C., Riahi, K., Schultz, M. G., Smith, S. J., Thompson, A., van Aardenne, J., van der Werf, G., and van Vuuren, D. P.: Evolution of anthropogenic and biomass burning emissions of air pollutants at global and regional scales during the 1980–2010 period, Climatic Change, 109, 163–190, https://doi.org/10.1007/s10584-011-0154-1, 2011.
Griscom, B. W., Adams, J., Ellis, P. W., Houghton, R. A., Lomax, G., Miteva, D. A., Schlesinger, W. H., Shoch, D., Siikamäki, J. V., Smith, P., Woodbury, P., Zganjar, C., Blackman, A., Campari, J., Conant, R. T., Delgado, C., Elias, P., Gopalakrishna, T., Hamsik, M. R., Herrero, M., Kiesecker, J., Landis, E., Laestadius, L., Leavitt, S. M., Minnemeyer, S., Polasky, S., Potapov, P., Putz, F. E., Sanderman, J., Silvius, M., Wollenberg, E., and Fargione, J.: Natural climate solutions, P. Natl. Acad. Sci. USA, 114, 11645–11650, https://doi.org/10.1073/pnas.1710465114, 2017.
Guenther, A., Karl, T., Harley, P., Wiedinmyer, C., Palmer, P. I., and Geron, C.: Estimates of global terrestrial isoprene emissions using MEGAN (Model of Emissions of Gases and Aerosols from Nature), Atmos. Chem. Phys., 6, 3181–3210, https://doi.org/10.5194/acp-6-3181-2006, 2006.
Guenther, A. B., Jiang, X., Heald, C. L., Sakulyanontvittaya, T., Duhl, T., Emmons, L. K., and Wang, X.: The Model of Emissions of Gases and Aerosols from Nature version 2.1 (MEGAN2.1): an extended and updated framework for modeling biogenic emissions, Geosci. Model Dev., 5, 1471–1492, https://doi.org/10.5194/gmd-5-1471-2012, 2012.
Harper, K. L. and Unger, N.: Global climate forcing driven by altered BVOC fluxes from 1990 to 2010 land cover change in maritime Southeast Asia, Atmos. Chem. Phys., 18, 16931–16952, https://doi.org/10.5194/acp-18-16931-2018, 2018.
Henze, D. K., Hakami, A., and Seinfeld, J. H.: Development of the adjoint of GEOS-Chem, Atmos. Chem. Phys., 7, 2413–2433, https://doi.org/10.5194/acp-7-2413-2007, 2007.
IPCC, 2014: Climate Change 2014: Synthesis Report, Contribution of Working Groups I, II and III to the Fifth Assessment Report of the Intergovernmental Panel on Climate Change, edited by: Core Writing Team, Pachauri, R. K. and Meyer, L. A., IPCC, Geneva, Switzerland, 151 pp., available at: https://www.ipcc.ch/report/ar5/syr/, last access: 22 October 2019, 2014.
Hooghiemstra, P. B., Krol, M. C., Meirink, J. F., Bergamaschi, P., van der Werf, G. R., Novelli, P. C., Aben, I., and Röckmann, T.: Optimizing global CO emission estimates using a four-dimensional variational data assimilation system and surface network observations, Atmos. Chem. Phys., 11, 4705–4723, https://doi.org/10.5194/acp-11-4705-2011, 2011.
Hooghiemstra, P. B., Krol, M. C., Bergamaschi, P., de Laat, A. T. J., van der Werf, G. R., Novelli, P. C., Deeter, M. N., Aben, I., and Röckmann, T.: Comparing optimized CO emission estimates using MOPITT or NOAA surface network observations, J. Geophys. Res., 117, D06309, https://doi.org/10.1029/2011JD017043, 2012.
Janicot, S., Thorncroft, C. D., Ali, A., Asencio, N., Berry, G., Bock, O., Bourles, B., Caniaux, G., Chauvin, F., Deme, A., Kergoat, L., Lafore, J.-P., Lavaysse, C., Lebel, T., Marticorena, B., Mounier, F., Nedelec, P., Redelsperger, J.-L., Ravegnani, F., Reeves, C. E., Roca, R., de Rosnay, P., Schlager, H., Sultan, B., Tomasini, M., Ulanovsky, A., and ACMAD forecasters team: Large-scale overview of the summer monsoon over West Africa during the AMMA field experiment in 2006, Ann. Geophys., 26, 2569–2595, https://doi.org/10.5194/angeo-26-2569-2008, 2008.
Jiang, Z., Jones, D. B. A., Worden, H. M., Deeter, M. N., Henze, D. K., Worden, J., Bowman, K. W., Brenninkmeijer, C. A. M., and Schuck, T. J.: Impact of model errors in convective transport on CO source estimates inferred from MOPITT CO retrievals, J. Geophys. Res.-Atmos., 118, 2073–2083, 2013.
Jiang, Z., Jones, D. B. A., Worden, J., Worden, H. M., Henze, D. K., and Wang, Y. X.: Regional data assimilation of multi-spectral MOPITT observations of CO over North America, Atmos. Chem. Phys., 15, 6801–6814, https://doi.org/10.5194/acp-15-6801-2015, 2015.
Jiang, Z., Worden, J. R., Worden, H., Deeter, M., Jones, D. B. A., Arellano, A. F., and Henze, D. K.: A 15-year record of CO emissions constrained by MOPITT CO observations, Atmos. Chem. Phys., 17, 4565–4583, https://doi.org/10.5194/acp-17-4565-2017, 2017.
Jones, D. B. A., Bowman, K. W., Palmer, P. I., Worden, J. R., Jacob, D. J., Hoffman, R. N., Bey, I., and Yantosca, R. M.: Potential of observations from the tropospheric emission spectrometer to constrain continental sources of carbon monoxide, J. Geophys. Res., 108, 4789, https://doi.org/10.1029/2003JD003702, 2003.
Kaiser, J., Jacob, D. J., Zhu, L., Travis, K. R., Fisher, J. A., González Abad, G., Zhang, L., Zhang, X., Fried, A., Crounse, J. D., St. Clair, J. M., and Wisthaler, A.: High-resolution inversion of OMI formaldehyde columns to quantify isoprene emission on ecosystem-relevant scales: application to the southeast US, Atmos. Chem. Phys., 18, 5483–5497, https://doi.org/10.5194/acp-18-5483-2018, 2018.
Kopacz, M., Jacob, D. J., Fisher, J. A., Logan, J. A., Zhang, L., Megretskaia, I. A., Yantosca, R. M., Singh, K., Henze, D. K., Burrows, J. P., Buchwitz, M., Khlystova, I., McMillan, W. W., Gille, J. C., Edwards, D. P., Eldering, A., Thouret, V., and Nedelec, P.: Global estimates of CO sources with high resolution by adjoint inversion of multiple satellite datasets (MOPITT, AIRS, SCIAMACHY, TES), Atmos. Chem. Phys., 10, 855–876, https://doi.org/10.5194/acp-10-855-2010, 2010.
Krinner, G., Viovy, N., de Noblet-Ducoudré, N., Ogee, J., Polcher, J., Friedlingstein, P., Ciais, P., Sitch, A., and Prentice, I.: A dynamical global vegetation model for studies of the coupled atmosphere-biosphere system, Global Biogeochem. Cy., 19, GB1015, https://doi.org/10.1029/2003GB002199, 2005.
Luyssaert, S., Marie, G., Valade, A., Chen, Y. Y., Njakou Djomo, S., Ryder, J. Otto, J., Naudts, K., Lanso, A. S., Ghattas, J., and McGrath, M. J.: Trade-offs in using European forests to meet climate objectives, Nature, 562, 259–262, https://doi.org/10.1038/s41586-018-0577-1, 2018.
Marais, E. A., Jacob, D. J., Kurosu, T. P., Chance, K., Murphy, J. G., Reeves, C., Mills, G., Casadio, S., Millet, D. B., Barkley, M. P., Paulot, F., and Mao, J.: Isoprene emissions in Africa inferred from OMI observations of formaldehyde columns, Atmos. Chem. Phys., 12, 6219–6235, https://doi.org/10.5194/acp-12-6219-2012, 2012.
Marais, E. A., Jacob, D. J., Guenther, A., Chance, K., Kurosu, T. P., Murphy, J. G., Reeves, C. E., and Pye, H. O. T.: Improved model of isoprene emissions in Africa using Ozone Monitoring Instrument (OMI) satellite observations of formaldehyde: implications for oxidants and particulate matter, Atmos. Chem. Phys., 14, 7693–7703, https://doi.org/10.5194/acp-14-7693-2014, 2014.
Miller, S. M., Matross, D. M., Andrews, A. E., Millet, D. B., Longo, M., Gottlieb, E. W., Hirsch, A. I., Gerbig, C., Lin, J. C., Daube, B. C., Hudman, R. C., Dias, P. L. S., Chow, V. Y., and Wofsy, S. C.: Sources of carbon monoxide and formaldehyde in North America determined from high-resolution atmospheric data, Atmos. Chem. Phys., 8, 7673–7696, https://doi.org/10.5194/acp-8-7673-2008, 2008.
Millet, D. B., Jacob, D. J., Boersma, K. F., Fu, T.-M., Kurosu, T. P., Chance, K., Heald, C. L., and Guenther, A.: Spatial distribution of isoprene emissions from North America derived from formaldehyde column measurements by the OMI satellite sensor, J. Geophys. Res., 113, D02307, https://doi.org/10.1029/2007JD008950, 2008.
Müller, J.‐F., Stavrakou, T., Bauwens, M., George, M., Hurtmans, D., Coheur, P.‐F., Clerbaux, C., and Sweeney, C.: Top‐down CO emissions based on IASI observations and hemispheric constraints on OH levels, Geophys. Res. Lett., 45, 1621–1629, https://doi.org/10.1002/2017GL076697, 2018.
Olivier, J. G. J. and Berdowski, J. J. M.: Global emissions sources and sinks, in: The Climate System, edited by: Berdowski, J., Guicherit, R., and Heij, B. J., A. A. Balkema Publishers/Swets & Zeitlinger Publishers, Lisse, the Netherlands, 33–78, 2001.
Palmer, P. I., Jacob, D. J., Fiore, A. M., Martin, R. V., Chance, K., and Kurosu, T. P.: Mapping isoprene emissions over North America using formaldehyde column observations from space, J. Geophys. Res., 108, 4180, https://doi.org/10.1029/2002JD002153, 2003.
Palmer, P. I., Abbot, D. S., Fu, T.-M., Jacob, D. J., Chance, K., Kurosu, T. P., Guenther, A., Wiedinmyer, C., Stanton, J. C., Pilling, M. J., Pressley, S. N., Lamb, B., and Sumner, A. L.: Quantifying the seasonal and interannual variability of North American isoprene emissions using satellite observations of the formaldehyde column, J. Geophys. Res., 111, D12315, https://doi.org/10.1029/2005JD006689, 2006.
Pfister, G. G., Emmons, L. K., Hess, P. G., Lamarque, J.-F., Orlando, J. J., Walters, S., Guenther, A., Palmer, P. I., and Lawrence, P. J.: Contribution of isoprene to chemical budgets: A model tracer study with the NCAR CTM MOZART-4, J. Geophys. Res., 113, D05308, https://doi.org/10.1029/2007JD008948, 2008.
Rienecker, M. M., Suarez, M. J., Gelaro, R., Todling, R., Bacmeister, J., Liu, E., Bosilovich, M. G., Schubert, S. D., Takacs, L., Kim, G., Bloom, S., Chen, J., Collins, D., Conaty, A., da Silva, A., Gu, W., Joiner, J., Koster, R. D., Lucchesi, R., Molod, A., Owens, T., Pawson, S., Pegion, P., Redder, C. R., Reichle, R., Robertson, F. R., Ruddick, A. G., Sienkiewicz, M., and Woollen, J.: MERRA: NASA's Modern-Era Retrospective Analysis for Research and Applications, J. Climate, 24, 3624–3648, https://doi.org/10.1175/JCLI-D-11-00015.1, 2011
Scott, C. E., Monks, S. A., Spracklen, D. V., Arnold, S. R., Forster, P. M., Rap, A., Äijälä, M., Artaxo, P., Carslaw, K. S., Chipperfield, M. P., Ehn, M., Gilardoni, S., Heikkinen, L., Kulmala, M., Petäjä, T., Reddington, C. L. S., Rizzo, L. V., Swietlicki, E., Vignati, E., and Wilson, C.: Impact on short-lived climate forcers increases projected warming due to deforestation, Nat. Commun., 9, 1–9, https://doi.org/10.1038/s41467-017-02412-4, 2018.
Shim, C., Wang, Y., Choi, Y., Palmer, P. I., Abbot, D. S., and Chance, K.: Constraining global isoprene emissions with Global Ozone Monitoring Experiment (GOME) formalde- hyde column measurements, J. Geophys. Res., 110, D24301, https://doi.org/10.1029/2004JD005629, 2005.
Stavrakou, T. and Müller, J.-F.: Grid-based vs. big-region approach for inverting CO emissions using Measurement of Pollution in the Troposphere (MOPITT) data, J. Geophys. Res., 111, D15304, https://doi.org/10.1029/2005JD006896, 2006.
Stavrakou, T., Müller, J.-F., De Smedt, I., Van Roozendael, M., van der Werf, G. R., Giglio, L., and Guenther, A.: Evaluating the performance of pyrogenic and biogenic emission inventories against one decade of space-based formaldehyde columns, Atmos. Chem. Phys., 9, 1037–1060, https://doi.org/10.5194/acp-9-1037-2009, 2009a.
Stavrakou, T., Müller, J.-F., De Smedt, I., Van Roozendael, M., van der Werf, G. R., Giglio, L., and Guenther, A.: Global emissions of non-methane hydrocarbons deduced from SCIAMACHY formaldehyde columns through 2003–2006, Atmos. Chem. Phys., 9, 3663–3679, https://doi.org/10.5194/acp-9-3663-2009, 2009b.
Stavrakou, T., Müller, J.-F., Bauwens, M., De Smedt, I., Van Roozendael, M., Guenther, A., Wild, M., and Xia, X.: Isoprene emissions over Asia 1979–2012: impact of climate and land-use changes, Atmos. Chem. Phys., 14, 4587–4605, https://doi.org/10.5194/acp-14-4587-2014, 2014.
Stavrakou, T., Müller, J.-F., Bauwens, M., De Smedt, I., Van Roozendael, M., De Mazière, M., Vigouroux, C., Hendrick, F., George, M., Clerbaux, C., Coheur, P.-F., and Guenther, A.: How consistent are top-down hydrocarbon emissions based on formaldehyde observations from GOME-2 and OMI?, Atmos. Chem. Phys., 15, 11861–11884, https://doi.org/10.5194/acp-15-11861-2015, 2015.
Strode, S. A., Worden, H. M., Damon, M., Douglass, A. R., Duncan, B. N., Emmons, L. K., Lamarque, J.-F., Manyin, M., Oman, L. D., Rodriguez, J. M., Strahan, S. E., and Tilmes, S.: Interpreting space-based trends in carbon monoxide with multiple models, Atmos. Chem. Phys., 16, 7285–7294, https://doi.org/10.5194/acp-16-7285-2016, 2016.
Unger, N.: Human land-use-driven reduction of forest volatiles cools global climate, Nat. Clim. Change, 4, 907–910, https://doi.org/10.1038/nclimate2347, 2014a.
Unger, N.: On the role of plant volatiles in anthropogenic global climate change, Geophys. Res. Lett., 41, 8563–8569, https://doi.org/10.1002/2014GL061616, 2014b.
van der Werf, G. R., Randerson, J. T., Giglio, L., Collatz, G. J., Mu, M., Kasibhatla, P. S., Morton, D. C., DeFries, R. S., Jin, Y., and van Leeuwen, T. T.: Global fire emissions and the contribution of deforestation, savanna, forest, agricultural, and peat fires (1997–2009), Atmos. Chem. Phys., 10, 11707–11735, https://doi.org/10.5194/acp-10-11707-2010, 2010.
van der Werf, G. R., Randerson, J. T., Giglio, L., van Leeuwen, T. T., Chen, Y., Rogers, B. M., Mu, M., van Marle, M. J. E., Morton, D. C., Collatz, G. J., Yokelson, R. J., and Kasibhatla, P. S.: Global fire emissions estimates during 1997–2016, Earth Syst. Sci. Data, 9, 697–720, https://doi.org/10.5194/essd-9-697-2017, 2017.
Warneke, C., de Gouw, J. A., Del Negro, L., Brioude, J., McKeen, S., Stark, H., Kuster, W. C., Goldan, P. D., Trainer, M., Fehsenfeld, F. C., Wiedinmyer, C., Guenther, A. B., Hansel, A., Wisthaler, A., Atlas, E., Holloway, J. S., Ryerson, T. B., Peischl, J., Huey, L. G., and Case Hanks, A. T.: Biogenic emission measurement and inventories determination of biogenic emissions in the eastern United States and Texas and comparison with biogenic emission inventories, J. Geophys. Res., 115, D00F18, https://doi.org/10.1029/2009JD012445, 2010.
Worden, J., Jiang, Z., Jones, D. B. A., Alvarado, M., Bowman K., Frankenberg, C., Kort, E. A., Kulawik, S. S., Lee, M., Liu, J., Payne, V., Wecht, K., and Worden, H.: El Niño, the 2006 Indonesian peat fires, and the distribution of atmospheric methane, Geophys. Res. Lett., 40, 4938–4943, https://doi.org/10.1002/grl.50937, 2013.
Worden, J. R., Bloom, A. A., Pandey, S., Jiang, Z., Worden, H. M., Walker, T. W., Houweling, S., and Röckmann, T.: Reduced biomass burning emissions reconcile conflicting estimates of the post-2006 atmospheric methane budget, Nat. Commun., 8, 2227, https://doi.org/10.1038/s41467-017-02246-0, 2017.
Yin, Y., Chevallier, F., Ciais, P., Broquet, G., Fortems-Cheiney, A., Pison, I., and Saunois, M.: Decadal trends in global CO emissions as seen by MOPITT, Atmos. Chem. Phys., 15, 13433–13451, https://doi.org/10.5194/acp-15-13433-2015, 2015.
Zheng, B., Chevallier, F., Ciais, P., Yin, Y., Deeter, M. N., Worden, H. M., Wang, Y., Zhang, Q., and He, K.: Rapid decline in carbon monoxide emissions and export from East Asia between years 2005 and 2016, Environ. Res. Lett., 13, 044007, https://doi.org/10.1088/1748-9326/aab2b3, 2018.
- Abstract
- Introduction
- CO flux estimation
- Bayesian CO flux attribution approach
- Uncertainty prediction and limitations
- Top-down isoprene estimates
- Global budgets of CO and C5H8 from biogenic emissions
- Seasonality of biogenic emissions – case study for the North African savannas
- Summary and future work
- Data availability
- Author contributions
- Competing interests
- Acknowledgements
- Financial support
- Review statement
- References
- Supplement
- Abstract
- Introduction
- CO flux estimation
- Bayesian CO flux attribution approach
- Uncertainty prediction and limitations
- Top-down isoprene estimates
- Global budgets of CO and C5H8 from biogenic emissions
- Seasonality of biogenic emissions – case study for the North African savannas
- Summary and future work
- Data availability
- Author contributions
- Competing interests
- Acknowledgements
- Financial support
- Review statement
- References
- Supplement