the Creative Commons Attribution 4.0 License.
the Creative Commons Attribution 4.0 License.
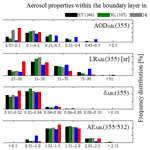
Interrelations between surface, boundary layer, and columnar aerosol properties derived in summer and early autumn over a continental urban site in Warsaw, Poland
Dongxiang Wang
Dominika Szczepanik
Iwona S. Stachlewska
PollyXT Raman polarization lidar observations were performed at the Remote Sensing Laboratory (RS-Lab) in Warsaw (52.2109∘ N, 20.9826∘ E), Poland, in the framework of the European Aerosol Research Lidar Network (EARLINET) and the Aerosol, Clouds, and Trace gases Research Infrastructure (ACTRIS) projects. Data collected in July, August, and September of 2013, 2015, and 2016 were analysed using the classical Raman approach. In total, 246 sets of intact profiles, each set comprising particle extinction (α) and backscatter coefficients (β) as well as linear particle depolarization ratios (δ) at 355 nm and 532 nm, were derived for statistical investigations and stored in the EARLINET/ACTRIS database. The main analysis was focused on intensive optical properties obtained within the atmospheric boundary layer (ABL). Their interrelations were discussed for different periods: the entire day; nighttime, with respect to the nocturnal boundary layer (NL) and the residual boundary layer (RL); at sunrise, with respect to the morning transition boundary layer (MTL); and from late afternoon until sunset, with respect to the well-mixed boundary layer (WML). Within the boundary layer, the lidar-derived optical properties (entire day, 246 sets) revealed a mean aerosol optical depth (AODABL) of 0.20±0.10 at 355 nm and 0.11±0.06 at 532 nm; a mean Ångström exponent (ÅEABL) of 1.54±0.37; a mean lidar ratio (LRABL) of 48±17 sr at 355 nm and 41±15 sr at 532 nm; a mean linear particle depolarization ratio (δABL) of 0.02±0.01 at 355 nm and 0.05±0.01 at 532 nm; and a mean water vapour mixing ratio (WVABL) of 8.28±2.46 g kg−1. In addition, the lidar-derived daytime boundary layer optical properties (for the MTL and WML) were compared with the corresponding daytime columnar aerosol properties derived from the multi-filter rotating shadowband radiometer (MFR-7) measuring within the National Aerosol Research Network (PolandAOD-NET) and the CE318 sun photometer of the Aerosol Robotic NETwork (AERONET). A high linear correlation of the columnar aerosol optical depth values from the two latter instruments was obtained in Warsaw (a correlation coefficient of 0.98 with a standard deviation of 0.02). The contribution of the aerosol load in the summer and early-autumn free troposphere can result in an AODCL value that is twice as high as the AODABL over Warsaw. The occurrence of a turbulence-driven aerosol burst from the boundary layer into the free troposphere can further increase this difference. Aerosol within the ABL and in the free troposphere was interpreted based on comparisons of the properties derived at different altitudes with values reported in the literature, which were characteristic for different aerosol types, in combination with backward trajectory calculations, satellite data, and model outputs. Within the boundary layer, the aerosol consisted of either urban anthropogenic pollution (∼ 61 %) or mixtures of anthropogenic aerosol with biomass-burning aerosol (< 14 %), local pollen (< 7 %), or Arctic marine particles (< 5 %). No significant contribution of mineral dust was found in the boundary layer. The lidar-derived atmospheric boundary layer height (ABLH) and the AODABL exhibited a positive correlation (R of 0.76), associated with the local anthropogenic pollution (most pronounced for the RL and WML). A positive correlation of the AODABL and LRABL and a negative correlation of the ÅEABL and LRABL, as well as the expected negative trends for the WVABL (and surface relative humidity, RH) and δABL, were observed. Relations of the lidar-derived aerosol properties within the ABL and the surface in situ measurements of particulate matter with an aerodynamic diameter less than 10 µm (PM10) and less than 2.5 µm (PM2.5) measured by the Warsaw Regional Inspectorate for Environmental Protection (WIOS) network, and the fine-to-coarse mass ratio (FCMR) were investigated. The FCMR and surface RH showed a positive correlation even at nighttime (R of 0.71 for the MTL, 0.63 for the WML, and 0.6 for the NL), which generally lacked statistically significant relations. A weak negative correlation of the FCMR and δABL (more pronounced at 532 nm at nighttime) and no casual relation between the FCMR and ÅEABL were found. Most interestingly, distinct differences were observed for the morning transition layer (MTL) and the well-mixed layer (WML). The MTL ranged up to 0.6–1 km, and was characterized by a lower AODABL(<0.12), wetter conditions (RH 50–80 %), smaller particles (ÅEABL of 1–2.2; FCMR from 0.5 to 3), and a low LRABL of between 20 and 40 sr. The WML ranged up to 1–2.5 km and exhibited a higher AODABL (reaching up to 0.45), drier conditions (RH 25–60 %), larger particles (ÅEABL of 0.8–1.7; FCMR of 0.2–1.5), and a higher LRABL of up to 90 sr.
Atmospheric aerosol can impact climate (e.g. Feingold et al., 2016; Seinfeld et al., 2016), weather (e.g. Fan et al., 2016; Gayatri et al., 2017), air quality (e.g. Fuzzi et al., 2015), and human health (e.g. Zheng et al., 2015; Trippetta et al., 2016). Aerosol particles affect the Earth's radiative budget, as they interact with the incoming solar short-wave radiation and the outgoing terrestrial long-wave radiation. Depending on the aerosol type, they can scatter and/or absorb the radiation, causing local warming or cooling of the atmosphere, at the surface and at the top of atmosphere (Kaufman et al., 2002). The variety of aerosol sources, those of natural and anthropogenic origin, as well as the influence of diverse meteorological conditions on aerosol characteristics and transport, lead to strongly variable aerosol contents within the troposphere. Aerosol optical properties, size, and composition are important for aerosol–cloud–radiation interaction studies (Seinfeld et al., 2016) and for radiative transfer modelling (Lolli et al., 2018).
The Intergovernmental Panel on Climate Change (IPCC) reported that the sparse and/or poorly-known information on the aerosol temporal and spatial variability causes high uncertainty in the assessment of their influence on the global radiation budget (Stocker et al., 2013). The latest IPCC report indicates that the reduction of uncertainties is still necessary, as it can improve the ability to accurately forecast global climate change (Masson-Delmotte et al., 2018). Several past studies have been dedicated to investigating and improving the above-mentioned uncertainties. Pan et al. (2015) identified the major discrepancies between the state-of-the-art global aerosol models and observations with respect to simulating aerosol loading over South Asia, thereby providing directions for future model improvements in this important region. Ghan et al. (2016) found that uncertainty regarding anthropogenic aerosol effects on cloud radiative forcing arises from uncertainty in several relationships, including the choice of parameter values and numerical integration methods. Koffi et al. (2016) provided further spatial and temporal details on the state-of-the-art AeroCom II global aerosol models and investigated the reasons for the model discrepancies and diversity, which provided a good foundation for further evaluation of the models' performance at a global scale. Kipling et al. (2016) investigated the impact of a wide range of processes (emission, transport, deposition, and microphysical and chemical processes) on aerosol vertical distribution in the aerosol–climate model via a series of limiting case process-based sensitivity tests; they showed that the processes that have the greatest impact on the vertical distribution vary both between different aerosol components and over the particle size spectrum. Seinfeld et al. (2016) provided strategies for improving estimates of aerosol–cloud relationships in climate models, for new remote sensing and in situ measurements, and for quantifying and reducing the model uncertainties.
Air pollution is one of the major environmental issues in metropolitan areas due to its adverse effects on human health (e.g. Chen et al., 2008; Lelieveld et al., 2015). Juda-Rezler et al. (2012) reported that air quality is related to anthropogenic emissions and natural emissions and that a change in temperature, water vapour, precipitation, wind speed, and wind direction can affect atmospheric chemical reactions, atmospheric transport, and deposition processes, as well as the rate of pollutant transport from urban environments to global-scale environments. The emission of pollutants changes the chemical composition of the atmosphere, which in turn has a feedback effect on the regional and global climate (Juda-Rezler et al., 2012). Strong emissions, e.g. from traffic, industry, or heating, can drastically decrease air quality, particularly when meteorological conditions prevent an exchange of polluted and clean air, (Juda-Rezler et al., 2011). In Europe, surface particulate matter (with an aerodynamic diameter below 10 µm, PM10, and 2.5 µm, PM2.5) is one of the most serious air quality problems (e.g. De Leeuw et al., 2001; Wolff and Perry, 2010). As atmospheric aerosol can affect air quality, health, and the environment, joint studies of the aerosol optical property measurements in combination with surface observations of PM10 (e.g. Guo et al., 2009, 2017; Stachlewska et al., 2017b; Popovici et al., 2018), PM2.5 (e.g. Schaap et al., 2009; Stachlewska et al., 2017b, 2018), and PM1 (e.g. Qin et al., 2018) concentrations can improve our knowledge of the atmospheric environment.
The atmospheric boundary layer (ABL) can affect the dispersion of pollutants within the mixing layer (Wałaszek et al., 2018). The knowledge of ABL characteristics and ABL dynamics, both related to ambient meteorological conditions, is helpful to model and predict mechanisms of importance in weather forecasting, air pollution, and climate change studies (Barlage et al., 2016). Therefore, it is meaningful to acquire knowledge of the ABL top height distribution along with the aerosol optical properties within the ABL.
Lidar techniques seem to be an optimal tool to provide height-resolved aerosol data products. Several lidar techniques suitable for aerosol studies have recently matured. In the last 10 years, rapid progress in laser technology, measurement techniques, and data acquisition systems has contributed to a much wider use of these techniques for aerosol monitoring, ranging from simple elastic backscatter lidar/ceilometer networks (Flentje et al., 2010; Lolli and Di Girolamo, 2015) to advanced multi-wavelength Raman lidar system networks (Baars et al., 2016). The European Aerosol Research Lidar Network (EARLINET; https://www.earlinet.orglast access: 15 October 2019) conducts lidar observations and provides relevant sets of lidar data products on the aerosol distribution over Europe, which are stored in a comprehensive, quantitative, and statistically significant database (Pappalardo et al., 2014). A quality assurance programme (Freudenthaler et al., 2018) and lidar data evaluation algorithms (Böckmann et al., 2004) have been developed and assessed at each lidar station, as well as during lidar intercomparison campaigns (e.g. Wandinger et al., 2016), to meet the accuracy standards required for calculations of aerosol radiative forcing. The unique dataset of lidar observations conducted over Europe allows for the classification of the aerosol type (e.g. Nicolae et al., 2018; Papagiannopoulos et al., 2018). The EARLINET network is an integral part of the Aerosol, Clouds, and Trace gases Research Infrastructure (ACTRIS; https://www.actris.eu, last access: 29 August 2019) – a pan-European initiative consolidating actions from European partners producing high quality observations of aerosol, clouds, and trace gases. As different atmospheric processes are increasingly becoming the focus of many societal and environmental challenges, such as air quality, health, sustainability, and climate change, ACTRIS initiatives aim at contributing to resolving these challenges by providing a platform for researchers to more effectively combine their efforts, and by making observational data of aerosol, clouds, and trace gases openly available to other external users.
The aerosol optical properties derived for the boundary layer from lidar have been studied using a statistical approach at several EARLINET sites in Europe (e.g. Matthias et al., 2004; Mattis et al., 2004; Amiridis et al., 2005; Sicard et al., 2011; Siomos et al., 2018). However, most of these studies have been restricted to the analysis of either AOD and/or LR values in the ABL (e.g. Matthias et al. 2004; Amiridis et al. 2005, 2009; Sicard et al., 2011), or relations between LRABL and AEABL (Giannakaki et al., 2010). Comprehensive investigations of interrelations of various aerosol optical properties have not been reported thus far. Therefore, the current study is the first to report on these interrelations (or lack of) for different times of day (nocturnal, sunrise and sunset, daytime) in summer (July and August) and early autumn (September) over Warsaw. The relations of surface PM2.5, PM10, and AODCL have been reported by e.g. Guo et al. (2009), Filip and Stefan (2011), Zang et al. (2017), and Szczepanik and Markowicz (2018), and the relations of AODCL and ambient RH were reported by e.g. Bergin et al. (2000) and Altaratz et al. (2013). Stachlewska et al. (2017b, 2018), investigated the relations of PM2.5, PM10, RH, LRABL, AODABL, AEABL, and δABL, based on case studies. In this paper, we analyse and report on those sets of properties based on long-term observations. The combination of such study with hygroscopic growth monitoring is the next step in the future (Navas Guzmán et al., 2019). The results reported in the current paper will enrich the state of knowledge on boundary layer aerosol optical properties by building a seasonal climatology over Warsaw (even if it is limited to July–September of the 3-year period – 2013, 2015, and 2016) and, thus, providing a reference for comparative studies with the other EARLINET (e.g. Papayannins et al., 2008) and AERONET sites (e.g. Siomos et al., 2018). The dataset obtained has excellent potential for aerosol microphysical parameter inversion (e.g. Veselovskii et al., 2002; Böckmann et al., 2005) and can also be used to test aerosol typing algorithms (e.g. Nicolae et al., 2018; Papagiannopoulos et al., 2018). This research provides parameters for studies of boundary layer meteorology, and the derived aerosol optical properties can serve to verify aerosol transport models (e.g. Binietoglou et al., 2015); furthermore, these properties can be used in the estimation of the aerosol radiative forcing effect in radiative transfer modelling (e.g. Lisok et al., 2018; Lolli et al., 2018) as well as for the validation of space-borne lidar (e.g. Illingworth et al., 2015; Papagiannopoulos et al., 2016; Proestakis et al., 2019).
In the framework of EARLINET, extensive observations at a continental, urban site in Warsaw at the Remote Sensing Laboratory (RS-Lab) of the Institute of Geophysics at the Faculty of Physics of the University of Warsaw have been performed since July 2013. Within the current paper the data products from the Warsaw site published in the EARLINET/ACTRIS database were utilized (The EARLINET Publishing Group, 2018). The study was dedicated to the calculation and analysis of the aerosol optical properties derived within the atmospheric boundary layer, at various times of the day, during summer (July and August) and early autumn (September) of 2013, 2015, and 2016, over a continental urban site in Warsaw. The following three specific goals were addressed: (i) investigation of interrelations between the different aerosol optical properties; (ii) investigation of relations between the aerosol optical properties, particulate matter (PM2.5 and PM10), and the relative humidity; and (iii) assessment of the quantitative contribution of the boundary aerosol optical depth to the columnar aerosol optical depth. In Sect. 2, the instrumentation and datasets are described. Section 3 presents the methodology regarding the boundary layer height derivation and the aerosol optical property retrieval approaches. Section 4 focuses on comparisons of different types of mean optical properties as derived within the boundary layer and in the atmospheric column: PMs, ABLHs, and near-surface relative humidity. Conclusions are given in Sect. 5.
The Raman polarization and water vapour lidar (52.2109∘ N, 20.9826∘ E, 112 m a.s.l.) is located at the Remote Sensing Laboratory (RS-Lab, https://www.igf.fuw.edu.pl/en/instruments, last access: 1 May 2019) of the Institute of Geophysics at the Faculty of Physics of the University of Warsaw, Poland. The location of the RS-Lab is shown in Fig. 1. The RS-Lab conducts observations as a part of the European Aerosol Research Lidar Network (EARLINET, https://www.earlinet.org, last access: 15 October 2019, Pappalardo et al., 2014) and provides regular measurements within the worldwide Polly.NET lidar network (http://polly.tropos.de/, last access: 23 September 2019, Baars et al., 2016) and within the National Aerosol Research Network PolandAOD-NET (http://www.polandaod.pl, last access: 15 October 2019, Supplement in Markowicz et al., 2016). The so-called “next generation PollyXT” lidar deployed in Warsaw was described in a great detail in Engelmann et al. (2016).
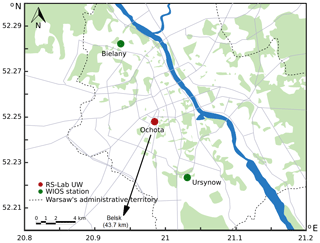
Figure 1The location of the Remote Sensing Laboratory (RS-Lab) at UW Ochota Campus in Warsaw, Poland (in red), the WIOS monitoring stations in Ursynow and Bielany (in green), and the Institute of Geophysics at the Polish Academy of Sciences (IGF-PAS) Observatory in Belsk (arrow).
Since July 2013, the PollyXT lidar has performed quasi-continuous 24/7 observations. Powerful laser pulses (180, 110, and 60 mJ) at 1064, 532, and 355 nm are emitted co-axially and vertically, with a 20 Hz repetition frequency, into the atmosphere. Detection is performed with a Newtonian telescope at eight channels (so-called 2 WV), which enables the determination of the particle extinction coefficient profiles (α) at 532 and 355 nm, the particle backscatter coefficient profiles (β) at 1064, 532, and 355 nm, the linear particle depolarization ratio profiles (δ) at 532 and 355 nm, and the water vapour mixing ratio (WV). The signals at all channels are recorded up to 48 km with a standard 7.5 m vertical and 30 s temporal resolution. Measured signals are affected by an incomplete geometrical overlap between the emitted laser beam and the receiving telescope; therefore, the signals in the range below an altitude of 400 m are rejected from further evaluation. The overlap-range issue posed a first constraint on the selected dataset, i.e. constraining analyses to the summer and early-autumn (July–September) data. In winter, the atmospheric boundary layer height derived at noon and midnight from the radiosounding profiles was found often enough below the complete lidar's overlap range. Hence, in winter, within the range in which the divergent lidar laser beam is not fully received by the full field of view of the lidar telescope, the detection of the boundary layer height by lidar is limited. In contrast, in summer and early autumn, the boundary layer height is always above the complete lidar's overlap range; thus, it does not affect the derived profiles. Therefore, we restricted the analyses of the optical properties within the boundary layer to the latter period. Moreover, the analysis was restricted to the complete sets of profiles of aerosol properties (i.e. 2 WV) derived in 2013, 2015, and 2016, as for the above-mentioned period, for this site, it was feasible to obtain the highest number of quality controlled lidar profiles. They were then calculated and stored in the EARLINET/ACTRIS database.
Although the PollyXT lidar in Warsaw performs quasi-continuous 24/7 observations, the data cannot be obtained every single day. Unfavourable weather conditions (e.g. precipitation or thick low-level clouds) significantly limit the number of observations in spring and late autumn, and practically prevent observations in winter. Instrumental issues occur (e.g. technical failures), which are season independent but in some periods lasted several weeks or months. Finally, even if observations were performed, it was not always feasible to derive the aforementioned full sets of aerosol properties, e.g. due to overly strong background light at daytime or an overly low (high) aerosol load. Note that, for the July–September period of 2013, 2015, and 2016, no other site provided such a high number of complete sets of measurements within the EARLINET/ACTRIS database.
Quality-controlled profiles of optical properties are stored in the EARLINET/ACTRIS database (http://www.earlinet.org, last access: 15 October 2019). The statistical analysis covers profiles derived for EARLINET regular measurements (Mondays and Thursdays, ±2 h from zenith and sunset) and for dedicated measurements (e.g. diurnal cycles, special alert events). From the Warsaw site, only cloud-screened profiles evaluated using the classical Raman approach are feed into the database. The profiles obtained for lidar observations in July, August, and September of 2013, 2015, and 2016 were analysed (2014 was excluded from analyses due to that fact that the data availability was too sparse). In total, 246 lidar profiles were collected for this study (denoted as contributing to “entire time”), 113 profiles were obtained for the “nocturnal time” (21:00–02:00 UTC), and 37 profiles were derived at sunrise (03:00–08:00 UTC) and 63 profiles at sunset (16:00–20:00 UTC), here defined as the “sunrise/sunset or transition time”. The precise sunrise and sunset times are available at http://www.timeanddate.com/sun/poland/warsaw (last access: 5 May 2019). The analysis period is separated into three periods, because the change in atmospheric conditions is driven by different processes during the designated times; this allows for change in the aerosol optical properties to be investigated. Note, that only 29 profiles were available under daytime conditions (08:00–16:00 UTC), which was considered to be too low to consider the daytime category separately – thus, these profiles joined the entire time category. However, comparisons of the optical properties derived from the lidar and photometer measurements were carried out for a subset of daytime profiles (03:00–19:00 UTC).
A multi-filter rotating shadowband radiometer (MFR-7; Yankee Environmental Systems) was used for continuous passive measurements at the RS-Lab in the frame of the PolandAOD-NET network activities. The instrument operates at six narrow-band channels (415, 500, 615, 673, 870, and 940 nm) and one broadband channel. It measures direct, diffuse, and total solar radiation, from which the spectrally resolved aerosol optical depth is obtained. In situ calibration using the classic Langley approach is applied on a regular basis. Details on the instrument design and uncertainty analyses are reported in Harrison et al. (1994). Cloud-screened products used in this study are the AODCL(415) and AODCL(500), with uncertainty at the ±0.025 level, and ÅECL(415/500), with uncertainty at the ±0.04 level. AODCL values less than 0.03 were excluded from the analyses.
A sun photometer (CE318; CIMEL Electronique) is operated at the Institute of Geophysics at the Polish Academy of Sciences (IGF-PAS) in the Observatory in Belsk (51.8366∘ N, 20.7916∘ E, 190 m a.s.l.), which is located 43.7 km south-west of the RS-Lab in Warsaw and provides the longest record of passive measurements in Poland. The same instrument was recently installed in the RS-Lab in Warsaw, and since January 2018 it has provided data to the AERONET database. Passive measurements of direct and diffuse solar irradiance and sky radiance at the Earth's surface at nine wavelengths in a spectral range from 340 to 1640 nm are used for retrieval of the AOD and ÅE. The data are calibrated once a year at the PHOTONS/AERONET-EUROPE calibration centre (http://loaphotons.univ-lille1.fr, last access: 3 September 2019) and processed by the Aerosol Robotic NETwork (AERONET, http://aeronet.gsfc.nasa.gov, last access: 15 October 2019, Holben et al., 1998). The Version 3 products (Giles et al., 2019) of AERONET Level 2.0 cloud-screened AODCL(380) and AODCL(500), with uncertainty at the ±0.01 level, and ÅECL(380/500), with uncertainty at the ±0.03 level, were used. AODCL values less than 0.03 were excluded from the analyses. Note that, the AODCL data from MFR-7 and CE318 are scaled using the Ångström power law (Ångström, 1929; Iqbal, 1983) to match either one another or the lidar wavelength.
The sun photometer is located about 2 km from the village of Belsk, in a typical agricultural region with fertile soil and trees. Note that the AERONET data in Belsk were only used in the current study to check data consistency and only as an indicator that the free-tropospheric aerosol load existed above Warsaw and in its vicinity; therefore, sun-photometer data from Belsk do not contribute to the core results. Note that, the AERONET CE318 sun photometer is also operated (since January 2018) at the RS-Lab of the University of Warsaw. The data from the latter instrument, specifically the data for the intercomparison of AODs obtained with the co-located CE318 and MFR-7 instruments at the RS-Lab, constitute a vital part of the results.
Particulate matter concentrations for particles with an aerodynamic diameter of less than 2.5 and 10 µm (referred to as PM2.5 and PM10 respectively) were measured at the air quality monitoring site of the Warsaw Regional Inspectorate for Environmental Protection (WIOS) in Ursynow, Warsaw, located 6.5 km from the RS-Lab. The daily and hourly averaged PM2.5 and PM10 data are available at http://sojp.wios.warszawa.pl/raport-dobowy-i-roczny (last access: 15 October 2019). The data measurements conducted at the State Environmental Monitoring stations are gathered in the JPOAT 2.0 air quality database of the National Chief Inspectorate for Environmental Protection (GIOS). These official, calibrated datasets of PM2.5 and PM10 are accessible at http://powietrze.gios.gov.pl/pjp/archive (last access: 15 October 2019). The measurement uncertainty is below 30 % for the hourly concentrations. Products used in this study are as follows: surface daily and hourly mean particulate matter concentrations for PM2.5 and PM10, and the fine-to-coarse mass ratio (FCMR) defined as PM2.5 ∕ (PM10 − PM2.5) (Zawadzka et al., 2013). A FCMR greater than 1.5 denotes “fine particle” domination (diameter < 2.5 µm); a FCMR less than 0.5 means “coarse particle” domination (diameter between 2.5 to 10 µm). FCMR values between 0.5 and 1.5 indicate that fine and coarse particles are distributed approximately equally.
The temperature, pressure, relative humidity, wind speed, and wind direction at the surface (p, T, RH, V, and Vdir), were measured by the WXT510 (Vaisala) weather transmitter mounted on the roof platform of the RS-Lab at 21 m above the ground's surface. The atmospheric pressure, temperature, and relative humidity profiles were obtained from the RS92 (Vaisala) radiosonde launched at two World Meteorological Organization sites located in Poland: WMO 12374 station in Legionowo (52.40∘ N, 20.96∘ E, 96 m a.s.l., 25 km north of Warsaw) and WMO 12425 station in Wroclaw (51.78∘ N, 16.88∘ E, 122 m a.s.l., 300 km south-west of Warsaw). The noon and midnight radiosounding profiles (launch at 11:15 and 23:15 UTC respectively, with a duration of circa 1.5 h) were visualized and downloaded from the University of Wyoming Upperair Air Data website (http://weather.uwyo.edu/upperair/sounding.html, last access: 27 August 2019).
Note that the shadowband radiometer-derived and the sun-photometer-derived AOD and the in situ measured PM concentration values are averaged so as to correspond to the time of the lidar-derived optical profiles available for given period for the Warsaw site in the EARLINET/ACTRIS database. Moreover, the MFR-7 and CE318 instruments only collected data at daytime. The measurement sites are shown in Fig. 1. The PM site is located in the residential suburb of Ursynow. The RS-Lab is located at the university campus in the suburb of Ochota, which is shrouded by green parks. Between the three sites Ochota and Ursynow (in Warsaw) and Belsk, there are no industrial pollution sources and the anthropogenic pollution in summertime is related to traffic (Zawadzka et al., 2013).
The atmospheric boundary layer is regarded as the lowest layer of the troposphere; it is directly influenced by the Earth's surface and reacts quickly to the surface forcing (Stull, 1988). The atmospheric boundary layer height (ABLH) can be derived from the lidar elastic-scattering aerosol backscatter signal, which relies on a higher aerosol load within the boundary layer than in the free troposphere. Dang et al. (2019) recently summarized the available methods for ABLH retrievals. The limitations and capabilities of mixing height retrieval algorithms for different lidar and ceilometer systems were investigated by Haeffelin et al. (2012). Comerón et al. (2013) discussed the wavelet correlation transform method and the gradient method for the determination of the ABLH by lidar. Stachlewska et al. (2012) used the latter method for the first retrievals of the ABLH in Warsaw. Wang et al. (2019) reported that the wavelet covariance transform method (WCT) is the most optimal technique for ABLH retrieval for the PollyXT lidar data in Warsaw. As for the WCT method applied in our study, the covariance transform Wf of the Haar wavelet function h, is defined as in Eq. (1):
where P(z) is the lidar elastic signal, z1 and z2 are the respective lower and upper limits of the signal, z is the altitude, and a and b are the dilation and the centre of the Haar wavelet function respectively. The WCT calculations were carried out with the dilation of 30 range bins applied on signals averaged over 7.5 m and 30 min, and the ABLH was derived at all three elastic wavelength signals (355, 532, and 1064 nm) and then averaged for a final result.
Aerosol optical properties can be derived in different ways. The Cloud-Aerosol Lidar and Infrared Pathfinder Satellite Observation (CALIPSO) can provide the range-resolved profiles of aerosol and clouds (Winker et al., 2007). The combined ground-based lidar and cloud radar is capable of obtaining the vertical structure of cloud and aerosol properties (e.g. Delanoë and Hogan, 2008). The high spectral resolution lidar technique allows for the separation of molecular and aerosol signals (e.g. Grund and Eloranta, 1991; Burton et al., 2012). The aerosol and cloud information can be retrieved by multi-wavelength Raman lidars (e.g. Müller et al., 2007; Giannakaki et al., 2010; Alados-Arboledas et al., 2011; Baars et al., 2016; Lolli et al., 2018; Ansmann et al., 2018). The latter lidar technique was used for this study.
Lidar signals stored in the EARLINET/ACTRIS database were evaluated using the classical Raman retrieval approach. The particle extinction coefficient profiles at 355 and 532 nm were calculated from the so-called Raman lidar equation using the Rayleigh law for molecules and the Ångström power law (usually with ÅE = 1) for aerosol particles. The particle extinction coefficient (αp) was derived as in Eq. (2):
where N denotes the molecular number density of the nitrogen, λ0 is the emitted wavelength (355 or 532 nm), λN is the wavelength of the nitrogen channel (387 or 607 nm), PN is the Raman signal at the nitrogen channel, z is the distance from the lidar, (i=0, N) is the molecular extinction coefficient at the emitted or the nitrogen wavelength, and k is the wavelength dependence of particle.
The particle backscatter coefficient profiles (βp) at 355, 532, and 1064 nm are derived with the use of the obtained particle extinction coefficient profiles at 355 and 532 nm (utilized for both larger wavelengths) and calibrated at the height range free of aerosol. More details on the exact procedure are given in Baars et al. (2016).
The linear particle depolarization ratio (δp) was derived as the ratio of the cross channel and the corresponding total channel at the same wavelength, using Eq. (3):
where δv is the linear volume depolarization ratio, δm is the linear depolarization ratio of air molecules, and βm is the molecule backscatter coefficient. The procedure for the ±45 depolarization calibration is used as in Engelmann et al. (2016).
The water vapour mixing ratio (WV) was obtained as the ratio of the Raman water vapour channel and the Raman nitrogen channel, as described in Stachlewska et al. (2017a), using Eq. (4):
where PλN is the Raman backscatter signal of the nitrogen channel wavelength λN=387 nm, is the Raman backscatter signal of water vapour channel wavelength λN=407 nm, and C is the calibration constant.
For all analysed data products, low- and mid-altitude clouds are screened prior to the retrieval. The sets of profiles (αp, βp, and σp) were averaged over the same time interval, which was either 60 min (60 %), 45 min (23 %), or 30 min (17 % of profiles used), depending on the variability of the atmospheric conditions and the signal-to-noise ratio. Iarlori et al. (2015) reported that smoothing procedures can affect the features of the lidar signal profile. For the EARLINET/ACTRIS database, we store the files with profiles smoothed with the running mean over 49 (b-file) and 101 (e-file) range bins (length of a single range bin is 7.5 m), which were found to be optimal for the PollyXT lidar data products derived at the Warsaw site. Typically, the αp profiles need higher smoothing than βp and δp profiles. However, we keep two versions of the smoothed profiles in the database. In this paper, the profiles smoothed over 49 range bins were used. Keeping the smoothing at the same level is not only advantageous for the error estimations and the profile comparisons but also for the microphysical parameter inversion (Janicka et al., 2017). The profiles of the WV were averaged over 30–60 min and 60 m with no smoothing applied. With respect to the lidar retrieval, the atmospheric profiles obtained by radiosounding at Legionowo or Wroclaw were used depending on the approaching direction of the air mass transport.
After the ABLH was determined, the mean values of different optical properties within the boundary layer were derived. For the incomplete overlap region, data in the lowermost altitude range required special treatment. The lowest value of the available particle extinction coefficient was assumed to be representative down to the ground surface, which is the commonly accepted approach in lidar studies, e.g. Matthias et al. (2004). Therefore, the mean extinction coefficient of the entire ABL was obtained by extrapolating the extinction profile using this value down to the ground. Similarly, the mean backscattering coefficient and the particle depolarization ratio of the ABL were extrapolated. Although the δ profiles can be derived almost to the ground, for the EARLINET/ACTRIS database profiles are only stored down to 400 m, so that extrapolation was also required here. The water vapour mixing ratio profiles were also extrapolated, from 100 m down to the ground. The WV profiles were calculated using the ratio of two signals at the 407 and 387 nm Raman channels. The overlap term of those two channels (which is close in spectral range – only 20 nm) practically cancels when calculating their ratio. Similarly, for the particle depolarization ratio (the cross and total ratio at the same wavelength), the overlap term also cancels. Therefore, the water vapour (and the depolarization ratio profile) can be obtained almost down to the ground. The water vapour nighttime detection in July–September is typically performed from 20:00 to 04:00 UTC; thus, only the data corresponding to the nocturnal time (21:00 to 02:00 UTC) were analysed.
Within the ABL, the vertical distribution of the lidar ratio (LRABL) was derived as a ratio of the aerosol extinction to backscatter coefficient profiles at 355 and 532 nm (LR ), and then the mean LRABL was calculated.
The vertical distribution of the Ångström exponent ÅEABL(355/532) was computed by using the profiles of the aerosol extinction coefficient (not AOD) at 355 and 532 nm; the mean ÅEABL was then calculated using Eq. (5):
The aerosol optical depth within the boundary layer (AODABL) was calculated by integrating the extrapolated (to the ground) aerosol extinction profile at 355 and 532 nm from the height of z1=0 to the height of the top of the boundary layer z2= ABLH, as in Eq. (6):
There is a threshold set on the mean values of an AODABL(355) of less than 0.05 and an AODABL(532) of less than 0.03 within the lowermost 1 km, i.e. the aerosol extinction coefficient profiles are extrapolated according to the AOD threshold for each wavelength. If the AOD within 1 km is below the threshold, re-extrapolation is applied from a range bin just above the initially chosen one down to the ground in an iterative manner until the AOD values within 1 km meet the above-mentioned thresholds.
Stachlewska et al. (2018) reported the uncertainty of the AODABL at 355 and 532 nm derived from the Raman extinction coefficient profiles as being less than 20 %, the uncertainty of LRABL derived by extinction-to-backscattering coefficient ratios at 355 and 532 nm as less than 35 %, and the uncertainty of δABL at 355 and 532 nm as less than 20 % of the derived value. The uncertainty of extinction-derived ÅEABL(355/532) is less than 30 %. The uncertainty of the ABLH retrieval from PollyXT lidar is ±70 m (Wang et al., 2019).
Table 1Literature review based on the values of the lidar-derived particle optical properties used for the interpretation of aerosol measured over the RS-Lab in Warsaw. The listed properties are assessed in each aerosol layer based on a manual approach in combination with case-to-case air mass transport analyses.
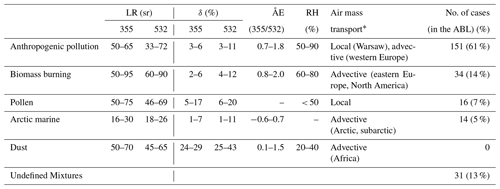
* Calculations of up to 10-day backward trajectories for aerosol layers in the free troposphere and in the boundary layer, and the assessment of possible sources of aerosol by interpreting them against satellite data (MODIS, MSG, and CALIPSO) and model outputs (NAAPS).
Finally, we attempt to interpret the aerosol measured within the boundary layer during the specified observational period over Warsaw. A number of publications have highlighted aerosol studies that use backward trajectories to interpret the possible aerosol source/type based on optical properties of observed aerosol and on the origin of air masses containing aerosol (e.g. Dörnbrack et al., 2010; Mona et al., 2012; Nemuc et al., 2014; Burton et al., 2015; Granados-Muñoz et al., 2015; Jung et al., 2016; Papagiannopoulos et al., 2016; Schmeisser et al., 2017; Szkop and Pietruczuk 2017; Ansmann et al., 2018; Di Biagio et al., 2018; Horvath et al., 2018; Foth et al., 2019). In this paper, the analysis was undertaken in a standard manner, case by case, based on the comparison of aerosol optical properties (LR, δ, ÅE, and RH) with the values reported in the literature (an excellent review of these values is provided by e.g. Nicolae et al., 2018), as well as by using the HYbrid Single-Particle Lagrangian Integrated Trajectory (HYSPLIT) model to calculate trajectories (Stein et al., 2015). The 246 profiles analysed were checked against the 3–10 day backward trajectories obtained in the boundary layer and the free troposphere, starting at altitudes of 0.5, 1.2, and 3 km, applied on the Global Data Assimilation System (GDAS) and CDC1 meteorological data (trajectories are available via the PolandAOD-NET website). We assessed possible aerosol sources by inspecting the trajectories and the aerosol optical properties against the satellite data, i.e. from the Moderate Resolution Imaging Spectroradiometer (MODIS), the Spinning Enhanced Visible and Infrared Imager (SEVIRI), and the Cloud-Aerosol Lidar and Infrared Pathfinder Satellite Observations (CALIPSO), and model outputs, i.e. the Navy Aerosol Analysis and Prediction System (NAAPS), http://www.nrlmry.navy.mil/aerosol, last access: 4 May 2019), as described by Stachlewska et al. (2018).
Roughly speaking, a LR greater than 75 sr can indicate the existence of aerosol particles related to biomass burning, values between 40 and 50 sr can indicate mineral dust, values between 50 and 60 sr can indicate anthropogenic pollution, and values between 20 and 30 sr can indicate Arctic marine particles, although these values depict an ideal case (a pure aerosol type rarely exists) and the values overlap for the different aerosol species, as indicated in Table 1.
The particle linear depolarization ratio (δp) can be used as a tracer of spherical and non-spherical particles: the low values (δ<0.01) can be regarded as being due to very small spherical particles (e.g. pollution); δ values between 0.2 and 0.35 can be regarded as beng due to dust (polluted dust values are lower – even as low as 0.1); δ values between 0.04 and 0.08 can be regarded as characteristic of biomass burning aerosol, values of approximately 0.1 indicate pollen, and a value less than 0.2 indicates urban pollution. Also here, values can overlap for the different aerosol species, as indicated in Table 1.
Table 2Mean values of the aerosol optical properties with standard deviations derived within the atmospheric boundary layer (ABL) from the PollyXT lidar at the EARLINET site, Warsaw, for measurements at 355 and 532 nm conducted for the July–September period of 2013, 2015, and 2016. Symbols denote the particle extinction coefficient (α), the particle backscatter coefficient (β), the aerosol optical depth (AOD), the lidar ratio (LR), the linear particle depolarization ratio (δ), the Ångström exponent (ÅE), and the atmospheric boundary layer height (ABLH). Mean values are obtained for different times of the day with respect to the boundary layer type.
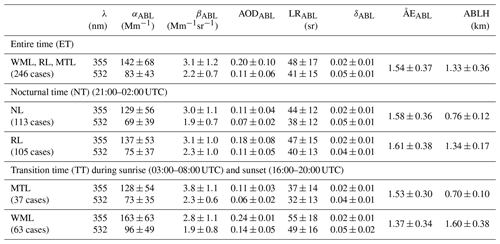
The Ångström exponent (ÅE) can be used as an indicator of the size of atmospheric aerosol particles. ÅE values of less than or equal to 1 indicate particle size distribution dominated by the coarse-mode aerosol (radii ≥ 0.5 µm, here referred to as “large particles”) that are typically associated with dust and sea salt particles (Perrone et al., 2014). ÅE values greater than or equal to 1.5 indicate size distributions dominated by the fine-mode aerosol (radii < 0.5 µm, here referred to as “small particles”) that are associated with urban pollution (Perrone et al., 2014). ÅE values between 1 and 1.5 belong to the accumulation mode (here referred to as “medium-size particles”) and are associated with biomass-burning aerosol (Janicka et al., 2017). The use of the ÅE nomenclature with respect to small, medium, and large particles is for clarity, as not to confuse them with the fine-to-coarse mass ratio (FCMR).
For brevity, hereafter the αp, βp, and δp are denoted as α, β, and δ.
Table 2 shows the mean particle extinction coefficient (α), the particle backscatter coefficient (β), the aerosol optical depth (AOD), the lidar ratio (LR), the linear particle depolarization ratio (δ), and the Ångström exponent (ÅE) derived at the 355 and 532 nm channels within the atmospheric boundary layer (ABL) for the entire (ET), nocturnal (NT), and transition (TT) times obtained for the July–September measurement period of 2013, 2015, and 2016. Different mechanisms govern the sunrise and sunset conditions; the first is driven by the development of the convective boundary layer; the latter lessens the convection which causes more stratification within the residual layer (RL). Thus, the ABLH algorithm developed determines the atmospheric boundary layer top as a morning transition layer (MTL), a well-mixed layer (WML), a nocturnal layer (NL), and/or a residual layer (RL).
The mean entire time (24 h) ABLH was 1.33±0.36 km for July–September of 2013, 2015, and 2016. Wang et al. (2019) reported that the decadal mean ABLH over Warsaw in summer was 1.34±0.15 km based on the 10-year dataset. This indicates that, in terms of the ABLH, the dataset analysed can be regarded as representative of the long-term analysis. The mean values of the αABL, AODABL, and LRABL calculated at the two wavelengths in the transition time are higher during the sunset period (WML) and lower during the sunrise period (MTL), the latter being similar to the NL. The mean ÅEABL in the given periods was high (1.37–1.61, in Table 2), indicating small particles. (Later on, we will show that the ÅEABL values ranged between 0.7 and 2.9 for the nocturnal period, whereas the range narrowed to 0.8–2.2 in the sunrise/sunset period.)
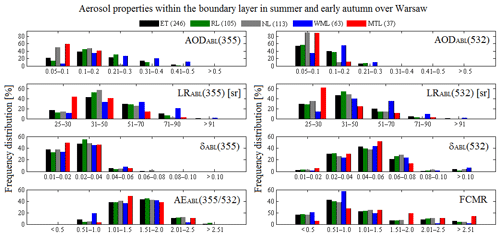
Figure 2Frequency distribution of the aerosol optical depth (AOD), the lidar ratio (LR), the linear particle depolarization ratio (δ), and the Ångström exponent (ÅE) at 355 and 532 nm derived within the atmospheric boundary layer at the EARLINET/ACTRIS lidar site, Warsaw, for the July–September period of 2013, 2015, and 2016. The corresponding fine-to-coarse mass ratio (FCMR) derived from measurements of surface particulate matter with an aerodynamic diameter below 10 µm (PM10) and below 2.5 µm (PM2.5) at the WIOS site in Ursynow, Warsaw. The period of measurement is divided into the following time spans: the entire time (ET; 24 h); the nocturnal time (NT), between 22:00 and 02:00 UTC – including the residual boundary layer (RL) and the nocturnal boundary layer (NL); and the transition time (TT), after sunrise between 03:00 and 08:00 for the morning transition layer (MTL) and before sunset between 16:00 and 20:00 UTC for the well-mixed boundary layer (WML).
The frequency distribution plots for the AODABL, LRABL, and ÅEABL derived at 355 and 532 nm, and the FCMR derived for PM2.5 and PM10 for the entire, nocturnal, and sunrise/sunset periods are shown in Fig. 2. The mean AODABL mainly ranges from 0.1 to 0.3: 80 % of the occurrences are attributed to AODABL(355) in the 0.1–0.3 range and AODABL(532) of below 0.2. The mean LRABL(355) and LRABL(532) values mainly range from 30 to 70 sr, which accounts for more than 75 % of total data. The majority of the ÅEABL(355/532) values are between 1.0 and 2.0 (more than 90 % of the total data), which indicates mid-sized and small particles (≤ 500 nm) within the boundary layer. The FCMR values between 0.5 and 1.5 constitute around 70 % of the total data, indicating a more or less equal distribution of fine and coarse particles with a size between 2.5 and 10 µm at the surface. Most of the AODABL in the MTL is below 0.2, and the LRABL in the MTL ranges from 25 to 50 sr at both wavelengths. The δABL in the MTL between 0.04 and 0.06 accounts for around 50 % of occurrences. The fine particles are expected in the MTL, as the majority of the FCMR values are above 1.5.
Amiridis et al. (2005) reported a 4-year mean AODABL of 0.44±0.16 at 355 nm and a mean LRABL of 49±25 at 355 nm in summer at Thessaloniki, Greece. According to Papayannis et al. (2008), this much higher value of AODABL(355) can be attributed to a significantly stronger impact from the summertime Saharan dust events on Thessaloniki than on Warsaw. Sicard et al. (2011) reported a low AODABL of 0.07±0.02 at 532 nm in north-eastern Spain, and attributed it to the influence of the sea breeze on the Barcelona area. Mattis et al. (2004) reported a 3-year mean AODABL(355) of 0.38 and a AODABL(532) of 0.18 for Leipzig, with a mean LRABL(355) of 58 sr and a LRABL(532) of 53 sr, and a mean ÅEABL(355/532) of 1.4. However, the ÅEABL was between 1.8 and 2.2 in the upper boundary layer during summer in Leipzig (Matthias et al., 2004); thus, only slightly larger particles are observed in the ABL in Warsaw during summer compared with Leipzig. Using Raman lidar observations at 10 EARLINET stations, Matthias et al. (2004) derived the lowest AODABL values in northwestern Europe (Aberystwyth) and the highest values in southeastern Europe (Athens), which was again attributed to the impact of Saharan dust events on the aerosol distribution in southern Europe.
The lidar ratio was used for the aerosol type characterization in various aerosol typing algorithms (e.g. Papagiannopoulos et al., 2018; Nicolae et al., 2018). Alados-Arboledas et al. (2011) reported lidar ratios for a fresh biomass-burning pollution plume of 60–65 sr at 355 and 532 nm at Granada. Müller et al. (2007) reported lidar ratios of 45–60 sr with a mean value of 53 sr at 532 nm, and a particle depolarization ratio at 532 nm of below 0.05 for Leipzig, under local and regional urban and anthropogenic haze conditions. Amiridis et al. (2005) reported a continental aerosol 4-year mean lidar ratio of 40–47 sr at 355 nm for Thessaloniki, and Giannakaki et al. (2010) reported a biomass-burning aerosol 7-year mean lidar ratio of 70 sr at 355 nm. Optical properties of eight aerosol types were derived by Burton et al. (2012) over North America for urban aerosol (lidar ratio of 53–70 sr at 532 nm with a particle depolarization ratio of 0.03–0.07) and for smoke particles (lidar ratio of 33–46 sr with a particle depolarization ratio of 0.04–0.09). A lidar ratio of marine particles of 20–26 sr at 532 nm was found in North Atlantic and the tropical Indian Ocean by Müller et al. (2007), and Masonis et al. (2003) reported a value of 25±4 sr at 532 nm in Hawaii. Dawson et al. (2015) presented a global mean lidar ratio for marine aerosol of 26 sr, with a range from 22±7 to 32±17 sr, depending on variation of the mean ocean surface wind speed. Haarig et al. (2017) reported lidar ratios varying from 19 to 27 sr at 355 nm and 23 to 25 sr at 532 nm for marine particles, and a particle depolarization ratio of between 0.05 and 0.12 sr at 355 nm and 0.07 and 0.15 sr at 532 nm. A review of aerosol types reported by Groß et al. (2013, 2015) includes a classification scheme based on the following values: the lidar ratio for the marine particles varies from 16 to 30 sr at 355 nm and 18 to 26 sr at 532 nm; biomass burning aerosol ranges from 50 to 95 sr at 355 nm and 60 to 90 sr at 532 nm; mineral dust ranges from 50 to 70 sr at 355 nm and 45 to 65 sr at 532 nm; and anthropogenic pollution ranges from 50 to 65 sr at 355 nm and 50 to 60 sr at 532 nm. In the current study, LRABL values in the range of 25–30 sr at both wavelengths were obtained in several cases (Fig. 2), and were interpreted as likely being due to the transport of a clean air mass of Arctic marine particles injected into the boundary layer in Warsaw during the analysis period. Such cold air masses can be transported from the Arctic to eastern Europe (Costa-Surós et al., 2015).
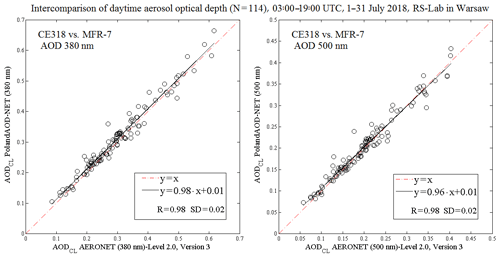
Figure 3Intercomparison of hourly averaged clear-sky daytime aerosol optical depth measured within the atmospheric column (AODCL) with the CE318 instrument at the AERONET site in Warsaw (Version 3 Level 2.0 data) and the MFR-7 shadowband radiometer at the PolandAOD-NET site in Warsaw (Level 1.5 data). Note the high agreement obtained for both wavelengths for a month (July 2018) of co-located measurements (instruments at a distance of 3 m and at the same altitude) at the RS-Lab in Warsaw. The AODCL at 415 nm from the MFR-7 was scaled to 380 nm using the Ångström power law.
The linear particle depolarization ratio is an indicator of non-spherical particles (Ansmann et al., 2009; Sakai et al., 2010; Gasteiger and Freudenthaler, 2014). The total depolarization ratio in dust episodes have been reported to be above 0.2, whereas anthropogenic pollution aerosol have a total depolarization ratio below 0.1 (Xie et al., 2008; Nemuc et al., 2013). Heese and Wiegner (2008) reported a particle depolarization ratio for dust (∼ 0.25) and biomass-burning aerosol (< 0.1) over Sahel (West Africa). Particle depolarization ratios of dust particles in the range of 0.1–0.25 were reported in Leipzig (Matthias et al., 2004), whereas values of 0.3–0.35 were found at Ouarzazate, Morocco (Freudenthaler et al., 2009). The particle depolarization ratios of urban haze and fire smoke have been reported to be less than 0.05 at different sites (Müller et al., 2007). A linear particle depolarization ratio for marine aerosol, in the range from 0.01 to 0.03, was reported by Groß et al. (2011). In the current study, the δABL values obtained (shown in Fig. 2) are within the range of the values listed above, characterizing different aerosol types. As δ is sensitive to the size of the sensed non-spherical particles, small particles (< 300 nm) sensed with a wavelength that is twice as large (532 nm) can be under the limit of detection (as seen in Fig. 2). The dust cases detected in the free troposphere during the given measurement period in Warsaw (e.g. Janicka et al., 2017) were excluded from the current analyses (four cases); however, the derived δ values of the entire observation time are less than 0.1, which means that there were no cases of dust particles being deposited or advected into the ABL, although the existence of polluted dust cannot be entirely excluded.
Overall, during the July to September period of 2013, 2015, and 2016 in Warsaw, the aerosol within the ABL consisted mainly of the following: (i) urban anthropogenic pollution of local origin or pollution transported from areas below or above of the Czech Republic via Silesia and/or Germany (61 %), and its mixtures with (ii) grass and peatland biomass-burning aerosol transported from Russia, the Ukraine, and Belarus (14 %), (iii) pollen emissions of strictly local origin from Warsaw's semi-natural green parks (7 %), and (iv) Arctic marine particles transported mainly from the Arctic over the Baltic Sea (5 %). For the remaining cases, the aerosol composition was regarded as being due to a mixture of more than two subcomponents (13 %), and was consequently not interpreted. The percentages given were derived by relating the number of profiles with an estimated origin to the total number of profiles, and are therefore given without uncertainties. No significant contribution of mineral dust into the boundary layer was found for the 246 profiles analysed, although transport pathways from the Sahara over the Iberian Peninsula or via Italy were identified for the upper troposphere.
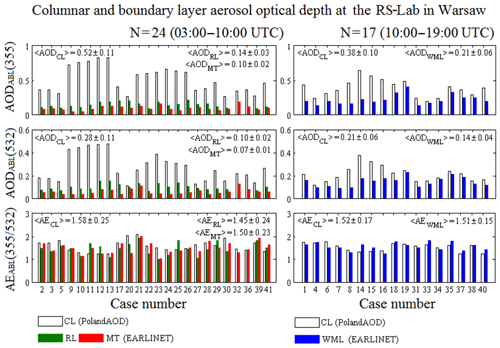
Figure 4Hourly averages of the aerosol optical depth (AOD) and the Ångström exponent (ÅE) derived within the atmospheric boundary layer at 355 and 532 nm from the PollyXT lidar at the EARLINET/ACTRIS site, Warsaw, plotted along with the corresponding MFR-7 shadowband radiometer values from the PolandAOD-NET site, Warsaw, for July–September 2013, 2015, and 2016 (leap year). These were co-located measurements at the RS-Lab in Warsaw. The AODCL from the MFR-7 measurements at 415 and 500 nm were scaled to 355 and 532 nm using the Ångström power law.
For the co-located, simultaneous Raman lidar measurements and the shadowband radiometer measurements, we assessed the AODABL contribution within the boundary layer to the AODCL within the column of air, keeping in mind that such co-located measurements never sample an identical section of air (lidar sampling in the zenith position vs. radiometer sampling at angles related to sun's elevation over the horizon). To make sure that the columnar measurements with the less commonly used (in the lidar community) shadowband radiometer do provide high quality data products, an intercomparison of the MFR-7 shadowband radiometer (PolandAOD-NET, Level 1.5 data, 415 nm and 500 nm) and the CE318 sun photometer (AERONET, Version 3, Level 2.0 data, 380 and 500 nm) was performed. The use of the above-mentioned level of data for both networks refers to clear-sky, manually cloud screened data products that have been calibrated within the past 12 months. One month of co-located daytime (03:00–19:00 UTC) measurements in July 2018 at the RS-Lab in Warsaw was chosen for the intercomparison and confirmed the high quality of the measurements performed using both instruments. Figure 3 shows high correlation coefficients (R=0.98) and low standard deviations (STD = 0.02) for 144 data points obtained at 380 and 500 nm for the intercomparison of the CE318 and the MFR-7. Note that the AODCL value at 415 nm from the MFR-7 was scaled to the value at 380 nm (corresponding to CE318 wavelength) using the Ångström power law (Fig. 3).
Figure 4 shows the daytime mean 30–60 min average of the aerosol optical depth within the atmospheric boundary layer, AODABL, at 355 and 532 nm, calculated from the mean extinction coefficient profiles of the EARLINET PollyXT lidar in Warsaw and the columnar daytime mean 1 h average (with a threshold of at least five data points) of the AODCL at 415 and 500 nm (scaled to lidar wavelengths) derived from the PolandAOD-NET MFR-7 shadowband radiometer measurements in Warsaw. Note that the AODCL values of the CE318 in Belsk were in good agreement with Warsaw results, with Belsk values being slightly lower than those in Warsaw (compare values in Table 3). Figure 4 also depicts the extinction-derived ÅEABL(355/532) of the lidar and the ÅECL(355/532) computed from the (scaled) shadowband radiometer AODCL. Products presented in Fig. 4 were derived for the July–September period of 2013, 2015, and 2016, for a subsample of cases when all three instruments were conducting observations at the same time (i.e. 41 cases).
Table 3The mean daytime (03:00–19:00 UTC) aerosol optical depth (AOD) and Ångström exponent (ÅE) with standard deviations derived within the atmospheric boundary layer at 355 and 532 nm from the PollyXT lidar at the EARLINET site, Warsaw, and in atmospheric column measured by the MFR-7 shadowband radiometer (415 and 500 nm) at the PolandAOD-NET site, Warsaw. For reference, the mean values derived from Version 3 Level 2.0 CE318 CIMEL (380 and 500 nm) at the AERONET site in Belsk are given. The AODCL of the CE318 and the MFR-7 were scaled to the lidar wavelength (355 and 532 nm respectively) using the Ångström power law. The mean values were obtained for July–September of 2013, 2015, and 2016, when instruments operated simultaneously (41 cases). The mean values derived for cases with no aerosol in the free troposphere (12 cases), as given in EARLINET/ACTRIS database, are shown in parentheses and emphasized using italic font.
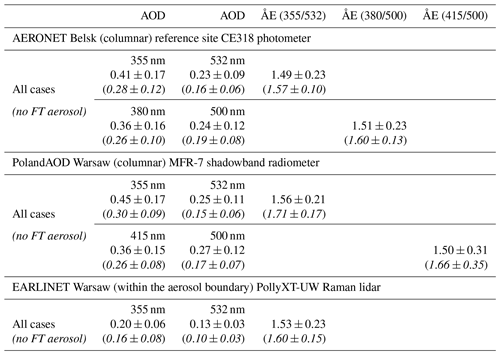
In Table 3, the CE318-derived mean AOD is 0.41±0.17 at 355 nm and 0.23±0.09 at 532 nm, the MFR-7-derived mean AOD is 0.45±0.17 at 355 and 0.25±0.11 at 532 nm, and the PollyXT-derived mean AODABL is 0.20±0.06 at 355 and 0.13±0.03 at 532 nm, for the 41 points. The columnar AODCL values of the two instruments are the same within the given uncertainty range, despite the 43.7 km distance between the two sites (Warsaw and Belsk). It can be expected that the AODABL is less than the AODCL (e.g. Sicard et al., 2011; Szczepanik and Markowicz, 2018). The results obtained indicate that the mean values of the AODABL are twice as large as the mean values of the AODCL. The high contribution of the aerosol load in the free troposphere can be related to the summertime long-range transport of the biomass-burning aerosol to Warsaw (e.g. Markowicz et al. 2016; Szkop and Pietruczuk, 2017; Janicka et al., 2017; Stachlewska et al., 2017a, 2018). This is why the mean values derived for the cases with no long-range transport in the free troposphere are listed in parentheses in Table 3 (i.e. no allocation to categories such as forest-fire or dust in the EARLINET/ACTRIS database). Excluding those cases from the mean results in an AODCL that is roughly 30 to 40 % lower in Warsaw and ∼ 30 % lower in Belsk. At the same time, the mean values of the AODABL do not decrease as much, i.e. the AODABL is less than 20 % lower. For conditions with no aerosol layers in the free troposphere, Szczepanik and Markowicz (2018) proposed the following approximation of the daytime boundary layer aerosol load for a rural mountainous site (Strzyzow, Poland, elevation 899 m a.s.l.): the AODABL(500) equals approximately 80 % of the AODCL(500). Based on the values listed in the Table 3, such approximation for the urban continental site in Warsaw (elevation 112 m a.s.l.) would indicate AODABL(532) ≈ 53 % AODCL(532) and AODABL(355) ≈ 67 % AODCL(355).
A closer look at Fig. 4 shows that the lidar-derived AODABL values are less than 0.2 on a few days (e.g. case numbers 9–13), although corresponding values of the radiometer-derived AODCL are more than 0.7. This is not an error. On 10 July 2013, biomass-burning aerosol from Canadian wildfires was detected by lidar in Warsaw, with an apparent optically thick aerosol layer suspended in the lower troposphere just above the top of the boundary layer, as reported by Janicka et al. (2017) and Ortiz-Amezcua et al. (2017). Due to the low ABLH (< 1000 m) on this day, not unusual under high-pressure system conditions over Poland e.g. Stachlewska et al. (2018), the optical depth contribution of the aerosol smoke layer in the free troposphere dominated over the optical depth contribution of the aerosol within the boundary layer, which explains the much higher columnar values compared with the boundary layer AOD. Markowicz et al. (2016) reported aerosol layers in the free troposphere having a significant (up to 55 %) contribution to the total optical depth, which is consistent with the results obtained in the current paper.
The results in Fig. 4, obtained for the ÅE with relation to the particle size, show that retrievals from different instruments have a similar trend of variation with time. The mean ÅECL values given in Table 3 are the same with respect to the given variability range for all 41 cases, despite the differences in the measurement wavelengths. The values in parentheses (no long-range transport of aerosol in free troposphere) show consistent results, i.e. higher ÅEABL values than ÅECL values were obtained, which indicates pollution dominating within the boundary layer.
4.1 Relation of the ABLH with optical properties and surface PM in summer and early autumn over Warsaw
The comparison of the AODCL and AODABL with the ABLH for 41 cases of temporally co-located measurements conducted with the MFR-7 and the PollyXT shows a positive correlation (0.74 at both wavelengths) of AODABL and ABLH, which is even higher than the correlation coefficient of 0.55 between AODABL and ABLH reported for Leipzig by Mattis et al. (2004). Similar results were reported for Warsaw by Stachlewska et al. (2017b, 2018), i.e. when there is no aerosol in the free troposphere above Warsaw; however, Stachlewska et al. (2017b, 2018) found that both the AODCL and AODABL increased with increasing ABLH. In the current study, no significant correlation was observed for the AODCL and ABLH, which is related to differences in the load and type of aerosol in the free troposphere and within the boundary layer. When an aerosol layer containing particles of dust, smoke, pollution, or a mixture of the above is suspended in the free troposphere, an increase in the columnar AODCL values can be observed. Marinou et al. (2017) reported that dust particles can be transported far from their source of origin and are frequently observed over central and northern Europe, with higher occurrences during summer. Dust particles can occur over Warsaw, mainly in the free troposphere (at a height above 2–3 km) during the spring and summer period (Chilinski et al., 2016; Janicka et al., 2017; Szczepanik et al., 2019). Biomass-burning particles and smoke layers were detected over central Europe in the summers of 2013 (Janicka et al., 2017; Ortiz-Amezcua et al., 2017; Trickl et al., 2015), 2015 (Stachlewska et al., 2017b; Szkop and Pietruczuk, 2017), and 2016 (Stachlewska et al., 2018). Biomass burning and dust were detected and analysed in southern Europe using a 10 year lidar dataset (Siomos et al., 2017, 2018). Canadian wildfire smoke detected in the troposphere and the stratosphere in summer 2017 over central Europe was also reported by Haarig et al. (2018), Ansmann et al. (2018), Hu et al. (2019), and Baars et al. (2019).
In cases where there is a lack of clouds (as in this study), the less sufficient growth of the ABLH could be explained as being partly due to the aerosol suspended in the free troposphere; this aerosol reduces the solar radiation reaching the surface and, thus, suppresses the thermal turbulence, leading to a lower boundary layer height. Hence, for certain conditions, the relation of AODCL and the ABLH can be expected to exhibit a negative correlation.
The majority of the 41 cases of daytime, clear-sky, summertime measurements analysed were related to observations of biomass-burning aerosol in the free troposphere. Over an urban site, the AODABL can increase due to pollution in the boundary layer, which adds to the growth of the ABLH due to the sun-driven turbulence. At the same time, the AODCL can decrease when free-tropospheric aerosol (e.g. absorbing particles) causes negative radiative effects at the surface. Another aspect that has to be accounted for is the presence of aerosol particles in the troposphere directly above the top of the boundary layer, which may follow from the dynamics of turbulent eddy structures in the layer. Even in the absence of convection, a typical feature of turbulent boundary layer flows is the presence of abrupt bursting and sweeping events (Pope, 2000). Bursts can eject aerosol particles to the upper regions of the boundary layer. The interactions between vortical structures are responsible for the balances of the particle concentration in the boundary layer flows (Béghein et al., 2014).
The AODABL is calculated as an integral of the extinction coefficient within the ABLH, and the ABLH is a variable of AODABL; therefore, the ABLH is not expected to be strongly related to the aerosol conditions above in the free troposphere. The aerosol optical depth is a unique parameter to determine the atmospheric aerosol load, and the ABLH derived by lidar relies on a higher aerosol concentration within the boundary layer than in the free troposphere. Therefore, a positive correlation between the AODABL and the ABLH can be expected. Note that although an intrusion of biomass-burning smoke into the boundary layer can contribute strongly to the suppression of the growth of the ABLH, as reported by Stachlewska et al. (2018), it still did not result in a negative correlation.
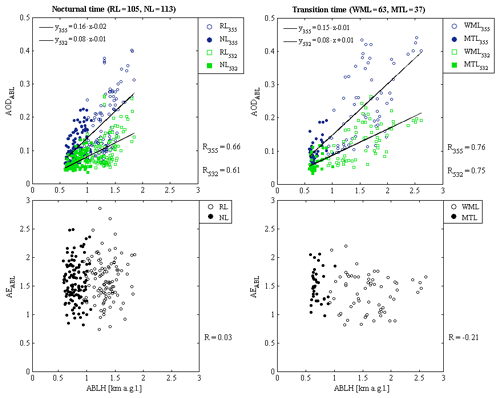
Figure 5Hourly averaged aerosol optical depth (AOD) and Ångström exponent (ÅE) derived within the boundary layer at 355 and 532 nm vs. the atmospheric boundary layer height (ABLH), all derived from the PollyXT lidar at the EARLINET/ACTRIS site of the RS-Lab,Warsaw, for July–September of 2013, 2015, and 2016. A linear fit to data points is shown for correlation coefficients (R) greater than 0.6.
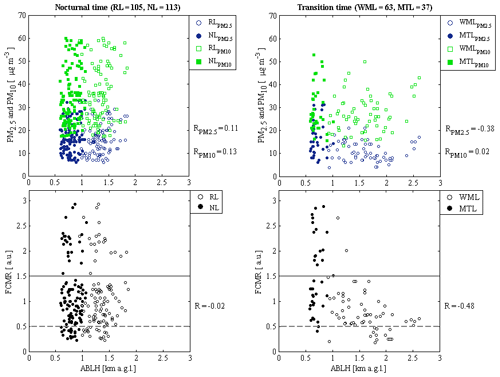
Figure 6Hourly averages of the surface fine-to-coarse mass ratio (FCMR) and particulate matter (PM2.5 and PM10) measured at the WIOS site in Ursynow, Warsaw, vs. the atmospheric boundary layer height (ABLH) derived from the PollyXT lidar at the EARLINET/ACTRIS site at the RS-Lab, Warsaw, for the July–September period of 2013, 2015, and 2016. Thresholds of the FCMR are marked using horizontal lines.
The relations of lidar-derived AODABL, ÅEABL, and the ABLH at different times of the day for the July–September period of 2013, 2015, and 2016 (246 cases) are depicted in Fig. 5. As the AODABL is related to the ABLH, there is a higher aerosol load in the ABL than in the free troposphere – thus, a positive correlation can be observed. A relatively high correlation coefficient (0.76 at 355 nm and 0.75 at 532 nm) between the ABLH and the AODABL occurred in the MTL, whereas their correlation coefficients were slightly weaker (0.66 and 0.61 respectively) during the nocturnal time (residual layer effect), when aerosol load within the ABL basically remained stable due to much weaker vertical mixing at night.
The mean ÅEABL is 1.54±0.37, indicating the dominance of relatively small particles during the observation period. No obvious relation between ÅEABL and the ABLH was obtained, but the highest values (ÅEABL > 2) were only observed at night, i.e. there was either more pollution or less humidity. Indeed, a higher concentration of PM2.5 was measured during the nocturnal period (16.75±6.86 µg m−3) compared with the other time spans (15.74±7.24 µg m−3 during sunrise and 10.94±4.13 µg m−3 during sunset). Note that the standard deviations given indicate high variability in the values obtained.
Figure 6 illustrates the relations between the ABLH, PM2.5, PM10, and the FCMR. A slightly negative trend between the FCMR and the ABLH can be observed for the well-mixed layer, which may suggest an increase in coarse particles (number or/and size) at the surface with an ascending ABLH. The fact that adiabatic effects have partly influenced the growth of particle size cannot be excluded. Schäfer et al. (2006) found a high negative correlation between PM10 and the ABLH in Hanover and Munich in winter. Rost et al. (2009) reported a strong negative relation between PM10 and the ABLH in Stuttgart. Similarly, Du et al. (2013) found that PM2.5 and the ABLH exhibited a negative correlation in Delhi and Xi'an. Geiß et al. (2017) reported that the link between PM and the ABLH can be attributed to several different reasons, such as meteorological conditions, terrain, local particle sources, and even the ABLH retrieval method itself. This was also the case for Warsaw during aerosol injections into the boundary layer that had undergone long-range transport (Stachlewska et al., 2017b, 2018). However, in general, no pronounced relationship between PM10 and the ABLH are expected for Warsaw, as also shown in Zawadzka et al. (2013). Furthermore, in the current study, no significant link between particulate matter (PM10 and PM2.5) and the ABLH was found for Warsaw during summer and early autumn (Fig. 6), which can be partly attributed to relatively low records of PM10 emissions (hourly values < 60 µg m−3) and relatively high summer ABLH values (up to 1.6 km, Table 2). The highest PM10 and PM2.5 values are observed at night (NL and RL), lower values are found for the MTL, and the lowest values are noted for the WML. Reizer and Juda-Rezler (2015) reported that either regional background pollution or local emission sources are mainly responsible for the high PM10 and PM2.5 concentrations in Polish urban areas. Clearly, the ABLH is not the main factor controlling the surface pollution in summer in Warsaw, which is consistent with reports by Bonn et al. (2016), Stachlewska et al. (2017b, 2018), and Geiß et al. (2017).
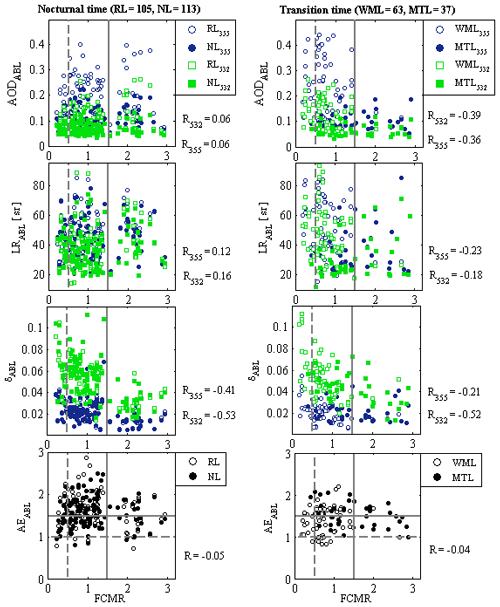
Figure 7Hourly averaged aerosol optical depth (AOD), lidar ratio (LR), linear particle depolarization ratio (δ), and Ångström exponent (ÅE) derived within the atmospheric boundary layer at 355 and 532 nm by the PollyXT lidar at the RS-Lab, EARLINET/ACTRIS site in Warsaw, for the July–September period of 2013, 2015, and 2016 vs. the surface fine-to-coarse mass ratio (FCMR) derived from particulate matter (PM2.5 and PM10) measured at the WIOS site in Ursynow, Warsaw. Thresholds of the ÅE and FCMR are marked using horizontal and vertical lines respectively.
4.2 Interrelations between different optical properties and with surface PM in summer and early autumn over Warsaw
Figure 7 presents the relationship of AODABL, ÅEABL, LRABL, δABL, and the surface FCMR for the nocturnal and transition times during July, August, and September of 2013, 2015, and 2016. The separation thresholds for the FCMR are defined as follows: an FCMR greater than 1.5 (vertical line) means that fine particles (< 2.5 µm) dominated and an FCMR less than 0.5 (vertical dashed line) means that coarse particles dominated (2.5–10 µm). The given thresholds for the ÅE roughly indicate the dominant particle size distribution mode, with separation thresholds of for small particles ÅE > 1.5 (horizontal line) and large particles ÅE < 1 (horizontal dashed line). However, the relation between the ÅE and the aerosol size distribution is complicated, as is the relationship with the FCMR.
For all time periods, FCMR values between 0.5 and 1.5 constitute the largest proportion in total. During the nocturnal period there are more fine particles than during sunrise (MTL) or sunset (WML). There is clear separation mark at a FCMR of 1.5 regardless the time period, and no clear correlation of ÅEABL with FCMR; however, for an ÅEABL between 1 and 2 during the nocturnal period and at sunrise, there is a higher PM2.5 contribution at the surface.
Because of weak vertical air motion (no convective mixing) and a lower ABLH during nighttime, relatively large aerosol particles are deposited in the ABL and most of small aerosol particles stack below the inversion of the top of the boundary layer (or residual layer). This can lead to an increase in the number of small particles accumulated within the nocturnal ABL, which manifests as a fine particles increase at the surface. In general, urban pollution, regarded as road traffic, industrial emissions, and the chemical reaction of gases (SO2, NO2, NOx), causes an increase in both PM10 and PM2.5 (He et al., 2008). The sunrise period in July–September (05:00–09:00 local time) corresponds to urban traffic emission, which can cause the lifting of particles from the ground (Zawadzka et al., 2013). The relationship of LRABL and the FCMR in Fig. 7 shows a clear separation of data, which is mainly the result of a higher abundance of fine particles in the MTL. On the contrary, more coarse particles (PM10 with a higher LRABL of 40–80 sr) occur in the WML.
The relationship of δABL and the FCMR in Fig. 7 indicates possible negative trends (stronger at 532 nm) for all time periods. The abundance of the fine (coarse) particles at the surface is related to the abundance of the spherical particles within the ABL.
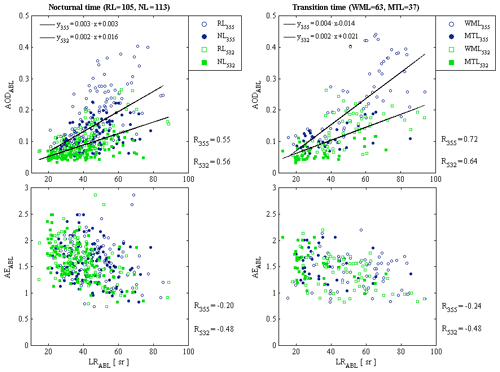
Figure 8Hourly averaged aerosol optical depth (AOD), Ångström exponent (ÅE), and lidar ratio (LR) derived within the atmospheric boundary layer at 355 and 532 nm from the PollyXT lidar at the RS-Lab, at the EARLINET/ACTRIS site in Warsaw, for the July–September period of 2013, 2015, and 2016. The lack of a correlation between the linear particle depolarization ratio (δ) and the lidar ratio (LR) is not shown for brevity. The linear fit to data points is shown for correlation coefficients (R) greater than 0.6.
Bennouna et al. (2016) reported that a significant positive correlation of PM10 and AODCL, and an increasing correlation coefficient for daily, monthly, and yearly averages, relays on the aerosol characteristics of the site. Zawadzka et al. (2013) reported a negative correlation between PM10 and AODCL for long-term monthly mean values in winter in Belsk and Warsaw, and a positive relation for unstable (meaning strong turbulent vertical mixing in summer) atmospheric conditions in Warsaw. The relation between optical properties and the surface aerosol mass concentration depends on boundary layer processes, chemical composition, source regions, weather conditions, and aerosol type, which are challenging to characterize well with the columnar AODABL and the surface PM concentrations alone. As depicted in Fig. 7, the AODABL and FCMR indicate a higher AODABL for coarse particles (PM10) in the WML and a lower AODABL for fine particles (PM2.5) in the MTL; however, no significant correlations of AODABL with PM10 or PM2.5 are reported in the current study.
Significant correlations (R>0.5) of AODCL and PM2.5 were reported mainly for the eastern cities of China (Guo et al., 2009; Zheng et al., 2015; Zang et al., 2017) and the United States (Liu et al., 2007; Hutchison et al., 2008; Wang and Christopher, 2003), where the main industrial regions with extreme pollution are located. In these cases, the majority of aerosol was expected to be present within the boundary layer. The anthropogenic pollution in Warsaw is much lower compared with the above-mentioned regions: Zawadzka et al. (2013) reported an R value of 0.42 between AODCL and PM2.5 in Warsaw, which was attributed to a significant load in the free troposphere affecting the relationship. The current study shows that there is also no significant correlation for AODABL and PM2.5, which can be explained by the low values of AODABL and PM2.5 measured during the investigated period; these low values of AODABL and PM2.5 are, in turn, attributed to the lack of high pollution events in summer and early autumn in Warsaw. Stachlewska et al. (2017b, 2018) showed that AODABL and PM2.5 in Warsaw can be correlated, under high aerosol load conditions, during events involving an injection of pollution or biomass burning that has undergone long-range transport into the boundary layers (but with no aerosol in the free troposphere).
As for the relation between AODABL and PM10, in the current study no significant linear correlation was found for the nocturnal time (similar to Filip and Stefan, 2011), and a weak positive relation was observed for the sunrise/sunset period (similar to Zawadzka et al. 2013). The ABL in summer is primarily driven by intensive convective mixing, resulting in a significantly higher ABLH than in other seasons (Wang et al., 2019). In summer, the ABL aerosol can be elevated by effective convection into the free troposphere, and this can lead to a decrease in the aerosol loading within the ABL, as reported by e.g. He et al. (2008) and Tian et al. (2017). The emission of PM10 in summer is lower than in other seasons in Warsaw (Zawadzka et al., 2013). Even lower urban emissions at night reduce the mass concentrations of surface PM10, and the aerosol properties within ABL are concurrently relatively stable due to the stable boundary layer at nighttime. Therefore, no apparent relationship can be observed during the nocturnal period.
Interrelations of optical properties within the ABL are given in Fig. 8. A positive correlation between the AODABL and LRABL is observed for all times, which is higher for sunrise and sunset (0.64–0.72) and lower for the nocturnal time (∼ 0.56). The AODABL and LRABL depend on the extinction coefficient derived within the ABL; thus, both values will increase when the fine particle contribution increases and/or when there is an increase in the absorption capability of the particles within the ABL, and vice versa. This may be partly due to the presence of biomass-burning particles inside the ABL, as reported e.g. in Stachlewska et al. (2018).
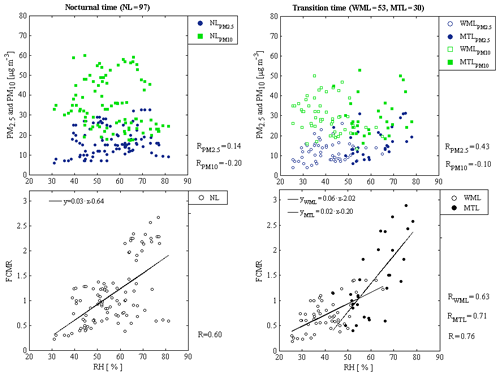
Figure 9Hourly averaged near-surface relative humidity (RH) measured by the WXT510 (Vaisala) weather transmitter at the RS-Lab in Warsaw for the July–September period of 2013, 2015, and 2016 vs. surface particulate matter (PM2.5 and PM10) measured at the WIOS site in Ursynow, Warsaw. The linear fit to data points is shown for correlation coefficients (R) greater than 0.6.
The relation between the ÅEABL and LRABL shows a weak negative trend during the analysed period, which may be due to larger size particles being injected into the ABL, particles growing in the ABL, or the smoke contribution in the composition of the ABL aerosol. As for the latter, the presence of smoke particles results in high negative correlations, e.g. correlations between LRABL and ÅEABL of −0.79 (Giannakaki et al., 2010) and −0.84 (Amiridis et al., 2009) were found for smoke particles. Moreover, Stachlewska et al. (2018) showed negative correlation of ÅEABL and LRABL for smoke particles. An alternative explanation could be the condensation of large organic molecules and particle coagulation from the upper atmosphere into the ABL, as reported by e.g. Pósfai et al. (2004) and Fiebig et al (2003). Giannakaki et al. (2010), showed no significant correlation between ÅEABL and LRABL for continental and urban aerosol related to anthropogenic pollution. Moreover, Mattis et al. (2004) reported no relationship between ÅEABL and LRABL when anthropogenic particles dominated in Leipzig. The results obtained in the current paper suggest that a mixture of local urban anthropogenic aerosol with a natural source aerosol (local or long-range transported into ABL) during summer and early autumn in Warsaw, might be a reasonable explanation for the weak negative tendency of ÅEABL and LRABL observed.
4.3 Relations of optical properties, surface PM, and relative humidity in summer and early autumn over Warsaw
Relations between the near-surface relative humidity (RH) and surface PM10 and PM2.5 and FCMR, and relations between the near-surface RH and the lidar-derived aerosol properties (AODABL ÅEABL, LRABL and δABL) were investigated for the entire, nocturnal, and sunrise/sunset times. Additionally, the nighttime relations between the lidar-derived water vapour mixing ratios (WVABL) and the aforementioned quantities were investigated.
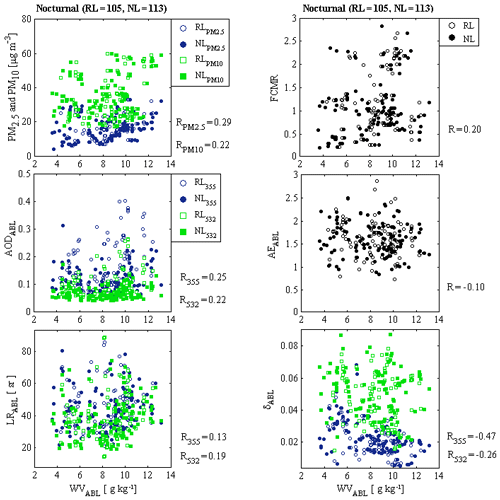
Figure 10Nighttime hourly averaged water vapour mixing ratio (WV) vs. aerosol optical depth (AOD), lidar ratio (LR), Ångström exponent (ÅE), and linear particle depolarization ratio (δ), all derived within the atmospheric boundary layer from the PollyXT lidar at the RS-Lab, at the EARLINET/ACTRIS site in Warsaw, and vs. the fine-to-coarse mass ratio (FCMR) and surface particulate matter (PM2.5 and PM10) measured at the WIOS site in Ursynow, Warsaw, during the July–September period of 2013, 2015, and 2016. Note that the lidar-derived WV is only available at nighttime.
Generally, as seen in Fig. 9, a weak positive trend of RH and PM2.5 is in agreement with the results of Sharma et al. (2017). The RH and FCMR exhibit a positive correlation, with correlation coefficients of 0.6 for the NT, 0.63 for the WML, and 0.71 for the MTL. Zhang et al. (2015) reported that high RH values can led to high PM2.5 in Beijing. In the summer urban environment, Li et al. (2017) showed that an increase of the RH can lead to growth of fine particles (PM2.5), but not to a growth of PM10 due to the hygroscopic effect on aerosol, and mainly attributed this to the effects of the wet scavenging under the high summer rainfall. The mean RH obtained in the current study was highest for the MTL at 63±10 %, was 57±12 % for the NL, and was lowest for the WML at 43±10 %. Small particles have a greater possibility to aggregate into relatively large particles at nighttime; thus, the lower correlation coefficients for RH and PM2.5 (and FCMR) are found for the nocturnal time.
Figure 10 presents no clear relation of WVABL with surface PM2.5, PM10, and FCMR at nighttime in Warsaw. The correlation coefficient of WVABL and PM2.5 is a little higher than WVABL and PM10, indicating that the water vapour in the ABL can affect the surface fine particles more than the surface coarse particles at night. This could be due to the presence of anthropogenic particles in the ABL, as these hygroscopic particles can absorb water vapour and gradually increase in size, although the growth of particles due to particle coagulation within the ABL cannot be excluded (Fiebig et al., 2003).
Figure 10 also does not depict a clear relation between WVABL and AODABL or ÅEABL and LRABL – only δABL and WVABL show a negative trend. The hygroscopicity of particles increases with decreasing particle size (Petters et al., 2009). At the same time, the more fine particles the lower the depolarization (see Fig. 7). Hence, an increase in water vapour and the presence of hygroscopic particles leads to a decrease in depolarization. When biomass-burning aerosol that has undergone long-range transport occurs over Warsaw, hygroscopic effects can be well captured with quasi-continuous profiling of the water vapour (Stachlewska et al., 2017a). Note that the relation of the AODABL, ÅEABL, and LRABL with the surface RH also does not show much of a trend for the NL, although for the WML the negative trends and property groupings becomes more visible (Fig. 11).
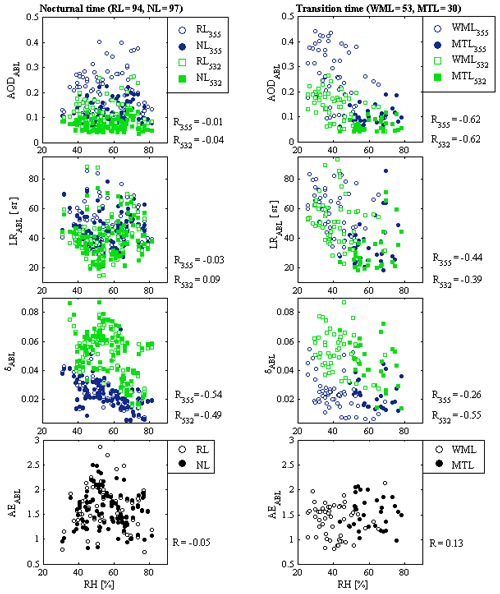
Figure 11Hourly averaged near-surface relative humidity (RH) measured by the WXT510 (Vaisala) weather transmitter at the RS-Lab, Warsaw, vs. the aerosol optical depth (AOD), the lidar ratio (LR), the linear particle depolarization ratio (δ), and the Ångström exponent (ÅE) derived within the boundary layer at 355 and 532 nm from the PollyXT lidar at the RS-Lab, at the EARLINET/ACTRIS site in Warsaw, for the July–September period of 2013, 2015, and 2016.
The AODCL was reported to increase with an increase in the ambient relative humidity for the hygroscopic particles due to hygroscopic growth (Bergin et al., 2000; Altaratz et al., 2013). In Fig. 11, a negative relation between AODABL and surface RH is found (with a higher surface RH, a lower AODABL is observed), except for during the nocturnal period (no trend in Fig. 11, similar to Fig. 10 for AODABL and WVABL). The size and optical properties can change as aerosol particles take up water and a higher RH is more favourable for hygroscopic growth of pollution particles (Tang, 1996). During the observational period, urban anthropogenic aerosol was observed most frequently within the boundary layer. An increase in RH led to an increase in the pollution particle size, which was visible at nighttime (ÅE and RH show slight negative trend). A slight positive trend between ÅEABL and RH is seen at transition times (different grouping in the moister MTL and drier WML), which is in accordance with the FCMR and ÅE scatterplots (more coarse particles in the MTL and more fine particles in the WML). Pollution particles within the boundary layer, due to a weaker convective mixing at nighttime, are prone to water uptake, which contributes to an increase in the aerosol particle size Cheng et al. (2008). The relation between LRABL and RH shows practically no correlation during the nocturnal time, but it is well separated in the transition time (a lower LR for the MTL). At nighttime, an increasing LR due to the accumulation of hygroscopic smoke particles was reported by Giannkaki et al. (2010). The convection and the energy exchange is stronger in the transition time (Stull, 1988), leading to anthropogenic aerosol being dominant in the atmospheric boundary layer. Increasing the surface RH results in a rise in the aerosol particles' size, and thus contributes to a decrease in the LRABL. The negative trend in the relation between δABL and the surface RH (clearly visible during the nocturnal time) is in agreement with the trend obtained for WVABL and δABL.
This study focuses on the optical properties derived within the atmospheric boundary layer, based on a dataset comprising measurements from July to September in 2013, 2015, and 2016 from the EARLINET/ACTRIS site in Warsaw. Interrelations between the different optical properties within ABL as well as relations between the optical properties and the surface PM concentrations and RH measurements were studied by conducting comparisons of various parameters during different periods: the entire time (24 h, ABL), nighttime (21:00–02:00 UTC; NL and RL), sunrise (03:00–08:00 UTC; MTL), and sunset (16:00–20:00 UTC; WML).
At both wavelengths, AODABL and LRABL at sunset were found to be higher (0.14–0.24 for AODABL and 49–55 sr for LRABL) than for the other two periods (0.06–0.18 for AODABL and 32–47 sr for LRABL). During the nocturnal period, ÅEABL values were higher (1.58±0.36 for NL, 1.61±0.37 for RL) than for the two remaining periods (1.53±0.30 for MTL, 1.37±0.34 for WML). For the entire dataset, the AEABL was distributed between 1 and 2 (> 90 % of data points) and the surface FCMR ranged between 0.5 and 1.5 (∼ 70 % of data points). The aerosol composition within the ABL in summer and early autumn in Warsaw consisted of urban anthropogenic pollution and its mixtures with local pollen and long-range transported biomass-burning aerosol and Arctic marine particles.
The boundary layer AODABL contribution to the columnar AODCL was assessed. The latter was more than twice as high when optically thick aerosol layers were observed above the ABL, and less than twice as high for cases with no aerosol layers in the free troposphere but with the presence of pollution aloft due to convection.
The AODABL and ABLH exhibit a positive correlation (∼ 0.75) during the observation period, which is highest for the WML and MTL. When the ABLH increases, a declining trend of the FCMR is observed for the WML, indicating an increase in the coarse particle fraction. However, there is no clearly apparent link between PM10 or PM2.5 and the ABLH. For the MTL, the ranges of ÅEABL and FCMR values obtained are higher than for the WML. A negative correlation of δABL and the FCMR was found for all time periods, which indicates the higher sphericity of fine particles. As reported in literature, different correlations obtained for AODABL and PM10 are attributed to complicated atmospheric and weather conditions. In summer and early autumn in Warsaw, high-pressure systems generally govern the dynamics of the atmosphere, there is significantly less traffic pollution (people on holidays, on bicycles), and the pollination of plants also plays a role.
The relation between AODABL and FCMR reported here displays a negative correlation at sunrise and sunset, which can be related to the traffic peaks. Due to lower urban emissions (no traffic nor domestic heating in summer) and a stable boundary layer, no apparent relationship between AODABL and the FCMR was observed at night. The AODABL and LRABL depend on the extinction coefficient within the ABL – thus, a positive correlation is observed. The relation of ÅEABL and LRABL reveals a weak negative trend. The positive trends of the RH and FCMR were found at night and for sunrise and sunset, although the sunrise trend was most pronounced.
The weak positive relation at night for WVABL and PM2.5 is higher than for PM10, indicating that the water vapour in the NL and RL affects surface fine particles more than surface coarse particles. The increasing WVABL (and RH at the surface) can lead to a decrease in depolarization (both relations are very similar at night) in the presence of hygroscopic particles. For high near-surface RH, lower AODABL values were derived in the MTL and WML, which was not seen in the NL and RL. A negative trend of δABL and WVABL and of δABL and RH is due to the hygroscopicity of particles. A negative relation between AODABL and surface RH is found for the transition time (in both the MTL and WML), which is followed by a weak negative correlation of LRABL and RH observed only during sunset (in the WML).
The co-located measurements conducted in Warsaw with the MFR-7 shadowband radiometer (PolandAOD-NET) and the CE318 sun photometer (AERONET) showed strong agreement with a correlation coefficient (R) of 0.98 and a standard deviation of 0.02 at both 380 and 500 nm.
The results obtained increase the current knowledge on the variability of optical properties within the summer and early-autumn atmospheric boundary layer at a continental urban site in central Europe. The relations found in previously published research, obtained using a case-study approach, do not necessarily apply and are not necessarily seen in the long-term study. Therefore, special care should be taken when interpreting and comparing the different results.
The bottom line is that regular, automated observations of the next generation PollyXT lidar conducted at the EARLINET site in the framework of the ACTRIS infrastructure activities allow for such studies. The excellent capabilities of this lidar enabled the combination of the results derived within this study with other data sources (e.g. AERONET, WIOS, and PolandAOD networks). Hypotheses regarding boundary layer aerosol properties interrelations were proposed and will be further verified using additional lidar data from regular observations in Warsaw. The expansion of the existing high quality lidar data sample would allow for an improvement in the investigation of the subgrouping of aerosol properties, which could provide statistically significant correlations. Moreover, more observations would allow for an extension of the investigation of differences to other seasons, would enable daytime analyses, and would aid with better distinguishing between sunset and sunrise aerosol property relationships; furthermore, a separation of aerosol properties accordingly to aerosol content, i.e. urban aerosol vs. urban aerosol mixtures with other aerosol types, would be possible (currently too few data exist in the mixed categories), followed by an estimation of their radiative effect.
The lidar data used in this study are available upon registration from http://data.earlinet.org (last access: 15 October 2019). The PM2.5 and PM10 data are publicly accessible via the data archive of the National Chief Inspectorate for Environmental Protection (GIOS) at http://powietrze.gios.gov.pl/pjp/archive (last access: 15 October 2019). The sun photometer AOD data are publicly available via the AERONET data website at https://AERONETl.gsfc.nasa.gov (last access: 15 October 2019). The shadowband radiometer AOD data and the surface RH data are available via the Polish aerosol Research Network PolandAOD-NET website at https://polandaod.pl (last access: 15 October 2019). The radiosounding profiles are available via the University of Wyoming Upperair Air Data website at http://weather.uwyo.edu/upperair/sounding.htm (last access: 15 October 2019).
Entire time | ET |
Nocturnal time | NT |
Transition rime | TT |
Atmospheric boundary layer (derived by lidar) | ABL |
Atmospheric boundary layer height (derived by lidar) | ABLH |
Residual layer (derived by lidar) | RL |
Nocturnal layer (derived by lidar) | NL |
Morning transition layer (derived by lidar) | MTL |
Well-mixed layer (derived by lidar) | WML |
Particle extinction coefficient (within the atmospheric boundary layer) | αABL |
Particle backscatter coefficient (within the atmospheric boundary layer) | βABL |
Aerosol optical depth (within the atmospheric boundary layer, derived by lidar) | AODABL(λ) |
Aerosol optical depth (columnar, derived by sun photometer or radiometer) | AODCL(λ) |
Lidar ratio (within the atmospheric boundary layer) | LRABL(λ) |
Linear particle depolarization ratio (within the atmospheric boundary layer) | δABL(λ) |
Ångström exponent (within the atmospheric boundary layer, derived by lidar) | ÅEABL(λ1∕λ2) |
Ångström exponent (columnar, derived by sun photometer or radiometer) | ÅECL(λ1∕λ2) |
Water vapour mixing ratio (within the atmospheric boundary layer) | WVABL |
Relative humidity (at the near-surface) | RH |
Particulate matter with diameter < 10 µm; < 2.5 µm | PM10; PM2.5 |
Fine to coarse mass ratio | FCMR |
Wavelength | λ |
ISS wrote the paper, obtained funding for the research, came up with the study approach, designed the methodology, performed the experiment, contributed to the development of the PollyXT lidar (Warsaw) and the lidar evaluation algorithms, took care of the quality assurance of lidar measurements and data products, and holistically interpreted the lidar results with respect to other data sources' results (AERONET, PolandAOD-NET, and WIOS Monitoring Network). DS was responsible for the calculation of the aerosol optical property profiles (α, β, and δ) and their categorization for the EARLINET/ACTRIS database. DW was responsible for an extensive literature review, wrote the codes for the ABLH and the WV retrieval algorithms, and performed statistical analysis of the optical properties (ÅE, LR, δ, and WV). All authors contributed to revisions of the paper.
The authors declare that they have no conflict of interest.
This article is part of the special issue “EARLINET aerosol profiling: contributions to atmospheric and climate research”. It is not associated with a conference.
This research was mainly carried out in the frame of the Technical assistance for Polish Radar and Lidar Mobile Observation System (POLIMOS) project funded by ESA-ESTEC. The PollyXT lidar (Warsaw) was developed via a scientific collaboration between the Faculty of Physics at the University of Warsaw (FUW) and the Institute of Tropospheric Research (TROPOS). The development was financed by the Polish Foundation of Science and Technology (FNTP). We especially acknowledge members of the PollyXT lidar group lead by Dietrich Althausen. The authors acknowledge the Warsaw Regional Inspectorate for Environmental Protection (WIOS) of the National Chief Inspectorate for Environmental Protection (GIOS) for the provision of the processed and quality assured PM2.5 and PM10 data. We acknowledge Brent Holben for processing the AERONET data; Aleksander Pietruczuk and Piotr Sobolewski of the Institute of Geophysics at the Polish Academy of Sciences for performing the AERONET measurements in Belsk; Wojciech Kumala of the Faculty of Physics at the University of Warsaw (FUW) for performing the AERONET measurements in Warsaw; Phillippe Golub for performing instrument calibrations at the PHOTONS/AERONET-EUROPE calibration centre. The AERONET sun photometer at the RS-Lab site in Warsaw is the property of the National Institute of Research and Development for Optoelectronics (INOE 2000) in Romania, and was kindly lent by the research group led by Doina Nicolae, within the framework of the SAtellite based Monitoring Initiative for Regional Air quality (SAMIRA) funded by ESA-ESRIN. We acknowledge Krzysztof Markowicz for processing the MFR-7 data within the Polish aerosol Research Network PolandAOD-NET. The purchase of the MFR-7 instrument was funded by the Polish Ministry of Science and High Education. The EARLINET/AERONET site in Warsaw established at RS-Lab at Faculty of Physics, University of Warsaw (FUW) acknowledges the vital support of the ACTRIS Research Infrastructure in developing the site.
This research has been supported by the European Space Agency ESA-ESTEC (grant no. 4000119961/16/NL/FF/mg), the Polish Foundation of Science and Technology (grant no. 519/FNITP/115/2010), the Polish Ministry of Science and High Education (grant no. 1283/B/P01/2010/38), and the European Space Agency ESA-ESRIN (grant no. 4000117393/16/INB). The EARLINET activities have been supported by the ACTRIS project, funded within the European Commission Seventh Framework Programme under the research infrastructures for atmospheric research (grant agreement no. 262254); the ACTRIS-2 project, funded by the European Union Research Infrastructures action under the H2020 specific programme for integrating and opening existing national and regional research infrastructures of European interest (grant agreement no. 654109); and the ACTRIS PPP 65 project supported by the European Commission under the Horizon 2020 – Research and Innovation Framework Programme, H2020INFRADEV-2016-2017 (grant agreement no. 739530).
This paper was edited by Lucia Mona and reviewed by three anonymous referees.
Altaratz, O., Bar-Or, R. Z., Wollner, U., and Koren, I.: Relative humidity and its effect on aerosol optical depth in the vicinity of convective clouds, Environ. Res. Lett., 8, 034025, https://doi.org/10.1088/1748-9326/8/3/034025, 2013.
Alados-Arboledas, L., Müller, D., Guerrero-Rascado, J., Navas-Guzmán, F., Pérez-Ramírez, D., and Olmo, F.: Optical and microphysical properties of fresh biomass burning aerosol retrieved by Raman lidar, and star- and sun-photometry, Geophys. Res. Lett., 38, L01807, https://doi.org/10.1029/2010GL045999, 2011.
Amiridis, V., Balis, D., Kazadzis, S., Bais, A., Giannakaki, E., Papayannis, A., and Zerefos, C.: Four-year aerosol observations with a Raman lidar at Thessaloniki, Greece, in the framework of European Aerosol Research Lidar Network (EARLINET), J. Geophys. Res., 110, D21203,https://doi.org/10.1029/2005JD006190, 2005.
Amiridis, V., Balis, D. S., Giannakaki, E., Stohl, A., Kazadzis, S., Koukouli, M. E., and Zanis, P.: Optical characteristics of biomass burning aerosols over Southeastern Europe determined from UV-Raman lidar measurements, Atmos. Chem. Phys., 9, 2431–2440, https://doi.org/10.5194/acp-9-2431-2009, 2009.
Ångström, A.: On the atmospheric transmission of sun radiation and on dust in the air, Geogr. Ann., 12, 156–166, https://doi.org/10.1080/20014422.1929.11880498, 1929.
Ansmann, A., Tesche, M., Knippertz, P., Bierwirth, E., Althausen, D., Mueller, D., and Schulz, O.: Vertical profiling of convective dust plumes in Southern Morocco during SAMUM, Tellus B, 61, 340–353, https://doi.org/10.1111/j.1600-0889.2008.00384.x, 2009.
Ansmann, A., Baars, H., Chudnovsky, A., Mattis, I., Veselovskii, I., Haarig, M., Seifert, P., Engelmann, R., and Wandinger, U.: Extreme levels of Canadian wildfire smoke in the stratosphere over central Europe on 21–22 August 2017, Atmos. Chem. Phys., 18, 11831–11845, https://doi.org/10.5194/acp-18-11831-2018, 2018.
Baars, H., Kanitz, T., Engelmann, R., Althausen, D., Heese, B., Komppula, M., Preißler, J., Tesche, M., Ansmann, A., Wandinger, U., Lim, J.-H., Ahn, J. Y., Stachlewska, I. S., Amiridis, V., Marinou, E., Seifert, P., Hofer, J., Skupin, A., Schneider, F., Bohlmann, S., Foth, A., Bley, S., Pfüller, A., Giannakaki, E., Lihavainen, H., Viisanen, Y., Hooda, R. K., Pereira, S. N., Bortoli, D., Wagner, F., Mattis, I., Janicka, L., Markowicz, K. M., Achtert, P., Artaxo, P., Pauliquevis, T., Souza, R. A. F., Sharma, V. P., van Zyl, P. G., Beukes, J. P., Sun, J., Rohwer, E. G., Deng, R., Mamouri, R.-E., and Zamorano, F.: An overview of the first decade of PollyNET: an emerging network of automated Raman-polarization lidars for continuous aerosol profiling, Atmos. Chem. Phys., 16, 5111–5137, https://doi.org/10.5194/acp-16-5111-2016, 2016.
Baars, H., Ansmann, A., Ohneiser, K., Haarig, M., Engelmann, R., Althausen, D., Hanssen, I., Gausa, M., Pietruczuk, A., Szkop, A., Stachlewska, I. S., Wang, D., Reichhardt, J., Skupin, A., Mattis, I., Trickl, T., Vogelmann, H., Navas-Guzmán, F., Haefele, A., Acheson, K., Ruth, A. A., Tatarov, B., Müller, D., Hu, Q., Podvin, T., Goloub, P., Vesselovski, I., Pietras, C., Haeffelin, M., Fréville, P., Sicard, M., Comerón, A., Fernández García, A. J., Molero Menéndez, F., Córdoba-Jabonero, C., Guerrero-Rascado, J. L., Alados-Arboledas, L., Bortoli, D., Costa, M. J., Dionisi, D., Liberti, G. L., Wang, X., Sannino, A., Papagiannopoulos, N., Boselli, A., Mona, L., D'Amico, G., Romano, S., Perrone, M. R., Belegante, L., Nicolae, D., Grigorov, I., Gialitaki, A., Amiridis, V., Soupiona, O., Papayannis, A., Mamouri, R.-E., Nisantzi, A., Heese, B., Hofer, J., Schechner, Y. Y., Wandinger, U., and Pappalardo, G.: The unprecedented 2017–2018 stratospheric smoke event: Decay phase and aerosol properties observed with EARLINET, Atmos. Chem. Phys. Discuss., https://doi.org/10.5194/acp-2019-615, in review, 2019.
Barlage, M., Miao, S., and Chen, F.: Impact of physics parameterizations on high-resolution weather prediction over two Chinese megacities, J. Geophys. Res., 121, 4487–4498, https://doi.org/10.1002/2015JD024450, 2016.
Bennouna, Y., Cachorro, V. E., Mateos, D., Burgos, M. A., Toledano, C., Torres, B., and de Frutos, A.: Long-term comparative study of columnar and surface mass concentration aerosol properties in a background environment, Atmos. Environ., 140, 261–272, https://doi.org/10.1016/j.atmosenv.2016.05.061, 2016.
Béghein C., Allery C., Wacławczyk C., and Pozorski J.: Application of POD-based dynamical systems to dispersion and deposition of particles in turbulent channel flow, Int. J. Multiphase Flow, 58, 97–113, https://doi.org/10.1016/j.ijmultiphaseflow.2013.09.001, 2014.
Bergin, M. H., Schwartz, S. E., Halthore, R. N., Ogren, J. A., and Hlavka, D. L.: Comparison of aerosol optical depth inferred surface measurements with that determined by Sun photometry for cloud-free conditions at a continental US site, J. Geophys. Res., 105, 6807–6816, https://doi.org/10.1029/1999JD900454, 2000.
Binietoglou, I., Basart, S., Alados-Arboledas, L., Amiridis, V., Argyrouli, A., Baars, H., Baldasano, J. M., Balis, D., Belegante, L., Bravo-Aranda, J. A., Burlizzi, P., Carrasco, V., Chaikovsky, A., Comerón, A., D'Amico, G., Filioglou, M., Granados-Muñoz, M. J., Guerrero-Rascado, J. L., Ilic, L., Kokkalis, P., Maurizi, A., Mona, L., Monti, F., Muñoz-Porcar, C., Nicolae, D., Papayannis, A., Pappalardo, G., Pejanovic, G., Pereira, S. N., Perrone, M. R., Pietruczuk, A., Posyniak, M., Rocadenbosch, F., Rodríguez-Gómez, A., Sicard, M., Siomos, N., Szkop, A., Terradellas, E., Tsekeri, A., Vukovic, A., Wandinger, U., and Wagner, J.: A methodology for investigating dust model performance using synergistic EARLINET/AERONET dust concentration retrievals, Atmos. Meas. Tech., 8, 3577–3600, https://doi.org/10.5194/amt-8-3577-2015, 2015.
Böckmann, C., Wandinger, U., Ansmann, A., Bösenberg, J., Amiridis, V., Boselli, A., Delaval, A., De Tomasi, F., Frioud, M., and Grigorov, I. V.: Aerosol lidar intercomparison in the framework of the EARLINET project. 2. Aerosol backscatter algorithms, Appl. Opt., 43, 977–989, https://doi.org/10.1364/AO.43.000977, 2004.
Böckmann, C., Mironova, I., Müller, D., Schneidenbach, L., and Nessler, R.: Microphysical aerosol parameters from multiwavelength lidar, J. Opt. Soc. Am. A, 22, 518–528, https://doi.org/10.1364/JOSAA.22.000518, 2005.
Bonn, B., von Schneidemesser, E., Andrich, D., Quedenau, J., Gerwig, H., Lüdecke, A., Kura, J., Pietsch, A., Ehlers, C., Klemp, D., Kofahl, C., Nothard, R., Kerschbaumer, A., Junkermann, W., Grote, R., Pohl, T., Weber, K., Lode, B., Schönberger, P., Churkina, G., Butler, T. M., and Lawrence, M. G.: BAERLIN2014 – the influence of land surface types on and the horizontal heterogeneity of air pollutant levels in Berlin, Atmos. Chem. Phys., 16, 7785–7811, https://doi.org/10.5194/acp-16-7785-2016, 2016.
Burton, S. P., Ferrare, R. A., Hostetler, C. A., Hair, J. W., Rogers, R. R., Obland, M. D., Butler, C. F., Cook, A. L., Harper, D. B., and Froyd, K. D.: Aerosol classification using airborne High Spectral Resolution Lidar measurements – methodology and examples, Atmos. Meas. Tech., 5, 73–98, https://doi.org/10.5194/amt-5-73-2012, 2012.
Burton, S. P., Hair, J. W., Kahnert, M., Ferrare, R. A., Hostetler, C. A., Cook, A. L., Harper, D. B., Berkoff, T. A., Seaman, S. T., Collins, J. E., Fenn, M. A., and Rogers, R. R.: Observations of the spectral dependence of linear particle depolarization ratio of aerosols using NASA Langley airborne High Spectral Resolution Lidar, Atmos. Chem. Phys., 15, 13453–13473, https://doi.org/10.5194/acp-15-13453-2015, 2015.
Chen, B. and Kan, H.: Air pollution and population health: A global challenge, Environ. Health Prev. Med., 13, 94–101, https://doi.org/10.1007/s12199-007-0018-5, 2008.
Cheng, Y. F., Wiedensohler, A., Eichler, H., Heintzenberg, J., Tesche, M., Ansmann, A., Wendisch, M., Su, H., Althausen, D., Herrmann, H., Gnauk, T., Brüggemann, E., Hu, M., and Zhang, Y. H.: Relative humidity dependence of aerosol optical properties and direct radiative forcing in the surface boundary layer at Xinken in Pearl River Delta of China: An observation based numerical study, Atmos. Environ., 42, 6373–6397, https://doi.org/10.1016/j.atmosenv.2008.04.009, 2008.
Chilinski, M. T., Markowicz, K. M., Zawadzka, O., Stachlewska, I. S., Kumala, W., Petelski, T., Makuch, P., Westphal, D. L., and Zagajewski, B.: Modelling and Observation of Mineral Dust Optical Properties over Central Europe, Acta Geophys., 64, 2550–2590, https://doi.org/10.1515/acgeo-2016-0069, 2016.
Comerón, A., Sicard, M., and Rocadenbosch, F.: Wavelet Correlation Transform Method and Gradient Method to Determine Aerosol Layering from Lidar Returns: Some Comments, J. Atmos. Ocean. Tech., 30, 1189–1193, https://doi.org/10.1175/JTECH-D-12-00233.1, 2013.
Costa-Surós, M., Stachlewska, I. S., Nemuc, A., Talianu, C., Heese, B., and Engelmann, R.: Study case of air-mass modification over Poland and Romania observed by the means of multiwavelength Raman depolarization lidars, 27th International Laser Radar Conference, New York, USA, 5–10 July 2015, 1–4, 2015.
Dang, R., Yang, Y., Hu, X.-M., Wang, Z., and Zhang, S.: A Review of Techniques for Diagnosing the Atmospheric Boundary Layer Height (ABLH) Using Aerosol Lidar Data, Remote Sens., 11, 1590, https://doi.org/10.3390/rs11131590, 2019.
Dawson, K. W., Meskhidze, N., Josset, D., and Gassó, S.: Spaceborne observations of the lidar ratio of marine aerosols, Atmos. Chem. Phys., 15, 3241–3255, https://doi.org/10.5194/acp-15-3241-2015, 2015.
Delanoë, J. and Hogan, R. J.: A variational scheme for retrieving ice cloud properties from combined radar, lidar, and infrared radiometer, J. Geophys. Res., 113, D07204, https://doi.org/10.1029/2007JD009000, 2008.
De Leeuw, F., Sluyter, R., van Breugel, P., and Bogman, F.: Air Pollution by ozone in Europe in 1999 and the summer of 2000, European Environmental Agency Topic Report number 1/2001, EEA, Copenhagen, Denmark, 2001.
Di Biagio, C., Pelon, J., Ancellet, G., Bazureau, A., and Mariage, V.: Sources, load, vertical distribution, and fate of wintertime aerosol north of Svalbard from combined V4 CALIOP data, ground-based IAOOS lidar observations and trajectory analysis, J. Geophys. Res.-Atmos., 123, 1363–1383, https://doi.org/10.1002/2017JD027530, 2018.
Dörnbrack, A., Stachlewska, I. S., Ritter, C., and Neuber, R.: Aerosol distribution around Svalbard during intense easterly winds, Atmos. Chem. Phys., 10, 1473–1490, https://doi.org/10.5194/acp-10-1473-2010, 2010.
Du, C., Liu, S., Yu, X., Li, X., Chen, C., Peng, Y., Dong, Y., Dong, Z., and Wang, F.: Urban boundary layer height characteristics and relationship with particulate matter mass concentrations in Xi'an, Central China, aerosol, Air Qual. Res., 13, 1598–1607, https://https://doi.org/10.4209/aaqr.2012.10.0274, 2013.
Engelmann, R., Kanitz, T., Baars, H., Heese, B., Althausen, D., Skupin, A., Wandinger, U., Komppula, M., Stachlewska, I. S., Amiridis, V., Marinou, E., Mattis, I., Linné, H., and Ansmann, A.: The automated multiwavelength Raman polarization and water-vapor lidar PollyXT: the neXT generation, Atmos. Meas. Tech., 9, 1767–1784, https://doi.org/10.5194/amt-9-1767-2016, 2016.
Fan, J., Wang, Y., Rosenfeld, D., and Liu, X.: Review of aerosol cloud interactions: Mechanisms, significance, and challenges, J. Atmos. Sci., 73, 4221–4252, https://doi.org/10.1175/JAS-D-16-0037.1, 2016.
Feingold, G., McComiskey, A., Yamaguchi, T., Johnson, J., Carslaw, K., and Schmidt, K. S.: New approaches to quantifying aerosol influence on the cloud radiative effect, P. Nat. Acad. Sci. USA, 113, 5812–5819, https://doi.org/10.1073/pnas.1514035112, 2016.
Fiebig, M., Stohl, A., Wendisch, M., Eckhardt, S., and Petzold, A.: Dependence of solar radiative forcing of forest fire aerosol on ageing and state of mixture, Atmos. Chem. Phys., 3, 881–891, https://doi.org/10.5194/acp-3-881-2003, 2003.
Filip, L. and Stefan, S.: Study of the correlation between the near-ground PM10 mass concentration and the aerosol optical Depth, J. Atmos. Sol.-Terr. Phys., 73, 1883–1889, https://doi.org/10.1016/j.jastp.2011.04.027, 2011.
Flentje, H., Heese, B., Reichardt, J., and Thomas, W.: Aerosol profiling using the ceilometer network of the German Meteorological Service, Atmos. Meas. Tech. Discuss., 3, 3643–3673, https://doi.org/10.5194/amtd-3-3643-2010, 2010.
Freudenthaler, V., Esselborn, M., Wiegner, M., Heese, B., Tesche, M., Ansmann, A., Müller, D., Althausen, D., Wirth, M., Fix, A., Ehret, G., Knippertz, P., Toledano, C., Gasteiger, J., Garhammer, M., and Seefeldner, M.: Depolarization ratio profiling at several wavelengths in pure Saharan dust during SAMUM 2006, Tellus B, 61, 165–179, https://https://doi.org/10.1111/j.1600-0889.2008.00396.x, 2009.
Freudenthaler, V., Linné, H., Chaikovski, A., Rabus, D., and Groß, S.: EARLINET lidar quality assurance tools, Atmos. Meas. Tech. Discuss., https://doi.org/10.5194/amt-2017-395, in review, 2018.
Foth, A., Kanitz, T., Engelmann, R., Baars, H., Radenz, M., Seifert, P., Barja, B., Fromm, M., Kalesse, H., and Ansmann, A.: Vertical aerosol distribution in the southern hemispheric midlatitudes as observed with lidar in Punta Arenas, Chile (53.2∘ S and 70.9∘ W), during ALPACA, Atmos. Chem. Phys., 19, 6217–6233, https://doi.org/10.5194/acp-19-6217-2019, 2019.
Fuzzi, S., Baltensperger, U., Carslaw, K., Decesari, S., Denier van der Gon, H., Facchini, M. C., Fowler, D., Koren, I., Langford, B., Lohmann, U., Nemitz, E., Pandis, S., Riipinen, I., Rudich, Y., Schaap, M., Slowik, J. G., Spracklen, D. V., Vignati, E., Wild, M., Williams, M., and Gilardoni, S.: Particulate matter, air quality and climate: lessons learned and future needs, Atmos. Chem. Phys., 15, 8217–8299, https://doi.org/10.5194/acp-15-8217-2015, 2015.
Gasteiger, J. and Freudenthaler, V.: Benefit of depolarization ratio at λ = 1064 nm for the retrieval of the aerosol microphysics from lidar measurements, Atmos. Meas. Tech., 7, 3773–3781, https://doi.org/10.5194/amt-7-3773-2014, 2014.
Gayatri, K., Patade, S., and Prabha, T. V.: Aerosol–Cloud interaction in deep convective clouds over the Indian Peninsula using spectral (bin) microphysics, J. Atmos. Sci., 74, 3145–3166, https://doi.org/10.1175/JAS-D-17-0034.1, 2017.
Geiß, A., Wiegner, M., Bonn, B., Schäfer, K., Forkel, R., von Schneidemesser, E., Münkel, C., Chan, K. L., and Nothard, R.: Mixing layer height as an indicator for urban air quality?, Atmos. Meas. Tech., 10, 2969–2988, https://doi.org/10.5194/amt-10-2969-2017, 2017.
Giannakaki, E., Balis, D. S., Amiridis, V., and Zerefos, C.: Optical properties of different aerosol types: seven years of combined Raman-elastic backscatter lidar measurements in Thessaloniki, Greece, Atmos. Meas. Tech., 3, 569–578, https://doi.org/10.5194/amt-3-569-2010, 2010.
Ghan, S. J., Wang, M., Zhang, S., Ferrachat, S., Gettleman, A., Griesfeller, J., Kipling, Z., Lohmann, U., Morrison, H., Neubauer, D., Partridge, D. G., Stier, P., Takemura, T., Wang, H., and Zhang, K.: Challenges in constraining anthropogenic aerosol effects on cloud radiative forcing using present-day spatiotemporal variability, P. Natl. Acad. Sci. USA, 113, 5804–5811, https://doi.org/10.1073/pnas.1514036113, 2016.
Giles, D. M., Sinyuk, A., Sorokin, M. G., Schafer, J. S., Smirnov, A., Slutsker, I., Eck, T. F., Holben, B. N., Lewis, J. R., Campbell, J. R., Welton, E. J., Korkin, S. V., and Lyapustin, A. I.: Advancements in the Aerosol Robotic Network (AERONET) Version 3 database – automated near-real-time quality control algorithm with improved cloud screening for Sun photometer aerosol optical depth (AOD) measurements, Atmos. Meas. Tech., 12, 169–209, https://doi.org/10.5194/amt-12-169-2019, 2019.
Granados-Muñoz, M. J., Navas-Guzmán, F., Bravo-Aranda, J. A., Guerrero-Rascado, J. L., Lyamani, H., Valenzuela, A., Titos, G., Fernández-Gálvez, J., and Alados-Arboledas, L.: Hygroscopic growth of atmospheric aerosol particles based on active remote sensing and radiosounding measurements: selected cases in southeastern Spain, Atmos. Meas. Tech., 8, 705–718, https://doi.org/10.5194/amt-8-705-2015, 2015.
Groß, S., Tesche, M., Freudenthaler, V., Toledano, C., Wiegner, M., Ansmann, A., Althausen, D., and Seefeldner, M.: Characterization of Saharan dust, marine aerosol and mixtures of biomass-burning aerosol and dust by means of multi-wavelength depolarization and Raman lidar measurements during SAMUM 2, Tellus B, 63, 706–724, https://doi.org/10.1111/j.1600-0889.2011.00556.x, 2011.
Groß, S., Esselborn, M., Weinzierl, B., Wirth, M., Fix, A., and Petzold, A.: Aerosol classification by airborne high spectral resolution lidar observations, Atmos. Chem. Phys., 13, 2487–2505, https://doi.org/10.5194/acp-13-2487-2013, 2013.
Groß, S., Freudenthaler, V., Schepanski, K., Toledano, C., Schäfler, A., Ansmann, A., and Weinzierl, B.: Optical properties of long-range transported Saharan dust over Barbados as measured by dual-wavelength depolarization Raman lidar measurements, Atmos. Chem. Phys., 15, 11067–11080, https://doi.org/10.5194/acp-15-11067-2015, 2015.
Grund, C. J. and Eloranta, E. W.: University of Wisconsin High Spectral Resolution Lidar, Opt. Eng., 30, 6–12, 1991.
Guo, H., Wang, Y., and Zhang, H.: Characterization of criteria air pollutants in Beijing during 2014–2015, Environ. Res., 154, 334–344, https://doi.org/10.1016/j.envres.2017.01.029, 2017.
Guo, J.-P., Zhang, X.-Y., Che, H.-Z., Gong, S.-L., An, X., Cao, C.-X., Guang, J., Zhang, H., Wang, Y.-Q., Zhang, X.-C., Xue, M., and Li, X.-W.: Correlation between PM concentrations and aerosol optical depth in eastern China, Atmos. Environ., 43, 5876–5886, https://doi.org/10.1016/j.atmosenv.2009.08.026, 2009.
Haarig, M., Ansmann, A., Gasteiger, J., Kandler, K., Althausen, D., Baars, H., Radenz, M., and Farrell, D. A.: Dry versus wet marine particle optical properties: RH dependence of depolarization ratio, backscatter, and extinction from multiwavelength lidar measurements during SALTRACE, Atmos. Chem. Phys., 17, 14199–14217, https://doi.org/10.5194/acp-17-14199-2017, 2017.
Haarig, M., Ansmann, A., Baars, H., Jimenez, C., Veselovskii, I., Engelmann, R., and Althausen, D.: Depolarization and lidar ratios at 355, 532, and 1064 nm and microphysical properties of aged tropospheric and stratospheric Canadian wildfire smoke, Atmos. Chem. Phys., 18, 11847–11861, https://doi.org/10.5194/acp-18-11847-2018, 2018.
Haeffelin, M., Angelini, F., Morille, Y., Martucci, G., Frey, S., Gobbi, G. P., Lolli, S., O'Dowd, C. D., Sauvage, L., XuerefRémy, I., Wastine, B., and Feist, D. G: Evaluation of mixing height retrievals from automatic profiling lidars and ceilometers in view of future integrated networks in Europe, Bound.-Lay. Meteorol., 143, 49–75, https://doi.org/10.1007/s10546-011-9643-z, 2012.
Harrison, L., Michalsky, J., and Berndt, J.: Automated multifilter rotating shadow-band radiometer: An instrument for optical depth and radiation measurements, Appl. Opt., 33, 5118–5125, https://doi.org/10.1364/AO.33.005118, 1994.
He, Q., Li, C., Mao, J., Lau, A. K. H., and Chu, D.: Analysis of aerosol vertical distribution and variability in Hong Kong, J. Geophys. Res., 113, D14211, https://doi.org/10.1029/2008JD009778, 2008.
Heese, B. and Wiegner, M.: Vertical aerosol profiles from Raman polarization lidar observations during the dry season AMMA field campaign, J. Geophys. Res., 113, D00C11, https://doi.org/10.1029/2007JD009487, 2008.
Holben, B. N., Eck, T. F., Slutsker, I., Tanre, D., Buis, J. P., Setzer, A., Vermote, E., Reagan, J. A., Kaufman, Y. J., Nakjima, T., Lavenu, F., Jankowiak, I., and Smirnov, A.: AERONET – A federated instrument network and data archive for aerosol characterization, Remote Sens. Environ., 66, 1–16, https://doi.org/10.1016/S0034-4257(98)00031-5, 1998.
Horvath, H., Alados Arboledas, L., and Olmo Reyes, F. J.: Angular scattering of the Sahara dust aerosol, Atmos. Chem. Phys., 18, 17735–17744, https://doi.org/10.5194/acp-18-17735-2018, 2018.
Hu, Q., Goloub, P., Veselovskii, I., Bravo-Aranda, J.-A., Popovici, I. E., Podvin, T., Haeffelin, M., Lopatin, A., Dubovik, O., Pietras, C., Huang, X., Torres, B., and Chen, C.: Long-range-transported Canadian smoke plumes in the lower stratosphere over northern France, Atmos. Chem. Phys., 19, 1173–1193, https://doi.org/10.5194/acp-19-1173-2019, 2019.
Hutchison, K. D., Faruqui, S. J., and Smith, S.: Improving correlations between MODIS aerosol optical thickness and ground-based PM2.5 observations through 3D spatial analyses, Atmos. Environ., 42, 530–543, https://doi.org/10.1016/j.atmosenv.2007.09.050, 2008.
Iarlori, M., Madonna, F., Rizi, V., Trickl, T., and Amodeo, A.: Effective resolution concepts for lidar observations, Atmos. Meas. Tech., 8, 5157–5176, https://doi.org/10.5194/amt-8-5157-2015, 2015.
Illingworth, A. J., Barker, H. W., Beljaars, A., Ceccaldi, M., Chepfer, H., Clerbaux, N., Cole, J., Delanoë, J., Domenech, C., Donovan, D. P., Fukuda, S., Hirakata, M., Hogan, R. J., Huenerbein, A., Kollias, P., Kubota, T., Nakajima, T., Nakajima, T.Y., Nishizawa, T., Ohno, Y., Okamoto, H., Oki, R., Sato, K., Satoh, M., Shephard, M., Velázquez-Blázquez, A., Wandinger, U., Wehr, T., and van Zadelhoff, G.-J.: The EarthCARE Satellite: The next step forward in global measurements of clouds, aerosol, precipitation and radiation, B. Am. Meteorol. Soc., 96, 1311–1332, https://doi.org/10.1175/BAMS-D-12-00227.1, 2015.
Iqbal, M.: An introduction to solar radiation, Acadamec Press, Ontario, 1983.
Janicka, L., Stachlewska, I. S., Veselovskii, I., and Baars, H.: Temporal variations in optical and microphysical properties of mineral dust and biomass burning aerosol derived from daytime Raman lidar observations over Warsaw, Poland, Atmos. Environ., 169, 162–174, https://doi.org/10.1016/j.atmosenv.2017.09.022, 2017.
Juda-Rezler, K., Reizer, M., and Oudinet, J. P.: Determination and analysis of PM10 source apportionment during episodes of air pollution in Central Eastern European urban areas: The case of wintertime 2006, Atmos. Environ., 45, 6557–6566, https://doi.org/10.1016/j.atmosenv.2011.08.020, 2011.
Juda-Rezler, K., Reizer, M., Huszar, P., Krueger, B., Zanis, P., Syrakov, D., Katragkou, E., Trapp, W., Melas, D., Chervenkov, H., Tegoulias, I., and Halenka, T.: Modelling the effects of climate change on air quality over central and Eastern Europe: concept, evaluation and projections, Clim. Res., 53, 179–203, https://doi.org/10.3354/cr01072, 2012.
Jung, E., Albrecht, B. A., Feingold, G., Jonsson, H. H., Chuang, P., and Donaher, S. L.: Aerosols, clouds, and precipitation in the North Atlantic trades observed during the Barbados aerosol cloud experiment – Part 1: Distributions and variability, Atmos. Chem. Phys., 16, 8643–8666, https://doi.org/10.5194/acp-16-8643-2016, 2016.
Kaufman, Y. J., Tanré, D., and Boucher, O.: A satellite view of aerosol in the climate system, Nature, 419, 215–223, https://doi.org/10.1038/nature01091, 2002.
Kipling, Z., Stier, P., Johnson, C. E., Mann, G. W., Bellouin, N., Bauer, S. E., Bergman, T., Chin, M., Diehl, T., Ghan, S. J., Iversen, T., Kirkevåg, A., Kokkola, H., Liu, X., Luo, G., van Noije, T., Pringle, K. J., von Salzen, K., Schulz, M., Seland, Ø., Skeie, R. B., Takemura, T., Tsigaridis, K., and Zhang, K.: What controls the vertical distribution of aerosol? Relationships between process sensitivity in HadGEM3–UKCA and inter-model variation from AeroCom Phase II, Atmos. Chem. Phys., 16, 2221–2241, https://doi.org/10.5194/acp-16-2221-2016, 2016.
Koffi, B., Schulz, M., Breon, F. M., Dentener, F., Steensen, B. M., Griesfeller, J., Winker, D., Balkanski, Y., Bauer, S. E., Bellouin, N., Berntsen, T., Bian, H. S., Chin, M., Diehl, T., Easter, R., Ghan, S., Hauglustaine, D. A., Iversen, T., Kirkevag, A., Liu, X. H., Lohmann, U., Myhre, G., Rasch, P., Seland, O., Skeie, R. B., Steenrod, S. D., Stier, P., Tackett, J., Takemura, T., Tsigaridis, K., Vuolo, M. R., Yoon, J., and Zhang, K.: Evaluation of the aerosol vertical distribution in global aerosol models through comparison against CALIOP measurements: AeroCom phase II results, J. Geophys. Res.-Atmos., 121, 7254–7283, https://doi.org/10.1002/2015JD024639, 2016.
Lelieveld, J., Evans, J. S., Fnais, M., Giannadaki, D., and Pozzer, A.: The contribution of outdoor air pollution sources to premature mortality on a global scale, Nature, 525, 367–371, https://doi.org/10.1038/nature15371, 2015.
Li, X., Ma, Y., Wang, Y., Liu, N., and Hong, Y.: Temporal and spatial analyses of particulate matter (PM10 and PM2.5) and its relationship with meteorological parameters over an urban city in Northeast China, Atmos. Res., 198, 185–193, https://doi.org/10.1016/j.atmosres.2017.08.023, 2017.
Lisok, J., Rozwadowska, A., Pedersen, J. G., Markowicz, K. M., Ritter, C., Kaminski, J. W., Struzewska, J., Mazzola, M., Udisti, R., Becagli, S., and Gorecka, I.: Radiative impact of an extreme Arctic biomass-burning event, Atmos. Chem. Phys., 18, 8829–8848, https://doi.org/10.5194/acp-18-8829-2018, 2018.
Liu, Y., Franklin, M., Kahn, R., and Koutrakis, P.: Using aerosol optical thickness to predict ground-level PM2.5 concentrations in the St. Louis area: A comparison between MISR and MODIS, Remote Sens. Environ., 107, 33–44, https://doi.org/10.1016/j.rse.2006.05.022, 2007.
Lolli, S. and Di Girolamo, P.: Principal Component Analysis Approach to Evaluate Instrument Performances in Developing a Cost-Effective Reliable Instrument Network for Atmospheric Measurements, J. Atmos. Ocean. Tech., 32, 1642–1649, https://doi.org/10.1175/JTECH-D-15-0085.1
Lolli, S., Madonna, F., Rosoldi, M., Campbell, J. R., Welton, E. J., Lewis, J. R., Gu, Y., and Pappalardo, G.: Impact of varying lidar measurement and data processing techniques in evaluating cirrus cloud and aerosol direct radiative effects, Atmos. Meas. Tech., 11, 1639–1651, https://doi.org/10.5194/amt-11-1639-2018, 2018.
Marinou, E., Amiridis, V., Binietoglou, I., Tsikerdekis, A., Solomos, S., Proestakis, E., Konsta, D., Papagiannopoulos, N., Tsekeri, A., Vlastou, G., Zanis, P., Balis, D., Wandinger, U., and Ansmann, A.: Three-dimensional evolution of Saharan dust transport towards Europe based on a 9-year EARLINET-optimized CALIPSO dataset, Atmos. Chem. Phys., 17, 5893–5919, https://doi.org/10.5194/acp-17-5893-2017, 2017.
Markowicz, K., Chilinski, M. T., Lisok, J., Zawadzka, O., Stachlewska, I. S., Janicka, L., Rozwadowska, A., Makuch, P., Pakszys, P., Zielinski, T., Petelski, T., Posyniak, M., Pietruczuk, A., Szkop, A., and Westphal, D. L.: Study of aerosol optical properties during long-range transport of biomass burning from Canada to Central Europe in July 2013, J. Aeros. Sci., 101, 156–173, https://doi.org/10.1016/j.jaerosci.2016.08.006, 2016.
Masonis, S. J., Anderson, T. L., Covert, D. S., Kapustin, V., Clarke, A. D., Howell, S., and Moore, K.: A study of the extinction-to-backscatter ratio of marine aerosol during the shoreline environment aerosol study, J. Atmos. Ocean. Tech., 20, 1388–1402, https://doi.org/10.1175/1520-0426(2003)020<1388:ASOTER>2.0.CO;2, 2003.
Masson-Delmotte, V., Zhai, P., Pörtner, H.-O., Roberts, D., Skea, J., Shukla, P. R., Pirani, A., Moufouma-Okia, W., Péan, C., Pidcock, R., Connors, S., Matthews, J. B. R., Chen, Y., Zhou, X., Gomis, M. I., Lonnoy, E., Maycock, T., Tignor, M., and Waterfield, T.: Global Warming of 1.5 ∘C, An IPCC Special Report on the impacts of global warming of 1.5 ∘C above pre-industrial levels and related global greenhouse gas emission pathways, in the context of strengthening the global response to the threat of climate change, sustainable development, and efforts to eradicate poverty, IPCC, 2018.
Matthias, V., Balis, D., Bösenberg, J., Eixmann, R., Iarlori, M., Komguem, L., Mattis, I., Papayannis, A., Pappalardo, G., and Perrone, M.: Vertical aerosol distribution over Europe: Statistical analysis of Raman lidar data from 10 European aerosol research lidar network (EARLINET) stations, J. Geophys. Res., 109, D18201, https://doi.org/10.1029/2004JD004638, 2004.
Mattis, I., Ansmann, A., Müller, D., Wandinger, U., and Althausen, D.: Multiyear aerosol observations with dual-wavelength Raman lidar in the framework of EARLINET, J. Geophys. Res., 109, D13203, https://doi.org/10.1029/2004JD004600, 2004.
Mona, L., Amodeo, A., D'Amico, G., Giunta, A., Madonna, F., and Pappalardo, G.: Multi-wavelength Raman lidar observations of the Eyjafjallajökull volcanic cloud over Potenza, southern Italy, Atmos. Chem. Phys., 12, 2229–2244, https://doi.org/10.5194/acp-12-2229-2012, 2012.
Müller, D., Ansmann, A., Mattis, I., Tesche, M., Wandinger, U., Althausen, D., and Pisani, G.: Aerosol-type-dependent lidar ratios observed with Raman lidar, J. Geophys. Res., 112, D16202, https://doi.org/10.1029/2006JD008292, 2007.
Navas-Guzmán, F., Martucci, G., Collaud Coen, M., Granados-Muñoz, M. J., Hervo, M., Sicard, M., and Haefele, A.: Characterization of aerosol hygroscopicity using Raman lidar measurements at the EARLINET station of Payerne, Atmos. Chem. Phys., 19, 11651–11668, https://doi.org/10.5194/acp-19-11651-2019, 2019.
Nemuc, A., Vasilescu, J., Talianu, C., Belegante, L., and Nicolae, D.: Assessment of aerosol's mass concentrations from measured linear particle depolarization ratio (vertically resolved) and simulations, Atmos. Meas. Tech., 6, 3243–3255, https://doi.org/10.5194/amt-6-3243-2013, 2013.
Nemuc, A., Stachlewska, I. S., Valilescu, J., Górska, A., Nicolae, D., and Talianu, C.: Optical Properties of Long-Range Transported Volcanic Ash over Romania and Poland During Eyjafjallajökull Eruption in 2010, Acta Geophys., 62, 350–366 https://doi.org/10.2478/s11600-013-0180-7, 2014.
Nicolae, D., Vasilescu, J., Talianu, C., Binietoglou, I., Nicolae, V., Andrei, S., and Antonescu, B.: A neural network aerosol-typing algorithm based on lidar data, Atmos. Chem. Phys., 18, 14511–14537, https://doi.org/10.5194/acp-18-14511-2018, 2018.
Ortiz-Amezcua, P., Guerrero-Rascado, J. L., Granados-Muñoz, M. J., Benavent-Oltra, J. A., Böckmann, C., Samaras, S., Stachlewska, I. S., Janicka, Ł., Baars, H., Bohlmann, S., and Alados-Arboledas, L.: Microphysical characterization of long-range transported biomass burning particles from North America at three EARLINET stations, Atmos. Chem. Phys., 17, 5931–5946, https://doi.org/10.5194/acp-17-5931-2017, 2017.
Pan, X., Chin, M., Gautam, R., Bian, H., Kim, D., Colarco, P. R., Diehl, T. L., Takemura, T., Pozzoli, L., Tsigaridis, K., Bauer, S., and Bellouin, N.: A multi-model evaluation of aerosols over South Asia: common problems and possible causes, Atmos. Chem. Phys., 15, 5903–5928, https://doi.org/10.5194/acp-15-5903-2015, 2015.
Papagiannopoulos, N., Mona, L., Alados-Arboledas, L., Amiridis, V., Baars, H., Binietoglou, I., Bortoli, D., D'Amico, G., Giunta, A., Guerrero-Rascado, J. L., Schwarz, A., Pereira, S., Spinelli, N., Wandinger, U., Wang, X., and Pappalardo, G.: CALIPSO climatological products: evaluation and suggestions from EARLINET, Atmos. Chem. Phys., 16, 2341–2357, https://doi.org/10.5194/acp-16-2341-2016, 2016.
Papagiannopoulos, N., Mona, L., Amodeo, A., D'Amico, G., Gumà Claramunt, P., Pappalardo, G., Alados-Arboledas, L., Guerrero-Rascado, J. L., Amiridis, V., Kokkalis, P., Apituley, A., Baars, H., Schwarz, A., Wandinger, U., Binietoglou, I., Nicolae, D., Bortoli, D., Comerón, A., Rodríguez-Gómez, A., Sicard, M., Papayannis, A., and Wiegner, M.: An automatic observation-based aerosol typing method for EARLINET, Atmos. Chem. Phys., 18, 15879–15901, https://doi.org/10.5194/acp-18-15879-2018, 2018.
Papayannis, A., Amiridis, V., Mona, L., Tsaknakis, G., Balis, D., Bosenberg, J., Chaikovski, A., De Tomasi, F., Grigorov, I., Mattis, I., Mitev, V., Muller, D., Nickovic, S., Perez, C., Pietruczuk, A., Pisani, G., Ravetta, F., Rizi, V., Sicard, M., Trickl, T., Wiegner, M., Gerding, M., Mamouri, R. E., D'Amico, G., and Pappalardo, G.: Systematic lidar observations of Saharan dust over Europe in the frame of EARLINET (2000–2002), J. Geophys. Res., 113, D10204, https://doi.org/10.1029/2007JD009028, 2008.
Pappalardo, G., Amodeo, A., Apituley, A., Comeron, A., Freudenthaler, V., Linné, H., Ansmann, A., Bösenberg, J., D'Amico, G., Mattis, I., Mona, L., Wandinger, U., Amiridis, V., Alados-Arboledas, L., Nicolae, D., and Wiegner, M.: EARLINET: towards an advanced sustainable European aerosol lidar network, Atmos. Meas. Tech., 7, 2389–2409, https://doi.org/10.5194/amt-7-2389-2014, 2014.
Perrone, M. R., De Tomasi, F., and Gobbi, G. P.: Vertically resolved aerosol properties by multi-wavelength lidar measurements, Atmos. Chem. Phys., 14, 1185–1204, https://doi.org/10.5194/acp-14-1185-2014, 2014.
Petters, M. D., Carrico, C. M., Kreidenweis, S. M., Prenni, A. J., DeMott, P. J., Collett, J. L., and Moosmüller, H.: Cloud condensation nucleation activity of biomass burning aerosol, J. Geophys. Res., 114, D22205, https://doi.org/10.1029/2009JD012353, 2009.
Pope, S. B.: Turbulent Flows. Cambridge University Press, UK, 771 pp., 2000.
Popovici, I. E., Goloub, P., Podvin, T., Blarel, L., Loisil, R., Unga, F., Mortier, A., Deroo, C., Victori, S., Ducos, F., Torres, B., Delegove, C., Choël, M., Pujol-Söhne, N., and Pietras, C.: Description and applications of a mobile system performing on-road aerosol remote sensing and in situ measurements, Atmos. Meas. Tech., 11, 4671–4691, https://doi.org/10.5194/amt-11-4671-2018, 2018.
Pósfai, M., Gelencsér, A., Simonics, R., Arató, K., Li, J., Hobbs, P. V., and Buseck, P. R.: Atmospheric tar balls: Particles from biomass and biofuel burning, J. Geophys. Res., 109, D06213, https://doi.org/10.1029/2003JD004169, 2004.
Proestakis, E., Amiridis, V., Marinou, E., Binietoglou, I., Ansmann, A., Wandinger, U., Hofer, J., Yorks, J., Nowottnick, E., Makhmudov, A., Papayannis, A., Pietruczuk, A., Gialitaki, A., Apituley, A., Szkop, A., Muñoz Porcar, C., Bortoli, D., Dionisi, D., Althausen, D., Mamali, D., Balis, D., Nicolae, D., Tetoni, E., Liberti, G. L., Baars, H., Mattis, I., Stachlewska, I. S., Voudouri, K. A., Mona, L., Mylonaki, M., Perrone, M. R., Costa, M. J., Sicard, M., Papagiannopoulos, N., Siomos, N., Burlizzi, P., Pauly, R., Engelmann, R., Abdullaev, S., and Pappalardo, G.: EARLINET evaluation of the CATS Level 2 aerosol backscatter coefficient product, Atmos. Chem. Phys., 19, 11743–11764, https://doi.org/10.5194/acp-19-11743-2019, 2019.
Qin, K., Zou, J., Guo, J., Lu, M., Bilal, M., Zhang, K., Ma, F., and Zhang, Y.: Estimating PM1 concentrations from MODIS over Yangtze River Delta of China during 2014–2017. Atmos. Environ., 195, 149–158, https://doi.org/10.1016/j.atmosenv.2018.09.054, 2018.
Reizer, M. and Juda-Rezler, K.: Explaining the high PM10 concentrations observed in Polish urban areas, Air Qual. Atmos Health., 9, 517–531, https://doi.org/10.1007/s11869-015-0358-z, 2015.
Rost, J., Holst, T., Sähn, E., Klingner, M., Anke, K., Ahrens, D., and Mayer, H.: Variability of PM10 concentrations dependent on meteorological conditions, Intern. J. Environ. Poll., 36, 3–18, https://doi.org/10.1504/IJEP.2009.021813, 2009.
Sakai, T., Nagai, T., Zaizen, Y., and Mano, Y.: Backscattering linear depolarization ratio measurements of mineral, sea-salt, and ammonium sulfate particles simulated in a laboratory chamber, Appl. Opt., 49, 4441–4449, https://doi.org/10.1364/AO.49.004441, 2010.
Schaap, M., Apituley, A., Timmermans, R. M. A., Koelemeijer, R. B. A., and de Leeuw, G.: Exploring the relation between aerosol optical depth and PM2.5 at Cabauw, the Netherlands, Atmos. Chem. Phys., 9, 909–925, https://doi.org/10.5194/acp-9-909-2009, 2009.
Schäfer, K., Emeis, S., Hoffmann, H., and Jahn, C.: Influence of mixing layer height upon air pollution in urban and sub-urban areas, Meteorol. Z., 15, 647–658, https://doi.org/10.1127/0941-2948/2006/0164, 2006.
Schmeisser, L., Andrews, E., Ogren, J. A., Sheridan, P., Jefferson, A., Sharma, S., Kim, J. E., Sherman, J. P., Sorribas, M., Kalapov, I., Arsov, T., Angelov, C., Mayol-Bracero, O. L., Labuschagne, C., Kim, S.-W., Hoffer, A., Lin, N.-H., Chia, H.-P., Bergin, M., Sun, J., Liu, P., and Wu, H.: Classifying aerosol type using in situ surface spectral aerosol optical properties, Atmos. Chem. Phys., 17, 12097–12120, https://doi.org/10.5194/acp-17-12097-2017, 2017.
Seinfeld, J. H., Bretherton, C., Carslaw, K. S., Coe, H., DeMott, P. J., Dunlea, E. J., Feingold, G., Ghan, S., Guenther, A. B., Kahn, R., Kraucunas, I., Kreidenweis, S. M., Molina, M. J., Nenes, A., Penner, J. E., Prather, K. A., Ramanathan, V., Ramaswamy, V., Rasch, P. J., Ravishankara, A. R., Rosenfeld, D., Stephens, G., and Wood, R.: Improving our fundamental understanding of the role of aerosol–cloud interactions in the climate system, P. Natl. Acad. Sci. USA, 113, 5781–5790, https://doi.org/10.1073/pnas.1514043113, 2016.
Sicard, M., Rocadenbosch, F., Reba, M. N. M., Comerón, A., Tomás, S., García-Vízcaino, D., Batet, O., Barrios, R., Kumar, D., and Baldasano, J. M.: Seasonal variability of aerosol optical properties observed by means of a Raman lidar at an EARLINET site over Northeastern Spain, Atmos. Chem. Phys., 11, 175–190, https://doi.org/10.5194/acp-11-175-2011, 2011.
Siomos, N., Balis, D. S., Poupkou, A., Liora, N., Dimopoulos, S., Melas, D., Giannakaki, E., Filioglou, M., Basart, S., and Chaikovsky, A.: Investigating the quality of modeled aerosol profiles based on combined lidar and sunphotometer data, Atmos. Chem. Phys., 17, 7003–7023, https://doi.org/10.5194/acp-17-7003-2017, 2017.
Siomos, N., Balis, D. S., Voudouri, K. A., Giannakaki, E., Filioglou, M., Amiridis, V., Papayannis, A., and Fragkos, K.: Are EARLINET and AERONET climatologies consistent? The case of Thessaloniki, Greece, Atmos. Chem. Phys., 18, 11885–11903, https://doi.org/10.5194/acp-18-11885-2018, 2018.
Sharma, A., Mandal, T., Sharma, S., Shukla, D., and Singh, S.: Relationships of surface ozone with its precursors, particulate matter and meteorology over Delhi, J. Atmos. Chem., 74, 451–474, https://doi.org/10.1007/s10874-016-9351-7, 2017.
Stachlewska, I., Piądłowski, M., Migacz, S., Szkop, A., Zielińska, A., and Swaczyna, P.: Ceilometer observations of the boundary layer over Warsaw, Poland, Acta Geophys., 60, 1386–1412, https://doi.org/10.2478/s11600-012-0054-4, 2012.
Stachlewska, I. S., Costa-Surós, M., and Althausen, D.: Raman lidar water vapour profiling over Warsaw, Poland, Atmos. Res., 194, 258–267, https://doi.org/10.1016/j.atmosres.2017.05.004, 2017a.
Stachlewska, I. S., Zawadzka, O., and Engelmann, R.: Effect of heat wave conditions on aerosol optical properties derived from satellite and ground-based remote sensing over Poland, Remote Sens., 9, 1199, https://doi.org/10.3390/rs9111199, 2017b.
Stachlewska, I. S., Samson, M., Zawadzka, O., Harenda, K. M., Janicka, L., Poczta, P., Szczepanik, D., Heese, B., Wang, D., and Borek, K. Tetoni, E., Proestakis, E., Siomos, N., Nemuc, A., Chojnicki, B. H., Markowicz, K. M., Pietruczuk, A., Szkop, A., Althausen, D., Stebel, K., Schuettemeyer, D., and Zehner, C.: Modification of local urban aerosol properties by long-range transport of biomass burning aerosol, Remote Sens., 10, 412, https://doi.org/10.3390/rs10030412, 2018.
Stein, A. F., Draxler, R. R., Rolph, G. D., Stunder, B. J. B., Cohen, M. D., and Ngan, F.: NOAA's HYSPLIT Atmospheric Transport and Dispersion Modeling System, B. Am. Meteorol. Soc., 96, 2059–2077, https://doi.org/10.1175/BAMS-D-14-00110.1, 2015.
Stocker, T., Qin, D., Plattner, G., Tignor, M., Allen, S., Boschung, J., Nauels, A., Xia, Y., Bex, V., and Midgley, P.: IPCC, 2013: Climate Change 2013: The Physical Science Basis, Contribution of Working Group I to the Fifth Assessment Report of the Intergovernmental Panel on Climate Change, Cambridge Univ. Press, Cambridge, UK, and New York, 1535 pp., 2013.
Stull, R. B.: An Introduction to Boundary Layer Meteorology, Kluwer Academic Publishers, Dordrecht, the Netherlands, 1988.
Szczepanik, D. and Markowicz, K.: The relation between columnar and surface aerosol optical properties in a background environment, Atmos. Poll. Res., 9, 246–256, https://doi.org/10.1016/j.apr.2017.10.001, 2018.
Szczepanik, D., Tetoni, E., Wang, D., and Stachlewska, I.: Lidar Based Separation of Polluted Dust Observed over Warsaw (Case Study on 09 August 2013), the 29th International Laser Radar Conference, Hefei, China, 24–28 June 2019, 1–5, 2019.
Szkop, A. and Pietruczuk, A.: Analysis of aerosol transport over southern Poland in August 2015 based on a synergy of remote sensing and backward trajectory techniques, J. Appl. Remote. Sens., 11, 016039, https://doi.org/10.1117/1.JRS.11.016039, 2017.
Tang, I. N.: Chemical and size effects of hygroscopic aerosol on light scattering coefficients, J. Geophys. Res., 101, 19245–19250, https://doi.org/10.1029/96JD03003, 1996.
The EARLINET publishing group 2000–2015: EARLINET All 2000–2015, World Data Center for Climate (WDCC) at DKRZ, https://doi.org/10.1594/WDCC/EARLINET_All_2000-2015, 2018.
Tian, P., Cao, X., Zhang, L., Sun, N., Sun, L., Logan, T., Shi, J., Wang, Y., Ji, Y., Lin, Y., Huang, Z., Zhou, T., Shi, Y., and Zhang, R.: Aerosol vertical distribution and optical properties over China from long-term satellite and ground-based remote sensing, Atmos. Chem. Phys., 17, 2509–2523, https://doi.org/10.5194/acp-17-2509-2017, 2017.
Trickl, T., Vogelmann, H., Flentje, H., and Ries, L.: Stratospheric ozone in boreal fire plumes – the 2013 smoke season over central Europe, Atmos. Chem. Phys., 15, 9631–9649, https://doi.org/10.5194/acp-15-9631-2015, 2015.
Trippetta, S., Sabia, S., and Caggiano, R.: Fine aerosol particles (PM1): Natural and anthropogenic contributions and health risk assessment, Air Qual. Atmos. Hlth., 9, 621–629, https://doi.org/10.1007/s11869-015-0373-0, 2016.
Veselovskii, I., Kolgotin, A., Griaznov, V., Müller, D., Wandinger, U., and Whiteman, D. N.: Inversion with regularization for the retrieval of tropospheric aerosol parameters from multiwavelength lidar sounding, Appl. Opt., 41, 3685–3699, https://doi.org/10.1364/AO.41.003685, 2002.
Wałaszek, K., Kryza, M., and Werner, M.: The role of precursor emissions on ground level ozone concentration during summer season in Poland, J. Atmos. Chem., 75, 181–204, https://doi.org/10.1007/s10874-017-9371-y, 2018.
Wandinger, U., Freudenthaler, V., Baars, H., Amodeo, A., Engelmann, R., Mattis, I., Groß, S., Pappalardo, G., Giunta, A., D'Amico, G., Chaikovsky, A., Osipenko, F., Slesar, A., Nicolae, D., Belegante, L., Talianu, C., Serikov, I., Linné, H., Jansen, F., Apituley, A., Wilson, K. M., de Graaf, M., Trickl, T., Giehl, H., Adam, M., Comerón, A., Muñoz-Porcar, C., Rocadenbosch, F., Sicard, M., Tomás, S., Lange, D., Kumar, D., Pujadas, M., Molero, F., Fernández, A. J., Alados-Arboledas, L., Bravo-Aranda, J. A., Navas-Guzmán, F., Guerrero-Rascado, J. L., Granados-Muñoz, M. J., Preißler, J., Wagner, F., Gausa, M., Grigorov, I., Stoyanov, D., Iarlori, M., Rizi, V., Spinelli, N., Boselli, A., Wang, X., Lo Feudo, T., Perrone, M. R., De Tomasi, F., and Burlizzi, P.: EARLINET instrument intercomparison campaigns: overview on strategy and results, Atmos. Meas. Tech., 9, 1001–1023, https://doi.org/10.5194/amt-9-1001-2016, 2016.
Wang, D., Stachlewska, I. S., Song, X., Heese, B., and Nemuc, A.: Variability of boundary layer over an urban continental site based on 10 years of active remote sensing observations in Warsaw, Remote Sens., in review, 2019.
Wang, J. and Christopher, S. A.: Intercomparison between satellite-derived aerosol optical thickness and PM2.5 mass: Implications for air quality studies, Geophys. Res. Lett., 30, 2095, https://doi.org/10.1029/2003GL018174, 2003.
Winker, D. M., Hunt, W. H., and McGill, M. J.: Initial performance assessment of CALIOP, Geophys. Res. Lett., 34, L19803, https://doi.org/10.1029/2007GL030135, 2007.
Wolff, H. and Perry, L.: Policy monitor: Trends in clean air legislation in Europe: Particulate matter and low emission zones, Rev. Environ. Econ. Policy, 4, 293–308, https://doi.org/10.1093/reep/req008, 2010.
Xie, C., Nishizawa, T., Sugimoto, N., Matsui, I., and Wang, Z.: Characteristics of aerosol optical properties in pollution and Asian dust episodes over Beijing, China, Appl. Opt., 47, 4945–4951, https://doi.org/10.1364/AO.47.004945, 2008.
Zang, Z. L., Wang, W. Q., You, W., Li, Y., Ye, F., and Wang, C. M.: Estimating ground-level PM2.5 concentrations in Beijing, China using aerosol optical depth and parameters of the temperature inversion layer, Sci. Total Environ., 575, 1219–1227, https://doi.org/10.1016/j.scitotenv.2016.09.186, 2017.
Zawadzka, O., Markowicz, K., Pietruczuk, A., Zielinski, T., and Jaroslawski, J.: Impact of urban pollution emitted in Warsaw on aerosol properties, Atmos. Environ., 69, 15–28, https://doi.org/10.1016/j.atmosenv.2012.11.065, 2013.
Zhang, H., Wang, Y., Hu, J., Ying, Q., and Hu, X.-M.: Relationships between meteorological parameters and criteria air pollutants in three megacities in China, Environ. Res., 140, 242–254, https://doi.org/10.1016/j.envres.2015.04.004, 2015.
Zheng, S., Pozzer, A., Cao, C. X., and Lelieveld, J.: Long-term (2001–2012) concentrations of fine particulate matter (PM2.5) and the impact on human health in Beijing, China, Atmos. Chem. Phys., 15, 5715–5725, https://doi.org/10.5194/acp-15-5715-2015, 2015.
- Abstract
- Introduction
- Instrumentation and dataset
- Methodology of lidar product retrieval
- Results and discussion
- Conclusions
- Data availability
- Appendix A: Lists of symbols and physical quantities
- Author contributions
- Competing interests
- Special issue statement
- Acknowledgements
- Financial support
- Review statement
- References
- Abstract
- Introduction
- Instrumentation and dataset
- Methodology of lidar product retrieval
- Results and discussion
- Conclusions
- Data availability
- Appendix A: Lists of symbols and physical quantities
- Author contributions
- Competing interests
- Special issue statement
- Acknowledgements
- Financial support
- Review statement
- References