the Creative Commons Attribution 4.0 License.
the Creative Commons Attribution 4.0 License.
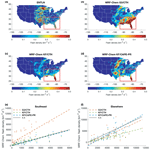
Lightning NO2 simulation over the contiguous US and its effects on satellite NO2 retrievals
Qindan Zhu
Joshua L. Laughner
Lightning is an important NOx source representing ∼10 % of the global source of odd N and a much larger percentage in the upper troposphere. The poor understanding of spatial and temporal patterns of lightning contributes to a large uncertainty in understanding upper tropospheric chemistry. We implement a lightning parameterization using the product of convective available potential energy (CAPE) and convective precipitation rate (PR) coupled with the Kain–Fritsch convective scheme (KF/CAPE-PR) into the Weather Research and Forecasting-Chemistry (WRF-Chem) model. Compared to the cloud-top height (CTH) lightning parameterization combined with the Grell 3-D convective scheme (G3/CTH), we show that the switch of convective scheme improves the correlation of lightning flash density in the southeastern US from 0.30 to 0.67 when comparing against the Earth Networks Total Lightning Network; the switch of lightning parameterization contributes to the improvement of the correlation from 0.48 to 0.62 elsewhere in the US. The simulated NO2 profiles using the KF/CAPE-PR parameterization exhibit better agreement with aircraft observations in the middle and upper troposphere. Using a lightning NOx production rate of 500 mol NO flash−1, the a priori NO2 profile generated by the simulation with the KF/CAPE-PR parameterization reduces the air mass factor for NO2 retrievals by 16 % on average in the southeastern US in the late spring and early summer compared to simulations using the G3/CTH parameterization. This causes an average change in NO2 vertical column density 4 times higher than the average uncertainty.
- Article
(4036 KB) - Full-text XML
-
Supplement
(1603 KB) - BibTeX
- EndNote
Nitrogen oxides () are key species in atmospheric chemistry, affecting the oxidative capacity in the troposphere by regulating the ozone and hydroxyl radical concentrations (Crutzen, 1979). Anthropogenic sources (mainly fossil fuel combustion) are the largest contributor to the NOx budget on a global scale. Natural sources of NOx are also non-negligible (Denman et al., 2007). While anthropogenic emissions of NOx are intensively studied, natural sources are less understood (e.g., Delmas et al., 1997; Lamsal et al., 2011; Miyazaki et al., 2012). Lightning contributes ∼10 % of the NOx budget on a global scale and represents over 80 % of NOx in the upper troposphere (UT) (Schumann and Huntrieser, 2007; Nault et al., 2017). Over the US, anthropogenic NOx emissions have been decreasing rapidly (Russell et al., 2012; Lu et al., 2015), making lightning an increasingly important source of NOx and an increasingly large fraction of the source of column NO2. Ozone (O3) in the UT has a long lifetime and leads to a more pronounced radiative effect than ozone elsewhere in the troposphere. Varying lightning NOx emissions (LNOx) by a factor of 4 (123 to 492 mol NO flash−1) yields up to 60 % enhancement of UT O3 and increases the mean net radiative flux by a factor of 3 (Liaskos et al., 2015). This range in the lightning NOx production rate is similar to the current uncertainty of estimated lightning emission rates. Further, incorrect representation of LNOx in a priori profiles for satellite NO2 retrievals leads to biases in the retrieved NO2 columns. This is exacerbated by the greater sensitivity of ultraviolet–visible (UV–Vis) NO2 retrievals to the UT (e.g., Laughner and Cohen, 2017; Travis et al., 2016).
When lightning occurs, NO is emitted as a result of high temperatures and NO2 forms through rapid photochemistry. Studies report that the estimated LNOx production rate ranges widely from 16 to 700 mol NO flash−1 (DeCaria et al., 2005; Hudman et al., 2007; Martin et al., 2007; Schumann and Huntrieser, 2007; Huntrieser et al., 2009; Beirle et al., 2010; Bucsela et al., 2010; Jourdain et al., 2010; Ott et al., 2010; Miyazaki et al., 2014; Liaskos et al., 2015; Pickering et al., 2016; Pollack et al., 2016; Laughner and Cohen, 2017; Nault et al., 2017).
Two categories of methods, one emphasizing the near field of lightning NOx and the other the far field, have previously been applied to estimate LNOx. In near-field approaches the total NOx from direct observation close to the lightning flashes is divided by the number of flashes from a lightning observation network to yield the NOx per flash (e.g., Schumann and Huntrieser, 2007; Huntrieser et al., 2009; Pollack et al., 2016). Near-field estimates of LNOx per flash have also been made through the use of cloud-resolved models with LNOx constrained by observed flashes and aircraft data from storm anvils (e.g., DeCaria et al., 2005; Ott et al., 2010; Cummings et al., 2013). In contrast, the far-field approach uses downwind observations to constrain a regional or global chemical transport model. The emission rate of lightning NOx is varied in the model (either ad hoc or through formal assimilation methods) until the modeled NOx agrees with the measurements of total NOx at the far-field location (Hudman et al., 2007; Martin et al., 2007; Jourdain et al., 2010; Miyazaki et al., 2014; Liaskos et al., 2015; Laughner and Cohen, 2017; Nault et al., 2017). In general, far-field approaches yield estimates of LNOx at the upper end of the reported range, while estimates from near-field studies are typically at the lower end of the range. Nault et al. (2017) showed that a large part of this discrepancy is because prior near-field studies assume a long NOx lifetime in the UT, while active peroxy radical chemistry in the near field leads to a short NOx lifetime (∼3 h). Without accounting for this chemical loss, the near-field and far-field estimates are biased low compared to each other. However, this effect cannot completely reconcile the discrepancy between LNOx reported from near- and far- field studies.
In chemical transport models, LNOx production is modeled by assuming that a fixed number of moles of NO is produced per lightning flash, typically 250 or 500 mol NO flash−1 (Zhao et al., 2009; Allen et al., 2010; Ott et al., 2010). This presents an additional challenge to far-field approaches to constrain LNOx, as errors in the simulation of the lightning flash rate will propagate into errors in the LNOx production per flash. However, explicitly simulating the cloud-scale processes that produce lightning is generally too computationally expensive to be applied in a regional or global model as it requires spatial resolution at the scale of cloud processes. Instead, the convection is parameterized using simplified convection schemes. Lightning is then parameterized by a suite of convection parameters. The most prevalent lightning parameterization relates lightning to the cloud-top height (CTH) (Price and Rind, 1992; Price et al., 1997). Price and Rind found a consistent proportionality between cloud-to-ground (CG) lightning flashes and the fifth power of cloud-top height. Other meteorological variables, including upward cloud mass flux (UMF), convective precipitation rate (CPR), convective available potential energy (CAPE) and cloud ice flux (ICEFLUX), have been suggested as alternative lightning proxies for CG flashes or in some cases total flashes (Allen and Pickering, 2002; Choi et al., 2005; Wong et al., 2013; Romps et al., 2014; Finney et al., 2014). When CG flashes are predicted, the total lightning rate, including CG and intra-cloud (IC) flashes, is derived by defining a regionally dependent CG : IC ratio (Boccippio et al., 2002).
Several previous studies have evaluated the performance of these lightning parameterizations in regional and global models. Tost et al. (2007) concluded that none of them accurately reproduce the observed lightning observations even though some are intercomparable. Wong et al. (2013) showed that a model using the Grell–Devenyi ensemble convective parameterization and the CTH lightning parameterization simulates an erroneous flash count frequency distribution over time, while the integrated lightning flash count is consistent with the observation. Luo et al. (2017) tested the single-variable parameterizations (CTH, CAPE, UMF, CPR) and the paired parameterizations based on the power-law relationship (CAPE-CTH, CAPE-UMF, UMF-CTH), each of which was coupled with the Kain–Fritsch convective scheme, and demonstrated that the two-variable parameterization using CAPE-CTH improves upon the previous single-variable parameterizations; it captures the temporal change in flash rates, but the simulated spatial distribution is still not satisfactory.
In this study, we implemented the CAPE-PR lightning parameterization (Romps et al., 2014) into WRF-Chem and assess the performance in reproducing lightning flash density. Our motivation is to produce a better representation of a proxy-based lightning parameterization in the regional chemistry transport model. We also evaluate the effect of modeled lightning NOx on both the a priori profiles used in satellite NO2 retrievals and the retrievals themselves.
2.1 WRF-Chem
This study applies the Weather Research and Forecast Model coupled with Chemistry (WRF-Chem) version 3.5.1 to the time periods May to June 2012 and August to September 2013. The model domain covers North America from 20 to 50∘ N with 12 km×12 km horizontal resolution and 29 vertical layers. The North American Regional Reanalysis (NARR) provides initial and boundary conditions. Temperature, wind direction, wind speed and water vapor are nudged every 3 h towards the NARR product. Chemistry initial and boundary conditions are provided by the Model for Ozone and Related Chemistry Tracers (MOZART; https://www.acom.ucar.edu/wrf-chem/mozart.shtml, last access: 21 October 2019). Anthropogenic emissions are driven by the National Emissions Inventory 2011 (NEI 11), with a scaling factor to match the total emissions to 2012 emission from the Environmental Protection Agency (EPA, 2016). Biogenic emissions are driven by the Model of Emissions of Gases and Aerosol from Nature (MEGAN; Guenther et al., 2006). We use a customized version of the Regional Atmospheric Chemistry Mechanism version 2 (RACM2); the details are described by Zare et al. (2018).
The default lightning parameterization used in WRF-Chem is based on cloud-top height (CTH). The parameterized lightning flash rates are proportional to a power of cloud-top height with linear scaling varied by region:
where f is the CG flash rate in each grid and H is the colocated cloud-top height in units of kilometers.
We also implement an alternative lightning parameterization wherein lightning flash rates are defined to be proportional to the product of the convective available potential energy (CAPE) and precipitation rate (PR).
Here, f is the CG flash rate in each grid cell, E the convective available potential energy and PR the convective precipitation rate. Southeastern CONUS in this context is the region between 94–76∘ W and 25–37∘ N. This parameterization was proposed by Romps et al. (2014). Romps et al. (2014) used a year-round observation of lightning and meteorological parameters and found a good correlation between observed lightning flash densities and observed CAPE times PR over CONUS. CAPE-PR was further examined in Tippett and Koshak (2018), who computed the proxy in a numerical forecast model and found a fairly good agreement between the spatial pattern of the daily CG flash rate and the forecast proxy over 2003–2016. To our knowledge the CAPE-PR parameterization has not previously been coupled with chemistry. Note that we compute these two meteorological variables every 72 s in our model setup and produce lightning flash rates in a much shorter time step compared to Romps et al. (2014) and Tippett and Koshak (2018). We also apply a regional scaling factor of 0.5 to the southeastern US (see Sect. 3.1).
We analyze WRF-Chem outputs from three model runs. The first run, referred to as G3/CTH, is consistent with Laughner and Cohen (2017); it selects the Grell 3-D ensemble cumulus convective scheme (Grell, 1993; Grell and Dévényi, 2002) and the CTH lightning parameterization. The Grell 3-D convective scheme readily computes the neutral buoyancy level that serves as the optimal proxy for cloud-top height (Wong et al., 2013). The G3/CTH run is the only option for the coupled convective–lighting parameterization used in WRF-Chem at a non-cloud-resolving resolution (12 km). In addition, we run WRF-Chem with the CTH lightning parameterization coupled with the Kain–Fritsch cumulus convective scheme (Kain and Fritsch, 1990; Kain, 2004) (KF/CTH) to test the effect of switching convective schemes. In the KF/CTH parameterization, the cloud-top height is the level at which the updraft vertical velocity equals zero. Another run, referred to as KF/CAPE-PR, selects the Kain–Fritsch cumulus convective scheme and the CAPE-PR lightning parameterization described above. Compared to the Grell 3-D convective scheme, the Kain–Fritsch uses the depletion of at least 90 % of the CAPE as the closure assumption and calculates CAPE on the basis of entraining parcels instead of undiluted parcels, which also improves the calculation of precipitation rate (Kain, 2004). The lightning NOx production rate is defined to be 500 mol NO flash−1. The CG : IC ratio and the LNOx post-convection vertical distribution are the same as used by Laughner and Cohen (2017).
2.2 ENTLN lightning observation network
To assess the performance of the lightning parameterizations we compare to lightning flashes from the Earth Networks Total Lightning Network (ENTLN). ENTLN employs over 100 sensors across the United States and observes both CG and IC pulses (https://www.earthnetworks.com/why-us/networks/lightning/, last access: 21 October 2019). All lightning pulses within 10 km and 700 ms of each other are grouped as a single flash. The IC and CG flashes are summed over the grid spacing defined in WRF-Chem.
Compared to the National Lightning Detection Network (NLDN), ENTLN is selected for high detection efficiencies of both CG and IC flashes. The average detection efficiency for total flashes observed by ENTLN was 88 % over CONUS relative to the space-based Tropical Rainfall Measurement Mission (TRMM) Lightning Imaging Sensor (LIS) (Lapierre et al., 2019; Jeff Lapierre, private communication, 2018). As shown in Fig. S2 in the Supplement, we matched the ENTLN data to LIS flashes both in time and space after the correction of LIS data based on its detection efficiency (Cecil et al., 2014) during 13 May–23 June 2012. It shows a median correlation (R2=0.51) with a slope of 1.0, indicating that the ENTLN data during the study time period are in agreement with the LIS observation. We use the ENTLN for analysis as reported and consider the detection efficiency of ENTLN as a source of uncertainty when comparing the modeled lightning flashes.
2.3 In situ aircraft measurements
We compare our simulations to observations from aircraft campaigns that focus on deep convection. The Deep Convective Clouds and Chemistry (DC3) campaign (Barth et al., 2015) took place during May and June of 2012 over Colorado, Oklahoma, Texas and Alabama. Studies of Emissions and Atmospheric Composition, Clouds, and Climate Coupling by Regional Surveys (SEAC4RS) (Toon et al., 2016) took place during August and September of 2013; most of the flight tracks occurred over the southeastern US. Both aircraft campaigns flew into and out of storms and sampled deep convection. The combination of these two aircraft campaigns covers the regions with the most active lightning in the domain.
2.4 Satellite measurements
The Ozone Monitoring Instrument (OMI) is an ultraviolet–visible (UV–Vis) nadir solar backscatter spectrometer launched in July 2004 onboard the Aura satellite. It detects backscattered radiance in the range of 270–500 nm and the spectra are used to derive column NO2 at a spatial resolution of 13 km×24 km at nadir (Levelt et al., 2006). The OMI overpass time is ∼ 13:30 local time.
We use the Berkeley High Resolution (BEHR) v3.0B OMI NO2 retrieval (Laughner et al., 2018). The air mass factor (AMF) is calculated based on the high-spatial-resolution a priori input data including surface reflectance, surface elevation and NO2 vertical profiles. In this study we apply an experimental branch of the BEHR product that differs from v3.0B in several ways. First, instead of calculation based on temperature profiles from WRF-Chem, the tropopause pressure is switched to GEOS-5 monthly tropopause pressure that is consistent with the NASA Standard Product (SP2) (Mak et al., 2018). Analysis shows that the algorithm used in BEHR v3.0B to calculate the WRF-derived tropopause pressure is very much dependent on the vertical spacing predefined in the WRF-Chem setup, which causes biases when the vertical layers are at a coarse resolution. Second, the NO2 vertical profiles are outputs using the modified lightning parameterization described in Eq. (2).
3.1 Comparison with observed lightning flash density
The lightning parameterizations are compared against observations from ENTLN in Fig. 1. Each of the datasets is averaged from 13 May to 23 June 2012, covering the DC3 field campaign. The ENTLN data are summed to the 12 km×12 km WRF grid. The G3/CTH parameterization fails to reproduce the spatial pattern of flashes observed by ENTLN over CONUS. Compared to the G3/CTH, the KF/CTH parameterization improves the spatial correlation in the southeast region of the US and yields a lower number of lightning flashes. It indicates that the KF convective scheme produces smaller cumulus cloud-top heights than the G3 scheme by including entrainment and detrainment processes during the convection. The result is consistent with Zhao et al. (2009). The KF/CAPE-PR parameterization better captures the spatial distribution of flash densities in both the southeast region and elsewhere in CONUS. However, the KF/CAPE-PR parameterization still fails to capture the gradients in flash occurrence within smaller regions. For instance, ENTLN shows that more lightning occurs along the east coast than the west coast in Florida; however, WRF-Chem generates a lightning flash density of the same magnitude over both areas. Nevertheless, the KF/CAPE-PR substantially improves the model performance in reproducing lightning spatial patterns.
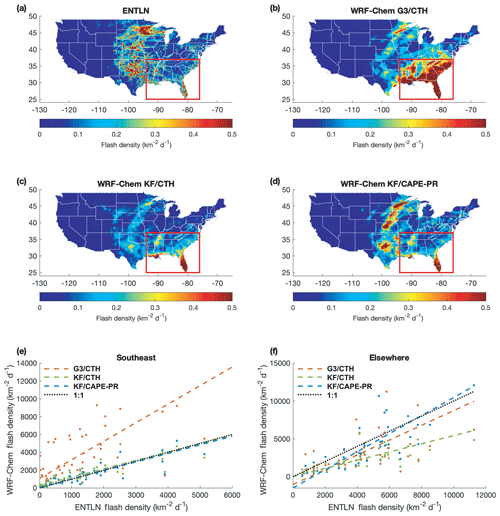
Figure 1Observed flash densities from the ENTLN dataset (a) and WRF-Chem using three coupled convective–lightning parameterizations, the G3/CTH parameterization (b), the KF/CTH parameterization (c) and the KF/CAPE-PR parameterization (d). The correlation of total flash density per day between WRF-Chem outputs and ENTLN for the southeastern US (denoted by the red box in panels a–d) is shown in panel (e) and the correlation for elsewhere in CONUS is shown in panel (f). The model using G3/CTH is in red, KF/CTH is in green and KF/CAPE-PR is in blue. Dashed lines are corresponding fits. For slope and R2, see Table 1.
To evaluate the agreement quantitatively, we regress the WRF daily regional average flash densities against those measured by ENTLN. The daily regional averaged flash density is calculated by summing the total flash rates and dividing them by the corresponding regional size. The regressions are shown in Fig. 1e and f; the correlation statistics are shown in Table 1. The regressions by forcing and intercept equal to zero are also tested, and the results are unaffected.
Table 1Correlation statistics between observed and modeled (G3/CTH, KF/CTH, KF/CAPE-PR) flash density per day averaged by region.
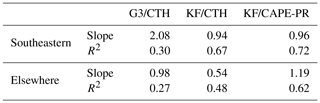
Both models using the KF/CTH and KF/CAPE-PR parameterizations improve the correlation between modeled and observed lightning flash densities over the US domain. In the southeastern US, changing from the G3 to KF convective scheme substantially increases the R2 from 0.30 to 0.67 and reduces the slope from 2.08 to 0.94. Switching from the CTH to CAPE-PR lightning parameterization only contributes a slight increment on the correlation. While the slopes are close to unity for both KF/CTH and KF/CAPE-PR, we note that the improved scaling of the slope in KF/CAPE-PR is mainly caused by the scaling factor of 0.5 applied to the southeast region. In this simulation, a constant linear coefficient for CAPE-PR is not adequate to represent the observed lightning over CONUS, in contrast to the finding of Romps et al. (2014). Elsewhere in CONUS, changes in both the convective scheme and lightning parameterization yield a better representation of lightning flash densities compared to the observation. The R2 for KF/CAPE-PR improves significantly to 0.62 compared to both G3/CTH and KF/CTH. The slope for KF/CAPE-PR is 1.19, which is within the uncertainty of the detection efficiency of ENTLN. In general the KF/CAPE-PR lightning parameterization captures the day-to-day variation in flash densities better than the G3/CTH and KF/CTH parameterizations, as shown by the improved R2 values.
3.2 Comparison with observed vertical profiles
We compare the WRF NO2 profile to the average vertical profile of NO2 measured during DC3 and SEAC4RS in Fig. 2. Data points are matched in time and space by finding the WRF-Chem output nearest in time and closest in space to a given observation. We only compare NO2 profiles from WRF-Chem using KF/CAPE-PR against the one using G3/CTH.
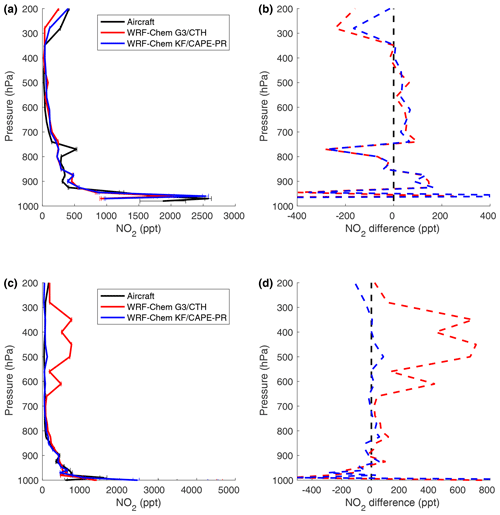
Figure 2Comparison of WRF-Chem and aircraft NO2 profiles from the (a, b) DC3 and (c, d) SEAC4RS campaigns. Vertical NO2 profiles are shown in panels (a) and (c); the solid line is the mean of all profiles and the bars are 1 standard deviation for each binned level. The corresponding absolute difference compared to observations is shown in panels (b) and (d). Aircraft measurements are shown in black, with WRF-Chem using the G3/CTH parameterization in red and WRF-Chem using the KF/CAPE-PR parameterization in blue.
The effect of lightning NOx on the profiles is indistinguishable close to the surface. In the upper and middle troposphere, both model simulations yield similar NO2 vertical profiles compared to the measurements from DC3. WRF-Chem using KF/CAPE-PR performs slightly better between 200 and 400 hPa, but the negative bias still exists. NOx from both the observations and the models is very small in the middle troposphere between 400 and 700 hPa.
Laughner et al. (2019) previously identified a high bias of WRF-Chem UT NO2 versus SEAC4RS in the southeast US when using the G3/CTH parameterization. The model using the KF/CAPE-PR parameterization reduces this high bias of NO2 in the middle and upper troposphere. The KF/CAPE-PR parameterization slightly overestimates NO2 in the middle troposphere (400–530 hPa) and underestimates it in the upper troposphere (<280 hPa), which is consistent with the comparison to observations from the DC3 campaign.
3.3 Impact on BEHR NO2 retrievals
In space-based retrievals of NO2, the AMF is required to convert the slant column density (SCD) obtained by fitting the observed radiances into a vertical column density (VCD). The AMF depends on scattering weights (which describe the sensitivity of the measurement to different levels of the atmosphere) and an NO2 profile that is either measured or simulated by a chemical transport model, such as WRF-Chem. Over a dark surface, the scattering weights in the UT are up to 10 times greater than near the surface due to the greater probability that a photon that reaches the lower troposphere will be absorbed by the surface. Therefore, errors in the UT NO2 profile can have large effects on the AMF (e.g., Laughner and Cohen, 2017). Here, we investigate how the NO2 profiles simulated by the KF/CAPE-PR parameterization affect the BEHR NO2 retrievals.
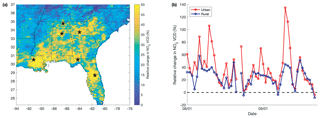
Figure 3Relative change in BEHR NO2 VCD over the southeastern US switching the source of a priori NO2 profiles from WRF-Chem outputs using G3/CTH to one using the KF/CAPE-PR lightning parameterization. Panel (a) shows the mean spatial distribution of the changes from 1 August to 23 September 2013 and panel (b) shows the temporal variation over urban and rural areas. Only observations with a cloud fraction less than 20 % are included. Medium to large cities are marked by stars in panel (a): Atlanta, GA; Huntsville, AL; Birmingham, AL; Tallahassee, FL; Orlando, FL; and Baton Rouge, LA.
Figure 3a shows the relative change in tropospheric VCD averaged between 1 August and 23 September 2013 induced by changing the a priori profiles from the model using G3/CTH to the one using the KF/CAPE-PR lightning parameterization. The relative enhancement of VCD is 19 % on average over the southeast US, but it varies significantly.
We follow the same algorithm used in Laughner and Cohen (2017) to determine if the result is significant. The overall uncertainty due to AMF calculation for BEHR v3.0B is smaller than 30 % during the study period (Sect. 6 in the Supplement from Laughner et al., 2019). Over 90 % of the uncertainty is attributed to the a prior NO2 profiles, the tropopause and cloud pressures. As each grid in Fig. 3a is the average of 45±9 pixels, the reduced uncertainty is less than 4.5 %. The overall change in VCD is 4 times larger than the reduced uncertainty. The switch of lightning parameterization leads to changes in VCD exceeding the averaged uncertainty in ∼94 % of pixels in the southeast region of the US.
The spatial pattern in Fig. 3a suggests that the magnitude of the improved representation of lightning is quite different in urban and rural areas. The cities indicated by stars and their vicinity regions are associated with a substantial increase in NO2 VCD. To quantify this, we define urban and rural areas by the difference in column NO2 calculated from WRF-Chem without LNOx. Urban ares are the top 5 % of columns with an average VCD of 2.2×1015 mole cm−2. The selected rural areas have the same size as urban areas and the average VCD is 0.72×1015 mole cm−2. Figure 3b shows the relative change in VCD over urban and rural areas as a function of time. The increase in VCD due to the change in profiles is more pronounced over urban areas, with an averaged relative change of ∼38 % compared to the average change of ∼24 % in rural areas. Changes in urban VCDs span −10 % to 135 %. In contrast, using the NO2 profiles produced by the KF/CAPE-PR simulation leads to a maximum increase of only 58.3 % in VCD over rural areas.
Table 2Differences for BEHR AMFs and tropospheric VCDs when using the a priori NO2 profiles from models with CTH vs. CAPE-PR parameterizations in the AMF calculation. For definitions of “urban” and “rural”, see the text.

Table 2 presents the AMF and VCD obtained using a priori profiles with the G3/CTH or KF/CAPE-PR lightning parameterization as well as the relative changes on 10 September and 24 August 2013. The corresponding a priori NO2 profiles and scattering weights over urban and rural areas are shown in Fig. S3. The G3/CTH parameterization has substantially more lightning than observed and thus places a large fraction of NO2 in the upper troposphere, whereas the KF/CAPE-PR has less lightning and is more consistent with observations. The resulting profiles of modeled NO2 are more dominated by boundary-layer NO2 and less sensitive to lightning. 10 September is an example of one day when the change in NO2 profiles has a very large impact on the NO2 VCDs. WRF-Chem using the G3/CTH parameterization places a large amount of NO2 between 200 and 600 hPa, with the maximum value comparable to near-surface NO2 over urban areas. The calculated AMF is predominantly determined by lightning NO2 due to the combination of higher scattering weight and larger NO2 in the middle and upper troposphere. The change in AMF is −56.0 % over urban areas and −32.0 % over rural areas; the corresponding VCD increases by 134.9 % and 44.9 %, respectively. In contrast, 24 August is an example in which the lightning parameterization has very little effect. While the positive bias in NO2 aloft is also observed by using the G3/CTH parameterization, the amount of NO2 in the middle and upper troposphere is smaller than on 10 September. It leads to lower sensitivity of AMF to the erroneous NO2 caused by the lightning parameterization. With a smaller relative change in AMF, the relative change in VCD is 3.1 % over urban areas and −4.6 % over rural areas.
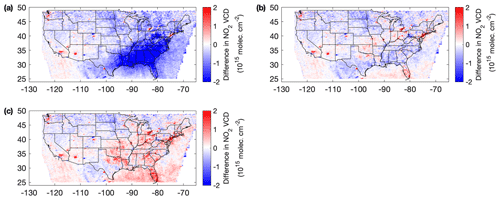
Figure 4Difference in NO2 VCD between BEHR retrievals and WRF-Chem (WRF-Chem − BEHR). Panel (a) excludes LNOx in model simulation, and panel (b) adds LNOx emissions with a production rate of 500 mol NO flash−1. Panel (c) includes the same LNOx emissions as panel (b) but uses NO2 profiles scaled upward by 60 % at pressure lower than 400 hPa. The average time covers 13 May to 23 June 2012. Pixels with a cloud fraction larger than 0.2 are filtered out in the analysis.
Here, we apply the improved KF/CAPE-PR simulation to the problem of constraining LNOx production over CONUS. To do so, we vary the lightning NOx production rate prescribed in WRF-Chem to produce the simulated map of NO2 VCD and compare against OMI NO2 retrievals using a priori profiles from model simulations with the same LNOx production rate. In our model–satellite comparisons the averaging kernel is applied to remove the representative errors introduced by a priori knowledge of NO2 vertical profiles (Boersma et al., 2016). Figure 4 shows the difference between satellite-retrieved NO2 VCD and model-simulated NO2 VCD without lightning NOx (a) and with a lightning NOx production rate of 500 mol NO flash−1 (b) averaged between 13 May and 23 June 2012. Figure S4 shows difference plots with varied lightning NOx production rates (400 and 665 mol NO flash−1). The corresponding root mean square errors (RMSEs) are included in Table S1. The LNOx production rate of 500 mol NO flash−1 yields the lowest RMSE of 0.41×1015 mole cm−2 between modeled and observed NO2 VCD over CONUS. This is at the high end of previous estimates of the lightning NOx production rate (16–700 mol NO flash−1).
The RMSE for urban areas (top 5 % of NO2 VCD simulated by WRF-Chem without LNOx) remains at a high value (∼0.9–1.3×1015 mole cm−2) when switching the LNOx production rate. It indicates that the bias in the modeled VCD over urban areas is more likely due to surface NO2. The RMSE for nonurban areas shows pronounced change with a varied LNOx production rate. Excluding urban areas lowers the RMSE to 0.37×1015 mole cm−2 for the LNOx production rate of 500 mol NO flash−1. The RMSEs are significant considering the uncertainty for retrievals. During the average time period, 32±6 pixels contribute to each value in the plots. While the global mean uncertainty for tropospheric NO2 VCD retrievals is 1×1015 mole cm−2 (Bucsela et al., 2013), the reduced uncertainty in our analysis is mole cm−2. The calculated RMSEs are twice the uncertainty.
However, we note that this lightning NOx estimate is systematically biased high due to the negative bias in the [NO2]∕[NOx] ratio in the middle and upper troposphere. The satellite-observed NO2 column serves as a proxy for total NOx emitted by lightning. The rapid interconversion between NO and NO2 reaches the photochemical steady state in a short time (∼120 s). Consequently, if the model kinetics result in an incorrect NO–NO2 photochemical steady-state ratio, this error will propagate into the LNOx production estimate. Comparisons against aircraft measurements show that the [NO2]∕[NOx] ratio in the WRF-Chem simulations is around 40 % smaller than observations in the upper troposphere (Fig. S5). Given that the simulated [NO2]∕[NOx] is too small, the model will simulate smaller NO2 VCDs per unit of LNOx emitted, requiring a greater LNOx production efficiency to match satellite NO2 VCD observations. A comparison of modeled NO2 columns, recalculated with NO2 profiles scaled up by 60 % (the ratio of observed and modeled [NO2]∕[NOx]) at pressure levels at which p<400 hPa, and observations is shown in Fig. 4c. This suggests that 500 mol NO flash−1 is greater than the actual LNOx production rate when the bias caused by the [NO2]∕[NOx] ratio is accounted for.
Several recent studies also report an underestimate in modeled [NO2]∕[NOx] ratios in the SE US (Travis et al., 2016; Silvern et al., 2018); both feature observations from the SEAC4RS field campaign to validate model simulations. Silvern et al. (2018) suggest that the underestimate is either caused by an unknown labile NOx reservoir species or an error in the reaction rate constant for the NO+O3 reaction and NO2 photolysis reaction. In contrast, Nault et al. (2017) utilize measurements from the DC3 field campaign and demonstrate a positive bias in the modeled [NO2]∕[NOx] ratio compared against observations. Understanding the difference in [NO2]∕[NOx] between models and observations requires additional study but is crucial to reducing the uncertainty in LNOx estimates.
We implement an alternative lightning parameterization based on convective available potential energy and precipitation rate into WRF-Chem and couple it with the Kain–Fritsch convective scheme. We first validate it by comparing against lightning observations and find that the model reproduces the day-to-day variation of lightning flashes in the southeastern US after the switch of convective scheme and the switch of lightning parameterization contribute to the improvement of the lightning representation elsewhere in the US. We also compare the simulated NO2 profiles against aircraft measurements and find that the simulated NO2 using KF/CAPE-PR is more consistent with observations in the middle and upper troposphere.
The improved lightning NO2 simulation has a significant impact on AMFs and VCD of NO2. Over the southeastern US the AMF is reduced by 16 % on average, leading to a 19 % increase in the NO2 VCD. The effects on AMF and on VCD are very locally dependent. The VCD increase over urban areas is more pronounced and can be over 100 %. This study indicates that the erroneous representation of lightning NO2 in a priori profiles is an important source of bias for satellite retrievals. The model–satellite NO2 column comparison suggests that 500 mol NO flash−1 is the upper bound for estimates of the lightning NOx production rate.
The experimental branch of the BEHR v3.0B product used in this study is hosted by UC Dash (Zhu et al., 2019a, b) as well as at https://behr.cchem.berkeley.edu/download-behr-data/ (last access: 21 October 2019). The BEHR algorithm is available at https://github.com/CohenBerkeleyLab/BEHR-core/ (last access: 21 October 2019; Laughner and Zhu, 2018). The revised WRF-Chem code is available at https://github.com/CohenBerkeleyLab/WRF-Chem-R2SMH/tree/lightning (last access: 21 October 2019; Zhu and Laughner, 2019).
The supplement related to this article is available online at: https://doi.org/10.5194/acp-19-13067-2019-supplement.
RCC directed the research and QZ, JLL and RCC designed this study; JLL and QZ developed BEHR products; QZ performed the analysis and prepared the paper with contributions from JLL and RCC. All authors have reviewed and edited the paper.
The authors declare that they have no conflict of interest.
We acknowledge use of the Savio computational cluster resource provided by the Berkeley Research Computing program at UC Berkeley that is supported by the UC Berkeley Chancellor, Vice Chancellor for Research, and Chief Information Officer. We thank the Earth Networks Company for providing the Earth Networks Total Lightning Network (ENTLN) datasets. We appreciate use of the WRF-Chem preprocessor tool (mozbc) provided by the Atmospheric Chemistry Observations and Modeling Lab (ACOM) of NCAR and use of MOZART-4 global model output available at https://www.acom.ucar.edu/wrf-chem/mozart.shtml (last access: 21 October 2019).
This research has been supported by NASA (grant nos. NNX14AK89H, NNX15AE37G, and 80NSSC18K0624) and the Smithsonian Astrophysical Observatory (grant no. SV3-83019).
This paper was edited by Andreas Richter and reviewed by Yuhang Wang and one anonymous referee.
Allen, D., Pickering, K., Duncan, B., and Damon, M.: Impact of lightning NO emissions on North American photochemistry as determined using the Global Modeling Initiative (GMI) model, J. Geophys. Res.-Atmos., 115, D22301, https://doi.org/10.1029/2010JD014062, 2010. a
Allen, D. J. and Pickering, K. E.: Evaluation of lightning flash rate parameterizations for use in a global chemical transport model, J. Geophys. Res.-Atmos., 107, ACH 15-1–ACH 15-21, https://doi.org/10.1029/2002JD002066, 2002. a
Barth, M. C., Cantrell, C. A., Brune, W. H., Rutledge, S. A., Crawford, J. H., Huntrieser, H., Carey, L. D., MacGorman, D., Weisman, M., Pickering, K. E., Bruning, E., Anderson, B., Apel, E., Biggerstaff, M., Campos, T., Campuzano-Jost, P., Cohen, R., Crounse, J., Day, D. A., Diskin, G., Flocke, F., Fried, A., Garland, C., Heikes, B., Honomichl, S., Hornbrook, R., Huey, L. G., Jimenez, J. L., Lang, T., Lichtenstern, M., Mikoviny, T., Nault, B., O’Sullivan, D., Pan, L. L., Peischl, J., Pollack, I., Richter, D., Riemer, D., Ryerson, T., Schlager, H., St. Clair, J., Walega, J., Weibring, P., Weinheimer, A., Wennberg, P., Wisthaler, A., Wooldridge, P. J., and Ziegler, C.: The Deep Convective Clouds and Chemistry (DC3) Field Campaign, B. Am. Meteorol. Soc., 96, 1281–1309, https://doi.org/10.1175/BAMS-D-13-00290.1, 2015. a
Beirle, S., Huntrieser, H., and Wagner, T.: Direct satellite observation of lightning-produced NOx, Atmos. Chem. Phys., 10, 10965–10986, https://doi.org/10.5194/acp-10-10965-2010, 2010. a
Boccippio, D. J., Koshak, W. J., and Blakeslee, R. J.: Performance Assessment of the Optical Transient Detector and Lightning Imaging Sensor. Part I: Predicted Diurnal Variability, J. Atmos. Ocean. Tech., 19, 1318–1332, https://doi.org/10.1175/1520-0426(2002)019<1318:PAOTOT>2.0.CO;2, 2002. a
Boersma, K. F., Vinken, G. C. M., and Eskes, H. J.: Representativeness errors in comparing chemistry transport and chemistry climate models with satellite UV–Vis tropospheric column retrievals, Geosci. Model Dev., 9, 875–898, https://doi.org/10.5194/gmd-9-875-2016, 2016. a
Bucsela, E. J., Pickering, K. E., Huntemann, T. L., Cohen, R. C., Perring, A., Gleason, J. F., Blakeslee, R. J., Albrecht, R. I., Holzworth, R., Cipriani, J. P., Vargas-Navarro, D., Mora-Segura, I., Pacheco-Hernández, A., and Laporte-Molina, S.: Lightning-generated NOx seen by the Ozone Monitoring Instrument during NASA's Tropical Composition, Cloud and Climate Coupling Experiment (TC4), J. Geophys. Res.-Atmos., 115, D00J10, https://doi.org/10.1029/2009JD013118, 2010. a
Bucsela, E. J., Krotkov, N. A., Celarier, E. A., Lamsal, L. N., Swartz, W. H., Bhartia, P. K., Boersma, K. F., Veefkind, J. P., Gleason, J. F., and Pickering, K. E.: A new stratospheric and tropospheric NO2 retrieval algorithm for nadir-viewing satellite instruments: applications to OMI, Atmos. Meas. Tech., 6, 2607–2626, https://doi.org/10.5194/amt-6-2607-2013, 2013. a
Cecil, D. J., Buechler, D. E., and Blakeslee, R. J.: Gridded lightning climatology from TRMM-LIS and OTD: Dataset description, Atmos. Res., 135–136, 404–414, https://doi.org/10.1016/j.atmosres.2012.06.028, 2014. a
Choi, Y., Wang, Y., Zeng, T., Martin, R. V., Kurosu, T. P., and Chance, K.: Evidence of lightning NOx and convective transport of pollutants in satellite observations over North America, Geophys. Res. Lett., 32, L02805, https://doi.org/10.1029/2004GL021436, 2005. a
Crutzen, P. J.: The Role of NO and NO2 in the Chemistry of the Troposphere and Stratosphere, Ann. Rev. Earth Pl. Sc., 7, 443–472, https://doi.org/10.1146/annurev.ea.07.050179.002303, 1979. a
Cummings, K. A., Huntemann, T. L., Pickering, K. E., Barth, M. C., Skamarock, W. C., Höller, H., Betz, H.-D., Volz-Thomas, A., and Schlager, H.: Cloud-resolving chemistry simulation of a Hector thunderstorm, Atmos. Chem. Phys., 13, 2757–2777, https://doi.org/10.5194/acp-13-2757-2013, 2013. a
DeCaria, A. J., Pickering, K. E., Stenchikov, G. L., and Ott, L. E.: Lightning-generated NOx and its impact on tropospheric ozone production: A three-dimensional modeling study of a Stratosphere-Troposphere Experiment: Radiation, Aerosols and Ozone (STERAO-A) thunderstorm, J. Geophys. Res.-Atmos., 110, D14303, https://doi.org/10.1029/2004JD005556, 2005. a, b
Delmas, R., Serça, D., and Jambert, C.: Global inventory of NOx sources, Nutr. Cycl. Agroecosyst., 48, 51–60, https://doi.org/10.1023/A:1009793806086, 1997. a
Denman, K. L., Brasseur, G., Chidthaisong, A., Ciais, P., Cox, P. M., Dickinson, R. E., Hauglustaine, D., Heinze, C., Holland, E., Jacob, D., Lohmann, U., Ramachandran, S., da Silva Dias, P. L., Wofsy, S. C., and Zhang, X.: Couplings Between Changes in the Climate System and Biogeochemistry ,in: Climate Change 2007: The Physical Science Basis. Contribution of Working Group I to the Fourth Assessment Report of the Intergovernmental Panel on Climate Change, edited by: Solomon, S., Qin, D., Manning, M., Chen, Z., Marquis, M., Averyt, K. B., Tignor, M., and Miller, H. L., Cambridge University Press, Cambridge, UK and New York, NY, USA, 2007. a
EPA: Air Pollutant Emissions Trends Data, available at: https://www.epa.gov/air-emissions-inventories/air-pollutant-emissions-trends-data (last access: 21 October 2019), 2016. a
Finney, D. L., Doherty, R. M., Wild, O., Huntrieser, H., Pumphrey, H. C., and Blyth, A. M.: Using cloud ice flux to parametrise large-scale lightning, Atmos. Chem. Phys., 14, 12665–12682, https://doi.org/10.5194/acp-14-12665-2014, 2014. a
Grell, G. A.: Prognostic Evaluation of Assumptions Used by Cumulus Parameterizations, Mon. Weather Rev., 121, 764–787, https://doi.org/10.1175/1520-0493(1993)121<0764:PEOAUB>2.0.CO;2, 1993. a
Grell, G. A. and Dévényi, D.: A generalized approach to parameterizing convection combining ensemble and data assimilation techniques, Geophys. Res. Lett., 29, 38-1–38-4, https://doi.org/10.1029/2002GL015311, 2002. a
Guenther, A., Karl, T., Harley, P., Wiedinmyer, C., Palmer, P. I., and Geron, C.: Estimates of global terrestrial isoprene emissions using MEGAN (Model of Emissions of Gases and Aerosols from Nature), Atmos. Chem. Phys., 6, 3181–3210, https://doi.org/10.5194/acp-6-3181-2006, 2006. a
Hudman, R. C., Jacob, D. J., Turquety, S., Leibensperger, E. M., Murray, L. T., Wu, S., Gilliland, A. B., Avery, M., Bertram, T. H., Brune, W., Cohen, R. C., Dibb, J. E., Flocke, F. M., Fried, A., Holloway, J., Neuman, J. A., Orville, R., Perring, A., Ren, X., Sachse, G. W., Singh, H. B., Swanson, A., and Wooldridge, P. J.: Surface and lightning sources of nitrogen oxides over the United States: Magnitudes, chemical evolution, and outflow, J. Geophys. Res. Atmos., 112, D12S05, https://doi.org/10.1029/2006JD007912, 2007. a, b
Huntrieser, H., Schlager, H., Lichtenstern, M., Roiger, A., Stock, P., Minikin, A., Höller, H., Schmidt, K., Betz, H.-D., Allen, G., Viciani, S., Ulanovsky, A., Ravegnani, F., and Brunner, D.: NOx production by lightning in Hector: first airborne measurements during SCOUT-O3/ACTIVE, Atmos. Chem. Phys., 9, 8377–8412, https://doi.org/10.5194/acp-9-8377-2009, 2009. a, b
Jourdain, L., Kulawik, S. S., Worden, H. M., Pickering, K. E., Worden, J., and Thompson, A. M.: Lightning NOx emissions over the USA constrained by TES ozone observations and the GEOS-Chem model, Atmos. Chem. Phys., 10, 107–119, https://doi.org/10.5194/acp-10-107-2010, 2010. a, b
Kain, J. S.: The Kain–Fritsch Convective Parameterization: An Update, J. Appl. Meteorol., 43, 170–181, https://doi.org/10.1175/1520-0450(2004)043<0170:TKCPAU>2.0.CO;2, 2004. a, b
Kain, J. S. and Fritsch, J. M.: A One-Dimensional Entraining/Detraining Plume Model and Its Application in Convective Parameterization, J. Atmos. Sci., 47, 2784–2802, https://doi.org/10.1175/1520-0469(1990)047<2784:AODEPM>2.0.CO;2, 1990. a
Lamsal, L. N., Martin, R. V., Padmanabhan, A., van Donkelaar, A., Zhang, Q., Sioris, C. E., Chance, K., Kurosu, T. P., and Newchurch, M. J.: Application of satellite observations for timely updates to global anthropogenic NOx emission inventories, Geophys. Res. Lett., 38, L05810, https://doi.org/10.1029/2010gl046476, 2011. a
Lapierre, J. L., Laughner, J. L., Geddes, J. A., Koshak, W., Cohen, R. C., and Pusede, S. E.: Observing regional variability in lightning NOx production rates, J. Geophys. Res., in review, 2019. a
Laughner, J. L. and Cohen, R. C.: Quantification of the effect of modeled lightning NO2 on UV–visible air mass factors, Atmos. Meas. Tech., 10, 4403–4419, https://doi.org/10.5194/amt-10-4403-2017, 2017. a, b, c, d, e, f, g
Laughner, J. L. and Zhu, Q.: CohenBerkeleyLab/BEHR-Core: BEHR Core code, Zenodo, https://doi.org/10.5281/zenodo.998275, 2018. a
Laughner, J. L., Zhu, Q., and Cohen, R. C.: The Berkeley High Resolution Tropospheric NO2 product, Earth Syst. Sci. Data, 10, 2069–2095, https://doi.org/10.5194/essd-10-2069-2018, 2018. a
Laughner, J. L., Zhu, Q., and Cohen, R. C.: Evaluation of version 3.0B of the BEHR OMI NO2 product, Atmos. Meas. Tech., 12, 129–146, https://doi.org/10.5194/amt-12-129-2019, 2019. a, b
Levelt, P., Oord, G., R. Dobber, M., Mälkki, A., Visser, H., Vries, J., Stammes, P., Lundell, J., and Saari, H.: The Ozone Monitoring Instrument, IEEE T. Geosci. Remote, 44, 1093–1101, https://doi.org/10.1109/TGRS.2006.872333, 2006. a
Liaskos, C. E., Allen, D. J., and Pickering, K. E.: Sensitivity of tropical tropospheric composition to lightning NOx production as determined by replay simulations with GEOS-5, J. Geophys. Res.-Atmos., 120, 8512–8534, https://doi.org/10.1002/2014JD022987, 2015. a, b, c
Lu, Z., Streets, D. G., de Foy, B., Lamsal, L. N., Duncan, B. N., and Xing, J.: Emissions of nitrogen oxides from US urban areas: estimation from Ozone Monitoring Instrument retrievals for 2005–2014, Atmos. Chem. Phys., 15, 10367–10383, https://doi.org/10.5194/acp-15-10367-2015, 2015. a
Luo, C., Wang, Y., and Koshak, W. J.: Development of a self-consistent lightning NOx simulation in large-scale 3-D models, J. Geophys. Res.-Atmos., 122, 3141–3154, https://doi.org/10.1002/2016JD026225, 2017. a
Mak, H. W. L., Laughner, J. L., Fung, J. C. H., Zhu, Q., and Cohen, R. C.: Improved Satellite Retrieval of Tropospheric NO2 Column Density via Updating of Air Mass Factor (AMF): Case Study of Southern China, Remote Sensing, 10, 1789, https://doi.org/10.3390/rs10111789, 2018. a
Martin, R., Sauvage, B., Folkins, I., Sioris, C., Boone, C., Bernath, P., and Ziemke, J.: Space-based constraints on the production of nitric oxide by lightning, J. Geophys. Res.-Atmos., 112, D09309, https://doi.org/10.1029/2006JD007831, 2007. a, b
Miyazaki, K., Eskes, H. J., and Sudo, K.: Global NOx emission estimates derived from an assimilation of OMI tropospheric NO2 columns, Atmos. Chem. Phys., 12, 2263–2288, https://doi.org/10.5194/acp-12-2263-2012, 2012. a
Miyazaki, K., Eskes, H. J., Sudo, K., and Zhang, C.: Global lightning NOx production estimated by an assimilation of multiple satellite data sets, Atmos. Chem. Phys., 14, 3277–3305, https://doi.org/10.5194/acp-14-3277-2014, 2014. a, b
Nault, B. A., Laughner, J. L., Wooldridge, P. J., Crounse, J. D., Dibb, J., Diskin, G., Peischl, J., Podolske, J. R., Pollack, I. B., Ryerson, T. B., Scheuer, E., Wennberg, P. O., and Cohen, R. C.: Lightning NOx Emissions: Reconciling Measured and Modeled Estimates With Updated NOx Chemistry, Geophys. Res. Lett., 44, 9479–9488, https://doi.org/10.1002/2017GL074436, 2017. a, b, c, d, e
Ott, L. E., Pickering, K. E., Stenchikov, G. L., Allen, D. J., DeCaria, A. J., Ridley, B., Lin, R.-F., Lang, S., and Tao, W.-K.: Production of lightning NOx and its vertical distribution calculated from three-dimensional cloud-scale chemical transport model simulations, J. Geophys. Res., 115, D04301, https://doi.org/10.1029/2009jd011880, 2010. a, b, c
Pickering, K. E., Bucsela, E., Allen, D., Ring, A., Holzworth, R., and Krotkov, N.: Estimates of lightning NOx production based on OMI NO2 observations over the Gulf of Mexico, J. Geophys. Res.-Atmos., 121, 8668–8691, https://doi.org/10.1002/2015JD024179, 2016. a
Pollack, I. B., Homeyer, C. R., Ryerson, T. B., Aikin, K. C., Peischl, J., Apel, E. C., Campos, T., Flocke, F., Hornbrook, R. S., Knapp, D. J., Montzka, D. D., Weinheimer, A. J., Riemer, D., Diskin, G., Sachse, G., Mikoviny, T., Wisthaler, A., Bruning, E., MacGorman, D., Cummings, K. A., Pickering, K. E., Huntrieser, H., Lichtenstern, M., Schlager, H., and Barth, M. C.: Airborne quantification of upper tropospheric NOx production from lightning in deep convective storms over the United States Great Plains, J. Geophys. Res.-Atmos., 121, 2002–2028, https://doi.org/10.1002/2015JD023941, 2016. a, b
Price, C. and Rind, D.: A simple lightning parameterization for calculating global lightning distributions, J. Geophys. Res.-Atmos., 97, 9919–9933, https://doi.org/10.1029/92JD00719, 1992. a
Price, C., Penner, J., and Prather, M.: NOx from lightning: 1. Global distribution based on lightning physics, J. Geophys. Res.-Atmos., 102, 5929–5941, https://doi.org/10.1029/96JD03504, 1997. a
Romps, D. M., Seeley, J. T., Vollaro, D., and Molinari, J.: Projected increase in lightning strikes in the United States due to global warming, Science, 346, 851–854, https://doi.org/10.1126/science.1259100, 2014. a, b, c, d, e, f
Russell, A. R., Valin, L. C., and Cohen, R. C.: Trends in OMI NO2 observations over the United States: effects of emission control technology and the economic recession, Atmos. Chem. Phys., 12, 12197–12209, https://doi.org/10.5194/acp-12-12197-2012, 2012. a
Schumann, U. and Huntrieser, H.: The global lightning-induced nitrogen oxides source, Atmos. Chem. Phys., 7, 3823–3907, https://doi.org/10.5194/acp-7-3823-2007, 2007. a, b, c
Silvern, R. F., Jacob, D. J., Travis, K. R., Sherwen, T., Evans, M. J., Cohen, R. C., Laughner, J. L., Hall, S. R., Ullmann, K., Crounse, J. D., Wennberg, P. O., Peischl, J., and Pollack, I. B.: Observed NO/NO2 Ratios in the Upper Troposphere Imply Errors in NO-NO2-O3 Cycling Kinetics or an Unaccounted NOx Reservoir, Geophys. Res. Lett., 45, 4466–4474, https://doi.org/10.1029/2018GL077728, 2018. a, b
Tippett, M. K. and Koshak, W. J.: A Baseline for the Predictability of U.S. Cloud-to-Ground Lightning, Geophys. Res. Lett., 45, 10719–10728, https://doi.org/10.1029/2018GL079750, 2018. a, b
Toon, O. B., Maring, H., Dibb, J., Ferrare, R., Jacob, D. J., Jensen, E. J., Luo, Z. J., Mace, G. G., Pan, L. L., Pfister, L., Rosenlof, K. H., Redemann, J., Reid, J. S., Singh, H. B., Thompson, A. M., Yokelson, R., Minnis, P., Chen, G., Jucks, K. W., and Pszenny, A.: Planning, implementation, and scientific goals of the Studies of Emissions and Atmospheric Composition, Clouds and Climate Coupling by Regional Surveys (SEAC4RS) field mission, J. Geophys. Res.-Atmos., 121, 4967–5009, https://doi.org/10.1002/2015JD024297, 2016. a
Tost, H., Jöckel, P., and Lelieveld, J.: Lightning and convection parameterisations – uncertainties in global modelling, Atmos. Chem. Phys., 7, 4553–4568, https://doi.org/10.5194/acp-7-4553-2007, 2007. a
Travis, K. R., Jacob, D. J., Fisher, J. A., Kim, P. S., Marais, E. A., Zhu, L., Yu, K., Miller, C. C., Yantosca, R. M., Sulprizio, M. P., Thompson, A. M., Wennberg, P. O., Crounse, J. D., St. Clair, J. M., Cohen, R. C., Laughner, J. L., Dibb, J. E., Hall, S. R., Ullmann, K., Wolfe, G. M., Pollack, I. B., Peischl, J., Neuman, J. A., and Zhou, X.: Why do models overestimate surface ozone in the Southeast United States?, Atmos. Chem. Phys., 16, 13561–13577, https://doi.org/10.5194/acp-16-13561-2016, 2016. a, b
Wong, J., Barth, M. C., and Noone, D.: Evaluating a lightning parameterization based on cloud-top height for mesoscale numerical model simulations, Geosci. Model Dev., 6, 429–443, https://doi.org/10.5194/gmd-6-429-2013, 2013. a, b, c
Zare, A., Romer, P. S., Nguyen, T., Keutsch, F. N., Skog, K., and Cohen, R. C.: A comprehensive organic nitrate chemistry: insights into the lifetime of atmospheric organic nitrates, Atmos. Chem. Phys., 18, 15419–15436, https://doi.org/10.5194/acp-18-15419-2018, 2018. a
Zhao, C., Wang, Y., Choi, Y., and Zeng, T.: Summertime impact of convective transport and lightning NOx production over North America: modeling dependence on meteorological simulations, Atmos. Chem. Phys., 9, 4315–4327, https://doi.org/10.5194/acp-9-4315-2009, 2009. a, b
Zhu, Q. and Laughner, J. L.: CohenBerkeleyLab/WRF-Chem-R2SMH: WRF-Chem code, Zenodo, https://doi.org/10.5281/zenodo.2585381, 2019. a
Zhu, Q., Laughner, J., and Cohen, R.: Berkeley High Resolution (BEHR) OMI NO2 v3.0C – Gridded pixels, daily profiles, v3, UC Berkeley Dash, Dataset, https://doi.org/10.6078/D16X1T, 2019a. a
Zhu, Q., Laughner, J., and Cohen, R.: Berkeley High Resolution (BEHR) OMI NO2 v3.0C – Native pixels, daily profiles, UC Berkeley Dash, Dataset, https://doi.org/10.6078/D1BM2B, 2019b. a