the Creative Commons Attribution 4.0 License.
the Creative Commons Attribution 4.0 License.
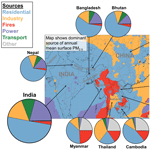
Exploring the impacts of anthropogenic emission sectors on PM2.5 and human health in South and East Asia
Carly L. Reddington
Luke Conibear
Christoph Knote
Ben J. Silver
Yong J. Li
Chak K. Chan
Steve R. Arnold
Dominick V. Spracklen
To improve poor air quality in Asia and inform effective emission-reduction strategies, it is vital to understand the contributions of different pollution sources and their associated human health burdens. In this study, we use the WRF-Chem regional atmospheric model to explore the air quality and human health benefits of eliminating emissions from six different anthropogenic sectors (transport, industry, shipping, electricity generation, residential combustion, and open biomass burning) over South and East Asia in 2014. We evaluate WRF-Chem against measurements from air quality monitoring stations across the region and find the model captures the spatial distribution and magnitude of PM2.5 (particulate matter with an aerodynamic diameter of no greater than 2.5 µm). We find that eliminating emissions from residential energy use, industry, or open biomass burning yields the largest reductions in population-weighted PM2.5 concentrations across the region. The largest human health benefit is achieved by eliminating either residential or industrial emissions, averting 467 000 (95 % uncertainty interval (95UI): 409 000–542 000) or 283 000 (95UI: 226 000–358 000) annual premature mortalities, respectively, in India, China, and South-east Asia, with fire prevention averting 28 000 (95UI: 24 000–32 000) annual premature mortalities across the region. We compare our results to previous sector-specific emission studies. Across these studies, residential emissions are the dominant cause of particulate pollution in India, with a multi-model mean contribution of 42 % to population-weighted annual mean PM2.5. Residential and industrial emissions cause the dominant contributions in China, with multi-model mean contributions of 29 % for both sectors to population-weighted annual mean PM2.5. Future work should focus on identifying the most effective options within the residential, industrial, and open biomass-burning emission sectors to improve air quality across South and East Asia.
- Article
(10870 KB) - Full-text XML
-
Supplement
(1556 KB) - BibTeX
- EndNote
Rapid industrialization and urbanization combined with slow implementation of environmental legislation and clean residential fuels have led to serious air quality problems across Asia. Exposure to poor air quality is associated with detrimental acute and chronic health effects, including premature mortality due to cardiopulmonary diseases and lung cancer (Burnett et al., 2014; Cohen et al., 2017), and reduced life expectancy (Apte et al., 2018). Specifically, exposure to ambient fine particulate matter (with an aerodynamic diameter of no greater than 2.5 µm; PM2.5) pollution is a leading risk factor for human health in Asia and is estimated to cause around 1 million premature deaths every year in both China and India (Global Burden of Diseases, Injuries, and Risk Factors Study 2016 (GBD2016); Cohen et al., 2017; Li et al., 2018; Burnett et al., 2018).
In China, the government has begun to tackle these air quality problems in recent years by introducing policies to reduce air pollutant emissions. Satellite and ground-based measurements indicate that concentrations of some air pollutants (PM2.5 and sulfur dioxide (SO2)) have begun to decline in China within the last decade (Ma et al., 2016; van der A et al., 2017; Lin et al., 2018; Silver et al., 2018; Zhai et al., 2019). India is also introducing policies aimed at addressing the health burden from air pollution (Sagar et al., 2016; Goldemberg et al., 2018). Many of these policies are due to be unified within the upcoming National Clean Air Programme (NCAP) to provide a framework for air quality management with the aim of attaining Indian air quality standards (Ministry of Environment Forests and Climate Change, 2018). However, despite these policies being introduced in China and India, ambient PM2.5 pollution remains a problem in both countries, with measured annual mean concentrations well in excess of the World Health Organization (WHO) Air Quality Guideline concentration of 10 µg m−3 (Brauer et al., 2016; Yang et al., 2018; Silver et al., 2018).
To improve poor air quality in Asia and inform effective emission-reduction strategies, it is vital to understand the major contributing sources and processes that lead to poor air quality and associated human health effects. Policies that have been implemented in North America and Europe to improve air quality may have limited effectiveness in Asia due to differences in emission sources. Therefore, there is a strong need for new research on source contributions specifically focussed on countries in Asia.
To quantify source contributions to PM2.5 and other air pollutants at a regional or national level, atmospheric chemistry-transport models can be applied (e.g. Ying et al., 2014; Hu et al., 2015; Wang et al., 2015; Shi et al., 2017; Timmermans et al., 2017; Qiao et al., 2018) using two main methods. The first method uses a “tagging” approach (also referred to as a “source-attribution” or “source-oriented” approach), where species in the model are tagged to trace the origin of the air pollutant of interest. This technique allows accurate quantification of the contributions of specified emission sources, model processes, and/or source regions to a given air pollutant. The second method uses a “removal” approach (also referred to as a “source-subtraction” approach or “sensitivity analysis”) where multiple model simulations are performed with different emission source sectors or source regions excluded (“zeroed out” or “switched off”). The effective contribution of the source of interest is calculated as the difference in simulated pollutant concentrations between the perturbed simulation and a control simulation (including all sources).
If the behaviour of air pollutants from emission to atmospheric concentration was linear, these two methods would yield the same results. However, the processing and resulting concentrations of certain air pollutants, particularly secondary pollutants (i.e. those partially or exclusively formed in the atmosphere), can be highly non-linear. Following this, the “removal” modelling approach allows accurate quantification of the change in past, current, or future air pollutant concentrations should the specified emission sector be eliminated or reduced as a result of emission control strategies or other reasons. This approach is better suited to testing the results of implementing planned or suggested emission controls on air pollutant concentrations than the “tagging” approach.
Using the “tagging” approach, Shi et al. (2017), Timmermans et al. (2017), and Qiao et al. (2018) analysed the source apportionment of PM2.5 across China. These studies consistently identified residential combustion and industry as the main contributing emission sectors to PM2.5, with some disagreement regarding the importance of the transport sector. Karagulian et al. (2017) used the “removal” approach and also found the largest relative contributions to PM2.5 in China were from the industrial and residential sectors, with the residential sector dominating contributions in India.
By combining atmospheric chemistry-transport models with concentration–response functions (from e.g. Burnett et al., 2014), several studies have quantified the disease burden associated with exposure to ambient PM2.5 from different emission sectors either globally (e.g. Lelieveld et al., 2015; Butt et al., 2016; Silva et al., 2016; Liang et al., 2018) or specifically for India and/or China (Archer-Nicholls et al., 2016; Global Burden of Disease from Major Air Pollution Sources (GBD-MAPS), 2016, 2018; Hu et al., 2017; Aunan et al., 2018; Gao et al., 2018; Gu et al., 2018; Upadhyay et al., 2018; Guo et al., 2018; Conibear et al., 2018a) and South-east Asia (Koplitz et al., 2017). Studies that consider contributions from multiple emission sectors generally find that PM2.5-related health effects are dominated in India by emissions from residential energy use (Lelieveld et al., 2015; Silva et al., 2016; GBD-MAPS, 2018; Upadhyay et al., 2018; Guo et al., 2018; Conibear et al., 2018a) and in China by emissions from residential energy use (Lelieveld et al., 2015; Silva et al., 2016; Liu et al., 2016) and industry (GBD-MAPS, 2016; Hu et al., 2017; Gu et al., 2018). However, the estimates of sectoral contributions to premature mortality from ambient PM2.5 exposure vary widely between the studies, largely caused by differences in the applied mortality estimation approaches (“attribution” or “substitution”; Conibear et al., 2018a), exposure–health impact functions, model processes and structure (including model grid resolution), anthropogenic emissions data, and population data. It is often challenging to distinguish the different methods used in these studies and to understand the implications of the different methods for the results presented.
The implications of using different approaches for estimating the health burden associated with PM2.5 exposure in India were explored and demonstrated recently by Conibear et al. (2018a). Conibear et al. (2018a) found that 52 % of population-weighted annual mean PM2.5 concentrations and 511 000 (95 % uncertainty interval (95UI): 340 000–697 000) annual premature mortalities in India were attributed to residential energy use (the “attribution” approach). However, removing residential emissions would avert only 256 000 (95UI: 162 000–340 000) annual premature mortalities (26 % of the total) (the “substitution” approach), due to the non-linear concentration–response relationship causing health effects to saturate at high PM2.5 concentrations.
To our knowledge, the potential averted disease burden from eliminating multiple different pollution sources has not yet been quantified, specifically for China and South-east Asia at high spatial resolution. Here we use the source-“removal” and mortality-“substitution” approaches in a high-resolution regional model (following Conibear et al., 2018a) to quantify the sector-specific air quality benefit and avoided disease burden in China, mainland Southeast Asia, and the Indian subcontinent. We focus on anthropogenic emission sectors (land transport, industry, agriculture, power generation, residential combustion, and shipping) and open biomass burning (including agricultural and deforestation fires).
In this paper, we also produce the most comprehensive summary to date of previous studies on sector-specific PM2.5 and disease-burden contributions in India and China. We document both the methods used and the results from these previous studies to enable more informed comparisons between them and also to develop a multi-model range in estimates of the sectoral contributions to PM2.5 and disease burden in India and China.
2.1 Model description
To simulate regional PM2.5 concentrations we used the Weather Research and Forecasting model coupled with Chemistry (WRF-Chem; Grell et al., 2005) version 3.7.1, which simulates the emission, transport, mixing, chemical transformation, and removal of trace gases and aerosol simultaneously with meteorology. We use the same model version and set-up as Conibear et al. (2018a), who give a detailed model description in the methods.
Aerosol physics and chemistry are treated using the Model for Simulating Aerosol Interactions and Chemistry (MOSAIC; Zaveri et al., 2008) scheme, including grid-scale aqueous chemistry and extended treatment of organic aerosol (Hodzic and Jimenez, 2011; Hodzic and Knote, 2014). The MOSAIC scheme treats major aerosol species, including sulfate, nitrate, chloride, ammonium, sodium, black carbon, primary and secondary organic aerosol, and other inorganics (including crustal and dust particles and residual primary PM2.5). Four discrete size bins are used within MOSAIC to represent the aerosol size distribution (with the following dry particle diameter ranges: 0.039–0.156, 0.156–0.625, 0.625–2.5, and 2.5–10 µm). Gas-phase chemical reactions are calculated using the Model for Ozone and Related Chemical Tracers, version 4 (MOZART-4) chemical mechanism (Emmons et al., 2010), with several updates to the photochemistry of aromatics, biogenic hydrocarbons, and other species relevant to regional air quality (Knote et al., 2014).
Simulated mesoscale meteorology is kept in line with analysed meteorology through grid nudging to the National Centre for Environmental Prediction (NCEP) Global Forecast System (GFS) analyses to limit errors in mesoscale transport (NCEP, 2000, 2007). The model meteorology was reinitialized every month to avoid drifting of WRF-Chem and spun up for 12 h, while chemistry and aerosol fields were retained to allow for pollution build-up and mesoscale pollutant transport phenomena to be captured. During the simulations, horizontal and vertical wind, potential temperature, and water vapour mixing ratio were nudged to GFS analyses in all model layers. Meteorological conditions were initialized by NCEP GFS 6-hourly analyses at 0.5∘ resolution. These, together with GFS 3 h forecasts in between, were also used for boundary conditions and grid analysis nudging (NCEP, 2000, 2007). MOZART-4/Goddard Earth Observing System Model version 5 (GEOS5) 6-hourly simulation data (NCAR, 2016) were used for chemical and aerosol boundary conditions.
We used two model domains, one over the Indian subcontinent and one over East Asia (including eastern and southern China and mainland South-east Asia). Both model domains use a Lambert conformal conical projection with a horizontal resolution of 30 km × 30 km. The model domain over the Indian subcontinent covers a 140×140 grid (Conibear et al., 2018a), while the model domain over East Asia covers a 130×124 grid. The domains have 33 vertical levels up to a minimum pressure of 10 hPa. We re-gridded the model output, using linear interpolation, onto a regular latitude–longitude grid at resolution. The results presented in Sect. 3 (including the model evaluation statistics, sectoral contributions to PM2.5, and health effects) were all calculated/obtained for the two model domains separately. The two model domains are combined in Fig. 1a for display purposes only (where the domains overlap, the grid cells with the maximum annual mean PM2.5 concentrations in the control simulation are shown).
We calculated the contribution of specific emission sectors to PM2.5 concentrations using the “removal” approach, i.e. by switching off emission sectors one at a time in individual simulations. When the emission sector is switched off in the model, pollution is no longer emitted from that specific source. In reality, the removed emission sector may be replaced by another pollution source, but this scenario was not tested in this study. The main emission sectors investigated were power generation (ENE), industrial non-power (IND), residential energy use (RES), land transport (TRA), and open biomass burning (BBU). We also investigated the agricultural sector (AGR) only in the South Asia domain and the shipping sector (SHP) only in the East Asia domain. All simulations were run for the same time period, with identical reinitialization intervals for the model meteorology (monthly). The simulation period was for 1 year from 00:00 UTC 9 January 2014 to 23:00 UTC 8 January 2015, with the first 8 days of January 2014 run as spin-up.
2.1.1 Description of emissions inventories
Anthropogenic emissions were taken from the Emission Database for Global Atmospheric Research with Task Force on Hemispheric Transport of Air Pollution (EDGAR-HTAP) version 2.2 at horizontal resolution (Janssens-Maenhout et al., 2015). For emissions over Asia EDGAR-HTAPv2.2 uses the Model Intercomparison Study for Asia Phase III (MIX) mosaic Asian anthropogenic emission inventory version 1.0 at horizontal resolution (M. Li et al., 2017). For China, MIX uses the Multiresolution Emission Inventory for China (MEIC) developed by Tsinghua University (http://www.meicmodel.org, last access: 10 September 2019) for 2010 and a high-resolution ammonia (NH3) emission inventory by Peking University (Huang et al., 2012) for 2006 to replace MEIC emissions for NH3 over China. For India, MIX uses the Indian emission inventory for 2010 provided by Argonne National Laboratory (Lu et al., 2011; Lu and Streets, 2012) for sulfur dioxide (SO2), black carbon (BC), and organic carbon (OC) for all sectors as well as nitrogen oxides (NOx) for power plants, and REAS2.1 (Kurokawa et al., 2013) for 2010 for other species. Gaps in EDGAR-HTAPv2.2 were filled by the EDGARv4.3 bottom–up global emission inventory.
The EDGAR-HTAPv2.2 inventory includes emissions of SO2, NOx, carbon monoxide (CO), non-methane volatile organic compounds (NMVOC), NH3, BC, and OC from the following source sectors: aviation, shipping, agriculture, power generation, industrial non-power, land transport, and residential energy use. The following descriptions of these emissions sectors are from Janssens-Maenhout et al. (2015). The aviation sector includes all international and domestic aviation. The shipping sector includes all international (marine) shipping, but not inland waterways. The industrial sector includes emissions from manufacturing, mining, metal, cement, chemical, and solvent industries. Land transport includes all transport by road, railway, inland waterways, pipeline, and other ground transport of mobile machinery. The agricultural sector includes emissions from livestock and crop cultivation, but not from agricultural waste burning or savannah/grassland burning. Emissions from residential energy include small-scale combustion devices for heating, cooking, lighting, and cooling in addition to supplementary engines for residential, commercial, agricultural, solid waste, and wastewater treatment.
Daily mean biomass-burning emissions were taken from the Fire Inventory from NCAR (FINN) version 1.5, with a spatial resolution of 1 km × 1 km (Wiedinmyer et al., 2011) for the year 2014. Biogenic emissions were calculated online by the Model of Emissions of Gases and Aerosol from Nature (MEGAN; Guenther et al., 2006). Dust emissions were calculated online through the Georgia Institute of Technology-Goddard Global Ozone Chemistry Aerosol Radiation and Transport (GOCART) model with Air Force Weather Agency (AFWA) modifications (LeGrand et al., 2019). Anthropogenic dust emissions (re-suspended road dust, construction dust, etc.) are not included. It is important to note that dust emissions may be underestimated across Asia in these simulations (Conibear et al., 2018a).
2.1.2 Health impact estimation
We calculated the disease burden due to exposure to ambient PM2.5 using the Integrated Exposure-Response (IER) functions from the Global Burden of Diseases, Injuries, and Risk Factors Study 2015 (GBD2015) with age-specific modifiers for each disease to estimate the relative risk of premature mortality due to exposure to various PM2.5 concentrations (GBD2015; Cohen et al., 2017). We estimated the disease burden from lower respiratory infection (LRI) for early, late, and post neonatal, and populations between 1 and 80 years upwards in 5-year groupings, and from ischaemic heart disease (IHD), cerebrovascular disease or stroke (STR), chronic obstructive pulmonary disease (COPD), and lung cancer (LC) for adults over 25 years old, split into 5-year age groups. We used the parameter distributions of α, β, and γ from the GBD2015 for 1000 simulations to derive the mean IER with 95 % uncertainty intervals (GBD2015; Cohen et al., 2017). The IER functions have uniform theoretical minimum risk exposure levels (TMREL) for PM2.5 between 2.4 and 5.9 µg m−3. The toxicity of PM2.5 is treated as homogenous regarding source, shape, and chemical composition, consistent with the GBD project, due to lack of composition-dependent exposure–response functions. The calculation of the disease burden and uncertainty is described in further detail in the Supplement (Sect. S1).
As in Conibear et al. (2018a), sector-specific mortality was calculated using the “subtraction” method. The “subtraction” method calculates the sector-specific premature mortality (MSECTOR) as the difference between the premature mortality from all sources (MALL) and the premature mortality when one sector has been removed (MSECTOR_OFF) as in Eq. (1):
We also calculated the sector-specific mortality using the “attribution” method (following Conibear et al., 2018a) to compare our results with previous studies that used this method. The “attribution” method first calculates the fractional sectoral reduction in PM2.5 concentrations from removing an emission sector (PM2.5_SECTOR_OFF) and then uses this fraction to scale the total premature mortality estimate (Eq. 2).
There is large uncertainty associated with calculating the health effects due to exposure to ambient PM2.5, with recent studies suggesting that the IER functions may underestimate relative risk (Yin et al., 2017; Li et al., 2018) and/or disease burden (Burnett et al., 2018). For example, recent epidemiological cohort studies in China suggest that the IER functions may underestimate the relative risk of cause-specific mortality due to long-term exposure to PM2.5 for PM2.5 concentrations experienced in China and other low- and middle-income countries (Yin et al., 2017; Li et al., 2018). These studies suggest that our premature mortality estimates, at least in China, may be conservative.
The population count (P) dataset at resolution was obtained from the Gridded Population of the World, Version 4 (GPWv4), created by the Centre for International Earth Science Information Network (CIESIN) and accessed from the National Aeronautics and Space Administration (NASA) Socioeconomic Data and Applications Centre (SEDAC) (GPWv4, 2016). The United Nations adjusted version was implemented for 2015 with total populations of 1.302 billion for India and 1.380 billion for China (1.402 billion for China and Taiwan). The WRF-Chem model domain used in this study (described in Sect. 2.1) includes 92 % of the population of China. Population age composition was taken from the GBD2015 population estimates for 2015 (GBD Collaborative Network, 2016).
2.2 Aerosol measurements
2.2.1 PM2.5 measurements
To evaluate our model-simulated surface PM concentrations, we used measured annual mean PM2.5 and PM10 concentrations from the WHO (2016, 2018). The database consists of city-average PM2.5 and PM10 concentrations obtained from multiple ground station measurements. Roughly 75 % of measurements are from urban areas of at least 20 000 inhabitants, with the remaining 25 % from smaller areas of up to 20 000 residents. The years of available measurements range from 2008 to 2016. Some cities in the database only have measurements of PM10 concentrations. For these locations, PM2.5 concentrations have been calculated by the WHO from the measured PM10 concentration using national conversion factors (PM2.5 ∕ PM10 ratio) either provided by the country or estimated as population-weighted averages of urban-specific conversion factors (estimated as the mean PM2.5 ∕ PM10 ratio of stations for the same year) for the country (WHO, 2016, 2018). These calculated PM2.5 concentrations make up 41 % of the measurements used in this study (see Table 1). For PM2.5 measurements in Vietnam, we found large differences between measured and converted concentrations and therefore only include measured concentrations in the model evaluation (Sect. 3.1.1) for this country.
Table 1Summary of annual mean PM2.5 measurements from the World Health Organization (WHO) Ambient (outdoor) air quality database (2016, 2018). The table shows the number of stations with available data, the year(s) the measurements were conducted, and the number of reported PM2.5 concentrations that were converted from PM10 measurements (see Sect. 2.3.1). The model normalized mean bias factor (NMBF; Yu et al., 2006) and Pearson's correlation coefficient (r) against observations are given for each country with available WHO measurements. The simulated population-weighted annual mean PM2.5 concentration is given for each country within the model domain (shown in Fig. 1) and the percentage of population “exposed to” (in the same model grid cell as) annual mean PM2.5 concentrations greater than the WHO Air Quality Guideline (AQG; 10 µg m−3) and WHO Interim Target 2 (IT-2; 25 µg m−3) (WHO, 2006, 2016).
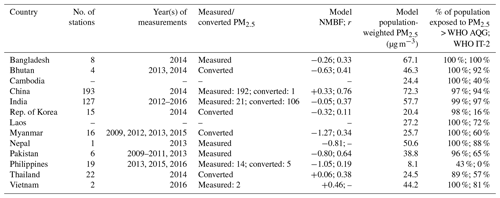
2.2.2 Aerosol composition measurements
To evaluate our model-simulated aerosol composition, we used measured mass concentrations of non-refractory particulate matter species (including organics, sulfate, nitrate, and ammonium) from 33 field campaigns that took place in different locations across China over a range of years (summarized in Table S1 in the Supplement). Measurements were made using aerosol mass spectrometer (AMS) systems and were collected together in a review by Y. J. Li et al. (2017). We also included AMS data from one field campaign in Guangzhou, China, from Qin et al. (2017).
2.2.3 Comparing simulated and measured aerosol concentrations
To evaluate model-simulated annual mean PM2.5 concentrations against measurements from the WHO (in Sect. 3.1.1), we selected measurement years to match or to be or as close as possible to the simulation year of 2014. The simulated annual mean surface PM2.5 concentrations from the control simulation were linearly interpolated to the location of the measurement station, using the longitude and latitude of the central part of the relevant town/city/municipality if the measurement represented an average of multiple stations.
To evaluate simulated aerosol composition against AMS measurements (in Sect. 3.1.2) we averaged total mass concentrations of individual aerosol components in the model (sulfate, nitrate, ammonium, and organic aerosol) over the matching month(s) of each measurement field campaign (Table S1) and linearly interpolated the simulated data to the location of the individual measurement site. Results are shown as an average across all field campaigns.
To quantify the agreement between model and observations, we use the Pearson correlation coefficient (r) and normalized mean bias factor (NMBF) as defined by Yu et al. (2006). A positive NMBF indicates the model overestimates the observations by a factor of NMBF+1. A negative NMBF indicates the model underestimates the observations by a factor of 1−NMBF.
3.1 Model evaluation
3.1.1 PM2.5 concentrations
The model captures the observed spatial distribution of annual mean PM2.5 concentrations, for the year 2014, particularly over China, India, Bangladesh, and Thailand (Fig. 1; r=0.55). Figure 1 compares simulated and measured annual mean PM2.5 concentrations over the Indian subcontinent, mainland South-east Asia, and eastern and southern China. Figure 1a shows that the model simulates high annual mean PM2.5 concentrations (∼80–160 µg m−3) over the Indo-Gangetic Plain in northern India and over the North China Plain and Sichuan Basin regions in China, with lower concentrations simulated over southern and western India, southern China, and mainland South-east Asia. The spatial agreement between model and measurements is improved when comparing against 2014 measurements only (r=0.76) or when we compare against measured PM2.5 only and discard values converted from PM10 (r=0.63).
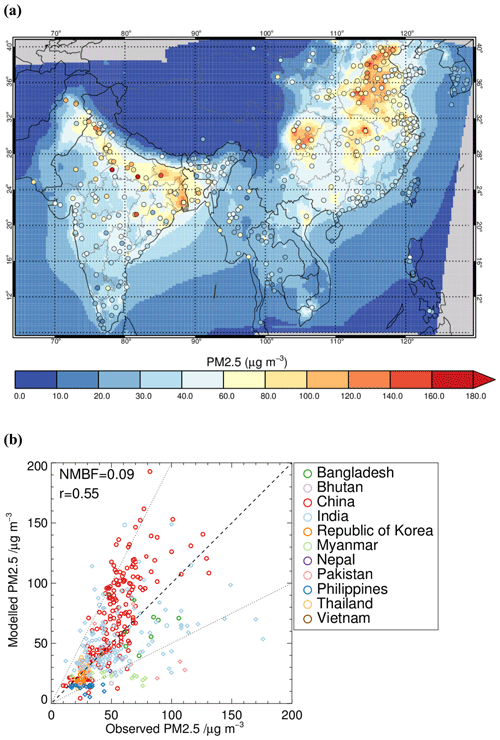
Figure 1Simulated and measured annual mean surface PM2.5 concentrations across South and East Asia. Observation data are from the World Health Organization database, 2016 and 2018. (a) Map of the simulated surface distribution of annual mean PM2.5 for 2014 (underlying colours); overlying circles show measured annual mean PM2.5 concentrations for available years (2009–2016). Regions in grey are outside the model domain. (b) Simulated versus measured annual mean PM2.5 concentrations. Circles show measured annual mean PM2.5 concentrations for the year 2014; diamonds show measured annual mean PM2.5 concentrations for years other than 2014. All simulated annual mean PM2.5 concentrations are for the year 2014. The normalized mean bias factor (NMBF; Yu et al., 2006) and Pearson's correlation coefficient (r) between simulated and measured values are displayed in the top left corner.
Over the whole domain, simulated annual mean PM2.5 concentrations are unbiased against the WHO measurements (Fig. 1b; NMBF = 0.09, equivalent to a factor 1.09 greater than measured values). On average, the model simulates annual mean PM2.5 concentrations within a factor 1.5 of the measurements in China (NMBF = 0.33; Table 1), Thailand (NMBF = 0.06), India (NMBF = −0.05), Bangladesh (NMBF = −0.26), Vietnam (NMBF = 0.46), and the Republic of Korea (NMBF = −0.32), and within a factor 2.3 in Myanmar (NMBF = −1.27), Nepal (NMBF = −0.81), and Bhutan (NMBF = −0.63). The negative model biases (up to a factor 2.27 underestimation) may be due to underestimation of open biomass-burning and anthropogenic emissions in some regions. Simulated PM2.5 concentrations and thus the estimated PM2.5-related disease burdens for countries with negative model biases are likely to be conservative.
In China, the model is positively biased against the measurements for annual mean PM2.5 concentrations above ∼60 µg m−3; this may be due to using anthropogenic emissions data from 2010 and comparing them with measurements from 2014. PM2.5 emissions, particularly those in the industrial and power generation sectors, are reported to have decreased across China between 2010 and 2014 (Zheng et al., 2018). It should be noted, however, that the large majority (89 %) of simulated values at individual stations in China are within a factor 2 of the measurements. Figure S1 in the Supplement shows the model is also able to capture daily variability in measured PM2.5 concentrations at three Chinese megacities, simulating daily mean concentrations within a factor 1.8 of the measurements (NMBF = 0.09–0.80; r=0.47–0.56).
In India, the model is generally unbiased against the measurements (NMBF = −0.05), as reported by Conibear et al. (2018a), who used Central Pollution Control Board (CPCB) measurement data for 2016 to evaluate simulated PM2.5 concentrations. The spatial correlation between simulated and measured annual mean PM2.5 in India (r=0.37; Table 1) is low relative to the model–measurement comparison in China (r=0.76). We suggest this is mainly due to the large range in measurement years for the WHO PM2.5 measurements in India (2012–2016; Table 1), with only 11 stations with measurements available for 2014 (the simulation year) and no available measurements for 2010 (the year of the emissions inventory used). Comparing simulated annual mean PM2.5 against measurements from 2014 only (11 stations), we obtain improved spatial correlation and bias between model and measurements (r=0.67, NMBF = −0.01).
The model is expected to underestimate measured concentrations in countries located towards the boundaries of the regional model domain (the Philippines, Pakistan, and the Republic of Korea) due to increased influence from the coarse-resolution global model and potential missing sources outside the regional model domain. Therefore, we do not present results for these countries in the following sections.
3.1.2 Aerosol composition
Section 3.1.1 shows that the model captures the magnitude of measured PM2.5 concentrations reasonably well across the model domain. Here, we compare the simulated and measured composition of non-refractory submicron particulate matter (NR-PM1) (including organics, sulfate, nitrate, and ammonium) across China (see Table S1). The measurements show that the average composition of NR-PM1 in China is 45.5 % organics, 21.9 % sulfate, 18.5 % nitrate, and 14.1 % ammonium (Fig. S2). In the model the average composition in China is 60.8 % organics, 27.8 % sulfate, 9.5 % nitrate, and 1.8 % ammonium, demonstrating that the model underestimates the contribution of nitrate and ammonium to particulate matter in these simulations. Therefore, we do not test the contribution of agricultural (ammonia) emissions to PM2.5 concentrations in this study. We note that the measurements used in this section are taken from field campaigns that took place over a range of years (2006–2014), with only two campaigns taking place within the simulation year (2014) and four campaigns in the year of the anthropogenic emissions (2010). Therefore, it is likely that some of the model discrepancy in aerosol mass concentrations is due to mismatching meteorology and/or anthropogenic emissions. Future work is needed to run the model over multiple years and match to the exact time of the measurements.
3.2 Contribution of emission sectors to ambient PM2.5 concentrations
3.2.1 Contribution of emission sectors to PM2.5 by country
Figure 2 shows the percentage contribution of each anthropogenic emission sector to the simulated population-weighted annual mean PM2.5 concentration for each country within the model domain. The relative contribution of each sector is calculated for each country as the percentage difference between the simulated population-weighted annual mean PM2.5 concentrations from the control simulation (with all sources included) and from each of the individual eliminated-sector simulations. Results for Afghanistan, Pakistan, the Philippines, and South Korea are not shown in Fig. 2 due to their proximity to the edges of the model domain (Sect. 3.1.1).
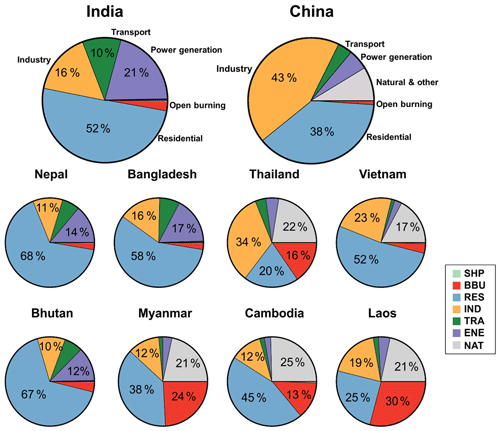
Figure 2Relative contributions of different anthropogenic emission sectors to population-weighted annual mean PM2.5 concentration by country in South and East Asia. Emission sectors include agriculture (AGR; South Asia only), power generation (ENE), industrial non-power (IND), residential energy use (RES), land transport (TRA), open biomass burning (BBU), and shipping (SHP; China and mainland South-east Asia only). Where the percentage contributions from each sector do not add up to 100 %, the residual fraction is assigned to “Natural and other sources” (NAT). Sectors are listed in the legend only if their contribution is visible in the pie charts. Relative contribution values of 10 % or greater are shown in the quadrants. Results are shown for the region of China contained within the model domain, which accounts for 92 % of the Chinese population (Sect. 2.2).
In China, the largest contributions to population-weighted annual mean PM2.5 concentrations are from the industrial (43 %) and residential (38 %) emission sectors, which is consistent with previous studies (see Sect. 4). The next largest contributions are from natural and other sources (including mineral dust, sea spray, biogenic SOA, and agricultural emissions) (9 %), power generation (5 %), and road transport (4 %). In India, the population-weighted annual mean PM2.5 is dominated by the contribution from the residential sector (52 %) as reported in Conibear et al. (2018a), with power generation, industry, and transport contributing 21 %, 16 %, and 10 %, respectively. Open biomass-burning emissions contribute relatively small fractions to the population-weighted annual mean PM2.5 in both China (1 %) and India (3 %). However, it is likely that fire emission datasets underestimate the emissions from agricultural fires in China (e.g. Zhang et al., 2016; Shen et al., 2019) and India (e.g. Cusworth et al., 2018).
In India, there is a noticeably larger fractional contribution of power generation emissions to the population-weighted annual mean PM2.5 concentration (21 %) compared with China (5 %). This is likely for multiple reasons, including lack of regulation, lack of flue-gas desulfurization, and low-energy efficiencies in India (Venkataraman et al., 2018), resulting in higher implied emission factors (emissions per unit of activity) for PM2.5 from power generation in India relative to China (Janssens-Maenhout et al., 2015) and higher fractional contributions of power generation to total primary PM2.5 emissions (16 % of the total in India; 7 % in China, M. Li et al., 2017). Conversely there is a larger contribution of industrial emissions to population-weighted annual mean PM2.5 concentration in China (43 %) than in India (16 %). This is likely due to a larger amount of heavy industry in China compared to in India (primary PM2.5 emissions from industry contribute 50 % to the total emitted PM2.5 in China compared to 18 % in India, M. Li et al., 2017). This is likely to change in the future in India, where industry is becoming dominant under current policies (Conibear et al., 2018b).
In Bangladesh, the contributions to population-weighted annual mean PM2.5 are very similar to those in India, with a larger contribution from the residential sector (58 %) and slightly smaller contributions from power generation (17 %) and transport (7 %) emissions. The contributions from industry (16 %) and open biomass burning (3 %) match those in India. In Nepal and Bhutan, residential emissions are even more dominant, contributing 67 %–68 % of population-weighted annual mean PM2.5.
The residential sector also dominates contributions to population-weighted annual PM2.5 in Myanmar (38 %), Vietnam (52 %), and Cambodia (45 %). Industrial emissions contribute the largest fraction of population-weighted PM2.5 in Thailand (34 %), with relatively large contributions in Laos (19 %) and Vietnam (23 %). In Laos, the population-weighted PM2.5 is dominated by emissions from open biomass burning (30 %). It is likely that open biomass-burning emissions are underestimated in South-east Asia (Reddington et al., 2016; Lasko et al., 2017), and so they may make a larger contribution to PM2.5 concentrations than reported here.
The contribution of natural sources (e.g. biogenic SOA, sea spray, and mineral dust) and other sources (e.g. agriculture and aviation) to population-weighted annual mean PM2.5 is relatively large in China and mainland South-east Asia compared to the Indian subcontinent. Shi et al. (2016) also found a relatively large combined contribution from windblown dust, SOA, and sea salt to province-average PM2.5 concentrations in China (17 %, calculated as the average over the provinces included in our model domain).
The residual PM2.5 concentration classed as from “natural and other” sources also depends on the non-linear effects of simulated air pollutant concentrations when emissions are eliminated in the model. Since the atmospheric chemistry, aerosol processes, and meteorology are fully coupled in WRF-Chem, eliminating primary air pollutant emissions may act to increase PM2.5 concentrations through changes in wind speed, boundary layer depth, secondary aerosol formation, aerosol removal, etc. This would act to increase the calculated contribution of “natural and other” sources to simulated population-weighted annual mean PM2.5 concentrations, although this is typically less than 1 %.
3.2.2 Contribution of emission sectors to PM2.5 by state or province
Figure 3 shows the contribution of each emission sector to the population-weighted annual mean PM2.5 concentration in each province in China (within the model domain) and each state in India. In all Chinese provinces, either industrial or residential emissions make the largest contributions to population-weighted annual mean PM2.5 concentrations, with the exception of Hainan, where natural and other sources make the largest contribution (Fig. 3a). The contributions from residential emissions range from 17 % to 50 %, in general with larger contributions from this sector in northern, western, and central provinces compared to southern and south-eastern provinces, e.g. contributions in Beijing (41 %), Sichuan (49 %), and Hubei (41 %) compared to Guangdong (26 %) and Shanghai (17 %). This is due to greater emissions from heating in colder northern and mountainous regions in winter months (Archer-Nicholls et al., 2016). The contribution of the industrial sector to population-weighted annual mean PM2.5 is prevalent across all provinces (range 23 % to 60 %), with the largest contributions in the major steel-producing provinces of Hebei (47 %) and Jiangsu (47 %), in the major coal-producing province of Shanxi (52 %), and in Shanghai (60 %).
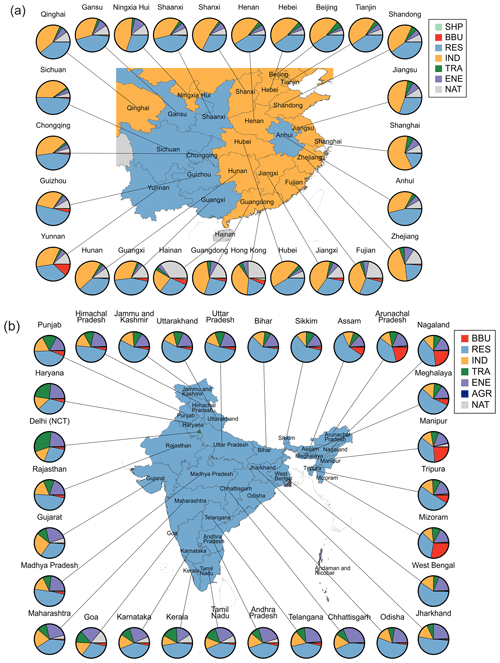
Figure 3Contribution of different emission sectors to population-weighted annual mean PM2.5 concentration (a) by province/municipality/region in China and (b) by state in India (Union Territories are not shown individually, apart from Delhi National Capital Territory (NCT)). The colour of each province in China and each state in India indicates the sector that dominates contributions to population-weighted annual mean PM2.5 in that province or state. The emission sectors are agriculture (AGR; India only), power generation (ENE), industrial non-power (IND), residential energy use (RES), land transport (TRA), open biomass burning (BBU), and shipping (SHP; China only). Where the percentage contributions from each sector do not add up to 100 %, the residual fraction is assigned to “Natural and other sources” (NAT).
The contributions from the other emission sectors (land transport, power generation, shipping, and open biomass burning) to population-weighted annual mean PM2.5 are relatively small (< 13 %) in all provinces. The contribution of power generation emissions ranges from 3 % to 11 %, with the greatest contribution in the provinces of Zhejiang (9 %) and Ningxia (11 %). The land transport sector generally makes the largest contributions in eastern and south-eastern provinces relative to provinces in other regions of China, with the largest contributions in Shanghai (6 %) and Beijng (6 %). We find that the contribution of shipping emissions across China are particularly small relative to the other sectors, with the largest contributions in the Special Administrative Region (SAR) of Hong Kong (2.5 %).
The largest contributions from open biomass-burning emissions are seen in the south-western and southern provinces of China, with the largest contribution in Yunnan province (12 %). These provinces are influenced by transport of smoke from fires in mainland South-east Asia and north-eastern India during the burning season (∼ February to April; see Fig. 5) (Huang et al., 2013; Zhu et al., 2017). Local fires also occur in these regions (Zhang et al., 2016; Zhu et al., 2017; Zhou et al., 2017), which will also contribute to simulated province-average PM2.5 concentrations.
In India (Fig. 3b), residential emissions make the largest contribution to population-weighted annual mean PM2.5 concentrations in all states (range 29 % to 64 %), with the exception of Delhi, where road transport contributes the largest fraction (as reported by Conibear et al., 2018a). In general, the contributions of residential emissions are larger than in Chinese provinces, particularly in the northern and north-eastern states, with the largest contributions in West Bengal (61 %), Sikkim (60 %), Assam (60 %), and Bihar (64 %). Land transport emissions also generally contribute a larger fraction to the population-weighted annual mean PM2.5 in Indian states (range 6 % to 34 %) compared to in Chinese provinces (range 1 % to 6 %), with the largest contributions in Delhi (34 %) and Haryana (25 %).
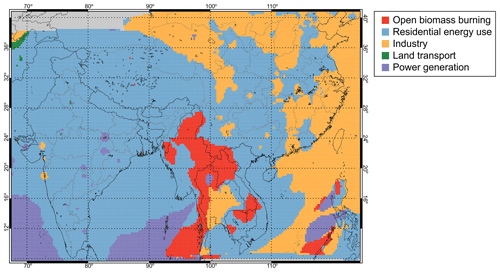
Figure 4Spatial distribution of the dominant anthropogenic emission sectors for annual mean PM2.5 in South and East Asia. The dominant emission sector is calculated for each model grid cell as the emission sector that gives the largest reduction in simulated annual mean surface PM2.5 concentration, i.e. results in the largest absolute difference in µg m−3 from the control simulation. Regions in grey are outside the model domain.
The power generation sector makes relatively large contributions to the population-weighted annual mean PM2.5 across India (range 13 % to 31 %), with larger contributions in all Indian states compared to Chinese provinces within the model domain (range 3 % to 10 %). The largest contributions of power generation emissions are in the states of central India: Chhattisgarh (31 %), Jharkhand (25 %), Maharashtra (24 %), and Andhra Pradesh (25 %), likely due to the large coal-fired power plants located in these states (clustered at the pit heads of coal mines; Guttikunda and Jawahar, 2014). In contrast, contributions from the industrial sector are smaller in almost all states in India (range 11 % to 26 %) compared to the provinces in China (range 23 % to 60 %), with the largest contributions in Gujarat (26 %) and Maharashtra (20 %).
Open biomass-burning emissions make relatively large contributions to PM2.5 in northern and north-eastern states in India, particularly in Mizoram (27 %), Manipur (23 %), and Nagaland (22 %). Agricultural fires (involving burning of crop residues) are widespread across northern India (Vadrevu et al., 2015), with substantial impacts on regional air quality (Liu et al., 2018; Sarkar et al., 2018). North-eastern states may also be affected by transported smoke from deforestation and agricultural fires in neighbouring Myanmar.
3.2.3 Dominant emission sector contributions to PM2.5
Figure 4 shows the spatial distribution of the anthropogenic emission sectors that yield the largest reduction in simulated annual mean surface PM2.5 concentrations. Over the majority of the Indian subcontinent, excluding residential emissions leads to the largest reduction in annual mean PM2.5. In some small regions of India, the largest reductions in PM2.5 are achieved by excluding the power generation (in parts of central-eastern India), transport (in Delhi), and industrial (in eastern Maharashtra and central Gujarat) sectors.
Excluding residential emissions also yields the largest reductions in annual mean PM2.5, relative to the other emission sectors, in Vietnam, southern Myanmar, central Laos and Cambodia, and southern and eastern parts of China. In central and south-eastern China and central Thailand, the largest reductions in annual mean PM2.5 are achieved by excluding industrial emissions. In other parts of mainland South-east Asia (northern and eastern regions of Myanmar and Thailand, and northern and southern regions of Cambodia and Laos), excluding fire emissions gives the largest reductions in simulated annual mean PM2.5 concentrations relative to the other emission sectors.
3.2.4 Seasonal variation in dominant emission sector contributions to PM2.5
Figure 5 shows the seasonal variation in the dominant emission sectors contributing to surface PM2.5 over the South Asia and East Asia model domains. Seasonal variation in anthropogenic sources contributing to PM2.5 is relatively low over much of the Indian subcontinent. Over this region, excluding emissions from residential energy use yields the largest reduction in seasonal mean PM2.5 concentrations throughout the year, with a small increase in the areas dominated by industrial emissions (in Maharashtra and Gujarat in western India) during March to August and power generation emissions (in central India) during March to May. In north-eastern India, the dominant emission sector switches from residential to open biomass burning during March to May. Open biomass-burning emissions can also be seen to dominate over residential emissions in northern India (states of Punjab and Haryana) during September to November, likely due to agricultural burning of rice residues.
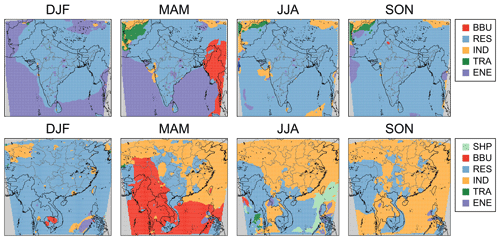
Figure 5Spatial distribution of the dominant anthropogenic emission sectors for seasonal mean PM2.5 in South Asia (top panel) and East Asia (bottom panel). DJF: December, January, February mean; MAM: March, April, May mean; JJA: June, July, August mean; SON: September, October, November mean. As for Fig. 4, the dominant emission sector is calculated for each model grid cell as the emission sector that gives the largest reduction in simulated seasonal mean surface PM2.5 concentration, i.e. results in the largest absolute difference in µg m−3 from the control simulation. Regions in grey are outside the model domain. The emission sectors shown are power generation (ENE), industrial non-power (IND), residential energy use (RES), land transport (TRA), open biomass burning (BBU), and shipping (SHP; East Asia only).
In contrast to India, there is strong seasonal variation in the dominant emission sectors in mainland South-east Asia. During December to February, excluding emissions from residential energy use yields the largest reduction in seasonal mean PM2.5 over much of the region, with fire emissions dominating seasonal mean PM2.5 in Cambodia. During March to May, excluding fire emissions yields the largest reduction in seasonal mean PM2.5 over most of mainland South-east Asia, but also in Taiwan, northern Philippines, eastern India, and south-western China. During July to November, the largest reductions in seasonal mean PM2.5 are achieved by excluding industrial emissions in central and southern Thailand (and Laos during September to November), power generation emissions in northern Thailand, and residential emissions in Myanmar, Cambodia, and Vietnam.
In China, excluding emissions from residential energy use yields the largest reduction in seasonal mean PM2.5 concentrations during the winter months (December to February), with the exception of the heavily industrialized regions of the Pearl River delta (PRD) and Yangtze River delta (YRD), where industrial emissions dominate. During March to November, excluding either residential or industrial emissions yields the largest reductions in seasonal mean PM2.5 in central, eastern, and south-eastern China, depending on the specific region.
3.3 Impacts of emission sectors on human health
Table 1 shows the percentage of population exposed to PM2.5 concentrations above the WHO Air Quality Guideline (AQG) limits for each country in the model domain. Our model simulations show that in 2014, the vast majority of the South and East Asian population was exposed to annual mean PM2.5 concentrations in excess of the WHO AQG of 10 µg m−3 (range per country: 43 %–100 %) and the WHO Level 2 Interim Target (IT-2) of 25 µg m−3 (range per country: 0 %–100 %).
Figure 6a shows the total annual premature mortality due to long-term exposure to ambient PM2.5 from all sources in India, China, and countries in mainland South-east Asia. The spatial distribution of PM2.5-related disease burden in South and East Asia is shown in Fig. S3. We estimate the total annual premature mortality in China (including Taiwan) to be 1 047 000 (95 % uncertainty interval (95UI): 846 000–1 287 000), with 19 679 000 (95UI: 15 622 000–24 580 000) years of life lost (YLL) compared to 990 000 (95UI: 660 000–1 350 000) annual premature mortalities and 24 606 000 (95UI: 14 567 000–32 698 000) YLL in India (Conibear et al., 2018a). The disease burden attributable to exposure to ambient PM2.5 in China is dominated by stroke (29 %; Fig. 6a) IHD (26 %) and COPD (26 %), with smaller contributions from LC (13 %) and LRI (6 %). In India, the fractions of mortality attributable to stroke (14 %) and LC (2 %) are less than in China, with larger fractions from COPD (31 %), IHD (35 %), and LRI (17 %).
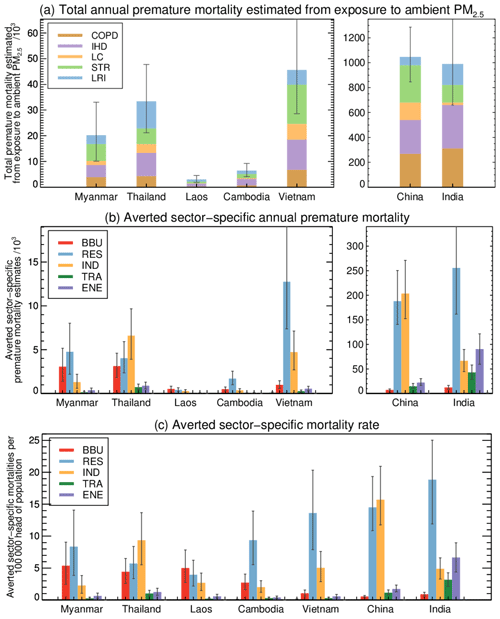
Figure 6(a) Total annual premature mortality per country due to long-term exposure to ambient PM2.5 from all emission sources. The colours show premature mortality by disease (chronic obstructive pulmonary disease (COPD), ischaemic heart disease (IHD), stroke (STR), lung cancer (LC), and lower respiratory infection (LRI)). (b) The number of averted annual premature mortalities due to a reduction in exposure to ambient PM2.5, achieved by eliminating emissions from each sector individually (agriculture (AGR; India only), power generation (ENE), industrial non-power (IND), residential energy use (RES), land transport (TRA), open biomass burning (BBU), and shipping (SHP; East Asia only)). Averted mortalities due to eliminating shipping emissions (in East Asia) and agricultural emissions (in India) are not visible on the plot scale and so are not displayed in the legend. (c) The number of averted annual premature mortalities per 100 000 head of population. Error bars in (a), (b), and (c) represent 95 % uncertainty intervals calculated from combining fractional errors in quadrature (see Sect. S1.1 in the Supplement). Mortality estimates for China include Hong Kong SAR, Macau SAR, and Taiwan.
In mainland South-east Asia, we estimate the total annual premature mortality as 109 000 (95UI: 66 000–160 000) with 2 304 000 (95UI: 1 309 000–3 540 000) YLL. The fraction of premature mortality estimated for each country in South-east Asia scales roughly with population, with the largest fractions in Vietnam (42 %) and Thailand (31 %) and the smallest in Laos (3 %). The disease burden is dominated by IHD in Cambodia (40 %) and Laos (37 %), by stroke in Vietnam (33 %) and Myanmar (33 %), and by LRI in Thailand (31 %).
Our estimates of the total premature mortality due to long-term exposure to ambient PM2.5 compare well with those from GBD2015 (Cohen et al., 2017) for China, India, and countries in South-east Asia (Fig. S4a). The mean estimates from this study lie well within the uncertainty bounds of the values reported by Cohen et al. (2017) for each country, with the exception of Myanmar. For Myanmar, the mean value of Cohen et al. (2017) is higher than the value from this study by a factor 1.5, but lies within our estimated uncertainty range.
Figure 6b and Table 2 show the sector-specific averted annual premature mortality due to a reduction in exposure to ambient PM2.5, using the “substitution” method as described in Sect. 2.2 and Conibear et al. (2018a). The spatial distribution of the averted disease burden is shown in Fig. S3b–h. The summation of sector contributions is 437 000 (95UI: 327 000–582 000) premature mortalities per year in China and Taiwan (42 % of the control simulation), 48 000 (95UI: 27 000–74 000) premature mortalities per year in South-east Asia (45 % of the control simulation), and 469 000 (95UI: 304 000–626 000) premature mortalities per year in India (47 % of the control simulation; Conibear et al., 2018a). It is important to note that these values are substantially lower than if we were to use the attribution method as used in other studies (e.g. Lelieveld et al., 2015; Archer-Nicholls et al., 2016; GBD-MAPS, 2016; Gao et al., 2018) because of the non-linear exposure–response relationship (Conibear et al., 2018a). When using the attribution method, Conibear et al. (2018a) obtained a summation of 1 012 000 (95UI: 675 000–1 381 000) annual premature mortalities in India, equivalent to 102 % of the control simulation.
Table 2Estimated total annual premature mortality due to exposure to ambient PM2.5 in countries in South and East Asia. Also shown is the averted annual premature mortality per country due to a reduction in exposure to ambient PM2.5, calculated using the substitution method. Averted premature mortality estimates are given for each emission sector: biomass burning (BBU), power generation (ENE), industrial non-power (IND), residential energy use (RES), shipping (SHP; East Asia only), and land transport (TRA). Values in bold show the emission sector that gives the largest averted premature mortality for each country/region. “SE Asia” includes Myanmar, Thailand, Laos, Cambodia, and Vietnam (results for these countries are also shown separately). China includes Hong Kong SAR, Macau SAR, and Taiwan. Values in parentheses represent the 95 % uncertainty intervals (95UI). Values are rounded to the nearest 100. Negative values represent increases in estimated premature mortality when an emission sector is removed (due to a increase in simulated PM2.5 concentrations).

The industrial emission sector is the dominant contributor to premature mortalities due to exposure to ambient PM2.5 in China and Thailand. Eliminating emissions from the industrial emission sector would avert 204 000 (95UI: 152 000–271 000) annual premature mortalities in China and 13 000 (8 000–20 000) annual premature mortalities across South-east Asia.
Residential energy use is the dominant contributor to premature mortalities due to exposure to ambient PM2.5 in Vietnam, Myanmar, and Cambodia and is the second largest contributor in China, Thailand, and Laos. Eliminating emissions from residential energy use would avert 188 000 (95UI: 141 000–250 000) and 24 000 (95UI: 13 000–36 000) annual premature mortalities in China and South-east Asia, respectively.
Open biomass burning is the dominant contributor to premature mortalities due to exposure to ambient PM2.5 in Laos. Preventing open biomass burning in East Asia would avert 8000 (95UI: 4000–13 000) annual premature mortalities across South-east Asia and 7000 (95UI: 6000–9000) annual premature mortalities in China.
The land transport and energy generation emission sectors are not dominant contributors to the national/regional annual premature mortality estimates in Fig. 6 and Table 2. However, eliminating emissions from these sectors would still yield a substantial human health benefit in China, averting 15 000 (95UI: 11 000–20 000) and 22 300 (95UI: 16 000–30 000) annual premature mortalities, respectively.
Table 3 summarizes the previous studies that have quantified the emission source/sector contributions to PM2.5 and associated health burden in China and India. These studies have used a range of different of approaches, methods, and tools, which lead to a wide range in estimates of sector-specific contributions to PM2.5 concentrations (Fig. 7; Tables 4 and 5) and annual premature mortalities (Fig. S4; Tables S2 and S3).
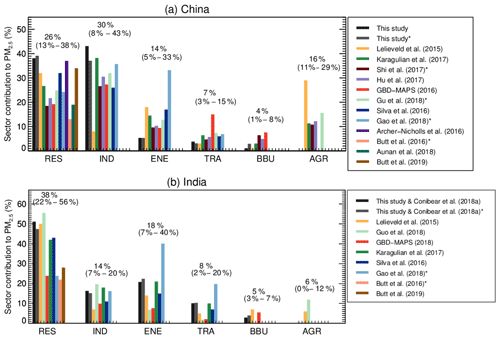
Figure 7Comparison of relative sector-specific contributions to annual mean PM2.5 concentrations in (a) China and (b) India from this study and previous studies. Bars show sector contributions to population-weighted annual mean PM2.5 concentrations, with the exception of the bars associated with studies shown in the legend with an asterisk (*), which show estimated sector contributions to surface area-weighted annual mean PM2.5 concentrations. In our study, population-weighted and area-weighted values differ by less than six percentage points. The mean relative contribution of each sector is shown above the bars with the range of values (minimum to maximum) in parentheses. The values for each study are also shown in Tables 4 and 5. The emission sectors are agriculture (AGR), power generation (ENE), industrial non-power (IND), residential energy use (RES), land transport (TRA), and open biomass burning (BBU). We note that the contribution of the agricultural section to PM2.5 is not quantified for China in this study.
For China we compare the total annual premature mortality estimate from this study to estimates from the previous studies listed in Table 3 (Fig. S4b). Our estimate (1 046 900 (95UI: 846 100–1 286 900)) sits well within the multi-model range of 916 000 to 1 357 000 (UI: 594 000–1 915 000) premature mortalities. Despite the large differences in modelling tools, emissions inventories and health functions used in these studies, our estimate (and uncertainty range) for China overlaps with all previous estimates in Fig. S4 apart from Lelieveld et al. (2015) (whose estimate also includes premature mortality due to exposure to ozone and does not report a UI specifically for China). We note that the larger mortality estimate from Lelieveld et al. (2015) will primarily be due to the GBD2010 IER function, which predicted much larger relative risks for cardiovascular diseases (IHD and stroke) compared to relative risks from GBD2015. The multi-model mean for China is 1 135 000 (UI: 746 000–1 398 000) annual premature mortalities. It is important to note that these estimates apply to a range of years (ranging from 2001 to 2014 in terms of meteorology and from 2005 to 2015 in terms of anthropogenic emissions; Table 3).
Table 3Summary of studies quantifying sector-specific contributions to PM2.5 concentrations and PM2.5-related disease burden in China and/or India (shown in order of publication year). The approaches used to estimate the sector-specific contributions are given based on descriptions included in the published papers and the Supplement. The model grid spacing/resoluton is given in terms of longitude × latitude (for grid resolutions in degrees, approximate conversions to kilometres at the Equator are also given).
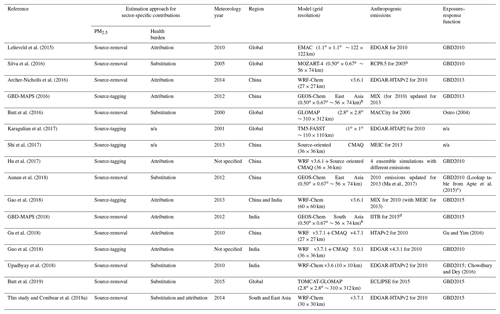
a Representative Concentration Pathway 8.5 global emissions inventory for 2005 (Riahi et al., 2011). b Spatially resolved fractional contributions of different source sectors estimated with GEOS-Chem simulations were multiplied by high-resolution ambient PM2.5 concentration estimates developed for the GBD2015 project to estimate the ambient PM2.5 concentrations attributable to each source sector. c Derived from the IER functions for exposure to PM2.5 and five mortality end-points, as established by Burnett et al. (2014). d IITB (the India Institute of Technology – Bombay) emission inventory (see GBD-MAPS, 2018). n/a – not applicable.
Figure 7 compares estimates of sector-specific contributions to annual mean PM2.5 concentrations in China and India. Previous studies consistently find that residential energy use and industry are the dominant emission sectors in China for annual mean PM2.5 (Fig. 7a and Table 4). Residential emissions contribute an average of 26 % (13 %–38 %) and industrial emissions contribute an average of 30 % (8 %–43 %) to annual mean PM2.5 concentrations in China (see Fig. 7a and Table 4). Other sectors make a smaller contribution, with emissions from power generation contributing an average of 14 % (range 5 %–33 %), land transport an average of 7 % (range 3 %–15 %), open biomass burning an average of 4 % (range 1 %–8 %), and agriculture an average of 16 % (range 11 %–29 %).
In India, previous studies consistently find that residential emissions dominate contributions to annual mean PM2.5 concentrations (Fig. 7b and Table 5), with an average contribution of 38 % (22 %–56 %) over all studies. Other sectors make a smaller contribution, with emissions from industry contributing an average of 14 % (range 7 %–20 %), power generation an average of 18 % (range 7 %–40 %), land transport an average of 8 % (range 2 %–20 %), open biomass burning an average of 5 % (range 3 %–7 %), and agriculture an average of 6 % (range 0.3 %–12 %).
Table 4Comparison of relative sector-specific contributions to simulated annual mean PM2.5 concentrations over China from this study and previous studies. Emission sectors are residential energy use (RES), industrial non-power (IND), power generation (ENE), land transport (TRA), biomass burning (BBU), agriculture (AGR), and shipping (SHP). The largest relative contribution for each study is in bold. The average over all studies (multi-model mean) is shown for population-weighted, area-weighted, and all annual mean PM2.5 concentrations and relative contributions.
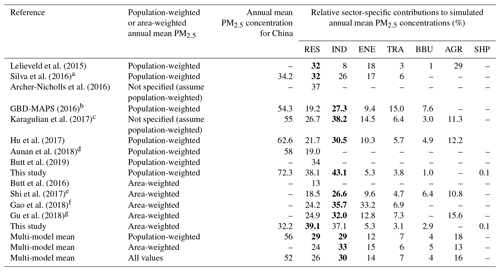
a Relative contributions are for all of East Asia (including China). b Relative contributions calculated using mean values from Table 6 of GBD-MAPS (2016). ENE: power plant coal; IND: industrial coal + non-coal industrial; RES: domestic coal + domestic biomass burning. c Relative contributions calculated from national annual mean PM2.5 concentrations in Sect. 3.1 of Karagulian et al. (2017). The missing sector for China (open biomass burning) was calculated from the remaining fraction of PM2.5 (Table T5 is missing from the report). d Relative contributions calculated from values of “population-weighted exposure to ambient air pollution” in Table 1 of Aunan et al. (2018). e Relative contributions calculated as average fractions across all provinces from Table 3 of Shi et al. (2017). f Relative contributions taken from Fig. S5 of Gao et al. (2018), showing sectoral contributions to area-weighted mean PM2.5 concentrations. g Relative contributions for the RES, IND, and TRA sectors taken from the text (Sect. Impacts on air quality of Gu et al., 2018) assuming these refer to area-weighted annual mean concentrations. Individual relative contributions for AGR and ENE sectors calculated from the combined value in the text (28.4 %) and relative contributions of population-weighted concentrations in Fig. 2 of Gu et al. (2018).
Table 5Comparison of relative sector-specific contributions to simulated annual mean PM2.5 concentrations over India from this study and previous studies. Emission sectors are residential energy use (RES), industrial non-power (IND), power generation (ENE), land transport (TRA), biomass burning (BBU), and agriculture (AGR). The largest relative contribution for each study is in bold. The average over all studies (multi-model mean) is shown for population-weighted, area-weighted, and all annual mean PM2.5 concentrations and relative contributions.
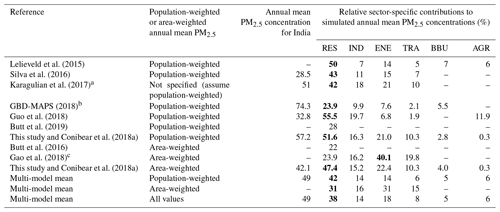
a Relative contributions calculated from national annual mean PM2.5 concentrations quoted in Sect. 3.1 of Karagulian et al. (2017). Two sectors are missing for India (biomass burning and agriculture), so we were unable to calculate these fractions (Table T5 is missing from the report). b Relative contributions taken from Table 2 of GBD-MAPS (2018). c Relative contributions taken from Fig. S5 of Gao et al. (2018), showing sectoral contributions to national mean PM2.5 concentrations. We assume the fraction quoted in the text (32 % in India; Gao et al., 2018) is the contribution to the population-weighted annual mean PM2.5 concentration.
Although previous studies consistently agree on the dominant emission sectors contributing to ambient PM2.5 concentrations in India and China, there is considerable variability in the estimated contribution from each sector. For most sectors the fractional contribution from any one sector varies by a factor of 2 to 5, with the largest range for open biomass burning (up to a factor of 8). Our study is the only one in Table 3 to quantify the contribution of shipping emissions to population-weighted annual mean PM2.5, and so the contribution of this sector is also likely to be uncertain. However, we note that the contribution of shipping emissions to PM2.5 concentrations is only likely to be important for coastal regions (Lv et al., 2018) and relatively small compared to other emission sectors.
We have not quantified the contribution of the agricultural sector to PM2.5 in China. Our model simulations underestimate ammonium concentrations over China (Sect. 3.1.2) and therefore it is likely that we would underestimate the contribution of the agriculture sector to PM2.5 concentrations. Previous studies have found this sector contributes as much as 11 %–29 % (mean 16 %; Table 4) in China and 0.3 %–12 % (mean 6 %; Table 5) in India to annual mean PM2.5 concentrations. There have been fewer studies quantifying the contribution of agriculture to PM2.5 concentrations in China and India relative to the other emission sectors, and the contribution of this sector has large uncertainty. Future work requires a detailed comparison of simulated and observed composition-resolved aerosol mass to help inform these sector-based emission studies.
The different model simulation and anthropogenic emission years will contribute to the range across previous studies, particularly since China and India have experienced rapid changes in emissions in the last decade (Saikawa et al., 2017; Zheng et al., 2018). Reducing the multi-model range in the future will require up-to-date and consistent anthropogenic emissions inventories (with improved quantification of the fractional contributions of the different sectors) to use in air quality models. It will also be important to run the same air quality models at different spatial resolutions to ensure that the fractional contributions of some sectors (e.g. land transport and residential energy use) to ambient PM2.5 concentrations are not underestimated due to missing or underrepresented sub-grid emission sources. Model grid resolution is also important to consider when estimating the health impacts of emissions from different sectors, particularly for land transport and residential energy use, where the exposure (or intake fraction) depends strongly on co-location of sources and high population (US National Research Council, 2012). Comparing model results of emission sector contributions with in situ source-apportionment measurements (as in Karagulian et al., 2017) may help to constrain the range in multi-model estimates.
The large variability in the disease burden estimates (Tables S2 and S3) is strongly influenced by the concentration–response function used in each study. The IER functions were developed for GBD2010 by Burnett et al. (2014). Each subsequent GBD study (2013, 2015, 2016, and 2017) updates the coefficients used to calculate relative risk within the IER functions (Sects. 2.2 and S1) due to the incorporation of more epidemiological evidence. In general, with the same PM2.5 concentration fields, applying coefficients from GBD2010 will yield the highest estimates of relative risk and mortality, applying coefficients from GBD2013 will yield the lowest estimates, while applying coefficients from GBD2015 and GBD2016 will yield medium estimates. Results from GBD2017 give slightly lower estimates of risk and mortality than GBD2015 and GBD2016, primarily due to the different approach to combining risk from household and ambient PM2.5 and avoiding overestimation for those exposed to both. A recent study that constructed a PM2.5-mortality hazard ratio function based only on cohort studies of ambient air pollution, rather than the IER approach of integrating several sources (ambient and household air pollution, passive and active smoking), finds estimates that are 120 % higher than the GBD2015 IER (Burnett et al., 2018). Future work should move to using consistent and up-to-date concentration–response functions to reduce the multi-model range in health impact estimates, although the associated uncertainty range will likely remain large.
In this study we used a high-resolution air quality model to explore the contribution of six different anthropogenic emission sectors to surface PM2.5 concentrations across South and East Asia, and calculated the human health impacts if emissions from each of these sectors were to be eliminated.
We found that the vast majority of the South and East Asian populations are exposed to annual mean PM2.5 concentrations exceeding the WHO Air Quality Guideline, which we estimated to cause 1 047 000 (95U: 846 000–1 287 000), 990 000 (95UI: 660 000–1 350 000), and 109 000 (95UI: 66 000–160 000) annual premature mortalities in China, India, and mainland South-east Asia, respectively. Emissions from the residential, industrial, and open biomass-burning sectors dominate contributions to population-weighted annual mean PM2.5 concentrations in South and East Asia. Eliminating emissions from these sources would substantially reduce the population exposed to ambient concentrations of PM2.5 above the WHO Air Quality Guideline and avert numerous PM2.5-related premature mortalities and years of life lost.
In China, we found that eliminating emissions from the industrial sector yielded the largest reduction in population-weighted annual mean PM2.5 concentrations (by 43 % in our study, on average 29 % across previous studies), averting the largest number of annual premature mortalities (204 000 (95UI: 152 000–271 000) in our study). Eliminating residential solid-fuel combustion also yielded substantial reductions in population-weighted annual mean PM2.5 concentrations (by 38 % in our study, on average 29 % across previous studies) and annual PM2.5-related premature mortalities (188 000 (95UI: 141 000–250 000) in our study).
In South-east Asia, eliminating emissions from residential solid-fuel combustion yielded the largest reductions in population-weighted annual mean PM2.5 in Myanmar (by 38 %), Vietnam (by 52 %), and Cambodia (by 45 %) and the second largest reductions in Thailand (by 20 %) and Laos (by 25 %). Removing this sector would avert 24 000 (95UI: 13 000–36 000) annual premature mortalities across the region. Other important emission sectors in this region are industry and open biomass burning: removing these emissions would avert 13 000 (95UI: 8000–20 000) and 8000 (95UI: 4000–13 000) annual premature mortalities in South-east Asia, respectively.
Future work should focus on identifying the most effective options within the residential, industrial, and open biomass-burning emission sectors to improve air quality across South and East Asia. For the residential sector, switching from solid-fuel combustion to combustion of clean fuels (such as liquefied petroleum gas (LPG)) will likely be the most effective option. Large reductions in ambient PM2.5 concentrations have already been achieved in China between 2005 and 2015, which may have been driven by a reduction in residential emissions from widespread adoption of clean fuels (due to increasing wealth and urbanization rather than control policies) (Zhao et al., 2018). However, despite reductions in ambient PM2.5 concentrations, exposure to air pollution in China remains a leading risk factor for human health. In India, there are programmes now in place to promote LPG to the poorest households (Goldemberg et al., 2018), aiming to increase the use of LPG from 30 % in 2015 to 90 % by the early 2020s. The air quality benefits of these programmes in India are yet to be explored.
Effective options also exist within the agricultural sector to reduce emissions from open biomass burning and improve air quality, including “no burn” alternatives to clearing agricultural residues and/or stricter enforcement of bans on open burning. The occurrence of wildfires is more difficult to control, but may be reduced by improving forest and land management and by employing fire prevention strategies. Emissions from agricultural fires are likely underestimated in China, India, and South-east Asia by the fire emissions dataset used in this study, and so open biomass burning may make a larger contribution to PM2.5 concentrations than reported here. Open biomass-burning emissions in some regions in Asia show strong inter-annual variation, and so contributions to PM2.5 concentrations may vary from year to year. The contribution of open biomass burning to air pollutant concentrations in Asia should be analysed in detail in future work, using additional observations for model constraint.
Anthropogenic emissions are changing rapidly across Asia, leading to large changes in air pollutant concentrations (e.g. Silver et al., 2018), so future work should include more up-to-date emission inventories that are becoming available for China and India to explore how the contributions of emission sectors to PM2.5 pollution have changed over time. There is a strong need for development of up-to-date anthropogenic emission inventories for countries in South-east Asia to improve our understanding of the contributions of pollution sources in this region for recent years.
Previous studies agree that emissions from the residential and industrial sectors dominate population-weighted PM2.5 concentrations in China and emissions from the residential sector dominate in India. Despite this qualitative agreement, we found the contribution of individual sectors varied by a factor of 2–5 or more. It will be important for future work to explore the reasons for these differences between model estimates of the contribution of different sources to air pollutant concentrations and the associated health burden.
This study can inform effective emission-reduction strategies at the local level across South and East Asia to improve air quality and reduce the substantial disease burden from air pollution exposure. Our work has demonstrated that the combustion of solid fuels dominates contributions to ambient PM2.5 concentrations and associated health effects in India, China, and mainland South-east Asia. We therefore recommend that emission-reduction strategies in these countries should focus on reducing the combustion of solid fuels in homes, industry, and through open burning.
Data from all WRF-Chem model simulations and post-processing codes are available from the corresponding author on request. Measured annual mean PM2.5 and PM10 concentrations from the World Health Organization database are available at https://www.who.int/airpollution/data/cities/en/ (WHO, 2018). Campaign-average aerosol mass spectrometer (AMS) measurements of aerosol composition are available from Y. J. Li et al. (2017) (Table S3) and Qin et al. (2017).
The supplement related to this article is available online at: https://doi.org/10.5194/acp-19-11887-2019-supplement.
CLR, DVS and SRA designed the research. CLR performed the WRF-Chem model simulations for the East Asia domain, analysed all the model data and wrote the manuscript. LC performed the WRF-Chem model simulations for the South Asia domain and the health impact calculations. YJL and CKC provided AMS measurement data to evaluate the model with. All authors contributed to scientific discussions and to the manuscript.
The authors declare that they have no conflict of interest.
We gratefully acknowledge the AIA Group Limited and Natural Environment Research Council for funding for this research. This work made use of the facilities of the N8 HPC Centre of Excellence, provided and funded by the N8 consortium and EPSRC (EP/K000225/1). We acknowledge use of the WRF-Chem preprocessor tools bio_emiss, anthro_emiss, fire_emiss, and mozbc provided by the Atmospheric Chemistry Observations and Modeling Lab (ACOM) of the NCAR. We acknowledge use of NCAR/ACOM MOZART-4 global model output available at http://www.acom.ucar.edu/wrf-chem/mozart.shtml (last access: 1 May 2017).
This research has been supported by the Natural Environment Research Council (grant no. NE/N006895/1) and the AIA Group Limited.
This paper was edited by Pedro Jimenez-Guerrero and reviewed by two anonymous referees.
Apte, J. S., Brauer, M., Cohen, A. J., Ezzati, M., and Pope III, C. A.: Ambient PM2.5 Reduces Global and Regional Life Expectancy, Environ. Sci. Technol. Lett., 5, 546–551, https://doi.org/10.1021/acs.estlett.8b00360, 2018.
Archer-Nicholls, S., Carter, E., Kumar, R., Xiao, Q., Liu, Y., Frostad, J., Forouzanfar, M. H., Cohen, A., Brauer, M., Baumgartner, J., and Wiedinmyer C.: The regional impacts of cooking and heating emissions on ambient air quality and disease burden in China, Environ. Sci. Technol., 50, 9416–9423, 2016.
Aunan, K., Ma, Q., Lund, M. T., and Wang, S.: Population-weighted exposure to PM2.5 pollution in China: An integrated approach, Environ. Int., 120, 111–120, https://doi.org/10.1016/j.envint.2018.07.042, 2018.
Brauer, M., Freedman, G., Frostad, J., van Donkelaar, A., Martin, R. V., Dentener, F., van Dingenen, R., Estep, K., Amini, H., Apte, J. S., Balakrishnan, K., Barregard, L., Broday, D., Feigin, V., Ghosh, S., Hopke, P. K., Knibbs, L. D., Kokubo, Y., Liu, Y., Ma, S., Morawska, L., Sangrador, J. L., Shaddick, G., Anderson, H. R., Vos, T., Forouzanfar, M. H., Burnett, R. T., and Cohen, A.: Ambient air pollution exposure estimation for the Global Burden of Disease 2013, Environ. Sci. Technol., 50, 79–88, 2016.
Burnett, R. T., Arden Pope, C., Ezzati, M., Olives, C., Lim, S. S., Mehta, S., Shin, H. H., Singh, G., Hubbell, B., Brauer, M., Anderson, H. R., Smith, K. R., Balmes, J. R., Bruce, N. G., Kan, H., Laden, F., Prüss-Ustün, A., Turner, M. C., Gapstur, S. M., Diver, W. R., and Cohen, A.: An integrated risk function for estimating the global burden of disease attributable to ambient fine particulate matter exposure, Environ. Health Perspect., 122, 397–403, https://doi.org/10.1289/ehp.1307049, 2014.
Burnett, R., Chen, H., Szyszkowicz, M., Fann, N., Hubbell, B., Pope III, C. A., Apte, J. S., Brauer, M., Cohen, A., Weichenthal, S., Coggins, J., Di, Q., Brunekreef, B., Frostad, J., Lim, S. S., Kan, H., Walker, K. D., Thurston, G. D., Hayes, R. B., Lim, C. C., Turner, M. C., Jerrett, M., Krewski, D., Gapstur, S. M., Diver, W. R., Ostro, B., Goldberg, D., Crouse, D. L., Martin, R. V., Peters, P., Pinault, L., Tjepkema, M., van Donkelaar, A., Villeneuve, P. J., Miller, A. B., Yin, P., Zhou, M., Wang, L., Janssen, N. A. H., Marra, M., Atkinson, R. W., Tsang, H., Quoc Thach, T., Cannon, J. B., Allen, R. T., Hart, J. E., Laden, F., Cesaroni, G., Forastiere, F., Weinmayr, G., Jaensch, A., Nagel, G., Concin, H., and Spadaro, J. V.: Global estimates of mortality associated with longterm exposure to outdoor fine particulate matter, P. Natl. Acad. Sci. USA, 115, 9592–9597, https://doi.org/10.1073/pnas.1803222115, 2018.
Butt, E. W., Rap, A., Schmidt, A., Scott, C. E., Pringle, K. J., Reddington, C. L., Richards, N. A. D., Woodhouse, M. T., Ramirez-Villegas, J., Yang, H., Vakkari, V., Stone, E. A., Rupakheti, M. S., Praveen, P. G., van Zyl, P., Beukes, J., Josipovic, M., Mitchell, E. J. S., Sallu, S. M., Forster, P. M., and Spracklen, D. V.: The impact of residential combustion emissions on atmospheric aerosol, human health, and climate, Atmos. Chem. Phys., 16, 873–905, https://doi.org/10.5194/acp-16-873-2016, 2016.
Butt, E. W., Pearce, H., Kilmont, Z., Heyes, C., McNorton, J., Conibear, L., Reddington, C. L., Arnold, S. R., and Spracklen, D. V.: Near-term global and regional air quality and health benefits in 2050 due to widespread adoption of clean residential combustion technologies, in preparation, 2019.
GPWv4: Center for International Earth Science Information Network (CIESIN) & NASA Socioeconomic Data and Applications Center (SEDAC), Gridded Population of the World, Version 4 (GPWv4): Population Density, https://doi.org/10.7927/H4NP22DQ, Columbia University, 2016.
Chowdhury, S. and Dey, S.: Cause-specific premature death from ambient PM2.5 exposure in India: Estimate adjusted for baseline mortality, Environ. Int., 91, 283–290, 2016.
Cohen, A. J., Brauer, M., Burnett, R., Anderson, H. R., Frostad, J., Estep, K., Balakrishnan, K., Brunekreef, B., Dandona, L., Dandona, R., Feigin, V., Freedman, G., Hubbell, B., Jobling, A., Kan, H., Knibbs, L., Liu, Y., Martin, R., Morawska, L., Pope III, C. A., Shin, H., Straif, K., Shaddick, G., Thomas, M., van Dingenen, R., van Donkelaar, A., Vos, T., Murray, C. J. L., and Forouzanfar, M. H.: Estimates and 25-year trends of the global burden of disease attributable to ambient air pollution: an analysis of data from the Global burden of Diseases Study 2015, Lancet, 389, 1907–1918, 2017.
Conibear, L., Butt, E. W., Knote, C., Arnold, S. R., and Spracklen, D. V.: Residential energy use emissions dominate health impacts from exposure to ambient particulate matter in India, Nat. Commun., 9, 617, https://doi.org/10.1038/s41467-018-02986-7, 2018a.
Conibear, L., Butt, E. W., Knote, C., Arnold, S. R., and Spracklen, D. V.: Stringent emission control policies can provide large improvements in air quality and public health in India, GeoHealth, 2, 196–211, https://doi.org/10.1029/2018GH000139, 2018b.
Cusworth, D. H., Mickley, L. J., Payer Sulprizio, M., Marlier, M. E., DeFries, R. S., Liu, T., and Guttikunda, S. K.: Quantifying the influence of agricultural fires in northwest India on urban air pollution in Delhi, India, Environ. Res. Lett., 13, 044018, https://doi.org/10.1088/1748-9326/aab303, 2018.
Emmons, L. K., Walters, S., Hess, P. G., Lamarque, J.-F., Pfister, G. G., Fillmore, D., Granier, C., Guenther, A., Kinnison, D., Laepple, T., Orlando, J., Tie, X., Tyndall, G., Wiedinmyer, C., Baughcum, S. L., and Kloster, S.: Description and evaluation of the Model for Ozone and Related chemical Tracers, version 4 (MOZART-4), Geosci. Model Dev., 3, 43–67, https://doi.org/10.5194/gmd-3-43-2010, 2010.
Gao, M., Beig, G., Song, S., Zhang, H., Hu, J., Ying, Q., Liang, F., Liu, Y., Wang, H., Lu, X., Zhu, T., Carmichael, G. R., Nielsen, C. P., and McElroy, M. B.: The impact of power generation emissions on ambient PM2.5 pollution and human health in China and India, Environ. Int., 121, 250–259, https://doi.org/10.1016/j.envint.2018.09.015, 2018.
GBD 2010 Risk Factors Collaborators: A comparative risk assessment of burden of disease and injury attributable to 67 risk factors and risk factor clusters in 21 regions, 1990–2010: a systematic analysis for the Global Burden of Disease Study 2010, Lancet, 380, 2224–2260, https://doi.org/10.1016/S0140-6736(12)61766-8, 2012.
GBD 2013 Risk Factors Collaborators: Global, regional, and national comparative risk assessment of 79 behavioural, environmental and occupational, and metabolic risks or clusters of risks in 188 countries, 1990–2013: a systematic analysis for the Global Burden of Disease Study 2013, Lancet, 386, 2287–2323, https://doi.org/10.1016/S0140-6736(15)00128-2, 2015.
GBD 2015 Risk Factors Collaborators: Global, regional, and national comparative risk assessment of 79 behavioural, environmental and occupational, and metabolic risks or clusters of risks, 1990–2015: a systematic analysis for the Global Burden of Disease Study 2015, Lancet, 388, 1659–1724, https://doi.org/10.1016/S0140-6736(16)31679-8, 2016.
GBD 2016 Risk Factors Collaborators: Global, regional, and national comparative risk assessment of 84 behavioural, environmental and occupational, and metabolic risks or clusters of risks, 1990–2016: a systematic analysis for the Global Burden of Disease Study 2016, Lancet, 390, 1345–1422, https://doi.org/10.1016/S0140-6736(17)32366-8, 2017.
GBD 2017 Risk Factors Collaborators: Global, regional, and national comparative risk assessment of 84 behavioural, environmental and occupational, and metabolic risks or clusters of risks for 195 countries and territories, 1990–2017: a systematic analysis for the Global Burden of Disease Study 2017, Lancet, 392, 1923–1994, https://doi.org/10.1016/S0140-6736(18)32225-6, 2018.
GBD Collaborative Network: Global Burden of Disease Study 2015 (GBD2015) Population Estimates 1970–2015, available at: http://ghdx.healthdata.org/record/ (last access: 27 August 2019), Seattle, United States: Institute for Health Metrics and Evaluation (IHME), 2016.
GBD-MAPS (Global Burden of Disease from Major Air Pollution Sources) Working Group: Burden of Disease Attributable to Coal-burning and Other Major Sources of Air Pollution in China, Special Report 20, Health Effects Institute, Boston, MA, available at: https://www.healtheffects.org/publication/ (last access: 25 September 2018), 2016.
GBD-MAPS (Global Burden of Disease from Major Air Pollution Sources) Working Group: Burden of Disease Attributable to Major Air Pollution Sources in India, Special Report 21. Health Effects Institute, Boston, MA, available at: https://www.healtheffects.org/publication/gbd-air-pollution-india (last access: 12 November 2018), 2018.
Goldemberg, J., Martinez-Gomez, J., Sagar, A., and Smith, K. R: Household air pollution, health, and climate change: cleaning the air, Environ. Res. Lett., 13, 030201, https://doi.org/10.1088/1748-9326/aaa49d, 2018.
Grell, G. A., Peckham, S. E., Schmitz, R., McKeen, S. A., Frost, G., Skamarock, W. C., and Edere, B.: Fully coupled “online” chemistry within the WRF model, Atmos. Environ., 39, 6957–6975, 2005.
Gu, Y. and Yim, S. H. L.: The air quality and health impacts of domestic trans-boundary pollution in various regions of China, Environ. Int., 97, 117–124, 2016.
Gu, Y., Wong, T. W., Law, C. K., Dong, G. H., Ho, K. F., Yang, Y., and Yim, S. H. L.: Impacts of sectoral emissions in China and the implications: air quality, public health, crop production, and economic costs, Environ. Res. Lett., 13, 084008, https://doi.org/10.1088/1748-9326/aad138, 2018.
Guenther, A., Karl, T., Harley, P., Wiedinmyer, C., Palmer, P. I., and Geron, C.: Estimates of global terrestrial isoprene emissions using MEGAN (Model of Emissions of Gases and Aerosols from Nature), Atmos. Chem. Phys., 6, 3181–3210, https://doi.org/10.5194/acp-6-3181-2006, 2006.
Guo, H., Kota, S. H., Chen, K., Sahu, S. K., Hu, J., Ying, Q., Wang, Y., and Zhang, H.: Source contributions and potential reductions to health effects of particulate matter in India, Atmos. Chem. Phys., 18, 15219–15229, https://doi.org/10.5194/acp-18-15219-2018, 2018.
Guttikunda, S. K. and Jawahar, P.: Atmospheric emissions and pollution from the coal- fired thermal power plants in India, Atmos. Environ., 92, 449–460, 2014.
Hodzic, A. and Jimenez, J. L.: Modeling anthropogenically controlled secondary organic aerosols in a megacity: a simplified framework for global and climate models, Geosci. Model Dev., 4, 901–917, https://doi.org/10.5194/gmd-4-901-2011, 2011.
Hodzic, A. and Knote, C.: WRF-Chem 3.6.1: MOZART gas-phase chemistry with MOSAIC aerosols, Atmospheric Chemistry Division (ACD), National Center for Atmospheric Research (NCAR), 1–9, 2014.
Hu, J., Wu, L., Zheng, B., Zhang, Q., He, K., Chang, Q., Li, X., Yang, F., Ying, Q., and Zhang, H.: Source contributions and regional transport of primary particulate matter in China, Environ. Pollut., 207, 31–42, 2015.
Hu, J., Huang, L., Chen, M., Liao, H., Zhang, H., Wang, S., Zhang, Q., and Ying, Q.: Premature mortality attributable to particulate matter in China: source contributions and responses to reductions, Environ. Sci. Technol., 51, 9950–9959, 2017.
Huang, X., Song, Y., Li, M., Li, J., Huo, Q., Cai, X., Zhu, T., Hu, M., and Zhang, H.: A high-resolution ammonia emission inventory in China, Global Biogeochem. Cy., 26, GB1030, https://doi.org/10.1029/2011GB004161, 2012.
Huang, K., Fu, J. S., Hsu, N. C., Gao, Y., Dong, X., Tsay, S.-C. and Lam, Y. F.: Impact assessment of biomass burning on air quality in Southeast and East Asia during BASE-ASIA, Atmos. Environ., 78, 291–302, 2013.
Janssens-Maenhout, G., Crippa, M., Guizzardi, D., Dentener, F., Muntean, M., Pouliot, G., Keating, T., Zhang, Q., Kurokawa, J., Wankmüller, R., Denier van der Gon, H., Kuenen, J. J. P., Klimont, Z., Frost, G., Darras, S., Koffi, B., and Li, M.: HTAP_v2.2: a mosaic of regional and global emission grid maps for 2008 and 2010 to study hemispheric transport of air pollution, Atmos. Chem. Phys., 15, 11411–11432, https://doi.org/10.5194/acp-15-11411-2015, 2015.
Karagulian, F., Van Dingenen, R., Belis, C. A., Janssens-Maenhout, G., Crippa, M., Guizzardi, D., and Dentener, F.: Attribution of anthropogenic PM2.5 to emission sources, EUR 28510 EN, 1–43, https://doi.org/10.2760/344371, 2017.
Knote, C., Hodzic, A., Jimenez, J. L., Volkamer, R., Orlando, J. J., Baidar, S., Brioude, J., Fast, J., Gentner, D. R., Goldstein, A. H., Hayes, P. L., Knighton, W. B., Oetjen, H., Setyan, A., Stark, H., Thalman, R., Tyndall, G., Washenfelder, R., Waxman, E., and Zhang, Q.: Simulation of semi-explicit mechanisms of SOA formation from glyoxal in aerosol in a 3-D model, Atmos. Chem. Phys., 14, 6213–6239, https://doi.org/10.5194/acp-14-6213-2014, 2014.
Koplitz, S. N., Jacob, D. J., Sulprizio, M. P., Myllyvirta, L., and Reid, C.: Burden of disease from rising coal-fired power plant emissions in Southeast Asia, Environ. Sci. Technol., 51, 1467–1476, 2017.
Kurokawa, J., Ohara, T., Morikawa, T., Hanayama, S., Janssens-Maenhout, G., Fukui, T., Kawashima, K., and Akimoto, H.: Emissions of air pollutants and greenhouse gases over Asian regions during 2000–2008: Regional Emission inventory in ASia (REAS) version 2, Atmos. Chem. Phys., 13, 11019–11058, https://doi.org/10.5194/acp-13-11019-2013, 2013.
Lasko, K., Vadrevu, K. P., Tran, V. T., Ellicott, E., Nguyen, T. T. N., Bui, H. Q., and Justice, C.: Satellites may underestimate rice residue and associated burning emissions in Vietnam, Environ. Res. Lett., 12, 085006, https://doi.org/10.1088/1748-9326/aa751d, 2017.
LeGrand, S. L., Polashenski, C., Letcher, T. W., Creighton, G. A., Peckham, S. E., and Cetola, J. D.: The AFWA dust emission scheme for the GOCART aerosol model in WRF-Chem v3.8.1, Geosci. Model Dev., 12, 131–166, https://doi.org/10.5194/gmd-12-131-2019, 2019.
Lelieveld, J., Evans, J. S., Fnais, M., Giannadaki, D., and Pozzer, A.: The contribution of outdoor air pollution sources to premature mortality on a global scale, Nature, 525, 367–371, 2015.
Li, M., Zhang, Q., Kurokawa, J.-I., Woo, J.-H., He, K., Lu, Z., Ohara, T., Song, Y., Streets, D. G., Carmichael, G. R., Cheng, Y., Hong, C., Huo, H., Jiang, X., Kang, S., Liu, F., Su, H., and Zheng, B.: MIX: a mosaic Asian anthropogenic emission inventory under the international collaboration framework of the MICS-Asia and HTAP, Atmos. Chem. Phys., 17, 935–963, https://doi.org/10.5194/acp-17-935-2017, 2017.
Li, Y. J., Sun, Y., Zhang, Q., Li, X., Li, M., Zhou, Z., and Chan, C. K.: Real-time chemical characterization of atmospheric particulate matter in China: A review, Atmos. Environ., 158, 270–304, https://doi.org/10.1016/j.atmosenv.2017.02.027, 2017.
Li, T., Zhang, Y., Wang, J., Xu, D., Yin, Z., Chen, H., Lv, Y., Luo, J., Zeng, Y., Liu, Y., Kinney, P. L., and Shi, X.: All-cause mortality risk associated with long-term exposure to ambient PM2.5 in China: a cohort study, The Lancet Public Health, 3, 470–477, 2018.
Liang, C.-K., West, J. J., Silva, R. A., Bian, H., Chin, M., Davila, Y., Dentener, F. J., Emmons, L., Flemming, J., Folberth, G., Henze, D., Im, U., Jonson, J. E., Keating, T. J., Kucsera, T., Lenzen, A., Lin, M., Lund, M. T., Pan, X., Park, R. J., Pierce, R. B., Sekiya, T., Sudo, K., and Takemura, T.: HTAP2 multi-model estimates of premature human mortality due to intercontinental transport of air pollution and emission sectors, Atmos. Chem. Phys., 18, 10497–10520, https://doi.org/10.5194/acp-18-10497-2018, 2018.
Lin, C. Q., Liu, G., Lau, A. K. H., Li, Y., Li, C. C., Fung, J. C. H., and Lao, X. Q.: High-resolution satellite remote sensing of provincial PM2.5 trends in China from 2001 to 2015, Atmos. Environ., 180, 110–116, https://doi.org/10.1016/j.atmosenv.2018.02.045, 2018.
Liu, J., Mauzerall, D. L., Chen, Q., Zhang, Q., Song, Y., Peng, W., Klimont, Z., Qiu, X., Zhang, S., Hu, M., Lin, W., Smith, K. R., and Zhu, T.: Air pollutant emissions from Chinese households: A major and underappreciated ambient pollution source, P. Natl. Acad. Sci. USA, 113, 7756–7761, https://doi.org/10.1073/pnas.1604537113, 2016.
Liu, T., Marlier, M. E., Defries, R. S., Westervelt, D. M., Xia, K. R., Fiore, A. M., Mickley, L. J., Cusworth, D. H., and Milly, G.: Seasonal impact of regional outdoor biomass burning on air pollution in three Indian cities: Delhi, Bengaluru, and Pune, Atmos. Environ., 172, 83–92, 2018.
Lu, Z., Zhang, Q., and Streets, D. G.: Sulfur dioxide and primary carbonaceous aerosol emissions in China and India, 1996–2010, Atmos. Chem. Phys., 11, 9839–9864, https://doi.org/10.5194/acp-11-9839-2011, 2011.
Lu, Z. and Streets, D. G.: Increase in NOx emissions from Indian thermal power plants during 1996–2010: unit-based inventories and multisatellite observations, Environ. Sci. Technol., 46, 7463–7470, 2012.
Lv, Z., Liu, H., Ying, Q., Fu, M., Meng, Z., Wang, Y., Wei, W., Gong, H., and He, K.: Impacts of shipping emissions on PM2.5 pollution in China, Atmos. Chem. Phys., 18, 15811–15824, https://doi.org/10.5194/acp-18-15811-2018, 2018.
Ma, Z., Hu, X., Sayer, A. M., Levy, R., Zhang, Q., Xue, Y., Tong, S., Bi, J., Huang, L., and Liu, Y.: Satellite-based spatiotemporal trends in PM2.5 concentrations: China, 2004–2013, Environ. Health Perspect., 124, 184–192, https://doi.org/10.1289/ehp.1409481, 2016.
Ministry of Environment Forests and Climate Change: National Clean Air Programme (NCAP) India, Retrieved September 14, 2018, available at: http://www.moef.gov.in/sites/default/files/NCAP with annex-ilovepdf-compressed.pdf, last access: 14 September 2018.
NCAR (National Center for Atmospheric Research): ACOM MOZART-4/GEOS-5 global model output, available at: http://www.acom.ucar.edu/wrf-chem/mozart.shtml (last access: 1 May 2017), UCAR, 2016.
NCEP: National Weather Service, NOAA & US Department of Commerce. NCEP Final (FNL) Operational Model Global Tropospheric Analyses, continuing from July 1999. Research Data Archive at the National Center for Atmospheric Research, Computational and Information Systems Laboratory, https://doi.org/10.5065/D6M043C6, 2000.
NCEP: National Weather Service, NOAA & US Department of Commerce. NCEP Global Forecast System (GFS) Analyses and Forecasts. Research Data Archive at the National Center for Atmospheric Research, Computational and Information Systems Laboratory, available at: http://rda.ucar.edu/datasets/ds084.6/, 2007.
Ostro, B.: Outdoor air pollution: Assessing the environmental burden of disease at national and local levels, WHO Environmental Burden of Disease Series No. 5, World Health Organization (WHO), Geneva, 2004.
Qiao, X., Ying, Q., Li, X., Zhang, H., Hu, J., Tang, Y., and Chen, X.: Source apportionment of PM2.5 for 25 Chinese provincial capitals and municipalities using a source-oriented community multiscale air quality model, Sci. Total Environ., 612, 462–471, 2018.
Qin, Y. M., Tan, H. B., Li, Y. J., Schurman, M. I., Li, F., Canonaco, F., Prévôt, A. S. H., and Chan, C. K.: Impacts of traffic emissions on atmospheric particulate nitrate and organics at a downwind site on the periphery of Guangzhou, China, Atmos. Chem. Phys., 17, 10245–10258, https://doi.org/10.5194/acp-17-10245-2017, 2017.
Riahi, K., Rao, S., Krey, V., Cho, C., Chirkov, V., Fischer, G., Kindermann, G., Nakicenovic, N., and Rafaj, P.: RCP8.5 – A scenario of comparatively high greenhouse gas emissions, Clim. Change, 109, 33 pp., https://doi.org/10.1007/s10584-011-0149-y, 2011.
Reddington, C. L., Spracklen, D. V., Artaxo, P., Ridley, D. A., Rizzo, L. V., and Arana, A.: Analysis of particulate emissions from tropical biomass burning using a global aerosol model and long-term surface observations, Atmos. Chem. Phys., 16, 11083–11106, https://doi.org/10.5194/acp-16-11083-2016, 2016.
Sagar, A., Balakrishnan, K., Guttikunda, S., Roychowdhury, A., and Smith, K. R.: India leads the way: A health-centered strategy for air pollution, Environ. Health Perspect., 124, 116–117, https://doi.org/10.1289/EHP90, 2016.
Saikawa, E., Trail, M., Zhong, M., Wu, Q., Young, C. L., Janssens-Maenhout, G., Kurokawa, J. I., Klimont, Z., Wagner, F., Naik, V., Horowitz, L. W., Zhao, Y., Nagpure, A., Gurjar, B., and Zhang, Q: Uncertainties in emissions estimates of greenhouse gases and air pollutants in India and their impacts on regional air quality, Environ. Res. Lett., 12, 065002, https://doi.org/10.1088/1748-9326/aa6cb4, 2017.
Sarkar, S., Singh, R. P., and Chauhan, A.: Crop residue burning in northern India: Increasing threat to Greater India, J. Geophys. Res.-Atmos., 123, 6920–6934, https://doi.org/10.1029/2018JD028428, 2018.
Shen, L., Jacob, D. J., Zhu, L., Zhang, Q., Zheng, B., Sulprizio, M. P., Li, K., De Smedt, I., González Abad, G., Cao, H., Fu, T.‐M., and Liao, H.: The 2005–2016 trends of formaldehyde columns over China observed by satellites: Increasing anthropogenic emissions of volatile organic compounds and decreasing agricultural fire emissions, Geophys. Res. Lett., 46, 4468–4475, https://doi.org/10.1029/2019GL082172, 2019.
Shi, Z., Li, J., Huang, L., Wang, P., Wu, L., Ying, Q., Zhang, H., Lu, L., Liu, X., Liao, H., and Hu, J.: Source apportionment of fine particulate matter in China in 2013 using a source-oriented chemical transport model, Sci. Total Environ., 601, 1476–1487, 2017.
Silva, R. A., Adelman, Z., Fry, M. M., and West, J. J.: The impact of individual anthropogenic emissions sectors on the global burden of human mortality due to ambient air pollution, Environ. Health Perspect., 124, 1776–1784, 2016.
Silver, B., Reddington, C. L., Arnold, S., and Spracklen, D. V.: Substantial changes in air pollution across China during 2015 to 2017, Environ. Res. Lett., 13, 114012, https://doi.org/10.1088/1748-9326/aae718, 2018.
Timmermans, R., Kranenburg, R., Manders, A., Hendriks, C., Segers, A., Dammers, E., Denier van der Gon, H., Schaap, M., Dammers, E., Zeng, L., Wang, L., and Liu, Z.: Source apportionment of PM2.5 across China using LOTOS-EUROS, Atmos. Environ., 164, 370–386, https://doi.org/10.1016/j.atmosenv.2017.06.003, 2017.
Upadhyay, A., Dey, S., Chowdhury, S., and Goyal, P.: Expected health benefits from mitigation of emissions from major anthropogenic PM2.5 sources in India: Statistics at state level, Environ. Pollut., 242, 1817–1826, https://doi.org/10.1016/j.envpol.2018.07.085, 2018.
US National Research Council: Exposure Science in the 21st Century: A Vision and a Strategy, Washington DC, The National Academies Press, 210 pp., https://doi.org/10.17226/13507, 2012.
Vadrevu, K. P., Lasko, K., Giglio, L., and Justice, C.: Vegetation fires, absorbing aerosols and smoke plume characteristics in diverse biomass burning regions of Asia, Environ. Res. Lett., 10, 105003, https://doi.org/10.1039/c4em00307a, 2015.
van der A, R. J., Mijling, B., Ding, J., Koukouli, M. E., Liu, F., Li, Q., Mao, H., and Theys, N.: Cleaning up the air: effectiveness of air quality policy for SO2 and NOx emissions in China, Atmos. Chem. Phys., 17, 1775–1789, https://doi.org/10.5194/acp-17-1775-2017, 2017.
Venkataraman, C., Brauer, M., Tibrewal, K., Sadavarte, P., Ma, Q., Cohen, A., Chaliyakunnel, S., Frostad, J., Klimont, Z., Martin, R. V., Millet, D. B., Philip, S., Walker, K., and Wang, S.: Source influence on emission pathways and ambient PM2.5 pollution over India (2015–2050), Atmos. Chem. Phys., 18, 8017–8039, https://doi.org/10.5194/acp-18-8017-2018, 2018.
Wang, L., Wei, Z., Wei, W., Fu, J. S., Meng, C., and Ma, S.: Source apportionment of PM2.5 in top polluted cities in Hebei, China using the CMAQ model, Atmos. Environ., 122, 723–736, 2015.
World Health Organization (WHO): Air quality guidelines for particulate matter, ozone, nitrogen dioxide and sulfur dioxide: Global update 2005, Summary of risk assessment, available at: http://www.who.int/phe/health_topics/outdoorair/outdoorair_aqg/en/ (last access: 27 August 2019), 2006.
World Health Organization (WHO): Ambient Air Pollution: A Global Assessment Of Exposure And Burden Of Disease, Vol. 121, World Health Organization, Geneva, 1–131, 2016.
WHO: WHO Global Air Quality Database, Version 1.0, Geneva, World Health Organization, available at: https://www.who.int/airpollution/data/cities/en/, last access: 30 August 2018.
Wiedinmyer, C., Akagi, S. K., Yokelson, R. J., Emmons, L. K., Al-Saadi, J. A., Orlando, J. J., and Soja, A. J.: The Fire INventory from NCAR (FINN): a high resolution global model to estimate the emissions from open burning, Geosci. Model Dev., 4, 625–641, https://doi.org/10.5194/gmd-4-625-2011, 2011.
Yang, X., Jiang, L., Zhao, W., Xiong, Q., Zhao, W., and Yan, X.: Comparison of ground-based PM2.5 and PM10 concentrations in China, India, and the US, Int. J. Environ. Res. Public Health, 15, 1382, https://doi.org/10.3390/ijerph15071382, 2018.
Yin, P., Brauer, M., Cohen, A., Burnett, R. T., Liu, J., Liu, Y., Liang, R., Wang, W., Qi, J., Wang, L., and Zhou, M.: Long-term fine particulate matter exposure and nonaccidental and cause-specific mortality in a large national cohort of Chinese men, Environ. Health Perspect., 125, 117002, https://doi.org/10.1289/EHP1673, 2017.
Ying, Q., Wu, L., and Zhang, H.: Local and inter-regional contributions to PM2.5 nitrate and sulfate in China, Atmos. Environ., 94, 582–592, 2014.
Yu, S., Eder, B., Dennis, R., Chu, S.-H., and Schwartz, S. E.: New unbiased symmetric metrics for evaluation of air quality models, Atmosph. Sci. Lett., 7, 26–34, https://doi.org/10.1002/asl.125, 2006.
Zaveri, R. A., Easter, R. C., Fast, J. D., and Peters, L. K.: Model for Simulating Aerosol Interactions and Chemistry (MOSAIC), J. Geophys. Res.-Atmos., 113, D13204, https://doi.org/10.1029/2007JD008782, 2008.
Zhai, S., Jacob, D. J., Wang, X., Shen, L., Li, K., Zhang, Y., Gui, K., Zhao, T., and Liao, H.: Fine particulate matter (PM2.5) trends in China, 2013–2018: contributions from meteorology, Atmos. Chem. Phys. Discuss., https://doi.org/10.5194/acp-2019-279, in review, 2019.
Zhang, L., Liu, Y., and Hao, L.: Contributions of open crop straw burning emissions to PM2.5 concentrations in China, Environ. Res. Lett., 11, 014014, https://doi.org/10.1088/1748-9326/11/1/014014, 2016.
Zhao, B, Zheng, H., Wang, S., Smith, K. R., Lu, X., Aunan, K., Gu, Y., Wang, Y., Ding, D., Xing, J., Fu, X., Yang, X., Liou, K.-N., and Hao, J.: Change in household fuels dominates the decrease in PM2.5 exposure and premature mortality in China in 2005–2015, P. Natl. Acad. Sci. USA, 115, 12401–12406, https://doi.org/10.1073/pnas.1812955115, 2018.
Zheng, B., Tong, D., Li, M., Liu, F., Hong, C., Geng, G., Li, H., Li, X., Peng, L., Qi, J., Yan, L., Zhang, Y., Zhao, H., Zheng, Y., He, K., and Zhang, Q.: Trends in China's anthropogenic emissions since 2010 as the consequence of clean air actions, Atmos. Chem. Phys., 18, 14095–14111, https://doi.org/10.5194/acp-18-14095-2018, 2018.
Zhou, Y., Xing, X., Lang, J., Chen, D., Cheng, S., Wei, L., Wei, X., and Liu, C.: A comprehensive biomass burning emission inventory with high spatial and temporal resolution in China, Atmos. Chem. Phys., 17, 2839–2864, https://doi.org/10.5194/acp-17-2839-2017, 2017.
Zhu, J., Xia, X., Wang, J., Zhang, J., Wiedinmyer, C., Fisher, J. A., and Keller, C. A.: Impact of Southeast Asian smoke on aerosol properties in Southwest China: First comparison of model simulations with satellite and ground observations, J. Geophys. Res.-Atmos., 122, 3904–3919, https://doi.org/10.1002/2016JD025793, 2017.