the Creative Commons Attribution 4.0 License.
the Creative Commons Attribution 4.0 License.
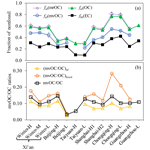
High contributions of fossil sources to more volatile organic aerosol
Haiyan Ni
Ru-Jin Huang
Junji Cao
Wenting Dai
Jiamao Zhou
Haoyue Deng
Anita Aerts-Bijma
Harro A. J. Meijer
Ulrike Dusek
Sources of particulate organic carbon (OC) with different volatility have rarely been investigated, despite the significant importance for better understanding of the atmospheric processes of organic aerosols. In this study we develop a radiocarbon-based (14C) approach for source apportionment of more volatile OC (mvOC) and apply to ambient aerosol samples collected in winter in six Chinese megacities. mvOC is isolated by desorbing organic carbon from the filter samples in helium (He) at 200 ∘C in a custom-made aerosol combustion system for 14C analysis. Evaluation of this new isolation method shows that the isolated mvOC amount agrees very well with the OC1 fraction (also desorbed at 200 ∘C in He) measured by a thermal–optical analyzer using the EUSAAR_2 protocol. The mvOC, OC and elemental carbon (EC) of 13 combined PM2.5 samples in six Chinese cities are analyzed for 14C to investigate their sources and formation mechanisms. The relative contribution of fossil sources to mvOC is 59±11 %, consistently larger than the contribution to OC (48±16 %) and smaller than that to EC (73±9 %), despite large differences in fossil contributions in different cities. The average difference in the fossil fractions between mvOC and OC is 13 % (range of 7 %–25 %), similar to that between mvOC and EC (13 %, with a range 4 %–25 %). Secondary OC (SOC) concentrations and sources are modeled based on the 14C-apportioned OC and EC and compared with concentrations and sources of mvOC. SOC concentrations (15.4±9.0 µg m−3) are consistently higher than those of mvOC (3.3±2.2 µg m−3), indicating that only a fraction of SOC is accounted for by the more volatile carbon fraction desorbed at 200 ∘C. The fossil fraction in SOC is 43 % (10 %–70 %), lower than that in mvOC (59 %, with a range of 45 %–78 %). Correlation between mvOC and SOC from nonfossil sources (mvOCnf vs. SOCnf) and from fossil sources (mvOCfossil vs. SOCfossil) is examined to further explore sources and formation processes of mvOC and SOC.
- Article
(2498 KB) -
Supplement
(695 KB) - BibTeX
- EndNote
Aerosol particles are of importance for atmospheric chemistry and physics and exert crucial influence on the climate system, air quality and human health (Fuzzi et al., 2015). The carbonaceous fraction of aerosol comprises a large fraction of the fine aerosol mass (20 %–80 %) (Cao et al., 2007; Tao et al., 2017). Carbonaceous aerosols are often operationally subdivided into organic carbon (OC) and elemental carbon (EC). OC consists of a large variety of organic species that cover a great range of volatilities and are not or only weakly light absorbing, while EC is nonvolatile, resistant to chemical transformation and strongly light absorbing (Pöschl, 2005). It should be noted that since no clear distinction between OC and EC exists, OC and EC are operationally defined based on measurement techniques (Petzold et al., 2013). When a thermal–optical method is used to separate and determine OC and EC, EC is described as a thermally refractory carbon continuum and OC is weakly refractory (Pöschl, 2003, 2005; Petzold et al., 2013).
EC is exclusively emitted as primary aerosols from incomplete combustion of biomass (e.g., wood, crop residues and grass) and fossil fuels (e.g., coal, gasoline and diesel). OC can be of both primary and secondary origin. Primary OC (POC) is emitted directly from nonfossil (e.g., biomass burning, biogenic emissions and cooking) and fossil sources. Secondary OC (SOC) is formed in the atmosphere via atmospheric oxidation of volatile organic compounds (VOCs) (Jacobson et al., 2000; Hallquist et al., 2009; Bond et al., 2013). High concentrations of aerosol particles have been observed in many cities, especially in China, where carbonaceous aerosols dominate particle mass concentrations, with SOC responsible for a large fraction of OC (Guo et al., 2014; Huang et al., 2014; Fang et al., 2017). Thus, understanding the sources, formation and transformation of EC, OC and SOC is important for better understanding of air pollution.
For aerosol source apportionment, radiocarbon (14C) analysis of OC and EC has been used to quantify their fossil and nonfossil fractions, based on the fact that emissions from fossil sources are 14C-free due to long-time decay, whereas nonfossil emissions contain the contemporary 14C content (e.g., Szidat et al., 2004, 2006; Dusek et al., 2013, 2017). Earlier 14C measurements of OC and EC found that fossil sources contribute more to EC than OC (Heal, 2014 and references therein). However, direct 14C measurements of SOC are not possible yet, due to the technical and conceptual difficulties in isolating the SOC fraction from filter samples. In positive-matrix-factorization-based (PMF) source apportionment of an aerosol mass spectrometer (Jayne et al., 2000) dataset, oxidized organic aerosols (OOA, also referred to as secondary organic aerosol, SOA) can be separated into semi-volatile OOA (SV-OOA) and low-volatility OOA (LV-OOA) based on their volatility (e.g., Huffman et al., 2009a; Wang et al., 2017). Volatility is an important physical property as it determines the partitioning between gas and particulate phases of organic species (Donahue et al., 2006, 2009). Direct measurement of OA volatility is challenging because OA is a mixture of thousands of individual organics spanning a wide range of volatilities (Donahue et al., 2011). Different approaches have been used to estimate the OA volatility. For example, using a thermodenuder (TD) coupled with an aerosol mass spectrometer (Burtscher et al., 2001; Wehner et al., 2002), the volatility of different OA components, such as hydrocarbon-like OA (HOA), biomass burning OA (BBOA) and OOA, can be estimated for ambient aerosols. Source and ambient studies indicate that BBOA and HOA are generally more volatile than OOA. Meanwhile, the volatility of BBOA can be quite variable, depending on type of biomass and the combustion conditions, and either higher or lower than that of HOA (e.g., Grieshop et al., 2009c; Huffman et al. 2009a; Paciga et al., 2016; Cao et al., 2018). For example, Huffman et al. (2009b) measured the volatility of primary OA from biomass burning and found that the more volatile BBOA was generally dominated by smoldering combustion, while the less volatile BBOA was more influenced by flaming combustion. Paciga et al. (2016) found that the BBOA was more volatile than HOA in an urban background site in Paris, with 50 % semi-volatile organic components (SVOCs with effective saturation concentrations C* of 1–100 µg m−3 range) and 30 % low-volatility organic compounds (LVOCs with C* of 10−3–0.1 µg m−3). Gaston et al. (2016) found that levoglucosan (i.e., the biomass burning tracer) was semi-volatile and had the potential to form low-volatility products during the chemical aging of ambient biomass burning aerosol, applying high-resolution time-of-flight chemical ionization mass spectrometer (HRTOF-CIMS) coupled to a Filter Inlet for Gases and AEROsols (FIGAERO) instrument for aerosol in the northwestern US.
In some recent studies, a thermal desorption approach has been used for analysis of filter samples at lower and higher temperatures as an indicator of volatility (Holzinger et al., 2013; Lopez-Hilfiker et al., 2014, 2015; Timkovsky et al., 2015; Masalaite et al., 2017, 2018). Even though the desorption temperature is not a direct measure of the particle volatility, OC desorbed from the filter at lower temperature tends to be more volatile, whereas the less volatile (i.e., more refractory) OC tends to be desorbed at higher temperatures. Vodička et al. (2015) and Masalaite et al. (2017) found that OC in urban samples was desorbed at lower temperatures compared to coastal, forest and rural background samples in Eastern Europe. Both studies suggest that OC is more volatile in urban areas close to the emission sources. Keller and Burtscher (2017) found that after aging of biomass burning emissions, less OC desorbed at lower temperatures. The reduction in volatility after aging agrees with previous TD aerosol mass spectrometer studies (Grieshop et al., 2009a, b). Even though the thermal desorption and TD measurements are different methods, results of both methods are usually in qualitative agreement and show similar trends. Earlier studies (e.g., Grieshop et al., 2009c; Chen et al., 2007) show similar trends qualitatively. Ma et al. (2016) made first efforts to link the OC fractions desorbed at different temperatures from filter samples on a thermal-optical analyzer to the volatility basis set, showing that OC fractions desorbed at lower temperatures (e.g., 140 and 280 ∘C) are semi-volatile.
Both POC and SOC can contribute to the OC fraction desorbed at lower temperatures. A number of previous studies have found that at least a part of POC from various sources is semi-volatile, including wood burning, gasoline and diesel vehicle exhausts and cooking (Lipsky and Robinson, 2006; Shrivastava et al., 2006; Robinson et al., 2007; Grieshop et al., 2009b, c; May et al., 2013a, b). Moreover, a significant part of freshly formed SOC is semi-volatile and will contribute to the more volatile OC fraction desorbed at lower temperatures (Holzinger et al., 2010; Salo et al., 2011; Meusinger et al., 2017; Gkatzelis et al., 2018). The relative contributions from fossil and nonfossil sources to OC can be dependent on the volatility. For example, recent studies during winter in Lithuania have shown that vehicular sources are associated with OC fractions desorbed at lower temperatures, while biomass burning (as a nonfossil source) contributes more strongly to less volatile OC fractions desorbed at higher temperatures (Masalaite et al., 2017, 2018).
Although an increasing number of studies has shown that OC from different emission sources and/or at different aging status may have different volatility, a quantitative study of the sources of OC with different volatility is scarce. In this study, wintertime fine particulate matter (PM2.5, particles with aerodynamic diameter < 2.5 µm) samples from six Chinese megacities are studied. Sources of carbonaceous aerosols, including different carbon fractions such as OC, EC and SOC, are estimated by 14C source apportionment. In addition, we operationally define a more volatile OC fraction (mvOC) as the carbon fraction desorbed from the filter samples at 200 ∘C in helium (He) and investigate sources of mvOC. The source contribution to mvOC is measured unambiguously by 14C.
2.1 Sampling
Simultaneous sampling was made during winter 2013/2014 in two northern (Beijing and Taiyuan) and three southern (Chongqing, Guangzhou and Shanghai) Chinese cities (Supplement Fig. S1). The samples from Xi'an in northern China were collected during winter 2015/2016. These sites were selected to represent urban-scale concentrations and located in the university or research center campus, > 100 m from local sources such as main roadways (Table S1 in the Supplement). The 24 h integrated PM2.5 samples were collected from 10:00 to 10:00 the next day (local standard time, LST). Filter samplers were deployed on rooftops about 6 to 20 m above ground level. In Xi'an, Beijing, Guangzhou and Shanghai, PM2.5 samples were collected on pre-baked (780 ∘C for 3 h) quartz fiber filter (QM-A, Whatman Inc., Clifton, NJ, USA, 20.3 cm ×25.4 cm) using a high-volume sampler (TE-6070 MFC, Tisch Inc., Cleveland, OH, USA) at a flow rate of 1.0 m3 min−1. In Taiyuan and Chongqing, samples were collected on pre-baked 47 mm Whatman quartz microfiber filters (QM-A) using mini-volume samplers at a flow rate of 5 L min−1 (Airmetrics, OR, USA). After sampling, all filters were packed in a pre-baked aluminum foil (450 ∘C for 3 h), sealed in polyethylene bags and stored in a freezer at −18 ∘C until analysis.
2.2 Determination of carbon fractions by thermal-optical analysis
For the PM2.5 samples collected in Xi'an, filter pieces of 1.5 cm2 were taken for OC and EC analysis using a carbon analyzer (Model 5L, Sunset Laboratory, Inc., Portland, OR, USA) following the thermal-optical transmittance protocol EUSAAR_2 (European Supersites for Atmospheric Aerosol Research; Cavalli et al., 2010). The EUSAAR_2 protocol defines OC1 as the carbon fraction that desorbs in helium (He) at 200 ∘C for 2 min. OC1 is compared with more volatile OC (mvOC) also extracted in He at 200 ∘C but for 5 min using our aerosol combustion system (ACS) (Dusek et al., 2014). No charred OC is observed by the transmittance signal in the OC1 stage during the thermal–optical analysis. For PM2.5 samples collected in Beijing, Taiyuan, Chongqing, Guangzhou and Shanghai, filter pieces of 0.5 cm2 were used to measure OC and EC using a Desert Research Institute (DRI) Model 2001 Thermal/Optical Carbon Analyzer (Atmoslytic Inc., Calabasas, CA, USA), following the IMPROVE_A thermal–optical reflectance (TOR) protocol (Chow et al., 2007). OC fractions of EUSAAR_2 protocol are desorbed in He at 200 ∘C (OC1), 300 ∘C (OC2), 450 ∘C (OC3) and 650 ∘C (OC4). Different from EUSAAR_2 protocol, IMPROVE_A (Interagency Monitoring of Protected Visual Environments) protocol defines OC fractions at stepwise temperature of 140, 280, 480 and 580 ∘C in He. Details of the carbon fraction measurements were described in our previous work (Cao et al., 2013; Zenker et al., 2017).
2.3 Radiocarbon (14C) measurements of mvOC, OC and EC
2.3.1 Sample selection for 14C analysis
For 14C analysis, we selected the samples carefully to cover periods of low and high total carbon (TC, the sum of OC and EC) and PM2.5 concentrations to get samples representative of various pollution conditions that did occur in each city. Two or three composite samples from each city representing high (H), medium (M) and low (L) TC concentrations were selected for 14C analysis of EC, OC and mvOC (Fig. S2). Each composite sample consists of two to four 24 h filter pieces with similar TC loadings and air mass backward trajectories (Fig. S2, Table S1).
2.3.2 Extraction of mvOC, OC and EC
Three separate extractions were performed for mvOC, OC and EC on our aerosol combustion system (ACS) (Dusek et al., 2014). The ACS consists of a combustion tube and a purification line. Aerosol filter pieces placed on the filter boat are heated at different temperatures in pure He or O2 and oxidized through the platinum catalyst in the combustion tube. The resulting CO2 is isolated and separated from other gases (e.g., NOx, water vapor) in the purification line. The purification line is equipped with an oven filled with copper grains and silver wire heated at 650 ∘C to remove NOx and liberated halogen, a U-type tube cooled with a dry ice–ethanol mixture for water removal, and a flask containing phosphorous pentoxide (P2O5) for removal of any trace water. The amount of purified CO2 is manometrically quantified and subsequently stored in flame-sealed glass ampoules.
mvOC is desorbed by heating the filter pieces at 200 ∘C in He for 5 min. After introducing the filter, the ovens are flushed with He for 10 min. Pilot tests show that the flushing time (10, 15 or 60 min) before heating the filter pieces does not affect the desorbed amount of mvOC (Table S2). OC is combusted by heating filter pieces at 375 ∘C in pure O2 for 10 min. EC is extracted from a separate filter piece after removing OC completely. First water-soluble OC is removed from the filter through water extraction (Dusek et al., 2014) to minimize the charring of organic materials (Yu et al., 2002; Zhang et al., 2012). Then, by heating the filter pieces at 375 ∘C in O2 for 10 min, most water-insoluble OC can be removed. Subsequently, the oven temperature is increased to 450 ∘C for 3 min to remove most refractory OC that is left on the filter. However, during this step some less refractory EC might be lost. Finally, the remaining EC is combusted by heating at 650 ∘C in O2 for 5 min (Dusek et al., 2014, 2017; Zenker et al., 2017).
Contamination introduced by the isolation procedure is determined by following exactly the same isolating procedures with either an empty filter boat or with preheated filters (at 650 ∘C in O2 for 10 min). The contamination introduced by the combustion process yields 0.72±0.44 µgC EC, 0.85±0.49 µgC OC and 0.52±0.31 µgC mvOC per extraction. Compared with our sample size of 30–391 µgC EC, 30–445 µgC OC and 15–121 µgC mvOC, the blanks are ≤7 % of the mvOC sample amount and < 3 % of OC and EC sample amount and thus relatively small compared to our sample sizes. The mvOC concentrations reported in this study are corrected for contamination by subtraction. OC and EC concentrations are measured following thermal-optical protocols using carbon analyzers (Sect. 2.2), thus are not affected by the isolation procedure using the ACS. For 14C values (Eq. 1), the contamination is assessed but not used for further 14C data correction for mvOC, OC and EC because the corrections for the small blanks will not introduce large uncertainties to the data, as explained in Supplement Sect. S3.
Two standards with known 14C∕12C ratios are combusted using the ACS as quality control for the combustion process: an oxalic acid standard and anthracite. Small amounts of solid standard materials are directly put on the filter boat of the combustion tube and heated in O2 at 650 ∘C for 10 min. In the further 14C analysis, the CO2 derived from combustion of the standards is treated exactly like the samples. Therefore, the contamination introduced by the combustion process can be estimated from the deviation of measured values from the nominal values of the standards (Table S3) (Dusek et al., 2014). The contamination inferred in this indirect way is below 1.5 µgC per extraction, which is slightly higher than the directly measured contamination of OC and EC separately but in the range of a TC contamination. It is also relatively small compared to the size of OC (30–445 µgC) and EC samples (30–391 µgC) in this study and thus can be neglected (Sect. S3). The F14C (Eq. 1) of the contamination can also be indirectly inferred and varied between 0.2 and 0.6 for standards measured on the ACS over the last 2 years. This is broadly within the range of our samples and implies that a correction for F14C will even be considerably smaller than 3 %.
2.3.3 14C measurements by accelerator mass spectrometer (AMS)
AMS measurements were conducted at the Centre for Isotope Research (CIO) at the University of Groningen. The extracted CO2 is released from the glass ampules and directed to a gas inlet system (Ruff et al., 2007), where the sample is diluted with He to 5 % CO2 (Salazar et al., 2015). The CO2∕He mixture is directly fed into the Cs sputter ion sources of the Mini Carbon Dating System (MICADAS) AMS at a constant rate (Synal et al., 2007).
The 14C∕12C ratio of an aerosol sample is usually reported relative to an oxalic acid standard (OXII) and expressed as fraction modern (F14C). The 14C∕12C ratio of the standard is related to the unperturbed atmosphere in the reference year of 1950 by multiplying it by a factor of 0.7459 (Mook and Van Der Plicht, 1999; Reimer et al., 2004):
where the 14C∕12C ratio of the sample and standard are both corrected for machine background and normalized to δ13C ‰. Aerosol carbon from fossil sources has F14C = 0 due to the extinction of 14C after long-time decay. Aerosol carbon from contemporary (or nonfossil) sources should have F14C ∼1 in an atmosphere undisturbed by human influences. However, the F14C values of contemporary carbon are higher than 1 due to the nuclear bomb tests that nearly doubled the 14CO2 in the atmosphere in the 1960s. The 14CO2 produced by the nuclear bomb tests has been taken up by oceans and the biosphere and diluted by the 14C-free CO2 emitted by the fossil fuel burning. Currently, F14C of the atmosphere CO2 is ∼1.04 (Levin et al., 2010).
The F14C values are corrected for cross contamination (also known as memory effect) (Wacker et al., 2010) using alternate measurements of OXII and 14C-free material as gaseous standards. Correction for instrument background (Salazar et al., 2015) is done by subtracting the memory-corrected F14C values of the 14C-free standard. Finally, the values are normalized to the average value of the (memory- and background-corrected) OXII standards. All standards used for the corrections are measured on the same day as the samples.
2.4 Estimation of source contributions to different carbon fractions
F14C of EC, OC and mvOC (i.e., F14C(EC), F14C(OC) and F14C(mvOC), respectively) were directly measured. We define “more refractory organic carbon” (mrOC) as the difference between OC and mvOC. F14C of mrOC (F14C(mrOC)) was calculated by isotope mass balance:
F14C(EC) was converted to the fraction of biomass burning (fbb(EC)) by dividing with an F14C value representative of typical biomass burning emissions (F14Cbb). Analogously, the fraction of nonfossil OC, mvOC and mrOC (i.e., fnf(OC), fnf(mvOC) andfnf(mrOC), respectively) were estimated from their corresponding F14C values and F14C of nonfossil sources (F14Cnf). F14Cbb and F14Cnf are estimated as 1.10±0.05 and 1.09±0.05 (Lewis et al., 2004; Mohn et al., 2008; Palstra and Meijer, 2014), respectively, based on tree-growth models and the assumption that wood burning dominates biomass burning. F14Cbb for EC is slightly bigger than F14Cnf for OC because, besides biomass burning, biogenic emissions also contribute to OC but have a smaller F14C than that of biomass burning. The estimation of F14Cbb and F14Cnf has been reported in our previous study (Ni et al., 2018).
Then, fbb(EC) was used to determine EC mass concentrations from nonfossil biomass (ECbb) and fossil fuel combustion (ECfossil):
Analogously, mass concentrations of OC, mvOC and mrOC from nonfossil sources (OCnf, mvOCnf and mrOCnf, respectively) and fossil sources (OCfossil, mvOCfossil and mrOCfossil, respectively) were determined.
Secondary OC (SOC) includes SOC from fossil (SOCfossil) and nonfossil sources (SOCnf):
Fraction fossil in total SOC (ffossil(SOC)) was formulated as follows:
For the following calculations we assume that SOCnf can be approximated by OC from nonfossil sources excluding primary biomass burning (OCo,nf; OC other nonfossil). In principle, OCo,nf includes SOCnf and nonfossil primary OC from vegetative detritus, bioaerosols, resuspended soil organic matter, or cooking. But the contributions from plant detritus, bioaerosols and spores to PM2.5 are likely small (Song et al., 2006; Hu et al., 2010; Guo et al., 2012). If cooking sources are significant then this assumption results in an upper limit of SOCnf.
where OCo,nf was calculated by the difference between OCnf and primary OC from biomass burning (POCbb). POCbb was calculated by multiplying ECbb with the primary OC∕EC ratio of biomass burning (rbb):
SOCfossil was calculated by subtracting primary fossil OC (POCfossil) from OCfossil. POCfossil was estimated by multiplying ECfossil with primary OC∕EC ratio of fossil fuel combustion (rfossil):
Fossil sources in China are almost exclusively from coal combustion and vehicle emissions, thus rfossil was estimated as follows:
where rcoal and rvehicle are the primary OC∕EC ratio of coal combustion and vehicle emissions, respectively. The rbb, rcoal and rvehicle varies with the fuel types and properties, combustion conditions, sampling and analysis methods, etc. Best estimate of rbb(4±1; average ± standard deviation), rcoal(2.38±0.44) and rvehicle(0.85±0.16) is done through a literature search and described in our earlier study (Ni et al., 2018). p is the fraction of EC from coal combustion (ECcoal) in ECfossil.
We used two different methods to estimate p. (1) Since both coal combustion and vehicle emissions do not contain 14C, they can not be distinguished by 14C measurements alone. Therefore, p is randomly chosen from 0 to 1, that is no constraint on p values. (2) EC from coal combustion is on average more enriched in the stable carbon isotope 13C compared to vehicle emissions. Therefore, complementing 14C results of EC with measurements of the 13C∕12C ratios of EC (expressed as δ13CEC in Eq. S1; Sect. S1) allows separation of ECfossil into ECcoal and EC from vehicle emissions (ECvehicle). Samples taken from Beijing, Shanghai and Guangzhou using high-volume samplers had enough material for analysis of both F14CEC and δ13CEC, thus ECcoal and ECvehicle are separated as described in detail in Sect. S2. In brief, the fraction of coal combustion and vehicle emissions in EC was calculated from measured F14CEC and δ13CEC for ambient EC combined with the source signatures. Bayesian Markov chain Monte Carlo (MCMC) calculations were used to account for the uncertainties in the source signatures and the measurement uncertainties (Andersson, 2011; Andersson et al., 2015). The results of the Bayesian calculations are the posterior probability density functions (PDFs) for the relative contributions of each source to EC (fcoal and fvehicle; Fig. S3). The p was formulated as follows:
The PDF of p was derived from the PDF of fcoal and fvehicle and shown in Fig. S4.
To propagate uncertainties, a Monte Carlo simulation with 10 000 individual calculations was conducted. For each individual calculation, F14C(EC), F14C(OC), F14C(mvOC), and concentrations of EC, OC and mvOC were randomly chosen from a normal distribution that is symmetric around the measured values with the experimental uncertainties as standard deviation (SD; Table S4). For F14Cbb, F14Cnf, rbb, rcoal and rvehicle, random values were chosen from a triangular frequency distribution, which has its maximum at the central value and at 0 at the lower limit and upper limit. For p ranging from 0 to 1 (no 13C constraints), p was randomly chosen from a uniform distribution. For p constrained by F14C and δ13C using MCMC (hereafter, 13C-constrained p), random values from the respective PDF of p were used (Fig. S4). In this way 10 000 different estimation of fbb(EC),fnf(OC), fnf(mvOC), fnf(mrOC), ECbb, ECfossil, OCnf, OCfossil, mvOCnf, mvOCfossil, mrOCnf, mrOCfossil, SOCnf, SOCfossil, SOC and ffossil(SOC) were calculated (Tables S5, S6, S7, S8). The derived average represents the best estimate and the SD represents the combined uncertainties.
3.1 Method evaluation and quality control for mvOC extraction
The separation of OC and EC for 14C analysis using our aerosol combustion system (ACS) were thoroughly evaluated by Dusek et al. (2014, 2017). It is thus necessary to validate the new extraction method for isolating mvOC. The reproducibility of the extracted mvOC amount was tested for two independent test filters with mvOC loadings of 6 and 18 µg cm−2, respectively (Fig. S5). The coefficient of variation was determined as a measure of reproducibility. The reproducibility was found to be ∼5 % (n=9).
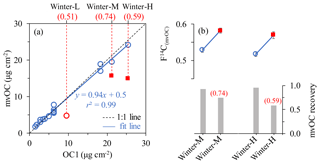
Figure 1(a) OC1 loading (µg cm−2) measured with a thermal–optical method (EUSAAR_2 protocol using a Sunset analyzer) and mvOC loading measured using our custom-made aerosol combustion system. The outliers are shown in red circle and square with their sample name on the top x axis. (b) F14C(mvOC) of winter-M and winter-H with high and low recoveries with respect to OC1. The red numbers in brackets in panels (a) and (b) give the respective outliers' mvOC recoveries to OC1.
Since carbon fractions (e.g., OC1) at different desorption temperatures have mostly been measured using the EUSAAR_2 protocol in many previous studies (e.g., Vodička et al., 2015; Keller and Burtscher, 2017), our goal is to define the mvOC fraction as representative of OC1. Therefore, the mvOC is desorbed at 200 ∘C, the same temperature as used for OC1 in the EUSAAR_2 protocol. However, the extracted amounts of the ACS system might differ due to different heating rates and length of the temperature step. The winter samples from Xi'an as well as the two test filters described above are used to compare mvOC concentrations from the ACS system to OC1. For most samples, excellent agreement was found between mvOC and OC1 (Fig. 1a) and most data points fall close to the 1:1 line. However, there are three data points deviating largely from the 1:1 line (red circle and square in Fig. 1). The two red squares represent the mvOC extraction for sample winter-H and winter-M using larger filter pieces (i.e., more mvOC in µgC per extraction). With larger filter pieces, the mvOC (µgC cm−2 filter area) extracted by the ACS system is significantly lower than that measured by the Sunset analyzer. The recoveries of these two outliers are 0.59 for sample winter-H and 0.74 for winter-M, calculated by dividing mvOC mass by OC1 mass. For winter-H, the low recovery is also repeatable with the same filter area (Fig. S5). The low recoveries for large filter pieces may result from the lower temperature (< 200 ∘C) towards the ends of the filter boat. At the relatively low temperature of 200 ∘C, only a 3.5 cm length at the center of a 12 cm combustion tube is maintained at 200 ∘C and outside of that 3.5 cm the temperature is lower than 200 ∘C, e.g., ∼170 ∘C at the end of the combustion tube as measured by a thermocouple. When filter pieces are large and placed outside the centered 3.5 cm, the desorption temperature for part of the filter pieces will lower than 200 ∘C, leading to lower desorbed mvOC amount. Another possibility is saturations of the catalyst (platinum, Pt) in the combustion line of ACS system. Pt works as catalyst by collecting oxygen atoms on the surface as has been demonstrated by direct observation of an ultra-thin oxygen layer on the Pt surface by a microscope (Spronsen et al., 2017). This is used to oxidize CO and hydrocarbons to CO2 in a reducing atmosphere. Thus, for large sample amounts it is possible that the oxygen on the catalyst could not be sufficient to oxidize all desorbed CO and hydrocarbons to CO2. However, we observed recoveries near 100 % for mvOC amounts up to 120 µg, which was higher than the total amount of desorbed mvOC for the winter-H sample. Therefore, limited catalyst capacity is not the likely explanation for the low recoveries. For subsequent experiments we consequently placed the filter pieces carefully in the 3.5 cm, 200 ∘C section of the combustion tube and avoided stacking multiple filter pieces to ensure a desorption temperature of 200 ∘C and sufficient He supply.
To examine the effect of the low recoveries of mvOC on the F14C(mvOC), we compare the F14C(mvOC) of winter-M and winter-H samples for both high and low mvOC recoveries. F14C(mvOC) for low recoveries is roughly 0.05 (absolute value, Fig. 1b) higher than for high recoveries, which is non-negligible compared to the measurement uncertainty. In addition, to validate the measured F14C(mvOC) for the combined sample winter-M, we also extracted mvOC separately from those three filters that were combined for the composite winter-M sample. Figure 2 shows the F14C(mvOC) of combined winter-M and those of the three individual filter samples. From the F14C(mvOC) and mvOC mass of the individual filters, we can estimate the expected F14C(mvOC) of the combined winter-M sample using the isotope mass balance equation. The F14C(mvOC) of winter-M calculated from individual filter pieces is 0.524, which is quite similar to the measured F14C(mvOC) of 0.529±0.007 for the combined winter-M with recovery close to 1.
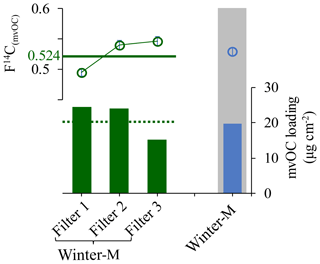
Figure 2F14C(mvOC) (shown in circle) and mvOC loading (vertical bar) of the three daily filters (“filter1”, “filter2” and “filter3” shown in dark green) that comprise winter-M and the composite winter-M (shown in blue in the gray area) with mvOC recovery of ∼1. The dashed dark green line indicates the expected mvOC loading and the horizontal solid green line indicates the F14C(mvOC) for the combined winter-M (weighted mean ± standard deviation; 0.524±0.028). The latter is calculated from the mvOC loading (µg cm−2) and F14C(mvOC) of the three daily filters using the isotope mass balance equation. F14C(mvOC) uncertainties are indicated but are too small to be visible.
We conclude that F14C(mvOC) of samples with mvOC recoveries of ∼1 are reliable and used in the following discussion. The mvOC recovery of sample winter-L (red circle in Fig. 1) is also low (0.51), and we could not repeat it due to the limited filter material. For sample winter-L, we take the OC1 concentrations as mvOC and the measured F14C(mvOC) values but assign a bigger absolute uncertainty of 0.05, due to its low mvOC recoveries. This is based on the difference in F14C(mvOC) for winter-H and winter-M with low and high mvOC recoveries, which is roughly 0.05 (Fig. 1b).
3.2 mvOC, OC and EC concentrations
Figure 3 shows the concentrations of mvOC, OC and EC and the mvOC contributions to OC (%) for the selected samples in the six studied Chinese megacities. mvOC and OC concentrations averaged 3.3±2.2 µg m−3 (range of 0.7–7.4 µg m−3) and 30.0±13.8 µg m−3 (8.8–50.4 µg m−3), respectively. EC concentrations ranged from 2.5 to 14.8 µg m−3, with an average of 6.9±3.6 µg m−3. High TC concentrations were found in Taiyuan (60 µg m−3 for sample Taiyuan-H), Chongqing (59 µg m−3 for Chongqing-H), Beijing (57 µg m−3 for Beijing-H) and Xi'an (57 µg m−3 for winter-H) in descending order. Of these cities, Chongqing is located in southern China, where there is no official heating season using coal in winter. This study nevertheless indicates severe pollution of carbonaceous aerosols in Chongqing. TC concentrations in the other southern Chinese cities (Shanghai and Guangzhou) were much lower than those in Chongqing (Fig. 3).
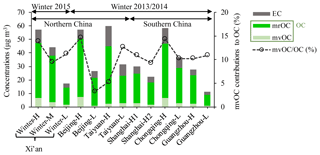
Figure 3Concentrations of mvOC, mrOC and EC (µg m−3) and contribution of mvOC to total OC (%) in three cities in northern China (Xi'an, Beijing and Taiyuan) and three in southern China (Shanghai, Chongqing, Guangzhou). mrOC concentrations are calculated by the difference between OC and mvOC. For details, see Table S4.
The fraction of mvOC in total OC (mvOC∕OC in Fig. 3) gives an indication of OC volatility. The mvOC contributed on average 10.5±3.3 % to OC, ranging from 3 % to 15 %. The mvOC∕OC varies between samples within the same city and between cities, indicating complicated sources and atmospheric processing of OC. The variations might also be partially attributed to the different protocols used for OC quantification. OC in Xi'an is measured with the EUSAAR_2 protocol (up to 650 ∘C for desorbing OC), whereas the IMPROVE_A protocol (up to 580 ∘C for OC) was used for the other five cities. However, Han et al. (2016) found that the absolute OC concentrations determined by EUSAAR_2 do not differ much from those determined by IMPROVE_A (22.6±12.0 µg m−3 vs. 19.7±10.7 µg m−3) for 1-year PM2.5 samples in Xi'an during 2012/2013. Because of the small differences of OC between the two protocols, we think the comparison of OC concentrations and mvOC∕OC amongst the six cities is justified. The rest of OC (∼90 %) was contributed to the mrOC. mrOC concentrations averaged 26.8±12.0 µg m−3, ranging from 7.9 µg m−3 for sample Guangzhou-L to 43.1 µg m−3 for Beijing-H.
Direct comparison of our results with previous works is somewhat difficult because different thermal–optical protocols were used. The averaged mvOC concentration (3.3±2.2 µg m−3) in winter for the six studied sites in China is higher than winter concentrations at an urban background site (1.6±1.7 µg m−3), a rural background site (0.7±0.6) in Prague (Vodička et al., 2015), where 200 ∘C in He was also applied to desorb this OC fraction using EUSAAR_2 protocol. The mvOC∕OC ratio in our study is smaller than that of ambient samples from various other locations and much smaller than that of fresh source samples. For example, the urban background site in Prague had a mvOC∕OC ratio of 28 % and the rural background site of 17 % (Vodička et al., 2015). The contribution of OC1 to total OC was as high as ∼60 % for primary biomass burning measured by EUSAAR_2 thermal–optical protocol (Keller and Burtscher, 2017). For vehicle emissions, the OC fractions desorbed at 140 and 280 ∘C are the major OC fractions measured by the IMPROVE_A protocol, contributing ∼30 % and ∼20 % to total OC in tunnels in Taiwan, respectively (Zhu et al., 2014, 2010). Using the same protocol for OC analysis, Tian et al. (2017) found that OC fraction desorbed at 140 and 280 ∘C contributed ∼13 % and ∼20 %, respectively, to primary OC from residential coal combustion in China. The mvOC desorbed at 200 ∘C falls between 140 and 280 ∘C, thus the mvOC fraction in total OC should be higher than the faction of OC desorbed at 140 ∘C in total OC and lower than the fraction of OC desorbed up to 280 ∘C in total OC.
OC from different emission sources has different volatility and the atmospheric processing can also alter its volatility. Keller and Burtscher (2017) found that aging reduces the volatility of OC from biomass burning; i.e., the contribution of OC1 fraction to total OC decreases from 60 % to 25 % after aging. The photochemical processing of OA can lead to accumulation of carbon in the more refractory organic fraction and also larger organic compounds (Masalaite et al., 2017). The mvOC fraction in OC of our ambient samples is much smaller than that of the primary sources, suggesting that atmospheric aging of OC plays an important role on modifying the volatility of OC.
3.3 Nonfossil and fossil fractions of different carbon fractions
Figure 4a shows the fraction of nonfossil carbon in mvOC, OC and EC (fnf(mvOC), fnf(OC) and fbb(EC), respectively). There are no considerable changes in fnf(mvOC), fnf(OC) and fbb(EC) between polluted days (“H” samples) and clean days (“L” samples) within each site (Fig. 4a), despite the very different concentrations of carbonaceous aerosols (Fig. 3). However, fnf(mvOC), fnf(OC) and fbb(EC) varied significantly among different sites: the lowest values are always found in Taiyuan and the highest are found in Chongqing. This implies that different pollution patterns exist in individual Chinese cities. The smallest fnf(mvOC), fnf(OC) and fbb(EC) being in Taiyuan suggests that fossil sources are the main contributor to mvOC, OC and EC, whereas the largest values being in Chongqing shows that the nonfossil contributions to mvOC, OC and EC are evidently higher in Chongqing than in other sites.
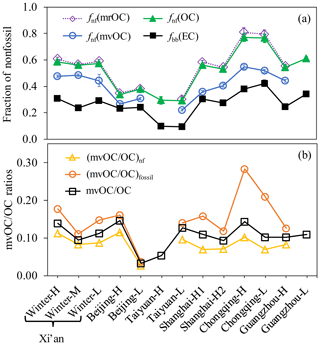
Figure 4(a) Fraction of nonfossil (fnf) in mvOC, OC and EC. fnf(mrOC) is calculated by the differences between OC and mvOC using isotope mass balance equation. (b) mvOC∕OC ratios for nonfossil, fossil and total OC.
Ranging from 22 % to 55 %, fnf(mvOC) (41±11 %) is consistently smaller than fnf(OC) (52±16 %, with a range of 29 %–77 %) and larger than fbb(EC) (27±9 %, with a range of 10 %–42 %), despite their variations among the cities. The absolute difference in the nonfossil fractions between mvOC and OC is 13 % (7 %–25 %), similar to that between mvOC and EC (13 %, with a range of 4 %–25 %). Consistently smaller fnf(mvOC) than fnf(OC) suggests that mvOC is more fossil (less nonfossil) than the total OC. Liu et al. (2017) also found that F14C of OC desorbed at lower temperature (up to 200 ∘C) in He was 0.389, smaller than F14C of total OC desorbed in the He phase of EUSAAR_2 protocol (F14C =0.495, up to 650 ∘C) for a single test sample collected in winter in Xinjiang, China. Agrios et al. (2016) had the qualitatively similar finding that F14C of OC extracted in O2 at 200 ∘C was smaller than F14C of OC extracted in O2 at higher temperatures for samples collected from an urban and rural site in Switzerland and from the Los Angeles Basin, USA. We can unambiguously conclude from 14C measurements of mvOC and OC that mvOC is more fossil than OC in six Chinese cites. The fraction of nonfossil carbon in mrOC (fnf(mrOC)) is calculated by the differences between OC and mvOC using the isotope mass balance. Since mvOC is only a small fraction of OC, fnf(mrOC) is very similar to fnf(OC).
Primary OC from vehicular emissions is generally more volatile (i.e., less refractory) than OC from biomass burning, which is in line with the ambient results presented. For example, Grieshop et al. (2009c) constrained the volatility distribution of primary OA from a diesel engine and wood burning using measurements of TD coupled to aerosol mass spectrometer and found that OA from wood burning was less volatile than from diesel exhaust. Chen et al. (2007) measured OC∕EC from fresh biomass burning emissions and found that high-temperature OC fractions (desorbed from 450 to 550 ∘C) are the major fraction of OC, in contrast to gasoline and diesel exhausts, where OC fractions desorbed at temperature lower than 250 ∘C are more abundant (Watson et al., 1994; Chow et al., 2004). Zhu et al. (2010, 2014) also found that OC desorbed at 140 ∘C is the major fraction of OC in fresh vehicle emissions in tunnel experiments. A more recent study of Masalaite et al. (2017) found that OC desorbed at 200 ∘C in He has higher contributions from vehicular emissions than OC desorbed at higher temperatures (250–350 ∘C) at three sites (urban, coastal and forest) in Lithuania, based on δ13C of OC desorbed at a different temperature step. However, the conclusions of this study remained qualitative because δ13C of organic carbon can be changed significantly by isotopic fractionation during atmospheric processing, whereas the 14C source apportionment used in our study is independent of atmospheric processing.
By comparing (mvOC∕OC)fossil (i.e., the fraction of mvOCfossil in OCfossil) and (mvOC∕OC)nf, we can get a measure of the volatility of OC from fossil and nonfossil sources, respectively:
Asfnf(mvOC) is smaller than fnf(OC), this is equivalent to (mvOC∕OC)fossil >(mvOC∕OC)nf (Eq. 13). That is, OC from fossil sources contains a larger, more volatile fraction than OC from nonfossil sources. This is true for all studied cities, despite the variation in fnf(mvOC) and fnf(OC) in different cities. (mvOC∕OC)fossil(14±6.6 %, with a range of 3.7 %–28 %) is consistently higher and more variable than (mvOC∕OC)nf (7.5±2.9 %, with a range of 2.6 %–11.6 %; Fig. 4b). (mvOC∕OC)fossil and (mvOC∕OC)nf in general tracks the variation in mvOC∕OC, except that (mvOC∕OC)fossil for Chongqing (28 % for Chongqing-H and 21 % for Chongqing-L) is much higher than the other sites (averaged 12 %). When comparing the mvOC∕OC within each city, it is found that (mvOC∕OC)fossil changes more strongly between H and L samples than (mvOC∕OC)nf, indicated by the bigger absolute differences of (mvOC∕OC)fossil between H and L samples than that of (mvOC∕OC)nf. Correlations between fnf(mvOC) and fnf(OC) and between fnf(mvOC) and fbb(EC) are examined. fnf(mvOC) correlates more closely with fnf(OC) (r2=0.89) than with fbb(EC) (r2=0.62) (Fig. 5).
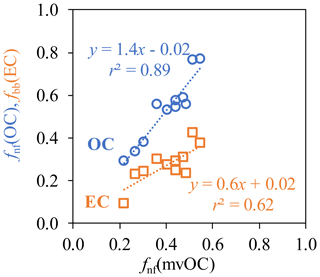
Figure 5Correlation between fnf(mvOC), fnf(OC), fnf(mvOC) and fbb(EC) for Xi'an, Beijing, Taiyuan, Shanghai, Chongqing and Guangzhou during winter.
It is likely that some of mvOC are of secondary origin, since SOC is formed in the atmosphere and subsequently condenses onto the aerosol particles (Hallquist et al., 2009; Jimenez et al., 2009). It has been shown that SOC formed in chambers initially desorbed at relatively low temperatures (e.g., 200 ∘C) (Holzinger et al., 2010; Salo et al., 2011; Meusinger et al., 2017; Gkatzelis et al., 2018). However, there is also evidence that the formation of highly oxidized low-volatility compounds can occur in SOA formation (Ehn et al., 2014). We thus compared concentrations and sources of SOC to those of mvOC. SOC concentrations and ffossil(SOC) are estimated based on the 14C-apportioned OC and EC in combination with p values of 0–1 (Sect. 2.4). Where δ13C measurements were available for high-volume filter samples (Beijing, Shanghai and Guangzhou), we compare the SOC concentrations and ffossil(SOC) derived from p values of 0–1 with p values constrained by δ13C. In these three cities, SOC concentrations and ffossil(SOC) without constraints on p value (0–1) is very similar to that with constraints on p value using δ13C, only with relatively larger uncertainties (Fig. 6). This indicates that the unconstrained p values do not lead to significant bias in SOC concentrations and ffossil(SOC) and the main gain in using δ13C is currently a decrease in uncertainty.
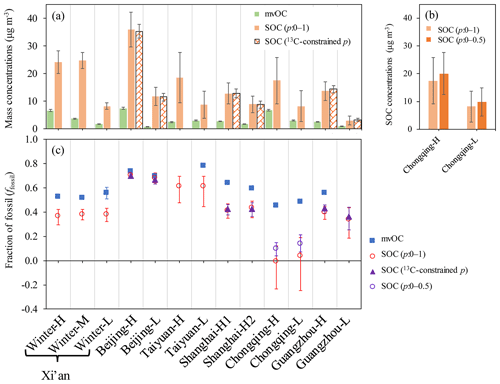
Figure 6(a) Observed mvOC concentrations (shown as a green bar) and estimated SOC concentrations by 14C-apportioned OC and EC in combination with p values without (p=0–1) and with constraints by δ13C (13C-constrained p), shown as solid orange bars and patterned orange bars, respectively. Error bars indicate the uncertainties of mvOC and SOC concentrations calculated by Monte Carlo error propagation. (b) For Chongqing, lower p values of 0–0.5 are used to estimate SOC concentrations and are compared with p values of 0–1. (c) Fraction of fossil (ffossil) in mvOC (blue square) and SOC. ffossil of SOC is estimated using p values without constraints (p=0–1) are shown as red circles and with constraints by δ13C (13C-constrained p) as purple triangles, respectively. For Chongqing, lower p values of 0–0.5 are used (purple circles). Interquartile ranges (25th–75th percentile) of the median ffossil(SOC) are shown as vertical bars, uncertainties of ffossil(mvOC) are indicated but are too small to be visible.
When choosing p randomly from 0 to 1, the calculated median ffossil(SOC) is smaller than 0 for samples in Chongqing, reflecting negative SOCfossil values. Since SOCfossil is calculated by subtracting POCfossil from OCfossil, this indicates POCfossil is overestimated in Chongqing. Because the primary OC∕EC ratio is higher for coal (rcoal) than vehicle (rvehicle) emissions, an overestimate of POCfossil is the direct result of overestimated p values, i.e. a too high fraction of coal burning in ECfossil (Eq. 11). Chongqing is in southern China, where there is no official heating using coal in winter and smaller contribution of coal combustion is expected than in northern China. Further, earlier studies found that vehicle emissions contributed more to EC than coal combustion in Chongqing (Chen et al., 2017). We thus tried to lower p values to 0–0.5. Lower p values lead to higher ffossil(SOC) (Fig. 6c) and, to a lesser extent, higher SOC concentrations (Fig. 6b). For Chongqing, we took p from 0 to 0.5 as a conservative estimate. For other cities except Chongqing, we kept p values of 0–1 due to the very similar ffossil(SOC) and SOC concentrations derived from p values with and without constraints by δ13C, especially for the two southern cities in China, e.g., Shanghai and Guangzhou.
ffossil(mvOC) ranged from 45 % to 78 %, with an average of 59 %, whereas ffossil(SOC) ranged from 10 % to 70 % and averaged 43 %. ffossil(mvOC) is always larger than ffossil(SOC) (Fig. 6c), showing that mvOC is more fossil than SOC. However, both SOC and mvOC have a larger contribution of fossil sources than the total OC. The smallest differences between ffossil(SOC) and ffossil(mvOC) are found in Beijing for both samples Beijing-H and Beijing-L, with an absolute difference of 3 % and 2 %, respectively, within the uncertainty of the measurements. Much higher differences are found in other cities, with the absolute differences ranging from 13 % to 35 %, well outside the measurement uncertainties.
Median values for SOC, SOCfossil and SOCnf are very close to their mean values (Fig. S6, Tables S7, S8). To compare with mvOC, here we use mean (±SD) as well for SOC, SOCfossil and SOCnf. The lowest SOC concentrations are found to be 2.95 µg m−3 for Guangzhou-L and the highest are found to be 35.9 µg m−3 for Beijing-H. SOC concentrations (15.4±9.0 µg m−3) are consistently higher than those of mvOC (3.3±2.2 µg m−3, Fig. 6a), showing that the contribution of SOC to total OC is considerably larger than that of mvOC. The major fraction of SOC has desorption temperatures above 200 ∘C and falls into our operationally defined mrOC. SOCfossil ranged from 0.90 to 25.3 µg m−3, with an average of 6.8±6.4 µg m−3, which is consistently higher than mvOCfossil ranging from 0.5 to 5.4 µg m−3 with an average of 2.1±1.4 µg m−3 (Table S6). mvOCnf is smaller than SOCnf as well (Fig. S7), averaged mvOCnf concentrations (1.5±1.1 µg m−3) are only one-fifth of that SOCnf(8.5±5 µg m−3).
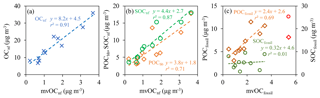
Figure 7Correlation between (a) OCnf and mvOCnf (blue cross), (b) POCbb and mvOCnf (orange diamond), SOCnf and mvOCnf (green circle), (c) POCfossil and mvOCfossil(diamond in dark orange), and SOCfossil and mvOCfossil(circle in dark green) for Xi'an, Beijing, Taiyuan, Shanghai, Chongqing and Guangzhou during winter. In panel (c), the data points marked in red are from sample Beijing-H with the considerably higher SOCfossil ∕ POCfossil ratio (3.1) than the other samples (an average of 0.8) and are not included in the regression.
We examined the correlation between mvOC and SOC from nonfossil sources (mvOCnf vs. SOCnf) and from fossil sources (mvOCfossil vs. SOCfossil). Comparing absolute concentrations between different cities and pollution levels is expected to result in reasonably positive correlations for all species because it can be expected that all carbon fractions are higher in polluted cities than in clean cities. However, it is still interesting to compare the correlation coefficients relative to one another. mvOCnf includes primary and secondary mvOC from nonfossil sources. Good correlation was found between mvOCnf and SOCnf(r2=0.87, bootstrapped 95 % confidence interval of [0.21, 0.94]; Fig. 7), which is not significantly different from the correlation between mvOCnf and POCbb (r2=0.71, bootstrapped 95 % confidence interval of [0.60, 0.99]). SOCnf is estimated by subtracting POCbb from OCnf, where POCbb is estimated by multiplying ECbb with the OC∕EC ratio of fresh biomass burning plumes (rbb) (Eq. 7). Due to the large uncertainty in rbb, separation between POCbb and SOCnf is rather uncertain and the r2 for a correlation of mvOCnf and SOCnf is therefore affected by rbb. For example, with lower rbb of 3 (mean), the correlation between SOCnf and mvOCnf is stronger (r2=0.92) than for our best estimate of rbb(4) (r2=0.87). For rbb of 5, it is slightly weaker (r2=0.76, Fig. S8). OCnf, the sum of POCbb and SOCnf, is well constrained by F14COC and not affected by rbb. mvOCnf correlates strongly with OCnf (r2=0.91), suggesting strong impacts on the variability of mvOCnf from nonfossil emissions, including the secondary formation from nonfossil sources and primary biomass burning emissions.
SOCfossil is produced by the oxidation of VOCs from fossil origins, including coal combustion and vehicle emissions, whereas mvOCfossil can have both primary and secondary sources. No correlation is found between mvOCfossil and SOCfossil (r2=0.01, Fig. 7c), implying that in cities or pollution conditions characterized by high concentrations of fossil SOC, mvOCfossil is not concurrently elevated. However, as demonstrated by the good correlation between POCfossil and mvOCfossil (r2=0.69; Fig. 7c) we conclude that primary fossil sources may play an important role on the variation in mvOCfossil. Larger ffossil(mvOC) than ffossil(SOC) also indicates that fossil sources are associated more with mvOC than SOC. In Fig. 7c there are two outlier data points from sample Beijing-H with strongly elevated SOCfossil and lower POCfossil (shown in red circle and diamond, respectively). The highest SOCfossil ∕ POCfossil ratio (3.1) is found in Beijing-H, suggesting the largest fossil SOC formation during polluted days in Beijing compared to clean days in Beijing (Beijing-L with SOCfossil ∕ POCfossil ratio of 1.4) and samples in other cities (average SOCfossil ∕ POCfossil ratio of 0.7). This is also found in an early study that during winter 2013, SOCfossil ∕ POCfossil ratios in Beijing (with an average of 4.2) were much higher than those in Xi'an, Shanghai and Guangzhou (average SOCfossil ∕ POCfossil ratio of 1.3) (Zhang et al., 2015). This pollution event of Beijing-H is thus not included in the regression.
In this study, samples are collected on single bare quartz (BQ) filters. mvOC may be influenced by adsorption of organic vapors to the quartz fibers (positive sampling artifact). In other words, the measured mvOC in the earlier discussion includes particulate mvOC and gaseous carbon adsorbed to the quartz filters that can also be desorbed from the filters up to 200 ∘C. This artifact can be estimated by using a backup quartz filter behind the BQ filter (quartz behind quartz, QBQ). The QBQ filter is assumed to be exposed to the same organic vapor concentrations as the front BQ filter and the carbon measured on the QBQ filter is mainly due to adsorbed organic vapor and can serve as an estimate of the positive artifact (Chow et al., 2010; Zhu et al., 2012).
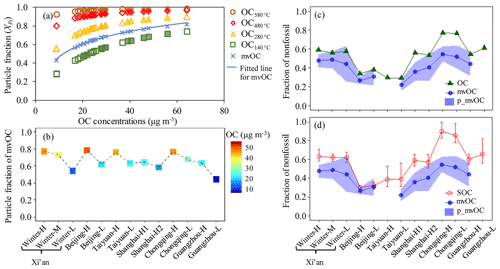
Figure 8(a) The predicted particulate fraction of OC fractions (i.e., OC, OC, OC, OC, where the subscript refers to the desorption temperature of the IMPROVE_A protocol) as function of OC concentrations (Sect. S4). Hollow shapes are used to indicate samples collected by high-volume samplers (HiVol) at a flow rate of 1.0 m3 min−1 and filled shapes by mini-volume samplers (MiniVol) at a flow rate of 5 L min−1. Particulate fraction of mvOC (Xp_mvOC, blue cross) is estimated by interpolating the particulate fraction of OC and OC. The blue solid line is the fitted line for the Xp_mvOC. (b) The Xp_mvOC for each sample corresponding to the fitted line in (a) for each sample; (c, d) fraction of nonfossil in mvOC collected on the single bare quartz (BQ) filter (fnf(mvOC), blue line and solid circle). The blue area indicates the possible range of the nonfossil fraction of particulate carbon after correction for the positive artifact (fnf(p_mvOC)), using an upper limit estimate for the positive artifact. The upper and lower limits of the range correspond to the extreme assumption that the F14C of the adsorbed organic vapor is 50 % lower and 50 % higher than measured F14C(mvOC), respectively. fnf(p_mvOC) is compared with fnf(OC) (solid green triangle) in (c) and fnf(SOC) (red circle) in (d). Interquartile range (25th–75th percentile) of the median ffossil (SOC) is shown as vertical bars.
Without the QBQ backup quartz filters in this study, we estimate the particulate fraction of mvOC (Xp_mvOC) on the BQ filters using the OC fractions measured by the thermal–optical carbon analyzer in combination with an empirical volatility basis set (VBS) model, following the method of Ma et al. (2016) (Sect. S4). The VBS bins the organic aerosol (OA) compounds according to the effective saturation concentrations (C*). For example, if C* is equal to the concentrations of OA (COA), then 50 % of OA mass will be in the particle phase (Donahue et al., 2006). Ma et al. (2016) provide empirical C* values for each desorption temperature corresponding to the measured particulate fraction on the filter, determined by the comparison of BQ and QBQ filters. They show that these empirical C* values (101.6 for OC, 101.1 for OC, 100.6 for OC, 100.1 for OC, where the subscript refers to the desorption temperature of the IMPROVE_A protocol) are useful to predict particulate fractions for various OC loadings and sampling environments. Assuming OA∕OC mass ratio is 1.8 for urban environment (Xing et al., 2013), the particulate fraction of the collected OC for each of the four IMPROVE_A temperature steps i(Xpi) can be estimated as a function of OC concentration, as shown in Fig. 8a. The particulate fraction of OC increases considerably from OC to OC and with increasing OC concentration. The desorption temperature for mvOC is 200 ∘C, falling within the 140 ∘C for OC and 280 ∘C for OC. We thus estimated the Xp_mvOC will fall within the Xp1 for OC and Xp2 for OC. Particulate fraction of mvOC is estimated by interpolating the particulate fraction of OC and OC (Fig. 8a). Xp_mvOC ranged from 0.54 for sample winter-L with the lowest OC concentration of 15 µg m−3 to 0.79 for sample Beijing-H with the highest OC concentration of 50 µg m−3 (Fig. 8b). However, for our study we consider this as a lower estimate of particulate fraction of mvOC (i.e., higher limits of the positive artifact).
Our sampling duration (24 h) is much longer than that of Ma et al. (2016), which is 45 min or 1 h. With longer sampling duration, the loading on the quartz filter of both particulate OC and adsorbed organic vapors increase initially; however, the latter will reach a saturation point and level out. Thus, the percent bias by the adsorbed organics is smaller for longer sampling duration (Turpin et al., 2000). For example, Turpin et al. (1994) found that the loading of adsorbed organics reached around 1.7 µg cm−2 and remained constant after 200 min of sampling time, but the aerosol loading increased linearly with the sampling time.
The empirically determined C* is affected by the sampling conditions, e.g., temperature. is defined at 300 K, higher than the average temperature in winter in China. The lower temperature drives the semi-volatile OC into the particle phase and leads to higher particulate fraction of each OC fraction (Donahue et al., 2006).
The adsorption of organic vapors is also influenced by the face velocity (the volumetric flow rate divided by the exposed filter area). As the face velocity decreases, the adsorption of organic gaseous species increases (McDow and Huntzicker, 1990; Turpin et al., 2000; Viana et al., 2006). In this study, most of samples were collected using high-volume samplers (HiVol) with a flow rate of 1 m3 min−1, including samples collected in Xi'an, Beijing, Guangzhou and Shanghai. Only samples in Taiyuan and Chongqing were collected using mini-volume samplers (MiniVol) at a flow rate of 5 L min−1. The face velocities for the HiVol and MiniVol sampler were 42 and 7 cm s−1, respectively. Turpin et al. (1994) found that the apparent OC concentrations on the front quartz filter were 22 % greater with a face velocity of 20 cm s−1 than those with a face velocity of 40 cm s−1. A more recent study of Kim et al. (2016) found that the positive artifacts for OC using sampler with the face velocity of 42 cm s−1 is 7.2 %, which is smaller than 12.2 % using sampler of a face velocity of 24 cm s−1 in spring and fall of 2014 in Korea. We do not take this into consideration and apply the same for HiVol and MiniVol samples, due to the lake of for different face velocities.
Due to the lack of information on how sampling duration, temperature and face velocity affect the C* for each carbon fraction, we treat the particulate fraction in Fig. 8b as a rough estimate. But with long sampling duration, low temperature and high face velocity for most of samples as well as high OC concentrations in winter in this study, the particulate fraction of mvOC on the collected filters should be considerably higher than the estimate derived from VBS. For example, Cheng and He (2015) found that the positive artifacts in OC were lowest (∼10 %) in winter, much lower than in summer (∼23 %) in Beijing, China. With the same face velocity of ∼10 cm s−1, the smaller positive artifacts in winter than that in summer can be attributed to higher OC concentrations (35 µg m−3 in winter vs. 12 µg m−3 in summer) and lower temperatures in winter. We consider the positive sampling artifact in this study is small and the collected mvOC on the bare quartz filter is dominated by the particulate mvOC.
Even though the positive artifact introduced by the adsorbed organic vapors is likely small, it is worth investigating its potential effect on the 14C results. The measured F14C(mvOC) on the BQ filter can be explained by the F14C of particulate mvOC (F14C(p_mvOC)) and adsorbed organic vapors (F14C(g_mvOC)). The F14C(p_mvOC) can be calculated as follows:
where Xp_mvOC is the estimated particulate fraction of mvOC, as shown in Fig. 8b, which is a much lower limit, as discussed earlier. (1–Xp_mvOC) is thus an estimate of positive artifact. How close the measured F14C(mvOC) is to the F14C(p_mvOC) depends on the F14C(g_mvOC).
Therefore, we conducted a sensitivity study based on the following assumption: the positive artifact is dominated by semi-volatile gases that actively partition between gas and particle phase. As the base case, we assume that F14C(g_mvOC)= F14C(mvOC) and consider extreme cases of F14C F14C(mvOC) and F14C F14C(mvOC), i.e. a variation of ±50 %. This allows F14C values for the adsorbed gas phase to be as low as for EC and higher than for OC in most cases. When the lower limit of F14C(g_mvOC) is used (50 % lower than F14C(mvOC)), the fnf(p_mvOC), calculated by dividing F14C(p_mvOC) with F14Cnf, is 0.04 to 0.19 (absolute value) higher than fnf(mvOC) (Fig. 8) but still lower than fnf(OC) and fnf(SOC) for most of samples, at least for highly polluted (H) samples with a higher particulate fraction of mvOC. Consequently, our conclusion that mvOC is more fossil than OC and SOC is still valid for most of samples, especially since this sensitivity study assumes (i) an upper limit of the positive artifact as well as (ii) a very large maximum difference between the F14C of the adsorbed gas phase and the semi-volatile particle phase. The upper limit of fnf(p_mvOC) corresponds to a semi-volatile gas phase with F14C values in the range of EC, which is probably not realistic.
Radiocarbon measurements are conducted for EC and OC of PM2.5 samples collected in six Chinese megacities during winter. In addition, the sources of mvOC are measured unambiguously using 14C. mvOC is isolated by desorbing organics in He at 200 ∘C using our custom-made aerosol combustion system with a reproducibility of ∼5 %. This new isolation method is evaluated by comparing with the OC1 fraction of the EUSAAR_2 protocol using a thermal–optical analyzer. The mvOC amount agrees very well with the OC1, despite the different heating rate and length of the temperature step, showing the representativeness of the isolated mvOC. The measured F14C(mvOC) for a combined sample (0.529±0.007), which consists of three individual daily filters, is quite similar to the weighted average of the individual filters. This further validates our isolation method for mvOC that the F14C(mvOC) is representative of the combined samples. With long sampling duration, low temperature and high face velocities as well as high OC concentrations in winter in this study, the positive artifacts to mvOC should be relatively small and play a limited role in the F14C(mvOC).
mvOC∕OC ranges from 3 % to 15 %, with an average of 10.5±3.3 %. The ambient samples in this study have a smaller mvOC∕OC than ambient samples from other places and have a much smaller mvOC fraction than fresh source samples, likely due to the effects from atmospheric aging processes. mvOC∕OC from fossil sources averages 14±6.6 %, consistently higher and more variable than that from nonfossil sources (7.5±2.9 %). That is, OC from fossil sources contains a larger more volatile fraction than that from nonfossil sources.
The nonfossil contribution to mvOC, OC and EC (fnf(mvOC), fnf(OC), fbb(EC)) varies significantly among samples in different sites: the lowest values are always found in Taiyuan and the highest values are found in Chongqing. This implies that different pollution patterns exist in individual Chinese cities. Despite the variations in fnf(mvOC), fnf(OC) and fbb(EC) in different cities, mvOC is more fossil than OC and less fossil than EC in all locations. The fossil contribution to mvOC, OC and EC are, on average, 59±11 %, 48±16 % and 73±9 %, respectively. Higher fossil contribution to mvOC than to OC is consistent with conclusions from δ13C of OC desorbed at different temperatures for ambient samples in other locations. fnf(mvOC) correlates better with fnf(OC) (r2=0.89) than fbb(EC) (r2=0.62).
SOC concentrations and sources are modeled based on the 14C-apportioned OC and EC. SOC concentrations (15.4±9.0 µg m−3) are consistently higher than those of mvOC (3.3±2.2 µg m−3), showing that the contribution of SOC to total OC is considerably larger than that of mvOC. A major fraction of SOC has desorption temperatures above 200 ∘C and falls into our operationally defined mrOC. mvOC is more fossil than SOC. The relative contribution of fossil sources to mvOC is 59 % on average (range of 45 %–78 %), which is higher than that to SOC (43 %, with a range of 10 %–70 %).
Good correlation is found between mvOCnf and OCnf(r2 = 0.91). This indicates strong impacts on the variability of mvOCnf from nonfossil emissions, including the secondary formation of OC from nonfossil sources and primary biomass burning. The variation in mvOCfossil is mainly explained by a good correlation with POCfossil (r2=0.69) and no correlation is found between mvOCfossil and SOCfossil (r2=0.01). This indicates that primary mvOC from fossil sources may play an important role in the variation in mvOCfossil.
All data needed to evaluate the conclusions in this study are present in the paper and the Supplement. Additional data related to this study are available upon request to the corresponding author.
The supplement related to this article is available online at: https://doi.org/10.5194/acp-19-10405-2019-supplement.
UD, RJH, HN and JC conducted the study design. HN, HD, WD and JZ carried out the experimental work. HN, UD, AAB and HAJM analyzed data. HN wrote the manuscript. HN, UD and RJH interpreted data and edited the manuscript. HN prepared display items. All authors commented on and discussed the manuscript.
The authors declare that they have no conflict of interest.
The authors acknowledge financial support from the Gratama Foundation. Special thanks are given to Dipayan Paul, Marc Bleeker and Henk Been for their help with the AMS measurements at CIO and to Dicky van Zonneveld for her help with 14C data correction at CIO.
This research has been supported by the National Key Research and Development Program of China (grant no. 2017YFC0212701), the National Natural Science Foundation of China (grant nos. 91644219 and 41877408), and the KNAW (grant no. 530-5CDP30).
This paper was edited by Rupert Holzinger and reviewed by Soenke Szidat and one anonymous referee.
Agrios, K., Salazar, G., and Szidat, S.: A continuous-flow gas interface of a thermal/optical analyzer With 14C AMS for source apportionment of atmospheric aerosols, Radiocarbon, 59, 921–932, https://doi.org/10.1017/RDC.2016.88, 2016.
Andersson, A.: A systematic examination of a random sampling strategy for source apportionment calculations, Sci. Total Environ., 412, 232–238, 2011.
Andersson, A., Deng, J., Du, K., Zheng, M., Yan, C., Sköld, M., and Gustafsson, Ö.: Regionally-varying combustion sources of the January 2013 severe haze events over eastern China, Environ. Sci. Technol., 49, 2038–2043, 2015.
Bond, T. C., Doherty, S. J., Fahey, D. W., Forster, P. M., Berntsen, T., DeAngelo, B. J., Flanner, M. G., Ghan, S., Kärcher, B., Koch, D., Kinne, S., Kondo, Y., Quinn, P. K., Sarofim, M. C., Schultz, M. G., Schulz, M., Venkataraman, C., Zhang, H., Zhang, S., Bellouin, N., Guttikunda, S. K., Hopke, P. K., Jacobson, M. Z., Kaiser, J. W., Klimont, Z., Lohmann, U., Schwarz, J. P., Shindell, D., Storelvmo, T., Warren, S. G., and Zender, C. S.: Bounding the role of black carbon in the climate system: a scientific assessment, J. Geophys. Res.-Atmos., 118, 5380–5552, https://doi.org/10.1002/jgrd.50171, 2013.
Burtscher, H., Baltensperger, U., Bukowiecki, N., Cohn, P., Hüglin, C., Mohr, M., Matter, U., Nyeki, S., Schmatloch, V., Streit, N., and Weingartner, E.: Separation of volatile and non-volatile aerosol fractions by thermodesorption: instrumental development and applications, J. Aerosol Sci., 32, 427–442, 2001.
Cao, J. J., Lee, S. C., Chow, J. C., Watson, J. G., Ho, K. F., Zhang, R. J., Jin, Z. D., Shen, Z. X., Chen, G. C., Kang, Y. M., Zou, S. C., Zhang, L. Z., Qi, S. H., Dai, M. H., Cheng, Y., and Hu, K.: Spatial and seasonal distributions of carbonaceous aerosols over China, J. Geophys. Res.-Atmos., 112, D22S11, https://doi.org/10.1029/2006JD008205, 2007.
Cao, J.-J., Zhu, C.-S., Tie, X.-X., Geng, F.-H., Xu, H.-M., Ho, S. S. H., Wang, G.-H., Han, Y.-M., and Ho, K.-F.: Characteristics and sources of carbonaceous aerosols from Shanghai, China, Atmos. Chem. Phys., 13, 803–817, https://doi.org/10.5194/acp-13-803-2013, 2013.
Cao, L.-M., Huang, X.-F., Li, Y.-Y., Hu, M., and He, L.-Y.: Volatility measurement of atmospheric submicron aerosols in an urban atmosphere in southern China, Atmos. Chem. Phys., 18, 1729–1743, https://doi.org/10.5194/acp-18-1729-2018, 2018.
Cavalli, F., Viana, M., Yttri, K. E., Genberg, J., and Putaud, J.-P.: Toward a standardised thermal-optical protocol for measuring atmospheric organic and elemental carbon: the EUSAAR protocol, Atmos. Meas. Tech., 3, 79–89, https://doi.org/10.5194/amt-3-79-2010, 2010.
Chen, L.-W. A., Moosmüller, H., Arnott, W. P., Chow, J. C., Watson, J. G., Susott, R. A., Babbitt, R. E., Wold, C. E., Lincoln, E. N., and Hao, W. M.: Emissions from laboratory combustion of wildland fuels: emission factors and source profiles, Environ. Sci. Technol., 41, 4317–4325, 2007.
Chen, Y., Xie, S.-D., Luo, B., and Zhai, C.-Z.: Particulate pollution in urban Chongqing of southwest China: historical trends of variation, chemical characteristics and source apportionment, Sci. Total Environ., 584–585, 523–534, https://doi.org/10.1016/j.scitotenv.2017.01.060, 2017.
Cheng, Y. and He, K. B.: Uncertainties in observational data on organic aerosol: An annual perspective of sampling artifacts in Beijing, China, Environ. Pollut., 206, 113–121, 2015.
Chow, J. C., Watson, J. G., Kuhns, H., Etyemezian, V., Lowenthal, D. H., Crow, D., Kohl, S. D., Engelbrecht, J. P., and Green, M. C.: Source profiles for industrial, mobile, and area sources in the Big Bend Regional Aerosol Visibility and Observational study, Chemosphere, 54, 185–208, https://doi.org/10.1016/j.chemosphere.2003.07.004, 2004.
Chow, J. C., Watson, J. G., Chen, L.-W. A., Chang, M. O., Robinson, N. F., Trimble, D., and Kohl, S.: The IMPROVE_A temperature protocol for thermal/optical carbon analysis: maintaining consistency with a long-term database, J. Air Waste Manage., 57, 1014–1023, 2007.
Chow, J. C., Watson, J. G., Chen, L.-W. A., Rice, J., and Frank, N. H.: Quantification of PM2.5 organic carbon sampling artifacts in US networks, Atmos. Chem. Phys., 10, 5223–5239, https://doi.org/10.5194/acp-10-5223-2010, 2010.
Donahue, N. M., Robinson, A. L., Stanier, C. O., and Pandis, S. N.: Coupled partitioning, dilution, and chemical aging of semivolatile organics, Environ. Sci. Technol., 40, 2635–2643, 2006.
Donahue, N. M., Robinson, A. L., and Pandis, S. N.: Atmospheric organic particulate matter: from smoke to secondary organic aerosol, Atmos. Environ., 43, 94–106, https://doi.org/10.1016/j.atmosenv.2008.09.055, 2009.
Donahue, N. M., Epstein, S. A., Pandis, S. N., and Robinson, A. L.: A two-dimensional volatility basis set: 1. organic-aerosol mixing thermodynamics, Atmos. Chem. Phys., 11, 3303–3318, https://doi.org/10.5194/acp-11-3303-2011, 2011.
Dusek, U., Ten Brink, H., Meijer, H., Kos, G., Mrozek, D., Röckmann, T., Holzinger, R., and Weijers, E.: The contribution of fossil sources to the organic aerosol in the Netherlands, Atmos. Environ., 74, 169–176, 2013.
Dusek, U., Monaco, M., Prokopiou, M., Gongriep, F., Hitzenberger, R., Meijer, H. A. J., and Röckmann, T.: Evaluation of a two-step thermal method for separating organic and elemental carbon for radiocarbon analysis, Atmos. Meas. Tech., 7, 1943–1955, https://doi.org/10.5194/amt-7-1943-2014, 2014.
Dusek, U., Hitzenberger, R., Kasper-Giebl, A., Kistler, M., Meijer, H. A. J., Szidat, S., Wacker, L., Holzinger, R., and Röckmann, T.: Sources and formation mechanisms of carbonaceous aerosol at a regional background site in the Netherlands: insights from a year-long radiocarbon study, Atmos. Chem. Phys., 17, 3233–3251, https://doi.org/10.5194/acp-17-3233-2017, 2017.
Ehn, M., Thornton, J. A., Kleist, E., Sipilä, M., Junninen, H., Pullinen, I., Springer, M., Rubach, F., Tillmann, R., Lee, B., Lopez-Hilfiker, F., Andres, S., Acir, I.-H., Rissanen, M., Jokinen, T., Schobesberger, S., Kangasluoma, J., Kontkanen, J., Nieminen, T., Kurtén, T., Nielsen, L. B., Jørgensen, S., Kjaergaard, H. G., Canagaratna, M., Maso, M. D., Berndt, T., Petäjä, T., Wahner, A., Kerminen, V.-M., Kulmala, M., Worsnop, D. R., Wildt, J., and Mentel, T. F.: A large source of low-volatility secondary organic aerosol, Nature, 506, 476–479, 2014.
Fang, W., Andersson, A., Zheng, M., Lee, M., Holmstrand, H., Kim, S.-W., Du, K., and Gustafsson, Ö.: Divergent evolution of carbonaceous aerosols during dispersal of East Asian haze, Sci. Rep.-UK, 7, 10422, https://doi.org/10.1038/s41598-017-10766-4, 2017.
Fuzzi, S., Baltensperger, U., Carslaw, K., Decesari, S., Denier van der Gon, H., Facchini, M. C., Fowler, D., Koren, I., Langford, B., Lohmann, U., Nemitz, E., Pandis, S., Riipinen, I., Rudich, Y., Schaap, M., Slowik, J. G., Spracklen, D. V., Vignati, E., Wild, M., Williams, M., and Gilardoni, S.: Particulate matter, air quality and climate: lessons learned and future needs, Atmos. Chem. Phys., 15, 8217–8299, https://doi.org/10.5194/acp-15-8217-2015, 2015.
Gaston, C. J., Lopez-Hilfiker, F. D., Whybrew, L. E., Hadley, O., McNair, F., Gao, H., Jaffe, D. A., and Thornton, J. A.: Online molecular characterization of fine particulate matter in Port Angeles, WA: Evidence for a major impact from residential wood smoke, Atmos. Environ., 138, 99–107, https://doi.org/10.1016/j.atmosenv.2016.05.013, 2016.
Gkatzelis, G. I., Tillmann, R., Hohaus, T., Müller, M., Eichler, P., Xu, K.-M., Schlag, P., Schmitt, S. H., Wegener, R., Kaminski, M., Holzinger, R., Wisthaler, A., and Kiendler-Scharr, A.: Comparison of three aerosol chemical characterization techniques utilizing PTR-ToF-MS: a study on freshly formed and aged biogenic SOA, Atmos. Meas. Tech., 11, 1481–1500, https://doi.org/10.5194/amt-11-1481-2018, 2018.
Grieshop, A. P., Donahue, N. M., and Robinson, A. L.: Laboratory investigation of photochemical oxidation of organic aerosol from wood fires 2: analysis of aerosol mass spectrometer data, Atmos. Chem. Phys., 9, 2227–2240, https://doi.org/10.5194/acp-9-2227-2009, 2009a.
Grieshop, A. P., Logue, J. M., Donahue, N. M., and Robinson, A. L.: Laboratory investigation of photochemical oxidation of organic aerosol from wood fires 1: measurement and simulation of organic aerosol evolution, Atmos. Chem. Phys., 9, 1263–1277, https://doi.org/10.5194/acp-9-1263-2009, 2009b.
Grieshop, A. P., Miracolo, M. A., Donahue, N. M., and Robinson, A. L.: Constraining the volatility distribution and gas-particle partitioning of combustion aerosols using isothermal dilution and thermodenuder measurements, Environ. Sci. Technol., 43, 4750–4756, 2009c.
Guo, S., Hu, M., Guo, Q., Zhang, X., Zheng, M., Zheng, J., Chang, C. C., Schauer, J. J., and Zhang, R.: Primary sources and secondary formation of organic aerosols in Beijing, China, Environ. Sci. Technol., 46, 9846–9853, 2012.
Guo, S., Hu, M., Zamora, M. L., Peng, J., Shang, D., Zheng, J., Du, Z., Wu, Z., Shao, M., Zeng, L., Molina, M. J., and Zhang, R: Elucidating severe urban haze formation in China, P. Natl. Acad. Sci. USA, 111, 17373–17378, https://doi.org/10.1073/pnas.1419604111, 2014.
Hallquist, M., Wenger, J. C., Baltensperger, U., Rudich, Y., Simpson, D., Claeys, M., Dommen, J., Donahue, N. M., George, C., Goldstein, A. H., Hamilton, J. F., Herrmann, H., Hoffmann, T., Iinuma, Y., Jang, M., Jenkin, M. E., Jimenez, J. L., Kiendler-Scharr, A., Maenhaut, W., McFiggans, G., Mentel, Th. F., Monod, A., Prévôt, A. S. H., Seinfeld, J. H., Surratt, J. D., Szmigielski, R., and Wildt, J.: The formation, properties and impact of secondary organic aerosol: current and emerging issues, Atmos. Chem. Phys., 9, 5155–5236, https://doi.org/10.5194/acp-9-5155-2009, 2009.
Han, Y. M., Chen, L.W., Huang, R.J., Chow, J. C., Watson, J. G., Ni, H. Y., Liu, S. X., Fung, K. K., Shen, Z. X, Wei, C., Wang, Q. Y., Tian, J., Zhao, Z. Z., Prévôt, A. S. H., and Cao, J. J.: Carbonaceous aerosols in megacity Xi'an, China: implications of thermal/optical protocols comparison, Atmos. Environ., 132, 58–68, 2016.
Heal, M. R.: The application of carbon-14 analyses to the source apportionment of atmospheric carbonaceous particulate matter: a review, Anal. Bioanal. Chem., 406, 81–98, 2014.
Holzinger, R., Williams, J., Herrmann, F., Lelieveld, J., Donahue, N. M., and Röckmann, T.: Aerosol analysis using a Thermal-Desorption Proton-Transfer-Reaction Mass Spectrometer (TD-PTR-MS): a new approach to study processing of organic aerosols, Atmos. Chem. Phys., 10, 2257–2267, https://doi.org/10.5194/acp-10-2257-2010, 2010.
Holzinger, R., Goldstein, A. H., Hayes, P. L., Jimenez, J. L., and Timkovsky, J.: Chemical evolution of organic aerosol in Los Angeles during the CalNex 2010 study, Atmos. Chem. Phys., 13, 10125–10141, https://doi.org/10.5194/acp-13-10125-2013, 2013.
Hu, D., Bian, Q., Lau, A. K. H., and Yu, J. Z.: Source apportioning of primary and secondary organic carbon in summer PM2.5 in Hong Kong using positive matrix factorization of secondary and primary organic tracer data, J. Geophys. Res.-Atmos., 115, D16204, https://doi.org/10.1029/2009jd012498, 2010.
Huang, R. J., Zhang, Y., Bozzeti, C., Ho, K. F., Cao, J. J., Han, Y., Daellenbach, K. R., Slowik, J.G., Platt, S. M., Canonaco, F., Zotter, P., Wolf, R., Pieber, S. M., Bruns, E. A., Crippa, M., Ciarelli, G., Piazzalunga, A., Schwikowski, M., Abbaszade, G., SchnelleKreis, J., Zimmermann, R., An, Z., Szidat, S., Baltensperger, U., El Haddad, I., and Prévôt, A. S. H.: High secondary aerosol contribution to particulate pollution during haze events in China, Nature, 514, 218–222, https://doi.org/10.1038/nature13774, 2014.
Huffman, J. A., Docherty, K. S., Aiken, A. C., Cubison, M. J., Ulbrich, I. M., DeCarlo, P. F., Sueper, D., Jayne, J. T., Worsnop, D. R., Ziemann, P. J., and Jimenez, J. L.: Chemically-resolved aerosol volatility measurements from two megacity field studies, Atmos. Chem. Phys., 9, 7161–7182, https://doi.org/10.5194/acp-9-7161-2009, 2009a.
Huffman, J., Docherty, K., Mohr, C., Cubison, M., Ulbrich, I., Ziemann, P., Onasch, T., and Jimenez, J.: Chemically-resolved volatility measurements of organic aerosol from different sources, Environ. Sci. Technol., 43, 5351–5357, 2009b.
Jacobson, M. C., Hansson, H. C., Noone, K. J., and Charlson, R. J.: Organic atmospheric aerosols: review and state of the science, Rev. Geophys., 38, 267–294, 2000.
Jayne, J. T., Leard, D. C., Zhang, X., Davidovits, P., Smith, K. A., Kolb, C. E., and Worsnop, D. R.: Development of an aerosol mass spectrometer for size and composition analysis of submicron particles, Aerosol Sci. Technol., 33, 49–70, https://doi.org/10.1080/027868200410840, 2000.
Jimenez, J. L., Canagaratna, M. R., Donahue, N. M., Prevot, A. S. H., Zhang, Q., Kroll, J. H., DeCarlo, P. F., Allan, J. D., Coe, H., Ng, N. L., Aiken, A. C., Docherty, K. S., Ulbrich, I. M., Grieshop, A. P., Robinson, A. L., Duplissy, J., Smith, J. D., Wilson, K. R., Lanz, V. A., Hueglin, C., Sun, Y. L., Tian, J., Laaksonen, A., Raatikainen, T., Rautiainen, J., Vaattovaara, P., Ehn, M., Kulmala, M., Tomlinson, J. M., Collins, D. R., Cubison, M. J., Dunlea, J., Huffman, J. A., Onasch, T. B., Alfarra, M. R., Williams, P. I., Bower, K., Kondo, Y., Schneider, J., Drewnick, F., Borrmann, S., Weimer, S., Demerjian, K., Salcedo, D., Cottrell, L., Griffin, R., Takami, A., Miyoshi, T., Hatakeyama, S., Shimono, A., Sun, J. Y., Zhang, Y. M., Dzepina, K., Kimmel, J. R., Sueper, D., Jayne, J. T., Herndon, S. C., Trimborn, A. M., Williams, L. R., Wood, E. C., Middlebrook, A. M., Kolb, C. E., Baltensperger, U., and Worsnop, D. R.: Evolution of organic aerosols in the atmosphere, Science, 326, 1525–1529, 2009.
Keller, A. and Burtscher, H.: Characterizing particulate emissions from wood burning appliances including secondary organic aerosol formation potential, J. Aerosol Sci., 114, 21–30, https://doi.org/10.1016/j.jaerosci.2017.08.014, 2017.
Kim, D., Jung, J., Lee, H., Choi, S.-H., Lee, S., and Oh, S.: Characterization of sampling artifacts in the measurement of carbonaceous particles using high- and low-volume samplers in Daejeon, Korea, Atmos. Environ., 139, 157–166, https://doi.org/10.1016/j.atmosenv.2016.05.029, 2016.
Levin, I., Naegler, T., Kromer, B., Diehl, M., Francey, R. J., Gomez-Pelaez, A. J., Steele, L., Wagenbach, D., Weller, R., and Worthy, D. E.: Observations and modelling of the global distribution and long-term trend of atmospheric 14CO2, Tellus B, 62, 26–46, 2010.
Lewis, C. W., Klouda, G. A., and Ellenson, W. D.: Radiocarbon measurement of the biogenic contribution to summertime PM2.5 ambient aerosol in Nashville, TN, Atmos. Environ., 38, 6053–6061, 2004.
Lipsky, E. M. and Robinson, A. L.: Effects of dilution on fine particle mass and partitioning of semivolatile organics in diesel exhaust and wood smoke, Environ. Sci. Technol., 40, 155–162, 2006.
Liu, J., Li, J., Ding, P., Zhang, Y., Liu, D., Shen, C., and Zhang, G.: Optimizing isolation protocol of organic carbon and elemental carbon for 14C analysis using fine particulate samples, Atmos. Environ., 154, 9–19, https://doi.org/10.1016/j.atmosenv.2017.01.027, 2017.
Lopez-Hilfiker, F. D., Mohr, C., Ehn, M., Rubach, F., Kleist, E., Wildt, J., Mentel, Th. F., Lutz, A., Hallquist, M., Worsnop, D., and Thornton, J. A.: A novel method for online analysis of gas and particle composition: description and evaluation of a Filter Inlet for Gases and AEROsols (FIGAERO), Atmos. Meas. Tech., 7, 983–1001, https://doi.org/10.5194/amt-7-983-2014, 2014.
Lopez-Hilfiker, F. D., Mohr, C., Ehn, M., Rubach, F., Kleist, E., Wildt, J., Mentel, Th. F., Carrasquillo, A. J., Daumit, K. E., Hunter, J. F., Kroll, J. H., Worsnop, D. R., and Thornton, J. A.: Phase partitioning and volatility of secondary organic aerosol components formed from α-pinene ozonolysis and OH oxidation: the importance of accretion products and other low volatility compounds, Atmos. Chem. Phys., 15, 7765–7776, https://doi.org/10.5194/acp-15-7765-2015, 2015.
Ma, J., Li, X., Gu, P., Dallmann, T. R., Presto, A. A., and Donahue, N. M.: Estimating ambient particulate organic carbon concentrations and partitioning using thermal optical measurements and the volatility basis set, Aerosol Sci. Tech., 50, 638–651, 2016.
Masalaite, A., Holzinger, R., Remeikis, V., Roeckmann, T., and Dusek, U.: Characteristics, sources and evolution of fine aerosol (PM1) at urban, coastal and forest background sites in Lithuania, Atmos. Environ., 148, 62–76, 2017.
Masalaite, A., Holzinger, R., Ceburnis, D., Remeikis, V., Ulevičius, V., Röckmann, T., and Dusek, U.: Sources and atmospheric processing of size segregated aerosol particles revealed by stable carbon isotope ratios and chemical speciation, Environ. Pollut., 240, 286–296, https://doi.org/10.1016/j.envpol.2018.04.073, 2018.
May, A. A., Presto, A. A., Hennigan, C. J., Nguyen, N. T., Gordon, T. D., and Robinson, A. L.: Gas-particle partitioning of primary organic aerosol emissions: (1) gasoline vehicle exhaust, Atmos. Environ., 77, 128–139, https://doi.org/10.1016/j.atmosenv.2013.04.060, 2013a.
May, A. A., Presto, A. A., Hennigan, C. J., Nguyen, N. T., Gordon, T. D., and Robinson, A. L.: Gas-particle partitioning of primary organic aerosol emissions:(2) diesel vehicles, Environ. Sci. Technol., 47, 8288–8296, 2013b.
McDow, S. R. and Huntzicker, J. J.: Vapor adsorption artifact in the sampling of organic aerosol: Face velocity effects, Atmos. Environ. A.-Gen., 24, 2563–2571, https://doi.org/10.1016/0960-1686(90)90134-9, 1990.
Meusinger, C., Dusek, U., King, S. M., Holzinger, R., Rosenørn, T., Sperlich, P., Julien, M., Remaud, G. S., Bilde, M., Röckmann, T., and Johnson, M. S.: Chemical and isotopic composition of secondary organic aerosol generated by α-pinene ozonolysis, Atmos. Chem. Phys., 17, 6373–6391, https://doi.org/10.5194/acp-17-6373-2017, 2017.
Mohn, J., Szidat, S., Fellner, J., Rechberger, H., Quartier, R., Buchmann, B., and Emmenegger, L.: Determination of biogenic and fossil CO2 emitted by waste incineration based on 14CO2 and mass balances, Bioresource Technol., 99, 6471–6479, 2008.
Mook, W. G. and van der Plicht, J.: Reporting 14C activities and concentrations, Radiocarbon, 41, 227–239, 1999.
Ni, H., Huang, R.-J., Cao, J., Liu, W., Zhang, T., Wang, M., Meijer, H. A. J., and Dusek, U.: Source apportionment of carbonaceous aerosols in Xi'an, China: insights from a full year of measurements of radiocarbon and the stable isotope 13C, Atmos. Chem. Phys., 18, 16363–16383, https://doi.org/10.5194/acp-18-16363-2018, 2018.
Paciga, A., Karnezi, E., Kostenidou, E., Hildebrandt, L., Psichoudaki, M., Engelhart, G. J., Lee, B.-H., Crippa, M., Prévôt, A. S. H., Baltensperger, U., and Pandis, S. N.: Volatility of organic aerosol and its components in the megacity of Paris, Atmos. Chem. Phys., 16, 2013–2023, https://doi.org/10.5194/acp-16-2013-2016, 2016.
Palstra, S. W. and Meijer, H. A.: Biogenic carbon fraction of biogas and natural gas fuel mixtures determined with 14C, Radiocarbon, 56, 7–28, 2014.
Petzold, A., Ogren, J. A., Fiebig, M., Laj, P., Li, S.-M., Baltensperger, U., Holzer-Popp, T., Kinne, S., Pappalardo, G., Sugimoto, N., Wehrli, C., Wiedensohler, A., and Zhang, X.-Y.: Recommendations for reporting “black carbon” measurements, Atmos. Chem. Phys., 13, 8365–8379, https://doi.org/10.5194/acp-13-8365-2013, 2013.
Pöschl, U.: Aerosol particle analysis: challenges and progress, Anal. Bioanal. Chem., 375, 30–32, 2003.
Pöschl, U.: Atmospheric aerosols: composition, transformation, climate and health effects, Angew. Chem. Int. Edit., 44, 7520–7540, 2005.
Reimer, P. J., Brown, T. A., and Reimer, R. W.: Discussion: reporting and calibration of post-bomb 14C data, Radiocarbon, 46, 1299–1304, 2004.
Robinson, A. L., Donahue, N. M., Shrivastava, M. K., Weitkamp, E. A., Sage, A. M., Grieshop, A. P., Lane, T. E., Pierce, J. R., and Pandis, S. N.: Rethinking organic aerosols: semivolatile emissions and photochemical aging, Science, 315, 1259–1262, 2007.
Ruff, M., Wacker, L., Gäggeler, H., Suter, M., Synal, H.-A., and Szidat, S.: A gas ion source for radiocarbon measurements at 200 kV, Radiocarbon, 49, 307–314, 2007.
Salazar, G., Zhang, Y. L., Agrios, K., and Szidat, S.: Development of a method for fast and automatic radiocarbon measurement of aerosol samples by online coupling of an elemental analyzer with a MICADAS AMS, Nucl. Instrum. Meth. B, 361, 163–167, https://doi.org/10.1016/j.nimb.2015.03.051, 2015.
Salo, K., Hallquist, M., Jonsson, Å. M., Saathoff, H., Naumann, K.-H., Spindler, C., Tillmann, R., Fuchs, H., Bohn, B., Rubach, F., Mentel, Th. F., Müller, L., Reinnig, M., Hoffmann, T., and Donahue, N. M.: Volatility of secondary organic aerosol during OH radical induced ageing, Atmos. Chem. Phys., 11, 11055–11067, https://doi.org/10.5194/acp-11-11055-2011, 2011.
Shrivastava, M. K., Lipsky, E. M., Stanier, C. O., and Robinson, A. L.: Modeling semivolatile organic aerosol mass emissions from combustion systems, Environ. Sci. Technol., 40, 2671–2677, 2006.
Song, Y., Zhang, Y., Xie, S., Zeng, L., Zheng, M., Salmon, L. G., Shao, M., and Slanina, S.: Source apportionment of PM2.5 in Beijing by positive matrix factorization, Atmos. Environ., 40, 1526–1537, https://doi.org/10.1016/j.atmosenv.2005.10.039, 2006.
Spronsen, M. A., Frenken, J. W., and Groot, I. M.: Observing the oxidation of platinum, Nat. Commun., 8, 429, https://doi.org/10.1038/s41467-017-00643-z, 2017.
Synal, H.-A., Stocker, M., and Suter, M.: MICADAS: A new compact radiocarbon AMS system, Nucl. Instrum. Meth. B, 259, 7–13, https://doi.org/10.1016/j.nimb.2007.01.138, 2007.
Szidat, S., Jenk, T. M., Gäggeler, H. W., Synal, H.-A., Fisseha, R., Baltensperger, U., Kalberer, M., Samburova, V., Reimann, S., Kasper-Giebl, A., and Hajdas, I.: Radiocarbon (14C)-deduced biogenic and anthropogenic contributions to organic carbon (OC) of urban aerosols from Zürich, Switzerland, Atmos. Environ., 38, 4035–4044, 2004.
Szidat, S., Jenk, T. M., Synal, H. A., Kalberer, M., Wacker, L., Hajdas, I., Kasper-Giebl, A., and Baltensperger, U.: Contributions of fossil fuel, biomass-burning, and biogenic emissions to carbonaceous aerosols in Zurich as traced by 14C, J. Geophys. Res.-Atmos., 111, D07206, https://doi.org/10.1029/2005JD006590, 2006.
Tao, J., Zhang, L., Cao, J., and Zhang, R.: A review of current knowledge concerning PM2.5 chemical composition, aerosol optical properties and their relationships across China, Atmos. Chem. Phys., 17, 9485–9518, https://doi.org/10.5194/acp-17-9485-2017, 2017.
Tian, J., Ni, H., Cao, J., Han, Y., Wang, Q., Wang, X., Chen, L. W. A., Chow, J. C., Watson, J. G., Wei, C., Sun, J., Zhang, T., and Huang, R.: Characteristics of carbonaceous particles from residential coal combustion and agricultural biomass burning in China, Atmos. Pollut. Res., 8, 521–527, https://doi.org/10.1016/j.apr.2016.12.006, 2017.
Timkovsky, J., Dusek, U., Henzing, J. S., Kuipers, T. L., Röckmann, T., and Holzinger, R.: Offline thermal-desorption proton-transfer-reaction mass spectrometry to study composition of organic aerosol, J. Aerosol Sci., 79, 1–14, https://doi.org/10.1016/j.jaerosci.2014.08.010, 2015.
Turpin, B. J., Huntzicker, J. J., and Hering, S. V.: Investigation of organic aerosol sampling artifacts in the Los Angeles Basin, Atmos. Environ., 28, 3061–3071, 1994.
Turpin, B. J., Saxena, P., and Andrews, E.: Measuring and simulating particulate organics in the atmosphere: problems and prospects, Atmos. Environ., 34, 2983–3013, https://doi.org/10.1016/S1352-2310(99)00501-4, 2000.
Viana, M., Chi, X., Maenhaut, W., Cafmeyer, J., Querol, X., Alastuey, A., Mikuška, P., and Večeřa, Z.: Influence of sampling artefacts on measured PM, OC, and EC levels in carbonaceous aerosols in an urban area, Aerosol Sci. Tech., 40, 107–117, 2006.
Vodička, P., Schwarz, J., Cusack, M., and Ždímal, V.: Detailed comparison of OC/EC aerosol at an urban and a rural Czech background site during summer and winter, Sci. Total Environ., 518–519, 424–433, https://doi.org/10.1016/j.scitotenv.2015.03.029, 2015.
Wacker, L., Christl, M., and Synal, H. A.: Bats: A new tool for AMS data reduction, Nucl. Instrum. Meth. B, 268, 976–979, https://doi.org/10.1016/j.nimb.2009.10.078, 2010.
Wang, Y. C., Huang, R. J., Ni, H. Y., Chen, Y., Wang, Q. Y., Li, G. H., Tie, X. X., Shen, Z. X., Huang, Y., Liu, S. X., Dong, W. M., Xue, P., Fröhlich, R., Canonaco, F., Elser, M., Daellenbach, K. R., El Haddad, I., Prevot, A. S. H., Canagaratna, M. R., Worsnop, D. R., Cao, J. J.: Chemical composition, sources and secondary processes of aerosols in Baoji city of north China, Atmos. Environ., 158, 128–137, 2017.
Watson, J. G., Chow, J. C., Lowenthal, D. H., Pritchett, L. C., Frazier, C. A., Neuroth, G. R., and Robbins, R.: Differences in the carbon composition of source profiles for diesel- and gasoline-powered vehicles, Atmos. Environ., 28, 2493–2505, https://doi.org/10.1016/1352-2310(94)90400-6, 1994.
Wehner, B., Philippin, S., and Wiedensohler, A.: Design and calibration of a thermodenuder with an improved heating unit to measure the size-dependent volatile fraction of aerosol particles, J. Aerosol Sci., 33, 1087–1093, 2002.
Xing, L., Fu, T.-M., Cao, J. J., Lee, S. C., Wang, G. H., Ho, K. F., Cheng, M.-C., You, C.-F., and Wang, T. J.: Seasonal and spatial variability of the OM∕OC mass ratios and high regional correlation between oxalic acid and zinc in Chinese urban organic aerosols, Atmos. Chem. Phys., 13, 4307–4318, https://doi.org/10.5194/acp-13-4307-2013, 2013.
Yu, J. Z., Xu, J., and Yang, H.: Charring characteristics of atmospheric organic particulate matter in thermal analysis, Environ. Sci. Technol., 36, 754–761, 2002.
Zenker, K., Vonwiller, M., Szidat, S., Calzolai, G., Giannoni, M., Bernardoni, V., Jedynska, A. D., Henzing, B., Meijer, H. A., and Dusek, U.: Evaluation and inter-comparison of oxygen-based OC-EC separation methods for radiocarbon analysis of ambient aerosol particle samples, Atmosphere, 8, 226, https://doi.org/10.3390/atmos8110226, 2017.
Zhang, Y. L., Perron, N., Ciobanu, V. G., Zotter, P., Minguillón, M. C., Wacker, L., Prévôt, A. S. H., Baltensperger, U., and Szidat, S.: On the isolation of OC and EC and the optimal strategy of radiocarbon-based source apportionment of carbonaceous aerosols, Atmos. Chem. Phys., 12, 10841–10856, https://doi.org/10.5194/acp-12-10841-2012, 2012.
Zhang, Y.-L., Huang, R.-J., El Haddad, I., Ho, K.-F., Cao, J.-J., Han, Y., Zotter, P., Bozzetti, C., Daellenbach, K. R., Canonaco, F., Slowik, J. G., Salazar, G., Schwikowski, M., Schnelle-Kreis, J., Abbaszade, G., Zimmermann, R., Baltensperger, U., Prévôt, A. S. H., and Szidat, S.: Fossil vs. non-fossil sources of fine carbonaceous aerosols in four Chinese cities during the extreme winter haze episode of 2013, Atmos. Chem. Phys., 15, 1299–1312, https://doi.org/10.5194/acp-15-1299-2015, 2015.
Zhu, C.-S., Chen, C.-C., Cao, J.-J., Tsai, C.-J., Chou, C. C.-K., Liu, S.-C., and Roam, G.-D.: Characterization of carbon fractions for atmospheric fine particles and nanoparticles in a highway tunnel, Atmos. Environ., 44, 2668–2673, 2010.
Zhu, C.-S., Tsai, C.-J., Chen, S.-C., Cao, J.-J., and Roam, G.-D.: Positive sampling artifacts of organic carbon fractions for fine particles and nanoparticles in a tunnel environment, Atmos. Environ., 54, 225–230, 2012.
Zhu, C.-S., Cao, J.-J., Tsai, C.-J., Shen, Z.-X., Han, Y.-M., Liu, S.-X., and Zhao, Z.-Z.: Comparison and implications of PM2.5 carbon fractions in different environments, Sci. Total Environ., 466–467, 203–209, https://doi.org/10.1016/j.scitotenv.2013.07.029, 2014.