the Creative Commons Attribution 4.0 License.
the Creative Commons Attribution 4.0 License.
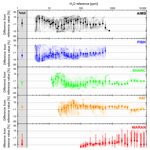
Intercomparison of midlatitude tropospheric and lower-stratospheric water vapor measurements and comparison to ECMWF humidity data
Christiane Voigt
Romy Heller
Tina Jurkat-Witschas
Martina Krämer
Christian Rolf
Martin Zöger
Andreas Giez
Bernhard Buchholz
Volker Ebert
Troy Thornberry
Ulrich Schumann
Accurate measurement of water vapor in the climate-sensitive region near the tropopause is very challenging. Unexplained systematic discrepancies between measurements at low water vapor mixing ratios made by different instruments on airborne platforms have limited our ability to adequately address a number of relevant scientific questions on the humidity distribution, cloud formation and climate impact in that region. Therefore, during the past decade, the scientific community has undertaken substantial efforts to understand these discrepancies and improve the quality of water vapor measurements. This study presents a comprehensive intercomparison of airborne state-of-the-art in situ hygrometers deployed on board the DLR (German Aerospace Center) research aircraft HALO (High Altitude and LOng Range Research Aircraft) during the Midlatitude CIRRUS (ML-CIRRUS) campaign conducted in 2014 over central Europe. The instrument intercomparison shows that the hygrometer measurements agree within their combined accuracy (±10 % to 15 %, depending on the humidity regime); total mean values agree within 2.5 %. However, systematic differences on the order of 10 % and up to a maximum of 15 % are found for mixing ratios below 10 parts per million (ppm) H2O. A comparison of relative humidity within cirrus clouds does not indicate a systematic instrument bias in either water vapor or temperature measurements in the upper troposphere. Furthermore, in situ measurements are compared to model data from the European Centre for Medium-Range Weather Forecasts (ECMWF) which are interpolated along the ML-CIRRUS flight tracks. We find a mean agreement within ±10 % throughout the troposphere and a significant wet bias in the model on the order of 100 % to 150 % in the stratosphere close to the tropopause. Consistent with previous studies, this analysis indicates that the model deficit is mainly caused by too weak of a humidity gradient at the tropopause.
- Article
(7452 KB) - Full-text XML
- BibTeX
- EndNote
Water vapor is one of the most important trace gases in Earth's atmosphere due to its large influence on the radiation budget and atmospheric dynamics. It absorbs and emits infrared radiation throughout the entire profile of the atmosphere (Kiehl and Trenberth, 1997). The radiative effect of small changes in water vapor concentration is most pronounced in the upper troposphere and lower stratosphere (UTLS), where absolute H2O mixing ratios are 2–4 orders of magnitude lower than at ground level (e.g., Ramanathan and Inamdar, 2006; Solomon et al., 2010; Riese et al., 2012). Besides the direct radiative effect, water vapor also provides one of the strongest feedback parameters to temperature changes in the atmosphere (Manabe and Wetherald, 1967; Dessler et al., 2008).
Additionally, water vapor is the most important parameter for cloud formation and lifetime. From an energy perspective, clouds not only influence the radiation balance but also redistribute energy through latent heat during condensation and evaporation. Changes in latent heat fluxes influence global dynamics like the Hadley circulation and extratropical storm tracks (Schneider et al., 2010). The radiative effect of clouds is more complex than the effect of greenhouse gases due to very inhomogeneous cloud cover and different microphysical and thus radiative properties of clouds at different altitudes. The opposing effects of the reflection of solar shortwave radiation and the trapping of longwave radiation determine the net radiative effect of clouds, whether cooling or heating, depending on cloud properties, surface albedo, sun elevation etc. (e.g., Liou, 1986; Lynch, 1996; Lee et al., 2009).
The various atmospheric processes related to water vapor impose challenges for its measurement. The measurement accuracy and resolution required to improve our understanding of the atmosphere strongly depend on the research question. Regarding the radiative effect of stratospheric H2O, the main challenge is the absolute accuracy at mixing ratios below 10 parts per million (ppm, equivalent to µmol mol−1) since small changes of less than 1 ppm significantly impact the radiation budget (Solomon et al., 2010). For cloud effects, the challenge is even bigger, especially in very cold ice clouds where ice supersaturation and cloud properties are strongly linked (Jensen et al., 2005; Shilling et al., 2006; Krämer et al., 2009). A 10 % difference in relative humidity with respect to ice (RHi), which falls within the combined uncertainty in water vapor and temperature measurements, can result in substantially different cloud properties.
During the past several decades, a number of H2O measurement intercomparisons during field campaigns including aircraft in situ, balloon-borne and satellite instruments revealed that the relative measurement uncertainty in water vapor mixing ratio was significantly higher than 10 %, even occasionally exceeding 100 % at the lowest mixing ratios in the lower stratosphere (e.g., Oltmans et al., 2000; Vömel et al., 2007; Weinstock et al., 2009). These large discrepancies motivated the comprehensive intercomparison campaign AquaVIT-1 at the AIDA (Aerosol Interaction and Dynamics in the Atmosphere) cloud chamber in Karlsruhe in 2007 (Fahey et al., 2014) and the follow-up but as-yet undocumented campaigns AquaVIT-2 and -3 in 2013 and 2015, respectively. In the controlled environment of the cloud chamber, the agreement between the instruments during AquaVIT-1 was better compared to the measurements on the different airborne platforms but still in the 20 % range for mixing ratios between 1 and 10 ppm. As a consequence, novel concepts and instruments (e.g., Thornberry et al., 2013; Kaufmann et al., 2014, 2016; Buchholz et al., 2017) and improved techniques for in-flight (Rollins et al., 2011) and ground calibration (Meyer et al., 2015) were developed to improve the accuracy of H2O measurements.
Since space and measurement time on research aircraft are limited and expensive, intercomparable airborne data sets of water vapor measurements are scarce (e.g., Kiemle et al., 2008; Jensen et al., 2017a). The most recent comprehensive intercomparison was conducted in 2011 on the NASA WB-57 high-altitude aircraft during the MACPEX (Mid-latitude Airborne Cirrus Properties EXperiment) campaign (Rollins et al., 2014). Similar to the present study, five different hygrometers using differing water vapor detection techniques were mounted on the aircraft. In the dry regime below 10 ppm, instruments were found to typically agree within their stated combined accuracies. However, the authors argue that the remaining discrepancies are very likely of systematic nature and result from undetermined offsets in flight (Rollins et al., 2014). Referring to the accuracy required to address the questions noted above, it seems that significant progress has been made in recent years. However, the current measurement accuracy still limits our ability to appropriately assess questions regarding, for instance, stratospheric water vapor trends.
The aim of this study is to provide another step towards a better understanding of the accuracy of airborne water vapor measurements. We present a comprehensive intercomparison of the primary airborne state-of-the-art hygrometers operated by the German research community. This unique data set is used to assess the performance of the individual instruments and to provide a solid basis for comparison to the Integrated Forecast System (IFS) of the European Centre for Medium-Range Weather Forecasts (ECMWF). Section 2 briefly describes the ML-CIRRUS campaign during which five independent in situ hygrometers were operated simultaneously. Section 3 provides a summary of the different instruments. The methodology of the intercomparison is described in Sect. 4, while the intercomparison itself is discussed in Sect. 5. In addition, this section also includes a comparison of relative humidity inside of cirrus clouds as well as an intercomparison of in situ measurements with ECMWF IFS model data.
The ML-CIRRUS campaign with the DLR (German Aerospace Center) research aircraft HALO (High Altitude and LOng Range Research Aircraft) took place in March and April 2014 with the aircraft based in Oberpfaffenhofen, Germany. A detailed summary of the scientific goals, the flight strategy and the instrumentation is given in Voigt et al. (2017). During the campaign period, HALO performed 16 research flights with 88 flight hours in total. The flights were designed for a comprehensive characterization of midlatitude cirrus and contrail cirrus using in situ as well as remote-sensing instruments. ML-CIRRUS aimed for a better understanding of cirrus cloud formation in different meteorological conditions (Krämer et al., 2016; Luebke et al., 2016; Wernli et al., 2016; Urbanek et al., 2017) to improve our estimation of the radiative impact of cirrus (Krisna et al., 2018) as well as for air traffic impacts on high cloud cover (Schumann et al., 2017; Grewe et al., 2017). Therefore, the flight plans were mainly designed to obtain a maximum number of flight hours either within cirrus clouds for in situ measurements or approximately 1 km above cirrus for lidar and dropsonde measurements. The implications of the flight strategy on the water vapor intercomparison are discussed in Sect. 4.1. Looking for cirrus cloud life cycle under different meteorological conditions, the flights covered almost the entire region of central Europe from the northern British coast down to Portugal (Fig. 1).
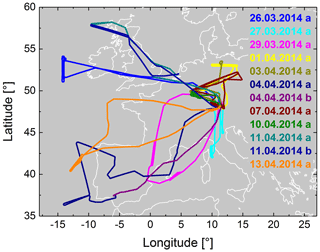
Figure 1Flight tracks of 12 research flights during the ML-CIRRUS campaign in March/April 2014 used for this study. Latitudes between 36 and 57∘ N were covered mainly over central and western Europe.
To achieve the scientific goals of the mission, the HALO payload for ML-CIRRUS comprised instruments to measure cloud particles, aerosols, trace gases and dynamic parameters. The aircraft cabin was equipped with several novel in situ instruments for trace gases and aerosols, dropsondes and a differential absorption lidar (DIAL) system for water vapor and cloud measurements. Furthermore, cloud particles and aerosols were measured in situ using a set of nine wing probes. Since this paper focusses on the intercomparison of the in situ water vapor measurements during ML-CIRRUS, only those instruments will be described here in detail. A full list of instruments, their descriptions and references can be found in Voigt et al. (2017).
The HALO payload for ML-CIRRUS included five different water vapor instruments, which provides the opportunity to compare different measurement methods and a comparison of both gas phase and total water measurements. In particular, three completely independent measurement principles for water vapor were used: mass spectrometry (AIMS-H2O), Lyman-α photofragment fluorescence spectroscopy (FISH) and tunable diode laser absorption spectroscopy (SHARC, HAI and WARAN). While AIMS-H2O and SHARC measured gas phase water vapor via a backward-facing inlet, FISH, HAI and WARAN measured total water (gas phase + evaporated cloud particles) using forward-facing inlets. A summary of key parameters for each instrument is given in Table 1.
3.1 AIMS-H2O
The Atmospheric Ionization Mass Spectrometer for water vapor (AIMS) is a linear quadrupole mass spectrometer designed to measure low water vapor mixing ratios typical for the upper troposphere and lower stratosphere (Kaufmann et al., 2016; Thornberry et al., 2013) and, in a different configuration, HCl, HNO3 and SO2 (Jurkat et al., 2016). The instrument samples gas phase water vapor through a backward-facing heated inlet. After passing a pressure regulation valve, sample air is directly ionized in an electrical discharge ion source. Inside the ion source multiple ion–molecule reactions form ion clusters with n = 0…2. The abundance of these ion clusters is then measured by the mass spectrometer and used to quantify the original water vapor molar mixing ratio in the ambient air. In order to accurately link the ion count rate with the H2O mixing ratio, the instrument is calibrated in flight by regularly adding a water vapor standard generated by the catalytic reaction of hydrogen and oxygen to form H2O on a heated Pt surface (Rollins et al., 2011). AIMS operates at a measurement range between 1 and 500 ppm with an overall accuracy of 7 % to 15 %, mainly depending on the actual water vapor concentration (Kaufmann et al., 2016). During ML-CIRRUS ambient air was sampled through 8.5 mm ID Synflex tubing, and a bypass flow was used to reduce the residence time of air in the inlet line to below 0.2 s. This results in a real measurement frequency of ∼ 4 Hz, corresponding to around 50 m horizontal resolution. In order to achieve the best possible accuracy of the instrument, it was calibrated once or twice during each research flight. The stability of the calibration standard was guaranteed by six ground reference measurements against a MBW 373LX dew point mirror during the campaign period.
3.2 FISH
FISH (Fast In situ Stratospheric Hygrometer) is a closed-cell Lyman-α photofragment fluorescence hygrometer which has been operated on various research aircraft for more than 20 years (Meyer et al., 2015; Schiller et al., 2009). The operating principle of the instrument is described in detail by Zöger et al. (1999). It uses the Lyman-α radiation of an UV lamp at 121.6 nm to dissociate water molecules into single H atoms and excited-state OH molecules. Returning to the ground state, the OH molecules emit radiation at a wavelength between 285 and 330 nm. The intensity of this radiation is proportional to the water vapor molar mixing ratio in the measurement cell and is quantified using a photomultiplier tube. FISH is calibrated regularly at ground level to relate the measured signal to the water vapor mixing ratio using a MBW DP30 dew point mirror as a reference instrument. A detailed description of the calibration procedure can be found in Meyer et al. (2015). FISH is able to measure water vapor mixing ratios in a range from 1 to 1000 ppm. The overall uncertainty during ML-CIRRUS was determined to be 6 % relative and ±0.4 ppm absolute offset uncertainty. FISH was connected to a forward-facing inlet to sample total water. The pressure difference between inlet (static + dynamic) and gas exhaust (only static) ensures a flow rate > 10 standard L min−1 and thus allows for fast measurements in UTLS and cirrus conditions.
3.3 SHARC
SHARC (Sophisticated Hygrometer for Atmospheric ResearCh) is a tunable diode laser (TDL) hygrometer developed at DLR Flight Experiments. It is a closed-cell hygrometer which uses the absorption line of water vapor at 1.37 µm. To cover a wide humidity range, SHARC uses a dual-path Herriott type cell with a single-pass absorption length of approximately 0.17 m and a multi-pass absorption length of approximately 8 m. The cell is completely fiber-coupled to minimize parasitic absorption outside the measurement volume and has a very compact volume of 83 cm3. The measurement range is from 10 to 50 000 ppm, constrained by the detection limit of the absorption signal at low water vapor mixing ratios. The overall uncertainty is 5 % relative and ±1 ppm absolute offset uncertainty. SHARC was operated with a 6.35 mm backward-facing stainless-steel inlet during ML-CIRRUS sampling gas phase H2O with a total flow of 15 standard L min−1 at ground level, decreasing to 1.5 standard L min−1 at the highest flight levels. The real-time data reduction uses a multi-line Voigt fit at 5 Hz to calculate the water vapor mixing ratio. For the intercomparison, the data were averaged to 1 Hz. The instrument was calibrated on the ground against a MBW 373LX dew point mirror.
3.4 HAI
HAI (Hygrometer for Atmospheric Investigations) is a four-channel TDL hygrometer which uses two different absorption wavelengths (1.37 and 2.6 µm) in both closed- and open-cell geometries (Buchholz et al., 2017). HAI uses a complete physical model in combination with spectral water absorption line parameters mostly measured at the Physikalisch-Technische Bundesanstalt Braunschweig (PTB) (Pogány et al., 2015) and monitors pressure, temperature and absorption path length in order to calculate the water vapor concentration for a given absorption spectrum without prior calibration. The accuracy of this approach was verified recently by a side-by-side comparison (Buchholz et al., 2014) of a previous PTB laser absorption spectrometer with the German national primary humidity standard. HAI has 1.5 m optical path length for the closed cell and 4.2 m for the open path. For this work, we use data from the 1.37 µm closed-cell channel of HAI in the range of 20 to 40 000 ppm since only that channel provided data within the required uncertainty margin during ML-CIRRUS. The overall uncertainty for this channel is 4.3 % relative and ±3 ppm absolute offset uncertainty. The closed cell was connected to a 12.7 mm forward-facing stainless-steel inlet and was actively pumped. The effective time resolution of the instrument is 0.7 s, corresponding to a spatial resolution at flight altitude of around 150 m.
3.5 WARAN
The WARAN (WAter vapoR ANalyzer) instrument consists of a commercial WVSS-II (SpectraSensors Inc., USA) tunable diode laser instrument in combination with a custom inlet and an additional pump for the flow through the measurement cell (Kaufmann et al., 2014; Groß et al., 2014). While the instrument was operated on other campaigns parallel to a frost point hygrometer (Heller et al., 2017), during ML-CIRRUS the WARAN was integrated in the AIMS rack and connected to a forward-facing inlet to sample total water. The inlet pylon was the same as used for AIMS-H2O. As for the other instruments operating with a forward-facing inlet, only cloud-free measurement sequences are used for the intercomparison. The instrument was calibrated on the ground after the ML-CIRRUS campaign using a MBW 373LX dew point mirror as a reference. Due to the high detection limit of the instrument (> 50 ppm, stated by the manufacturer), the intercomparison of this instrument is limited to tropospheric conditions. During ML-CIRRUS the WARAN was mainly used to detect cloud water. Due to the enhancement of ice particles in the inlet by a factor between 20 and 35, measured total-water mixing ratios are relatively high (Afchine et al., 2018). Hence the instrument detection limit allows for cloud water quantification for most clouds except for very thin cirrus.
3.6 Additional instrumentation
For data evaluation with respect to relative humidity, cloud detection and model intercomparison, we use additional parameters measured on board HALO during ML-CIRRUS. Static pressure and static temperature are measured by the Basis HALO Measurement and Sensor System (BAHAMAS; Krautstrunk and Giez, 2012; Giez et al., 2017). The accuracy of the pressure sensor is 0.3 hPa; the accuracy of the static temperature measurement is 0.5 K. The SHARC hygrometer (see Sect. 3.3) is also part of BAHAMAS. Cloud detection was done using data from the Cloud and Aerosol Spectrometer with Detection of Polarization (CAS-DPOL), which was mounted under the wing of HALO (Baumgardner et al., 2001; Voigt et al., 2017). The cloud probe measures particles in a size range between 0.5 and 50 µm and is thus sensitive to natural cirrus as well as contrail ice particles.
Similar to previous approaches (e.g., Rollins et al., 2014; Fahey et al., 2014; Meyer et al., 2015) we use the data set from the entire ML-CIRRUS campaign in order to achieve good statistics. This section describes the framework of the intercomparison and the methodology of the data evaluation including the determination of a water vapor reference value.
4.1 Flight strategy
A discussion of the flight strategy during ML-CIRRUS is important since the campaign did not aim for a statistically uniform sampling in terms of water vapor but rather the investigation of cirrus clouds. The flight patterns typically consist of three components:
-
sampling inside cirrus clouds in order to obtain in situ information on particle distribution and their interaction with trace gases and aerosols,
-
remote-sensing segments of cirrus clouds by lidar and radiation measurements where HALO flew ∼ 1 km above the cirrus,
-
transferring flight segments to approach specific weather systems like warm conveyor belts or mountain lee wave regions over western Europe (dark blue and magenta flight tracks in Fig. 1).
In total, we have around 160 000 1 Hz data points in the UTLS with H2O mixing ratios between 3 and 1000 ppm. Of those data points, approximately 22 % are in stratospheric conditions (θ > 350 K), and 33 % are in-cloud measurements.
The dedicated search for cirrus conditions leads to a higher detection frequency of both in-cirrus and above-cirrus sampling relative to their natural occurrence. Since we expect the mode value of the RHi distribution to be close to 100 % inside cirrus (e.g., Ovarlez et al., 2002; Jensen et al., 2017b), this allows for an independent check of the absolute values of the gas phase water vapor measurements. However, extensive in situ sampling in cirrus limits the data for intercomparison of total and gas phase instruments. The remote-sensing legs and the transfer segments provide a comprehensive water vapor data set within the lower stratosphere. The lidar requires a certain vertical distance from the cirrus upper edge; hence most of the stratospheric data were sampled roughly 1 km above that level. Directly above cirrus level fewer data points are sampled. During the transfer segments, flight altitude and horizontal position of the aircraft are independent of meteorological conditions; however, due to the typical high flight altitude of HALO, most of these data points are within the lower stratosphere.
Overall, the ML-CIRRUS flight strategy shifts the sampling of water vapor compared to unbiased sampling of the UTLS in a way that there is a higher detection frequency of humid upper-tropospheric air within cirrus clouds, higher detection frequency of stratospheric measurements at a distance of around 1 to 1.5 km to the tropopause and only a small detection frequency of data in dry tropospheric conditions and directly above the tropopause. However, the measurement strategy should only affect the amount of data in certain water vapor ranges and not the performance of each instrument within its specification.
4.2 Data processing and filtering
In order to construct a consistent data set from all five water vapor instruments on board HALO, the specific time resolutions and response characteristics are considered for each instrument. The goal is to retain as much information as possible while minimizing data-processing-related artifacts. Since all instruments reported data either with a non-uniform frequency or in 1 Hz intervals, the latter was used to unify the data. For AIMS, the 1 Hz data are created by averaging over three data points. Data from FISH are on a 1 Hz integer time basis. For SHARC and HAI, the 1 Hz resolution data are interpolated onto integer values. The only instrument with a lower time resolution than 1 Hz is the WARAN with ∼ 0.4 Hz. Since it is not useful to interpolate this data set onto a 1 Hz interval, each measured value is assigned to the closest integer time value. This processing allows comparison of the H2O measurements directly without imposing any substantial interpolation artifacts in the measured values which could affect the interpretation of the intercomparison.
Since three instruments (FISH, HAI and WARAN) measured total water, cloud sequences were filtered out for the comparison of gas phase H2O. The cloud filtering was done in a two-step process using both the total water measurements themselves and cloud probe particle measurements by the CAS-DPOL. To make sure that in-cloud data are definitely filtered out, all data with total water concentrations above saturation are flagged as “in-cloud”. However, this implies that supersaturated cloud-free conditions are left out as well. As a quality check for the filtering procedure, particle concentrations measured by the CAS-DPOL are used to double-check the cloud mask. In this step, very few additional data points are rejected, which might be due to very thin sublimating clouds or the different positions of cloud probe under the wing and water vapor inlets at the top fuselage.
Further data filtering was applied manually in order to clear data that suffer from obvious sampling artifacts. Concerning AIMS, the pressure regulation of the instrument (Kaufmann et al., 2016) during ML-CIRRUS was not fast enough to compensate for the pressure drop during the fast first ascent on each flight. For this reason, H2O data in that region are not reliable and not included in the archived data set. Furthermore, there are a few ascent and descent sequences where one or more instruments showed a significant time lag of a couple of seconds compared to the other instruments. The causes of these lags and their intermittent occurrence are not clear, and the respective sequences are filtered out.
4.3 Reference value
The determination of a reference value for the intercomparison is guided by various considerations. One possibility is the agreement on a common reference instrument. The airborne intercomparison during MACPEX (Rollins et al., 2014), for example, used the Harvard Lyman-α as a single instrument reference. However this approach is complicated for the instrument combination deployed during ML-CIRRUS since there was no instrument on HALO which measured gas phase H2O and simultaneously covered the complete range of mixing ratios. For that reason, we follow the approach of the AquaVIT campaign described in Fahey et al. (2014), where the mean value of a set of instruments was used as a reference. This allows for a combined intercomparison of data in the lower stratosphere (AIMS, FISH) and in the upper troposphere in cirrus clouds (AIMS, SHARC) and clear sky (AIMS, FISH, SHARC, HAI). We further compare the middle troposphere at higher H2O mixing ratios (SHARC, HAI, WARAN). The reference value for each 1 s step is calculated as the mean of AIMS, FISH, SHARC and HAI data points with the condition that at least two instruments provided valid data for a single time step. For the lower stratosphere, the reference is the mean value of AIMS and FISH measurements. For the troposphere, generally all four instruments are used for the calculation of the reference except for cloud sequences and depending on data availability. Data from the WARAN are not included in the reference calculation since their uncertainty is significantly higher.
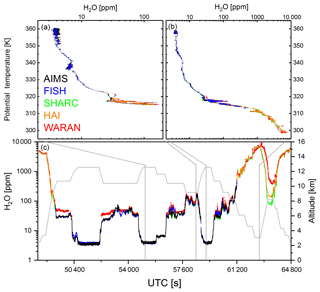
Figure 2Water vapor molar mixing ratio measurements for the research flight on 3 April 2014. AIMS (black) and SHARC (green) measured in situ gas phase H2O, while FISH (blue), HAI (orange) and WARAN (red) measured total water. Panels (a) and (b) are profiles of H2O in situ measurements plotted against potential temperature, showing the descent between 54 904 and 58 522 s and the descent between 59 139 and 61 398 s, respectively. Panel (c) is the time series of the complete flight including the HALO flight altitude in gray.
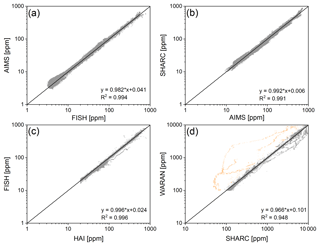
Figure 3Scatterplots of data from the five in situ water vapor instruments on HALO during ML-CIRRUS. (a) Clear-sky measurements of AIMS and FISH covering the stratosphere and upper troposphere, (b) AIMS and SHARC measuring gas phase H2O. This plot thus includes in-cloud gas phase H2O data. (c) HAI vs. FISH for clear-sky upper-tropospheric mixing ratios. (d) WARAN vs. SHARC data extending up to 10 000 ppm with a lower cutoff of the WARAN at 100 ppm. The strong wet bias of the WARAN that occasionally occurs during the first ascent of the plane is marked orange. These data points are left out for the further intercomparison.
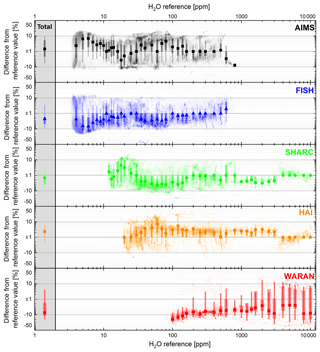
Figure 4Relative difference of the measurements of AIMS, FISH, SHARC, HAI and WARAN from the mean H2O molar mixing ratio value which is used as a reference (details see text). The small dots are the single measurement points (1 Hz values). The big squares, triangle and circle are mean values of the relative difference for specific bins of H2O mixing ratio. The broad bars represent the 25th–75th percentile, while the narrow bars stand for the 10th–90th percentile within the bins. All points with a deviation between −1 % and +1 % fall on the ±1 line. Values in the gray box on the left-hand side represent the overall mean values for the different instruments.
The basis for the intercomparison of H2O data during ML-CIRRUS is time series from each instrument, an example sequence of which is shown in Fig. 2c for the flight on 3 April 2014. For all total-water instruments, only cloud-free data are used for the intercomparison. This flight aimed for in situ and remote measurements of thin cirrus over Germany which were potentially influenced by Saharan dust (Weger et al., 2018). Flight altitude and water vapor mixing ratios in Fig. 2 show the alternation of tropospheric in situ legs (H2O ∼ 30…120 ppm) and lidar legs in the stratosphere (H2O ∼ 5 ppm). Except for the WARAN, which seems to measure too high at the beginning of the flight, all instruments agree reasonably well in both upper troposphere and lower stratosphere. Figure 2a shows a profile for the upper troposphere and lower stratosphere using data from the second descent (indicated by dotted lines in Fig. 2c). The instruments follow the same structures in both regions with a much higher variation in H2O mixing ratios in the upper troposphere. The agreement also holds for the second profile down to 3 km altitude (Fig. 2b); however mixing ratios there are too high to be measured by AIMS and FISH. The short ascent to 8 km after the profile shows a significant deviation between SHARC, HAI and WARAN. Both total-water instruments (HAI and WARAN) measure higher values than the SHARC, which is most likely due to wet contamination of their measurement cells when encountering liquid clouds during the descent. Sequences with such contamination are identified for the entire data set and filtered out for the intercomparison (less than 1 % of the data).
5.1 Correlation of single instruments
To investigate the overall performance of the different measurement systems, 12 ML-CIRRUS flights were combined similar to the one shown in Fig. 2. This complete data set is used to produce the scatterplots in Fig. 3, where selections of four combinations of instrument pairs are displayed. The scatterplot of AIMS and FISH in Fig. 3a shows a very close correlation from below 4 ppm up to ∼ 600 ppm corresponding, to the upper limit of AIMS. For stratospheric mixing ratios below 10 ppm the correlation broadens, with AIMS exhibiting a tendency to higher humidity values and FISH to lower humidity values. Figure 3b shows the correlation between AIMS and SHARC, the two instruments measuring solely gas phase H2O, and is thus the only correlation plot where in-cloud data are displayed together with clear-sky data. Consistent with Fig. 3a this correlation is very narrow, slightly widening only for the high concentrations at the upper AIMS measurement limit. A similar narrow correlation is found for HAI vs. FISH (Fig. 3c) from 20 ppm up to 1000 ppm. For all three scatterplots (Fig. 3a–c) correlation coefficients are higher than 0.99. In contrast to panels a–c, Fig. 3d spans the range to higher humidity from 10 to 10 000 ppm, displaying data from WARAN and SHARC. Between 100 and 300 ppm, the WARAN shows a slight dry bias, which disappears for higher mixing ratios. Compared to the other instruments, the WARAN exhibits a significantly larger scatter, with complete sequences lying well above the one-to-one line. These sequences are associated with initial ascent during the flights, where the WARAN occasionally shows a wet bias (data points marked orange in Fig. 3d). These data points are omitted from the intercomparison. The dry bias and larger scatter are also reflected in the correlation coefficient, which is 0.94 for Fig. 3d. The comparison with WARAN measurements during other campaigns suggests that the deviations are likely caused by systematic offsets in the original calibration of the instrument. Thus, the analysis is probably only valid for this specific instrument during the ML-CIRRUS campaign. Overall, the correlation plots indicate a good agreement for AIMS, FISH, SHARC and HAI throughout the entire campaign.
5.2 Deviation with respect to reference value
In order to quantify the performance of each instrument, the deviations of each instrument from the reference value (see Sect. 4.3) are displayed in Fig. 4, similar to previous studies (Fahey et al., 2014; Rollins et al., 2014). On the x axis, the H2O reference value is shown. The y axis denotes the relative difference for each instrument from that reference value. The small dots are the measured 1 Hz values; the big symbols are mean values for logarithmic bins in H2O. Additionally, the broad bars represent the interquartile range in each bin, and the narrow bars are the 10th–90th percentiles. In the gray box on the left, mean values and respective percentiles for the entire data set of each instrument are shown. As shown in Table 2, the mean deviations of AIMS, FISH, SHARC and HAI are below 2.5 %, indicating that there is no consistent systematic bias when averaging over the entire data set. The situation looks different for the WARAN instrument, where the dry bias at low H2O mixing ratios can be clearly seen in the H2O-resolved deviation but not in the overall mean (Fig. 4e).
Table 2Statistic summary of the five instruments including number of points entering the comparison, mean deviation and spread of the data.
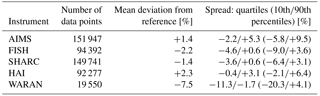
When looking at Fig. 4a and b in more detail, the agreement between AIMS and FISH in the lower stratosphere below 10 ppm seems good with single values of both instruments mostly falling within ±15 %. Since these are the only two instruments measuring in the low ppm range, the plot is a direct comparison of both instruments. In fact, there is a systematic difference between both instruments for humidity conditions between 4 and 10 ppm. In that region the mean values of the instruments differ by 4 % to 16 %, with AIMS measuring higher and FISH measuring lower mixing ratios. Interestingly, the difference between the instruments for the driest conditions (3.5 to 4.5 ppm) is smaller than for the next several bins (2.4 % vs. 6.5 %). However, the spread in the data is too large to judge if this difference is significant. Examining all of the time series plots from the campaign (not shown) reveals that there are some distinct stratospheric legs where AIMS is up to 1 ppm higher than FISH (corresponding to a relative deviation of ∼ 20 %). The reason for this deviation is not completely clear; one explanation could be a contamination of the AIMS vacuum system. However, it is unlikely that this is the only cause since the behavior changes occasionally from one leg to another within the same flight. For upper-tropospheric measurements (where more than the two instruments contribute to the reference value), the agreement of the mean values with the reference is better than 5 %. The same holds for the SHARC measurements (Fig. 4c) throughout its complete range with a slight tendency to lower mixing ratios (3 % to 4 %) compared to the reference between 30 and 200 ppm. HAI data (Fig. 4d) also fall in the same range of variation, with mean values being consistently slightly higher by about 3 % than the reference value in the range between 30 and 2000 ppm. For both SHARC and HAI, the single measurement scatter is within ±20 % with respect to the reference. Considering the fact that all four instruments contribute to the reference value, one can state that FISH and SHARC tend to consistently report slightly lower mixing ratios than AIMS and HAI. The WARAN measurements (Fig. 4e) fall off compared to the other four instruments, exhibiting a significant low bias for mixing ratios below 300 ppm. However, these data are still within the uncertainty specifications of the instrument (see Table 1).
5.3 Comparison of relative humidity in clouds
The comparison of relative humidity measurements in clouds can be considered as a further measure for the quality of the H2O measurements which is independent from any kind of reference value. In contrast to measurements in liquid clouds, much stronger deviations of RHi from saturation are possible in ice clouds due to their higher thermodynamic inertia. RHi inside cirrus clouds can be very variable due to advection as well as small-scale turbulence inside the cloud (e.g., Gettelman et al., 2006; Petzold et al., 2017). However, if the measurements include a sufficiently even sampling of meteorological conditions, a distribution of RHi with a mode value close to 100 % would be expected. In order to calculate RHi from the measured H2O mixing ratios, we have used the static temperature and static pressure measurements on board HALO to calculate water vapor partial pressure and saturation pressure. The saturation pressure over ice is calculated using Eq. (7) from Murphy and Koop (2005).
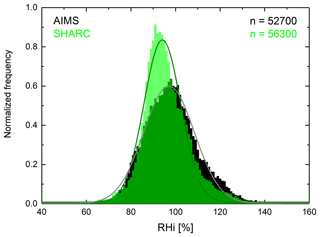
Figure 5PDFs of relative humidity with respect to ice calculated from AIMS (black) and SHARC (green) data and the static air temperature measurement on HALO inside cirrus clouds for the entire campaign. Dark green indicates overlap regions. The cloud flag is the same used for filtering the total water measurements. The center of the respective distribution is 94 % for SHARC and 97 % for AIMS.
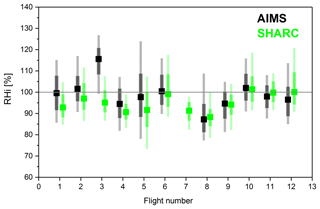
Figure 6Mean values for RHi inside cirrus measured by AIMS (black) and SHARC (green) for each ML-CIRRUS flight. Broad bars denote the interquartile range; narrow bars denote the 10th–90th-percentile range.
Here, we compare in-cloud measurements of RHi for the two water vapor instruments with backward-facing inlets, AIMS and SHARC (see also Fig. 3b). In total, more than 50 000 in-cloud data points were acquired during ML-CIRRUS, with numbers varying between 2000 and 11 000 for individual flights. The frequency distribution of RHi for the entire data set of the ML-CIRRUS campaign is shown in Fig. 5. Data from both instruments are almost normally distributed, with mean values slightly below ice saturation. Fitting a normal distribution to both data sets, they peak at RHi = 97 % for AIMS (52 700 data points) and 94 % for SHARC (56 300 data points). The full width at half maximum of the distribution is 26.7 % for AIMS and 19.4 % for SHARC. Both distributions are slightly asymmetric with a tail towards higher supersaturation which is more pronounced in the SHARC measurements. This agrees with results from Ovarlez et al. (2002), who find similar asymmetric distributions for temperatures below −40 ∘C.
Considering the instrumental uncertainties, both distributions appear reasonable. However, the question remains whether the slight shift of the center of the RHi distribution relative to 100 % is caused by systematic instrument biases (H2O and temperature), inlet issues (e.g., sucking in and evaporating ice particles) or a sampling bias in the flight strategy. If the sampling were biased toward either forming/growing cirrus or evaporating cirrus, one would expect a positive or negative RHi bias with respect to saturation, respectively. During ML-CIRRUS, individual flights typically targeted specific meteorological conditions, e.g., the updraft region of warm conveyor belts or mountain wave cirrus. Hence, a sampling bias for individual flights is very likely. In order to investigate that, Figure 6 shows the mean values for the in-cloud RHi distributions of AIMS and SHARC including interquartile ranges and 10th–90th-percentile ranges for each flight. For flight nos. 1–5, AIMS and SHARC deviate by 4 % to 8 % with one exception on flight no. 3, where the deviation is around 20 %. These data originate from a two-step profile through cirrus clouds with high updraft velocities over the Balearic Islands. During that flight, there is a systematic difference between AIMS and SHARC which is most pronounced during the two cirrus transects (difference of around 20 % compared to 7 % to 10 % during the rest of the flight). From the high updraft velocity, one would rather expect supersaturation inside the cirrus. For flight no. 7, there is not enough in-cloud data from AIMS to produce a reasonable RHi distribution. For flight nos. 8, 9 and 10, the agreement of both instruments is almost perfect, while for the last two flights AIMS tended to measure slightly lower RHi values than SHARC but with a difference of less than 3 %. The spread of the RHi measurements is similar for both instruments (AIMS interquartile range: 10 % to 20 %; SHARC interquartile range: 8 % to 17 %), with the lower values for SHARC arising from a slightly better precision.
The observed trend could be an indication of instrumental drift over the campaign period; however we cannot state which instrument is subject to a drift. Flights with mean super- or subsaturation are almost evenly distributed for AIMS, while SHARC measurements are slightly sub-saturated, especially during the first half of the campaign. From the present data, we do not have clear evidence for an overall sampling bias during the campaign. A possible bias affecting RHi derived from both instruments could be a bias in the static temperature measurement on board HALO since we use the same temperature information for both instruments. However, the median and mean values of the distributions deviate by less than 6 % from saturation for most of the flights, indicating that temperature is not significantly off.
5.4 Comparison to the ECMWF numerical weather prediction model
The extensive ML-CIRRUS in situ data set of upper-tropospheric and lower-stratospheric humidity further enables an evaluation of the accuracy of UTLS humidity in the ECMWF (European Centre for Medium-Range Weather Forecasts) numerical weather prediction (NWP) model. A correct representation of water vapor is crucial for weather and climate prediction via various pathways. Besides the troposphere, where water vapor is obviously important for cloud formation and precipitation, the stratospheric mean state also influences the predictability in the troposphere (Douville, 2009). Moreover, biases in modeled stratospheric water vapor can induce a frequently observed cold bias in the extratropics (e.g., Boer et al., 1992; Stenke et al., 2008; Chen and Rasch, 2012).
The model data used for analysis of ML-CIRRUS are provided by the Integrated Forecasting System (IFS) of the ECMWF (IFS Version 40r1). For analysis, we use a combination of analysis data with hourly forecasts starting every 12 h from the analysis at 00:00 and 12:00 UTC. The data set covers the region of 20–70∘ N, 60∘ W–20∘ E. The model includes 137 vertical model levels, with pressure intervals of 18 hPa near 7 km altitude and 7 hPa near 15 km height. For typical flight altitudes near 11.5 km (200 hPa) the vertical resolution is around 300 m (10 hPa). The horizontal resolution of the data used is 0.5∘. Higher horizontal resolution would be available from IFS but would not provide more information due to the hourly time resolution. The data are interpolated linearly to the measurement position for a given HALO position (latitude and longitude) above the WGS84 reference ellipsoid. Vertical interpolation is performed in the logarithm of pressure fields (which varies more smoothly than pressure) based on the static pressure measured by HALO-BAHAMAS (Schumann et al., 2015). The output frequency is 0.1 Hz along the flight track, resulting in a distance of roughly 2 km between adjacent data points. The reference H2O mixing ratio is averaged accordingly over 10 s intervals. Except for the time resolution, the methodology of the intercomparison of model data and measurements is the same as used in Sect. 5.2, simply treating the interpolated model data as a “new” instrument. In Fig. 7, the relative deviation of the ECMWF data is plotted against the measured reference H2O value (same method as used for Fig. 4). The small dots represent the interpolated model data point for each valid reference value (see Sect. 4.3). Similarly to in Fig. 4, the black triangles denote bin-wise mean values of the relative difference, while the gray bars and whiskers represent the interquartile range and the 10th–90th-percentile range, respectively. In order to get an idea if the sampled air mass is of stratospheric or tropospheric origin, the individual data points are color-coded with potential temperature averaged from the HALO onboard measurements.
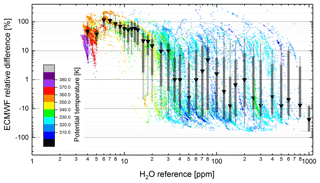
Figure 7Relative difference of H2O mixing ratio between ECMWF analysis and measurement reference for all ML-CIRRUS flights. Model data are interpolated in space and time on each flight track. The reference value on the x axis is the same as in Fig. 4. As in Fig. 4, the triangles, broad bars and narrow bars represent the mean values, 25th–75th percentiles and 10th–90th percentiles, respectively. Single data points are color-coded with potential temperature.
As can be seen in Fig. 7, the comparison between the model and measurements is different in two distinct humidity regimes. At the higher tropospheric mixing ratios above 30 ppm, there is a remarkably good agreement between mean bin values, and the interquartile range is mostly within ±10 %. The single values exhibit a larger scatter, resulting in 10th and 90th percentiles of around −30 % and +20 %, respectively. This could be expected considering the high natural variability in water vapor compared to the model resolution. The distribution of mean relative differences suggests a slight bias in that region, with ECMWF being slightly lower. With a mean value near 3 %, this bias is very small when considering the overall scatter of the data and the interpolation of the model onto the flight path. The interpolation procedure is also the reason for the single data points resembling the shape of a mirrored S. This behavior results from comparing the measurement signal with high spatial variability with the rather smooth model data. When using a logarithmic y scale and the more variable measured mixing ratio as a reference on the x axis, it results in an S-like shape in the individual data points.
The character of the intercomparison differs for lower mixing ratios below 30 ppm found in the tropopause region and the lower stratosphere. In that region, the model significantly overestimates the humidity. The biggest differences between measurement and model occur at mixing ratios between 5 and 8 ppm, typical values for the region directly above the tropopause. The maximum difference is found in the bin between 5.5 and 6.5 ppm, where the mean difference is 115 % (statistics from 382 data points). The difference decreases again for mixing ratios below 5 ppm, indicating a better agreement between measurement and model with increasing distance to the tropopause. The mean difference for the driest bin (3.5 to 4.5 ppm with 2383 data points) of 46 % is less than half of the more humid neighboring bins. However, it still is significant and positive, meaning that ECMWF shows a systematic wet bias for the entire probed region in the lower stratosphere in spring.
The maximal differences close to tropopause mixing ratios indicate that the difference between measurement and model is caused by too weak of a humidity gradient at the tropopause, partially explained by the model grid resolution of about 300 m vertically near the tropopause. Here, narrow inversions may form between subsiding dry stratospheric air and upward mixing of humid cold tropospheric air (Birner et al., 2002) which might not be covered by the coarse resolution of a global model. The difference in humidity gradients is directly evident in the humidity profiles. Figure 8 shows one ascent (Fig. 8a) and one descent (Fig. 8b) through the entire tropopause region on 11 April 2014. Consistent with Fig. 7, we observe a good agreement between model and measurement in the troposphere. Directly above the tropopause, the humidity gradient in the model is weaker compared to the measurements for both profiles, resulting in overestimation of water vapor by the model in that region. This feature is independent from the absolute height of the tropopause (∼ 11.8 km in Fig. 8a, ∼ 10.4 km in Fig. 8b), which is well represented in the model when comparing measured and modeled temperature profiles. With increasing vertical distance to the tropopause, measurement and model approach similar values, which is consistent with the overall intercomparison in Fig. 7. The region above the tropopause where we observe a significant difference between measurements and model varies from around 1 km above the tropopause in Fig. 8a to around 3 km in Fig. (8b). Thus, the weaker gradient is certainly no artifact of the vertical interpolation of the model.
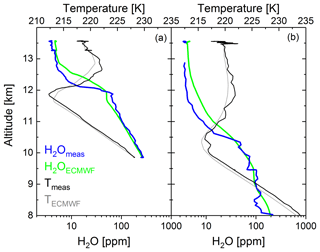
Figure 8Profiles of water vapor mixing ratio and temperature from in-situ measurements and the ECMWF model. The blue line is the water vapor reference value from in-situ observations; the green line is the interpolated ECMWF model data. Data shown here originate from one ascent (a) and one descent (b) through the tropopause on 11 April 2014 (flight #11). The water vapor profiles agree well in the upper troposphere; in the lower stratosphere we observe a stronger gradient in the measurements compared to the model. The vertical position of the thermal tropopause (black: measured by HALO; gray: ECMWF) is well represented in the model.
Table 3Relative difference between ECMWF IFS data and measurements for different potential temperatures.
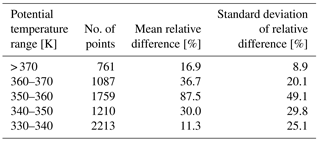
Our results support previous studies (e.g., Kunz et al., 2014; Dyroff et al., 2015). The latter study shows a good agreement between measurement and model for vertical distances to the tropopause of 6 km and higher and model wet bias between 2 and 6 km above the tropopause for the extratropics. During ML-CIRRUS, the maximum distance above the tropopause was 3.5 km; hence the measurements are probably not stratospheric enough to leave the wet-bias region. However, the trend towards better agreement deeper in the stratosphere can be seen in the color coding in Fig. 7 as well as in Table 3 where mean difference are binned by potential temperature rather than the mixing ratio. It turns out that the wet bias strongly peaks at potential temperatures between 350 and 360 K (mean difference of 88 %), whereas it decreases from there with increasing altitude in the stratosphere (higher potential temperature) as well as into the troposphere (lower potential temperature). Both single profiles and the overall intercomparison allow the observed differences in lower-stratospheric humidity to be attributed to the too-weak humidity gradient of ECMWF above the tropopause compared to the observations in European spring conditions.
We intercompare water vapor measurements from different state-of-the-art in situ instruments on board the DLR research aircraft HALO during the midlatitude UTLS field project ML-CIRRUS. It is the first comprehensive intercomparison of all primary airborne hygrometers operated by the German research community including three TDL instruments (HAI, SHARC and WARAN), one mass spectrometer (AIMS) and the established Lyman-α hygrometer FISH. The intercomparison includes a large span of humidity conditions from lower-stratospheric to lower-tropospheric H2O molar mixing ratios, with different instruments covering different parts of the mixing ratio spectrum. This work focusses on the intercomparison of gas phase water vapor measurements, meaning that only clear-sky data are used from instruments measuring total water (HAI, FISH and WARAN). The flight strategy of ML-CIRRUS focused on the investigation of midlatitude cirrus clouds with in situ and remote-sensing (lidar) instrumentation. Hence, the majority of data points originate from the midlatitude upper troposphere and lower stratosphere above Europe and the western Atlantic in spring 2014.
The agreement between the in situ instruments, expressed by the relative difference to a reference value (mean value of at least two instruments), is generally good and consistent with previous intercomparison studies (Rollins et al., 2014). For all instruments except the WARAN, the overall mean deviation from the reference value is below 2.5 %. This is an indication for the successful efforts to improve the accuracy of UTLS H2O measurements during the past decade, motivated by large discrepancies that have been found before (Fahey et al., 2014). Still, systematic discrepancies remain between the instruments in specific regimes which need to be addressed in order to improve our understanding of the humidity budget in the lowermost stratosphere or of cirrus formation under very cold conditions (Gao et al., 2004; Krämer et al., 2009; Jensen et al., 2017a). One major issue is the difference between FISH and AIMS for stratospheric mixing ratios below 10 ppm. The observation that the mass spectrometer AIMS measures systematically higher mixing ratios than FISH is similar to the findings during the MACPEX intercomparison (Rollins et al., 2014). During that campaign, the maximum difference of bin mean values is 13.7 % in the range 5.5–6.5 ppm. Although this difference is still within the combined uncertainty of the instruments, it hampers the detailed investigation of trends in the lower-stratospheric water vapor budget, which are of the same order of magnitude and highly uncertain, even in their sign (e.g., Hegglin et al., 2014; Lossow et al., 2018).
We investigate RHi measurements in cirrus clouds from AIMS and SHARC as an independent metric of the absolute accuracy of the H2O measurements. This is not straightforward, as RHi in cirrus clouds is known to differ significantly from saturation depending on the dynamics of the cloud. Still, considering a sufficiently large database, the data can be used as an independent indicator of the absolute accuracy of the measurements under UTLS conditions. Data from both instruments have a mode value close to ice saturation (less than 10 % difference of mean value for all flights). An overall instrumental or sampling bias seems unlikely since flights with mean super- and subsaturation in clouds are almost evenly distributed. The same holds for a possible bias in the aircraft temperature measurement which would similarly propagate into the RHi distribution. However, we do observe a drift between the in-cloud measurements of the two instruments over the course of the measurement campaign. While AIMS measures higher RHi values than SHARC in the beginning of the campaign, mean RHi values agree much better during the second half of the campaign. When considering the entire data set (including clear-sky data), this drift is not apparent, which makes a change in the performance of one instrument unlikely.
A comparison of the measured H2O mixing ratios with ECMWF IFS data is accomplished using the same methodology as for the instrument intercomparison. The gridded ECMWF data are interpolated in space and time along the flight path of HALO with a resolution of 0.1 Hz. Measurement and model show generally good agreement throughout the upper troposphere with bin-wise mean values of the difference typically within ±10 % (consistent with, e.g., Flentje et al., 2007) with a slight tendency towards a model dry bias which, however, is not statistically significant. Below mixing ratios of 30 ppm, we observe a significant wet bias in the ECMWF model with highest mean deviation from the measurements around 6 ppm or at a potential temperature of 355 K. In that regime, mean deviations are on the order of 100 % with an interquartile range of 70 to 140 %. The large wet bias of the model in the tropopause region is consistent with findings in previous studies (e.g., Kunz et al., 2014; Dyroff et al., 2015). The model wet bias decreases substantially at higher potential temperatures, leading to a mean difference of only 17 % at potential temperatures above 370 K. The fact that the model bias shows a clear maximum at the tropopause indicates that this issue is likely caused by too strong numerical smoothing reducing humidity gradients near the tropopause rather than an overall bias of stratospheric mixing ratios. Kunz et al. (2014) found a similar feature with good agreement between FISH measurements and ECMWF reanalysis data at altitudes higher than 6 km above the tropopause. The issue of too-weak gradients at the tropopause is discussed extensively by, for example, Birner et al. (2002), Gray et al. (2014) and Saffin et al. (2017). In particular, the lower-stratospheric wet bias is very sensitive to the horizontal interpolation of the specific humidity field in the semi-Langrangian IFS model (Diamantakis, 2014), leading to a too high diffusivity, which in turn causes a cold bias at the extratropical tropopause (Stenke et al., 2008). However, it is difficult and cost intensive to address the issue in the model since it would require adjusting core dynamical model processes or increasing the model resolution (Saffin et al., 2017; Pope et al., 2001). Additionally, the model suffers from a lack of assimilated information on lower-stratospheric water vapor since specific humidity data from radiosondes are only assimilated below a certain threshold pressure level (depending on the type of sonde; see Andersson et al., 2007). Given the large model uncertainty in H2O concentrations close to the tropopause, it is difficult, for example, to correctly evaluate the radiative effects of water vapor in that region where the atmosphere is very sensitive to even small changes in H2O (Solomon et al., 2010; Riese et al., 2012).
Despite the limitation to one-dimensional data for the in situ measurements, high-spatial-resolution data as obtained from aircraft can help to point out important small-scale differences which are difficult to assess when comparing model to satellite data due to their limited (especially vertical) resolution (e.g., Lamquin et al., 2009). The intercomparison shows that our approach to comparing in situ data with model data can be particularly useful for investigating model performance around the tropopause. Hence, it could be worthwhile to extend this type of intercomparison to reanalysis data like the new climate reanalysis data set (ERA-5) of the ECMWF or include further NWP models like the Icosahedral Nonhydrostatic (ICON) model from the German Weather Service.
Data are accessible via the HALO database (https://halo-db.pa.op.dlr.de/mission/2) (HALO database, 2018). They can be accessed after signing a data agreement. Operational meteoro-logical analyses are archived in the MARS archive at ECMWF (https://www.ecmwf.int/en/forecasts/documentation-and-support/ changes-ecmwf-model/ifs-documentation, ECMWF, 2018)
SK, CV, RH and TJW performed the AIMS measurements; MK and CR performed the FISH measurements; MZ and AG performed the SHARC measurements; BB and VE performed the HAI measurements; US provided and prepared the ECMWF data; and SK performed the study and wrote the manuscript with help from CV, TT and US. All authors commented on the manuscript.
The authors declare that there is no conflict of interest.
This article is part of the special issue “ML-CIRRUS – the airborne experiment on natural cirrus and contrail cirrus in mid-latitudes with the high-altitude long-range research aircraft HALO (ACP/AMT inter-journal SI)”. It is not associated with a conference.
We thank the DLR flight department and Andreas Minikin for great support
during the campaign and Klaus Gierens for helpful comments on the manuscript.
Support by the Helmholtz Association under contract W2/W3-60 and by the
German Science Foundation within the DFG-SPP HALO 1294 via grant VO1504/4-1
(CV), JU3059/1-1 (TJ), KR 2957/1-1 (MK) and SCHI-872/2-2 (CR) is greatly
acknowledged. The article processing charges for this
open-access
publication were covered by a Research
Centre of the Helmholtz Association.
Edited by: Darrel Baumgardner
Reviewed by: three anonymous
referees
Afchine, A., Rolf, C., Costa, A., Spelten, N., Riese, M., Buchholz, B., Ebert, V., Heller, R., Kaufmann, S., Minikin, A., Voigt, C., Zöger, M., Smith, J., Lawson, P., Lykov, A., Khaykin, S., and Krämer, M.: Ice particle sampling from aircraft – influence of the probing position on the ice water content, Atmos. Meas. Tech., 11, 4015–4031, https://doi.org/10.5194/amt-11-4015-2018, 2018.
Andersson, E., Hólm, E., Bauer, P., Beljaars, A., Kelly, G. A., McNally, A. P., Simmons, A. J., Thépaut, J. N., and Tompkins, A. M.: Analysis and forecast impact of the main humidity observing systems, Q. J. Roy. Meteorol. Soc., 133, 1473–1485, https://doi.org/10.1002/qj.112, 2007.
Baumgardner, D., Jonsson, H., Dawson, W., O'Connor, D., and Newton, R.: The cloud, aerosol and precipitation spectrometer: a new instrument for cloud investigations, Atmos. Res., 59–60, 251–264, https://doi.org/10.1016/S0169-8095(01)00119-3, 2001.
Birner, T., Dörnbrack, A., and Schumann, U.: How sharp is the tropopause at midlatitudes?, Geophys. Res. Lett., 29, 45-41–45-44, https://doi.org/10.1029/2002GL015142, 2002.
Boer, G. J., Arpe, K., Blackburn, M., Déqué, M., Gates, W. L., Hart, T. L., Treut, H. l., Roeckner, E., Sheinin, D. A., Simmonds, I., Smith, R. N. B., Tokioka, T., Wetherald, R. T., and Williamson, D.: Some results from an intercomparison of the climates simulated by 14 atmospheric general circulation models, J. Geophys. Res.-Atmos., 97, 12771–12786, https://doi.org/10.1029/92JD00722, 1992.
Buchholz, B., Böse, N., and Ebert, V.: Absolute validation of a diode laser hygrometer via intercomparison with the German national primary water vapor standard, Appl. Phys. B, 116, 883–899, https://doi.org/10.1007/s00340-014-5775-4, 2014.
Buchholz, B., Afchine, A., Klein, A., Schiller, C., Krämer, M., and Ebert, V.: HAI, a new airborne, absolute, twin dual-channel, multi-phase TDLAS-hygrometer: background, design, setup, and first flight data, Atmos. Meas. Tech., 10, 35–57, https://doi.org/10.5194/amt-10-35-2017, 2017.
Chen, C.-C. and Rasch, P. J.: Climate Simulations with an Isentropic Finite-Volume Dynamical Core, J. Clim., 25, 2843–2861, https://doi.org/10.1175/2011jcli4184.1, 2012.
Dessler, A. E., Zhang, Z., and Yang, P.: Water-vapor climate feedback inferred from climate fluctuations, 2003–2008, Geophys. Res. Lett., 35, L20704, https://doi.org/10.1029/2008GL035333, 2008.
Diamantakis, M.: The semi-Lagrangian technique in atmospheric modelling: current status and future challenges, Seminar on Recent Developments in Numerical Methods for Atmosphere and Ocean Modelling, 2–5 September 2013, Shinfield Park, Reading, 2014.
Douville, H.: Stratospheric polar vortex influence on Northern Hemisphere winter climate variability, Geophys. Res. Lett., 36, L18703, https://doi.org/10.1029/2009GL039334, 2009.
Dyroff, C., Zahn, A., Christner, E., Forbes, R., Tompkins, A. M., and Velthoven, P. F. J. v.: Comparison of ECMWF analysis and forecast humidity data with CARIBIC upper troposphere and lower stratosphere observations, Q. J. Roy. Meteorol. Soc., 141, 833–844, https://doi.org/10.1002/qj.2400, 2015.
ECMWF: CY40R1, available at: https://www.ecmwf.int/en/ forecasts/documentation-and-support/changes-ecmwf-model/ifs-documentation (last access: 26 November 2018), 2018.
Fahey, D. W., Gao, R.-S., Möhler, O., Saathoff, H., Schiller, C., Ebert, V., Krämer, M., Peter, T., Amarouche, N., Avallone, L. M., Bauer, R., Bozóki, Z., Christensen, L. E., Davis, S. M., Durry, G., Dyroff, C., Herman, R. L., Hunsmann, S., Khaykin, S. M., Mackrodt, P., Meyer, J., Smith, J. B., Spelten, N., Troy, R. F., Vömel, H., Wagner, S., and Wienhold, F. G.: The AquaVIT-1 intercomparison of atmospheric water vapor measurement techniques, Atmos. Meas. Tech., 7, 3177–3213, https://doi.org/10.5194/amt-7-3177-2014, 2014.
Flentje, H., Dörnbrack, A., Fix, A., Ehret, G., and H'”olm, E.: Evaluation of ECMWF water vapour fields by airborne differential absorption lidar measurements: a case study between Brazil and Europe, Atmos. Chem. Phys., 7, 5033–5042, https://doi.org/10.5194/acp-7-5033-2007, 2007.
Gao, R. S., Popp, P. J., Fahey, D. W., Marcy, T. P., Herman, R. L., Weinstock, E. M., Baumgardner, D. G., Garrett, T. J., Rosenlof, K. H., Thompson, T. L., Bui, P. T., Ridley, B. A., Wofsy, S. C., Toon, O. B., Tolbert, M. A., Karcher, B., Peter, T., Hudson, P. K., Weinheimer, A. J., and Heymsfield, A. J.: Evidence that nitric acid increases relative humidity in low-temperature cirrus clouds, Science, 303, 516–520, https://doi.org/10.1126/science.1091255, 2004.
Gettelman, A., Fetzer, E. J., Eldering, A., and Irion, F. W.: The Global Distribution of Supersaturation in the Upper Troposphere from the Atmospheric Infrared Sounder, J. Clim., 19, 6089–6103, https://doi.org/10.1175/jcli3955.1, 2006.
Giez, A., Mallaun, C., Zöger, M., Dörnbrack, A., and Schumann, U.: Static Pressure from Aircraft Trailing-Cone Measurements and Numerical Weather-Prediction Analysis, J. Aircraft, 54, 1728–1737, https://doi.org/10.2514/1.C034084, 2017.
Gray, S. L., Dunning, C. M., Methven, J., Masato, G., and Chagnon, J. M.: Systematic model forecast error in Rossby wave structure, Geophys. Res. Lett., 41, 2979–2987, https://doi.org/10.1002/2014GL059282, 2014.
Grewe, V., Dahlmann, K., Flink, J., Frömming, C., Ghosh, R., Gierens, K., Heller, R., Hendricks, J., Jöckel, P., Kaufmann, S., Kölker, K., Linke, F., Luchkova, T., Lührs, B., Van Manen, J., Matthes, S., Minikin, A., Niklaß, M., Plohr, M., Righi, M., Rosanka, S., Schmitt, A., Schumann, U., Terekhov, I., Unterstrasser, S., Vázquez-Navarro, M., Voigt, C., Wicke, K., Yamashita, H., Zahn, A., and Ziereis, H.: Mitigating the Climate Impact from Aviation: Achievements and Results of the DLR WeCare Project, Aerospace, 4, 34, 2017.
Groß, S., Wirth, M., Schäfler, A., Fix, A., Kaufmann, S., and Voigt, C.: Potential of airborne lidar measurements for cirrus cloud studies, Atmos. Meas. Tech., 7, 2745–2755, https://doi.org/10.5194/amt-7-2745-2014, 2014.
Hegglin, M. I., Plummer, D. A., Shepherd, T. G., Scinocca, J. F., Anderson, J., Froidevaux, L., Funke, B., Hurst, D., Rozanov, A., Urban, J., von Clarmann, T., Walker, K. A., Wang, H. J., Tegtmeier, S., and Weigel, K.: Vertical structure of stratospheric water vapour trends derived from merged satellite data, Nat. Geosci., 7, 768–776, https://doi.org/10.1038/ngeo2236, 2014.
HALO database, https://halo-db.pa.op.dlr.de, https://doi.org/10.17616/R39Q0T, 2018.
Heller, R., Voigt, C., Beaton, S., Dörnbrack, A., Giez, A., Kaufmann, S., Mallaun, C., Schlager, H., Wagner, J., Young, K., and Rapp, M.: Mountain waves modulate the water vapor distribution in the UTLS, Atmos. Chem. Phys., 17, 14853–14869, https://doi.org/10.5194/acp-17-14853-2017, 2017.
Jensen, E. J., Smith, J. B., Pfister, L., Pittman, J. V., Weinstock, E. M., Sayres, D. S., Herman, R. L., Troy, R. F., Rosenlof, K., Thompson, T. L., Fridlind, A. M., Hudson, P. K., Cziczo, D. J., Heymsfield, A. J., Schmitt, C., and Wilson, J. C.: Ice supersaturations exceeding 100 % at the cold tropical tropopause: implications for cirrus formation and dehydration, Atmos. Chem. Phys., 5, 851–862, https://doi.org/10.5194/acp-5-851-2005, 2005.
Jensen, E. J., Pfister, L., Jordan, D. E., Bui, T. V., Ueyama, R., Singh, H. B., Thornberry, T. D., Rollins, A. W., Gao, R.-S., Fahey, D. W., Rosenlof, K. H., Elkins, J. W., Diskin, G. S., DiGangi, J. P., Lawson, R. P., Woods, S., Atlas, E. L., Rodriguez, M. A. N., Wofsy, S. C., Pittman, J., Bardeen, C. G., Toon, O. B., Kindel, B. C., Newman, P. A., McGill, M. J., Hlavka, D. L., Lait, L. R., Schoeberl, M. R., Bergman, J. W., Selkirk, H. B., Alexander, M. J., Kim, J.-E., Lim, B. H., Stutz, J., and Pfeilsticker, K.: The NASA Airborne Tropical Tropopause Experiment: High-Altitude Aircraft Measurements in the Tropical Western Pacific, B. Am. Meteor. Soc., 98, 129–143, https://doi.org/10.1175/bams-d-14-00263.1, 2017a.
Jensen, E. J., Thornberry, T. D., Rollins, A. W., Ueyama, R., Pfister, L., Bui, T., Diskin, G. S., DiGangi, J. P., Hintsa, E., Gao, R.-S., Woods, S., Lawson, R. P., and Pittman, J.: Physical processes controlling the spatial distributions of relative humidity in the tropical tropopause layer over the Pacific, J. Geophys. Res.-Atmos., 122, 6094–6107, https://doi.org/10.1002/2017JD026632, 2017b.
Jurkat, T., Kaufmann, S., Voigt, C., Schäuble, D., Jeßberger, P., and Ziereis, H.: The airborne mass spectrometer AIMS – Part 2: Measurements of trace gases with stratospheric or tropospheric origin in the UTLS, Atmos. Meas. Tech., 9, 1907–1923, https://doi.org/10.5194/amt-9-1907-2016, 2016.
Kaufmann, S., Voigt, C., Jeßberger, P., Jurkat, T., Schlager, H., Schwarzenboeck, A., Klingebiel, M., and Thornberry, T.: In situ measurements of ice saturation in young contrails, Geophys. Res. Lett., 41, 702–709, https://doi.org/10.1002/2013GL058276, 2014.
Kaufmann, S., Voigt, C., Jurkat, T., Thornberry, T., Fahey, D. W., Gao, R.-S., Schlage, R., Schäuble, D., and Zöger, M.: The airborne mass spectrometer AIMS – Part 1: AIMS-H2O for UTLS water vapor measurements, Atmos. Meas. Tech., 9, 939–953, https://doi.org/10.5194/amt-9-939-2016, 2016.
Kiehl, J. T. and Trenberth, K. E.: Earth's annual global mean energy budget, B. Am. Meteorol. Soc., 78, 197–208, https://doi.org/10.1175/1520-0477(1997)078<0197:eagmeb>2.0.CO;2, 1997.
Kiemle, C., Wirth, M., Fix, A., Ehret, G., Schumann, U., Gardiner, T., Schiller, C., Sitnikov, N., and Stiller, G.: First airborne water vapor lidar measurements in the tropical upper troposphere and mid-latitudes lower stratosphere: accuracy evaluation and intercomparisons with other instruments, Atmos. Chem. Phys., 8, 5245–5261, https://doi.org/10.5194/acp-8-5245-2008, 2008.
Krämer, M., Schiller, C., Afchine, A., Bauer, R., Gensch, I., Mangold, A., Schlicht, S., Spelten, N., Sitnikov, N., Borrmann, S., Reus, M. D., and Spichtinger, P.: Ice supersaturation and cirrus cloud crystal numbers, Atmos. Chem. Phys., 9, 3505–3522, 2009.
Krämer, M., Rolf, C., Luebke, A., Afchine, A., Spelten, N., Costa, A., Meyer, J., Zöger, M., Smith, J., Herman, R. L., Buchholz, B., Ebert, V., Baumgardner, D., Borrmann, S., Klingebiel, M., and Avallone, L.: A microphysics guide to cirrus clouds – Part 1: Cirrus types, Atmos. Chem. Phys., 16, 3463–3483, https://doi.org/10.5194/acp-16-3463-2016, 2016.
Krautstrunk, M. and Giez, A.: The Transition From FALCON to HALO Era Airborne Atmos. Res., in: Atmospheric Physics, 1 edition, Berlin, edited by: Schumann, U., Springer, 609–624, 2012.
Krisna, T. C., Wendisch, M., Ehrlich, A., Jäkel, E., Werner, F., Weigel, R., Borrmann, S., Mahnke, C., Pöschl, U., Andreae, M. O., Voigt, C., and Machado, L. A. T.: Comparing airborne and satellite retrievals of cloud optical thickness and particle effective radius using a spectral radiance ratio technique: two case studies for cirrus and deep convective clouds, Atmos. Chem. Phys., 18, 4439–4462, https://doi.org/10.5194/acp-18-4439-2018, 2018.
Kunz, A., Spelten, N., Konopka, P., Müller, R., Forbes, R. M., and Wernli, H.: Comparison of Fast In situ Stratospheric Hygrometer (FISH) measurements of water vapor in the upper troposphere and lower stratosphere (UTLS) with ECMWF (re)analysis data, Atmos. Chem. Phys., 14, 10803–10822, https://doi.org/10.5194/acp-14-10803-2014, 2014.
Lamquin, N., Gierens, K., Stubenrauch, C. J., and Chatterjee, R.: Evaluation of upper tropospheric humidity forecasts from ECMWF using AIRS and CALIPSO data, Atmos. Chem. Phys., 9, 1779–1793, https://doi.org/10.5194/acp-9-1779-2009, 2009.
Lee, J., Yang, P., Dessler, A. E., Gao, B.-C., and Platnick, S.: Distribution and Radiative Forcing of Tropical Thin Cirrus Clouds, J. Atmos. Sci., 66, 3721–3731, https://doi.org/10.1175/2009jas3183.1, 2009.
Liou, K. N.: Influence of Cirrus Clouds on Weather and Climate Processes – A Global Perspective, Mon. Weather Rev., 114, 1167–1199, https://doi.org/10.1175/1520-0493(1986)114<1167:ioccow>2.0.CO;2, 1986.
Lossow, S., Hurst, D. F., Rosenlof, K. H., Stiller, G. P., von Clarmann, T., Brinkop, S., Dameris, M., Jöckel, P., Kinnison, D. E., Plieninger, J., Plummer, D. A., Ploeger, F., Read, W. G., Remsberg, E. E., Russell, J. M., and Tao, M.: Trend differences in lower stratospheric water vapour between Boulder and the zonal mean and their role in understanding fundamental observational discrepancies, Atmos. Chem. Phys., 18, 8331–8351, https://doi.org/10.5194/acp-18-8331-2018, 2018.
Luebke, A. E., Afchine, A., Costa, A., Grooß, J.-U., Meyer, J., Rolf, C., Spelten, N., Avallone, L. M., Baumgardner, D., and Krämer, M.: The origin of midlatitude ice clouds and the resulting influence on their microphysical properties, Atmos. Chem. Phys., 16, 5793–5809, https://doi.org/10.5194/acp-16-5793-2016, 2016.
Lynch, D. K.: Cirrus clouds: Their role in climate and global change, Acta Astronaut., 38, 859–863, https://doi.org/10.1016/S0094-5765(96)00098-7, 1996.
Manabe, S. and Wetherald, R. T.: Thermal Equilibrium of the Atmosphere with a Given Distribution of Relative Humidity, J. Atmos. Sci., 24, 241–259, https://doi.org/10.1175/1520-0469(1967)024<0241:teotaw>2.0.CO;2, 1967.
Meyer, J., Rolf, C., Schiller, C., Rohs, S., Spelten, N., Afchine, A., Zöger, M., Sitnikov, N., Thornberry, T. D., Rollins, A. W., Bozóki, Z., Tátrai, D., Ebert, V., Kühnreich, B., Mackrodt, P., Möhler, O., Saathoff, H., Rosenlof, K. H., and Krämer, M.: Two decades of water vapor measurements with the FISH fluorescence hygrometer: a review, Atmos. Chem. Phys., 15, 8521–8538, https://doi.org/10.5194/acp-15-8521-2015, 2015.
Murphy, D. M. and Koop, T.: Review of the vapour pressures of ice and supercooled water for atmospheric applications, Q. J. Roy. Meteorol. Soc., 131, 1539–1565, https://doi.org/10.1256/qj.04.94, 2005.
Oltmans, S., Rosenlof, K., Michelsen, H., Nedoluha, G., Pan, L., Read, W., Remsberg, E., and Schiller, C.: SPARC Report No. 2: Upper Tropospheric and Stratospheric Water Vapour, Chapter 2, 2000.
Ovarlez, J., Gayet, J. F., Gierens, K., Strom, J., Ovarlez, H., Auriol, F., Busen, R., and Schumann, U.: Water vapour measurements inside cirrus clouds in Northern and Southern hemispheres during INCA, Geophys. Res. Lett., 29, 1813, https://doi.org/10.1029/2001gl014440, 2002.
Petzold, A., Kramer, M., Neis, P., Rolf, C., Rohs, S., Berkes, F., Smit, H. G. J., Gallagher, M., Beswick, K., Lloyd, G., Baumgardner, D., Spichtinger, P., Nedelec, P., Ebert, V., Buchholz, B., Riese, M., and Wahner, A.: Upper tropospheric water vapour and its interaction with cirrus clouds as seen from IAGOS long-term routine in situ observations, Faraday Discuss., 200, 229–249, https://doi.org/10.1039/C7FD00006E, 2017.
Pogány, A., Klein, A., and Ebert, V.: Measurement of water vapor line strengths in the 1.4–2.7 µm range by tunable diode laser absorption spectroscopy, J. Quant. Spectrosc. Ra., 165, 108–122, https://doi.org/10.1016/j.jqsrt.2015.06.023, 2015.
Pope, V. D., Pamment, J. A., Jackson, D. R., and Slingo, A.: The Representation of Water Vapor and Its Dependence on Vertical Resolution in the Hadley Centre Climate Model, J. Clim., 14, 3065–3085, https://doi.org/10.1175/1520-0442(2001)014<3065:trowva>2.0.CO;2, 2001.
Ramanathan, V. and Inamdar, A.: The radiative forcing due to clouds and water vapor, in: Frontiers of Climate Modeling, edited by: Kiehl, J. T. and Ramanathan, V., 119–151, Cambridge University Press, 2006.
Riese, M., Ploeger, F., Rap, A., Vogel, B., Konopka, P., Dameris, M., and Forster, P.: Impact of uncertainties in atmospheric mixing on simulated UTLS composition and related radiative effects, J. Geophys. Res.-Atmos., 117, D16305, https://doi.org/10.1029/2012JD017751, 2012.
Rollins, A. W., Thornberry, T. D., Gao, R.-S., Hall, B. D., and Fahey, D. W.: Catalytic oxidation anf H2 on platinum: a robust method for generating low mixing ratio H2O standards, Atmos. Meas. Tech., 4, 2059–2064, https://doi.org/10.5194/amt-4-2059-2011, 2011.
Rollins, A. W., Thornberry, T. D., Gao, R. S., Smith, J. B., Sayres, D. S., Sargent, M. R., Schiller, C., Krämer, M., Spelten, N., Hurst, D. F., Jordan, A. F., Hall, E. G., Vömel, H., Diskin, G. S., Podolske, J. R., Christensen, L. E., Rosenlof, K. H., Jensen, E. J., and Fahey, D. W.: Evaluation of UT/LS hygrometer accuracy by intercomparison during the NASA MACPEX mission, J. Geophys. Res.-Atmos., 119, 1915–1935, https://doi.org/10.1002/2013JD020817, 2014.
Saffin, L., Gray, S. L., Methven, J., and Williams, K. D.: Processes Maintaining Tropopause Sharpness in Numerical Models, J. Geophys. Res.-Atmos., 122, 9611–9627, https://doi.org/10.1002/2017JD026879, 2017.
Schiller, C., Grooß, J.-U., Konopka, P., Plöger, F., Silva dos Santos, F. H., and Spelten, N.: Hydration and dehydration at the tropical tropopause, Atmos. Chem. Phys., 9, 9647–9660, https://doi.org/10.5194/acp-9-9647-2009, 2009.
Schneider, T., O'Gorman, P. A., and Levine, X. J.: Water vapor and the dynamics of climate changes, Rev. Geophys., 48, RG3001, https://doi.org/10.1029/2009RG000302, 2010.
Schumann, U., Graf, K., Bugliaro, L., Dörnbrack, A., Voigt, C., Wirth, M., Ziereis, H., Giez, A., and Minikin, A.: Contrail predictions for ML-CIRRUS – Method and Experiences, 4th International Conference on Transport, Atmosphere and Climate, Bad Kohlgrub, Germany, 132–1138, 2015.
Schumann, U., Baumann, R., Baumgardner, D., Bedka, S. T., Duda, D. P., Freudenthaler, V., Gayet, J.-F., Heymsfield, A. J., Minnis, P., Quante, M., Raschke, E., Schlager, H., Vázquez-Navarro, M., Voigt, C., and Wang, Z.: Properties of individual contrails: a compilation of observations and some comparisons, Atmos. Chem. Phys., 17, 403–438, https://doi.org/10.5194/acp-17-403-2017, 2017.
Shilling, J. E., Tolbert, M. A., Toon, O. B., Jensen, E. J., Murray, B. J., and Bertram, A. K.: Measurements of the vapor pressure of cubic ice and their implications for atmospheric ice clouds, Geophys. Res. Lett., 33, L17801, https://doi.org/10.1029/2006GL026671, 2006.
Solomon, S., Rosenlof, K. H., Portmann, R. W., Daniel, J. S., Davis, S. M., Sanford, T. J., and Plattner, G. K.: Contributions of Stratospheric Water Vapor to Decadal Changes in the Rate of Global Warming, Science, 327, 1219–1223, https://doi.org/10.1126/science.1182488, 2010.
Stenke, A., Grewe, V., and Ponater, M.: Lagrangian transport of water vapor and cloud water in the ECHAM4 GCM and its impact on the cold bias, Clim. Dynam., 31, 491–506, https://doi.org/10.1007/s00382-007-0347-5, 2008.
Thornberry, T. D., Rollins, A. W., Gao, R. S., Watts, L. A., Ciciora, S. J., McLaughlin, R. J., Voigt, C., Hall, B., and Fahey, D. W.: Measurement of low-ppm mixing ratios of water vapor in the upper troposphere and lower stratosphere using chemical ionization mass spectrometry, Atmos. Meas. Tech., 6, 1461–1475, https://doi.org/10.5194/amt-6-1461-2013, 2013.
Urbanek, B., Groß, S., Schäfler, A., and Wirth, M.: Determining stages of cirrus evolution: a cloud classification scheme, Atmos. Meas. Tech., 10, 1653–1664, https://doi.org/10.5194/amt-10-1653-2017, 2017.
Voigt, C., Schumann, U., Minikin, A., Abdelmonem, A., Afchine, A., Borrmann, S., Boettcher, M., Buchholz, B., Bugliaro, L., Costa, A., Curtius, J., Dollner, M., Dörnbrack, A., Dreiling, V., Ebert, V., Ehrlich, A., Fix, A., Forster, L., Frank, F., Fütterer, D., Giez, A., Graf, K., Grooß, J.-U., Groß, S., Heimerl, K., Heinold, B., Hüneke, T., Järvinen, E., Jurkat, T., Kaufmann, S., Kenntner, M., Klingebiel, M., Klimach, T., Kohl, R., Krämer, M., Krisna, T. C., Luebke, A., Mayer, B., Mertes, S., Molleker, S., Petzold, A., Pfeilsticker, K., Port, M., Rapp, M., Reutter, P., Rolf, C., Rose, D., Sauer, D., Schäfler, A., Schlage, R., Schnaiter, M., Schneider, J., Spelten, N., Spichtinger, P., Stock, P., Walser, A., Weigel, R., Weinzierl, B., Wendisch, M., Werner, F., Wernli, H., Wirth, M., Zahn, A., Ziereis, H., and Zöger, M.: ML-CIRRUS: The Airborne Experiment on Natural Cirrus and Contrail Cirrus with the High-Altitude Long-Range Research Aircraft HALO, B. Am. Meteorol. Soc., 98, 271–288, https://doi.org/10.1175/bams-d-15-00213.1, 2017.
Vömel, H., David, D. E., and Smith, K.: Accuracy of tropospheric and stratospheric water vapor measurements by the cryogenic frost point hygrometer: Instrumental details and observations, J. Geophys. Res.-Atmos., 112, D08305, https://doi.org/10.1029/2006jd007224, 2007.
Weinstock, E. M., Smith, J. B., Sayres, D. S., Pittman, J. V., Spackman, J. R., Hintsa, E. J., Hanisco, T. F., Moyer, E. J., St Clair, J. M., Sargent, M. R., and Anderson, J. G.: Validation of the Harvard Lyman-alpha in situ water vapor instrument: Implications for the mechanisms that control stratospheric water vapor, J. Geophys. Res.-Atmos., 114, D23301, https://doi.org/10.1029/2009jd012427, 2009.
Wernli, H., Boettcher, M., Joos, H., Miltenberger, A. K., and Spichtinger, P.: A trajectory-based classification of ERA-Interim ice clouds in the region of the North Atlantic storm track, Geophys. Res. Lett., 43, 6657–6664, https://doi.org/10.1002/2016GL068922, 2016.
Zöger, M., Afchine, A., Eicke, N., Gerhards, M. T., Klein, E., McKenna, D. S., Morschel, U., Schmidt, U., Tan, V., Tuitjer, F., Woyke, T., and Schiller, C.: Fast in situ stratospheric hygrometers: A new family of balloon-borne and airborne Lyman alpha photofragment fluorescence hygrometers, J. Geophys. Res.-Atmos., 104, 1807–1816, https://doi.org/10.1029/1998jd100025, 1999.