the Creative Commons Attribution 4.0 License.
the Creative Commons Attribution 4.0 License.
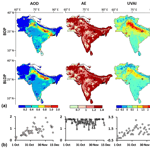
Aerosol chemistry, transport, and climatic implications during extreme biomass burning emissions over the Indo-Gangetic Plain
Nandita Singh
Tirthankar Banerjee
Made P. Raju
Karine Deboudt
Meytar Sorek-Hamer
Ram S. Singh
Rajesh K. Mall
The large-scale emissions of airborne particulates from burning of agricultural residues particularly over the upper Indo-Gangetic Plain (IGP) have often been associated with frequent formation of haze, adverse health impacts, and modification in aerosol climatology and thereby aerosol impact on regional climate. In this study, short-term variations in aerosol climatology during extreme biomass burning emissions over the IGP were investigated. Size-segregated particulate concentration was initially measured and submicron particles (PM1.1) were found to dominate particulate mass within the fine mode (PM2.1). Particulate-bound water-soluble ions were mainly secondary in nature and primarily composed of sulfate and nitrate. There was evidence of gaseous NH3 dominating neutralization of acidic aerosol species () in submicron particles, in contrast to crustal-dominating neutralization in coarser particulates. Diurnal variation in black carbon (BC) mass ratio was primarily influenced by regional meteorology, while gradual increase in BC concentration was consistent with the increase in Delta-C, referring to biomass burning emissions. The influence of biomass burning emissions was established using specific organic (levoglucosan), inorganic (K+ and ), and satellite-based (UV aerosol index, UVAI) tracers. Levoglucosan was the most abundant species within submicron particles (649±177 ng m−3), with a very high ratio (> 50) to other anhydrosugars, indicating exclusive emissions from burning of agriculture residues. Spatiotemporal distribution of aerosol and a few trace gases (CO and NO2) was evaluated using both spaceborne active and passive sensors. A significant increase in columnar aerosol loading (aerosol optical depth, AOD: 0.98) was evident, with the presence of absorbing aerosols (UVAI > 1.5) having low aerosol layer height (∼ 1.5 km). A strong intraseasonality in the aerosol cross-sectional altitudinal profile was even noted from CALIPSO, referring to the dominance of smoke and polluted continental aerosols across the IGP. A possible transport mechanism of biomass smoke was established using cluster analysis and concentration-weighted air mass back trajectories. Short-wave aerosol radiative forcing (ARF) was further simulated considering intraseasonality in aerosol properties, which resulted in a considerable increase in atmospheric ARF (135 W m−2) and heating rate (4.3 K day−1) during extreme biomass burning emissions compared to the non-dominating period (56 W m−2, 1.8 K day−1). Our analysis will be useful to improve understanding of short-term variation in aerosol chemistry over the IGP and to reduce uncertainties in regional aerosol–climate models.
- Article
(8868 KB) - Full-text XML
-
Supplement
(145 KB) - BibTeX
- EndNote
Aerosols are studied systematically in terms of their potential to influence the transfer of radiant energy and distribution of latent heat, by which they modify the Earth's weather and climate. Aerosols are also associated with nutrient recycling and governing atmospheric chemistry (Kanakidou et al., 2018). Aerosol interaction with radiation mainly constitutes its radiative forcing of climate change (Bellouin et al., 2005; Bond et al., 2013) while it also modifies the climate by means of cloud formation processes (Seinfeld et al., 2016). Aerosol–radiation interaction necessitates understanding of spectrally varying aerosol optical properties, which are associated with particle size distribution, chemical composition, morphology, and mixing states. The representation of aerosol processes in global and regional climate models varies considerably and thereby estimates of aerosol–radiation interaction still contain significant uncertainties (Myhre et al., 2013). This necessitates extensive regional investigation in terms of aerosol composition and properties for improved parametrization of aerosol schemes in the regional and global climate model.
The Indo-Gangetic plain (IGP) in South Asia is especially unique in terms of aerosol loading and diversity that varies over the seasons (Singh et al., 2017a, b; Sen et al., 2017; Sayer et al., 2014; Kumar et al., 2018). The IGP is often projected to be one of the most vulnerable region in terms of aerosol-induced negative health impacts (Apte et al., 2015) and therefore numerous observational and modeling studies were performed for better characterization of aerosols (Sen et al., 2017; Moorthy et al., 2008, and references therein). Recently, Singh et al. (2017a) concluded the presence of spatial and seasonal variations in aerosol sources over South Asia, with vehicular emissions, followed by industrial emissions and secondary aerosols, contributes most to fine particulates. Additionally, episode-specific emissions like from biomass burning (Wan et al., 2017; Rajput et al., 2011, 2014; Rajput and Sarin, 2014) and the use of fire crackers (Kumar et al., 2016) also induce sudden large-scale changes in aerosol properties and necessitate extensive investigation for better representation in regional aerosol models. Post-harvest agricultural residue burning, especially over the upper IGP, is projected to release 400 Gg of particulate-bound organic aerosol (OA) and 40 Gg of black carbon (BC; Rajput et al., 2014), almost entirely (90 %) from the burning of rice husks (Rajput et al., 2011). The OA mostly constitutes the fine particulate mass (20 %–90 %) and is reported to be hydrophilic in nature (Rajput and Sarin, 2014), therefore having the potential to act as cloud condensation nuclei molecules or at the most compete with sulfate particles (Singh et al., 2017b). Nevertheless, the presence of such a huge amount of OA may lead to either a reduction in mean evaporation and modify regional precipitation or may reduce cloud formation processes by inducing additional heat in the system (Riipinen et al., 2011; Sun and Ariya, 2006). Biomass burning aerosols also impact the Earth's surface albedo by depositing on glaciers. The net radiative forcing of biomass burning aerosols by aerosol–radiation interactions is close to neutral, i.e., −0.0 (−0.20 to +0.20) W m−2, having a gradient with negative forcing from OA and positive forcing from BC (Myhre et al., 2013). Biomass burning aerosols even evolve due to oxidation (Jimenez et al., 2009; Vakkari et al., 2014), from gas-phase precursors to semi-volatile secondary OA (SOA) and finally to highly volatile oxidized gases (e.g., CO and CO2), thus warranting molecular characterization and specific understanding in terms of composition, atmospheric chemistry, transport, and radiative forcing (Singh et al., 2017b).
Several investigations were made over the IGP to understand the characteristics of biomass burning aerosols. A few attempts were made using solely ground-based information, e.g., aerosol emission budget (Rajput et al., 2014), organic mass-to-organic carbon ratio (Rajput and Sarin, 2014), emissions of PAHs (Rajput et al., 2011), organic molecular tracers (Wan et al., 2017; Li et al., 2014), and radiative forcing (Sharma et al., 2017; Alam et al., 2011); however, few have explored remote-sensing observations to interpret fire (Vadrevu et al., 2012) and aerosol plume characteristics (Kaskaoutis et al., 2014). However, there is a need to integrate both ground and contemporary satellite-based information so that spatiotemporal characterization of aerosols and their climatic impacts are assessed more realistically. In the present analysis complementary measurements from both ground- and space-based platforms are therefore combined to trace the vital signatures of extreme biomass burning emissions and their chemical evolution, transport, and aerosol radiative forcing. Initially, chemical speciations of size-segregated aerosols are made, supported by BC dynamics and molecular tracers of biomass burning emissions; they are then further explored in terms of their relevance to regional meteorology. The spatial extent of aerosol emission and transport was obtained using Modern-Era Retrospective Analysis for Research and Applications (MERRA) atmospheric reanalysis data, Global Data Assimilation System (GDAS) archives, and NCEP/NCAR Reanalysis data. Further, visualization from the A-Train satellite constellation, including spaceborne passive sensors like the Moderate Resolution Imaging Spectroradiometer (MODIS) and the Ozone Monitoring Instrument (OMI) and an active sensor like the Cloud-Aerosol Lidar and Infrared Pathfinder Satellite Observation (CALIPSO), are included. Briefly, the results are explored to highlight three exclusive but interrelated mechanisms, i.e., aerosol chemistry, regional transport, and radiative forcing, as well as their intraseasonal variations over the middle IGP, which may well be useful in regional climate models.
2.1 Site description
Ground-based aerosol measurements were made at the institutional premises of Banaras Hindu University, Varanasi (25.26∘ N, 82.98∘ E, 82 m a.m.s.l.). The ground station typically experiences a humid subtropical climate, with no localized effects of oceans or mountains (Fig. 1). The predominating wind profile is northwesterly, which is projected to subsidize over a section of the middle IGP, coinciding well with the ground monitoring station, thereby facilitating gradual accumulation of aerosols (Kumar et al., 2018). Interestingly enough, the region also experiences a significant diurnal variation in atmospheric boundary layer (ABL) associated with high convective turbulence that usually redistributes aerosols to a greater height (M. Kumar et al., 2015, 2017a; R. Kumar et al., 2015). Particulates emitted from crustal sources, road dust resuspension, vehicular exhaust, and biomass/waste burning are often reported to constitute the regional aerosols (Singh et al., 2017a).
2.2 Micrometeorology, ABL, and wind field
The 24 h average meteorological parameters, e.g., temperature, relative humidity (RH), and wind speed (WS), were obtained from Weather Underground (https://www.wunderground.com/) and validated with regional weather monitoring station data. The ABL heights at specific coordinates were retrieved from Global Data Assimilation System (GDAS) archives hosted at NOAA Air Resources Laboratory, which provides simulated meteorological observations at a gridded scale. The 3-hourly ABL data (0.5∘) were averaged on a daily basis in parallel with the period of particulate measurement. The NCEP/NCAR Reanalysis data were used to analyze the variation in 3-D wind fields at the near surface (1000 m) with a horizontal resolution of . Vector wind composite mean (m s−1) for 925 hPa was plotted for the defined coordinate (6–38∘ N, 50–105∘ E) to understand the synoptic pattern of the wind field.
2.3 Ground-based measurements
2.3.1 Size-segregated aerosol mass concentration
Size-segregated aerosols were collected on the pre-combusted quartz fiber filter using an Anderson eight-stage cascade impactor (Tisch Environmental Inc., USA). Sampling was continued once a week from 1 October to 15 December 2016, continuously for 72 h (each week) to obtain representative deposition of particulates. The instrument was run with a fixed flow rate of 28.3 L min−1, having an aerodynamic cutoff diameter of < 0.43, 0.65, 1.1, 2.1, 3.3, 4.7, 5.8, and > 9.0 µm (with 50 % collection efficiency). The individual stages of each sample were then segregated into three groups on the basis of cutoff diameter, with (i) coarse mode (PM> 2.1) comprising the stages with an aerodynamic diameter > 2.1 µm, (ii) fine mode (PM1.1–2.1) for the stages with a diameter of 1.1 to 2.1 µm, and (iii) submicron mode (PM< 1.1) for the last two stages with a diameter < 1.1 µm.
2.3.2 Black carbon mass concentration
The BC real-time mass concentration was measured using a seven-channel Aethalometer (model AE-42, Magee Scientific Inc., USA), with a constant flow rate of 3 L min−1 at 5 min resolution. The Aethalometer measures the attenuated beam of light transmitted through aerosol samples on filter tape at seven wavelengths (370, 470, 520, 590, 660, 880, and 950 nm), while attenuation at 880 nm was considered for BC (Bodhaine, 1995). The BC concentration is estimated based on the concept of linearity between the light attenuation and BC mass deposited on the quartz filter. An absorption efficiency of 16.6 m2 g−1 (provided by the manufacturer) was used to measure BC after correction of loading effect. The mechanism for estimation of BC is described in Wang et al. (2011) and Kumar et al. (2017a). BC measured at two wavelengths, e.g., 370 nm (indicating absorption by wood-smoke particles) and 880 nm (by both fossil fuel and wood burning emissions), was used to compute Delta-C (BC370 nm–BC880 nm). Delta-C is reported to symbolize smoke emissions (Wang et al., 2011; Kumar et al., 2016) and, therefore, was used as a tracer for biomass burning emissions.
2.3.3 Aerosol chemical constituents
Water-soluble ions
The particulate deposits on filter were extracted with deionized water in an ultrasonic bath (Microclean-109, Oscar, India) for 30 min, and extracts were further filtered through syringe filters (pore size 0.2 µm). The water-soluble ionic constituents (WSICs) were analyzed using an ion exchange chromatograph (ICS 3000, Dionex, USA). For measurement of anions (Cl−, , , and ), the ion chromatograph was equipped with a micromembrane suppressor (AERS-300, 4 mm; Dionex) with an IonPac analytical column (AS11-HC × 250 mm) connected with a guard column IonPac (AG11-HC, 4×50 mm; Dionex). Cations (, Na+, K+, Mg2+, Ca2+) were measured through a suppressor (CERS-300, 4 mm; Dionex) with an analytical column (IonPac CS12A-HC, 4×250 mm; Dionex) and a guard column (IonPac CG11-HC, 4×50 mm; Dionex, USA). The background contamination was removed by subtracting the blank filter value from sample values (Kumar et al., 2017b).
Trace metals
The trace metals were extracted from filter discs as per U.S. EPA Method IO-3.2 (EPA, 1999). The filters were cut into pieces and digested in an acid mixture solution (5.55 % HNO3 with 16.67 % HCl) on a hot plate for 2 h. The extracts were filtered, stored at 4 ∘C, and analyzed with an atomic absorption spectrophotometer (Avanta version 2.01, GBC) for Ca, Na, K, Cu, Mn, Fe, Cd, Cr, Pb, Ni, Co, and Zn.
Organic compounds
For determining the aerosol organic constituents, the filter composites of each group were extracted by ultrasonicating the filters initially with a dichloromethane–hexane mixture (1:1), followed by a dichloromethane–methanol mixture (1:1). Both solvent extracts were combined and concentrated using a vacuum rotatory evaporator and nitrogen evaporator to a volume of 100 µL (Hu et al., 2013). The extracts were derivatized by silylation with N, O-bis-(trimethylsilyl)-trifluoroacetamide and 1 % trimethylchlorosilane prior to analysis. After derivatization, the residue was redissolved in hexane and analyzed with a gas chromatograph–mass spectrometer (GCMS-QP2010 Ultra, Shimadzu, Japan) equipped with a Rxi-5ms fused silica capillary column having the dimensions 30 m × 0.25 mm ID × 0.25 µm (Restek, Bellefonte, PA, USA). The sample was injected in the GC–MS at 260 ∘C injector temperature in splitless mode. The column oven temperature program was started at 50 ∘C with 2 min of the isothermal hold, which was further increased to 120 ∘C (linear elevation at 30 ∘C min−1) and 300 ∘C (linear elevation at 6 ∘C min−1) followed by an isothermal hold of 11 min. The electron impact ionization was used to produce molecular ions at 70 eV with the ion source and interface temperatures of 230 and 270 ∘C. The molecular ions were scanned for a wide range of m∕z values from 40 to 650. The target compounds were identified based on retention time and fragmentation pattern from the National Institute of Standards and Technology (NIST) library and standard solutions of analytes. The average recoveries (relative standard deviation, RSD) of the n-alkanes (28 compounds) varied from 72 % to 92 % (1 %–12 %), 75 % to 88 % (2 %–7 %) for phthalates (six compounds), 74 % to 92 % (1 %–9 %) for FAMES, 73 % to 93 % (1 %–10 %) for PAHs, and 75 % to 80 % (4 %–6 %) for anhydrosugars (three compounds).
2.4 Satellite-based observations
2.4.1 Aqua and Terra MODIS data
The aerosol optical depth (AOD) at 550 nm was retrieved daily from MODIS onboard the Aqua satellite in parallel to ground-based aerosol monitoring. The level 2 collection 6 AOD at 10 km resolution was retrieved using MODIS merged DT–DB AOD (AOD_550_-Dark_Target_Deep_Blue_Combined; Levy et al., 2013). The selection of merged DT–DB for retrieving AOD was based on higher retrieval number and accuracy across the IGP (Mhawish et al., 2017). The AOD over the ground station was calculated as the average of 5×5 pixels, surrounding the monitoring site. The Ångström exponent (AE, α) was retrieved using MODIS C6 level 2 DB AOD and the relation between AOD and AE was used to measure the aerosol loading and the particle size (M. Kumar et al., 2015; Mhawish et al., 2017). Columnar water vapor content (CWV) was retrieved from Aqua MODIS collection 6 level 2 infrared channel at 1 km spatial resolution. To illustrate the impact of biomass burning, the fire spots were retrieved over the IGP from Aqua and Terra MODIS fire mapper product (collection 6, spatial resolution 1×1 km2) provided by the Fire Information for Resource Management System (FIRMS, https://firms.modaps.eosdis.nasa.gov, last access: 2 August 2017). Details about MODIS fire products and their algorithms can be found elsewhere (Justice et al., 2006).
2.4.2 Aura OMI and MERRA-2 reanalysis data
OMI onboard the AURA satellite has a typical daily global coverage with 13×24 km2 spatial resolution at the nadir and measures solar backscatter irradiation in the UV–visible spectrum (264–504 nm; Levelt et al., 2006). Ultraviolet aerosol index (UVAI), tropospheric NO2, total columnar ozone (TCO), and single-scattering albedo (SSA) were retrieved from Aura OMI available at NASA Goddard Earth Sciences Data and Information Services Centre (GES DISC). OMI UVAI is capable of detecting aerosol absorption from satellite measured radiances without any prior assumption on aerosol composition (Torres et al., 2013). It is a qualitative parameter and is widely used to identify the UV absorbing aerosols (e.g., smoke plumes, soot, and mineral dust; Torres et al., 2013; Mhawish et al., 2018). The UVAI based on the OMI near-UV aerosol retrieval algorithm (OMAERUV) was extracted from the level 2G, version 003 aerosol product containing 1 day's level 2 dataset of original pixels (13×24 km2) into grids. The NO2 tropospheric column density was retrieved from the cloud-screened (cloud fraction < 30 %) level 3, version 003, daily gridded OMNO2d product (Krotkov et al., 2017). To estimate TCO, level 3e data (OMDOAO3) at a spatial resolution of were used. SSA at 550 nm was retrieved from the OMI level 2G product (OMAERUV) at resolution. The carbon monoxide (CO) surface concentration (in parts per billion by volume) was retrieved from Modern-Era Retrospective Analysis for Research and Applications version 2 (MERRA-2) atmospheric reanalysis data available at resolution from GES DISC.
2.4.3 CALIPSO–CALIOP observations
CALIPSO products were used to examine the vertical distribution of aerosols, altitude of aerosol layers, clouds, aerosol types, and their properties at visible (532 nm) and near-IR wavelengths (1064 nm). The v4.10 CALIOP Level 2 altitude–orbit cross-section profiles retrieved from the CALIPSO sub-setting web application (https://www-calipso.larc.nasa.gov, last access: 9 August 2017) were used. The lidar profiles were processed for images of vertical feature masks, aerosol subtypes, and extinction coefficients (at 532 nm) at 30 m vertical resolution over the selected grid (80–86∘ N and 22–28∘ E). Details about data products, calibration, and uncertainty are discussed in Rogers et al. (2011).
2.5 Air mass back trajectory
The NOAA HYSPLIT model (Draxler and Rolph, 2003) was used to simulate particle back trajectories in a three-dimensional system. HYSPLIT was run using the Global Data Assimilation System data (GDAS, ) available from the archive dataset (http://ready.arl.noaa.gov/gdas1.php) to predict 120 h air mass back trajectories (00:00, 06:00, 12:00, and 18:00 IST, Indian standard time) starting from October to December 2016. Trajectories for different aerosol loading periods were then overlaid on the MODIS fire map to study the transboundary movement of emissions from biomass burning. The trajectory analysis was made using the GIS-based software TrajStat (Wang et al., 2009). Concentration-weighted trajectories (CWTs) were also drawn considering columnar aerosol loading to evaluate potential aerosol source fields and mechanisms of aerosol transport. The specificities of the models' parameters and algorithms are detailed elsewhere (Wang et al., 2009; Kumar et al., 2018).
2.6 Aerosol optical properties, radiative forcing, and heating rate
Aerosol-induced shortwave (0.2–4.0 µm) direct radiative forcing (ARF) was estimated using the Santa Barbara DISORT Atmospheric Radiative Transfer (SBDART) model (Ricchiazzi et al., 1998). SBDART estimates plane-parallel radiative transfer under clear-sky conditions for both Earth's top of the atmosphere (TOA) and at the surface (SUF), while atmospheric forcing (ATM) is calculated as the difference between them. The standard atmospheric profile is used together with input variables, e.g., AOD, SSA, CWV, TCO, and an asymmetry parameter (ASP) derived through the OPAC model (Optical Properties of Aerosols and Clouds; Hess et al., 1998). OPAC provides aerosol optical properties over a wide range of wavelengths and delivers necessary input to SBDART. Mean mass concentrations of aerosol water soluble (WSIC) and insoluble (dust and organics) components along with BC mass concentration were converted to particle number densities and introduced to OPAC to derive aerosol optical properties. The AOD and SSA were reconstructed to match modeled and satellite-derived values within ±5 % deviation.
The weekly mean values of AOD, SSA, ASP, CWV, TCO, visibility, and AE were included as inputs to SBDART. SBDART includes multiple scattering in a vertically inhomogeneous, non-isothermal plane-parallel media and is reported to be efficient in resolving the radiative transfer equation (Raju et al., 2016). The ARF was calculated using 10 solar zenith angles (0 to 89, with increments of 10) and was used for conditions like “with aerosols” or “without aerosols”. The surface albedo was decided based on visual observation considering a combination of snow, ocean, sand, and vegetation. Overall uncertainty in the estimated ARF was in the range of 10 %–15 % (Alam et al., 2011). The ATM–ARF was further used to compute atmospheric heating rate (, K day−1), using Eq. (1):
where ΔP is the difference in forcing, ΔP is the pressure difference between the top and bottom boundary layer, Cp is specific heat capacity of air at constant pressure, and g is the acceleration due to gravity (Kumar et al., 2017a).
3.1 General characteristics of aerosols
The weekly variation in particulate concentrations in different size fractions is presented in Fig. 2 with the descriptive statistics included in Supplement Table S1. The total aerosol mass concentration has high intraseasonal variations (median: 370; range: 134–734 µg m−3), mainly influenced by coarse-mode particles (PM> 2.1) contributing 63±15 % of particulate mass. In contrast, the contribution of submicron (PM< 1.1: 27±12 %) and fine-mode particles (PM1.1–2.1: 10±4 %) to total aerosol loading was relatively less (< 37 %). The average (±1σ) mass concentration of PM2.1 (PM< 1.1 + PM1.1–2.1) and total aerosol loading was 162 (±123) and 390 (±199) µg m−3, which were approximately 98 % (against PM2.5) and 92 % higher compared to annual averages observed over the monitoring station (Murari et al., 2017; Prajapati and Tripathi, 2008). To our knowledge, until submission of the paper, there was no published report on submicron particles over the ground station. Time series analysis of size-segregated particulates (Fig. 2) indicates the submicron (PM< 1.1) and fine-mode particles (PM1.1–2.1) only had a late rise in mass concentration, while the coarse-mode particulates (PM> 2.1) did not show any trend. However, there was a definite increasing pattern in fine-to-coarse particle ratio (PM2.1 ∕ PM> 2.1; mean: 0.7±0.5; range: 0.2–1.5) due to a continuous increase in the fine mode from mid-November to the end of monitoring. Thus, the contribution of fine-mode particles to total aerosol loading increased from mid-November (> 40 %) and contributed almost 60 % of particulate mass during the month of December. The submicron particles also indicate a high median concentration (96 µg m−3) compared to the fine mode (33 µg m−3), and the particle ratio (PM< 1.1 ∕ PM1.1–2.1) remains > 1 throughout, only to exceed values > 2.5 from November to December. This clearly indicates the dominance of submicron particles within fine-mode fractions, possibly associated with anthropogenic emissions and also influenced by local meteorological conditions, e.g., low temperature (mean ± SD: 20±3 ∘C), calm wind (mean: 0.6 m s−1), and shallow boundary layer height (mean ± SD: 379±89 m).
3.2 Aerosol chemical speciations
3.2.1 Water-soluble inorganic species (WSIS)
Temporal variation in WSIS in size-segregated airborne particulates is presented in Fig. 3a. It indicates the major contribution of WSIS to submicron (21 %) and fine-particle mass (21 %) compared to coarser particles (13 %). Secondary inorganic aerosols (SIA = ) together accounted for 17 % of the submicron particle mass, with major contributions from sulfate (9 %) and nitrate (6 %). Similarly, the case for fine particulates as SIA contributed to almost 17 % of aerosol mass with predominant contributions from sulfate (8 %) and nitrate (6 %) and a relatively small proportion of ammonia (4 %). In contrast, the relative contribution of SIA to coarse particulate was lower (7 %), also primarily associated with sulfate (5 %) and nitrate compounds (2 %). This indicates the secondary nature of the origin of fine and submicron particles, which possibly evolve through gas-phase photochemical conversion of SO2 and NO2, eventually neutralized by crustal species like carbonate salts (CaCO3 and MgCO3) associated with airborne dust. The time series of SIA contribution to particulate mass (Fig. 3a) indicate a dominance (although in different extent) of secondary aerosols in PM1.1–2.1 and PM> 2.1 only during November.
Among the WSIS, was invariably the most abundant species within each particulate size fraction (PM< 1.1: 39 %, PM1.1–2.1: 32 %, PM> 2.1: 36 %), followed by (PM< 1.1: 27 %, PM1.1–2.1: 29 %, PM> 2.1: 17 %). The ratio was considered to be an indicator of the mobile and stationary source contribution to nitrogen and sulfur (Tian et al., 2016). An average ratio varying from 0.62 to 1.92 was noted for all size-segregated particulates, testifying dominance of both sources, although on different timescales. In the later phase, the ionic ratio () was enhanced (> 1) in submicron and fine-mode particles, identical to the reported haze events over Guangzhou (Tan et al., 2009) and Suzhou, China (Tian et al., 2016). A very high ratio (3.2±1.3) was only noted in fine aerosols during October, mainly due to a lower concentration of sulfate. The next two dominant contributors to WSIS were (PM< 1.1: 14 %, PM1.1–2.1: 19 %, PM> 2.1: 5 %) and K+ (PM< 1.1: 8 %, PM1.1–2.1: 5 %, PM> 2.1: 2 %), both considered to be molecular tracers for biomass burning emission (Banerjee et al., 2015). They constitute the greater proportion of WSIS in PM< 1.1 and PM1.1–2.1, especially from the last week of October until the end of November, signifying an elevated contribution of biomass/agro-residue burning emissions to these particle sizes. Further, a strong correlation (R2=0.9) between and and high equivalent ratio (0.9±0.2) for submicron particulates indicate the abundance of gaseous NH3 to neutralize acidic species () by forming (NH4)2SO4 and/or NH4HSO4. The equivalent ratio gradually increased from week 5 (mean: 1.2; range: 0.9–1.3), possibly due to abundant emission of from biomass burning emissions. Unlike submicron particles, the low equivalent ratios (< 0.7, mean: 0.4) in coarse-mode particles indicate the predominant neutralization by crustal minerals.
Unlike the other WSISs, Na+ and Ca2+ were found to contribute the most to PM> 2.1 (Na+: 2 %; Ca2+: 3 %), indicating their crustal origin. The relative abundance of Cl− in size-segregated aerosols was roughly equal for each size fraction, contributing almost identically to total WSIS in PM< 1.1 (6 %), PM1.1–2.1 (5 %), and PM> 2.1 (4 %). The possible origin of Cl− in PM> 2.1 could be the aged sea salt, transported from the Bay of Bengal, but its association with PM< 1.1 was most likely due to biomass burning emissions (Pavuluri at al., 2011; Murari et al., 2015). The temporal variations in WSISs in all particulate size fractions were consistent except for Mg2+ and contributing < 0.2 % of particulate mass and having non-biomass-specific emission sources. A strong correlation between the anion and cation equivalents within all the size groups (0.7–0.9) indicates that most ions were from the filter samples. The total ion equivalent ratio (anions to cation) refers to a cationic imbalance (PM< 1.1: 1.2, PM1.1–2.1: 0.8 and PM> 2.1: 0.6) with excess cations in fine- and coarse-mode particles, possibly due to unmeasured components like carbonates and bicarbonates.
3.2.2 Trace metals
Total metallic contribution to particulate mass was found at a maximum in PM1.1–2.1 (24 %), followed by PM> 2.1 (11 %) and was the least in PM< 1.1 (7 %, Fig. 3b). The most abundant elements were Na, Ca, K, and Zn for all size fractions, contributing 90 %–98 % of total identified metals, while the remaining fractions were primarily constituted by Fe (1 %–10 %). Within the detectable level of metals, Ca and Na share 88 % of metal concentrations in PM< 1.1 and 7 % of submicron particulate mass, without having any specific temporal trend. However, Ca and Na were found to be high in PM1.1–2.1 (Ca: 10 %; Na: 7 %), indicating their origin from resuspension of crustal materials and road dust.
There is little evidence of trace metal emissions from burning of biomass. Wang et al. (2015) have concluded that biomass combustion is the most prominent source of Fe concentration in submicron particles. For this analysis, although Fe was measured at a maximum in PM> 2.1, the relative increase in Fe concentration in submicron (PM1.1: 59 %) and fine aerosols (PM1.1–2.1: 415 %) during week 6 to week 9 possibly indicates the added contribution of biomass burning emissions. In addition to Fe, there are also reports of trace metal emissions, particularly K, Cu, S, Zn, and Pb, from burning of rice straw (Ryu et al., 2004); organic bound Fe2+, Cu2+, Ni2+, and Zn2+ from hardwood burning (Chang-Graham et al., 2011); and Cu, Pb, Ni, and As from the burning of biomass fuel (Zhang et al., 2014). In our case, a massive increase in K (PM1.1: 528 %; PM1.1–2.1: 119 %) was also noted between weeks 6 and 9. This contrasted with coarse-particle-bound Fe and K, which are primarily of crustal origin (Banerjee et al., 2015), and we recorded only a 15 % (Fe) and 83 % (K) increase in concentration within weeks 6 to 9. Zinc was found to be considerably high in PM> 2.1 (3 %) and relatively low in PM1.1–2.1 (2 %). The major sources of atmospheric Zn are burning of residual oil, refuse, and garbage (Gonzalez et al., 2016), which possibly leads to higher mass fractions in coarser particulates. Here, a relatively high Zn concentration was noted in the later phase of monitoring irrespective of particulate size coinciding with the winter-specific burning of waste refuse (Kumar et al., 2017b). The relative contribution of the rest of the trace metals (e.g., Mn, Pb, Cd, Ni, Cu, Cr, and Co) to particulate mass was insignificant (< 0.05 %), without having any specific temporal pattern.
3.3 Characteristics of BC mass loading
Daily means of BC concentration and Delta-C (BC370–BC880) are plotted in Fig. 4 with some data gaps. The 24 h average BC concentration varied from 2.0 to 15.4 µg m−3 with a seasonal mean (±1σ) of 8.3 (±2.9) µg m−3. The season-specific BC average was 80 % higher in comparison to the annual mean (4.6 µg m−3; Kumar et al., 2017a), while there were also reports of winter-specific very high BC concentration (22 µg m−3; Murari et al., 2016) that usually persisted over the region. A distinct diurnal profile with a high BC concentration during nighttime (> 9 µg m−3; 23:00–07:00 IST) and low daytime concentrations (< 6 µg m−3, 11:00–17:00 IST) was also noted. A gradual rise in BC mass loading from 17:00 IST could be mainly attributed to the regional meteorology, which is reported as the most influential factor in regulating BC concentrations compared to anthropogenic sources (R. Kumar et al., 2015; M. Kumar et al., 2017). Boundary layer height and transport are two fundamental processes that influence the diurnal BC variation, mainly by means of regulating horizontal and vertical transport (R. Kumar et al., 2015). Therefore, the diurnal variation in BC concentration was the consequence of change in boundary layer height with occasional inflow of emissions from large-scale burning of agriculture residues–biomass–waste and from vehicular emissions.
The BC time series shows an enhanced BC concentration from the end of October (week 4) until the end of November (week 9). Such an increase in BC concentration was, however, possibly due to increased source strength as there was no significant variation in meteorological variables (like ABL) within this timeframe (Table S1). To understand the variation in BC sources, 24 h average Delta-C concentration (mean ± SD: 2.3±1.0 µg m−3) is also included in Fig. 4, which refers to the emission of smoke particles (Wang et al., 2011; Kumar et al., 2016). Except for a few exceptions, high Delta-C (> 2.3 µg m−3) was observed particularly in the month of November (80 % of days) compared to October (23 %) and December (46 %), referring to the added contribution of biomass burning emissions.
3.4 Composition of organic aerosols
Size-segregated particle-bound OAs were analyzed for 22 n-alkanes (C13-C34), three anhydrosugars (levoglucosan, mannosan, and galactosan), four PAHs, and 10 n-alkanoic acids (C12-C26) (Fig. 5). Considerable variation in the concentration and size distributions of these OAs was noted. Contributions of OA to size-segregated particulates were relatively low because of partial characterization through GC–MS. Among the identified species, n-alkanes were invariably the highest within PM< 1.1 (mean ± SD: 484±103 ng m−3) compared to fine-mode (267±43 ng m−3) and coarse-mode aerosols (308±93 ng m−3). The molecular distribution of n-alkane homologues in all three size fractions showed a slight dominance of odd-numbered n-alkanes. The CPI (Carbon Preference Index) remains close to unity (CPI range: 1.2–2.1; mean ± SD: 1.5±0.5), indicating dominance of anthropogenic emissions like combustion of fossil fuels and biomass burning. The higher-molecular-weight homologue (> C25) concentration was found to be the highest in PM< 1.1 with an oscillating pattern, having an odd molecule concentration higher than the adjacent even molecules (Fig. 5b). In contrast the low-molecular-weight homologues (< C25) showed no such specific pattern of odd–even dominance. The sources of higher homologues (C27, C29, and C31) are probably the surface-deposited plant litter for coarse-mode aerosols and biomass burning for fine-mode aerosols, while low-molecular-weight homologues (< C25) primarily originate from fossil fuel combustion (Kang et al., 2016). Saturated fatty acids were found to constitute a larger fraction of solvent-extractable organics within coarse-mode particles (439±38 ng m−3) and submicron particles (357±162 ng m−3) in comparison to fine-mode particles (171±57 ng m−3). For all three size fractions, total low-molecular-weight fatty acid (≤ C20) concentration was found to be higher than the high-molecular-weight fatty acids (≥ C20), implicating anthropogenic emissions like vehicular, residential biomass burning, and energy practices. The presence of high concentrations of C12 and C15 implicates the dominance of cooking oil combustion. The high concentration of C22 further suggests the influence of biomass burning, which potentially emits both high and low fatty acids (Mochida et al., 2007). The fatty acid amide was found in trace amounts and could possibly be derived from fatty acid and ammonia during burning processes. The presence of PAHs was also measured for size-segregated particulates and was found to be the highest in submicron particulates (7.0 ng m−3) compared to PM1.1–2.1 (3.6 ng m−3) and PM> 2.1 (3.1 ng m−3). PAHs are mainly produced due to incomplete combustion of fuels like fossil fuels and biomass at relatively high temperatures (Singh et al., 2017b; Chen et al., 2015). There was no previous report of submicron particulate-bound PAHs during biomass burning emissions over the IGP to compare, except for PM2.5-bound PAHs in Patiala (27–40 ng m−3; Rajput et al., 2011, 2014), Agra (9 ng m−3; Villalobos et al., 2015), and Kanpur (3 ng m−3; Villalobos et al., 2015) and total-aerosol-bound PAHs in Kathmandu (320 ng m−3, Chen et al., 2015).
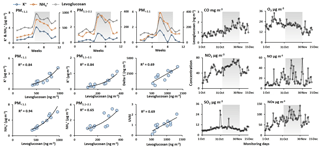
Figure 6Temporal variation in trace gases and biomass burning signature molecules (, K+, levoglucosan) and their associations within different aerosol size fractions.
Levoglucosan was found to be the most abundant anhydrosugar in submicron particles with an average (±1σ) of 649 (±177) ng m−3. In contrast, concentration in fine (229±87 ng m−3) and coarse particles (162±68 ng m−3) was relatively low, indicating the influence of biomass burning emissions for submicron particles. Levoglucosan concentration measured in this study is comparable to other reported observations, especially with the cases that have accounted for the influence of biomass burning emissions, e.g., New Delhi (1978 ng m−3, Li et al., 2014), Mt. Tai, China (391 ng m−3, Fu et al., 2008), Gent, Belgium (477 ng m−3, Zdrahal et al., 2002), Lumbini, Nepal (734 ng m−3, Wan et al., 2017), and Beijing, China (590 ng m−3, Cheng et al., 2013). In addition to levoglucosan, the relative concentration of other anhydrosugars (mannosan and galactosan) in all size-segregated aerosols was negligible (< 70 ng m−3, not shown).
3.5 Signature of biomass burning emissions
Biomass primarily consists of different biopolymers (e.g., cellulose, hemicellulose, lignin, suberin, sporopollenin, and chitin) with a small proportion of lipids and terpenoids. During thermal combustion, such biomass emits different types of organic molecules, some of which have the potential to be considered a signature molecule based on their long residence time and chemical stability (Banerjee et al., 2015). The major combustion product of cellulose and hemicellulose includes anhydrosugars like levoglucosan (1,6-anhydro-β-D-glucopyranose, C6H10O5) and its two isomers (mannosan and galactosan). Among these, levoglucosan is a robust and widely used tracer for biomass burning emissions, both globally (Simoneit et al., 1999; Schkolnik et al., 2005; Cheng et al., 2013) and over the IGP (Li et al., 2014; Banerjee et al., 2015; Wan et al., 2017). In our case, levoglucosan was abundant in submicron particles with a peak during November (weeks 6 to 9; Fig. 6). The rise in concentration was universal in each particulate size fraction, but typically in submicron (837±83 ng m−3) and fine particulates (311±47 ng m−3), having a 54 %–70 % rise compared to the rest of the monitoring period. This could correspond to a short-term variation in emissions source strength, which possibly influenced the aerosol properties. A ratio between levoglucosan and the rest of the anhydrosugars (mannosan and galactosan) was also considered to indicate the dominating type of biomass burning, with a ratio < 10 specific for softwood combustion and > 10 for burning of hardwood and crop residue (Cheng et al., 2013). Even a ratio > 40 was reported from physical experiments using rice straw, wheat straw, and maize stalks (Engling et al., 2009). Although the presence of mannosan and galactosan was not frequent in our case, an overall ratio > 50 indicates the exclusive dominance of agriculture residue burning across the IGP.
The possibility of considering K+ and as biomass burning tracers was investigated in terms of their association with levoglucosan for submicron and fine particulates. In general, the temporal trend in levoglucosan coincided well with both K+ and , and all these tracers registered a gradual rise in concentration during November. A highly significant correlation (R2) between levoglucosan and K+ (PM1.1: 0.80, PM1.1–2.1: 0.76; p < 0.01), and levoglucosan and (PM1.1: 0.95, PM1.1–2.1: 0.60; p < 0.01), was noted at the 99 % confidence interval. That definitely indicates that levoglucosan, K+, and have similar biogenic sources over the IGP that predominately contribute to the aerosol loading, especially in PM1.1 and PM1.1–2.1. The relation between levoglucosan and K+ and further appeared to be nonlinear, with an exponential fit for submicron (R2: 0.84, 0.94) and for fine particulates (R2: 0.83, 0.65). Nonlinear correlations between levoglucosan and K+ are also reported in the Amazon (Schkolnik et al., 2005) and in Beijing (Cheng et al., 2013) during extreme biomass burning emissions. There was also evidence that was better associated with levoglucosan compared to K+, indicating the presence of additional K+ sources across the region (like fireworks; Kumar et al., 2016). However, in the absence of aerosol organic carbon content, contribution of biomass burning to aerosol mass was not computed.
In addition to using conventional biomass burning tracers, we also evaluated the association of submicron and fine-particulate-bound levoglucosan with weekly averages of Delta-C and UVAI (Fig. 6). Both Delta-C and UVAI are the measures of identifying the relative dominance of absorbing aerosols. In all scenarios, a significant correlation (R2) was noted between levoglucosan with Delta-C (0.65, p < 0.01) and UVAI (0.66, p < 0.01). In addition to the ground-based aerosol measurement, dynamic profiles of trace gas concentration, especially for trace gases that behave as aerosol precursors, are assessed from the Real-time Air Quality Data inventory of the Central Pollution Control Board (https://app.cpcbccr.com/ccr, last access: 12 March 2018). The hourly average concentrations of individual trace gases were initially checked for data quality and outliers and further averaged to 24 h. No such universal trend in concentration of all the trace gases was evident, except an overall increasing trend for NO, NO2, NOx, and CO, while SO2 remained stable and there was a negative trend for O3. The most striking feature was an increase in concentration particularly during November, although of a different magnitude. This was also evident in the variation in particulate-bound biomass tracers, which inspired us to consider two different aerosol loading scenarios, namely scenario 1 for the biomass-burning-dominated period (weeks 6 to 9, BDP) and scenario 2 for the less dominant biomass burning period (weeks 1–5 and weeks 10–11, BLDP). Such classification was intended to recognize if there was any variation in aerosol source fields over the IGP and in aerosol-induced radiative forcing.
3.6 Spatiotemporal nature of aerosol columnar properties
Spatiotemporal variations in aerosol columnar properties and trace gases are plotted in Fig. 7a, including the daily variations at the ground station (Fig. 7b). Instead of considering the columnar properties for the entire season, spatial plots are generated for two different scenarios like BDP and BLDP.
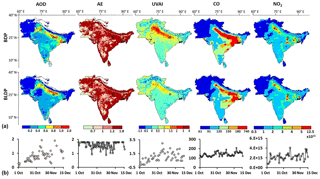
Figure 7Episode-specific spatial distribution of AOD, AE, UVAI, surface CO (ppbv), and tropospheric NO2 (molecules cm−2) over (a) South Asia and (b) at the ground station.
The spatial pattern in aerosol columnar properties was typical, having a very high aerosol loading exclusively over the IGP (area-weighted AOD mean ± SD: 0.55±0.21) in comparison to the rest of South Asia (0.31±0.21). However, there was no such temporal variation particularly over the IGP as both BDPAOD (0.56±0.23) and BLDPAOD (0.53±0.23) were similar. The BDPAOD was slightly higher (12 %) than that of the reported decadal average (0.50±0.25; Kumar et al., 2018) and was comparable to the season-specific average over the IGP (0.55±0.20; Kumar et al., 2018). It should be noted that the area-weighted AOD average includes all the pixels retrieved across the region, some of which may not represent the biomass burning emissions. This leads us to further retrieve and compare AOD particularly over the ground station. In this case, the mean AOD was significantly high during the post-monsoon season (0.81±0.39), 44 % higher for BDPAOD (0.98±0.42) with respect to BLDPAOD (0.68±0.32). The BDPAOD was 46 % higher compared to the decadal average for the station (0.67±0.28; Kumar et al., 2018). Figure 7a also includes a comparison of the relative dominance of aerosol types in terms of AE, and in both conditions fine particles (AE; BDP: 1.5, BLDP: 1.7) were found to dominate with a season-specific mean (±1σ) of 1.6 (±0.2).
Following the evidence of persisting high AOD and high AE indicating dominance of fine particulates of anthropogenic origin, the nature of aerosols in terms of absorbing and/or scattering was distinguished using OMI UVAI. UVAI has been widely used to detect dust (Badarinath et al., 2010), biomass burning aerosols (Torres et al., 2013; Kaskaoutis et al., 2014), and soot particles (Kumar et al., 2016), and has also been used in combination with CALIPSO to detect the height of the aerosol layer (Guan et al., 2010). In our experiment, the daily UVAI varied from (−) 0.34 to (+) 2.24 with a seasonal mean (±1σ) of 0.99 (±0.49) over the IGP, which is considerably higher than the seasonal mean for all of South Asia (0.47±0.46). Interestingly, negative UVAI was only evident during early October (week 1), signifying the presence of non-absorbing aerosols (like sulfate), while UV absorbing aerosols such as smoke and/or mineral dust was mainly evident during the rest of the season. During BDP, the high UVAI values (> 1.5) were mainly found to concentrate over the upper to middle IGP with 72 % of observations > 1.0. This clearly indicates the larger abundance of fresh UV-absorbing particles and is similar to the reported UVAI (< 2.0) over the Himalayas during peak burning season (Kumar et al., 2011; Vadrevu et al., 2012). There was also a considerable difference between the periodical mean UVAI for BDP (1.47±0.64) and BLDP (0.75±0.58) over the ground station. Further, following Guan et al. (2010) to use UVAI as a proxy to compute aerosol height, we found a low average height of the aerosol layer (∼1.5 km), possibly due to low-altitude injection of plumes from burning of agricultural residue.
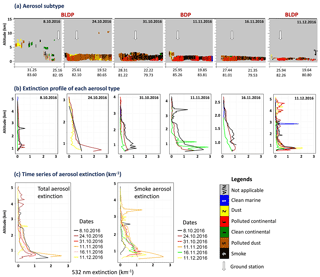
Figure 8Aerosol vertical profiles from selected CALIPSO overpasses across ground station (a) aerosol subtypes, (b) extinction coefficients of each aerosol type, and (c) time series of the extinction profile for total and smoke aerosols.
Apart from aerosols, spatial variation in a few trace gases (e.g., CO and NO2) directly emitted from biomass burning is also estimated. The MERRA-2 reanalysis surface CO profile was consistent with the observed UVAI, with a high CO surface concentration over the IGP (mean ± SD: 156±62 ppbv) in contrast to South Asia (114±52 ppbv). Similarly, in the case for tropospheric NO2 column density Aura OMI observations show high NO2 concentration across the IGP ( mol cm−2) compared to South Asia ( mol cm−2). Dominance of CO and NO2 across the IGP clearly reflects the influence of anthropogenic emissions from industries, vehicles, and biomass burning. Likewise, higher surface NO2 concentrations (> 5×1015 mol cm2) were particularly evident over urban/industrial hotspots like Punjab and Delhi, over industrial sectors in the Chhattisgarh region, and in the lower IGP (particularly over Dhaka). Temporal variations in mean CO (143 to 169 ppbv) and NO2 concentrations (2.3 to 2.5×1015 mol cm−2) were not so severe both across the IGP and over the ground station (CO: 140–142 ppbv; NO2: 2.3–2.5×1015 mol cm−2). The possible explanation for such minimum episode-specific variation may be the short residence time of NO2 and CO, as NO2 rapidly photodissociates by reaction with OH radicals, while CO gradually oxidizes to form CO2. Overall, the spatiotemporal nature of aerosols and trace gases was consistent with the observed trend at the ground station and was helpful for establishing the influence of biomass burning emissions over the region.
3.7 Vertical distribution of aerosols
Vertically resolved aerosol subtypes from spaceborne lidar for selected overpasses across the IGP are plotted in Fig. 8a with the corresponding extinction coefficient of aerosol type (Fig. 8b). The CALIPSO–CALIOP profile clearly indicates a temporal change in aerosol type, without any considerable change in the height of the aerosol layer. During initial days (in October), dominance of polluted dust (dust mixed with biomass burning smoke) was noted across the IGP, with occasional prevalence of smoke (biomass burning aerosols), clean continental (clean background aerosol), and dust aerosols. However, the contribution of polluted dust to total aerosol extinction was higher compared to the rest of the aerosol types. The height of aerosol layer was relatively low (< 2 km), corresponding to a low plume injection height and thereby posing limited potential for dispersion. The aerosol vertical profile, however, was modified from the end of October due to biomass burning emissions, with dominance of smoke particles, mainly persisting at low altitude (< 1.5 km). The height of smoke layer was consistent with that of OMI UVAI projected aerosol height. Smoke particles were found to associate with polluted dust, clean continental, and polluted continental aerosols, with overlapping profiles. Overall, smoke was the most frequent aerosol type with a high aerosol extinction coefficient (1–2.5 km−1 at 532 nm), and the altitude of the largest occurrence frequency of smoke remained below ∼1.5 km. The low injection height of smoke plumes from biomass burning may serve as a key input for aerosol transport modeling over the IGP as it critically regulates the distance and direction of the particle dispersion (Guan et al., 2010; Banerjee et al., 2011).
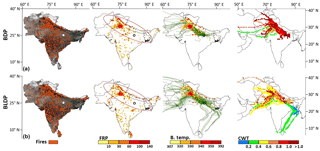
Figure 9Episode-specific MODIS fire count, fire radiative power (FRP, MW), brightness temperature (B. temp., K), and 5-day air mass back trajectory along with CWT.
The daily variation in total aerosol extinction and aerosol extinction only by smoke particles were also included in Fig. 8c. Total aerosol extinction indicates a corresponding increase during biomass burning, which peaks particularly in November, with low smoke injection height. Clear evidence of a gradual increase in smoke particle aerosol extinction was also noted. A single piece of evidence of high smoke extinction (> 1 km−1) at a greater height (∼3.4 km) was noted on 11 November, which may be associated with particle long-range transport and/or by the influence of the ABL. Overall, the CALIOP aerosol profiles were in accordance with the ground observations and OMI UVAI, indicating exclusive dominance of high-UV-absorbing aerosols across the plain during intense biomass burning.
3.8 Potential aerosol sources and transport
Active fire counts from the Terra and Aqua MODIS fire and thermal anomalies (with ≥70 % confidence) clearly indicate that fire spots were predominately over the upper IGP, mainly concentrated over the Indian states of Punjab, Haryana, and western Uttar Pradesh, and in the Punjab state of Pakistan (Fig. 9). However, there was a temporal shift in the total number of fire counts (Fig. 9, within the marked region) from the biomass-burning-dominated period (BDP: 5272) to a less dominating period (BLDP: 4466). The fire radiative power (FRP), i.e., the rate of energy released in unit time, indicates a relative change in amount and strength of biomass burning emissions, mainly during BDP (138 366 MW) in comparison to BLDP (112 168 MW). The total FRP was higher during BDP mainly due to a higher number of fire counts and fire strength, as the rate of release of thermal radiation is related to the amount of biomass burnt and smoke being released (Schroeder et al., 2010). The MODIS fire spots (with brightness temperature), specially subset over the IGP, were plotted against 5-day air mass back trajectories and simulated and integrated at three vertical heights (100, 300, and 500 m) over the ground station. Vertical heights were selected based on the average planetary boundary layer height (402±81 m) for the monitoring period. The air mass back trajectories indicate the upper IGP as the sole source of aerosols during BDP, which was otherwise influenced by both continental and marine air masses during the non-dominating period. The air mass back trajectories during BDP overlap precisely with the fire spots that correspond to higher brightness temperature, indicating greater relevance to FRP. The air masses for individual episodes were further subject to cluster and CWT analysis considering columnar aerosol load, and the result was consistent with our prior observations. High CWT (> 0.8) during BDP was clearly attributed to the regional pollution, mainly originating from the upper IGP. In contrast, relatively low CWT was noted during BLDP, originating from the upper IGP (CWT < 0.8), western dry region (CWT < 0.6), and an oceanic environment (CWT < 0.4). This leads us to conclude with confidence that there was a strong temporal gradient in post-monsoon-specific biomass burning emissions over the upper IGP, which greatly influences the regional aerosol climatology and thereby influences aerosol-induced health effects and regional climate.
3.9 Aerosol radiative forcing and atmospheric heating
Daily satellite-retrieved AOD, TCO, CWV, SSA, ground-based BC mass concentration, and aerosol water-soluble and water-insoluble fractions were used as an input to the OPAC model to simulate ARF (at 0.2–4.0 µm). Within the period, TCO varied between 237 and 277 DU without any difference between BDP (257±10 DU) and BLDP (256±12 DU). The SSA (at 550 nm), designating the fraction of scattered light over total light extinction, was lower during BDP (0.86±0.05) compared to BLDP (0.98±0.04), suggesting an abundance of strong absorbing aerosols especially during BDP. The CWV also fluctuates considerably (range: 0.28–3.92 cm), with an overall season-specific mean (±σ) of 2.0 (±0.7) cm.
The direct ARF and heating rate were estimated under clear-sky conditions with the SBDART model using OPAC output. The composite ARF was calculated for individual episodes at surface (SRF), top of the atmosphere (TOA), and atmosphere (ATM) (Fig. 10). Overall, the ARF at TOA and SRF were negative, indicating the aerosol cooling effect at the surface and top of the atmosphere. There was a slight temporal change in TOA radiative forcing (BDP: −28; BLDP: −23 W m−2) compared to the considerable intraseasonal variation in SRF forcing (BDP: −163; BLDP: −79 W m−2). The variation in SRF forcing was mainly induced by the surface BC (mean; BDP, BLDP: 9, 7 µg m−3), aerosol mass concentration (501, 327 µg m−3), and WSIC fractions, particularly in (38, 15 µg m−3), (19, 12 µg m−3), and (11, 4 µg m−3). Since the ATM forcing is the balance of attenuation of radiation at TOA and SRF, the resultant atmospheric forcing was found to be very high, especially during the biomass-burning-dominated period (BDP: 135 W m−2), compared to the non-dominating one (BLDP: 56 W m−2). Overall, there was a clear indication of intraseasonal variation in ARF, which needs to be considered in parametrization of aerosol schemes for regional climate models. Similarly, the corresponding heat rate was substantially high during BDP (4.3 K day−1), possibly influenced by more absorbing aerosols, compared to BLDP (1.8 K day−1). The computed ARF during the post-monsoon season was comparable to other urban sites in the IGP that are reported to be influenced by biomass burning, e.g., Delhi (44–131 W m−2, Bisht et al., 2015), Patiala (57–63 W m−2, Sharma et al. 2017), Kanpur (30–43 W m−2, Kaskaoutis et al., 2013), and over Karachi (35–84 W m−2, Alam et al., 2011). However, none of the earlier reports noted the intraseasonality in ARF by means of change in driving factors, which appeared to be significant and necessitate proper address in regional model simulation. Intraseasonality in ARF was reported earlier over Varanasi during winter (ARF: 31–47 W m−2, Kumar et al., 2017b), while the change in forcing was not as drastic as evident during the post-monsoon season. Therefore, it is extremely likely that intraseasonality in aerosol properties significantly influences the aerosol–climate–health interactions over the IGP and therefore must need to be taken into account for uncertainty analysis in the regional aerosol– climate model.
The influence of biomass burning emissions on aerosol properties, transport, and radiative forcing was evaluated over the Indo-Gangetic Plain, South Asia. Very high concentrations of total and fine-mode aerosol (PM2.1) were observed during the post-monsoon season, with a significant increase in fine-to-coarse particle ratio (> 1) particularly from November. Submicron particles dominate the aerosol fine mode, with the PM1.1-to-PM1.1–2.1 ratio frequently exceeding 2.5. The WSIS was found to constitute a greater proportion of submicron and fine-particle mass compared to the coarser particles. The WSIS was mainly of secondary nature, with major contributions from sulfate and nitrate ions. A strong correlation between and and a high equivalent ratio in submicron particulates indicate the abundance of gaseous NH3 to neutralize acidic species (). This contrasted with coarse-mode particles for which a low equivalent ratio indicates the predominant neutralization by crustal minerals. The -to- ratio for submicron and fine-mode particles also increased (> 1) during extreme biomass burning emissions, as expected considering other reported observations of haze events over Asia. A rise in black carbon with a corresponding increase in Delta-C refer to the added contribution of biomass burning emissions. The influence of emissions was further quantified using specific organic (levoglucosan), inorganic (K+ and ), and satellite (UVAI) tracers. Levoglucosan was the most abundant species in submicron particles, with a very high ratio (> 50) with other anhydrosugars denoting exclusive emissions from burning of agriculture residue. The temporal variation in levoglucosan was consistent with inorganic tracers (K+ and ), with a sharp rise during November, and a strong correlation among these three indicates their biogenic sources. The association between levoglucosan and K+ or was nonlinear, with an exponential fit for submicron and fine particulates. The spatiotemporal distribution of aerosols was evaluated in terms of area-weighted mean over both the IGP and over the selected transect across the ground station. During the biomass-burning-dominated period, a considerable increase in columnar aerosol loading was highlighted (AOD: 0.98), consisting of absorbing aerosols (UVAI > 1.5) with a corresponding low plume height (∼1.5 km). Moreover, the variation in a few trace gases associated with biomass burning emissions (CO and NO2) was consistent with AOD, allowing a definite spatial signature of emission sources and transport across the IGP. The CALIPSO–CALIOP cross-sectional altitudinal profiles clearly illustrate the intraseasonality in aerosol types that were dominated by smoke and polluted continental aerosols during biomass burning emissions, which are otherwise associated with clean continental, polluted dust, and dust aerosols. The possible pathway for regional transport of aerosols from the upper IGP to the ground station was noted using cluster analysis and concentration-weighted air mass back trajectories. Finally, aerosol optical and microphysical properties were used in combination to simulate direct aerosol radiative forcing (ARF) and atmospheric heating. There was evidence of strong intraseasonality in ARF with a very high atmospheric forcing (135 W m−2) and heating rate (4.3 K day−1) during the biomass-burning-dominated period compared to the non-dominating one (56 W m−2, 1.8 K day−1).
Considering that the duration of these biomass burning emissions represents several weeks per year, their impact on ARF and by consequence on the regional climate is not negligible. We therefore conclude with a reasonable level of confidence that intraseasonality in aerosol properties must be seriously considered in the regional aerosol–climate model for improving assessment and forecasting of aerosol–climate–health interactions across the IGP.
MODIS data are available from the Level 1 and Atmosphere Archive & Distribution System (LAADS) at https://ladsweb.nascom.nasa.gov (last access: 12 March 2018). Aura OMI and MERRA-2 reanalysis data are available from the Mirador NASA Goddard Earth Sciences Data and Information Center (GES DISC) (https://mirador.gsfc.nasa.gov, last access: 12 March 2018). CALIPSO data are available from the NASA Atmospheric Science Data Center (https://eosweb.larc.nasa.gov, last access: 12 March 2018). Planetary boundary layer height and air mass back trajectories are retrieved from Global Data Assimilation System (GDAS) archives hosted at the NOAA Air Resource Laboratory (https://ready.arl.noaa.gov, last access: 12 March 2018). MODIS fire products are obtained from the Fire Information for Resource Management System (FIRMS) (https://firms.modaps.eosdis.nasa.gov). Trace gas data at the ground station are available at the Real time Air Quality Data inventory of the Central Pollution Control Board (https://app.cpcbccr.com/ccr).
The supplement related to this article is available online at: https://doi.org/10.5194/acp-18-14197-2018-supplement.
NS and TB designed the experiment while NS, MPR, and TB carried out the experiment and analyzed the data. NS, MPR, KD, TB, RSS, RKM, and MSH interpreted the observations and NS, TB, and KD drafted the paper.
The authors declare that they have no conflict of interest.
This research is supported by the Science and Engineering Research Board (SERB),
Department of Science and Technology (DST), New Delhi (SR/FTP/ES-52/2014).
Tirthankar Banerjee acknowledges the financial support from the University Grants
Commission (UGC) under the UGC-ISF bilateral project (6-11/2018 IC), Ram S. Singh
acknowledges the Indian Space Research Organization under ARFI (code
P-32-13), and Rajesh K. Mall acknowledges financial support from the Climate Change
Programme of DST (DST/CCP/CoE/80/2017-G). Nandita Singh acknowledges
financial support under the DST Women Scientist scheme (SR/WOS-A/EA-1012/2015)
and Meytar Sorek-Hamer acknowledges the NASA postdoctoral fellowship,
administered by USRA. The authors duly acknowledge the guidance and cooperation
provided by the dean and director of IESD-BHU.
Edited by: Rolf Müller
Reviewed by: two anonymous
referees
Alam, K., Trautmann, T., and Blaschke, T.: Aerosol optical properties and radiative forcing over mega-city Karachi, Atmos. Res., 101, 773–782, https://doi.org/10.1016/j.atmosres.2011.05.007, 2011.
Apte, J. S., Marshall, J. D., Cohen, A. J., and Brauer, M.: Addressing global mortality from ambient PM2.5, Environ. Sci. Technol., 49, 8057–8066, https://doi.org/10.1021/acs.est.5b01236, 2015.
Badarinath, K. V. S., Sharma, A. R., Kaskaoutis, D. G., Kharol, S. K., and Kambezidis, H. D.: Solar dimming over the tropical urban region of Hyderabad, India: Effect of increased cloudiness and increased anthropogenic aerosols, J. Geophys. Res.-Atmos., 115, D21208, https://doi.org/10.1029/2009JD013694, 2010.
Banerjee, T., Barman, S. C., and Srivastava, R. K.: Application of air pollution dispersion modeling for source-contribution assessment and model performance evaluation at Integrated Industrial Estate-Pantnagar, Environ. Policy, 159, 865–875, https://doi.org/10.1016/j.envpol.2010.12.026, 2011.
Banerjee, T., Murari, V., Kumar, M., and Raju, M. P.: Source apportionment of airborne particulates through receptor modeling: Indian scenario, Atmos. Res., 164, 167–187, https://doi.org/10.1016/j.atmosres.2015.04.017, 2015.
Bellouin, N., Boucher, O., Haywood, J., and Reddy, M. S.: Global estimate of aerosol direct radiative forcing from satellite measurements, Nature, 438, 1138–1141, https://doi.org/10.1038/nature04348, 2005.
Bisht, D. S., Dumka, U. C., Kaskaoutis, D. G., Pipal, A. S., Srivastava, A. K., Soni, V. K., Attri, S. D., Sateesh, M., and Tiwari, S.: Carbonaceous aerosols and pollutants over Delhi urban environment: temporal evolution, source apportionment and radiative forcing, Sci. Total Environ., 521, 431–445, https://doi.org/10.1016/j.scitotenv.2015.03.083, 2015.
Bodhaine, B. A.: Aerosol absorption measurements at Barrow, Mauna Loa and the south pole, J. Geophys. Res.-Atmos., 100, 8967–8975, https://doi.org/10.1029/95JD00513, 1995.
Bond, T. C., Doherty, S. J., Fahey, D. W., Forster, P. M., Berntsen, T., DeAngelo, B. J., Flanner, M. G., Ghan, S., Kärcher, B., Koch, D., and Kinne, S.: Bounding the role of black carbon in the climate system: A scientific assessment, J. Geophys. Res.-Atmos., 118, 5380–5552, https://doi.org/10.1002/jgrd.50171, 2013.
Chang-Graham, A. L., Profeta, L. T., Johnson, T. J., Yokelson, R. J., Laskin, A., and Laskin, J.: Case study of water-soluble metal containing organic constituents of biomass burning aerosol, Environ. Sci. Technol., 45, 1257–1263, https://doi.org/10.1021/es103010j, 2011.
Chen, P., Kang, S., Li, C., Rupakheti, M., Yan, F., Li, Q., Ji, Z., Zhang, Q., Luo, W., and Sillanpää, M.: Characteristics and sources of polycyclic aromatic hydrocarbons in atmospheric aerosols in the Kathmandu Valley, Nepal, Sci. Total Environ. 538, 86–92, https://doi.org/10.1016/j.scitotenv.2015.08.006, 2015.
Cheng, Y., Engling, G., He, K.-B., Duan, F.-K., Ma, Y.-L., Du, Z.-Y., Liu, J.-M., Zheng, M., and Weber, R. J.: Biomass burning contribution to Beijing aerosol, Atmos. Chem. Phys., 13, 7765–7781, https://doi.org/10.5194/acp-13-7765-2013, 2013.
Draxler, R. R. and Rolph, G. D.: HYSPLIT (HYbrid single-particle Lagrangian integrated trajectory) model access via NOAA ARL READY, NOAA Air Resources Laboratory, Silver Spring, MD, Dostupno na, available at: http://ready.arl.noaa.gov/HYSPLIT.php (last access: 6 June 2010), 2003.
Engling, G., Lee, J. J., Tsai, Y. W., Lung, S. C. C., Chou, C. C. K., and Chan, C. Y.: Size-resolved anhydrosugar composition in smoke aerosol from controlled field burning of rice straw, Aerosol Sci. Tech., 43, 662–672, https://doi.org/10.1080/02786820902825113, 2009.
EPA: Compendium Method IO-3.2 Determination of metals in ambient particulate matter using atomic absorption (AA) spectroscopy, Center for Environmental Research Information Office of Research and Development, U.S. Environmental Protection Agency, 1999.
Fu, P., Kawamura, K., Okuzawa, K., Aggarwal, S. G., Wang, G., Kanaya, Y., and Wang, Z.: Organic molecular compositions and temporal variations of summertime mountain aerosols over Mt. Tai, North China Plain, J. Geophys. Res.-Atmos., 113, D19107, https://doi.org/10.1029/2008JD009900, 2008.
Gonzalez, R. O., Strekopytov, S., Amato, F., Querol, X., Reche, C., and Weiss, D.: New insights from zinc and copper isotopic compositions into the sources of atmospheric particulate matter from two major European cities, Environ. Sci. Technol., 50, 9816–9824, https://pubs.acs.org/doi/10.1021/acs.est.6b00863, 2016.
Guan, H., Esswein, R., Lopez, J., Bergstrom, R., Warnock, A., Follette-Cook, M., Fromm, M., and Iraci, L. T.: A multi-decadal history of biomass burning plume heights identified using aerosol index measurements, Atmos. Chem. Phys., 10, 6461–6469, https://doi.org/10.5194/acp-10-6461-2010, 2010.
Hess, M., Koepke, P., and Schult, I.: Optical properties of aerosols and clouds: The software package OPAC, B. Am. Meteorol. Soc., 79, 831–844, https://doi.org/10.1175/1520-0477(1998)079<0831:OPOAAC>2.0.CO;2, 1998.
Hu, Q. H., Xie, Z. Q., Wang, X. M., Kang, H., and Zhang, P.: Levoglucosan indicates high levels of biomass burning aerosols over oceans from the Arctic to Antarctic, Sci. Rep., 3, 3119, https://doi.org/10.1038/srep03119, 2013.
Jimenez, J. L., Canagaratna, M. R., Donahue, N. M., Prevot, A. S. H., Zhang, Q., Kroll, J. H., DeCarlo, P. F., Allan, J. D., Coe, H., Ng, N. L., and Aiken, A. C.: Evolution of organic aerosols in the atmosphere, Science, 326, 1525–1529, https://doi.org/10.1126/science.1180353, 2009.
Justice, C., Giglio, L., Boschetti, L., Roy, D., Csiszar, I., Morisette, J., and Kaufman, Y.: MODIS Fire Products Algorithm Technical Background Document, MODIS Science Team, 2006.
Kanakidou, M., Myriokefalitakis, S., and Tsigaridis, K.: Aerosols in atmospheric chemistry and biogeochemical cycles of nutrients. Environ. Res. Lett., 13, 063004, https://doi.org/10.1088/1748-9326/aabcdb, 2018.
Kang, M., Fu, P., Aggarwal, S. G., Kumar, S., Zhao, Y., Sun, Y., and Wang, Z.: Size distributions of n-alkanes, fatty acids and fatty alcohols in springtime aerosols from New Delhi, India, Environ. Pollut., 219, 957–966, https://doi.org/10.1016/j.envpol.2016.09.077, 2016.
Kaskaoutis, D. G., Sinha, P. R., Vinoj, V., Kosmopoulos, P. G., Tripathi, S. N., Misra, A., Sharma, M., and Singh, R. P.: Aerosol properties and radiative forcing over Kanpur during severe aerosol loading conditions, Atmos. Environ., 79, 7–19, https://doi.org/10.1016/j.atmosenv.2013.06.020, 2013.
Kaskaoutis, D. G., Kumar, S., Sharma, D., Singh, R. P., Kharol, S. K., Sharma, M., Singh, A. K., Singh, S., Singh, A., and Singh, D.: Effects of crop residue burning on aerosol properties, plume characteristics, and long-range transport over northern India, J. Geophys. Res.-Atmos., 119, 5424–5444, https://doi.org/10.1002/2013JD021357, 2014.
Krotkov, N. A., Lamsal, L. N., Celarier, E. A., Swartz, W. H., Marchenko, S. V., Bucsela, E. J., Chan, K. L., Wenig, M., and Zara, M.: The version 3 OMI NO2 standard product, Atmos. Meas. Tech., 10, 3133–3149, https://doi.org/10.5194/amt-10-3133-2017, 2017.
Kumar, M., Tiwari, S., Murari, V., Singh, A. K., and Banerjee, T.: Wintertime characteristics of aerosols at middle Indo-Gangetic Plain: Impacts of regional meteorology and longrange transport, Atmos. Environ., 104, 162–175, https://doi.org/10.1016/j.atmosenv.2015.01.014, 2015.
Kumar, M., Singh, R. K., Murari, V., Singh, A. K., Singh, R. S., and Banerjee, T.: Fireworks induced particle pollution: a spatio-temporal analysis, Atmos. Res., 180, 78–91, https://doi.org/10.1016/j.atmosres.2016.05.014, 2016.
Kumar, M., Raju, M. P., Singh, R. S., and Banerjee, T.: Impact of drought and normal monsoon scenarios on aerosol induced radiative forcing and atmospheric heating in Varanasi over middle Indo-Gangetic Plain, J. Aerosol Sci., 113, 95–107, https://doi.org/10.1016/j.jaerosci.2017.07.016, 2017a.
Kumar, M., Raju, M. P., Singh, R. K., Singh, A. K., Singh, R. S., and Banerjee, T.: Wintertime characteristics of aerosols over middle Indo-Gangetic Plain: Vertical profile, transport and radiative forcing, Atmos. Res., 183, 268–282, https://doi.org/10.1016/j.atmosres.2016.09.012, 2017b.
Kumar, M., Parmar, K. S., Kumar, D. B., Mhawish, A., Broday, D. M., Mall, R. K., and Banerjee, T.: Long-term aerosol climatology over Indo-Gangetic Plain: Trend, prediction and potential source fields, Atmos. Environ., 180, 37–50, https://doi.org/10.1016/j.atmosenv.2018.02.027, 2018.
Kumar, R., Naja, M., Satheesh, S. K., Ojha, N., Joshi, H., Sarangi, T., Pant, P., Dumka, U. C., Hegde, P., and Venkataramani, S.: Influences of the springtime northern Indian biomass burning over the central Himalayas, J. Geophys. Res.-Atmos., 116, D19302, https://doi.org/10.1029/2010JD015509, 2011.
Kumar, R., Barth, M. C., Pfister, G. G., Nair, V. S., Ghude, S. D., and Ojha, N.: What controls the seasonal cycle of black carbon aerosols in India?, J. Geophys. Res.-Atmos., 120, 7788–7812, https://doi.org/10.1002/2015JD023298, 2015.
Levelt, P. F., van den Oord, G. H., Dobber, M. R., Malkki, A., Visser, H., de Vries, J., Stammes, P., Lundell, J. O., and Saari, H.: The ozone monitoring instrument, IEEE T. Geosci. Remote, 44, 1093–1101, https://doi.org/10.1109/TGRS.2006.872333, 2006.
Levy, R. C., Mattoo, S., Munchak, L. A., Remer, L. A., Sayer, A. M., Patadia, F., and Hsu, N. C.: The Collection 6 MODIS aerosol products over land and ocean, Atmos. Meas. Tech., 6, 2989–3034, https://doi.org/10.5194/amt-6-2989-2013, 2013.
Li, J., Wang, G., Aggarwal, S. G., Huang, Y., Ren, Y., Zhou, B., Singh, K., Gupta, P. K., Cao, J., and Zhang, R.: Comparison of abundances, compositions and sources of elements, inorganic ions and organic compounds in atmospheric aerosols from Xi'an and New Delhi, two megacities in China and India, Sci. Total Environ., 476, 485–495, https://doi.org/10.1016/j.scitotenv.2014.01.011, 2014.
Mhawish, A., Banerjee, T., Broday, D. M., Misra, A., and Tripathi, S. N.: Evaluation of MODIS Collection 6 aerosol retrieval algorithms over Indo-Gangetic Plain: Implications of aerosols types and mass loading, Remote Sens. Environ., 201, 297–313, https://doi.org/10.1016/j.rse.2017.09.016, 2017.
Mhawish, A., Kumar, M., Mishra, A. K., Srivastava, P. K., and Banerjee, T.: Remote sensing of aerosols from space: retrieval of properties and applications, Remote Sensing of Aerosols, Clouds, and Precipitation, 45–83, https://doi.org/10.1016/B978-0-12-810437-8.00003-7, 2018.
Mochida, M., Umemoto, N., Kawamura, K., Lim, H. J., and Turpin, B. J.: Bimodal size distributions of various organic acids and fatty acids in the marine atmosphere: Influence of anthropogenic aerosols, Asian dusts, and sea spray off the coast of East Asia, J. Geophys. Res.-Atmos., 112, D15209, https://doi.org/10.1029/2006JD007773, 2007.
Moorthy, K. K., Satheesh, S. K., Babu, S. S., and Dutt, C. B. S.: Integrated Campaign for Aerosols, gases and Radiation Budget (ICARB): An overview, J. Earth Syst. Sci., 117, 243–262, https://doi.org/10.1007/s12040-008-0029-7, 2008.
Murari, V., Kumar, M., Barman, S. C., and Banerjee, T.: Temporal variability of MODIS aerosol optical depth and chemical characterization of airborne particulates in Varanasi, India, Environ. Sci. Pollut. Res., 22, 1329–1343, https://doi.org/10.1007/s11356-014-3418-2, 2015.
Murari, V., Kumar, M., Singh, N., Singh, R. S., and Banerjee, T.: Particulate morphology and elemental characteristics: variability at middle Indo-Gangetic Plain, J. Atmos. Chem., 73, 165–179, https://doi.org/10.1007/s10874-015-9321-5, 2016.
Murari, V., Kumar, M., Mhawish, A., Barman, S. C., and Banerjee, T.: Airborne particulate in Varanasi over middle Indo-Gangetic Plain: variation in particulate types and meteorological influences, Environ. Monit. Assess., 189, 157, https://doi.org/10.1007/s10661-017-5859-9, 2017.
Myhre, G., Samset, B. H., Schulz, M., Balkanski, Y., Bauer, S., Berntsen, T. K., Bian, H., Bellouin, N., Chin, M., Diehl, T., Easter, R. C., Feichter, J., Ghan, S. J., Hauglustaine, D., Iversen, T., Kinne, S., Kirkevåg, A., Lamarque, J.-F., Lin, G., Liu, X., Lund, M. T., Luo, G., Ma, X., van Noije, T., Penner, J. E., Rasch, P. J., Ruiz, A., Seland, Ø., Skeie, R. B., Stier, P., Takemura, T., Tsigaridis, K., Wang, P., Wang, Z., Xu, L., Yu, H., Yu, F., Yoon, J.-H., Zhang, K., Zhang, H., and Zhou, C.: Radiative forcing of the direct aerosol effect from AeroCom Phase II simulations, Atmos. Chem. Phys., 13, 1853–1877, https://doi.org/10.5194/acp-13-1853-2013, 2013.
Pavuluri, C. M., Kawamura, K., Aggarwal, S. G., and Swaminathan, T.: Characteristics, seasonality and sources of carbonaceous and ionic components in the tropical aerosols from Indian region, Atmos. Chem. Phys., 11, 8215–8230, https://doi.org/10.5194/acp-11-8215-2011, 2011.
Prajapati, S. K. and Tripathi, B. D.: Seasonal variation of leaf dust accumulation and pigment content in plant species exposed to urban particulates pollution, J. Environ. Qual., 37, 865–870, https://doi.org/10.2134/jeq2006.0511, 2008.
Rajput, P. and Sarin, M. M.: Polar and non-polar organic aerosols from large-scale agricultural-waste burning emissions in Northern India: implications to organic mass-to-organic carbon ratio, Chemosphere, 103, 74–79, https://doi.org/10.1016/j.chemosphere.2013.11.028, 2014.
Rajput, P., Sarin, M. M., Rengarajan, R., and Singh, D.: Atmospheric polycyclic aromatic hydrocarbons (PAHs) from post-harvest biomass burning emissions in the Indo-Gangetic Plain: isomer ratios and temporal trends, Atmos. Environ., 45, 6732–6740, https://doi.org/10.1016/j.atmosenv.2011.08.018, 2011.
Rajput, P., Sarin, M. M., Sharma, D., and Singh, D.: Characteristics and emission budget of carbonaceous species from post-harvest agricultural-waste burning in source region of the Indo-Gangetic Plain, Tellus B, 66, 21026, https://doi.org/10.3402/tellusb.v66.21026, 2014.
Raju, M. P., Safai, P. D., Vijayakumar, K., Devara, P. C. S., Naidu, C. V., Rao, P. S. P., and Pandithurai, G.: Atmospheric abundances of black carbon aerosols and their radiative impact over an urban and a rural site in SW India, Atmos. Environ., 125, 429–436, https://doi.org/10.1016/j.atmosenv.2015.09.023, 2016.
Ricchiazzi, P., Yang, S., Gautier, C., and Sowle, D.: SBDART: A research and teaching software tool for plane-parallel radiative transfer in the Earth's atmosphere, B. Am. Meteorol. Soc., 79, 2101–2114, https://doi.org/10.1175/1520-0477(1998)079<2101:SARATS>2.0.CO;2, 1998.
Riipinen, I., Pierce, J. R., Yli-Juuti, T., Nieminen, T., Häkkinen, S., Ehn, M., Junninen, H., Lehtipalo, K., Petäjä, T., Slowik, J., Chang, R., Shantz, N. C., Abbatt, J., Leaitch, W. R., Kerminen, V.-M., Worsnop, D. R., Pandis, S. N., Donahue, N. M., and Kulmala, M.: Organic condensation: a vital link connecting aerosol formation to cloud condensation nuclei (CCN) concentrations, Atmos. Chem. Phys., 11, 3865–3878, https://doi.org/10.5194/acp-11-3865-2011, 2011.
Rogers, R. R., Hostetler, C. A., Hair, J. W., Ferrare, R. A., Liu, Z., Obland, M. D., Harper, D. B., Cook, A. L., Powell, K. A., Vaughan, M. A., and Winker, D. M.: Assessment of the CALIPSO Lidar 532 nm attenuated backscatter calibration using the NASA LaRC airborne High Spectral Resolution Lidar, Atmos. Chem. Phys., 11, 1295–1311, https://doi.org/10.5194/acp-11-1295-2011, 2011.
Ryu, S. Y., Kim, J. E., Zhuanshi, H., Kim, Y. J., and Kang, G. U.: Chemical composition of post-harvest biomass burning aerosols in Gwangju, Korea, J. Air Waste Manage., 54, 1124–1137, https://doi.org/10.1080/10473289.2004.10471018, 2004.
Sayer, A. M., Munchak, L. A., Hsu, N. C., Levy, R. C., Bettenhausen, C., and Jeong, M. J.: MODIS Collection 6 aerosol products: Comparison between Aqua's e-Deep Blue, Dark Target, and “merged” data sets, and usage recommendations, J. Geophys. Res.-Atmos., 119, 13965–13989, https://doi.org/10.1002/2014JD022453, 2014.
Schkolnik, G., Falkovich, A. H., Rudich, Y., Maenhaut, W., and Artaxo, P.: New analytical method for the determination of levoglucosan, polyhydroxy compounds, and 2-methylerythritol and its application to smoke and rainwater samples, Environ. Sci. Technol., 39, 2744–2752, https://doi.org/10.1021/es048363c, 2005.
Schroeder, W., Csiszar, I., Giglio, L., and Schmidt, C. C.: On the use of fire radiative power, area, and temperature estimates to characterize biomass burning via moderate to coarse spatial resolution remote sensing data in the Brazilian Amazon, J. Geophys. Res.-Atmos., 115, D21121, https://doi.org/10.1029/2009JD013769, 2010.
Seinfeld, J. H., Bretherton, C., Carslaw, K. S., Coe, H., DeMott, P. J., Dunlea, E. J., Feingold, G., Ghan, S., Guenther, A. B., Kahn, R., and Kraucunas, I.: Improving our fundamental understanding of the role of aerosol-cloud interactions in the climate system, P. Natl. Acad. Sci. USA, 113, 5781–5790, https://doi.org/10.1073/pnas.1514043113, 2016.
Sen, A., Abdelmaksoud, A. S., Ahammed, Y. N., Banerjee, T., Bhat, M. A., Chatterjee, A., Choudhuri, A. K., Das, T., Dhir, A., Dhyani, P. P., and Gadi, R.: Variations in particulate matter over Indo-Gangetic Plains and Indo-Himalayan Range during four field campaigns in winter monsoon and summer monsoon: role of pollution pathways, Atmos. Environ., 154, 200–224, https://doi.org/10.1016/j.atmosenv.2016.12.054, 2017.
Sharma, D., Srivastava, A. K., Ram, K., Singh, A., and Singh, D.: Temporal variability in aerosol characteristics and its radiative properties over Patiala, northwestern part of India: Impact of agricultural biomass burning emissions, Environ. Pollut., 231, 1030–1041, https://doi.org/10.1016/j.envpol.2017.08.052, 2017.
Simoneit, B. R., Schauer, J. J., Nolte, C. G., Oros, D. R., Elias, V. O., Fraser, M. P., Rogge, W. F., and Cass, G. R.: Levoglucosan, a tracer for cellulose in biomass burning and atmospheric particles, Atmos. Environ., 33, 173–182, https://doi.org/10.1016/S1352-2310(98)00145-9, 1999.
Singh, N., Murari, V., Kumar, M., Barman, S. C., and Banerjee, T.: Fine particulates over South Asia: review and meta-analysis of PM2.5 source apportionment through receptor model, Environ. Pollut., 223, 121–136, https://doi.org/10.1016/j.envpol.2016.12.071, 2017a.
Singh, N., Mhawish, A., Deboudt, K., Singh, R. S., and Banerjee, T.: Organic aerosols over Indo-Gangetic Plain: Sources, distributions and climatic implications, Atmos. Environ., 157, 59–74, https://doi.org/10.1016/j.atmosenv.2017.03.008, 2017b.
Sun, J. and Ariya, P. A.: Atmospheric organic and bio-aerosols as cloud condensation nuclei (CCN): A review, Atmos. Environ. 40, 795–820, https://doi.org/10.1016/j.atmosenv.2005.05.052, 2006.
Tan, J. H., Duan, J. C., Chen, D. H., Wang, X. H., Guo, S. J., Bi, X. H., Sheng, G. Y., He, K. B., and Fu, J. M.: Chemical characteristics of haze during summer and winter in Guangzhou, Atmos. Res., 94, 238–245, https://doi.org/10.1016/j.atmosres.2009.05.016, 2009.
Tian, M., Wang, H., Chen, Y., Yang, F., Zhang, X., Zou, Q., Zhang, R., Ma, Y., and He, K.: Characteristics of aerosol pollution during heavy haze events in Suzhou, China, Atmos. Chem. Phys., 16, 7357–7371, https://doi.org/10.5194/acp-16-7357-2016, 2016.
Torres, O., Ahn, C., and Chen, Z.: Improvements to the OMI near-UV aerosol algorithm using A-train CALIOP and AIRS observations, Atmos. Meas. Tech., 6, 3257–3270, https://doi.org/10.5194/amt-6-3257-2013, 2013.
Vadrevu, K. P., Ellicott, E., Giglio, L., Badarinath, K. V. S., Vermote, E., Justice, C., and Lau, W. K.: Vegetation fires in the himalayan region–Aerosol load, black carbon emissions and smoke plume heights, Atmos. Environ., 47, 241–251, https://doi.org/10.1016/j.atmosenv.2011.11.009, 2012.
Vakkari, V., Kerminen, V. M., Beukes, J. P., Tiitta, P., Zyl, P. G., Josipovic, M., Venter, A. D., Jaars, K., Worsnop, D. R., Kulmala, M., and Laakso, L.: Rapid changes in biomass burning aerosols by atmospheric oxidation, Geophys. Res. Lett., 41, 2644–2651, https://doi.org/10.1002/2014GL059396, 2014.
Villalobos, A. M., Amonov, M. O., Shafer, M. M., Devi, J. J., Gupta, T., Tripathi, S. N., Rana, K. S., Mckenzie, M., Bergin, M. H., and Schauer, J. J: Source apportionment of carbonaceous fine particulate matter (PM2.5) in two contrasting cities across the Indo-Gangetic plain, Atmos. Pollut. Res., 6, 398–405, https://doi.org/10.5094/APR.2015.044, 2015.
Wan, X., Kang, S., Li, Q., Rupakheti, D., Zhang, Q., Guo, J., Chen, P., Tripathee, L., Rupakheti, M., Panday, A. K., Wang, W., Kawamura, K., Gao, S., Wu, G., and Cong, Z.: Organic molecular tracers in the atmospheric aerosols from Lumbini, Nepal, in the northern Indo-Gangetic Plain: influence of biomass burning, Atmos. Chem. Phys., 17, 8867–8885, https://doi.org/10.5194/acp-17-8867-2017, 2017.
Wang, R., Balkanski, Y., Boucher, O., Bopp, L., Chappell, A., Ciais, P., Hauglustaine, D., Peñuelas, J., and Tao, S.: Sources, transport and deposition of iron in the global atmosphere, Atmos. Chem. Phys., 15, 6247–6270, https://doi.org/10.5194/acp-15-6247-2015, 2015.
Wang, Y., Hopke, P. K., Rattigan, O. V., Xia, X., Chalupa, D. C., and Utell, M. J.: Characterization of residential wood combustion particles using the two-wavelength aethalometer, Environ. Sci. Technol., 45, 7387–7393, https://doi.org/10.1021/es2013984, 2011.
Wang, Y. Q., Zhang, X. Y., and Draxler, R. R.: TrajStat: GIS-based software that uses various trajectory statistical analysis methods to identify potential sources from long-term air pollution measurement data, Environ. Modell. Softw., 24, 938–939, https://doi.org/10.1016/j.envsoft.2009.01.004, 2009.
Zdrahal, Z., Oliveira, J., Vermeylen, R., Claeys, M., and Maenhaut, W.: Improved method for quantifying levoglucosan and related monosaccharide anhydrides in atmospheric aerosols and application to samples from urban and tropical locations, Environ. Sci. Technol., 36, 747–753, https://doi.org/10.1021/es015619v, 2002.
Zhang, W., Tong, Y., Wang, H., Chen, L., Ou, L., Wang, X., Liu, G., and Zhu, Y.: Emission of metals from pelletized and uncompressed biomass fuels combustion in rural household stoves in China, Sci. Rep., 4, 5611, https://doi.org/10.1038/srep05611, 2014.